- Privacy Policy

Home » Case Study – Methods, Examples and Guide
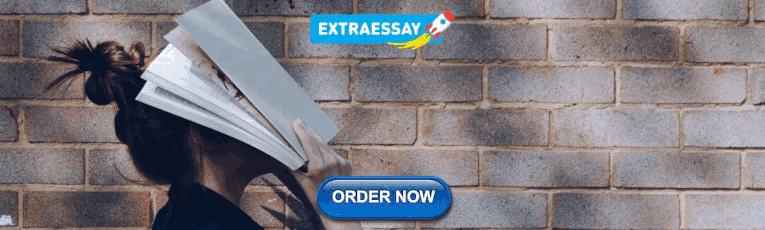
Case Study – Methods, Examples and Guide
Table of Contents

A case study is a research method that involves an in-depth examination and analysis of a particular phenomenon or case, such as an individual, organization, community, event, or situation.
It is a qualitative research approach that aims to provide a detailed and comprehensive understanding of the case being studied. Case studies typically involve multiple sources of data, including interviews, observations, documents, and artifacts, which are analyzed using various techniques, such as content analysis, thematic analysis, and grounded theory. The findings of a case study are often used to develop theories, inform policy or practice, or generate new research questions.
Types of Case Study
Types and Methods of Case Study are as follows:
Single-Case Study
A single-case study is an in-depth analysis of a single case. This type of case study is useful when the researcher wants to understand a specific phenomenon in detail.
For Example , A researcher might conduct a single-case study on a particular individual to understand their experiences with a particular health condition or a specific organization to explore their management practices. The researcher collects data from multiple sources, such as interviews, observations, and documents, and uses various techniques to analyze the data, such as content analysis or thematic analysis. The findings of a single-case study are often used to generate new research questions, develop theories, or inform policy or practice.
Multiple-Case Study
A multiple-case study involves the analysis of several cases that are similar in nature. This type of case study is useful when the researcher wants to identify similarities and differences between the cases.
For Example, a researcher might conduct a multiple-case study on several companies to explore the factors that contribute to their success or failure. The researcher collects data from each case, compares and contrasts the findings, and uses various techniques to analyze the data, such as comparative analysis or pattern-matching. The findings of a multiple-case study can be used to develop theories, inform policy or practice, or generate new research questions.
Exploratory Case Study
An exploratory case study is used to explore a new or understudied phenomenon. This type of case study is useful when the researcher wants to generate hypotheses or theories about the phenomenon.
For Example, a researcher might conduct an exploratory case study on a new technology to understand its potential impact on society. The researcher collects data from multiple sources, such as interviews, observations, and documents, and uses various techniques to analyze the data, such as grounded theory or content analysis. The findings of an exploratory case study can be used to generate new research questions, develop theories, or inform policy or practice.
Descriptive Case Study
A descriptive case study is used to describe a particular phenomenon in detail. This type of case study is useful when the researcher wants to provide a comprehensive account of the phenomenon.
For Example, a researcher might conduct a descriptive case study on a particular community to understand its social and economic characteristics. The researcher collects data from multiple sources, such as interviews, observations, and documents, and uses various techniques to analyze the data, such as content analysis or thematic analysis. The findings of a descriptive case study can be used to inform policy or practice or generate new research questions.
Instrumental Case Study
An instrumental case study is used to understand a particular phenomenon that is instrumental in achieving a particular goal. This type of case study is useful when the researcher wants to understand the role of the phenomenon in achieving the goal.
For Example, a researcher might conduct an instrumental case study on a particular policy to understand its impact on achieving a particular goal, such as reducing poverty. The researcher collects data from multiple sources, such as interviews, observations, and documents, and uses various techniques to analyze the data, such as content analysis or thematic analysis. The findings of an instrumental case study can be used to inform policy or practice or generate new research questions.
Case Study Data Collection Methods
Here are some common data collection methods for case studies:
Interviews involve asking questions to individuals who have knowledge or experience relevant to the case study. Interviews can be structured (where the same questions are asked to all participants) or unstructured (where the interviewer follows up on the responses with further questions). Interviews can be conducted in person, over the phone, or through video conferencing.
Observations
Observations involve watching and recording the behavior and activities of individuals or groups relevant to the case study. Observations can be participant (where the researcher actively participates in the activities) or non-participant (where the researcher observes from a distance). Observations can be recorded using notes, audio or video recordings, or photographs.
Documents can be used as a source of information for case studies. Documents can include reports, memos, emails, letters, and other written materials related to the case study. Documents can be collected from the case study participants or from public sources.
Surveys involve asking a set of questions to a sample of individuals relevant to the case study. Surveys can be administered in person, over the phone, through mail or email, or online. Surveys can be used to gather information on attitudes, opinions, or behaviors related to the case study.
Artifacts are physical objects relevant to the case study. Artifacts can include tools, equipment, products, or other objects that provide insights into the case study phenomenon.
How to conduct Case Study Research
Conducting a case study research involves several steps that need to be followed to ensure the quality and rigor of the study. Here are the steps to conduct case study research:
- Define the research questions: The first step in conducting a case study research is to define the research questions. The research questions should be specific, measurable, and relevant to the case study phenomenon under investigation.
- Select the case: The next step is to select the case or cases to be studied. The case should be relevant to the research questions and should provide rich and diverse data that can be used to answer the research questions.
- Collect data: Data can be collected using various methods, such as interviews, observations, documents, surveys, and artifacts. The data collection method should be selected based on the research questions and the nature of the case study phenomenon.
- Analyze the data: The data collected from the case study should be analyzed using various techniques, such as content analysis, thematic analysis, or grounded theory. The analysis should be guided by the research questions and should aim to provide insights and conclusions relevant to the research questions.
- Draw conclusions: The conclusions drawn from the case study should be based on the data analysis and should be relevant to the research questions. The conclusions should be supported by evidence and should be clearly stated.
- Validate the findings: The findings of the case study should be validated by reviewing the data and the analysis with participants or other experts in the field. This helps to ensure the validity and reliability of the findings.
- Write the report: The final step is to write the report of the case study research. The report should provide a clear description of the case study phenomenon, the research questions, the data collection methods, the data analysis, the findings, and the conclusions. The report should be written in a clear and concise manner and should follow the guidelines for academic writing.
Examples of Case Study
Here are some examples of case study research:
- The Hawthorne Studies : Conducted between 1924 and 1932, the Hawthorne Studies were a series of case studies conducted by Elton Mayo and his colleagues to examine the impact of work environment on employee productivity. The studies were conducted at the Hawthorne Works plant of the Western Electric Company in Chicago and included interviews, observations, and experiments.
- The Stanford Prison Experiment: Conducted in 1971, the Stanford Prison Experiment was a case study conducted by Philip Zimbardo to examine the psychological effects of power and authority. The study involved simulating a prison environment and assigning participants to the role of guards or prisoners. The study was controversial due to the ethical issues it raised.
- The Challenger Disaster: The Challenger Disaster was a case study conducted to examine the causes of the Space Shuttle Challenger explosion in 1986. The study included interviews, observations, and analysis of data to identify the technical, organizational, and cultural factors that contributed to the disaster.
- The Enron Scandal: The Enron Scandal was a case study conducted to examine the causes of the Enron Corporation’s bankruptcy in 2001. The study included interviews, analysis of financial data, and review of documents to identify the accounting practices, corporate culture, and ethical issues that led to the company’s downfall.
- The Fukushima Nuclear Disaster : The Fukushima Nuclear Disaster was a case study conducted to examine the causes of the nuclear accident that occurred at the Fukushima Daiichi Nuclear Power Plant in Japan in 2011. The study included interviews, analysis of data, and review of documents to identify the technical, organizational, and cultural factors that contributed to the disaster.
Application of Case Study
Case studies have a wide range of applications across various fields and industries. Here are some examples:
Business and Management
Case studies are widely used in business and management to examine real-life situations and develop problem-solving skills. Case studies can help students and professionals to develop a deep understanding of business concepts, theories, and best practices.
Case studies are used in healthcare to examine patient care, treatment options, and outcomes. Case studies can help healthcare professionals to develop critical thinking skills, diagnose complex medical conditions, and develop effective treatment plans.
Case studies are used in education to examine teaching and learning practices. Case studies can help educators to develop effective teaching strategies, evaluate student progress, and identify areas for improvement.
Social Sciences
Case studies are widely used in social sciences to examine human behavior, social phenomena, and cultural practices. Case studies can help researchers to develop theories, test hypotheses, and gain insights into complex social issues.
Law and Ethics
Case studies are used in law and ethics to examine legal and ethical dilemmas. Case studies can help lawyers, policymakers, and ethical professionals to develop critical thinking skills, analyze complex cases, and make informed decisions.
Purpose of Case Study
The purpose of a case study is to provide a detailed analysis of a specific phenomenon, issue, or problem in its real-life context. A case study is a qualitative research method that involves the in-depth exploration and analysis of a particular case, which can be an individual, group, organization, event, or community.
The primary purpose of a case study is to generate a comprehensive and nuanced understanding of the case, including its history, context, and dynamics. Case studies can help researchers to identify and examine the underlying factors, processes, and mechanisms that contribute to the case and its outcomes. This can help to develop a more accurate and detailed understanding of the case, which can inform future research, practice, or policy.
Case studies can also serve other purposes, including:
- Illustrating a theory or concept: Case studies can be used to illustrate and explain theoretical concepts and frameworks, providing concrete examples of how they can be applied in real-life situations.
- Developing hypotheses: Case studies can help to generate hypotheses about the causal relationships between different factors and outcomes, which can be tested through further research.
- Providing insight into complex issues: Case studies can provide insights into complex and multifaceted issues, which may be difficult to understand through other research methods.
- Informing practice or policy: Case studies can be used to inform practice or policy by identifying best practices, lessons learned, or areas for improvement.
Advantages of Case Study Research
There are several advantages of case study research, including:
- In-depth exploration: Case study research allows for a detailed exploration and analysis of a specific phenomenon, issue, or problem in its real-life context. This can provide a comprehensive understanding of the case and its dynamics, which may not be possible through other research methods.
- Rich data: Case study research can generate rich and detailed data, including qualitative data such as interviews, observations, and documents. This can provide a nuanced understanding of the case and its complexity.
- Holistic perspective: Case study research allows for a holistic perspective of the case, taking into account the various factors, processes, and mechanisms that contribute to the case and its outcomes. This can help to develop a more accurate and comprehensive understanding of the case.
- Theory development: Case study research can help to develop and refine theories and concepts by providing empirical evidence and concrete examples of how they can be applied in real-life situations.
- Practical application: Case study research can inform practice or policy by identifying best practices, lessons learned, or areas for improvement.
- Contextualization: Case study research takes into account the specific context in which the case is situated, which can help to understand how the case is influenced by the social, cultural, and historical factors of its environment.
Limitations of Case Study Research
There are several limitations of case study research, including:
- Limited generalizability : Case studies are typically focused on a single case or a small number of cases, which limits the generalizability of the findings. The unique characteristics of the case may not be applicable to other contexts or populations, which may limit the external validity of the research.
- Biased sampling: Case studies may rely on purposive or convenience sampling, which can introduce bias into the sample selection process. This may limit the representativeness of the sample and the generalizability of the findings.
- Subjectivity: Case studies rely on the interpretation of the researcher, which can introduce subjectivity into the analysis. The researcher’s own biases, assumptions, and perspectives may influence the findings, which may limit the objectivity of the research.
- Limited control: Case studies are typically conducted in naturalistic settings, which limits the control that the researcher has over the environment and the variables being studied. This may limit the ability to establish causal relationships between variables.
- Time-consuming: Case studies can be time-consuming to conduct, as they typically involve a detailed exploration and analysis of a specific case. This may limit the feasibility of conducting multiple case studies or conducting case studies in a timely manner.
- Resource-intensive: Case studies may require significant resources, including time, funding, and expertise. This may limit the ability of researchers to conduct case studies in resource-constrained settings.
About the author
Muhammad Hassan
Researcher, Academic Writer, Web developer
You may also like

Mixed Methods Research – Types & Analysis

Basic Research – Types, Methods and Examples

Correlational Research – Methods, Types and...

Observational Research – Methods and Guide

Focus Groups – Steps, Examples and Guide

Phenomenology – Methods, Examples and Guide
Have a language expert improve your writing
Run a free plagiarism check in 10 minutes, generate accurate citations for free.
- Knowledge Base
Methodology
- What Is a Case Study? | Definition, Examples & Methods
What Is a Case Study? | Definition, Examples & Methods
Published on May 8, 2019 by Shona McCombes . Revised on November 20, 2023.
A case study is a detailed study of a specific subject, such as a person, group, place, event, organization, or phenomenon. Case studies are commonly used in social, educational, clinical, and business research.
A case study research design usually involves qualitative methods , but quantitative methods are sometimes also used. Case studies are good for describing , comparing, evaluating and understanding different aspects of a research problem .
Table of contents
When to do a case study, step 1: select a case, step 2: build a theoretical framework, step 3: collect your data, step 4: describe and analyze the case, other interesting articles.
A case study is an appropriate research design when you want to gain concrete, contextual, in-depth knowledge about a specific real-world subject. It allows you to explore the key characteristics, meanings, and implications of the case.
Case studies are often a good choice in a thesis or dissertation . They keep your project focused and manageable when you don’t have the time or resources to do large-scale research.
You might use just one complex case study where you explore a single subject in depth, or conduct multiple case studies to compare and illuminate different aspects of your research problem.
Research question | Case study |
---|---|
What are the ecological effects of wolf reintroduction? | Case study of wolf reintroduction in Yellowstone National Park |
How do populist politicians use narratives about history to gain support? | Case studies of Hungarian prime minister Viktor Orbán and US president Donald Trump |
How can teachers implement active learning strategies in mixed-level classrooms? | Case study of a local school that promotes active learning |
What are the main advantages and disadvantages of wind farms for rural communities? | Case studies of three rural wind farm development projects in different parts of the country |
How are viral marketing strategies changing the relationship between companies and consumers? | Case study of the iPhone X marketing campaign |
How do experiences of work in the gig economy differ by gender, race and age? | Case studies of Deliveroo and Uber drivers in London |
Receive feedback on language, structure, and formatting
Professional editors proofread and edit your paper by focusing on:
- Academic style
- Vague sentences
- Style consistency
See an example
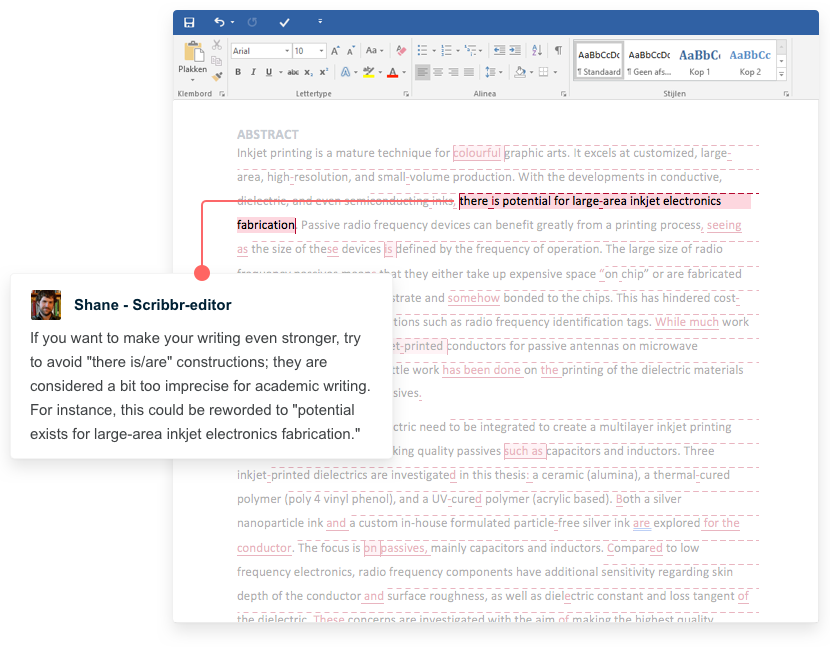
Once you have developed your problem statement and research questions , you should be ready to choose the specific case that you want to focus on. A good case study should have the potential to:
- Provide new or unexpected insights into the subject
- Challenge or complicate existing assumptions and theories
- Propose practical courses of action to resolve a problem
- Open up new directions for future research
TipIf your research is more practical in nature and aims to simultaneously investigate an issue as you solve it, consider conducting action research instead.
Unlike quantitative or experimental research , a strong case study does not require a random or representative sample. In fact, case studies often deliberately focus on unusual, neglected, or outlying cases which may shed new light on the research problem.
Example of an outlying case studyIn the 1960s the town of Roseto, Pennsylvania was discovered to have extremely low rates of heart disease compared to the US average. It became an important case study for understanding previously neglected causes of heart disease.
However, you can also choose a more common or representative case to exemplify a particular category, experience or phenomenon.
Example of a representative case studyIn the 1920s, two sociologists used Muncie, Indiana as a case study of a typical American city that supposedly exemplified the changing culture of the US at the time.
While case studies focus more on concrete details than general theories, they should usually have some connection with theory in the field. This way the case study is not just an isolated description, but is integrated into existing knowledge about the topic. It might aim to:
- Exemplify a theory by showing how it explains the case under investigation
- Expand on a theory by uncovering new concepts and ideas that need to be incorporated
- Challenge a theory by exploring an outlier case that doesn’t fit with established assumptions
To ensure that your analysis of the case has a solid academic grounding, you should conduct a literature review of sources related to the topic and develop a theoretical framework . This means identifying key concepts and theories to guide your analysis and interpretation.
There are many different research methods you can use to collect data on your subject. Case studies tend to focus on qualitative data using methods such as interviews , observations , and analysis of primary and secondary sources (e.g., newspaper articles, photographs, official records). Sometimes a case study will also collect quantitative data.
Example of a mixed methods case studyFor a case study of a wind farm development in a rural area, you could collect quantitative data on employment rates and business revenue, collect qualitative data on local people’s perceptions and experiences, and analyze local and national media coverage of the development.
The aim is to gain as thorough an understanding as possible of the case and its context.
Prevent plagiarism. Run a free check.
In writing up the case study, you need to bring together all the relevant aspects to give as complete a picture as possible of the subject.
How you report your findings depends on the type of research you are doing. Some case studies are structured like a standard scientific paper or thesis , with separate sections or chapters for the methods , results and discussion .
Others are written in a more narrative style, aiming to explore the case from various angles and analyze its meanings and implications (for example, by using textual analysis or discourse analysis ).
In all cases, though, make sure to give contextual details about the case, connect it back to the literature and theory, and discuss how it fits into wider patterns or debates.
If you want to know more about statistics , methodology , or research bias , make sure to check out some of our other articles with explanations and examples.
- Normal distribution
- Degrees of freedom
- Null hypothesis
- Discourse analysis
- Control groups
- Mixed methods research
- Non-probability sampling
- Quantitative research
- Ecological validity
Research bias
- Rosenthal effect
- Implicit bias
- Cognitive bias
- Selection bias
- Negativity bias
- Status quo bias
Cite this Scribbr article
If you want to cite this source, you can copy and paste the citation or click the “Cite this Scribbr article” button to automatically add the citation to our free Citation Generator.
McCombes, S. (2023, November 20). What Is a Case Study? | Definition, Examples & Methods. Scribbr. Retrieved August 21, 2024, from https://www.scribbr.com/methodology/case-study/
Is this article helpful?
Shona McCombes
Other students also liked, primary vs. secondary sources | difference & examples, what is a theoretical framework | guide to organizing, what is action research | definition & examples, what is your plagiarism score.

