Information
- Author Services
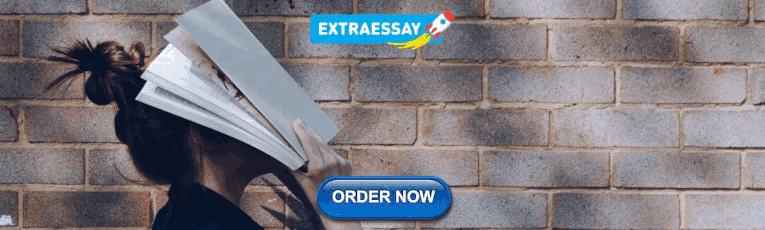
Initiatives
You are accessing a machine-readable page. In order to be human-readable, please install an RSS reader.
All articles published by MDPI are made immediately available worldwide under an open access license. No special permission is required to reuse all or part of the article published by MDPI, including figures and tables. For articles published under an open access Creative Common CC BY license, any part of the article may be reused without permission provided that the original article is clearly cited. For more information, please refer to https://www.mdpi.com/openaccess .
Feature papers represent the most advanced research with significant potential for high impact in the field. A Feature Paper should be a substantial original Article that involves several techniques or approaches, provides an outlook for future research directions and describes possible research applications.
Feature papers are submitted upon individual invitation or recommendation by the scientific editors and must receive positive feedback from the reviewers.
Editor’s Choice articles are based on recommendations by the scientific editors of MDPI journals from around the world. Editors select a small number of articles recently published in the journal that they believe will be particularly interesting to readers, or important in the respective research area. The aim is to provide a snapshot of some of the most exciting work published in the various research areas of the journal.
Original Submission Date Received: .
- Active Journals
- Find a Journal
- Proceedings Series
- For Authors
- For Reviewers
- For Editors
- For Librarians
- For Publishers
- For Societies
- For Conference Organizers
- Open Access Policy
- Institutional Open Access Program
- Special Issues Guidelines
- Editorial Process
- Research and Publication Ethics
- Article Processing Charges
- Testimonials
- Preprints.org
- SciProfiles
- Encyclopedia
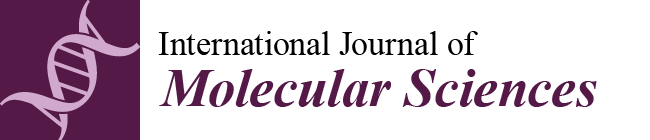
Article Menu
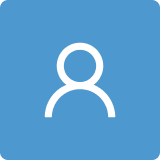
- Subscribe SciFeed
- Recommended Articles
- PubMed/Medline
- Google Scholar
- on Google Scholar
- Table of Contents
Find support for a specific problem in the support section of our website.
Please let us know what you think of our products and services.
Visit our dedicated information section to learn more about MDPI.
JSmol Viewer
Pathophysiology of atherosclerosis.
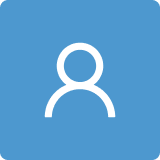
1. Introduction
2. endothelium, 3. atherosclerosis initiation and fatty streak formation, 3.1. endothelial dysfunction in atherosclerosis development, 3.1.1. hemodynamic forces and endothelial dysfunction, 3.1.2. the role of nitric oxide in endothelial dysfunction, 3.2. ldl infiltration, ldl modifications in the intima, 3.3. endothelial activation, 3.4. monocyte recruitment and foam cell formation, 3.5. contribution of vsmcs to foam cell population, 4. fibrous plaque development, 4.1. fibrous cap, 4.2. necrotic core, 4.3. plaque calcification, 5. plaque stability and rupture, 5.1. vulnerable plaque, 5.2. plaque rupture and thrombus formation, 5.3. clinical complications, 6. inflammation in atherosclerosis, 7. post-transcriptional regulation of atherosclerotic plaques, 7.1. mirnas, 7.1.1. mirnas in atherosclerotic plaque initiation and progression, 7.1.2. mirnas and atherosclerotic plaque rupture, 7.1.3. distinct mirna regulation of vsmc and ec function, 7.2. lncrnas, 8. microbiota, 9. sex as an important risk factor in atherosclerosis, 9.1. impact of plaque size and morphology between sexes, 9.2. clinical implications, 10. cigarette-smoking-induced atherosclerosis, molecular mechanisms underlying clinical smoking-induced atherosclerosis, 11. conclusions, author contributions, institutional review board statement, informed consent statement, conflicts of interest.
- Reitsma, S.; Slaaf, D.W.; Vink, H.; van Zandvoort, M.A.M.J.; Oude Egbrink, M.G. The endothelial glycocalyx: Composition, functions, and visualization. Pflug. Arch. Eur. J. Physiol. 2007 , 454 , 345–359. [ Google Scholar ] [ CrossRef ] [ PubMed ] [ Green Version ]
- Rhodin, J.A. Ultrastructure of mammalian venous capillaries, venules, and small collecting veins. J. Ultrastruct. Res. 1968 , 25 , 452–500. [ Google Scholar ] [ CrossRef ]
- Zhang, B.; Gu, J.; Qian, M.; Niu, L.; Zhou, H.; Ghista, D. Correlation between quantitative analysis of wall shear stress and intima-media thickness in atherosclerosis development in carotid arteries. Biomed. Eng. Online 2017 , 16 , 137. [ Google Scholar ] [ CrossRef ] [ Green Version ]
- Roger, V.L.; Weston, S.A.; Killian, J.M.; Pfeifer, E.A.; Belau, P.G.; Kottke, T.E.; Frye, R.L.; Bailey, K.R.; Jacobsen, S.J. Time trends in the prevalence of atherosclerosis: A population-based autopsy study. Am. J. Med. 2001 , 110 , 267–273. [ Google Scholar ] [ CrossRef ]
- Targonski, P. Referral to Autopsy: Effect of Antemortem Cardiovascular Disease A Population-Based Study in Olmsted County, Minnesota. Ann. Epidemiol. 2001 , 11 , 264–270. [ Google Scholar ] [ CrossRef ]
- Böyum, A. Isolation of mononuclear cells and granulocytes from human blood. Isolation of monuclear cells by one centrifugation, and of granulocytes by combining centrifugation and sedimentation at 1 g. Scand. J. Clin. Lab. Investig. Suppl. 1968 , 97 , 77–89. [ Google Scholar ]
- Hays, A.G.; Kelle, S.; Hirsch, G.A.; Soleimanifard, S.; Yu, J.; Agarwal, H.K.; Gerstenblith, G.; Schär, M.; Stuber, M.; Weiss, R.G. Regional Coronary Endothelial Function Is Closely Related to Local Early Coronary Atherosclerosis in Patients With Mild Coronary Artery Disease. Circ. Cardiovasc. Imaging 2012 , 5 , 341–348. [ Google Scholar ] [ CrossRef ] [ PubMed ] [ Green Version ]
- Senior, R.M.; Campbell, E.J.; Landis, J.A.; Cox, F.R.; Kuhn, C.; Koren, H.S. Elastase of U-937 Monocytelike Cells. J. Clin. Investig. 1982 , 69 , 384–393. [ Google Scholar ] [ CrossRef ] [ Green Version ]
- Favero, G.; Paganelli, C.; Buffoli, B.; Rodella, L.F.; Rezzani, R. Endothelium and Its Alterations in Cardiovascular Diseases: Life Style Intervention. BioMed Res. Int. 2014 , 2014 , 801896. [ Google Scholar ] [ CrossRef ] [ Green Version ]
- Campinho, P.; Vilfan, A.; Vermot, J. Blood Flow Forces in Shaping the Vascular System: A Focus on Endothelial Cell Behavior. Front. Physiol. 2020 , 11 , 552. [ Google Scholar ] [ CrossRef ]
- Davies, P.F. Flow-mediated endothelial mechanotransduction. Physiol. Rev. 1995 , 75 , 519–560. [ Google Scholar ] [ CrossRef ] [ PubMed ]
- Esper, R.J.; Nordaby, R.A.; Vilariño, J.O.; Paragano, A.; Cacharrón, J.L.; Machado, R.A. Endothelial Dysfunction: A Comprehensive Appraisal. Cardiovasc. Diabetol. 2006 , 18 , 1–18. [ Google Scholar ]
- Gimbrone, M.A., Jr.; García-Cardeña, G. Vascular Endothelium, Hemodynamics, and the Pathobiology of Atherosclerosis. Cardiovasc. Pathol. 2013 , 22 , 9–15. [ Google Scholar ] [ CrossRef ] [ PubMed ] [ Green Version ]
- Natural history of aortic and coronary atherosclerotic lesions in youth. Findings from the PDAY Study. Pathobiological Determinants of Atherosclerosis in Youth (PDAY) Research Group. Arter. Thromb. A J. Vasc. Biol. 1993 , 13 , 1291–1298. [ Google Scholar ] [ CrossRef ] [ Green Version ]
- Rubanyi, G.M. The Role of Endothelium in Cardiovascular Homeostasis and Diseases. J. Cardiovasc. Pharmacol. 1993 , 22 , S1–S14. [ Google Scholar ] [ CrossRef ]
- Vanhoutte, P.M. How to assess endothelial function in human blood vessels. J. Hypertens. 1999 , 17 , 1047–1058. [ Google Scholar ] [ CrossRef ]
- Hermida, N.; Balligand, J.-L. Low-Density Lipoprotein-Cholesterol-Induced Endothelial Dysfunction and Oxidative Stress: The Role of Statins. Antioxid. Redox Signal. 2014 , 20 , 1216–1237. [ Google Scholar ] [ CrossRef ]
- Mundi, S.; Massaro, M.; Scoditti, E.; Carluccio, M.A.; van Hinsbergh, V.W.M.; Iruela-Arispe, M.L.; De Caterina, R. Endothelial permeability, LDL deposition, and cardiovascular risk factors—A review. Cardiovasc. Res. 2018 , 114 , 35–52. [ Google Scholar ] [ CrossRef ]
- Yu, X.-H.; Fu, Y.-C.; Zhang, D.-W.; Yin, K.; Tang, C.-K. Foam cells in atherosclerosis. Clin. Chim. Acta 2013 , 424 , 245–252. [ Google Scholar ] [ CrossRef ] [ Green Version ]
- Allahverdian, S.; Chehroudi, A.C.; McManus, B.M.; Abraham, T.; Francis, G.A. Contribution of Intimal Smooth Muscle Cells to Cholesterol Accumulation and Macrophage-Like Cells in Human Atherosclerosis. Circulation 2014 , 129 , 1551–1559. [ Google Scholar ] [ CrossRef ] [ Green Version ]
- Stary, H.C.; Chandler, A.B.; Glagov, S.; Guyton, J.R.; Insull, W.; Rosenfeld, M.E.; Schaffer, S.A.; Schwartz, C.J.; Wagner, W.D.; Wissler, R.W. A definition of initial, fatty streak, and intermediate lesions of atherosclerosis. A report from the Committee on Vascular Lesions of the Council on Arteriosclerosis, American Heart Association. Circulation 1994 , 89 , 2462–2478. [ Google Scholar ] [ CrossRef ] [ PubMed ] [ Green Version ]
- Sun, H.-J.; Wu, Z.-Y.; Nie, X.-W.; Bian, J.-S. Role of Endothelial Dysfunction in Cardiovascular Diseases: The Link between Inflammation and Hydrogen Sulfide. Front. Pharmacol. 2020 , 10 , 1568. [ Google Scholar ] [ CrossRef ] [ PubMed ] [ Green Version ]
- Verma, S.; Anderson, T.J. Fundamentals of Endothelial Function for the Clinical Cardiologist. Circulation 2002 , 105 , 546–549. [ Google Scholar ] [ CrossRef ] [ PubMed ] [ Green Version ]
- Verma, S.; Buchanan, M.R.; Anderson, T.J. Endothelial Function Testing as a Biomarker of Vascular Disease. Circulation 2003 , 108 , 2054–2059. [ Google Scholar ] [ CrossRef ]
- Ardestani, S.B.; Eftedal, I.; Pedersen, M.; Jeppesen, P.B.; Nørregaard, R.; Matchkov, V.V. Endothelial dysfunction in small arteries and early signs of atherosclerosis in ApoE knockout rats. Sci. Rep. 2020 , 10 , 15296. [ Google Scholar ] [ CrossRef ]
- Mudau, M.; Genis, A.; Lochner, A.; Strijdom, H. Endothelial dysfunction: The early predictor of atherosclerosis. Cardiovasc. J. Afr. 2012 , 23 , 222–231. [ Google Scholar ] [ CrossRef ]
- Gordon, E.; Schimmel, L.; Frye, M. The Importance of Mechanical Forces for in vitro Endothelial Cell Biology. Front. Physiol. 2020 , 11 , 684. [ Google Scholar ] [ CrossRef ]
- Gimbrone, M.A., Jr.; García-Cardeña, G. Endothelial Cell Dysfunction and the Pathobiology of Atherosclerosis. Circ. Res. 2016 , 118 , 620–636. [ Google Scholar ] [ CrossRef ] [ Green Version ]
- Chiu, J.-J.; Chien, S. Effects of Disturbed Flow on Vascular Endothelium: Pathophysiological Basis and Clinical Perspectives. Physiol. Rev. 2011 , 91 , 327–387. [ Google Scholar ] [ CrossRef ] [ Green Version ]
- Ross, R.; Glomset, J.A. The Pathogenesis of Atherosclerosis. N. Engl. J. Med. 1976 , 295 , 369–377. [ Google Scholar ] [ CrossRef ]
- Chistiakov, D.A.; Orekhov, A.N.; Bobryshev, Y.V. Effects of shear stress on endothelial cells: Go with the flow. Acta Physiol. 2017 , 219 , 382–408. [ Google Scholar ] [ CrossRef ] [ PubMed ]
- Kang, H.; Cancel, L.M.; Tarbell, J.M. Effect of shear stress on water and LDL transport through cultured endothelial cell monolayers. Atherosclerosis 2014 , 233 , 682–690. [ Google Scholar ] [ CrossRef ] [ PubMed ] [ Green Version ]
- Caro, C.G.; Fitz-Gerald, J.M.; Schroter, R.C. Atheroma and arterial wall shear—Observation, correlation and proposal of a shear dependent mass transfer mechanism for atherogenesis. Proc. R. Soc. London. Ser. B Boil. Sci. 1971 , 177 , 109–133. [ Google Scholar ] [ CrossRef ]
- Zhou, J.; Li, Y.-S.; Chien, S. Shear Stress–Initiated Signaling and Its Regulation of Endothelial Function. Arterioscler. Thromb. Vasc. Biol. 2014 , 34 , 2191–2198. [ Google Scholar ] [ CrossRef ] [ PubMed ] [ Green Version ]
- Baeyens, N.; Bandyopadhyay, C.; Coon, B.G.; Yun, S.; Schwartz, M.A. Endothelial fluid shear stress sensing in vascular health and disease. J. Clin. Investig. 2016 , 126 , 821–828. [ Google Scholar ] [ CrossRef ]
- Souilhol, C.; Serbanovic-Canic, J.; Fragiadaki, M.; Chico, T.J.; Ridger, V.; Roddie, H.; Evans, P.C. Endothelial responses to shear stress in atherosclerosis: A novel role for developmental genes. Nat. Rev. Cardiol. 2020 , 17 , 52–63. [ Google Scholar ] [ CrossRef ]
- Chien, S.; Shyy, J.Y.J. Effects of hemodynamic forces on gene expression and signal transduction in endothelial cells. Biol. Bull. 1998 , 194 , 390–393. [ Google Scholar ] [ CrossRef ]
- Gimbrone, M.A.; Topper, J.N.; Nagel, T.; Anderson, K.R.; Garcia-Cardeña, G. Endothelial Dysfunction, Hemodynamic Forces, and Atherogenesisa. Ann. N. Y. Acad. Sci. 2006 , 902 , 230–240. [ Google Scholar ] [ CrossRef ]
- Resnick, N.; Yahav, H.; Shay-Salit, A.; Shushy, M.; Schubert, S.; Zilberman, L.C.M.; Wofovitz, E. Fluid shear stress and the vascular endothelium: For better and for worse. Prog. Biophys. Mol. Biol. 2003 , 81 , 177–199. [ Google Scholar ] [ CrossRef ]
- Chiu, J.-J.; Usami, S.; Chien, S. Vascular endothelial responses to altered shear stress: Pathologic implications for atherosclerosis. Ann. Med. 2009 , 41 , 19–28. [ Google Scholar ] [ CrossRef ]
- Chien, S. Role of shear stress direction in endothelial mechanotransduction. Mol. Cell. Biomech. 2008 , 5 , 1–8. [ Google Scholar ] [ PubMed ]
- García-Cardeña, G.; Gimbrone, M.A. Biomechanical Modulation of Endothelial Phenotype: Implications for Health and Disease. In The Vascular Endothelium II ; Moncada, S., Higgs, A., Eds.; Handbook of Experimental Pharmacology; Springer: Berlin/Heidelberg, Germany, 2006; Volume 176/II, pp. 79–95. ISBN 978-3-540-36027-8. [ Google Scholar ]
- Shyy, J.Y.; Lin, M.C.; Han, J.; Lu, Y.; Petrime, M.; Chien, S. The cis-acting phorbol ester “12-O-tetradecanoylphorbol 13-acetate”-responsive element is involved in shear stress-induced monocyte chemotactic protein 1 gene expression. Proc. Natl. Acad. Sci. USA 1995 , 92 , 8069–8073. [ Google Scholar ] [ CrossRef ] [ PubMed ] [ Green Version ]
- Hsiai, T.K.; Cho, S.K.; Reddy, S.; Hama, S.; Navab, M.; Demer, L.; Honda, H.M.; Ho, C.-M. Pulsatile Flow Regulates Monocyte Adhesion to Oxidized Lipid-Induced Endothelial Cells. Arterioscler. Thromb. Vasc. Biol. 2001 , 21 , 1770–1776. [ Google Scholar ] [ CrossRef ] [ PubMed ] [ Green Version ]
- Hsiai, T.K.; Cho, S.K.; Wong, P.K.; Ing, M.; Salazar, A.; Sevanian, A.; Navab, M.; Demer, L.; Ho, C.-M. Monocyte recruitment to endothelial cells in response to oscillatory shear stress. FASEB J. 2003 , 17 , 1648–1657. [ Google Scholar ] [ CrossRef ] [ Green Version ]
- Kraiss, L.W.; Geary, R.L.; Mattsson, E.J.; Vergel, S.; Au, Y.T.; Clowes, A.W. Acute Reductions in Blood Flow and Shear Stress Induce Platelet-Derived Growth Factor-A Expression in Baboon Prosthetic Grafts. Circ. Res. 1996 , 79 , 45–53. [ Google Scholar ] [ CrossRef ]
- Malek, A.M.; Gibbons, G.H.; Dzau, V.J.; Izumo, S. Fluid shear stress differentially modulates expression of genes encoding basic fibroblast growth factor and platelet-derived growth factor B chain in vascular endothelium. J. Clin. Investig. 1993 , 92 , 2013–2021. [ Google Scholar ] [ CrossRef ] [ Green Version ]
- Wilcox, J.N.; Smith, K.M.; Williams, L.T.; Schwartz, S.M.; Gordon, D. Platelet-derived growth factor mRNA detection in human atherosclerotic plaques by in situ hybridization. J. Clin. Investig. 1988 , 82 , 1134–1143. [ Google Scholar ] [ CrossRef ] [ Green Version ]
- Silberman, M.; Barac, Y.D.; Yahav, H.; Wolfovitz, E.; Einav, S.; Resnick, N.; Binah, O. Shear stress-induced transcriptional regulation via hybrid promoters as a potential tool for promoting angiogenesis. Angiogenesis 2009 , 12 , 231–242. [ Google Scholar ] [ CrossRef ]
- Davis, M.E.; Grumbach, I.; Fukai, T.; Cutchins, A.; Harrison, D.G. Shear Stress Regulates Endothelial Nitric-oxide Synthase Promoter Activity through Nuclear Factor κB Binding. J. Biol. Chem. 2004 , 279 , 163–168. [ Google Scholar ] [ CrossRef ] [ Green Version ]
- Drummond, G.; Cai, H.; Davis, M.E.; Ramasamy, S.; Harrison, D.G. Transcriptional and Posttranscriptional Regulation of Endothelial Nitric Oxide Synthase Expression by Hydrogen Peroxide. Circ. Res. 2000 , 86 , 347–354. [ Google Scholar ] [ CrossRef ]
- Almendro, N.; Bellón, T.; Rius, C.; Lastres, P.; Langa, C.; Corbí, A.; Bernabeu, C. Cloning of the human platelet endothelial cell adhesion molecule-1 promoter and its tissue-specific expression. Structural and functional characterization. J. Immunol. 1996 , 157 , 5411–5421. [ Google Scholar ] [ PubMed ]
