Have a language expert improve your writing
Run a free plagiarism check in 10 minutes, generate accurate citations for free.
- Knowledge Base
- Dissertation
- What Is a Research Methodology? | Steps & Tips
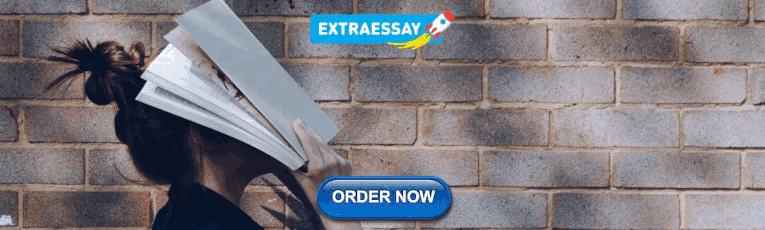
What Is a Research Methodology? | Steps & Tips
Published on August 25, 2022 by Shona McCombes and Tegan George. Revised on November 20, 2023.
Your research methodology discusses and explains the data collection and analysis methods you used in your research. A key part of your thesis, dissertation , or research paper , the methodology chapter explains what you did and how you did it, allowing readers to evaluate the reliability and validity of your research and your dissertation topic .
It should include:
- The type of research you conducted
- How you collected and analyzed your data
- Any tools or materials you used in the research
- How you mitigated or avoided research biases
- Why you chose these methods
- Your methodology section should generally be written in the past tense .
- Academic style guides in your field may provide detailed guidelines on what to include for different types of studies.
- Your citation style might provide guidelines for your methodology section (e.g., an APA Style methods section ).
Instantly correct all language mistakes in your text
Upload your document to correct all your mistakes in minutes
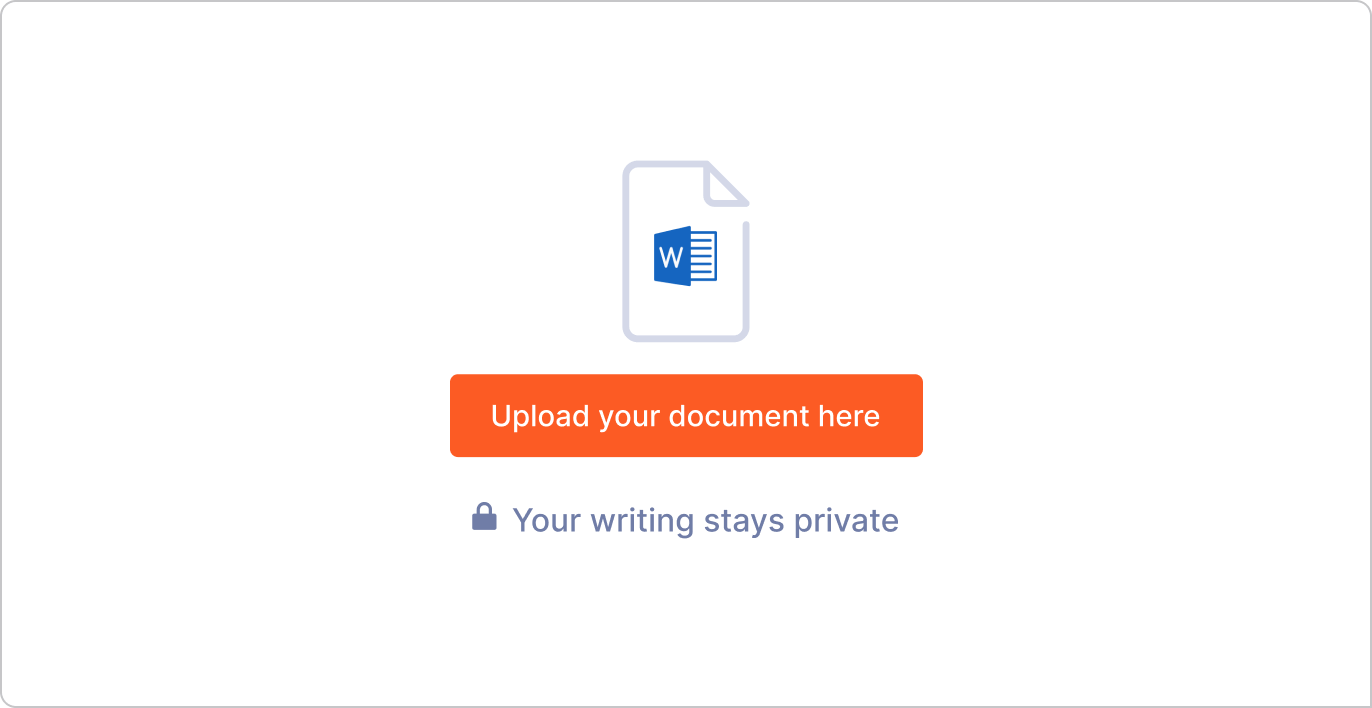
Table of contents
How to write a research methodology, why is a methods section important, step 1: explain your methodological approach, step 2: describe your data collection methods, step 3: describe your analysis method, step 4: evaluate and justify the methodological choices you made, tips for writing a strong methodology chapter, other interesting articles, frequently asked questions about methodology.
Don't submit your assignments before you do this
The academic proofreading tool has been trained on 1000s of academic texts. Making it the most accurate and reliable proofreading tool for students. Free citation check included.
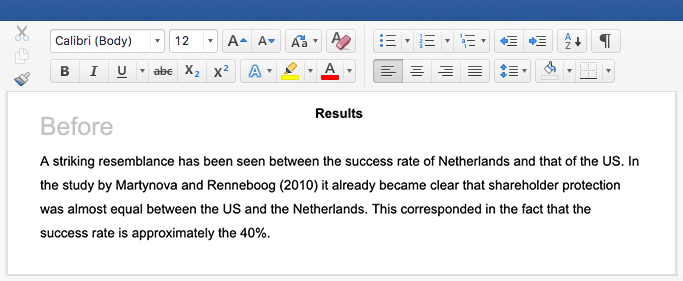
Try for free
Your methods section is your opportunity to share how you conducted your research and why you chose the methods you chose. It’s also the place to show that your research was rigorously conducted and can be replicated .
It gives your research legitimacy and situates it within your field, and also gives your readers a place to refer to if they have any questions or critiques in other sections.
You can start by introducing your overall approach to your research. You have two options here.
Option 1: Start with your “what”
What research problem or question did you investigate?
- Aim to describe the characteristics of something?
- Explore an under-researched topic?
- Establish a causal relationship?
And what type of data did you need to achieve this aim?
- Quantitative data , qualitative data , or a mix of both?
- Primary data collected yourself, or secondary data collected by someone else?
- Experimental data gathered by controlling and manipulating variables, or descriptive data gathered via observations?
Option 2: Start with your “why”
Depending on your discipline, you can also start with a discussion of the rationale and assumptions underpinning your methodology. In other words, why did you choose these methods for your study?
- Why is this the best way to answer your research question?
- Is this a standard methodology in your field, or does it require justification?
- Were there any ethical considerations involved in your choices?
- What are the criteria for validity and reliability in this type of research ? How did you prevent bias from affecting your data?
Once you have introduced your reader to your methodological approach, you should share full details about your data collection methods .
Quantitative methods
In order to be considered generalizable, you should describe quantitative research methods in enough detail for another researcher to replicate your study.
Here, explain how you operationalized your concepts and measured your variables. Discuss your sampling method or inclusion and exclusion criteria , as well as any tools, procedures, and materials you used to gather your data.
Surveys Describe where, when, and how the survey was conducted.
- How did you design the questionnaire?
- What form did your questions take (e.g., multiple choice, Likert scale )?
- Were your surveys conducted in-person or virtually?
- What sampling method did you use to select participants?
- What was your sample size and response rate?
Experiments Share full details of the tools, techniques, and procedures you used to conduct your experiment.
- How did you design the experiment ?
- How did you recruit participants?
- How did you manipulate and measure the variables ?
- What tools did you use?
Existing data Explain how you gathered and selected the material (such as datasets or archival data) that you used in your analysis.
- Where did you source the material?
- How was the data originally produced?
- What criteria did you use to select material (e.g., date range)?
The survey consisted of 5 multiple-choice questions and 10 questions measured on a 7-point Likert scale.
The goal was to collect survey responses from 350 customers visiting the fitness apparel company’s brick-and-mortar location in Boston on July 4–8, 2022, between 11:00 and 15:00.
Here, a customer was defined as a person who had purchased a product from the company on the day they took the survey. Participants were given 5 minutes to fill in the survey anonymously. In total, 408 customers responded, but not all surveys were fully completed. Due to this, 371 survey results were included in the analysis.
- Information bias
- Omitted variable bias
- Regression to the mean
- Survivorship bias
- Undercoverage bias
- Sampling bias
Qualitative methods
In qualitative research , methods are often more flexible and subjective. For this reason, it’s crucial to robustly explain the methodology choices you made.
Be sure to discuss the criteria you used to select your data, the context in which your research was conducted, and the role you played in collecting your data (e.g., were you an active participant, or a passive observer?)
Interviews or focus groups Describe where, when, and how the interviews were conducted.
- How did you find and select participants?
- How many participants took part?
- What form did the interviews take ( structured , semi-structured , or unstructured )?
- How long were the interviews?
- How were they recorded?
Participant observation Describe where, when, and how you conducted the observation or ethnography .
- What group or community did you observe? How long did you spend there?
- How did you gain access to this group? What role did you play in the community?
- How long did you spend conducting the research? Where was it located?
- How did you record your data (e.g., audiovisual recordings, note-taking)?
Existing data Explain how you selected case study materials for your analysis.
- What type of materials did you analyze?
- How did you select them?
In order to gain better insight into possibilities for future improvement of the fitness store’s product range, semi-structured interviews were conducted with 8 returning customers.
Here, a returning customer was defined as someone who usually bought products at least twice a week from the store.
Surveys were used to select participants. Interviews were conducted in a small office next to the cash register and lasted approximately 20 minutes each. Answers were recorded by note-taking, and seven interviews were also filmed with consent. One interviewee preferred not to be filmed.
- The Hawthorne effect
- Observer bias
- The placebo effect
- Response bias and Nonresponse bias
- The Pygmalion effect
- Recall bias
- Social desirability bias
- Self-selection bias
Mixed methods
Mixed methods research combines quantitative and qualitative approaches. If a standalone quantitative or qualitative study is insufficient to answer your research question, mixed methods may be a good fit for you.
Mixed methods are less common than standalone analyses, largely because they require a great deal of effort to pull off successfully. If you choose to pursue mixed methods, it’s especially important to robustly justify your methods.
Next, you should indicate how you processed and analyzed your data. Avoid going into too much detail: you should not start introducing or discussing any of your results at this stage.
In quantitative research , your analysis will be based on numbers. In your methods section, you can include:
- How you prepared the data before analyzing it (e.g., checking for missing data , removing outliers , transforming variables)
- Which software you used (e.g., SPSS, Stata or R)
- Which statistical tests you used (e.g., two-tailed t test , simple linear regression )
In qualitative research, your analysis will be based on language, images, and observations (often involving some form of textual analysis ).
Specific methods might include:
- Content analysis : Categorizing and discussing the meaning of words, phrases and sentences
- Thematic analysis : Coding and closely examining the data to identify broad themes and patterns
- Discourse analysis : Studying communication and meaning in relation to their social context
Mixed methods combine the above two research methods, integrating both qualitative and quantitative approaches into one coherent analytical process.
Above all, your methodology section should clearly make the case for why you chose the methods you did. This is especially true if you did not take the most standard approach to your topic. In this case, discuss why other methods were not suitable for your objectives, and show how this approach contributes new knowledge or understanding.
In any case, it should be overwhelmingly clear to your reader that you set yourself up for success in terms of your methodology’s design. Show how your methods should lead to results that are valid and reliable, while leaving the analysis of the meaning, importance, and relevance of your results for your discussion section .
- Quantitative: Lab-based experiments cannot always accurately simulate real-life situations and behaviors, but they are effective for testing causal relationships between variables .
- Qualitative: Unstructured interviews usually produce results that cannot be generalized beyond the sample group , but they provide a more in-depth understanding of participants’ perceptions, motivations, and emotions.
- Mixed methods: Despite issues systematically comparing differing types of data, a solely quantitative study would not sufficiently incorporate the lived experience of each participant, while a solely qualitative study would be insufficiently generalizable.
Remember that your aim is not just to describe your methods, but to show how and why you applied them. Again, it’s critical to demonstrate that your research was rigorously conducted and can be replicated.
1. Focus on your objectives and research questions
The methodology section should clearly show why your methods suit your objectives and convince the reader that you chose the best possible approach to answering your problem statement and research questions .
2. Cite relevant sources
Your methodology can be strengthened by referencing existing research in your field. This can help you to:
- Show that you followed established practice for your type of research
- Discuss how you decided on your approach by evaluating existing research
- Present a novel methodological approach to address a gap in the literature
3. Write for your audience
Consider how much information you need to give, and avoid getting too lengthy. If you are using methods that are standard for your discipline, you probably don’t need to give a lot of background or justification.
Regardless, your methodology should be a clear, well-structured text that makes an argument for your approach, not just a list of technical details and procedures.
If you want to know more about statistics , methodology , or research bias , make sure to check out some of our other articles with explanations and examples.
- Normal distribution
- Measures of central tendency
- Chi square tests
- Confidence interval
- Quartiles & Quantiles
Methodology
- Cluster sampling
- Stratified sampling
- Thematic analysis
- Cohort study
- Peer review
- Ethnography
Research bias
- Implicit bias
- Cognitive bias
- Conformity bias
- Hawthorne effect
- Availability heuristic
- Attrition bias
Methodology refers to the overarching strategy and rationale of your research project . It involves studying the methods used in your field and the theories or principles behind them, in order to develop an approach that matches your objectives.
Methods are the specific tools and procedures you use to collect and analyze data (for example, experiments, surveys , and statistical tests ).
In shorter scientific papers, where the aim is to report the findings of a specific study, you might simply describe what you did in a methods section .
In a longer or more complex research project, such as a thesis or dissertation , you will probably include a methodology section , where you explain your approach to answering the research questions and cite relevant sources to support your choice of methods.
In a scientific paper, the methodology always comes after the introduction and before the results , discussion and conclusion . The same basic structure also applies to a thesis, dissertation , or research proposal .
Depending on the length and type of document, you might also include a literature review or theoretical framework before the methodology.
Quantitative research deals with numbers and statistics, while qualitative research deals with words and meanings.
Quantitative methods allow you to systematically measure variables and test hypotheses . Qualitative methods allow you to explore concepts and experiences in more detail.
Reliability and validity are both about how well a method measures something:
- Reliability refers to the consistency of a measure (whether the results can be reproduced under the same conditions).
- Validity refers to the accuracy of a measure (whether the results really do represent what they are supposed to measure).
If you are doing experimental research, you also have to consider the internal and external validity of your experiment.
A sample is a subset of individuals from a larger population . Sampling means selecting the group that you will actually collect data from in your research. For example, if you are researching the opinions of students in your university, you could survey a sample of 100 students.
In statistics, sampling allows you to test a hypothesis about the characteristics of a population.
Cite this Scribbr article
If you want to cite this source, you can copy and paste the citation or click the “Cite this Scribbr article” button to automatically add the citation to our free Citation Generator.
McCombes, S. & George, T. (2023, November 20). What Is a Research Methodology? | Steps & Tips. Scribbr. Retrieved August 21, 2024, from https://www.scribbr.com/dissertation/methodology/
Is this article helpful?
Shona McCombes
Other students also liked, what is a theoretical framework | guide to organizing, what is a research design | types, guide & examples, qualitative vs. quantitative research | differences, examples & methods, what is your plagiarism score.
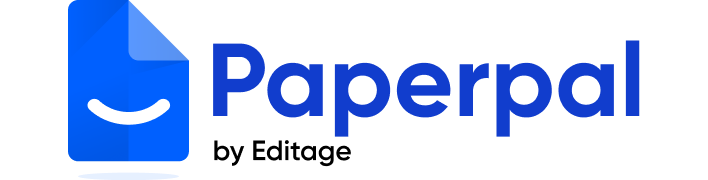
What is Research Methodology? Definition, Types, and Examples
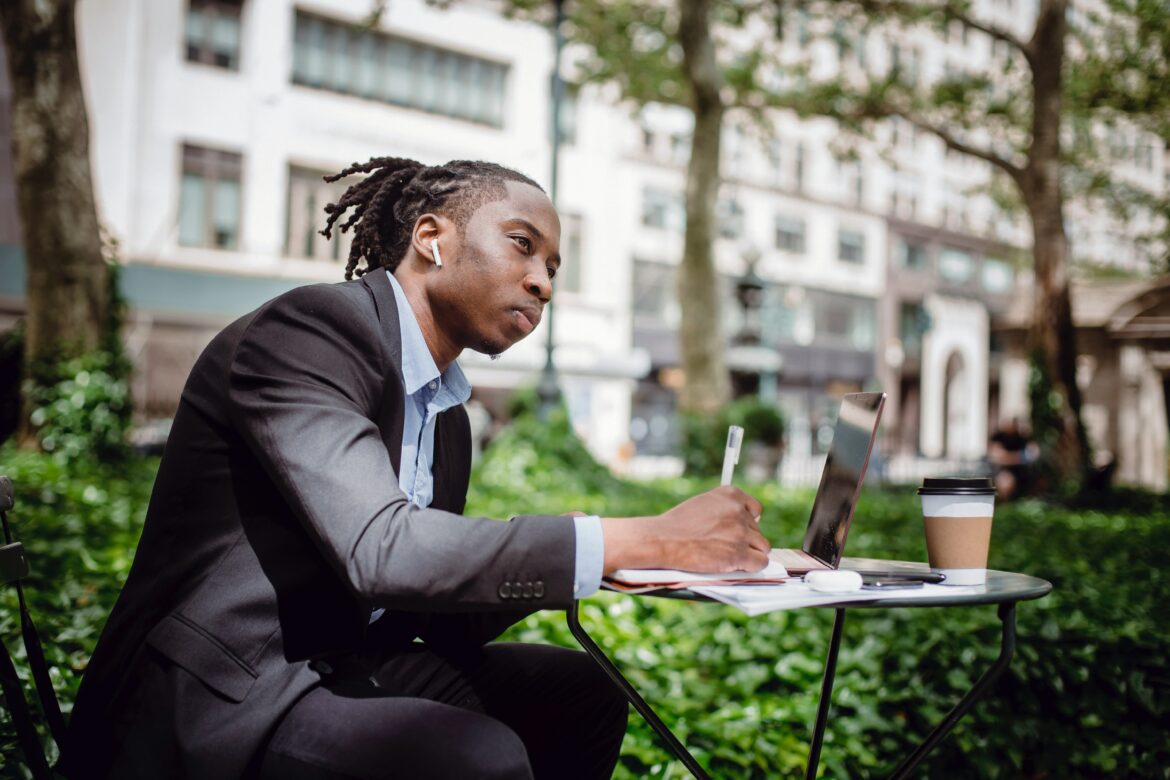
Research methodology 1,2 is a structured and scientific approach used to collect, analyze, and interpret quantitative or qualitative data to answer research questions or test hypotheses. A research methodology is like a plan for carrying out research and helps keep researchers on track by limiting the scope of the research. Several aspects must be considered before selecting an appropriate research methodology, such as research limitations and ethical concerns that may affect your research.
The research methodology section in a scientific paper describes the different methodological choices made, such as the data collection and analysis methods, and why these choices were selected. The reasons should explain why the methods chosen are the most appropriate to answer the research question. A good research methodology also helps ensure the reliability and validity of the research findings. There are three types of research methodology—quantitative, qualitative, and mixed-method, which can be chosen based on the research objectives.
What is research methodology ?
A research methodology describes the techniques and procedures used to identify and analyze information regarding a specific research topic. It is a process by which researchers design their study so that they can achieve their objectives using the selected research instruments. It includes all the important aspects of research, including research design, data collection methods, data analysis methods, and the overall framework within which the research is conducted. While these points can help you understand what is research methodology, you also need to know why it is important to pick the right methodology.
Why is research methodology important?
Having a good research methodology in place has the following advantages: 3
- Helps other researchers who may want to replicate your research; the explanations will be of benefit to them.
- You can easily answer any questions about your research if they arise at a later stage.
- A research methodology provides a framework and guidelines for researchers to clearly define research questions, hypotheses, and objectives.
- It helps researchers identify the most appropriate research design, sampling technique, and data collection and analysis methods.
- A sound research methodology helps researchers ensure that their findings are valid and reliable and free from biases and errors.
- It also helps ensure that ethical guidelines are followed while conducting research.
- A good research methodology helps researchers in planning their research efficiently, by ensuring optimum usage of their time and resources.
Writing the methods section of a research paper? Let Paperpal help you achieve perfection
Types of research methodology.
There are three types of research methodology based on the type of research and the data required. 1
- Quantitative research methodology focuses on measuring and testing numerical data. This approach is good for reaching a large number of people in a short amount of time. This type of research helps in testing the causal relationships between variables, making predictions, and generalizing results to wider populations.
- Qualitative research methodology examines the opinions, behaviors, and experiences of people. It collects and analyzes words and textual data. This research methodology requires fewer participants but is still more time consuming because the time spent per participant is quite large. This method is used in exploratory research where the research problem being investigated is not clearly defined.
- Mixed-method research methodology uses the characteristics of both quantitative and qualitative research methodologies in the same study. This method allows researchers to validate their findings, verify if the results observed using both methods are complementary, and explain any unexpected results obtained from one method by using the other method.
What are the types of sampling designs in research methodology?
Sampling 4 is an important part of a research methodology and involves selecting a representative sample of the population to conduct the study, making statistical inferences about them, and estimating the characteristics of the whole population based on these inferences. There are two types of sampling designs in research methodology—probability and nonprobability.
- Probability sampling
In this type of sampling design, a sample is chosen from a larger population using some form of random selection, that is, every member of the population has an equal chance of being selected. The different types of probability sampling are:
- Systematic —sample members are chosen at regular intervals. It requires selecting a starting point for the sample and sample size determination that can be repeated at regular intervals. This type of sampling method has a predefined range; hence, it is the least time consuming.
- Stratified —researchers divide the population into smaller groups that don’t overlap but represent the entire population. While sampling, these groups can be organized, and then a sample can be drawn from each group separately.
- Cluster —the population is divided into clusters based on demographic parameters like age, sex, location, etc.
- Convenience —selects participants who are most easily accessible to researchers due to geographical proximity, availability at a particular time, etc.
- Purposive —participants are selected at the researcher’s discretion. Researchers consider the purpose of the study and the understanding of the target audience.
- Snowball —already selected participants use their social networks to refer the researcher to other potential participants.
- Quota —while designing the study, the researchers decide how many people with which characteristics to include as participants. The characteristics help in choosing people most likely to provide insights into the subject.
What are data collection methods?
During research, data are collected using various methods depending on the research methodology being followed and the research methods being undertaken. Both qualitative and quantitative research have different data collection methods, as listed below.
Qualitative research 5
- One-on-one interviews: Helps the interviewers understand a respondent’s subjective opinion and experience pertaining to a specific topic or event
- Document study/literature review/record keeping: Researchers’ review of already existing written materials such as archives, annual reports, research articles, guidelines, policy documents, etc.
- Focus groups: Constructive discussions that usually include a small sample of about 6-10 people and a moderator, to understand the participants’ opinion on a given topic.
- Qualitative observation : Researchers collect data using their five senses (sight, smell, touch, taste, and hearing).
Quantitative research 6
- Sampling: The most common type is probability sampling.
- Interviews: Commonly telephonic or done in-person.
- Observations: Structured observations are most commonly used in quantitative research. In this method, researchers make observations about specific behaviors of individuals in a structured setting.
- Document review: Reviewing existing research or documents to collect evidence for supporting the research.
- Surveys and questionnaires. Surveys can be administered both online and offline depending on the requirement and sample size.
Let Paperpal help you write the perfect research methods section. Start now!
What are data analysis methods.
The data collected using the various methods for qualitative and quantitative research need to be analyzed to generate meaningful conclusions. These data analysis methods 7 also differ between quantitative and qualitative research.
Quantitative research involves a deductive method for data analysis where hypotheses are developed at the beginning of the research and precise measurement is required. The methods include statistical analysis applications to analyze numerical data and are grouped into two categories—descriptive and inferential.
Descriptive analysis is used to describe the basic features of different types of data to present it in a way that ensures the patterns become meaningful. The different types of descriptive analysis methods are:
- Measures of frequency (count, percent, frequency)
- Measures of central tendency (mean, median, mode)
- Measures of dispersion or variation (range, variance, standard deviation)
- Measure of position (percentile ranks, quartile ranks)
Inferential analysis is used to make predictions about a larger population based on the analysis of the data collected from a smaller population. This analysis is used to study the relationships between different variables. Some commonly used inferential data analysis methods are:
- Correlation: To understand the relationship between two or more variables.
- Cross-tabulation: Analyze the relationship between multiple variables.
- Regression analysis: Study the impact of independent variables on the dependent variable.
- Frequency tables: To understand the frequency of data.
- Analysis of variance: To test the degree to which two or more variables differ in an experiment.
Qualitative research involves an inductive method for data analysis where hypotheses are developed after data collection. The methods include:
- Content analysis: For analyzing documented information from text and images by determining the presence of certain words or concepts in texts.
- Narrative analysis: For analyzing content obtained from sources such as interviews, field observations, and surveys. The stories and opinions shared by people are used to answer research questions.
- Discourse analysis: For analyzing interactions with people considering the social context, that is, the lifestyle and environment, under which the interaction occurs.
- Grounded theory: Involves hypothesis creation by data collection and analysis to explain why a phenomenon occurred.
- Thematic analysis: To identify important themes or patterns in data and use these to address an issue.
How to choose a research methodology?
Here are some important factors to consider when choosing a research methodology: 8
- Research objectives, aims, and questions —these would help structure the research design.
- Review existing literature to identify any gaps in knowledge.
- Check the statistical requirements —if data-driven or statistical results are needed then quantitative research is the best. If the research questions can be answered based on people’s opinions and perceptions, then qualitative research is most suitable.
- Sample size —sample size can often determine the feasibility of a research methodology. For a large sample, less effort- and time-intensive methods are appropriate.
- Constraints —constraints of time, geography, and resources can help define the appropriate methodology.
Got writer’s block? Kickstart your research paper writing with Paperpal now!
How to write a research methodology .
A research methodology should include the following components: 3,9
- Research design —should be selected based on the research question and the data required. Common research designs include experimental, quasi-experimental, correlational, descriptive, and exploratory.
- Research method —this can be quantitative, qualitative, or mixed-method.
- Reason for selecting a specific methodology —explain why this methodology is the most suitable to answer your research problem.
- Research instruments —explain the research instruments you plan to use, mainly referring to the data collection methods such as interviews, surveys, etc. Here as well, a reason should be mentioned for selecting the particular instrument.
- Sampling —this involves selecting a representative subset of the population being studied.
- Data collection —involves gathering data using several data collection methods, such as surveys, interviews, etc.
- Data analysis —describe the data analysis methods you will use once you’ve collected the data.
- Research limitations —mention any limitations you foresee while conducting your research.
- Validity and reliability —validity helps identify the accuracy and truthfulness of the findings; reliability refers to the consistency and stability of the results over time and across different conditions.
- Ethical considerations —research should be conducted ethically. The considerations include obtaining consent from participants, maintaining confidentiality, and addressing conflicts of interest.
Streamline Your Research Paper Writing Process with Paperpal
The methods section is a critical part of the research papers, allowing researchers to use this to understand your findings and replicate your work when pursuing their own research. However, it is usually also the most difficult section to write. This is where Paperpal can help you overcome the writer’s block and create the first draft in minutes with Paperpal Copilot, its secure generative AI feature suite.
With Paperpal you can get research advice, write and refine your work, rephrase and verify the writing, and ensure submission readiness, all in one place. Here’s how you can use Paperpal to develop the first draft of your methods section.