The Ultimate Guide to Qualitative Research - Part 1: The Basics
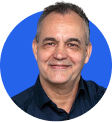
- Introduction and overview
- What is qualitative research?
- What is qualitative data?
- Examples of qualitative data
- Qualitative vs. quantitative research
- Mixed methods
- Qualitative research preparation
- Theoretical perspective
- Theoretical framework
- Literature reviews
Research question
- Conceptual framework
- Conceptual vs. theoretical framework
Data collection
- Qualitative research methods
- Focus groups
- Observational research
What is a case study?
Applications for case study research, what is a good case study, process of case study design, benefits and limitations of case studies.
- Ethnographical research
- Ethical considerations
- Confidentiality and privacy
- Power dynamics
- Reflexivity
Case studies
Case studies are essential to qualitative research , offering a lens through which researchers can investigate complex phenomena within their real-life contexts. This chapter explores the concept, purpose, applications, examples, and types of case studies and provides guidance on how to conduct case study research effectively.
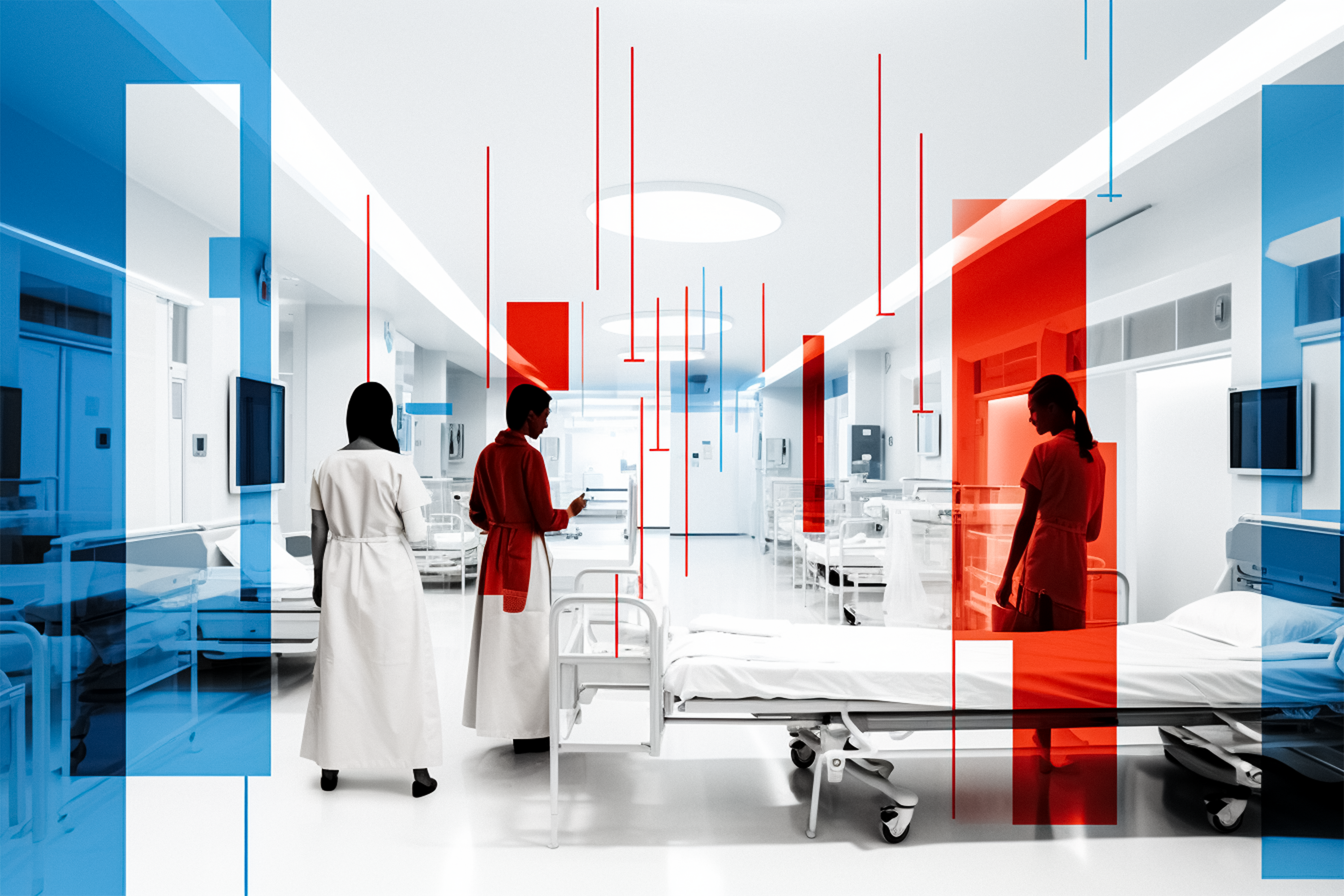
Whereas quantitative methods look at phenomena at scale, case study research looks at a concept or phenomenon in considerable detail. While analyzing a single case can help understand one perspective regarding the object of research inquiry, analyzing multiple cases can help obtain a more holistic sense of the topic or issue. Let's provide a basic definition of a case study, then explore its characteristics and role in the qualitative research process.
Definition of a case study
A case study in qualitative research is a strategy of inquiry that involves an in-depth investigation of a phenomenon within its real-world context. It provides researchers with the opportunity to acquire an in-depth understanding of intricate details that might not be as apparent or accessible through other methods of research. The specific case or cases being studied can be a single person, group, or organization – demarcating what constitutes a relevant case worth studying depends on the researcher and their research question .
Among qualitative research methods , a case study relies on multiple sources of evidence, such as documents, artifacts, interviews , or observations , to present a complete and nuanced understanding of the phenomenon under investigation. The objective is to illuminate the readers' understanding of the phenomenon beyond its abstract statistical or theoretical explanations.
Characteristics of case studies
Case studies typically possess a number of distinct characteristics that set them apart from other research methods. These characteristics include a focus on holistic description and explanation, flexibility in the design and data collection methods, reliance on multiple sources of evidence, and emphasis on the context in which the phenomenon occurs.
Furthermore, case studies can often involve a longitudinal examination of the case, meaning they study the case over a period of time. These characteristics allow case studies to yield comprehensive, in-depth, and richly contextualized insights about the phenomenon of interest.
The role of case studies in research
Case studies hold a unique position in the broader landscape of research methods aimed at theory development. They are instrumental when the primary research interest is to gain an intensive, detailed understanding of a phenomenon in its real-life context.
In addition, case studies can serve different purposes within research - they can be used for exploratory, descriptive, or explanatory purposes, depending on the research question and objectives. This flexibility and depth make case studies a valuable tool in the toolkit of qualitative researchers.
Remember, a well-conducted case study can offer a rich, insightful contribution to both academic and practical knowledge through theory development or theory verification, thus enhancing our understanding of complex phenomena in their real-world contexts.
What is the purpose of a case study?
Case study research aims for a more comprehensive understanding of phenomena, requiring various research methods to gather information for qualitative analysis . Ultimately, a case study can allow the researcher to gain insight into a particular object of inquiry and develop a theoretical framework relevant to the research inquiry.
Why use case studies in qualitative research?
Using case studies as a research strategy depends mainly on the nature of the research question and the researcher's access to the data.
Conducting case study research provides a level of detail and contextual richness that other research methods might not offer. They are beneficial when there's a need to understand complex social phenomena within their natural contexts.
The explanatory, exploratory, and descriptive roles of case studies
Case studies can take on various roles depending on the research objectives. They can be exploratory when the research aims to discover new phenomena or define new research questions; they are descriptive when the objective is to depict a phenomenon within its context in a detailed manner; and they can be explanatory if the goal is to understand specific relationships within the studied context. Thus, the versatility of case studies allows researchers to approach their topic from different angles, offering multiple ways to uncover and interpret the data .
The impact of case studies on knowledge development
Case studies play a significant role in knowledge development across various disciplines. Analysis of cases provides an avenue for researchers to explore phenomena within their context based on the collected data.
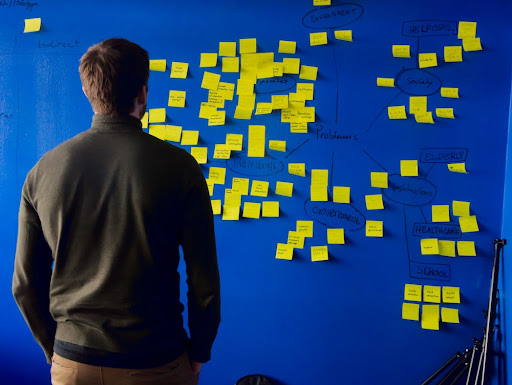
This can result in the production of rich, practical insights that can be instrumental in both theory-building and practice. Case studies allow researchers to delve into the intricacies and complexities of real-life situations, uncovering insights that might otherwise remain hidden.
Types of case studies
In qualitative research , a case study is not a one-size-fits-all approach. Depending on the nature of the research question and the specific objectives of the study, researchers might choose to use different types of case studies. These types differ in their focus, methodology, and the level of detail they provide about the phenomenon under investigation.
Understanding these types is crucial for selecting the most appropriate approach for your research project and effectively achieving your research goals. Let's briefly look at the main types of case studies.
Exploratory case studies
Exploratory case studies are typically conducted to develop a theory or framework around an understudied phenomenon. They can also serve as a precursor to a larger-scale research project. Exploratory case studies are useful when a researcher wants to identify the key issues or questions which can spur more extensive study or be used to develop propositions for further research. These case studies are characterized by flexibility, allowing researchers to explore various aspects of a phenomenon as they emerge, which can also form the foundation for subsequent studies.
Descriptive case studies
Descriptive case studies aim to provide a complete and accurate representation of a phenomenon or event within its context. These case studies are often based on an established theoretical framework, which guides how data is collected and analyzed. The researcher is concerned with describing the phenomenon in detail, as it occurs naturally, without trying to influence or manipulate it.
Explanatory case studies
Explanatory case studies are focused on explanation - they seek to clarify how or why certain phenomena occur. Often used in complex, real-life situations, they can be particularly valuable in clarifying causal relationships among concepts and understanding the interplay between different factors within a specific context.
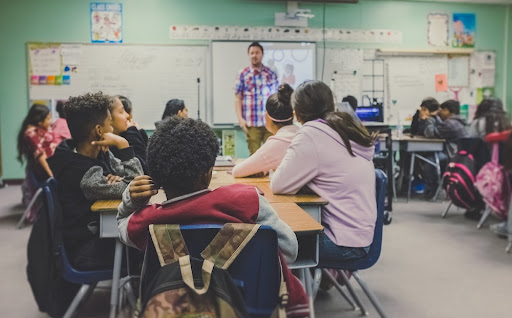
Intrinsic, instrumental, and collective case studies
These three categories of case studies focus on the nature and purpose of the study. An intrinsic case study is conducted when a researcher has an inherent interest in the case itself. Instrumental case studies are employed when the case is used to provide insight into a particular issue or phenomenon. A collective case study, on the other hand, involves studying multiple cases simultaneously to investigate some general phenomena.
Each type of case study serves a different purpose and has its own strengths and challenges. The selection of the type should be guided by the research question and objectives, as well as the context and constraints of the research.
The flexibility, depth, and contextual richness offered by case studies make this approach an excellent research method for various fields of study. They enable researchers to investigate real-world phenomena within their specific contexts, capturing nuances that other research methods might miss. Across numerous fields, case studies provide valuable insights into complex issues.
Critical information systems research
Case studies provide a detailed understanding of the role and impact of information systems in different contexts. They offer a platform to explore how information systems are designed, implemented, and used and how they interact with various social, economic, and political factors. Case studies in this field often focus on examining the intricate relationship between technology, organizational processes, and user behavior, helping to uncover insights that can inform better system design and implementation.
Health research
Health research is another field where case studies are highly valuable. They offer a way to explore patient experiences, healthcare delivery processes, and the impact of various interventions in a real-world context.
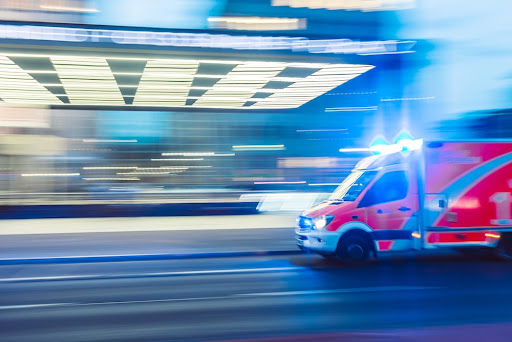
Case studies can provide a deep understanding of a patient's journey, giving insights into the intricacies of disease progression, treatment effects, and the psychosocial aspects of health and illness.
Asthma research studies
Specifically within medical research, studies on asthma often employ case studies to explore the individual and environmental factors that influence asthma development, management, and outcomes. A case study can provide rich, detailed data about individual patients' experiences, from the triggers and symptoms they experience to the effectiveness of various management strategies. This can be crucial for developing patient-centered asthma care approaches.
Other fields
Apart from the fields mentioned, case studies are also extensively used in business and management research, education research, and political sciences, among many others. They provide an opportunity to delve into the intricacies of real-world situations, allowing for a comprehensive understanding of various phenomena.
Case studies, with their depth and contextual focus, offer unique insights across these varied fields. They allow researchers to illuminate the complexities of real-life situations, contributing to both theory and practice.
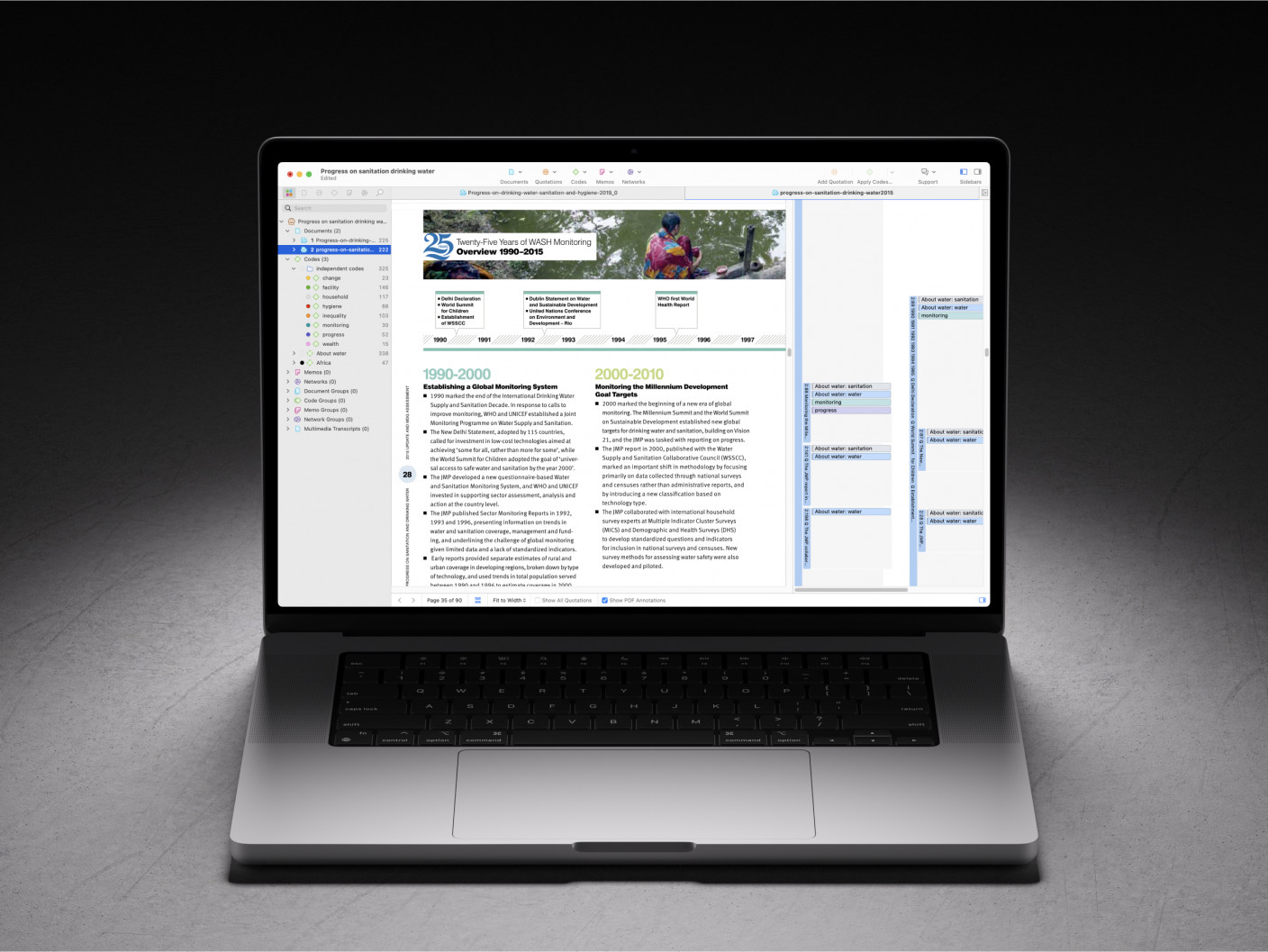
Whatever field you're in, ATLAS.ti puts your data to work for you
Download a free trial of ATLAS.ti to turn your data into insights.
Understanding the key elements of case study design is crucial for conducting rigorous and impactful case study research. A well-structured design guides the researcher through the process, ensuring that the study is methodologically sound and its findings are reliable and valid. The main elements of case study design include the research question , propositions, units of analysis, and the logic linking the data to the propositions.
The research question is the foundation of any research study. A good research question guides the direction of the study and informs the selection of the case, the methods of collecting data, and the analysis techniques. A well-formulated research question in case study research is typically clear, focused, and complex enough to merit further detailed examination of the relevant case(s).
Propositions
Propositions, though not necessary in every case study, provide a direction by stating what we might expect to find in the data collected. They guide how data is collected and analyzed by helping researchers focus on specific aspects of the case. They are particularly important in explanatory case studies, which seek to understand the relationships among concepts within the studied phenomenon.
Units of analysis
The unit of analysis refers to the case, or the main entity or entities that are being analyzed in the study. In case study research, the unit of analysis can be an individual, a group, an organization, a decision, an event, or even a time period. It's crucial to clearly define the unit of analysis, as it shapes the qualitative data analysis process by allowing the researcher to analyze a particular case and synthesize analysis across multiple case studies to draw conclusions.
Argumentation
This refers to the inferential model that allows researchers to draw conclusions from the data. The researcher needs to ensure that there is a clear link between the data, the propositions (if any), and the conclusions drawn. This argumentation is what enables the researcher to make valid and credible inferences about the phenomenon under study.
Understanding and carefully considering these elements in the design phase of a case study can significantly enhance the quality of the research. It can help ensure that the study is methodologically sound and its findings contribute meaningful insights about the case.
Ready to jumpstart your research with ATLAS.ti?
Conceptualize your research project with our intuitive data analysis interface. Download a free trial today.
Conducting a case study involves several steps, from defining the research question and selecting the case to collecting and analyzing data . This section outlines these key stages, providing a practical guide on how to conduct case study research.
Defining the research question
The first step in case study research is defining a clear, focused research question. This question should guide the entire research process, from case selection to analysis. It's crucial to ensure that the research question is suitable for a case study approach. Typically, such questions are exploratory or descriptive in nature and focus on understanding a phenomenon within its real-life context.
Selecting and defining the case
The selection of the case should be based on the research question and the objectives of the study. It involves choosing a unique example or a set of examples that provide rich, in-depth data about the phenomenon under investigation. After selecting the case, it's crucial to define it clearly, setting the boundaries of the case, including the time period and the specific context.
Previous research can help guide the case study design. When considering a case study, an example of a case could be taken from previous case study research and used to define cases in a new research inquiry. Considering recently published examples can help understand how to select and define cases effectively.
Developing a detailed case study protocol
A case study protocol outlines the procedures and general rules to be followed during the case study. This includes the data collection methods to be used, the sources of data, and the procedures for analysis. Having a detailed case study protocol ensures consistency and reliability in the study.
The protocol should also consider how to work with the people involved in the research context to grant the research team access to collecting data. As mentioned in previous sections of this guide, establishing rapport is an essential component of qualitative research as it shapes the overall potential for collecting and analyzing data.
Collecting data
Gathering data in case study research often involves multiple sources of evidence, including documents, archival records, interviews, observations, and physical artifacts. This allows for a comprehensive understanding of the case. The process for gathering data should be systematic and carefully documented to ensure the reliability and validity of the study.
Analyzing and interpreting data
The next step is analyzing the data. This involves organizing the data , categorizing it into themes or patterns , and interpreting these patterns to answer the research question. The analysis might also involve comparing the findings with prior research or theoretical propositions.
Writing the case study report
The final step is writing the case study report . This should provide a detailed description of the case, the data, the analysis process, and the findings. The report should be clear, organized, and carefully written to ensure that the reader can understand the case and the conclusions drawn from it.
Each of these steps is crucial in ensuring that the case study research is rigorous, reliable, and provides valuable insights about the case.
The type, depth, and quality of data in your study can significantly influence the validity and utility of the study. In case study research, data is usually collected from multiple sources to provide a comprehensive and nuanced understanding of the case. This section will outline the various methods of collecting data used in case study research and discuss considerations for ensuring the quality of the data.
Interviews are a common method of gathering data in case study research. They can provide rich, in-depth data about the perspectives, experiences, and interpretations of the individuals involved in the case. Interviews can be structured , semi-structured , or unstructured , depending on the research question and the degree of flexibility needed.
Observations
Observations involve the researcher observing the case in its natural setting, providing first-hand information about the case and its context. Observations can provide data that might not be revealed in interviews or documents, such as non-verbal cues or contextual information.
Documents and artifacts
Documents and archival records provide a valuable source of data in case study research. They can include reports, letters, memos, meeting minutes, email correspondence, and various public and private documents related to the case.
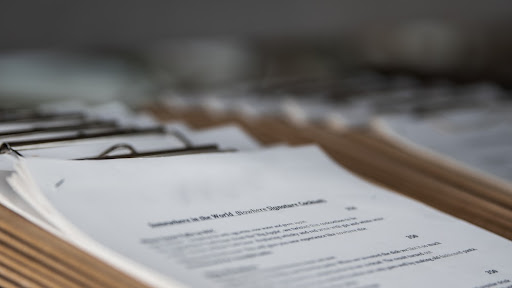
These records can provide historical context, corroborate evidence from other sources, and offer insights into the case that might not be apparent from interviews or observations.
Physical artifacts refer to any physical evidence related to the case, such as tools, products, or physical environments. These artifacts can provide tangible insights into the case, complementing the data gathered from other sources.
Ensuring the quality of data collection
Determining the quality of data in case study research requires careful planning and execution. It's crucial to ensure that the data is reliable, accurate, and relevant to the research question. This involves selecting appropriate methods of collecting data, properly training interviewers or observers, and systematically recording and storing the data. It also includes considering ethical issues related to collecting and handling data, such as obtaining informed consent and ensuring the privacy and confidentiality of the participants.
Data analysis
Analyzing case study research involves making sense of the rich, detailed data to answer the research question. This process can be challenging due to the volume and complexity of case study data. However, a systematic and rigorous approach to analysis can ensure that the findings are credible and meaningful. This section outlines the main steps and considerations in analyzing data in case study research.
Organizing the data
The first step in the analysis is organizing the data. This involves sorting the data into manageable sections, often according to the data source or the theme. This step can also involve transcribing interviews, digitizing physical artifacts, or organizing observational data.
Categorizing and coding the data
Once the data is organized, the next step is to categorize or code the data. This involves identifying common themes, patterns, or concepts in the data and assigning codes to relevant data segments. Coding can be done manually or with the help of software tools, and in either case, qualitative analysis software can greatly facilitate the entire coding process. Coding helps to reduce the data to a set of themes or categories that can be more easily analyzed.
Identifying patterns and themes
After coding the data, the researcher looks for patterns or themes in the coded data. This involves comparing and contrasting the codes and looking for relationships or patterns among them. The identified patterns and themes should help answer the research question.
Interpreting the data
Once patterns and themes have been identified, the next step is to interpret these findings. This involves explaining what the patterns or themes mean in the context of the research question and the case. This interpretation should be grounded in the data, but it can also involve drawing on theoretical concepts or prior research.
Verification of the data
The last step in the analysis is verification. This involves checking the accuracy and consistency of the analysis process and confirming that the findings are supported by the data. This can involve re-checking the original data, checking the consistency of codes, or seeking feedback from research participants or peers.
Like any research method , case study research has its strengths and limitations. Researchers must be aware of these, as they can influence the design, conduct, and interpretation of the study.
Understanding the strengths and limitations of case study research can also guide researchers in deciding whether this approach is suitable for their research question . This section outlines some of the key strengths and limitations of case study research.
Benefits include the following:
- Rich, detailed data: One of the main strengths of case study research is that it can generate rich, detailed data about the case. This can provide a deep understanding of the case and its context, which can be valuable in exploring complex phenomena.
- Flexibility: Case study research is flexible in terms of design , data collection , and analysis . A sufficient degree of flexibility allows the researcher to adapt the study according to the case and the emerging findings.
- Real-world context: Case study research involves studying the case in its real-world context, which can provide valuable insights into the interplay between the case and its context.
- Multiple sources of evidence: Case study research often involves collecting data from multiple sources , which can enhance the robustness and validity of the findings.
On the other hand, researchers should consider the following limitations:
- Generalizability: A common criticism of case study research is that its findings might not be generalizable to other cases due to the specificity and uniqueness of each case.
- Time and resource intensive: Case study research can be time and resource intensive due to the depth of the investigation and the amount of collected data.
- Complexity of analysis: The rich, detailed data generated in case study research can make analyzing the data challenging.
- Subjectivity: Given the nature of case study research, there may be a higher degree of subjectivity in interpreting the data , so researchers need to reflect on this and transparently convey to audiences how the research was conducted.
Being aware of these strengths and limitations can help researchers design and conduct case study research effectively and interpret and report the findings appropriately.
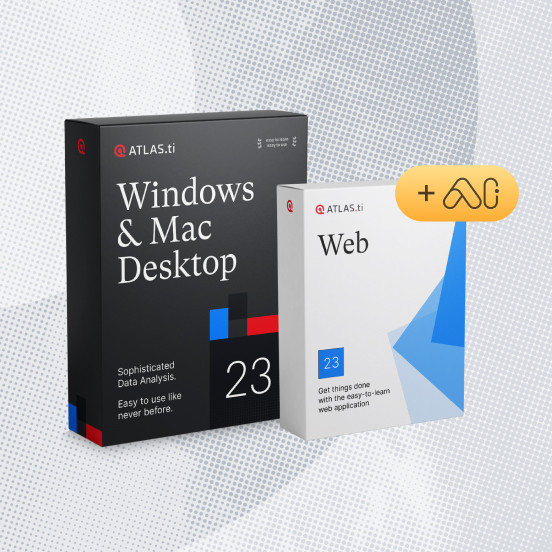
Ready to analyze your data with ATLAS.ti?
See how our intuitive software can draw key insights from your data with a free trial today.
- Open access
- Published: 27 June 2011
The case study approach
- Sarah Crowe 1 ,
- Kathrin Cresswell 2 ,
- Ann Robertson 2 ,
- Guro Huby 3 ,
- Anthony Avery 1 &
- Aziz Sheikh 2
BMC Medical Research Methodology volume 11 , Article number: 100 ( 2011 ) Cite this article
794k Accesses
1104 Citations
42 Altmetric
Metrics details
The case study approach allows in-depth, multi-faceted explorations of complex issues in their real-life settings. The value of the case study approach is well recognised in the fields of business, law and policy, but somewhat less so in health services research. Based on our experiences of conducting several health-related case studies, we reflect on the different types of case study design, the specific research questions this approach can help answer, the data sources that tend to be used, and the particular advantages and disadvantages of employing this methodological approach. The paper concludes with key pointers to aid those designing and appraising proposals for conducting case study research, and a checklist to help readers assess the quality of case study reports.
Peer Review reports
Introduction
The case study approach is particularly useful to employ when there is a need to obtain an in-depth appreciation of an issue, event or phenomenon of interest, in its natural real-life context. Our aim in writing this piece is to provide insights into when to consider employing this approach and an overview of key methodological considerations in relation to the design, planning, analysis, interpretation and reporting of case studies.
The illustrative 'grand round', 'case report' and 'case series' have a long tradition in clinical practice and research. Presenting detailed critiques, typically of one or more patients, aims to provide insights into aspects of the clinical case and, in doing so, illustrate broader lessons that may be learnt. In research, the conceptually-related case study approach can be used, for example, to describe in detail a patient's episode of care, explore professional attitudes to and experiences of a new policy initiative or service development or more generally to 'investigate contemporary phenomena within its real-life context' [ 1 ]. Based on our experiences of conducting a range of case studies, we reflect on when to consider using this approach, discuss the key steps involved and illustrate, with examples, some of the practical challenges of attaining an in-depth understanding of a 'case' as an integrated whole. In keeping with previously published work, we acknowledge the importance of theory to underpin the design, selection, conduct and interpretation of case studies[ 2 ]. In so doing, we make passing reference to the different epistemological approaches used in case study research by key theoreticians and methodologists in this field of enquiry.
This paper is structured around the following main questions: What is a case study? What are case studies used for? How are case studies conducted? What are the potential pitfalls and how can these be avoided? We draw in particular on four of our own recently published examples of case studies (see Tables 1 , 2 , 3 and 4 ) and those of others to illustrate our discussion[ 3 – 7 ].
What is a case study?
A case study is a research approach that is used to generate an in-depth, multi-faceted understanding of a complex issue in its real-life context. It is an established research design that is used extensively in a wide variety of disciplines, particularly in the social sciences. A case study can be defined in a variety of ways (Table 5 ), the central tenet being the need to explore an event or phenomenon in depth and in its natural context. It is for this reason sometimes referred to as a "naturalistic" design; this is in contrast to an "experimental" design (such as a randomised controlled trial) in which the investigator seeks to exert control over and manipulate the variable(s) of interest.
Stake's work has been particularly influential in defining the case study approach to scientific enquiry. He has helpfully characterised three main types of case study: intrinsic , instrumental and collective [ 8 ]. An intrinsic case study is typically undertaken to learn about a unique phenomenon. The researcher should define the uniqueness of the phenomenon, which distinguishes it from all others. In contrast, the instrumental case study uses a particular case (some of which may be better than others) to gain a broader appreciation of an issue or phenomenon. The collective case study involves studying multiple cases simultaneously or sequentially in an attempt to generate a still broader appreciation of a particular issue.
These are however not necessarily mutually exclusive categories. In the first of our examples (Table 1 ), we undertook an intrinsic case study to investigate the issue of recruitment of minority ethnic people into the specific context of asthma research studies, but it developed into a instrumental case study through seeking to understand the issue of recruitment of these marginalised populations more generally, generating a number of the findings that are potentially transferable to other disease contexts[ 3 ]. In contrast, the other three examples (see Tables 2 , 3 and 4 ) employed collective case study designs to study the introduction of workforce reconfiguration in primary care, the implementation of electronic health records into hospitals, and to understand the ways in which healthcare students learn about patient safety considerations[ 4 – 6 ]. Although our study focusing on the introduction of General Practitioners with Specialist Interests (Table 2 ) was explicitly collective in design (four contrasting primary care organisations were studied), is was also instrumental in that this particular professional group was studied as an exemplar of the more general phenomenon of workforce redesign[ 4 ].
What are case studies used for?
According to Yin, case studies can be used to explain, describe or explore events or phenomena in the everyday contexts in which they occur[ 1 ]. These can, for example, help to understand and explain causal links and pathways resulting from a new policy initiative or service development (see Tables 2 and 3 , for example)[ 1 ]. In contrast to experimental designs, which seek to test a specific hypothesis through deliberately manipulating the environment (like, for example, in a randomised controlled trial giving a new drug to randomly selected individuals and then comparing outcomes with controls),[ 9 ] the case study approach lends itself well to capturing information on more explanatory ' how ', 'what' and ' why ' questions, such as ' how is the intervention being implemented and received on the ground?'. The case study approach can offer additional insights into what gaps exist in its delivery or why one implementation strategy might be chosen over another. This in turn can help develop or refine theory, as shown in our study of the teaching of patient safety in undergraduate curricula (Table 4 )[ 6 , 10 ]. Key questions to consider when selecting the most appropriate study design are whether it is desirable or indeed possible to undertake a formal experimental investigation in which individuals and/or organisations are allocated to an intervention or control arm? Or whether the wish is to obtain a more naturalistic understanding of an issue? The former is ideally studied using a controlled experimental design, whereas the latter is more appropriately studied using a case study design.
Case studies may be approached in different ways depending on the epistemological standpoint of the researcher, that is, whether they take a critical (questioning one's own and others' assumptions), interpretivist (trying to understand individual and shared social meanings) or positivist approach (orientating towards the criteria of natural sciences, such as focusing on generalisability considerations) (Table 6 ). Whilst such a schema can be conceptually helpful, it may be appropriate to draw on more than one approach in any case study, particularly in the context of conducting health services research. Doolin has, for example, noted that in the context of undertaking interpretative case studies, researchers can usefully draw on a critical, reflective perspective which seeks to take into account the wider social and political environment that has shaped the case[ 11 ].
How are case studies conducted?
Here, we focus on the main stages of research activity when planning and undertaking a case study; the crucial stages are: defining the case; selecting the case(s); collecting and analysing the data; interpreting data; and reporting the findings.
Defining the case
Carefully formulated research question(s), informed by the existing literature and a prior appreciation of the theoretical issues and setting(s), are all important in appropriately and succinctly defining the case[ 8 , 12 ]. Crucially, each case should have a pre-defined boundary which clarifies the nature and time period covered by the case study (i.e. its scope, beginning and end), the relevant social group, organisation or geographical area of interest to the investigator, the types of evidence to be collected, and the priorities for data collection and analysis (see Table 7 )[ 1 ]. A theory driven approach to defining the case may help generate knowledge that is potentially transferable to a range of clinical contexts and behaviours; using theory is also likely to result in a more informed appreciation of, for example, how and why interventions have succeeded or failed[ 13 ].
For example, in our evaluation of the introduction of electronic health records in English hospitals (Table 3 ), we defined our cases as the NHS Trusts that were receiving the new technology[ 5 ]. Our focus was on how the technology was being implemented. However, if the primary research interest had been on the social and organisational dimensions of implementation, we might have defined our case differently as a grouping of healthcare professionals (e.g. doctors and/or nurses). The precise beginning and end of the case may however prove difficult to define. Pursuing this same example, when does the process of implementation and adoption of an electronic health record system really begin or end? Such judgements will inevitably be influenced by a range of factors, including the research question, theory of interest, the scope and richness of the gathered data and the resources available to the research team.
Selecting the case(s)
The decision on how to select the case(s) to study is a very important one that merits some reflection. In an intrinsic case study, the case is selected on its own merits[ 8 ]. The case is selected not because it is representative of other cases, but because of its uniqueness, which is of genuine interest to the researchers. This was, for example, the case in our study of the recruitment of minority ethnic participants into asthma research (Table 1 ) as our earlier work had demonstrated the marginalisation of minority ethnic people with asthma, despite evidence of disproportionate asthma morbidity[ 14 , 15 ]. In another example of an intrinsic case study, Hellstrom et al.[ 16 ] studied an elderly married couple living with dementia to explore how dementia had impacted on their understanding of home, their everyday life and their relationships.
For an instrumental case study, selecting a "typical" case can work well[ 8 ]. In contrast to the intrinsic case study, the particular case which is chosen is of less importance than selecting a case that allows the researcher to investigate an issue or phenomenon. For example, in order to gain an understanding of doctors' responses to health policy initiatives, Som undertook an instrumental case study interviewing clinicians who had a range of responsibilities for clinical governance in one NHS acute hospital trust[ 17 ]. Sampling a "deviant" or "atypical" case may however prove even more informative, potentially enabling the researcher to identify causal processes, generate hypotheses and develop theory.
In collective or multiple case studies, a number of cases are carefully selected. This offers the advantage of allowing comparisons to be made across several cases and/or replication. Choosing a "typical" case may enable the findings to be generalised to theory (i.e. analytical generalisation) or to test theory by replicating the findings in a second or even a third case (i.e. replication logic)[ 1 ]. Yin suggests two or three literal replications (i.e. predicting similar results) if the theory is straightforward and five or more if the theory is more subtle. However, critics might argue that selecting 'cases' in this way is insufficiently reflexive and ill-suited to the complexities of contemporary healthcare organisations.
The selected case study site(s) should allow the research team access to the group of individuals, the organisation, the processes or whatever else constitutes the chosen unit of analysis for the study. Access is therefore a central consideration; the researcher needs to come to know the case study site(s) well and to work cooperatively with them. Selected cases need to be not only interesting but also hospitable to the inquiry [ 8 ] if they are to be informative and answer the research question(s). Case study sites may also be pre-selected for the researcher, with decisions being influenced by key stakeholders. For example, our selection of case study sites in the evaluation of the implementation and adoption of electronic health record systems (see Table 3 ) was heavily influenced by NHS Connecting for Health, the government agency that was responsible for overseeing the National Programme for Information Technology (NPfIT)[ 5 ]. This prominent stakeholder had already selected the NHS sites (through a competitive bidding process) to be early adopters of the electronic health record systems and had negotiated contracts that detailed the deployment timelines.
It is also important to consider in advance the likely burden and risks associated with participation for those who (or the site(s) which) comprise the case study. Of particular importance is the obligation for the researcher to think through the ethical implications of the study (e.g. the risk of inadvertently breaching anonymity or confidentiality) and to ensure that potential participants/participating sites are provided with sufficient information to make an informed choice about joining the study. The outcome of providing this information might be that the emotive burden associated with participation, or the organisational disruption associated with supporting the fieldwork, is considered so high that the individuals or sites decide against participation.
In our example of evaluating implementations of electronic health record systems, given the restricted number of early adopter sites available to us, we sought purposively to select a diverse range of implementation cases among those that were available[ 5 ]. We chose a mixture of teaching, non-teaching and Foundation Trust hospitals, and examples of each of the three electronic health record systems procured centrally by the NPfIT. At one recruited site, it quickly became apparent that access was problematic because of competing demands on that organisation. Recognising the importance of full access and co-operative working for generating rich data, the research team decided not to pursue work at that site and instead to focus on other recruited sites.
Collecting the data
In order to develop a thorough understanding of the case, the case study approach usually involves the collection of multiple sources of evidence, using a range of quantitative (e.g. questionnaires, audits and analysis of routinely collected healthcare data) and more commonly qualitative techniques (e.g. interviews, focus groups and observations). The use of multiple sources of data (data triangulation) has been advocated as a way of increasing the internal validity of a study (i.e. the extent to which the method is appropriate to answer the research question)[ 8 , 18 – 21 ]. An underlying assumption is that data collected in different ways should lead to similar conclusions, and approaching the same issue from different angles can help develop a holistic picture of the phenomenon (Table 2 )[ 4 ].
Brazier and colleagues used a mixed-methods case study approach to investigate the impact of a cancer care programme[ 22 ]. Here, quantitative measures were collected with questionnaires before, and five months after, the start of the intervention which did not yield any statistically significant results. Qualitative interviews with patients however helped provide an insight into potentially beneficial process-related aspects of the programme, such as greater, perceived patient involvement in care. The authors reported how this case study approach provided a number of contextual factors likely to influence the effectiveness of the intervention and which were not likely to have been obtained from quantitative methods alone.
In collective or multiple case studies, data collection needs to be flexible enough to allow a detailed description of each individual case to be developed (e.g. the nature of different cancer care programmes), before considering the emerging similarities and differences in cross-case comparisons (e.g. to explore why one programme is more effective than another). It is important that data sources from different cases are, where possible, broadly comparable for this purpose even though they may vary in nature and depth.
Analysing, interpreting and reporting case studies
Making sense and offering a coherent interpretation of the typically disparate sources of data (whether qualitative alone or together with quantitative) is far from straightforward. Repeated reviewing and sorting of the voluminous and detail-rich data are integral to the process of analysis. In collective case studies, it is helpful to analyse data relating to the individual component cases first, before making comparisons across cases. Attention needs to be paid to variations within each case and, where relevant, the relationship between different causes, effects and outcomes[ 23 ]. Data will need to be organised and coded to allow the key issues, both derived from the literature and emerging from the dataset, to be easily retrieved at a later stage. An initial coding frame can help capture these issues and can be applied systematically to the whole dataset with the aid of a qualitative data analysis software package.
The Framework approach is a practical approach, comprising of five stages (familiarisation; identifying a thematic framework; indexing; charting; mapping and interpretation) , to managing and analysing large datasets particularly if time is limited, as was the case in our study of recruitment of South Asians into asthma research (Table 1 )[ 3 , 24 ]. Theoretical frameworks may also play an important role in integrating different sources of data and examining emerging themes. For example, we drew on a socio-technical framework to help explain the connections between different elements - technology; people; and the organisational settings within which they worked - in our study of the introduction of electronic health record systems (Table 3 )[ 5 ]. Our study of patient safety in undergraduate curricula drew on an evaluation-based approach to design and analysis, which emphasised the importance of the academic, organisational and practice contexts through which students learn (Table 4 )[ 6 ].
Case study findings can have implications both for theory development and theory testing. They may establish, strengthen or weaken historical explanations of a case and, in certain circumstances, allow theoretical (as opposed to statistical) generalisation beyond the particular cases studied[ 12 ]. These theoretical lenses should not, however, constitute a strait-jacket and the cases should not be "forced to fit" the particular theoretical framework that is being employed.
When reporting findings, it is important to provide the reader with enough contextual information to understand the processes that were followed and how the conclusions were reached. In a collective case study, researchers may choose to present the findings from individual cases separately before amalgamating across cases. Care must be taken to ensure the anonymity of both case sites and individual participants (if agreed in advance) by allocating appropriate codes or withholding descriptors. In the example given in Table 3 , we decided against providing detailed information on the NHS sites and individual participants in order to avoid the risk of inadvertent disclosure of identities[ 5 , 25 ].
What are the potential pitfalls and how can these be avoided?
The case study approach is, as with all research, not without its limitations. When investigating the formal and informal ways undergraduate students learn about patient safety (Table 4 ), for example, we rapidly accumulated a large quantity of data. The volume of data, together with the time restrictions in place, impacted on the depth of analysis that was possible within the available resources. This highlights a more general point of the importance of avoiding the temptation to collect as much data as possible; adequate time also needs to be set aside for data analysis and interpretation of what are often highly complex datasets.
Case study research has sometimes been criticised for lacking scientific rigour and providing little basis for generalisation (i.e. producing findings that may be transferable to other settings)[ 1 ]. There are several ways to address these concerns, including: the use of theoretical sampling (i.e. drawing on a particular conceptual framework); respondent validation (i.e. participants checking emerging findings and the researcher's interpretation, and providing an opinion as to whether they feel these are accurate); and transparency throughout the research process (see Table 8 )[ 8 , 18 – 21 , 23 , 26 ]. Transparency can be achieved by describing in detail the steps involved in case selection, data collection, the reasons for the particular methods chosen, and the researcher's background and level of involvement (i.e. being explicit about how the researcher has influenced data collection and interpretation). Seeking potential, alternative explanations, and being explicit about how interpretations and conclusions were reached, help readers to judge the trustworthiness of the case study report. Stake provides a critique checklist for a case study report (Table 9 )[ 8 ].
Conclusions
The case study approach allows, amongst other things, critical events, interventions, policy developments and programme-based service reforms to be studied in detail in a real-life context. It should therefore be considered when an experimental design is either inappropriate to answer the research questions posed or impossible to undertake. Considering the frequency with which implementations of innovations are now taking place in healthcare settings and how well the case study approach lends itself to in-depth, complex health service research, we believe this approach should be more widely considered by researchers. Though inherently challenging, the research case study can, if carefully conceptualised and thoughtfully undertaken and reported, yield powerful insights into many important aspects of health and healthcare delivery.
Yin RK: Case study research, design and method. 2009, London: Sage Publications Ltd., 4
Google Scholar
Keen J, Packwood T: Qualitative research; case study evaluation. BMJ. 1995, 311: 444-446.
Article CAS PubMed PubMed Central Google Scholar
Sheikh A, Halani L, Bhopal R, Netuveli G, Partridge M, Car J, et al: Facilitating the Recruitment of Minority Ethnic People into Research: Qualitative Case Study of South Asians and Asthma. PLoS Med. 2009, 6 (10): 1-11.
Article Google Scholar
Pinnock H, Huby G, Powell A, Kielmann T, Price D, Williams S, et al: The process of planning, development and implementation of a General Practitioner with a Special Interest service in Primary Care Organisations in England and Wales: a comparative prospective case study. Report for the National Co-ordinating Centre for NHS Service Delivery and Organisation R&D (NCCSDO). 2008, [ http://www.sdo.nihr.ac.uk/files/project/99-final-report.pdf ]
Robertson A, Cresswell K, Takian A, Petrakaki D, Crowe S, Cornford T, et al: Prospective evaluation of the implementation and adoption of NHS Connecting for Health's national electronic health record in secondary care in England: interim findings. BMJ. 2010, 41: c4564-
Pearson P, Steven A, Howe A, Sheikh A, Ashcroft D, Smith P, the Patient Safety Education Study Group: Learning about patient safety: organisational context and culture in the education of healthcare professionals. J Health Serv Res Policy. 2010, 15: 4-10. 10.1258/jhsrp.2009.009052.
Article PubMed Google Scholar
van Harten WH, Casparie TF, Fisscher OA: The evaluation of the introduction of a quality management system: a process-oriented case study in a large rehabilitation hospital. Health Policy. 2002, 60 (1): 17-37. 10.1016/S0168-8510(01)00187-7.
Stake RE: The art of case study research. 1995, London: Sage Publications Ltd.
Sheikh A, Smeeth L, Ashcroft R: Randomised controlled trials in primary care: scope and application. Br J Gen Pract. 2002, 52 (482): 746-51.
PubMed PubMed Central Google Scholar
King G, Keohane R, Verba S: Designing Social Inquiry. 1996, Princeton: Princeton University Press
Doolin B: Information technology as disciplinary technology: being critical in interpretative research on information systems. Journal of Information Technology. 1998, 13: 301-311. 10.1057/jit.1998.8.
George AL, Bennett A: Case studies and theory development in the social sciences. 2005, Cambridge, MA: MIT Press
Eccles M, the Improved Clinical Effectiveness through Behavioural Research Group (ICEBeRG): Designing theoretically-informed implementation interventions. Implementation Science. 2006, 1: 1-8. 10.1186/1748-5908-1-1.
Article PubMed Central Google Scholar
Netuveli G, Hurwitz B, Levy M, Fletcher M, Barnes G, Durham SR, Sheikh A: Ethnic variations in UK asthma frequency, morbidity, and health-service use: a systematic review and meta-analysis. Lancet. 2005, 365 (9456): 312-7.
Sheikh A, Panesar SS, Lasserson T, Netuveli G: Recruitment of ethnic minorities to asthma studies. Thorax. 2004, 59 (7): 634-
CAS PubMed PubMed Central Google Scholar
Hellström I, Nolan M, Lundh U: 'We do things together': A case study of 'couplehood' in dementia. Dementia. 2005, 4: 7-22. 10.1177/1471301205049188.
Som CV: Nothing seems to have changed, nothing seems to be changing and perhaps nothing will change in the NHS: doctors' response to clinical governance. International Journal of Public Sector Management. 2005, 18: 463-477. 10.1108/09513550510608903.
Lincoln Y, Guba E: Naturalistic inquiry. 1985, Newbury Park: Sage Publications
Barbour RS: Checklists for improving rigour in qualitative research: a case of the tail wagging the dog?. BMJ. 2001, 322: 1115-1117. 10.1136/bmj.322.7294.1115.
Mays N, Pope C: Qualitative research in health care: Assessing quality in qualitative research. BMJ. 2000, 320: 50-52. 10.1136/bmj.320.7226.50.
Mason J: Qualitative researching. 2002, London: Sage
Brazier A, Cooke K, Moravan V: Using Mixed Methods for Evaluating an Integrative Approach to Cancer Care: A Case Study. Integr Cancer Ther. 2008, 7: 5-17. 10.1177/1534735407313395.
Miles MB, Huberman M: Qualitative data analysis: an expanded sourcebook. 1994, CA: Sage Publications Inc., 2
Pope C, Ziebland S, Mays N: Analysing qualitative data. Qualitative research in health care. BMJ. 2000, 320: 114-116. 10.1136/bmj.320.7227.114.
Cresswell KM, Worth A, Sheikh A: Actor-Network Theory and its role in understanding the implementation of information technology developments in healthcare. BMC Med Inform Decis Mak. 2010, 10 (1): 67-10.1186/1472-6947-10-67.
Article PubMed PubMed Central Google Scholar
Malterud K: Qualitative research: standards, challenges, and guidelines. Lancet. 2001, 358: 483-488. 10.1016/S0140-6736(01)05627-6.
Article CAS PubMed Google Scholar
Yin R: Case study research: design and methods. 1994, Thousand Oaks, CA: Sage Publishing, 2
Yin R: Enhancing the quality of case studies in health services research. Health Serv Res. 1999, 34: 1209-1224.
Green J, Thorogood N: Qualitative methods for health research. 2009, Los Angeles: Sage, 2
Howcroft D, Trauth E: Handbook of Critical Information Systems Research, Theory and Application. 2005, Cheltenham, UK: Northampton, MA, USA: Edward Elgar
Book Google Scholar
Blakie N: Approaches to Social Enquiry. 1993, Cambridge: Polity Press
Doolin B: Power and resistance in the implementation of a medical management information system. Info Systems J. 2004, 14: 343-362. 10.1111/j.1365-2575.2004.00176.x.
Bloomfield BP, Best A: Management consultants: systems development, power and the translation of problems. Sociological Review. 1992, 40: 533-560.
Shanks G, Parr A: Positivist, single case study research in information systems: A critical analysis. Proceedings of the European Conference on Information Systems. 2003, Naples
Pre-publication history
The pre-publication history for this paper can be accessed here: http://www.biomedcentral.com/1471-2288/11/100/prepub
Download references
Acknowledgements
We are grateful to the participants and colleagues who contributed to the individual case studies that we have drawn on. This work received no direct funding, but it has been informed by projects funded by Asthma UK, the NHS Service Delivery Organisation, NHS Connecting for Health Evaluation Programme, and Patient Safety Research Portfolio. We would also like to thank the expert reviewers for their insightful and constructive feedback. Our thanks are also due to Dr. Allison Worth who commented on an earlier draft of this manuscript.
Author information
Authors and affiliations.
Division of Primary Care, The University of Nottingham, Nottingham, UK
Sarah Crowe & Anthony Avery
Centre for Population Health Sciences, The University of Edinburgh, Edinburgh, UK
Kathrin Cresswell, Ann Robertson & Aziz Sheikh
School of Health in Social Science, The University of Edinburgh, Edinburgh, UK
You can also search for this author in PubMed Google Scholar
Corresponding author
Correspondence to Sarah Crowe .
Additional information
Competing interests.
The authors declare that they have no competing interests.
Authors' contributions
AS conceived this article. SC, KC and AR wrote this paper with GH, AA and AS all commenting on various drafts. SC and AS are guarantors.
Rights and permissions
This article is published under license to BioMed Central Ltd. This is an Open Access article distributed under the terms of the Creative Commons Attribution License ( http://creativecommons.org/licenses/by/2.0 ), which permits unrestricted use, distribution, and reproduction in any medium, provided the original work is properly cited.
Reprints and permissions
About this article
Cite this article.
Crowe, S., Cresswell, K., Robertson, A. et al. The case study approach. BMC Med Res Methodol 11 , 100 (2011). https://doi.org/10.1186/1471-2288-11-100
Download citation
Received : 29 November 2010
Accepted : 27 June 2011
Published : 27 June 2011
DOI : https://doi.org/10.1186/1471-2288-11-100
Share this article
Anyone you share the following link with will be able to read this content:
Sorry, a shareable link is not currently available for this article.
Provided by the Springer Nature SharedIt content-sharing initiative
- Case Study Approach
- Electronic Health Record System
- Case Study Design
- Case Study Site
- Case Study Report
BMC Medical Research Methodology
ISSN: 1471-2288
- General enquiries: [email protected]
Have a language expert improve your writing
Run a free plagiarism check in 10 minutes, automatically generate references for free.
- Knowledge Base
- Methodology
- Case Study | Definition, Examples & Methods
Case Study | Definition, Examples & Methods
Published on 5 May 2022 by Shona McCombes . Revised on 30 January 2023.
A case study is a detailed study of a specific subject, such as a person, group, place, event, organisation, or phenomenon. Case studies are commonly used in social, educational, clinical, and business research.
A case study research design usually involves qualitative methods , but quantitative methods are sometimes also used. Case studies are good for describing , comparing, evaluating, and understanding different aspects of a research problem .
Table of contents
When to do a case study, step 1: select a case, step 2: build a theoretical framework, step 3: collect your data, step 4: describe and analyse the case.
A case study is an appropriate research design when you want to gain concrete, contextual, in-depth knowledge about a specific real-world subject. It allows you to explore the key characteristics, meanings, and implications of the case.
Case studies are often a good choice in a thesis or dissertation . They keep your project focused and manageable when you don’t have the time or resources to do large-scale research.
You might use just one complex case study where you explore a single subject in depth, or conduct multiple case studies to compare and illuminate different aspects of your research problem.
Research question | Case study |
---|---|
What are the ecological effects of wolf reintroduction? | Case study of wolf reintroduction in Yellowstone National Park in the US |
How do populist politicians use narratives about history to gain support? | Case studies of Hungarian prime minister Viktor Orbán and US president Donald Trump |
How can teachers implement active learning strategies in mixed-level classrooms? | Case study of a local school that promotes active learning |
What are the main advantages and disadvantages of wind farms for rural communities? | Case studies of three rural wind farm development projects in different parts of the country |
How are viral marketing strategies changing the relationship between companies and consumers? | Case study of the iPhone X marketing campaign |
How do experiences of work in the gig economy differ by gender, race, and age? | Case studies of Deliveroo and Uber drivers in London |
Prevent plagiarism, run a free check.
Once you have developed your problem statement and research questions , you should be ready to choose the specific case that you want to focus on. A good case study should have the potential to:
- Provide new or unexpected insights into the subject
- Challenge or complicate existing assumptions and theories
- Propose practical courses of action to resolve a problem
- Open up new directions for future research
Unlike quantitative or experimental research, a strong case study does not require a random or representative sample. In fact, case studies often deliberately focus on unusual, neglected, or outlying cases which may shed new light on the research problem.
If you find yourself aiming to simultaneously investigate and solve an issue, consider conducting action research . As its name suggests, action research conducts research and takes action at the same time, and is highly iterative and flexible.
However, you can also choose a more common or representative case to exemplify a particular category, experience, or phenomenon.
While case studies focus more on concrete details than general theories, they should usually have some connection with theory in the field. This way the case study is not just an isolated description, but is integrated into existing knowledge about the topic. It might aim to:
- Exemplify a theory by showing how it explains the case under investigation
- Expand on a theory by uncovering new concepts and ideas that need to be incorporated
- Challenge a theory by exploring an outlier case that doesn’t fit with established assumptions
To ensure that your analysis of the case has a solid academic grounding, you should conduct a literature review of sources related to the topic and develop a theoretical framework . This means identifying key concepts and theories to guide your analysis and interpretation.
There are many different research methods you can use to collect data on your subject. Case studies tend to focus on qualitative data using methods such as interviews, observations, and analysis of primary and secondary sources (e.g., newspaper articles, photographs, official records). Sometimes a case study will also collect quantitative data .
The aim is to gain as thorough an understanding as possible of the case and its context.
In writing up the case study, you need to bring together all the relevant aspects to give as complete a picture as possible of the subject.
How you report your findings depends on the type of research you are doing. Some case studies are structured like a standard scientific paper or thesis, with separate sections or chapters for the methods , results , and discussion .
Others are written in a more narrative style, aiming to explore the case from various angles and analyse its meanings and implications (for example, by using textual analysis or discourse analysis ).
In all cases, though, make sure to give contextual details about the case, connect it back to the literature and theory, and discuss how it fits into wider patterns or debates.
Cite this Scribbr article
If you want to cite this source, you can copy and paste the citation or click the ‘Cite this Scribbr article’ button to automatically add the citation to our free Reference Generator.
McCombes, S. (2023, January 30). Case Study | Definition, Examples & Methods. Scribbr. Retrieved 21 August 2024, from https://www.scribbr.co.uk/research-methods/case-studies/
Is this article helpful?
Research Writing and Analysis
- NVivo Group and Study Sessions
- SPSS This link opens in a new window
- Statistical Analysis Group sessions
- Using Qualtrics
- Dissertation and Data Analysis Group Sessions
- Defense Schedule - Commons Calendar This link opens in a new window
- Research Process Flow Chart
- Research Alignment Chapter 1 This link opens in a new window
- Step 1: Seek Out Evidence
- Step 2: Explain
- Step 3: The Big Picture
- Step 4: Own It
- Step 5: Illustrate
- Annotated Bibliography
- Literature Review This link opens in a new window
- Systematic Reviews & Meta-Analyses
- How to Synthesize and Analyze
- Synthesis and Analysis Practice
- Synthesis and Analysis Group Sessions
- Problem Statement
- Purpose Statement
- Conceptual Framework
- Theoretical Framework
- Locating Theoretical and Conceptual Frameworks This link opens in a new window
- Quantitative Research Questions
- Qualitative Research Questions
- Trustworthiness of Qualitative Data
- Analysis and Coding Example- Qualitative Data
- Thematic Data Analysis in Qualitative Design
- Dissertation to Journal Article This link opens in a new window
- International Journal of Online Graduate Education (IJOGE) This link opens in a new window
- Journal of Research in Innovative Teaching & Learning (JRIT&L) This link opens in a new window
Writing a Case Study

What is a case study?

A Case study is:
- An in-depth research design that primarily uses a qualitative methodology but sometimes includes quantitative methodology.
- Used to examine an identifiable problem confirmed through research.
- Used to investigate an individual, group of people, organization, or event.
- Used to mostly answer "how" and "why" questions.
What are the different types of case studies?

Descriptive | This type of case study allows the researcher to: | How has the implementation and use of the instructional coaching intervention for elementary teachers impacted students’ attitudes toward reading? |
Explanatory | This type of case study allows the researcher to: | Why do differences exist when implementing the same online reading curriculum in three elementary classrooms? |
Exploratory | This type of case study allows the researcher to:
| What are potential barriers to student’s reading success when middle school teachers implement the Ready Reader curriculum online? |
Multiple Case Studies or Collective Case Study | This type of case study allows the researcher to: | How are individual school districts addressing student engagement in an online classroom? |
Intrinsic | This type of case study allows the researcher to: | How does a student’s familial background influence a teacher’s ability to provide meaningful instruction? |
Instrumental | This type of case study allows the researcher to: | How a rural school district’s integration of a reward system maximized student engagement? |
Note: These are the primary case studies. As you continue to research and learn
about case studies you will begin to find a robust list of different types.
Who are your case study participants?

|
This type of study is implemented to understand an individual by developing a detailed explanation of the individual’s lived experiences or perceptions.
|
| This type of study is implemented to explore a particular group of people’s perceptions. |
| This type of study is implemented to explore the perspectives of people who work for or had interaction with a specific organization or company. |
| This type of study is implemented to explore participant’s perceptions of an event. |
What is triangulation ?
Validity and credibility are an essential part of the case study. Therefore, the researcher should include triangulation to ensure trustworthiness while accurately reflecting what the researcher seeks to investigate.

How to write a Case Study?
When developing a case study, there are different ways you could present the information, but remember to include the five parts for your case study.

|
|
|
|
|

Was this resource helpful?
- << Previous: Thematic Data Analysis in Qualitative Design
- Next: Journal Article Reporting Standards (JARS) >>
- Last Updated: Aug 21, 2024 7:54 AM
- URL: https://resources.nu.edu/researchtools


An official website of the United States government
The .gov means it’s official. Federal government websites often end in .gov or .mil. Before sharing sensitive information, make sure you’re on a federal government site.
The site is secure. The https:// ensures that you are connecting to the official website and that any information you provide is encrypted and transmitted securely.
- Publications
- Account settings
Preview improvements coming to the PMC website in October 2024. Learn More or Try it out now .
- Advanced Search
- Journal List
- BMC Med Res Methodol