- Houston, P.; White, B.P.; Campbell, C.J.; Braddock, M. Delivery and Expression of Fluid Shear Stress-Inducible Promoters to the Vessel Wall: Applications for Cardiovascular Gene Therapy. Hum. Gene Ther. 1999 , 10 , 3031–3044. [ Google Scholar ] [ CrossRef ] [ PubMed ]
- Landmesser, U.; Hornig, B.; Drexler, H. Endothelial Dysfunction in Hypercholesterolemia: Mechanisms, Pathophysiological Importance, and Therapeutic Interventions. Semin. Thromb. Hemost. 2000 , 26 , 529–538. [ Google Scholar ] [ CrossRef ] [ PubMed ]
- Archer, S.L.; Huang, J.M.; Hampl, V.; Nelson, D.P.; Shultz, P.J.; Weir, E.K. Nitric oxide and cGMP cause vasorelaxation by activation of a charybdotoxin-sensitive K channel by cGMP-dependent protein kinase. Proc. Natl. Acad. Sci. USA 1994 , 91 , 7583–7587. [ Google Scholar ] [ CrossRef ] [ Green Version ]
- Aggio, A.; Grassi, D.; Onori, E.; D’Alessandro, A.; Masedu, F.; Valenti, M.; Ferri, C. Endothelium/nitric oxide mechanism mediates vasorelaxation and counteracts vasoconstriction induced by low concentration of flavanols. Eur. J. Nutr. 2012 , 52 , 263–272. [ Google Scholar ] [ CrossRef ]
- Cooke, J.P.; Tsao, P.S. Is NO an endogenous antiatherogenic molecule? Arter. Thromb. A J. Vasc. Biol. 1994 , 14 , 653–655. [ Google Scholar ] [ CrossRef ] [ Green Version ]
- Libby, P. Current Concepts of the Pathogenesis of the Acute Coronary Syndromes. Circulation 2001 , 104 , 365–372. [ Google Scholar ] [ CrossRef ] [ Green Version ]
- Marx, N.; Sukhova, G.K.; Collins, T.; Libby, P.; Plutzky, J. PPARα Activators Inhibit Cytokine-Induced Vascular Cell Adhesion Molecule-1 Expression in Human Endothelial Cells. Circulation 1999 , 99 , 3125–3131. [ Google Scholar ] [ CrossRef ] [ Green Version ]
- Chen, J.-Y.; Ye, Z.-X.; Wang, X.-F.; Chang, J.; Yang, M.-W.; Zhong, H.; Hong, F.-F.; Yang, S.-L. Nitric oxide bioavailability dysfunction involves in atherosclerosis. Biomed. Pharmacother. 2018 , 97 , 423–428. [ Google Scholar ] [ CrossRef ]
- Carlström, M. Nitric oxide signalling in kidney regulation and cardiometabolic health. Nat. Rev. Nephrol. 2021 , 17 , 575–590. [ Google Scholar ] [ CrossRef ]
- Schulz, E.; Gori, T.; Münzel, T. Oxidative stress and endothelial dysfunction in hypertension. Hypertens. Res. 2011 , 34 , 665–673. [ Google Scholar ] [ CrossRef ] [ PubMed ]
- Förstermann, U.; Xia, N.; Li, H. Roles of Vascular Oxidative Stress and Nitric Oxide in the Pathogenesis of Atherosclerosis. Circ. Res. 2017 , 120 , 713–735. [ Google Scholar ] [ CrossRef ] [ PubMed ]
- Casino, P.R.; Kilcoyne, C.M.; A Quyyumi, A.; Hoeg, J.M.; A Panza, J. The role of nitric oxide in endothelium-dependent vasodilation of hypercholesterolemic patients. Circulation 1993 , 88 , 2541–2547. [ Google Scholar ] [ CrossRef ] [ PubMed ] [ Green Version ]
- A Panza, J.; Casino, P.R.; Kilcoyne, C.M.; A Quyyumi, A. Role of endothelium-derived nitric oxide in the abnormal endothelium-dependent vascular relaxation of patients with essential hypertension. Circulation 1993 , 87 , 1468–1474. [ Google Scholar ] [ CrossRef ] [ PubMed ] [ Green Version ]
- Alexander, R.W. Hypertension and the Pathogenesis of Atherosclerosis. Hypertension 1995 , 25 , 155–161. [ Google Scholar ] [ CrossRef ] [ PubMed ]
- Michel, C.C.; Curry, F.E. Microvascular Permeability. Physiol. Rev. 1999 , 79 , 703–761. [ Google Scholar ] [ CrossRef ]
- Rippe, B.; Haraldsson, B. Transport of macromolecules across microvascular walls: The two-pore theory. Physiol. Rev. 1994 , 74 , 163–219. [ Google Scholar ] [ CrossRef ] [ PubMed ]
- Rippe, B.; Rosengren, B.-I.; Carlsson, O.; Venturoli, D. Transendothelial Transport: The Vesicle Controversy. J. Vasc. Res. 2002 , 39 , 375–390. [ Google Scholar ] [ CrossRef ]
- Pappenheimer, J.R.; Renkin, E.M.; Borrero, L.M. Filtration, Diffusion and Molecular Sieving Through Peripheral Capillary Membranes. Am. J. Physiol. Content 1951 , 167 , 13–46. [ Google Scholar ] [ CrossRef ]
- Jang, E.; Robert, J.; Rohrer, L.; von Eckardstein, A.; Lee, W.L. Transendothelial transport of lipoproteins. Atheroscler. 2020 , 315 , 111–125. [ Google Scholar ] [ CrossRef ]
- Zhang, X.; Sessa, W.C.; Fernández-Hernando, C. Endothelial Transcytosis of Lipoproteins in Atherosclerosis. Front. Cardiovasc. Med. 2018 , 5 , 130. [ Google Scholar ] [ CrossRef ] [ PubMed ]
- Goldstein, J.L.; Brown, M.S. The LDL Receptor. Arterioscler. Thromb. Vasc. Biol. 2009 , 29 , 431–438. [ Google Scholar ] [ CrossRef ] [ Green Version ]
- Babitt, J.; Trigatti, B.; Rigotti, A.; Smart, E.J.; Anderson, R.G.W.; Xu, S.; Krieger, M. Murine SR-BI, a High Density Lipoprotein Receptor That Mediates Selective Lipid Uptake, Is N-Glycosylated and Fatty Acylated and Colocalizes with Plasma Membrane Caveolae. J. Biol. Chem. 1997 , 272 , 13242–13249. [ Google Scholar ] [ CrossRef ] [ PubMed ] [ Green Version ]
- Santibanez, J.F.; Blanco, F.J.; Garrido-Martin, E.M.; Sanz-Rodriguez, F.; del Pozo, M.A.; Bernabeu, C. Caveolin-1 interacts and cooperates with the transforming growth factor-β type I receptor ALK1 in endothelial caveolae. Cardiovasc. Res. 2008 , 77 , 791–799. [ Google Scholar ] [ CrossRef ] [ PubMed ] [ Green Version ]
- Rothberg, K.G.; Heuser, J.E.; Donzell, W.C.; Ying, Y.-S.; Glenney, J.R.; Anderson, R.G. Caveolin, a protein component of caveolae membrane coats. Cell 1992 , 68 , 673–682. [ Google Scholar ] [ CrossRef ]
- Fernández-Hernando, C.; Yu, J.; Suárez, Y.; Rahner, C.; Dávalos, A.; Lasunción, M.A.; Sessa, W.C. Genetic Evidence Supporting a Critical Role of Endothelial Caveolin-1 during the Progression of Atherosclerosis. Cell Metab. 2009 , 10 , 48–54. [ Google Scholar ] [ CrossRef ] [ Green Version ]
- Frank, P.G.; Pavlides, S.; Lisanti, M.P. Caveolae and transcytosis in endothelial cells: Role in atherosclerosis. Cell Tissue Res. 2009 , 335 , 41–47. [ Google Scholar ] [ CrossRef ]
- Wang, D.-X.; Pan, Y.-Q.; Liu, B.; Dai, L. Cav-1 promotes atherosclerosis by activating JNK-associated signaling. Biochem. Biophys. Res. Commun. 2018 , 503 , 513–520. [ Google Scholar ] [ CrossRef ]
- Ramírez, C.M.; Zhang, X.; Bandyopadhyay, C.; Rotllan, N.; Sugiyama, M.G.; Aryal, B.; Liu, X.; He, S.; Kraehling, J.R.; Ulrich, V.; et al. Caveolin-1 Regulates Atherogenesis by Attenuating Low-Density Lipoprotein Transcytosis and Vascular Inflammation Independently of Endothelial Nitric Oxide Synthase Activation. Circulation 2019 , 140 , 225–239. [ Google Scholar ] [ CrossRef ]
- Zhang, X.; Fernández-Hernando, C. Transport of LDLs into the arterial wall: Impact in atherosclerosis. Curr. Opin. Lipidol. 2020 , 31 , 279–285. [ Google Scholar ] [ CrossRef ]
- Yang, Y.; Schmidt, E.P. The endothelial glycocalyx. Tissue Barriers 2013 , 1 , e23494. [ Google Scholar ] [ CrossRef ] [ PubMed ] [ Green Version ]
- Ziegler, T.; Abdel Rahman, F.; Jurisch, V.; Kupatt, C. Atherosclerosis and the Capillary Network; Pathophysiology and Potential Therapeutic Strategies. Cells 2019 , 9 , 50. [ Google Scholar ] [ CrossRef ] [ PubMed ] [ Green Version ]
- Skålén, K.; Gustafsson, M.; Rydberg, E.K.; Hultén, L.M.; Wiklund, O.; Innerarity, T.L.; Borén, J. Subendothelial retention of atherogenic lipoproteins in early atherosclerosis. Nature 2002 , 417 , 750–754. [ Google Scholar ] [ CrossRef ] [ PubMed ]
- Khalil, M.F.; Wagner, W.D.; Goldberg, I.J. Molecular Interactions Leading to Lipoprotein Retention and the Initiation of Atherosclerosis. Arterioscler. Thromb. Vasc. Biol. 2004 , 24 , 2211–2218. [ Google Scholar ] [ CrossRef ]
- Carmena, R.; Ascaso, J.F.; Camejo, G.; Varela, G.; Hurt-Camejo, E.; Ordovas, J.; Martinez-Valls, J.; Bergstöm, M.; Wallin, B. Effect of olive and sunflower oils on low density lipoprotein level, composition, size, oxidation and interaction with arterial proteoglycans. Atherosclerosis 1996 , 125 , 243–255. [ Google Scholar ] [ CrossRef ]
- Levitan, I.; Volkov, S.; Subbaiah, P.V. Oxidized LDL: Diversity, Patterns of Recognition, and Pathophysiology. Antioxid. Redox Signal. 2010 , 13 , 39–75. [ Google Scholar ] [ CrossRef ] [ Green Version ]
- Sheedy, F.J.; Grebe, A.; Rayner, K.J.; Kalantari, P.; Ramkhelawon, B.; Carpenter, S.B.; E Becker, C.; Ediriweera, H.N.; E Mullick, A.; Golenbock, D.T.; et al. CD36 coordinates NLRP3 inflammasome activation by facilitating intracellular nucleation of soluble ligands into particulate ligands in sterile inflammation. Nat. Immunol. 2013 , 14 , 812–820. [ Google Scholar ] [ CrossRef ] [ Green Version ]
- Witztum, J.L.; Steinberg, D. The Oxidative Modification Hypothesis of Atherosclerosis Does It Hold for Humans? Trends Cardiovasc. Med. 2001 , 11 , 93–102. [ Google Scholar ] [ CrossRef ]
- Steinbrecher, U.P.; Zhang, H.; Lougheed, M. Role of oxidatively modified LDL in atherosclerosis. Free Radic. Biol. Med. 1990 , 9 , 155–168. [ Google Scholar ] [ CrossRef ]
- Carr, A.C.; McCall, M.R.; Frei, B. Oxidation of LDL by Myeloperoxidase and Reactive Nitrogen Species. Arterioscler. Thromb. Vasc. Biol. 2000 , 20 , 1716–1723. [ Google Scholar ] [ CrossRef ] [ Green Version ]
- Pitanga, T.N.; França, L.D.A.; Rocha, V.C.J.; Meirelles, T.; Borges, V.M.; Gonçalves, M.S.; Pontes-De-Carvalho, L.C.; Noronha-Dutra, A.A.; Dos-Santos, W.L.C. Neutrophil-derived microparticles induce myeloperoxidase-mediated damage of vascular endothelial cells. BMC Cell Biol. 2014 , 15 , 21. [ Google Scholar ] [ CrossRef ] [ Green Version ]
- Nakajima, H.; Ikeda, M.; Hirata, Y.; Matsumoto, M.; Seike, M.; Kodama, H. Low-density lipoprotein is oxidized by phospholipase A 2 and lipoxygenase in xanthoma lesions. Eur. J. Lipid Sci. Technol. 2007 , 109 , 1053–1059. [ Google Scholar ] [ CrossRef ]
- Takahashi, Y.; Zhu, H.; Yoshimoto, T. Essential Roles of Lipoxygenases in LDL Oxidation and Development of Atherosclerosis. Antioxid. Redox Signal. 2005 , 7 , 425–431. [ Google Scholar ] [ CrossRef ]
- Parthasarathy, S.; Wieland, E.; Steinberg, D. A role for endothelial cell lipoxygenase in the oxidative modification of low density lipoprotein. Proc. Natl. Acad. Sci. USA 1989 , 86 , 1046–1050. [ Google Scholar ] [ CrossRef ] [ PubMed ] [ Green Version ]
- Esterbauer, H.; Jürgens, G.; Quehenberger, O.; Koller, E. Autoxidation of human low density lipoprotein: Loss of polyunsaturated fatty acids and vitamin E and generation of aldehydes. J. Lipid Res. 1987 , 28 , 495–509. [ Google Scholar ] [ CrossRef ]
- Jialal, I.; Norkus, E.P.; Cristol, L.; Grundy, S.M. β-Carotene inhibits the oxidative modification of low-density lipoprotein. Biochim. Et Biophys. Acta (BBA)-Lipids Lipid Metab. 1991 , 1086 , 134–138. [ Google Scholar ] [ CrossRef ]
- Esterbauer, H.; Waeg, G.; Puhl, H.; Dieber-Rotheneder, M.; Tatzber, F. Inhibition of LDL oxidation by antioxidants. Free Radic. Aging 1992 , 62 , 145–157. [ Google Scholar ] [ CrossRef ]
- Heinecke, J.W. Mechanisms of oxidative damage of low density lipoprotein in human atherosclerosis. Curr. Opin. Lipidol. 1997 , 8 , 268–274. [ Google Scholar ] [ CrossRef ]
- Parthasarathy, S.; Raghavamenon, A.; Garelnabi, M.O.; Santanam, N. Oxidized Low-Density Lipoprotein. In Free Radicals and Antioxidant Protocols ; Uppu, R.M., Murthy, S.N., Pryor, W.A., Parinandi, N.L., Eds.; Methods in Molecular Biology; Humana Press: Totowa, NJ, USA, 2010; Volume 610, pp. 403–417. ISBN 978-1-58829-710-5. [ Google Scholar ]
- Berliner, J.A.; Navab, M.; Fogelman, A.M.; Frank, J.S.; Demer, L.; Edwards, P.A.; Watson, A.D.; Lusis, A.J. Atherosclerosis: Basic Mechanisms. Circulation 1995 , 91 , 2488–2496. [ Google Scholar ] [ CrossRef ]
- Park, J.S.; Bae, S.H. Interplay between Saturated Free Fatty Acids and mmLDL Induces Inflammation in LPS-stimulated Macrophages. Korean Circ. J. 2021 , 51 , 81–82. [ Google Scholar ] [ CrossRef ]
- Ann, S.-J.; Kim, K.-K.; Cheon, E.J.; Noh, H.-M.; Hwang, I.; Yu, J.-W.; Park, S.; Kang, S.-M.; Manabe, I.; Miller, Y.I.; et al. Palmitate and minimally-modified low-density lipoprotein cooperatively promote inflammatory responses in macrophages. PLoS ONE 2018 , 13 , e0193649. [ Google Scholar ] [ CrossRef ] [ PubMed ] [ Green Version ]
- Kraaijenhof, J.M.; Hovingh, G.K.; Stroes, E.S.; Kroon, J. The iterative lipid impact on inflammation in atherosclerosis. Curr. Opin. Lipidol. 2021 , 32 , 286–292. [ Google Scholar ] [ CrossRef ] [ PubMed ]
- Chistiakov, D.A.; Bobryshev, Y.V.; Orekhov, A.N. Macrophage-mediated cholesterol handling in atherosclerosis. J. Cell. Mol. Med. 2016 , 20 , 17–28. [ Google Scholar ] [ CrossRef ] [ Green Version ]
- Moore, K.J.; Freeman, M.W. Scavenger Receptors in Atherosclerosis. Arterioscler. Thromb. Vasc. Biol. 2006 , 26 , 1702–1711. [ Google Scholar ] [ CrossRef ] [ PubMed ] [ Green Version ]
- Younis, N.; Sharma, R.; Soran, H.; Charlton-Menys, V.; Elseweidy, M.; Durrington, P.N. Glycation as an atherogenic modification of LDL. Curr. Opin. Lipidol. 2008 , 19 , 378–384. [ Google Scholar ] [ CrossRef ]
- Greaves, D.R.; Gordon, S. The macrophage scavenger receptor at 30 years of age: Current knowledge and future challenges. J. Lipid Res. 2009 , 50 , S282–S286. [ Google Scholar ] [ CrossRef ] [ Green Version ]
- Öörni, K.; Pentikäinen, M.O.; Annila, A.; Kovanen, P.T. Oxidation of Low Density Lipoprotein Particles Decreases Their Ability to Bind to Human Aortic Proteoglycans. J. Biol. Chem. 1997 , 272 , 21303–21311. [ Google Scholar ] [ CrossRef ] [ Green Version ]
- Ling, W.; Lougheed, M.; Suzuki, H.; Buchan, A.; Kodama, T.; Steinbrecher, U.P. Oxidized or acetylated low density lipoproteins are rapidly cleared by the liver in mice with disruption of the scavenger receptor class A type I/II gene. J. Clin. Investig. 1997 , 100 , 244–252. [ Google Scholar ] [ CrossRef ] [ Green Version ]
- Lu, M.; Gursky, O. Aggregation and fusion of low-density lipoproteins in vivo and in vitro. Biomol. Concepts 2013 , 4 , 501–518. [ Google Scholar ] [ CrossRef ] [ Green Version ]
- Libby, P. The changing landscape of atherosclerosis. Nature 2021 , 592 , 524–533. [ Google Scholar ] [ CrossRef ]
- van den Boogert, M.A.; Larsen, L.E.; Ali, L.; Kuil, S.D.; Chong, P.L.; Loregger, A.; Kroon, J.; Schnitzler, J.G.; Schimmel, A.W.; Peter, J.; et al. N-Glycosylation Defects in Humans Lower Low-Density Lipoprotein Cholesterol Through Increased Low-Density Lipoprotein Receptor Expression. Circulation 2019 , 140 , 280–292. [ Google Scholar ] [ CrossRef ] [ PubMed ]
- Liao, J.K. Linking endothelial dysfunction with endothelial cell activation. J. Clin. Investig. 2013 , 123 , 540–541. [ Google Scholar ] [ CrossRef ] [ PubMed ]
- Pober, J.S.; Sessa, W.C. Evolving functions of endothelial cells in inflammation. Nat. Rev. Immunol. 2007 , 7 , 803–815. [ Google Scholar ] [ CrossRef ] [ PubMed ]
- Yu, X.-H.; Zheng, X.-L.; Tang, C.-K. Nuclear Factor-ΚB Activation as a Pathological Mechanism of Lipid Metabolism and Atherosclerosis. In Advances in Clinical Chemistry ; Elsevier: Amsterdam, The Netherlands, 2015; Volume 70, pp. 1–30. ISBN 978-0-12-803316-6. [ Google Scholar ]
- Liu, T.; Zhang, L.; Joo, D.; Sun, S.-C. NF-κB signaling in inflammation. Signal Transduct. Target. Ther. 2017 , 2 , 17023. [ Google Scholar ] [ CrossRef ] [ Green Version ]
- Ropraz, P.; Imhof, B.A.; Matthes, T.; Wehrle-Haller, B.; Sidibé, A. Simultaneous Study of the Recruitment of Monocyte Subpopulations Under Flow In Vitro. J. Vis. Exp. 2018 , 58509 , e58509. [ Google Scholar ] [ CrossRef ] [ PubMed ]
- Gerrity, R.G. The role of the monocyte in atherogenesis: I. Transition of blood-borne monocytes into foam cells in fatty lesions. Am. J. Pathol. 1981 , 103 , 181–190. [ Google Scholar ]
- Gerhardt, T.; Ley, K. Monocyte trafficking across the vessel wall. Cardiovasc. Res. 2015 , 107 , 321–330. [ Google Scholar ] [ CrossRef ] [ PubMed ] [ Green Version ]
- Teh, Y.C.; Ding, J.L.; Ng, L.G.; Chong, S.Z. Capturing the Fantastic Voyage of Monocytes through Time and Space. Front. Immunol. 2019 , 10 , 834. [ Google Scholar ] [ CrossRef ]
- Ramos, C.L.; Huo, Y.; Jung, U.; Ghosh, S.; Manka, D.R.; Sarembock, I.J.; Ley, K. Direct Demonstration of P-Selectin–and VCAM-1–Dependent Mononuclear Cell Rolling in Early Atherosclerotic Lesions of Apolipoprotein E–Deficient Mice. Circ. Res. 1999 , 84 , 1237–1244. [ Google Scholar ] [ CrossRef ] [ Green Version ]