- Generate an outline: Input some details about your research to instantly generate an outline for your methods section
- Develop the section: Use the outline and suggested sentence templates to expand your ideas and develop the first draft.
- P araph ras e and trim : Get clear, concise academic text with paraphrasing that conveys your work effectively and word reduction to fix redundancies.
- Choose the right words: Enhance text by choosing contextual synonyms based on how the words have been used in previously published work.
- Check and verify text : Make sure the generated text showcases your methods correctly, has all the right citations, and is original and authentic. .
You can repeat this process to develop each section of your research manuscript, including the title, abstract and keywords. Ready to write your research papers faster, better, and without the stress? Sign up for Paperpal and start writing today!
Frequently Asked Questions
Q1. What are the key components of research methodology?
A1. A good research methodology has the following key components:
- Research design
- Data collection procedures
- Data analysis methods
- Ethical considerations
Q2. Why is ethical consideration important in research methodology?
A2. Ethical consideration is important in research methodology to ensure the readers of the reliability and validity of the study. Researchers must clearly mention the ethical norms and standards followed during the conduct of the research and also mention if the research has been cleared by any institutional board. The following 10 points are the important principles related to ethical considerations: 10
- Participants should not be subjected to harm.
- Respect for the dignity of participants should be prioritized.
- Full consent should be obtained from participants before the study.
- Participants’ privacy should be ensured.
- Confidentiality of the research data should be ensured.
- Anonymity of individuals and organizations participating in the research should be maintained.
- The aims and objectives of the research should not be exaggerated.
- Affiliations, sources of funding, and any possible conflicts of interest should be declared.
- Communication in relation to the research should be honest and transparent.
- Misleading information and biased representation of primary data findings should be avoided.
Q3. What is the difference between methodology and method?
A3. Research methodology is different from a research method, although both terms are often confused. Research methods are the tools used to gather data, while the research methodology provides a framework for how research is planned, conducted, and analyzed. The latter guides researchers in making decisions about the most appropriate methods for their research. Research methods refer to the specific techniques, procedures, and tools used by researchers to collect, analyze, and interpret data, for instance surveys, questionnaires, interviews, etc.
Research methodology is, thus, an integral part of a research study. It helps ensure that you stay on track to meet your research objectives and answer your research questions using the most appropriate data collection and analysis tools based on your research design.
Accelerate your research paper writing with Paperpal. Try for free now!
- Research methodologies. Pfeiffer Library website. Accessed August 15, 2023. https://library.tiffin.edu/researchmethodologies/whatareresearchmethodologies
- Types of research methodology. Eduvoice website. Accessed August 16, 2023. https://eduvoice.in/types-research-methodology/
- The basics of research methodology: A key to quality research. Voxco. Accessed August 16, 2023. https://www.voxco.com/blog/what-is-research-methodology/
- Sampling methods: Types with examples. QuestionPro website. Accessed August 16, 2023. https://www.questionpro.com/blog/types-of-sampling-for-social-research/
- What is qualitative research? Methods, types, approaches, examples. Researcher.Life blog. Accessed August 15, 2023. https://researcher.life/blog/article/what-is-qualitative-research-methods-types-examples/
- What is quantitative research? Definition, methods, types, and examples. Researcher.Life blog. Accessed August 15, 2023. https://researcher.life/blog/article/what-is-quantitative-research-types-and-examples/
- Data analysis in research: Types & methods. QuestionPro website. Accessed August 16, 2023. https://www.questionpro.com/blog/data-analysis-in-research/#Data_analysis_in_qualitative_research
- Factors to consider while choosing the right research methodology. PhD Monster website. Accessed August 17, 2023. https://www.phdmonster.com/factors-to-consider-while-choosing-the-right-research-methodology/
- What is research methodology? Research and writing guides. Accessed August 14, 2023. https://paperpile.com/g/what-is-research-methodology/
- Ethical considerations. Business research methodology website. Accessed August 17, 2023. https://research-methodology.net/research-methodology/ethical-considerations/
Paperpal is a comprehensive AI writing toolkit that helps students and researchers achieve 2x the writing in half the time. It leverages 21+ years of STM experience and insights from millions of research articles to provide in-depth academic writing, language editing, and submission readiness support to help you write better, faster.
Get accurate academic translations, rewriting support, grammar checks, vocabulary suggestions, and generative AI assistance that delivers human precision at machine speed. Try for free or upgrade to Paperpal Prime starting at US$19 a month to access premium features, including consistency, plagiarism, and 30+ submission readiness checks to help you succeed.
Experience the future of academic writing – Sign up to Paperpal and start writing for free!
Related Reads:
- Dangling Modifiers and How to Avoid Them in Your Writing
- Webinar: How to Use Generative AI Tools Ethically in Your Academic Writing
- Research Outlines: How to Write An Introduction Section in Minutes with Paperpal Copilot
- How to Paraphrase Research Papers Effectively
Language and Grammar Rules for Academic Writing
Climatic vs. climactic: difference and examples, you may also like, dissertation printing and binding | types & comparison , what is a dissertation preface definition and examples , how to write a research proposal: (with examples..., how to write your research paper in apa..., how to choose a dissertation topic, how to write a phd research proposal, how to write an academic paragraph (step-by-step guide), maintaining academic integrity with paperpal’s generative ai writing..., research funding basics: what should a grant proposal..., how to write an abstract in research papers....
- USC Libraries
- Research Guides
Organizing Your Social Sciences Research Paper
- 6. The Methodology
- Purpose of Guide
- Design Flaws to Avoid
- Independent and Dependent Variables
- Glossary of Research Terms
- Reading Research Effectively
- Narrowing a Topic Idea
- Broadening a Topic Idea
- Extending the Timeliness of a Topic Idea
- Academic Writing Style
- Applying Critical Thinking
- Choosing a Title
- Making an Outline
- Paragraph Development
- Research Process Video Series
- Executive Summary
- The C.A.R.S. Model
- Background Information
- The Research Problem/Question
- Theoretical Framework
- Citation Tracking
- Content Alert Services
- Evaluating Sources
- Primary Sources
- Secondary Sources
- Tiertiary Sources
- Scholarly vs. Popular Publications
- Qualitative Methods
- Quantitative Methods
- Insiderness
- Using Non-Textual Elements
- Limitations of the Study
- Common Grammar Mistakes
- Writing Concisely
- Avoiding Plagiarism
- Footnotes or Endnotes?
- Further Readings
- Generative AI and Writing
- USC Libraries Tutorials and Other Guides
- Bibliography
The methods section describes actions taken to investigate a research problem and the rationale for the application of specific procedures or techniques used to identify, select, process, and analyze information applied to understanding the problem, thereby, allowing the reader to critically evaluate a study’s overall validity and reliability. The methodology section of a research paper answers two main questions: How was the data collected or generated? And, how was it analyzed? The writing should be direct and precise and always written in the past tense.
Kallet, Richard H. "How to Write the Methods Section of a Research Paper." Respiratory Care 49 (October 2004): 1229-1232.
Importance of a Good Methodology Section
You must explain how you obtained and analyzed your results for the following reasons:
- Readers need to know how the data was obtained because the method you chose affects the results and, by extension, how you interpreted their significance in the discussion section of your paper.
- Methodology is crucial for any branch of scholarship because an unreliable method produces unreliable results and, as a consequence, undermines the value of your analysis of the findings.
- In most cases, there are a variety of different methods you can choose to investigate a research problem. The methodology section of your paper should clearly articulate the reasons why you have chosen a particular procedure or technique.
- The reader wants to know that the data was collected or generated in a way that is consistent with accepted practice in the field of study. For example, if you are using a multiple choice questionnaire, readers need to know that it offered your respondents a reasonable range of answers to choose from.
- The method must be appropriate to fulfilling the overall aims of the study. For example, you need to ensure that you have a large enough sample size to be able to generalize and make recommendations based upon the findings.
- The methodology should discuss the problems that were anticipated and the steps you took to prevent them from occurring. For any problems that do arise, you must describe the ways in which they were minimized or why these problems do not impact in any meaningful way your interpretation of the findings.
- In the social and behavioral sciences, it is important to always provide sufficient information to allow other researchers to adopt or replicate your methodology. This information is particularly important when a new method has been developed or an innovative use of an existing method is utilized.
Bem, Daryl J. Writing the Empirical Journal Article. Psychology Writing Center. University of Washington; Denscombe, Martyn. The Good Research Guide: For Small-Scale Social Research Projects . 5th edition. Buckingham, UK: Open University Press, 2014; Lunenburg, Frederick C. Writing a Successful Thesis or Dissertation: Tips and Strategies for Students in the Social and Behavioral Sciences . Thousand Oaks, CA: Corwin Press, 2008.
Structure and Writing Style
I. Groups of Research Methods
There are two main groups of research methods in the social sciences:
- The e mpirical-analytical group approaches the study of social sciences in a similar manner that researchers study the natural sciences . This type of research focuses on objective knowledge, research questions that can be answered yes or no, and operational definitions of variables to be measured. The empirical-analytical group employs deductive reasoning that uses existing theory as a foundation for formulating hypotheses that need to be tested. This approach is focused on explanation.
- The i nterpretative group of methods is focused on understanding phenomenon in a comprehensive, holistic way . Interpretive methods focus on analytically disclosing the meaning-making practices of human subjects [the why, how, or by what means people do what they do], while showing how those practices arrange so that it can be used to generate observable outcomes. Interpretive methods allow you to recognize your connection to the phenomena under investigation. However, the interpretative group requires careful examination of variables because it focuses more on subjective knowledge.
II. Content
The introduction to your methodology section should begin by restating the research problem and underlying assumptions underpinning your study. This is followed by situating the methods you used to gather, analyze, and process information within the overall “tradition” of your field of study and within the particular research design you have chosen to study the problem. If the method you choose lies outside of the tradition of your field [i.e., your review of the literature demonstrates that the method is not commonly used], provide a justification for how your choice of methods specifically addresses the research problem in ways that have not been utilized in prior studies.
The remainder of your methodology section should describe the following:
- Decisions made in selecting the data you have analyzed or, in the case of qualitative research, the subjects and research setting you have examined,
- Tools and methods used to identify and collect information, and how you identified relevant variables,
- The ways in which you processed the data and the procedures you used to analyze that data, and
- The specific research tools or strategies that you utilized to study the underlying hypothesis and research questions.
In addition, an effectively written methodology section should:
- Introduce the overall methodological approach for investigating your research problem . Is your study qualitative or quantitative or a combination of both (mixed method)? Are you going to take a special approach, such as action research, or a more neutral stance?
- Indicate how the approach fits the overall research design . Your methods for gathering data should have a clear connection to your research problem. In other words, make sure that your methods will actually address the problem. One of the most common deficiencies found in research papers is that the proposed methodology is not suitable to achieving the stated objective of your paper.
- Describe the specific methods of data collection you are going to use , such as, surveys, interviews, questionnaires, observation, archival research. If you are analyzing existing data, such as a data set or archival documents, describe how it was originally created or gathered and by whom. Also be sure to explain how older data is still relevant to investigating the current research problem.
- Explain how you intend to analyze your results . Will you use statistical analysis? Will you use specific theoretical perspectives to help you analyze a text or explain observed behaviors? Describe how you plan to obtain an accurate assessment of relationships, patterns, trends, distributions, and possible contradictions found in the data.
- Provide background and a rationale for methodologies that are unfamiliar for your readers . Very often in the social sciences, research problems and the methods for investigating them require more explanation/rationale than widely accepted rules governing the natural and physical sciences. Be clear and concise in your explanation.
- Provide a justification for subject selection and sampling procedure . For instance, if you propose to conduct interviews, how do you intend to select the sample population? If you are analyzing texts, which texts have you chosen, and why? If you are using statistics, why is this set of data being used? If other data sources exist, explain why the data you chose is most appropriate to addressing the research problem.
- Provide a justification for case study selection . A common method of analyzing research problems in the social sciences is to analyze specific cases. These can be a person, place, event, phenomenon, or other type of subject of analysis that are either examined as a singular topic of in-depth investigation or multiple topics of investigation studied for the purpose of comparing or contrasting findings. In either method, you should explain why a case or cases were chosen and how they specifically relate to the research problem.
- Describe potential limitations . Are there any practical limitations that could affect your data collection? How will you attempt to control for potential confounding variables and errors? If your methodology may lead to problems you can anticipate, state this openly and show why pursuing this methodology outweighs the risk of these problems cropping up.
NOTE: Once you have written all of the elements of the methods section, subsequent revisions should focus on how to present those elements as clearly and as logically as possibly. The description of how you prepared to study the research problem, how you gathered the data, and the protocol for analyzing the data should be organized chronologically. For clarity, when a large amount of detail must be presented, information should be presented in sub-sections according to topic. If necessary, consider using appendices for raw data.
ANOTHER NOTE: If you are conducting a qualitative analysis of a research problem , the methodology section generally requires a more elaborate description of the methods used as well as an explanation of the processes applied to gathering and analyzing of data than is generally required for studies using quantitative methods. Because you are the primary instrument for generating the data [e.g., through interviews or observations], the process for collecting that data has a significantly greater impact on producing the findings. Therefore, qualitative research requires a more detailed description of the methods used.
YET ANOTHER NOTE: If your study involves interviews, observations, or other qualitative techniques involving human subjects , you may be required to obtain approval from the university's Office for the Protection of Research Subjects before beginning your research. This is not a common procedure for most undergraduate level student research assignments. However, i f your professor states you need approval, you must include a statement in your methods section that you received official endorsement and adequate informed consent from the office and that there was a clear assessment and minimization of risks to participants and to the university. This statement informs the reader that your study was conducted in an ethical and responsible manner. In some cases, the approval notice is included as an appendix to your paper.
III. Problems to Avoid
Irrelevant Detail The methodology section of your paper should be thorough but concise. Do not provide any background information that does not directly help the reader understand why a particular method was chosen, how the data was gathered or obtained, and how the data was analyzed in relation to the research problem [note: analyzed, not interpreted! Save how you interpreted the findings for the discussion section]. With this in mind, the page length of your methods section will generally be less than any other section of your paper except the conclusion.
Unnecessary Explanation of Basic Procedures Remember that you are not writing a how-to guide about a particular method. You should make the assumption that readers possess a basic understanding of how to investigate the research problem on their own and, therefore, you do not have to go into great detail about specific methodological procedures. The focus should be on how you applied a method , not on the mechanics of doing a method. An exception to this rule is if you select an unconventional methodological approach; if this is the case, be sure to explain why this approach was chosen and how it enhances the overall process of discovery.
Problem Blindness It is almost a given that you will encounter problems when collecting or generating your data, or, gaps will exist in existing data or archival materials. Do not ignore these problems or pretend they did not occur. Often, documenting how you overcame obstacles can form an interesting part of the methodology. It demonstrates to the reader that you can provide a cogent rationale for the decisions you made to minimize the impact of any problems that arose.
Literature Review Just as the literature review section of your paper provides an overview of sources you have examined while researching a particular topic, the methodology section should cite any sources that informed your choice and application of a particular method [i.e., the choice of a survey should include any citations to the works you used to help construct the survey].
It’s More than Sources of Information! A description of a research study's method should not be confused with a description of the sources of information. Such a list of sources is useful in and of itself, especially if it is accompanied by an explanation about the selection and use of the sources. The description of the project's methodology complements a list of sources in that it sets forth the organization and interpretation of information emanating from those sources.
Azevedo, L.F. et al. "How to Write a Scientific Paper: Writing the Methods Section." Revista Portuguesa de Pneumologia 17 (2011): 232-238; Blair Lorrie. “Choosing a Methodology.” In Writing a Graduate Thesis or Dissertation , Teaching Writing Series. (Rotterdam: Sense Publishers 2016), pp. 49-72; Butin, Dan W. The Education Dissertation A Guide for Practitioner Scholars . Thousand Oaks, CA: Corwin, 2010; Carter, Susan. Structuring Your Research Thesis . New York: Palgrave Macmillan, 2012; Kallet, Richard H. “How to Write the Methods Section of a Research Paper.” Respiratory Care 49 (October 2004):1229-1232; Lunenburg, Frederick C. Writing a Successful Thesis or Dissertation: Tips and Strategies for Students in the Social and Behavioral Sciences . Thousand Oaks, CA: Corwin Press, 2008. Methods Section. The Writer’s Handbook. Writing Center. University of Wisconsin, Madison; Rudestam, Kjell Erik and Rae R. Newton. “The Method Chapter: Describing Your Research Plan.” In Surviving Your Dissertation: A Comprehensive Guide to Content and Process . (Thousand Oaks, Sage Publications, 2015), pp. 87-115; What is Interpretive Research. Institute of Public and International Affairs, University of Utah; Writing the Experimental Report: Methods, Results, and Discussion. The Writing Lab and The OWL. Purdue University; Methods and Materials. The Structure, Format, Content, and Style of a Journal-Style Scientific Paper. Department of Biology. Bates College.
Writing Tip
Statistical Designs and Tests? Do Not Fear Them!
Don't avoid using a quantitative approach to analyzing your research problem just because you fear the idea of applying statistical designs and tests. A qualitative approach, such as conducting interviews or content analysis of archival texts, can yield exciting new insights about a research problem, but it should not be undertaken simply because you have a disdain for running a simple regression. A well designed quantitative research study can often be accomplished in very clear and direct ways, whereas, a similar study of a qualitative nature usually requires considerable time to analyze large volumes of data and a tremendous burden to create new paths for analysis where previously no path associated with your research problem had existed.
To locate data and statistics, GO HERE .
Another Writing Tip
Knowing the Relationship Between Theories and Methods
There can be multiple meaning associated with the term "theories" and the term "methods" in social sciences research. A helpful way to delineate between them is to understand "theories" as representing different ways of characterizing the social world when you research it and "methods" as representing different ways of generating and analyzing data about that social world. Framed in this way, all empirical social sciences research involves theories and methods, whether they are stated explicitly or not. However, while theories and methods are often related, it is important that, as a researcher, you deliberately separate them in order to avoid your theories playing a disproportionate role in shaping what outcomes your chosen methods produce.
Introspectively engage in an ongoing dialectic between the application of theories and methods to help enable you to use the outcomes from your methods to interrogate and develop new theories, or ways of framing conceptually the research problem. This is how scholarship grows and branches out into new intellectual territory.
Reynolds, R. Larry. Ways of Knowing. Alternative Microeconomics . Part 1, Chapter 3. Boise State University; The Theory-Method Relationship. S-Cool Revision. United Kingdom.
Yet Another Writing Tip
Methods and the Methodology
Do not confuse the terms "methods" and "methodology." As Schneider notes, a method refers to the technical steps taken to do research . Descriptions of methods usually include defining and stating why you have chosen specific techniques to investigate a research problem, followed by an outline of the procedures you used to systematically select, gather, and process the data [remember to always save the interpretation of data for the discussion section of your paper].
The methodology refers to a discussion of the underlying reasoning why particular methods were used . This discussion includes describing the theoretical concepts that inform the choice of methods to be applied, placing the choice of methods within the more general nature of academic work, and reviewing its relevance to examining the research problem. The methodology section also includes a thorough review of the methods other scholars have used to study the topic.
Bryman, Alan. "Of Methods and Methodology." Qualitative Research in Organizations and Management: An International Journal 3 (2008): 159-168; Schneider, Florian. “What's in a Methodology: The Difference between Method, Methodology, and Theory…and How to Get the Balance Right?” PoliticsEastAsia.com. Chinese Department, University of Leiden, Netherlands.
- << Previous: Scholarly vs. Popular Publications
- Next: Qualitative Methods >>
- Last Updated: Aug 21, 2024 8:54 AM
- URL: https://libguides.usc.edu/writingguide
- How it works
"Christmas Offer"
Terms & conditions.
As the Christmas season is upon us, we find ourselves reflecting on the past year and those who we have helped to shape their future. It’s been quite a year for us all! The end of the year brings no greater joy than the opportunity to express to you Christmas greetings and good wishes.
At this special time of year, Research Prospect brings joyful discount of 10% on all its services. May your Christmas and New Year be filled with joy.
We are looking back with appreciation for your loyalty and looking forward to moving into the New Year together.
"Claim this offer"
In unfamiliar and hard times, we have stuck by you. This Christmas, Research Prospect brings you all the joy with exciting discount of 10% on all its services.
Offer valid till 5-1-2024
We love being your partner in success. We know you have been working hard lately, take a break this holiday season to spend time with your loved ones while we make sure you succeed in your academics
Discount code: RP23720
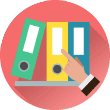
Published by Nicolas at March 21st, 2024 , Revised On March 12, 2024
The Ultimate Guide To Research Methodology
Research methodology is a crucial aspect of any investigative process, serving as the blueprint for the entire research journey. If you are stuck in the methodology section of your research paper , then this blog will guide you on what is a research methodology, its types and how to successfully conduct one.
Table of Contents
What Is Research Methodology?
Research methodology can be defined as the systematic framework that guides researchers in designing, conducting, and analyzing their investigations. It encompasses a structured set of processes, techniques, and tools employed to gather and interpret data, ensuring the reliability and validity of the research findings.
Research methodology is not confined to a singular approach; rather, it encapsulates a diverse range of methods tailored to the specific requirements of the research objectives.
Here is why Research methodology is important in academic and professional settings.
Facilitating Rigorous Inquiry
Research methodology forms the backbone of rigorous inquiry. It provides a structured approach that aids researchers in formulating precise thesis statements , selecting appropriate methodologies, and executing systematic investigations. This, in turn, enhances the quality and credibility of the research outcomes.
Ensuring Reproducibility And Reliability
In both academic and professional contexts, the ability to reproduce research outcomes is paramount. A well-defined research methodology establishes clear procedures, making it possible for others to replicate the study. This not only validates the findings but also contributes to the cumulative nature of knowledge.
Guiding Decision-Making Processes
In professional settings, decisions often hinge on reliable data and insights. Research methodology equips professionals with the tools to gather pertinent information, analyze it rigorously, and derive meaningful conclusions.
This informed decision-making is instrumental in achieving organizational goals and staying ahead in competitive environments.
Contributing To Academic Excellence
For academic researchers, adherence to robust research methodology is a hallmark of excellence. Institutions value research that adheres to high standards of methodology, fostering a culture of academic rigour and intellectual integrity. Furthermore, it prepares students with critical skills applicable beyond academia.
Enhancing Problem-Solving Abilities
Research methodology instills a problem-solving mindset by encouraging researchers to approach challenges systematically. It equips individuals with the skills to dissect complex issues, formulate hypotheses , and devise effective strategies for investigation.
Understanding Research Methodology
In the pursuit of knowledge and discovery, understanding the fundamentals of research methodology is paramount.
Basics Of Research
Research, in its essence, is a systematic and organized process of inquiry aimed at expanding our understanding of a particular subject or phenomenon. It involves the exploration of existing knowledge, the formulation of hypotheses, and the collection and analysis of data to draw meaningful conclusions.
Research is a dynamic and iterative process that contributes to the continuous evolution of knowledge in various disciplines.
Types of Research
Research takes on various forms, each tailored to the nature of the inquiry. Broadly classified, research can be categorized into two main types:
- Quantitative Research: This type involves the collection and analysis of numerical data to identify patterns, relationships, and statistical significance. It is particularly useful for testing hypotheses and making predictions.
- Qualitative Research: Qualitative research focuses on understanding the depth and details of a phenomenon through non-numerical data. It often involves methods such as interviews, focus groups, and content analysis, providing rich insights into complex issues.
Components Of Research Methodology
To conduct effective research, one must go through the different components of research methodology. These components form the scaffolding that supports the entire research process, ensuring its coherence and validity.
Research Design
Research design serves as the blueprint for the entire research project. It outlines the overall structure and strategy for conducting the study. The three primary types of research design are:
- Exploratory Research: Aimed at gaining insights and familiarity with the topic, often used in the early stages of research.
- Descriptive Research: Involves portraying an accurate profile of a situation or phenomenon, answering the ‘what,’ ‘who,’ ‘where,’ and ‘when’ questions.
- Explanatory Research: Seeks to identify the causes and effects of a phenomenon, explaining the ‘why’ and ‘how.’
Data Collection Methods
Choosing the right data collection methods is crucial for obtaining reliable and relevant information. Common methods include:
- Surveys and Questionnaires: Employed to gather information from a large number of respondents through standardized questions.
- Interviews: In-depth conversations with participants, offering qualitative insights.
- Observation: Systematic watching and recording of behaviour, events, or processes in their natural setting.
Data Analysis Techniques
Once data is collected, analysis becomes imperative to derive meaningful conclusions. Different methodologies exist for quantitative and qualitative data:
- Quantitative Data Analysis: Involves statistical techniques such as descriptive statistics, inferential statistics, and regression analysis to interpret numerical data.
- Qualitative Data Analysis: Methods like content analysis, thematic analysis, and grounded theory are employed to extract patterns, themes, and meanings from non-numerical data.
The research paper we write have:
- Precision and Clarity
- Zero Plagiarism
- High-level Encryption
- Authentic Sources
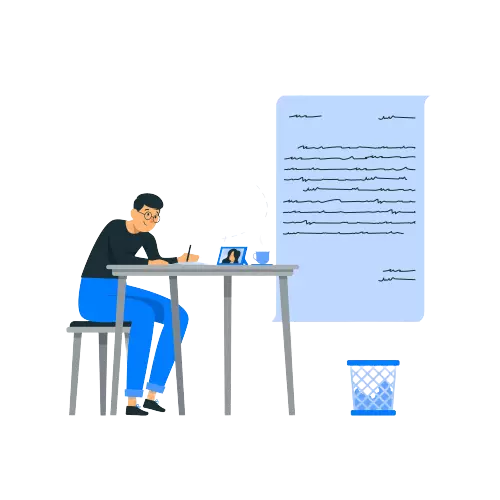
Choosing a Research Method
Selecting an appropriate research method is a critical decision in the research process. It determines the approach, tools, and techniques that will be used to answer the research questions.
Quantitative Research Methods
Quantitative research involves the collection and analysis of numerical data, providing a structured and objective approach to understanding and explaining phenomena.
Experimental Research
Experimental research involves manipulating variables to observe the effect on another variable under controlled conditions. It aims to establish cause-and-effect relationships.
Key Characteristics:
- Controlled Environment: Experiments are conducted in a controlled setting to minimize external influences.
- Random Assignment: Participants are randomly assigned to different experimental conditions.
- Quantitative Data: Data collected is numerical, allowing for statistical analysis.
Applications: Commonly used in scientific studies and psychology to test hypotheses and identify causal relationships.
Survey Research
Survey research gathers information from a sample of individuals through standardized questionnaires or interviews. It aims to collect data on opinions, attitudes, and behaviours.
- Structured Instruments: Surveys use structured instruments, such as questionnaires, to collect data.
- Large Sample Size: Surveys often target a large and diverse group of participants.
- Quantitative Data Analysis: Responses are quantified for statistical analysis.
Applications: Widely employed in social sciences, marketing, and public opinion research to understand trends and preferences.
Descriptive Research
Descriptive research seeks to portray an accurate profile of a situation or phenomenon. It focuses on answering the ‘what,’ ‘who,’ ‘where,’ and ‘when’ questions.
- Observation and Data Collection: This involves observing and documenting without manipulating variables.
- Objective Description: Aim to provide an unbiased and factual account of the subject.
- Quantitative or Qualitative Data: T his can include both types of data, depending on the research focus.
Applications: Useful in situations where researchers want to understand and describe a phenomenon without altering it, common in social sciences and education.
Qualitative Research Methods
Qualitative research emphasizes exploring and understanding the depth and complexity of phenomena through non-numerical data.
A case study is an in-depth exploration of a particular person, group, event, or situation. It involves detailed, context-rich analysis.
- Rich Data Collection: Uses various data sources, such as interviews, observations, and documents.
- Contextual Understanding: Aims to understand the context and unique characteristics of the case.
- Holistic Approach: Examines the case in its entirety.