The case study approach
Sarah crowe.
1 Division of Primary Care, The University of Nottingham, Nottingham, UK
Kathrin Cresswell
2 Centre for Population Health Sciences, The University of Edinburgh, Edinburgh, UK
Ann Robertson
3 School of Health in Social Science, The University of Edinburgh, Edinburgh, UK
Anthony Avery
Aziz sheikh.
The case study approach allows in-depth, multi-faceted explorations of complex issues in their real-life settings. The value of the case study approach is well recognised in the fields of business, law and policy, but somewhat less so in health services research. Based on our experiences of conducting several health-related case studies, we reflect on the different types of case study design, the specific research questions this approach can help answer, the data sources that tend to be used, and the particular advantages and disadvantages of employing this methodological approach. The paper concludes with key pointers to aid those designing and appraising proposals for conducting case study research, and a checklist to help readers assess the quality of case study reports.
Introduction
The case study approach is particularly useful to employ when there is a need to obtain an in-depth appreciation of an issue, event or phenomenon of interest, in its natural real-life context. Our aim in writing this piece is to provide insights into when to consider employing this approach and an overview of key methodological considerations in relation to the design, planning, analysis, interpretation and reporting of case studies.
The illustrative 'grand round', 'case report' and 'case series' have a long tradition in clinical practice and research. Presenting detailed critiques, typically of one or more patients, aims to provide insights into aspects of the clinical case and, in doing so, illustrate broader lessons that may be learnt. In research, the conceptually-related case study approach can be used, for example, to describe in detail a patient's episode of care, explore professional attitudes to and experiences of a new policy initiative or service development or more generally to 'investigate contemporary phenomena within its real-life context' [ 1 ]. Based on our experiences of conducting a range of case studies, we reflect on when to consider using this approach, discuss the key steps involved and illustrate, with examples, some of the practical challenges of attaining an in-depth understanding of a 'case' as an integrated whole. In keeping with previously published work, we acknowledge the importance of theory to underpin the design, selection, conduct and interpretation of case studies[ 2 ]. In so doing, we make passing reference to the different epistemological approaches used in case study research by key theoreticians and methodologists in this field of enquiry.
This paper is structured around the following main questions: What is a case study? What are case studies used for? How are case studies conducted? What are the potential pitfalls and how can these be avoided? We draw in particular on four of our own recently published examples of case studies (see Tables Tables1, 1 , ,2, 2 , ,3 3 and and4) 4 ) and those of others to illustrate our discussion[ 3 - 7 ].
Example of a case study investigating the reasons for differences in recruitment rates of minority ethnic people in asthma research[ 3 ]
Minority ethnic people experience considerably greater morbidity from asthma than the White majority population. Research has shown however that these minority ethnic populations are likely to be under-represented in research undertaken in the UK; there is comparatively less marginalisation in the US. |
To investigate approaches to bolster recruitment of South Asians into UK asthma studies through qualitative research with US and UK researchers, and UK community leaders. |
Single intrinsic case study |
Centred on the issue of recruitment of South Asian people with asthma. |
In-depth interviews were conducted with asthma researchers from the UK and US. A supplementary questionnaire was also provided to researchers. |
Framework approach. |
Barriers to ethnic minority recruitment were found to centre around: |
1. The attitudes of the researchers' towards inclusion: The majority of UK researchers interviewed were generally supportive of the idea of recruiting ethnically diverse participants but expressed major concerns about the practicalities of achieving this; in contrast, the US researchers appeared much more committed to the policy of inclusion. |
2. Stereotypes and prejudices: We found that some of the UK researchers' perceptions of ethnic minorities may have influenced their decisions on whether to approach individuals from particular ethnic groups. These stereotypes centred on issues to do with, amongst others, language barriers and lack of altruism. |
3. Demographic, political and socioeconomic contexts of the two countries: Researchers suggested that the demographic profile of ethnic minorities, their political engagement and the different configuration of the health services in the UK and the US may have contributed to differential rates. |
4. Above all, however, it appeared that the overriding importance of the US National Institute of Health's policy to mandate the inclusion of minority ethnic people (and women) had a major impact on shaping the attitudes and in turn the experiences of US researchers'; the absence of any similar mandate in the UK meant that UK-based researchers had not been forced to challenge their existing practices and they were hence unable to overcome any stereotypical/prejudicial attitudes through experiential learning. |
Example of a case study investigating the process of planning and implementing a service in Primary Care Organisations[ 4 ]
Health work forces globally are needing to reorganise and reconfigure in order to meet the challenges posed by the increased numbers of people living with long-term conditions in an efficient and sustainable manner. Through studying the introduction of General Practitioners with a Special Interest in respiratory disorders, this study aimed to provide insights into this important issue by focusing on community respiratory service development. |
To understand and compare the process of workforce change in respiratory services and the impact on patient experience (specifically in relation to the role of general practitioners with special interests) in a theoretically selected sample of Primary Care Organisations (PCOs), in order to derive models of good practice in planning and the implementation of a broad range of workforce issues. |
Multiple-case design of respiratory services in health regions in England and Wales. |
Four PCOs. |
Face-to-face and telephone interviews, e-mail discussions, local documents, patient diaries, news items identified from local and national websites, national workshop. |
Reading, coding and comparison progressed iteratively. |
1. In the screening phase of this study (which involved semi-structured telephone interviews with the person responsible for driving the reconfiguration of respiratory services in 30 PCOs), the barriers of financial deficit, organisational uncertainty, disengaged clinicians and contradictory policies proved insurmountable for many PCOs to developing sustainable services. A key rationale for PCO re-organisation in 2006 was to strengthen their commissioning function and those of clinicians through Practice-Based Commissioning. However, the turbulence, which surrounded reorganisation was found to have the opposite desired effect. |
2. Implementing workforce reconfiguration was strongly influenced by the negotiation and contest among local clinicians and managers about "ownership" of work and income. |
3. Despite the intention to make the commissioning system more transparent, personal relationships based on common professional interests, past work history, friendships and collegiality, remained as key drivers for sustainable innovation in service development. |
It was only possible to undertake in-depth work in a selective number of PCOs and, even within these selected PCOs, it was not possible to interview all informants of potential interest and/or obtain all relevant documents. This work was conducted in the early stages of a major NHS reorganisation in England and Wales and thus, events are likely to have continued to evolve beyond the study period; we therefore cannot claim to have seen any of the stories through to their conclusion. |
Example of a case study investigating the introduction of the electronic health records[ 5 ]
Healthcare systems globally are moving from paper-based record systems to electronic health record systems. In 2002, the NHS in England embarked on the most ambitious and expensive IT-based transformation in healthcare in history seeking to introduce electronic health records into all hospitals in England by 2010. |
To describe and evaluate the implementation and adoption of detailed electronic health records in secondary care in England and thereby provide formative feedback for local and national rollout of the NHS Care Records Service. |
A mixed methods, longitudinal, multi-site, socio-technical collective case study. |
Five NHS acute hospital and mental health Trusts that have been the focus of early implementation efforts. |
Semi-structured interviews, documentary data and field notes, observations and quantitative data. |
Qualitative data were analysed thematically using a socio-technical coding matrix, combined with additional themes that emerged from the data. |
1. Hospital electronic health record systems have developed and been implemented far more slowly than was originally envisioned. |
2. The top-down, government-led standardised approach needed to evolve to admit more variation and greater local choice for hospitals in order to support local service delivery. |
3. A range of adverse consequences were associated with the centrally negotiated contracts, which excluded the hospitals in question. |
4. The unrealistic, politically driven, timeline (implementation over 10 years) was found to be a major source of frustration for developers, implementers and healthcare managers and professionals alike. |
We were unable to access details of the contracts between government departments and the Local Service Providers responsible for delivering and implementing the software systems. This, in turn, made it difficult to develop a holistic understanding of some key issues impacting on the overall slow roll-out of the NHS Care Record Service. Early adopters may also have differed in important ways from NHS hospitals that planned to join the National Programme for Information Technology and implement the NHS Care Records Service at a later point in time. |
Example of a case study investigating the formal and informal ways students learn about patient safety[ 6 ]
There is a need to reduce the disease burden associated with iatrogenic harm and considering that healthcare education represents perhaps the most sustained patient safety initiative ever undertaken, it is important to develop a better appreciation of the ways in which undergraduate and newly qualified professionals receive and make sense of the education they receive. | |
---|---|
To investigate the formal and informal ways pre-registration students from a range of healthcare professions (medicine, nursing, physiotherapy and pharmacy) learn about patient safety in order to become safe practitioners. | |
Multi-site, mixed method collective case study. | |
: Eight case studies (two for each professional group) were carried out in educational provider sites considering different programmes, practice environments and models of teaching and learning. | |
Structured in phases relevant to the three knowledge contexts: | |
Documentary evidence (including undergraduate curricula, handbooks and module outlines), complemented with a range of views (from course leads, tutors and students) and observations in a range of academic settings. | |
Policy and management views of patient safety and influences on patient safety education and practice. NHS policies included, for example, implementation of the National Patient Safety Agency's , which encourages organisations to develop an organisational safety culture in which staff members feel comfortable identifying dangers and reporting hazards. | |
The cultures to which students are exposed i.e. patient safety in relation to day-to-day working. NHS initiatives included, for example, a hand washing initiative or introduction of infection control measures. | |
1. Practical, informal, learning opportunities were valued by students. On the whole, however, students were not exposed to nor engaged with important NHS initiatives such as risk management activities and incident reporting schemes. | |
2. NHS policy appeared to have been taken seriously by course leaders. Patient safety materials were incorporated into both formal and informal curricula, albeit largely implicit rather than explicit. | |
3. Resource issues and peer pressure were found to influence safe practice. Variations were also found to exist in students' experiences and the quality of the supervision available. | |
The curriculum and organisational documents collected differed between sites, which possibly reflected gatekeeper influences at each site. The recruitment of participants for focus group discussions proved difficult, so interviews or paired discussions were used as a substitute. |
What is a case study?
A case study is a research approach that is used to generate an in-depth, multi-faceted understanding of a complex issue in its real-life context. It is an established research design that is used extensively in a wide variety of disciplines, particularly in the social sciences. A case study can be defined in a variety of ways (Table (Table5), 5 ), the central tenet being the need to explore an event or phenomenon in depth and in its natural context. It is for this reason sometimes referred to as a "naturalistic" design; this is in contrast to an "experimental" design (such as a randomised controlled trial) in which the investigator seeks to exert control over and manipulate the variable(s) of interest.
Definitions of a case study
Author | Definition |
---|---|
Stake[ ] | (p.237) |
Yin[ , , ] | (Yin 1999 p. 1211, Yin 1994 p. 13) |
• | |
• (Yin 2009 p18) | |
Miles and Huberman[ ] | (p. 25) |
Green and Thorogood[ ] | (p. 284) |
George and Bennett[ ] | (p. 17)" |
Stake's work has been particularly influential in defining the case study approach to scientific enquiry. He has helpfully characterised three main types of case study: intrinsic , instrumental and collective [ 8 ]. An intrinsic case study is typically undertaken to learn about a unique phenomenon. The researcher should define the uniqueness of the phenomenon, which distinguishes it from all others. In contrast, the instrumental case study uses a particular case (some of which may be better than others) to gain a broader appreciation of an issue or phenomenon. The collective case study involves studying multiple cases simultaneously or sequentially in an attempt to generate a still broader appreciation of a particular issue.
These are however not necessarily mutually exclusive categories. In the first of our examples (Table (Table1), 1 ), we undertook an intrinsic case study to investigate the issue of recruitment of minority ethnic people into the specific context of asthma research studies, but it developed into a instrumental case study through seeking to understand the issue of recruitment of these marginalised populations more generally, generating a number of the findings that are potentially transferable to other disease contexts[ 3 ]. In contrast, the other three examples (see Tables Tables2, 2 , ,3 3 and and4) 4 ) employed collective case study designs to study the introduction of workforce reconfiguration in primary care, the implementation of electronic health records into hospitals, and to understand the ways in which healthcare students learn about patient safety considerations[ 4 - 6 ]. Although our study focusing on the introduction of General Practitioners with Specialist Interests (Table (Table2) 2 ) was explicitly collective in design (four contrasting primary care organisations were studied), is was also instrumental in that this particular professional group was studied as an exemplar of the more general phenomenon of workforce redesign[ 4 ].
What are case studies used for?
According to Yin, case studies can be used to explain, describe or explore events or phenomena in the everyday contexts in which they occur[ 1 ]. These can, for example, help to understand and explain causal links and pathways resulting from a new policy initiative or service development (see Tables Tables2 2 and and3, 3 , for example)[ 1 ]. In contrast to experimental designs, which seek to test a specific hypothesis through deliberately manipulating the environment (like, for example, in a randomised controlled trial giving a new drug to randomly selected individuals and then comparing outcomes with controls),[ 9 ] the case study approach lends itself well to capturing information on more explanatory ' how ', 'what' and ' why ' questions, such as ' how is the intervention being implemented and received on the ground?'. The case study approach can offer additional insights into what gaps exist in its delivery or why one implementation strategy might be chosen over another. This in turn can help develop or refine theory, as shown in our study of the teaching of patient safety in undergraduate curricula (Table (Table4 4 )[ 6 , 10 ]. Key questions to consider when selecting the most appropriate study design are whether it is desirable or indeed possible to undertake a formal experimental investigation in which individuals and/or organisations are allocated to an intervention or control arm? Or whether the wish is to obtain a more naturalistic understanding of an issue? The former is ideally studied using a controlled experimental design, whereas the latter is more appropriately studied using a case study design.
Case studies may be approached in different ways depending on the epistemological standpoint of the researcher, that is, whether they take a critical (questioning one's own and others' assumptions), interpretivist (trying to understand individual and shared social meanings) or positivist approach (orientating towards the criteria of natural sciences, such as focusing on generalisability considerations) (Table (Table6). 6 ). Whilst such a schema can be conceptually helpful, it may be appropriate to draw on more than one approach in any case study, particularly in the context of conducting health services research. Doolin has, for example, noted that in the context of undertaking interpretative case studies, researchers can usefully draw on a critical, reflective perspective which seeks to take into account the wider social and political environment that has shaped the case[ 11 ].
Example of epistemological approaches that may be used in case study research
Approach | Characteristics | Criticisms | Key references |
---|---|---|---|
Involves questioning one's own assumptions taking into account the wider political and social environment. | It can possibly neglect other factors by focussing only on power relationships and may give the researcher a position that is too privileged. | Howcroft and Trauth[ ] Blakie[ ] Doolin[ , ] | |
Interprets the limiting conditions in relation to power and control that are thought to influence behaviour. | Bloomfield and Best[ ] | ||
Involves understanding meanings/contexts and processes as perceived from different perspectives, trying to understand individual and shared social meanings. Focus is on theory building. | Often difficult to explain unintended consequences and for neglecting surrounding historical contexts | Stake[ ] Doolin[ ] | |
Involves establishing which variables one wishes to study in advance and seeing whether they fit in with the findings. Focus is often on testing and refining theory on the basis of case study findings. | It does not take into account the role of the researcher in influencing findings. | Yin[ , , ] Shanks and Parr[ ] |
How are case studies conducted?
Here, we focus on the main stages of research activity when planning and undertaking a case study; the crucial stages are: defining the case; selecting the case(s); collecting and analysing the data; interpreting data; and reporting the findings.
Defining the case
Carefully formulated research question(s), informed by the existing literature and a prior appreciation of the theoretical issues and setting(s), are all important in appropriately and succinctly defining the case[ 8 , 12 ]. Crucially, each case should have a pre-defined boundary which clarifies the nature and time period covered by the case study (i.e. its scope, beginning and end), the relevant social group, organisation or geographical area of interest to the investigator, the types of evidence to be collected, and the priorities for data collection and analysis (see Table Table7 7 )[ 1 ]. A theory driven approach to defining the case may help generate knowledge that is potentially transferable to a range of clinical contexts and behaviours; using theory is also likely to result in a more informed appreciation of, for example, how and why interventions have succeeded or failed[ 13 ].
Example of a checklist for rating a case study proposal[ 8 ]
Clarity: Does the proposal read well? | |
Integrity: Do its pieces fit together? | |
Attractiveness: Does it pique the reader's interest? | |
The case: Is the case adequately defined? | |
The issues: Are major research questions identified? | |
Data Resource: Are sufficient data sources identified? | |
Case Selection: Is the selection plan reasonable? | |
Data Gathering: Are data-gathering activities outlined? | |
Validation: Is the need and opportunity for triangulation indicated? | |
Access: Are arrangements for start-up anticipated? | |
Confidentiality: Is there sensitivity to the protection of people? | |
Cost: Are time and resource estimates reasonable? |
For example, in our evaluation of the introduction of electronic health records in English hospitals (Table (Table3), 3 ), we defined our cases as the NHS Trusts that were receiving the new technology[ 5 ]. Our focus was on how the technology was being implemented. However, if the primary research interest had been on the social and organisational dimensions of implementation, we might have defined our case differently as a grouping of healthcare professionals (e.g. doctors and/or nurses). The precise beginning and end of the case may however prove difficult to define. Pursuing this same example, when does the process of implementation and adoption of an electronic health record system really begin or end? Such judgements will inevitably be influenced by a range of factors, including the research question, theory of interest, the scope and richness of the gathered data and the resources available to the research team.
Selecting the case(s)
The decision on how to select the case(s) to study is a very important one that merits some reflection. In an intrinsic case study, the case is selected on its own merits[ 8 ]. The case is selected not because it is representative of other cases, but because of its uniqueness, which is of genuine interest to the researchers. This was, for example, the case in our study of the recruitment of minority ethnic participants into asthma research (Table (Table1) 1 ) as our earlier work had demonstrated the marginalisation of minority ethnic people with asthma, despite evidence of disproportionate asthma morbidity[ 14 , 15 ]. In another example of an intrinsic case study, Hellstrom et al.[ 16 ] studied an elderly married couple living with dementia to explore how dementia had impacted on their understanding of home, their everyday life and their relationships.
For an instrumental case study, selecting a "typical" case can work well[ 8 ]. In contrast to the intrinsic case study, the particular case which is chosen is of less importance than selecting a case that allows the researcher to investigate an issue or phenomenon. For example, in order to gain an understanding of doctors' responses to health policy initiatives, Som undertook an instrumental case study interviewing clinicians who had a range of responsibilities for clinical governance in one NHS acute hospital trust[ 17 ]. Sampling a "deviant" or "atypical" case may however prove even more informative, potentially enabling the researcher to identify causal processes, generate hypotheses and develop theory.
In collective or multiple case studies, a number of cases are carefully selected. This offers the advantage of allowing comparisons to be made across several cases and/or replication. Choosing a "typical" case may enable the findings to be generalised to theory (i.e. analytical generalisation) or to test theory by replicating the findings in a second or even a third case (i.e. replication logic)[ 1 ]. Yin suggests two or three literal replications (i.e. predicting similar results) if the theory is straightforward and five or more if the theory is more subtle. However, critics might argue that selecting 'cases' in this way is insufficiently reflexive and ill-suited to the complexities of contemporary healthcare organisations.
The selected case study site(s) should allow the research team access to the group of individuals, the organisation, the processes or whatever else constitutes the chosen unit of analysis for the study. Access is therefore a central consideration; the researcher needs to come to know the case study site(s) well and to work cooperatively with them. Selected cases need to be not only interesting but also hospitable to the inquiry [ 8 ] if they are to be informative and answer the research question(s). Case study sites may also be pre-selected for the researcher, with decisions being influenced by key stakeholders. For example, our selection of case study sites in the evaluation of the implementation and adoption of electronic health record systems (see Table Table3) 3 ) was heavily influenced by NHS Connecting for Health, the government agency that was responsible for overseeing the National Programme for Information Technology (NPfIT)[ 5 ]. This prominent stakeholder had already selected the NHS sites (through a competitive bidding process) to be early adopters of the electronic health record systems and had negotiated contracts that detailed the deployment timelines.
It is also important to consider in advance the likely burden and risks associated with participation for those who (or the site(s) which) comprise the case study. Of particular importance is the obligation for the researcher to think through the ethical implications of the study (e.g. the risk of inadvertently breaching anonymity or confidentiality) and to ensure that potential participants/participating sites are provided with sufficient information to make an informed choice about joining the study. The outcome of providing this information might be that the emotive burden associated with participation, or the organisational disruption associated with supporting the fieldwork, is considered so high that the individuals or sites decide against participation.
In our example of evaluating implementations of electronic health record systems, given the restricted number of early adopter sites available to us, we sought purposively to select a diverse range of implementation cases among those that were available[ 5 ]. We chose a mixture of teaching, non-teaching and Foundation Trust hospitals, and examples of each of the three electronic health record systems procured centrally by the NPfIT. At one recruited site, it quickly became apparent that access was problematic because of competing demands on that organisation. Recognising the importance of full access and co-operative working for generating rich data, the research team decided not to pursue work at that site and instead to focus on other recruited sites.
Collecting the data
In order to develop a thorough understanding of the case, the case study approach usually involves the collection of multiple sources of evidence, using a range of quantitative (e.g. questionnaires, audits and analysis of routinely collected healthcare data) and more commonly qualitative techniques (e.g. interviews, focus groups and observations). The use of multiple sources of data (data triangulation) has been advocated as a way of increasing the internal validity of a study (i.e. the extent to which the method is appropriate to answer the research question)[ 8 , 18 - 21 ]. An underlying assumption is that data collected in different ways should lead to similar conclusions, and approaching the same issue from different angles can help develop a holistic picture of the phenomenon (Table (Table2 2 )[ 4 ].
Brazier and colleagues used a mixed-methods case study approach to investigate the impact of a cancer care programme[ 22 ]. Here, quantitative measures were collected with questionnaires before, and five months after, the start of the intervention which did not yield any statistically significant results. Qualitative interviews with patients however helped provide an insight into potentially beneficial process-related aspects of the programme, such as greater, perceived patient involvement in care. The authors reported how this case study approach provided a number of contextual factors likely to influence the effectiveness of the intervention and which were not likely to have been obtained from quantitative methods alone.
In collective or multiple case studies, data collection needs to be flexible enough to allow a detailed description of each individual case to be developed (e.g. the nature of different cancer care programmes), before considering the emerging similarities and differences in cross-case comparisons (e.g. to explore why one programme is more effective than another). It is important that data sources from different cases are, where possible, broadly comparable for this purpose even though they may vary in nature and depth.
Analysing, interpreting and reporting case studies
Making sense and offering a coherent interpretation of the typically disparate sources of data (whether qualitative alone or together with quantitative) is far from straightforward. Repeated reviewing and sorting of the voluminous and detail-rich data are integral to the process of analysis. In collective case studies, it is helpful to analyse data relating to the individual component cases first, before making comparisons across cases. Attention needs to be paid to variations within each case and, where relevant, the relationship between different causes, effects and outcomes[ 23 ]. Data will need to be organised and coded to allow the key issues, both derived from the literature and emerging from the dataset, to be easily retrieved at a later stage. An initial coding frame can help capture these issues and can be applied systematically to the whole dataset with the aid of a qualitative data analysis software package.
The Framework approach is a practical approach, comprising of five stages (familiarisation; identifying a thematic framework; indexing; charting; mapping and interpretation) , to managing and analysing large datasets particularly if time is limited, as was the case in our study of recruitment of South Asians into asthma research (Table (Table1 1 )[ 3 , 24 ]. Theoretical frameworks may also play an important role in integrating different sources of data and examining emerging themes. For example, we drew on a socio-technical framework to help explain the connections between different elements - technology; people; and the organisational settings within which they worked - in our study of the introduction of electronic health record systems (Table (Table3 3 )[ 5 ]. Our study of patient safety in undergraduate curricula drew on an evaluation-based approach to design and analysis, which emphasised the importance of the academic, organisational and practice contexts through which students learn (Table (Table4 4 )[ 6 ].
Case study findings can have implications both for theory development and theory testing. They may establish, strengthen or weaken historical explanations of a case and, in certain circumstances, allow theoretical (as opposed to statistical) generalisation beyond the particular cases studied[ 12 ]. These theoretical lenses should not, however, constitute a strait-jacket and the cases should not be "forced to fit" the particular theoretical framework that is being employed.
When reporting findings, it is important to provide the reader with enough contextual information to understand the processes that were followed and how the conclusions were reached. In a collective case study, researchers may choose to present the findings from individual cases separately before amalgamating across cases. Care must be taken to ensure the anonymity of both case sites and individual participants (if agreed in advance) by allocating appropriate codes or withholding descriptors. In the example given in Table Table3, 3 , we decided against providing detailed information on the NHS sites and individual participants in order to avoid the risk of inadvertent disclosure of identities[ 5 , 25 ].
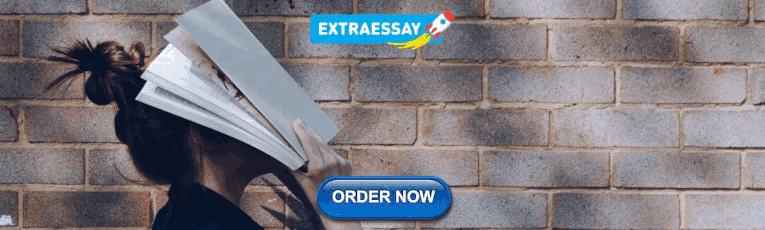
What are the potential pitfalls and how can these be avoided?
The case study approach is, as with all research, not without its limitations. When investigating the formal and informal ways undergraduate students learn about patient safety (Table (Table4), 4 ), for example, we rapidly accumulated a large quantity of data. The volume of data, together with the time restrictions in place, impacted on the depth of analysis that was possible within the available resources. This highlights a more general point of the importance of avoiding the temptation to collect as much data as possible; adequate time also needs to be set aside for data analysis and interpretation of what are often highly complex datasets.
Case study research has sometimes been criticised for lacking scientific rigour and providing little basis for generalisation (i.e. producing findings that may be transferable to other settings)[ 1 ]. There are several ways to address these concerns, including: the use of theoretical sampling (i.e. drawing on a particular conceptual framework); respondent validation (i.e. participants checking emerging findings and the researcher's interpretation, and providing an opinion as to whether they feel these are accurate); and transparency throughout the research process (see Table Table8 8 )[ 8 , 18 - 21 , 23 , 26 ]. Transparency can be achieved by describing in detail the steps involved in case selection, data collection, the reasons for the particular methods chosen, and the researcher's background and level of involvement (i.e. being explicit about how the researcher has influenced data collection and interpretation). Seeking potential, alternative explanations, and being explicit about how interpretations and conclusions were reached, help readers to judge the trustworthiness of the case study report. Stake provides a critique checklist for a case study report (Table (Table9 9 )[ 8 ].
Potential pitfalls and mitigating actions when undertaking case study research
Potential pitfall | Mitigating action |
---|---|
Selecting/conceptualising the wrong case(s) resulting in lack of theoretical generalisations | Developing in-depth knowledge of theoretical and empirical literature, justifying choices made |
Collecting large volumes of data that are not relevant to the case or too little to be of any value | Focus data collection in line with research questions, whilst being flexible and allowing different paths to be explored |
Defining/bounding the case | Focus on related components (either by time and/or space), be clear what is outside the scope of the case |
Lack of rigour | Triangulation, respondent validation, the use of theoretical sampling, transparency throughout the research process |
Ethical issues | Anonymise appropriately as cases are often easily identifiable to insiders, informed consent of participants |
Integration with theoretical framework | Allow for unexpected issues to emerge and do not force fit, test out preliminary explanations, be clear about epistemological positions in advance |
Stake's checklist for assessing the quality of a case study report[ 8 ]
1. Is this report easy to read? |
2. Does it fit together, each sentence contributing to the whole? |
3. Does this report have a conceptual structure (i.e. themes or issues)? |
4. Are its issues developed in a series and scholarly way? |
5. Is the case adequately defined? |
6. Is there a sense of story to the presentation? |
7. Is the reader provided some vicarious experience? |
8. Have quotations been used effectively? |
9. Are headings, figures, artefacts, appendices, indexes effectively used? |
10. Was it edited well, then again with a last minute polish? |
11. Has the writer made sound assertions, neither over- or under-interpreting? |
12. Has adequate attention been paid to various contexts? |
13. Were sufficient raw data presented? |
14. Were data sources well chosen and in sufficient number? |
15. Do observations and interpretations appear to have been triangulated? |
16. Is the role and point of view of the researcher nicely apparent? |
17. Is the nature of the intended audience apparent? |
18. Is empathy shown for all sides? |
19. Are personal intentions examined? |
20. Does it appear individuals were put at risk? |
Conclusions
The case study approach allows, amongst other things, critical events, interventions, policy developments and programme-based service reforms to be studied in detail in a real-life context. It should therefore be considered when an experimental design is either inappropriate to answer the research questions posed or impossible to undertake. Considering the frequency with which implementations of innovations are now taking place in healthcare settings and how well the case study approach lends itself to in-depth, complex health service research, we believe this approach should be more widely considered by researchers. Though inherently challenging, the research case study can, if carefully conceptualised and thoughtfully undertaken and reported, yield powerful insights into many important aspects of health and healthcare delivery.
Competing interests
The authors declare that they have no competing interests.
Authors' contributions
AS conceived this article. SC, KC and AR wrote this paper with GH, AA and AS all commenting on various drafts. SC and AS are guarantors.
Pre-publication history
The pre-publication history for this paper can be accessed here:
http://www.biomedcentral.com/1471-2288/11/100/prepub
Acknowledgements
We are grateful to the participants and colleagues who contributed to the individual case studies that we have drawn on. This work received no direct funding, but it has been informed by projects funded by Asthma UK, the NHS Service Delivery Organisation, NHS Connecting for Health Evaluation Programme, and Patient Safety Research Portfolio. We would also like to thank the expert reviewers for their insightful and constructive feedback. Our thanks are also due to Dr. Allison Worth who commented on an earlier draft of this manuscript.
- Yin RK. Case study research, design and method. 4. London: Sage Publications Ltd.; 2009. [ Google Scholar ]
- Keen J, Packwood T. Qualitative research; case study evaluation. BMJ. 1995; 311 :444–446. [ PMC free article ] [ PubMed ] [ Google Scholar ]
- Sheikh A, Halani L, Bhopal R, Netuveli G, Partridge M, Car J. et al. Facilitating the Recruitment of Minority Ethnic People into Research: Qualitative Case Study of South Asians and Asthma. PLoS Med. 2009; 6 (10):1–11. [ PMC free article ] [ PubMed ] [ Google Scholar ]
- Pinnock H, Huby G, Powell A, Kielmann T, Price D, Williams S, The process of planning, development and implementation of a General Practitioner with a Special Interest service in Primary Care Organisations in England and Wales: a comparative prospective case study. Report for the National Co-ordinating Centre for NHS Service Delivery and Organisation R&D (NCCSDO) 2008. http://www.sdo.nihr.ac.uk/files/project/99-final-report.pdf
- Robertson A, Cresswell K, Takian A, Petrakaki D, Crowe S, Cornford T. et al. Prospective evaluation of the implementation and adoption of NHS Connecting for Health's national electronic health record in secondary care in England: interim findings. BMJ. 2010; 41 :c4564. [ PMC free article ] [ PubMed ] [ Google Scholar ]
- Pearson P, Steven A, Howe A, Sheikh A, Ashcroft D, Smith P. the Patient Safety Education Study Group. Learning about patient safety: organisational context and culture in the education of healthcare professionals. J Health Serv Res Policy. 2010; 15 :4–10. doi: 10.1258/jhsrp.2009.009052. [ PubMed ] [ CrossRef ] [ Google Scholar ]
- van Harten WH, Casparie TF, Fisscher OA. The evaluation of the introduction of a quality management system: a process-oriented case study in a large rehabilitation hospital. Health Policy. 2002; 60 (1):17–37. doi: 10.1016/S0168-8510(01)00187-7. [ PubMed ] [ CrossRef ] [ Google Scholar ]
- Stake RE. The art of case study research. London: Sage Publications Ltd.; 1995. [ Google Scholar ]
- Sheikh A, Smeeth L, Ashcroft R. Randomised controlled trials in primary care: scope and application. Br J Gen Pract. 2002; 52 (482):746–51. [ PMC free article ] [ PubMed ] [ Google Scholar ]
- King G, Keohane R, Verba S. Designing Social Inquiry. Princeton: Princeton University Press; 1996. [ Google Scholar ]
- Doolin B. Information technology as disciplinary technology: being critical in interpretative research on information systems. Journal of Information Technology. 1998; 13 :301–311. doi: 10.1057/jit.1998.8. [ CrossRef ] [ Google Scholar ]
- George AL, Bennett A. Case studies and theory development in the social sciences. Cambridge, MA: MIT Press; 2005. [ Google Scholar ]
- Eccles M. the Improved Clinical Effectiveness through Behavioural Research Group (ICEBeRG) Designing theoretically-informed implementation interventions. Implementation Science. 2006; 1 :1–8. doi: 10.1186/1748-5908-1-1. [ PMC free article ] [ PubMed ] [ CrossRef ] [ Google Scholar ]
- Netuveli G, Hurwitz B, Levy M, Fletcher M, Barnes G, Durham SR, Sheikh A. Ethnic variations in UK asthma frequency, morbidity, and health-service use: a systematic review and meta-analysis. Lancet. 2005; 365 (9456):312–7. [ PubMed ] [ Google Scholar ]
- Sheikh A, Panesar SS, Lasserson T, Netuveli G. Recruitment of ethnic minorities to asthma studies. Thorax. 2004; 59 (7):634. [ PMC free article ] [ PubMed ] [ Google Scholar ]
- Hellström I, Nolan M, Lundh U. 'We do things together': A case study of 'couplehood' in dementia. Dementia. 2005; 4 :7–22. doi: 10.1177/1471301205049188. [ CrossRef ] [ Google Scholar ]
- Som CV. Nothing seems to have changed, nothing seems to be changing and perhaps nothing will change in the NHS: doctors' response to clinical governance. International Journal of Public Sector Management. 2005; 18 :463–477. doi: 10.1108/09513550510608903. [ CrossRef ] [ Google Scholar ]
- Lincoln Y, Guba E. Naturalistic inquiry. Newbury Park: Sage Publications; 1985. [ Google Scholar ]
- Barbour RS. Checklists for improving rigour in qualitative research: a case of the tail wagging the dog? BMJ. 2001; 322 :1115–1117. doi: 10.1136/bmj.322.7294.1115. [ PMC free article ] [ PubMed ] [ CrossRef ] [ Google Scholar ]
- Mays N, Pope C. Qualitative research in health care: Assessing quality in qualitative research. BMJ. 2000; 320 :50–52. doi: 10.1136/bmj.320.7226.50. [ PMC free article ] [ PubMed ] [ CrossRef ] [ Google Scholar ]
- Mason J. Qualitative researching. London: Sage; 2002. [ Google Scholar ]
- Brazier A, Cooke K, Moravan V. Using Mixed Methods for Evaluating an Integrative Approach to Cancer Care: A Case Study. Integr Cancer Ther. 2008; 7 :5–17. doi: 10.1177/1534735407313395. [ PubMed ] [ CrossRef ] [ Google Scholar ]
- Miles MB, Huberman M. Qualitative data analysis: an expanded sourcebook. 2. CA: Sage Publications Inc.; 1994. [ Google Scholar ]
- Pope C, Ziebland S, Mays N. Analysing qualitative data. Qualitative research in health care. BMJ. 2000; 320 :114–116. doi: 10.1136/bmj.320.7227.114. [ PMC free article ] [ PubMed ] [ CrossRef ] [ Google Scholar ]
- Cresswell KM, Worth A, Sheikh A. Actor-Network Theory and its role in understanding the implementation of information technology developments in healthcare. BMC Med Inform Decis Mak. 2010; 10 (1):67. doi: 10.1186/1472-6947-10-67. [ PMC free article ] [ PubMed ] [ CrossRef ] [ Google Scholar ]
- Malterud K. Qualitative research: standards, challenges, and guidelines. Lancet. 2001; 358 :483–488. doi: 10.1016/S0140-6736(01)05627-6. [ PubMed ] [ CrossRef ] [ Google Scholar ]
- Yin R. Case study research: design and methods. 2. Thousand Oaks, CA: Sage Publishing; 1994. [ Google Scholar ]
- Yin R. Enhancing the quality of case studies in health services research. Health Serv Res. 1999; 34 :1209–1224. [ PMC free article ] [ PubMed ] [ Google Scholar ]
- Green J, Thorogood N. Qualitative methods for health research. 2. Los Angeles: Sage; 2009. [ Google Scholar ]
- Howcroft D, Trauth E. Handbook of Critical Information Systems Research, Theory and Application. Cheltenham, UK: Northampton, MA, USA: Edward Elgar; 2005. [ Google Scholar ]
- Blakie N. Approaches to Social Enquiry. Cambridge: Polity Press; 1993. [ Google Scholar ]
- Doolin B. Power and resistance in the implementation of a medical management information system. Info Systems J. 2004; 14 :343–362. doi: 10.1111/j.1365-2575.2004.00176.x. [ CrossRef ] [ Google Scholar ]
- Bloomfield BP, Best A. Management consultants: systems development, power and the translation of problems. Sociological Review. 1992; 40 :533–560. [ Google Scholar ]
- Shanks G, Parr A. Proceedings of the European Conference on Information Systems. Naples; 2003. Positivist, single case study research in information systems: A critical analysis. [ Google Scholar ]

An official website of the United States government
The .gov means it’s official. Federal government websites often end in .gov or .mil. Before sharing sensitive information, make sure you’re on a federal government site.
The site is secure. The https:// ensures that you are connecting to the official website and that any information you provide is encrypted and transmitted securely.
- Publications
- Account settings
- My Bibliography
- Collections
- Citation manager
Save citation to file
Email citation, add to collections.
- Create a new collection
- Add to an existing collection
Add to My Bibliography
Your saved search, create a file for external citation management software, your rss feed.
- Search in PubMed
- Search in NLM Catalog
- Add to Search
Case study: design? Method? Or comprehensive strategy?
Affiliation.
- 1 Faculty of Health & Applied Social Sciences, School of Nursing & Healthcare Studies, Liverpool John Moores University, Liverpool, UK.
- PMID: 15065485
- DOI: 10.7748/nr2004.04.11.3.70.c6206
As the case study approach gains popularity in nursing research, questions arise with regard to what it exactly is, and where it appears to fit paradigmatically. Is it a method, a design, are such distinctions important? Colin Jones and Christina Lyons review some of the key issues, with specific emphasis on the use of case study within an interpretevist philosophy.
PubMed Disclaimer
Similar articles
- Reliability and validity in research. Roberts P, Priest H. Roberts P, et al. Nurs Stand. 2006 Jul 12-18;20(44):41-5. doi: 10.7748/ns2006.07.20.44.41.c6560. Nurs Stand. 2006. PMID: 16872117 Review.
- Case study: a bridge across the paradigms. Luck L, Jackson D, Usher K. Luck L, et al. Nurs Inq. 2006 Jun;13(2):103-9. doi: 10.1111/j.1440-1800.2006.00309.x. Nurs Inq. 2006. PMID: 16700753 Review.
- [How to relate concepts and data]. Angot J, Milano P. Angot J, et al. Rech Soins Infirm. 2005 Mar;(80):12-27. Rech Soins Infirm. 2005. PMID: 15861914 Review. French.
- "Are we in Kansas yet, Toto?" The construction of chronic illness in research. Paterson BL. Paterson BL. Can J Nurs Res. 2000 Dec;32(3):11-7. Can J Nurs Res. 2000. PMID: 11928129 Review. No abstract available.
- [The mixed design in nursing sciences or when a question of research calls for qualitative and quantitative strategies]. Bourgault P, Gallagher F, Michaud C, Saint-Cyr-Tribble D. Bourgault P, et al. Rech Soins Infirm. 2010 Dec;(103):20-8. Rech Soins Infirm. 2010. PMID: 21322192 French.
- Participant Outcomes and Facilitator Experiences Following a Community Living Skills Program for Adult Mental Health Consumers. Sammells E, Logan A, Sheppard L. Sammells E, et al. Community Ment Health J. 2023 Apr;59(3):428-438. doi: 10.1007/s10597-022-01020-x. Epub 2022 Sep 8. Community Ment Health J. 2023. PMID: 36074286 Free PMC article.
- Young registered nurses' intention to leave the profession and professional turnover in early career: a qualitative case study. Flinkman M, Isopahkala-Bouret U, Salanterä S. Flinkman M, et al. ISRN Nurs. 2013 Aug 20;2013:916061. doi: 10.1155/2013/916061. eCollection 2013. ISRN Nurs. 2013. PMID: 24027640 Free PMC article.
Publication types
- Search in MeSH
LinkOut - more resources
Full text sources.
- Ovid Technologies, Inc.
Research Materials
- NCI CPTC Antibody Characterization Program
- Citation Manager
NCBI Literature Resources
MeSH PMC Bookshelf Disclaimer
The PubMed wordmark and PubMed logo are registered trademarks of the U.S. Department of Health and Human Services (HHS). Unauthorized use of these marks is strictly prohibited.
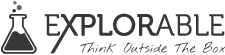
- Foundations
- Write Paper
Search form
- Experiments
- Anthropology
- Self-Esteem
- Social Anxiety

Case Study Research Design
The case study research design have evolved over the past few years as a useful tool for investigating trends and specific situations in many scientific disciplines.
This article is a part of the guide:
- Research Designs
- Quantitative and Qualitative Research
- Literature Review
- Quantitative Research Design
- Descriptive Research
Browse Full Outline
- 1 Research Designs
- 2.1 Pilot Study
- 2.2 Quantitative Research Design
- 2.3 Qualitative Research Design
- 2.4 Quantitative and Qualitative Research
- 3.1 Case Study
- 3.2 Naturalistic Observation
- 3.3 Survey Research Design
- 3.4 Observational Study
- 4.1 Case-Control Study
- 4.2 Cohort Study
- 4.3 Longitudinal Study
- 4.4 Cross Sectional Study
- 4.5 Correlational Study
- 5.1 Field Experiments
- 5.2 Quasi-Experimental Design
- 5.3 Identical Twins Study
- 6.1 Experimental Design
- 6.2 True Experimental Design
- 6.3 Double Blind Experiment
- 6.4 Factorial Design
- 7.1 Literature Review
- 7.2 Systematic Reviews
- 7.3 Meta Analysis
The case study has been especially used in social science, psychology, anthropology and ecology.
This method of study is especially useful for trying to test theoretical models by using them in real world situations. For example, if an anthropologist were to live amongst a remote tribe, whilst their observations might produce no quantitative data, they are still useful to science.
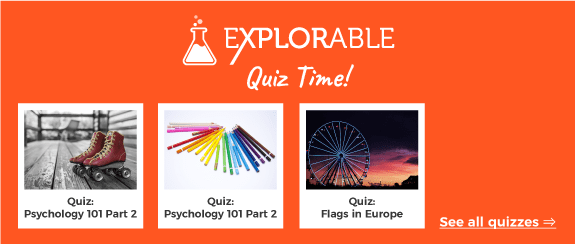
What is a Case Study?
Basically, a case study is an in depth study of a particular situation rather than a sweeping statistical survey . It is a method used to narrow down a very broad field of research into one easily researchable topic.
Whilst it will not answer a question completely, it will give some indications and allow further elaboration and hypothesis creation on a subject.
The case study research design is also useful for testing whether scientific theories and models actually work in the real world. You may come out with a great computer model for describing how the ecosystem of a rock pool works but it is only by trying it out on a real life pool that you can see if it is a realistic simulation.
For psychologists, anthropologists and social scientists they have been regarded as a valid method of research for many years. Scientists are sometimes guilty of becoming bogged down in the general picture and it is sometimes important to understand specific cases and ensure a more holistic approach to research .
H.M.: An example of a study using the case study research design.
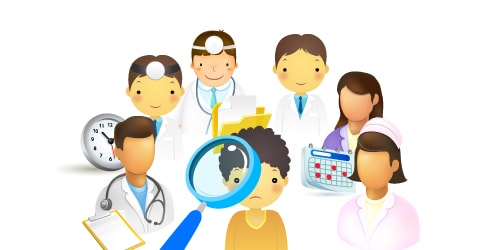
The Argument for and Against the Case Study Research Design
Some argue that because a case study is such a narrow field that its results cannot be extrapolated to fit an entire question and that they show only one narrow example. On the other hand, it is argued that a case study provides more realistic responses than a purely statistical survey.
The truth probably lies between the two and it is probably best to try and synergize the two approaches. It is valid to conduct case studies but they should be tied in with more general statistical processes.
For example, a statistical survey might show how much time people spend talking on mobile phones, but it is case studies of a narrow group that will determine why this is so.
The other main thing to remember during case studies is their flexibility. Whilst a pure scientist is trying to prove or disprove a hypothesis , a case study might introduce new and unexpected results during its course, and lead to research taking new directions.
The argument between case study and statistical method also appears to be one of scale. Whilst many 'physical' scientists avoid case studies, for psychology, anthropology and ecology they are an essential tool. It is important to ensure that you realize that a case study cannot be generalized to fit a whole population or ecosystem.
Finally, one peripheral point is that, when informing others of your results, case studies make more interesting topics than purely statistical surveys, something that has been realized by teachers and magazine editors for many years. The general public has little interest in pages of statistical calculations but some well placed case studies can have a strong impact.
How to Design and Conduct a Case Study
The advantage of the case study research design is that you can focus on specific and interesting cases. This may be an attempt to test a theory with a typical case or it can be a specific topic that is of interest. Research should be thorough and note taking should be meticulous and systematic.
The first foundation of the case study is the subject and relevance. In a case study, you are deliberately trying to isolate a small study group, one individual case or one particular population.
For example, statistical analysis may have shown that birthrates in African countries are increasing. A case study on one or two specific countries becomes a powerful and focused tool for determining the social and economic pressures driving this.
In the design of a case study, it is important to plan and design how you are going to address the study and make sure that all collected data is relevant. Unlike a scientific report, there is no strict set of rules so the most important part is making sure that the study is focused and concise; otherwise you will end up having to wade through a lot of irrelevant information.
It is best if you make yourself a short list of 4 or 5 bullet points that you are going to try and address during the study. If you make sure that all research refers back to these then you will not be far wrong.
With a case study, even more than a questionnaire or survey , it is important to be passive in your research. You are much more of an observer than an experimenter and you must remember that, even in a multi-subject case, each case must be treated individually and then cross case conclusions can be drawn .
How to Analyze the Results
Analyzing results for a case study tends to be more opinion based than statistical methods. The usual idea is to try and collate your data into a manageable form and construct a narrative around it.
Use examples in your narrative whilst keeping things concise and interesting. It is useful to show some numerical data but remember that you are only trying to judge trends and not analyze every last piece of data. Constantly refer back to your bullet points so that you do not lose focus.
It is always a good idea to assume that a person reading your research may not possess a lot of knowledge of the subject so try to write accordingly.
In addition, unlike a scientific study which deals with facts, a case study is based on opinion and is very much designed to provoke reasoned debate. There really is no right or wrong answer in a case study.
- Psychology 101
- Flags and Countries
- Capitals and Countries
Martyn Shuttleworth (Apr 1, 2008). Case Study Research Design. Retrieved Aug 21, 2024 from Explorable.com: https://explorable.com/case-study-research-design
You Are Allowed To Copy The Text
The text in this article is licensed under the Creative Commons-License Attribution 4.0 International (CC BY 4.0) .
This means you're free to copy, share and adapt any parts (or all) of the text in the article, as long as you give appropriate credit and provide a link/reference to this page.
That is it. You don't need our permission to copy the article; just include a link/reference back to this page. You can use it freely (with some kind of link), and we're also okay with people reprinting in publications like books, blogs, newsletters, course-material, papers, wikipedia and presentations (with clear attribution).
Want to stay up to date? Follow us!
Get all these articles in 1 guide.
Want the full version to study at home, take to school or just scribble on?
Whether you are an academic novice, or you simply want to brush up your skills, this book will take your academic writing skills to the next level.
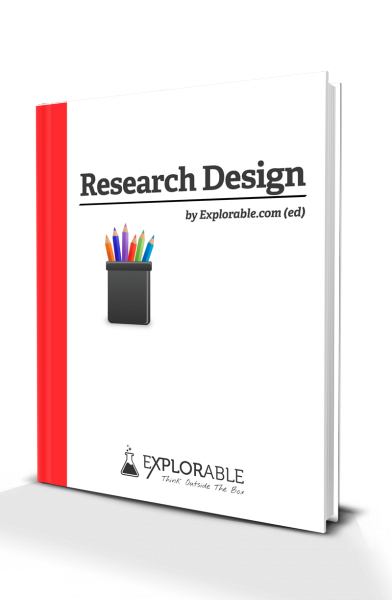
Download electronic versions: - Epub for mobiles and tablets - PDF version here
Save this course for later
Don't have time for it all now? No problem, save it as a course and come back to it later.
Footer bottom
- Privacy Policy
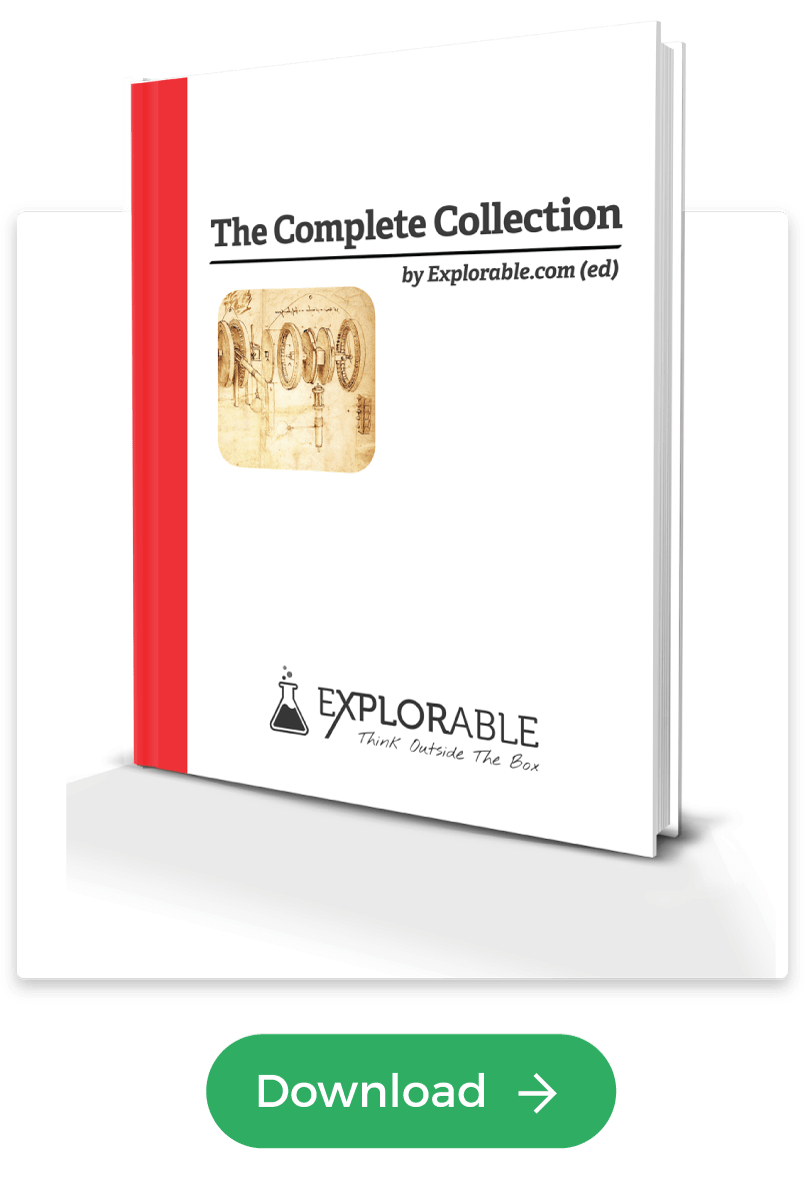
- Subscribe to our RSS Feed
- Like us on Facebook
- Follow us on Twitter

Case Study Research: Methods and Designs
Case study research is a type of qualitative research design. It’s often used in the social sciences because it involves…