- Ley, K.; Laudanna, C.; Cybulsky, M.I.; Nourshargh, S. Getting to the site of inflammation: The leukocyte adhesion cascade updated. Nat. Rev. Immunol. 2007 , 7 , 678–689. [ Google Scholar ] [ CrossRef ]
- Galkina, E.; Ley, K. Immune and Inflammatory Mechanisms of Atherosclerosis. Annu. Rev. Immunol. 2009 , 27 , 165–197. [ Google Scholar ] [ CrossRef ] [ PubMed ] [ Green Version ]
- Campbell, J.; Qin, S.; Bacon, K.B.; Mackay, C.; Butcher, E.C. Biology of chemokine and classical chemoattractant receptors: Differential requirements for adhesion-triggering versus chemotactic responses in lymphoid cells. J. Cell Biol. 1996 , 134 , 255–266. [ Google Scholar ] [ CrossRef ] [ PubMed ] [ Green Version ]
- Campbell, J.J.; Hedrick, J.; Zlotnik, A.; Siani, M.A.; Thompson, D.A.; Butcher, E.C. Chemokines and the Arrest of Lymphocytes Rolling Under Flow Conditions. Science 1998 , 279 , 381–384. [ Google Scholar ] [ CrossRef ]
- Ley, K.; Miller, Y.I.; Hedrick, C.C. Monocyte and Macrophage Dynamics during Atherogenesis. Arterioscler. Thromb. Vasc. Biol. 2011 , 31 , 1506–1516. [ Google Scholar ] [ CrossRef ] [ PubMed ] [ Green Version ]
- Springer, T.A. Traffic signals for lymphocyte recirculation and leukocyte emigration: The multistep paradigm. Cell 1994 , 76 , 301–314. [ Google Scholar ] [ CrossRef ]
- Sozzani, S.; Molino, M.; Locati, M.; Luini, W.; Cerletti, C.; Vecchi, A.; Mantovani, A. Receptor-activated calcium influx in human monocytes exposed to monocyte chemotactic protein-1 and related cytokines. J. Immunol. 1993 , 150 , 1544–1553. [ Google Scholar ] [ PubMed ]
- Uguccioni, M.; D’Apuzzo, M.; Loetscher, M.; Dewald, B.; Baggiolini, M. Actions of the chemotactic cytokines MCP-1, MCP-2, MCP-3, RANTES, MIP-1α and MIP-1β on human monocytes. Eur. J. Immunol. 1995 , 25 , 64–68. [ Google Scholar ] [ CrossRef ]
- Kakkar, V.; Lu, X. Impact of MCP -1 in Atherosclerosis. Curr. Pharm. Des. 2014 , 20 , 4580–4588. [ Google Scholar ] [ CrossRef ]
- Nourshargh, S.; Alon, R. Leukocyte Migration into Inflamed Tissues. Immunity 2014 , 41 , 694–707. [ Google Scholar ] [ CrossRef ] [ Green Version ]
- Allport, J.R.; Muller, W.A.; Luscinskas, F.W. Monocytes Induce Reversible Focal Changes in Vascular Endothelial Cadherin Complex during Transendothelial Migration under Flow. J. Cell Biol. 2000 , 148 , 203–216. [ Google Scholar ] [ CrossRef ] [ Green Version ]
- Shaw, S.K.; Bamba, P.S.; Perkins, B.N.; Luscinskas, F.W. Real-Time Imaging of Vascular Endothelial-Cadherin during Leukocyte Transmigration Across Endothelium. J. Immunol. 2001 , 167 , 2323–2330. [ Google Scholar ] [ CrossRef ] [ PubMed ] [ Green Version ]
- Vestweber, D. Regulation of endothelial cell contacts during leukocyte extravasation. Curr. Opin. Cell Biol. 2002 , 14 , 587–593. [ Google Scholar ] [ CrossRef ]
- Muller, W.A. Leukocyte–endothelial-cell interactions in leukocyte transmigration and the inflammatory response. Trends Immunol. 2003 , 24 , 327–334. [ Google Scholar ] [ CrossRef ]
- Carman, C.V.; Springer, T.A. A transmigratory cup in leukocyte diapedesis both through individual vascular endothelial cells and between them. J. Cell Biol. 2004 , 167 , 377–388. [ Google Scholar ] [ CrossRef ] [ PubMed ] [ Green Version ]
- Carman, C.V.; Sage, P.T.; Sciuto, T.E.; de la Fuente, M.; Geha, R.S.; Ochs, H.D.; Dvorak, H.F.; Dvorak, A.M.; Springer, T.A. Transcellular Diapedesis Is Initiated by Invasive Podosomes. Immunity 2007 , 26 , 784–797. [ Google Scholar ] [ CrossRef ] [ Green Version ]
- Angelovich, T.A.; Hearps, A.C.; Maisa, A.; Kelesidis, T.; Jaworowski, A. Quantification of Monocyte Transmigration and Foam Cell Formation from Individuals with Chronic Inflammatory Conditions. J. Vis. Exp. 2017 , 128 , e56293. [ Google Scholar ] [ CrossRef ]
- Hallmann, R.; Horn, N.; Selg, M.; Wendler, O.; Pausch, F.; Sorokin, L.M. Expression and Function of Laminins in the Embryonic and Mature Vasculature. Physiol. Rev. 2005 , 85 , 979–1000. [ Google Scholar ] [ CrossRef ] [ Green Version ]
- Italiani, P.; Boraschi, D. From Monocytes to M1/M2 Macrophages: Phenotypical vs. Functional Differentiation. Front. Immunol. 2014 , 5 , 514. [ Google Scholar ] [ CrossRef ] [ Green Version ]
- Shapouri-Moghaddam, A.; Mohammadian, S.; Vazini, H.; Taghadosi, M.; Esmaeili, S.-A.; Mardani, F.; Seifi, B.; Mohammadi, A.; Afshari, J.T.; Sahebkar, A. Macrophage plasticity, polarization, and function in health and disease. J. Cell. Physiol. 2018 , 233 , 6425–6440. [ Google Scholar ] [ CrossRef ]
- Ferrante, C.J.; Pinhal-Enfield, G.; Elson, G.; Cronstein, B.N.; Hasko, G.; Outram, S.; Leibovich, S.J. The Adenosine-Dependent Angiogenic Switch of Macrophages to an M2-Like Phenotype is Independent of Interleukin-4 Receptor Alpha (IL-4Rα) Signaling. Inflammation 2013 , 36 , 921–931. [ Google Scholar ] [ CrossRef ]
- De Paoli, F.; Staels, B.; Chinetti-Gbaguidi, G. Macrophage Phenotypes and Their Modulation in Atherosclerosis. Circ. J. 2014 , 78 , 1775–1781. [ Google Scholar ] [ CrossRef ] [ PubMed ] [ Green Version ]
- Chen, J.; Li, M.; Yang, C.; Yin, X.; Duan, K.; Wang, J.; Feng, B. Macrophage phenotype switch by sequential action of immunomodulatory cytokines from hydrogel layers on titania nanotubes. Colloids Surfaces B Biointerfaces 2018 , 163 , 336–345. [ Google Scholar ] [ CrossRef ] [ PubMed ]
- Peled, M.; Fisher, E.A. Dynamic Aspects of Macrophage Polarization during Atherosclerosis Progression and Regression. Front. Immunol. 2014 , 5 , 579. [ Google Scholar ] [ CrossRef ] [ PubMed ] [ Green Version ]
- Bobryshev, Y.V. Monocyte recruitment and foam cell formation in atherosclerosis. Micron 2006 , 37 , 208–222. [ Google Scholar ] [ CrossRef ] [ PubMed ]
- Wilfling, F.; Wang, H.; Haas, J.T.; Krahmer, N.; Gould, T.J.; Uchida, A.; Cheng, J.-X.; Graham, M.; Christiano, R.; Fröhlich, F.; et al. Triacylglycerol Synthesis Enzymes Mediate Lipid Droplet Growth by Relocalizing from the ER to Lipid Droplets. Dev. Cell 2013 , 24 , 384–399. [ Google Scholar ] [ CrossRef ] [ Green Version ]
- Ouimet, M.; Barrett, T.J.; Fisher, E.A. HDL and Reverse Cholesterol Transport. Circ. Res. 2019 , 124 , 1505–1518. [ Google Scholar ] [ CrossRef ]
- Chinetti, G.; Baron, M.; Bouhlel, M.A.; Vanhoutte, J.; Copin, C.; Sebti, Y.; Derudas, B.; Mayi, T.; Bories, G.; Tailleux, A.; et al. Human Atherosclerotic Plaque Alternative Macrophages Display Low Cholesterol Handling but High Phagocytosis Because of Distinct Activities of the PPARγ and LXRα Pathways. Circ. Res. 2011 , 108 , 985–995. [ Google Scholar ] [ CrossRef ]
- Maitra, U.; Parks, J.S.; Li, L. An Innate Immunity Signaling Process Suppresses Macrophage ABCA1 Expression through IRAK-1-Mediated Downregulation of Retinoic Acid Receptor α and NFATc2. Mol. Cell. Biol. 2009 , 29 , 5989–5997. [ Google Scholar ] [ CrossRef ] [ Green Version ]
- Kim, T.W.; Febbraio, M.; Robinet, P.; Dugar, B.; Greene, D.; Cerny, A.; Latz, E.; Gilmour, R.; Staschke, K.; Chisolm, G.; et al. The Critical Role of IL-1 Receptor-Associated Kinase 4-Mediated NF-κB Activation in Modified Low-Density Lipoprotein-Induced Inflammatory Gene Expression and Atherosclerosis. J. Immunol. 2011 , 186 , 2871–2880. [ Google Scholar ] [ CrossRef ]
- Rekhter, M.; Staschke, K.; Estridge, T.; Rutherford, P.; Jackson, N.; Gifford-Moore, D.; Foxworthy, P.; Reidy, C.; Huang, X.-D.; Kalbfleisch, M.; et al. Genetic ablation of IRAK4 kinase activity inhibits vascular lesion formation. Biochem. Biophys. Res. Commun. 2008 , 367 , 642–648. [ Google Scholar ] [ CrossRef ]
- Takahashi, T.; Huang, Y.; Yamamoto, K.; Hamano, G.; Kakino, A.; Kang, F.; Imaizumi, Y.; Takeshita, H.; Nozato, Y.; Nozato, S.; et al. The endocytosis of oxidized LDL via the activation of the angiotensin II type 1 receptor. iScience 2021 , 24 , 102076. [ Google Scholar ] [ CrossRef ]
- Nasiri, M.; Janoudi, A.; Vanderberg, A.; Frame, M.; Flegler, C.; Flegler, S.; Abela, G.S. Role of cholesterol crystals in atherosclerosis is unmasked by altering tissue preparation methods. Microsc. Res. Tech. 2015 , 78 , 969–974. [ Google Scholar ] [ CrossRef ] [ PubMed ]
- Levene, C.I. The early lesions of atheroma in the coronary arteries. J. Pathol. Bacteriol. 1956 , 72 , 79–82. [ Google Scholar ] [ CrossRef ] [ PubMed ]
- Katz, S.S.; Shipley, G.G.; Small, D.M. Physical chemistry of the lipids of human atherosclerotic lesions. Demonstration of a lesion intermediate between fatty streaks and advanced plaques. J. Clin. Investig. 1976 , 58 , 200–211. [ Google Scholar ] [ CrossRef ] [ PubMed ] [ Green Version ]
- Bogren, H.; Larsson, K. An X-ray-diffraction study of crystalline cholesterol in some pathological deposits in man. Biochim. Et Biophys. Acta 1963 , 75 , 65–69. [ Google Scholar ] [ CrossRef ]
- Suhalim, J.L.; Chung, C.-Y.; Lilledahl, M.B.; Lim, R.S.; Levi, M.; Tromberg, B.J.; Potma, E.O. Characterization of Cholesterol Crystals in Atherosclerotic Plaques Using Stimulated Raman Scattering and Second-Harmonic Generation Microscopy. Biophys. J. 2012 , 102 , 1988–1995. [ Google Scholar ] [ CrossRef ] [ Green Version ]
- Baumer, Y.; Mehta, N.N.; Dey, A.K.; Powell-Wiley, T.M.; A Boisvert, W. Cholesterol crystals and atherosclerosis. Eur. Heart J. 2020 , 41 , 2236–2239. [ Google Scholar ] [ CrossRef ]
- Abela, G.S. Cholesterol crystals piercing the arterial plaque and intima trigger local and systemic inflammation. J. Clin. Lipidol. 2010 , 4 , 156–164. [ Google Scholar ] [ CrossRef ]
- Guo, H.; Callaway, J.B.; Ting, J.P.-Y. Inflammasomes: Mechanism of action, role in disease, and therapeutics. Nat. Med. 2015 , 21 , 677–687. [ Google Scholar ] [ CrossRef ] [ Green Version ]
- Hornung, V.; Bauernfeind, F.; Halle, A.; O Samstad, E.; Kono, H.; Rock, K.L.; Fitzgerald, K.; Latz, E. Silica crystals and aluminum salts activate the NALP3 inflammasome through phagosomal destabilization. Nat. Immunol. 2008 , 9 , 847–856. [ Google Scholar ] [ CrossRef ]
- Zhou, R.; Yazdi, A.S.; Menu, P.; Tschopp, J. A role for mitochondria in NLRP3 inflammasome activation. Nature 2011 , 469 , 221–225, Erratum in Nature 2011 , 475 , 122. [ Google Scholar ] [ CrossRef ]
- Mietus-Snyder, M.; Gowri, M.S.; Pitas, R.E. Class A Scavenger Receptor Up-regulation in Smooth Muscle Cells by Oxidized Low Density Lipoprotein. J. Biol. Chem. 2000 , 275 , 17661–17670. [ Google Scholar ] [ CrossRef ] [ PubMed ] [ Green Version ]
- Yan, P.; Xia, C.; Duan, C.; Li, S.; Mei, Z. Biological Characteristics of Foam Cell Formation in Smooth Muscle Cells Derived from Bone Marrow Stem Cells. Int. J. Biol. Sci. 2011 , 7 , 937–946. [ Google Scholar ] [ CrossRef ] [ PubMed ]
- Beyea, M.M.; Reaume, S.; Sawyez, C.G.; Edwards, J.Y.; O’Neil, C.; Hegele, R.A.; Pickering, J.G.; Huff, M.W. The Oxysterol 24 (S), 25-Epoxycholesterol Attenuates Human Smooth Muscle–Derived Foam Cell Formation Via Reduced Low-Density Lipoprotein Uptake and Enhanced Cholesterol Efflux. J. Am. Heart Assoc. 2012 , 1 , e000810. [ Google Scholar ] [ CrossRef ] [ PubMed ] [ Green Version ]
- Ricciarelli, R.; Zingg, J.-M.; Azzi, A. Vitamin E Reduces the Uptake of Oxidized LDL by Inhibiting CD36 Scavenger Receptor Expression in Cultured Aortic Smooth Muscle Cells. Circulation 1999 , 102 , 82–87. [ Google Scholar ] [ CrossRef ] [ PubMed ]
- Aoyama, T.; Chen, M.; Fujiwara, H.; Masaki, T.; Sawamura, T. LOX-1 mediates lysophosphatidylcholine-induced oxidized LDL uptake in smooth muscle cells. FEBS Lett. 2000 , 467 , 217–220. [ Google Scholar ] [ CrossRef ] [ Green Version ]
- Choi, H.Y.; Rahmani, M.; Wong, B.W.; Allahverdian, S.; McManus, B.M.; Pickering, J.G.; Chan, T.; Francis, G.A. ATP-Binding Cassette Transporter A1 Expression and Apolipoprotein A-I Binding Are Impaired in Intima-Type Arterial Smooth Muscle Cells. Circulation 2009 , 119 , 3223–3231. [ Google Scholar ] [ CrossRef ] [ Green Version ]
- Lusis, A.J. Atherosclerosis. Nature 2000 , 407 , 233–241. [ Google Scholar ] [ CrossRef ]
- Fisher, E.A.; Feig, J.E.; Hewing, B.; Hazen, S.L.; Smith, J.D. High-Density Lipoprotein Function, Dysfunction, and Reverse Cholesterol Transport. Arterioscler. Thromb. Vasc. Biol. 2012 , 32 , 2813–2820. [ Google Scholar ] [ CrossRef ] [ Green Version ]
- Puri, R.; Nissen, S.E.; Ballantyne, C.M.; Barter, P.J.; Chapman, M.J.; Erbel, R.; Libby, P.; Raichlen, J.S.; John, J.S.; Wolski, K.; et al. Factors underlying regression of coronary atheroma with potent statin therapy. Eur. Heart J. 2013 , 34 , 1818–1825. [ Google Scholar ] [ CrossRef ]
- Chamié, D.; Wang, Z.; Bezerra, H.; Rollins, A.M.; Costa, M.A. Optical Coherence Tomography and Fibrous Cap Characterization. Curr. Cardiovasc. Imaging Rep. 2011 , 4 , 276–283. [ Google Scholar ] [ CrossRef ] [ PubMed ] [ Green Version ]
- Watson, M.G.; Byrne, H.M.; Macaskill, C.; Myerscough, M.R. A two-phase model of early fibrous cap formation in atherosclerosis. J. Theor. Biol. 2018 , 456 , 123–136. [ Google Scholar ] [ CrossRef ] [ PubMed ] [ Green Version ]
- Schaper, W.; Ito, W.D. Molecular Mechanisms of Coronary Collateral Vessel Growth. Circ. Res. 1996 , 79 , 911–919. [ Google Scholar ] [ CrossRef ] [ PubMed ]
- Wolf, C.; Cai, W.-J.; Vosschulte, R.; Koltai, S.; Mousavipour, D.; Scholz, D.; Afsah-Hedjri, A.; Schaper, W.; Schaper, J. Vascular Remodeling and Altered Protein Expression During Growth of Coronary Collateral Arteries. J. Mol. Cell. Cardiol. 1998 , 30 , 2291–2305. [ Google Scholar ] [ CrossRef ]
- Zalewski, A.; Shi, Y.; Johnson, A.G. Diverse Origin of Intimal Cells. Circ. Res. 2002 , 91 , 652–655. [ Google Scholar ] [ CrossRef ] [ Green Version ]
- Louis, S.F.; Zahradka, P. Vascular smooth muscle cell motility: From migration to invasion. Exp. Clin. Cardiol. 2010 , 15 , e75–e85. [ Google Scholar ]
- Bennett, M.R.; Sinha, S.; Owens, G.K. Vascular Smooth Muscle Cells in Atherosclerosis. Circ. Res. 2016 , 118 , 692–702. [ Google Scholar ] [ CrossRef ]
- Stiko-Rahm, A.; Hultgårdh-Nilsson, A.; Regnström, J.; Hamsten, A.; Nilsson, J. Native and oxidized LDL enhances production of PDGF AA and the surface expression of PDGF receptors in cultured human smooth muscle cells. Arter. Thromb. A J. Vasc. Biol. 1992 , 12 , 1099–1109. [ Google Scholar ] [ CrossRef ] [ Green Version ]
- Kohno, M.; Yokokawa, K.; Yasunari, K.; Minami, M.; Kano, H.; Hanehira, T.; Yoshikawa, J. Induction by lysophosphatidylcholine, a major phospholipid component of atherogenic lipoproteins, of human coronary artery smooth muscle cell migration. Circulation 1998 , 98 , 353–359. [ Google Scholar ] [ CrossRef ]
- Ross, R. The Pathogenesis of Atherosclerosis—An Update. N. Engl. J. Med. 1986 , 314 , 488–500. [ Google Scholar ] [ CrossRef ]
- Raines, E.W.; Dower, S.K.; Ross, R. Interleukin-1 Mitogenic Activity for Fibroblasts and Smooth Muscle Cells Is Due to PDGF-AA. Science 1989 , 243 , 393–396. [ Google Scholar ] [ CrossRef ] [ PubMed ]
- Forsyth, E.A.; Aly, H.M.; Neville, R.F.; Sidawy, A.N. Proliferation and extracellular matrix production by human infragenicular smooth muscle cells in response to interleukin-1β. J. Vasc. Surg. 1997 , 26 , 1002–1008. [ Google Scholar ] [ CrossRef ] [ Green Version ]
- Bentzon, J.F.; Otsuka, F.; Virmani, R.; Falk, E. Mechanisms of Plaque Formation and Rupture. Circ. Res. 2014 , 114 , 1852–1866. [ Google Scholar ] [ CrossRef ] [ PubMed ]
- Shen, C.-M.; Mao, S.J.; Huang, G.; Yang, P.-C.; Chu, R.-M. Stimulation of smooth muscle cell proliferation by ox-LDL- and acetyl LDL-induced macrophage-derived foam cells. Life Sci. 2001 , 70 , 443–452. [ Google Scholar ] [ CrossRef ]
- Sorokin, V.; Vickneson, K.; Kofidis, T.; Woo, C.C.; Lin, X.Y.; Foo, R.; Shanahan, C.M. Role of Vascular Smooth Muscle Cell Plasticity and Interactions in Vessel Wall Inflammation. Front. Immunol. 2020 , 11 , 599415. [ Google Scholar ] [ CrossRef ]
- Badimon, L.; Padro, T.; Vilahur, G. Atherosclerosis, platelets and thrombosis in acute ischaemic heart disease. Eur. Heart J. Acute Cardiovasc. Care 2012 , 1 , 60–74. [ Google Scholar ] [ CrossRef ] [ PubMed ]
- Zhang, Z.; Wang, M.; Fan, X.-H.; Chen, J.-H.; Guan, Y.-Y.; Tang, Y.-B. Upregulation of TRPM7 Channels by Angiotensin II Triggers Phenotypic Switching of Vascular Smooth Muscle Cells of Ascending Aorta. Circ. Res. 2012 , 111 , 1137–1146. [ Google Scholar ] [ CrossRef ] [ PubMed ] [ Green Version ]
- Mack, C.P. Signaling Mechanisms That Regulate Smooth Muscle Cell Differentiation. Arterioscler. Thromb. Vasc. Biol. 2011 , 31 , 1495–1505. [ Google Scholar ] [ CrossRef ] [ Green Version ]