Applications: Common in social sciences, psychology, and business to investigate complex and specific instances.
Ethnography
Ethnography involves immersing the researcher in the culture or community being studied to gain a deep understanding of their behaviours, beliefs, and practices.
- Participant Observation: Researchers actively participate in the community or setting.
- Holistic Perspective: Focuses on the interconnectedness of cultural elements.
- Qualitative Data: In-depth narratives and descriptions are central to ethnographic studies.
Applications: Widely used in anthropology, sociology, and cultural studies to explore and document cultural practices.
Grounded Theory
Grounded theory aims to develop theories grounded in the data itself. It involves systematic data collection and analysis to construct theories from the ground up.
- Constant Comparison: Data is continually compared and analyzed during the research process.
- Inductive Reasoning: Theories emerge from the data rather than being imposed on it.
- Iterative Process: The research design evolves as the study progresses.
Applications: Commonly applied in sociology, nursing, and management studies to generate theories from empirical data.
Research design is the structural framework that outlines the systematic process and plan for conducting a study. It serves as the blueprint, guiding researchers on how to collect, analyze, and interpret data.
Exploratory, Descriptive, And Explanatory Designs
Exploratory design.
Exploratory research design is employed when a researcher aims to explore a relatively unknown subject or gain insights into a complex phenomenon.
- Flexibility: Allows for flexibility in data collection and analysis.
- Open-Ended Questions: Uses open-ended questions to gather a broad range of information.
- Preliminary Nature: Often used in the initial stages of research to formulate hypotheses.
Applications: Valuable in the early stages of investigation, especially when the researcher seeks a deeper understanding of a subject before formalizing research questions.
Descriptive Design
Descriptive research design focuses on portraying an accurate profile of a situation, group, or phenomenon.
- Structured Data Collection: Involves systematic and structured data collection methods.
- Objective Presentation: Aims to provide an unbiased and factual account of the subject.
- Quantitative or Qualitative Data: Can incorporate both types of data, depending on the research objectives.
Applications: Widely used in social sciences, marketing, and educational research to provide detailed and objective descriptions.
Explanatory Design
Explanatory research design aims to identify the causes and effects of a phenomenon, explaining the ‘why’ and ‘how’ behind observed relationships.
- Causal Relationships: Seeks to establish causal relationships between variables.
- Controlled Variables : Often involves controlling certain variables to isolate causal factors.
- Quantitative Analysis: Primarily relies on quantitative data analysis techniques.
Applications: Commonly employed in scientific studies and social sciences to delve into the underlying reasons behind observed patterns.
Cross-Sectional Vs. Longitudinal Designs
Cross-sectional design.
Cross-sectional designs collect data from participants at a single point in time.
- Snapshot View: Provides a snapshot of a population at a specific moment.
- Efficiency: More efficient in terms of time and resources.
- Limited Temporal Insights: Offers limited insights into changes over time.
Applications: Suitable for studying characteristics or behaviours that are stable or not expected to change rapidly.
Longitudinal Design
Longitudinal designs involve the collection of data from the same participants over an extended period.
- Temporal Sequence: Allows for the examination of changes over time.
- Causality Assessment: Facilitates the assessment of cause-and-effect relationships.
- Resource-Intensive: Requires more time and resources compared to cross-sectional designs.
Applications: Ideal for studying developmental processes, trends, or the impact of interventions over time.
Experimental Vs Non-experimental Designs
Experimental design.
Experimental designs involve manipulating variables under controlled conditions to observe the effect on another variable.
- Causality Inference: Enables the inference of cause-and-effect relationships.
- Quantitative Data: Primarily involves the collection and analysis of numerical data.
Applications: Commonly used in scientific studies, psychology, and medical research to establish causal relationships.
Non-Experimental Design
Non-experimental designs observe and describe phenomena without manipulating variables.
- Natural Settings: Data is often collected in natural settings without intervention.
- Descriptive or Correlational: Focuses on describing relationships or correlations between variables.
- Quantitative or Qualitative Data: This can involve either type of data, depending on the research approach.
Applications: Suitable for studying complex phenomena in real-world settings where manipulation may not be ethical or feasible.
Effective data collection is fundamental to the success of any research endeavour.
Designing Effective Surveys
Objective Design:
- Clearly define the research objectives to guide the survey design.
- Craft questions that align with the study’s goals and avoid ambiguity.
Structured Format:
- Use a structured format with standardized questions for consistency.
- Include a mix of closed-ended and open-ended questions for detailed insights.
Pilot Testing:
- Conduct pilot tests to identify and rectify potential issues with survey design.
- Ensure clarity, relevance, and appropriateness of questions.
Sampling Strategy:
- Develop a robust sampling strategy to ensure a representative participant group.
- Consider random sampling or stratified sampling based on the research goals.
Conducting Interviews
Establishing Rapport:
- Build rapport with participants to create a comfortable and open environment.
- Clearly communicate the purpose of the interview and the value of participants’ input.
Open-Ended Questions:
- Frame open-ended questions to encourage detailed responses.
- Allow participants to express their thoughts and perspectives freely.
Active Listening:
- Practice active listening to understand areas and gather rich data.
- Avoid interrupting and maintain a non-judgmental stance during the interview.
Ethical Considerations:
- Obtain informed consent and assure participants of confidentiality.
- Be transparent about the study’s purpose and potential implications.
Observation
1. participant observation.
Immersive Participation:
- Actively immerse yourself in the setting or group being observed.
- Develop a deep understanding of behaviours, interactions, and context.
Field Notes:
- Maintain detailed and reflective field notes during observations.
- Document observed patterns, unexpected events, and participant reactions.
Ethical Awareness:
- Be conscious of ethical considerations, ensuring respect for participants.
- Balance the role of observer and participant to minimize bias.
2. Non-participant Observation
Objective Observation:
- Maintain a more detached and objective stance during non-participant observation.
- Focus on recording behaviours, events, and patterns without direct involvement.
Data Reliability:
- Enhance the reliability of data by reducing observer bias.
- Develop clear observation protocols and guidelines.
Contextual Understanding:
- Strive for a thorough understanding of the observed context.
- Consider combining non-participant observation with other methods for triangulation.
Archival Research
1. using existing data.
Identifying Relevant Archives:
- Locate and access archives relevant to the research topic.
- Collaborate with institutions or repositories holding valuable data.
Data Verification:
- Verify the accuracy and reliability of archived data.
- Cross-reference with other sources to ensure data integrity.
Ethical Use:
- Adhere to ethical guidelines when using existing data.
- Respect copyright and intellectual property rights.
2. Challenges and Considerations
Incomplete or Inaccurate Archives:
- Address the possibility of incomplete or inaccurate archival records.
- Acknowledge limitations and uncertainties in the data.
Temporal Bias:
- Recognize potential temporal biases in archived data.
- Consider the historical context and changes that may impact interpretation.
Access Limitations:
- Address potential limitations in accessing certain archives.
- Seek alternative sources or collaborate with institutions to overcome barriers.
Common Challenges in Research Methodology
Conducting research is a complex and dynamic process, often accompanied by a myriad of challenges. Addressing these challenges is crucial to ensure the reliability and validity of research findings.
Sampling Issues
Sampling bias:.
- The presence of sampling bias can lead to an unrepresentative sample, affecting the generalizability of findings.
- Employ random sampling methods and ensure the inclusion of diverse participants to reduce bias.
Sample Size Determination:
- Determining an appropriate sample size is a delicate balance. Too small a sample may lack statistical power, while an excessively large sample may strain resources.
- Conduct a power analysis to determine the optimal sample size based on the research objectives and expected effect size.
Data Quality And Validity
Measurement error:.
- Inaccuracies in measurement tools or data collection methods can introduce measurement errors, impacting the validity of results.
- Pilot test instruments, calibrate equipment, and use standardized measures to enhance the reliability of data.
Construct Validity:
- Ensuring that the chosen measures accurately capture the intended constructs is a persistent challenge.
- Use established measurement instruments and employ multiple measures to assess the same construct for triangulation.
Time And Resource Constraints
Timeline pressures:.
- Limited timeframes can compromise the depth and thoroughness of the research process.
- Develop a realistic timeline, prioritize tasks, and communicate expectations with stakeholders to manage time constraints effectively.
Resource Availability:
- Inadequate resources, whether financial or human, can impede the execution of research activities.
- Seek external funding, collaborate with other researchers, and explore alternative methods that require fewer resources.
Managing Bias in Research
Selection bias:.
- Selecting participants in a way that systematically skews the sample can introduce selection bias.
- Employ randomization techniques, use stratified sampling, and transparently report participant recruitment methods.
Confirmation Bias:
- Researchers may unintentionally favour information that confirms their preconceived beliefs or hypotheses.
- Adopt a systematic and open-minded approach, use blinded study designs, and engage in peer review to mitigate confirmation bias.
Tips On How To Write A Research Methodology
Conducting successful research relies not only on the application of sound methodologies but also on strategic planning and effective collaboration. Here are some tips to enhance the success of your research methodology:
Tip 1. Clear Research Objectives
Well-defined research objectives guide the entire research process. Clearly articulate the purpose of your study, outlining specific research questions or hypotheses.
Tip 2. Comprehensive Literature Review
A thorough literature review provides a foundation for understanding existing knowledge and identifying gaps. Invest time in reviewing relevant literature to inform your research design and methodology.
Tip 3. Detailed Research Plan
A detailed plan serves as a roadmap, ensuring all aspects of the research are systematically addressed. Develop a detailed research plan outlining timelines, milestones, and tasks.
Tip 4. Ethical Considerations
Ethical practices are fundamental to maintaining the integrity of research. Address ethical considerations early, obtain necessary approvals, and ensure participant rights are safeguarded.
Tip 5. Stay Updated On Methodologies
Research methodologies evolve, and staying updated is essential for employing the most effective techniques. Engage in continuous learning by attending workshops, conferences, and reading recent publications.
Tip 6. Adaptability In Methods
Unforeseen challenges may arise during research, necessitating adaptability in methods. Be flexible and willing to modify your approach when needed, ensuring the integrity of the study.
Tip 7. Iterative Approach
Research is often an iterative process, and refining methods based on ongoing findings enhance the study’s robustness. Regularly review and refine your research design and methods as the study progresses.
Frequently Asked Questions
What is the research methodology.
Research methodology is the systematic process of planning, executing, and evaluating scientific investigation. It encompasses the techniques, tools, and procedures used to collect, analyze, and interpret data, ensuring the reliability and validity of research findings.
What are the methodologies in research?
Research methodologies include qualitative and quantitative approaches. Qualitative methods involve in-depth exploration of non-numerical data, while quantitative methods use statistical analysis to examine numerical data. Mixed methods combine both approaches for a comprehensive understanding of research questions.
How to write research methodology?
To write a research methodology, clearly outline the study’s design, data collection, and analysis procedures. Specify research tools, participants, and sampling methods. Justify choices and discuss limitations. Ensure clarity, coherence, and alignment with research objectives for a robust methodology section.
How to write the methodology section of a research paper?
In the methodology section of a research paper, describe the study’s design, data collection, and analysis methods. Detail procedures, tools, participants, and sampling. Justify choices, address ethical considerations, and explain how the methodology aligns with research objectives, ensuring clarity and rigour.
What is mixed research methodology?
Mixed research methodology combines both qualitative and quantitative research approaches within a single study. This approach aims to enhance the details and depth of research findings by providing a more comprehensive understanding of the research problem or question.
You May Also Like
Learn everything about meta synthesis literature review in this comprehensive guide. From definition and process to its types and challenges.
Are you in need of captivating and achievable research topics within the field of biology? Your quest for the best […]
How to write date in Canada – ISO 8601 format – YYYY-MM-DD – For example, January 4, 2024, will be written as 2024-01-04.
Ready to place an order?
USEFUL LINKS
Learning resources.
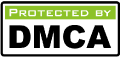
COMPANY DETAILS
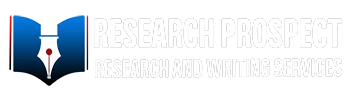
- How It Works
- Privacy Policy

Home » Evaluating Research – Process, Examples and Methods
Evaluating Research – Process, Examples and Methods
Table of Contents

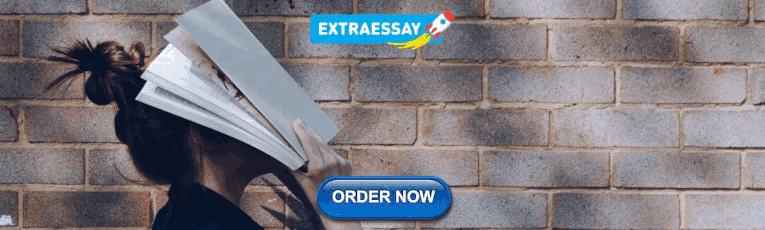
Evaluating Research
Definition:
Evaluating Research refers to the process of assessing the quality, credibility, and relevance of a research study or project. This involves examining the methods, data, and results of the research in order to determine its validity, reliability, and usefulness. Evaluating research can be done by both experts and non-experts in the field, and involves critical thinking, analysis, and interpretation of the research findings.
Research Evaluating Process
The process of evaluating research typically involves the following steps:
Identify the Research Question
The first step in evaluating research is to identify the research question or problem that the study is addressing. This will help you to determine whether the study is relevant to your needs.
Assess the Study Design
The study design refers to the methodology used to conduct the research. You should assess whether the study design is appropriate for the research question and whether it is likely to produce reliable and valid results.
Evaluate the Sample
The sample refers to the group of participants or subjects who are included in the study. You should evaluate whether the sample size is adequate and whether the participants are representative of the population under study.
Review the Data Collection Methods
You should review the data collection methods used in the study to ensure that they are valid and reliable. This includes assessing the measures used to collect data and the procedures used to collect data.
Examine the Statistical Analysis
Statistical analysis refers to the methods used to analyze the data. You should examine whether the statistical analysis is appropriate for the research question and whether it is likely to produce valid and reliable results.
Assess the Conclusions
You should evaluate whether the data support the conclusions drawn from the study and whether they are relevant to the research question.
Consider the Limitations
Finally, you should consider the limitations of the study, including any potential biases or confounding factors that may have influenced the results.
Evaluating Research Methods
Evaluating Research Methods are as follows:
- Peer review: Peer review is a process where experts in the field review a study before it is published. This helps ensure that the study is accurate, valid, and relevant to the field.
- Critical appraisal : Critical appraisal involves systematically evaluating a study based on specific criteria. This helps assess the quality of the study and the reliability of the findings.
- Replication : Replication involves repeating a study to test the validity and reliability of the findings. This can help identify any errors or biases in the original study.
- Meta-analysis : Meta-analysis is a statistical method that combines the results of multiple studies to provide a more comprehensive understanding of a particular topic. This can help identify patterns or inconsistencies across studies.
- Consultation with experts : Consulting with experts in the field can provide valuable insights into the quality and relevance of a study. Experts can also help identify potential limitations or biases in the study.
- Review of funding sources: Examining the funding sources of a study can help identify any potential conflicts of interest or biases that may have influenced the study design or interpretation of results.
Example of Evaluating Research
Example of Evaluating Research sample for students:
Title of the Study: The Effects of Social Media Use on Mental Health among College Students
Sample Size: 500 college students
Sampling Technique : Convenience sampling
- Sample Size: The sample size of 500 college students is a moderate sample size, which could be considered representative of the college student population. However, it would be more representative if the sample size was larger, or if a random sampling technique was used.
- Sampling Technique : Convenience sampling is a non-probability sampling technique, which means that the sample may not be representative of the population. This technique may introduce bias into the study since the participants are self-selected and may not be representative of the entire college student population. Therefore, the results of this study may not be generalizable to other populations.
- Participant Characteristics: The study does not provide any information about the demographic characteristics of the participants, such as age, gender, race, or socioeconomic status. This information is important because social media use and mental health may vary among different demographic groups.
- Data Collection Method: The study used a self-administered survey to collect data. Self-administered surveys may be subject to response bias and may not accurately reflect participants’ actual behaviors and experiences.
- Data Analysis: The study used descriptive statistics and regression analysis to analyze the data. Descriptive statistics provide a summary of the data, while regression analysis is used to examine the relationship between two or more variables. However, the study did not provide information about the statistical significance of the results or the effect sizes.
Overall, while the study provides some insights into the relationship between social media use and mental health among college students, the use of a convenience sampling technique and the lack of information about participant characteristics limit the generalizability of the findings. In addition, the use of self-administered surveys may introduce bias into the study, and the lack of information about the statistical significance of the results limits the interpretation of the findings.
Note*: Above mentioned example is just a sample for students. Do not copy and paste directly into your assignment. Kindly do your own research for academic purposes.
Applications of Evaluating Research
Here are some of the applications of evaluating research:
- Identifying reliable sources : By evaluating research, researchers, students, and other professionals can identify the most reliable sources of information to use in their work. They can determine the quality of research studies, including the methodology, sample size, data analysis, and conclusions.
- Validating findings: Evaluating research can help to validate findings from previous studies. By examining the methodology and results of a study, researchers can determine if the findings are reliable and if they can be used to inform future research.
- Identifying knowledge gaps: Evaluating research can also help to identify gaps in current knowledge. By examining the existing literature on a topic, researchers can determine areas where more research is needed, and they can design studies to address these gaps.
- Improving research quality : Evaluating research can help to improve the quality of future research. By examining the strengths and weaknesses of previous studies, researchers can design better studies and avoid common pitfalls.
- Informing policy and decision-making : Evaluating research is crucial in informing policy and decision-making in many fields. By examining the evidence base for a particular issue, policymakers can make informed decisions that are supported by the best available evidence.
- Enhancing education : Evaluating research is essential in enhancing education. Educators can use research findings to improve teaching methods, curriculum development, and student outcomes.
Purpose of Evaluating Research
Here are some of the key purposes of evaluating research:
- Determine the reliability and validity of research findings : By evaluating research, researchers can determine the quality of the study design, data collection, and analysis. They can determine whether the findings are reliable, valid, and generalizable to other populations.
- Identify the strengths and weaknesses of research studies: Evaluating research helps to identify the strengths and weaknesses of research studies, including potential biases, confounding factors, and limitations. This information can help researchers to design better studies in the future.
- Inform evidence-based decision-making: Evaluating research is crucial in informing evidence-based decision-making in many fields, including healthcare, education, and public policy. Policymakers, educators, and clinicians rely on research evidence to make informed decisions.
- Identify research gaps : By evaluating research, researchers can identify gaps in the existing literature and design studies to address these gaps. This process can help to advance knowledge and improve the quality of research in a particular field.
- Ensure research ethics and integrity : Evaluating research helps to ensure that research studies are conducted ethically and with integrity. Researchers must adhere to ethical guidelines to protect the welfare and rights of study participants and to maintain the trust of the public.
Characteristics Evaluating Research
Characteristics Evaluating Research are as follows:
- Research question/hypothesis: A good research question or hypothesis should be clear, concise, and well-defined. It should address a significant problem or issue in the field and be grounded in relevant theory or prior research.
- Study design: The research design should be appropriate for answering the research question and be clearly described in the study. The study design should also minimize bias and confounding variables.
- Sampling : The sample should be representative of the population of interest and the sampling method should be appropriate for the research question and study design.
- Data collection : The data collection methods should be reliable and valid, and the data should be accurately recorded and analyzed.
- Results : The results should be presented clearly and accurately, and the statistical analysis should be appropriate for the research question and study design.
- Interpretation of results : The interpretation of the results should be based on the data and not influenced by personal biases or preconceptions.
- Generalizability: The study findings should be generalizable to the population of interest and relevant to other settings or contexts.
- Contribution to the field : The study should make a significant contribution to the field and advance our understanding of the research question or issue.
Advantages of Evaluating Research
Evaluating research has several advantages, including:
- Ensuring accuracy and validity : By evaluating research, we can ensure that the research is accurate, valid, and reliable. This ensures that the findings are trustworthy and can be used to inform decision-making.
- Identifying gaps in knowledge : Evaluating research can help identify gaps in knowledge and areas where further research is needed. This can guide future research and help build a stronger evidence base.
- Promoting critical thinking: Evaluating research requires critical thinking skills, which can be applied in other areas of life. By evaluating research, individuals can develop their critical thinking skills and become more discerning consumers of information.
- Improving the quality of research : Evaluating research can help improve the quality of research by identifying areas where improvements can be made. This can lead to more rigorous research methods and better-quality research.
- Informing decision-making: By evaluating research, we can make informed decisions based on the evidence. This is particularly important in fields such as medicine and public health, where decisions can have significant consequences.
- Advancing the field : Evaluating research can help advance the field by identifying new research questions and areas of inquiry. This can lead to the development of new theories and the refinement of existing ones.
Limitations of Evaluating Research
Limitations of Evaluating Research are as follows:
- Time-consuming: Evaluating research can be time-consuming, particularly if the study is complex or requires specialized knowledge. This can be a barrier for individuals who are not experts in the field or who have limited time.
- Subjectivity : Evaluating research can be subjective, as different individuals may have different interpretations of the same study. This can lead to inconsistencies in the evaluation process and make it difficult to compare studies.
- Limited generalizability: The findings of a study may not be generalizable to other populations or contexts. This limits the usefulness of the study and may make it difficult to apply the findings to other settings.
- Publication bias: Research that does not find significant results may be less likely to be published, which can create a bias in the published literature. This can limit the amount of information available for evaluation.
- Lack of transparency: Some studies may not provide enough detail about their methods or results, making it difficult to evaluate their quality or validity.
- Funding bias : Research funded by particular organizations or industries may be biased towards the interests of the funder. This can influence the study design, methods, and interpretation of results.
About the author
Muhammad Hassan
Researcher, Academic Writer, Web developer
You may also like

Dissertation vs Thesis – Key Differences

Delimitations in Research – Types, Examples and...

Survey Instruments – List and Their Uses

Research Paper – Structure, Examples and Writing...

Data Analysis – Process, Methods and Types

Scope of the Research – Writing Guide and...
Reference management. Clean and simple.
What is research methodology?
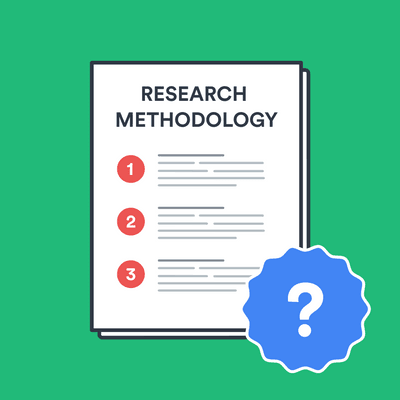
The basics of research methodology
Why do you need a research methodology, what needs to be included, why do you need to document your research method, what are the different types of research instruments, qualitative / quantitative / mixed research methodologies, how do you choose the best research methodology for you, frequently asked questions about research methodology, related articles.
When you’re working on your first piece of academic research, there are many different things to focus on, and it can be overwhelming to stay on top of everything. This is especially true of budding or inexperienced researchers.
If you’ve never put together a research proposal before or find yourself in a position where you need to explain your research methodology decisions, there are a few things you need to be aware of.
Once you understand the ins and outs, handling academic research in the future will be less intimidating. We break down the basics below:
A research methodology encompasses the way in which you intend to carry out your research. This includes how you plan to tackle things like collection methods, statistical analysis, participant observations, and more.
You can think of your research methodology as being a formula. One part will be how you plan on putting your research into practice, and another will be why you feel this is the best way to approach it. Your research methodology is ultimately a methodological and systematic plan to resolve your research problem.
In short, you are explaining how you will take your idea and turn it into a study, which in turn will produce valid and reliable results that are in accordance with the aims and objectives of your research. This is true whether your paper plans to make use of qualitative methods or quantitative methods.
The purpose of a research methodology is to explain the reasoning behind your approach to your research - you'll need to support your collection methods, methods of analysis, and other key points of your work.
Think of it like writing a plan or an outline for you what you intend to do.
When carrying out research, it can be easy to go off-track or depart from your standard methodology.
Tip: Having a methodology keeps you accountable and on track with your original aims and objectives, and gives you a suitable and sound plan to keep your project manageable, smooth, and effective.
With all that said, how do you write out your standard approach to a research methodology?
As a general plan, your methodology should include the following information:
- Your research method. You need to state whether you plan to use quantitative analysis, qualitative analysis, or mixed-method research methods. This will often be determined by what you hope to achieve with your research.
- Explain your reasoning. Why are you taking this methodological approach? Why is this particular methodology the best way to answer your research problem and achieve your objectives?
- Explain your instruments. This will mainly be about your collection methods. There are varying instruments to use such as interviews, physical surveys, questionnaires, for example. Your methodology will need to detail your reasoning in choosing a particular instrument for your research.
- What will you do with your results? How are you going to analyze the data once you have gathered it?
- Advise your reader. If there is anything in your research methodology that your reader might be unfamiliar with, you should explain it in more detail. For example, you should give any background information to your methods that might be relevant or provide your reasoning if you are conducting your research in a non-standard way.
- How will your sampling process go? What will your sampling procedure be and why? For example, if you will collect data by carrying out semi-structured or unstructured interviews, how will you choose your interviewees and how will you conduct the interviews themselves?
- Any practical limitations? You should discuss any limitations you foresee being an issue when you’re carrying out your research.
In any dissertation, thesis, or academic journal, you will always find a chapter dedicated to explaining the research methodology of the person who carried out the study, also referred to as the methodology section of the work.
A good research methodology will explain what you are going to do and why, while a poor methodology will lead to a messy or disorganized approach.
You should also be able to justify in this section your reasoning for why you intend to carry out your research in a particular way, especially if it might be a particularly unique method.
Having a sound methodology in place can also help you with the following:
- When another researcher at a later date wishes to try and replicate your research, they will need your explanations and guidelines.
- In the event that you receive any criticism or questioning on the research you carried out at a later point, you will be able to refer back to it and succinctly explain the how and why of your approach.
- It provides you with a plan to follow throughout your research. When you are drafting your methodology approach, you need to be sure that the method you are using is the right one for your goal. This will help you with both explaining and understanding your method.
- It affords you the opportunity to document from the outset what you intend to achieve with your research, from start to finish.
A research instrument is a tool you will use to help you collect, measure and analyze the data you use as part of your research.
The choice of research instrument will usually be yours to make as the researcher and will be whichever best suits your methodology.
There are many different research instruments you can use in collecting data for your research.
Generally, they can be grouped as follows:
- Interviews (either as a group or one-on-one). You can carry out interviews in many different ways. For example, your interview can be structured, semi-structured, or unstructured. The difference between them is how formal the set of questions is that is asked of the interviewee. In a group interview, you may choose to ask the interviewees to give you their opinions or perceptions on certain topics.
- Surveys (online or in-person). In survey research, you are posing questions in which you ask for a response from the person taking the survey. You may wish to have either free-answer questions such as essay-style questions, or you may wish to use closed questions such as multiple choice. You may even wish to make the survey a mixture of both.
- Focus Groups. Similar to the group interview above, you may wish to ask a focus group to discuss a particular topic or opinion while you make a note of the answers given.
- Observations. This is a good research instrument to use if you are looking into human behaviors. Different ways of researching this include studying the spontaneous behavior of participants in their everyday life, or something more structured. A structured observation is research conducted at a set time and place where researchers observe behavior as planned and agreed upon with participants.
These are the most common ways of carrying out research, but it is really dependent on your needs as a researcher and what approach you think is best to take.
It is also possible to combine a number of research instruments if this is necessary and appropriate in answering your research problem.
There are three different types of methodologies, and they are distinguished by whether they focus on words, numbers, or both.
Data type | What is it? | Methodology |
---|---|---|
Quantitative | This methodology focuses more on measuring and testing numerical data. What is the aim of quantitative research? | Surveys, tests, existing databases. |
Qualitative | Qualitative research is a process of collecting and analyzing both words and textual data. | Observations, interviews, focus groups. |
Mixed-method | A mixed-method approach combines both of the above approaches. | Where you can use a mixed method of research, this can produce some incredibly interesting results. This is due to testing in a way that provides data that is both proven to be exact while also being exploratory at the same time. |
➡️ Want to learn more about the differences between qualitative and quantitative research, and how to use both methods? Check out our guide for that!
