Click through the PLOS taxonomy to find articles in your field.
For more information about PLOS Subject Areas, click here .
Loading metrics
Open Access
Peer-reviewed
Research Article
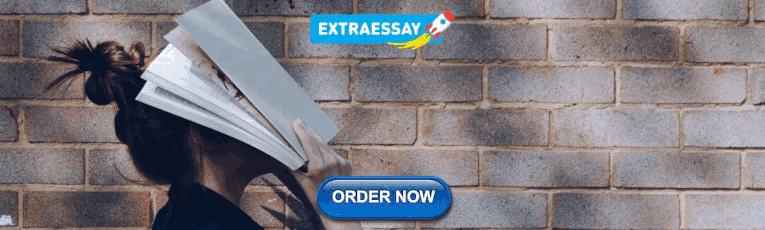
Mobile phones: The effect of its presence on learning and memory
Roles Conceptualization, Data curation, Investigation, Writing – original draft
Affiliation Department of Psychology, Sunway University, Selangor, Malaysia
Roles Formal analysis, Investigation, Methodology, Resources, Supervision, Writing – original draft, Writing – review & editing
* E-mail: [email protected]

- Clarissa Theodora Tanil,
- Min Hooi Yong
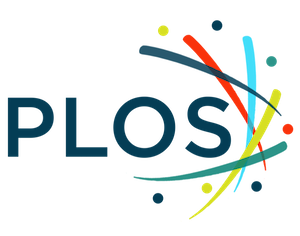
- Published: August 13, 2020
- https://doi.org/10.1371/journal.pone.0219233
- See the preprint
- Peer Review
- Reader Comments
Our aim was to examine the effect of a smartphone’s presence on learning and memory among undergraduates. A total of 119 undergraduates completed a memory task and the Smartphone Addiction Scale (SAS). As predicted, those without smartphones had higher recall accuracy compared to those with smartphones. Results showed a significant negative relationship between phone conscious thought, “how often did you think about your phone”, and memory recall but not for SAS and memory recall. Phone conscious thought significantly predicted memory accuracy. We found that the presence of a smartphone and high phone conscious thought affects one’s memory learning and recall, indicating the negative effect of a smartphone proximity to our learning and memory.
Citation: Tanil CT, Yong MH (2020) Mobile phones: The effect of its presence on learning and memory. PLoS ONE 15(8): e0219233. https://doi.org/10.1371/journal.pone.0219233
Editor: Barbara Dritschel, University of St Andrews, UNITED KINGDOM
Received: June 17, 2019; Accepted: July 30, 2020; Published: August 13, 2020
Copyright: © 2020 Tanil, Yong. This is an open access article distributed under the terms of the Creative Commons Attribution License , which permits unrestricted use, distribution, and reproduction in any medium, provided the original author and source are credited.
Data Availability: All relevant data are within the manuscript.
Funding: MHY received funding from Sunway University (GRTIN-RRO-104-2020 and INT-RRO-2018-49).
Competing interests: The authors have declared that no competing interests exist.
Introduction
Smartphones are a popular communication form worldwide in this century and likely to remain as such, especially among adolescents [ 1 ]. The phone has evolved from basic communicative functions–calls only–to being a computer-replacement device, used for web browsing, games, instant communication on social media platforms, and work-related productivity tools, e.g. word processing. Smartphones undoubtedly keep us connected; however, many individuals are now obsessed with them [ 2 , 3 ]. This obsession can lead to detrimental cognitive functions and mood/affective states, but these effects are still highly debated among researchers.
Altmann, Trafton, and Hambrick suggested that as little as a 3-second distraction (e.g. reaching for a cell phone) is adequate to disrupt attention while performing a cognitive task [ 4 ]. This distraction is disadvantageous to subsequent cognitive tasks, creating more errors as the distraction period increases, and this is particularly evident in classroom settings. While teachers and parents are for [ 5 ] or against cell phones in classrooms [ 6 ], empirical evidence showed that students who used their phones in class took fewer notes [ 7 ] and had poorer overall academic performance, compared to those who did not [ 8 , 9 ]. Students often multitask in classrooms and even more so with smartphones in hand. One study showed no significant difference in in-class test scores, regardless of whether they were using instant messaging [ 10 ]. However, texters took a significantly longer time to complete the in-class test, suggesting that texters required more cognitive effort in memory recall [ 10 ]. Other researchers have posited that simply the presence of a cell phone may have detrimental effects on learning and memory as well. Research has shown that a mobile phone left next to the participant while completing a task, is a powerful distractor even when not in use [ 11 , 12 ]. Their findings showed that mobile phone participants could perform similarly to control groups on simple versions of specific tasks (e.g. visual spatial search, digit cancellation), but performed much poorer in the demanding versions. In another study, researchers controlled for the location of the smartphone by taking the smartphones away from participants (low salience, LS), left the smartphone next to them (high salience/HS), or kept the smartphones in bags or pockets (control) [ 13 ]. Results showed that participants in LS condition performed significantly better compared to HS, while no difference was established between control and HS conditions. Taken together, these findings confirmed that the smartphone is a distractor even when not in use. Further, smartphone presence also increases cognitive load, because greater cognitive effort is required to inhibit distractions.
Reliance on smartphones has been linked to a form of psychological dependency, and this reliance has detrimental effect on our affective ‘mood’ states. For example, feelings of anxiety when one is separated from their smartphones can interfere with the ability to attend to information. Cheever et al. observed that heavy and moderate mobile phone users reported increased anxiety when their mobile phone was taken away as early as 10 minutes into the experiment [ 14 ]. They noted that high mobile phone usage was associated with higher risk of experiencing ‘nomophobia’ (no mobile phone phobia), a form of anxiety characterized by constantly thinking about one’s own mobile phones and the desire to stay in contact with the device [ 15 ]. Other studies reported similar separation-anxiety and other unpleasant thoughts in participants when their smartphones were taken away [ 16 ] or the usage was prohibited [ 17 , 18 ]. Participants also reported having frequent thoughts about their smartphones, despite their device being out of sight briefly (kept in bags or pockets), to the point of disrupting their task performance [ 13 ]. Taken together, these findings suggest that strong attachment towards a smartphone has immediate and lasting negative effects on mood and appears to induce anxiety.
Further, we need to consider the relationship between cognition and emotion to understand how frequent mobile phone use affects memory e.g. memory consolidation. Some empirical findings have shown that anxious individuals have attentional biases toward threats and that these biases affect memory consolidation [ 19 , 20 ]. Further, emotion-cognition interaction affects efficiency of specific cognitive functions, and that one’s affective state may enhance or hinder these functions rapidly, flexibly, and reversibly [ 21 ]. Studies have shown that positive affect improves visuospatial attention [ 22 ], sustained attention [ 23 ], and working memory [ 24 ]. The researchers attributed positive affect in participants’ improved controlled cognitive processing and less inhibitory control. On the other hand, participants’ negative affect had fewer spatial working memory errors [ 23 ] and higher cognitive failures [ 25 ]. Yet, in all of these studies–the direction of modulation, intensity, valence of experiencing a specific affective state ranged widely and primarily driven by external stimuli (i.e. participants affective states were induced from watching videos), which may not have the same motivational effect generated internally.
Present study
Prior studies have demonstrated the detrimental effects of one’s smartphone on cognitive function (e.g. working memory [ 13 ], visual spatial search [ 12 ], attention [ 11 ]), and decreased cognitive ability with increasing attachment to one’s phone [ 14 , 16 , 26 ]. Further, past studies have demonstrated the effect of affective state on cognitive performance [ 19 , 20 , 22 – 25 , 27 ]. To our knowledge, no study has investigated the effect of positive or negative affective states resulting from smartphone separation on memory recall accuracy. One study showed that participants reporting an increased level of anxiety as early as 10 minutes [ 14 ]. We also do not know the extent of smartphone addiction and phone conscious thought effects on memory recall accuracy. One in every four young adults is reported to have problematic smartphone use and this is accompanied by poor mental health e.g. higher anxiety, stress, depression [ 28 ]. One report showed that young adults reached for their phones 86 times in a day on average compared to 47 times in other age groups [ 29 ]. Young adults also reported that they “definitely” or “probably” used their phone too much, suggesting that they recognised their problematic smartphone use.
We had two main aims in this study. First, we replicated [ 13 ] to determine whether ‘phone absent’ (LS) participants had higher memory accuracy compared to the ‘phone present’ (HS). Second, we predicted that participants with higher smartphone addiction scores (SAS) and higher phone conscious thought were more likely to have lower memory accuracy. With regards to separation from their smartphone, we hypothesised that LS participants will experience an increase of negative affect or a decrease in positive affect and that this will affect memory recall negatively. We will also examine whether these predictor variables–smartphone addiction, phone conscious thought and affect differences—predict memory accuracy.
Materials and methods
Participants.
A total of 119 undergraduate students (61 females, M age = 20.67 years, SD age = 2.44) were recruited from a private university in an Asian capital city. To qualify for this study, the participant must own a smartphone and does not have any visual or auditory deficiencies. Using G*Power v. 3.1.9.2 [ 30 ], we require at least 76 participants with an effect size of d = .65, α = .05 and power of (1-β) = .8 based on Thornton et al.’s [ 11 ] study, or 128 participants from Ward’s study [ 13 ].
Out of 119 participants, 43.7% reported using their smartphone mostly for social networking, followed by communication (31.1%) and entertainment (17.6%) (see Table 1 for full details on smartphone usage). Participants reported an average smartphone use of 8.16 hours in a day ( SD = 4.05). There was no significant difference between daily smartphone use for participants in the high salience (HS) and low salience groups (LS), t (117) = 1.42, p = .16, Cohen’s d = .26. Female participants spent more time using their smartphones over a 24-hour period ( M = 9.02, SD = 4.10) compared to males, ( M = 7.26, SD = 3.82), t (117) = 2.42, p = .02, Cohen’s d = .44.
- PPT PowerPoint slide
- PNG larger image
- TIFF original image
https://doi.org/10.1371/journal.pone.0219233.t001
Ethical approval and informed consent
The study was conducted in accordance with the protocol approved by the Department of Psychology Research Ethics Committee at Sunway University (approval code: 20171090). All participants provided written consent before commencing the study and were not compensated for their participation in the study.
Study design
Our experimental study was a mixed design, with smartphone presence (present vs absent) as a between-subjects factor, and memory task as a within-subjects factor. Participants who had their smartphone out of sight formed the ‘Absent’ or low-phone salience (LS) condition, and the other group had their smartphone placed next to them throughout the study, ‘Present’ or high-phone salience (HS) condition. The dependent variable was recall accuracy from the memory test.
Working memory span test.
A computerized memory span task ‘Operation Span (OS)’ retrieved from software Wadsworth CogLab 2.0 was used to assess working memory [ 31 ]. A working memory span test was chosen as a measure to test participants’ memory ability for two reasons. First, participants were required to learn and memorize three types of stimuli thus making this task complex. Second, the duration of task completion took approximately 20 minutes. This was advantageous because we wanted to increase separation-anxiety [ 16 ] as well as having the most pronounced effect on learning and memory without the presence of their smartphone [ 9 ].
The test comprised of three stimulus types, namely words (long words such as computer, refrigerator and short words like pen, cup), letters (similar sound E, P, B, and non-similar sound D, H, L) and digits (1 to 9). The test began by showing a sequence of items on the left side of the screen, with each item presented for one second. After that, participants were required to recall the stimulus from a 9-button box located on the right side of the screen. In order to respond correctly, participants were required to click on the buttons for the items in the corresponding order they were presented. A correct response increases the length of stimulus presented by one item (for each stimulus category), while an incorrect response decreases the length of the stimulus by one item. Each trial began with five stimuli and increased or decreased depending on the participants’ performance. The minimum length possible was one while the maximum was ten. Each test comprised of 25 trials with no time limit and without breaks between trials. Working memory ability was measured through the number of correct responses over total trials: scores ranged from 0 to 25, with the highest score representing superior working memory.
Positive and Negative Affect Scale (PANAS).
We used PANAS to assess the current mood/affective state of the participants with state/feeling-descriptive statements [ 32 ]. PANAS has ten PA statements e.g. interested, enthusiastic, proud, and ten NA statements e.g. guilty, nervous, hostile. Each statement was measured using a five-point Likert scale ranging from very slightly or not at all to extremely, and then totalled to form overall PA or NA score with higher scores representing higher levels of PA or NA. In the current study, the internal reliability of PANAS was good with a Cronbach’s alpha coefficient of .819, and .874 for PA and NA respectively.
Smartphone Addiction Scale (SAS)
SAS is a 33-item self-report scale used to examine participants’ smartphone addiction [ 33 ]. SAS contained six sub-factors; daily-life disturbance that measures the extent to which mobile phone use impairs one’s activities during everyday tasks (5 statements), positive anticipation to describe the excitement of using phone and de-stressing with the use of mobile phone (8 statements), withdrawal refers to the feeling of anxiety when separated from one’s mobile phone (6 statements), cyberspace-oriented relationship refers to one’s opinion on online friendship (7 statements), overuse measures the excessive use of mobile phone to the extent that they have become inseparable from their device (4 statements), and tolerance points to the cognitive effort to control the usage of one’s smartphone (3 statements). Each statement was measured using a six-point Likert scale from strongly disagree to strongly agree, and total SAS was identified by totalling all 33 statements. Higher SAS scores represented higher degrees of compulsive smartphone use. In the present study, the internal reliability of SAS was identified with Cronbach's alpha correlation coefficient of .918.
Phone conscious thought and perceived effect on learning
We included a one-item question for phone conscious thought: “During the memory test how often do you think of your smartphone?”. The aim of this question was two-fold; first was to capture endogenous interruption experienced by the separation, and second to complement the smartphone addiction to reflect current immediate experience. Participants rated this item on a scale of one (none to hardly) to seven (all the time). We also included a one-item question on how much they perceived their smartphone use has affected their learning and attention: “In general, how much do you think your smartphone affects your learning performance and attention span?”. This item was similarly rated on a scale of one (not at all) to seven (very much).
We randomly assigned participants to one of two conditions: low-phone salience (LS) and high-phone salience (HS). Participants were tested in groups of three to six people in a university computer laboratory and seated two seats apart from each other to prevent communication. Each group was assigned to the same experimental condition to ensure similar environmental conditions. Participants in the HS condition were asked to place their smartphone on the left side of the table with the screen facing down. LS participants were asked to hand their smartphone to the researcher at the start of the study and the smartphones were kept on the researcher’s table throughout the task at a distance between 50cm to 300cm from the participants depending on their seat location, and located out of sight behind a small panel on the table.
At the start of the experiment, participants were briefed on the rules in the experimental lab, such as no talking and no smartphone use (for HS only). Participants were also instructed to silence their smartphones. They filled in the consent form and demographic form before completing the PANAS questionnaire. They were then directed to CogLab software and began the working memory test. Upon completion, participants were asked to complete the PANAS again followed by the SAS, phone conscious thought, and their perception of their phone use on their learning performance and attention span. The researcher thanked the participants and returned the smartphones (LS condition only) at the end of the task.
Statistical analysis
We examined for normality in our data using the Shapiro-Wilk results and visual inspection of the histogram. For the normally distributed data, we analysed our data using independent-sample t -test for comparison between groups (HS or LS), paired-sample t test for within groups (e.g. before and after phone separation), and Pearson r for correlation. Non-normally distributed or ranked data were analysed using Spearman rho for correlation.
Preliminary analyses
Our female participants reported using their smartphone significantly longer than males, and so we examined the effects of gender on memory recall accuracy. We found no significant difference between males and females on memory recall accuracy, t (117) = .18, p = .86, Cohen’s d = .03. Subsequently, data were collapsed, analysed and reported on in the aggregate.
Smartphone presence and memory recall accuracy
An independent-sample t- test was used to examine whether participants’ performance on a working memory task was influenced by the presence (HS) or absence (LS) of their smartphone. Results showed that participants in the LS condition had higher accuracy ( M = 14.21, SD = 2.61) compared to HS ( M = 13.08, SD = 2.53), t (117) = 2.38, p = .02, Cohen’s d = .44 (see Fig 1 ). The effect size ᶇ 2 = .44 indicates that smartphone presence/salience has a moderate effect on participant working memory ability and a sensitivity power of .66.
https://doi.org/10.1371/journal.pone.0219233.g001
Relationship between Smartphone Addiction Score (SAS), higher phone conscious thought and memory recall accuracy
Sas and memory recal..
We first examined participants’ SAS scores between the two conditions. Results showed no significant difference between the LS (M = 104.64, SD = 24.86) and HS (M = 102.70, SD = 20.45) SAS scores, t (117) = .46, p = .64, Cohen’s d = .09. We predicted that those with higher SAS scores will have lower memory accuracy, and thus we examined the relationship between SAS and memory recall accuracy using Pearson correlation coefficient. Results showed that there was no significant relationship between SAS and memory recall accuracy, r = -.03, n = 119, p = .76. We also examined the SAS scores between the LS and HS groups on memory recall accuracy scores. In the LS group, no significant relationship was established between SAS score and memory accuracy, r = -.04, n = 58, p = .74. Similarly, there was no significant relationship between SAS score and memory accuracy in the HS group, r = .10, n = 61, p = .47. In the event that one SAS subscale may have a larger impact, we examined the relationship between each subscale and memory recall accuracy. Results showed no significant relationship between each sub-factor of SAS scores and memory accuracy, all p s > .12 (see Table 2 ).
https://doi.org/10.1371/journal.pone.0219233.t002
Phone conscious thought and memory accuracy.
We found a significant negative relationship between phone conscious thought and memory recall accuracy, r S = -.25, n = 119, p = .01. We anticipated a higher phone conscious thought for the LS group since their phone was kept away from them during the task and examined the relationship for each condition. Results showed a significant negative relationship between phone conscious thought and memory accuracy in the HS condition, r S = -.49, n = 61, p = < .001, as well as the LS condition, r S = -.27, n = 58, p = .04.
Affect/mood changes after being separated from their phone
We anticipated that our participants may have experienced either an increase in negative affect (NA) or a decrease in positive affect (PA) after being separated from their phone (LS condition).
We first computed the mean difference (After minus Before) for both positive ‘PA difference’ and negative affect ‘NA difference’. A repeated-measures 2 (Mood change: PA difference, NA difference) x 2 (Conditions: LS, HS) ANOVA was conducted to determine whether there is an interaction between mood change and condition. There was no interaction effect of mood change and condition, F (1, 117) = .38, p = .54, n p 2 = .003. There was a significant effect of Mood change, F (1, 117) = 13.01, p < .001, n p 2 = .10 (see Fig 2 ).
https://doi.org/10.1371/journal.pone.0219233.g002
Subsequent post-hoc analyses showed a significant decrease in participants’ positive affect before ( M = 31.12, SD = 5.79) and after ( M = 29.36, SD = 6.58) completing the memory task in the LS participants, t (57) = 2.48, p = .02, Cohen’s d = .28 but not for the negative affect, Cohen’s d = .07. A similar outcome was also shown in the HS condition, in which there was a significant decrease in positive affect only, t (60) = 3.45, p = .001, Cohen’s d = .37 (see Fig 2 ).
PA/NA difference on memory accuracy.