- Richardson, P.; Davies, M.; Born, G. Influence of Plaque Configuration and Stress Distribution on Fissuring of Coronary Atherosclerotic Plaques. Lancet 1989 , 334 , 941–944. [ Google Scholar ] [ CrossRef ]
- Li, Z.-Y.; Howarth, S.P.; Tang, T.; Gillard, J.H. How Critical Is Fibrous Cap Thickness to Carotid Plaque Stability? Stroke 2006 , 37 , 1195–1199. [ Google Scholar ] [ CrossRef ] [ Green Version ]
- Anlamlert, W.; Lenbury, Y.; Bell, J. Modeling fibrous cap formation in atherosclerotic plaque development: Stability and oscillatory behavior. Adv. Differ. Equations 2017 , 2017 , 195. [ Google Scholar ] [ CrossRef ] [ Green Version ]
- Motoyama, S.; Sarai, M.; Harigaya, H.; Anno, H.; Inoue, K.; Hara, T.; Naruse, H.; Ishii, J.; Hishida, H.; Wong, N.D.; et al. Computed Tomographic Angiography Characteristics of Atherosclerotic Plaques Subsequently Resulting in Acute Coronary Syndrome. J. Am. Coll. Cardiol. 2009 , 54 , 49–57. [ Google Scholar ] [ CrossRef ] [ PubMed ] [ Green Version ]
- Visscher, M.; Moerman, A.M.; Burgers, P.C.; Van Beusekom, H.M.M.; Luider, T.M.; Verhagen, H.J.M.; Van der Steen, A.F.W.; Van der Heiden, K.; Van Soest, G. Data Processing Pipeline for Lipid Profiling of Carotid Atherosclerotic Plaque with Mass Spectrometry Imaging. J. Am. Soc. Mass Spectrom. 2019 , 30 , 1790–1800. [ Google Scholar ] [ CrossRef ] [ PubMed ] [ Green Version ]
- Thim, T.; Hagensen, M.K.; Bentzon, J.F.; Falk, E. From vulnerable plaque to atherothrombosis. J. Intern. Med. 2008 , 263 , 506–516. [ Google Scholar ] [ CrossRef ] [ PubMed ]
- Linton, M.F.; Babaev, V.R.; Huang, J.; Linton, E.F.; Tao, H.; Yancey, P.G. Macrophage Apoptosis and Efferocytosis in the Pathogenesis of Atherosclerosis. Circ. J. 2016 , 80 , 2259–2268. [ Google Scholar ] [ CrossRef ] [ Green Version ]
- Cabrera, J.T.O.; Makino, A. Efferocytosis of vascular cells in cardiovascular disease. Pharmacol. Ther. 2021 , 107919. [ Google Scholar ] [ CrossRef ]
- Gonzalez, L.; Trigatti, B.L. Macrophage Apoptosis and Necrotic Core Development in Atherosclerosis: A Rapidly Advancing Field with Clinical Relevance to Imaging and Therapy. Can. J. Cardiol. 2017 , 33 , 303–312. [ Google Scholar ] [ CrossRef ]
- Coornaert, I.; Hofmans, S.; Devisscher, L.; Augustyns, K.; Van der Veken, P.; De Meyer, G.; Martinet, W. Novel drug discovery strategies for atherosclerosis that target necrosis and necroptosis. Expert Opin. Drug Discov. 2018 , 13 , 477–488. [ Google Scholar ] [ CrossRef ]
- Wanke, F.; Gutbier, S.; Rümmelin, A.; Steinberg, M.; Hughes, L.D.; Koenen, M.; Komuczki, J.; Regan-Komito, D.; Wagage, S.; Hesselmann, J.; et al. Ligand-dependent kinase activity of MERTK drives efferocytosis in human iPSC-derived macrophages. Cell Death Dis. 2021 , 12 , 538. [ Google Scholar ] [ CrossRef ]
- Quillard, T.; Araújo, H.A.; Franck, G.; Tesmenitsky, Y.; Libby, P. Matrix Metalloproteinase-13 Predominates Over Matrix Metalloproteinase-8 as the Functional Interstitial Collagenase in Mouse Atheromata. Arterioscler. Thromb. Vasc. Biol. 2014 , 34 , 1179–1186. [ Google Scholar ] [ CrossRef ] [ Green Version ]
- Galis, Z.S.; Sukhova, G.K.; Kranzhöfer, R.; Clark, S.; Libby, P. Macrophage foam cells from experimental atheroma constitutively produce matrix-degrading proteinases. Proc. Natl. Acad. Sci. USA 1995 , 92 , 402–406. [ Google Scholar ] [ CrossRef ] [ PubMed ] [ Green Version ]
- Schneider, F.; Sukhova, G.K.; Aikawa, M.; Canner, J.; Gerdes, N.; Tang, S.-M.T.; Shi, G.-P.; Apte, S.; Libby, P. Matrix Metalloproteinase-14 Deficiency in Bone Marrow–Derived Cells Promotes Collagen Accumulation in Mouse Atherosclerotic Plaques. Circulation 2008 , 117 , 931–939. [ Google Scholar ] [ CrossRef ] [ Green Version ]
- Mulay, S.R.; Anders, H.-J. Crystallopathies. N. Engl. J. Med. 2016 , 374 , 2465–2476. [ Google Scholar ] [ CrossRef ] [ PubMed ]
- Nidorf, S.M.; Fiolet, A.; Abela, G.S. Viewing atherosclerosis through a crystal lens: How the evolving structure of cholesterol crystals in atherosclerotic plaque alters its stability. J. Clin. Lipidol. 2020 , 14 , 619–630. [ Google Scholar ] [ CrossRef ] [ PubMed ]
- Warnatsch, A.; Ioannou, M.; Wang, Q.; Papayannopoulos, V. Neutrophil extracellular traps license macrophages for cytokine production in atherosclerosis. Science 2015 , 349 , 316–320. [ Google Scholar ] [ CrossRef ] [ PubMed ] [ Green Version ]
- Johnson, J.L.; Jenkins, N.P.; Huang, W.-C.; Di Gregoli, K.; Sala-Newby, G.B.; Scholtes, V.P.W.; Moll, F.L.; Pasterkamp, G.; Newby, A.C. Relationship of MMP-14 and TIMP-3 Expression with Macrophage Activation and Human Atherosclerotic Plaque Vulnerability. Mediat. Inflamm. 2014 , 2014 , 276457. [ Google Scholar ] [ CrossRef ] [ Green Version ]
- García-García, H.M.; Klauss, V.; Gonzalo, N.; Garg, S.; Onuma, Y.; Hamm, C.W.; Wijns, W.; Shannon, J.; Serruys, P.W. Relationship between cardiovascular risk factors and biomarkers with necrotic core and atheroma size: A serial intravascular ultrasound radiofrequency data analysis. Int. J. Cardiovasc. Imaging 2012 , 28 , 695–703. [ Google Scholar ] [ CrossRef ] [ Green Version ]
- Martinet, W.; Schrijvers, D.; De Meyer, G. Necrotic cell death in atherosclerosis. Basic Res. Cardiol. 2011 , 106 , 749–760. [ Google Scholar ] [ CrossRef ]
- Steffel, J.; Lüscher, T.F.; Tanner, F.C. Tissue Factor in Cardiovascular Diseases. Circulation 2006 , 113 , 722–731. [ Google Scholar ] [ CrossRef ] [ Green Version ]
- Nakahara, T.; Dweck, M.; Narula, N.; Pisapia, D.; Narula, J.; Strauss, H.W. Coronary Artery Calcification. JACC Cardiovasc. Imaging 2017 , 10 , 582–593. [ Google Scholar ] [ CrossRef ]
- Shi, X.; Gao, J.; Lv, Q.; Cai, H.; Wang, F.; Ye, R.; Liu, X. Calcification in Atherosclerotic Plaque Vulnerability: Friend or Foe? Front. Physiol. 2020 , 11 , 56. [ Google Scholar ] [ CrossRef ] [ PubMed ]
- Bobryshev, Y.V.; Killingsworth, M.C.; Lord, R.S.A.; Grabs, A.J. Matrix vesicles in the fibrous cap of atherosclerotic plaque: Possible contribution to plaque rupture. J. Cell. Mol. Med. 2008 , 12 , 2073–2082. [ Google Scholar ] [ CrossRef ] [ PubMed ] [ Green Version ]
- Anderson, H.C. Mechanisms of Pathologic Calcification. Rheum. Dis. Clin. N. Am. 1988 , 14 , 303–319. [ Google Scholar ] [ CrossRef ]
- Kapustin, A.N.; Chatrou, M.L.; Drozdov, I.; Zheng, Y.; Davidson, S.M.; Soong, D.; Furmanik, M.; Sanchis, P.; de Rosales, R.T.M.; Alvarez-Hernandez, D.; et al. Vascular Smooth Muscle Cell Calcification Is Mediated by Regulated Exosome Secretion. Circ. Res. 2015 , 116 , 1312–1323. [ Google Scholar ] [ CrossRef ] [ PubMed ] [ Green Version ]
- New, S.E.P.; Goettsch, C.; Aikawa, M.; Marchini, J.; Shibasaki, M.; Yabusaki, K.; Libby, P.; Shanahan, C.; Croce, K.; Aikawa, E. Macrophage-Derived Matrix Vesicles. Circ. Res. 2013 , 113 , 72–77. [ Google Scholar ] [ CrossRef ]
- Canfield, A.; Sutton, A.; Hoyland, J.; Schor, A. Association of thrombospondin-1 with osteogenic differentiation of retinal pericytes in vitro. J. Cell Sci. 1996 , 109 , 343–353. [ Google Scholar ] [ CrossRef ]
- Lomashvili, K.A.; Cobbs, S.; Hennigar, R.A.; Hardcastle, K.I.; O’Neill, W.C. Phosphate-Induced Vascular Calcification: Role of Pyrophosphate and Osteopontin. J. Am. Soc. Nephrol. 2004 , 15 , 1392–1401. [ Google Scholar ] [ CrossRef ] [ Green Version ]
- Otsuka, F.; Sakakura, K.; Yahagi, K.; Joner, M.; Virmani, R. Has Our Understanding of Calcification in Human Coronary Atherosclerosis Progressed? Arterioscler. Thromb. Vasc. Biol. 2014 , 34 , 724–736. [ Google Scholar ] [ CrossRef ] [ Green Version ]
- Proudfoot, D.; Skepper, J.N.; Shanahan, C.M.; Weissberg, P.L. Calcification of Human Vascular Cells in Vitro Is Correlated With High Levels of Matrix Gla Protein and Low Levels of Osteopontin Expression. Arterioscler. Thromb. Vasc. Biol. 1998 , 18 , 379–388. [ Google Scholar ] [ CrossRef ] [ Green Version ]
- Demer, L.L.; Tintut, Y. Vascular Calcification: Pathobiology of a multifaceted disease. Circulation 2008 , 117 , 2938–2948. [ Google Scholar ] [ CrossRef ]
- Reynolds, J.L.; Joannides, A.J.; Skepper, J.N.; McNair, R.; Schurgers, L.J.; Proudfoot, D.; Jahnen-Dechent, W.; Weissberg, P.L.; Shanahan, C.M. Human Vascular Smooth Muscle Cells Undergo Vesicle-Mediated Calcification in Response to Changes in Extracellular Calcium and Phosphate Concentrations: A Potential Mechanism for Accelerated Vascular Calcification in ESRD. J. Am. Soc. Nephrol. 2004 , 15 , 2857–2867. [ Google Scholar ] [ CrossRef ] [ PubMed ] [ Green Version ]
- Reith, S.; Milzi, A.; Dettori, R.; Marx, N.; Burgmaier, M. Predictors for target lesion microcalcifications in patients with stable coronary artery disease: An optical coherence tomography study. Clin. Res. Cardiol. 2018 , 107 , 763–771. [ Google Scholar ] [ CrossRef ] [ PubMed ]
- Pugliese, G.; Iacobini, C.; Fantauzzi, C.B.; Menini, S. The dark and bright side of atherosclerotic calcification. Atherosclerosis 2015 , 238 , 220–230. [ Google Scholar ] [ CrossRef ] [ PubMed ]
- Slager, C.J.; Wentzel, J.J.; Gijsen, F.J.H.; Schuurbiers, J.C.H.; van der Wal, A.; Van Der Steen, A.F.W.; Serruys, P.W. The role of shear stress in the generation of rupture-prone vulnerable plaques. Nat. Clin. Pract. Cardiovasc. Med. 2005 , 2 , 401–407. [ Google Scholar ] [ CrossRef ]
- Stefanadis, C.; Antoniou, C.; Tsiachris, D.; Pietri, P. Coronary Atherosclerotic Vulnerable Plaque: Current Perspectives. J. Am. Heart Assoc. 2017 , 6 , e005543. [ Google Scholar ] [ CrossRef ] [ Green Version ]
- Cuadrado, I.; Saura, M.; Castejón, B.; Martin, A.M.; Herruzo, I.; Balatsos, N.; Zamorano, J.L.; Zaragoza, C. Preclinical models of atherosclerosis. The future of Hybrid PET/MR technology for the early detection of vulnerable plaque. Expert Rev. Mol. Med. 2016 , 18 , E6. [ Google Scholar ] [ CrossRef ]
- Sodhi, N.; Brown, D.L. Pathophysiology of Acute Coronary Syndromes. In Cardiac Intensive Care ; Elsevier BV: Amsterdam, The Netherlands, 2019; pp. 68–80.e2. [ Google Scholar ]
- Libby, P. Inflammation in atherosclerosis. Nature 2002 , 420 , 868–874. [ Google Scholar ] [ CrossRef ]
- Kovanen, P.T.; Kaartinen, M.; Paavonen, T. Infiltrates of Activated Mast Cells at the Site of Coronary Atheromatous Erosion or Rupture in Myocardial Infarction. Circulation 1995 , 92 , 1084–1088. [ Google Scholar ] [ CrossRef ]
- Sarén, P.; Welgus, H.G.; Kovanen, P.T. TNF-alpha and IL-1beta selectively induce expression of 92-kDa gelatinase by human macrophages. J. Immunol. 1996 , 157 , 4159–4165. [ Google Scholar ]
- Pasterkamp, G.; Schoneveld, A.H.; van der Wal, A.C.; Hijnen, D.-J.; van Wolveren, W.J.A.; Plomp, S.; Teepen, H.L.J.M.; Borst, C. Inflammation of the Atherosclerotic Cap and Shoulder of the Plaque Is a Common and Locally Observed Feature in Unruptured Plaques of Femoral and Coronary Arteries. Arterioscler. Thromb. Vasc. Biol. 1999 , 19 , 54–58. [ Google Scholar ] [ CrossRef ] [ Green Version ]
- Groen, H.C.; Gijsen, F.J.; Van Der Lugt, A.; Ferguson, M.S.; Hatsukami, T.S.; Van Der Steen, A.F.; Yuan, C.; Wentzel, J.J. Plaque Rupture in the Carotid Artery Is Localized at the High Shear Stress Region. Stroke 2007 , 38 , 2379–2381. [ Google Scholar ] [ CrossRef ] [ Green Version ]
- Vergallo, R.; Crea, F. Atherosclerotic Plaque Healing. N. Engl. J. Med. 2020 , 383 , 846–857. [ Google Scholar ] [ CrossRef ] [ PubMed ]
- Yun, S.-H.; Sim, E.-H.; Goh, R.-Y.; Park, J.-I.; Han, J.-Y. Platelet Activation: The Mechanisms and Potential Biomarkers. BioMed Res. Int. 2016 , 2016 , 9060143. [ Google Scholar ] [ CrossRef ] [ PubMed ] [ Green Version ]
- Osaki, T.; Ichinose, A. [Current views of activating and regulatory mechanisms of blood coagulation]. Nihon rinsho. Jpn. J. Clin. Med. 2014 , 72 , 1206–1211. [ Google Scholar ]
- Mackman, N.; Tilley, R.E.; Key, N.S. Role of the extrinsic pathway of blood coagulation in hemostasis and thrombosis. Arterioscler. Thromb. Vasc. Biol. 2007 , 27 , 1687–1693. [ Google Scholar ] [ CrossRef ] [ PubMed ] [ Green Version ]
- Grainger, D.J.; Wakefield, L.; Bethell, H.W.; Farndale, R.W.; Metcalfe, J.C. Release and activation of platelet latent TGF–β in blood clots during dissolution with plasmin. Nat. Med. 1995 , 1 , 932–937. [ Google Scholar ] [ CrossRef ]
- Epstein, F.H.; Fuster, V.; Badimon, L.; Badimon, J.J.; Chesebro, J.H. The Pathogenesis of Coronary Artery Disease and the Acute Coronary Syndromes. N. Engl. J. Med. 1992 , 326 , 310–318. [ Google Scholar ] [ CrossRef ] [ PubMed ]
- Théroux, P.; Fuster, V. Acute Coronary Syndromes. Circulation 1998 , 97 , 1195–1206. [ Google Scholar ] [ CrossRef ] [ Green Version ]
- Thanvi, B.; Robinson, T. Complete occlusion of extracranial internal carotid artery: Clinical features, pathophysiology, diagnosis and management. Postgrad. Med. J. 2007 , 83 , 95–99. [ Google Scholar ] [ CrossRef ]
- Lyaker, M.R.; Tulman, D.B.; Dimitrova, G.T.; Pin, R.H.; Papadimos, T.J. Arterial embolism. Int. J. Crit. Illn. Inj. Sci. 2013 , 3 , 77–87. [ Google Scholar ] [ CrossRef ]
- Ridker, P.M.; Howard, C.P.; Walter, V.; Everett, B.; Libby, P.; Hensen, J.; Thuren, T. Effects of Interleukin-1β Inhibition With Canakinumab on Hemoglobin A1c, Lipids, C-Reactive Protein, Interleukin-6, and Fibrinogen. Circulation 2012 , 126 , 2739–2748. [ Google Scholar ] [ CrossRef ] [ PubMed ] [ Green Version ]
- Meiliana, A.; Dewi, N.M.; Wijaya, A. Advanced in Molecular Mechanisms of Atherosclerosis: From Lipids to Inflammation. Indones. Biomed. J. 2018 , 10 , 104–122. [ Google Scholar ] [ CrossRef ]
- Cushing, S.D.; A Berliner, J.; Valente, A.J.; Territo, M.C.; Navab, M.; Parhami, F.; Gerrity, R.; Schwartz, C.J.; Fogelman, A.M. Minimally modified low density lipoprotein induces monocyte chemotactic protein 1 in human endothelial cells and smooth muscle cells. Proc. Natl. Acad. Sci. USA 1990 , 87 , 5134–5138. [ Google Scholar ] [ CrossRef ] [ PubMed ] [ Green Version ]
- Quinn, M.T.; Parthasarathy, S.; Fong, L.G.; Steinberg, D. Oxidatively modified low density lipoproteins: A potential role in recruitment and retention of monocyte/macrophages during atherogenesis. Proc. Natl. Acad. Sci. USA 1987 , 84 , 2995–2998. [ Google Scholar ] [ CrossRef ] [ Green Version ]
- Soehnlein, O.; Drechsler, M.; Döring, Y.; Lievens, D.; Hartwig, H.; Kemmerich, K.; Ortega-Gomez, A.; Mandl, M.; Vijayan, S.; Projahn, D.; et al. Distinct functions of chemokine receptor axes in the atherogenic mobilization and recruitment of classical monocytes. EMBO Mol. Med. 2013 , 5 , 471–481. [ Google Scholar ] [ CrossRef ]
- Chávez-Sánchez, L.; Madrid-Miller, A.; Chávez-Rueda, K.; Legorreta-Haquet, M.; Tesoro-Cruz, E.; Blanco-Favela, F. Activation of TLR2 and TLR4 by minimally modified low-density lipoprotein in human macrophages and monocytes triggers the inflammatory response. Hum. Immunol. 2010 , 71 , 737–744. [ Google Scholar ] [ CrossRef ]
- Miller, Y.I.; Viriyakosol, S.; Worrall, D.S.; Boullier, A.; Butler, S.; Witztum, J.L. Toll-Like Receptor 4–Dependent and –Independent Cytokine Secretion Induced by Minimally Oxidized Low-Density Lipoprotein in Macrophages. Arterioscler. Thromb. Vasc. Biol. 2005 , 25 , 1213–1219. [ Google Scholar ] [ CrossRef ] [ Green Version ]
- Schroder, K.; Tschopp, J. The Inflammasomes. Cell 2010 , 140 , 821–832. [ Google Scholar ] [ CrossRef ] [ Green Version ]
- Lopes-Virella, M.F.; Virella, G. Pathogenic Role of Modified LDL Antibodies and Immune Complexes in Atherosclerosis. J. Atheroscler. Thromb. 2013 , 20 , 743–754. [ Google Scholar ] [ CrossRef ] [ Green Version ]
- Rhoads, J.P.; Lukens, J.R.; Wilhelm, A.J.; Moore, J.L.; Mendez-Fernandez, Y.; Kanneganti, T.-D.; Major, A.S. Oxidized Low-Density Lipoprotein Immune Complex Priming of the Nlrp3 Inflammasome Involves TLR and FcγR Cooperation and Is Dependent on CARD9. J. Immunol. 2017 , 198 , 2105–2114. [ Google Scholar ] [ CrossRef ] [ Green Version ]
- Duewell, P.; Kono, H.; Rayner, K.J.; Sirois, C.M.; Vladimer, G.; Bauernfeind, F.G.; Abela, G.S.; Franchi, L.; Nuñez, G.; Schnurr, M.; et al. NLRP3 inflammasomes are required for atherogenesis and activated by cholesterol crystals. Nature 2010 , 464 , 1357–1361. [ Google Scholar ] [ CrossRef ] [ PubMed ] [ Green Version ]