Case study research is a type of qualitative research design. It’s often used in the social sciences because it involves observing subjects, or cases, in their natural setting, with minimal interference from the researcher.
In the case study method , researchers pose a specific question about an individual or group to test their theories or hypothesis. This can be done by gathering data from interviews with key informants.
Here’s what you need to know about case study research design .
What Is The Case Study Method?
Main approaches to data collection, case study research methods, how case studies are used, case study model.
Case study research is a great way to understand the nuances of a matter that can get lost in quantitative research methods. A case study is distinct from other qualitative studies in the following ways:
- It’s interested in the effect of a set of circumstances on an individual or group.
- It begins with a specific question about one or more cases.
- It focuses on individual accounts and experiences.
Here are the primary features of case study research:
- Case study research methods typically involve the researcher asking a few questions of one person or a small number of people—known as respondents—to test one hypothesis.
- Case study in research methodology may apply triangulation to collect data, in which the researcher uses several sources, including documents and field data. This is then analyzed and interpreted to form a hypothesis that can be tested through further research or validated by other researchers.
- The case study method requires clear concepts and theories to guide its methods. A well-defined research question is crucial when conducting a case study because the results of the study depend on it. The best approach to answering a research question is to challenge the existing theories, hypotheses or assumptions.
- Concepts are defined using objective language with no reference to preconceived notions that individuals might have about them. The researcher sets out to discover by asking specific questions on how people think or perceive things in their given situation.
They commonly use the case study method in business, management, psychology, sociology, political science and other related fields.
A fundamental requirement of qualitative research is recording observations that provide an understanding of reality. When it comes to the case study method, there are two major approaches that can be used to collect data: document review and fieldwork.
A case study in research methodology also includes literature review, the process by which the researcher collects all data available through historical documents. These might include books, newspapers, journals, videos, photographs and other written material. The researcher may also record information using video cameras to capture events as they occur. The researcher can also go through materials produced by people involved in the case study to gain an insight into their lives and experiences.
Field research involves participating in interviews and observations directly. Observation can be done during telephone interviews, events or public meetings, visits to homes or workplaces, or by shadowing someone for a period of time. The researcher can conduct one-on-one interviews with individuals or group interviews where several people are interviewed at once.
Let’s look now at case study methodology.
The case study method can be divided into three stages: formulation of objectives; collection of data; and analysis and interpretation. The researcher first makes a judgment about what should be studied based on their knowledge. Next, they gather data through observations and interviews. Here are some of the common case study research methods:
One of the most basic methods is the survey. Respondents are asked to complete a questionnaire with open-ended and predetermined questions. It usually takes place through face-to-face interviews, mailed questionnaires or telephone interviews. It can even be done by an online survey.
2. Semi-structured Interview
For case study research a more complex method is the semi-structured interview. This involves the researcher learning about the topic by listening to what others have to say. This usually occurs through one-on-one interviews with the sample. Semi-structured interviews allow for greater flexibility and can obtain information that structured questionnaires can’t.
3. Focus Group Interview
Another method is the focus group interview, where the researcher asks a few people to take part in an open-ended discussion on certain themes or topics. The typical group size is 5–15 people. This method allows researchers to delve deeper into people’s opinions, views and experiences.
4. Participant Observation
Participant observation is another method that involves the researcher gaining insight into an experience by joining in and taking part in normal events. The people involved don’t always know they’re being studied, but the researcher observes and records what happens through field notes.
Case study research design can use one or several of these methods depending on the context.
Case studies are widely used in the social sciences. To understand the impact of socio-economic forces, interpersonal dynamics and other human conditions, sometimes there’s no other way than to study one case at a time and look for patterns and data afterward.
It’s for the same reasons that case studies are used in business. Here are a few uses:
- Case studies can be used as tools to educate and give examples of situations and problems that might occur and how they were resolved. They can also be used for strategy development and implementation.
- Case studies can evaluate the success of a program or project. They can help teams improve their collaboration by identifying areas that need improvements, such as team dynamics, communication, roles and responsibilities and leadership styles.
- Case studies can explore how people’s experiences affect the working environment. Because the study involves observing and analyzing concrete details of life, they can inform theories on how an individual or group interacts with their environment.
- Case studies can evaluate the sustainability of businesses. They’re useful for social, environmental and economic impact studies because they look at all aspects of a business or organization. This gives researchers a holistic view of the dynamics within an organization.
- We can use case studies to identify problems in organizations or businesses. They can help spot problems that are invisible to customers, investors, managers and employees.
- Case studies are used in education to show students how real-world issues or events can be sorted out. This enables students to identify and deal with similar situations in their lives.
And that’s not all. Case studies are incredibly versatile, which is why they’re used so widely.
Human beings are complex and they interact with each other in their everyday life in various ways. The researcher observes a case and tries to find out how the patterns of behavior are created, including their causal relations. Case studies help understand one or more specific events that have been observed. Here are some common methods:
1. Illustrative case study
This is where the researcher observes a group of people doing something. Studying an event or phenomenon this way can show cause-and-effect relationships between various variables.
2. Cumulative case study
A cumulative case study is one that involves observing the same set of phenomena over a period. Cumulative case studies can be very helpful in understanding processes, which are things that happen over time. For example, if there are behavioral changes in people who move from one place to another, the researcher might want to know why these changes occurred.
3. Exploratory case study
An exploratory case study collects information that will answer a question. It can help researchers better understand social, economic, political or other social phenomena.
There are several other ways to categorize case studies. They may be chronological case studies, where a researcher observes events over time. In the comparative case study, the researcher compares one or more groups of people, places, or things to draw conclusions about them. In an intervention case study, the researcher intervenes to change the behavior of the subjects. The study method depends on the needs of the research team.
Deciding how to analyze the information at our disposal is an important part of effective management. An understanding of the case study model can help. With Harappa’s Thinking Critically course, managers and young professionals receive input and training on how to level up their analytic skills. Knowledge of frameworks, reading real-life examples and lived wisdom of faculty come together to create a dynamic and exciting course that helps teams leap to the next level.
Explore Harappa Diaries to learn more about topics such as Objectives Of Research , What are Qualitative Research Methods , How To Make A Problem Statement and How To Improve your Cognitive Skills to upgrade your knowledge and skills.
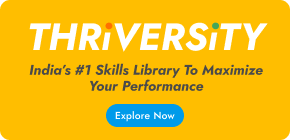
Information
- Author Services
Initiatives
You are accessing a machine-readable page. In order to be human-readable, please install an RSS reader.
All articles published by MDPI are made immediately available worldwide under an open access license. No special permission is required to reuse all or part of the article published by MDPI, including figures and tables. For articles published under an open access Creative Common CC BY license, any part of the article may be reused without permission provided that the original article is clearly cited. For more information, please refer to https://www.mdpi.com/openaccess .
Feature papers represent the most advanced research with significant potential for high impact in the field. A Feature Paper should be a substantial original Article that involves several techniques or approaches, provides an outlook for future research directions and describes possible research applications.
Feature papers are submitted upon individual invitation or recommendation by the scientific editors and must receive positive feedback from the reviewers.
Editor’s Choice articles are based on recommendations by the scientific editors of MDPI journals from around the world. Editors select a small number of articles recently published in the journal that they believe will be particularly interesting to readers, or important in the respective research area. The aim is to provide a snapshot of some of the most exciting work published in the various research areas of the journal.
Original Submission Date Received: .
- Active Journals
- Find a Journal
- Proceedings Series
- For Authors
- For Reviewers
- For Editors
- For Librarians
- For Publishers
- For Societies
- For Conference Organizers
- Open Access Policy
- Institutional Open Access Program
- Special Issues Guidelines
- Editorial Process
- Research and Publication Ethics
- Article Processing Charges
- Testimonials
- Preprints.org
- SciProfiles
- Encyclopedia
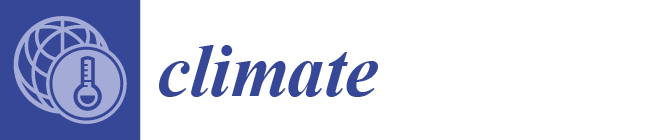
Article Menu
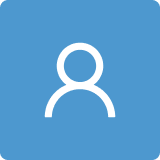
- Subscribe SciFeed
- Recommended Articles
- Author Biographies
- Google Scholar
- on Google Scholar
- Table of Contents
Find support for a specific problem in the support section of our website.
Please let us know what you think of our products and services.
Visit our dedicated information section to learn more about MDPI.
JSmol Viewer
Adaptation and coping strategies of women to reduce food insecurity in an era of climate change: a case of chireya district, zimbabwe, 1. introduction, framework for climate change, food security, and sustaining livelihoods, 2. methodology, 3.1. data presentation and analysis, 3.1.1. respondents’ gender distribution, 3.1.2. respondent ages, 3.1.3. respondents’ number of years in farming experience, 3.2. family size of the residents, 3.3. the effects of climate change on livelihoods, 3.4. coping and effectiveness of strategies to reduce food insecurity, 3.5. effectiveness of the coping strategies, 3.6. stakeholders assisting the communities to cope with the effects of food insecurity.
- Changing planting dates;
- Switching to early maturity drought-resistant crops;
- Crop rotation and diversification;
- Increasing the amount of land under cultivation;
- Digging the wells along the flood plains;
- Gardening (growing vegetables) to supplement diet;
- Water harvesting/dam construction;
- Income generating projects (IGPs) for both men and women.
3.7. Implications of the Findings
- The need for awareness among farmers regarding the harmful effects of environmental practices such as deforestation, veld fires, stream bank cultivation, and overuse of inorganic chemicals is emphasized. Government institutions responsible for environmental management should actively engage with rural communities to provide this awareness. Additionally, there is a call for the integration and implementation of a comprehensive framework for capacity building. This involves collaboration among government bodies such as the Ministry of Agriculture, Environmental Management Agency, and Local Government to educate farmers about the adverse impacts of environmental degradation.
- The Agriculture Research and Extension Services (Agritex) requires improvement to provide guidance on various drought-resistant crops, such as sorghum, and animals like donkeys. Additionally, there is a necessity to educate farmers about the significance of organic fertilizers and equip them with skills to adapt to fluctuations in precipitation and temperature. Furthermore, integrating indigenous knowledge systems is crucial for addressing climate challenges effectively.
- Government institutions, in collaboration with local communities, should implement improved water harvesting methods. These initiatives would assist communities in accessing domestic and drinking water for both humans and animals in the aftermath of rainfall. Additionally, this harvested water could be utilized for gardening purposes, thereby enhancing food supplies and dietary diversity.
- Early warning systems utilizing efficient information and communication technology are essential to swiftly distribute information to rural communities at the village level. Additionally, it is advisable to provide comprehensive training to farmers on managing various diseases.
- The Agriculture Research and Extension Services (Agritex) should promote the expansion and enhancement of non-farming income streams. This could involve implementing projects aimed at generating income, enhancing skills, and improving living standards for many individuals, enabling households to better withstand the impacts of climate change.
- To combat livestock illnesses, it is imperative to enhance production capabilities swiftly to mitigate losses and enhance productivity. Increasing veterinary extension services and providing training to existing personnel are highly recommended actions to decrease livestock mortality rates and enhance production levels.
- The Ministry of Women’s Affairs, along with its partners, must raise awareness about the significance of empowering girls, and work towards diminishing instances of gender-based violence.
Author Contributions
Data availability statement, conflicts of interest.
- IPCC. Climate Change 2014: Impacts, Adaptation, and Vulnerability. In Part A: Global and Sectoral Aspects. Contribution of Working Group II to the Fifth Assessment Report of the Intergovernmental Panel on Climate Change ; IPCC: Geneva, Switzerland, 2014; Chapter 24; p. 21. [ Google Scholar ]
- Mikhaylov, A.; Moiseev, N.; Aleshin, K.; Burkhardt, T. Global climate change and greenhouse effect. Entrep. Sustain. Issues 2020 , 7 , 2897. [ Google Scholar ] [ CrossRef ]
- Phiri, K.; Nhliziyo, M.; Madzivire, S.; Sithole, M.; Nyathi, D. Understanding climate smart agriculture and the resilience of smallholder farmers in Umguza district, Zimbabwe. Cogent Soc. Sci. 2021 , 7 , 1970425. [ Google Scholar ] [ CrossRef ]
- Chanza, N.; Musakwa, W. Ecological and hydrological indicators of climate change observed by dryland communities of Malipati in Chiredzi, Zimbabwe. Diversity 2022 , 14 , 541. [ Google Scholar ] [ CrossRef ]
- Rangarirai, W.; Marnani, C.; Rahmat, A. Analyzing the impact of community-based approaches on disaster preparedness to the risk of tropical cyclone induced flooding in Chimanimani district, Zimbabwe. IOP Conf. Ser. Earth Environ. Sci. 2023 , 1173 , 012070. [ Google Scholar ] [ CrossRef ]
- Govere, S.; Nyamangara, J.; Nyakatawa, E. Review: Climate change and the water footprint of wheat production in Zimbabwe. Water SA 2019 , 45 , 513–526. [ Google Scholar ] [ CrossRef ]
- Matsa, M. Climate change and lessons from world indigenous minority communities. In Climate Change and Agriculture in Zimbabwe ; Springer: Berlin/Heidelberg, Germany, 2021; pp. 11–20. [ Google Scholar ]
- Mushore, T.D.; Mhizha, T.; Manjowe, M.; Mashawi, L.; Matandirotya, E.; Mashonjowa, E.; Mutasa, C.; Gwenzi, J.; Mushambi, G.T. Climate change adaptation and mitigation strategies for small holder farmers: A case of Nyang district in Zimbabwe. Front. Clim. 2021 , 3 , 676495. [ Google Scholar ] [ CrossRef ]
- Waha, K.; Müller, C.; Bondeau, A.; Dietrich, J.P.; Kurukulasuriya, P.; Heinke, J.; Lotze-Campen, H. Adaptation to climate change through the choice of cropping system and sowing date in sub-Saharan Africa. Glob. Environ. Chang. 2012 , 23 , 130–143. [ Google Scholar ] [ CrossRef ]
- Sujakhu, N.; Ranjitkar, S.; He, J.; Schmidt-Vogt, D.; Su, Y.; Xu, J. Assessing the livelihood vulnerability of rural indigenous households to climate changes in central Nepal, Himalaya. Sustainability 2019 , 11 , 2977. [ Google Scholar ] [ CrossRef ]
- Perkins, A.K. “The poor feel it the most”: The Antilles bishops, the poor, and climate change. Am. J. Econ. Sociol. 2021 , 80 , 1155–1172. [ Google Scholar ] [ CrossRef ]
- Dewi, K.H.; Sari, D.P.; Latifa, A. Sustainability of climate change issues in Indonesia: The voices of women’s NGOs. IOP Conf. Ser. Earth Environ. Sci. 2022 , 1105 , 012005. [ Google Scholar ] [ CrossRef ]
- Hlahla, S. Gender perspectives of the water, energy, land, and food security nexus in sub-Saharan Africa. Front. Sustain. Food Syst. 2022 , 6 , 719913. [ Google Scholar ] [ CrossRef ]
- D’Exelle, B.; Riedl, A. Gender inequality and resource sharing: Evidence from rural Nicaragua. J. Dev. Perspect. 2018 , 2 , 62–87. [ Google Scholar ] [ CrossRef ]
- Tigabie, A.; Teferra, B.; Abe, A. Access and control of resources by rural women in north Shewa zone, Amhara region, Ethiopia. Res. World Agric. Econ. 2022 , 3 , 36–47. [ Google Scholar ] [ CrossRef ]
- Amporfu, E.; Grépin, K. Measuring and explaining changing patterns of inequality in institutional deliveries between urban and rural women in Ghana: A decomposition analysis. Int. J. Equity Health 2019 , 18 , 123. [ Google Scholar ] [ CrossRef ] [ PubMed ]
- Otu, J.; Anam, B. The role of women in food production and poverty reduction in rural communities in cross river state. Adv. Soc. Sci. Res. J. 2016 , 3 . [ Google Scholar ] [ CrossRef ]
- Rahman, M.M.; Dana, L.; Moral, I.H.; Anjum, N.; Rahaman, M.S. Challenges of rural women entrepreneurs in Bangladesh to survive their family entrepreneurship: A narrative inquiry through storytelling. J. Fam. Bus. Manag. 2022 , 13 , 645–664. [ Google Scholar ] [ CrossRef ]
- Tiwari, R.; Gupta, A.; Agrawal, A.; Gandhi, A.; Gupta, M.; Das, M. Women and tobacco use: Discrepancy in the knowledge, belief and behavior towards tobacco consumption among urban and rural women in Chhattisgarh, central India. Asian Pac. J. Cancer Prev. 2015 , 16 , 6365–6373. [ Google Scholar ] [ CrossRef ] [ PubMed ]
- Shukla, R.; Kanaan, M.; Siddiqi, K. Tobacco use among 1,310,716 women of reproductive age (15–49 years) in 42 low- and middle-income countries: Secondary data analysis from the 2010–2016 demographic and health surveys. SSRN Electron. J. 2021 , 23 , 2019–2027. [ Google Scholar ] [ CrossRef ]
- Füssel, H.M.; Kein, R. Climate change vulnerability assessments: An evolution of conceptual thinking. Clim. Chang. 2006 , 75 , 301–329. [ Google Scholar ] [ CrossRef ]
- Füssel, H. Vulnerability: A generally applicable conceptual framework for climate change research. Glob. Environ. Chang. 2007 , 17 , 155–167. [ Google Scholar ] [ CrossRef ]
- Kongolo, M.; Dlamini, D.K. Small-scale livestock farming in developing areas of Swaziland and South Africa. AFRREV STECH An Int. J. Sci. Technol. Bahir Dar Ethiop. 2012 , 1 , 100–111. [ Google Scholar ]
- Zenda, M.; Malan, P.J. The sustainability of small-scale sheep farming systems in the Northern Cape (Hantam Karoo), South Africa. S. Afr. J. Agric. Extension 2021 , 49 , 105–121. [ Google Scholar ] [ CrossRef ]
- Parrish, R.; Colbourn, T.; Lauriola, P.; Leonardi, G.; Hajat, S.; Zeka, A. A critical analysis of the drivers of human migration patterns in the presence of climate change: A new conceptual model. Int. J. Environ. Res. Public Health 2020 , 17 , 6036. [ Google Scholar ] [ CrossRef ] [ PubMed ]
- Li, Z. A review of research on the dilemma of motherhood in contemporary China. In 2022 6th International Seminar on Education, Management and Social Sciences ; Atlantis Press: Amsterdam, The Netherlands, 2022; pp. 1503–1509. [ Google Scholar ] [ CrossRef ]
- Paniw, M.; James, T.D.; Ruth Archer, C.; Römer, G.; Levin, S.; Compagnoni, A.; Che-Castaldo, J.; Bennett, J.M.; Mooney, A.; Childs, D.Z.; et al. The myriad of complex demographic responses of terrestrial mammals to climate change and gaps of knowledge: A global analysis. J. Anim. Ecol. 2021 , 90 , 1398–1407. [ Google Scholar ] [ CrossRef ] [ PubMed ]
- Hertel, T.W.; Rosch, S. Climate change, agriculture, and poverty. Appl. Econ. Perspect. Policy 2010 , 32 , 355–385. [ Google Scholar ] [ CrossRef ]
- Baker, R.; Anttila-Hughes, J. Characterizing the contribution of high temperatures to child undernourishment in sub-Saharan Africa. Sci. Rep. 2020 , 10 , 18796. [ Google Scholar ] [ CrossRef ] [ PubMed ]
- Abid, M.; Scheffran, J.; Schneider, U.A.; Ashfaq, M. Farmers’ perceptions of and adaptation strategies to climate change and their determinants: The case of Punjab province, Pakistan. Earth Syst. Dyn. 2015 , 6 , 225–243. [ Google Scholar ] [ CrossRef ]
- Wu, J. Impact of relocation in response to climate change on farmers’ livelihood capital in minority areas: A case study of Yunnan province. Int. J. Clim. Change Strateg. Manag. 2023 , 15 , 790–809. [ Google Scholar ] [ CrossRef ]
- Hlophe-Ginindza, S.; Mpandeli, N.S. The role of small-scale farmers in ensuring food security in Africa. In Food Security in Africa ; IntechOpen: London, UK, 2021. [ Google Scholar ] [ CrossRef ]
- Challinor, A.J.; Wheeler, T.; Garforth, C.; Craufurd, P.; Kassam, A. Assessing the vulnerability of food crop systems in Africa to climate change. Clim. Chang. 2007 , 83 , 381–399. [ Google Scholar ] [ CrossRef ]
- Ribeiro, P.F.; Rodriguez, A.V.C. Emerging advanced technologies to mitigate the impact of climate change in Africa. Plants 2020 , 9 , 381. [ Google Scholar ] [ CrossRef ]
- Pickson, R.B.; Boateng, E. Climate change: A friend or foe to food security in Africa? Environ. Dev. Sustain. 2021 , 24 , 4387–4412. [ Google Scholar ] [ CrossRef ] [ PubMed ]
- Adepoju, A.O.; Obialo, A. Agricultural labour productivity growth and food insecurity transitions among maize farming households in rural Nigeria. Ekon. Poljopr. 2022 , 69 , 1093–1107. [ Google Scholar ] [ CrossRef ]
- Bashir, M.K.; Schilizzi, S. The nexus between food security and livelihoods: A study on the determinants of food security in rural areas of Pakistan. Food Secur. 2013 , 5 , 327–337. [ Google Scholar ]
- Schnitter, R.; Berry, P. The climate change, food security and human health nexus in Canada: A framework to protect population health. Int. J. Environ. Res. Public Health 2019 , 16 , 2531. [ Google Scholar ] [ CrossRef ] [ PubMed ]
- Scoones, I. Sustainable Rural Livelihoods: A Framework for Analysis ; IDS Working Paper 72; Institute of Development Studies: Brighton, UK, 1998. [ Google Scholar ]
- Carney, D.; Drinkwater, M.; Rusinow, T.; Neefjes, K.; Wanmali, S.; Singh, N. Livelihoods Approaches Compared ; Department for International Development: London, UK, 1999.
- Murniati, K.; Mutolib, A. The impact of climate change on the household food security of upland rice farmers in Sidomulyo, Lampung province, Indonesia. Biodiversitas J. Biol. Divers. 2020 , 21 . [ Google Scholar ] [ CrossRef ]
- Toimil, A.; Camus, P.; Losada, Í.J.; Cozannet, G.L.; Nicholls, R.J.; Idier, D.; Maspataud, A. Climate change-driven coastal erosion modelling in temperate sandy beaches: Methods and uncertainty treatment. Earth-Sci. Rev. 2020 , 202 , 103110. [ Google Scholar ] [ CrossRef ]
- Houessou, M.D.; Cassee, A.; Sonneveld, B. The effects of the COVID-19 pandemic on food security in rural and urban settlements in benin: Do allotment gardens soften the blow? Sustainability 2021 , 13 , 7313. [ Google Scholar ] [ CrossRef ]
- Okesanya, O.J.; Alnaeem, K.F.H.; Hassan, H.K.; Oso, A.T.; Adigun, O.A.; Bouaddi, O.; Olaleke, N.O.; Kheir, S.G.M.; Haruna, U.A.; Shomuyiwa, D.O.; et al. The intersectional impact of climate change and gender inequalities in Africa. Public Health Chall. 2024 , 3 , e169. [ Google Scholar ] [ CrossRef ]
- Andersen, L.V.; Davis, M.D. Climate change and the epidemiology of selected tick-borne and mosquito-borne diseases: Update from the international society of dermatology climate change task force. Int. J. Dermatol. 2016 , 56 , 252–259. [ Google Scholar ]
- Suranny, L.E.; Gravitiani, E.; Rahardjo, M. Impact of climate change on the agriculture sector and its adaptation strategies. IOP Conf. Ser. Earth Environ. Sci. 2022 , 1016 , 012038. [ Google Scholar ] [ CrossRef ]
- Hamidazada, M.; Cruz, A.M.; Yokomatsu, M. Vulnerability factors of Afghan rural women to disasters. Int. J. Disaster Risk Sci. 2019 , 10 , 573–590. [ Google Scholar ] [ CrossRef ]
- Memon, F. Climate change and violence against women: Study of a flood-affected population in the rural area of sindh, pakistan. Pak. J. Women Stud. Alam-E-Niswan 2020 , 27 , 65–85. [ Google Scholar ] [ CrossRef ]
- Kretz, E.; Itaru, A.; Glas, M.; Waswa, L.; Jordan, I. Is responsive feeding difficult? a case study in teso south sub-county, kenya. Nutrients 2022 , 14 , 4677. [ Google Scholar ] [ CrossRef ] [ PubMed ]
- Harris-Fry, H.; Nur, H.; Shankar, B.; Zanello, G.; Srinivasan, C.; Kadiyala, S. The impact of gender equity in agriculture on nutritional status, diets, and household food security: A mixed-methods systematic review. BMJ Global Health 2020 , 5 , e002173. [ Google Scholar ] [ CrossRef ] [ PubMed ]
- Bridge, J. Barriers to accessing medical services: A review of economic and cultural factors. J. Health Econ. 2008 , 15 , 123–135. [ Google Scholar ]
- Anderson, W.; Seager, R.; Baethgen, W.E.; Cane, M.A.; You, L. Synchronous crop failures and climate-forced production variability. Sci. Adv. 2019 , 5 , eaaw1976. [ Google Scholar ] [ CrossRef ] [ PubMed ]
- Kulkarni, M.A.; Duguay, C.; Ost, K. Charting the evidence for climate change impacts on the global spread of malaria and dengue and adaptive responses: A scoping review of reviews. Glob. Health 2022 , 18 , 1–8. [ Google Scholar ] [ CrossRef ] [ PubMed ]
- True, J. Gendered violence in natural disasters: Learning from New Orleans, Haiti and Christchurch. Aotearoa N. Z. Soc. Work 2016 , 25 , 78–89. [ Google Scholar ] [ CrossRef ]
- Leslie, E.; Wilson, R. Sheltering in place and domestic violence: Evidence from calls for service during COVID-19. J. Public Econ. 2020 , 189 , 104241. [ Google Scholar ] [ CrossRef ]
- Below, T.B.; Mutabazi, K.D.; Kirschke, D.; Franke, C.; Sieber, S.; Siebert, R.; Tscherning, K. Can farmers’ adaptation to climate change be explained by socio-economic household-level variables? Glob. Environ. Chang. 2012 , 22 , 223–235. [ Google Scholar ] [ CrossRef ]
- Jacoby, H.; Skoufias, E. Testing Theories of Consumption Behaviour Using Information on Aggregate Shocks: Income Seasonality and Rainfall in Rural India. Am. J. Agric. Econ. 1997 , 80 , 1–14. [ Google Scholar ] [ CrossRef ]
- Kochar, A. Explaining household vulnerability to idiosyncratic income shocks. Am. Econ. Rev. 1999 , 89 , 159–164. [ Google Scholar ]
- Morduch, J. Income smoothing and consumption smoothing. J. Econ. Perspect. 1995 , 9 , 103–114. [ Google Scholar ] [ CrossRef ]
- Skoufias, E.; Rabassa, M.; Olivieri, S.; Brahmbhatt, M. The poverty impacts of climate Change. PREM Policy Brief 2011 , 51 , 1–35. [ Google Scholar ]
Click here to enlarge figure
Gender Distribution | Percentage |
---|---|
Women | 62 |
Men | 33 |
Village heads | 5 |
Ages | |
50–60 | 58 |
61–70 | 40 |
75+ | 2 |
Education level | |
Never went to school | 3 |
Finished primary education | 58 |
Secondary education | 36 |
Tertiary education | 3 |
Farming experience in years | |
0–4 | 9 |
5–9 | 12 |
10–20 | 22 |
21 and above | 57 |
Coping Strategy | Percentage of Women Using the Coping Strategy | Effectiveness |
---|---|---|
Selling items such as plows, harrows, and cultivators. | 100% | 60% |
Altering the time of planting. | 90% | 80% |
Selling domestic animals like cattle and goats, as well as domestic birds like chickens. | 90% | 65% |
Borrowing from kins, friends, and from the chief. | 90% | 60% |
Transitioning to drought-tolerant varieties of crops such as sorghum. | 88% | 75% |
Informal employment. | 80% | 50% |
Decreasing the frequency of daily meals. | 80% | 50% |
Bartering pieces of land for grains. | 80% | 50% |
Unlawful trade of firewood. | 72% | 35% |
Buying on credit and paying after harvests or selling an asset. | 70% | 50% |
Exchanging livestock and assets in return for crops. | 70% | 50% |
Illegal mining activities. | 70% | 45% |
Adding wild fruits to their diet as a supplement. | 70% | 30% |
Depending on funding bodies or contributors. | 67% | 55% |
Excavating deep wells alongside the flood plains. | 60% | 55% |
Reducing the quantity and quality of meals. | 60% | 40% |
Vending untamed fruits in rural hubs and urban areas. | 50% | 40% |
Food for work and money for work programs. | 36% | 42% |
Temporarily relocating children to more prosperous relatives, such as in-laws. | 26% | 20% |
Consuming grains left aside as seeds. | 26% | 20% |
Help from friends. | 20% | 20% |
Arranging marriages for their daughters. | 12% | 10% |
Stakeholder | Duties |
---|---|
Local Government | |
Agritex | |
Grain Marketing Board | |
Women affairs | |
NGOs | Food and money distribution during the period in food insecurity situations. |
Social welfare | |
Environmental Management | Protect the environment from being exploited. They reported that it helped to mitigate veld fires; land degradation; siltation; deforestation. |
The statements, opinions and data contained in all publications are solely those of the individual author(s) and contributor(s) and not of MDPI and/or the editor(s). MDPI and/or the editor(s) disclaim responsibility for any injury to people or property resulting from any ideas, methods, instructions or products referred to in the content. |
Share and Cite
Magwegwe, E.; Zivengwa, T.; Zenda, M. Adaptation and Coping Strategies of Women to Reduce Food Insecurity in an Era of Climate Change: A Case of Chireya District, Zimbabwe. Climate 2024 , 12 , 126. https://doi.org/10.3390/cli12080126
Magwegwe E, Zivengwa T, Zenda M. Adaptation and Coping Strategies of Women to Reduce Food Insecurity in an Era of Climate Change: A Case of Chireya District, Zimbabwe. Climate . 2024; 12(8):126. https://doi.org/10.3390/cli12080126
Magwegwe, Everjoy, Taruberekerwa Zivengwa, and Mashford Zenda. 2024. "Adaptation and Coping Strategies of Women to Reduce Food Insecurity in an Era of Climate Change: A Case of Chireya District, Zimbabwe" Climate 12, no. 8: 126. https://doi.org/10.3390/cli12080126
Article Metrics
Further information, mdpi initiatives, follow mdpi.
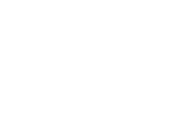
Subscribe to receive issue release notifications and newsletters from MDPI journals
Open Access is an initiative that aims to make scientific research freely available to all. To date our community has made over 100 million downloads. It’s based on principles of collaboration, unobstructed discovery, and, most importantly, scientific progression. As PhD students, we found it difficult to access the research we needed, so we decided to create a new Open Access publisher that levels the playing field for scientists across the world. How? By making research easy to access, and puts the academic needs of the researchers before the business interests of publishers.
We are a community of more than 103,000 authors and editors from 3,291 institutions spanning 160 countries, including Nobel Prize winners and some of the world’s most-cited researchers. Publishing on IntechOpen allows authors to earn citations and find new collaborators, meaning more people see your work not only from your own field of study, but from other related fields too.
Brief introduction to this section that descibes Open Access especially from an IntechOpen perspective
Want to get in touch? Contact our London head office or media team here
Our team is growing all the time, so we’re always on the lookout for smart people who want to help us reshape the world of scientific publishing.
Home > Books > Exploring the World of Drilling
Completion Strategy: A Case Study of Using Autonomous Inflow Control Technology to Manage Water
Submitted: 21 March 2024 Reviewed: 11 April 2024 Published: 04 June 2024
DOI: 10.5772/intechopen.114988
Cite this chapter
There are two ways to cite this chapter:
From the Edited Volume
Exploring the World of Drilling
Edited by Sonny Irawan
To purchase hard copies of this book, please contact the representative in India: CBS Publishers & Distributors Pvt. Ltd. www.cbspd.com | [email protected]
Chapter metrics overview
23 Chapter Downloads
Impact of this chapter
Total Chapter Downloads on intechopen.com
The issue of high-water cut will have repercussions for every oil operator on the planet. The presence of scales, corrosion, and deterioration, along with the expense of separation, are all exacerbated by an overabundance of water production, driving up the price of lifting fluids. To understand the challenges faced by the many wells there, data from one of the offshore oil fields had to be analyzed. The autonomous inflow control valves (AICVs) method is used for water control in different reservoirs. Swell packers are used in the AICV method to permanently seal off the well. Additionally, the completion strategy for handling the high-water cut has been outlined. It also discusses the availability of many inflow control systems for well completion to stop undesired water production, as well as the efficacy of various water shut-off techniques. The study offers a complete approach for water control in horizontal wells for the future rehabilitation plan of the Offshore Field by analyzing the effectiveness of these technologies via a variety of case studies and literature research. After analyzing the reservoir and fluid properties of the western offshore field, a completion strategy was suggested for the future redevelopment plan.
- horizontal wells
- autonomous inflow control system
- water control
- heterogeneous formation
- offshore field
Author Information
Vinayak s. wadgaonkar *.
- School of Petroleum Engineering, Dr. Vishwanath Karad MIT World Peace University, Pune, India
Siraj Bhatkar
Dinesh bhutada.
- School of Chemical Engineering, Dr. Vishwanath Karad MIT World Peace University, Pune, India
Pratiksha Khurpade
Himangi neve, pallavi tatpate.
*Address all correspondence to: [email protected]
1. Introduction
To prolong the period the wellbore is in direct contact with the reservoir, horizontal wells are increasingly being drilled. Horizontal wells are a good alternative to vertical wells when they are not available or when a corporation wants to reduce the number of vertical wells [ 1 ]. These are occasionally drilled to increase the area of contact with the pay zones or to access targets buried beneath nearby strata. Due to the angle at which these horizontal wells are dug, a variety of problems that could lower production rates are more likely to affect them. An existing heterogeneous well’s level of heterogeneity is influenced by fractures, solution channels, near-water zones, high permeability zones, fractures connecting water aquifers, heterogeneous oil-water contact, and heterogeneous permeability [ 2 ]. Poor oil output, drastically declining rates of oil production, and a shorter economic production life for the well are the results of this non-uniform flow sweep in the wellbore [ 3 , 4 , 5 ].
The issue of high-water cut will have repercussions for every oil operator on the planet. This occurs in both older and more recently constructed wells [ 6 ]. Many factories feel the effects of this in their bottom lines. The presence of scales, corrosion, and deterioration, along with the expense of separation, are all exacerbated by an overabundance of water production, driving up the price of lifting fluids. Production well efficiency and lifespan are both negatively impacted. Therefore, it is vital to resolve water shortage concerns. Therefore, knowing the formation and field inside and out during wellbore design [ 7 , 8 , 9 , 10 , 11 ] can help to cut down on water output that is not needed.
There is hardly one cookie-cutter answer to any problem. It is necessary to comprehend the nature of the issue to correctly address a given water scenario. There are several approaches to handle high-water cuttings [ 12 ]. The mechanical and chemical processes are two examples. During mechanical water shut-off procedures, expandable and non-expandable packers are used to isolate the wellbore’s water-producing parts. Only a few examples of chemical techniques include in situ gels, polymer and swelling agents, water swelling polymers, Micro Matrix Cement, and HWSO plugging agents. These are applied in different ways depending on the specifics of the well’s water level-lowering problem. There are a lot of potential answers to the issue of wasteful water production, but it is difficult to put these solutions into practice and successfully reduce water consumption [ 4 , 13 , 14 , 15 , 16 , 17 , 18 , 19 ].
Engineers investigated the problem of water cuts at a functioning oil field in the Indian Western Offshore Basin (WOB) in this study. In 1985, it was determined that the area was a field. In addition to three gas caps, the field has a reasonably reliable bottom aquifer. The field has a reserve of 42.54 MMT and an estimated OIIP of 112.48 MMT. Water injections started in July 1994, and initial production started in March 1990. With an average water cut of 90%, the field is now injecting water at a rate of 85,000 BWPD and producing oil at a rate of 14,400 BPD. The field had produced 35.54 MMT (31.6% OIIP) as of March 2019. Both newly drilled and side-tracked wells as well as older wells have experienced a sharp increase in the ratio of water to oil production (W/C).
2. Geological setup
The field is crescent-shaped, with two summits separated by a mild saddle and a shared OWC at 1477 m. Tectonic activities linked with fault reactivation have most likely resulted in significant fracture of carbonate rocks. The crack has likely caused karstification by acting as a conduit/pathway for the movement of meteoric water. Core investigations have shown three types of diagnostic traits in reservoir development.
Zone of Intense Karstification: This is the highest zone of Bassein pay. The formation of vugs, solution channels, and collapse breccia on this face indicate significant Karstification in the meteoric zone.
Tight Zone: Sparitization is abundant in the tight zone under the severely karstified zone. There are several types of fractures. Fractures, vugs, and faunal chambers from earlier generations are mainly sparitized, contributing to a reduction in porosity.
Changed Limestone: This zone is very permeable and mostly composed of altered chalky limestone. Because of the breakdown of lime mud, it forms microporosity.
3. Problems with offshore field’s water cut and well treatments applied
The field began production in March 1990 through four wells of the P-1 platform beginning in 1993–1994. In 1993–1994, the field had a peak oil output of around 95,000 BOPD. In July 1994, as shown in Figure 1 , water injection began from the W-1 and W-2 platforms in the field’s southernmost section. Despite beginning in April 1994, water reduction increased steadily until it reached 66% in 1999. The rise in water cut was not consistent over the field because of the field’s highly varied aerial and vertical structure. Oil is produced from the naturally fractured carbonate reservoir found in the field known as Bassein pay. There are karstified zones, solution channels, cracks, and vugs in this reservoir. This issue has always been a source of mud leaks and water injection failures. From 1994 to 1998, the water cut in the field increased by a factor of 40, and from 1998 to 2002, it increased by another 60–70. Numerous factors, including the presence of fractures, wormholes, channeling from injectors and aquifer, completion in the water zone, high permeability variation, water coning, and so on, have contributed to the field’s current extremely high water output. From no water reduction in 1994 to a 70% reduction in 2002, the field is now facing a situation where more than 90% of the water used in production must be conserved. There are a total of 92 oil-producing wells in this western offshore area, 71 of which use horizontal wells with slotted tubing and 21 of which are vertical or inclined. These oil-producing wells, which include horizontal wells, have had over 90% of the water removed and are back to normal operation. Horizontal wells may use slotted or perforated tubing as a final touch, making it difficult to trace the water’s origin [ 20 ].

Production-injection performance of the Western offshore field.
The water cut worries are greater in the northern half of the field, where the thickness is only 15–20 m than in the southern part of the field, where the thickness is 50–100 m (70 m). Due to thickness differences and inherent unpredictability, some areas recovered more quickly than others. The average productivity of the northern sector is 0.036 MMt/string, whereas the combined productivity of the northern and southern sectors is 0.185 MMt/string. The field’s production and injection performance through time is depicted in the following graphs.
The asset routinely implemented corrective measures, such as chemical water shut-off works, to curb the output of water from horizontal wells that was deemed excessive. These procedures were conducted in conjunction with a number of institutions and other service providers in an effort to control the excessive output of water from horizontal wells. Chemical water shut-off activities were carried out in the field based on the Western Offshore Field’s geology and reservoir characteristics. The selection of appropriate water shut-off tasks was guided by geological factors, reservoir characteristics, fluid properties, and challenges encountered during water cuts. These decisions were substantiated through in-lab experiments and literature reviews. Because of the detrimental effects the excessive water production had, these procedures were shifted to horizontal wells with high-water cut concerns [ 21 ].
Polymer gel technology, more precisely “PHPA Polymer with Metallic Cross Linker” and “PHPA Polymer with Organic Cross Linker,” was employed for chemical water shut-off operations in the western offshore region. Water shut-off jobs utilizing this polymer gel technology in a large number of horizontal wells had promising early results, but the wells continued to produce a high-water cut after a few months due to an increase in field water cut and movement of water due to water coning and water breakthrough. The job done by chemical water shut-off in high-water cut wells is illustrated by the bar graphs below ( Figures 2 – 5 ). It shows the production status of the well before and after the water was turned off.

Performance of the wells pre-and post-WSO jobs.

Some success was had with using polymer gel water shut-off to address water cut difficulties in horizontal wells, however, these results were inconsistent. Eight of the 18 wells given the water shut-off job saw significant improvements in oil rates and water cut, three saw moderate gains, two saw none, and five saw no change in productivity. Wells that had no improvement in water cut difficulties after implementing polymer gel jobs were more likely to experience a high number of water cuts much sooner than other wells [ 22 ]. After a polymer gel was applied, the number of productive wells stayed rather constant (3–6 months). However, these readings did not last long, as water cuts increased at the wells in tandem with a drop in oil rates as a result of water coning and water breakthrough. The water cut in the field rose again after a certain length of time had passed, and this rise was in concert with an increase in water cut in these wells as a result of WSO jobs. Water cut cannot be controlled throughout a well’s life even if a successful water shut-off scheme is implemented at the beginning of the well’s life.
4. Completion strategy for different reservoir properties
A thorough understanding of reservoir parameters during drilling and logging can aid in the development of an effective water control completion strategy. Taking these reservoir qualities into account and applying the appropriate technique for those properties might assist delay and control water production. The following are some examples of completion strategies for various reservoir parameters.
Unconsolidated sandstone reservoir
A long wellbore with near water aquifer support
Presence of low-viscosity hydrocarbon in the formation
A long and thin pay zone thickness with low permeability variation
Reliability
Limited hydrocarbon reserves and low flow rate

Completion strategy for unconsolidated sandstone formation using ICDs.
According to the aforementioned reservoir features, adopting an inflow control device (ICD) will be the most appropriate strategy for effectively producing hydrocarbons while preventing the creation of undesirable water. To prevent wellbore collapse in an unconsolidated sandstone formation, the production formation must be cased, cemented, and perforated along the length of the horizontal wellbore. To finish a well with an ICD, the well must be segregated into sections with packers calculated by reservoir modeling and simulation software for the specific reservoir parameters [ 14 , 23 , 24 ].
The ability of the inflow regulate device to filter sand using screens before flowing into the device can effectively control the probability of increasing sand production from the unconsolidated sandstone deposit. Wells are less likely to experience early water breakthrough due to excessive water coning if fluid inflow is uniform from all directions, as is the case when using an ICD [ 25 ]. Thus, the ICD will ensure uniform inflow across the entire wellbore and suppress the production of water in areas close to the oil-water interface ( Figure 6 ). ICDs will successfully create light oil by providing lower pressure drops for light oil zones and bigger pressure drops for heavy oil zones since they are ideally adapted to low-viscosity fluids. ICD would help overcome the well cost and give effective good production, making the well economical by equalizing the fluid flow along the wellbore length in the low permeability variation formation. The great reliability of channel-type ICDs in overcoming clogging and erosion could aid in the prevention.
of system failure [ 26 ]. The ICD is more capable of producing low flowrate fluid formations and including artificial lift in the form of gas lift to produce remaining reserves for maximum oil recovery in a well. As a result, based on the limited reserves in the well, ICDs will efficiently generate the most hydrocarbons, making the well profitable [ 27 , 28 , 29 , 30 ].
High permeability variation wellbore
A wellbore with a thin pay zone, high water aquifer support, and gas cap
Presence of low-viscosity hydrocarbons in the formation
Heterogeneous oil-water contact and near water zones
Presence of some fractures connecting bottom water aquifer

Completion strategy for consolidated sandstone formation using AICDs.
Depending on the aforementioned reservoir parameters, incorporating an AICD into the completion strategy will be the most efficient means of producing hydrocarbons. The above-mentioned cemented carbonate formation can be extracted via open-hole well completion from the well. Using reservoir data and wellbore modeling and simulator software, the number and location of autonomous inflow control devices (AICDs) and packers in the wellbore can be estimated, allowing for a successful completion with an AICD. Depending on the reservoir and fluid qualities, this will aid in the production of fluids from each zone of the wellbore [ 31 , 32 , 33 , 34 , 35 ]. The occurrence of considerable permeability variation along the wellbore can result in non-uniform production in wellbore zones. The AICD maintains productive wells by regulating oil and water flows independently. This can also assist minimize water coning at the well’s heel by lessening the “heel-toe effect” that happens in long wellbores. Perforated tubing can be used to complete zones with exceptionally low permeability, resulting in negligible pressure drop and efficient production. AICDs can sustain hydrocarbon displacement by the below heterogeneous oil-water contact along the horizontal wellbore by balancing the inflow throughout the wellbore. Producing hydrocarbons with varying viscosities is one of several applications for the autonomous ICD [ 36 , 37 , 38 , 39 , 40 , 41 ].
AICDs are rate-dependent, along with fluid property identification, and provide effective light oil production, resulting in increased oil output while preventing the flow of undesirable fluids ( Figure 7 ). Because of the AICD’s sensitivity, oil can generate and restrict water and gas, effectively regulating water cut and GOR [ 42 ]. Fractures are the primary source of variability that contributes to extremely high permeability zones. If connected to a water source, these zones may give very high hydrocarbon production and early water breakthrough. AICDs can successfully produce an extra pressure drop in these high permeability zones while managing wellbore input and preventing early water breakthrough. When AICDs achieve breakthrough, they can effectively limit water and gas production by imposing significant resistance to their flow while enabling oil to flow through the least resistance. AICDs aid water breakthrough from these zones by creating an equal amount of hydrocarbons across the wellbore.
A long wellbore with high permeability variation
Presence of high viscosity hydrocarbon in the formation
Heterogeneous oil-water contact with near water zones
Presence of a gas cap in the formation along with high-water aquifer support
Presence of high permeability fractures connecting the below water zones

Completion strategy for highly heterogeneous carbonate formation using AICVs.
The production of cemented carbonates eliminates the problem of wellbore collapse. Because the formation is highly heterogeneous, the well should be completed utilizing effective zonal isolation by producing a well into segments. To maximize oil recovery while delaying or controlling water output, the optimal well completion strategy given the aforementioned reservoir characteristics is to employ autonomous inflow control valves (AICVs). By isolating each zone of the wellbore with packers determined by reservoir modeling and simulation software for the precise reservoir parameters, AICVs can be placed anywhere along the wellbore length to take advantage of various reservoir features. The zone contributing to very high permeability due to the existence of a large number of cracks can be totally segregated without the installation of AICV, resulting in early and very high-water production right from the start [ 43 , 44 ].
The AICVs will create hydrocarbon in each segment of the wellbore with a homogeneous influx of fluids, ensuring consistent production across the whole length of the wellbore. In high permeability zones, autonomous inflow control valves will provide an extra pressure drop, permitting equal fluid flow in each segment of the wellbore [ 45 ]. It will also lessen the possibility of water coning in the lengthy wellbore due to the heel-toe phenomenon. AICVs are extremely effective in producing high-viscosity fluids (oil) by allowing low restrictions to their flow into the wellbore, while allowing low-viscosity fluids (water and gas) to flow through heavy restrictions and produce in negligible amounts. AICVs essentially allow high-viscosity fluids to flow freely while effectively shutting them down for low-viscosity fluids.
AICVs can fully shut down output from a zone when water breaks through from nearby water zones or moving oil-water contact occurs owing to heterogeneous oil-water contact ( Figure 8 ). The existence of a gas cap contributing to high GOR can be controlled with AICV by turning off the operation and enabling oil in other zones to retain well productivity due to its applicability for shutting off production for low-viscosity fluids. AICV is the best device for shutting down production in a zone connected with a high-permeability fracture because it restricts the flow of water the most. Since water can acquire the entire wellbore section through high permeability cracks connecting the water aquifer, the AICVs successfully avoid these zones by shutting down production while preserving well productivity by producing through other zones [ 46 , 47 ].
A highly heterogeneous formation with a high length and thick pay zone containing a very high amount of reserves
There is considerable permeability variation in the formation and plentiful water aquifers
There is the presence of low-viscosity oil.
Presence of a large amount of high- and low-permeability fractures
A gas cap formation and the need for artificial lift in future

Completion strategy for highly heterogeneous carbonate formation using IWC.
Since the aforementioned formation is a cemented carbonate formation, an open hole well completion can be used to eliminate the possibility of hole collapse. Carbonate formations like this one require state-of-the-art intelligent well completion equipment due to its high reserves and complicated geology. It is necessary to partition the well into sub-sections according to the various requirements of the reservoir for the intelligent well completion system to function properly. This can assist in producing independently from each zone of the wellbore to balance the overall well output [ 48 ].
The surface-controlled inflow control valves will be the best choice for monitoring the behavior of each zone and controlling the valves based on the need to produce from that zone ( Figure 9 ). Due to the limited installation downhole, an electrically driven inflow control valve would be the preferred choice to produce the big formation over hydraulic inflow control valves [ 49 , 50 ]. This intelligent well completion can help to monitor reservoir and fluid parameters from each zone, as well as manage flow through varying openings in each zone. This will allow you to manually respond to changing behavior in each zone while remaining productive. The temperature, pressure, flow, and composition of fluid flowing in each zone are monitored by a sensor and transmitted to the surface analysis system, where the appropriate approach to the behavior is decided and controlled from the surface with the varying openings of the inflow control valves [ 51 ]. Using an intelligent well-completion system, controlling each zone based on its features would aid in recovering maximum reserves in a very heterogeneous formation [ 46 , 52 ].
Indicators of the formation’s heterogeneity include the presence of both high- and low-permeability cracks and the presence of a wide range of permeability fluctuations. Intelligent good completion is autonomous in identifying fluid features because it is not automatic but rather is operated by carefully examining the fluid’s qualities. As a result, water and gas breakthroughs may be easily monitored by operators due to high permeability fractures or gas caps, and necessary actions are done by totally shutting off the valves while continuing to produce oil and other zones. Intelligent well completion enables artificial lift such as in-situ gas lift, gas lift, or ESP to recover maximum reserves from the formation in the future for the production of remaining reserves under low pressure.
An intelligent well completion system is an incredibly expensive system that can only be installed in formations with a large number of reserves existing, such as the above formation, where the well cost can be overcome and the end recovery results in a good profit, making the well profitable. The western offshore field is a highly heterogeneous cemented carbonate formation. It has a strong water aquifer support beneath the pay zone and a gas cap. Its remarkable heterogeneity is dominated by cracks, vugs, and solution channels. Water can easily flow from the aquifer to the wellbore as a result of these fissures, some of which are connected to the bottom water aquifer support. Because of water coning in the adjacent water zones and close to the oil-water interface, water breakthrough occurs early. The northern part of the field is thinner, making the well more susceptible to water coning (15–20 m).
Oil-water contact is consistent across the field, with a common oil-water contact at 1477 m in both the northern and southern portions. Permeability changes dramatically along the field’s length due to significant variability. A reservoir pressure of 2000 to 2500 psi and a temperature of 100 to 120°C are typical in this field. The carbonate deposit has a permeability range of 1–500 millidarcy and a porosity range of 15–25%. In comparison to the formation water, the oil in this deposit has a low API gravity of about 35 to 42°C. In reservoir circumstances, oil has a viscosity of 0.9 cP, formation water is 0.4 cP, and gas has a viscosity of 0.02 cP. The field’s initial oil in situ is expected to be 112.48 MMT, with final reserves of 42.54 MMT. 35.54 MMT (31.6 OIIP) of which has been generated. A redevelopment plan is being implemented, with 9 additional wells being added to the current 92 wells in order to collect the remaining reserves from the field.
According to literature research [ 6 , 7 , 12 , 13 , 20 , 21 , 22 ] examining reservoir characteristics and water cut issues that are similar to the Western Offshore Field, AICD is the best completion method for reducing water cut. Using swell packers to permanently seal off the well is a crucial aspect of the completion technique when implementing AICD. By separating the well into sectors using zonal isolation and installing AICD in each sector, it is possible to generate a homogeneous influx along the horizontal wellbore. Optimizing the placement and number of swell packers and AICDs can be done by modeling the well with a NETool or Static Simulator Software and comparing input numbers from other wells or drilling and logging data. The highly diverse Western Offshore Field can now maximize oil production with the aid of AICD by effectively preventing and controlling water breakthrough. High permeability zones can contribute to an unbalanced input of fluids along the wellbore’s length, however, AICD will effectively restrict their flow [ 53 ]. If fluids are constantly moving down the wellbore, water is less likely to condense in high-permeability areas and rush out the well before it is ready. Careful input management [ 54 , 55 ] can reduce the possibility of an early water breakthrough caused by the displacement of water from neighboring water zones and close oil-water interaction.
Due to the formation’s high heterogeneity caused by the presence of fractures, vugs, and solution channels, it is crucial to regulate inflow to prevent fluids from these conduits from dominating the flow into the wellbore and limiting flow from other zones. AICD will be able to control the flow from these pipes and maintain a steady flow of water into the well [ 56 ]. If these pipes are connected to a water supply, water will flow through them instead of oil, making a breakthrough nearly impossible to prevent. The development of AICD will permit oil and water to be efficiently managed together. Water going through AICD will be severely limited due to its oblique path around the output, but oil will encounter fewer restrictions thanks to its radial flow pattern. AICD will lead to increased oil production and better gas output regulation. This thin oil rim deposit has a stable water aquifer and a gas cap that regulates the amount of water and gas that can be taken, making it a good candidate for hydrocarbon extraction using AICD. With AICD, the input across the wellbore will be managed to avoid premature water breakthrough in the very heterogeneous Western Offshore Field formation, and after water breakthrough has occurred, AICD will efficiently control water production to ensure maximum oil recovery.
Engineers determined that a Range 1 Fluidic Diode AICD would be the most effective ICD for the Western Offshore Field due to fluid characteristics being similar to Completion Strategy Case 2. When AICD detects certain fluid qualities, it is able to shut off the water supply. The temperature of all the oil in the field is between 35°C and 42°C on the API scale, and its viscosity is only 0.9 cP. Additionally, the viscosity difference between field oil (0.9 cP) and water is rather small (0.4 cP). The AICD (0.3–1.5 cP) Range 1 Fluidic Diode type excels for making light oil and identifying the minute differences between fluids. If the tiny difference in viscosity between light oil and water can be determined [ 57 ], then AICDs of the Range 1 Fluidic Diode type can be utilized to restrict water flow and boost oil output. AICD’s ability to stabilize input is especially helpful in regions where permeability fluctuates significantly (more than 0.1 mD but less than 500 mD). Therefore, the completion technique using a Range 1 Fluidic Diode type AICD and Zonal Isolation was more appropriate for the Western Offshore Field. To this end, it was planned to implement a completion approach wherein the wells involved in the field’s rehabilitation plan would have AICDs of the Range 1 Fluidic Diode type installed with Zonal Isolation.
Two newly side-tracked wells were completed in accordance with the AICD Completion Strategy as part of the Field Redevelopment Program and began production in August 2021. Placement and quantity of packers and AICDs installed were determined by NETool software modeling of a well, and zonal isolation was achieved by using swell packers and AICDs of the Range 1 Fluidic Diode type (0.3–1.5 cP).
When the production performance of these wells was analyzed, the wells performed similarly to other wells (without an Inflow Control System) in the initial phase of production, with 6 and 11% of water cut. However, after 9 months of operation, the water cut of these wells climbed to 38 and 47%, respectively, when compared to other wells where a steady increase in water cut was noted ( Figure 10 ). The other wells achieved greater than 80% water cut within 4–5 months after going into production. When compared to other wells, the wells finished with a Completion Strategy performed exceptionally well in terms of limiting the water cut and producing adequate amounts of hydrocarbon, thereby recovering the residual hydrocarbon efficiently. The Completion Strategy performed admirably, as expected, with Zonal Isolation using swell packers producing hydrocarbons from each zone specifically to maintain uniform oil production across the wellbore, whereas AICDs assisted in producing high amounts of hydrocarbons and effectively controlling water production. The best solution to manage the water cut issue was found to be completing the wells as part of the Field Redevelopment Program using a Completion Strategy that included Zonal Isolation with Swell Packers and Range 1 Fluidic Diode type AICD (0.3–1.5 cP).