We predicted that LS participants will experience either an increase in NA and/or a decrease in PA since their smartphones were taken away and that this will affect memory recall negatively. Results showed that LS participants who experienced a higher NA difference had poorer memory recall accuracy ( r s = -.394, p = .002). We found no significant relationship between NA difference and memory recall accuracy for HS participants ( r s = -.057, p = .663, n = 61) and no significant relationship for PA difference in both HS ( r s = .217, p = .093) and LS conditions ( r s = .063, p = .638).
Relationship between phone conscious thought, smartphone addiction scale and mood changes to memory recall accuracy
Preliminary analyses were conducted to ensure no violation of the assumptions of normality, linearity, multicollinearity and homoscedasticity. There was a significant positive relationship between SAS scores and phone conscious thought, r S = .25, n = 119, p = .007. Using the enter method, we found that phone conscious thought explained by the model as a whole was 19.9%, R 2 = .20, R 2 Adjusted = .17, F (4, 114) = 7.10, p < .001. Phone conscious thought significantly predicted memory recall accuracy, b = -.63, t (114) = 4.76, p < .001, but not for the SAS score, b = .02, t (114) = 1.72, p = .09, PA difference score, b = .05, t (114) = 1.29, p = .20, and NA difference score, b = .06, t (114) = 1.61, p = .11.
Perception between phone usage and learning
For the participants’ perception of their phone usage on their learning and attention span, we found no significant difference between LS ( M = 4.22, SD = 1.58) and HS participants ( M = 4.07, SD = 1.62), t (117) = .54, p = .59, Cohen’s d = .09. There was also no significant correlation between perceived cognitive interference and memory accuracy, r = .07, p = .47.
We aimed [ 1 ] to examine the effect of smartphone presence on memory recall accuracy and [ 2 ] to investigate the relationship between affective states, phone conscious thought, and smartphone addiction to memory recall accuracy. For the former, our results were consistent with prior studies [ 11 – 13 ] in that participants had lower accuracy when their smartphone was next to them (HS) and higher accuracy when separated from their smartphones (LS). For the latter, we predicted that the short-term separation from their smartphone would evoke some anxiety, identified by either lower PA or higher NA post-test. Our results showed that both groups had experienced a decrease in PA post-test, suggesting that the reduced PA is likely to have stemmed from the prohibited usage (HS) and/or separation from their phone (LS). Our results also showed lower memory recall in the LS group who experienced higher NA providing some evidence that separation from their smartphone does contribute to feelings of anxiety. This is consistent with past studies in which participants reported increased anxiety over time when separated from their phones [ 14 ], or when smartphone usage was prohibited [ 17 ].
We also examined another variable–phone conscious thought–described in past studies [ 11 , 13 ], as a measure of smartphone addiction. Our findings showed that phone conscious thought is negatively correlated to memory recall in both HS and LS groups, and uniquely contributed 19.9% in our regression model. We propose that phone conscious thought is more relevant and meaningful compared to SAS as a measure of smartphone addiction [ 15 ] because unlike the SAS, this question can capture endogenous interruptions from their smartphone behaviour and participants were to simply report their behaviour within the last hour. The SAS is better suited to describe problematic smartphone use as the statements described behaviours over a longer duration. Further, SAS statements included some judgmental terms such as fretful, irritated, and this might have influenced participants’ ability in recalling such behaviour. We did not find any support for high smartphone addiction to low memory recall accuracy. Our participants in both HS and LS groups had similar high SAS scores, and they were similar to Kwon et al. [ 33 ] study, providing further evidence that smartphone addiction is relatively high in the student population compared to other categories such as employees, professionals, unemployed. Our participants’ high SAS scores and primary use of the smartphone was for social media signals potential problematic users [ 34 ]. Students’ usage of social networking (SNS) is common and the fear of missing out (FOMO) may fuel the SNS addiction [ 35 ]. Frequent checks on social media is an indication of lower levels of self-control and may indicate a need for belonging.
Our results for the presence of a smartphone and frequent phone conscious thought on memory recall is likely due to participants’ cognitive load ‘bandwidth effect’ that contributed to poor memory recall rather than a failure in their memory processes. Past studies have shown that participants with smartphones could generally perform simple cognitive tasks as well as those without, suggesting that memory failure in participants themselves to be an unlikely reason [ 1 , 3 , 5 ]. Due to our study design, we are unable to tease apart whether the presence of the smartphone had interfered with encoding, consolidation, or recall stage in our participants. This is certainly something of consideration for future studies to determine which aspects of memory processes are more susceptible to smartphone presence.
There are several limitations in our study. First, we did not ask the phone conscious thought at specific time points during the study. Having done so might have determined whether such thoughts impaired encoding, consolidating, or retrieval. Second, we did not include the simple version of this task as a comparison to rule out possible confounds within the sample. We did maintain similar external stimuli in their environment during testing, e.g. all participants were in one specific condition, lab temperature, lab noise, and thereby ruling out possible external factors that may have interfered with their memory processes. Third, the OS task itself. This task is complex and unfamiliar, which may have caused some disadvantages to some participants. However, the advantage of an unfamiliar task requires more cognitive effort to learn and progress and therefore demonstrates the limited cognitive load capacity in our brain, and whether such limitation is easily affected by the presence of a smartphone. Future studies could consider allowing participants to use their smartphone in both conditions and including eye-tracking measures to determine their smartphone attachment behaviour.
Implications
Future studies should look into the online learning environment. Students are often users of multiple electronic devices and are expected to use their devices frequently to learn various learning materials. Because students frequently use their smartphones for social media and communication during lessons [ 34 , 36 ], the online learning environment becomes far more challenging compared to a face-to-face environment. It is highly unlikely that we can ban smartphones despite evidence showing that students performed poorer academically with their smartphones presented next to them. The challenge is then to engage students to remain focused on their lessons while minimising other content. Some online platforms (e.g. Kahoot and Mentimeter) create a fun interactive experience to which students complete tasks on their smartphones and allow the instructor to monitor their performance from a computer. Another example is to use Twitter as a classroom tool [ 37 ].
The ubiquitous nature of the smartphone in our lives also meant that our young graduates are constantly connected to their smartphones and very likely to be on SNS even at work. Our findings showed that the most frequently used feature was the SNS sites e.g. Instagram, Facebook, and Twitter. Being frequently on SNS sites may be a challenge in the workforce because these young adults need to maintain barriers between professional and social lives. Young adults claim that SNS can be productive at work [ 38 ], but many advise to avoid crossing boundaries between professional and social lives [ 39 , 40 ]. Perhaps a more useful approach is to recognise a good balance when using SNS to meet both social and professional demands for the young workforce.
In conclusion, the presence of the smartphone and frequent thoughts of their smartphone significantly affected memory recall accuracy, demonstrating that they contributed to an increase in cognitive load ‘bandwidth effect’ interrupting participants’ memory processes. Our initial hypothesis that experiencing higher NA or lower PA would have reduced their memory recall was not supported, suggesting that other factors not examined in this study may have influenced our participants’ affective states. With the rapid rise in the e-learning environment and increasing smartphone ownership, smartphones will continue to be present in the classroom and work environment. It is important that we manage or integrate the smartphones into the classroom but will remain a contentious issue between instructors and students.
Acknowledgments
We would like to thank our participants for volunteering to participate in this study, and comments on earlier drafts by Louisa Lawrie and Su Woan Wo. We would also like to thank one anonymous reviewer for commenting on the drafts.
- View Article
- Google Scholar
- 2. Perlow LA. Sleeping with Your Smartphone: How to Break the 24/7 Habit and Change the Way You Work. Harvard Business Press; 2012. 286 p.
- 3. G D. 45 Smartphone Addiction Stats In 2020 [The Scary Nomophobia] [Internet]. Tech Jury. 2019 [cited 2020 Apr 13]. Available from: https://techjury.net/stats-about/smartphone-addiction/
- PubMed/NCBI
- 31. Francis G, Neath I, VanHorn D. CogLab On A CD, Version 2.0. Belmont, CA: Wadsworth; 2008.
- 39. Wang Y, Norcie G, Komanduri S, Acquisti A, Leon PG, Cranor LF. ‘I Regretted the Minute I Pressed Share’: A qualitative study of regrets on Facebook. In: Proceedings of the Seventh Symposium on Usable Privacy and Security [Internet]. New York, NY, USA: ACM; 2011 [cited 2019 Apr 23]. p. 10:1–10:16. (SOUPS ‘11). Available from: http://doi.acm.org/10.1145/2078827.2078841
- 40. Skeels MM, Grudin J. When social networks cross boundaries: A case study of workplace use of Facebook and Linkedin. In: Proceedings of the ACM 2009 International Conference on Supporting Group Work [Internet]. New York, NY, USA: ACM; 2009 [cited 2019 Apr 23]. p. 95–104. (GROUP ‘09). Available from: http://doi.acm.org/10.1145/1531674.1531689
From “brick” to smartphone: the evolution of the mobile phone
- FEATURES POSTERMINARIES
- Published: 05 March 2021
- Volume 46 , pages 287–288, ( 2021 )
Cite this article
- Steve Moss 1
7995 Accesses
1 Altmetric
Explore all metrics
Avoid common mistakes on your manuscript.
Telephony began in the 1870s with the invention of the telephone. Alexander Graham Bell filed a patent for his version of the telephone at the US Patent Office in Washington, DC, on February 14, 1876, just a few hours before his competitor Elisha Gray filed his patent based upon independent work. 1 Since then, materials research has pushed this field of technology involving the development, application, and deployment of telecommunication services, particularly in recent years.
The proliferation of telephones did not make a great overnight leap. In fact, as late as 1950, only 62% of American households contained a telephone, 2 and that number had been significantly smaller before World War II. Most communications were by telegram, letter, or through face-to-face discussions. One significant hindrance to communication was natural disasters, which often led to long periods of no information between family and friends.
On the evening of January 3, 1949, a devastating tornado struck the small town of Warren, Ark. 3 The tornado left more than 50 dead and more than 300 injured. My dad’s parents, brother, sister-in-law, niece, and nephew, as well as many friends, lived in Warren at that time and were affected. Telephone lines were destroyed. My parents and I lived far away and had no way to get in contact with them. More than a week passed before we received word that they were okay.
My father later accepted a faculty position at Arkansas A&M College in Monticello, 16 miles from Warren, to be closer to his family. When we moved to a farm near the campus, our first telephone line was a party line, which consisted of a single channel shared by many people. This offered little in the way of privacy, as others outside of your household could listen to conversations. We eventually upgraded to a private landline. The phone was located in a central part of the house, and the cord was only a few feet long, which meant that you were essentially tethered to that spot when making or answering a call, not ideal for any teenager craving privacy.
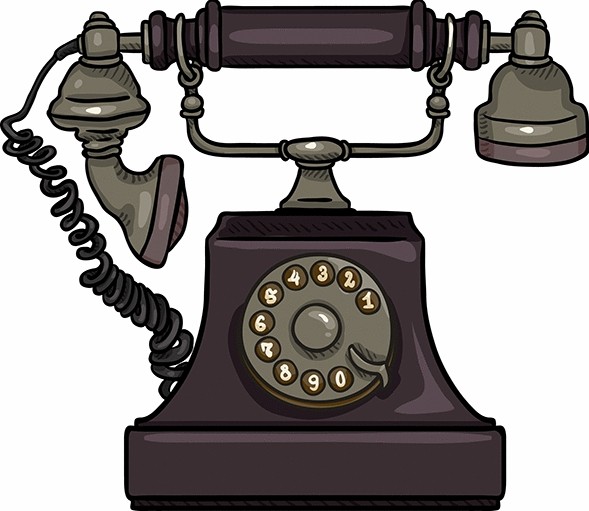
People then didn’t have the luxury of cell phones, and instead often used pay phones by inserting money or calling collect. Jim Croce has a wonderful song, “Operator,” 4 about an unsuccessful attempt to connect with some old friends. I often wonder if younger people understand the significance of the lyrics, including the phrase “You can keep the dime.”
Today, humanity is more connected than ever through the use of cell phones. However, mobile phones didn’t start in their current, sleek style. The first mobile phone by Motorola in 1983 5 was so big and heavy that it was nicknamed “the brick.” Current phones are significantly more lightweight and compact and have the capability to text, email, access social media, access the Internet, and much more.
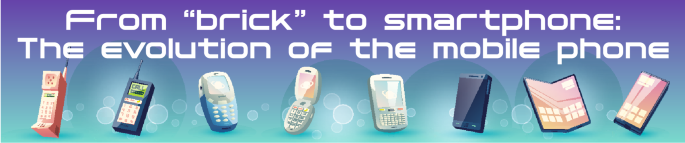
According to recent surveys, 75% of the world’s population owns a cell phone. 6 , 7 Surveys in 2019 indicated that there were 5.11 billion unique mobile phone users, and that 2.71 billion of them used smartphones. People from China (> 782 million users) and India (> 386 million users) are the largest consumers of smartphones, followed by the United States (> 235 million users).
If you search for technological advances that facilitated progress to the current state of cell phone technology, you will find lists that include the Internet, global positioning systems, touch screens, cameras, high-speed modems, displays, batteries, and a host of other materials and technologies. 8 , 9
The computers that drive recent smartphones have 64-bit architectures. 10 , 11 They are usually fabricated as a system-on-a-chip and include multiple cores and extra features, such as neural engines and embedded motion coprocessors. They contain cameras with more than 10 megapixels and multi-element lens systems and include zoom capabilities and two-axis stabilization. The phones support a wide variety of standard communication protocols, including accessibility features for those who wear hearing aids. Recent smartphone microprocessors have been built with fin field-effect transistors (finFETs) 12 manufactured at the 10 nm, 7 nm, and 5 nm processing scales. They also include a range of sensors, including for facial identification, a barometer, a three-axis gyro, an accelerometer, a proximity sensor, an ambient light sensor, a Hall sensor, and a RGB light sensor. 10 , 11
These systems are also designed to take advantage of fifth-generation (5G) cell phone networks with advantages in bandwidth and data rates (eventually up to 10 Gbps). 13
Integrating even a fraction of these capabilities into the early Motorola mobile phone would have likely expanded the size, weight, and power requirements well beyond what one person could have easily carried. (As I write this, an image of a famous body-builder, Arnold Schwarzenegger, struggling to lift this enhanced “brick” popped into my head, as he was trailed by a large generator on wheels to power the phone.) This does not factor in the fact that many of these technologies did not exist at the time.
Microelectronics has evolved through a range of technologies and materials developments over the years 14 , 15 that have affected transistors (bipolar junction transistors, various metal–oxide–semiconductor field-effect transistors, including finFETs), dielectrics (thermal oxides, high- k dielectrics), metallization (aluminum, polysilicon, copper, tungsten vias), high levels of integration, including multilayer metallization, and integration of billions of transistors per chip. Fabrication of modern microchips involves many hundreds of process steps that have to be performed within narrow tolerances. It is remarkable that these fabrication lines yield in numbers high enough to be economically viable. If any step falls outside of the tolerances, then yield can fall catastrophically. This would kick off an investigation to determine the root cause(s) of the problem and can shut down fabrication lines for long periods of time—an expensive proposition. Developing these technologies and the processes that allow them to be inserted into high-yield fabrication lines have occupied hordes of materials researchers for decades.
I could write similar discussions of materials advances in batteries, displays, touch screens, and camera systems that have relied on similar hordes of materials researchers. However, I’m out of space for this article, so those stories will have to wait until another time.
The features described, the ease of carrying modern cell phones, and their economic affordability are driving the surge in worldwide usage. Access to information is only as good as the information. We are constantly bombarded with inaccurate information as well as disinformation. Filtering all of that can be difficult and time consuming. Instantaneous access to information using cell phone and other electronic technologies provides the unwary with an opportunity to make huge mistakes quickly.
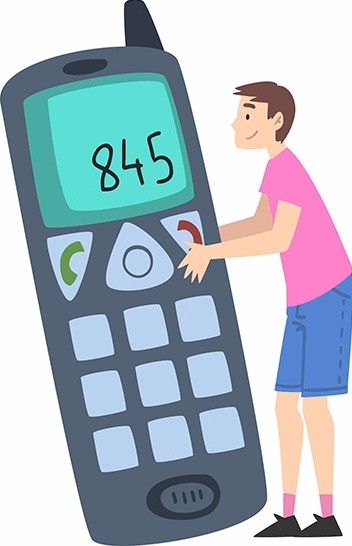
The use of landline phones reached a peak in the 2000s. Now they are down to around 40% of American households and declining. 16 I am one of those neo-Luddites who has chosen to keep my landline. I find that, for now, it gives me some comfort to have it available.
Warts and all, the proliferation of cell phone systems is good. Widespread outages due to local events are unlikely to destroy all cell towers in a local community. Therefore, people are likely to maintain some capability for communication, even if impacted by tornados such as the one on January 3, 1949, in Warren, Ark.
The invention of the telephone - Ericsson
https://www.statista.com/statistics/189959/housing-units-with-telephones-in-the-united-states-since-1920/
S. Beitler, A.R. Warren, Tornado destroys towns. GenDisasters (1949); http://www.gendisasters.com/arkansas/11233/warren-ar-tornado-destroys-towns-jan-1949
Operator (That's Not the Way It Feels) - Wikipedia
S. Wolpin, The first cellphone went on sale 30 years ago for $4,000. Mashable (2014); https://mashable.com/2014/03/13/first-cellphone-on-sale/
75% of the world's population owns a cell phone - PhoneArena
D. Metev, 39+ smartphone statistics you should know in 2020, Review 42 (2020); 39+ smartphone statistics you should know in 2020 (review42.com)
J. Gold, 11 tech breakthroughs that led to today’s smartphones. Computerworld (2017), https://www.computerworld.com/article/3222855/11-tech-breakthroughs-that-led-to-todays-smartphones.html
https://www.sciencemuseum.org.uk/objects-and-stories/computer-your-pocket-rise-smartphones
Apple iPhone 12 Pro Max - Full phone specifications ( gsmarena.com )
Specs | Samsung Galaxy S21 5G, S21+ 5G and S21 Ultra 5G | The Official Samsung Galaxy Site
https://en.wikipedia.org/wiki/FinFET#:~:text=A%20fin%20field%2Deffect%20transistor,forming%20a%20double%20gate%20structure
https://en.wikipedia.org/wiki/5G
B. Davari, R.H. Dennard, G.G. Shahidi, CMOS scaling for high performance and low power-the next ten years. Proc. IEEE 83 (4), 595 (1995)
Article Google Scholar
D.M. Fleetwood, Radiation effects in a post-Moore world. 2020 IEEE Nuclear & Space Radiation Effects Conference Short Course , K.F. Galloway, Ed. (2020), chapter I, pp. 1–81
E.K. Lieser, Study: only 40 percent of U.S. households have a landline. The National Interest (2020), https://nationalinterest.org/blog/buzz/study-only-40-percent-us-households-have-landline-135212#:~:text=Today%2C%20that%20figure%20has%20plummeted,households%20utilized%20only%20wireless%20service
Download references
Author information
Authors and affiliations.
Cambridge, UK
You can also search for this author in PubMed Google Scholar
Rights and permissions
Reprints and permissions
About this article
Moss, S. From “brick” to smartphone: the evolution of the mobile phone. MRS Bulletin 46 , 287–288 (2021). https://doi.org/10.1557/s43577-021-00067-7
Download citation
Published : 05 March 2021
Issue Date : March 2021
DOI : https://doi.org/10.1557/s43577-021-00067-7
Share this article
Anyone you share the following link with will be able to read this content:
Sorry, a shareable link is not currently available for this article.