- Mofidi, R.; Crotty, T.B.; McCarthy, P.; Sheehan, S.J.; Mehigan, D.; Keaveny, T.V. Association between plaque instability, angiogenesis and symptomatic carotid occlusive disease. Br. J. Surg. 2002 , 88 , 945–950. [ Google Scholar ] [ CrossRef ] [ PubMed ]
- Spagnoli, L.G.; Bonanno, E.; Sangiorgi, G.; Mauriello, A. Role of Inflammation in Atherosclerosis. J. Nucl. Med. 2007 , 48 , 1800–1815. [ Google Scholar ] [ CrossRef ] [ Green Version ]
- Akhmedov, A.; Sawamura, T.; Chen, C.-H.; Kraler, S.; Vdovenko, D.; Lüscher, T.F. Lectin-like oxidized low-density lipoprotein receptor-1 (LOX-1): A crucial driver of atherosclerotic cardiovascular disease. Eur. Heart J. 2021 , 42 , 1797–1807. [ Google Scholar ] [ CrossRef ] [ PubMed ]
- Chen, L.; Yang, G.; Monslow, J.; Todd, L.; Cormode, D.P.; Tang, J.; Grant, G.R.; DeLong, J.H.; Tang, S.Y.; Lawson, J.A.; et al. Myeloid cell microsomal prostaglandin E synthase-1 fosters atherogenesis in mice. Proc. Natl. Acad. Sci. USA 2014 , 111 , 6828–6833. [ Google Scholar ] [ CrossRef ] [ Green Version ]
- Yang, G.; Chen, L. An Update of Microsomal Prostaglandin E Synthase-1 and PGE2Receptors in Cardiovascular Health and Diseases. Oxidative Med. Cell. Longev. 2016 , 2016 , 5249086. [ Google Scholar ] [ CrossRef ] [ Green Version ]
- Haemmig, S.; Simion, V.; Yang, D.; Deng, Y.; Feinberg, M.W. Long noncoding RNAs in cardiovascular disease, diagnosis, and therapy. Curr. Opin. Cardiol. 2017 , 32 , 776–783. [ Google Scholar ] [ CrossRef ]
- Freedman, J.E.; Miano, J.M. Challenges and Opportunities in Linking Long Noncoding RNAs to Cardiovascular, Lung, and Blood Diseases. Arterioscler. Thromb. Vasc. Biol. 2017 , 37 , 21–25. [ Google Scholar ] [ CrossRef ] [ Green Version ]
- Boettger, T.; Braun, T. A New Level of Complexity. Circ. Res. 2012 , 110 , 1000–1013. [ Google Scholar ] [ CrossRef ] [ Green Version ]
- Wang, R.; Dong, L.-D.; Meng, X.-B.; Shi, Q.; Sun, W.-Y. Unique MicroRNA signatures associated with early coronary atherosclerotic plaques. Biochem. Biophys. Res. Commun. 2015 , 464 , 574–579. [ Google Scholar ] [ CrossRef ]
- Di Gregoli, K.; Jenkins, N.; Salter, R.; White, S.; Newby, A.C.; Johnson, J.L. MicroRNA-24 Regulates Macrophage Behavior and Retards Atherosclerosis. Arterioscler. Thromb. Vasc. Biol. 2014 , 34 , 1990–2000. [ Google Scholar ] [ CrossRef ] [ PubMed ] [ Green Version ]
- Di Gregoli, K.; Anuar, N.N.M.; Bianco, R.; White, S.J.; Newby, A.C.; George, S.J.; Johnson, J.L. MicroRNA-181b Controls Atherosclerosis and Aneurysms Through Regulation of TIMP-3 and Elastin. Circ. Res. 2017 , 120 , 49–65. [ Google Scholar ] [ CrossRef ] [ PubMed ] [ Green Version ]
- Raitoharju, E.; Lyytikäinen, L.-P.; Levula, M.; Oksala, N.; Mennander, A.; Tarkka, M.; Klopp, N.; Illig, T.; Kähönen, M.; Karhunen, P.J.; et al. miR-21, miR-210, miR-34a, and miR-146a/b are up-regulated in human atherosclerotic plaques in the Tampere Vascular Study. Atherosclerosis 2011 , 219 , 211–217. [ Google Scholar ] [ CrossRef ] [ PubMed ]
- Denecke, B.; Rostalsky, A.; Hristov, M.; Koeppel, T.A.; Bidzhekov, K.; Gan, L.; Zernecke, A.; Weber, C. microRNA expression signatures and parallels between monocyte subsets and atherosclerotic plaque in humans. Thromb. Haemost. 2012 , 107 , 619–625. [ Google Scholar ] [ CrossRef ] [ PubMed ]
- Cipollone, F.; Felicioni, L.; Sarzani, R.; Ucchino, S.; Spigonardo, F.; Mandolini, C.; Malatesta, S.; Bucci, M.; Mammarella, C.; Santovito, D.; et al. A Unique MicroRNA Signature Associated With Plaque Instability in Humans. Stroke 2011 , 42 , 2556–2563. [ Google Scholar ] [ CrossRef ] [ PubMed ] [ Green Version ]
- Zhu, N.; Zhang, D.; Chen, S.; Liu, X.; Lin, L.; Huang, X.; Guo, Z.; Liu, J.; Wang, Y.; Yuan, W.; et al. Endothelial enriched microRNAs regulate angiotensin II-induced endothelial inflammation and migration. Atherosclerosis 2011 , 215 , 286–293. [ Google Scholar ] [ CrossRef ] [ PubMed ]
- Lee, D.-Y.; Yang, T.-L.; Huang, Y.-H.; Lee, C.-I.; Chen, L.-J.; Shih, Y.-T.; Wei, S.-Y.; Wang, W.-L.; Wu, C.-C.; Chiu, J.-J. Induction of microRNA-10a using retinoic acid receptor-α and retinoid x receptor-α agonists inhibits atherosclerotic lesion formation. Atherosclerosis 2018 , 271 , 36–44. [ Google Scholar ] [ CrossRef ]
- Suárez, Y.; Wang, C.; Manes, T.D.; Pober, J.S. Cutting Edge: TNF-Induced MicroRNAs Regulate TNF-Induced Expression of E-Selectin and Intercellular Adhesion Molecule-1 on Human Endothelial Cells: Feedback Control of Inflammation. J. Immunol. 2010 , 184 , 21–25. [ Google Scholar ] [ CrossRef ] [ Green Version ]
- Fish, J.E.; Santoro, M.M.; Morton, S.U.; Yu, S.; Yeh, R.-F.; Wythe, J.D.; Ivey, K.N.; Bruneau, B.G.; Stainier, D.Y.R.; Srivastava, D. miR-126 Regulates Angiogenic Signaling and Vascular Integrity. Dev. Cell 2008 , 15 , 272–284. [ Google Scholar ] [ CrossRef ] [ Green Version ]
- Sun, X.; Icli, B.; Wara, A.K.; Belkin, N.; He, S.; Kobzik, L.; Hunninghake, G.M.; Pinilla-Vera, M.; Blackwell, T.S.; Baron, R.M.; et al. MicroRNA-181b regulates NF-κB–mediated vascular inflammation. J. Clin. Investig. 2012 , 122 , 1973–1990. [ Google Scholar ] [ CrossRef ]
- Yang, K.; He, Y.S.; Wang, X.Q.; Lu, L.; Chen, Q.J.; Liu, J.; Sun, Z.; Shen, W.F. MiR-146a inhibits oxidized low-density lipoprotein-induced lipid accumulation and inflammatory response via targeting toll-like receptor 4. FEBS Lett. 2011 , 585 , 854–860. [ Google Scholar ] [ CrossRef ] [ Green Version ]
- Chen, T.; Huang, Z.; Wang, L.; Wang, Y.; Wu, F.; Meng, S.; Wang, C. MicroRNA-125a-5p partly regulates the inflammatory response, lipid uptake, and ORP9 expression in oxLDL-stimulated monocyte/macrophages. Cardiovasc. Res. 2009 , 83 , 131–139. [ Google Scholar ] [ CrossRef ] [ PubMed ] [ Green Version ]
- Andreou, I.; Sun, X.; Stone, P.H.; Edelman, E.R.; Feinberg, M.W. miRNAs in atherosclerotic plaque initiation, progression, and rupture. Trends Mol. Med. 2015 , 21 , 307–318. [ Google Scholar ] [ CrossRef ]
- Wu, W.; Xiao, H.; Laguna-Fernandez, A.; Villarreal, G., Jr.; Wang, K.-C.; Geary, G.G.; Zhang, Y.; Wang, W.-C.; Huang, H.-D.; Zhou, J.; et al. Flow-Dependent Regulation of Krüppel-Like Factor 2 Is Mediated by MicroRNA-92a. Circulation 2011 , 124 , 633–641. [ Google Scholar ] [ CrossRef ] [ PubMed ] [ Green Version ]
- Loyer, X.; Potteaux, S.; Vion, A.-C.; Guérin, C.L.; Boulkroun, S.; Rautou, P.-E.; Ramkhelawon, B.; Esposito, B.; Dalloz, M.; Paul, J.-L.; et al. Inhibition of MicroRNA-92a Prevents Endothelial Dysfunction and Atherosclerosis in Mice. Circ. Res. 2014 , 114 , 434–443. [ Google Scholar ] [ CrossRef ] [ Green Version ]
- Hsu, P.; Lin, W.; Lin, R.; Juo, S.H. MicroRNA let-7g inhibits angiotensin II-induced endothelial senescence via the LOX-1-independent mechanism. Int. J. Mol. Med. 2018 , 41 , 2243–2251. [ Google Scholar ] [ CrossRef ] [ Green Version ]
- Yang, S.; Mi, X.; Chen, Y.; Feng, C.; Hou, Z.; Hui, R.; Zhang, W. MicroRNA-216a induces endothelial senescence and inflammation via Smad3/IκBα pathway. J. Cell. Mol. Med. 2018 , 22 , 2739–2749. [ Google Scholar ] [ CrossRef ]
- Chaudhuri, A.A.; So, A.Y.-L.; Sinha, N.; Gibson, W.S.J.; Taganov, K.D.; O’Connell, R.M.; Baltimore, D. MicroRNA-125b Potentiates Macrophage Activation. J. Immunol. 2011 , 187 , 5062–5068. [ Google Scholar ] [ CrossRef ]
- Wei, Y.; Nazari-Jahantigh, M.; Chan, L.; Zhu, M.; Heyll, K.; Corbalán-Campos, J.; Hartmann, P.; Thiemann, A.; Weber, C.; Schober, A. The microRNA-342-5p Fosters Inflammatory Macrophage Activation Through an Akt1- and microRNA-155–Dependent Pathway during Atherosclerosis. Circulation 2013 , 127 , 1609–1619. [ Google Scholar ] [ CrossRef ] [ Green Version ]
- Price, N.L.; Goedeke, L.; Suárez, Y.; Fernández-Hernando, C. miR-33 in cardiometabolic diseases: Lessons learned from novel animal models and approaches. EMBO Mol. Med. 2021 , 13 , e12606. [ Google Scholar ] [ CrossRef ] [ PubMed ]
- Alshanwani, A.R.; Riches-Suman, K.; O’Regan, D.J.; Wood, I.C.; Turner, N.; Porter, K.E. MicroRNA-21 drives the switch to a synthetic phenotype in human saphenous vein smooth muscle cells. IUBMB Life 2018 , 70 , 649–657. [ Google Scholar ] [ CrossRef ] [ PubMed ]
- Liu, X.; Cheng, Y.; Zhang, S.; Lin, Y.; Yang, J.; Zhang, C. A Necessary Role of miR-221 and miR-222 in Vascular Smooth Muscle Cell Proliferation and Neointimal Hyperplasia. Circ. Res. 2009 , 104 , 476–487. [ Google Scholar ] [ CrossRef ] [ PubMed ] [ Green Version ]
- Leeper, N.J.; Raiesdana, A.; Kojima, Y.; Chun, H.; Azuma, J.; Maegdefessel, L.; Kundu, R.K.; Quertermous, T.; Tsao, P.S.; Spin, J.M. MicroRNA-26a is a novel regulator of vascular smooth muscle cell function. J. Cell. Physiol. 2011 , 226 , 1035–1043. [ Google Scholar ] [ CrossRef ] [ PubMed ] [ Green Version ]
- Torella, D.; Iaconetti, C.; Catalucci, D.; Ellison, G.; Leone, A.; Waring, C.D.; Bochicchio, A.; Vicinanza, C.; Aquila, I.; Curcio, A.; et al. MicroRNA-133 Controls Vascular Smooth Muscle Cell Phenotypic Switch In Vitro and Vascular Remodeling In Vivo. Circ. Res. 2011 , 109 , 880–893. [ Google Scholar ] [ CrossRef ] [ Green Version ]
- Talasila, A.; Yu, H.; Ackers-Johnson, M.; Bot, M.; Van Berkel, T.; Bennett, M.R.; Bot, I.; Sinha, S. Myocardin Regulates Vascular Response to Injury Through miR-24/-29a and Platelet-Derived Growth Factor Receptor-β. Arterioscler. Thromb. Vasc. Biol. 2013 , 33 , 2355–2365. [ Google Scholar ] [ CrossRef ] [ Green Version ]
- Li, P.; Zhu, N.; Yi, B.; Wang, N.; Chen, M.; You, X.; Zhao, X.; Solomides, C.C.; Qin, Y.; Sun, J. MicroRNA-663 Regulates Human Vascular Smooth Muscle Cell Phenotypic Switch and Vascular Neointimal Formation. Circ. Res. 2013 , 113 , 1117–1127. [ Google Scholar ] [ CrossRef ] [ Green Version ]
- Xu, F.; Ahmed, A.; Kang, X.; Hu, G.; Liu, F.; Zhang, W.; Zhou, J. MicroRNA-15b/16 Attenuates Vascular Neointima Formation by Promoting the Contractile Phenotype of Vascular Smooth Muscle Through Targeting YAP. Arterioscler. Thromb. Vasc. Biol. 2015 , 35 , 2145–2152. [ Google Scholar ] [ CrossRef ] [ Green Version ]
- Ouimet, M.; Ediriweera, H.N.; Gundra, U.M.; Sheedy, F.; Ramkhelawon, B.; Hutchison, S.B.; Rinehold, K.; Van Solingen, C.; Fullerton, M.D.; Cecchini, K.; et al. MicroRNA-33–dependent regulation of macrophage metabolism directs immune cell polarization in atherosclerosis. J. Clin. Investig. 2015 , 125 , 4334–4348. [ Google Scholar ] [ CrossRef ] [ Green Version ]
- Stary, H.C.; Blankenhorn, D.H.; Chandler, A.B.; Glagov, S.; Insull, W.; Richardson, M.; E Rosenfeld, M.; A Schaffer, S.; Schwartz, C.J.; Wagner, W.D. A definition of the intima of human arteries and of its atherosclerosis-prone regions. A report from the Committee on Vascular Lesions of the Council on Arteriosclerosis, American Heart Association. Circulation 1992 , 85 , 391–405. [ Google Scholar ] [ CrossRef ] [ Green Version ]
- Fuster, V.; Moreno, P.R.; Fayad, Z.A.; Corti, R.; Badimon, J.J. Atherothrombosis and High-Risk Plaque: Part I: Evolving Concepts. J. Am. Coll. Cardiol. 2005 , 46 , 937–954. [ Google Scholar ] [ CrossRef ] [ Green Version ]
- Chen, Y.-C.; Bui, A.V.; Diesch, J.; Manasseh, R.; Hausding, C.; Rivera, J.; Haviv, I.; Agrotis, A.; Htun, N.M.; Jowett, J.; et al. A Novel Mouse Model of Atherosclerotic Plaque Instability for Drug Testing and Mechanistic/Therapeutic Discoveries Using Gene and MicroRNA Expression Profiling. Circ. Res. 2013 , 113 , 252–265. [ Google Scholar ] [ CrossRef ] [ Green Version ]
- Son, D.J.; Kumar, S.; Takabe, W.; Kim, C.W.; Ni, C.-W.; Alberts-Grill, N.; Jang, I.-H.; Kim, S.; Kim, W.; Kang, S.W.; et al. The atypical mechanosensitive microRNA-712 derived from pre-ribosomal RNA induces endothelial inflammation and atherosclerosis. Nat. Commun. 2013 , 4 , 3000. [ Google Scholar ] [ CrossRef ] [ PubMed ]
- Shi, L.; Yu, C.; Tian, X.; Ma, C.; Wang, L.; Xia, D.; Cui, C.; Chen, X.; Jiang, T.; Gu, Y.; et al. Effect of microRNA-133a-3p/matrix metalloproteinase-9 axis on the growth of atherosclerotic vascular smooth muscle cells. Exp. Ther. Med. 2019 . [ Google Scholar ] [ CrossRef ] [ PubMed ]
- Wezel, A.; Welten, S.M.J.; Razawy, W.; Lagraauw, H.M.; de Vries, M.R.; Goossens, E.A.C.; Boonstra, M.C.; Hamming, J.F.; Kandimalla, E.R.; Kuiper, J.; et al. Inhibition of MicroRNA-494 Reduces Carotid Artery Atherosclerotic Lesion Development and Increases Plaque Stability. Ann. Surg. 2015 , 262 , 841–848. [ Google Scholar ] [ CrossRef ] [ PubMed ]
- Ma, F.; Xu, S.; Liu, X.; Zhang, Q.; Xu, X.; Liu, M.; Hua, M.; Li, N.; Yao, H.; Cao, X. The microRNA miR-29 controls innate and adaptive immune responses to intracellular bacterial infection by targeting interferon-γ. Nat. Immunol. 2011 , 12 , 861–869. [ Google Scholar ] [ CrossRef ]
- Boon, R.A.; Seeger, T.; Heydt, S.; Fischer, A.; Hergenreider, E.; Horrevoets, A.J.; Vinciguerra, M.; Rosenthal, N.; Sciacca, S.; Pilato, M.; et al. MicroRNA-29 in Aortic Dilation: Implications for Aneurysm Formation. Circ. Res. 2011 , 109 , 1115–1119. [ Google Scholar ] [ CrossRef ] [ Green Version ]
- Das, A.; Ganesh, K.; Khanna, S.; Sen, C.K.; Roy, S. Engulfment of Apoptotic Cells by Macrophages: A Role of MicroRNA-21 in the Resolution of Wound Inflammation. J. Immunol. 2014 , 192 , 1120–1129. [ Google Scholar ] [ CrossRef ] [ Green Version ]
- Haneklaus, M.; Gerlic, M.; Kurowska-Stolarska, M.; Rainey, A.-A.; Pich, D.; McInnes, I.B.; Hammerschmidt, W.; O’Neill, L.A.J.; Masters, S.L. Cutting Edge: miR-223 and EBV miR-BART15 Regulate the NLRP3 Inflammasome and IL-1β Production. J. Immunol. 2012 , 189 , 3795–3799. [ Google Scholar ] [ CrossRef ] [ Green Version ]
- Ghorpade, D.S.; Leyland, R.; Kurowska-Stolarska, M.; Patil, S.A.; Balaji, K.N. MicroRNA-155 Is Required for Mycobacterium bovis BCG-Mediated Apoptosis of Macrophages. Mol. Cell. Biol. 2012 , 32 , 2239–2253. [ Google Scholar ] [ CrossRef ] [ Green Version ]
- Gao, Y.; Peng, J.; Ren, Z.; He, N.-Y.; Li, Q.; Zhao, X.-S.; Wang, M.-M.; Wen, H.-Y.; Tang, Z.-H.; Jiang, Z.-S.; et al. Functional regulatory roles of microRNAs in atherosclerosis. Clin. Chim. Acta 2016 , 460 , 164–171. [ Google Scholar ] [ CrossRef ]
- Santulli, G.; Wronska, A.; Uryu, K.; Diacovo, T.G.; Gao, M.; Marx, S.O.; Kitajewski, J.; Chilton, J.M.; Akat, K.M.; Tuschl, T.; et al. A selective microRNA-based strategy inhibits restenosis while preserving endothelial function. J. Clin. Investig. 2014 , 124 , 4102–4114. [ Google Scholar ] [ CrossRef ] [ PubMed ]
- Cordes, K.R.; Sheehy, N.T.; White, M.P.; Berry, E.C.; Morton, S.U.; Muth, A.N.; Lee, T.-H.; Miano, J.M.; Ivey, K.N.; Srivastava, D. miR-145 and miR-143 regulate smooth muscle cell fate and plasticity. Nature 2009 , 460 , 705–710. [ Google Scholar ] [ CrossRef ] [ PubMed ] [ Green Version ]
- Avgeris, M.; Mavridis, K.; Tokas, T.; Stravodimos, K.; Fragoulis, E.G.; Scorilas, A. Uncovering the clinical utility of miR-143, miR-145 and miR-224 for predicting the survival of bladder cancer patients following treatment. Carcinogenesis 2015 , 36 , 528–537. [ Google Scholar ] [ CrossRef ] [ PubMed ] [ Green Version ]
- Engelhardt, S.; Leierseder, S. Coinciding functions for miR-145 in vascular smooth muscle and cardiac fibroblasts. J. Mol. Cell. Cardiol. 2013 , 65 , 105–107. [ Google Scholar ] [ CrossRef ] [ PubMed ]
- Elia, L.; Quintavalle, M.; Zhang, J.; Contu, R.; Cossu, L.; Latronico, M.V.G.; Peterson, K.L.; Indolfi, C.; Catalucci, D.; Chen, J.; et al. The knockout of miR-143 and -145 alters smooth muscle cell maintenance and vascular homeostasis in mice: Correlates with human disease. Cell Death Differ. 2009 , 16 , 1590–1598. [ Google Scholar ] [ CrossRef ]
- Climent, M.; Quintavalle, M.; Miragoli, M.; Chen, J.; Condorelli, G.; Elia, L. TGFβ Triggers miR-143/145 Transfer From Smooth Muscle Cells to Endothelial Cells, Thereby Modulating Vessel Stabilization. Circ. Res. 2015 , 116 , 1753–1764. [ Google Scholar ] [ CrossRef ] [ Green Version ]
- Wang, S.; Aurora, A.B.; Johnson, B.A.; Qi, X.; McAnally, J.; Hill, J.A.; Richardson, J.A.; Bassel-Duby, R.; Olson, E.N. The Endothelial-Specific MicroRNA miR-126 Governs Vascular Integrity and Angiogenesis. Dev. Cell 2008 , 15 , 261–271. [ Google Scholar ] [ CrossRef ] [ Green Version ]
- Feng, X.; Tan, W.; Cheng, S.; Wang, H.; Ye, S.; Yu, C.; He, Y.; Zeng, J.; Cen, J.; Hu, J.; et al. Upregulation of microRNA-126 in Hepatic Stellate Cells May Affect Pathogenesis of Liver Fibrosis Through the NF-κB Pathway. DNA Cell Biol. 2015 , 34 , 470–480. [ Google Scholar ] [ CrossRef ]