If you've done your due diligence, you'll have an idea of which methodology approach is best suited to your research.
It’s likely that you will have carried out considerable reading and homework before you reach this point and you may have taken inspiration from other similar studies that have yielded good results.
Still, it is important to consider different options before setting your research in stone. Exploring different options available will help you to explain why the choice you ultimately make is preferable to other methods.
If proving your research problem requires you to gather large volumes of numerical data to test hypotheses, a quantitative research method is likely to provide you with the most usable results.
If instead you’re looking to try and learn more about people, and their perception of events, your methodology is more exploratory in nature and would therefore probably be better served using a qualitative research methodology.
It helps to always bring things back to the question: what do I want to achieve with my research?
Once you have conducted your research, you need to analyze it. Here are some helpful guides for qualitative data analysis:
➡️ How to do a content analysis
➡️ How to do a thematic analysis
➡️ How to do a rhetorical analysis
Research methodology refers to the techniques used to find and analyze information for a study, ensuring that the results are valid, reliable and that they address the research objective.
Data can typically be organized into four different categories or methods: observational, experimental, simulation, and derived.
Writing a methodology section is a process of introducing your methods and instruments, discussing your analysis, providing more background information, addressing your research limitations, and more.
Your research methodology section will need a clear research question and proposed research approach. You'll need to add a background, introduce your research question, write your methodology and add the works you cited during your data collecting phase.
The research methodology section of your study will indicate how valid your findings are and how well-informed your paper is. It also assists future researchers planning to use the same methodology, who want to cite your study or replicate it.
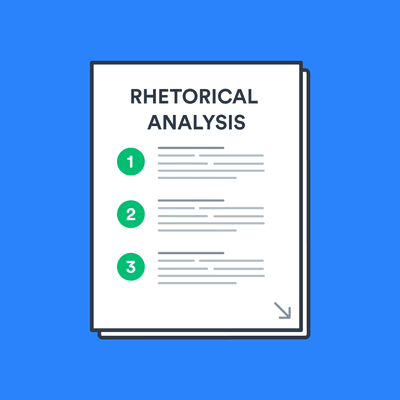

An official website of the United States government
The .gov means it’s official. Federal government websites often end in .gov or .mil. Before sharing sensitive information, make sure you’re on a federal government site.
The site is secure. The https:// ensures that you are connecting to the official website and that any information you provide is encrypted and transmitted securely.
- Publications
- Account settings
Preview improvements coming to the PMC website in October 2024. Learn More or Try it out now .
- Advanced Search
- Journal List
- BMC Med Res Methodol

A tutorial on methodological studies: the what, when, how and why
Lawrence mbuagbaw.
1 Department of Health Research Methods, Evidence and Impact, McMaster University, Hamilton, ON Canada
2 Biostatistics Unit/FSORC, 50 Charlton Avenue East, St Joseph’s Healthcare—Hamilton, 3rd Floor Martha Wing, Room H321, Hamilton, Ontario L8N 4A6 Canada
3 Centre for the Development of Best Practices in Health, Yaoundé, Cameroon
Daeria O. Lawson
Livia puljak.
4 Center for Evidence-Based Medicine and Health Care, Catholic University of Croatia, Ilica 242, 10000 Zagreb, Croatia
David B. Allison
5 Department of Epidemiology and Biostatistics, School of Public Health – Bloomington, Indiana University, Bloomington, IN 47405 USA
Lehana Thabane
6 Departments of Paediatrics and Anaesthesia, McMaster University, Hamilton, ON Canada
7 Centre for Evaluation of Medicine, St. Joseph’s Healthcare-Hamilton, Hamilton, ON Canada
8 Population Health Research Institute, Hamilton Health Sciences, Hamilton, ON Canada
Associated Data
Data sharing is not applicable to this article as no new data were created or analyzed in this study.
Methodological studies – studies that evaluate the design, analysis or reporting of other research-related reports – play an important role in health research. They help to highlight issues in the conduct of research with the aim of improving health research methodology, and ultimately reducing research waste.
We provide an overview of some of the key aspects of methodological studies such as what they are, and when, how and why they are done. We adopt a “frequently asked questions” format to facilitate reading this paper and provide multiple examples to help guide researchers interested in conducting methodological studies. Some of the topics addressed include: is it necessary to publish a study protocol? How to select relevant research reports and databases for a methodological study? What approaches to data extraction and statistical analysis should be considered when conducting a methodological study? What are potential threats to validity and is there a way to appraise the quality of methodological studies?
Appropriate reflection and application of basic principles of epidemiology and biostatistics are required in the design and analysis of methodological studies. This paper provides an introduction for further discussion about the conduct of methodological studies.
The field of meta-research (or research-on-research) has proliferated in recent years in response to issues with research quality and conduct [ 1 – 3 ]. As the name suggests, this field targets issues with research design, conduct, analysis and reporting. Various types of research reports are often examined as the unit of analysis in these studies (e.g. abstracts, full manuscripts, trial registry entries). Like many other novel fields of research, meta-research has seen a proliferation of use before the development of reporting guidance. For example, this was the case with randomized trials for which risk of bias tools and reporting guidelines were only developed much later – after many trials had been published and noted to have limitations [ 4 , 5 ]; and for systematic reviews as well [ 6 – 8 ]. However, in the absence of formal guidance, studies that report on research differ substantially in how they are named, conducted and reported [ 9 , 10 ]. This creates challenges in identifying, summarizing and comparing them. In this tutorial paper, we will use the term methodological study to refer to any study that reports on the design, conduct, analysis or reporting of primary or secondary research-related reports (such as trial registry entries and conference abstracts).
In the past 10 years, there has been an increase in the use of terms related to methodological studies (based on records retrieved with a keyword search [in the title and abstract] for “methodological review” and “meta-epidemiological study” in PubMed up to December 2019), suggesting that these studies may be appearing more frequently in the literature. See Fig. 1 .

Trends in the number studies that mention “methodological review” or “meta-
epidemiological study” in PubMed.
The methods used in many methodological studies have been borrowed from systematic and scoping reviews. This practice has influenced the direction of the field, with many methodological studies including searches of electronic databases, screening of records, duplicate data extraction and assessments of risk of bias in the included studies. However, the research questions posed in methodological studies do not always require the approaches listed above, and guidance is needed on when and how to apply these methods to a methodological study. Even though methodological studies can be conducted on qualitative or mixed methods research, this paper focuses on and draws examples exclusively from quantitative research.
The objectives of this paper are to provide some insights on how to conduct methodological studies so that there is greater consistency between the research questions posed, and the design, analysis and reporting of findings. We provide multiple examples to illustrate concepts and a proposed framework for categorizing methodological studies in quantitative research.
What is a methodological study?
Any study that describes or analyzes methods (design, conduct, analysis or reporting) in published (or unpublished) literature is a methodological study. Consequently, the scope of methodological studies is quite extensive and includes, but is not limited to, topics as diverse as: research question formulation [ 11 ]; adherence to reporting guidelines [ 12 – 14 ] and consistency in reporting [ 15 ]; approaches to study analysis [ 16 ]; investigating the credibility of analyses [ 17 ]; and studies that synthesize these methodological studies [ 18 ]. While the nomenclature of methodological studies is not uniform, the intents and purposes of these studies remain fairly consistent – to describe or analyze methods in primary or secondary studies. As such, methodological studies may also be classified as a subtype of observational studies.
Parallel to this are experimental studies that compare different methods. Even though they play an important role in informing optimal research methods, experimental methodological studies are beyond the scope of this paper. Examples of such studies include the randomized trials by Buscemi et al., comparing single data extraction to double data extraction [ 19 ], and Carrasco-Labra et al., comparing approaches to presenting findings in Grading of Recommendations, Assessment, Development and Evaluations (GRADE) summary of findings tables [ 20 ]. In these studies, the unit of analysis is the person or groups of individuals applying the methods. We also direct readers to the Studies Within a Trial (SWAT) and Studies Within a Review (SWAR) programme operated through the Hub for Trials Methodology Research, for further reading as a potential useful resource for these types of experimental studies [ 21 ]. Lastly, this paper is not meant to inform the conduct of research using computational simulation and mathematical modeling for which some guidance already exists [ 22 ], or studies on the development of methods using consensus-based approaches.
When should we conduct a methodological study?
Methodological studies occupy a unique niche in health research that allows them to inform methodological advances. Methodological studies should also be conducted as pre-cursors to reporting guideline development, as they provide an opportunity to understand current practices, and help to identify the need for guidance and gaps in methodological or reporting quality. For example, the development of the popular Preferred Reporting Items of Systematic reviews and Meta-Analyses (PRISMA) guidelines were preceded by methodological studies identifying poor reporting practices [ 23 , 24 ]. In these instances, after the reporting guidelines are published, methodological studies can also be used to monitor uptake of the guidelines.
These studies can also be conducted to inform the state of the art for design, analysis and reporting practices across different types of health research fields, with the aim of improving research practices, and preventing or reducing research waste. For example, Samaan et al. conducted a scoping review of adherence to different reporting guidelines in health care literature [ 18 ]. Methodological studies can also be used to determine the factors associated with reporting practices. For example, Abbade et al. investigated journal characteristics associated with the use of the Participants, Intervention, Comparison, Outcome, Timeframe (PICOT) format in framing research questions in trials of venous ulcer disease [ 11 ].
How often are methodological studies conducted?
There is no clear answer to this question. Based on a search of PubMed, the use of related terms (“methodological review” and “meta-epidemiological study”) – and therefore, the number of methodological studies – is on the rise. However, many other terms are used to describe methodological studies. There are also many studies that explore design, conduct, analysis or reporting of research reports, but that do not use any specific terms to describe or label their study design in terms of “methodology”. This diversity in nomenclature makes a census of methodological studies elusive. Appropriate terminology and key words for methodological studies are needed to facilitate improved accessibility for end-users.
Why do we conduct methodological studies?
Methodological studies provide information on the design, conduct, analysis or reporting of primary and secondary research and can be used to appraise quality, quantity, completeness, accuracy and consistency of health research. These issues can be explored in specific fields, journals, databases, geographical regions and time periods. For example, Areia et al. explored the quality of reporting of endoscopic diagnostic studies in gastroenterology [ 25 ]; Knol et al. investigated the reporting of p -values in baseline tables in randomized trial published in high impact journals [ 26 ]; Chen et al. describe adherence to the Consolidated Standards of Reporting Trials (CONSORT) statement in Chinese Journals [ 27 ]; and Hopewell et al. describe the effect of editors’ implementation of CONSORT guidelines on reporting of abstracts over time [ 28 ]. Methodological studies provide useful information to researchers, clinicians, editors, publishers and users of health literature. As a result, these studies have been at the cornerstone of important methodological developments in the past two decades and have informed the development of many health research guidelines including the highly cited CONSORT statement [ 5 ].
Where can we find methodological studies?
Methodological studies can be found in most common biomedical bibliographic databases (e.g. Embase, MEDLINE, PubMed, Web of Science). However, the biggest caveat is that methodological studies are hard to identify in the literature due to the wide variety of names used and the lack of comprehensive databases dedicated to them. A handful can be found in the Cochrane Library as “Cochrane Methodology Reviews”, but these studies only cover methodological issues related to systematic reviews. Previous attempts to catalogue all empirical studies of methods used in reviews were abandoned 10 years ago [ 29 ]. In other databases, a variety of search terms may be applied with different levels of sensitivity and specificity.
Some frequently asked questions about methodological studies
In this section, we have outlined responses to questions that might help inform the conduct of methodological studies.
Q: How should I select research reports for my methodological study?
A: Selection of research reports for a methodological study depends on the research question and eligibility criteria. Once a clear research question is set and the nature of literature one desires to review is known, one can then begin the selection process. Selection may begin with a broad search, especially if the eligibility criteria are not apparent. For example, a methodological study of Cochrane Reviews of HIV would not require a complex search as all eligible studies can easily be retrieved from the Cochrane Library after checking a few boxes [ 30 ]. On the other hand, a methodological study of subgroup analyses in trials of gastrointestinal oncology would require a search to find such trials, and further screening to identify trials that conducted a subgroup analysis [ 31 ].
The strategies used for identifying participants in observational studies can apply here. One may use a systematic search to identify all eligible studies. If the number of eligible studies is unmanageable, a random sample of articles can be expected to provide comparable results if it is sufficiently large [ 32 ]. For example, Wilson et al. used a random sample of trials from the Cochrane Stroke Group’s Trial Register to investigate completeness of reporting [ 33 ]. It is possible that a simple random sample would lead to underrepresentation of units (i.e. research reports) that are smaller in number. This is relevant if the investigators wish to compare multiple groups but have too few units in one group. In this case a stratified sample would help to create equal groups. For example, in a methodological study comparing Cochrane and non-Cochrane reviews, Kahale et al. drew random samples from both groups [ 34 ]. Alternatively, systematic or purposeful sampling strategies can be used and we encourage researchers to justify their selected approaches based on the study objective.
Q: How many databases should I search?
A: The number of databases one should search would depend on the approach to sampling, which can include targeting the entire “population” of interest or a sample of that population. If you are interested in including the entire target population for your research question, or drawing a random or systematic sample from it, then a comprehensive and exhaustive search for relevant articles is required. In this case, we recommend using systematic approaches for searching electronic databases (i.e. at least 2 databases with a replicable and time stamped search strategy). The results of your search will constitute a sampling frame from which eligible studies can be drawn.
Alternatively, if your approach to sampling is purposeful, then we recommend targeting the database(s) or data sources (e.g. journals, registries) that include the information you need. For example, if you are conducting a methodological study of high impact journals in plastic surgery and they are all indexed in PubMed, you likely do not need to search any other databases. You may also have a comprehensive list of all journals of interest and can approach your search using the journal names in your database search (or by accessing the journal archives directly from the journal’s website). Even though one could also search journals’ web pages directly, using a database such as PubMed has multiple advantages, such as the use of filters, so the search can be narrowed down to a certain period, or study types of interest. Furthermore, individual journals’ web sites may have different search functionalities, which do not necessarily yield a consistent output.
Q: Should I publish a protocol for my methodological study?
A: A protocol is a description of intended research methods. Currently, only protocols for clinical trials require registration [ 35 ]. Protocols for systematic reviews are encouraged but no formal recommendation exists. The scientific community welcomes the publication of protocols because they help protect against selective outcome reporting, the use of post hoc methodologies to embellish results, and to help avoid duplication of efforts [ 36 ]. While the latter two risks exist in methodological research, the negative consequences may be substantially less than for clinical outcomes. In a sample of 31 methodological studies, 7 (22.6%) referenced a published protocol [ 9 ]. In the Cochrane Library, there are 15 protocols for methodological reviews (21 July 2020). This suggests that publishing protocols for methodological studies is not uncommon.
Authors can consider publishing their study protocol in a scholarly journal as a manuscript. Advantages of such publication include obtaining peer-review feedback about the planned study, and easy retrieval by searching databases such as PubMed. The disadvantages in trying to publish protocols includes delays associated with manuscript handling and peer review, as well as costs, as few journals publish study protocols, and those journals mostly charge article-processing fees [ 37 ]. Authors who would like to make their protocol publicly available without publishing it in scholarly journals, could deposit their study protocols in publicly available repositories, such as the Open Science Framework ( https://osf.io/ ).
Q: How to appraise the quality of a methodological study?
A: To date, there is no published tool for appraising the risk of bias in a methodological study, but in principle, a methodological study could be considered as a type of observational study. Therefore, during conduct or appraisal, care should be taken to avoid the biases common in observational studies [ 38 ]. These biases include selection bias, comparability of groups, and ascertainment of exposure or outcome. In other words, to generate a representative sample, a comprehensive reproducible search may be necessary to build a sampling frame. Additionally, random sampling may be necessary to ensure that all the included research reports have the same probability of being selected, and the screening and selection processes should be transparent and reproducible. To ensure that the groups compared are similar in all characteristics, matching, random sampling or stratified sampling can be used. Statistical adjustments for between-group differences can also be applied at the analysis stage. Finally, duplicate data extraction can reduce errors in assessment of exposures or outcomes.
Q: Should I justify a sample size?
A: In all instances where one is not using the target population (i.e. the group to which inferences from the research report are directed) [ 39 ], a sample size justification is good practice. The sample size justification may take the form of a description of what is expected to be achieved with the number of articles selected, or a formal sample size estimation that outlines the number of articles required to answer the research question with a certain precision and power. Sample size justifications in methodological studies are reasonable in the following instances:
- Comparing two groups
- Determining a proportion, mean or another quantifier
- Determining factors associated with an outcome using regression-based analyses
For example, El Dib et al. computed a sample size requirement for a methodological study of diagnostic strategies in randomized trials, based on a confidence interval approach [ 40 ].
Q: What should I call my study?
A: Other terms which have been used to describe/label methodological studies include “ methodological review ”, “methodological survey” , “meta-epidemiological study” , “systematic review” , “systematic survey”, “meta-research”, “research-on-research” and many others. We recommend that the study nomenclature be clear, unambiguous, informative and allow for appropriate indexing. Methodological study nomenclature that should be avoided includes “ systematic review” – as this will likely be confused with a systematic review of a clinical question. “ Systematic survey” may also lead to confusion about whether the survey was systematic (i.e. using a preplanned methodology) or a survey using “ systematic” sampling (i.e. a sampling approach using specific intervals to determine who is selected) [ 32 ]. Any of the above meanings of the words “ systematic” may be true for methodological studies and could be potentially misleading. “ Meta-epidemiological study” is ideal for indexing, but not very informative as it describes an entire field. The term “ review ” may point towards an appraisal or “review” of the design, conduct, analysis or reporting (or methodological components) of the targeted research reports, yet it has also been used to describe narrative reviews [ 41 , 42 ]. The term “ survey ” is also in line with the approaches used in many methodological studies [ 9 ], and would be indicative of the sampling procedures of this study design. However, in the absence of guidelines on nomenclature, the term “ methodological study ” is broad enough to capture most of the scenarios of such studies.
Q: Should I account for clustering in my methodological study?
A: Data from methodological studies are often clustered. For example, articles coming from a specific source may have different reporting standards (e.g. the Cochrane Library). Articles within the same journal may be similar due to editorial practices and policies, reporting requirements and endorsement of guidelines. There is emerging evidence that these are real concerns that should be accounted for in analyses [ 43 ]. Some cluster variables are described in the section: “ What variables are relevant to methodological studies?”
A variety of modelling approaches can be used to account for correlated data, including the use of marginal, fixed or mixed effects regression models with appropriate computation of standard errors [ 44 ]. For example, Kosa et al. used generalized estimation equations to account for correlation of articles within journals [ 15 ]. Not accounting for clustering could lead to incorrect p -values, unduly narrow confidence intervals, and biased estimates [ 45 ].
Q: Should I extract data in duplicate?
A: Yes. Duplicate data extraction takes more time but results in less errors [ 19 ]. Data extraction errors in turn affect the effect estimate [ 46 ], and therefore should be mitigated. Duplicate data extraction should be considered in the absence of other approaches to minimize extraction errors. However, much like systematic reviews, this area will likely see rapid new advances with machine learning and natural language processing technologies to support researchers with screening and data extraction [ 47 , 48 ]. However, experience plays an important role in the quality of extracted data and inexperienced extractors should be paired with experienced extractors [ 46 , 49 ].
Q: Should I assess the risk of bias of research reports included in my methodological study?
A : Risk of bias is most useful in determining the certainty that can be placed in the effect measure from a study. In methodological studies, risk of bias may not serve the purpose of determining the trustworthiness of results, as effect measures are often not the primary goal of methodological studies. Determining risk of bias in methodological studies is likely a practice borrowed from systematic review methodology, but whose intrinsic value is not obvious in methodological studies. When it is part of the research question, investigators often focus on one aspect of risk of bias. For example, Speich investigated how blinding was reported in surgical trials [ 50 ], and Abraha et al., investigated the application of intention-to-treat analyses in systematic reviews and trials [ 51 ].
Q: What variables are relevant to methodological studies?
A: There is empirical evidence that certain variables may inform the findings in a methodological study. We outline some of these and provide a brief overview below:
- Country: Countries and regions differ in their research cultures, and the resources available to conduct research. Therefore, it is reasonable to believe that there may be differences in methodological features across countries. Methodological studies have reported loco-regional differences in reporting quality [ 52 , 53 ]. This may also be related to challenges non-English speakers face in publishing papers in English.
- Authors’ expertise: The inclusion of authors with expertise in research methodology, biostatistics, and scientific writing is likely to influence the end-product. Oltean et al. found that among randomized trials in orthopaedic surgery, the use of analyses that accounted for clustering was more likely when specialists (e.g. statistician, epidemiologist or clinical trials methodologist) were included on the study team [ 54 ]. Fleming et al. found that including methodologists in the review team was associated with appropriate use of reporting guidelines [ 55 ].
- Source of funding and conflicts of interest: Some studies have found that funded studies report better [ 56 , 57 ], while others do not [ 53 , 58 ]. The presence of funding would indicate the availability of resources deployed to ensure optimal design, conduct, analysis and reporting. However, the source of funding may introduce conflicts of interest and warrant assessment. For example, Kaiser et al. investigated the effect of industry funding on obesity or nutrition randomized trials and found that reporting quality was similar [ 59 ]. Thomas et al. looked at reporting quality of long-term weight loss trials and found that industry funded studies were better [ 60 ]. Kan et al. examined the association between industry funding and “positive trials” (trials reporting a significant intervention effect) and found that industry funding was highly predictive of a positive trial [ 61 ]. This finding is similar to that of a recent Cochrane Methodology Review by Hansen et al. [ 62 ]
- Journal characteristics: Certain journals’ characteristics may influence the study design, analysis or reporting. Characteristics such as journal endorsement of guidelines [ 63 , 64 ], and Journal Impact Factor (JIF) have been shown to be associated with reporting [ 63 , 65 – 67 ].
- Study size (sample size/number of sites): Some studies have shown that reporting is better in larger studies [ 53 , 56 , 58 ].
- Year of publication: It is reasonable to assume that design, conduct, analysis and reporting of research will change over time. Many studies have demonstrated improvements in reporting over time or after the publication of reporting guidelines [ 68 , 69 ].
- Type of intervention: In a methodological study of reporting quality of weight loss intervention studies, Thabane et al. found that trials of pharmacologic interventions were reported better than trials of non-pharmacologic interventions [ 70 ].
- Interactions between variables: Complex interactions between the previously listed variables are possible. High income countries with more resources may be more likely to conduct larger studies and incorporate a variety of experts. Authors in certain countries may prefer certain journals, and journal endorsement of guidelines and editorial policies may change over time.
Q: Should I focus only on high impact journals?
A: Investigators may choose to investigate only high impact journals because they are more likely to influence practice and policy, or because they assume that methodological standards would be higher. However, the JIF may severely limit the scope of articles included and may skew the sample towards articles with positive findings. The generalizability and applicability of findings from a handful of journals must be examined carefully, especially since the JIF varies over time. Even among journals that are all “high impact”, variations exist in methodological standards.
Q: Can I conduct a methodological study of qualitative research?
A: Yes. Even though a lot of methodological research has been conducted in the quantitative research field, methodological studies of qualitative studies are feasible. Certain databases that catalogue qualitative research including the Cumulative Index to Nursing & Allied Health Literature (CINAHL) have defined subject headings that are specific to methodological research (e.g. “research methodology”). Alternatively, one could also conduct a qualitative methodological review; that is, use qualitative approaches to synthesize methodological issues in qualitative studies.
Q: What reporting guidelines should I use for my methodological study?
A: There is no guideline that covers the entire scope of methodological studies. One adaptation of the PRISMA guidelines has been published, which works well for studies that aim to use the entire target population of research reports [ 71 ]. However, it is not widely used (40 citations in 2 years as of 09 December 2019), and methodological studies that are designed as cross-sectional or before-after studies require a more fit-for purpose guideline. A more encompassing reporting guideline for a broad range of methodological studies is currently under development [ 72 ]. However, in the absence of formal guidance, the requirements for scientific reporting should be respected, and authors of methodological studies should focus on transparency and reproducibility.
Q: What are the potential threats to validity and how can I avoid them?
A: Methodological studies may be compromised by a lack of internal or external validity. The main threats to internal validity in methodological studies are selection and confounding bias. Investigators must ensure that the methods used to select articles does not make them differ systematically from the set of articles to which they would like to make inferences. For example, attempting to make extrapolations to all journals after analyzing high-impact journals would be misleading.
Many factors (confounders) may distort the association between the exposure and outcome if the included research reports differ with respect to these factors [ 73 ]. For example, when examining the association between source of funding and completeness of reporting, it may be necessary to account for journals that endorse the guidelines. Confounding bias can be addressed by restriction, matching and statistical adjustment [ 73 ]. Restriction appears to be the method of choice for many investigators who choose to include only high impact journals or articles in a specific field. For example, Knol et al. examined the reporting of p -values in baseline tables of high impact journals [ 26 ]. Matching is also sometimes used. In the methodological study of non-randomized interventional studies of elective ventral hernia repair, Parker et al. matched prospective studies with retrospective studies and compared reporting standards [ 74 ]. Some other methodological studies use statistical adjustments. For example, Zhang et al. used regression techniques to determine the factors associated with missing participant data in trials [ 16 ].
With regard to external validity, researchers interested in conducting methodological studies must consider how generalizable or applicable their findings are. This should tie in closely with the research question and should be explicit. For example. Findings from methodological studies on trials published in high impact cardiology journals cannot be assumed to be applicable to trials in other fields. However, investigators must ensure that their sample truly represents the target sample either by a) conducting a comprehensive and exhaustive search, or b) using an appropriate and justified, randomly selected sample of research reports.
Even applicability to high impact journals may vary based on the investigators’ definition, and over time. For example, for high impact journals in the field of general medicine, Bouwmeester et al. included the Annals of Internal Medicine (AIM), BMJ, the Journal of the American Medical Association (JAMA), Lancet, the New England Journal of Medicine (NEJM), and PLoS Medicine ( n = 6) [ 75 ]. In contrast, the high impact journals selected in the methodological study by Schiller et al. were BMJ, JAMA, Lancet, and NEJM ( n = 4) [ 76 ]. Another methodological study by Kosa et al. included AIM, BMJ, JAMA, Lancet and NEJM ( n = 5). In the methodological study by Thabut et al., journals with a JIF greater than 5 were considered to be high impact. Riado Minguez et al. used first quartile journals in the Journal Citation Reports (JCR) for a specific year to determine “high impact” [ 77 ]. Ultimately, the definition of high impact will be based on the number of journals the investigators are willing to include, the year of impact and the JIF cut-off [ 78 ]. We acknowledge that the term “generalizability” may apply differently for methodological studies, especially when in many instances it is possible to include the entire target population in the sample studied.
Finally, methodological studies are not exempt from information bias which may stem from discrepancies in the included research reports [ 79 ], errors in data extraction, or inappropriate interpretation of the information extracted. Likewise, publication bias may also be a concern in methodological studies, but such concepts have not yet been explored.
A proposed framework
In order to inform discussions about methodological studies, the development of guidance for what should be reported, we have outlined some key features of methodological studies that can be used to classify them. For each of the categories outlined below, we provide an example. In our experience, the choice of approach to completing a methodological study can be informed by asking the following four questions:
- What is the aim?
A methodological study may be focused on exploring sources of bias in primary or secondary studies (meta-bias), or how bias is analyzed. We have taken care to distinguish bias (i.e. systematic deviations from the truth irrespective of the source) from reporting quality or completeness (i.e. not adhering to a specific reporting guideline or norm). An example of where this distinction would be important is in the case of a randomized trial with no blinding. This study (depending on the nature of the intervention) would be at risk of performance bias. However, if the authors report that their study was not blinded, they would have reported adequately. In fact, some methodological studies attempt to capture both “quality of conduct” and “quality of reporting”, such as Richie et al., who reported on the risk of bias in randomized trials of pharmacy practice interventions [ 80 ]. Babic et al. investigated how risk of bias was used to inform sensitivity analyses in Cochrane reviews [ 81 ]. Further, biases related to choice of outcomes can also be explored. For example, Tan et al investigated differences in treatment effect size based on the outcome reported [ 82 ].