Provided by the Springer Nature SharedIt content-sharing initiative
- Find a journal
- Publish with us
- Track your research
Thank you for visiting nature.com. You are using a browser version with limited support for CSS. To obtain the best experience, we recommend you use a more up to date browser (or turn off compatibility mode in Internet Explorer). In the meantime, to ensure continued support, we are displaying the site without styles and JavaScript.
- View all journals
- Explore content
- About the journal
- Publish with us
- Sign up for alerts
- Open access
- Published: 15 June 2022
Mobile phones are hazardous microbial platforms warranting robust public health and biosecurity protocols
- Matthew Olsen 1 ,
- Rania Nassar 3 , 4 ,
- Abiola Senok 3 ,
- Susan Moloney 1 , 8 ,
- Anna Lohning 1 ,
- Peter Jones 1 , 7 ,
- Gary Grant 5 ,
- Mark Morgan 1 ,
- Dinesh Palipana 6 ,
- Simon McKirdy 7 ,
- Rashed Alghafri 1 , 2 , 7 , 9 &
- Lotti Tajouri 1 , 2 , 7
Scientific Reports volume 12 , Article number: 10009 ( 2022 ) Cite this article
11k Accesses
9 Citations
649 Altmetric
Metrics details
- Public health
- Risk factors
Advancements in technology and communication have revolutionised the twenty-first century with the introduction of mobile phones and smartphones. These phones are known to be platforms harbouring microbes with recent research shedding light on the abundance and broad spectrum of organisms they harbour. Mobile phone use in the community and in professional sectors including health care settings is a potential source of microbial dissemination. To identify the diversity of microbial genetic signature present on mobile phones owned by hospital medical staff. Twenty-six mobile phones of health care staff were swabbed. DNA extraction for downstream next generation sequencing shotgun metagenomic microbial profiling was performed. Survey questionnaires were handed to the staff to collect information on mobile phone usage and users’ behaviours. Each of the 26 mobile phones of this study was contaminated with microbes with the detection of antibiotic resistance and virulent factors. Taken together the sum of microbes and genes added together across all 26 mobile phones totalised 11,163 organisms (5714 bacteria, 675 fungi, 93 protists, 228 viruses, 4453 bacteriophages) and 2096 genes coding for antibiotic resistance and virulent factors. The survey of medical staff showed that 46% (12/26) of the participants used their mobile phones in the bathroom. Mobile phones are vectors of microbes and can contribute to microbial dissemination and nosocomial diseases worldwide. As fomites, mobile phones that are not decontaminated may pose serious risks for public health and biosecurity.
Similar content being viewed by others

A pilot metagenomic study reveals that community derived mobile phones are reservoirs of viable pathogenic microbes
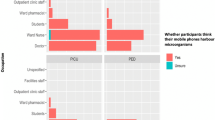
Mobile phones of paediatric hospital staff are never cleaned and commonly used in toilets with implications for healthcare nosocomial diseases
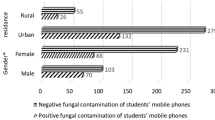
Fungal contamination of medical students’ mobile phones from the University of Belgrade, Serbia: a cross-sectional study
Introduction.
Mobile phones are ubiquitous and are used as primary communication devices. There are accounting for over 5 billion mobile phone users globally (over two-thirds of the world’s population) with an increase of 100 million unique mobile phone users each year 1 . According to Statista in 2020, the number of mobile phone users accessing popular messaging apps to communicate was 2.77 billion 2 . There have been many risks identified linked to the use of mobile phones including addiction 3 , vision impairment in children 4 , dangerous driving 5 , distracted pedestrians 6 , psychological stress and general anxiety 7 . Mobile phones are used up to 3 h and 37 min per person and touched with hands more than 2000 times a day 8 . A previously underestimated risk of mobile phones is associated with their role as fomite and several recent studies have confirmed the presence of viable microbes on their surface 9 , 10 . The United States Centre for Disease Control and Prevention (CDC) outlined that up to 80% of all infectious diseases was transmitted via hands 11 . Researchers have shown that mobile phones are reservoirs of microbes, users neglect and rarely decontaminate these devices, high rates of use and touch contact of mobile phone surfaces and individual’ tendencies to touch their face regularly (up to 23 times an hour) 12 or/and other surrounding surfaces 13 . Olsen et al. (2020) stated that mobile phones act as ‘Trojan horse’ devices which: (i) bypass gold standard hand hygiene practices; (ii) are likely linked to pathogen movement via cross-contamination transmission pathways during epidemics and pandemics 14 ; and (iii) contribute to global population infections and hospitalisations due to nosocomial infections. A recently published survey of 165 healthcare workers (HCW) demonstrated that 52% (86/165) of participants used their mobile phone in the bathroom/toilet and that 57% reported that they never wash their devices 15 . Mobile phones are platforms that host microbial vectors leading to the dissemination of infectious diseases. The use of phones by all professional sectors makes them ideal platform niches for micro-organism contamination 10 . Despite a massive increase in published articles describing the role of mobile phones as fomites there is still poor global awareness with continuing poor practices of standardised sanitisation. In 2020, a global scoping review of 56 studies identified that on average, 68% of mobile phones were contaminated with microbes with many harbouring antibiotic resistant bacteria 9 . While such scoping review was informative, microbial characterisation and identification from these studies most probably underestimated the overall spectrum and richness of microbes on mobile phones. These studies were based on traditional agar-based growths, biochemical testings or orthogonal polymerase chain reaction amplification of microbial genomic sequences 9 .
Improved methodology using a sequencing approach with 16S rRNA primers for metagenomic sequencing also highlighted the inadequacy of traditional culture-dependent identification techniques to capture the entire globality of microbes present on mobile phones 16 . A 2021 pilot next generation sequencing project was able to capture a wider population of micro-organisms with all mobile phones found to be contaminated with microbes. The findings consisted of 235 bacteria, 8 fungi, 8 protists and 53 bacteriophages reported from only five mobile phones derived swabs 14 . However, this study still could be considered as an underestimation of microbial finding as the samples were pre-cultured on agar plates prior to next generation sequencing metagenomic profiling 14 . A similar study used a metagenomic shotgun sequencing-based approach of viable pre-cultured microbes collected from 30 mobile phones of HCW. These phones were swabbed across 4 different hospital wards and plated on five different agar plates (Horse Blood agar, Nutrient agar, MacConkey agar, Bile Esculin agar, Mannitol Salt agar) before being subject to next generation sequencing 10 . The study identified a large range of microbial organisms with 399 operational taxonomic units (OTUs) bacteria, 155 bacteriophage OTUs and the identification of 134 antibiotic resistant genes (ARGs) and 347 virulence factor genes (VFGs).
To address the limitations identified in previous studies, this study collected swabs from mobile phones of health care staff working in a hospital. These swabs were subject to a direct shotgun next generation sequencing to identify the metagenomic presence of micro-organisms on these surfaces.
Participant recruitment and sample collection
Informed consent was obtained from all subjects of this study with a total of 26 health care workers from the Paediatric Intensive Care Unit (PICU) and the General Paediatric Department (GPD) of the Gold Coast University Hospital, Australia. An information sheet was provided to all participants, detailing the nature of the research, with no personal identifying information collected. Informed consent was provided verbally and agreeing to participate on the day of sampling. Samples were collected each of the 26 mobile phones using culture swab EZ II swabs (Becton Dickson) pre-moistened with sterile saline. During the sample collection phase, gloves were worn and changed between participants to prevent cross-contamination. The mobile phones were swabbed on both the front and back of the devices with swabs then placed in portable containers and transported immediately to the laboratory for processing.
Survey questionnaire
The complete survey data set has been published previously 15 , however, for this paper some results have been extracted to enable comparison with the microorganisms discovered. The 26 questionnaire survey responses were included in this paper.
Swab and DNA extraction
The preliminary step of the DNA extraction process involved the use of bead beating with 0.1 mm diameter glass beads (BioSpec Products, Bartlesville, OK USA) on a Powerlyser 24 homogenizer (Mo-Bio, Carlsbad, CA USA) at the Australian Centre for Ecogenomics (ACE), Brisbane, Australia. Briefly, samples were transferred to a bead tube and 800 µl of bead solution (Qiagen, Germantown, MD USA) was added and bead-beat for five minutes at 2000 rpm, then centrifuged at 10,000 g for one minute. Following the addition of 60 µl of cell lysis buffer, tubes were vortexed and then heated at 65 °C for 10 min (while mixing at 1000 rpm), then vortexed again for 30 s and stored at −20 °C pending DNA extraction. Prior to DNA extraction, samples were thawed at room temperature; vortexed and centrifuged for one minute at 10,000 g. The resulting lysate was transferred to a new collection tube and DNA extraction carried out using DNeasy Powersoil Kit (Qiagen), as per manufacturer protocol with a final elution volume of 50 µl using sterile, EDTA-free elution buffer.
Metagenomic sequencing and bioinformatic analysis
Libraries were prepared according to the manufacturer’s protocol using Nextera DNA Flex Library Preparation Kit (Illumina San Diego, CA USA). Preparation and bead clean-up were run on the Mantis Liquid Handler (Formulatrix) and Epmotion (Eppendorf) automated platform. On completion of the library prep protocol, each library was quantified, and quality control (QC) was performed using the Quant-iT™ dsDNA HS Assay Kit (Invitrogen, Carlsbad, CA USA) and Agilent D1000 HS tapes on the TapeStation 4200 (Agilent Technologies, Santa Clara, CA USA) as per manufacturer’s protocol. Library Pooling, QC and Loading Nextera DNA Flex libraries were pooled at equimolar amounts of 2 nM per library to create a sequencing pool. The library pool was quantified in triplicates using the Qubit™ dsDNA HS Assay Kit (Invitrogen). Sequencing was carried out on the NextSeq500 (Illumina) using NextSeq 500/550 High Output v2 2 × 150 bp paired end chemistry according to manufacturer’s protocol 12 . The post-sequencing derived raw data were retained and transferred into Illumina base space platform ( https://basespace.illumina.com ). Following the sequencing runs, data as demultiplexed FASTQ files were uploaded into CosmosID platform ( https://www.cosmosid.com/ ). Raw datasets Fastq files were analysed using the CosmosID software to identify bacteria, fungi, virulence factor genes and antibiotic resistance genes. The datasets were then analysed with proper mining bio-informatic analytic tools using high performance data-mining k-mer algorithm and highly dynamic comparator databases (GenBook®). Through this process, the raw data of millions of short reads can be distinctively aligned against sequences of microbial genomes and genes (CosmosID Metagenomics Cloud).
Microbial ‘Richness’ corresponds to the cumulative amount of all distinct microbes detected across all phones whereas the number of occurrences across all phones for each of these distinct microbes are represented by Hits.
Ethical approval was obtained from Bond University Human Research Ethics Committee (16,004) and the GCUH Human Research Ethics committee with Site Specific approval (GC HREA 46,569). All methods were performed in accordance with the relevant guidelines and regulations.
Participant features and questionnaire findings
In total, there were 26 health care workers who participated in this study: 16 nurses, 8 doctors, 1 outpatient clinical staff and 1 unspecified participant. 16 staff members were from the General Paediatric Department and 10 were from the Paediatric Intensive Care Unit. Majority of the participants (77%; N = 20/26) were completing their shift and whilst 23% (n/N = 6/26) commencing their shift. 77% (20/26) reported using their mobile phones at work with 88% (23/26) believing their mobile phones were essential tools for their job. 96% (25/26) of participants believed their mobile phones would harbour potentially pathogenic microorganisms. Concerning the hygiene habits associated with mobile phone use in the professional setting, 46% (12/26) of the participants had recently used their mobile phones in the bathroom. Of the medical staff using mobile phones in the bathroom, 58% (7/12) reported using their devices for social media access, 25% (3/12) did not specify the purpose of use and 16% (2/12) reported using their phone for work-related purposes. Over half of the participants (54%; n/N = 14/26) of participants had never cleaned their mobile phone. Of the 46% (12/26) of participants who had cleaned their mobile phones at some point, 25% (3/12) did so within the past year, 33% (4/12) did so within the past month, 16% (2/12) did so within the past week and 25% (3/12) did so within the past day. Of those who reported cleaning their phones, 41% (5/12) used an alcohol-based wipe and 33% (4/12) used a disinfectant spray.
Illumina derived next generation sequencing datasets
The average amount of sequencing reads per mobile phone was approximately 53 million reads. Sample 26 (NS313-110) contained the lowest (33 million) and sample 12 (NS250-72) the highest number of reads (156 million) respectively.
The sequencing fastq dataset files of all sequencing samples of this study are available and processed in the SRA database with the SRA BioProject accession number PRJNA828402 that can be available in Entrez ( https://www.ncbi.nlm.nih.gov/sra/PRJNA828402 ). Each detailed accession number of the 26 datasets generated and analysed during the current study are available in the NCBI repository, ( PRJNA828402—SRA—NCBI(nih.gov) ).”
Sequencing reads and metagenomic overview
A total of 11,163 microorganisms and 2096 genes coding for antibiotic and virulent factors were identified in this metagenomic shotgun next generation sequencing study. In total, there were 5714 bacteria, 675 fungi, 93 protists, 228 viruses, 4453 bacteriophages, 560 antibiotic resistant genes and 1 536 virulence factor genes identified across the 26 mobile phones from GPD and PICU (Table 1 ).
On average, mobile phones from the GPD contained higher amounts of pathogens and genes, compared to the phones sampled from PICU. Additionally, mobile phones of nurses contained in average a slightly higher number of microbes compared to doctors with 460.2 and 403.6 respectively. Across all 26 mobile phones, the average number of micro-organisms was calculated to be 429 with an average of 477.7 on the GPD phones and 361.6 in the PICU phones. Microbial numbers ranged from 138 to 669 per phone and genes (ARG and VRG) ranged from 7 to 144 per phone. (Table 1 ). Bacteria and bacteriophages represented the largest proportion of the microorganism distribution (Fig. 1 ).
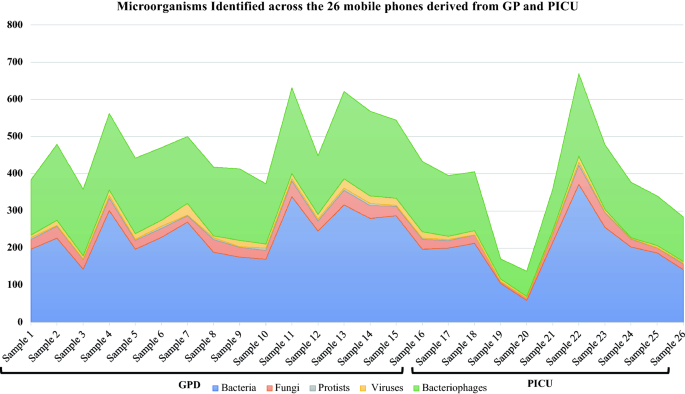
Distribution of different types of microorganisms across the 26 mobile phone samples.
Bacterial identification
1307 bacterial different strains were found with a richness across all 26 mobile phones accounting for 5714 hits. Clinically relevant species were found and include bacteria responsible for nosocomial diseases. 143 ‘ESKAPE’ type bacteria were found and consisted of Enterobacteriaceae: [46 hits on 19 phones (73%; 19/26) ], Staphylococcus aureus [25 hits; 25 phones (96%; 25/26)], Klebsiella pneumoniae [2 hits; 2 phones (7.7%; 2/26)], Acinetobacter baumannii [33 hits; 22 mobile phones (84.6%; 22/26)], Pseudomonas aeruginosa [21 hits, 21 mobile phones (80.8%; 21/26], Enterococcus faecalis / E. faecium [14 hits; with 50% of all 26 phones contaminated]. Of note, different strains of Pseudomonas and Acinetobacter species accounted for 187 and 205 richness hits respectively across the 26 mobile phones.
Additionally, community-acquired pathogenic HACEK group gram-negative bacteria accounted for 180 richness hits across the mobile phones swabbed. The highest hits were attributed to Haemophilus spp, and Aggregatibacter spp with 110 and 38 hits respectively while Cardiobacterium hominis , Eikenella corrodens , and Kingella spp corresponded to 14, 12 and 6 hits respectively. Every single phone swab harboured at least one Haemophilus spp.
Coagulase negative staphylococci (CONS) was found on all the mobile phones accounting for a total of 272 richness hits. All phones within that study harboured CONS with S. epidermidis , S. hominis , S. warneri ., S. haemolyticus. S. lugdunensis was identified on 92% (24/26) of mobile phones. While S. capitis and S. pasteuri in 88% and 81% of phones respectively.
Neisseria spp were identified with 152 richness hits. N. flavescens , N. subflava , N. elongate, N. sicca , and N. mucosa were the most represented with 21, 16, 16, 16 and 14 hits respectively. Noteworthy, N. meningitidis were present on 27% of phones (7/26) and N. gonorrhoeae was retrieved from one phone.
Streptococci strains accounted for 404 richness hits across the 26 mobile phones and included S. thermophilus , S. sanguninis , S. parasanguinis , S. salivarius , S. pseudopnemoniae , S. oralis , S. mitis , S. intermedius, S. infantis , S. infantarius , S. cristatus , S. australis , S. anginosus , and S. agalactiae . S. pneumoniae was found on the surface of 81% of the mobile phones (21/26) (Fig. 2 ).
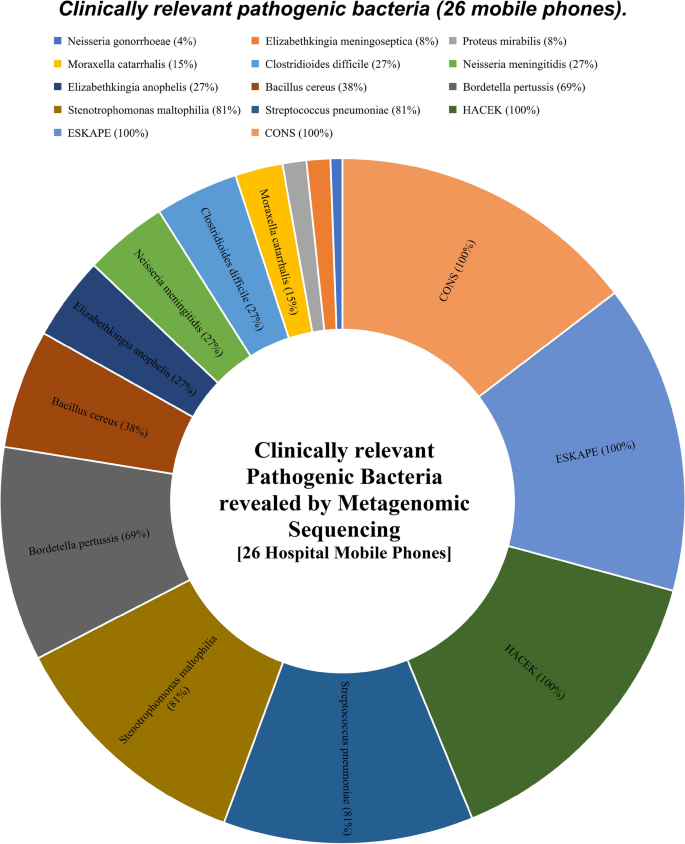
Clinically relevant pathogenic bacteria identified across all 26 mobile phones.