- Rohde, J.-H.; Weigand, J.E.; Suess, B.; Dimmeler, S. A Universal Aptamer Chimera for the Delivery of Functional microRNA-126. Nucleic Acid Ther. 2015 , 25 , 141–151. [ Google Scholar ] [ CrossRef ]
- Shibayama, Y.; Kondo, T.; Ohya, H.; Fujisawa, S.-I.; Teshima, T.; Iseki, K. Upregulation of microRNA-126-5p is associated with drug resistance to cytarabine and poor prognosis in AML patients. Oncol. Rep. 2015 , 33 , 2176–2182. [ Google Scholar ] [ CrossRef ] [ Green Version ]
- Hu, J.; Zeng, L.; Huang, J.; Wang, G.; Lu, H. miR-126 promotes angiogenesis and attenuates inflammation after contusion spinal cord injury in rats. Brain Res. 2015 , 1608 , 191–202. [ Google Scholar ] [ CrossRef ] [ PubMed ] [ Green Version ]
- Nakano, M.; Fukushima, Y.; Yokota, S.-I.; Fukami, T.; Takamiya, M.; Aoki, Y.; Yokoi, T.; Nakajima, M. CYP2A7 Pseudogene Transcript Affects CYP2A6 Expression in Human Liver by Acting as a Decoy for miR-126*. Drug Metab. Dispos. 2015 , 43 , 703–712. [ Google Scholar ] [ CrossRef ] [ PubMed ] [ Green Version ]
- Meng, Q.; Wang, W.; Yu, X.; Li, W.; Kong, L.; Qian, A.; Li, C.; Li, X. Upregulation of MicroRNA-126 Contributes to Endothelial Progenitor Cell Function in Deep Vein Thrombosis via Its Target PIK3R2. J. Cell. Biochem. 2015 , 116 , 1613–1623. [ Google Scholar ] [ CrossRef ] [ PubMed ]
- Mocharla, P.; Briand, S.; Giannotti, G.; Dörries, C.; Jakob, P.; Paneni, F.; Lüscher, T.; Landmesser, U. AngiomiR-126 expression and secretion from circulating CD34+ and CD14+ PBMCs: Role for proangiogenic effects and alterations in type 2 diabetics. Blood 2013 , 121 , 226–236. [ Google Scholar ] [ CrossRef ] [ PubMed ] [ Green Version ]
- Novák, J.; Vašků, J.B.; Souček, M. Long non-coding RNAs in the pathophysiology of atherosclerosis. Vnitřní Lékařství 2018 , 64 , 77–82. [ Google Scholar ] [ CrossRef ]
- Sallam, T.; Jones, M.; Thomas, B.J.; Wu, X.; Gilliland, T.; Qian, K.; Eskin, A.; Casero, D.; Zhang, Z.; Sandhu, J.; et al. Transcriptional regulation of macrophage cholesterol efflux and atherogenesis by a long noncoding RNA. Nat. Med. 2018 , 24 , 304–312. [ Google Scholar ] [ CrossRef ]
- Arslan, S.; Berkan, Ö.; Lalem, T.; Özbilüm, N.; Göksel, S.; Korkmaz, Ö; Çetin, N.; Devaux, Y. Long non-coding RNAs in the atherosclerotic plaque. Atherosclerosis 2017 , 266 , 176–181. [ Google Scholar ] [ CrossRef ]
- Huangfu, N.; Xu, Z.; Zheng, W.; Wang, Y.; Cheng, J.; Chen, X. LncRNA MALAT1 regulates oxLDL-induced CD36 expression via activating β-catenin. Biochem. Biophys. Res. Commun. 2018 , 495 , 2111–2117. [ Google Scholar ] [ CrossRef ]
- Hennessy, E.J. Cardiovascular Disease and Long Noncoding RNAs. Circ. Cardiovasc. Genet. 2017 , 10 , e001556. [ Google Scholar ] [ CrossRef ]
- Hennessy, E.J.; Van Solingen, C.; Scacalossi, K.R.; Ouimet, M.; Afonso, M.; Prins, J.; Koelwyn, G.J.; Sharma, M.; Ramkhelawon, B.; Carpenter, S.; et al. The long noncoding RNA CHROME regulates cholesterol homeostasis in primates. Nat. Metab. 2019 , 1 , 98–110. [ Google Scholar ] [ CrossRef ]
- Lu, W.; He, X.; Su, L.; Miao, J. Long Noncoding RNA-CERNA1 Stabilized Atherosclerotic Plaques in apolipoprotein E−/− Mice. J. Cardiovasc. Transl. Res. 2019 , 12 , 425–434. [ Google Scholar ] [ CrossRef ] [ PubMed ]
- Haemmig, S.; Yang, D.; Sun, X.; Das, D.; Ghaffari, S.; Molinaro, R.; Chen, L.; Deng, Y.; Freeman, D.; Moullan, N.; et al. Long noncoding RNA SNHG12 integrates a DNA-PK–mediated DNA damage response and vascular senescence. Sci. Transl. Med. 2020 , 12 , eaaw1868. [ Google Scholar ] [ CrossRef ] [ PubMed ]
- Hu, Y.-W.; Guo, F.-X.; Xu, Y.-J.; Li, P.; Lu, Z.-F.; McVey, D.G.; Zheng, L.; Wang, Q.; Ye, J.; Kang, C.-M.; et al. Long noncoding RNA NEXN-AS1 mitigates atherosclerosis by regulating the actin-binding protein NEXN. J. Clin. Investig. 2019 , 129 , 1115–1128. [ Google Scholar ] [ CrossRef ] [ PubMed ]
- Leisegang, M.S.; Bibli, S.-I.; Günther, S.; Pflüger-Müller, B.; A Oo, J.; Höper, C.; Seredinski, S.; Yekelchyk, M.; Schmitz-Rixen, T.; Schürmann, C.; et al. Pleiotropic effects of laminar flow and statins depend on the Krüppel-like factor-induced lncRNA MANTIS. Eur. Heart J. 2019 , 40 , 2523–2533. [ Google Scholar ] [ CrossRef ] [ Green Version ]
- Bell, R.D.; Long, X.; Lin, M.; Bergmann, J.H.; Nanda, V.; Cowan, S.L.; Zhou, Q.; Han, Y.; Spector, D.L.; Zheng, D.; et al. Identification and Initial Functional Characterization of a Human Vascular Cell–Enriched Long Noncoding RNA. Arterioscler. Thromb. Vasc. Biol. 2014 , 34 , 1249–1259. [ Google Scholar ] [ CrossRef ] [ Green Version ]
- Boulberdaa, M.; Scott, E.; Ballantyne, M.; Garcia, R.; Descamps, B.; Angelini, G.; Brittan, M.; Hunter, A.; McBride, M.; McClure, J.; et al. A Role for the Long Noncoding RNA SENCR in Commitment and Function of Endothelial Cells. Mol. Ther. 2016 , 24 , 978–990. [ Google Scholar ] [ CrossRef ] [ Green Version ]
- Zhang, Y.; Zheng, L.; Xu, B.-M.; Tang, W.-H.; Ye, Z.-D.; Huang, C.; Ma, X.; Zhao, J.-J.; Guo, F.-X.; Kang, C.-M.; et al. LncRNA-RP11-714G18.1 suppresses vascular cell migration via directly targeting LRP2BP. Immunol. Cell Biol. 2018 , 96 , 175–189. [ Google Scholar ] [ CrossRef ]
- Ballantyne, M.D.; Pinel, K.; Dakin, R.; Vesey, A.T.; Diver, L.; MacKenzie, R.; Garcia, R.; Welsh, P.; Sattar, N.; Hamilton, G.; et al. Smooth Muscle Enriched Long Noncoding RNA (SMILR) Regulates Cell Proliferation. Circulation 2016 , 133 , 2050–2065. [ Google Scholar ] [ CrossRef ]
- Lu, Y.-B.; Shi, C.; Yang, B.; Lu, Z.-F.; Wu, Y.-L.; Zhang, R.-Y.; He, X.; Li, L.-M.; Hu, B.; Hu, Y.-W.; et al. Long noncoding RNA ZNF800 suppresses proliferation and migration of vascular smooth muscle cells by upregulating PTEN and inhibiting AKT/mTOR/HIF-1α signaling. Atherosclerosis 2020 , 312 , 43–53. [ Google Scholar ] [ CrossRef ]
- Shan, K.; Jiang, Q.; Wang, X.Q.; Wang, Y.N.Z.; Yang, H.; Yao, M.D.; Liu, C.; Li, X.M.; Yao, J.; Liu, B.; et al. Role of long non-coding RNA-RNCR3 in atherosclerosis-related vascular dysfunction. Cell Death Dis. 2016 , 7 , e2248. [ Google Scholar ] [ CrossRef ]
- Wu, G.; Cai, J.; Han, Y.; Chen, J.; Huang, Z.-P.; Chen, C.; Cai, Y.; Huang, H.; Yang, Y.; Liu, Y.; et al. LincRNA-p21 Regulates Neointima Formation, Vascular Smooth Muscle Cell Proliferation, Apoptosis, and Atherosclerosis by Enhancing p53 Activity. Circulation 2014 , 130 , 1452–1465. [ Google Scholar ] [ CrossRef ] [ PubMed ] [ Green Version ]
- Khyzha, N.; Khor, M.; DiStefano, P.V.; Wang, L.; Matic, L.; Hedin, U.; Wilson, M.D.; Maegdefessel, L.; Fish, J.E. Regulation of CCL2 expression in human vascular endothelial cells by a neighboring divergently transcribed long noncoding RNA. Proc. Natl. Acad. Sci. USA 2019 , 116 , 16410–16419. [ Google Scholar ] [ CrossRef ] [ PubMed ] [ Green Version ]
- Weber, D.G.; Casjens, S.; Brik, A.; Raiko, I.; Lehnert, M.; Taeger, D.; Gleichenhagen, J.; Kollmeier, J.; Bauer, T.T.; Brüning, T.; et al. Circulating long non-coding RNA GAS5 (growth arrest-specific transcript 5) as a complement marker for the detection of malignant mesothelioma using liquid biopsies. Biomark. Res. 2020 , 8 , 15. [ Google Scholar ] [ CrossRef ] [ PubMed ]
- Ye, J.; Wang, C.; Wang, D.; Yuan, H. LncRBA GSA5, up-regulated by ox-LDL, aggravates inflammatory response and MMP expression in THP-1 macrophages by acting like a sponge for miR-221. Exp. Cell Res. 2018 , 369 , 348–355. [ Google Scholar ] [ CrossRef ]
- Zhong, X.; Ma, X.; Zhang, L.; Li, Y.; Li, Y.; He, R. MIAT promotes proliferation and hinders apoptosis by modulating miR-181b/STAT3 axis in ox-LDL-induced atherosclerosis cell models. Biomed. Pharmacother. 2018 , 97 , 1078–1085. [ Google Scholar ] [ CrossRef ]
- Li, H.; Liu, X.; Zhang, L.; Li, X. LncRNA BANCR facilitates vascular smooth muscle cell proliferation and migration through JNK pathway. Oncotarget 2017 , 8 , 114568–114575. [ Google Scholar ] [ CrossRef ] [ Green Version ]
- Holdt, L.M.; Beutner, F.; Scholz, M.; Gielen, S.; Gäbel, G.; Bergert, H.; Schuler, G.; Thiery, J.; Teupser, D. ANRIL Expression Is Associated With Atherosclerosis Risk at Chromosome 9p21. Arterioscler. Thromb. Vasc. Biol. 2010 , 30 , 620–627. [ Google Scholar ] [ CrossRef ] [ Green Version ]
- Holdt, L.M.; Teupser, D. Long Noncoding RNA ANRIL: Lnc-ing Genetic Variation at the Chromosome 9p21 Locus to Molecular Mechanisms of Atherosclerosis. Front. Cardiovasc. Med. 2018 , 5 , 145. [ Google Scholar ] [ CrossRef ]
- Holdt, L.M.; Stahringer, A.; Sass, K.; Pichler, G.; Kulak, N.A.; Wilfert, W.; Kohlmaier, A.; Herbst, A.; Northoff, B.H.; Nicolaou, A.; et al. Circular non-coding RNA ANRIL modulates ribosomal RNA maturation and atherosclerosis in humans. Nat. Commun. 2016 , 7 , 12429. [ Google Scholar ] [ CrossRef ] [ Green Version ]
- Sonnenburg, J.L.; Bäckhed, F. Diet–microbiota interactions as moderators of human metabolism. Nature 2016 , 535 , 56–64. [ Google Scholar ] [ CrossRef ]
- Gilbert, J.A.; Quinn, R.A.; Debelius, J.; Xu, Z.Z.; Morton, J.; Garg, N.; Jansson, J.K.; Dorrestein, P.C.; Knight, R. Microbiome-wide association studies link dynamic microbial consortia to disease. Nature 2016 , 535 , 94–103. [ Google Scholar ] [ CrossRef ] [ PubMed ]
- Karlsson, F.; Fåk, F.; Nookaew, I.; Tremaroli, V.; Fagerberg, B.; Petranovic, D.; Bäckhed, F.; Nielsen, J. Symptomatic atherosclerosis is associated with an altered gut metagenome. Nat. Commun. 2012 , 3 , 1245. [ Google Scholar ] [ CrossRef ] [ PubMed ] [ Green Version ]
- Jonsson, A.L.; Bäckhed, F. Role of gut microbiota in atherosclerosis. Nat. Rev. Cardiol. 2017 , 14 , 79–87. [ Google Scholar ] [ CrossRef ] [ PubMed ]
- Sharma, V.; Aggarwal, A. Helicobacter pylori: Does it add to risk of coronary artery disease. World J. Cardiol. 2015 , 7 , 19–25. [ Google Scholar ] [ CrossRef ]
- Shen, X.; Li, L.; Sun, Z.; Zang, G.; Zhang, L.; Shao, C.; Wang, Z. Gut Microbiota and Atherosclerosis—Focusing on the Plaque Stability. Front. Cardiovasc. Med. 2021 , 8 , 668532. [ Google Scholar ] [ CrossRef ]
- Clemente, J.C.; Ursell, L.K.; Parfrey, L.W.; Knight, R. The Impact of the Gut Microbiota on Human Health: An Integrative View. Cell 2012 , 148 , 1258–1270. [ Google Scholar ] [ CrossRef ] [ Green Version ]
- Li, J.; Jia, H.; Cai, X.; Zhong, H.; Feng, Q.; Sunagawa, S.; Arumugam, M.; Kultima, J.R.; Prifti, E.; Nielsen, T.; et al. An integrated catalog of reference genes in the human gut microbiome. Nat. Biotechnol. 2014 , 32 , 834–841. [ Google Scholar ] [ CrossRef ]
- Tang, W.W.; Kitai, T.; Hazen, S.L. Gut Microbiota in Cardiovascular Health and Disease. Circ. Res. 2017 , 120 , 1183–1196. [ Google Scholar ] [ CrossRef ] [ Green Version ]
- Yamashiro, K.; Tanaka, R.; Urabe, T.; Ueno, Y.; Yamashiro, Y.; Nomoto, K.; Takahashi, T.; Tsuji, H.; Asahara, T.; Hattori, N. Gut dysbiosis is associated with metabolism and systemic inflammation in patients with ischemic stroke. PLoS ONE 2017 , 12 , e0171521, Correction in PLoS ONE 2017 , 12 , e0176062. [ Google Scholar ] [ CrossRef ]
- Cani, P.D.; Bibiloni, R.; Knauf, C.; Waget, A.; Neyrinck, A.M.; Delzenne, N.M.; Burcelin, R. Changes in Gut Microbiota Control Metabolic Endotoxemia-Induced Inflammation in High-Fat Diet-Induced Obesity and Diabetes in Mice. Diabetes 2008 , 57 , 1470–1481. [ Google Scholar ] [ CrossRef ] [ Green Version ]
- Eshghjoo, S.; Jayaraman, A.; Sun, Y.; Alaniz, R.C. Microbiota-Mediated Immune Regulation in Atherosclerosis. Molecules 2021 , 26 , 179. [ Google Scholar ] [ CrossRef ] [ PubMed ]
- He, Z.; Chen, Z.-Y. What Are Missing Parts in the Research Story of Trimethylamine-N-oxide (TMAO)? J. Agric. Food Chem. 2017 , 65 , 5227–5228. [ Google Scholar ] [ CrossRef ]
- Sun, X.; Jiao, X.; Ma, Y.; Liu, Y.; Zhang, L.; He, Y.; Chen, Y. Trimethylamine N-oxide induces inflammation and endothelial dysfunction in human umbilical vein endothelial cells via activating ROS-TXNIP-NLRP3 inflammasome. Biochem. Biophys. Res. Commun. 2016 , 481 , 63–70. [ Google Scholar ] [ CrossRef ] [ PubMed ]
- Wang, Z.; Klipfell, E.; Bennett, B.J.; Koeth, R.; Levison, B.S.; DuGar, B.; Feldstein, A.E.; Britt, E.B.; Fu, X.; Chung, Y.-M.; et al. Gut Flora Metabolism of Phosphatidylcholine Promotes Cardiovascular Disease. Nature 2011 , 472 , 57–63. [ Google Scholar ] [ CrossRef ] [ PubMed ] [ Green Version ]
- Koren, O.; Spor, A.; Felin, J.; Fåk, F.; Stombaugh, J.; Tremaroli, V.; Behre, C.J.; Knight, R.; Fagerberg, B.; Ley, R.E.; et al. Human oral, gut, and plaque microbiota in patients with atherosclerosis. Proc. Natl. Acad. Sci. USA 2010 , 108 , 4592–4598. [ Google Scholar ] [ CrossRef ] [ PubMed ] [ Green Version ]
- De Oliveira, C.; Watt, R.; Hamer, M. Toothbrushing, inflammation, and risk of cardiovascular disease: Results from Scottish Health Survey. BMJ 2010 , 340 , c2451. [ Google Scholar ] [ CrossRef ] [ PubMed ] [ Green Version ]
- Fåk, F.; Tremaroli, V.; Bergström, G.; Bäckhed, F. Oral microbiota in patients with atherosclerosis. Atherosclerosis 2015 , 243 , 573–578. [ Google Scholar ] [ CrossRef ]
- Pietiäinen, M.; Liljestrand, J.M.; Kopra, E.; Pussinen, P.J. Mediators between oral dysbiosis and cardiovascular diseases. Eur. J. Oral Sci. 2018 , 126 , 26–36. [ Google Scholar ] [ CrossRef ] [ Green Version ]
- Yu, E.; Rimm, E.; Qi, L.; Rexrode, K.; Albert, C.; Sun, Q.; Willett, W.C.; Hu, F.B.; Manson, J.E. Diet, Lifestyle, Biomarkers, Genetic Factors, and Risk of Cardiovascular Disease in the Nurses’ Health Studies. Am. J. Public Heal. 2016 , 106 , 1616–1623. [ Google Scholar ] [ CrossRef ]
- Berger, J.S.; Hochman, J.; Lobach, I.; Adelman, M.; Riles, T.S.; Rockman, C. Modifiable risk factor burden and the prevalence of peripheral artery disease in different vascular territories. J. Vasc. Surg. 2013 , 58 , 673–681.e1. [ Google Scholar ] [ CrossRef ] [ Green Version ]
- Perk, J.; De Backer, G.; Gohlke, H.; Graham, I.; Reiner, Z.; Verschuren, M.; Verschuren, M.; Albus, C.; Benlian, P.; Boysen, G.; et al. European Guidelines on cardiovascular disease prevention in clinical practice (version 2012). The Fifth Joint Task Force of the European Society of Cardiology and Other Societies on Cardiovascular Disease Prevention in Clinical Practice (constituted by representatives of nine societies and by invited experts). Eur. Heart J. 2012 , 33 , 1635–1701. [ Google Scholar ] [ CrossRef ] [ PubMed ] [ Green Version ]
- Galicia-Garcia, U.; Benito-Vicente, A.; Jebari, S.; Larrea-Sebal, A.; Siddiqi, H.; Uribe, K.B.; Ostolaza, H.; Martín, C. Pathophysiology of Type 2 Diabetes Mellitus. Int. J. Mol. Sci. 2020 , 21 , 6275. [ Google Scholar ] [ CrossRef ] [ PubMed ]
- Dawber, T.R.; Meadors, G.F.; Moore, F.E., Jr. Epidemiological Approaches to Heart Disease: The Framingham Study. Am. J. Public Health 1951 , 41 , 279–286. [ Google Scholar ] [ CrossRef ] [ PubMed ]
- Yusuf, S.; Hawken, S.; Ôunpuu, S.; Dans, T.; Avezum, A.; Lanas, F.; McQueen, M.; Budaj, A.; Pais, P.; Varigos, J.; et al. Effect of potentially modifiable risk factors associated with myocardial infarction in 52 countries (the INTERHEART study): Case-control study. Lancet 2004 , 364 , 937–952. [ Google Scholar ] [ CrossRef ]
- Aggarwal, N.R.; Patel, H.N.; Mehta, L.S.; Sanghani, R.M.; Lundberg, G.P.; Lewis, S.J.; Mendelson, M.A.; Wood, M.J.; Volgman, A.S.; Mieres, J.H. Sex Differences in Ischemic Heart Disease. Circ. Cardiovasc. Qual. Outcomes 2018 , 11 , e004437. [ Google Scholar ] [ CrossRef ]
- Okunrintemi, V.; Tibuakuu, M.; Virani, S.S.; Sperling, L.S.; Volgman, A.S.; Gulati, M.; Cho, L.; Leucker, T.M.; Blumenthal, R.S.; Michos, E.D. Sex Differences in the Age of Diagnosis for Cardiovascular Disease and Its Risk Factors Among US Adults: Trends From 2008 to 2017, the Medical Expenditure Panel Survey. J. Am. Heart Assoc. 2020 , 9 , e018764. [ Google Scholar ] [ CrossRef ]
- Virani, S.S.; Alonso, A.; Benjamin, E.J.; Bittencourt, M.S.; Callaway, C.W.; Carson, A.P.; Chamberlain, A.M.; Chang, A.R.; Cheng, S.; Delling, F.N.; et al. Heart Disease and Stroke Statistics—2020 Update: A Report from the American Heart Association. Circulation 2020 , 141 , e139–e596. [ Google Scholar ] [ CrossRef ]
- Man, J.J.; Beckman, J.A.; Jaffe, I.Z. Sex as a Biological Variable in Atherosclerosis. Circ. Res. 2020 , 126 , 1297–1319. [ Google Scholar ] [ CrossRef ]
- World Health Organization Statistical Information System 2004. Available online: www.who.int/whosis (accessed on 21 November 2021).