Completion strategy for initial flow rate vs. post flow rate.
5. Results and discussion
Water’s low viscosity allows it to flow through oil and into the entire wellbore section, and this phenomenon, known as water cut, is shown to worsen with time once it has established itself in a well. This was uncovered after an exhaustive study of the finished Western Offshore Field and an appreciation of the multiple water shut-off jobs utilized to regulate water output revealed the truth. Since water can flow through any channel connecting the wellbore when water cut problem arises, it is very challenging to regulate water production. Water shut-off can be accomplished in a number of ways, both mechanically and chemically. Most methods of water shut-off used to lessen the severity of water shortage worries are not particularly effective, and they cannot be relied upon to halt water output for long [ 58 ]. Water shut-off techniques can only limit the water cut to a certain level for a few period of time before the well starts producing an enhanced volume of water. This is because halting water in a highly permeable heterogeneous field is particularly tough. As a whole, it is rather challenging to manage the water once it has begun to flow, which makes the goods costly and the well uneconomical. Rather than relying on reactive methods to regulate future water production, this method takes preventative action throughout a well’s lifetime. Using modern inflow control systems, such as ICDs, AICDs, ICVs, AICVs, and intelligent wells, in conjunction with zonal isolation based on reservoir properties, can prevent uncontrolled production and water breakthrough. This method of well finishing can help increase the well’s profitability by increasing its useful life, decreasing the need for well intervention, increasing hydrocarbon production, and decreasing water production. In addition, the chapter provides several illustrations of various reservoir characteristics with suitable completion processes for regulating water production.
6. Conclusion
To identify problems and find solutions for decreasing water cut and increasing field output, we examined well-by-well production and injection behavior using a variety of classical plots; analyzed pre-existing in-house water shut-off methods (both mechanical and chemical); and investigated global technologies.
Studies of the Indian Western Offshore Field, its water cut issues, and the various water shut-off methods implemented in its wells, as well as several water shut-off case studies, revealed that: It is difficult, ineffective, and nearly impossible to increase the productivity of a well for a longer period of time by attempting to control water production after the fact. Mechanical and chemical water shut-off jobs are difficult to execute down hole, and even if they are, they only give temporary respite from water scarcity. Therefore, water shut-off solutions cannot ensure water cut mitigation.
If a completion plan is developed to prevent and manage water output, it may be possible to avoid well intervention for water shut-off actions. The proper completion method will serve as an efficient water control measure even before the problem occurs, rather than applying for water shut-off work after the fact, which has a low success rate and is less effective. This technique can reduce water output by delaying the emergence of water breakthroughs in a well through the use of preventative measures. For one thing, it employs Zonal Isolation in tandem with a reservoir-specific Inflow Control System (ICD, AICD, ICVs, or AICVs).
It recognizes water’s individual qualities and limits its flow to restrict its breakthrough output, whereas looser restrictions promote oil production. Early water breakthrough can be prevented with the help of an Inflow Control System and Zonal Isolation, which work together to equalize the inflow across the wellbore and then isolate the source of the water flow after it has broken through, all while keeping oil production at a steady rate.
A completion strategy involving a Range 1 Fluidic Diode type AICD with Zonal Isolation utilizing Swell Packers was proposed to install in horizontal wells for the future redevelopment plan of the field after studying the reservoir and fluid properties of the Western Offshore Field with the issue of high-water cut. This finishing method can be created for deployment in a field’s wells to efficiently eliminate water cut problems and boost future wells’ productivity by utilizing simulator software to decide the placement and number of AICDs and Packers. Possible effects of this completion approach include a decrease in water production and an increase in cumulative oil output from the very heterogeneous western offshore field.
Comprehensive completion plans are suggested for the future redevelopment of the field, which will involve drilling or side-tracking additional wells. It was agreed that Range 1 Fluidic Diode AICDs will be employed to complete subsequent wells. Two new side-tracked wells on the P-3 and P-4 Platforms were completed utilizing the completion technique of zonal isolation using swell packers and Range 1 Fluidic Diode type AICD (0.3–1.5 cP), resulting in excellent control over water cut and good hydrocarbon rates. As a result, the project of Completion Strategy for controlling water cut in the field was found to be successful, and it was agreed that future wells to be drilled and produced to recover the remaining hydrocarbons would be completed using the same Completion Strategy.
Acknowledgments
The authors are grateful to the Dr. Vishwanath Karad MIT World Peace University for providing us with all the facilities that were required work.
- 1. James EJ, Hossain MM. Evaluation of Factors Influencing the Effectiveness of Passive and Autonomous Inflow Control Devices; SPE-186926-MS. Society of Petroleum Engineering (SPE); 2017. pp. 1-15
- 2. Elverhoy AB, Aakre H, Mathiesen V. Autonomous Inflow Control for Reduced Water Cut and/or Gas-Oil Ratio; OTC-28860-MS. Society of Petroleum Engineering (SPE); 2018. pp. 1-15
- 3. Qian J, Shen Z, Huang P, Xue J. The Application of Water Shut-off Technique in Jidong Oil Field; SPE-188098-MS. Society of Petroleum Engineering (SPE); 2017
- 4. Turner B, SPE, TIORCO, Zahner B, SPE, VENOCO, Inc. Polymer Gel Water Shut-off Applications Combined with Stimulation Increase Oil Production and Life of Wells in the Monterey Formation Offshore California; SPE-121194. Society of Petroleum Engineering (SPE); 2009
- 5. Harlan TD, SPE, Chevron, USA, Reed SD, SPE, Forman JM, SPE. Foamed Cement Selection for Horizontal Liners Proves Effective for Zonal Isolation- Case History; SPE-71055. Society of Petroleum Engineering (SPE); 2001
- 6. Igbal F, Iskandar R, Radwan E, Doulk H. Autonomous Inflow Control Device: A Case Study of First Successful Field Trial in GCC for Water Conformance; SPE-177927-MS. Society of Petroleum Engineering (SPE); 2015. pp. 1-11
- 7. Corona G, Yin W, Fripp M, et al. Testing of a Novel Autonomous ICD with Low-viscosity Multiphase Fluids; OTC-27789-MS. Society of Petroleum Engineering (SPE); 2017. pp. 1-14
- 8. Arangath RS, SPE-EE, Mkpasi, Mobil Producing Nigeria (ExxonMobil Subsidiary), SPE. Water Shut-off Treatments in Open Hole Horizontal Wells Completed with Slotted Liners; SPE-74806. Society of Petroleum Engineering (SPE); 2002
- 9. Kosztin B, Ferdiansyah E, Zadjali F. Petroleum Development Oman LLC. Joint Stimulation-Water Shut-off Technology Leads to Extra Oil from Mature Fields; SPE-149658-MS. Society of Petroleum Engineering (SPE); 2012
- 10. Cottin C, Ai-Amrie O, Barrois E, Total ABK, Templier A, POWELTEC. Chemical Water Shut-off Pilot in Mature Offshore Carbonate Field; SPE-188871-MS. Society of Petroleum Engineering (SPE); 2017
- 11. Caili D. China University of Petroleum, Qing Y, Peking University and China University, Fulin Z, China University of Petroleum. Case Study on Polymer Gel to Control Water Coning for Horizontal Well in Offshore Oil Field; OTC-21125. Society of Petroleum Engineering (SPE); 2011
- 12. Shahreyar N, Corona G, Miller B. Multilateral Well Construction with Fluidic Diode AICD Completion Technologies: Case Study, North West Shelf, Western Australia; OTC-30445-MS. Society of Petroleum Engineering (SPE); 2020. pp. 1-12
- 13. Hajeri NA, Osann Y, Safar AI, et al. Simple and Reliable Innovative Fluid Dynamic Technology Maximizes Oil Recovery in Upper Burgan Reservoir; SPE-183167-MS. Society of Petroleum Engineering (SPE); 2016. pp. 1-10
- 14. Cooper RE, Pt. Dowell Schlumberger Indonesia, Troncoso JC, Atlantic Rich field Indonesia Inc. An Overview of Horizontal Well Completion Technology; SPE-17582. Society of Petroleum Engineering (SPE); 1988. pp. 335-350
- 15. Taha A, Amani M. Overview of water shut-off operations in oil and gas wells. Chemical Engineering. 2019; 3 (2):51
- 16. Xindi S, Daojun B. Missouri university of science and technology, comprehensive review of water shut-off methods for horizontal wells. Petroleum Exploration and Development. 2017; 44 (6):1022-1029
- 17. Seright RS, SPE, New Mexico Petroleum Recovery Research Center, Lane RH, SPE, Northstar Technologies IntI., and Sydansk RD, SPE, Sydansk Consulting Services, A Strategy for Attacking Excess Water Production; SPE 70067. 2001
- 18. Reddy BR, Eoff L, Dwyann Dalrymple E, Black K, Brown D, Rietjens M, A Natural Polymer Based Cross-Linker System Conformance Gel Systems; SPE-84937-PA. 2003
- 19. Kazemi S. Review of polymer gels for conformance control in oil reservoirs, University of Vindsor [thesis]. 2019
- 20. Ariffin MHM, Bakar H, Kumar A. Revitalizing Low Contrast Viscosities Between Oil and Water Reservoir Using Autonomous Inflow Control Device AICD Completion Strategy; SPE-202445-MS. Society of Petroleum Engineering (SPE); 2020. pp. 1-12
- 21. Ismail IM, Tendeka TGI, Tom F. Increased Oil Production in Super Thin Oil Rim Using the Application of Autonomous Inflow Control Devices; SPE-191590-MS. Society of Petroleum Engineering (SPE); 2018. pp. 1-21
- 22. Triandi M, Chigbo I, Khunmek T, Ismail IM. Field Case: Use of Autonomous Inflow Control Devices to Increase Oil Production in a Thin Oil Rim Reservoir in the Gulf of Thailand; SPE-193305-MS. Society of Petroleum Engineering (SPE); 2018. pp. 1-12
- 23. James EJ, Hossain MM. Curtin University, Evaluation of Factors Influencing the Effectiveness of Passive and Autonomous Inflow Control Devices; SPE-186926-MS. Society of Petroleum Engineering (SPE); 2017
- 24. Joseph BH, Galimzyanov A, Hughes B, Nazarenko P, Hughes B. Increasing Oil Recovery from Long Horizontal Wells Using Advanced Lower Completion Systems; SPE-170754-MS. Society of Petroleum Engineering (SPE); 2014
- 25. Fernandes P, Li Z. Understanding the Roles of Inflow Control Devices in Optimizing Horizontal Well Performance; SPE-124677. Society of Petroleum Engineering (SPE); 2009. pp. 1-12
- 26. Ratterman EE, Vool BA, Augustine JR. New Technology Applications to Extend Field Economic Life by Creating Uniform Flow Profiles in Horizontal Wells: Case Study and Technology Overview; OTC-17548. Society of Petroleum Engineering (SPE); 2005. pp. 1-8
- 27. Minulina P, Shahin AS, George Z, Bouchard M. The Design, Implementation and Use of Inflow Control Devices for Improving the Production Performance of Horizontal Wells; SPE-15745. Society of Petroleum Engineering (SPE); 2012
- 28. Shaker W, Majed S, Aishuwaikhat Ali A, Farooq Q. Unique Real Time Production Optimization Methodology for a Multilateral Well with Hydraulic and Electrical Inflow Control Valves; IPTC-19799. International Petroleum Technology Conference (IPTC); 2020
- 29. Xu Z. J. Phys, Research and Field Application of Adaptive Inflow Control Technology. Conference Series 1176 042091; 2019
- 30. Duku Oliver MM. Application of inflow control device (ICD) for optimizing horizontal well performance, thesis report, Universiti Teknologi Petronas. 2012
- 31. Elverhoy AB, Aakre H, Mathiesen V. Autonomous Inflow Control for Reduced Water Cut and/or Gas-Oil Ratio, Inflow Control; OTC-28860-MS. 2018
- 32. Peirce JW, Hutcherson MR, Jensen MD. SPE, Conoco Phillips, Brice BW, SPE, BP Alaska, Woods A, Schlumberge. An Overview of Conformance Control Efforts for the West Sak Field on the North Slope of Alaska; SPE-169073-MS. 2014
- 33. Igbal F, Iskandar R, Radwan E, Doulk H. Abu Dhabi Company for Onshore Petroleum Operations Ltd. (ADCO), Autonomous Inflow Control Device- A Case Study of First Successful Field Trial in GCC for Water Conformance; SPE-177927-MS. 2015
- 34. Corona G, Yin W, Maxime Coffin MF, Shahreyar N. Halliburton, Testing of a Novel Autonomous ICD with Low- Viscosity Multiphase Fluids; OTC-27789-MS. 2017
- 35. Shahreyar N, Corona G, Miller B. Multilateral Well Construction with Fluidic Diode AICD Completion Technologies: Case Study, North West Shelf, Western Australia; OTC-30445-MS. 2020
- 36. Ai-Hajeri N, Osann Y, Safar AI, Ai-Muttar B. Kuwait Oil Company, Simple and Reliable Innovative Fluid Dynamic Technology Maximizes Oil Recovery in Upper Burgan Reservoir; SPE-183167-MS. 2016
- 37. Hafizi M, Ariffin M, Asmizah Bakar H, Kumar A. PETRONAS Carigali Sdn. Bhd. Revitalizing Low Contrast Viscosities between Oil and Water Reservoir Using Autonomous Inflow Control Device AICD Completion Strategy; SPE-202445-MS. 2020
- 38. Ismarullizam Mohd Ismail T, tan Giok L, Tom F. Sapura Energy; Hillis F, Tendeka, Increased Oil Production in Super Thin Oil Rim Using the Application of Autonomous Inflow Control Devices; SPE-191590-MS. 2018
- 39. Triandi M, Chigbo I, Khunmek T. Mubadala Petroleum; Ismail IM, Tendeka, Field Case: Use of Autonomous Inflow Control Devices to Increase Oil Production in a Thin Oil Rim Reservoir in the Gulf of Thailand; SPE-193305-MS. 2018
- 40. Ai-Kadam M, Lee BO, Aramco S, Brandon Least H. First Autonomous ICD Installation in Saudi Arabia- Modelling a Field Case; SPE-177997-MS. Society of Petroleum Engineering (SPE); 2015
- 41. Corona G, Yin W, Fripp M, Coffin M, Shahreyar N. Testing of Novel Autonomous ICD with Low Viscosity Multiphase Fluids; OTC-27789-MS. Society of Petroleum Engineering (SPE); 2017
- 42. Liao C, Zhang W, Huang P, Qian J. The Study and Application of an Electric Intelligent Well Completion System with Electrically Driven Inflow Control Device and Long-Term Monitoring; SPE-186271-MS. Society of Petroleum Engineering (SPE); 2017. pp. 1-12
- 43. Bjerke A, Aakre H, Mathiesen V. Integrated Downhole Multiphase Flowmeter and Autonomous Inflow Control Valve, Inflow Control; SPE-197460-MS. Society of Petroleum Engineering (SPE); 2019
- 44. Jorden J, Jackson A, Marzouqi MA. Integrated Drilling, Completion, and Stimulation Approach Facilitates High Productivity Horizontal Well with 10,000-ft Pay Interval; IADC/SPE-151231. Society of Petroleum Engineering (SPE); 2012
- 45. Joseph P, Galimzyanov A, Nazarenko P. Increasing Oil Recovery from Long Horizontal Wells Using Advanced Lower Completion Systems; SPE-170754-MS. Society of Petroleum Engineering (SPE); 2014. pp. 1-10
- 46. Shaker W, Majed S, Aishuwaikhat Ali A, Farooq Q , Schlumberge. Unique Real Time Production Optimization Methodology for a Multilateral Well with Hydraulic and Electrical Inflow Control Valves; IPTC-19799. International Petroleum Technology Conference (IPTC); 2020
- 47. Aakre H. Inflow Control/Telemark University; Britt Halvorsen, Telemark University; Vidar Mathiesen, Inflow Control, Smart Well with Autonomous Inflow Control Valve Technology; SPE-164348-MS. Society of Petroleum Engineering (SPE); 2013
- 48. Liao C, Zhang W, Huang P, Qian J. Research Institute of Petroleum Exploration & Development, CNPC, The Study and Application of an Electric Intelligent Well Completion System with Electrically Driven Inflow Control Device and Long-Term Monitoring; SPE-186271-MS. Society of Petroleum Engineering (SPE); 2017
- 49. Bjerke A, Aakre H, Mathiesen V. Integrated Downhole Multiphase Flowmeter and Autonomous Inflow Control Valve; SPE-197460-MS. Society of Petroleum Engineering (SPE); 2019. pp. 1-9
- 50. Shaker W, Majed S, Ali A, Farooq Q. Unique Real-Time Optimization Methodology for a Multilateral Well with Hydraulic and Electrical Inflow Control Valves; IPTC-19799. International Petroleum Technology Conference (IPTC); 2020. pp. 1-11
- 51. Bhatkar S, Topare NS, Ahmed B. Analyzing corrosion prediction and dose optimization of corrosion inhibitor in oil field production. Techno-Societal. 2020; 2021 :861-868
- 52. Rodriguez JC, Figueroa AR. Penex, Intelligent Completions and Horizontal Wells Increase Production and Reduce Free- Gas and Water in Mature Fields; SPE-139404. Society of Petroleum Engineering (SPE); 2010
- 53. Jumah AA, Gokmen M, Harrasi A, Abri I, Buwaiqi S, Urdaneta G. Field Application of the Autonomous Inflow Control Device AICD for Optimized Heavy Oil Production in South Sultanate of Oman; SPE-200279-MS. Society of Petroleum Engineering (SPE). pp. 1-12
- 54. Ai-khelaiwi FT, Birchenko VM, Davies DR. SPE, Heriot-Watt University, SPE Well Dynamics, Advanced Wells: A Comprehensive Approach to the Selection between Passive and Active Inflow Control Completions; IPTC-1245. International Petroleum Technology Conference (IPTC); 2008
- 55. Huiyaun M, Chenggang Y, Liangliang D, Yukun F, Hanwen S. Review of intelligent well technology. Petroleum. 2020; 6 (3):226-233
- 56. Kalyani T, Corona G, Ross K. Fluidic Diode Autonomous ICD Selection Criteria, Design Methodology, and Performance Analysis for Multiple Completion Designs: Case Studies; SPE-200255-MS. Society of Petroleum Engineering (SPE); 2022. pp. 1-15
- 57. Agrawal P, Yousif S, Shokry A, Saqib T, Keshtta O, Bigno Y, et al. Unlocking by-passed Oil with Autonomous Inflow Control Devices through an Integrated Approach; SPE-207569-MS. Society of Petroleum Engineering (SPE); 2021. pp. 1-12
- 58. Kadam MA, Lee BO, Least B. First Autonomous ICD Installation in Saudi Arabia – Modelling a Field Case; SPE-177997-MS. Society of Petroleum Engineering (SPE); 2015. pp. 1-12
© 2024 The Author(s). Licensee IntechOpen. This chapter is distributed under the terms of the Creative Commons Attribution 3.0 License , which permits unrestricted use, distribution, and reproduction in any medium, provided the original work is properly cited.
Continue reading from the same book
Published: 21 August 2024
By Muhammad Hammad Rasool, Maqsood Ahmad, Ahsan Jawaa...
59 downloads
By Alexis Koulidis and Shehab Ahmed
47 downloads
By Ioana Stanciu
31 downloads
IntechOpen Author/Editor? To get your discount, log in .
Discounts available on purchase of multiple copies. View rates
Local taxes (VAT) are calculated in later steps, if applicable.
Support: [email protected]
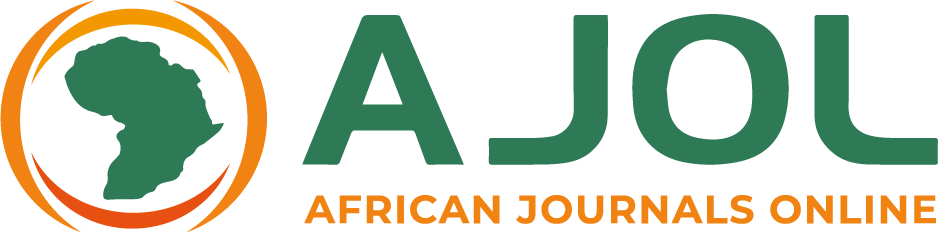
Global Journal of Educational Research Journal / Global Journal of Educational Research / Vol. 23 No. 3 (2024) / Articles (function() { function async_load(){ var s = document.createElement('script'); s.type = 'text/javascript'; s.async = true; var theUrl = 'https://www.journalquality.info/journalquality/ratings/2408-www-ajol-info-gjedr'; s.src = theUrl + ( theUrl.indexOf("?") >= 0 ? "&" : "?") + 'ref=' + encodeURIComponent(window.location.href); var embedder = document.getElementById('jpps-embedder-ajol-gjedr'); embedder.parentNode.insertBefore(s, embedder); } if (window.attachEvent) window.attachEvent('onload', async_load); else window.addEventListener('load', async_load, false); })();
Article sidebar.
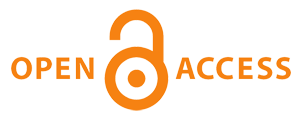
Article Details
© Bachudo Science Co. Ltd. This work is licensed under the creative commons Attribution 4.0 International license.
Olufowoke Mary Oyeyipo
Guidance and Counselling, School Director, Shepherd's Academy, Shepherd's Porch Church, Off MCC, Akai efai, Calabar, CRS, Nigeria
Main Article Content
Girl child early marriage and its impact on education: a case study of cross river state.
This study sought to investigate primarily the extent of girl child marriage and how it impacts education in Cross River State. Four purpose, research questions and hypothesis guided the study. The research design adopted for this study was the survey research design. The population for the study was all parents with school aged girls in their homes in Cross River State, Nigeria. Stratified random sampling technique was used to select nine local government for the study out of the 18 local government in the state. The actual sample respondents of 378 parents with school aged girls in their homes in Cross River State was drawn for the study from the sampled LGA. Three experts validated the instruments both the used to collect data for the study. Instrument was used for the study, the instrument was titled “Girl Child Early Marriage and Education questionnaire” was administered to the respondents to elicit their responses. The instruments was 23 items with 4-option answer format and 3 items to elicit the respondent’s demographic data. A pilot study was conducted on a sample of 40 participants and internal consistency coefficient ranging from .80-.83 was obtained using Cronbach alpha reliability estimate methods. The researchers administered the instruments on the sampled respondents in the selected faculty. The data that was collected was analyzed using and the hypothesis was tested using independent t-test, one way analysis of variance and Pearson product moment correlations at 0.05 level of significance. The result showed that Rate of Girl child marriage, Girl child family Social economic status, and Girl child Parental type education does significantly influence education in Cross River State. It was further fund that there is a strong positive relationship between Old folktales about girl child early marriage and education in Cross-River State. Above all it was recommended that government should create and implement policy that will efficiently improve educational accessibility among minority groups.
AJOL is a Non Profit Organisation that cannot function without donations. AJOL and the millions of African and international researchers who rely on our free services are deeply grateful for your contribution. AJOL is annually audited and was also independently assessed in 2019 by E&Y.
Your donation is guaranteed to directly contribute to Africans sharing their research output with a global readership.
- For annual AJOL Supporter contributions, please view our Supporters page.
Journal Identifiers
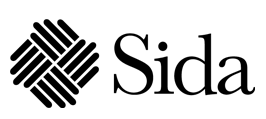
NTRS - NASA Technical Reports Server
Available downloads, related records.
Thank you for visiting nature.com. You are using a browser version with limited support for CSS. To obtain the best experience, we recommend you use a more up to date browser (or turn off compatibility mode in Internet Explorer). In the meantime, to ensure continued support, we are displaying the site without styles and JavaScript.
- View all journals
- Explore content
- About the journal
- Publish with us
- Sign up for alerts
- Open access
- Published: 22 August 2024
Improved efficacy behavioral modeling of microwave circuits through dimensionality reduction and fast global sensitivity analysis
- Slawomir Koziel 1 , 2 ,
- Anna Pietrenko-Dabrowska 2 &
- Leifur Leifsson 3
Scientific Reports volume 14 , Article number: 19465 ( 2024 ) Cite this article
Metrics details
- Electrical and electronic engineering
- Engineering
Behavioral models have garnered significant interest in the realm of high-frequency electronics. Their primary function is to substitute costly computational tools, notably electromagnetic (EM) analysis, for repetitive evaluations of the structure under consideration. These evaluations are often necessary for tasks like parameter tuning, statistical analysis, or multi-criterial design. However, constructing reliable surrogate models faces several challenges, including the nonlinearity of circuit characteristics and the vast size of the parameter space, encompassing both dimensionality and design variable ranges. Additionally, ensuring the validity of the model across broad geometry/material parameter and frequency ranges is crucial for its utility in design. The purpose of this paper is to introduce an innovative approach to cost-effective and dependable behavioral modeling of microwave passives. Central to our method is a fast global sensitivity analysis (FGSA) procedure, which is devised to identify correlations between design parameters and quantify their impacts on circuit characteristics. The most significant directions identified through FGSA are utilized to establish a reduced-dimensionality domain. Within this domain, the model may be constructed using a limited amount of data samples while capturing a significant portion of the circuit response variability, rendering it suitable for design purposes. The outstanding predictive capability of the proposed model, its superiority over traditional techniques, and its readiness for design applications are demonstrated through the analysis of three microstrip circuits of diverse characteristics.
Introduction
Computational tools have become indispensable in high-frequency electronics, among others, microwave design. These include both equivalent network simulators 1 , 2 , and full-wave electromagnetic (EM) analysis 3 , 4 . Versatility and reliability of the latter has been unquestionable, especially when it comes to quantifying phenomena difficult to be accounted for otherwise (EM cross-coupling, substrate anisotropy, the effects of surrounding components such as connectors, feed radiation, etc.). In particular, EM simulation is imperative for accurate evaluation of numerous modern circuits, e.g., compact structures obtained using line meandering 5 , the employment of slow-wave phenomenon 6 , 7 , multi-layer implementations 8 , or defected ground structures 9 .
The downside of EM analysis is it high computational cost, which is usually acceptable for one-time design verification or limited-scope parametric studies, but becomes a bottleneck for procedures requiring massive analyses (parameter tuning 10 , 11 , uncertainty quantification 12 , 13 , 14 , global optimization 15 , 16 , multi-criterial design 17 , 18 ). Globalized search is the most troublesome as it is often performed using nature-inspired algorithms 19 , 20 , 21 , 22 , 23 , 24 , operating at the budgets corresponding to many thousands of system evaluations. Not surprisingly, considerable research has been focused on development of accelerated algorithms. Some of available techniques include the employment of adjoint sensitivities 25 , sparse Jacobian updating schemes 26 , 27 , 28 , parallelization 29 , mesh deformation methods 30 (all of the above used to lower the expenses pertinent to sensitivity estimation in gradient-based algorithms), feature-based technology 31 , cognition-driven design 32 , multi-resolution techniques (e.g., space mapping 33 , response correction 34 , 35 , 36 ), dimensionality reduction approaches 37 , including model-order reduction 38 , 39 . A growing number of approaches incorporates surrogate modelling methods 33 , 40 , 41 , often in the form of iterative design procedures 42 , 43 (also referred to as machine learning 44 , 45 , 46 ). Some of popular modelling methods include polynomial regression 47 , radial basis functions 48 , kriging 49 , support vector machines 50 , Gaussian process regression (GPR) 51 , neural networks 52 , 53 , 54 , ensemble learning 55 , or polynomial chaos expansion 56 .
As mentioned earlier, surrogate modelling (especially behavioral, or data-driven) is a potentially attractive way to mitigate the challenges associated with high cost of multiple EM analyses. Replacing expensive simulations with a low-cost metamodel altogether would be a desirable solution to expedite procedures such as design optimization. Nevertheless, constructing models of microwave components is a challenging task for several reasons: nonlinearity of circuit outputs, curse of dimensionality 57 , and utility requirements (which entails coverage of wide geometry and material parameter ranges but also frequencies). Addressing these issues is a daunting task. Some of the methods attempt to make a better use of available data (e.g., adjust the very structure of the model to the distribution and nature of the input information, e.g., ensemble learning 58 , 59 ), efficiently handle large datasets (e.g., deep neural networks 60 , 61 ), or target a specific structure of the modeled responses (e.g., high-dimensional model representation (HDMR) 62 , orthogonal matching pursuit 63 ). On the other hand, the improvement of computational efficiency can be achieved using multi-resolution methods, for example, co-kriging 64 , Bayesian model fusion 65 , or two-stage GPR 66 . Yet another option is performance-driven modelling 67 , 68 , 69 , 70 . The techniques of this class capitalize on defining the surrogate’s domain so that it covers the region encapsulating high-quality designs 67 , and can be generalized to multi-resolution regime 71 , and employ deep learning methods 72 . The limitation is that the model setup is specific to a given set of performance specifications 68 , and domain definition itself requires reference designs that need to be acquired beforehand 69 . The latter increases the cost of the process. Recently, reference-design-free variations have been suggested 73 to mitigate this particular issue.
This research proposes a procedure for improved-efficiency generation of design-ready behavioral models of microwave passive circuits. An inherent part of our methodology is a procedure for fast global sensitivity analysis (FGSA), which has been developed to determine correlations between design parameters (both geometry and material), and establish their effects on the circuit responses. The results of FGSA allow for defining a reduced-dimensionality model domain, which is constructed using a set of directions responsible for the largest variability of the circuit responses. A rendition of a reliable data-driven model in restricted regions necessitates a limited amount of training points compared to traditional approaches. Nonetheless, the model accounts for the majority of the circuit response changes, which makes it suitable for design purposes. Comprehensive numerical studies conducted using three microstrip circuits demonstrate excellent dependability and accuracy of the proposed surrogate, and its superiority over modeling in conventional domains. The model design utility is corroborated through application case studies, in particular, circuit optimization under different scenarios concerning a variety of performance specifications.
Behavioral modeling by fast global sensitivity evaluation and dimensionality reduction
In this part of the manuscript, we elaborate on the algorithmic components of the modeling approach introduced in the study. The surrogate modeling task is stated in Section " Modelling problem statement ". Section " Low-cost global sensitivity analysis " discusses a fast global sensitivity analysis (FGSA) procedure introduced to determine the relationships between design variables and frequency characteristics of the system. The latter is expressed in terms of an orthonormal set of directions, which influences the circuit responses most significantly. In Section " Domain Definition ", these directions are employed to define a reduced-dimensionality domain of the behavioral model. The operation of the entire modeling framework is elucidated in Section " Complete modelling procedure ".
Modelling problem statement
In the following, we mark as R f ( x ) a response of the high-fidelity circuit model, evaluated through EM analysis. Here, x stands for a vector of designable parameters (cf. Table 1 for modelling-related terminology). Typically, we are interested in scattering parameters S kj ( x , f ), where k and j stand for the respective circuit ports; frequency is marked as f . The goal of behavioral modeling is to render a fast replacement model (surrogate) R s ( x ) of R f ( x ), valid over the region of interest (parameter space) X . The latter is normally an interval determined by the lower and upper parameter bounds (cf. Table 1 ). The surrogate is supposed to represent the system responses as well as possible in a given sense. The performance metric utilized here is the relative RMS error, defined as || R s ( x )– R f ( x )||/|| R f ( x )||. The model accuracy will be estimated using the average error E aver , evaluated using an independent set of testing points { x t ( k ) } k = 1, …, Nt , and defined as
The relative error is typically more intuitive than absolute metrics because it is independent of the actual value (and the unit) of the model output. In engineering applications, the error level of a few (e.g., five) percent, typically makes the model sufficient for design purposes. A discussion of alternative error measures can be found, e.g., in 74 , 75 .
Low-cost global sensitivity analysis
In Section " Introduction ", we discussed some fundamental challenges encountered in the behavioral modeling of microwave components. These challenges include the curse of dimensionality, the nonlinearity of frequency characteristics, and the necessity for the model to remain valid across wide ranges of geometry and material parameters. This latter requirement is particularly crucial for practical applications, such as design optimization. Among these challenges, those associated with the number of design variables are particularly critical, as they often underpin other difficulties. Consider a scenario where the parameter space X is assumed to be uniform, meaning that typical response nonlinearity remains consistent across all regions within X . Whatever technique is employed for constructing a surrogate model (radial basis function 48 , kriging 49 , neural networks 52 , etc.), the modelling error is primarily a function of the mean distance between the data samples used for model training. More specifically, the smaller the distance, the better accuracy of the surrogate. The average distance, in turn, depends on the cardinality N of the dataset, and dimensionality of the space n : it is proportional to (1/ N ) 1/ n . Note that dimensionality n plays a major role here, e.g., to reduce the average distance by a factor of two for n = 3, the training dataset has to be enlarged by a factor of eight, whereas for n = 10, the corresponding factor exceeds 1,000.
Thus, dimensionality reduction is critical to facilitate a reliable behavioural modelling of microwave components. One of possible approaches is variable screening, where the system variables having the least significance are identified and eliminated from the problem. Some of available techniques include the Morris method 76 , Pearson correlation coefficients 77 , partial or correlation coefficients 78 . Another approach is global sensitivity analysis (GSA) (Sobol indices 79 , Jansen method 80 , regression-based methods 81 ), the goal of which is similar, i.e., to determine the relative importance of the system parameters, and to potentially exclude those that are of minor importance. Notwithstanding, the mentioned techniques are computationally expensive: estimation of the sensitivity indicators is based on large numbers of data samples. Another issue is that for most microwave components, dropping out individual variables is not possible without impairing the system capability to reach any particular set of target operating conditions. Most parameters (including the material ones, e.g., substrate permittivity) act in synergy and affect the system characteristics through their interactions.
In this research, an alternative technique for global sensitivity analysis is developed, which is to satisfy the following requirements:
It is cheap to execute, i.e., involves less than a hundred EM analyses of the system being modelled;
It allows for identifying important parameter space directions rather than to determine important variables; the ‘importance’ is understood in terms of the effects on the circuit response variability.
The operating flow of the proposed fast GSA technique (further referred to as FGSA) has been shown in Fig. 1 . The eigenvectors e j of the relocation matrix S form represent the parameter space directions that have a decreasing impact on the response variability. The latter is quantified by the corresponding eigenvalues λ j . By definition, the eigenvectors form an orthonormal basis in X . It should be mentioned that FGSA has a certain resemblance to the active subspace (AS) approaches (cf. 82 , 83 ). Therein, the objective is also to identify a lower-dimensionality parameter subspace corresponding to the largest response variations. However, the spectral analysis is carried out on the matrix composed of gradient vectors of the (scalar) function of interest obtained for a set of random observables. This makes AS computationally more expensive than FGSA, especially for higher-dimensional problems. On the other hand, similarly to GSA methods mentioned earlier, AS is likely to provide higher accuracy.
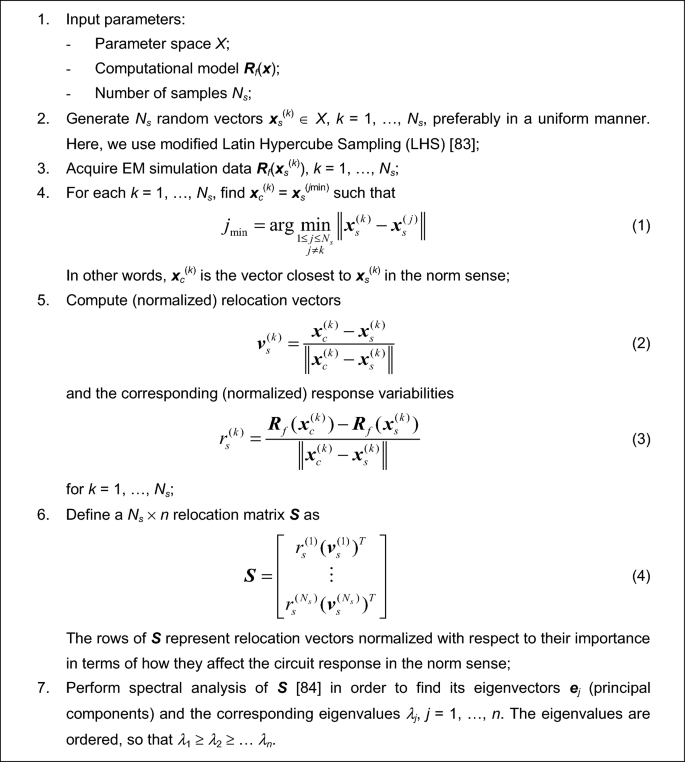
Pseudocode of the proposed fast global sensitivity analysis (FGSA). The eigenvectors e j represent the parameter space directions having major effects on the circuit characteristics; the importance is quantified using the eigenvalues λ j .
FGSA is employed as a tool for defining a reduced-dimensionality region for surrogate model establishment. For that purpose, we use a few most important eigenvectors, the number of which is determined as the smallest integer N d ∈ {1, 2, …, n } that satisfies
In other words, N d is the smallest number of vectors for which the corresponding (joint) relative least-square variability exceeds the user-defined threshold C min . In Section " Verification results ", we use C min = 0.9; accordingly, the selected directions should account for at least ninety percent of the overall circuit response variability.
The operation of FGSA is illustrated using several examples. The first instance is a linear function f ( x ) = f ([ x 1 x 2 ] T ) = 3 x 1 – 2 x 2 , shown in Fig. 2 . This function has been chosen because due to linearity, the direction of the maximum function variability can be readily identified as the gradient vector g = [3–2] T . This has been confirmed by FGSA, here based on twenty random points, cf. Figure 2 b. Figure 3 shows two more examples involving nonlinear functions defined so that the directions of maximum function variability can be easily assessed visually (specifically, as the vectors perpendicular to the directions of the f ( x ) ‘ripples’). Also in these cases, FGSA involving twenty random points correctly identified the relevant directions.
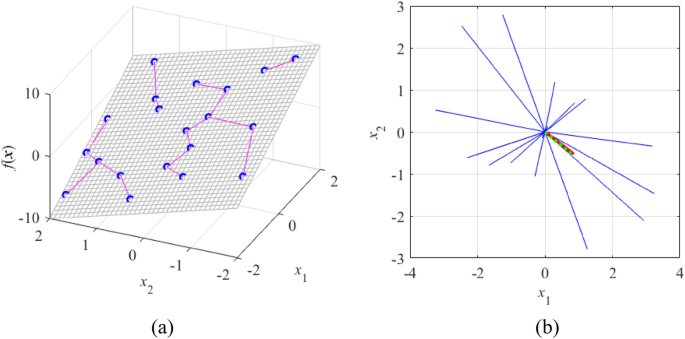
FGSA illustration using a linear function f ( x ) = f ([ x 1 x 2 ] T ) = 3 x 1 –2 x 2 : ( a ) surface plot of the function (gray), twenty random observables x s ( k ) (circles), and relocation vectors x c ( k ) – x s ( k ) (line segments); ( b ) relocation matrix vectors r s ( k ) v s ( k ) (thin lines), the largest principal component e 1 (thick solid line), and the normalized gradient g = [3–2] T /13 1/2 (thick dotted line). In this example, all function variability occurs along the gradient g (the function is constant in the direction orthogonal to g ), which is well aligned with the vector e 1 , obtained using the proposed FGSA.
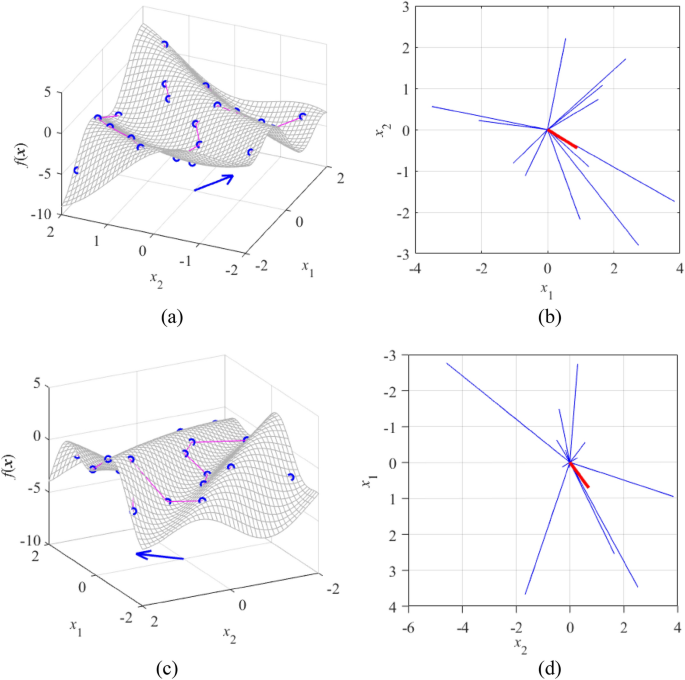
FGSA illustration using nonlinear functions of two variables: ( a ) surface plot of the first function f 1 ( x ) = f 1 ([ x 1 x 2 ] T ) = 2sin(2.5 x 1 )–1.5 x 2 –2exp( x 2 /5) + x 1 x 2 (gray), twenty random observables x s ( k ) (circles), and relocation vectors x c ( k ) – x s ( k ) (line segments), as well as the principal component e 1 (thick arrow); ( b ) relocation matrix vectors r s ( k ) v s ( k ) (thin lines), and the largest principal component e 1 (thick solid line); ( c ) and ( d ) surface plot and relocation matrix vectors for the second function f 2 ( x ) = f 2 ([ x 1 x 2 ] T ) = 1.5sin(3 x 1 + 2 x 2 )–1.1 x 1 2 –0.3 x 2 2 . It can be noticed that the vector e 1 obtained using FGSA visually corresponds to the direction associated with the highest variability of f ( x ).
Our final example is a miniaturized microstrip coupling structure shown in Fig. 4 a. The circuit features six design variables, x = [ l 1 l 2 l 3 d w w 1 ] T . Here, FGSA is carried out based on fifty random points uniformly allocated in the design space X . The latter is determined by the lower and upper bounds l = [2.0 7.0 12.5 0.2 0.7 0.2] T , and u = [4.5 12.5 22.0 0.65 1.5 0.9] T . Figure 4 b illustrates the EM-evaluated scattering parameters at a random parameter vector x and designs perturbed along the eigenvectors identified by FGSA, x + h e k , k = 1, …, n . As it can be observed, the response variability is the largest for k = 1, and gradually diminishes for increasing k .
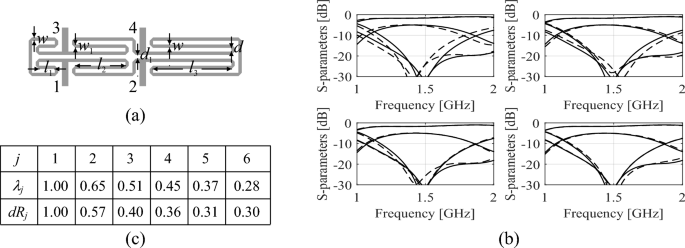
FGSA applied to a miniaturized rat-race coupler: ( a ) circuit topology, ( b ) scattering characteristics at a random vector x and designs perturbed along the principal components, x + h e k (here, h = 0.1) for the first four vectors (from top left to bottom right) obtained using FGSA, solid lines denote S -parameters at design x (| S 11 | through | S 41 |), whereas dashed lines denote corresponding S -parameters at the perturbed design; ( c ) normalized eigenvalues of the relocation matrix S obtained using FSGA based on twenty random samples, as well as average EM-simulated variability indicators dR j computed as in (6). It can be observed that response variability is gradually reduced for increasing k , which demonstrates that subsequent eigenvectors correspond to directions having less and less effect on circuit characteristics.
To estimate the actual response variability, N r = 20 random designs have been allocated in the parameter space, x r ( k ) , k = 1, …., N r , along with the perturbations x r ( k.j ) = x r ( k ) + h e j , j = 1, …, n . Having acquired the EM simulation data R f ( x r ( k ) ), k = 1, …, N r , and R f ( x r ( k.j ) ), k ∈ {1, …, N r }, j ∈ {1, …, n }, the variability indicators have been computed as
for j = 1, …, n . In (6), the norm \(\left\| {{\mathbf{R}}_{f} ({\mathbf{x}}) - {\mathbf{R}}_{f} ({\mathbf{y}})} \right\|\) is computed for all circuit responses, here, the scattering parameters S j 1 ( x , f ), j = 1, 2, 3, 4, and takes the form
where F is the simulation frequency range. The number dR j represents an average response variability in the direction e j . Upon normalization, dR j agree well with the normalized eigenvalues λ j , as shown in Fig. 4 (c), which demonstrates relevance of the presented sensitivity analysis approach.
Note that FGSA is computationally efficient. Many of the global sensitivity analysis techniques mentioned earlier (e.g., Sobol indices 79 , regression-based methods 81 ) require from a few hundred to a few thousands of data samples to yield accurate sensitivity assessment, depending on the problem size. FGSA is carried out based on a few dozen points. More specifically, in Section " Verification results ", only fifty samples are utilized. The sensitivity estimation accuracy is somehow compromised as compared to the expensive methods, but it is sufficient of our needs. It should also be reiterated that FGSA generates a set of principal directions that generally do not coincide with the coordinate system axes. Thus, instead of eliminating individual parameters, our method enables exploration of variable interactions and their joint effects on the circuit responses.
Domain Definition
The eigenvectors e j generated by FGSA are employed to establish the model domain X d , which is determined by N d most significant vectors e j , j = 1, …, N d . Recall that N d has been determined using (5). This arrangement allows for the domain to account for the majority of the circuit response variability within X (more specifically, the fraction equal or higher than C min , cf. Section " Low-cost global sensitivity analysis "). The formal definition of the domain is
i.e., the set encapsulating parameter vectors x c + a 1 e 1 + … + a Nd e Nd , where x c = [ l + u ]/2 is the centre of the original domain X (cf. Table 1 ), and a j , j = 1, …, N d , are real numbers. Figure 5 provides a graphical illustration of generating X d .
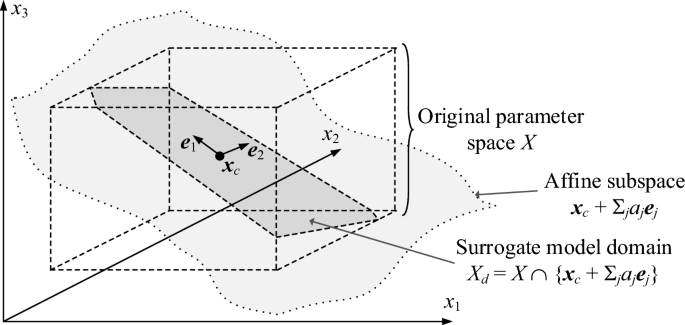
Constructing reduced-dimensionality domain X d . In the example shown, the original parameter space is three dimensional, whereas X d is determined by two principal vectors e 1 and e 2 . Note that X d is a set theory intersection of X and the affine subspace x c + Σ j =1,2 a j e j .
Again, while dim( X d ) < n , the domain accounts for the parameter space directions that are significant for the circuit response variability. This is necessary to secure design utility of the behavioural model to be established in X d . In this work, the underlying modelling technique is kriging 84 . However, a particular choice of the modelling method unimportant: our main goal is to investigate the benefits of dimensionality reduction by means of FGSA.
Complete modelling procedure
Figure 6 showcases the modelling process pseudocode. Meanwhile, Fig. 7 illustrates the flow diagram. The procedures consists of the three major stages: (i) fast global sensitivity analysis, FGSA (Stage I), (ii) domain definition (Stage II), and acquisition of the training data as well as surrogate model identification (Stage III). It should be emphasized that there is only one control parameter, which is the variability threshold C min . It is set to 0.9 in the verification studies considered in Section " Verification results ".
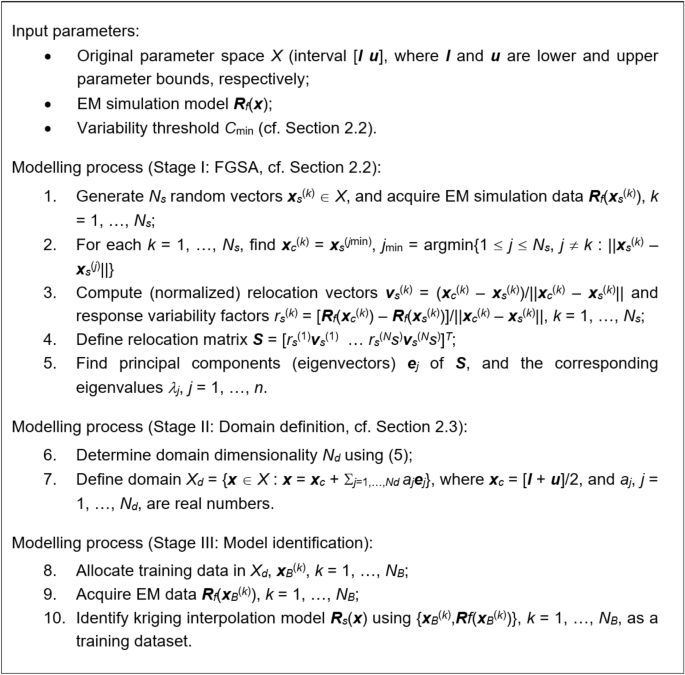
Surrogate modelling of microwave circuits using FGSA and reduced-dimensionality surrogates.
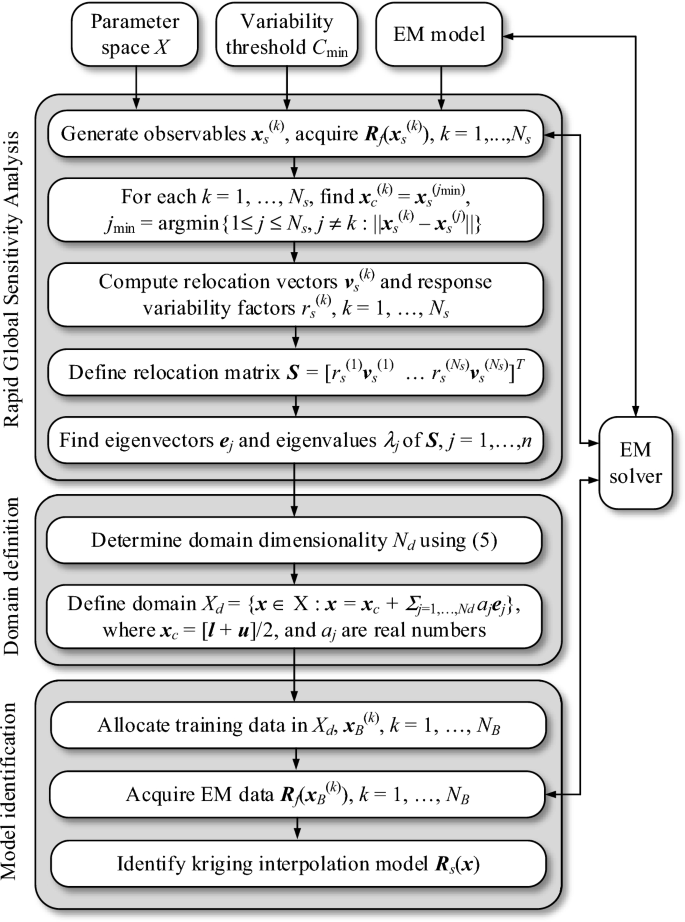
Flow diagram of the behavioral modeling procedure using FGSA and reduced-dimensionality domain.
Verification results
The modeling methodology outlined in Section " Behavioral modeling by fast global sensitivity evaluation and dimensionality reduction " is validated in this section through three examples of microwave components. The modeling captures the scattering parameters of these structures across broad ranges of geometry dimensions and frequencies. Reduced-dimensionality surrogates are compared to traditional metamodels concerning both accuracy and computational cost during model setup. Additionally, we explore how the predictive power of the model scales with the size of the training dataset. The practical applicability of the proposed approach will be further discussed in Section " Application case studies ".
Validation of the modeling framework introduced in Section " Behavioral modeling by fast global sensitivity evaluation and dimensionality reduction " is realized with the help of three microwave circuits. These are:
A miniaturized rat-race coupler (Circuit I) 85 ;
A branch-line coupler using compact microstrip resonant cells (CMRCs) (Circuit II) 86 ;
A dual-band equal-split power divider (Circuit III 87 .
The architectures of the circuits have been shown in Figs. 8 a, 9 a, and 10 a, respectively. Information about material parameters (substrate permittivity and height), design variables, parameter spaces, as well as modeled characteristics have been included in Figs. 8 b, 9 b, and 10 b for Circuit I, II, and III. One can note that the considered modeling problems are intricate. The parameter spaces are of relatively high dimensionality (when juxtaposing them with what is typically available in the literature), whereas the parameter ranges are wide. In particular, the mean values of the ratio between upper and lower bounds is close to three for Circuit I and II, and about nine for Circuit II. Furthermore, the modeling is conducted over broad ranges of frequency (0.5 GHz to 2.5 GHz for Circuit I, 0.1 GHz to 2.5 GHz for Circuit II, and 0.5 GHz to 7.0 GHz for Circuit III). Finally, the surrogates are to represent several complex responses of each structure.
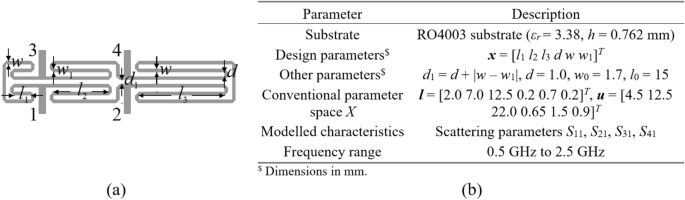
Test Circuit I: ( a ) parameterized geometry, ( b ) important data.
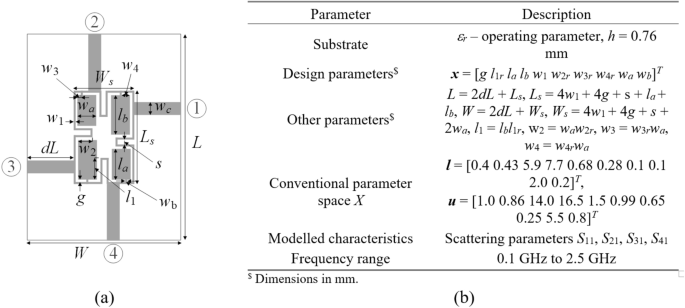
Test Circuit II: ( a ) parameterized geometry, ( b ) important data.
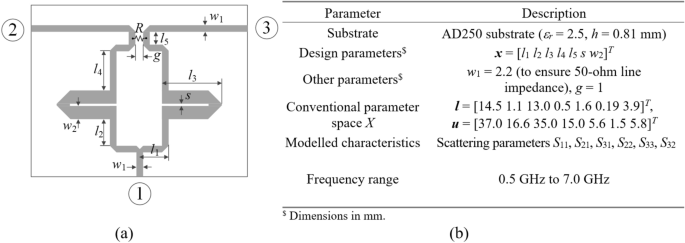
Test Circuit III: ( a ) parameterized geometry, ( b ) important data.
Experimental setup
The validation studies have been carried out to verify the two hypotheses concerning the properties of our modelling methodology:
Dimensionality reduction by means of the fast GSA of Section " Low-cost global sensitivity analysis " enables a considerable enhancement of the model accuracy and scalability, i.e., more favourable relation between the training set size and the model’s predictive power;
Dimensionality reduction and the computational benefits associated with it are not detrimental to design suitability of the surrogate.
The second hypothesis will be discussed in Section " Application case studies ". Its meaning is whether operating in a reduced space leaves sufficient flexibility for surrogate to be used for design purposes. The first hypothesis will be addressed in the remaining part of this section. To investigate the features of the presented modelling approach, we compare surrogate models rendered in the full-dimensional space X with those obtained within the reduced set. The FGSA procedure is conducted based on fifty random samples allocated in X by means of modified Latin Hypercube Sampling 88 . The dimensionality N d of the model domain is computed for the threshold C min = 0.9 in (5), cf. Section " Low-cost global sensitivity analysis ", i.e., the domain is to account for ninety percent of the system response variability or more. Furthermore, the surrogate models are established using sample sets of several sizes N B = 50, 100, 200, 400, and 800. This will allow us to determine the scalability of the model predictive power as a function of N B .
In this study, the modelling routine of choice is kriging (setup: 2nd-order polynomial as a regression function, Gaussian correlation function). However, this is of secondary importance as our goal is mainly to determine potential benefits of using FGSA and dimensionality-reduced domains. As mentioned earlier, the model accuracy is estimated using the relative root-mean square (RMS) error, || R s ( x )– R f ( x )||/|| R f ( x )||, where R s and R f stand for the model-predicted and EM-evaluated circuit responses. To compute the error we utilize one hundred independent test samples randomly distributed within the model domain.
The first point for discussion is the sensitivity analysis of Section " Behavioral modeling by fast global sensitivity evaluation and dimensionality reduction ". Table 2 includes the data on the eigenvalues λ k generated by FGSA, and the dimensionality N d of the model domain determined according to (5) with C min = 0.9. Note that in practice, N d corresponding to variability factor slightly lower than C min was approved in order to keep the domain dimensionality as small as possible. One can observe that N d is considerably smaller than the number n of the circuit design variables. The (multiplicative) dimensionality reduction factor is 2.0 (Circuit I), 2.8 (Circuit II), and 1.8 (Circuit III). It is the smallest for Circuit III, which is the most difficult case for two reasons: (i) the modelled characteristics are defined over broad range of frequencies, and (ii) the circuit responses are highly nonlinear over the entire frequency spectrum. Furthermore, it should be emphasized that for this structure, the eigenvalues are much less reduced between λ 1 and λ n (here, from 1.00 to 0.45), which indicates low parameter redundancy. This is in contrast to Circuits I and especially Circuit II, where the eigenvalues drop down much faster. This behaviour is typical for miniaturized components, both CMRC-based and those employing line meandering, and enables a more efficient dimensionality reduction.
The reliability of the conventional and the proposed surrogates constructed using training datasets of sizes 50 through 800 samples have been shown in Table 3 . Only for Circuit III, the models were also constructed using 1,600 samples, which is because it is the most challenging case, for which it was not possible to reduce the modelling error below ten percent with the 800-sample training set. The plots of the metamodel-predicted and EM-evaluated scattering parameters of Circuit I through III for chosen test points can be found in Figs. 11 , 12 , and 13 , respectively.
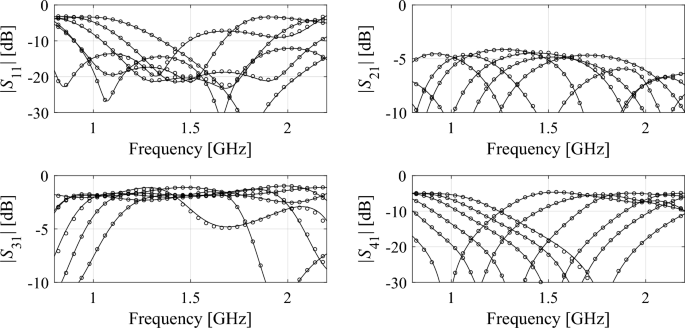
Circuit I: scattering parameters at the chosen testing points: EM analysis (—), and the introduced FGSA-based model (o). The training set size N B = 400.
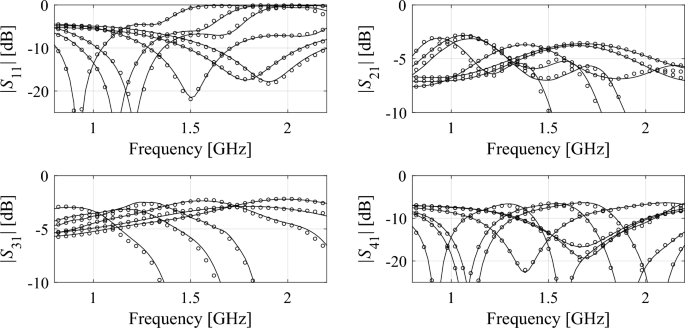
Circuit II: scattering parameters at the chosen testing points: EM analysis (—), and the introduced FGSA-based model (o). The training set size N B = 800.
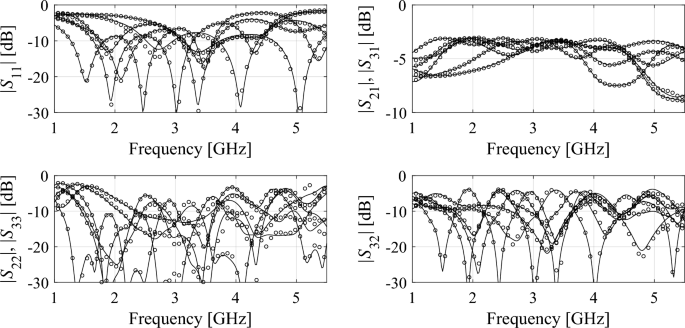
Circuit III: scattering parameters at the chosen testing points: EM analysis (—), and the introduced FGSA-based model (o). The training set size N B = 1,600.
The data encapsulated in Table 3 corroborates that dimensionality reduction greatly affects the surrogate model accuracy. Circuit I is the only case where modeling in the original parameter space is capable of rendering surrogates that feature relative error below ten percent. For this structure, modeling in a reduced-dimensionality domain allows for keeping the error below five percent already for training sample sets as small as 100 samples. Circuit II is considerably more challenging. Here, the conventional model is clearly unusable (RMS error over twenty percent) even for the largest dataset. At the same time, dimensionality reduction using fast GSA enables a dramatic accuracy improvement to about six percent. Finally, for Circuit III, the most difficult case, conventional modeling is exceptionally poor, also when the training dataset cardinality is enlarged to 1,600 samples. Dimensionality reduction enables error level reduction to only eight percent, which allows us to expect that the surrogate might be usable as a design aid.
Application case studies
The numerical results presented in Section " Results " unanimously demonstrate the benefits of reducing domain dimensionality through the proposed fast global sensitivity analysis. In particular, it makes it possible to render accurate surrogate models using reasonably small numbers of training points, as shown in Figs. 11 , 12 , 13 . Nevertheless, a more important question is whether domain restriction is not detrimental for design utility of the model. Although a dimensionality-reduced domain is smaller than the original one, recall that it is spanned along the directions that correspond to the maximum response variability (i.e., those associated with the largest eigenvalues of the variability matrix S of (4)). Consequently, limiting the number of dimensions should not affect the ability of the surrogate to accommodate the optimum designs to a large extent. In order to verify this property, the GSA-based models are employed in this section to optimize Circuits I through III under different design scenarios. As the modeling process has been carried out over broad frequency spectra, the surrogate are utilized to optimize the respective circuit for a variety of operating frequencies, but—in the case of Circuit II—also different substrate materials (note that substrate permittivity ε r is one of the design parameters for Circuit II, with the range from 2.0 to 5.0).
Table 4 delineates performance requirements for the considered devices. It should be noted that for Circuit III, equal power division is ensured by the structure’s symmetry. The parameters of each circuit were tuned for four distinct target values of the operating parameters, which are f 0 and K P for Circuit I, f 0 and ε r for Circuit II, as well as f 1 and f 2 for Circuit III. The findings have been compiled in Tables 5 , 6 , and 7 , while Figs. 14 , 15 , and 16 illustrate the optimized circuit responses for Circuit I, II, and III. Notably, optimization of the proposed surrogate model produces satisfactory designs across all cases. Moreover, there is excellent alignment between the model-predicted characteristics and those simulated through electromagnetic (EM) methods. These results collectively support the practical applicability of the modeling methodology discussed in this study, especially its effectiveness in designing circuits across wide ranges of operating conditions, such as center frequencies and power split ratios.
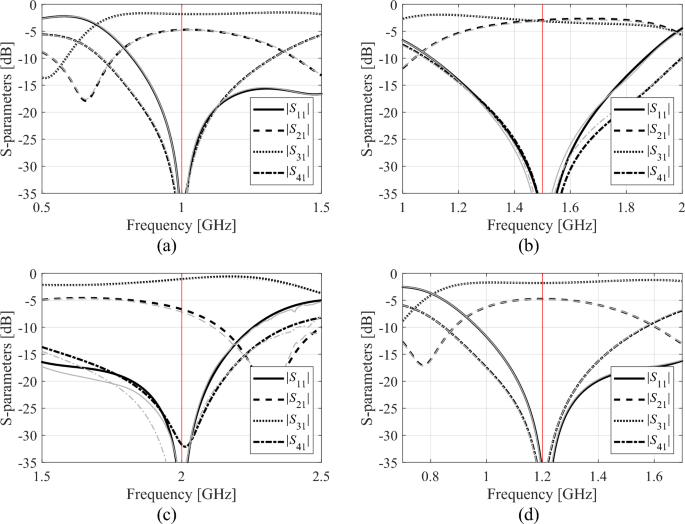
Optimization results for Circuit I: surrogate- (gray) and EM-simulation (black) at designs found by optimizing the proposed surrogate ( N B = 400). Operating frequency marked using the vertical line: ( a ) Case 1, ( b ) Case 2, ( c ) Case 3, ( d ) Case 4.
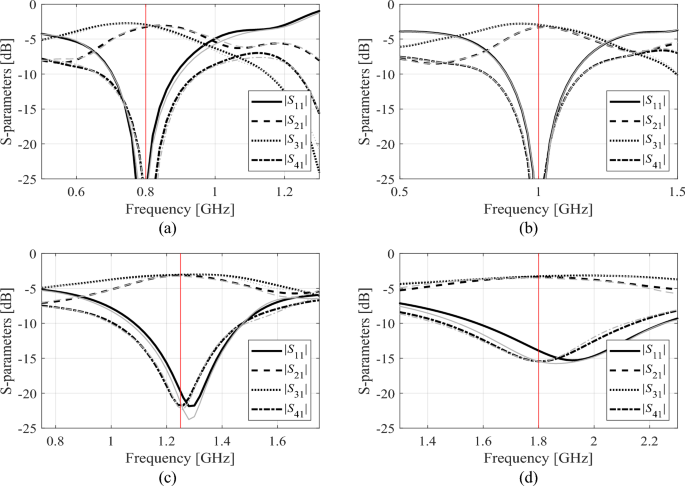
Optimization results for Circuit II: surrogate- (gray) and EM-simulation (black) at designs found by optimizing the proposed surrogate ( N B = 800). Operating frequency marked using the vertical line: ( a ) Case 1, ( b ) Case 2, ( c ) Case 3, ( d ) Case 4.
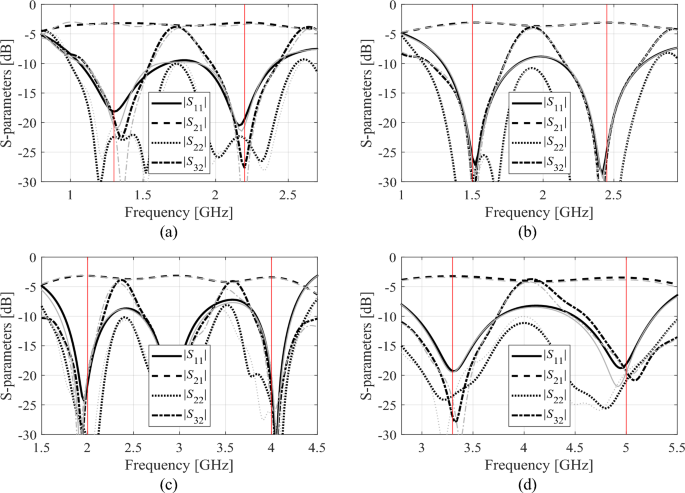
Optimization results for Circuit I: surrogate- (gray) and EM-simulation (black) at designs found by optimizing the proposed surrogate ( N B = 1600). Operating frequencies marked using the vertical lines: ( a ) Case 1, ( b ) Case 2, ( c ) Case 3, ( d ) Case 4.
This study presents a method for enhancing the accuracy of surrogate modeling for passive microwave components. The approach introduced is grounded in fast global sensitivity analysis (FGSA), which has been developed to identify, at a low cost, the parameter space directions that exert the most significant influence on circuit responses. By employing a small subset of these critical directions, determined through eigenvalue analysis, the surrogate model domain is effectively spanned. This setup facilitates the creation of reliable metamodels across wide frequency ranges, as well as varying geometry and material parameters, using relatively modest training datasets. Importantly, this approach preserves the design versatility of the surrogates, as the directions defining the domain capture the majority of circuit response variability.
The proposed technique has undergone comprehensive validation using three microstrip components, including two compact couplers and a dual-band power divider. The results demonstrate a significant improvement in predictive power compared to conventional methods, with relative RMS errors reaching only a few percent even with just a few hundred training samples. This improvement is notable despite considering wide frequency ranges (0.5 GHz to 2.5 GHz, 0.1 GHz to 2.5 GHz, and 0.5 GHz to 7.0 GHz for the first, second, and third circuits, respectively) and material parameter variations (substrate permittivity ranging from 2.0 to 5.0 for the second circuit). At the same time, modeling in the original domains results in remarkably poor performance and unusable models featuring relative errors of over twenty percent (except the simplest case of a rat-race coupler).
The aforementioned investigations have been supplemented by the application case studies. In these experiments, the proposed surrogate models were employed to optimize the test circuits under a variety of scenarios (different center frequencies, power division ratios, substrate permittivity values). The obtained results allowed us to establish that dimensionality reduction as described in the paper is not detrimental to design utility of the models. The findings of this work suggest that the presented methodology may be viewed an attractive alternative to state-of-the-art modeling techniques. Although demonstrated using kriging interpolation, it can be coupled with any specific approximation method. Furthermore, it is generic as opposed to some of the recent methods, which are also domain-oriented (e.g., performance-driven techniques). Finally, it is easy to implement, which is of a practical value for researchers with less experience in the fields of numerical modeling and optimization.
It should also be emphasized that due to its data-driven nature, the proposed modelling methodology can be applied to a broader class of microwave structures. In particular, the constructed surrogate models and the dimensionality reduction process are entirely based on acquired EM simulation data. The microstrip circuits employed in Section " Application case studies " were merely illustration examples. Some of worth mentioning areas include components for 5G/6G applications (e.g., antennas operating within frequency bands beyond 24 GHz), as well as broadband systems implemented on fiberglass substrates (challenging due to geometrical complexity, position-based permittivity variations, and multi-resonance outputs).
Data availability
The datasets used and/or analyzed during the current study available from the corresponding author on reasonable request.
ADS (Advanced Design System), Keysight Technologies, Fountaingrove Parkway 1400, Santa Rosa, CA 95403–1799, 2021.
AWR Microwave Office, Cadence Design Systems, Inc. San Jose, CA 95134, USA, 2022.
CST Microwave Studio, https://www.3ds.com/products-services/simulia/products/cst-studio-suite/ , Dassault Systems, Rue Marcel Dassault, 78140 Velizy-Villacoublay, France, 2022.
HFSS, Release 19.0, ANSYS, http://www.ansoft.com/products/hf/hfss/ , 2600 Ansys Dr., Canonsburg, PA 15317, USA, 2019.
Hou, Z. J. et al. A compact and low-loss bandpass filter using self-coupled folded-line resonator with capacitive feeding technique. IEEE Electron Dev. Lett. 39 (10), 1584–1587 (2018).
CAS Google Scholar
Shum, K. M., Mo, T. T., Xue, Q. & Chan, C. H. A compact bandpass filter with two tuning transmission zeros using a CMRC resonator. IEEE Trans. Microwave Theory Techn. 53 (3), 895–900 (2005).
ADS Google Scholar
Chen, S. et al. A frequency synthesizer based microwave permittivity sensor using CMRC structure. IEEE Access 6 , 8556–8563 (2018).
Google Scholar
Wu, D.-S., Li, Y. C., Xue, Q. & Mou, J. “LTCC bandstop filters with controllable bandwidths using transmission zeros pair. IEEE Trans. Circ. Syst. II: Express Briefs 67 (6), 1034–1038 (2020).
Sen, S. & Moyra, T. “Compact microstrip low-pass filtering power divider with wide harmonic suppression. IET Microwaves Ant. Propag. 13 (12), 2026–2031 (2019).
Dong, Y., Yang, B., Yu, Z. & Zhou, J. Robust fast electromagnetic optimization of SIW filters using model-based deviation estimation and Jacobian matrix update. IEEE Access 8 , 2708–2722 (2020).
Koziel, S., Pietrenko-Dabrowska, A. & Plotka, P. Design specification management with automated decision-making for reliable optimization of miniaturized microwave components. Sci. Rep. 12 , 829 (2022).
ADS CAS PubMed PubMed Central Google Scholar
Ren, Z., He, S., Zhang, D., Zhang, Y. & Koh, C. S. A possibility-based robust optimal design algorithm in preliminary design state of electromagnetic devices. IEEE Trans. Magn. 52 (3), 7001–7504 (2016).
Prasad, A. K., Ahadi, M. & Roy, S. Multidimensional uncertainty quantification of microwave/RF networks using linear regression and optimal design of experiments. IEEE Trans. Microwave Theory Techn. 64 (8), 2433–2446 (2016).
Rayas-Sanchez, J. E. & Gutierrez-Ayala, V. EM-based monte carlo analysis and yield prediction of microwave circuits using linear-input neural-output space mapping. IEEE Trans. Microwave Theory Techn. 54 (12), 4528–4537 (2006).
Liu, B., Yang, H. & Lancaster, M. J. Global optimization of microwave filters based on a surrogate model-assisted evolutionary algorithm. IEEE Trans. Microwave Theory Techn. 65 (6), 1976–1985 (2017).
Torun, H. M. & Swaminathan, M. High-dimensional global optimization method for high-frequency electronic design. IEEE Trans. Microwave Theory Techn. 67 (6), 2128–2142 (2019).
Lim, D. K., Yi, K. P., Jung, S. Y., Jung, H. K. & Ro, J. S. Optimal design of an interior permanent magnet synchronous motor by using a new surrogate-assisted multi-objective optimization. IEEE Trans. Magn. 51 (11), 8207504 (2015).
Koziel, S. & Pietrenko-Dabrowska, A. Constrained multi-objective optimization of compact microwave circuits by design triangulation and Pareto front interpolation. Eur. J. Op. Res. 299 (1), 302–312 (2022).
Luo, X., Yang, B. & Qian, H. J. Adaptive synthesis for resonator-coupled filters based on particle swarm optimization. IEEE Trans. Microwave Theory Techn. 67 (2), 712–725 (2019).
Li, X., Duan, B., Zhou, J., Song, L. & Zhang, Y. Planar array synthesis for optimal microwave power transmission with multiple constraints. IEEE Ant. Wireless Propag. Lett. 16 , 70–73 (2017).
Oyelade, O. N., Ezugwu, A.E.-S., Mohamed, T. I. A. & Abualigah, L. Ebola optimization search algorithm: A new nature-inspired metaheuristic optimization algorithm. IEEE Access 10 , 16150–16177 (2022).
Majumder, A., Chatterjee, S., Chatterjee, S., Sinha Chaudhari, S. & Poddar, D. R. Optimization of small-signal model of GaN HEMT by using evolutionary algorithms. IEEE Microwave Wireless Comp. Lett. 27 (4), 362–364 (2017).
Li, X. & Luk, K. M. The grey wolf optimizer and its applications in electromagnetics. IEEE Trans. Ant. Prop. 68 (3), 2186–2197 (2020).
Ding, D., Zhang, Q., Xia, J., Zhou, A. & Yang, L. Wiggly parallel-coupled line design by using multiobjective evolutionary algorithm. IEEE Microwave Wireless Comp. Lett. 28 (8), 648–650 (2018).
Wang, J., Yang, X. S. & Wang, B. Z. Efficient gradient-based optimisation of pixel antenna with large-scale connections. IET Microwaves Ant. Prop. 12 (3), 385–389 (2018).
Koziel, S. & Pietrenko-Dabrowska, A. Reduced-cost electromagnetic-driven optimization of antenna structures by means of trust-region gradient-search with sparse Jacobian updates. IET Microwaves Ant. Prop. 13 (10), 1646–1652 (2019).
S. Koziel and A. Pietrenko-Dabrowska, “Variable-fidelity simulation models and sparse gradient updates for cost-efficient optimization of compact antenna input characteristics,” Sensors , 19(8), (2019).
Pietrenko-Dabrowska, A. & Koziel, S. Computationally-efficient design optimization of antennas by accelerated gradient search with sensitivity and design change monitoring. IET Microwaves Ant. Prop. 14 (2), 165–170 (2020).
Zhang, W. et al. EM-centric multiphysics optimization of microwave components using parallel computational approach. IEEE Trans. Microwave Theory Techn. 68 (2), 479–489 (2020).
Feng, F. et al. Coarse- and fine-mesh space mapping for EM optimization incorporating mesh deformation. IEEE Microwave Wireless Comp. Lett. 29 (8), 510–512 (2019).
Koziel, S. Fast simulation-driven antenna design using response-feature surrogates. Int. J. RF Micr. CAE 25 (5), 394–402 (2015).
Zhang, C., Feng, F., Gongal-Reddy, V., Zhang, Q. J. & Bandler, J. W. Cognition-driven formulation of space mapping for equal-ripple optimization of microwave filters. IEEE Trans. Microwave Theory Techn. 63 (7), 2154–2165 (2015).
ADS CAS Google Scholar
Cheng, Q. S., Rautio, J. C., Bandler, J. W. & Koziel, S. Progress in simulator-based tuning—the art of tuning space mapping. IEEE Microwave Magazine 11 (4), 96–110 (2010).
Koziel, S. & Unnsteinsson, S. D. Expedited design closure of antennas by means of trust-region-based adaptive response scaling. IEEE Ant. Wireless Propag. Lett. 17 (6), 1099–1103 (2018).
Su, Y., Li, J., Fan, Z. and Chen, R. Shaping optimization of double reflector antenna based on manifold mapping, Int. Applied Comp. Electromagnetics Soc. Symp. , ACES , pp. 1–2, Suzhou, China, 1–4. (2017).
Koziel, S. & Leifsson, L. Simulation-Driven Design by Knowledge-based Response Correction Techniques (Springer, New York, 2016).
Pietrenko-Dabrowska, A. & Koziel, S. Reliable surrogate modeling of antenna input characteristics by means of domain confinement and principal components. Electronics 9 (5), 1–16 (2020).
Spina, D., Ferranti, F., Antonini, G., Dhaene, T. & Knockaert, L. Efficient variability analysis of electromagnetic systems via polynomial chaos and model order reduction. IEEE Trans. Comp. Packaging Manuf. Technal. 4 (6), 1038–1051 (2014).
Zhang, J., Feng, F. & Zhang, Q.-J. Rapid yield estimation of microwave passive components using model-order reduction based neuro-transfer function models. IEEE Microwave Wireless Comp. Lett. 31 (4), 333–336 (2021).
MathSciNet Google Scholar
Feng, F. et al. Adaptive feature zero assisted surrogate-based EM optimization for microwave filter design. IEEE Microwave Wireless Comp. Lett. 29 (1), 2–4 (2019).
Hassan, A. K. S. O., Etman, A. S. & Soliman, E. A. Optimization of a novel nano antenna with two radiation modes using kriging surrogate models. IEEE Photonic J. 10 (4), 4800807 (2018).
Liu, B. et al. An efficient method for antenna design optimization based on evolutionary computation and machine learning techniques. IEEE Trans. Ant. Propag. 62 (1), 7–18 (2014).
Zhang, Z., Chen, H. C. & Cheng, Q. S. Surrogate-assisted quasi-Newton enhanced global optimization of antennas based on a heuristic hypersphere sampling. IEEE Trans. Ant. Propag. 69 (5), 2993–2998 (2021).
Wu, Q., Wang, H. & Hong, W. Multistage collaborative machine learning and its application to antenna modeling and optimization. IEEE Trans. Ant. Propag. 68 (5), 3397–3409 (2020).
Taran, N., Ionel, D. M. & Dorrell, D. G. Two-level surrogate-assisted differential evolution multi-objective optimization of electric machines using 3-D FEA. IEEE Trans. Magn. 54 (11), 8107605 (2018).
Wu, Q., Chen, W., Yu, C., Wang, H. and Hong, W. Multilayer machine learning-assisted optimization-based robust design and its applications to antennas and arrays, IEEE Trans. Ant. Prop. , Early view, (2021).
Chávez-Hurtado, J. L. & Rayas-Sánchez, J. E. Polynomial-based surrogate modeling of RF and microwave circuits in frequency domain exploiting the multinomial theorem. IEEE Trans. Microwave Theory Tech. 64 (12), 4371–4381 (2016).
Zhou, Q. et al. An active learning radial basis function modeling method based on self-organization maps for simulation-based design problems. Knowl.-Based Syst. 131 , 10–27 (2017).
de Villiers, D. I. L., Couckuyt, I. and Dhaene, T. Multi-objective optimization of reflector antennas using kriging and probability of improvement, Int. Symp. Ant. Prop. , pp. 985–986, San Diego, USA, (2017).
Cai, J., King, J., Yu, C., Liu, J. & Sun, L. Support vector regression-based behavioral modeling technique for RF power transistors. IEEE Microwave Wireless Comp. Lett. 28 (5), 428–430 (2018).
Jacobs, J. P. Characterization by Gaussian processes of finite substrate size effects on gain patterns of microstrip antennas. IET Microwaves Ant. Prop. 10 (11), 1189–1195 (2016).
Dong, J., Qin, W. & Wang, M. “Fast multi-objective optimization of multi-parameter antenna structures based on improved BPNN surrogate model. IEEE Access 7 , 77692–77701 (2019).
Calik, N., Belen, M., Mahouti, P. & Koziel, S. Accurate modeling of frequency selective surfaces using fully-connected regression model with automated architecture determination and parameter selection based on Bayesian optimization. IEEE Access 9 , 38396–38410 (2021).
Koziel, S., Mahouti, P., Calik, N., Belen, M. A. & Szczepanski, S. Improved modeling of miniaturized microwave structures using performance-driven fully-connected regression surrogate. IEEE Access 9 , 71470–71481 (2021).
Yang, J. & Wang, F. Auto-ensemble: an adaptive learning rate scheduling based deep learning model ensembling. IEEE Access 8 , 217499–217509 (2020).
Du, J. & Roblin, C. Stochastic surrogate models of deformable antennas based on vector spherical harmonics and polynomial chaos expansions: Application to textile antennas. IEEE Trans. Ant. Prop. 66 (7), 3610–3622 (2018).
Pang, Y., Zhou, B. & Nie, F. “Simultaneously learning neighborship and projection matrix for supervised dimensionality reduction. IEEE Trans. Neural Netw. Learn. Syst. 30 (9), 2779–2793 (2019).
MathSciNet PubMed Google Scholar
Müller, D., Soto-Rey, I. & Kramer, F. An analysis on ensemble learning optimized medical image classification with deep convolutional neural networks. IEEE Access 10 , 66467–66480 (2022).
Zhang, L., Ni, Q., Zhai, M., Moreno, J. & Briso, C. An ensemble learning scheme for indoor-outdoor classification based on KPIs of LTE network. IEEE Access 7 , 63057–63065 (2019).
Jin, J. et al. A novel deep neural network topology for parametric modeling of passive microwave components. IEEE Access 8 , 82273–82285 (2020).
Jin, J. et al. Deep neural network technique for high-dimensional microwave modeling and applications to parameter extraction of microwave filters. IEEE Trans. Microwave Theory Tech. 67 (10), 4140–4155 (2019).
Yücel, A. C., Bağcı, H. & Michielssen, E. “An ME-PC enhanced HDMR method for efficient statistical analysis of multiconductor transmission line networks. IEEE Trans. Comp. Packaging Manuf. Tech. 5 (5), 685–696 (2015).
Becerra, J. A. et al. A doubly orthogonal matching pursuit algorithm for sparse predistortion of power amplifiers. IEEE Microwave Wireless Comp. Lett. 28 (8), 726–728 (2018).
Kennedy, M. C. & O’Hagan, A. Predicting the output from complex computer code when fast approximations are available. Biometrika 87 , 1–13 (2000).
Wang, F. et al. “Bayesian model fusion: large-scale performance modeling of analog and mixed-signal circuits by reusing early-stage data. IEEE Trans. Comput.-Aided Design Integr. Circ. Syst. 35 (8), 1255–1268 (2016).
Jacobs, J. P. & Koziel, S. Two-stage framework for efficient Gaussian process modeling of antenna input characteristics. IEEE Trans. Antennas Prop. 62 (2), 706–713 (2014).
Koziel, S. & Pietrenko-Dabrowska, A. Performance-Driven Surrogate Modeling of High-Frequency Structures (Springer, New York, 2020).
Koziel, S. & Pietrenko-Dabrowska, A. Performance-based nested surrogate modeling of antenna input characteristics. IEEE Trans. Ant. Prop. 67 (5), 2904–2912 (2019).
Koziel, S. Low-cost data-driven surrogate modeling of antenna structures by constrained sampling. IEEE Antennas Wireless Prop. Lett. 16 , 461–464 (2017).
Koziel, S. & Sigurdsson, A. T. Triangulation-based constrained surrogate modeling of antennas. IEEE Trans. Ant. Prop. 66 (8), 4170–4179 (2018).
Pietrenko-Dabrowska, A. & Koziel, S. Antenna modeling using variable-fidelity EM simulations and constrained co-kriging. IEEE Access 8 (1), 91048–91056 (2020).
Koziel, S., Calik, N., Mahouti, P. & Belen, M. A. Accurate modeling of antenna structures by means of domain confinement and pyramidal deep neural networks. IEEE Trans. Ant. Prop. 70 (3), 2174–2188 (2022).
Koziel, S. and Pietrenko-Dabrowska, A. Knowledge-based performance-driven modeling of antenna structures, Knowledge Based Systems , paper no. 107698, (2021).
Li, X. R. & Zhao, Z. Evaluation of estimation algorithms part I: Incomprehensive measures of performance. IEEE Trans. Aerospace Electr. Syst. 42 (4), 1340–1358 (2006).
Gorissen, D., Crombecq, K., Couckuyt, I., Dhaene, T. & Demeester, P. A surrogate modeling and adaptive sampling toolbox for computer based design. J. Mach. Learn. Res. 11 , 2051–2055 (2010).
Morris, M. D. Factorial sampling plans for preliminary computational experiments. Technometrics 33 , 161–174 (1991).
Iooss, B. & Lemaitre, P. A Review on Global Sensitivity Analysis Methods. In Uncertainty Management in Simulation-Optimization of Complex Systems (eds Dellino, G. & Meloni, C.) 101–122 (Springer, 2015).
Tian, W. A review of sensitivity analysis methods in building energy analysis. Renew. Sustain. Energy Rev. 20 , 411–419 (2013).
Saltelli, A. Making best use of model evaluations to compute sensitivity indices. Com. Physics. Comm. 145 , 280–297 (2002).
Jansen, M. J. W. Analysis of variance designs for model output. Comp. Phys. Comm. 117 , 25–43 (1999).
Kovacs, I., Topa, M., Buzo, A., Rafaila, M. & Pelz, G. Comparison of sensitivity analysis methods in high-dimensional verification spaces. Acta Tecnica Napocensis. Electron. Telecommun. 57 (3), 16–23 (2016).
Constantine, P. G., Dow, E. & Wang, Q. Active subspace methods in theory and practice: Applications to kriging surfaces. SIAM J. Sci. Comp. 36 (4), 1500–1524 (2014).
Ma, H., Li, E. P., Cangellaris, A. C. & Chen, X. Support vector regression-based active subspace (SVR-AS) modeling of high-speed links for fast and accurate sensitivity analysis. IEEE Access 8 , 74339–74348 (2020).
Forrester, A. I. J. & Keane, A. J. Recent advances in surrogate-based optimization. Prog. Aerospace Sci. 45 , 50–79 (2009).
Koziel, S. & Sigurdsson, A. T. Performance-driven modeling of compact couplers in restricted domains. Int. J. RF Microwave CAE 28 (6), e21296 (2018).
Tseng, C. & Chang, C. A rigorous design methodology for compact planar branch-line and rat-race couplers with asymmetrical T-structures. IEEE Trans. Microwave Theory Tech. 60 (7), 2085–2092 (2012).
Lin, Z. & Chu, Q.-X. A novel approach to the design of dual-band power divider with variable power dividing ratio based on coupled-lines. Prog. Electromagn. Res. 103 , 271–284 (2010).
Beachkofski, B. and Grandhi, R. Improved distributed hypercube sampling, American Institute of Aeronautics and Astronautics , paper AIAA 2002–1274, 2002.
Download references
Acknowledgement
The authors would like to thank Dassault Systemes, France, for making CST Microwave Studio available. This work is partially supported by the Icelandic Centre for Research (RANNIS) Grant 239858 and by National Science Centre of Poland Grant 2022/47/B/ST7/00072
Author information
Authors and affiliations.
Engineering Optimization & Modeling Center, Reykjavik University, 101, Reykjavik, Iceland
Slawomir Koziel
Faculty of Electronics, Telecommunications and Informatics, Gdansk University of Technology, 80-233, Gdansk, Poland
Slawomir Koziel & Anna Pietrenko-Dabrowska
School of Aeronautics and Astronautics, Purdue University, West Lafayette, IN, 47907, USA
Leifur Leifsson
You can also search for this author in PubMed Google Scholar
Contributions
Conceptualization, S.K., A.P.; methodology, S.K. and A.P.; data generation, S.K. and L.L.; investigation, S.K. and A.P.; writing—original draft preparation, S.K. and A.P.; writing—review and editing, S.K. and L.L.; visualization, S.K. and L.L.; supervision, S.K.; project administration, S.K. and A.P.
Corresponding author
Correspondence to Slawomir Koziel .
Ethics declarations
Competing interests.
The authors declare no competing interests.
Additional information
Publisher's note.
Springer Nature remains neutral with regard to jurisdictional claims in published maps and institutional affiliations.
Rights and permissions
Open Access This article is licensed under a Creative Commons Attribution-NonCommercial-NoDerivatives 4.0 International License, which permits any non-commercial use, sharing, distribution and reproduction in any medium or format, as long as you give appropriate credit to the original author(s) and the source, provide a link to the Creative Commons licence, and indicate if you modified the licensed material. You do not have permission under this licence to share adapted material derived from this article or parts of it. The images or other third party material in this article are included in the article’s Creative Commons licence, unless indicated otherwise in a credit line to the material. If material is not included in the article’s Creative Commons licence and your intended use is not permitted by statutory regulation or exceeds the permitted use, you will need to obtain permission directly from the copyright holder. To view a copy of this licence, visit http://creativecommons.org/licenses/by-nc-nd/4.0/ .
Reprints and permissions
About this article
Cite this article.
Koziel, S., Pietrenko-Dabrowska, A. & Leifsson, L. Improved efficacy behavioral modeling of microwave circuits through dimensionality reduction and fast global sensitivity analysis. Sci Rep 14 , 19465 (2024). https://doi.org/10.1038/s41598-024-70246-4
Download citation
Received : 29 April 2024
Accepted : 14 August 2024
Published : 22 August 2024
DOI : https://doi.org/10.1038/s41598-024-70246-4
Share this article
Anyone you share the following link with will be able to read this content:
Sorry, a shareable link is not currently available for this article.
Provided by the Springer Nature SharedIt content-sharing initiative
- Microwave engineering
- Passive circuits
- Global sensitivity analysis
- Behavioral modeling
- Simulation-driven design
- Dimensionality reduction
By submitting a comment you agree to abide by our Terms and Community Guidelines . If you find something abusive or that does not comply with our terms or guidelines please flag it as inappropriate.
Quick links
- Explore articles by subject
- Guide to authors
- Editorial policies
Sign up for the Nature Briefing newsletter — what matters in science, free to your inbox daily.