Mobile phones microbial composition varied with a subset of microbes uniquely present in either department: 170 and 317 bacteria in PICU and GPD respectively. These unique ward bacterial signatures showed different bacterial phylum profiles with the bacterial Actinobacteria phylum demonstrating the larger signature subset of PICU derived mobile phones while Bacteroidetes , Firmicutes , and Proteobacteria phylum were predominant in GPD derived devices (Fig. 3 ).
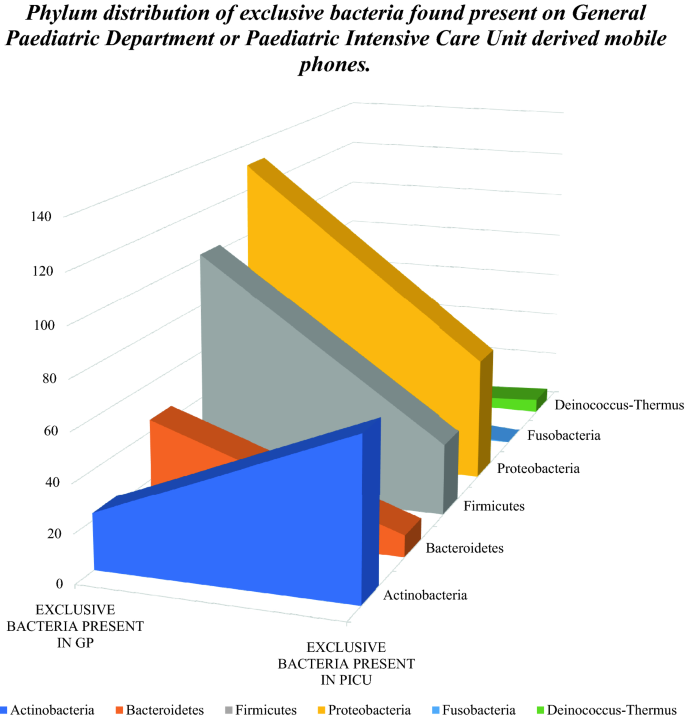
Phylum distribution of exclusive bacteria found present on General Paediatric Department or Paediatric Intensive Care Unit derived mobile phones.
Bacteriophage identification
In total there were 512 different bacteriophage viruses accounting for 4453 hits. Figure 4 illustrates the various bacteriophages identified from mobile phones of the GPD and PICU hospital departments. The highest hits corresponded to Propionibacterium virus , Streptococcus virus , Lactococcus virus , Staphylococcus virus, Pseudomonas virus with 29% (1 283/4 453), ~ 17.5% (777/4 453), ~ 17% (755/4 453), ~ 14.5% (646/4 453), ~ 3% (128/4 453) respectively (Fig. 4 ).
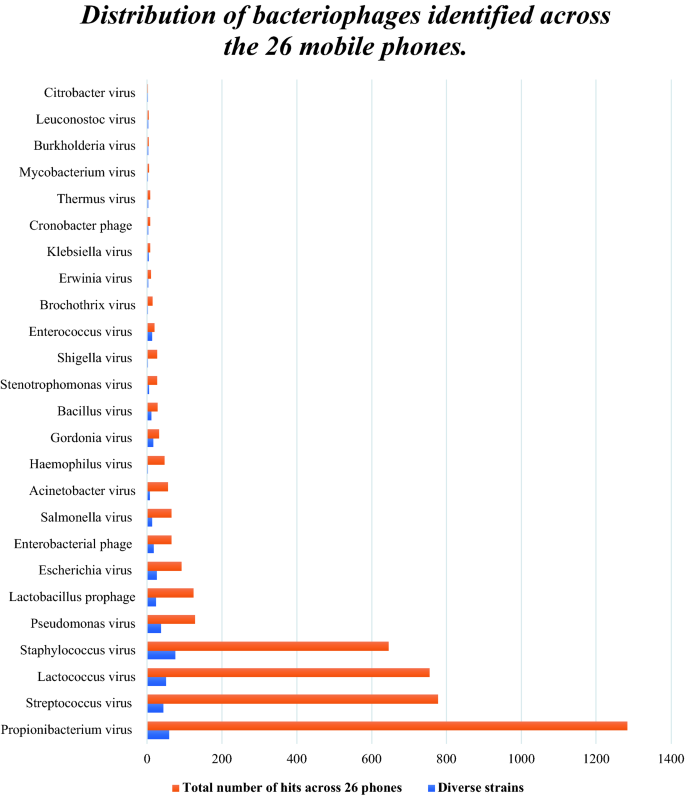
Distribution of bacteriophages identified across the 26 mobile phones.
A significant difference in the number of bacteriophages was observed between the two wards (GPD and PICU) ( P -value: 0.0022) (Wilcoxon Rank Sum Test) (Fig. 5 ).
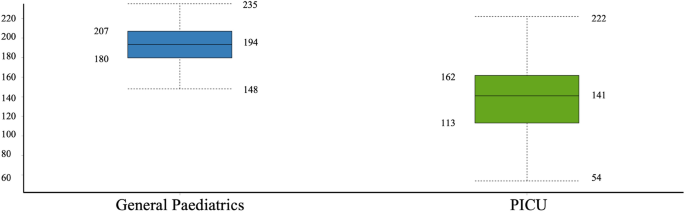
Boxplot of bacteriophages in GPD versus PICU wards (CHAO1 representation).
Viral identification
Sixty-seven different viruses accounting for 228 richness hits was found on the mobile phones. Seven different human herpes viruses (HHV) were identified and corresponded to a total richness of 29 hits. 15 phones had at least one HHV and in one phone alone 5 HHVs could be retrieved [ Herpes Simplex virus 1 , Epstein bar Virus , cytomegalovirus , Roseolovirus 6 and 7 ]. Twenty-nine different strains of Human papillomavirus were found which corresponded to 95 total hit richness across the mobile phones swabbed in this experiment. Seven pathogenic Human Papilloma Viruses (HPVs) (24%;7/29) were present and these accounted for 45% (43/95 hits) of all the 95 HPV hits. Of note, one phone alone had 5 pathogenic HPVs (HPV-3, -4, -5, -9 and -49). Polyomaviruses such as the Human polyomavirus 6 , MW and STL polyomavirus were identified. Noteworthy, the Merkel cell polyomavirus was retrieved on six mobile phones.
Protist identification
12 different protists were found representing 93 total hits. Figure 6 highlights the range of protozoa identified with several amoebae of the protozoal group Sarcodina with Acanthamoeba polyphaga , Acanthamoeba palestinensis , Naegleria fowleri , Entamoeba dispar , Entamoeba histolytica (Fig. 6 ).
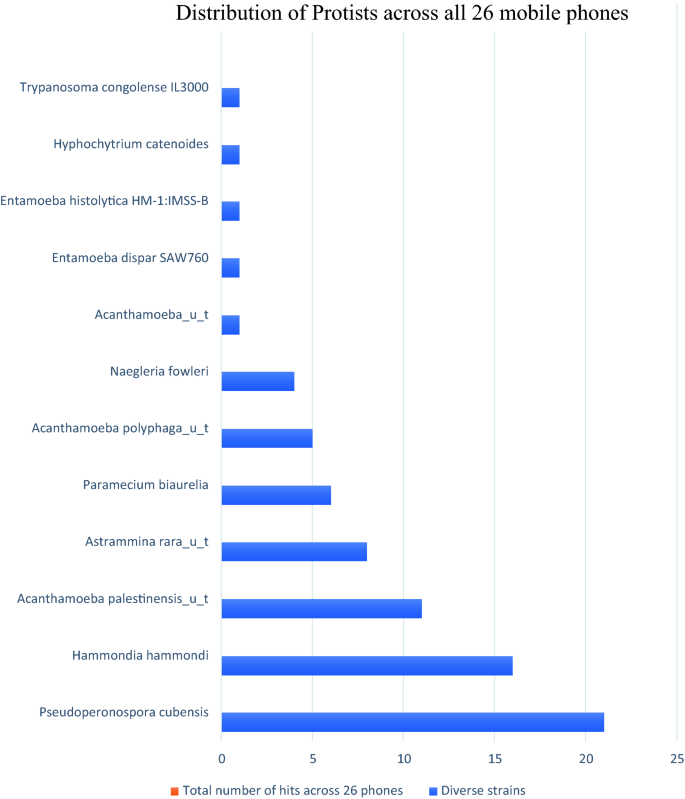
Distribution of protists identified across 26 mobile phones.
Resistome and virulome
Antibiotic resistance genes.
The metagenomic analysis revealed the presence of 134 different (distinct) antibiotic resistance genes with a cumulative richness number across all the mobile phones of 560 ARGs. Figure 7 represents the distribution of grouped antibiotic resistant genes. Resistance genes to Macrolides (19 genes), beta-lactams (32 genes), aminoglycosides (26 genes), and tetracycline (13 genes) corresponded to richness hits of 167, 98, 97 and 50 respectively (Fig. 7 ). Multi-type of antibiotics was targeted by efflux pumps (17 genes) and pump-regulator genes (13 genes) which together accounted for 89 richness hits. Less richness was found for other antibiotics resistance genes acting on bacterial metabolism (sul2 gene acting on Sulphonamides; dfrC and dfrG genes acting on Trimethoprim), on cell wall (PBP1b/2b and vanXY genes acting on transpeptidases and vancomycin), on bacterial DNA (norA, oqxA, bleomycin binding protein genes) and on protein translation [genes like cmx, dha1, cm acting on phenicols; fusC gene acting on the bacterial elongation factor (EF)] Fig. 8 (and Supplementary Fig. 1 ).
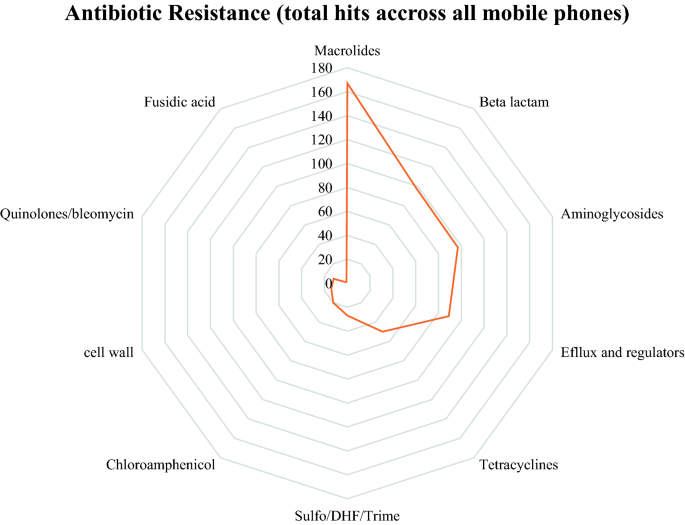
Antibiotic Resistant gene distribution across all wards of 26 mobile phones.
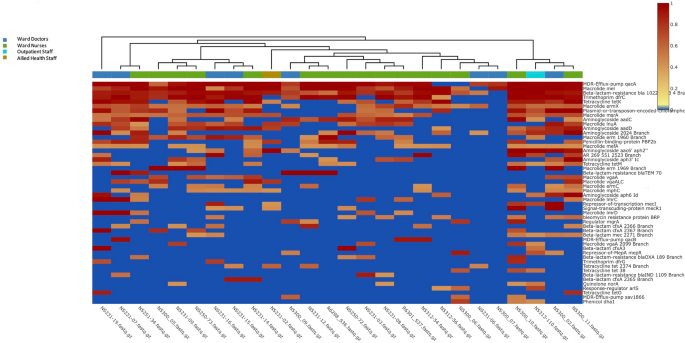
Heatmap representation of antibiotic resistant genes found on mobile phones owned by health care staff (heatmap clustered by staff occupation).
Virulence factor genes (VFGs)
Across the mobile phones swabbed, this study identified 419 different (distinct) virulent factor genes with 1536 hits. 35% of all these hits (552/1536) were attributed to 28 different VFGs genes that were all in at least 50% of mobile phones and included Klebsiella pneumoniae GENE tnpA, Proteus mirabilis GENE tnpA, Enterococcus faecalis GENE repB & GENE mob, Enterococcus faecium GENE ermB, Streptococcus pyogenes GENE msrD, Staphylococcus epidermidis GeneID SEA1545, Staphylococcus lentus GENE tetK & GENE repL & GENE repC & GENE pre & GENE ermC, Staphylococcus aureus GENE qacC & GENE dfrA & GENE blaZ & GENE blaR1 & GENE blaI & GENE thyA (Fig. 9 and Supplementary Fig. 2 ).
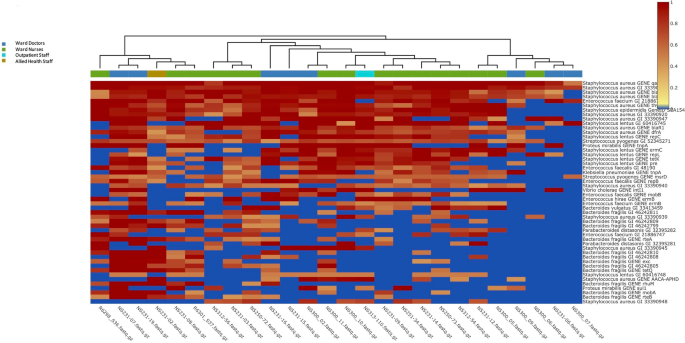
Heatmap representation by healthcare occupation of the 419 distinct virulence factor genes identified on mobile phones by means of metagenomic analysis.
This study performed metagenomic profiling of swabs derived from 26 mobile phones of health care workers, predominantly doctors and nurses, in a Paediatric Intensive Care Unit and a General Paediatric Department). Alongside the shotgun next generation sequencing experimentation, a questionnaire was competed by all participants. Results showed that all phones were contaminated with microbes including bacteria, viruses, fungi and protozoa. The average microbial burden on the mobile phone showed that phones derived from GPD had the greatest number of bacteria, fungi, viruses and protists with 235, 29, 15 and 4 micro-organism respectively. Mobile phones from the PICU harboured on average 195 bacteria, 22 fungi, 7 viruses. Interestingly average number of bacteriophages were also more common on mobile phones from the GPD versus PICU with 194 and 141 respectively (Fig. 5 ). This ward microbial burden difference was observed in both nursing and medical staff. The reduction of mobile phone microbial burden in PICU might be associated with higher frequency of hand hygiene practices or more stringent infection control measures. Interestingly, the average number of microbes irrespective of the ward was always higher in mobile phones owned by nurses than doctors with the exception of fungi and protists that were found in higher number on doctor phones from the GPD. Additionally, mobile phones of the doctors from the GPD had a higher number of antibiotic resistant and virulent factor genes than those of nurses. However, in PICU, nurses’ mobile phones had a higher number of antibiotic resistant and virulent factor genes compared to doctors within that department. Overall, the microbial load on phones from both departments was at levels that should be considered problematic. For bacteria alone, this metagenomic analysis identified 1307 different strains accounting for 5714 hits from 26 mobile phones. Well-known nosocomial organisms including HACEK bacteria causing endocarditis [ Haemophilus spp, Aggregatibacter spp Cardiobacterium hominis , Eikenella corrodens , and Kingella spp] and ESKAPE type bacteria [Enterobacteriaceae, Staphylococcus aureus , Klebsiella pneumoniae, Acinetobacter baumannii , Pseudomonas aeruginosa and Enterococcus faecalis / E. faecium organisms] were present on all 26 mobile phones swabbed in this study. This study has identified a serious general hospital infection control concern that may escalate to future public health threats.
The study also identified other microbial presence on mobile phones that raises concerns. Clinically relevant pathogens such as Bordetella pertussis , responsible for whooping cough was present on 69% of all phones studied, Streptococcus pneumoniae and the emergent nosocomial bacteria Stenotrophomonas maltophilia were each present on 81% of all phones studied (21/26).
Food borne bacteria ( Bacillus cereus ) was identified on HCW mobile phones. While this study was done in a hospital setting, it confirms that other industries such as the food industry are also at risk of microbial cross contamination from mobile phones. Other concerning organisms including Clostridioides difficile, Moraxella catarrhalis, Proteus mirabilis, Elizabethkingia meningoseptica and the sexually transmitted infectious bacteria Neisseria gonorrhoeae were identified on phones in this study. Clostridioides difficile infections has been shown to spread from contaminated surfaces with the risk of infection higher when using bathrooms preceded by infected individuals 17 . Finding HCW mobile phones to be microbial laden fomites possibly confers appropriate conditions to disseminate infections to susceptible hosts and immune-compromised patients and is a real public health risk. One example is finding Elizabethkingi a meningoseptica , a nosocomial bacterium that has disastrous consequences on premature babies with known past outbreaks linked to phone receivers 18 .
Human behaviours and the constant contact with mobile phones in toilets provide cumulative evidence that such devices are exposed to unsanitary conditions leading to the presence of a range of viable microbes on these platforms. Based on this study and others, it appears mobile phones are rarely or ever cleaned and even when cleaned this may occur in an ineffective manner. Mobile phones act as fomites turning these devices into ideal platforms for disease transmission either by means of self-inoculation when touching your own mobile phone and face orby simple microbial dissemination in the environment, public places, or professional sectors.
Bacteriophages were also found in association with bacteria with 512 different phages found and accounting for 4453 hits across 26 HCW mobile phones. Additionally, 67 different viruses including animal and human viruses were detected. These consisted of seven different human herpes viruses with 15 phones found with at least one HHV and one phone harboured up to 5 HHVs. including Herpes Simplex virus 1 , Epstein bar Virus , cytomegalovirus and Roseolovirus 6 and 7 . 29 different strains of Human papillomavirus were found with seven clinically important pathogenic HPVs and Merkel cell polyomavirus responsible for a rare but highly aggressive form of cancer was retrieved on six mobile phones from HCW suggesting a role for transfer of significant viral infections from mobile phones.
This study has also highlighted the risk posed by the presence of a large profile of antimicrobial drug resistome and pathogenic virulome on the surface of mobile phones. The bacterial resistome found in the study showed antibiotic resistant genes that counteract with all antibiotic modes of action on bacteria. Antibiotics normally actively targeting bacterial cell wall, cell membrane, cellular metabolism, DNA transcription & replication and protein translational synthesis may be impacted by the expression of these antibiotic resistance genes. Of note, 17 genes coding for drug efflux pumps were found in this study demonstrating that the resistome capacity of bacteria present on mobile phones is equipped with sophisticated expulsion processes protecting them from ‘undesirable’ antibiotics.
Along with the antibiotic resistome profile, the bacteria found on mobile phones show strong virulence capacity with 419 different virulent factor identified genes (1536 hits across all 26 mobile phones). High amount of VFGs were the signature of Klebsiella pneumoniae , Proteus mirabilis , Enterococcus faecalis , Enterococcus faecium , Streptococcus pyogenes , Staphylococcus epidermidis , Staphylococcus lentus , Staphylococcus aureus .
In hospitals, it is now commonplace for mobile phones to be used by the majority of HCW, they may however be counteracting the World Health Organisation hand hygiene campaigns. The efforts to limit exposure of microbes to patients may be nullified if mobile phones are not decontaminated regularly 19 . The number of microbes identified on phones does suggest that new measures of infection control in these vulnerable areas should be implemented. This should include mobile phone sanitisation as a corollary to the Five Moments of Hand Hygiene 20 . Mobile phones should now be considered as the ‘third hand’ from their users and subject to frequent decontaminations in hospitals (both health care staff and patients/visitors). An infographic shows the dissemination route of microbes derived from healthcare staff users and users of the community (Fig. 10 ).