Methodological studies may report quality of reporting against a reporting checklist (i.e. adherence to guidelines) or against expected norms. For example, Croituro et al. report on the quality of reporting in systematic reviews published in dermatology journals based on their adherence to the PRISMA statement [ 83 ], and Khan et al. described the quality of reporting of harms in randomized controlled trials published in high impact cardiovascular journals based on the CONSORT extension for harms [ 84 ]. Other methodological studies investigate reporting of certain features of interest that may not be part of formally published checklists or guidelines. For example, Mbuagbaw et al. described how often the implications for research are elaborated using the Evidence, Participants, Intervention, Comparison, Outcome, Timeframe (EPICOT) format [ 30 ].
Sometimes investigators may be interested in how consistent reports of the same research are, as it is expected that there should be consistency between: conference abstracts and published manuscripts; manuscript abstracts and manuscript main text; and trial registration and published manuscript. For example, Rosmarakis et al. investigated consistency between conference abstracts and full text manuscripts [ 85 ].
In addition to identifying issues with reporting in primary and secondary studies, authors of methodological studies may be interested in determining the factors that are associated with certain reporting practices. Many methodological studies incorporate this, albeit as a secondary outcome. For example, Farrokhyar et al. investigated the factors associated with reporting quality in randomized trials of coronary artery bypass grafting surgery [ 53 ].
Methodological studies may also be used to describe methods or compare methods, and the factors associated with methods. Muller et al. described the methods used for systematic reviews and meta-analyses of observational studies [ 86 ].
Some methodological studies synthesize results from other methodological studies. For example, Li et al. conducted a scoping review of methodological reviews that investigated consistency between full text and abstracts in primary biomedical research [ 87 ].
Some methodological studies may investigate the use of names and terms in health research. For example, Martinic et al. investigated the definitions of systematic reviews used in overviews of systematic reviews (OSRs), meta-epidemiological studies and epidemiology textbooks [ 88 ].
In addition to the previously mentioned experimental methodological studies, there may exist other types of methodological studies not captured here.
- 2. What is the design?
Most methodological studies are purely descriptive and report their findings as counts (percent) and means (standard deviation) or medians (interquartile range). For example, Mbuagbaw et al. described the reporting of research recommendations in Cochrane HIV systematic reviews [ 30 ]. Gohari et al. described the quality of reporting of randomized trials in diabetes in Iran [ 12 ].
Some methodological studies are analytical wherein “analytical studies identify and quantify associations, test hypotheses, identify causes and determine whether an association exists between variables, such as between an exposure and a disease.” [ 89 ] In the case of methodological studies all these investigations are possible. For example, Kosa et al. investigated the association between agreement in primary outcome from trial registry to published manuscript and study covariates. They found that larger and more recent studies were more likely to have agreement [ 15 ]. Tricco et al. compared the conclusion statements from Cochrane and non-Cochrane systematic reviews with a meta-analysis of the primary outcome and found that non-Cochrane reviews were more likely to report positive findings. These results are a test of the null hypothesis that the proportions of Cochrane and non-Cochrane reviews that report positive results are equal [ 90 ].
- 3. What is the sampling strategy?
Methodological reviews with narrow research questions may be able to include the entire target population. For example, in the methodological study of Cochrane HIV systematic reviews, Mbuagbaw et al. included all of the available studies ( n = 103) [ 30 ].
Many methodological studies use random samples of the target population [ 33 , 91 , 92 ]. Alternatively, purposeful sampling may be used, limiting the sample to a subset of research-related reports published within a certain time period, or in journals with a certain ranking or on a topic. Systematic sampling can also be used when random sampling may be challenging to implement.
- 4. What is the unit of analysis?
Many methodological studies use a research report (e.g. full manuscript of study, abstract portion of the study) as the unit of analysis, and inferences can be made at the study-level. However, both published and unpublished research-related reports can be studied. These may include articles, conference abstracts, registry entries etc.
Some methodological studies report on items which may occur more than once per article. For example, Paquette et al. report on subgroup analyses in Cochrane reviews of atrial fibrillation in which 17 systematic reviews planned 56 subgroup analyses [ 93 ].
This framework is outlined in Fig. 2 .

A proposed framework for methodological studies
Conclusions
Methodological studies have examined different aspects of reporting such as quality, completeness, consistency and adherence to reporting guidelines. As such, many of the methodological study examples cited in this tutorial are related to reporting. However, as an evolving field, the scope of research questions that can be addressed by methodological studies is expected to increase.
In this paper we have outlined the scope and purpose of methodological studies, along with examples of instances in which various approaches have been used. In the absence of formal guidance on the design, conduct, analysis and reporting of methodological studies, we have provided some advice to help make methodological studies consistent. This advice is grounded in good contemporary scientific practice. Generally, the research question should tie in with the sampling approach and planned analysis. We have also highlighted the variables that may inform findings from methodological studies. Lastly, we have provided suggestions for ways in which authors can categorize their methodological studies to inform their design and analysis.
Acknowledgements
Abbreviations.
CONSORT | Consolidated Standards of Reporting Trials |
EPICOT | Evidence, Participants, Intervention, Comparison, Outcome, Timeframe |
GRADE | Grading of Recommendations, Assessment, Development and Evaluations |
PICOT | Participants, Intervention, Comparison, Outcome, Timeframe |
PRISMA | Preferred Reporting Items of Systematic reviews and Meta-Analyses |
SWAR | Studies Within a Review |
SWAT | Studies Within a Trial |
Authors’ contributions
LM conceived the idea and drafted the outline and paper. DOL and LT commented on the idea and draft outline. LM, LP and DOL performed literature searches and data extraction. All authors (LM, DOL, LT, LP, DBA) reviewed several draft versions of the manuscript and approved the final manuscript.
This work did not receive any dedicated funding.
Availability of data and materials
Ethics approval and consent to participate.
Not applicable.
Consent for publication
Competing interests.
DOL, DBA, LM, LP and LT are involved in the development of a reporting guideline for methodological studies.
Publisher’s Note
Springer Nature remains neutral with regard to jurisdictional claims in published maps and institutional affiliations.
- Research Process
- Manuscript Preparation
- Manuscript Review
- Publication Process
- Publication Recognition
- Language Editing Services
- Translation Services
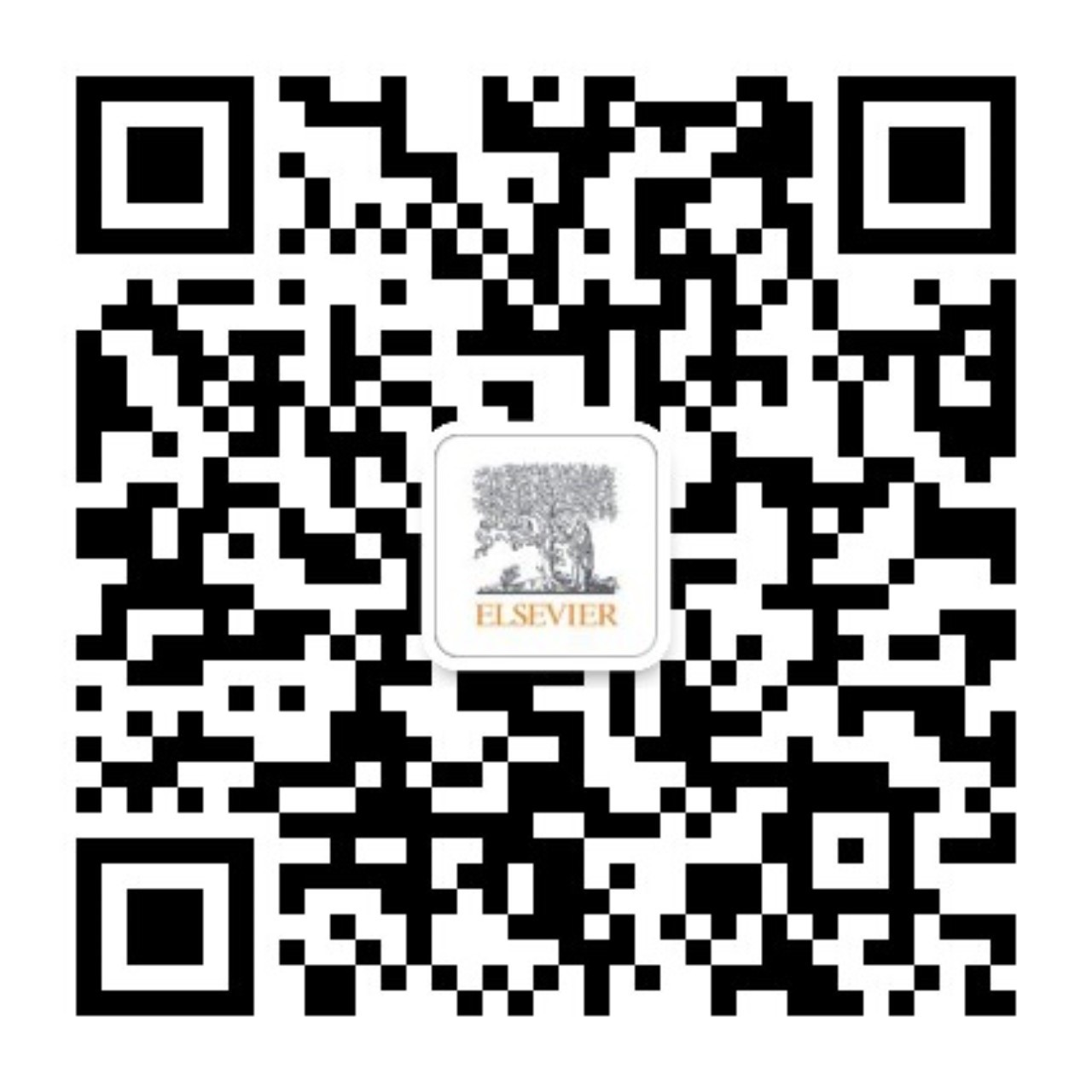
Choosing the Right Research Methodology: A Guide for Researchers
- 3 minute read
- 49.5K views
Table of Contents
Choosing an optimal research methodology is crucial for the success of any research project. The methodology you select will determine the type of data you collect, how you collect it, and how you analyse it. Understanding the different types of research methods available along with their strengths and weaknesses, is thus imperative to make an informed decision.
Understanding different research methods:
There are several research methods available depending on the type of study you are conducting, i.e., whether it is laboratory-based, clinical, epidemiological, or survey based . Some common methodologies include qualitative research, quantitative research, experimental research, survey-based research, and action research. Each method can be opted for and modified, depending on the type of research hypotheses and objectives.
Qualitative vs quantitative research:
When deciding on a research methodology, one of the key factors to consider is whether your research will be qualitative or quantitative. Qualitative research is used to understand people’s experiences, concepts, thoughts, or behaviours . Quantitative research, on the contrary, deals with numbers, graphs, and charts, and is used to test or confirm hypotheses, assumptions, and theories.
Qualitative research methodology:
Qualitative research is often used to examine issues that are not well understood, and to gather additional insights on these topics. Qualitative research methods include open-ended survey questions, observations of behaviours described through words, and reviews of literature that has explored similar theories and ideas. These methods are used to understand how language is used in real-world situations, identify common themes or overarching ideas, and describe and interpret various texts. Data analysis for qualitative research typically includes discourse analysis, thematic analysis, and textual analysis.
Quantitative research methodology:
The goal of quantitative research is to test hypotheses, confirm assumptions and theories, and determine cause-and-effect relationships. Quantitative research methods include experiments, close-ended survey questions, and countable and numbered observations. Data analysis for quantitative research relies heavily on statistical methods.
Analysing qualitative vs quantitative data:
The methods used for data analysis also differ for qualitative and quantitative research. As mentioned earlier, quantitative data is generally analysed using statistical methods and does not leave much room for speculation. It is more structured and follows a predetermined plan. In quantitative research, the researcher starts with a hypothesis and uses statistical methods to test it. Contrarily, methods used for qualitative data analysis can identify patterns and themes within the data, rather than provide statistical measures of the data. It is an iterative process, where the researcher goes back and forth trying to gauge the larger implications of the data through different perspectives and revising the analysis if required.
When to use qualitative vs quantitative research:
The choice between qualitative and quantitative research will depend on the gap that the research project aims to address, and specific objectives of the study. If the goal is to establish facts about a subject or topic, quantitative research is an appropriate choice. However, if the goal is to understand people’s experiences or perspectives, qualitative research may be more suitable.
Conclusion:
In conclusion, an understanding of the different research methods available, their applicability, advantages, and disadvantages is essential for making an informed decision on the best methodology for your project. If you need any additional guidance on which research methodology to opt for, you can head over to Elsevier Author Services (EAS). EAS experts will guide you throughout the process and help you choose the perfect methodology for your research goals.
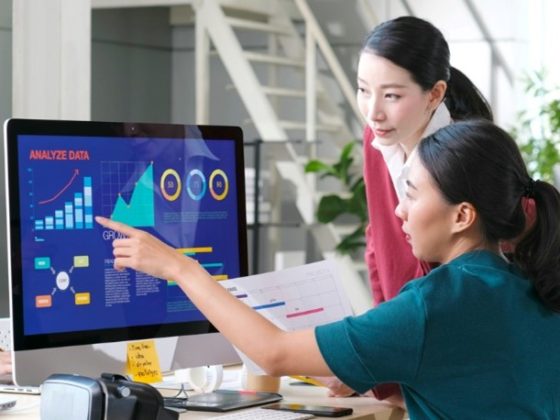
Why is data validation important in research?
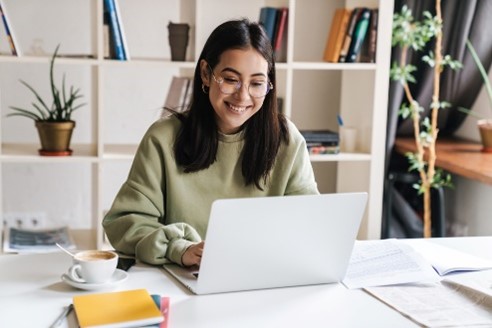
When Data Speak, Listen: Importance of Data Collection and Analysis Methods
You may also like.
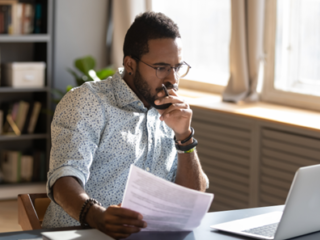
Descriptive Research Design and Its Myriad Uses
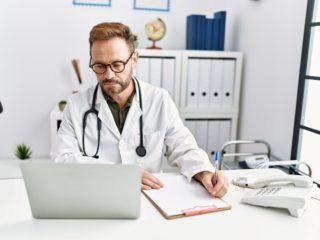
Five Common Mistakes to Avoid When Writing a Biomedical Research Paper
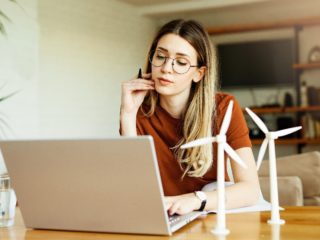
Making Technical Writing in Environmental Engineering Accessible
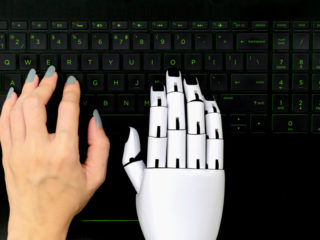
To Err is Not Human: The Dangers of AI-assisted Academic Writing
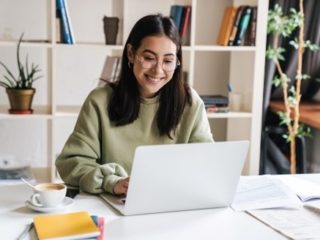
Writing a good review article
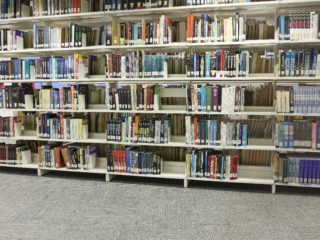
Scholarly Sources: What are They and Where can You Find Them?
Input your search keywords and press Enter.
Service update: Some parts of the Library’s website will be down for maintenance on August 11.
Secondary menu
- Log in to your Library account
- Hours and Maps
- Connect from Off Campus
- UC Berkeley Home
Search form
Research methods--quantitative, qualitative, and more: overview.
- Quantitative Research
- Qualitative Research
- Data Science Methods (Machine Learning, AI, Big Data)
- Text Mining and Computational Text Analysis
- Evidence Synthesis/Systematic Reviews
- Get Data, Get Help!
About Research Methods
This guide provides an overview of research methods, how to choose and use them, and supports and resources at UC Berkeley.
As Patten and Newhart note in the book Understanding Research Methods , "Research methods are the building blocks of the scientific enterprise. They are the "how" for building systematic knowledge. The accumulation of knowledge through research is by its nature a collective endeavor. Each well-designed study provides evidence that may support, amend, refute, or deepen the understanding of existing knowledge...Decisions are important throughout the practice of research and are designed to help researchers collect evidence that includes the full spectrum of the phenomenon under study, to maintain logical rules, and to mitigate or account for possible sources of bias. In many ways, learning research methods is learning how to see and make these decisions."
The choice of methods varies by discipline, by the kind of phenomenon being studied and the data being used to study it, by the technology available, and more. This guide is an introduction, but if you don't see what you need here, always contact your subject librarian, and/or take a look to see if there's a library research guide that will answer your question.
Suggestions for changes and additions to this guide are welcome!
START HERE: SAGE Research Methods
Without question, the most comprehensive resource available from the library is SAGE Research Methods. HERE IS THE ONLINE GUIDE to this one-stop shopping collection, and some helpful links are below:
- SAGE Research Methods
- Little Green Books (Quantitative Methods)
- Little Blue Books (Qualitative Methods)
- Dictionaries and Encyclopedias
- Case studies of real research projects
- Sample datasets for hands-on practice
- Streaming video--see methods come to life
- Methodspace- -a community for researchers
- SAGE Research Methods Course Mapping
Library Data Services at UC Berkeley
Library Data Services Program and Digital Scholarship Services
The LDSP offers a variety of services and tools ! From this link, check out pages for each of the following topics: discovering data, managing data, collecting data, GIS data, text data mining, publishing data, digital scholarship, open science, and the Research Data Management Program.
Be sure also to check out the visual guide to where to seek assistance on campus with any research question you may have!
Library GIS Services
Other Data Services at Berkeley
D-Lab Supports Berkeley faculty, staff, and graduate students with research in data intensive social science, including a wide range of training and workshop offerings Dryad Dryad is a simple self-service tool for researchers to use in publishing their datasets. It provides tools for the effective publication of and access to research data. Geospatial Innovation Facility (GIF) Provides leadership and training across a broad array of integrated mapping technologies on campu Research Data Management A UC Berkeley guide and consulting service for research data management issues
General Research Methods Resources
Here are some general resources for assistance:
- Assistance from ICPSR (must create an account to access): Getting Help with Data , and Resources for Students
- Wiley Stats Ref for background information on statistics topics
- Survey Documentation and Analysis (SDA) . Program for easy web-based analysis of survey data.
Consultants
- D-Lab/Data Science Discovery Consultants Request help with your research project from peer consultants.
- Research data (RDM) consulting Meet with RDM consultants before designing the data security, storage, and sharing aspects of your qualitative project.
- Statistics Department Consulting Services A service in which advanced graduate students, under faculty supervision, are available to consult during specified hours in the Fall and Spring semesters.
Related Resourcex
- IRB / CPHS Qualitative research projects with human subjects often require that you go through an ethics review.
- OURS (Office of Undergraduate Research and Scholarships) OURS supports undergraduates who want to embark on research projects and assistantships. In particular, check out their "Getting Started in Research" workshops
- Sponsored Projects Sponsored projects works with researchers applying for major external grants.
- Next: Quantitative Research >>
- Last Updated: Aug 6, 2024 3:06 PM
- URL: https://guides.lib.berkeley.edu/researchmethods
Repetitive research: a conceptual space and terminology of replication, reproduction, revision, reanalysis, reinvestigation and reuse in digital humanities
- Original Paper
- Open access
- Published: 06 November 2023
- Volume 5 , pages 373–403, ( 2023 )
Cite this article
You have full access to this open access article
- Christof Schöch ORCID: orcid.org/0000-0002-4557-2753 1
1934 Accesses
2 Citations
4 Altmetric
Explore all metrics
This article is motivated by the ‘reproducibility crisis’ that is being discussed intensely in fields such as Psychology or Biology but is also becoming increasingly relevant to Artificial Intelligence, Natural Language Processing and Digital Humanities, not least in the context of Open Science. Using the phrase ‘repetitive research’ as an umbrella term for a range of practices from replication to follow-up research, and with the objective to provide clarity and help establish best practices in this area, this article focuses on two issues: First, the conceptual space of repetitive research is described across five key dimensions, namely those of the research question or hypothesis, the dataset, the method of analysis, the team, and the results or conclusions. Second, building on this new description of the conceptual space and on earlier terminological work, a specific set of terms for recurring scenarios of repetitive research is proposed. For each scenario, its position in the conceptual space is defined, its typical purpose and added value in the research process are discussed, the requirements for enabling it are described, and illustrative examples from the domain of Computational Literary Studies are provided. The key contribution of this article, therefore, is a proposal for a transparent terminology underpinned by a systematic model of the conceptual space of repetitive research.
Similar content being viewed by others
Emergent Theory and/as Doctoral Research
Open Science, Replicability, and Transparency in Modelling
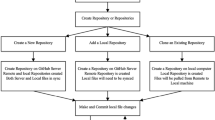
Furthering Open Science in Behavior Analysis: An Introduction and Tutorial for Using GitHub in Research
Explore related subjects.
- Artificial Intelligence
Avoid common mistakes on your manuscript.
1 Introduction
Why on earth should the study of space configurations and their structure be beneficial when dealing with meaning in language? - Dominic Widdows, Geometry and Meaning , 2004.
The aim of this contribution is to systematically describe, using a multi-dimensional conceptual space and a set of descriptive terms for recurring scenarios within that conceptual space, a specific research practice in the field of Digital Humanities (DH), a mode that, broadly defined, could be be termed repetitive research (RR).
Firstly, the mode of research I’d like to describe is one that repeats , in the sense that studies following this mode actively seek to align their research questions or hypotheses, their datasets and/or their methods of analysis, with research practiced and published earlier. This is done with the explicit aim to approximate an earlier study, but conscious also of the fact that perfectly identical repetition is virtually impossible to achieve. In many cases - and this might be a particularity of this kind of research in the humanities, where influential research may remain relevant for many decades -, this also means that the earlier research that is to be repeated has been practiced within the non-computational, or at least in the non-digital, paradigm. Any attempt at exact repetition can in fact only, in such a scenario, result in a reenactment and an approximation of earlier research.
Secondly, this mode of research is repeatable , in the sense that it (typically) makes all the efforts it can to provide the data, code, and explanatory information that make it possible for others, at a later point in time, to perform the same (or very similar) research again. Of course, an earlier study that aimed to be repeatable will be more amenable to a later study repeating it than one that did not consider this to be an important goal. In that sense, not only does repeatability foster repetition, but also the other way around; both issues are in fact two sides of the same coin. Actually, most research that repeated earlier research also aims to be repeatable, because this kind of repeatability, closely related to transparency and sustainability, is something that researchers come to value when they practice the hardships of repeating earlier research.
Repetitive research has important functions in the research process and makes essential contributions to the production of knowledge that are closely related to core values of the scholarly enterprise such as reliability, trustworthiness, transparency and sustainability, as discussed further below. As formulated elsewhere: “In this way, this kind of research is located between past and future: a (never identical) reenactment of past research, and an invitation for (never identical) further reenactments in the future. This mode of research is practiced with the conviction, or at least in the hope, that this cycle of repetitions is not a futile treading in the same place, but a productive, insightful upwards spiral.” (Schöch, 2023b )
In terms of the scope of this contribution’s argument, the examples and use cases employed in the present contribution for illustration and clarification pertain to the growing subfield within DH called Computational Literary Studies (CLS). Most of the arguments with respect to the role of data and methods in RR are valid primarily for research that operates with datasets that represent the domain being investigated as well as with algorithmic implementations of the method of analysis. This means that the basic ideas are likely to be applicable only to other fields within the Digital Humanities that use algorithmic methods applied to evidence available in the form of digital data, sometimes collectively called Computational Humanities. Such studies are most amenable to RR but clearly do not represent all or even most of research in DH.
The remainder of this paper is structured as follows. In Section 2 , a trailblazing example of RR is provided as a first way of approaching the issue. Then, Section 3 briefly motivates the paper, mostly by way of a defense of RR as a useful and indeed necessary answer to the limited transparency, corroboration and sustainability that characterizes much of present research. In Section 4 , earlier work on the topic is discussed, with a focus on the terminological issues surrounding RR. In Section 5 , a solution to this situation is proposed, based on a systematic description of the practice of RR as a multi-dimensional semantic space. From this space, one can derive not only an understanding of the structure of RR, but also a clear and well-motivated terminology for recurring scenarios within RR. In Section 6 , several such scenarios are defined, discussed and illustrated. By way of a conclusion, in Section 7 , some of the benefits and limitations of the proposed terminology, but also of RR more generally, are discussed.
In 2015, Geoffrey Rockwell gave a talk at the University of Würzburg titled “Replication as a way of knowing” (Rockwell, 2015 ). In this talk, he presented work he had done together with Stéfan Sinclair on reenacting a classic, very early, stylometric study by Thomas C. Mendenhall. This talk is an early example of the idea of RR, not just as a practice, but as a programmatic research principle, in the domain of CLS.
The story starts in 1887, when the US-American scientist Thomas C. Mendenhall (1841-1924) published an article in Science titled: “The Characteristic Curves of Composition” (Mendenhall, 1887 ). His fundamental idea was that it was possible to identify the author of a text by the characteristic distribution of word lengths in his or her texts. For example, Fig. 1 shows the word length distribution plot that Mendenhall obtained for the first 1000 words of the novel Oliver Twist by British 19-century author Charles Dickens.
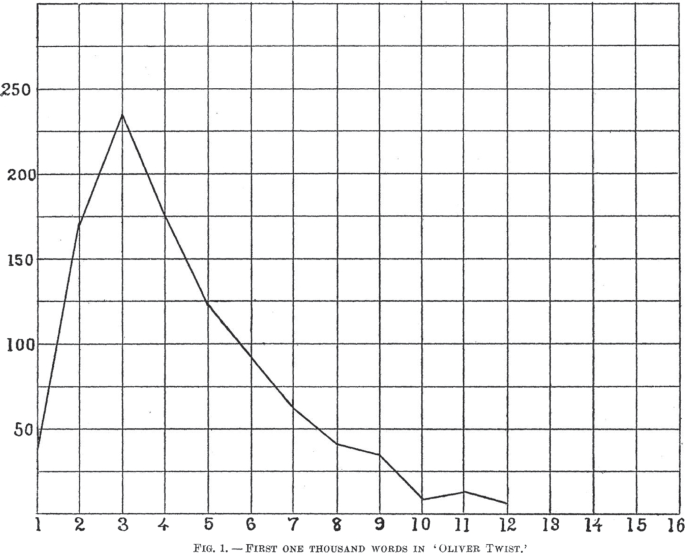
Plot from Thomas C. Mendenhall’s study “The Characteristic Curves of Composition”, here showing the word length distribution for the first 1000 words of Charles Dicken’s novel Oliver Twist
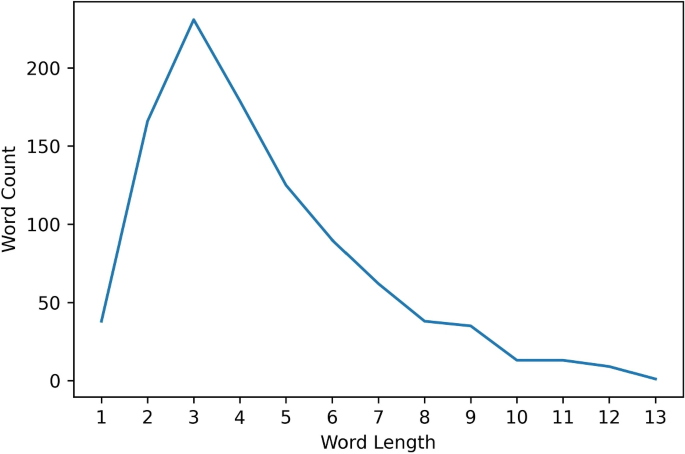
Plot obtained by Sinclair and Rockwell for the word length distribution for Charles Dicken’s novel Oliver Twist , based on the first 1000 words
In their repeating study, Stéfan Sinclair and Geoffrey Rockwell started out with the idea to implement Mendenhall’s study once more, but using digital texts and some simple algorithms (Sinclair & Rockwell, 2015 ). When they did so, they obtained nearly identical results (Fig. 2 ). Then, however, they repeated the same analysis for the entire novel and now obtained a distribution that, despite being recognizably related, clearly deviated from Mendenhall’s results as an effect of the much longer text being analysed (Fig. 3 ).