Grab your spot at the free arXiv Accessibility Forum
Help | Advanced Search
Computer Science > Neural and Evolutionary Computing
Title: evaluation framework for ai-driven molecular design of multi-target drugs: brain diseases as a case study.
Abstract: The widespread application of Artificial Intelligence (AI) techniques has significantly influenced the development of new therapeutic agents. These computational methods can be used to design and predict the properties of generated molecules. Multi-target Drug Discovery (MTDD) is an emerging paradigm for discovering drugs against complex disorders that do not respond well to more traditional target-specific treatments, such as central nervous system, immune system, and cardiovascular diseases. Still, there is yet to be an established benchmark suite for assessing the effectiveness of AI tools for designing multi-target compounds. Standardized benchmarks allow for comparing existing techniques and promote rapid research progress. Hence, this work proposes an evaluation framework for molecule generation techniques in MTDD scenarios, considering brain diseases as a case study. Our methodology involves using large language models to select the appropriate molecular targets, gathering and preprocessing the bioassay datasets, training quantitative structure-activity relationship models to predict target modulation, and assessing other essential drug-likeness properties for implementing the benchmarks. Additionally, this work will assess the performance of four deep generative models and evolutionary algorithms over our benchmark suite. In our findings, both evolutionary algorithms and generative models can achieve competitive results across the proposed benchmarks.
Comments: | 8 pages, 1 figure, published in 2024 IEEE Congress on Evolutionary Computation (CEC) |
Subjects: | Neural and Evolutionary Computing (cs.NE); Artificial Intelligence (cs.AI) |
Cite as: | [cs.NE] |
(or [cs.NE] for this version) | |
Focus to learn more arXiv-issued DOI via DataCite | |
Journal reference: | 2024 IEEE Congress on Evolutionary Computation (CEC), Yokohama, Japan, 2024, pp. 1-8 |
: | Focus to learn more DOI(s) linking to related resources |
Submission history
Access paper:.
- HTML (experimental)
- Other Formats
References & Citations
- Google Scholar
- Semantic Scholar
BibTeX formatted citation