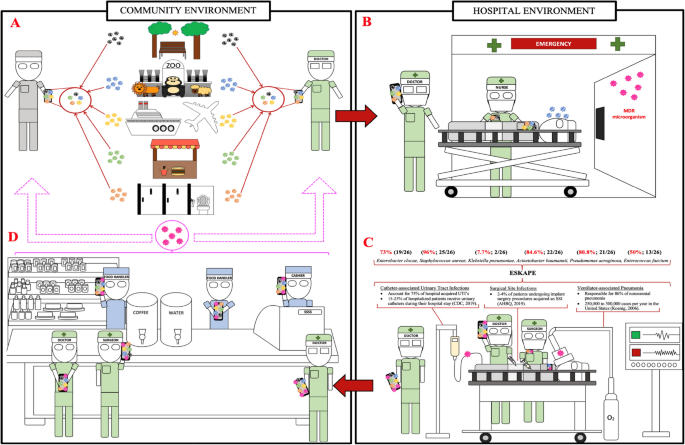
Contaminated mobile phones potential vectors of dissemination of germs in and out healthcare and community settings.
Figure 10 illustrates the transmission dynamics of microbes derived from mobile phones and the possible inter-related dissemination of germs in and out healthcare and community settings. A. Mobile phones exposed to all sorts of community environments will harbour diverse microbes from the user’s hands. These organisms may persist on the surface of mobile phones and be a source of further downstream dissemination in other areas.
B. illustrates a patient of the community admitted at the hospital and both healthcare and patient’s mobile phones are contaminated. Germs in pink represent multi drug resistant nosocomial pathogens in hospitals.
C. on duty medical staff with their (non-sanitised) mobile phones might be the cause of nosocomial diseases contracted by vulnerable immuno-compromised patients during various procedures (ventilators, catheters, injections, open wound surgery.).
D. While nosocomial pathogenic and resistant microbes are present in hospitals, health care workers on duty might acquire such pathogens on the surface of their phones. At lunch or at the end of their shift medical professionals may disseminate these pathogens in the community.
Author’s recommendation
This direct swab to metagenomic analysis study has revealed that hospital derived mobile phones used by health care workers, are accommodating niches for large amount of diverse pathogenic germs that are equipped with an arsenal of virulence genes and large spectrum of antibiotic resistance.
While this study took place in a hospital, the research highlights the need for the scientific community and public health authorities to further investigate the role mobile phones play as fomites. The potential for them to be vehicles for transmission and propagation of infectious microbes across health care settings needs to be addressed. Additionally, mobile phones harbouring a plethora of viable microbes are in circulation, with billions currently owned globally, and may be the means to establish, maintain or spread epidemics and pandemics. As an example, SARS-CoV-2 was detected on mobile phones and shown to survive on such platforms up to 28 days 21 . Undetected introductions of biothreats and invasive pathological organisms might be due to the billions of passengers travelling around the globe with ‘uncleaned’ mobile phones. Presence of SARS-CoV-2 omicron or delta variants on mobile phones need to be investigated.
Additionally, this research emphasises that the density of microbes found on mobile phones may be the ideal platforms for horizontal genetic transfers to occur among different species of micro-organisms such as transformation, conjugation, and transduction. Mobile phones may act as platforms for microbial multiplication and as a dynamic training ‘school’ for superbugs to evolve (and disseminate).
Mobile phones are dynamically contaminated with all sorts of microbes touched by the hands of their users thousands of times a day, even while in bathrooms. Mobile phones therefore have become our third hand. They are ‘dirty’ as are infrequently cleaned/sanitised and are completely negating first the worldwide gold standard hygienic hand washing practices and secondly the cost-effective public health and biosecurity prophylactic measures. Mitigation resides in sanitising mobile phones as frequently as we wash our hands with the adoption of new technology driven solution a like safety-certified enclosed ultraviolet-C emitting mobile phone sanitisers to clean phones in 10–20 s. This fast and efficient technology driven sanitisation of phones is practical as could be performed while health care workers practise hand hygiene. Presence in healthcare facilities of stations that can decontaminate both hands and mobile phones will prevent the risks of cross contamination and should be implemented in the five moments of hand washing.
It also sends a strong message to the general community to prevent further global microbial dissemination. These metagenomics analysis findings revealed a real biosecurity concern with possible economically important disease repercussions that authorities must take seriously. Not only were some microbes on mobile phones highly resistant to multiple antibiotics, but cancer related viruses such as herpes viruses, polyomaviruses and human papillomaviruses are also of high concern for public health if mobile phones are not decontaminated in a daily basis. With 134 different antibiotic resistance genes and 419 different virulent factor genes found across all 26 mobile phones, the United Nation sustainable development goal number #3 ‘Good health and well-being’, is in peril. SDG#3 will undoubtfully fail to reach that goal by 2030 because of multiple factors that include: (i) a discovery void era of new antibiotics, (ii) paucity of research for alternative antimicrobial solutions and (iii) ‘third hand’ microbial laden mobile phones with multi drug resistant superbugs 22 . Hundreds of trillions of micro-organisms on the surface of billions of mobile phone fomites cross borders, by means of modern transport, un-noticed as Trojan horses. Custom security officers are not aware nor trained to prevent and stop the entry of these viable germs present on mobile phone. No measures or regulations exist in our hospitals or in our airports to decontaminate these mobile ‘petri-dishes harbouring in total impunity an array of pathogens. In the hands of billions of people mobile phones enter our health care settings, land in our countries and act as vectors to disseminating germs in all corners of the globe. Public Health and Biosecurity authorities should work ‘hands in hands’ to stop this silent ‘third hand’ driven pandemic and implement urgently regulations to actively decontaminate mobile phones as niches and reservoirs of viable microbes. The consequences for national and global biosecurity are outlined in Fig. 11 .
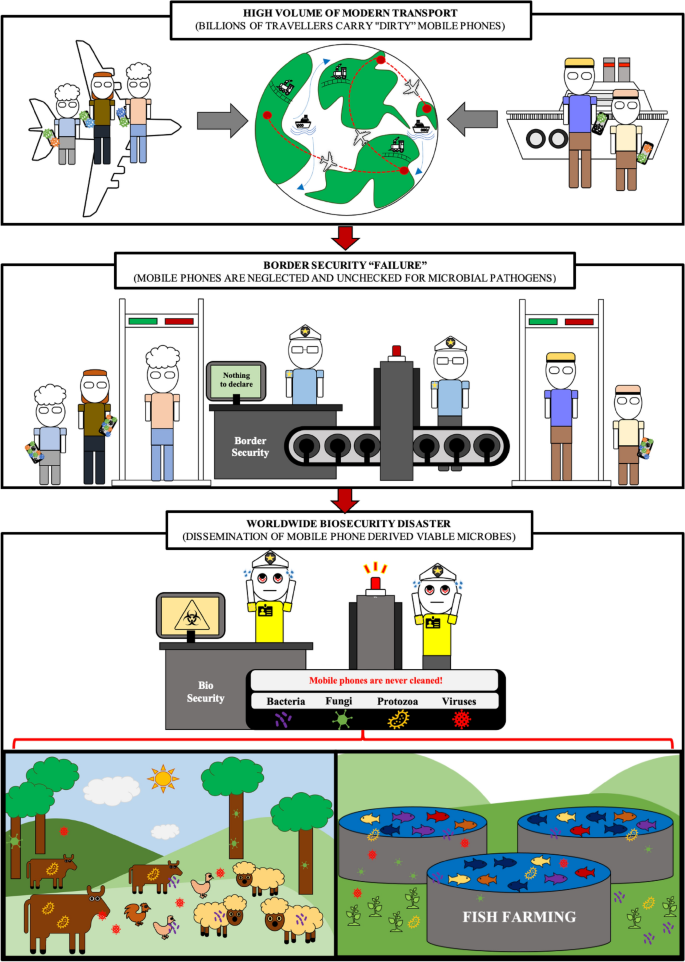
Mobile phone contaminated with microbes pose national and global biosecurity threats.
Figure 11 Passengers of modern transport are per billions and carry with them billions of phones. While traveling around the globe, passengers returning home or in holiday trips pass through the customs without officer’s awareness that mobile phones carry all sorts of pathogens (viruses, bacteria, fungi, and protists) and proper sanitisation logistics to clean phones. The entry of billions of pathogens (including probably hard to control invasive germs) pass borders un-noticed and enter countries every day of the year. Downstream repercussions of un-controlled passage of all these viable microbes by means of trojan horse mobile phones are yet to be quantified in terms of economic losses due to inadequate biosecurity measures to decontaminate mobile phones at borders. Impacts on agriculture, native flora, marine fauna and native fauna as well as all livestock and aquatic farms from these invasive biothreats may be astronomical but yet not considered a national biosecurity priority.
Supplementary data
Complete and extended of Figs. 8 and 9 heatmaps are available online as supplementary datasets (Supplementary Figs. 1 and 2).
Additionally, an excel sheet entitled “Supplementary data_Olsen et al-2022_Hits_abundance across all 26 samples“ is also available online as a supplementary data. That supplementary information provides details regarding the taxonomy Ids and abundance of microbes and genes found present or absent across the 26 samples investigated in that study.
Data availability
The sequencing fastq dataset files of all sequencing samples of this study are available and processed in the SRA database with the SRA BioProject accession number PRJNA828402 that can be available in Entrez ( https://www.ncbi.nlm.nih.gov/sra/PRJNA828402 ). Each detailed accession number of the 26 datasets generated and analysed during the current study are available in the NCBI repository, PRJNA828402—SRA—NCBI(nih.gov) .”
Kemp, S. Digital 2020: October global statshot . Datareportal: Kepios Analysis https://datareportal.com/reports/digital-2020-october-global-statshot (2020).
Statista Research Department. Number of mobile phone messaging app users worldwide from 2018 to 2022 (in billions) . https://www.statista.com/statistics/483255/number-of-mobile-messaging-users-worldwide/ (2021).
Shoukat, S. Cell phone addiction and psychological and physiological health in adolescents. EXCLI J. 18 , 47–50 (2019).
PubMed PubMed Central Google Scholar
Wang, J., Li, M., Zhu, D. & Cao, Y. Smartphone overuse and visual impairment in children and young adults: Systematic review and meta-analysis. J. Med. Internet Res. 22 (12), e21923 (2020).
Article Google Scholar
Zatezalo, N., Erdogan, M. & Green, R. Road traffic injuries and fatalities among drivers distracted by mobile devices. J. Emerg. Trauma Shock 11 (3), 175–182 (2018).
Mikusova, M., Wachnicka, J. & Zukowska, J. Research on the use of mobile devices and headphones on pedestrian crossings—Pilot case study from Slovakia. Safety 7 (1), 17 (2021).
Thomée, S. Mobile phone use and mental health. A review of the research that takes a psychological perspective on exposure. Int. J. Environ. Res. Public health 15 (12), 2692 (2018).
Winnick M. Putting a Finger on Our Phone Obsession . Descout report https://blog.dscout.com/mobile-touches (2016).
Olsen, M. et al. Mobile phones represent a pathway for microbial transmission: A scoping review. Travel Med. Infect. Dis. 35 , 101704 (2020).
Tajouri, L. et al. The role of mobile phones as a possible pathway for pathogen movement, a cross-sectional microbial analysis. Travel Med. Infect. Dis. 43 , 102095. https://doi.org/10.1016/j.tmaid.2021.102095 (2021).
Article PubMed Google Scholar
McCalla, S., Thomas, R. & Reilly, M. Hand hygiene compliance: “Going Back to Basics”. Am. J. Infect. Control 42 (6), S134 (2014).
Kwok, Y. L. A., Gralton, J. & McLaws, M. Face touching: A frequent habit that has implications for hand hygiene. Am. J. Infect. Control 43 (2), 112–114 (2015).
Zhang, N., Li, Y. & Huang, H. Surface touch and its network growth in a graduate student office. Indoor Air 28 (6), 963–972 (2018).
Olsen, M. et al. A pilot metagenomic study reveals that community derived mobile phones are reservoirs of viable pathogenic microbes. Sci. Rep. 11 , 14102. https://doi.org/10.1038/s41598-021-93622-w (2021).
Article ADS CAS PubMed PubMed Central Google Scholar
Olsen, M. et al. Mobile phones of paediatric hospital staff are never cleaned and commonly used in toilets with implications for healthcare nosocomial diseases. Sci. Rep. 11 , 12999. https://doi.org/10.1038/s41598-021-92360-3 (2021).
Simmonds, R. & Hayhust, E. Mobile phone contamination in clinical environments ‘The True Extent’. Access Microbial. https://doi.org/10.1099/acmi.fis2019.po0001 (2020).
Australian Guidelines for the Prevention and Control of Infection in Healthcare, Canberra: National Health and Medical Research Council (2019).
Ceyhan, M. et al. A Chryseobacterium meningosepticum outbreak observed in 3 clusters involving both neonatal and non-neonatal pediatric patients. Am. J Infect. Control 36 (6), 453–457 (2008).
Beuvink, Y. & Hackett, S. Are infection control measures helpful in reducing paediatric ward infections?. Paediatr. Child Health 28 (6), 282–288 (2018).
World Health Organization. Hand hygiene in outpatient and home-based care and long-term care facilities: a guide to the application of the WHO multimodal hand hygiene improvement strategy and the “My Five Moments For Hand Hygiene” approach. (2012).
Riddell, S., Goldie, S., Hill, A., Eagles, D. & Drew, T. W. The effect of temperature on persistence of SARS-CoV-2 on common surfaces. Virol. J. 17 (1), 1–145 (2020).
Boucherabine, S. et al. Metagenomic sequencing and reverse transcriptase PCR reveal that mobile phones and environmental surfaces are reservoirs of multidrug-resistant superbugs and SARS-CoV-2. Front. Cell. Infect. Microbiol. 12 , 806077 (2022).
Download references
Acknowledgements
Funding for the DNA sequencing was made available thanks to a consultation research-based account owned by LT and administered at Bond University.
Author information
Authors and affiliations.
Faculty of Health Sciences and Medicine, Genomics and Molecular Biology, Bond University, Robina, Gold Coast, QLD, 4229, Australia
Matthew Olsen, Susan Moloney, Anna Lohning, Peter Jones, Mark Morgan, Rashed Alghafri & Lotti Tajouri
Dubai Police Scientists Council, Dubai Police, Dubai, UAE
Rashed Alghafri & Lotti Tajouri
Mohammed Bin Rashid University of Medicine and Health Sciences, Dubai, UAE
Rania Nassar & Abiola Senok
Oral and Biomedical Sciences, School of Dentistry, College of Biomedical and Life Sciences, Cardiff University, Cardiff, UK
Rania Nassar
School of Pharmacy and Medical Sciences, Griffith University, Gold Coast, Australia
School of Medicine Gold Coast, Griffith University, Gold Coast, Australia
Dinesh Palipana
Harry Butler Institute, Murdoch University, Murdoch, WA, 6150, Australia
Peter Jones, Simon McKirdy, Rashed Alghafri & Lotti Tajouri
Department of Paediatrics, Gold Coast University Hospital, Southport, Australia
Susan Moloney
General Department of Forensic Sciences and Criminology, Dubai Police, Dubai, UAE
Rashed Alghafri
You can also search for this author in PubMed Google Scholar
Contributions
M.O., L.T. and R.A. wrote the main manuscript. M.O. and L.T. prepared all figures. M.O, L.T., S.M. and P.J. processed the samples. All authors reviewed the manuscript. S.M., R.A. and L.T. are the chief investigators.
Corresponding author
Correspondence to Lotti Tajouri .
Ethics declarations
Competing interests.
The authors declare no competing interests.
Additional information
Publisher's note.
Springer Nature remains neutral with regard to jurisdictional claims in published maps and institutional affiliations.
Supplementary Information
Supplementary information 1., supplementary information 2., supplementary information 3., rights and permissions.
Open Access This article is licensed under a Creative Commons Attribution 4.0 International License, which permits use, sharing, adaptation, distribution and reproduction in any medium or format, as long as you give appropriate credit to the original author(s) and the source, provide a link to the Creative Commons licence, and indicate if changes were made. The images or other third party material in this article are included in the article's Creative Commons licence, unless indicated otherwise in a credit line to the material. If material is not included in the article's Creative Commons licence and your intended use is not permitted by statutory regulation or exceeds the permitted use, you will need to obtain permission directly from the copyright holder. To view a copy of this licence, visit http://creativecommons.org/licenses/by/4.0/ .
Reprints and permissions
About this article
Cite this article.
Olsen, M., Nassar, R., Senok, A. et al. Mobile phones are hazardous microbial platforms warranting robust public health and biosecurity protocols. Sci Rep 12 , 10009 (2022). https://doi.org/10.1038/s41598-022-14118-9
Download citation
Received : 29 November 2021
Accepted : 01 June 2022
Published : 15 June 2022
DOI : https://doi.org/10.1038/s41598-022-14118-9
Share this article
Anyone you share the following link with will be able to read this content:
Sorry, a shareable link is not currently available for this article.
Provided by the Springer Nature SharedIt content-sharing initiative
By submitting a comment you agree to abide by our Terms and Community Guidelines . If you find something abusive or that does not comply with our terms or guidelines please flag it as inappropriate.
Quick links
- Explore articles by subject
- Guide to authors
- Editorial policies
Sign up for the Nature Briefing newsletter — what matters in science, free to your inbox daily.


An official website of the United States government
The .gov means it’s official. Federal government websites often end in .gov or .mil. Before sharing sensitive information, make sure you’re on a federal government site.
The site is secure. The https:// ensures that you are connecting to the official website and that any information you provide is encrypted and transmitted securely.
- Publications
- Account settings
- My Bibliography
- Collections
- Citation manager
Save citation to file
Email citation, add to collections.
- Create a new collection
- Add to an existing collection
Add to My Bibliography
Your saved search, create a file for external citation management software, your rss feed.
- Search in PubMed
- Search in NLM Catalog
- Add to Search
Healthcare workers' informal uses of mobile phones and other mobile devices to support their work: a qualitative evidence synthesis
Affiliations.
- 1 Department of Health and Functioning, Western Norway University of Applied Sciences, Bergen, Norway.
- 2 Department of Health Sciences, Oslo Metropolitan University, Oslo, Norway.
- 3 Department of International Health, Johns Hopkins Bloomberg School of Public Health, Baltimore, Maryland, USA.
- 4 Center for Global Digital Health Innovation, Johns Hopkins University, Baltimore, USA.
- 5 Global Health Cluster, Norwegian Institute of Public Health, Oslo, Norway.
- 6 Child Health and Development Centre, Makerere University, Kampala, Uganda.
- 7 Department of Information Systems, School of Computing and Informatics Technology, Makerere University, Kampala, Uganda.
- 8 School of Medicine, Makerere University College of Health Sciences, Kampala, Uganda.
- 9 College of Health Sciences, Makerere University, Kampala, Uganda.
- 10 College of Education and External Studies, Department of Adult and Community Education, Makerere University, Kampala, Uganda.
- 11 Institute of Health and Society, University of Oslo, Oslo, Norway.
- 12 Centre for Development and the Environment, University of Oslo, Oslo, Norway.
- 13 Healthcare Information For All, Chipping Norton, UK.
- 14 Department of Science, Information and Dissemination, WHO Regional Office for the Eastern Mediterranean, Cairo, Egypt.