- Ramirez, F.D.; Hibbert, B. Letter by Ramirez and Hibbert Regarding Article, “Consideration of Sex Differences in Design and Reporting of Experimental Arterial Pathology Studies: A Statement from the Arteriosclerosis, Thrombosis, and Vascular Biology council. Arterioscler. Thromb. Vasc. Biol. 2018 , 38 , e99–e100. [ Google Scholar ] [ CrossRef ] [ Green Version ]
- Lu, H.S.; Schmidt, A.M.; Hegele, R.A.; Mackman, N.; Rader, D.J.; Weber, C.; Daugherty, A. Reporting Sex and Sex Differences in Preclinical Studies. Arterioscler. Thromb. Vasc. Biol. 2018 , 38 , e171–e184. [ Google Scholar ] [ CrossRef ] [ Green Version ]
- Lu, H.S.; Schmidt, A.M.; Hegele, R.A.; Mackman, N.; Rader, D.J.; Weber, C.; Daugherty, A. Annual Report on Sex in Preclinical Studies. Arterioscler. Thromb. Vasc. Biol. 2020 , 40 , e1–e9. [ Google Scholar ] [ CrossRef ] [ PubMed ]
- Libby, P.; Buring, J.E.; Badimon, L.; Hansson, G.K.; Deanfield, J.; Bittencourt, M.S.; Tokgözoğlu, L.; Lewis, E.F. Atherosclerosis. Nat. Rev. Dis. Primers 2019 , 5 , 56. [ Google Scholar ] [ CrossRef ] [ PubMed ]
- Sturlaugsdottir, R.; Aspelund, T.; Bjornsdottir, G.; Sigurdsson, S.; Thorsson, B.; Eiriksdottir, G.; Gudnason, V. Prevalence and determinants of carotid plaque in the cross-sectional REFINE-Reykjavik study. BMJ Open 2016 , 6 , e012457. [ Google Scholar ] [ CrossRef ] [ Green Version ]
- Lansky, A.J.; Ng, V.G.; Maehara, A.; Weisz, G.; Lerman, A.; Mintz, G.S.; De Bruyne, B.; Farhat, N.; Niess, G.; Jankovic, I.; et al. Gender and the Extent of Coronary Atherosclerosis, Plaque Composition, and Clinical Outcomes in Acute Coronary Syndromes. JACC Cardiovasc. Imaging 2012 , 5 , S62–S72. [ Google Scholar ] [ CrossRef ] [ PubMed ] [ Green Version ]
- Plank, F.; Beyer, C.; Friedrich, G.; Wildauer, M.; Feuchtner, G. Sex differences in coronary artery plaque composition detected by coronary computed tomography: Quantitative and qualitative analysis. Neth. Heart J. 2019 , 27 , 272–280. [ Google Scholar ] [ CrossRef ] [ Green Version ]
- Kataoka, Y.; Puri, R.; Hammadah, M.; Duggal, B.; Uno, K.; Kapadia, S.R.; Tuzcu, E.M.; Nissen, S.E.; King, P.; Nicholls, S.J. Sex Differences in Nonculprit Coronary Plaque Microstructures on Frequency-Domain Optical Coherence Tomography in Acute Coronary Syndromes and Stable Coronary Artery Disease. Circ. Cardiovasc. Imaging 2016 , 9 , e004506. [ Google Scholar ] [ CrossRef ] [ Green Version ]
- Wendorff, C.; Wendorff, H.; Pelisek, J.; Tsantilas, P.; Zimmermann, A.; Zernecke, A.; Kuehnl, A.; Eckstein, H.-H. Carotid Plaque Morphology Is Significantly Associated With Sex, Age, and History of Neurological Symptoms. Stroke 2015 , 46 , 3213–3219. [ Google Scholar ] [ CrossRef ] [ Green Version ]
- Vrijenhoek, J.E.; Ruijter, H.M.D.; De Borst, G.J.; de Kleijn, D.P.; De Vries, J.-P.P.; Bots, M.L.; Van de Weg, S.M.; Vink, A.; Moll, F.L.; Pasterkamp, G. Sex Is Associated With the Presence of Atherosclerotic Plaque Hemorrhage and Modifies the Relation Between Plaque Hemorrhage and Cardiovascular Outcome. Stroke 2013 , 44 , 3318–3323. [ Google Scholar ] [ CrossRef ] [ Green Version ]
- Halvorsen, D.S.; Johnsen, S.H.; Mathiesen, E.B.; Njølstad, I. The Association between Inflammatory Markers and Carotid Atherosclerosis Is Sex Dependent: The Tromsø Study. Cerebrovasc. Dis. 2009 , 27 , 392–397. [ Google Scholar ] [ CrossRef ]
- Jia, H.; Kubo, T.; Akasaka, T.; Yu, B. Optical Coherence Tomography Guidance in Management of Acute Coronary Syndrome Caused by Plaque Erosion. Circ. J. 2018 , 82 , 302–308. [ Google Scholar ] [ CrossRef ] [ Green Version ]
- Sato, T.; Minami, Y.; Asakura, K.; Katamine, M.; Kato, A.; Katsura, A.; Muramatsu, Y.; Kakizaki, R.; Nemoto, T.; Hashimoto, T.; et al. Age- and Gender-Related Differences in Coronary Lesion Plaque Composition on Optical Coherence Tomography. Circ. J. 2020 , 84 , 463–470. [ Google Scholar ] [ CrossRef ] [ PubMed ] [ Green Version ]
- Salami, J.A.; Warraich, H.; Valero-Elizondo, J.; Spatz, E.S.; Desai, N.R.; Rana, J.S.; Virani, S.S.; Blankstein, R.; Khera, A.; Blaha, M.J.; et al. National Trends in Statin Use and Expenditures in the US Adult Population From 2002 to 2013. JAMA Cardiol. 2017 , 2 , 56–65. [ Google Scholar ] [ CrossRef ] [ PubMed ] [ Green Version ]
- Peterson, E.D.; Delong, E.R.; Masoudi, F.A.; O’Brien, S.M.; Peterson, P.N.; Rumsfeld, J.S.; Shahian, D.M.; Shaw, R.E. ACCF/AHA 2010 Position Statement on Composite Measures for Healthcare Performance Assessment. Circulation 2010 , 121 , 1780–1791. [ Google Scholar ] [ CrossRef ] [ PubMed ]
- Nanna, M.G.; Wang, T.Y.; Xiang, Q.; Goldberg, A.C.; Robinson, J.G.; Roger, V.L.; Virani, S.S.; Wilson, P.W.; Louie, M.J.; Koren, A.; et al. Sex Differences in the Use of Statins in Community Practice. Circ. Cardiovasc. Qual. Outcomes 2019 , 12 , e005562. [ Google Scholar ] [ CrossRef ]
- Tsujita, K.; Sugiyama, S.; Sumida, H.; Shimomura, H.; Yamashita, T.; Yamanaga, K.; Komura, N.; Sakamoto, K.; Oka, H.; Nakao, K.; et al. Impact of Dual Lipid-Lowering Strategy With Ezetimibe and Atorvastatin on Coronary Plaque Regression in Patients With Percutaneous Coronary Intervention. J. Am. Coll. Cardiol. 2015 , 66 , 495–507. [ Google Scholar ] [ CrossRef ] [ Green Version ]
- Dayspring, T.D.; Varvel, S.A.; Ghaedi, L.; Thiselton, D.L.; Bruton, J.; McConnell, J.P. Biomarkers of cholesterol homeostasis in a clinical laboratory database sample comprising 667,718 patients. J. Clin. Lipidol. 2015 , 9 , 807–816. [ Google Scholar ] [ CrossRef ] [ Green Version ]
- Fujino, A.; Mintz, G.S.; Matsumura, M.; Lee, T.; Kim, S.-Y.; Hoshino, M.; Usui, E.; Yonetsu, T.; Haag, E.S.; Shlofmitz, R.A.; et al. A new optical coherence tomography-based calcium scoring system to predict stent underexpansion. EuroIntervention 2018 , 13 , 2182–2189. [ Google Scholar ] [ CrossRef ]
- Maejima, N.; Hibi, K.; Saka, K.; Akiyama, E.; Konishi, M.; Endo, M.; Iwahashi, N.; Tsukahara, K.; Kosuge, M.; Ebina, T.; et al. Relationship Between Thickness of Calcium on Optical Coherence Tomography and Crack Formation After Balloon Dilatation in Calcified Plaque Requiring Rotational Atherectomy. Circ. J. 2016 , 80 , 1413–1419. [ Google Scholar ] [ CrossRef ] [ Green Version ]
- Kubo, T.; Shimamura, K.; Ino, Y.; Yamaguchi, T.; Matsuo, Y.; Shiono, Y.; Taruya, A.; Nishiguchi, T.; Shimokado, A.; Teraguchi, I.; et al. Superficial Calcium Fracture After PCI as Assessed by OCT. JACC Cardiovasc. Imaging 2015 , 8 , 1228–1229. [ Google Scholar ] [ CrossRef ] [ Green Version ]
- Kim, Y.; Wilkens, L.R.; Park, S.-Y.; Goodman, M.T.; Monroe, K.R.; Kolonel, L.N. Association between various sedentary behaviours and all-cause, cardiovascular disease and cancer mortality: The Multiethnic Cohort Study. Int. J. Epidemiol. 2013 , 42 , 1040–1056. [ Google Scholar ] [ CrossRef ] [ Green Version ]
- Meisinger, C.; Heier, M.; Löwel, H.; Schneider, A.; Döring, A. Sleep Duration and Sleep Complaints and Risk of Myocardial Infarction in Middle-aged Men and Women from the General Population: The MONICA/KORA Augsburg Cohort Study. Sleep 2007 , 30 , 1121–1127. [ Google Scholar ] [ CrossRef ] [ PubMed ]
- Pan, B.; Jin, X.; Jun, L.; Qiu, S.; Zheng, Q.; Pan, M. The relationship between smoking and stroke. Medicine 2019 , 98 , e14872. [ Google Scholar ] [ CrossRef ]
- Larsson, S.C.; Burgess, S.; Michaëlsson, K. Smoking and stroke: A mendelian randomization study. Ann. Neurol. 2019 , 86 , 468–471. [ Google Scholar ] [ CrossRef ] [ PubMed ] [ Green Version ]
- National Center for Chronic Disease Prevention and Health Promotion (US); Office on Smoking and Health. The Health Consequences of Smoking—50 Years of Progress: A Report of the Surgeon General ; Reports of the Surgeon General; Centers for Disease Control and Prevention (US): Atlanta, GA, USA, 2014.
- Thun, M.J.; Carter, B.D.; Feskanich, D.; Freedman, N.D.; Prentice, R.; Lopez, A.D.; Hartge, P.; Gapstur, S.M. 50-Year Trends in Smoking-Related Mortality in the United States. N. Engl. J. Med. 2013 , 368 , 351–364. [ Google Scholar ] [ CrossRef ] [ Green Version ]
- Gambardella, J.; Sardu, C.; Sacra, C.; DEL Giudice, C.; Santulli, G. Quit smoking to outsmart atherogenesis: Molecular mechanisms underlying clinical evidence. Atherosclerosis 2017 , 257 , 242–245. [ Google Scholar ] [ CrossRef ]
- Cheezum, M.K.; Kim, A.; Bittencourt, M.S.; Kassop, D.; Nissen, A.; Thomas, D.; Nguyen, B.; Glynn, R.J.; Shah, N.R.; Villines, T.C. Association of tobacco use and cessation with coronary atherosclerosis. Atherosclerosis 2017 , 257 , 201–207. [ Google Scholar ] [ CrossRef ] [ PubMed ]
- Stein, J.H.; Smith, S.S.; Hansen, K.M.; Korcarz, C.E.; Piper, M.E.; Fiore, M.C.; Baker, T.B. Longitudinal effects of smoking cessation on carotid artery atherosclerosis in contemporary smokers: The Wisconsin Smokers Health Study. Atherosclerosis 2020 , 315 , 62–67. [ Google Scholar ] [ CrossRef ]
- Tollefson, A.K.; Oberley-Deegan, R.E.; Butterfield, K.T.; Nicks, M.E.; Weaver, M.R.; Remigio, L.K.; Decsesznak, J.; Chu, H.; Bratton, D.L.; Riches, D.W.; et al. Endogenous enzymes (NOX and ECSOD) regulate smoke-induced oxidative stress. Free Radic. Biol. Med. 2010 , 49 , 1937–1946. [ Google Scholar ] [ CrossRef ] [ Green Version ]
- Collins, T. Endothelial nuclear factor-kappa B and the initiation of the atherosclerotic lesion. Lab. Investig. 1993 , 68 , 499–508. [ Google Scholar ]
- Barbieri, S.S.; Weksler, B.B. Tobacco smoke cooperates with interleukin-1β to alter β-catenin trafficking in vascular endothelium resulting in increased permeability and induction of cyclooxygenase-2 expression in vitro and in vivo. FASEB J. 2007 , 21 , 1831–1843. [ Google Scholar ] [ CrossRef ] [ Green Version ]
- Wang, C.-N.; Yang, G.-H.; Wang, Z.-Q.; Liu, C.-W.; Li, T.-J.; Lai, Z.-C.; Miao, S.-Y.; Wang, L.-F.; Liu, B. Role of perivascular adipose tissue in nicotine-induced endothelial cell inflammatory responses. Mol. Med. Rep. 2016 , 14 , 5713–5718. [ Google Scholar ] [ CrossRef ] [ PubMed ] [ Green Version ]
- Demirjian, L.; Abboud, R.T.; Li, H.; Duronio, V. Acute effect of cigarette smoke on TNF-α release by macrophages mediated through the erk1/2 pathway. Biochim. Et Biophys. Acta (BBA)-Mol. Basis Dis. 2006 , 1762 , 592–597. [ Google Scholar ] [ CrossRef ] [ PubMed ] [ Green Version ]
- Csordas, A.; Bernhard, D. The biology behind the atherothrombotic effects of cigarette smoke. Nat. Rev. Cardiol. 2013 , 10 , 219–230. [ Google Scholar ] [ CrossRef ] [ PubMed ]
- Raveendran, M.; Senthil, D.; Utama, B.; Shen, Y.; Dudley, D.; Wang, J.; Zhang, Y.; Wang, X.L. Cigarette suppresses the expression of P4Hα and vascular collagen production. Biochem. Biophys. Res. Commun. 2004 , 323 , 592–598. [ Google Scholar ] [ CrossRef ] [ PubMed ]
- O’Toole, T.E.; Zheng, Y.-T.; Hellmann, J.; Conklin, D.J.; Barski, O.; Bhatnagar, A. Acrolein activates matrix metalloproteinases by increasing reactive oxygen species in macrophages. Toxicol. Appl. Pharmacol. 2009 , 236 , 194–201. [ Google Scholar ] [ CrossRef ] [ Green Version ]
- Ljungberg, L.U.; Persson, K.; Eriksson, A.C.; Gréen, H.; Whiss, P.A. Effects of nicotine, its metabolites and tobacco extracts on human platelet function in vitro. Toxicol. Vitr. 2013 , 27 , 932–938. [ Google Scholar ] [ CrossRef ] [ Green Version ]
- Feinberg, M.W.; Moore, K.J. MicroRNA Regulation of Atherosclerosis. Circ. Res. 2016 , 118 , 703–720. [ Google Scholar ] [ CrossRef ] [ Green Version ]
- Yokoyama, Y.; Mise, N.; Suzuki, Y.; Tada-Oikawa, S.; Izuoka, K.; Zhang, L.; Zong, C.; Takai, A.; Yamada, Y.; Ichihara, S. MicroRNAs as Potential Mediators for Cigarette Smoking Induced Atherosclerosis. Int. J. Mol. Sci. 2018 , 19 , 1097. [ Google Scholar ] [ CrossRef ] [ Green Version ]
- Zhou, J.; Wang, K.C.; Wu, W.; Subramaniam, S.; Shyy, J.Y.; Chiu, J.J.; Li, J.Y.; Chien, S. MicroRNA-21 targets peroxisome proliferators-activated receptor-α in an autoregulatory loop to modulate flow-induced endothelial inflammation. Proc. Natl. Acad. Sci. USA 2011 , 108 , 10355–10360. [ Google Scholar ] [ CrossRef ] [ Green Version ]
- Bala, S.; Csak, T.; Saha, B.; Zatsiorsky, J.; Kodys, K.; Catalano, D.; Satishchandran, A.; Szabo, G. The pro-inflammatory effects of miR-155 promote liver fibrosis and alcohol-induced steatohepatitis. J. Hepatol. 2016 , 64 , 1378–1387. [ Google Scholar ] [ CrossRef ] [ Green Version ]
- Gu, W.; Yao, L.; Li, L.; Zhang, J.; Place, A.T.; Minshall, R.D.; Liu, G. ICAM-1 regulates macrophage polarization by suppressing MCP-1 expression via miR-124 upregulation. Oncotarget 2017 , 8 , 111882–111901. [ Google Scholar ] [ CrossRef ] [ PubMed ] [ Green Version ]
- Cerutti, C.; Soblechero-Martin, P.; Wu, D.; Lopez-Ramirez, M.A.; De Vries, H.; Sharrack, B.; Male, D.K.; Romero, I.A. MicroRNA-155 contributes to shear-resistant leukocyte adhesion to human brain endothelium in vitro. Fluids Barriers CNS 2016 , 13 , 8. [ Google Scholar ] [ CrossRef ] [ PubMed ] [ Green Version ]
- Deshmane, S.L.; Kremlev, S.; Amini, S.; Sawaya, B.E. Monocyte Chemoattractant Protein-1 (MCP-1): An Overview. J. Interf. Cytokine Res. 2009 , 29 , 313–326. [ Google Scholar ] [ CrossRef ]
- Weber, M.; Baker, M.B.; Moore, J.P.; Searles, C.D. MiR-21 is induced in endothelial cells by shear stress and modulates apoptosis and eNOS activity. Biochem. Biophys. Res. Commun. 2010 , 393 , 643–648. [ Google Scholar ] [ CrossRef ] [ PubMed ] [ Green Version ]
- Liu, Y.; Pan, Q.; Zhao, Y.; He, C.; Bi, K.; Chen, Y.; Zhao, B.; Chen, Y.; Ma, X. MicroRNA-155 Regulates ROS Production, NO Generation, Apoptosis and Multiple Functions of Human Brain Microvessel Endothelial Cells Under Physiological and Pathological Conditions. J. Cell. Biochem. 2015 , 116 , 2870–2881. [ Google Scholar ] [ CrossRef ] [ PubMed ]
Click here to enlarge figure
Location | Upregulated | Downregulated |
---|---|---|
Coronary arteries | miR-29, miR-100, miR-155, miR-199, miR-221, miR-363, miR-497, miR-508 and miR-181 [ , , ]. | miR-1273, miR-490, miR-24 and miR-1284 [ , , ]. |
Aorta, femoral, and carotid arteries | miR-21, miR-34, miR-146 and miR-210 [ ]. Only in carotid plaques: miR-15, miR-26, miR-30, miR-98, miR-125, miR-152, miR-181, miR-100, miR-127, miR-133, miR-145 and miR-422 [ , ]. | Only in carotid plaques: miR-520, miR-105 [ , ]. |
Cell Line | Athero-Protective | Pro-Atherogenic |
---|---|---|
Endothelial cells | miR-155 [ ] miR-10a [ ], miR-31 and miR-17-3p [ ] miR-146a [ ] miR-181b [ ] miR-92a [ , ] miR-let-7g [ ] | miR-216a [ ] |
Macrophages | miR-146a [ ] miR-125a [ ] miR-223 [ ] | miR-125b [ ] miR-342-p [ ] miR-33 [ ] |
VSMCs * | miR-21 [ ] miR-221 and miR-222 [ ] miR-26a [ ] miR-663 [ ] | miR-143, miR-145 and miR-1 [ ] miR-29a and miR-24 [ ] miR-133a [ ] miR-15b and miR-16 [ ] |
Anti-Atherogenic | Pro-Atherogenic |
---|---|
miR-24 [ ] | miR-322 [ ] |
miR-133a [ ] | miR-712 [ ] |
miR-29 [ , ] | miR-494 [ ] |
miR-21 [ ] | miR-155 [ ] |
miR-223 [ ] | miR-365 [ ] |
Athero-Protective | Pro-Atherogenic |
---|---|
MeXis [ ] MALAT1 [ , ] CERNA1 [ ] SNHG12 [ ] NEXN-AS1 [ ] MANTIS [ ] SENCR [ ] RP11-714G18.1 [ ] CHROME [ , ] circANRIL [ ] | SMILR [ ] CCL2 [ ] GAS5 [ , ] MIAT [ , ] BANCR [ ] ANRIL [ , ] |
MDPI stays neutral with regard to jurisdictional claims in published maps and institutional affiliations. |
Share and Cite
Jebari-Benslaiman, S.; Galicia-García, U.; Larrea-Sebal, A.; Olaetxea, J.R.; Alloza, I.; Vandenbroeck, K.; Benito-Vicente, A.; Martín, C. Pathophysiology of Atherosclerosis. Int. J. Mol. Sci. 2022 , 23 , 3346. https://doi.org/10.3390/ijms23063346
Jebari-Benslaiman S, Galicia-García U, Larrea-Sebal A, Olaetxea JR, Alloza I, Vandenbroeck K, Benito-Vicente A, Martín C. Pathophysiology of Atherosclerosis. International Journal of Molecular Sciences . 2022; 23(6):3346. https://doi.org/10.3390/ijms23063346
Jebari-Benslaiman, Shifa, Unai Galicia-García, Asier Larrea-Sebal, Javier Rekondo Olaetxea, Iraide Alloza, Koen Vandenbroeck, Asier Benito-Vicente, and César Martín. 2022. "Pathophysiology of Atherosclerosis" International Journal of Molecular Sciences 23, no. 6: 3346. https://doi.org/10.3390/ijms23063346
Article Metrics
Article access statistics, further information, mdpi initiatives, follow mdpi.
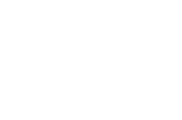
Subscribe to receive issue release notifications and newsletters from MDPI journals
CASE REPORT article
Case report: coronary atherosclerosis in a patient with long-standing very low ldl-c without lipid-lowering therapy.
- 1 Department of Medicine, Section of Cardiovascular Medicine, Yale School of Medicine, New Haven, CT, United States
- 2 Division of Cardiology, Beth Israel Deaconess Medical Center and Harvard Medical School, Boston, MA, United States
- 3 Department of Radiology & Biomedical Imaging, Section of Vascular & Interventional Radiology, Yale School of Medicine, New Haven, CT, United States
Background: ApoB-containing lipoproteins including low-density lipoprotein cholesterol (LDL-C) are necessary for the development of atherosclerosis, and lifelong exposure to low serum levels of LDL-C have been associated with a substantial reduction of cardiovascular risk. Although plaque regression has been observed in patients with serum LDL-C less than 70–80 mg/dl on lipid-lowering therapy, an LDL-C level under which atherosclerosis cannot develop has not been established.
Case presentation: In this case we describe a 60-year-old man with well-controlled diabetes mellitus and hypertension who presented to the hospital after an acute stroke likely due to an atrial myxoma discovered on imaging. A coronary computed tomography angiography scan performed in preparation for the planned surgical myxoma resection revealed an anomalous origin of the right coronary artery as well as evidence of nonobstructive coronary atherosclerosis in the right coronary and non-anomalous left coronary system. Despite not having ever been on any lipid-lowering therapy, this patient was found to have low LDL-C levels (<40 mg/dl) during this admission and on routine laboratory data collected over the prior 16 years. His family history strongly suggested heterozygous familial hypobetalipoproteinemia as a possible diagnosis.
Conclusions: This case illustrates that even long-standing, very low levels of LDL-C may be insufficient to completely prevent atherosclerosis and emphasizes the importance of primordial prevention of all cardiovascular risk factors.
Introduction
While the biological mechanisms underlying the pathogenesis of atherosclerosis involve numerous factors, apolipoprotein B (ApoB)-containing lipoproteins inclusive of low-density lipoprotein cholesterol (LDL-C) are necessary for its development ( 1 ). Mendelian randomization studies and prospective studies of individuals with genetic variants leading to naturally low LDL-C levels have demonstrated that lifelong exposure to low LDL-C results in substantial reductions in risk of atherosclerotic cardiovascular disease (ASCVD) even in the presence of other risk factors ( 2 ). Atherosclerosis detected by coronary calcification can also occur in asymptomatic middle-aged adults without other risk factors and at serum LDL-C levels below 100 mg/dl ( 3 ). Reduction in atherogenic lipoproteins early in life is therefore central to primary prevention of ASCVD, though the serum levels of ApoB or LDL-C at which atherosclerosis fails to develop has not been firmly established and likely varies among individuals. It has been hypothesized that lifelong LDL-C of less than 30 mg/dl would be sufficient to completely prevent atherosclerosis ( 4 ), though studies of patients with established ASCVD have demonstrated plaque regression with serum LDL-C less than 70–80 mg/dl on lipid-lowering therapy ( 5 ).
Case description
A 60-year-old man presented to his local hospital with new-onset weakness, ataxia and paresthesia in the left upper extremity and was found to have an acute ischemic stroke based on MRI imaging showing multiple areas of acute cerebral and cerebellar infarction. A transthoracic echocardiogram was performed which showed a 4.3 × 2.0 cm mass in the left atrium ( Supplementary Video S1 ), consistent with an atrial myxoma which was thought to be the cause of his acute stroke. He was subsequently transferred to a tertiary medical center for further work-up and evaluation for cardiothoracic surgery. Prior to surgery for surgical myxoma resection, inpatient cardiology was consulted for preoperative evaluation. Aside from mild residual weakness, ataxia and paresthesia in his left upper extremity, the patient was asymptomatic and had been physically active. On physical exam, he was noted to have mild weakness of his left upper extremity (4+/5 strength in shoulder abduction) and trace dysmetria of the left hand. His cardiac exam was notable for a low-pitched sound heard early in diastole with no associated murmurs. His physical exam was otherwise unremarkable.
The patient reported being diagnosed with hypertension and type 2 diabetes mellitus approximately 7 years prior to admission. He was currently taking amlodipine and losartan and reported his systolic blood pressure was typically less than 130 mmHg at all recent clinic visits. At the time of diabetes diagnosis, his hemoglobin A1c (HbA1c) was 12.3 and he reported his diet contained high amounts of processed foods, saturated fat, and sugar-sweetened beverages. He was prescribed metformin and subsequently stopped drinking sugar-sweetened beverages, made other healthful changes to his diet and started exercising on a regular basis. He subsequently lost 20 pounds and his HbA1c after one year was markedly improved at 5.0. On admission his HbA1c was 6.2 with a body mass index of 27.9 Kg/m 2 . He was also noted to have hepatic steatosis on an outpatient liver ultrasound prior to admission. He denied ever using tobacco or drugs and rarely consumed alcohol.
On admission the patient's standard lipid panel showed a serum LDL-C of 32 mg/dl and a non-HDL-C of 46 mg/dl. The patient had never been on lipid-lowering therapy and reported being previously told he had naturally very low blood cholesterol levels, which was confirmed on outpatient labs over the prior 16 years ( Table 1 ). He recalled being told his mother and maternal grandmother had very low levels of LDL-C. He had no family history of premature ASCVD. Further testing during his hospitalization revealed an ApoB level of 35 mg/dl, directly measured LDL-C of 48 mg/dl, and lipoprotein(a) of 36 nmol/L.