Bibliographic and Citation Tools
Code, data and media associated with this article, recommenders and search tools.
- Institution
arXivLabs: experimental projects with community collaborators
arXivLabs is a framework that allows collaborators to develop and share new arXiv features directly on our website.
Both individuals and organizations that work with arXivLabs have embraced and accepted our values of openness, community, excellence, and user data privacy. arXiv is committed to these values and only works with partners that adhere to them.
Have an idea for a project that will add value for arXiv's community? Learn more about arXivLabs .
A DQN based approach for large-scale EVs charging scheduling
- Original Article
- Open access
- Published: 21 August 2024
Cite this article
You have full access to this open access article
- Yingnan Han 1 ,
- Tianyang Li ORCID: orcid.org/0009-0001-5545-8700 1 &
- Qingzhu Wang 1
This paper addresses the challenge of large-scale electric vehicle (EV) charging scheduling during peak demand periods, such as holidays or rush hours. The growing EV industry has highlighted the shortcomings of current scheduling plans, which struggle to manage surge large-scale charging demands effectively, thus posing challenges to the EV charging management system. Deep reinforcement learning, known for its effectiveness in solving complex decision-making problems, holds promise for addressing this issue. To this end, we formulate the problem as a Markov decision process (MDP). We propose a deep Q-learning (DQN) based algorithm to improve EV charging service quality as well as minimizing average queueing times for EVs and average idling times for charging devices (CDs). In our proposed methodology, we design two types of states to encompass global scheduling information, and two types of rewards to reflect scheduling performance. Based on this designing, we developed three modules: a fine-grained feature extraction module for effectively extracting state features, an improved noise-based exploration module for thorough exploration of the solution space, and a dueling block for enhancing Q value evaluation. To assess the effectiveness of our proposal, we conduct three case studies within a complex urban scenario featuring 34 charging stations and 899 scheduled EVs. The results of these experiments demonstrate the advantages of our proposal, showcasing its superiority in effectively locating superior solutions compared to current methods in the literature, as well as its efficiency in generating feasible charging scheduling plans for large-scale EVs. The code and data are available by accessing the hyperlink: https://github.com/paperscodeyouneed/A-Noisy-Dueling-Architecture-for-Large-Scale-EV-ChargingScheduling/tree/main/EV%20Charging%20Scheduling .
Explore related subjects
- Artificial Intelligence
Avoid common mistakes on your manuscript.
Introduction
Electric Vehicles (EVs) contribute to numerous benefits such as reduced using cost, environment friendly, and decreased consumption of fossil fuels [ 1 ]. As a result, the amount of EVs has been increasing rapidly [ 2 , 3 ]. However, the lack of convenient charging infrastructure and low effective charging scheduling algorithm for large-scale EVs charging scheduling becomes the key barrier to the EVs penetration [ 4 ]. In some specific scenarios, such as rush hours or extreme weather with low temperatures, there is a noticeable increase in the demand for charging scheduling services [ 4 ]. Uncoordinated charging scheduling strategy could lead to a long queuing time for EVs and traffic congestion [ 5 ]. Therefore, it remains crucial to develop an effective algorithm for managing the large-scale EVs charging schedules while improving the quality of EVs charging service by minimizing the average queuing time of EVs and maximizing charging performance for both EV and charging stations (CSs).
Previous research about EVs charging scheduling has predominantly employed the metaheuristic-based and the Reinforcement Learning (RL)-based methods [ 6 ]. Some of the studies use the metaheuristic-based method to deal with the large-scale real-time scheduling problems. In such scenarios, the genetic algorithm (GA) [ 7 , 8 , 9 ], artificial bee colony (ABC) [ 10 , 11 , 12 ] and particle swarm optimization (PSO) [ 13 , 14 , 15 ] are widely used to search the optimal/suboptimal solution. However, in other studies, the reinforcement learning based algorithms are used to get a better scheduling plan for larger or more complex scenarios. For example, studies in [ 16 , 17 , 19 ] aimed to employ reinforcement learning based algorithm to generate a feasible EV charging scheduling plan to reduce charging costs for users. Similarly, some studies focus on reinforcement learning based algorithms to balance electric load [ 16 , 19 , 20 , 21 ] and increase CSs revenue [ 22 ]. Moreover, some studies proposed the model-based algorithms [ 16 , 23 , 24 ] or multi-agent reinforcement learning (MARL) [ 16 , 21 , 25 ] in different scheduling environments. Generally, metaheuristic-based algorithms can find the optimal/suboptimal solution in real-time, but they need lots of searching time. The aforementioned reinforcement learning based studies primarily generated scheduling plan by using the historical data (such as time series, this needs more steps to update the state/reward information rather get feedback from environment directly) instead of states in real-time. Consequently, these algorithms are difficult to deal with the high real-time EVs charging scheduling problems with high requirement of quick response. To deal with this difficulty, some studies formulated the EVs charging scheduling problem as a sophisticate sequences decision problem or Markov Decision Process (MDP) [ 16 , 21 , 25 ] and the deep reinforcement learning (DRL) is utilized to generate EV charging scheduling plan in the complex situation with multiple of constraints. While, due to the lack of reasonable design of states and exploration mechanism, this type of RL based methods hardly cope with the large state/solution space and cannot find the optimal/suboptimal scheduling solution, which means the large-scale EVs charging scheduling problem may be solved in an inefficient way.
To overcome these gaps, creating an effective deep reinforcement learning-based approach must be undertaken. The states that could represent the global information should be firstly designed and the design of reward should consider the appropriate optimizing target which reflects the service quality of EVs charging scheduling. Furthermore, for obtaining the optimal scheduling plan, DRL-based networks that need to consider the utilization of historical experience of environment interaction and high global search ability should be designed based on the scheduling process. Finally, the training algorithm of the models should pay more attention to scheduling details to avoid being trapped into local optimal solutions.
Despite the importance of providing an effective DRL-based approach to improve service quality (to get lower average queueing time for EVs and lower average idling time for CSs), current research only focuses on historical experience utilization or inefficient solution space explorations. Moreover, existing DRL-based EVs charging scheduling methods only focus on optimizing EV-side or CS-side targets, without providing a comprehensive approach to optimizing service quality from both perspectives. The lack of effective method precedent raises three questions:
What is the state and reward patterns that cover comprehensive information for both EVs and CSs sides?
How to design deep models for solving MDP of EVs charging scheduling efficiently?
How to train the deep models to effectively obtain the optimal EVs charging scheduling solution while avoiding the local optimal and exploring the action space thoroughly?
To solve these questions, on the basis of our pervious works [ 4 ], we proposed an innovative DQN based approach, including a fined-grained state representation that contains the global information, the reward based on an appropriate optimization target for guiding models to improve service qualities and deep neural networks with novel training algorithms. The deep neural networks, combined with the advantages of dueling net [ 26 ] and noisy layer [ 27 ], is designed to not only make effectively use of historical experience, but also improve the ability of space exploring, which makes the proposed approach have the extraordinary performance. Through a series of experiments, we demonstrate the superiority and effectiveness of our proposal.
The remainder of this paper is organized as follows. “Related Works” review the research related to RL/DRL-based EVs charging scheduling. “Problem Formulation” provides the mathematical background of our proposal. Next, MDP formulation of large-scale EVs charging scheduling is introduced. The “DQN based scheduling network and training algorithm” section details the designing of our proposal. “Experiments and Discussion” shows the efficiency and superiority of our algorithm by a series of experiments and analyzes and discusses the effective mechanism of our proposal from the perspective of capability of solution space exploring”. Finally, the “Conclusion” section summarizes our work, provides an outlook for our research.
Related works
With the rapid penetration of EVs in urban transportation systems, there has been a corresponding increase in related research about EVs charging scheduling [ 6 , 28 , 29 ]. Many studies have focused on utilizing DRL-based methods to address EVs charging scheduling problems from different perspectives. In recent research, more attentions were paid to the design of the optimizing target, state, and deep model.
The design of the optimizing target varies across different studies. A well-designed optimizing target can guide the model effectively achieving the optimal goals. In research [ 30 − 32 ], the authors presented the DRL-based algorithms to reduce the energy costs by managing the scheduling process and predicting the price trends. Similarly, authors in [ 19 , 33 , 34 ] developed the DRL based strategies to maximize the revenue or welfare for charging station in energy distributed system. On another hand, some study focuses on optimizing multiple optimizing targets or the weighted sum (of multiple optimizing targets). For instance, a double-Q learning based algorithm in [ 35 ] was designed to optimize the optimizing targets such as satisfying EV charging demand, minimizing charging operation costs, and reducing transformer overloading at same time. Additionally, studies in [ 36 , 37 ] combined waiting time with energy costs as their optimization targets.
The designing of the state usually depends on the optimizing targets [ 38 ]. For example, studies that aimed to meet EVs charging requirements, such as studies [ 37 , 39 ], used the battery state of charge as a part of state. Parameters like charging or discharging costs were usually included in the state representation to optimize the operational costs [ 40 , 41 ]. Other factors, such as the waiting time [ 42 ]and charging price, were also considered when designing state representations to improve the individual satisfaction, decrease energy consumption levels or the expected charging duration. However, there is still a lack of appropriate state representation that incorporates the global information to determine the EVs scheduling priorities and charging station selection in large-scale EVs scheduling scenarios. Based on carefully designed states, suitable actions for minimizing or maximizing the optimizing goal can be generated by the models. Generally, a model used to map the state to an action-value can take the form of a table that consisting of state-action values or a combination of several neural networks.
For simple problems with low-dimensional action and state spaces, such as the problem in study [ 43 ], a Q-Table was commonly employed as a state-action value function to predict the quality of the charging scheduling. However, this method is not widely applicable to real-world problems due to the complexity and randomness [ 44 ]. For more sophisticated situations, the most commonly used algorithms are DQN, Deep Deterministic Policy Gradient (DDPG) and their variants [ 38 ]. In study [ 40 ], the model combining DDPG and DQN was proposed to predict the action-values based on the features of historical data extracted by a long short-term memory (LSTM) and to obtain feasible solutions. Besides, the MARL was used in some studies, such as [ 45 ] and [ 41 ], where it was used to generate EVs charging scheduling strategies by deeply exploring the state and action spaces. The advantages of the multi-agent over the single agent are that it can easily get the local optimum in a large space and is suitable for more complex scenarios such as high dimensions of features or action spaces [ 6 ]. While, effectively obtaining an optimal scheduling plan remains challenging due to the complexity of surging EVs charging scheduling demands. Existing literature pays little attention to this problem.
In summary, the current studies mainly addressed EVs charging scheduling problems with a small state space or low-dimensional action space from an economic or operational perspective. Some of them focused on getting feasible solutions from historical experience, the others devoted to gain the local optimum. Few studies focus on finding the optimal solution in large-scale state an action spaces, especially for scenarios of EVs charging scheduling with surging demands to improve the quality of charging service. Therefore, an effective scheduling method is needed for managing the surging requests in large-scale EVs charging. To solve this problem, a DRL-based algorithm should be proposed with: (i) well-defined state that can represent the global information of both EVs and CSs sides, and reward that could represent the service quality; (ii) deep networks and training algorithms that can solve MDP of EVs charging scheduling and accurately map the state to action values while incorporating an action space exploration mechanism.
Problem formulation
Entity definition.
The EVs charging scheduling problem typically involves three key entities: EVs, CSs and Charging Devices (CDs). The EV and CD can be represented as a quadruple < i , elo i , ltd i , ct i > and a five-tuple < j , k , ot (k, j) , qn kj > respectively, while the CS can be described as a triple < j , clo j , dn j > .
Without loss of generality, we consider the scheduling of EVs with specific charging requirements. Each EV numbered with i , denoted as EV i , is located at position elo i (represents the current position of EV i ) and requires ct i (represents the charging duration of EV i ) minutes for charging. Each charging station numbered with j , denoted as CS j , is equipped with dn j (the number of charging devices on CS j ) available CDs. The scheduling of EVs charging is to assign a charging station CS j located at position clo j (the position of charging station CS j ) for each EV i with the limitation ltd i (the max left travel distance by remaining battery capacity of EV i ).
We represent the k th CD of CS j as CD kj . Additionally, ot (k, j) denotes the working time of CD kj that occupied by the assigned qn kj (the number of EVs that scheduled at CD kj ) EVs.
In this study, we focus on the scheduling of large-scale EVs charging in a city scenario with a complex traffic situation. Both the EVs and the CSs have the capability to communicate with a Global Aggregator which is a third party that interested in EVs charging scheduling. Our approach may help GA to determine the scheduling sequence of EVs for an appropriate CS with the purpose of high charging efficiency in terms of minimized queueing time of EVs and idling time of CDs.
Mathematical background
In general, an EV will submit a charging request when the battery is low, which mainly includes the expected charging time and the remaining travel distance. Specifically, for any given time, the travel time from EV i to CS j , which is denoted as tt ij , is determined by equation tt ij = d (i, j) /(α × v i ) , where the d (i, j) represents the distance between EV i to CS j , the v i represents the average driving speed of EV i and the α ∈ [0, 1] represents a factor that indicates the level of traffic congestion of route between EV i to CS j . The value of α is determined based on the real-time traffic situation and obtained from the data interface of map Apps (Such as Google map or Baidu map). A lower value of α indicates a higher level of congestion, while a higher value suggests a lower congestion level. If EV i is scheduled at CD kj , which is occupied before EV i arrives, the expected queueing time qt ijk can be calculated by qt ijk = (ot (k, j) –tt ij ) × I (ot (k, j) > tt ij ) . Otherwise, if CD kj is not occupied, its idling time it jk can be updated as it jk = (tt ij –ot (k, j) ) × I (ot (k, j) ≤ tt ij ) . I (x) denote the indicator function and can be described as ( 1 ).
Additionally, the occupied time of CD jk can be updated as ( 2 ):
For improving the charging efficiency and quality for both EV and CS sides, the target of charging decision is to minimize the expected queueing time of each EV and the idling time of each CD. To simplify the problem, we transform the EVs charging scheduling problem from a multi-objective optimization to a single-objective optimization. The optimization objective is formulated as ( 3 ), where ω 1 and ω 2 are the hyper-parameters.
Markov decision process of large-scale EVs charging scheduling
The EVs charging scheduling problem can be defined within a discrete MDP considering a discrete time model. Based on the updated state information provided by GA (GA, i.e., global aggregator, is a third party which provides the information of the state for EVs and CSs in real-time; the state of EVs and CSs are denoted as SEV and SCS separately), deep models which include the EV selection model and CS selection model are used as the agent to make decisions regarding which EV should be prioritized for charging and where it should be scheduled. The state, action and reward in our approach are denoted as follows:
STATE : SEV t represents the state used to select which EV is to be scheduled at time step t (the selected EV is denoted as EV s ). It includes the global state information of the CSs and all the not scheduled EVs. Similarly, SCS t refers to the state used to select the optimal CS for EV i at time step t . It includes the information of selected EV and all available CSs.
ACTION : a et represents the action taken at time step t to select an EV that is scheduled. The range of a et is from 0 to N ev , where N ev is the total number of schedulable EVs, indicating the available actions for selecting a schedulable EV. Similarly, a ct represents the action used to select a CS for EV s . The range of a ct is from 0 to N cs , where N cs denotes the total number of available charging stations.
REWARD : In our proposal, we have designed two types of rewards r e and r c . Each reward is based on the weighted values of average queueing time and idling time. The weights are hyperparameters. r e is designed for training the EV selection model. It is a K -steps discounted weighted value with discounted factor γ , where K is a hyper-parameter. This allows to consider the long-term consequences of the selected actions. Additionally, r c is the average queueing time returned from the environment after each step is executed. This reward provides direct feedback on the impact of the chosen charging station for the EVs.
Improved DQN based networks and training algorithms
In the large-scale EV charging scheduling scenario, the high-dimensional action space and state space pose the challenges to find the optima solution effectively when applying the current DRL-based algorithms. To overcome this disadvantage, we carefully designed the state representation and reward setting. Based on this, an improved DQN-based network with feature extracting module is proposed for enhancing EV charging scheduling performance. In addition, for efficiently utilizing the scheduling experience that is obtained from the interaction between the agent and environment, a scheduling-order fine-tunning algorithm is proposed.
Designing of state, action, and reward
Designing of state.
For a schedulable EV, its feature is formed as SE i = [ dis_to_cs(i), ct i , ltd i , scheduled_flag ], which includes the distance to all available charging stations (denoted as dis_to_cs(i) ), the expected charging time (denoted as ct i ), the remaining travel distance of the EV i (denoted as ltd i ) and the scheduling flag (denoted as scheduled_flag ) used to indicate whether the EV i is scheduled.
In addition, feature of CSs consist of AQT , AIT , CNS and occup_T . Therein, the AQT is a list contains the average queueing time of EVs in each CS, AIT denotes a list contains the average idling time of EVs in each CS and the CNS is a list that contains the number of EVs scheduled at each charging station. Therefore, the state of charging station is collectively denoted as SC = [ AQT, AIT, CNS, occup_T ].
With above defined features, the state SEV t used to the EV selection model can be represented as SEV = [ SE 1 , \( \cdot \cdot \cdot \) , SE Nev , SC ] with dimensions = ( N ev + 4) × L , where L represents the dimensions of SE i . For the CS selection that is used to select a charging station for EV s (the selected EV at time step t ), the state SCS is constructed as SCS = [ SE s , SC ] with dimensions = 5 × L .
Designing of action and reward
At each time step t , the models interact with the environment by using the current states SEVt, SCSt to select the optimal EV and CS. The EV selection model operates on schedulable EVs, each output with an associated Q -value representing the expected reward upon selection. Similarly, the CS selection model involves reachable CSs for the chosen EV, each output linked to a Q-value denoting the expected rewards for allocating the CS to EV.
The rewards acquired from the environment rely on both the states and actions taken for optimal EV and CS selection. The reward re for EV selection is a K -step discounted weighted sum of the queueing time about EVi and idling times of the target CDkj , enabling long-term information acquisition. It can be collectively described as \({r}_{et}={\sum }_{m=0}^{K}{\gamma }^{m}\times \left({\omega }_{1}\times {it}_{kj\left(t+m\right)}+{\omega }_{2}\times {qt}_{ikj\left(t+m\right)}\right)\) where itkj ( t + m ) represents the idling time of CDkj due to waiting for the arrival of m th EV that scheduled at CDkj from time step t and qtijk ( t + m ) represents the queueing time of the m th EV that scheduled at CDkj from time step t . Specifically, the discounted factor γ is set as 0.8 and K is set as 2 (as an experience value) enabling long-term information acquisition.
For CS selection, the reward is the weighted sum of the current average idling and average queueing times of the selected EV and their target CS, providing direct scheduling feedback within a single time-step.
Architecture of Neural networks
In this section, the detail of the overall structure of deep models is introduced, which shown as Fig. 1 . For improving the performance of feature extraction from states of EV selection, a fine-grained feature.