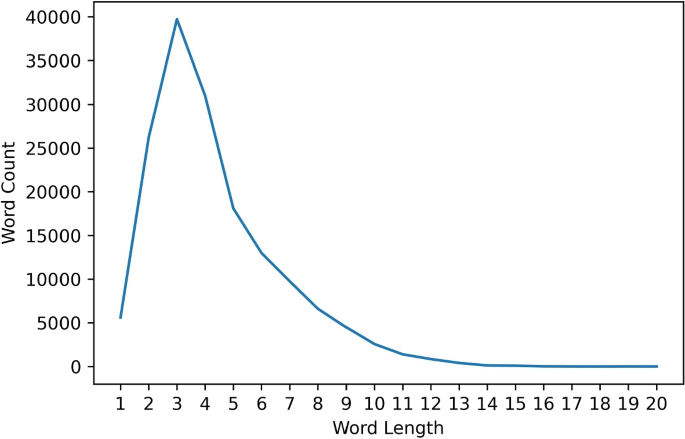
Plot obtained by Sinclair and Rockwell for the word length distribution for Charles Dicken’s novel Oliver Twist , based on the entire novel
Many of the typical properties of RR are already present in this seemingly simple study. The starting point is a more or less famous example of early quantitative (in this case of course non-digital) research. We find a close (but most likely not perfect) alignment in terms of the data: the digital edition Rockwell and Sinclair used (from Project Gutenberg ) is likely to be based on a print edition that is roughly contemporary, and therefore very similar, to the one Mendenhall used. Given that the process of scanning and text recognition is likely to introduce errors and deviations, even if exactly same edition had been used, it seems fair to assume that the data is not strictly identical (at the level of the character sequence). Also, given that this repetition involves crossing the analog-digital domain, it shares the basic methodological approach of determining the number of words of any given length, but makes use of a functionally-identical, but fundamentally different, algorithmic implementation of this method: Mendenhall must have tabulated and visualized this information by hand, whereas Sinclair and Rockwell have of course implemented the same process algorithmically, in Python.
In addition, we can notice efforts to approximate the earlier results, but also an attention for the slight and inevitable deviations. Note in Fig. 2 , for example, that there seem to be a few words with 13 letters that Sinclair and Rockwell found but Mendenhall did not. This might be an effect of differences in the underlying text (e.g. due to OCR errors, or to slightly deviating editions of the text), of differing understandings of what an individual word form or token is (Mendenhall says nothing about this), or of a (systematic or accidental) difference in the way that Mendenhall and Sinclair/Rockwell established the word length counts. It becomes clear that the authors employ this research strategy not so much as a way of checking the work of Mendenhall for flaws or errors (as would be the case in a strict replication study primarily designed as a verification of quality and integrity, basically inconceivable in this scenario), but in order to better understand his approach and, in the process, also think about their own computational methodology. One can also see a display of the advantages of the digital paradigm in terms of scale: once the code works with 1000 words, to which Mendenhall limited his investigation for obvious pragmatic reasons, it is trivial to expand the analysis to entire novels. This second, modified step in their approach, where the additional data used is purposefully distinct but still closely related to Mendenhall’s original data, places Sinclair and Rockwell’s study even further away from a strict replication. Given that replicating was not the goal, and that Sinclair and Rockwell’s results were quite similar to those of Mendenhall, one may still say that they confirmed Mendenhall’s findings. In the terminology proposed below, this research would best be described as a (successful and relatively close) reinvestigation (of a question) .
What Rockwell and Sinclair do not do, however, is follow Mendenhall’s further claims and actually try to perform authorship attribution with this kind of data. C.B. Williams had, in fact, in 1975, determined that this does not work very well. The primary reason for this is that word length count distributions appear to be at least as strongly affected by form (notably, by the distinction between texts in verse and in prose) as they are by authorship (Williams, 1975 ). So while Sinclair and Rockwell confirm that Mendenhall had worked with considerable accuracy, when they reimplemented his method, Williams had shown that the more general methodological conclusions regarding authorship attribution that Mendenhall had hoped to prove based on his experiments do not hold up to scrutiny.
Finally, Sinclair and Rockwell understood very well the link between repeating earlier research and making one’s research repeatable. Therefore, they made their own research processes transparent and easily repeatable by providing a Jupyter notebook that not only contains the code and the required data, but also some explanatory prose that helps others understand how the study works and how to easily run the code themselves. Footnote 1
3 Motivation
One may ask, however, why a more systematic approach to RR, in terms both of practice and theory, appears to be particularly useful today. The first motivation is the “reproducibility crisis” in a range of academic fields, as discussed further below. Footnote 2 The term has appeared around the year 2015 and has served to highlight findings from a study conducted by the Center for Open Science in Charlottesville (Open Science Collaboration, 2015 ). In this meta-study, the authors attempted to reproduce the results of 100 papers from several key journals in psychology, all first published in 2008. They were able to do so in just 40 percent of the cases. This was a shocking finding, despite the fact that there are many good reasons for such a result: Not only is it hard to avoid any and all so-called “questionable research practices” threatening reproducibility. The studies were also actually gathering relevant empirical data once more from scratch, not just checking the integrity of datasets and code provided. Finally, there are arguments that a certain level of non-reproducibility is to be expected as a matter of course (Hedges, 2019 ; Bird, 2021 ; Grieve, 2021 ). Still, a fundamental principle of the scientific method appears questioned by these results. The argument here is not that research as practiced today is not connected to the past and is not open to the future. Of course, we all read, learn from and quote earlier research extensively and hope that our own research will be read and quoted by others in the future. Research as practiced today is also not all unsound. In fact, it is an open question whether a field like DH can and should even be held to the kinds of standards relevant in the natural sciences (see, e.g., Peels & Bouter, 2018 ; Peels , 2019 ; Penders et al. , 2019 ). Different answers probably apply to different areas within DH, with the more immediately computational areas such as CLS more closely aligned with RR than other areas. But it seems clear that RR can be an important aspect to make sure our research is well-integrated into the research tradition and will be useful, trusted and well-received in the future.
The second motivation, more specific to DH, are fears of a disconnect between DH and the established Humanities. There is an ongoing discourse expressing fears of a disconnect or a mismatch between the contextualizing, interpretive, detail-oriented modes of research typical of disciplines in the Humanities, on the one hand, and formalizing, quantitative approaches in DH, on the other (e.g. Marche , 2012 ; Eyers , 2013 ; Da , 2019 . One of the main reasons for this fear is the assumption that, for instance, literary texts, historical documents or cultural artefacts are simply not suitable for elucidation through formal modeling, algorithms and computational analysis, because they are complex, semiotic, non-deterministic, contextual artefacts. Inversely, this would mean that CLS and other similar subfields within DH that ultimately rely on counting surface features, can only ask and answer a specific set of questions (such as authorship attribution) but are unable to make meaningful contributions to established, qualitative research in these fields (such as literary history or textual interpretation). This is of course a highly disputed position and the paradigm of RR, in particular, with its explicit focus on modeling and operationalisation across an earlier and a later study, can be a useful bridge between established, qualitative research on the one hand, and computational or quantitative research, on the other.
The third motivating factor is the most closely connected to CLS: it is the 2019 paper by Nan Z. Da in the influential journal Critical Inquiry , with the title “The Computational Case Against Computational Literary Studies” (Da, 2019 ). The author basically argues that computational, quantitative approaches are fundamentally unsuited for investigations into literary texts. And she argues that the selection of studies she looked at either had statistically solid results that were meaningless, or seemingly meaningful results that were not statistically sound. This paper is highly problematic and has been commented on and criticized extensively, for a large number of good reasons. Footnote 3 However, it also points to some serious and relevant challenges for the field of CLS: notably, the difficulty to reproduce work in this field, starting with issues of access to data and code, but also concerning questions of lacking reporting standards, limited scholarly recognition, and missing community commitment and capacity that would all be needed to foster a culture of RR in CLS and beyond Romero ( 2018 ).
4 Earlier work
Moving on from an understanding of the general relevance of RR, it appears useful to now consider more closely two key issues in selected contributions to the rather large body of existing conceptual work on RR: first, regarding terminological discussions around the definitions of repeatability, reproducibility, reproduction, replication, reanalysis and others; and second, regarding the purposes, functions and epistemological added value of RR in the research process.
The disciplinary focus of the present paper notwithstanding, the principle of RR is of course not limited to this field, quite the contrary. The “reproducibility crisis” (Baker, 2016 ) has first become very visible in fields such as social psychology, biology and medicine (e.g. Open Science Collaboration , 2015 ; Freedman & Inglese, 2014 ; Hunter , 2017 ) and has more recently become a major issue in Artificial Intelligence, Natural Language Processing and Linguistics (e.g. Hutson , 2018 ; Cohen et al. , 2018 ; Porte & McManus, 2019 ; Belz et al. , 2021 ; Berez-Kroeker et al. , 2022 ), three fields closely related to CLS. In recent years, the issue has also begun to be discussed in the (Digital) Humanities (e.g. KNAW , 2018 ; Peels & Bouter, 2018 ; Schöch et al. , 2018 ; Herrmann et al. , 2023 ), with the most prominent and controversial work applying replication in CLS certainly being the one, already mentioned, by Da ( 2019 ). However, the debate about the definition of relevant terms has occurred mostly outside the domain of DH.
The terminological situation, which can certainly be described as complex and confusing, is in itself another motivating factor for this paper, but earlier efforts to get to grips with the conceptual space and the terminology also present a substantial learning opportunity. The fact that the terminology has developed over time and often in parallel in different fields is part of the reason why it has become so unwieldy. Researchers have variously noted this, for example Goodman and colleagues, who note that “the language and conceptual framework of ‘research reproducibility’ are nonstandard and unsettled across the sciences" (Goodman et al. , 2016 , 1). Cohen and colleagues remark that, in addition to using terms with varying definitions, “it is not uncommon to see reproducibility and replicability or repeatability used interchangeably in the same paper” (Cohen et al. , 2018 , 156). Hans Plesser describes his contribution as “a history of a confused terminology” (Plesser , 2018 , 1). As Cohen and colleagues also note, “[t]his lack of consensus [in] definitions related to reproducibility is a problem because without them, we cannot compare studies of reproducibility (Cohen et al. , 2018 , 156).
Hans Plesser has summarized the “Claerbout terminology”, in which reproducing means the exact repetition of earlier research (where data, implementation and results should be identical), whereas replicating means using a new implementation and reaching similar conclusions (Plesser, 2018 ). Footnote 4 Plesser also explains that this is at odds with terminology in the sciences, for instance in Chemistry, where repeatability is understood as exact repetition within a lab under identical conditions (to measure within-run precision), and reproducibility means repetition of the same experiment, but performed by a different person in a different lab with different conditions (to measure between-run precision, so robustness). Since 2020, the ACM uses repeatability for the exact repetition of the same experimental setup by the same team, reproducibility for repetition of the same experimental setup by a different team, and replicability for repetition of a different experimental setup by a different team, swapping the latter two terms relative to earlier ACM usage (ACM, 2020 ). The ACM terminology, however, does not differentiate between several distinct aspects of the experimental setup, notably concerning the dataset and the method of analysis.
The contribution by Prasad Patil and colleagues is helpful not so much for the clarification of the terminology, but rather for a clear understanding of the conceptual space around reproducibility and replication (Patil et al., 2016 ). They address this issue by identifying a relatively large number of features (around a dozen) that can be used to describe the relationship between an earlier, original study and a later, repeating study. However, they do not attempt to directly relate the terms they propose to these descriptive dimensions.
Another contribution that explicitly aims to achieve a clarification of the terminological confusion is by Goodman et al. ( 2016 ). Their contribution is quite systematic in the way it considers key dimensions of RR, such as data, method and results. They propose research reproducibility as the cover term and note that an essential difference between different kinds of such research practices is whether or not they use new evidence, that is newly-gathered or otherwise additional data. They also note that the terms reproducibility and replication are frequently used to mark this difference, but without consistency as to which of the two terms is used for which scenario. Their proposal is to only use the term reproducibility , but to characterize it further depending on the specific scenario, in order to distinguish methods reproducibility (the exact repetition of earlier research), results reproducibility (using additional data to corroborate earlier results) and inferential reproducibility (where the methods of analysis and the conclusions drawn from results may be different). As far as I can see, this terminology has not been adopted widely.
A further terminological tradition, proposed for example by Drummond ( 2009 ) and explained very clearly by Huber et al. ( 2020 ) in the context of Natural Language Processing (NLP), is the following: “We use the term replication to refer to the activity of running the same code on the same dataset with the aim of producing the same (or sufficiently similar) measurements presented in the original paper. We use the term reproduction to refer to the activity of verifying the claims with experimental settings that are different from the ones in the original paper. For NLP experiments, this typically means reimplementation of the method(s) and the use of different datasets and/or languages” (Huber et al. , 2020 , 5604). In another contribution to the terminological debate within NLP, Cohen et al. ( 2018 ) follow this terminological tradition, but use reproducibility also as a relatively broad cover term. Footnote 5 Another terminological strand using replication as terminological point of departure is the one found in applied linguistics, where strict, approximate and conceptual replication are sometimes distinguished (see e.g. Porte and McManus , 2019 ).
What also becomes clear from the research literature is that there are not only different kinds of RR, but that they also have different functions in the research process or make different contributions to the production of knowledge. Indeed, these functions and contributions are closely related to core values of the scholarly enterprise such as reliability, trustworthiness, transparency, sustainability and progress, fundamental not only in the context of Open Science. As formulated by Freedman and Inglese: “Research advances build upon the validity and reproducibility of previously published data and findings” (Freedman & Inglese, 2014 ). As a consequence, functions of RR mentioned in the research literature include the “verification of a previously observed finding” (Gomez et al., 2010 ); “enhanc[ing] the reliability of research” Gomez et al. ( 2010 ); the “corroboration” of earlier evidence, claims or findings (Babin et al. , 2021 ; Goodman et al. , 2016 ); converting “tentative belief to accepted knowledge” (Berthon et al., 2002 ), also in a Bayesian perspective of accumulating evidence (Sprenger, 2019 ); establishing the degree of robustness and/or generalizability of results (Goodman et al., 2016 ); and verifying the quality and integrity of research, for example with respect to identifying “(i) fraud, falsification, and plagiarism, (ii) questionable research practices, partly due to unhealthy research systems with perverse publication incentives, (iii) human error, (iv) changes in conditions and circumstances, (v) lack of effective peer review, and (vi) lack of rigor” (Peels , 2019 , 1). In summary, one may say with the authors of the Open Science Collaboration report: “Replication can increase certainty when findings are reproduced and promote innovation when they are not” (Open Science Collaboration , 2015 , 943). Being aware of these important functions may also help address what has been called a “publication bias” in replication studies, where (among other effects) research corroborating earlier work has a lesser chance of being submitted and published than work revising earlier conclusions (Berinsky et al., 2021 ).
Several conclusions can be drawn from this brief discussion: First of all, given that NLP is a field closely related to CLS, and for further reasons explained below, I propose to accept the terminology in the tradition of Drummond, where replication refers to the exact repetition of earlier research, adding further terms as necessary to describe research scenarios that differ, in some defined way, from replication. Second, it appears useful to use a limited number of relevant dimensions to describe the conceptual space of RR, notably research question, dataset, method, team, and results. And third, the discussion of the dimensions of the conceptual space and the distinct scenarios of RR should not only describe them in terms of their position in the conceptual space, but also in terms of their functions or purpose in the research process.
5 The conceptual space of RR
In this section, I would like to propose a typology of and terminology for RR that is based on a simplifying but useful multi-dimensional description of the conceptual space of RR. Describing the conceptual space in a systematic manner helps clarify which aspects of RR are relevant and what the meaning is of any term proposed in the terminology, because its meaning can be described by specifying the subspace in the overall conceptual space that is covered by the term. It also shows the similarities and differences as well as the distances between the meaning of specific pairs of terms and, of course, the relationships between studies that can be described using those terms.
The fundamental assumption behind this description of the conceptual space is that any research can be described, not fully of course, but fundamentally and usefully in the context of RR, by five dimensions:
(Q) The key research question being studied (or the key hypothesis or claim to be verified)
(D) The dataset used (or more generally, the empirical basis of enquiry)
(M) The research method employed (and its implementation, e.g. in a code-based algorithm or tool)
(T) The team performing the research (including, of course, the case of a one-person team)
(R) The result of the research (and the claims or conclusions supported by the results)
In addition, this model of the conceptual space assumes that the relationship between an earlier study that is being repeated and a later study that repeats it can, for any of these five aspects, be described as corresponding to one out of three simplifying types, conceived not so much as distinct categories, but as contiguous areas on a gradient from perfect identity to complete unrelatedness:
(1) Identical (exactly or virtually the same)
(2) Similar (more or less closely related)
(3) Unrelated (largely dissimilar or entirely different)
It is true, of course, that assuming just five dimensions and three possible values for each dimension is a simplification. It is also true that it may not always be possible to clearly distinguish between a scenario, for instance, where the dataset is functionally identical and one where the dataset is very similar to that of an earlier study, as the three values are meant to describe a gradient rather than sharply distinct categories. However, considering that this conceptual space defines no less than 3 5 =243 theoretically possible positions, I would argue that it provides more than enough differentiation to support the definition of a clear descriptive vocabulary that allows to characterize any repeating study with relatively little ambiguity. For some recurrent scenarios, we can define labels that function as shortcuts. For others, we may prefer to describe their exact position in the conceptual space. This gives us three ways to describe an instance of RR: We can use one of the terms proposed below, if one is applicable, for recurring scenarios of RR. We can describe a particular instance using a verbal description containing the five dimensions and three values. And we can express such a verbal description in the condensed form of a vector-like representation.
As a first illustration, we could describe Sinclair and Rockwell’s study repeating earlier research by Mendenhall (discussed above) in the following way, focusing in a first step on the initial part of their study: it pursues an identical research question or hypothesis (Q=1), uses very similar data (D=2) and a method that, while aiming to be functionally identical, is realized in quite a different manner (M=2), was performed by an entirely unrelated team (T=3) and obtained very similar, though not entirely identical, results (R=1). The vector-like representation of this scenario would therefore be RR (Q,D,M,T,R) = (1,2,2,3,1, respectively). With regard to the set of terms proposed below, this scenario is best characterized as a (rather close) reinvestigation (of the question) . Footnote 6 One could further deduce from the description that the repeating study likely corroborated the earlier study because with additional relevant data, it came to a very similar conclusion to the same research question.
6 A terminology of RR
The question that arises from the description of the multi-dimensional conceptual space is how some of the recurrent scenarios within this semantic space can be delimited and labeled. This is likely to always remain controversial, but once the conceptual space is clearly defined, the terminology in fact becomes somewhat less of a matter, because it is always possible to define terms with respect to the conceptual space, or to describe a specific scenario independently of a given term. In this perspective, the terms become convenient shortcuts that have their usefulness and importance, but that are ultimately not essential.
As mentioned, 3 5 =243 possible combinations or different scenarios of RR are clearly more than we would care to qualify using individual terms. One part of the terminological complexity in the field stems from the fact that, even if one were to agree on the structure of this conceptual space, it can be divided up in multiple ways; another stems from the fact that the inventory of semantically suitable terms for different areas of the conceptual space contains many terms that are very similar to each other in form and meaning and, as a consequence, have been used interchangeably at different times and in different fields (see Section 4 ).
However, if we set aside for a moment the dimensions four and five (team and results) - important descriptive aspects that do not require, however, to be included in distinctions between recurring scenarios of RR -, we obtain a three-dimensional conceptual space that can be visualized as a cube and that, with its three possible values in each dimension, distinguishes just 3 3 =27 distinct subspaces (see Fig. 4 ).
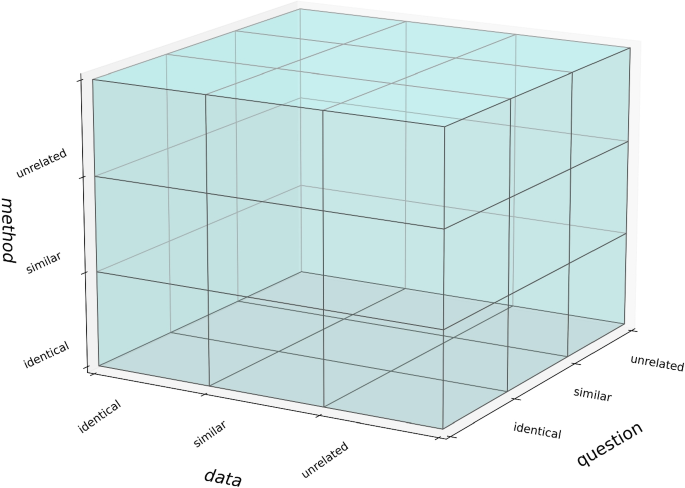
The three-dimensional conceptual space of repeating research. - The corner at the front, bottom, left side of the cube represents the closest possible relationship between an earlier and a later study (1,1,1 - replication), whereas the corner at the back, top, right side of the cube represents the area of maximum difference between two studies (3,3,3 - unrelated research)
Within this cube, we can define specific subspaces and provide terms for them. I propose to distinguish the following terms, as shown in Table 1 with a short definition and their vector shortcut, to provide an overview before describing them in more detail in the following sections:
These terms and the scenarios of RR they designate fall into three groups: (1) Replication (of research) , the only scenario that implies an exact (strictly identical or very close) repetition of earlier research; (2) reproduction (of results) , revision (of method) and reinvestigation (of the question) , in which the research question remains the same but the data, or the method, or both deviate to a limited degree and which can be understood to be approximate repetitions, due to the clear and close relationship that they establish between an original study and a repeating study; and (3) the remaining scenarios, reanalysis (of data) , reuse (of data) , reuse (of method) and follow-up research , scenarios that can still be called related research, but are further removed from the original study than the previous group, given that they deal with a differing research question and include additional differences. The exact subspace that each term covers as well as the best term for a given subspace may be subject to debate and revision, of course; a debate that is, however, supported by the explicit conceptual space that underpins the terminology.
Note that the recurring scenarios of RR described here do not exhaust the space defined by the three dimensions and three values. Certain combinations do not make a lot of sense and are unlikely to be a recurring practice in research. For example, investigating an entirely different or unrelated research question with the same data and method, while a theoretical possibility of the conceptual space (3,1,1), does not appear to be easily feasible. Similarly, the scenario where question and data are identical, but the method is unrelated (1,1,3), appears simply unrealistic and would probably rather be a case of revision (of method) (1,1,2). Finally, it is of course possible to envision research that would employ a question, data and method that are all unrelated to earlier research (3,3,3), but in the absence of any systematic relationship to earlier work, this scenario cannot be meaningfully included under the category of RR.
Note also that the terms proposed here for the recurring scenarios of repeating research, as defined in Table 1 , are meant to describe the relationship between an earlier, original study and a later, repeating study. However, if the concern is to characterize a given study with respect to the degree to which it enables repetition, rather than performing it, then the corresponding terms expressing the ability to perform a certain type of RR can be used, that is replicability (of research), reproducibility (of results), reanalysability (of data) and reusability (of data or code). More than one term may be used to describe a given study, in this case, for example to say that the reusability of its data is high (e.g. because the data is encoded in a widespread data format and is well-documented), but the replicability of the research as a whole is expected to be low (e.g. because the code has not been provided). Finally, the terms or values, respectively, may be further characterized by describing a study, for instance, as being a ‘close reproduction’ or a dataset as being ‘broadly similar’, etc.
The following discussions of the scenarios describe each scenario’s location in the conceptual space, including by way of a visualization, argue what constitutes a successful repetition in that scenario, comment on the functions or purpose of this scenario in the research process, name the requirements for enabling this scenario and provide one or several examples of this scenario from CLS.
6.1 Replication (of research)
Within the terminology proposed here, the term replication (of research) designates practices of RR in which the research question, the dataset and the method of analysis of the repeating study are all identical (or virtually identical) to the original study (1,1,1) (see Fig. 5 ). The term can be used irrespective of the team and the results. The term replication is preferred for this configuration of strict repetition without any significant modification because it is etymologically related to Italian ‘replica’ (copy, repetition) and Latin ‘replicare’ (to duplicate, to repeat) and because this is the accepted meaning of the term in Natural Language Processing, a field closely related to CLS (as discussed in Section 4 ).
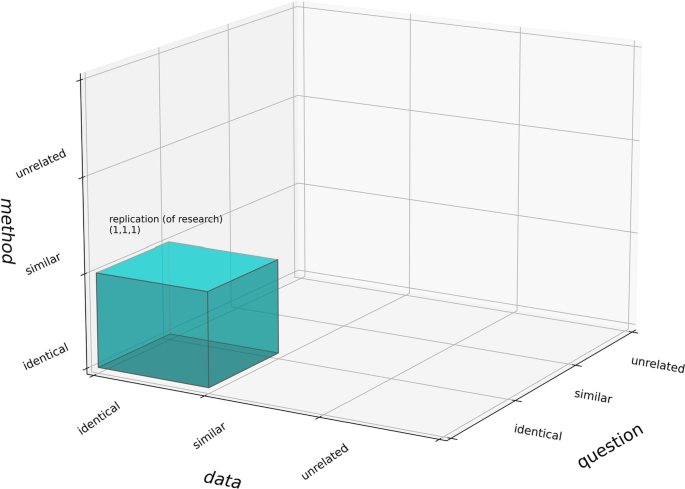
The scenario replication (of research) (1,1,1) within the conceptual space of RR. - Note that the boundaries are meant to be fuzzy, and that even a single cell within the cube encompasses a range of degrees of similarity and difference
Within a strict replication , only if the results of the repeating study are exactly identical to the original study, can such a replication be said to be successful, while all other outcomes would signal some flaw in the data and/or the code and would mean the replication was unsuccessful (but see remarks below). In this sense, the purpose of replication is to check the integrity and correctness of the code when applied to the data. Generally speaking, then, such a replication does not add new knowledge about the domain or research question but rather serves as a quality check. If the team performing the replication is identical or very similar, for example coming from the same research group, the function of this replication would amount to an internal check of the research as a whole, ensuring for example the completeness of the dataset, the functionality of the implementation and the correct match between data and code. If the team is unrelated, for example when a replication is performed as part of a peer-review process, the function of this replication would be quite similar, but would likely also be helpful in order to ensure the completeness of documentation that allows a third party to run the code on the dataset; in addition, it would serve to ensure the integrity of the research process in the sense of verifying that no manual manipulation has occurred in the original study that would affect reported numerical results or data visualisations. Compared to other scenarios, strict replication , then, is important for quality assurance rather than for advancing knowledge.