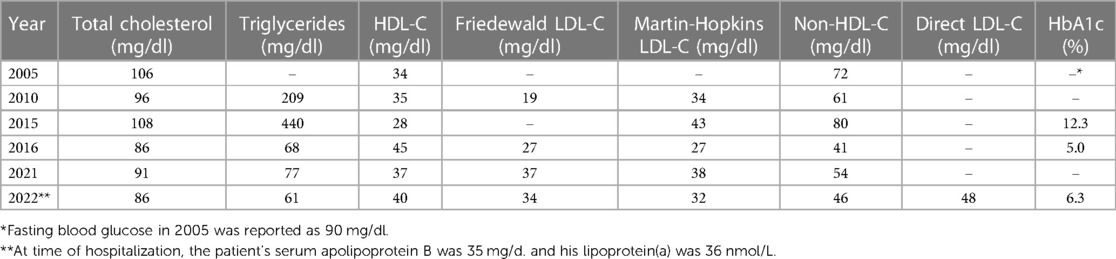
Table 1 . Patient laboratory tests for Serum lipids and HbA1c.
As part of pre-operative planning for cardiac surgery, the patient underwent coronary computed tomography angiography (CCTA) which demonstrated an anomalous right coronary artery (RCA; Figures 1A,B ) at the origin of the left sinus with mild atherosclerotic plaques in the RCA. Mild atherosclerotic plaques were also noted in the left anterior descending artery (LAD) and left circumflex artery (LCx; Figures 1C,D ), with normal LAD and LCx origins. To assess for ischemia due to the anomalous RCA, pharmacologic myocardial perfusion imaging with positron emission tomography was performed which showed a small-sized, mild-intensity, reversible perfusion defect in the apical inferior wall which was thought to be clinically insignificant due to lack of any exertional symptoms.
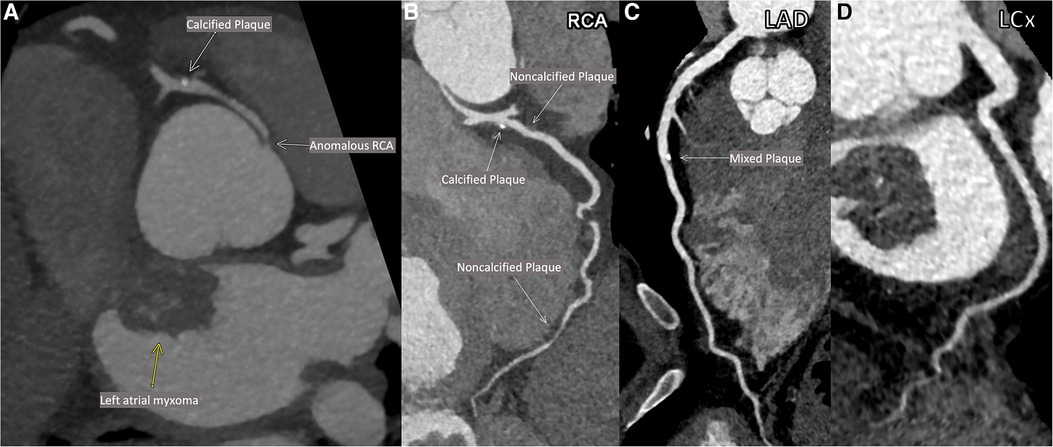
Figure 1 . Patient's coronary computed tomographic angiography (CCTA). Anomalous origin of the right coronary artery (RCA; A ). Multiplanar reconstructions of the RCA ( B ), the left anterior descending artery (LAD; C ) and the left circumflex artery (LCx; D ).
The patient underwent successful robotic-assisted resection of the left atrial mass (confirmed to be a myxoma on pathology) with no intervention performed to the RCA. He was discharged on post-operative day 4 with rosuvastatin and metoprolol. He was also recommended for consideration of outpatient genetic screening for hypobetalipoproteinemia.
Despite chronic and presumably life-long levels of LDL-C typically less than 40 mg/dl, the patient presented here was found to have mild atherosclerosis in multiple coronary arteries by CCTA. Genetic testing was not performed in this patient, though his family history, long-standing low blood cholesterol levels and hepatic steatosis suggest his low LDL-C is likely due to heterozygous familial hypobetalipoproteinemia, a genetic cause of low LDL-C with a ∼1:1000 prevalence in most populations ( 6 ). Patients with familial hypobetalipoproteinemia typically have LDL-C levels between 20 and 50 mg/dl and though the APOB mutations often associated with this condition lead to substantially lower risk of ASCVD ( 7 ), prevalence of coronary atherosclerosis specifically in this population has not been well described.
Importantly, this case demonstrates that coronary atherosclerosis can occur even with long-standing LDL-C less than 40 mg/dl when other risk factors such as hypertension and diabetes are present and otherwise well controlled. Reports of atherosclerosis in individuals with lifelong very low LDL-C are extremely rare ( 8 ). The patient in this instance did have uncontrolled diabetes mellitus on initial diagnosis with a HbA1c of 12.3 in 2015, and due to lack of regular follow-up it is unclear how long that level of hyperglycemia may have occurred and contributed to plaque development. His serum ApoB was not assessed at that time and his LDL-C and non-HDL-C were higher than on all his other laboratory checks, though still at very low levels. Notably, his diabetes rapidly improved with only lifestyle changes and metformin. At the time of hospitalization, the patient's serum ApoB was concordantly low and his lipoprotein(a) level was also low, and therefore these factors are unlikely to explain development of atherosclerosis. There is little evidence available on whether anomalous coronary artery origin directly contributes to atherosclerosis; it has previously been shown that anomalous right-sided arteries with a retroaortic course may develop atherosclerosis earlier than non-anomalous arteries within the same patient. However, the case patient was also observed to have atherosclerosis in the non-anomalous left coronary system ( 9 ). To our knowledge, this is the first case report showing calcified coronary plaque on CCTA in a patient with long-standing LDL-C less than 40–50 mg/dl while not on lipid-lowering therapy.
This case indicates that even lifelong LDL-C at very low levels, as could theoretically be achieved with early initiation of statins and PCSK9 inhibition with medications or gene editing ( 10 – 12 ), may be insufficient to completely prevent atherosclerosis when other risk factors are present. Therefore, primordial prevention of risk factors, rather than only managing them after they have developed, remains critical to reducing prevalence of atherosclerosis and ASCVD across populations. However, atherosclerosis specifically in patients with familial hypobetalipoproteinemia is not well understood, and there may be unique factors that promote development in this population. Given that our observations were made from a single patient, definitive conclusions about coronary plaque development cannot be made from this report. Further study will be needed to determine what specific factors drive development of atherosclerosis in the setting of low LDL-C, including in patients with familial hypobetalipoproteinemia.
Data availability statement
The original contributions presented in the study are included in the article/ Supplementary Material , further inquiries can be directed to the corresponding author.
Ethics statement
Additional written informed consent was not required because we had obtained verbal informed consent from the patient to publish this case report prior to submission. Informed consent for publication was documented in the patient's medical record.
Author contributions
GM: Conceptualization, Data curation, Visualization, Writing – original draft, Writing – review & editing. FW: Conceptualization, Data–curation, Writing – review & editing. HM: Conceptualization, Visualization, Writing – review & editing. KF: Conceptualization, Supervision, Visualization, Writing – original draft, Writing – review editing, Funding acquisition.
The author(s) declare that no financial support was received for the research, authorship, and/or publication of this article.
Conflict of interest
HM is a consultant for Inari Medical and receives an educational grant from the same company.
The remaining authors declare that the research was conducted in the absence of any commercial or financial relationships that could be construed as a potential conflict of interest.
Publisher's note
All claims expressed in this article are solely those of the authors and do not necessarily represent those of their affiliated organizations, or those of the publisher, the editors and the reviewers. Any product that may be evaluated in this article, or claim that may be made by its manufacturer, is not guaranteed or endorsed by the publisher.
Supplementary material
The Supplementary Material for this article can be found online at: https://www.frontiersin.org/articles/10.3389/fcvm.2023.1272944/full#supplementary-material
1. Ference BA, Ginsberg HN, Graham I, Ray KK, Packard CJ, Bruckert E, et al. Low-density lipoproteins cause atherosclerotic cardiovascular disease. 1. Evidence from genetic, epidemiologic, and clinical studies. A consensus statement from the European atherosclerosis society consensus panel. Eur Heart J . (2017) 38(32):2459–72. doi: 10.1093/eurheartj/ehx144
PubMed Abstract | CrossRef Full Text | Google Scholar
2. Ference BA, Yoo W, Alesh I, Mahajan N, Mirowska KK, Mewada A, et al. Effect of long-term exposure to lower low-density lipoprotein cholesterol beginning early in life on the risk of coronary heart disease: a Mendelian randomization analysis. J Am Coll Cardiol . (2012) 60(25):2631–9. doi: 10.1016/j.jacc.2012.09.017
3. Fernández-Friera L, Fuster V, López-Melgar B, Oliva B, García-Ruiz JM, Mendiguren J, et al. Normal LDL-cholesterol levels are associated with subclinical atherosclerosis in the absence of risk factors. J Am Coll Cardiol . (2017) 70(24):2979–91. doi: 10.1016/j.jacc.2017.10.024
CrossRef Full Text | Google Scholar
4. Libby P, Buring JE, Badimon L, Hansson GK, Deanfield J, Bittencourt MS, et al. Atherosclerosis. Nature Reviews Disease Primers . (2019) 5(1):56. doi: 10.1038/s41572-019-0106-z
5. Ahmadi A, Argulian E, Leipsic J, Newby DE, Narula J. From subclinical atherosclerosis to plaque progression and acute coronary events: JACC state-of-the-art review. J Am Coll Cardiol . (2019) 74(12):1608–17. doi: 10.1016/j.jacc.2019.08.012
6. Olsson AG, Angelin B, Assmann G, Binder CJ, Björkhem I, Cedazo-Minguez A, et al. Can LDL cholesterol be too low? Possible risks of extremely low levels. J Intern Med . (2017) 281(6):534–53. doi: 10.1111/joim.12614
7. Welty FK. Hypobetalipoproteinemia and abetalipoproteinemia: liver disease and cardiovascular disease. Curr Opin Lipidol . (2020) 31(2):49–55. doi: 10.1097/MOL.0000000000000663
8. Welty FK, Ordovas J, Schaefer EJ, Wilson PW, Young SG. Identification and molecular analysis of two apoB gene mutations causing low plasma cholesterol levels. Circulation . (1995) 92(8):2036–40. doi: 10.1161/01.CIR.92.8.2036
9. Samarendra P, Kumari S, Hafeez M, Vasavada BC, Sacchi TJ. Anomalous circumflex coronary artery: benign or predisposed to selective atherosclerosis. Angiology . (2001) 52(8):521–6. doi: 10.1177/000331970105200803
10. Braunwald E. How to live to 100 before developing clinical coronary artery disease: a suggestion. Eur Heart J . (2022) 43(4):249–50. doi: 10.1093/eurheartj/ehab532
11. Lee RG, Mazzola AM, Braun MC, Platt C, Vafai SB, Kathiresan S, et al. Efficacy and safety of an investigational single-course CRISPR base-editing therapy targeting PCSK9 in nonhuman primate and mouse models. Circulation . (2023) 147(3):242–53. doi: 10.1161/CIRCULATIONAHA.122.062132
12. Oostveen RF, Khera AV, Kathiresan S, Stroes ESG, Fitzgerald K, Harms MJ, et al. New approaches for targeting PCSK9: small-interfering ribonucleic acid and genome editing. Arterioscler Thromb Vasc Biol . (2023) 43(7):1081–92. doi: 10.1161/ATVBAHA.122.317963
Keywords: LDL-C, atherosclerosis, familial hypobetalipoproteinemia (FHBL), primordial prevention of CVD, coronary artery disease
Citation: Mottola G, Welty FK, Mojibian HR and Faridi KF (2023) Case report: Coronary atherosclerosis in a patient with long-standing very low LDL-C without lipid-lowering therapy. Front. Cardiovasc. Med. 10:1272944. doi: 10.3389/fcvm.2023.1272944
Received: 4 August 2023; Accepted: 8 September 2023; Published: 19 September 2023.
Reviewed by:
© 2023 Mottola, Welty, Mojibian and Faridi. This is an open-access article distributed under the terms of the Creative Commons Attribution License (CC BY) . The use, distribution or reproduction in other forums is permitted, provided the original author(s) and the copyright owner(s) are credited and that the original publication in this journal is cited, in accordance with accepted academic practice. No use, distribution or reproduction is permitted which does not comply with these terms.
*Correspondence: Kamil F. Faridi [email protected]
Disclaimer: All claims expressed in this article are solely those of the authors and do not necessarily represent those of their affiliated organizations, or those of the publisher, the editors and the reviewers. Any product that may be evaluated in this article or claim that may be made by its manufacturer is not guaranteed or endorsed by the publisher.

Create Free Account or

- Acute Coronary Syndromes
- Anticoagulation Management
- Arrhythmias and Clinical EP
- Cardiac Surgery
- Cardio-Oncology
- Cardiovascular Care Team
- Congenital Heart Disease and Pediatric Cardiology
- COVID-19 Hub
- Diabetes and Cardiometabolic Disease
- Dyslipidemia
- Geriatric Cardiology
- Heart Failure and Cardiomyopathies
- Invasive Cardiovascular Angiography and Intervention
- Noninvasive Imaging
- Pericardial Disease
- Pulmonary Hypertension and Venous Thromboembolism
- Sports and Exercise Cardiology
- Stable Ischemic Heart Disease
- Valvular Heart Disease
- Vascular Medicine
- Clinical Updates & Discoveries
- Advocacy & Policy
- Perspectives & Analysis
- Meeting Coverage
- ACC Member Publications
- ACC Podcasts
- View All Cardiology Updates
- Earn Credit
- View the Education Catalog
- ACC Anywhere: The Cardiology Video Library
- CardioSource Plus for Institutions and Practices
- ECG Drill and Practice
- Heart Songs
- Nuclear Cardiology
- Online Courses
- Collaborative Maintenance Pathway (CMP)
- Understanding MOC
- Image and Slide Gallery
- Annual Scientific Session and Related Events
- Chapter Meetings
- Live Meetings
- Live Meetings - International
- Webinars - Live
- Webinars - OnDemand
- Certificates and Certifications
- ACC Accreditation Services
- ACC Quality Improvement for Institutions Program
- CardioSmart
- National Cardiovascular Data Registry (NCDR)
- Advocacy at the ACC
- Cardiology as a Career Path
- Cardiology Careers
- Cardiovascular Buyers Guide
- Clinical Solutions
- Clinician Well-Being Portal
- Diversity and Inclusion
- Infographics
- Innovation Program
- Mobile and Web Apps
A 52-Year-Old Man With Atherosclerosis
A 52-year-old executive was referred to our clinic for risk factor management after undergoing coronary computed tomography angiography (CTA) as part of an Executive Physical. He has no history of coronary artery disease and exercises regularly without experiencing anginal symptoms.
His family history is notable for a myocardial infarction (MI) in his father at the age of 52 years. He is a lifelong non-smoker. He does not take medications.
His blood pressure was 110/75. His exam was notable for being overweight with a BMI of 27, but was otherwise unremarkable.
His total cholesterol is 206 mg/dL, HDL-C is 46 mg/dL, triglycerides are 178 mg/dL, calculated LDL-C is 124 mg/dL, and non HDL-C is 160 mg/dL. His fasting glucose is 86 mg/dL. His Hgb A1c is 5.6%.
His 10-year risk based on the 2013 ACC/AHA pooled ASCVD risk estimator is 3.7%.
His coronary artery calcium (CAC) score is 120, which places him in the 87th percentile for his age, gender, and ethnicity.
His coronary CTA shows the following in the proximal LAD:
COMMENTS
The patient received a coronary artery bypass surgery at just 21 years of age. He exhibits traditional risk factors of CHD; hypertension, dyslipidemia, cigarette smoking, and obesity. CHD may be more prevalent in the younger population than once was thought, and it is important that risk factor reduction starts in early in life.>.
The patient received a coronary artery bypass surgery at just 21 years of age. He exhibits traditional risk factors of CHD; hypertension, dyslipidemia, cigarette smoking, and obesity. CHD may be more prevalent in the younger population than once was thought, and it is important that risk factor reduction starts in early in life.>.
Importantly, this case demonstrates that coronary atherosclerosis can occur even with long-standing LDL-C less than 40 mg/dl when other risk factors such as hypertension and diabetes are present and otherwise well controlled. Reports of atherosclerosis in individuals with lifelong very low LDL-C are extremely rare . The patient in this instance ...
This case is an important reminder to all clinicians that atherosclerosis often involves multiple arterial territories. The weaknesses of the case report include significant omitted details regarding the history, physical examination, and objective findings. The timeline of the patient's management is also unclear.
Between October 29, 2004, and June 8, 2006, a total of 697 patients with acute coronary syndromes were enrolled after they had undergone successful percutaneous coronary intervention ( Table 1 ...
Atherosclerosis is a chronic condition in which arteries harden through build-up of plaques. Main classical risk factors for atherosclerosis include dyslipoproteinaemia, di-abetes, cigarette smoking, hypertension and genetic abnor-malities. In this review, we present an update on the patho-physiology of atherosclerosis and related current and ...
Case Report A 65 year old asymptomatic female was suspected to have a distal ascending aortic aneurysm and a moderate Aortic Regurgitation with some aortic sclerosis on routine health checkup.
Introduction. Cardiovascular disease (CVD) is the leading cause of mortality and morbidity worldwide and represents the highest proportion of costs to health care systems (1,2).Global CVD prevalence is growing, and if this trend continues, it will threaten the ability of health care systems to cope with its consequences ().Most forms of CVD originate in atherosclerosis, which is thus the main ...
Arteriosclerosis and Atherosclerosis 1215. 3. Sawabe M, Takahashi R, Matsushita S, Ozawa T, Arai T, Hamamatsu A, Nakahara K, Chida K, Yamanouchi H, Murayama S, Tanaka N. Aortic pulse wave velocity and the degree of atherosclerosis in the elderly: a pathological study based on 304 autopsy cases. Atherosclerosis. 2005; 179:345-351.
A Multiscale and Patient-specific Computational Framework of Atherosclerosis Formation and Progression: A Case Study in the Aorta and Peripheral Arteri es.pdf Content available from CC BY-NC-ND 4.0:
Atherosclerosis is the main risk factor for cardiovascular disease (CVD), which is the leading cause of mortality worldwide. Atherosclerosis is initiated by endothelium activation and, followed by a cascade of events (accumulation of lipids, fibrous elements, and calcification), triggers the vessel narrowing and activation of inflammatory pathways. The resultant atheroma plaque, along with ...
Importantly, this case demonstrates that coronary atherosclerosis can occur even with long-standing LDL-C less than 40 mg/dl when other risk factors such as hypertension and diabetes are present and otherwise well controlled. Reports of atherosclerosis in individuals with lifelong very low LDL-C are extremely rare . The patient in this instance ...
Discovery of these common variants comes at the heel of large genome-wide association studies now being conducted on an increasingly larger scale. 2,3 As the name of these ... Atherosclerosis. 2011;219:799-806. doi: 10.1016/j.atherosclerosis ... a prospective case-control study. Lancet Diabetes Endocrinol. 2015;3:507-513. doi: 10. ...
Atherosclerosis is the major cause of morbidities and mortalities worldwide. In this study we aimed to review the mechanism of atherosclerosis and its risk factors, focusing on new findings in atherosclerosis markers and its risk factors. Furthermore, the role of antioxidants and medicinal herbs in atherosclerosis and endothelial damage has ...
Between October 29, 2004, and June 8, 2006, a total of 697 patients with acute coronary syn-dromes were enrolled after they had undergone successful percutaneous coronary intervention (Table 1 ...
In this issue of the Journal of the American Heart Association (JAHA), Duncan et al apply the technique of trajectory analysis to a more‐contemporary cohort of the Framingham Heart Study, followed for 35 years to determine the relationship of lifelong exposure to elevated LDL cholesterol and low high‐density lipoprotein cholesterol to ASCVD and total mortality. 6 For LDL cholesterol, they ...
Aim: The purpose of the study was to determine the presence of cardiovascular disease in young patient with a rare monogenic form of diabetes mellitus (MODY12). Clinical case: The development of atherosclerosis in a patient 28 years old with 12 mody diabetes - Atherosclerosis
His family history is notable for a myocardial infarction (MI) in his father at the age of 52 years. He is a lifelong non-smoker. He does not take medications. His blood pressure was 110/75. His exam was notable for being overweight with a BMI of 27, but was otherwise unremarkable. His total cholesterol is 206 mg/dL, HDL-C is 46 mg/dL ...
Atherosclerosis is a chronic inflammatory disease of the arteries and is the underlying cause of about 50% of all deaths in westernized society. It is principally a lipid-driven process initiated by the accumulation of low-density lipoprotein and remnant lipoprotein particles and an active inflammatory process in focal areas of arteries particularly at regions of disturbed non-laminar flow at ...
Epidemiologic studies have identified hyperhomocysteinemia as a possible risk factor for atherosclerosis. We determined the risk of carotid-artery atherosclerosis in relation to both plasma ...
Aging is a complex and multifactorial process of physiological changes strongly associated with various human diseases, including cardiovascular diseases (CVDs), diabetes, neurodegeneration and ...
Many animal models have been used to study atherosclerosis, as summarized in the recent American Heart Association Scientific Statement, 3 which provide suggestions on selecting appropriate animal models for a specific atherosclerosis study. Given its value and feasibility as a proof-of-principle model, mouse models have continued to be the most common species to study atherosclerosis.
The microscopic study of the coronary arteries revealed recent partial thrombosis on the seventh cm of the right coronary artery, in addition to atherosclerosis with 80% obstruction. The anterior interventricular and circumflex branches of the left coronary artery had stents placed several years before and were submitted to special processing ...
Nervous System case study Name:_____ Going Under the Knife: A Case on Membrane Structure and Function Twenty-year-old Kevin groaned and clutched his abdomen as he lay on the emergency room gurney. He had just been diagnosed with acute appendicitis and was waiting to be taken to the operating room (OR). Although he desperately wanted the pain to stop, Kevin was terrified of having general ...
This is the case report of a 66-year-old man with systemic hypertension and a chronic smoker that presented with acute severe chest pain. After medical assessment, he was diagnosed with acute myocardial infarction and the patient underwent coronary angiography, which disclosed proximal occlusion of the left anterior descending artery with ...