The overall structure of our proposal
extraction module (FFEM) is proposed for EV selection model. Based on the extracted fine-grained features, an improved noisy-based exploring module (INEM) is employed to explore the solution space thoroughly. In the end, a dueling module is used to improve the fitting of performance of Q-value.
Fine-grained feature extracting module (FFEM)
In traditional reinforcement learning/deep reinforcement learning based EV charging scheduling methods, states that acquired from the environment are usually featured with low-dimensional or can be easily transformed into a simpler form [ 6 ]. However, for the large-scale EV charging scheduling scenario, states are high-dimensional and based on the total number of EVs and CSs, which cannot be transformed into a simpler form. Moreover, it is difficult to extract features for interacting with the scheduling environment. Therefore, a more effective feature extraction module is proposed.
For solving this problem, a fine-grained feature extracting module (FFEM) is proposed. The FFEM, which used for helping to extract the feature of the input state SEV, contains two same sub-modules. For each sub-module, as shown in the Fig. 2 , a 3 × 3 convolutional layer is used to extract the key information of the input feature map. Given that fact that the feature of the feature map might be sparse in some scheduling scenarios, a batch normalization is therefore adopted to prevent the disappearance of the gradient. Furthermore, the ReLU (rectifier linear unit) is adopted after each batch normalization layer to improve the ability of nonlinear sub-module feature extracting. In each sub-module, two of sub-structure, i.e., Convolutional-Batch Normlized-ReLU combination, is concatenated linearly and a 1 × 1 convolutional layer is adopted which links the input and the output of the sub-module. It implements the cross-channel integration of input and output features, significantly increasing nonlinearity of the sub-model. Given the reality that the complexity of state space and for extracting more refined features of the input states, the proposed FFEM consists of two linearly concatenated sub-modules.
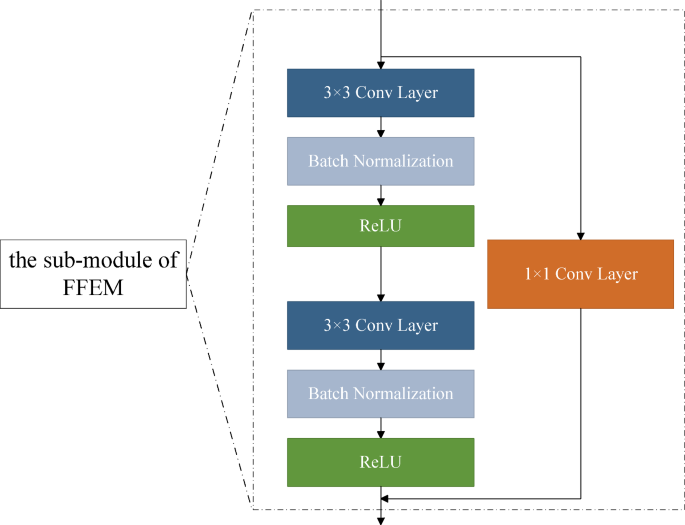
The overall sub-module structure of FFEM
For each sub-module of FFEM, let X denotes the input state, W 1 , B 1 , W 2 , B 2 denotes the weights and biases of the convolutional layers and W 3 , B 3 denotes the weight and bias of the 1 × 1 convolutional layer. Each sub-module of fine-grained feature extraction module can be collectively summarized as ( 4 ) and ( 5 ):
where σ 1 and σ 2 are the standard deviation of the X and Y , μ 1 and μ 2 are the mean of the X and Y , ε 1 and ε 2 are the hyper-parameters that bigger than 0, β 1 , β 2 , γ 1 , γ 2 are the learnable parameters.
It can be concluded from ( 4 ) and ( 5 ) that the proposed module introduces more weights and biases parameters. By combining with the ReLU function, this structure help improves the ability for the complex non-linearized Q-value function fitting and feature extraction in larger state space. Therein, the ReLU activation function is defined as f ( x ) = max ( 0, x ), introducing nonlinearity into the model. By allowing only positive values to pass through and setting negative values to zero, ReLU facilitates the learning of complex nonlinear relationships in the data. Furthermore, its sparse activation property promotes feature extraction by selecting relevant features from the input. When integrated into the proposed module, ReLU enhances the model's capability to capture complex patterns in the Q-value function and extract meaningful features, particularly in larger state spaces. The introduction of the proposed module, through the incorporation of additional weights and biases parameters along with the utilization of the ReLU activation function, significantly improves the model's performance in fitting complex nonlinear Q-value functions and extracting features. This approach proves particularly advantageous in scenarios involving larger state spaces, where traditional models may struggle to adequately capture the underlying relationships. Future research could explore further improvements and applications of this framework in various reinforcement learning tasks.
Improved noisy-based exploring module (INEM)
In our research, the selection of an appropriate exploration strategy within the solution space is crucial for identifying the optimal electric vehicle charging scheduling scheme. Generally, traditional strategies such as ε -greedy and Boltzmann probability-based methods are two primary approaches for exploring the solution space. However, when dealing with large-scale, high-dimensional solution spaces, these methods exhibit inefficiencies in the exploration and the balancing between exploration and exploitation [ 27 ]. To address this issue, we proposed an improved noisy-based exploring module (INEM) that integrates the dropout and noisy linear layer. The aim is to augment the model's exploration capabilities.
The Fig. 3 illustrates the structure of INEM, which comprising three key neural network layers. Initially, the Dropout layer processes the state features extracted by the FFEM module. During training stage, it stochastically deactive a portion of neurons with a probability of p = 0.5 to inhibit their forward and backward propagation. Following the dropout processing of features, they are fed into two layers of noisy layers designed to incorporate random noise subject to normal distribution, enhancing the model’s generalization capabilities. Each noisy linear layer is adopted the ReLU function for non-linearity, thereby augmenting the module's capacity.
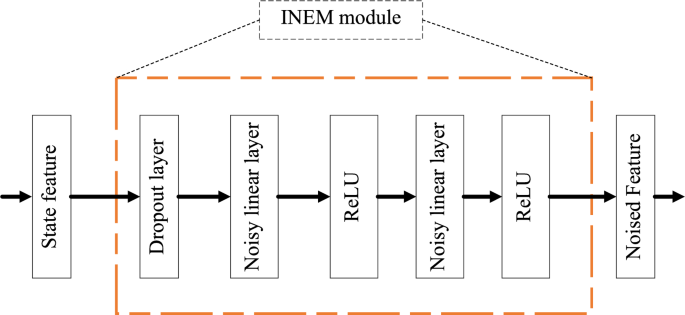
The structure of INEM
Specifically, for the Dropout layer, during each forward propagation, a portion of neurons is randomly deactivated with a probability of p = 0.5, discarding their original computed results and setting their outputs as 0. Furthermore, concerning these deactivated neurons, their parameter updates are halted during backpropagation. This allows effectively training a sub-model of the original model in each training iteration. This strategy helps reduce reliance between specific neurons, thereby enhancing the model's generalization and mitigating overfitting risks. Mathematically, this layer can be represented as Y = X ⨂ m , where ⨂ denotes element-wise multiplication of matrices, and m follows a Bernoulli distribution ( p = 0.5).
For the noisy linear layers within the module, the generation of parameterized noise adopts the factorized gaussian noise method proposed in [ 27 ]. Based on this method, the representation of the noisy layer can be summarized as Y n = ( μ ω + σ ω ⨂ ε ω ) × X n + ( μ b + σ b ⨂ ε b ), where the X n and Y n denotes the input and output of the layer, and the μ ε , μ b , σ ε , σ b are the parameter matrices of the noisy linear layer and each value in the matrices is subjected to normal distribution. The introduction of noisy layers aims to enhance the model's robustness and generalization capabilities. By introducing Gaussian noise at each layer, the model can better adapt to different data distributions and noise conditions, thereby improving its generalization and solution space exploration ability. Additionally, noisy layers help prevent overfitting, thereby enhancing the model’s performance in practical applications.
Combining the dropout skill, the noisy linear layer described in this section, and the Fig. 3 , our proposed module can be mathematically summarized as the form shown in Eq. ( 6 ).
where f in represents the input to the model, \(\mu^{{\omega_{i} }} ,\mu^{{b_{i} }} ,\sigma^{{\omega_{i} }} ,\sigma^{{b_{i} }}\) are the hyper-parameters of the ith noisy linear layer, and \(\varepsilon^{{\omega_{{\text{i}}} }} ,\varepsilon^{{b_{{\text{i}}} }}\) signify the noise introduced by the Noise layer’s weights and biases, respectively.
This module's design yields significant advantages for the model in various aspects. Primarily, it contributes to enhancing the overall training speed, crucial for efficiently handling large-scale tasks. Secondly, the introduction of the Noise layer amplifies the collective adaptability among neural nodes, notably enhancing the model's robustness and performance when dealing with intricate datasets. Additionally, the incorporation of the Noise layer aids in balancing the model's exploration and exploitation, thereby reinforcing its generalization capabilities. These combined factors propel the model towards better adaptation to the challenges posed by large-scale electric vehicle charging scheduling tasks.
Dueling block for a better Q-value fitting
When addressing problems involving large-scale action spaces and high-dimensional state spaces, directly mapping from states to different actions through state-action values (i.e., Q-values) typically demands increased computational resources and struggles to accurately reflect the value information of diverse states. To mitigate this issue, we devised a Q-value estimation module based on Noised Features, employing the structure of a Dueling Network to tackle these challenges.
The Fig. 4 illustrates this module, which takes processed features from the Noisy Linear Layer as input and ultimately produces Q-values for actions. In contrast to other networks (such as the Q-network in DQN), the dueling module employs two parallel sub-network branches that separately estimate the advantage value of state-action pairs and the value of the current state. Here, the advantage value refers to the relative merit of an action concerning the state value, representing the relative value of a particular action in a specific state. To merge the outputs of these two branches into the actual Q-values, an Aggregation Layer is employed to integrate the outputs of these sub-networks.
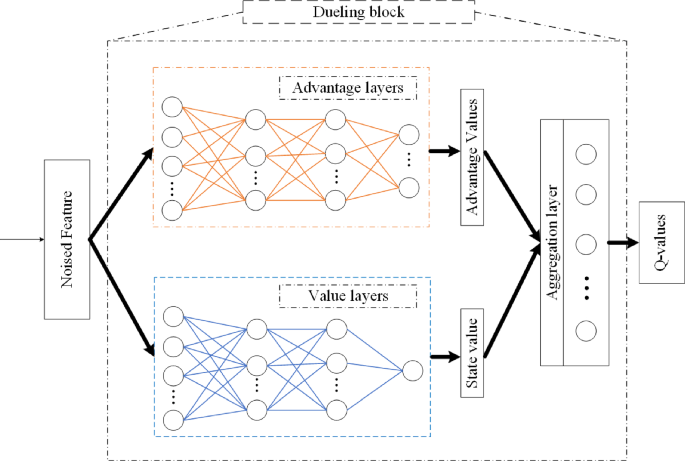
The dueling module
Let the noised feature as shown in the Fig. 4 be denoted as S noised , the v η and a ψ represent the state value predictor branch and advantage value predictor branch respectively. Therein, η and ψ represents the parameters of the v η and a ψ branches. In this context, a signifies the action chosen in the current state,
while a′ represents the action chosen according to the current action-selection policy in the subsequent state s′ . Hence, the mathematical formalization of this module can be articulated as follows:
where θ = { η, ψ }.
In the proposed module, the s noised is separately fed into the v η and a ψ branches, thereby decomposing the estimation of Q-values into estimations of the advantage value A and the state value V . Subsequently, the aggregation layer integrating outputs derived from both branches into the Q-value. The design uniquely determines the action advantage values and state values [ 26 ]. This structure aids in optimizing the model's training stage, enhancing both the speed and precision of state value estimation, thus improving the capability for policy evaluation. Simultaneously, this decomposition stabilizes the model's exploration of solution spaces, enhancing its ability to search for the optimal solution to electric vehicle charging scheduling problems within large-scale solution spaces.
Training algorithm
For training the proposed architecture effectively, an assist algorithm which are used to scheduling order fine-tunning is proposed. Based on this algorithm, the models are trained by the sampled experiences.
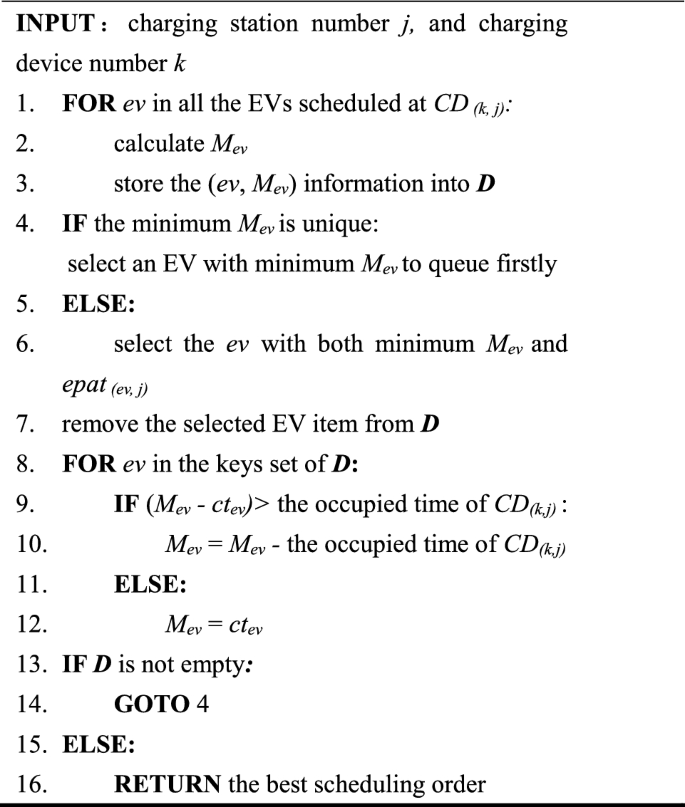
Charging order fine-tunning
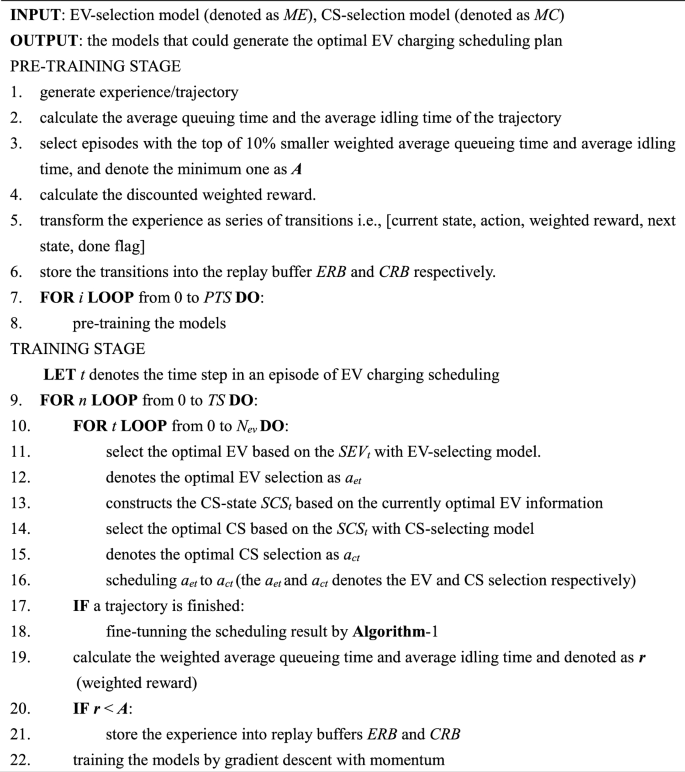
EV charging scheduling models training algorithm
In a large-scale action space, it is difficult to directly identify a scheduling scheme with relatively good performance at the beginning of the training stage. This can lead to a relatively poor quality of experience gathered by the model while interacting with the environment (longer EV queueing times, longer charging equipment idling times). As a result, models trained based on the experience typically perform poorly on EV charging scheduling. To minimize this deficiency, this paper proposes a charging sequence fine-tunning algorithm, which is outlined as Algorithm 1 . In this algorithm, the epat(ev, j) denotes the predicted driving time to the target charging station j of EV ev in current state . And the ct ev denotes the charging time for EV ev .
The main idea of the proposed algorithm is derived from SJF (shortest job first), i.e., to have the shortest process time of the scheduling process is to schedule the not scheduled job/EVs with shortest processing time firstly. However, the situation becomes more complex in the EV charging scheduling process, i.e., both the traveling time and charging time could be the key point for the scheduling result.
To solve this problem, two of skills is introduced. Firstly, calculated the expected occupied time of each EV need to be scheduled at CD (k, j) , which could be described as the sum of the charging time and the expected traveling time (the 1–3 lines of Algorithm 1 ). Based on the calculating results, the optimal EV selection can be simply defined as the EV with minimum summation (i.e., M ev ) (the 4–5 lines of Algorithm 1 ). However, the 6–7 lines of Algorithm 1 shows a special situation. For situations that more than one EVs has the same M ev -value, select the EV that with minimum epat (ev, j) can derive the better plan. This can be simply proved as follows.
Considering the situation where two EVs (denoted as A and B ) need to be scheduled at the charging station CD (j, k) , let C A and C B denote the charging time of A and B , and R A and R B denote the expected travel time to the charging station. If C A > C B , R A < R B , and C A + C B = R A + R B . If A is firstly selected, then the total finish time of the charging process can be calculated as follows (Eq. 8 ):
otherwise, the total finish time is calculated as ( 9 ):
It can be derived from ( 8 ) and ( 9 ) that,
Based on the assumption, it can be derived that L—F < 0. Therefore, to select an EV which is closer to the charging station could lead to a better scheduling performance when the M -value of EVs is equal.
The 9–13 lines gives a scheme to update the M -value of EVs, which ensures that the re-arranged scheduling order could lead to a maximum performance on both EV (minimized queueing time) and CS (minimized idling time).
In our model training algorithm, the training process can be divided into two-main part i.e., the pre-training stage and training stage. In this algorithm, two experience storage, i.e., the prioritized replay buffer (PER) [ 35 ], is denoted as ERB and CRB for storing the experience that used to training the EV-selecting model and CS-selecting model respectively. Therein, the PER is used for enhancing the utilization of collected experiences. Based on the experience, the models are pre-trained for PTS time trained for TS times where both the PTS and TS are scalars. The training process is summarized as Algorithm-2.
In Algorithm 2 , it can be divided into two main parts, the pre-training stage and training stage. The advantages of this training setting can be summarized as follows: given the fact that the problem setting has the large-scale and large-dimensional action space and state space, a pre-training process is introduced to accelerating the convergence of models. This could scale the search space back and exclude some solutions with relatively worse performance to alleviate some of the unnecessary exploration in the subsequent training stage.
At the pre-training stage (i.e., the line 1–8), the algorithm collected experiences by Monte Carlo with a sampling process. A sampling mechanism is introduced to re-sampling the original sampled experiences and only 30% of experiences with better scheduling performance is keep. This allows that the pre-training process could lead to a faster performance enhancing in EV charging scheduling task.
The training stage (i.e., the line 9–22) has two main processes, the interacting and training. In interacting process, the models interact with the environment to explore the solution space (i.e., the line 10–16). To prevent introducing the worse experience into replay buffer, the scheduling order fine-tunning is introduced (i.e., the line 17–18). In line 20–21, each trajectory is re-evaluated after fine-tunning. If the re-evaluated experience is better than in the replay buffer, this experience will be added to the experience buffer. This is beneficial for improving the overall quality of the training model. This can be simply explained as follows:
Assuming that N A trajectories are added into the replay buffer with the final weighted reward A , and a new experience is sampled with the final weighted reward r . If the new experiences are added into the replay buffer, the change of A (denoted as B ) follows:
It follows that:
if and only if r < A . It shows that, this experience collection method helps to improve the quality of experience used for training models, which is beneficial for accelerating model training.
Experiments and discussion
To demonstrate the superiority of our proposal, we have designed the case studies to showcase its performance. The experiments in each case study were conducted using PyCharm Integrated Development Environment version 2019.1.1 with Python 3.7 on a PC equipped with an 11th Gen Intel(R) Core (TM) i7-11800H processor running at 2.30 GHz, 32 GB of memory, and an Nvidia RTX 3060 graphics card with 6 GB of memory.
Yardstick and dataset
In our experiments, we collected a real-world dataset from an EV charging service company inter-face. The dataset consists of 1000 EVs and 34 charging stations (CSs) of a city. Each CS is equipped with 5 high voltage charging devices. The 1000 EVs are located at different positions and need charging services. During our research, we identified that 101 EVs were unable to be scheduled for charging due to poor battery conditions. These EVs required battery exchanging services. Consequently, only 899 EVs were able to reach at least one charging station with their remaining battery capacity. The details of the dataset can be summarized as Table 1 .
Experiment setting
In our experiment setting, three sets of case studies were conducted to showcase the performance of our model from different perspectives.
In case study I, we investigate the influences of different reward setting, hyperparameters setting on the performance of models in EV charging scheduling. In this part, a group of optimal hyper-parameter setting is identified. The experiments discussed after this section are all conducted based on the optimal parameters shows in this part.
In case study II, we investigated the effectiveness of each proposed module (fine-grained features extraction module, Improved noisy-based exploring module and dueling module) and scheduling order fine-tunning algorithm. A series ablation experiments, and analysis are conducted/proposed based on the results of experiments.
In case study III, some heuristic-based, DRL-based, and dispatching rule-based EV charging scheduling algorithms are compared. The results showcase the advantages of our proposal over other algorithms.
Case study I
In this section, our primary focus lies in presenting and discussing the influence of different reward settings, neural network optimizers and hyperparameters, such as learning rates, on the exploration of the solution space. To investigate this topic comprehensively, we examine various reward combinations (with learning rate = 0.0001and optimizer Adagrad for EV-model, Adam for CS-model), as depicted in Table 2 . Based on these reward settings, a series of controlled experiments are conducted (therein, we sampled experience by Monte Carlo to pre-training the models), and the experiment results are illustrated in Fig. 5 . Furthermore, the effects of different hyper-parameter (mainly about the learning rate) on the performance of the models are then discussed based on the optimal reward setting.
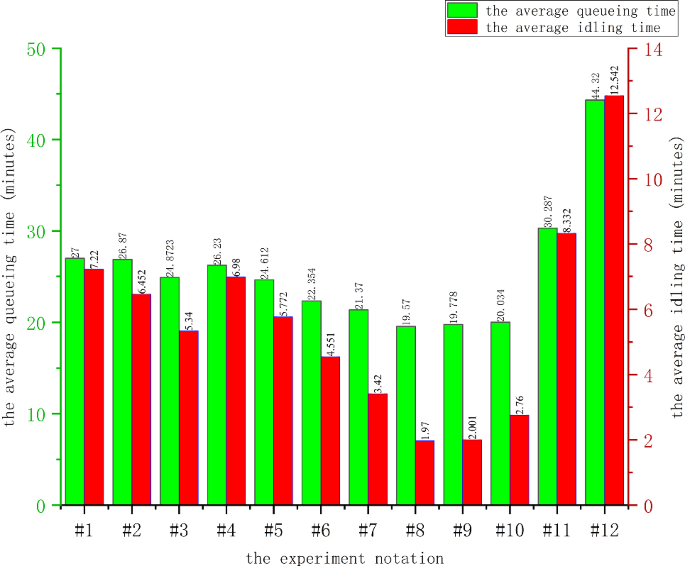
The result of compare experiments
As shown in the Table 2 and Fig. 5 , the result #1—#8 indicates that the bigger weight for the queueing time can lead to a better scheduling performance to some extent. For some extremely situation (i.e., one of the factors (queueing time or idling time) is neglect, such as the #11—#12 version of the control experiment), however, the performance has a downward trend. Therefore, it can be concluded from the Fig. 5 that the performance for EV charging scheduling cannot be improved by simply increasing the weight of the queueing time. Given the fact that the idling time increasing far slower than the queueing time, it can be therefore concluded that it is easier to find the optimal EV charging scheduling solution by considering the target that changes more frequently (such as the queuing time) in this type of problem.
Learning rate and optimizer are of the most important elements in the models training. However, given the complexity of the problem (large solution space) that it is hard to find an appropriate learning rate or an optimizer in large-scale searching space (i.e., solution space in our proposal). Therefore, we conduct a series of experiments to investigate the influence of optimizer selecting and learning rate setting on scheduling results.
In these experiments, we adopt different optimizers and learning rate for training EV-selection model and CS-selection model for comparison. The mainly tested optimizers are Adagrad, Adam, SGD, and the learning rate we adopt are 0.1, 0.01, 0.05, 0.001, 0.005, 0.0001. For simplifying the criterion of scheduling results, we adopt the weighted sum of the average queueing time and weighted idling time (with ω 1 = 0.8, ω 2 = 0.2). The result of experiments is shown as Table 3 .
It can be concluded from the Table 3 that the combination of Adagrad for EV-selecting model training and Adam for CS-selecting model training has the best performance among the scheduling result. It can be inferred that the features of Adagrad (suitable for sparse data and able to automatically adjust the learning rate) is suitable for the solution space of EV charging scheduling with high dimensionality. Therefore, there exists the sparsity in the solution space of EV charging scheduling problem to some extent can be then inferred (i.e., the solution space of EV selecting). This might be contributed to the scheduling constraints that not all the EV could be scheduled in the current state. Given the complexity of solution space, the learning rate adjusts automatically lead to a more convenient paradigm to training the model without introducing the additional hand labor. On the other hand, it could lead to best results by adopt the Adam for CS-model training. This might be since the high-efficient in gradient calculating which lead to a better performance in EV charging scheduling. Therefore, based on the result shown in the Table 3 , all the following comparison experiments are conducted in the following manners:
The optimizers are set as Adam for CS-selecting model with learning rate = 0.0001 and Adagrad for EV-selecting model with learning rate = 0.0001.
The reward for EV selecting is set as the discounted weighted reward with ω 1 = 0.8 , ω 2 = 0.2 and the discounted factor is set as 0.7 (the 0.7 is experienced value).
Case study II
This part, we conducted a series of ablation/compare experiments to investigate the advantages of our proposal. Firstly, we study the feature extract module to show that it is beneficial for enhancing the features extraction for state representing in the large-scale state space. Then, the advantage for noisy block in exploring solution space is analyzed by comparing the results with exploring by Boltzmann probability and ε -greedy. After that, the merits for advantage-value module are further analyzed by the ablation experiments.
For investigating the advantages of the feature extract module, a group of ablation experiments are conducted. In the compared module, the 1 × 1 conv shortcut, batch normalize are erased. As the results shown as the Figs. 6 and 7 , the overall trend from the experimental results suggests that adding a feature extraction module leads to a better large-scale EV scheduling result. On the one hand, the average queuing time corresponding to the overall scheduling result has a decreasing trend compared to the control group as shown in Fig. 7 , which suggests that the enhanced extraction of features helps to improve the ability of the model in generating scheduling plan. On the other hand, it can be concluded from the Fig. 6 that the average idling time corresponding to the scheduling results generated in the model training stage has a greater than its control group in some time steps. This is since the refined feature extraction layer helps to some extent in the exploration of the solution space by other subsequent modules (e.g., the noise module in the EV selecting model) (the oscillations in the idle time indicate that the queuing order is constantly changing during this stage, which suggests that the model is exploring the solution space thoroughly).
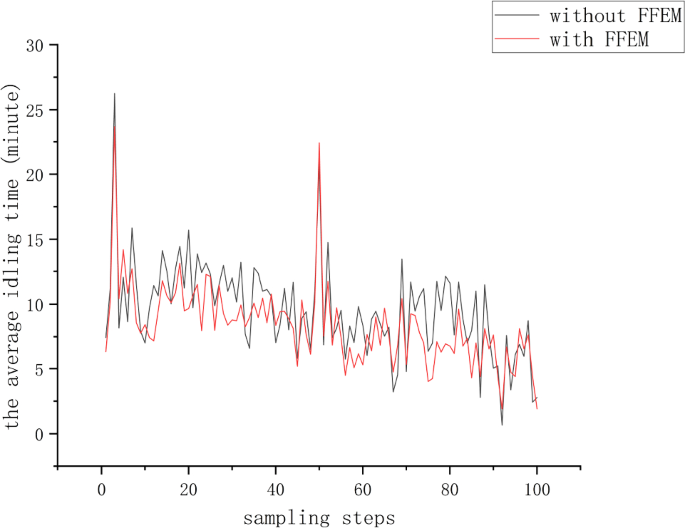
The result of average idling time
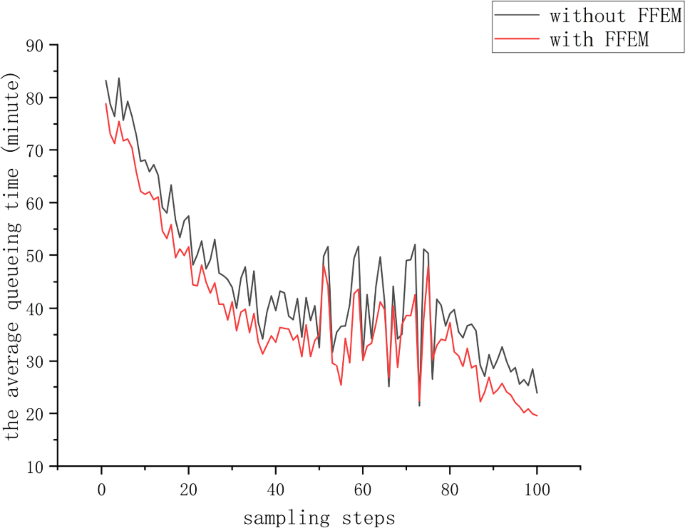
The result of average queueing time
Based on the features extracted by the proposed feature extraction module, we further investigate the important role played by the noise layer in our proposed network architecture for the exploration of the solution spaces. For clarifying the comparison, we use the weighted sum of the average idling time and the average queueing time with weight ( ω 1 = 0.8 , ω 2 = 0.2) as the indicator to measure the scheduling performance.
To investigate the effectiveness of the INEM, the ablation experiment is conducted. In the ablation control experiment, we uniformly sampled 50 samples at equal intervals during both the pre-training and training stages in the control group and our proposed model. The horizontal axis represents sample steps, where 0–50 denotes the pre-training stage, and 51–100 represents the training stage. The vertical axis represents the weighted criterion, i.e., the weighted sum of the average queue time of electric vehicles and the average idle time of CS after each scheduling, with weights set as ω 1 = 0.8 and ω 2 = 0.2. As shown in Fig. 8 , during the pre-training stage (steps 0–50), our proposal and the version without INEM exhibit similar convergence trends. However, our model shows superior performance compared to the control group. This might attribute to the fact that the model with the IENM module has more parameters, a relatively complex structure, i.e., higher model fitting capacity, resulting in a better fit to the pre-training data. Furthermore, in the training stage (steps 51–100), our proposal demonstrates significant fluctuation in the optimal weighted criterion sampled at different time points compared to the control group. This fluctuation stabilizes after a certain period. This phenomenon is attributed to the Noisy Linear Layer embedded in the INEM module, which introduces learnable noise following a normal distribution. In the early stages of model training, these noise in Noise Linear Layer guides the model to explore the solution space thoroughly. By leveraging the scheduling order fine-tuning algorithm, the model can explore strategies with better scheduling results. The trends in the curve from step 70 to step 100 indicate that the model acquires the capability to generate high-quality scheduling strategies. Moreover, the change in the weighted criterion becomes gradually smoother, possibly because the model adapts to the impact of learnable noise and could effectively removing non-critical information from the state features for generating optimal scheduling strategies. These crucial factors contribute to the superiority of INEM over the control group.
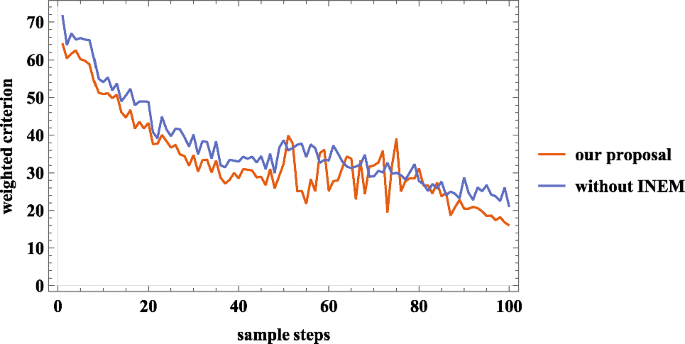
The results of the ablation for INEM
We compare the proposed improved noisy-based exploring module with the ε -greedy and Boltzmann probability, where the ε -greedy based algorithm is to select an action with the optimal Q-value by a probability ε , and select the actions randomly by a probability 1– ε . In the Boltzmann probability, the selection probability of each action is proportional to its Q-value, The Boltzmann probability of an action is shown as \(pt(a|st) = e^{Q(st,a)} /\sum\nolimits_{a} {e^{Q(st,a)} }\) where the p t (a |s t ) denotes the selection probability of action a in the state s t and the e denotes the Euler’s number (which equals to 2.71828).
The Fig. 9 shows the results of the comparison for different exploration mechanism ( ε -greedy, Boltzmann probability and our proposal). It can be inferred that the epsilon-greedy based exploration mechanism is less capable of exploring on larger scale action spaces than the other two approaches. This may be because this method only considers a simple trade-off between "utilization" and "exploration", and the actual environmental information is not considered in the exploration stage. As can be seen in the green part of the figure, in a large-scale solution space, the Boltzmann probability-based exploration mechanism tends to explore more solutions in the space (i.e., it is able to search a much larger solution space than the epsilon-greedy-based method). This is reflected in the larger fluctuations in the reward values obtained from the environment during the search stage. However, this property might introduce instability to problems with large solution spaces. Unlike the first two classes of traditional methods, our proposed module has a gentler character in the exploration stage from an overall perspective. Additionally, the final exploration results are better compared to the two types of traditional schemes. This may be since the model directly adds noise to the states during the exploration of the solution space, which on the one hand introduces randomness, and on the other hand the model adapts to the effects brought by the noise as it approaches convergence. This indirectly leads to a strong adaptive ability of the model, which makes the model perform relatively well in solving problems concerning large-scale action space exploration.
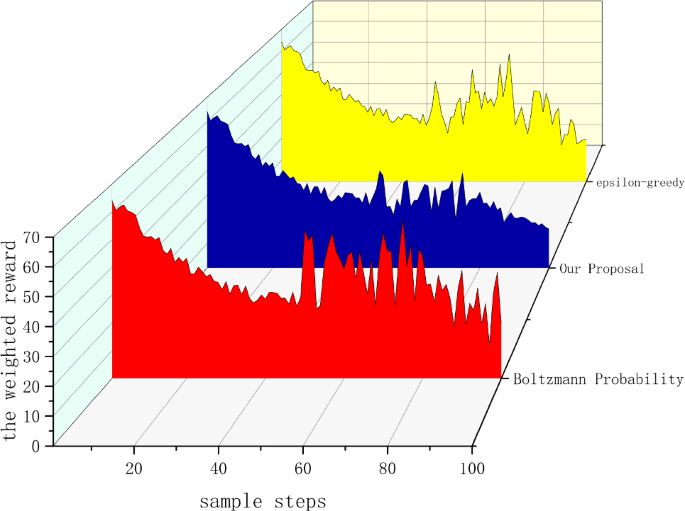
The result of weighted reward comparison
Dueling block
For exploring the advantages of the proposed advantage-value module, a group of comparison experiments is conducted. In this experiment, the dueling block is replaced by the fully connected layers.
The structure of the compared model has similarities compare to the original however, the part of value-module is eliminated. Therefore, it can be viewed as a DQN-shaped structure. The experiment result is collectively shown as the Table 4 .
It can be concluded that our proposed structure performs better than the conventional DQN for large-scale EV charging scheduling. This may be due to the decoupling of Q- values by the dueling structure. This makes the advantage value, which really reflects the importance of the action, separated from the original Q-value, as well as the network is used to predict the state value more specialized, which also enhances the ability to fit the actual state value function at same time.
To validate the effect of the Dueling Block on improving the accuracy of Q-value evaluation, we conducted further ablation experiments, with results presented in rows 1 and 3 of Table 5 . The experimental findings indicate that removing the Dueling module resulted in a 16% increase in the weighted reward corresponding to the scheduling policies generated by the model compared to the control group. This suggests that simply using the output of the noise layer as Q -values cannot meet the accuracy requirements for predicting state-action values. On the other hand, experimentally, both the Dueling Block and the CNN module were able to reasonably predict Q-values and maintain their results within appropriate ranges. However, there was variation in prediction accuracy, with a difference of approximately 1.8% observed in the evaluation metrics corresponding to the optimal scheduling strategy for large-scale electric vehicle charging. This indicates that in larger-scale electric vehicle charging scheduling scenarios, our proposed architecture demonstrates a more pronounced advantage, generating strategies more suitable for large-scale electric vehicle charging scheduling.
Case study III
In this case study, we demonstrate the advantages of our proposal over other EV charging scheduling algorithms in our scheduling scenarios and investigate the mechanism of solution space exploration. To achieve this, we surveyed six categories (consisting of fourteen kinds) of algorithms. The aim was to assess their effectiveness in generating feasible EV charging scheduling plans. We conducted rigorous experiments on all these algorithms within our carefully designed EV charging scheduling environment. The summarized results of all comparison experiments can be found in Table 5 .
From Table 5 , it is evident that experiments 1 and 2 yield poorer scheduling performance compared to the others. This suggests that these two algorithms result in a gathering of EVs at the charging station, leading to longer average queuing times. Different from our proposal, solely considering the user or the charging service provider for charging scheduling may overlook global information, resulting in subpar performance of the scheduling strategy generated. Among experiments 3, 4, and 5, there is a notable improvement in EV charging scheduling results compared to experiments 1 and 2. This indicates that the GA-based algorithm effectively addresses the problem. However, searching through the vast solution space with GA may lead to local optima or slow search speeds. The EDA-based algorithm (lines 6 and 7 in Table 5 ) demonstrates better results in solution space exploration. Nevertheless, increasing the number of iterations does not necessarily yield better results for large-scale EV charging scheduling (as observed in experiments 6 and 7, where more iterations led to worse scheduling results). This suggests instability in the EDA-GA based algorithm when tackling such problems. Despite the potential for better solutions with more iterations, the long runtime for solution space exploration is impractical for real-time applications. We further tested the EDA-GA based algorithm with more than 3000 iterations, such as 3500 and 5000. Although some experiments showed improved results, the extended runtime remains a barrier for practical application.
Clearly, the DRL/RL based algorithm proves to be more effective than other algorithms. In the realm of electric vehicle (EV) charging scheduling, deep reinforcement learning (DRL) methods exhibit significant advantages over genetic algorithms (GAs), dispatching rules, and other conventional methods. Firstly, DRL autonomously explores optimal charging strategies through continual trial and error learning, effectively adapting to intricate and ever-changing environments and requirements. In contrast, GAs necessitates evaluating numerous individuals in each generation and rely on randomness to explore solution spaces, potentially resulting in inefficient exploration particularly in high-dimensional and complex problem domains. Dispatching rules, often based on static rules or heuristic methods, lack the flexibility to adjust to real-time changes. Secondly, DRL methods dynamically adjust strategies based on environmental feedback, facilitating adaptation to fluctuating charging demands and grid conditions, thereby enhancing charging efficiency and grid utilization. This dynamic adaptability is lacking in traditional approaches. Consequently, RL offers superior adaptability and efficiency in EV charging scheduling, better aligning with practical application requirements.
For instance, when comparing scheduling outcomes in similar scenarios, such as our observations from experiments 11 and 6, we found that scheduling strategies based on reinforcement learning methods achieve computational speeds approximately 47 times faster than other methods when addressing the large-scale electric vehicle charging scheduling problem. This finding isn't merely reflective of a single experimental result but has been validated through multiple repeated experiments. Moreover, our approach notably outperforms other prominent reinforcement learning-based scheduling algorithms, especially those based on Deep Q-Networks (DQN) and Deep Deterministic Policy Gradients (DDPG). The superiority of our approach can be attributed to several key factors. Firstly, our model incorporates a refined feature extraction module, significantly enhancing the model's capability to extract state features. By fully leveraging this refined state feature extraction module, coupled with pre-training and constrained solution space exploration, our method efficiently explores the solution space while mitigating the issue of excessive randomization. Secondly, we judiciously decompose the actual Q-values, enabling the model to understand the value of each state more accurately, thereby making more effective decisions when exploring the solution space. Such design choices result in our model performing commendably in addressing the large-scale electric vehicle charging scheduling problem. It's worth noting that compared to other reinforcement learning methods, such as Advantage Actor-Critic (A2C) based on policy gradient methods, our proposed approach still holds a slight advantage. This observation underscores the practicality and efficiency of our method over A2C in the field of electric vehicle scheduling. This is because our proposed model architecture bears similarity to the A2C method in predicting Q-values, whereby rational decomposition of Q -values enhances model stability and prediction accuracy. However, in addition, effective exploration mechanisms and a sound understanding of state features within the vast state space are more advantageous for the model to attain optimal solutions compared to traditional Actor-Critic frameworks.
In our study, we conducted experiments comparing the use of Multi-Agent Reinforcement Learning (MARL) and Single-Agent Reinforcement Learning methods for addressing the large-scale electric vehicle (EV) charging scheduling problem. Surprisingly, the experimental results indicated that the MARL-based approach did not exhibit the anticipated superiority in solving this problem and, in fact, performed worse compared to the Single-Agent Reinforcement Learning method. This outcome may stem from the inherent complexity of the problem itself and the associated technical challenges. Firstly, the involvement of numerous electric vehicles in large-scale EV systems led to a proportional increase in the number of agents within the MARL framework. As the number of agents increased, the complexity of interactions within the system grew exponentially. Seeking the optimal scheduling strategy inevitably introduced additional interaction parameters among the agents. However, these supplementary interaction parameters often did not directly correlate with the essence of the problem but rather augmented the computational complexity and uncertainty of the system. Furthermore, the collaboration and competition dynamics inherent in multi-agent systems may have contributed to the performance degradation. When addressing the EV charging scheduling problem, individual agents may engage in competition due to conflicting interests, thereby resulting in instability, and decreased overall system performance. In contrast, our proposal offered a more straightforward approach, enabling more effective exploration of the optimal solution within the solution space and avoiding the complexities of interactions and competition present in multi-agent systems.
Additionally, given the widespread application of Long Short-Term Memory (LSTM) in decision problems involving time series data, we conducted a comparative analysis of our proposal against several LSTM-based decision models (Experiment 12 and Experiment 13). In the control experiments, considering the characteristics of LSTM and the electric vehicle charging scheduling problem, we utilized these models for decision-making regarding the sequence of electric vehicle scheduling, while maintaining the original charging station selection network unchanged. The experimental results, as presented in the "Other" column of Table 5 , indicate that Algorithms 12 and 13 achieved results like our proposal. However, models based on the INEM module exhibited a more thorough exploration of the solution space, particularly in generating optimal strategies for EV charging schedules. This might be attributed to the fact that, in the context of EV charging scheduling problems, the design of states incorporates current global information. On the other hand, LSTM-based models, in the process of addressing sequential decision-making problems, appropriately utilize memory gates to retain information from the previous state. Due to this, the combination of this information with the current state might adversely impact the accuracy of the Q-value prediction module, potentially hindering the overall accuracy of Q-value predictions. From an experimental standpoint, it is observed that algorithm 12 and 13 exhibit slightly longer inference speeds compared to our proposal. Consequently, our proposal demonstrates a superior advantage in exploring the solution space.
Based on the three proposed modules, we further investigate the mechanism of action space exploration. As illustrated in Fig. 10 , the training process of our proposed model can be divided into two parts: the pre-training stage and the training stage with exploration. During the pre-training stage, the EV charging service quality, represented as the weighted sum of the average queueing time of EVs and the average idling time of charging stations, shows a gradual improvement for the solutions (depicted as blue dots). This improvement suggests that the model's performance in electric vehicle scheduling tasks has been enhanced through pre-training. The solutions tend to concentrate within the solution space and gradually extend towards the optimal solution (represented by the blue dot). This observation indicates that training the model using pretraining samples obtained from better-performing trajectories can expedite the model's training progress in the initial stage. However, as depicted in the figure, it can be concluded that solely conducting pre-training may result in a limited exploration of the solution space. During the model's training and exploration stages (represented by yellow dots), the positions of these solutions obtained through our model exhibit relatively more dispersion. This dispersion signifies that sufficient exploration of the solution space has taken place during the model training process. In summary, the pre-training stage contributes to the initial performance improvement of the model in EV scheduling tasks. While pre-training accelerates the training speed and leads to concentrated solutions, further training and exploration stages are essential for achieving a more diverse and comprehensive exploration of the solution space. This two-stage training approach and our proposed architecture/modules ensure thorough exploration of solutions and enhance the algorithm’s capability to find optimal solutions for EV scheduling.
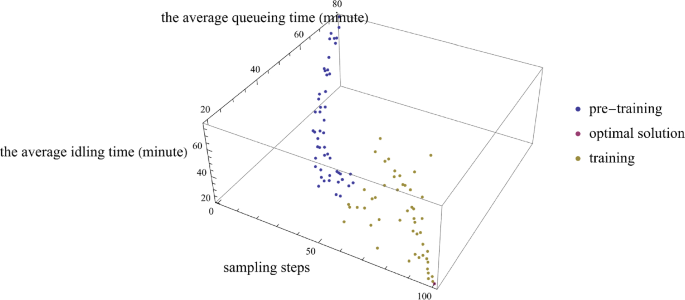
The pattern for exploration
The advantage of our model lies in the appropriate combination of the proposed three modules i.e., the fine-grained features extraction module (FFEM), the Improved noisy-based exploring module (INEM) and the dueling block. The model utilizes the fine-grained features extraction module and the dueling block to efficiently extract state features to fit the empirical data to roughly determine the approximate location of the subspace where the optimal solution is located. Based on this, the model introduces constrained noise through the improved noisy-based exploring module in the training stage to help the model explore the subspace adequately. In this process, our proposed an effective empirical filtering strategy (refer to the line 20–21 in Algorithm 2 ) which ensures that the model can explore the solution space and identifies the location of the optimal solution by iteratively. The reasonable combination of the three main modules in the training process can enable the model to efficiently obtain the global optimal solution in a large-scale solution space (This phenomenon can be well verified in Fig. 10 ). Unlike our proposal, the swarm intelligence algorithm can only continue to explore those subspaces that have already been explored. This model has some drawbacks in the search of global optimal solutions, i.e., the swarm intelligence algorithm can only generate new solutions based on the existing solutions through basic operations (e.g., crossover or mutation operation in genetic algorithms), which is relatively easy to ignore the subspace of the global optimal solutions.
Based on the analysis, our proposed model helps to improve the current large-scale EV charging efficiency and has higher execution efficiency compared to traditional algorithms as well as other reinforcement learning based algorithms. This has an enlightening significance for further research on large-scale electric vehicle charging scheduling methods in more complex scenarios.
The growing significance of large-scale EV charging scheduling challenges across diverse scenarios underscores the urgent need for effective solutions in this domain. In this study, we introduce a novel model architecture tailored specifically to tackle the complexities inherent in large-scale EV charging scheduling. Our approach revolves around the creation of two distinct state representations encapsulating the global scheduling information. Furthermore, we introduce a performance metric aimed at quantifying the quality of EV charging service. To optimize scheduling performance, we employ the Fine-Grained Feature Extraction Module (FFEM), the Improved Noise-Based Exploration Module (INEM), and a dueling block for enhancing feature extraction, solution space exploration ability, and Q-value prediction accuracy, respectively. To ensure effective model training, we propose a two-stage algorithm encompassing model pretraining proficient trajectory sampling, and action space exploration.
The effectiveness of our proposed methodology is rigorously demonstrated through three comprehensive case studies. Our experimental findings clearly indicate that our approach excels in generating optimal EV charging schedules, outperforms existing methodologies in terms of efficiency and effectivity. Overall, our proposal furnishes valuable insights for large-scale EV charging scheduling service providers and furnishes a roadmap for addressing challenges characterized by extensive action spaces using Deep Reinforcement Learning (DRL) techniques.
Our future research endeavors will be geared towards further enhancing the performance of the EV charging algorithm. By focusing on improving computational efficiency and optimizing the scheduling process, we aim to elevate the overall efficiency and effectiveness of EV charging services.
Hussain S et al (2023) Enhancing the efficiency of electric vehicles charging stations based on novel fuzzy integer linear programming. IEEE Trans Intell Transp Syst 24(9):9150–9164. https://doi.org/10.1109/TITS.2023.3274608
Google Scholar
Long T, Jia QS, Wang G, Yang Y (2021) Efficient real-time EV charging scheduling via ordinal optimization. IEEE Trans Smart Grid 12(5):4029–4038. https://doi.org/10.1109/TSG.2021.3078445
V. Global (2021) "The global electric vehicle market overview In 2021. Statistics & Forecasts 1:2022
Li T, Li X, He T, Zhang Y (2022) "An EDA-based Genetic Algorithm for EV Charging Scheduling under Surge Demand," In: 2022 IEEE International Conference on Services Computing (SCC) , 10–16 July 2022, pp. 231–238, https://doi.org/10.1109/SCC55611.2022.00042
Rahman MM, Al-Ammar EA, Das HS, Ko WS (2020) Comprehensive impact analysis of electric vehicle charging scheduling on load-duration curve. Comput Electr Eng 85:106673. https://doi.org/10.1016/j.compeleceng.2020.106673
Abdullah HM, Gastli A, Ben-Brahim L (2021) Reinforcement learning based EV charging management systems-a review. IEEE Access, Rev 9:41506–41531. https://doi.org/10.1109/ACCESS.2021.3064354
Wu J, Su H, Meng JH, Lin MQ (2023) Electric vehicle charging scheduling considering infrastructure constraints. Energy 278:127806. https://doi.org/10.1016/j.energy.2023.127806
Mishra S, Mondal A, Mondal S (2023) A multi-objective optimization framework for electric vehicle charge scheduling with adaptable charging ports. IEEE Trans Veh Technol 72(5):5702–5714. https://doi.org/10.1109/tvt.2022.3231901
Amin A, Mahmood A, Khan AR, Arshad K, Assaleh K, Zoha A (2023) A two-stage multi-agent EV charging coordination scheme for maximizing grid performance and customer satisfaction. Sensors 23(6):2925. https://doi.org/10.3390/s23062925
Falabretti D, Gulotta F (2022) A nature-inspired algorithm to enable the E-mobility participation in the ancillary service market. Energies 15(9):3023. https://doi.org/10.3390/en15093023
Cai W, Vosoogh M, Reinders B, Toshin DS, Ebadi AG (2019) Application of quantum artificial bee colony for energy management by considering the heat and cooling storages. Appl Thermal Eng 157:113742. https://doi.org/10.1016/j.applthermaleng.2019.113742
Comert SE, Yazgan HR (2023) A new approach based on hybrid ant colony optimization-artificial bee colony algorithm for multi-objective electric vehicle routing problems. Eng Appl Artif Intell 123:106375
Yang Q, Huang Y, Zhang Q, Zhang J (2023) A bi-level optimization and scheduling strategy for charging stations considering battery degradation. Energies 16(13):5070. https://doi.org/10.3390/en16135070
Das P, Samantaray S, Kayal P (2023) Evaluation of distinct EV scheduling at residential charging points in an unbalanced power distribution system. IETE J Res. https://doi.org/10.1080/03772063.2023.2187891
Sukumar B, Aslam S, Karthikeyan N, Rajesh P (2023) A hybrid BCMPO technique for optimal scheduling of electric vehicle aggregators under market price uncertainty. IETE J Res. https://doi.org/10.1080/03772063.2023.2177756
Fu L, Wang T, Song M, Zhou Y, Gao S (2023) Electric vehicle charging scheduling control strategy for the large-scale scenario with non-cooperative game-based multi-agent reinforcement learning. Int J Electr Power Energy Syst 153:109348
Poddubnyy A, Nguyen P, Slootweg H (2023) "Online EV charging controlled by reinforcement learning with experience replay. Sustain Energy Grids Netw 36:101162
Sykiotis S, Menos-Aikateriniadis C, Doulamis A, Doulamis N, Georgilakis PS (2023) A self-sustained EV charging framework with N-step deep reinforcement learning. Sustain Energy, Grids Netw 35:101124
Lee S, Choi D-H (2023) Two-stage scheduling of smart electric vehicle charging stations and inverter-based Volt-VAR control using a prediction error-integrated deep reinforcement learning method. Energy Rep 10:1135–1150
Sultanuddin SJ, Vibin R, Rajesh Kumar A, Behera NR, Pasha MJ, Baseer KK (2023) Development of improved reinforcement learning smart charging strategy for electric vehicle fleet. J Energy Storage 64:106987
Park K, Moon I (2022) Multi-agent deep reinforcement learning approach for EV charging scheduling in a smart grid. Appl Energy 328:120111
Wang S, Bi S, Zhang YA (2021) Reinforcement learning for real-time pricing and scheduling control in EV charging stations. IEEE Trans Industr Inf 17(2):849–859. https://doi.org/10.1109/TII.2019.2950809
Tan M, Dai Z, Su Y, Chen C, Wang L, Chen J (2023) Bi-level optimization of charging scheduling of a battery swap station based on deep reinforcement learning. Eng Appl Artif Intell 118:105557
Li H et al (2023) Constrained large-scale real-time EV scheduling based on recurrent deep reinforcement learning. Int J Electr Power Energy Syst 144:108603
Alqahtani M, Scott MJ, Hu M (2022) Dynamic energy scheduling and routing of a large fleet of electric vehicles using multi-agent reinforcement learning. Comput Ind Eng 169:108180
Wang Z, Schaul T, Hessel M, Hasselt H, Lanctot M, Freitas N (2016) "Dueling network architectures for deep reinforcement learning," presented at the Proceedings of The 33rd International Conference on Machine Learning, Proceedings of Machine Learning Research
Fortunato M et al. (2017) "Noisy networks for exploration," arXiv preprint arXiv:1706.10295
Chen Q, Folly KA (2023) Application of artificial intelligence for EV charging and discharging scheduling and dynamic pricing: a review. Energies, Rev 16(1):146. https://doi.org/10.3390/en16010146
Singh PP, Wen F, Palu I, Sachan S, Deb S (2023) electric vehicles charging infrastructure demand and deployment: challenges and solutions. Energ, Rev 16(1):7. https://doi.org/10.3390/en16010007
Ren M, Liu X, Yang Z, Zhang J, Guo Y, Jia Y (2022) A novel forecasting based scheduling method for household energy management system based on deep reinforcement learning. Sustain Cities Soc 76:103207
Svetozarevic B, Baumann C, Muntwiler S, Di Natale L, Zeilinger MN, Heer P (2022) Data-driven control of room temperature and bidirectional EV charging using deep reinforcement learning: simulations and experiments. Appl Energy 307:118127
Jin R, Zhou Y, Lu C, Song J (2022) Deep reinforcement learning-based strategy for charging station participating in demand response. Appl Energy 328:120140
Hussain A, Bui V-H, Musilek P (2023) Local demand management of charging stations using vehicle-to-vehicle service: a welfare maximization-based soft actor-critic model. Etransportation 18:100280
Qiu D, Ye Y, Papadaskalopoulos D, Strbac G (2020) A deep reinforcement learning method for pricing electric vehicles with discrete charging levels. IEEE Trans Ind Appl 56(5):5901–5912
Zhang Y, Rao X, Liu C, Zhang X, Zhou Y (2023) A cooperative EV charging scheduling strategy based on double deep Q-network and Prioritized experience replay. Eng Appl Artif Intell 118:105642
Qian T, Shao C, Wang X, Shahidehpour M (2019) Deep reinforcement learning for EV charging navigation by coordinating smart grid and intelligent transportation system. IEEE Trans Smart Grid 11(2):1714–1723
Ur Rehman U, Riaz M (2018) "Real time controlling algorithm for vehicle to grid system under price uncertainties," In: 2018 1st International Conference on Power, Energy and Smart Grid (ICPESG), IEEE, pp. 1–7
Abdullah HM, Gastli A, Ben-Brahim L (2021) Reinforcement learning based EV charging management systems–a review. IEEE Access 9:41506–41531
Sun J, Zheng Y, Hao J, Meng Z, Liu Y (2020) Continuous multiagent control using collective behavior entropy for large-scale home energy management. Proceed AAAI Conf Artif Intell 34(01):922–929
Zhang F, Yang Q, An D (2021) CDDPG: a deep-reinforcement-learning-based approach for electric vehicle charging control. IEEE Internet Things J 8(5):3075–3087. https://doi.org/10.1109/JIOT.2020.3015204
Shin M, Choi D-H, Kim J (2019) Cooperative management for PV/ESS-enabled electric vehicle charging stations: a multiagent deep reinforcement learning approach. IEEE Trans Industr Inf 16(5):3493–3503
Liu J, Guo H, Xiong J, Kato N, Zhang J, Zhang Y (2019) Smart and resilient EV charging in SDN-enhanced vehicular edge computing networks. IEEE J Sel Areas Commun 38(1):217–228
Wen Z, O’Neill D, Maei H (2015) Optimal demand response using device-based reinforcement learning. IEEE Transactions on Smart Grid 6(5):2312–2324
Li H, Wan Z, He H (2019) Constrained EV charging scheduling based on safe deep reinforcement learning. IEEE Trans Smart Grid 11(3):2427–2439
Dusparic I, Harris C, Marinescu A, Cahill V, Clarke S (2013) "Multi-agent residential demand response based on load forecasting," In: 2013 1st IEEE conference on technologies for sustainability (SusTech), IEEE, pp. 90–96
Zhang A, Liu Q, Liu J, Cheng L (2024) CASA: cost-effective EV charging scheduling based on deep reinforcement learning. Neural Comput Appl. https://doi.org/10.1007/s00521-024-09530-3
Liu D, Zeng P, Cui S, Song C (2023) Deep reinforcement learning for charging scheduling of electric vehicles considering distribution network voltage stability. Sensors 23(3):1618
Jin J, Xu Y (2022) Shortest-path-based deep reinforcement learning for EV charging routing under stochastic traffic condition and electricity prices. IEEE Internet Things J 9(22):22571–22581. https://doi.org/10.1109/JIOT.2022.3181613
MathSciNet Google Scholar
Wang S, Fan Y, Jin S, Takyi-Aninakwa P, Fernandez C (2023) Improved anti-noise adaptive long short-term memory neural network modeling for the robust remaining useful life prediction of lithium-ion batteries. Reliab Eng Syst Saf 230:108920
Wang S, Wu F, Takyi-Aninakwa P, Fernandez C, Stroe D-I, Huang Q (2023) Improved singular filtering-Gaussian process regression-long short-term memory model for whole-life-cycle remaining capacity estimation of lithium-ion batteries adaptive to fast aging and multi-current variations. Energy 284:128677
Download references
This work was supported by the Scientific Research Funds of Northeast Electric Power University (No. BSZT07202107) and the science and technology development program of Jilin province (No. 20240101362JC).
Author information
Authors and affiliations.
School of Computer Science, Northeast Electric Power University, Jilin, China
Yingnan Han, Tianyang Li & Qingzhu Wang
You can also search for this author in PubMed Google Scholar
Corresponding author
Correspondence to Qingzhu Wang .
Ethics declarations
Conflict of interest.
There are no financial or non-financial interests that are directly or indirectly related to the work submitted for publication.
Additional information
Publisher's note.
Springer Nature remains neutral with regard to jurisdictional claims in published maps and institutional affiliations.
Rights and permissions
Open Access This article is licensed under a Creative Commons Attribution-NonCommercial-NoDerivatives 4.0 International License, which permits any non-commercial use, sharing, distribution and reproduction in any medium or format, as long as you give appropriate credit to the original author(s) and the source, provide a link to the Creative Commons licence, and indicate if you modified the licensed material. You do not have permission under this licence to share adapted material derived from this article or parts of it. The images or other third party material in this article are included in the article’s Creative Commons licence, unless indicated otherwise in a credit line to the material. If material is not included in the article’s Creative Commons licence and your intended use is not permitted by statutory regulation or exceeds the permitted use, you will need to obtain permission directly from the copyright holder. To view a copy of this licence, visit http://creativecommons.org/licenses/by-nc-nd/4.0/ .
Reprints and permissions
About this article
Han, Y., Li, T. & Wang, Q. A DQN based approach for large-scale EVs charging scheduling. Complex Intell. Syst. (2024). https://doi.org/10.1007/s40747-024-01587-w
Download citation
Received : 16 December 2023
Accepted : 06 July 2024
Published : 21 August 2024
DOI : https://doi.org/10.1007/s40747-024-01587-w
Share this article
Anyone you share the following link with will be able to read this content:
Sorry, a shareable link is not currently available for this article.
Provided by the Springer Nature SharedIt content-sharing initiative
- Deep Q-network
- Charging scheduling
- Surge demand
- Deep reinforcement learning
- Find a journal
- Publish with us
- Track your research
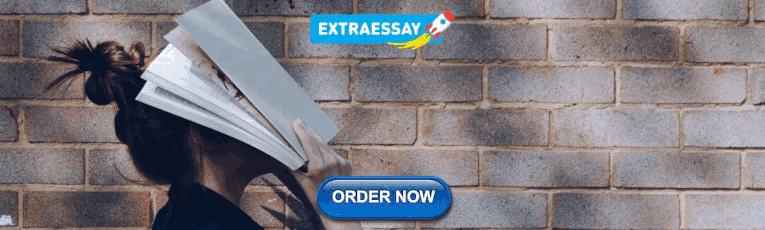
IMAGES
COMMENTS
The term case study is confusing because the same term is used multiple ways. The term can refer to the methodology, that is, a system of frameworks used to design a study, or the methods used to conduct it. Or, case study can refer to a type of academic writing that typically delves into a problem, process, or situation.
Defnition: A case study is a research method that involves an in-depth examination and analysis of a particular phenomenon or case, such as an individual, organization, community, event, or situation. It is a qualitative research approach that aims to provide a detailed and comprehensive understanding of the case being studied.
A case study is one of the most commonly used methodologies of social research. This article attempts to look into the various dimensions of a case study research strategy, the different epistemological strands which determine the particular case study type and approach adopted in the field, discusses the factors which can enhance the effectiveness of a case study research, and the debate ...
Revised on November 20, 2023. A case study is a detailed study of a specific subject, such as a person, group, place, event, organization, or phenomenon. Case studies are commonly used in social, educational, clinical, and business research. A case study research design usually involves qualitative methods, but quantitative methods are ...
Case study research: Design and methods (3rd ed.). Thousand Oaks, CA: Sage. 559 The Qualitative Report December 2008. Author Note . Dr. Pamela Baxter is an assistant prof essor at McMaster ...
Case study method is the most widely used method in academia for researchers interested in qualitative research (Baskarada, 2014). Research students select the case study as a method without understanding array of factors that can affect the outcome of their research. ... Case study research: Design and methods. Thousand Oaks, CA: Sage. Google ...
A case study protocol outlines the procedures and general rules to be followed during the case study. This includes the data collection methods to be used, the sources of data, and the procedures for analysis. Having a detailed case study protocol ensures consistency and reliability in the study.
A case study is a research approach that is used to generate an in-depth, multi-faceted understanding of a complex issue in its real-life context. It is an established research design that is used extensively in a wide variety of disciplines, particularly in the social sciences. A case study can be defined in a variety of ways (Table 5 ), the ...
The term case study is confusing because the same term is used multiple ways. It can refer to the methodology, that is, a system of frameworks used to design a study, or the methods used to conduct it. Or, case study can refer to a type of academic writing that typically delves into a problem, process, or situation.
A case study is a detailed study of a specific subject, such as a person, group, place, event, organisation, or phenomenon. Case studies are commonly used in social, educational, clinical, and business research. A case study research design usually involves qualitative methods, but quantitative methods are sometimes also used.
1967) or with case study design by Eisenhardt (1989) and Eisenhardt and Graebner (2007) and it is often surprising that authors quote these three sources together with Miles and Huberman (1994) in order to legitimate the qualitative design of their study. The design of case studies in Yin's book relates data to propositions, e.g. by pattern ...
Case study research is typically extensive; it draws on multiple methods of data collection and involves multiple data sources. The researcher begins by identifying a specific case or set of cases to be studied. Each case is an entity that is described within certain parameters, such as a specific time frame, place, event, and process.
Providing a complete portal to the world of case study research, the Fourth Edition of Robert K. Yin's bestselling text Case Study Research offers comprehensive coverage of the design and use of the case study method as a valid research tool. This thoroughly revised text now covers more than 50 case studies (approximately 25% new), gives fresh attention to quantitative analyses, discusses ...
The case study method is particularly useful for researching educational interventions because it provides a rich description of all the interrelated factors. ... Jack S (2008). Qualitative case study methodology: Study design and implementation for novice researchers. The Qualitative Report, 13(4), 544-559. Google Scholar. Converse M. (2012 ...
This new edition of the best-selling Case Study Research has been carefully revised, updated, and expanded while retaining virtually all of the features and coverage of the Second Edition. Robert Yin's comprehensive presentation covers all aspects of the case study method--from problem definition, design, and data collection, to data analysis and composition and reporting.
A Case study is: An in-depth research design that primarily uses a qualitative methodology but sometimes includes quantitative methodology. Used to examine an identifiable problem confirmed through research. Used to investigate an individual, group of people, organization, or event. Used to mostly answer "how" and "why" questions.
Method Using multiple case studies (seven children) and a qualitative research design, the self-identity (personal and social) of six- to ten-year-old TRA children was explored.
treatment of case study methods we encourage you to read Hancock and Algozzine's, Doing case study research: A practical guide for beginning researchers (2006). Philosophical Underpinnings . First, both Stake (1995) and Yin (2003) base their approach to case study on a constructivist paradigm.
Definitions of qualitative case study research. Case study research is an investigation and analysis of a single or collective case, intended to capture the complexity of the object of study (Stake, 1995).Qualitative case study research, as described by Stake (), draws together "naturalistic, holistic, ethnographic, phenomenological, and biographic research methods" in a bricoleur design ...
A case study is a research approach that is used to generate an in-depth, multi-faceted understanding of a complex issue in its real-life context. It is an established research design that is used extensively in a wide variety of disciplines, particularly in the social sciences. A case study can be defined in a variety of ways (Table 5 ), the ...
As the case study approach gains popularity in nursing research, questions arise with regard to what it exactly is, and where it appears to fit paradigmatically. Is it a method, a design, are such distinctions important? Colin Jones and Christina Lyons review some of the key issues, with specific emphasis on the use of case study within an ...
How to Design and Conduct a Case Study. The advantage of the case study research design is that you can focus on specific and interesting cases. This may be an attempt to test a theory with a typical case or it can be a specific topic that is of interest. Research should be thorough and note taking should be meticulous and systematic.
Case study research is a type of qualitative research design. It's often used in the social sciences because it involves observing subjects, or cases, in their natural setting, with minimal interference from the researcher. In the case study method, researchers pose a specific question about an individual or group to test their theories or ...
This study employed an embedded mixed method case study design. An embedded mixed methods case study design involves integrating quantitative and qualitative methods within a case study. This is done to gain a more complete understanding of a particular case or situation. A rural village in Gokwe 22 kilometers from the Midlands Capital (Gweru ...
Studies of the Indian Western Offshore Field, its water cut issues, and the various water shut-off methods implemented in its wells, as well as several water shut-off case studies, revealed that: It is difficult, ineffective, and nearly impossible to increase the productivity of a well for a longer period of time by attempting to control water ...
This study sought to investigate primarily the extent of girl child marriage and how it impacts education in Cross River State. Four purpose, research questions and hypothesis guided the study. The research design adopted for this study was the survey research design. The population for the study was all parents with school aged girls in their homes in Cross River State, Nigeria.
In an effort to modernize engineering design standards to appropriately consider the higher practical strength of dynamically loaded structures, this overarching research project seeks to develop an experimental test procedure for quantifying the dynamic strength of metallic alloys as a function of excitation frequency. In the ideal case, the ...
Central to our method is a fast global sensitivity analysis (FGSA) procedure, which is devised to identify correlations between design parameters and quantify their impacts on circuit characteristics.
The widespread application of Artificial Intelligence (AI) techniques has significantly influenced the development of new therapeutic agents. These computational methods can be used to design and predict the properties of generated molecules. Multi-target Drug Discovery (MTDD) is an emerging paradigm for discovering drugs against complex disorders that do not respond well to more traditional ...
Electric Vehicles (EVs) contribute to numerous benefits such as reduced using cost, environment friendly, and decreased consumption of fossil fuels [].As a result, the amount of EVs has been increasing rapidly [2, 3].However, the lack of convenient charging infrastructure and low effective charging scheduling algorithm for large-scale EVs charging scheduling becomes the key barrier to the EVs ...