Note, however, that even within this relatively narrow scenario, some degree of variation is conceivable. In any case, entirely exact replication will frequently not be feasible, and strictly identical results can therefore often not be expected. The implementation and the results obtained from applying it to the data may deviate from the original results, for example based on the properties of the statistical and/or probabilistic methods employed in it or because the algorithms are run in an environment with fundamental technical properties related to hardware and/or operating system that are distinct from the original study. In any case, the greatest epistemological value lies not in strict replication, but in subtle and controlled departures from the original study design, as also noted, e.g. by Porte and McManus ( 2019 ) or Hedges ( 2019 ) and as described in the following scenarios. Research designed for repetition can also purposefully enable such insight through opening up paths for controlled deviations, for example by making it easy and explicit, in the code, to explore alternative choices with respect e.g. to selection of data or features, parameters for preprocessing, or use of specific measures or calculations.
The requirements for enabling replication (of research) are quite high, as the complete dataset and all code need to be available in a form that allows running the code without modifications or new implementation. It can be noted that providing data (and code) with publications - supporting both transparency, replicability and sustainability - is becoming increasingly common, and sometimes even expected, in CLS. Footnote 7 If data and code are available and can be run, then performing the replication itself is comparatively trivial. This usually requires some degree of documentation, although at minimum or in a first step, a replication can treat the code and data as a black box, focusing on the comparison of the results reported in the original study and those obtained by running the code on the data once more. However, as soon as something does not quite work as expected, documentation and insight into the code and data become crucial, of course. Also, sustainability is an issue here, when underlying libraries and environments become incompatible over time. Depending on how strict the replication is meant to be, specific versions of operating systems, programming languages and packages may need to be used, or data and code may even need to be packaged within a containerized environment Börner et al. ( 2023 ). Footnote 8
The study by Nan Z. Da mentioned above can be understood to have been intended, in part, as a replication study, although it is not quite clear from the paper and the relevant Github repositories to what extent individual studies were actually replicated in their entirety. It appears that for the most part, either data or code, or both, were not available and a full replication of the research was therefore not possible. In any case, such complete replications have not been documented online. Footnote 9 As mentioned, another scenario where strict replication happens is either as a project-internal quality-assurance measure or, more formally, as part of the peer-review process. However, a full replication step in the peer-review process is still the exception in DH-related journals and in most cases, there would be no publicly available record of this process, except in an open peer-review scenario. Footnote 10
6.2 Reproduction (of results)
Another recurring configuration is the one in which research question and method of analysis remain identical, with respect to the original study, but in which the dataset, instead of also being identical, may be more or less similar, but not unrelated (1,2,1). (See this and the following scenarios, all instances of approximate repetition departing from replication in a particular way, in Fig. 6 ). Again, this is independent of the team and the results. The term that appears best suited to describe this configuration is reproduction (of results) , because this is a frequent scenario and the term is well-established, albeit not always in this precise meaning. In addition, one may motivate it by its etymology, in the sense that it is derived from biological reproduction which, at least in the case of sexual reproduction, does not imply the production of organisms that are identical to their parents, but rather the production of somewhat modified organisms.
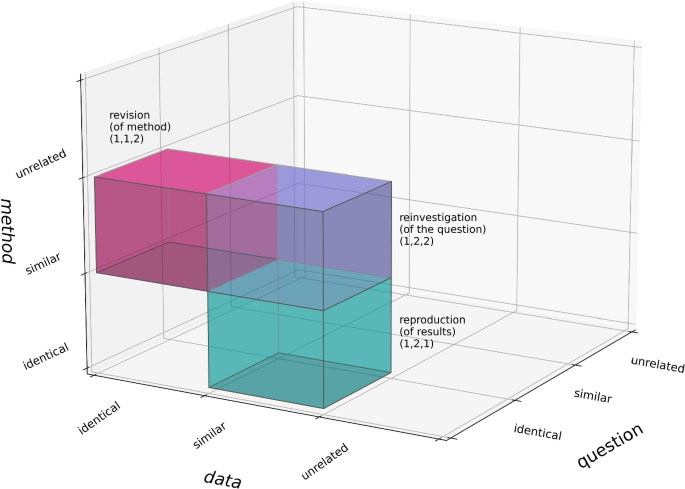
Three scenarios of approximate repetition within the conceptual space of RR. - Reproduction (of research) , revision (of method) and reinvestigation (of the question) are distinct ways of a limited departure from the replication scenario
In this scenario, the function or purpose is to verify whether or not, with another set of similar (i.e., relevant but not identical) data, the results of the original study can be confirmed. If the reproduction of the results is successful, then the results of the original study are corroborated in the sense of that the initial results, being valid across more than one dataset, are in this way shown to be valid more generally or more broadly than if they hold true only for one particular dataset. Such a success is also a confirmation that the method of analysis is robust across multiple datasets or that a theory or claim holds up even when it is tested on another relevant dataset (on theory testing, see Brendel et al. , 2021 ). The more dissimilar the data, the stronger the corroboration and generalizability, because the results can be understood to be more robust and supported by more evidence.
The requirements for enabling reproduction (of results) are relatively high, in the sense that either the original implementation of the method needs to be available in order for a (very close) reproduction to be able to reuse it, or the method needs to be documented with sufficient detail and precision for a functionally-identical reimplementation and thus a somewhat looser reproduction to be possible. In addition, because it is important to be able to determine the exact degree of similarity between the dataset of the original study and that of the repeating study, in order to correctly interpret any differences in results, the dataset needs to be available or at least described in sufficient detail.
This scenario appears to be frequent in the sciences, for example in the famous case of the Open Science Collaboration cited above (Open Science Collaboration, 2015 ), where new but equivalent data was obtained empirically in order to verify whether applying the same analysis to the data would lead to a confirmation of the results obtained in the original studies. In CLS, where we have very different conditions for obtaining new but equivalent data, studies that can be described as a reproduction (of results) occur especially in the context of the development and evaluation of methods. For example, in stylometric authorship attribution, methods and measures have typically been proposed using English-language corpora (classic cases being Burrows’ Delta and Zeta, see Burrows , 2002 , Burrows , 2007 ). There are quite a number of studies implementing these measures as closely as possible (given that Burrows described the measures, but did not provide a code-based implementation) or reusing a reference implementation in a tool such as stylo (Eder, 2016 ) and evaluating the measures using additional datasets containing different literary genres and/or different languages, both for Delta and Zeta (see Hoover , 2004 ; Rybicki & Eder, 2011 ; Evert et al. , 2017 ; Craig & Kinney, 2009 ; Schöch et al. , 2018 ). Footnote 11 A different example of such a study is Du ( 2023 ), in which the author performed an identical evaluation study twice, once with a dataset consisting of newspaper texts, and once more with a comparable dataset that, however, consists of literary texts. The purpose of this approach is clearly to reach increased generalizability of the results.
6.3 Revision (of method)
This scenario involves an identical research question and an identical dataset, but the use of a method of analysis that is only more or less similar, rather than identical, to the original one. This scenario can be termed revision (of method) , whether it concerns a functionally-similar reimplementation of an earlier method or a revised but closely-related method or measure. Again, this is irrespective of the team or the results. The idea here is to use a similar method to investigate the same question using the same data, as a way of verifying whether or not it is possible to arrive at the same conclusions using a new, but functionally-similar implementation or a different, possibly superior, version of the same method of inquiry.
The requirements for enabling such a revision of an earlier method are similar to those for reanalysis (of data) described below, namely that the dataset be made available. In addition, it is essential in this scenario that the research question be defined with considerable precision, so that exactly the same issue can be investigated. In case the method used in the original study was not implemented algorithmically or the code is not made available, a very detailed, step-by-step description of the method is required for an equivalent reimplementation to be feasible.
The purpose of a revision of the method using the same data is to investigate the robustness of earlier results or to propose an improved method. If a non-identical but similar method (for example involving a distinct but related statistical measure) investigating the same features of the same data nevertheless produces the same findings or solves an issue with comparable performance, then the results of the earlier research are corroborated. Similarly, if the reimplemented method involves the selection of dissimilar features in the same dataset, and still comes to the same results, this is again a corroboration of the earlier results. Note that in terms of systematic progress in scientific knowledge construction, the controlled departures from replication that are constituted by reproduction and revision are probably the most useful approaches. Scenarios that depart from the original study in more than one dimension at a time make it harder to clearly identify the source of any differences in results.
Papers in stylometric authorship attribution proposing a new measure of textual similarity (that follows the same distance-based methodological paradigm) and using one or several datasets for evaluation identical to those used in earlier research on similar methods are good examples of revision (of method) . A surprisingly rare example of such a systematic study is Smith and Aldridge ( 2011 ). The purpose of conducting such a study lies, in this case, in the fact that only by reusing the same dataset can results (e.g. the performance of a classifier or an attribution based on a distance measure) be comparable across studies and differences be precisely attributed to the new measure. A very different example of RR that includes a clear example of a revision (of method) is a review of Nicholas D. Paige’s book Technologies of the Novel (Paige, 2020 ) published by myself (Schöch, 2023a ). Footnote 12 Paige has made the full dataset used for the book publicly available and describes his analyses in detail in the book. However, he has not provided the code used to analyse and visualize the data. In a first step, most repetitions that I performed were therefore attempts to reconstruct specific plots contained in the book by developing reverse-engineered code that would functionally approximate the analysis that the author must have performed to obtain the plots, in order to verify that the plots are really based precisely on the data provided. This is probably as close to a replication as one can get when the code is not provided, but my Python code is likely to be sufficiently different from the method Paige used to generate the plots to call this a revision (of method) . In a second step, I purposefully departed from the original study by using slightly different analyses of the data and proposing alternative visualizations based on the same data, which places the study firmly in the domain of revision (of method) .
6.4 Reinvestigation (of the question)
The term reinvestigation (of the question) is used for the scenario where the research question is identical, but both data and method are more or less similar rather than identical, but not unrelated or radically different (1,2,2). This scenario is therefore somewhat more removed from an exact replication than both reproduction (of results) (where only the data is not identical) and revision (of method) (where only the method is not identical), but because the research question is still the same, it is assigned to the group of approximate repetition .
This is a rather frequent scenario, despite the fact that its purpose and usefulness are somewhat complicated by the fact that, with the repeating study differing from the original study in two key factors rather than just one, differences in results are difficult to relate specifically to either one of these two factors. This means it can neither serve as a quality check nor to evaluate a new method or to support generalization. However, it still allows to approximate an earlier study and to acquire further information on the earlier study’s research question. The reason for this scenario’s frequency is most likely pragmatic: Indeed, with proper benchmarking datasets still being rare in CLS, both the original data and the original method are often not readily available for reuse. In such cases, they have to be reconstructed with more or less accuracy from verbal descriptions or documentation. Also, a new dataset is often of greater interest to the researchers than earlier ones, for example because of its language or genre. In such cases, a strict reproduction (of results) or a revision (of method) is excluded.
As spelled out above, the study by Rockwell and Sinclair presented initially falls into this category. This approach is also typical of studies proposing, for example, a new method of stylometric authorship attribution and evaluate it on a new dataset, rather than on a dataset that had already been used earlier in similar authorship attribution studies. An example is Evert et al. ( 2017 ), who used datasets previously not analysed in distance-based authorship attribution evaluation. The recommended best practice here would be to perform three scenarios, when introducing a new method or measure: test the earlier method on a dataset used in earlier work, to prove the equivalence of one’s own implementation ( replication ); test the new method on the earlier dataset, for a comparison of performance ( revision ); and test the new method on a new dataset, to demonstrate the generalizability of the new method’s usefulness. A rare example actually following this best practice is, again, (Smith & Aldridge, 2011 ).
Outside of the development of measures and methods for authorship attribution, a study by a French team on new methods for direct speech recognition reusing but also modifying multiple earlier, suitably-annotated literary corpora falls into this scenario (Durandard et al., 2023 ). Also, my own study on sentence length in books by Belgian writer Georges Simenon, and in books by contemporary authors (Schöch, 2016 ), can be understood as an attempt to repeat earlier work on sentence length using a non-digital but clearly quantitative approach by Richaudeau ( 1982 ). The research question was the same, namely, whether Simenon’s success could be explained by his use of particularly short sentences. In a first step, I aimed to approximate the corpus of the original study, with limited success, then used a substantially expanded corpus of texts. Also, with the (manual) method of establishing sentence length not documented in detail by Richaudeau, my algorithmic procedure for this task was almost certainly quite different, even if the basic methodological approach was still fundamentally the same. This means the study combined a relatively close reinvestigation and a somewhat looser one.
6.5 Reanalysis (of data)
The scenario I propose to call reanalysis (of data) differs from revision (of method) in that in addition to the method of analysis of the repeating study not being identical to the original study’s method, the question being investigated can also diverge to some degree, while the data remains the same (2,1,2). In fact, a changing method of analysis may of course induce the research question to shift to some extent, whether intended or not by the researchers conducting the reanalysis. The term reanalysis appears fitting because the data remains the same and the overall perspective is still closely related to the earlier study. In contrast to this scenario, I propose the term reuse (of data) (described in Section 6.6 ) for a scenario in which question and method are clearly distinct from the original context and the same dataset is hence used for a largely unrelated purpose. (For a visual overview of three of the scenarios grouped under the term related research, see Fig. 7 ). Footnote 13
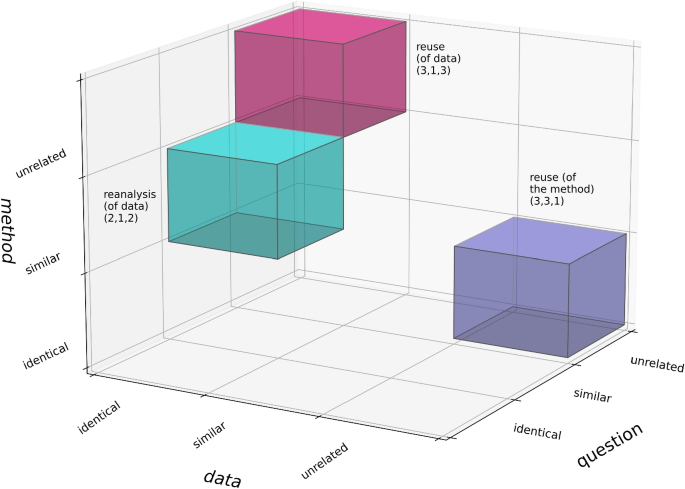
Three scenarios of related research within the conceptual space of RR: reanalysis (of data) , reuse (of data) and reuse (of method)
The function of reanalysis (of data) is primarily to examine a similar research question from a new but related methodological angle. If the reanalysis is successful in the sense of producing results supporting conclusions that are identical to those of the original study, then these conclusions are corroborated and their robustness is confirmed. Depending on how closely related the method used in such a reanalysis is to the original method, more or less similar results are to be expected. If the results turn out to be different, the reanalysis can be said to have been unsuccessful, pointing, however, to a potential flaw not only in the original study, but possibly also in the reanalysis of the data. Only once this can be ruled out would a differing result point to a potential flaw in the original study’s method and results.
The requirements for enabling reanalysis (of data) are clearly lower than for replication (of research) , because strictly speaking, only the dataset needs to be available in identical form, whereas the other aspects of the research will deviate from the original study in any case (but see the remarks on reusable datasets in Section 6.6 .
The practice of reanalysis is typically based on well-established datasets or corpora that have been available for a considerable amount of time. For example, there is a considerable number of studies broadly concerned with distinctions of text types or genres using the Brown Corpus (Francis & Kucera, 1979 ) but employing a wide range of more or less similar methods and approaches for a range of distinct, albeit related, research questions. For example, Karlgren and Cutting ( 1994 ) used discriminant analysis to support a classification mechanism for genres, with a focus on identifying discriminatory features. Some years later, Kessler et al. ( 1997 ) again used the Brown Corpus for genre classification, but using a different set of features and classification methods (logistic regression and neural networks), with a focus on classification accuracy. More recently, Kazmi ( 2022 ) again used the Brown Corpus for genre classification, but focusing on the fiction/non-fiction distinction and using logistic regression as the key method. Each time, the same dataset is analysed with a similar question and a related method, as well as with various degrees of success.
6.6 Reuse (of data)
For the scenario where the dataset used in a later study is identical to an earlier study, but the research question and method of analysis are very different or unrelated, I propose the term reuse (of data) (3,1,3). This scenario is therefore even further removed from strict replication than reanalysis (of data) . Note, however, the adjacent position of the two scenarios in the conceptual space and the potential overlap between the two terms.
The requirements for enabling reuse (of data) are comparatively simple: the dataset needs to be publicly available. However, as in all other cases where the dataset is required, this seemingly simple condition hides considerable complexity. Not only does the dataset need to be available, but it also needs to be understandable, interoperable, sufficiently well-documented and suitable for an inquiry into the new research question. For example, corpora need extensive metadata and a detailed documentation of the provenance, encoding and annotation of the included texts, while tabular datasets need a clear documentation of how the data was obtained and of the meaning of the various column headers. Footnote 14 As a consequence, in many cases where reuse of data is the goal, considerable effort is first invested into augmenting, cleaning, annotating or otherwise enhancing the dataset. The upside of such efforts is both the potential attention for one’s data by other researchers and a contribution to the sustainability of research.
These departures from the original study in terms of question and method are legitimate, in this scenario, because we are here in the domain of related research, where the function of reusing the data is not to check its quality or the quality of the study it was used in, but simply to save time and effort by reusing an existing dataset rather than creating a new one.
With the publication of corpora and datasets becoming increasingly common in CLS, there are many cases of reuse of data . While project-specific, research-driven datasets are sometimes not easily reused, others, in particular curation-driven corpora that are large in size, contain reliable texts, use standardized encoding, include rich metadata and provide detailed documentation, are routinely being reused. Examples for such corpora relevant to CLS include the Oxford Text Archive , the Deutsches Textarchiv , DraCor or the European Literary Text Collection (ELTeC). Footnote 15 Among the many examples of reuses of these corpora in CLS, one may mention a study of the chorus in a Spanish-language drama corpus included in DraCor (Dabrowsa & Fernández, 2020 ) or a study of the titles of novels across multiple languages included in the European Literary Text Collection (ELTeC) (Patras et al., 2021 ). Reuse of data is not limited to analysis, of course, but can also be performed when one or several existing corpora are used to create a new corpus, as in the case of the KOLIMO corpus which was created using texts from the TextGrid Digital Library , the Deutsches Textarchiv and Gutenberg-DE (Herrmann & Lauer, 2018 ).
6.7 Reuse (of method)
When the research question is similar, different or unrelated and the dataset is different or unrelated with respect to earlier research, and only the method or code is used in identical or very similar form, then we may speak of reuse (of method) (or of code) (3,3,1). Depending on how similar the dataset is and on how flexibly the code can be used, the research question may of necessity be more or less closely related to that of the original study.
Again, this scenario does not fall into the realm of exact or approximate repetition of earlier research, but it is nevertheless a specific mode of RR. And it does have a specific function, which is to save implementation time and increase the reliability and robustness of an implementation. In a sense, any use of a tools or software packages developed by others is reuse in this sense. Using existing tools such as stylo, MALLET or TXM (see Eder , 2016 ; McCallum , 2002 ; Heiden et al. , 2010 ) for one’s own purposes is, of course, a normal practice in Digital Humanities and CLS, so mentioning specific examples does not appear particularly useful in this case.
The main requirements for enabling efficient reuse (of method) are the availability of the code, software package or tool with a minimum of (financial or technical) hurdles as well as a detailed and understandable documentation.
6.8 Follow-up research
If the research question remains similar, but the dataset used as well as the method of analysis are either similar or unrelated, then I propose to use the term follow-up research (2,2-3,2-3). This relatively broad scenario is clearly in the domain of related research, rather than exact or approximate repetition, because there is a comparatively distant relationship between earlier studies and the later study in this case, linked essentially by the similar research question (see Fig. 8 , covering four subcubes). If the research question is different, but either data or method are identical, then reuse (of data) or reuse (of method) are more applicable scenarios. If all three dimensions are different, there is no (relevant) relationship anymore between the earlier and the later study and the scenario falls outside the scope of RR.
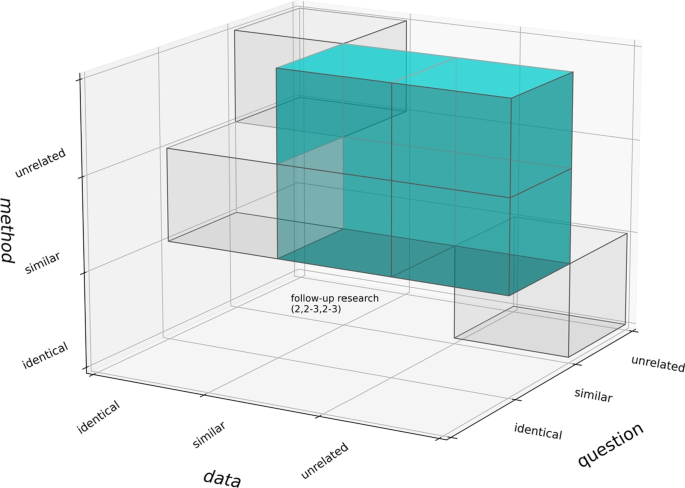
The scenario follow-up research) within the conceptual space of RR. - The other scenarios of related research are provided for visual context
In terms of functions of follow-up research , a later study on the same question that obtains similar results using distinct data and methods can certainly be understood as a corroboration of earlier studies on the topic, showing that these results are robust against variations in the dataset and the method used to elucidate the question. However, the relationship between the studies being much looser than in the scenarios of approximate repetition, differing results based on a follow-up study do not necessarily indicate that anything is wrong in the earlier study.
The requirements for enabling follow-up research are comparatively low, as research question, data and method or code do not need to be and usually are not identical and therefore need not be readily available for reuse. Pushed even further, for example by focusing on a research question that is different or unrelated to any other, earlier research questions, and this scenario can be understood either to break the chain of incremental increase in our knowledge about a domain, or to break entirely new ground.
My own study repeating a classic study by stylistician Leo Spitzer about French playwright Jean Racine is an example of both reinvestigation and follow-up research (Spitzer, 1931 , 1969 ; Schöch, 2023b ). The original intent was to perform a very close reenactment of this non-computational and qualitative study using digital data and methods, but it became clear rather quickly that a strict replication was impossible and that even a revision (of method) was hardly feasible, given the analog-digital and the qualitative-quantitative divides as well as the lack of information about the exact editions used by Spitzer. As a consequence, this instance of RR proceeded in multiple steps that became increasingly distant from the original study: Things started out with a most likely very similar corpus and a closely-related but distinct method (hence, reinvestigation , 1,2,2), but ended with a larger and more diverse corpus, a mixed-methods approach combining modeling of stylistic devices with statistical analysis that ended up even shifting the research question from Racine himself to Racine’s position among the contemporary authors: clearly, follow-up research , rather than any more specific type of repetitive research.
7 Conclusion
As a way to conclude, it appears useful to briefly reflect on the affordances and limitations of the proposed conceptual space and terminology of RR.
The primary affordance of the description of RR as structured into a five-dimensional conceptual space appears to be that this allows us to describe, with considerable granularity, precision and transparency, and without necessarily using a given terminology, the relationship between an earlier study and a later, related study. The main advantage of the set of terms proposed above is that they are clearly defined with respect to the conceptual space and that they provide us with convenient and distinct labels for a certain number of recurring scenarios within the conceptual space of RR. Several studies that have been designated with the same term, within this terminology, can reasonably be expected to show a considerable amount of similarity. Taken together, especially when considering that the conceptual space and the set of terms also come with a description of functions and requirements, the hope is that this conceptual work can support the community of researchers in CLS and DH more broadly to more clearly understand, value more appropriately, and more frequently practice RR.
A possible limitation of the conceptual space is the choice of the five dimensions. One may argue that the concrete implementation of a method, for example as an executable algorithm implemented in a programming language, should have been separated out from the method understood broadly and turned into an additional dimension. A similar argument may be made for separating the claims or conclusions from the raw results, instead of treating them as one shared dimension of research. That is true but doing so would come at the cost of additional complexity not only of the conceptual space, but also of the terminology it supports. Another potential limitation of the terminology as it is proposed here is that the terms identical , similar and unrelated , while clear enough in everyday settings, are of course not categorically separate classes, but three rather fuzzy areas on a continuum. This fuzziness, however, is embraced here. Specifications like functionally or strictly identical, or like closely or broadly similar may help clarify the usage in certain cases. Another possible limitation of the terminology is, of course, that despite efforts to minimize gaps between the terms, some degree of this appears to be inevitable if the number of terms is to remain manageable and if terms are reserved for particularly frequent or important scenarios. Footnote 16 Finally, people may disagree with the choice of terms themselves, in one or several cases. Fortunately, given that the terms and the conceptual space are defined quite explicitly, alternative ways of dividing up that space, alternative definitions of terms, or alternative terms for a given scenario can easily be proposed and exchanged.
With respect to the practice of RR itself, whether in CLS or in DH more broadly, there are some challenges, among them the considerable effort required to enable or perform RR, especially in the case of replication and the various forms of approximate repetition. In addition, there is the issue of a potential (or apparent) conflict with disciplinary values. Clearly, RR may be perceived to be at odds with the ways in which value is usually ascribed to research. Research usually needs to be original, innovative, ground-breaking, relevant, timely in order to be considered valuable. Apart from replication as quality control, the kind of research I advocate for here, in contrast, is fundamentally concerned with repeating research that has been completed years or even decades ago. Can such research be said to be valuable in this sense, or to foster excellence in research? Of course it can, precisely because it serves such important and varied functions in the research process, whether it is quality assurance and building trust (as in strict replication ), corroboration or generalization of results (as in several different scenarios), efficiency and sustainability (as in the reuse of data or tools) or incrementally but methodically pushing the boundaries of knowledge (as in reinvestigations and follow-up research ). In addition, practicing RR appears to be a learning opportunity to me, because one understands previous research much better when trying to replicate, reproduce or otherwise repeat it, including its strengths and limitations. More generally speaking, we also need RR as a way of guaranteeing the continuity, over time, of the disciplinary context of our work, especially in the Digital Humanities. Finally, and maybe most importantly, many of the functions of RR constitute or support best practices in the perspective of Open Science.
Availability of data and materials
Not applicable, but see data provided in Schöch ( 2016 ) and Schöch ( 2023b ).
Code Availability
Not applicable, but see code provided in Schöch ( 2016 ) and Schöch ( 2023b ).
See: https://github.com/sgsinclair/epistemologica .
Please note that the terminology varies widely, both between and within fields, and a systematic terminology will only be introduced in Section 6 .
Some of the reactions can be read on the blog section “Computational Literary Studies: A Critical Inquiry Online Forum” with responses invited by Critical Inquiry at https://critinq.wordpress.com/2019/03/31/computational-literary-studies-a-critical-inquiry-online-forum/ , others in the “Commentary” section of the Journal of Cultural Analytics at https://culturalanalytics.org/section/1580 .
Other authors following this understanding are Branco et al. ( 2020 ); Peng ( 2015 ).
Earlier papers from outside the NLP domain and also using this terminology are Berthon et al. ( 2002 ) and Gomez et al. ( 2010 ), the latter using reanalysis as their third term with a definition that is similar to the one proposed below.
One may argue over the issue of knowing whether this case represents a rather loose reproduction or a reasonably close reinvestigation , depending on how much weight one gives to the differences in dataset and method. The fact that the study crosses the analog-digital divide with respect to both data and method is my main argument for placing it in the latter category. The point of this paper, however, is not primarily to solve such arguments, but rather to enable their precise formulation.