- 15 Global Healthcare Information Network, Oxford, UK.
- 16 UNDP/UNFPA/UNICEF/World Bank Special Program of Research, Development and Research Training in Human Reproduction (HRP), Department of Sexual and Reproductive Health and Research, World Health Organization, Geneva, Switzerland.
- 17 Department of Health Sciences Ålesund, Norwegian University of Science and Technology (NTNU), Ålesund, Norway.
- 18 Health Systems Research Unit, South African Medical Research Council, Cape Town, South Africa.
- 19 Centre for Epidemic Interventions Research (CEIR), Norwegian Institute of Public Health, Oslo, Norway.
- PMID: 39189465
- PMCID: PMC11348462
- DOI: 10.1002/14651858.CD015705.pub2
Background: Healthcare workers sometimes develop their own informal solutions to deliver services. One such solution is to use their personal mobile phones or other mobile devices in ways that are unregulated by their workplace. This can help them carry out their work when their workplace lacks functional formal communication and information systems, but it can also lead to new challenges.
Objectives: To explore the views, experiences, and practices of healthcare workers, managers and other professionals working in healthcare services regarding their informal, innovative uses of mobile devices to support their work.
Search methods: We searched MEDLINE, Embase, CINAHL and Scopus on 11 August 2022 for studies published since 2008 in any language. We carried out citation searches and contacted study authors to clarify published information and seek unpublished data.
Selection criteria: We included qualitative studies and mixed-methods studies with a qualitative component. We included studies that explored healthcare workers' views, experiences, and practices regarding mobile phones and other mobile devices, and that included data about healthcare workers' informal use of these devices for work purposes.
Data collection and analysis: We extracted data using an extraction form designed for this synthesis, assessed methodological limitations using predefined criteria, and used a thematic synthesis approach to synthesise the data. We used the 'street-level bureaucrat' concept to apply a conceptual lens to our findings and prepare a line of argument that links these findings. We used the GRADE-CERQual approach to assess our confidence in the review findings and the line-of-argument statements. We collaborated with relevant stakeholders when defining the review scope, interpreting the findings, and developing implications for practice.
Main results: We included 30 studies in the review, published between 2013 and 2022. The studies were from high-, middle- and low-income countries and covered a range of healthcare settings and healthcare worker cadres. Most described mobile phone use as opposed to other mobile devices, such as tablets. We have moderate to high confidence in the statements in the following line of argument. The healthcare workers in this review, like other 'street-level bureaucrats', face a gap between what is expected of them and the resources available to them. To plug this gap, healthcare workers develop their own strategies, including using their own mobile phones, data and airtime. They also use other personal resources, including their personal time when taking and making calls outside working hours, and their personal networks when contacting others for help and advice. In some settings, healthcare workers' personal phone use, although unregulated, has become a normal part of many work processes. Some healthcare workers therefore experience pressure or expectations from colleagues and managers to use their personal phones. Some also feel driven to use their phones at work and at home because of feelings of obligation towards their patients and colleagues. At best, healthcare workers' use of their personal phones, time and networks helps humanise healthcare. It allows healthcare workers to be more flexible, efficient and responsive to the needs of the patient. It can give patients access to individual healthcare workers rather than generic systems and can help patients keep their sensitive information out of the formal system. It also allows healthcare workers to communicate with each other in more personalised, socially appropriate ways than formal systems allow. All of this can strengthen healthcare workers' relationships with community members and colleagues. However, these informal approaches can also replicate existing social hierarchies and deepen existing inequities among healthcare workers. Personal phone use costs healthcare workers money. This is a particular problem for lower-level healthcare workers and healthcare workers in low-income settings as they are likely to be paid less and may have less access to work phones or compensation. Out-of-hours use may also be more of a burden for lower-level healthcare workers, as they may find it harder to ignore calls when they are at home. Healthcare workers with poor access to electricity and the internet are less able to use informal mobile phone solutions, while healthcare workers who lack skills and training in how to appraise unendorsed online information are likely to struggle to identify trustworthy information. Informal digital channels can help healthcare workers expand their networks. But healthcare workers who rely on personal networks to seek help and advice are at a disadvantage if these networks are weak. Healthcare workers' use of their personal resources can also lead to problems for patients and can benefit some patients more than others. For instance, when healthcare workers store and share patient information on their personal phones, the confidentiality of this information may be broken. In addition, healthcare workers may decide to use their personal resources on some types of patients, but not others. Healthcare workers sometimes describe using their personal phones and their personal time and networks to help patients and clients whom they assess as being particularly in need. These decisions are likely to reflect their own values and ideas, for instance about social equity and patient 'worthiness'. But these may not necessarily reflect the goals, ideals and regulations of the formal healthcare system. Finally, informal mobile phone use plugs gaps in the system but can also weaken the system. The storing and sharing of information on personal phones and through informal channels can represent a 'shadow IT' (information technology) system where information about patient flow, logistics, etc., is not recorded in the formal system. Healthcare workers may also be more distracted at work, for instance, by calls from colleagues and family members or by social media use. Such challenges may be particularly difficult for weak healthcare systems.
Authors' conclusions: By finding their own informal solutions to workplace challenges, healthcare workers can be more efficient and more responsive to the needs of patients, colleagues and themselves. But these solutions also have several drawbacks. Efforts to strengthen formal health systems should consider how to retain the benefits of informal solutions and reduce their negative effects.
Copyright © 2024 The Authors. Cochrane Database of Systematic Reviews published by John Wiley & Sons, Ltd. on behalf of The Cochrane Collaboration.
PubMed Disclaimer
Conflict of interest statement
Claire Glenton (CG) declares a grant from Norges Forskningsråd (Research Council in Norway) paid to institution. Until 2022, CG was an Editor with Cochrane Effective Practice and Organisation of Care (EPOC). She was not involved in the editorial process for this review.
Elizabeth Paulsen (EP) declares that she has no conflicts of interest.
Smisha Agarwal (SA) declares grants and contracts from the Gates Foundation to study digital health (to understand how countries are using digital tools to improve primary healthcare services). SA has several academic papers in this area of work. SA serves on the Board of Directors (non‐fiduciary) of Reach Digital (South Africa), a not for profit company that provides services that develops mobile based health programs.
Unni Gopinathan (UG) declares a grant from Norges Forskningsråd (Research Council of Norway, project no. 316145); paid to institution (to cover salary and operational costs of the project).
Marit Johansen (MJ): until January 2023, MJ was the Information Specialist for Cochrane EPOC, hosted by the Norwegian Institute for Public Health. MJ was not involved in the editorial process for this review.
David Kyaddondo (DK) declares that he has no conflicts of interest.
Susan Munabi‐Babigumira (SMB) works in health services research at the Norwegian Public health Institute. She is a former Editor with Cochrane EPOC. She was not involved in the editorial process for this review.
Josephine Nabukenya (JNAB) declares that she has no conflicts of interest.
Immaculate Nakityo (IN) declares that she has no conflicts of interest.
Rehema Namaganda (RN) declares that she has no conflicts of interest.
Josephine Namitala (JNAM) is a PhD candidate on the mHEALTH INNOVATE PROJECT.
Tom Neumark (TN) declares that he has no conflicts of interest.
Allen Nsangi (AN) declares that she has no conflicts of interest.
Neil Pakenham‐Walsh (NMPW) is a global health professional and runs a non‐profit global network called Healthcare Information For All (HIFA; www.hifa.org ). HIFA is supported by more than 400 health and development organisations including Cochrane. A number of these may have an opinion or interest, or both, in the topic.
Arash Rashidian (AR) is a health professional by background, and now works as a director within the WHO, who have a stated policy to support countries by enhancing the use of digital health to support health systems and respond to people needs. He declares that he has published papers on digital health. AR is an EPOC Editor, but was not involved in the editorial process for this review. AR also declares that he holds a professorship position in health policy (however, he is on unpaid leave from this position while he works for the WHO).
Geoff Royston (GR) is a member of the Steering Group for HIFA ( www.hifa.org ), who have declared an opinion or position on the topic. GR reports that he is a co‐author of two papers relevant to the review: (PAPER 1) Royston G, Pakenham‐Walsh N, Zielinski C. Universal access to essential health information: accelerating progress towards universal health coverage and other SDG health targets. BMJ Global Health 2020;5:e002475. DOI:10.1136/ bmjgh‐2020‐002475; and (PAPER 2) Royston G, Hagar C, Long L‐A, et al. Mobile health‐care information for all: a global challenge. Lancet Glob Health 2015;3:e356–7.
Nelson Sewankambo (NS) declares that he has no conflicts of interest.
Tigest Tamrat (TT) declares that she has no conflicts of interest.
Simon Lewin (SL) declares a grant from the Research Council of Norway – he is Co‐investigator on the mHEALTH‐INNOVATE research project ( prosjektbanken.forskningsradet.no/en/project/FORISS/325476 ), paid to institution but managed by SL (covered person‐time related to this review, including writing the review). SL is the Fiduciary Officer and CoLead for Cochrane Person‐Centred Care, Health Systems and Public Health Thematic Group; unpaid position. SL was employed as the Joint Co‐ordinating Editor of Cochrane EPOC, paid to institution. He was not involved in the editorial process for this review. SL declares that he was on the Trial Steering Committee, which received no funding, for a trial that was linked to one of the included studies (Anstey 2018).
A number of non‐financial issues, including personal, political, and academic factors, could have influenced the review authors’ input when conducting this review. The review authors have discussed this further in the sections on reflexivity in the Methods section.
PRISMA flow diagram
- doi: 10.1002/14651858.CD015705
References to studies included in this review
Abane 2021 {published data only}.
- Abane AM, Mariwah S, Owusu SA, Kasim A, Robson E, Hampshire K. Mobile phone use and the welfare of community health nurses in Ghana: an analysis of unintended costs. World Development Perspectives 2021;23:100317. [DOI: 10 1016/j wdp 2021 100317] - PMC - PubMed
Anstey 2018 {published data only}
- Anstey Watkins JOT, Goudge J, Gomez-Olive FX, Griffiths F. Mobile phone use among patients and health workers to enhance primary healthcare: a qualitative study in rural South Africa. Social Science & Medicine 2018;198:139-47. [DOI: 10 1016/j socscimed 2018 01 011] - PubMed
Barnor‐Ahiaku 2016 {published data only}
- Barnor-Ahiaku E. Exploring the use of smartphones and tablets by medical House Officers in Korle-Bu Teaching Hospital. Ghana Medical Journal 2016;50(1):50-6. [DOI: ] - PMC - PubMed
Bautista 2016 {published data only}
- Bautista JR, Lin TT. Sociotechnical analysis of nurses' use of personal mobile phones at work. International Journal of Medical Informatics 2016;95:71-80. [DOI: 10 1016/j ijmedinf 2016 09 002] - PubMed
Bautista 2017 {published data only}
- Bautista JR, Lin TTC. Nurses' use of mobile instant messaging applications: a uses and gratifications perspective. International Journal of Nursing Practice 2017;23(5):1-9. [DOI: 10 1111/ijn 12577] - PubMed
Bautista 2020 {published data only}
- Bautista JR, Lin TTC, Theng YL. Influence of organizational issues on nurse administrators' support to staff nurses' use of smartphones for work purposes in the Philippines: focus group study. JMIR Nursing 2020;3(1):e17040. [DOI: 10 2196/17040] - PMC - PubMed
Bhat 2021 {published data only}
- Bhat KS, Jain M, Kumar N. Infrastructuring telehealth in (in)formal patient-doctor contexts. Proceedings of the ACM on Human-Computer Interaction 2021;5(CSCW2):1-28. [DOI: 10 1145/3476064]
Brandt 2016 {published data only}
- Brandt J, Katsma D. Calling in at work: acute care nursing cell phone policies. Nursing Management 2016;47(7):20-7. [DOI: 10 1097/01 NUMA 0000484474 76112 a3] - PubMed
Burns 2013 {published data only}
- Burns K, Belton S. Clinicians and their cameras: policy, ethics and practice in an Australian tertiary hospital. Australian Health Review 2013;37(4):437-41. [DOI: 10 1071/ah12039] - PubMed
Chiang 2016 {published data only}
- Chiang KF, Wang HH. Nurses' experiences of using a smart mobile device application to assist home care for patients with chronic disease: a qualitative study. Journal of Clinical Nursing 2016;25(13-4):2008-17. [DOI: 10 1111/jocn 13231] - PubMed
Chib 2013 {published data only}
- Chib A, Phuong TK, Si CW, Hway NS. Enabling informal digital guanxi for rural doctors in Shaanxi, China. Chinese Journal of Communication 2013;6(1):62-80. [DOI: 10 1080/17544750 2013 753500]
Hampshire 2017 {published data only}
- Hampshire K, Porter G, Mariwah S, Munthali A, Robson E, Owusu SA, et al. Who bears the cost of 'informal mhealth'? Health-workers' mobile phone practices and associated political-moral economies of care in Ghana and Malawi. Health Policy & Planning 2017;32(1):34-42. [DOI: 10 1093/heapol/czw095] - PMC - PubMed
Hampshire 2021 {published data only}
- Hampshire K, Mwase-Vuma T, Alemu K, Abane A, Munthali A, Awoke T, et al. Informal mhealth at scale in Africa: opportunities and challenges. World Development 2021;140:105257. [DOI: 10 1016/j worlddev 2020 105257] - PMC - PubMed
Hussain 2022 {published data only}
- Hussain A, Rossi T, Rynne S. ‘I keep my brain on my iPhone’–being and becoming an emergency physician in a technological age. Studies in Continuing Education 2022;45(2):1-16. [DOI: 10 1080/0158037x 2022 2028762]
Ismail 2019 {published data only}
- Ismail A, Kumar N. Empowerment on the margins: the online experiences of community health workers. In: CHI '19: Conference on Human Factors in Computing Systems; 2019 May 4-9; Glasgow, Scotland. 2019:1-15. [DOI: 10.1145/3290605.3300329] - DOI
Karusala 2020 {published data only}
- Karusala N, Wang D, O'Neill J. Making chat at home in the hospital: exploring chat use by nurses. In: CHI '20: Conference on Human Factors in Computing Systems; 2020 April 25-30; Honolulu, Hawai'i. 2020:1-15. [DOI: 10 1145/3313831 3376166]
Ling 2020 {published data only}
- Ling R, Poorisat T, Chib A. Mobile phones and patient referral in Thai rural healthcare: a structuration view. Information Communication and Society 2020;23(3):358-73. [DOI: 10 1080/1369118x 2018 1503698]
Mariwah 2022 {published data only}
- Mariwah S, Machistey Abane A, Asiedu Owusu S, Kasim A, Robson E, Castelli M, et al. Formalising 'informal' mHealth in Ghana: opportunities and challenges for Universal Health Coverage (UHC). Global Public Health 2022;17(5):768-81. [DOI: 10 1080/17441692 2021 1874467] - PubMed
Mather 2018 {published data only}
- Mather C, Cummings E, Gale F. Nurses as stakeholders in the adoption of mobile technology in Australian health care environments: interview study. JMIR Nursing 2019;2(1):e14279. [DOI: 10 2196/14279] - PMC - PubMed
- Mather CA, Cummings EA, Gale F. Advancing mobile learning in Australian healthcare environments: nursing profession organisation perspectives and leadership challenges. BMC Nursing 2018;17:44. [DOI: 10 1186/s12912-018-0313-z] - PMC - PubMed
Moyer 2014 {published data only}
- Moyer E. Peer mentors, mobile phone and pills: collective monitoring and adherence in Kenyatta National Hospital's HIV treatment programme. Anthropology & Medicine 2014;21(2):149-61. [DOI: 10 1080/13648470 2014 925083] - PMC - PubMed
Nerminathan 2017 {published data only}
- Nerminathan A, Harrison A, Phelps M, Alexander S, Scott KM. Doctors' use of mobile devices in the clinical setting: a mixed methods study. Internal Medicine Journal 2017;47(3):291-8. [DOI: 10 1111/imj 13349] - PubMed
Ologeanu‐Taddei 2019 {published data only}
- Ologeanu-Taddei R, Wessel L, Bourdon I. Persistent paradoxes in pluralistic organizations: a case study of continued use of shadow-it in a French hospital. In: ICIS 2019; 2019 December 15-18; Munich, Germany. Vol. 16. 2019:1-17. [URL: aisel.aisnet.org/icis2019/is_development/is_development/16 ]
Pimmer 2018 {published data only}
- Pimmer C, Lee A, Mwaikambo L. Mobile instant messaging: new knowledge tools in global health? Knowledge Management & E-Learning: An International Journal 2018;10(3):334-49. [URL: files.eric.ed.gov/fulltext/EJ1247624.pdf ]
Rathbone 2020 {published data only}
- Rathbone AP, Norris R, Parker P, Lindsley A, Robinson A, Baqir W, et al. Exploring the use of WhatsApp in out-of-hours pharmacy services: a multi-site qualitative study. Research In Social & Administrative Pharmacy 2020;16(4):503-10. [DOI: 10 1016/j sapharm 2019 06 019] - PubMed
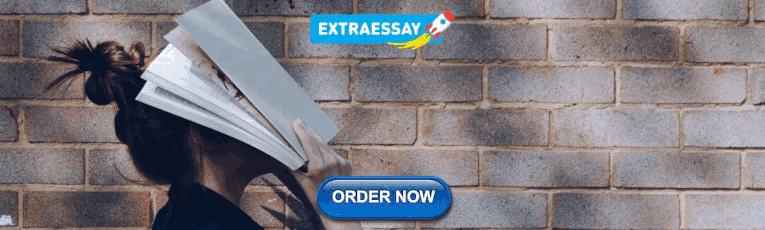
Schwartz 2013 {published data only}
- Schwartz A, Bhavsar M, Cutrell E, Donner J, Densmore M. Balancing burden and benefit: non-prescribed use of employer-issued mobile devices. In: ICTD '13: Sixth International Conference on Information and Communications Technologies and Development: Notes- Volume 2; 2013 December 7-10 ; Cape Town, South Africa. 2013:140-3. [DOI: 10.1145/2517899.2517911] - DOI
Shenouda 2018 {published data only}
- Shenouda JEA, Davies BS, Haq I. The role of the smartphone in the transition from medical student to foundation trainee: a qualitative interview and focus group study. BMC Medical Education 2018;18(1):175. [DOI: 10 1186/s12909-018-1279-y] - PMC - PubMed
Spink 2020 {published data only}
- Spink P, Burstein F. Mobile technology use in a multidisciplinary healthcare team - factors and challenges. In: 53rd Annual Hawaii International Conference on System Sciences; 2020 January 7-10; Maui, Hawai'i. 2020:3508-18. [DOI: 10 24251/hicss 2020 431]
Tran 2014 {published data only}
- Tran K, Morra D, Lo V, Quan S, Wu R. The use of smartphones on general internal medicine wards: a mixed methods study. Applied Clinical Informatics 2014;5(3):814-23. [DOI: 10 4338/aci-2014-02-ra-0011] - PMC - PubMed
Venkataraghavan 2022 {published data only}
- Venkataraghavan M, Rani P, Ashok L, Rao CR, Chandra SV, Krishnapriya TK. "Through their eyes, I can work" – rural physicians' perceptions about mobile phone use among community health workers – a qualitative analysis. Health Education 2022;122(2):180-201. [DOI: 10 1108/he-12-2020-0123]
Watkins 2018 {published data only}
- Watkins J, Baulch E. Chapter 5. Identifying grassroots opportunities and barriers to mHealth design for HIV/AIDS using a communicative ecologies framework. In: Baulch E, Watkins J, Tariq A, editors(s). mHealth Innovation in Asia: Grassroots Challenges and Practical Interventions. Dordrecht, The Netherlands: Springer Open, 2018:69-90. [DOI: 10 1007/978-94-024-1251-2]
References to studies awaiting assessment
Anderson 2013 {published data only}.