This is evidenced, for example, in the submission guidelines of the Journal of Computational Literary Studies or the recent Calls for papers of the Computational Humanities Research conference. See the JCLS submission guidelines at https://jcls.io/site/guidelines/ (section “Data and Code Availability”) or, for instance, the 2023 CfP of CHR at https://2023.computational-humanities-research.org/cfp/ , which clearly expects authors to include code and data repositories when they submit papers. Similarly, the Journal of Open Humanities Data provides visibility for datasets and supports the recognition of dataset curation as a scholarly endeavor; see https://openhumanitiesdata.metajnl.com/ .
More generally, see the reservations and challenges discussed by Arvan et al. ( 2022 ) in Natural Language Processing, broadly applicable also to CLS.
In addition to the paper (Da, 2019 ), see the Github repositories at https://github.com/nan-da .
At The Programming Historian , the review process happens openly on Github, and testing any instructions or executing any code included in a lesson is certainly part of the review process. See https://github.com/programminghistorian/ph-submissions/issues for examples. Among the journals immediately relevant to CLS, only the Journal of Computational Literary Studies routinely expects code and data to be made available when papers are submitted but does not, by default, include a full replication step in the peer-review process (see JCLS , 2023 ).
Note that it is very common for such studies to start out with or include a measure that is virtually identical to previously proposed measures, but then also include tweaks to the measures and/or features used that go beyond the earlier proposals, meaning the studies move from reproduction (of results) towards revision (of method) (see Section 6.3 ) or even, when they also add new datasets, to reinvestigation (of the question) (see Section 6.4 ).
See, in particular, the Github repository accompanying the review at https://github.com/christofs/paige .
One may consider expanding the coverage of the three terms in this group to their adjacent subspaces, adding (3,1,2) to reanalysis (of data) , (2,1,3) to reuse (of data) and (3,2,1) or even (2,3,1) and/or (2,2,1) to reuse (of method) . These subspaces are not covered, in the present proposal, by other terms.
The sustainability and interoperability of data in the Digital Humanities is a well-researched topic of its own that is beyond the scope of this paper; standardized data models such as XML-TEI (for text) or RDF (for Linked Open Data) as well as controlled vocabularies and ontologies have an important role to play here; see e.g. Rehm and Witt ( 2008 ); García et al. ( 2016 ).
On these corpora, see: Morrison ( 1999 ); Haaf et al. ( 2022 ); Fischer et al. ( 2019 ); Schöch et al. ( 2021 ).
However, only once RR is practiced more frequently, will it become possible to observe which scenarios are indeed recurring, potentially leading to an adjustment of the terminology.
ACM. (2020). Artifact Review and Badging - Current. ACM Publications Policies and Procedures https://www.acm.org/publications/policies/artifact-review-and-badging-current
Arvan, M., Pina, L., Parde, N. (2022). Reproducibility in Computational Linguistics: Is Source Code Enough? In: Conference on Empirical Methods in Natural Language Processing. ACM, pp 2350-2361, https://aclanthology.org/2022.emnlp-main.150/
Babin, B. J., Ortinau, D. J., Herrmann, J. L., et al. (2021). Science is about corroborating empirical evidence, even in academic business research journals. Journal of Business Research, 126 , 504–511. https://doi.org/10.1016/j.jbusres.2020.06.002
Article Google Scholar
Baker, M. (2016). Is there a reproducibility crisis? Nature, 533 (7604), 452–454. https://doi.org/10.1038/533452a
Belz, A., Agarwal S., Shimorina A., et al. (2021). A Systematic Review of Reproducibility Research in Natural Language Processing. Proceedings of the 16th Conference of the European Chapter of the Association for Computational Linguistics: Main Volume pp 381–393. https://doi.org/10.18653/v1/2021.eacl-main.29
Berez-Kroeker, A.L., McDonnell, B., Koller, E., et al. (2022). Data, Data Management, and Reproducible Research in Linguistics: On the Need for The Open Handbook of Linguistic Data Management. In: Berez-Kroeker, A.L., McDonnell, B., Koller, E., et al. (eds) The Open Handbook of Linguistic Data Management. The MIT Press, https://doi.org/10.7551/mitpress/12200.001.0001
Berinsky, A. J., Druckman, J. N., & Yamamoto, T. (2021). Publication Biases in Replication Studies. Political Analysis, 29 (3), 370–384. https://doi.org/10.1017/pan.2020.34
Berthon, P., Pitt, L., Ewing, M., et al. (2002). Potential Research Space in MIS: A Framework for Envisioning and Evaluating Research Replication, Extension, and Generation. Information Systems Research., 13 (4), 416–427. https://doi.org/10.1287/isre.13.4.416.71
Bird, A. (2021). Understanding the Replication Crisis as a Base Rate Fallacy. The British Journal for the Philosophy of Science, 72 (4), 965–993. https://doi.org/10.1093/bjps/axy051
Börner, I., Trilcke, P., Milling, C. et al. (2023). Dockerizing DraCor - A Container-based Approach to Reproducibility in Computational Literary Studies. In: Book of Abstracts of the Digital Humanities Conference 2023 ADHO, Graz. https://doi.org/10.5281/zenodo.8107836
Branco, A., Calzolari, N., Vossen, P., et al. (2020). A Shared Task of a New, Collaborative Type to Foster Reproducibility: A First Exercise in the Area of Language Science and Technology with REPROLANG2020. In: Proceedings of the 12th Language Resources and Evaluation Conference. ELRA, Marseille, France, pp 5539–5545. https://www.aclweb.org/anthology/2020.lrec-1.680
Brendel, A. B., Diederich, S., & Niederman, F. (2021). An immodest proposal-going “All in” on replication research in information systems. European Journal of Information Systems, 1–10. https://doi.org/10.1080/0960085X.2021.1944822
Burrows, J. (2002). ‘Delta’: A Measure of Stylistic Difference and a Guide to Likely Authorship. Literary and Linguistic Computing, 17 (3), 267–287. https://doi.org/10.1093/llc/17.3.267
Burrows, J. (2007). All the Way Through: Testing for Authorship in Different Frequency Strata. Literary and Linguistic Computing, 22 (1), 27–47. https://doi.org/10.1093/llc/fqi067
Cohen, K., Xia, J., Zweigenbaum, P., et al. (2018). Three Dimensions of Reproducibility in Natural Language Processing. In: Proceedings of the 12th Language Resources and Evaluation Conference. ELRA, Marseille, France, https://aclanthology.org/L18-1025.pdf
Craig, H., Kinney, AF., (eds). (2009). Shakespeare, Computers, and the Mystery of Authorship, 1st edn. Cambridge University Press
Da, N. Z. (2019). The Computational Case against Computational Literary Studies. Critical Inquiry, 45 (3), 601–639. https://doi.org/10.1086/702594
Dabrowsa, M., Fernández, MTSM. (2020). Análisis del coro como personaje en la dramaturgia grecolatina y española incluida en DraCor. In: Digital Humanities Conference 2020: Book of Abstracts. ADHO. https://hcommons.org/deposits/item/hc:31881/
Drummond, C. (2009) Replicability is not Reproducibility: Nor is it Good Science. In: Proceedings of the Evaluation Methods for Machine Learning Workshop at the 26th ICML. National Research Council of Canada, Montréal
Du, K. (2023) Zum Verständnis des LDA Topic Modeling: eine Evaluation aus Sicht der Digital Humanities. Ph.D. Thesis. Würzburg University, Würzburg Durandard N, Tran VA, Michel G, et al (2023) Automatic Annotation of Direct Speech in Written French Narratives. https://doi.org/10.48550/arXiv.2306.15634,2306.15634
Durandard N, Tran VA, Michel G, et al (2023) Automatic Annotation of Direct Speech in Written French Narratives. https://doi.org/10.48550/arXiv.2306.15634,2306.1563
Eder, M., Kestemont, M., Rybicki, J. (2016). Stylometry with R: A package for computational text analysis. The R Journal, 16(1),1–15. https://journal.r-project.org/archive/2016/RJ-2016-007/index.html
Evert, S., Jannidis, F., Proisl, T., et al. (2017). Understanding and Explaining Distance Measures for Authorship Attribution. Digital Scholarship in the Humanities, 32,ii4–ii16. https://doi.org/10.1093/llc/fqx023
Eyers, T. (2013). The Perils of the ‘Digital Humanities’: New Positivisms and the Fate of Literary Theory. Postmodern Culture, 23(2). https://doi.org/10.1353/pmc.2013.0038
Fischer, F., Börner, I., Göbel, M., et al. (2019). Programmable corpora: Introducing dracor, an infrastructure for the research on european drama. In: Book of Abstracts of the Digital Humanities Conference 2019. ADHO, Utrecht. https://doi.org/10.5281/zenodo.4284001
Francis, W., Kucera, H. (1979). Brown Corpus Manual. https://korpus.uib.no/icame/manuals/BROWN/INDEX.HTM
Freedman, L. P., & Inglese, J. (2014). The Increasing Urgency for Standards in Basic Biological Research. Cancer research, 74 (15), 4024–4029. https://doi.org/10.1158/0008-5472.can-14-0925
García, EGB., Manailescu, M., Ros, S. (2016). From syllables, lines and stanzas to linked open data: Standardization, interoperability and multilingual challenges for digital humanities. Proceedings of the Fourth International Conference on Technological Ecosystems for Enhancing Multiculturality pp 979–983. https://doi.org/10.1145/3012430.3012635
Gomez, O.S., Juristo, N., Vegas, S. (2010). Replication, Reproduction and Reanalysis: Three ways for verifying experimental findings. In: International Symposium on Workshop on Replication in Empirical Software Engineering Research. ACM, Cape Town
Goodman, S.N., Fanelli, D., Ioannidis, J.P.A. (2016). What does research reproducibility mean? Science Translational Medicine, 8(341),341ps12–341ps12. https://doi.org/10.1126/scitranslmed.aaf5027
Grieve, J. (2021). Observation, experimentation, and replication in linguistics. Linguistics, 59 (5), 1343–1356. https://doi.org/10.1515/ling-2021-0094
Haaf, S., Boenig, M., Hug, M. (2022). Das Deutsche Textarchiv gestern und heute. Mitteilungen des Deutschen Germanistenverbandes, 69(2),127–134. https://doi.org/10.14220/mdge.2022.69.2.127
Hedges, L. V. (2019). The Statistics of Replication. Methodology, 15 (Supplement 1), 3–14. https://doi.org/10.1027/1614-2241/a000173
Heiden, S., Magué, J.P., Pincemin, B. (2010). TXM : Une plateforme logicielle opensource pour la textométrie–conception et développement. In: Statistical Analysis of Textual Data–Proceedings of 10th International Conference Journées d’Analyse Statistique Des Données Textuelles, pp 1021–1032, http://halshs.archives-ouvertes.fr/halshs-00549779
Herrmann, J.B., Lauer, G. (2018). Korpusliteraturwissenschaft. Zur Konzeption und Praxis am Beispiel eines Korpus zur literarischen Moderne. Osnabrücker Beiträge zur Sprachtheorie, 2018(92),127–156. http://nbn-resolving.de/urn:nbn:de:0070-pub-29556320
Herrmann, J.B., Bories, A.S., Frontini, F., et al. (2023). Tool criticism in practice. On methods, tools and aims of computational literary studies. Digital Humanities Quarterly 17(2) Hoover DL (2004) Testing Burrows’s Delta. Literary and Linguistic Computing, 19(4),453–475. https://doi.org/10.1093/llc/19.4.453
Hoover, D. L. (2004). Testing Burrows’s Delta. Literary and Linguistic Computing, 19 (4), 453–475. https://doi.org/10.1093/llc/19.4.453
Huber, E., Çöltekin, Ç. (2020). Reproduction and Replication: A Case Study with Automatic Essay Scoring. In: Proceedings of the 12th Language Resources and Evaluation Conference. ELRA, Marseille, France, pp 5603-5613, https://www.aclweb.org/anthology/2020.lrec-1.688
Hunter, P. (2017). The reproducibility ‘crisis’. EMBO Reports, 18(9),1493–1496. https://doi.org/10.15252/embr.201744876
Hutson, M. (2018). Artificial intelligence faces reproducibility crisis. Science, 359 (6377), 725–726. https://doi.org/10.1126/science.359.6377.725
JCLS. (2023). Code and data review. Submission Guidelines. https://jcls.io/site/code-data-review/
Karlgren, J., Cutting, D. (1994). Recognizing text genres with simple metrics using discriminant analysis. In: Proceedings of the 15th Conference on Computational Linguistics , vol 2. Association for Computational Linguistics, Kyoto, Japan, p 1071, https://doi.org/10.3115/991250.991324
Kazmi, A., Ranjan, S., Sharma, A., et al. (2022). Linguistically Motivated Features for Classifying Shorter Text into Fiction and Non-Fiction Genre. In: Proceedings of the 29th International Conference on Computational Linguistics. International Committee on Computational Linguistics, Gyeongju, Republic of Korea, pp 922–937. https://aclanthology.org/2022.coling-1.77
Kessler, B., Nunberg, G., Schuetze, H. (1997). Automatic Detection of Text Genre. https://doi.org/10.48550/arXiv.cmp-lg/9707002,cmp-lg/9707002
KNAW. (2018). Replication Studies . KNAW-Royal Netherlands Academy of Arts and Sciences, Amsterdam: Improving Reproducibility in the Empirical Sciences. Advisory Report. Tech. rep.
Google Scholar
Marche, S. (2012). Literature is not Data: Against Digital Humanities. Los Angeles Review of Books. http://lareviewofbooks.org/essay/literature-is-not-data-against-digital-humanities#
McCallum, A.K. (2002). Mallet: A machine learning for language toolkit, http://mallet.cs.umass.edu
Mendenhall, T.C. (1887) The Characteristic Curves of Composition. Science, 9(214),237–249. http://www.jstor.org/stable/1764604
Morrison, A. (1999). Delivering Electronic Texts Over the Web: The Current and Planned Practices of the Oxford Text Archive. Computers and the Humanities, 33 (1), 193–198. https://doi.org/10.1023/a:1001726011322
Open Science Collaboration. (2015). Estimating the reproducibility of psychological science. Science, 349(6251),aac4716. https://doi.org/10.1126/science.aac4716
Paige, N. D. (2020). Technologies of the Novel: Quantitative Data and the Evolution of Literary Systems . New York: Cambridge University Press.
Book Google Scholar
Patil, P., Peng, R.D., Leek, J.T. (2016). A statistical definition for reproducibility and replicability. bioRxiv p 066803. https://doi.org/10.1101/066803
Patras, R., Odebrecht, C., Galleron, I., et al. (2021). Thresholds to the “Great Unread”: Titling Practices in Eleven ELTeC Collections. Interférences littéraires/Literaire interferenties, 25,163–187. http://interferenceslitteraires.be/index.php/illi/article/view/1102
Peels, R. (2019). Replicability and replication in the humanities. Research Integrity and Peer Review, 4 (1), 2. https://doi.org/10.1186/s41073-018-0060-4
Peels, R., & Bouter, L. (2018). The possibility and desirability of replication in the humanities. Palgrave Communications, 4 (1), 1–4. https://doi.org/10.1057/s41599-018-0149-x
Penders, B., Holbrook, J. B., & de Rijcke, S. (2019). Rinse and Repeat: Understanding the Value of Replication across Different Ways of Knowing. Publications, 7 (3), 1–15. https://doi.org/10.3390/publications7030052
Peng, R. (2015). The reproducibility crisis in science: A statistical counterattack. Significance, 12 (3), 30–32. https://doi.org/10.1111/j.1740-9713.2015.00827.x
Plesser, H.E. (2018). Reproducibility vs. Replicability: A Brief History of a Confused Terminology. Frontiers in Neuroinformatics 11. https://doi.org/10.3389/fninf.2017.00076
Porte, G. K., & McManus, K. (2019). Doing Replication Research in Applied Linguistics . Routledge, New York, NY: Second Language Acquisition Research Series.
Rehm, G., Witt, A. (2008). Aspects of Sustainability in Digital Humanities. In: Digital Humanities Conference (DH2008): Book of Abstracts. ADHO. http://georg-re.hm/pdf/Rehm-et-al-DH2008.pdf
Richaudeau, F. (1982). Simenon : uneécriture pas si simple quón le penserait. Communication et langages, 53 (1), 11–32. https://doi.org/10.3406/colan.1982.1484
Rockwell, G. (2015). Replication as a way of knowing in the Digital Humanities. In: Lectures in Digital Humanities, University of Würzburg
Romero, F. (2018). Who Should Do Replication Labor? Advances in Methods and Practices in Psychological Science, 1 (4), 516–537. https://doi.org/10.1177/2515245918803619
Rybicki, J., & Eder, M. (2011). Deeper Delta across genres and languages: Do we really need the most frequent words? Literary and Linguistic Computing, 26 (3), 315–321. https://doi.org/10.1093/llc/fqr031
Schöch, C. (2016). Does Shorter Sell Better? Belgian author George Simenon’s use of sentence length. The Dragonfly’s Gaze [blog]. https://dragonfly.hypotheses.org/922
Schöch, C. (2023a). Nicholas D. Paige: Technologies of the novel: Quantitative data and the evolution of literary systems (Cambridge University Press, 2020) [review]. H-France Review 23(22). https://h-france.net/vol23reviews/vol23no22schoch.pdf
Schöch, C. (2023b) Spitzer on Racine. A Replication Study. In: Hesselbach R, Henny-Kramer U, Calvo Tello J, et al (eds) Digital Stylistics in Romance Studies and Beyond. Heidelberg University Press, Heidelberg
Schöch, C., Schlör, D., Zehe, A., et al. (2018). Burrows’ Zeta: Exploring and Evaluating Variants and Parameters. In: Book of Abstracts of the Digital Humanities Conference. ADHO, Mexico City. https://dh2018.adho.org/burrows-zeta-exploring-and-evaluating-variants-and-parameters/
Schöch, C., van Dalen-Oskam, K., Jannidis, F., et al. (2020). Panel: Replication and Computational Literary Studies. In: Digital Humanities 2020: Book of Abstracts. ADHO, Ottawa. https://hcommons.org/deposits/item/hc:30439
Schöch, C., Patras, R., Erjavec, T., et al. (2021). Creating the European Literary Text Collection (ELTeC): Challenges and Perspectives. Modern Languages Open, 1 , 25. https://doi.org/10.3828/mlo.v0i0.364
Sinclair, S., Rockwell, G. (2015). Epistemologica. Tech. rep., Github.com. https://github.com/sgsinclair/epistemologica
Smith, P. W. H., & Aldridge, W. (2011). Improving Authorship Attribution: Optimizing Burrows’ Delta Method. Journal of Quantitative Linguistics, 18 (1), 63–88. https://doi.org/10.1080/09296174.2011.533591
Spitzer, L. (1931). Die klassische Dämpfung bei Racine (1928). Romanische Stil-und Literaturstudien I (pp. 135–268). Marburg: Elwert.
Spitzer, L. (1969). The muting effect of classical style in Racine. In R. Knight (Ed.), Racine (pp. 117–131). Modern Judgements: Aurora Publishers.
Chapter Google Scholar
Sprenger, J. (2019) Degree of Corroboration: An Antidote to the Replication Crisis. In: PhilSci Archive. http://philsci-archive.pitt.edu/16047/
Widdows, D. (2004). Geometry and Meaning . Stanford: CSLI Publications.
Williams, C. B. (1975). Mendenhall’s studies of word-length distribution in the works of Shakespeare and Bacon. Biometrika, 62 (1), 207–212. https://doi.org/10.1093/biomet/62.1.207
Download references
Acknowledgements
My thanks go to colleagues from the CLS and Distant Reading community who have provided invaluable feedback on some of the ideas proposed here on the occasion of several invited talks on the subject in Bern (2017), Montpellier (2019), Budapest (2019) and Munich (2020), in particular Katherine Bode, Fotis Jannidis, Melanie Andresen and one anonymous reviewer, who all helped me sharpen my argument. All errors remain my own. - The epigraph comes from (Widdows , 2004 , 2)
Open Access funding enabled and organized by Projekt DEAL. Part of this work was supported by the European Commission through the COST Action ‘Distant Reading for European Literary History’ (CA16204).
Author information
Authors and affiliations.
Trier Center for Digital Humanities & Department for Computational Linguistics and Digital Humanities, University of Trier, Am Universitätsring 15, Trier, 54296, Germany
Christof Schöch
You can also search for this author in PubMed Google Scholar
Contributions
(following the CRediT taxonomy): CS is responsible for Conceptualization, Data curation, Formal Analysis, Funding acquisition, Investigation, Methodology, Project administration, Resources, Software, Validation, Visualization, Writing - original draft, Writing - review & editing.
Corresponding author
Correspondence to Christof Schöch .
Ethics declarations
Competing interests, ethics approval.
Not applicable.
Consent to participate
Consent for publication, rights and permissions.
Open Access This article is licensed under a Creative Commons Attribution 4.0 International License, which permits use, sharing, adaptation, distribution and reproduction in any medium or format, as long as you give appropriate credit to the original author(s) and the source, provide a link to the Creative Commons licence, and indicate if changes were made. The images or other third party material in this article are included in the article’s Creative Commons licence, unless indicated otherwise in a credit line to the material. If material is not included in the article’s Creative Commons licence and your intended use is not permitted by statutory regulation or exceeds the permitted use, you will need to obtain permission directly from the copyright holder. To view a copy of this licence, visit http://creativecommons.org/licenses/by/4.0/ .
Reprints and permissions
About this article
Schöch, C. Repetitive research: a conceptual space and terminology of replication, reproduction, revision, reanalysis, reinvestigation and reuse in digital humanities. Int J Digit Humanities 5 , 373–403 (2023). https://doi.org/10.1007/s42803-023-00073-y
Download citation
Received : 05 March 2023
Accepted : 04 October 2023
Published : 06 November 2023
Issue Date : November 2023
DOI : https://doi.org/10.1007/s42803-023-00073-y
Share this article
Anyone you share the following link with will be able to read this content:
Sorry, a shareable link is not currently available for this article.
Provided by the Springer Nature SharedIt content-sharing initiative
- Replication
- Reproduction
- Terminology
- Computational Literary Studies
- Open Science
Advertisement
- Find a journal
- Publish with us
- Track your research
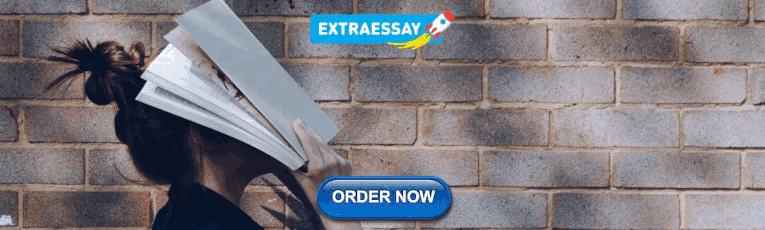
COMMENTS
Replicate 2. Evaluate, Research Methodology, -Subjects and Methods, -Research Methodology or simply Methods. and more. ... What are the Research Methodology section is written with two purposes in mind. Research Methodology. provide enough detail for a competent reader to replicate the study and reproduce the results.
Research Methodology refers to the systematic and scientific approach used to conduct research, investigate problems, and gather data and information for a specific purpose. It involves the techniques and procedures used to identify, collect, analyze, and interpret data to answer research questions or solve research problems.
Step 1: Explain your methodological approach. Step 2: Describe your data collection methods. Step 3: Describe your analysis method. Step 4: Evaluate and justify the methodological choices you made. Tips for writing a strong methodology chapter. Other interesting articles.
Definition, Types, and Examples. Research methodology 1,2 is a structured and scientific approach used to collect, analyze, and interpret quantitative or qualitative data to answer research questions or test hypotheses. A research methodology is like a plan for carrying out research and helps keep researchers on track by limiting the scope of ...
I. Groups of Research Methods. There are two main groups of research methods in the social sciences: The empirical-analytical group approaches the study of social sciences in a similar manner that researchers study the natural sciences.This type of research focuses on objective knowledge, research questions that can be answered yes or no, and operational definitions of variables to be measured.
Research methodology can be defined as the systematic framework that guides researchers in designing, conducting, and analyzing their investigations. It encompasses a structured set of processes, techniques, and tools employed to gather and interpret data, ensuring the reliability and validity of the research findings.
Definition: Evaluating Research refers to the process of assessing the quality, credibility, and relevance of a research study or project. This involves examining the methods, data, and results of the research in order to determine its validity, reliability, and usefulness. Evaluating research can be done by both experts and non-experts in the ...
Replication is a research methodology used to verify, consolidate, and advance knowledge and understanding within empirical fields of study. A replication study works toward this goal by repeating a study's methodology with or without changes followed by systematic comparison to better understand the nature, repeatability, and generalizability of its findings.
The purpose of a research methodology is to explain the reasoning behind your approach to your research - you'll need to support your collection methods, methods of analysis, and other key points of your work. Think of it like writing a plan or an outline for you what you intend to do. When carrying out research, it can be easy to go off-track ...
The research design is a fundamental aspect of research methodology, outlining the overall strategy and structure of the study. It includes decisions regarding the research type (e.g., descriptive, experimental), the selection of variables, and the determination of the study's scope and timeframe. We must carefully consider the design to ...
2. Provide the rationality behind your chosen approach. Based on logic and reason, let your readers know why you have chosen said research methodologies. Additionally, you have to build strong arguments supporting why your chosen research method is the best way to achieve the desired outcome. 3.
2.1 Research Methodology. Method can be described as a set of tools and techniques for finding something out, or for reducing levels of uncertainty. According to Saunders (2012) method is the technique and procedures used to obtain and analyse research data, including for example questionnaires, observation, interviews, and statistical and non-statistical techniques [].
and make for good science and scientific research. The purpose of this introductory chapter is to convey what methodology is, why it is needed, and the key tenets that guide what we do as scientists. These foci may seem obvious—after all, everyone knows what methodology is and why it is needed. Perhaps so, but the answers are not all so obvious.
In scientific research, a replication is commonly defined as a study that is conducted using the same or similar methods as the original investigation, in order to evaluate whether consistent results can be obtained [].Often carried out by researchers independent from the original investigators, replications are designed to assess the robustness and generalizability of the original findings [2,3].
In this tutorial paper, we will use the term methodological study to refer to any study that reports on the design, conduct, analysis or reporting of primary or secondary research-related reports (such as trial registry entries and conference abstracts). In the past 10 years, there has been an increase in the use of terms related to ...
Following the approximate replication paradigm, the study has the same purpose as the original study with a very similar design and will thus contribute to the self-correcting nature of scientific ...
1.1 purpose and significance of research methodology The p urpose and significance of research methodology lie in its role as the systematic and structured approach to conducting research studies.
Your Methods Section contextualizes the results of your study, giving editors, reviewers and readers alike the information they need to understand and interpret your work. Your methods are key to establishing the credibility of your study, along with your data and the results themselves. A complete methods section should provide enough detail for a skilled researcher to replicate your process ...
Conclusion: Choosing an optimal research methodology is crucial for the success of any research project. The methodology you select will determine the type of data you collect, how you collect it, and how you analyse it. Understanding the different types of research methods available along with their strengths and weaknesses, is thus imperative ...
About Research Methods. This guide provides an overview of research methods, how to choose and use them, and supports and resources at UC Berkeley. As Patten and Newhart note in the book Understanding Research Methods, "Research methods are the building blocks of the scientific enterprise. They are the "how" for building systematic knowledge.
Research is a rigorous problem-solving process whose ultimate goal is the discovery of new knowledge. Research may include the description of a new phenomenon, definition of a new relationship, development of a new model, or application of an existing principle or procedure to a new context. Research is systematic, logical, empirical, reductive, replicable and transmittable, and generalizable.
6.1 Replication (of research) Within the terminology proposed here, the term replication (of research) designates practices of RR in which the research question, the dataset and the method of analysis of the repeating study are all identical (or virtually identical) to the original study (1,1,1) (see Fig. 5). The term can be used irrespective ...
Research methodology is written with 2 purposes in mind: 1. Replicate 2. Evaluate In this part, the researcher writes each sub-section concisely yet completely to provide enough detail for a competent reader to replicate the study and reproduce the results. This section also ensures that the study has undergone scientific process and that the tools