- Anderson E, Esper G. Mneurology: Shaping the future of patient care. Neurology 2013;80(Suppl 7):P05.011. [DOI: 10.1212/WNL.80.7_supplement.P05.011] - DOI
Kundasamy 2019 {published data only}
- Kundasamy P, Abdelrahman I, Hill G. Taking clinical pictures with smartphones in emergency and trauma; a change in hospital policy; a quality improvement project. British Journal of Surgery 2019;106(Suppl 6):147-8. [DOI: 10.1002/bjs.11344] - DOI
Lee 2015 {published data only}
- Lee JL, Beach MC, Wu AW. Communication access: why some patients can email their doctors and others can't. Journal of General Internal Medicine 2015;2:S125.
Negarandeh 2022 {published data only}
- Negarandeh R, Molaee S, Mohammadnejad E, Aazami S. Challenges of using personal cell phone by nurses in care setting: a qualitative study. JMIR Publications 2022 [Preprint]. [DOI: 10 2196/preprints 39635]
Patel 2011 {published data only}
- Patel M, Dine J, Asch D. Resident use of smartphones while providing patient care. Journal of General Internal Medicine 2011;26(1):S103-4. [DOI: 10 1007/s11606-011-1730-9]
Simeonov 2012 {published data only}
- Simeonov IM, Hamm KM. Mobile technology use by promotoras: a small qualitative study in California. Clinical Toxicology 2012;50(7):631. [DOI: 10 3109/15563650 2012 700015]
Williams 2013 {published data only}
- Williams CG. The role of mobile phones in delivering emergency health care to a rural population in eastern Uganda. BJOG: An International Journal of Obstetrics and Gynaecology 2013;120(Suppl 1):334. [ABSTRACT NUMBER: EP5.46] [DOI: 10 1111/1471-0528 12297]
Additional references
Agarwal 2018.
- Agarwal S, Vasudevan L, Tamrat T, Glenton C, Lewin S, Bergman H, et al. Digital tracking, provider decision support systems, and targeted client communication via mobile devices to improve primary health care. Cochrane Database of Systematic Reviews 2018, Issue 1. Art. No: CD012925. [DOI: 10.1002/14651858.CD012925] - DOI
Agarwal 2020
- Agarwal S, Glenton C, Henschke N, Tamrat T, Bergman H, Fønhus MS, et al. Tracking health commodity inventory and notifying stock levels via mobile devices: a mixed methods systematic review. Cochrane Database of Systematic Reviews 2020, Issue 10. Art. No: CD012907. [DOI: 10.1002/14651858.CD012907.pub2] - DOI - PMC - PubMed
Agarwal 2021
- Agarwal S, Glenton C, Tamrat T, Henschke N, Maayan N, Fønhus MS, et al. Decision-support tools via mobile devices to improve quality of care in primary healthcare settings. Cochrane Database of Systematic Reviews 2021, Issue 7. Art. No: CD012944. [DOI: 10.1002/14651858.CD012944.pub2] - DOI - PMC - PubMed
Alshallaqi 2022
- Alshallaqi M. The complexities of digitization and street-level discretion: a socio-materiality perspective. Public Management Review 2022;26(1):25-47. [DOI: 10.1080/14719037.2022.2042726] - DOI
- Alwy Al-Beity F, Pembe AB, Kwezi HA, Massawe SN, Hanson C, Baker U. "We do what we can do to save a woman" health workers' perceptions of health facility readiness for management of postpartum haemorrhage. Global Health Action 2020;13(1):1707403. [DOI: 10.1080/16549716.2019.1707403] - DOI - PMC - PubMed
- Ames HM, Glenton C, Lewin S, Tamrat T, Akama E, Leon N. Clients' perceptions and experiences of targeted digital communication accessible via mobile devices for reproductive, maternal, newborn, child, and adolescent health: a qualitative evidence synthesis. Cochrane Database of Systematic Reviews 2019, Issue 10. Art. No: CD013447. [DOI: 10.1002/14651858.CD013447] - DOI - PMC - PubMed
Anstey 2018
- Anstey Watkins JOT, Goudge J, Gómez-Olivé FX, Griffiths F. Mobile phone use among patients and health workers to enhance primary healthcare: a qualitative study in rural South Africa. Social Science & Medicine 2018;198:139-47. [DOI: 10.1016/j.socscimed.2018.01.011] - DOI - PubMed
Backes 2007
- Backes DS, Koerich MS, Erdmann AL. Humanizing care through the valuation of the human being: resignifcation of values and principles by health professionals. Revista Latino-Americana de Enfermagem 2007;15(1):34-41. [DOI: 10.1590/s0104-11692007000100006] - DOI - PubMed
Barnett‐Page 2009
- Barnett-Page E, Thomas J. Methods for the synthesis of qualitative research: a critical review. BMC Medical Research Methodology 2009;9(1):1-11. [DOI: 10.1186/1471-2288-9-59] - DOI - PMC - PubMed
Blavatnik 2020
- Blavatnik School of Government. ‘Public institutional integrity' defined. integrity.bsg.ox.ac.uk/sites/default/files/integrity/documents/media/ins... (accessed August 2023).
Bowker 1995
- Bowker GC, Timmermans S, Star SL. Infrastructure and organizational transformation: classifying nurses' work. In: Orlikowski W, Walsham G, Jones M, DeGross J, editors(s). Information Technology and Changes in Organizational Work. London (UK): Chapman and Hall, 1995:344-70. [DOI: 10.1007/978-0-387-34872-8] - DOI
- Braun V, Clarke V. Using thematic analysis in psychology. Qualitative Research in Psychology 2006;3(2):77-101. [DOI: 10.1191/1478088706qp063oa] - DOI
Buchely 2015
- Buchely L. Bureaucratic Activism and Colombian Community Mothers: The Daily Construction of the Rule of Law. Rapoport Center Human Rights Working Paper Series. Austin (TX): University of Texas School of Law, 2015.
- Busch IM, Moretti F, Travaini G, Wu AW, Rimondini M. Humanization of care: key elements identifed by patients, caregivers, and healthcare providers. A systematic review. The Patient - Patient-Centered Outcomes Research 2019 ;12(5):461-74. [DOI: 10.1007/s40271-019-00370-1] - DOI - PubMed
- Critical Appraisal Skills Programme (CASP). CASP Qualitative Studies Checklist. casp-uk.net/images/checklist/documents/CASP-Qualitative-Studies-Checklis... (accessed 28 March 2023).
Dixon‐Woods 2006
- Dixon-Woods M, Cavers D, Agarwal S, Annandale E, Arthur A, Harvey J, et al. Conducting a critical interpretive synthesis of the literature on access to healthcare by vulnerable groups. BMC Medical Research Methodology 2006;6(1):1-13. [DOI: 10.1186/1471-2288-6-35] - DOI - PMC - PubMed
Dubois 2010
- Dubois V. The Bureaucrat and the Poor. Encounters in French Welfare Offices. Aldershot (UK): Ashgate, 2010.
- Cochrane Effective Practice and Organisation of Care Group (EPOC). EPOC Qualitative Evidence Syntheses guidance on when to sample and how to develop a purposive sampling frame; 2017. Available at epoc.cochrane.org/resources/epoc-resources-review-authors .
Finlay 2009
- Finlay S, Sandall J. "Someone's rooting for you": continuity, advocacy and street-level bureaucracy in UK maternal healthcare. Social Science & Medicine 2009;69(8):1228-35. [DOI: 10.1016/j.socscimed.2009.07.029] - DOI - PubMed
- Gates A, Guitard S, Pillay J, Elliott SA, Dyson MP, Newton AS, et al. Performance and usability of machine learning for screening in systematic reviews: a comparative evaluation of three tools. Systematic Reviews 2019;8(1):278. [DOI: 10.1186/s13643-019-1222-2] - DOI - PMC - PubMed
Gillingham 2010
- Gillingham P, Humphreys C. Child protection practitioners and decision-making tools: observations and reflections from the front line. British Journal of Social Work 2010;40:2598-616. [DOI: 10.1093/bjsw/bcp155] - DOI
Glenton 2019
- Glenton C, Lewin S, Lawrie TA, Barreix M, Downe S, Finlayson KW, et al. Qualitative Evidence Synthesis (QES) for Guidelines: Paper 3 - using qualitative evidence syntheses to develop implementation considerations and inform implementation processes. Health Research Policy and Systems 2019;17(1):74. [DOI: 10.1186/s12961-019-0450-1] - DOI - PMC - PubMed
Glenton 2021
- Glenton C, Carlsen B, Lewin S, Wennekes MD, Winje BA, Eilers R. Healthcare workers' perceptions and experiences of communicating with people over 50 years of age about vaccination: a qualitative evidence synthesis. Cochrane Database of Systematic Reviews 2021, Issue 7. Art. No: CD013706. [DOI: 10.1002/14651858.CD013706.pub2] - DOI - PMC - PubMed
Glenton 2022
- Glenton C, Nabukenya J, Agarwal S, Meltzer M, Mukendi E, Nakityo I, et al. Using an online community of practice to explore the informal use of mobile phones by health workers. Oxford Open Digital Health 2023;1:1-6. [DOI: 10.1093/oodh/oqac003] - DOI
Glenton 2023
- Glenton C, Bohren MA, Downe S, Paulsen EJ, Lewin S. EPOC Qualitative Evidence Synthesis: protocol and review template, version 1.4; 6 October 2023. Available at zenodo.org/records/10050961 .
Gonçalves‐Bradley 2020
- Gonçalves-Bradley DC, Maria ARJ, Ricci-Cabello I, Villanueva G, Fønhus MS, Glenton C, et al. Mobile technologies to support healthcare provider to healthcare provider communication and management of care. Cochrane Database of Systematic Reviews 2020, Issue 8. Art. No: CD012927. [DOI: 10.1002/14651858.CD012927.pub2] - DOI - PMC - PubMed
Gonçalves‐Bradley 2018
- Gonçalves‐Bradley DC, Buckley BS, Fønhus MS, Glenton C, Henschke N, Lewin S, et al. Mobile‐based technologies to support client to healthcare provider communication and management of care. Cochrane Database of Systematic Reviews 2018, Issue 1. Art. No: CD012928. [DOI: 10.1002/14651858.CD012928] - DOI - PMC - PubMed
GRADE‐CERQual 2022 [Computer program]
- GRADE-CERQual Interactive Summary of Qualitative Findings (iSoQ), Version 1.0 (Available at isoq.epistemonikos.org ). Oslo, Norway: Norwegian Institute of Public Health, accessed 18 October 2022. Available at isoq.epistemonikos.org .
- Green J, Thorogood N. Qualitative Methods for Health Research. London (UK): SAGE Publications Ltd, 2018.
Hampshire 2021
- Hampshire K, Mwase-Vuma T, Alemu K, Abane A, Munthali A, Awoke T, et al. Informal mhealth at scale in Africa: opportunities and challenges. World Development 2021;140:105257. [DOI: 10.1016/j.worlddev.2020.105257] - DOI - PMC - PubMed
Harris 2013
- Harris B, Eyles J, Penn-Kekana L, Fried J, Nyathela H, Thomas L, et al. Bringing justice to unacceptable health care services? Street-level reflections from urban South Africa. International Journal of Transitional Justice 2013;8(1):141-61. [DOI: 10.1093/ijtj/ijt028] - DOI
Hatton 2017
- Hatton E. Mechanisms of invisibility: rethinking the concept of invisible work. Work, Employment and Society 2017;31(2):336-51. [DOI: 10.1177/0950017016674894] - DOI
- Hupe P, Buffat A. A public service gap: capturing contexts in a comparative approach of street-level bureaucracy. Public Management Review 2014;16(4):548-69. [DOI: 10.1080/14719037.2013.854401] - DOI
- International Telecommunication Union. Global ICT developments. www.itu.int/ITU-D/ict/statistics/ict/ (accessed 18 October 2022).
Karimi‐Shahanjarini 2019
- Karimi-Shahanjarini A, Shakibazadeh E, Rashidian A, Hajimiri K, Glenton C, Noyes J, et al. Barriers and facilitators to the implementation of doctor-nurse substitution strategies in primary care: a qualitative evidence synthesis. Cochrane Database of Systematic Reviews 2019, Issue 4. Art. No: CD010412. [DOI: 10.1002/14651858.CD010412.pub2] - DOI - PMC - PubMed
- Lavee E. Who is in charge? The provision of informal personal resources at the street level. Journal of Public Administration Research and Theory 2021;31(1):4-20. [DOI: 10.1093/jopart/muaa025] - DOI
- Lavee E. Walking the talk of social equity? Street-level bureaucrats’ decisionmaking about the provision of personal resources. American Review of Public Administration 2022;52(1):3-15. [DOI: 10.1177/02750740211050577] - DOI
- Lewin S, Booth A, Glenton C, Munthe-Kaas H, Rashidian A, Wainwright M, et al. Applying GRADE-CERQual to qualitative evidence synthesis findings: introduction to the series. Implement Science 2018;13(Suppl 1):2. [DOI: 10.1186/s13012-017-0688-3] - DOI - PMC - PubMed
Lipsky 1980
- Lipsky M. Street-Level Bureaucracy: Dilemmas of the Individual in Public Services. New York (NY): Russel Sage Foundation, 1980.
Mather 2019
- May C, Finch T, Mair F, Ballini L, Dowrick C, Eccles M, et al. Understanding the implementation of complex interventions in health care: the normalization process model. BMC Health Services Research 2007;7:148. [DOI: 10.1186/1472-6963-7-148] - DOI - PMC - PubMed
Maynard‐Moody 2000
- Maynard-Moody S, Musheno M. State agent or citizen agent: two narratives of discretion. Journal of Public Administration Research and Theory 2000;10(2):329-58. [DOI: 10.1093/oxfordjournals.jpart.a024272] - DOI
Merriam‐Webster 2023
- Innovation. www.merriam-webster.com/dictionary/innovation (accessed 9 November 2023).
Microsoft Excel 2022 [Computer program]
- Microsoft Excel. Version 2204. Microsoft Corporation, 2022. Available from https://office.microsoft.com/excel .
Microsoft Word 2022 [Computer program]
- Microsoft Word. Version 2204. Microsoft Corporation, 2022. Available from https://office.microsoft.com/word .
Muller 2021
- Muller AE, Ames HM, Himmels JP, Jardim PS, Nguyen HL, Rose CJ, et al. Implementation of machine learning in evidence syntheses in the Cluster for Reviews and Health Technology Assessments: Final report 2020-2021. Norwegian Institute of Public Health. www.fhi.no/globalassets/dokumenterfiler/rapporter/2021/implementation-of... (accessed 5 June 2023).
Munabi‐Babigumira 2019
- Munabi-Babigumira S, Glenton C, Willcox M, Nabudere H. Ugandan health workers' and mothers' views and experiences of the quality of maternity care and the use of informal solutions: a qualitative study. PLOS One 2019;14(3):e0213511. [DOI: 10.1371/journal.pone.0213511] - DOI - PMC - PubMed
Munthe‐Kaas 2020
- Munthe-Kaas H, Nøkleby H, Lewin S, Glenton C. The TRANSFER Approach for assessing the transferability of systematic review findings. BMC Medical Research Methodology 2020;20(1):11. [DOI: 10.1186/s12874-019-0834-5] - DOI - PMC - PubMed
- Naeem M, Ozuem W, Howell K, Ranfagni S. A step-by-step process of thematic analysis to develop a conceptual model in qualitative research. International Journal of Qualitative Methods 2023;22:1-18. [DOI: 10.1177/16094069231205789] - DOI
Neumark 2020
- Neumark T. Trusting the poor: cash grants and the caring bureaucrat in Kenya. Anthropological Quarterly 2020;93(2):119-49. [DOI: 10.1353/anq.2020.0028] - DOI
Odendaal 2020
- Odendaal WA, Anstey Watkins J, Leon N, Goudge J, Griffiths F, Tomlinson M, et al. Health workers' perceptions and experiences of using mHealth technologies to deliver primary healthcare services: a qualitative evidence synthesis. Cochrane Database of Systematic Reviews 2020, Issue 3. Art. No: CD011942. [DOI: 10.1002/14651858.CD011942.pub2] - DOI - PMC - PubMed
- Page MJ, McKenzie JE, Bossuyt PM, Boutron I, Hoffmann TC, Mulrow CD, et al. The PRISMA 2020 statement: an updated guideline for reporting systematic reviews. BMJ 2021;372:n71. [DOI: 10.1136/bmj.n71] - DOI - PMC - PubMed
- Page MJ, Moher D, Bossuyt PM, Boutron I, Hoffmann TC, Mulrow CD, et al. PRISMA 2020 explanation and elaboration: updated guidance and exemplars for reporting systematic reviews. BMJ 2021;372:n160. [DOI: 10.1136/bmj.n160] - DOI - PMC - PubMed
Palmer 2020a
- Palmer MJ, Henschke N, Bergman H, Villanueva G, Maayan N, Tamrat T, et al. Targeted client communication via mobile devices for improving maternal, neonatal, and child health. Cochrane Database of Systematic Reviews 2020, Issue 8. Art. No: CD013679. [DOI: 10.1002/14651858.CD013679] - DOI - PMC - PubMed
Palmer 2020b
- Palmer MJ, Henschke N, Villanueva G, Maayan N, Bergman H, Glenton C, et al. Targeted client communication via mobile devices for improving sexual and reproductive health. Cochrane Database of Systematic Reviews 2020, Issue 8. Art. No: CD013680. [DOI: 10.1002/14651858.CD013680] - DOI - PMC - PubMed
Phillips 2015
- Phillips W, Lee H, Ghobadian A, O'Regan N, James P. Social innovation and social entrepreneurship: a systematic review. Group & Organization Management 2015;40(3):428-61. [DOI: 10.1177/1059601114560063] - DOI
Phills 2008
- Phills JA, Deiglmeier K, Miller DT. Rediscovering social innovation. Stanford Social Innovation Review 2008;6:34-43. [DOI: 10.48558/gbjy-gj47] - DOI
Pollock 2022
- Pollock A, Morley R, Watts C. Involving people: a learning resource for systematic review authors. training.cochrane.org/involving-people (accessed 21 June 2022).
Sheikh 2020
- Sheikh K, Agyepong I, Jhalani M, Ammar W, Hafeez A, Pyakuryal S, et al. Learning health systems: an empowering agenda for low-income and middle-income countries. Lancet 2020;395(10223):476-7. [DOI: 10.1016/S0140-6736(19)33134-4] - DOI - PubMed
Thomas 2008
- Thomas J, Harden A. Methods for the thematic synthesis of qualitative research in systematic reviews. BMC Medical Research Methodology 2008;8(1):1-10. [DOI: 10.1186/1471-2288-8-45] - DOI - PMC - PubMed
Thomas 2010 [Computer program]
- EPPI-Reviewer 4: software for research synthesis. EPPI-Centre Software. Thomas J, Brunton J, Graziosi S, Version 4. London: Social Science Research Unit, UCL Institute of Education, 2010.
Tummers 2015
- Tummers LL, Bekkers V, Vink E, Musheno M. Coping during public service delivery: a conceptualization and systematic review of the literature. Journal of Public Administration Research and Theory 2015;25(4):1099–126. [DOI: 10.1093/jopart/muu056] - DOI
van Niekerk 2017
- Niekerk L, Chater R, Naydenova E, Lim J, Chamas L, Manderson L, et al. Social innovation in health: case studies and lessons learned from low- and middle-income countries; 2017. apps.who.int/iris/handle/10665/259187 .
Vasudevan 2021
- Vasudevan L, Glenton C, Henschke N, Maayan N, Eyers J, Fønhus MS, et al. Birth and death notification via mobile devices: a mixed methods systematic review. Cochrane Database of Systematic Reviews 2021, Issue 7. Art. No: CD012909. [DOI: 10.1002/14651858.CD012909.pub2] - DOI - PMC - PubMed
- World Health Organization. WHA71.7 Digital health: resolutions and decisions; 26 May 2018. Available at www.who.int/publications/i/item/10665-279505 (accessed 5 June 2023).
- World Health Organization. WHO Recommendations on Digital Interventions for Health Systems Strengthening. Geneva (Switzerland): World Health Organization, 2019.
- World Health Organization. WHO releases first guideline on digital health interventions; 17 April 2019. Available at www.who.int/news/item/17-04-2019-who-releases-first-guideline-on-digital... .
- World Health Organization. Global strategy on digital health 2020-2025. www.who.int/docs/default-source/documents/gs4dhdaa2a9f352b0445bafbc79ca7... (accessed 5 June 2023).
Wikipedia 2022
- Wikipedia. Shadow IT. en.wikipedia.org/w/index.php?title=Shadow_IT&oldid=1114504202 (accessed 18 October 2022).
- Wu R, Rossos P, Quan S, Reeves S, Lo V, Wong B, et al. An evaluation of the use of smartphones to communicate between clinicians: a mixed-methods study. Journal of Medical Internet Research 2011;13(3):e59. [DOI: 10.2196/jmir.1655: 10.2196/jmir.1655] - PMC - PubMed
References to other published versions of this review
Glenton 2023b.
- Glenton C, Paulsen E, Agarwal S, Gopinathan U, Johansen M, Kyaddondo D, et al. Healthcare workers’ informal uses of mobile devices to support their work: a qualitative evidence synthesis. Cochrane Database of Systematic Reviews 2023, Issue 7. Art. No: CD015705. [DOI: 10.1002/14651858.CD015705] - DOI
Publication types
- Search in MeSH
LinkOut - more resources
Full text sources, miscellaneous.
- NCI CPTAC Assay Portal

- Citation Manager
NCBI Literature Resources
MeSH PMC Bookshelf Disclaimer
The PubMed wordmark and PubMed logo are registered trademarks of the U.S. Department of Health and Human Services (HHS). Unauthorized use of these marks is strictly prohibited.

An official website of the United States government
The .gov means it’s official. Federal government websites often end in .gov or .mil. Before sharing sensitive information, make sure you’re on a federal government site.
The site is secure. The https:// ensures that you are connecting to the official website and that any information you provide is encrypted and transmitted securely.
- Publications
- Account settings
Preview improvements coming to the PMC website in October 2024. Learn More or Try it out now .
- Advanced Search
- Journal List
- Springer Nature - PMC COVID-19 Collection

The impact of smartphone use on learning effectiveness: A case study of primary school students
Jen chun wang.
1 Department of Industry Technology Education, National Kaohsiung Normal University, 62, Shenjhong Rd., Yanchao District, Kaohsiung, 82446 Taiwan
Chia-Yen Hsieh
2 Department of Early Childhood Education, National PingTung University, No.4-18, Minsheng Rd., Pingtung City, Pingtung County 900391 Taiwan
Shih-Hao Kung
Associated data.
The datasets generated during and/or analysed during the current study are available from the corresponding author upon request.
This study investigated the effects of smartphone use on the perceived academic performance of elementary school students. Following the derivation of four hypotheses from the literature, descriptive analysis, t testing, one-way analysis of variance (ANOVA), Pearson correlation analysis, and one-way multivariate ANOVA (MANOVA) were performed to characterize the relationship between smartphone behavior and academic performance with regard to learning effectiveness. All coefficients were positive and significant, supporting all four hypotheses. We also used structural equation modeling (SEM) to determine whether smartphone behavior is a mediator of academic performance. The MANOVA results revealed that the students in the high smartphone use group academically outperformed those in the low smartphone use group. The results indicate that smartphone use constitutes a potential inequality in learning opportunities among elementary school students. Finally, in a discussion of whether smartphone behavior is a mediator of academic performance, it is proved that smartphone behavior is the mediating variable impacting academic performance. Fewer smartphone access opportunities may adversely affect learning effectiveness and academic performance. Elementary school teachers must be aware of this issue, especially during the ongoing COVID-19 pandemic. The findings serve as a reference for policymakers and educators on how smartphone use in learning activities affects academic performance.
Introduction
The advent of the Fourth Industrial Revolution has stimulated interest in educational reforms for the integration of information and communication technology (ICT) into instruction. Smartphones have become immensely popular ICT devices. In 2019, approximately 96.8% of the global population had access to mobile devices with the coverage rate reaching 100% in various developed countries (Sarker et al., 2019 ). Given their versatile functions, smartphones have been rapidly integrated into communication and learning, among other domains, and have become an inseparable part of daily life for many. Smartphones are perceived as convenient, easy-to-use tools that promote interaction and multitasking and facilitate both formal and informal learning (Looi et al., 2016 ; Yi et al., 2016 ). Studies have investigated the impacts of smartphones in education. For example, Anshari et al. ( 2017 ) asserted that the advantages of smartphones in educational contexts include rich content transferability and the facilitation of knowledge sharing and dynamic learning. Modern students expect to experience multiple interactive channels in their studies. These authors also suggested incorporating smartphones into the learning process as a means of addressing inappropriate use of smartphones in class (Anshari et al., 2017 ). For young children, there are differences in demand and attributes and some need for control depending upon the daily smartphone usage of the children (Cho & Lee, 2017 ). To avoid negative impacts, including interference with the learning process, teachers should establish appropriate rules and regulations. In a study by Bluestein and Kim ( 2017 ) on the use of technology in the classroom they examined three themes: acceptance of tablet technology, learning excitement and engagement, and the effects of teacher preparedness and technological proficiency. They suggested that teachers be trained in application selection and appropriate in-class device usage. Cheng et al. ( 2016 ) found that smartphone use facilitated English learning in university students. Some studies have provided empirical evidence of the positive effects of smartphone use, whereas others have questioned the integration of smartphone use into the academic environment. For example, Hawi and Samaha ( 2016 ) investigated whether high academic performance was possible for students at high risk of smartphone addiction. They provided strong evidence of the adverse effects of smartphone addiction on academic performance. Lee et al. ( 2015 ) found a negative correlation between smartphone addiction and learning in university students. There has been a lot of research on the effectiveness of online teaching, but the results are not consistent. Therefore, this study aims to further explore the effects of independent variables on smartphone use behavior and academic performance.
The COVID-19 pandemic has caused many countries to close schools and suspend in-person classes, enforcing the transition to online learning. Carrillo and Flores ( 2020 ) suggested that because of widespread school closures, teachers must learn to manage the online learning environment. Online courses have distinct impacts on students and their families, requiring adequate technological literacy and the formulation of new teaching or learning strategies (Sepulveda-Escobar & Morrison, 2020 ). Since 2020, numerous studies have been conducted on parents’ views regarding the relationship of online learning, using smartphones, computers, and other mobile devices, with learning effectiveness. Widely inconsistent findings have been reported. For instance, in a study by Hadad et al. ( 2020 ), two thirds of parents were opposed to the use of smartphones in school, with more than half expressing active opposition ( n = 220). By contrast, parents in a study by Garbe et al. ( 2020 ) agreed to the school closure policy and allowed their children to use smartphones to attend online school. Given the differences in the results, further scholarly discourse on smartphone use in online learning is essential.
Questions remain on whether embracing smartphones in learning systems facilitates or undermines learning (i.e., through distraction). Only a few studies have been conducted on the impacts of smartphone use on academic performance in elementary school students (mostly investigating college or high school students). Thus, we investigated the effects of elementary school students’ smartphone use on their academic performance.
Literature review
Mobile technologies have driven a paradigm shift in learning; learning activities can now be performed anytime, anywhere, as long as the opportunity to obtain information is available (Martin & Ertzberger, 2013 ).
Kim et al. ( 2014 ) focused on identifying factors that influence smartphone adoption or use. Grant and Hsu ( 2014 ) centered their investigation on user behavior, examining the role of smartphones as learning devices and social interaction tools. Although the contribution of smartphones to learning is evident, few studies have focused on the connection between smartphones and learning, especially in elementary school students. The relationship between factors related to learning with smartphones among this student population is examined in the following sections.
Behavioral intentions of elementary school students toward smartphone use
Children experience rapid growth and development during elementary school and cultivate various aspects of the human experience, including social skills formed through positive peer interactions. All these experiences exert a substantial impact on the establishment of self-esteem and a positive view of self. Furthermore, students tend to maintain social relationships by interacting with others through various synchronous or asynchronous technologies, including smartphone use (Guo et al., 2011 ). Moreover, students favor communication through instant messaging, in which responses are delivered rapidly. However, for this type of interaction, students must acquire knowledge and develop skills related to smartphones or related technologies which has an impact on social relationships (Kang & Jung, 2014 ; Park & Lee, 2012 ).
Karikoski and Soikkeli ( 2013 ) averred that smartphone use promotes human-to-human interaction both through verbal conversation and through the transmission of textual and graphic information, and cn stimulate the creation and reinforcement of social networks. Park and Lee ( 2012 ) examined the relationship between smartphone use and motivation, social relationships, and mental health. The found smartphone use to be positively correlated with social intimacy. Regarding evidence supporting smartphone use in learning, Firmansyah et al. ( 2020 ) concluded that smartphones significantly benefit student-centered learning, and they can be used in various disciplines and at all stages of education. They also noted the existence of a myriad smartphone applications to fulfill various learning needs. Clayton and Murphy ( 2016 ) suggested that smartphones be used as a mainstay in classroom teaching, and that rather than allowing them to distract from learning, educators should help their students to understand how smartphones can aid learning and facilitate civic participation. In other words, when used properly, smartphones have some features that can lead to better educational performance. For example, their mobility can allow students access to the same (internet-based) services as computers, anytime, anywhere (Lepp et al., 2014 ). Easy accessibility to these functionalities offers students the chance to continuously search for study-related information. Thus, smartphones can provide a multi-media platform to facilitate learning which cannot be replaced by simply reading a textbook (Zhang et al., 2014 ). Furthermore, social networking sites and communication applications may also contribute to the sharing of relevant information. Faster communication between students and between students and faculty may also contribute to more efficient studying and collaboration (Chen et al., 2015 ). College students are more likely to have access to smartphones than elementary school students. The surge in smartphone ownership among college students has spurred interest in studying the impact of smartphone use on all aspects of their lives, especially academic performance. For example, Junco and Cotton ( 2012 ) found that spending a fair amount of time on smartphones while studying had a negative affect on the university student's Grade Point Average (GPA). In addition, multiple studies have found that mobile phone use is inversely related to academic performance (Judd, 2014 ; Karpinski et al., 2013 ). Most research on smartphone use and academic performance has focused on college students. There have few studies focused on elementary school students. Vanderloo ( 2014 ) argued that the excessive use of smartphones may cause numerous problems for the growth and development of children, including increased sedentary time and reduced physical activity. Furthermore, according to Sarwar and Soomro ( 2013 ), rapid and easy access to information and its transmission may hinder concentration and discourage critical thinking and is therefore not conducive to children’s cognitive development.
To sum up, the evidence on the use of smartphones by elementary school students is conflicting. Some studies have demonstrated that smartphone use can help elementary school students build social relationships and maintain their mental health, and have presented findings supporting elementary students’ use of smartphones in their studies. Others have opposed smartphone use in this student population, contending that it can impede growth and development. To take steps towards resolving this conflict, we investigated smartphone use among elementary school students.
In a study conducted in South Korea, Kim ( 2017 ) reported that 50% of their questionnaire respondents reported using smartphones for the first time between grades 4 and 6. Overall, 61.3% of adolescents reported that they had first used smartphones when they were in elementary school. Wang et al. ( 2017 ) obtained similar results in an investigation conducted in Taiwan. However, elementary school students are less likely to have access to smartphones than college students. Some elementary schools in Taiwan prohibit their students from using smartphones in the classroom (although they can use them after school). On the basis of these findings, the present study focused on fifth and sixth graders.
Jeong et al. ( 2016 ), based on a sample of 944 respondents recruited from 20 elementary schools, found that people who use smartphones for accessing Social Network Services (SNS), playing games, and for entertainment were more likely to be addicted to smartphones. Park ( 2020 ) found that games were the most commonly used type of mobile application among participants, comprised of 595 elementary school students. Greater smartphone dependence was associated with greater use of educational applications, videos, and television programs (Park, 2020 ). Three studies in Taiwan showed the same results, that elementary school students in Taiwan enjoy playing games on smartphones (Wang & Cheng, 2019 ; Wang et al., 2017 ). Based on the above, it is reasonable to infer that if elementary school students spend more time playing games on their smartphones, their academic performance will decline. However, several studies have found that using smartphones to help with learning can effectively improve academic performance. In this study we make effort to determine what the key influential factors that affect students' academic performance are.
Kim ( 2017 ) reported that, in Korea, smartphones are used most frequentlyfrom 9 pm to 12 am, which closely overlaps the corresponding period in Taiwan, from 8 to 11 pm In this study, we not only asked students how they obtained their smartphones, but when they most frequently used their smartphones, and who they contacted most frequently on their smartphones were, among other questions. There were a total of eight questions addressing smartphone behavior. Recent research on smartphones and academic performance draws on self-reported survey data on hours and/or minutes of daily use (e.g. Chen et al., 2015 ; Heo & Lee, 2021 ; Lepp et al., 2014 ; Troll et al., 2021 ). Therefore, this study also uses self-reporting to investigate how much time students spend using smartphones.
Various studies have indicated that parental attitudes affect elementary school students’ behavioral intentions toward smartphone use (Chen et al., 2020 ; Daems et al., 2019 ). Bae ( 2015 ) determined that a democratic parenting style (characterized by warmth, supervision, and rational explanation) was related to a lower likelihood of smartphone addiction in children. Park ( 2020 ) suggested that parents should closely monitor their children’s smartphone use patterns and provide consistent discipline to ensure appropriate smartphone use. In a study conducted in Taiwan, Chang et al. ( 2019 ) indicated that restrictive parental mediation reduced the risk of smartphone addiction among children. In essence, parental attitudes critically influence the behavioral intention of elementary school students toward smartphone use. The effect of parental control on smartphone use is also investigated in this study.
Another important question related to student smartphone use is self-control. Jeong et al. ( 2016 ) found that those who have lower self-control and greater stress were more likely to be addicted to smartphones. Self-control is here defined as the ability to control oneself in the absence of any external force, trying to observe appropriate behavior without seeking immediate gratification and thinking about the future (Lee et al., 2015 ). Those with greater self-control focus on long-term results when making decisions. People are able to control their behavior through the conscious revision of automatic actions which is an important factor in retaining self-control in the mobile and on-line environments. Self-control plays an important role in smartphone addiction and the prevention thereof. Previous studies have revealed that the lower one’s self-control, the higher the degree of smartphone dependency (Jeong et al., 2016 ; Lee et al., 2013 ). In other words, those with higher levels of self-control are likely to have lower levels of smartphone addiction. Clearly, self-control is an important factor affecting smartphone usage behavior.
Reviewing the literature related to self-control, we start with self-determination theory (SDT). The SDT (Deci & Ryan, 2008 ) theory of human motivation distinguishes between autonomous and controlled types of behavior. Ryan and Deci ( 2000 ) suggested that some users engage in smartphone communications in response to perceived social pressures, meaning their behavior is externally motivated. However, they may also be intrinsically motivated in the sense that they voluntarily use their smartphones because they feel that mobile communication meets their needs (Reinecke et al., 2017 ). The most autonomous form of motivation is referred to as intrinsic motivation. Being intrinsically motivated means engaging in an activity for its own sake, because it appears interesting and enjoyable (Ryan & Deci, 2000 ). Acting due to social pressure represents an externally regulated behavior, which SDT classifies as the most controlled form of motivation (Ryan & Deci, 2000 ). Individuals engage in such behavior not for the sake of the behavior itself, but to achieve a separable outcome, for example, to avoid punishment or to be accepted and liked by others (Ryan & Deci, 2006 ). SDT presumes that controlled and autonomous motivations are not complementary, but “work against each other” (Deci et al., 1999 , p. 628). According to the theory, external rewards alter the perceived cause of action: Individuals no longer voluntarily engage in an activity because it meets their needs, but because they feel controlled (Deci et al., 1999 ). For media users, the temptation to communicate through the smartphone is often irresistible (Meier, 2017 ). Researchers who have examined the reasons why users have difficulty controlling media use have focused on their desire to experience need gratification, which produces pleasurable experiences. The assumption here is that users often subconsciously prefer short-term pleasure gains from media use to the pursuit of long-term goals (Du et al., 2018 ). Accordingly, self-control is very important. Self-control here refers to the motivation and ability to resist temptations (Hofmann et al., 2009 ). Dispositional self-control is a key moderator of yielding to temptation (Hofmann et al., 2009 ). Ryan and Deci ( 2006 ) suggested that people sometimes perform externally controlled behaviors unconsciously, that is, without applying self-control.
Sklar et al. ( 2017 ) described two types of self-control processes: proactive and reactive. They suggested that deficiencies in the resources needed to inhibit temptation impulses lead to failure of self-control. Even when impossible to avoid a temptation entirely, self-control can still be made easier if one avoids attending to the tempting stimulus. For example, young children instructed to actively avoid paying attention to a gift and other attention-drawing temptations are better able to resist the temptation than children who are just asked to focus on their task. Therefore, this study more closely investigates students' self-control abilities in relation to smartphone use asking the questions, ‘How did you obtain your smartphone?’ (to investigate proactivity), and ‘How much time do you spend on your smartphone in a day?’ (to investigate the effects of self-control).
Thus, the following hypotheses are advanced.
- Hypothesis 1: Smartphone behavior varies with parental control.
- Hypothesis 2: Smartphone behavior varies based on students' self-control.
Parental control, students' self-control and their effects on learning effectiveness and academic performance
Based on Hypothesis 1 and 2, we believe that we need to focus on two factors, parental control and student self-control and their impact on academic achievement. In East Asia, Confucianism is one of the most prevalent and influential cultural values which affect parent–child relations and parenting practice (Lee et al., 2016 ). In Taiwan, Confucianism shapes another feature of parenting practice: the strong emphasis on academic achievement. The parents’ zeal for their children’s education is characteristic of Taiwan, even in comparison to academic emphasis in other East Asian countries. Hau and Ho ( 2010 ) noted that, in Eastern Asian (Chinese) cultures, academic achievement does not depend on the students’ interests. Chinese students typically do not regard intelligence as fixed, but trainable through learning, which enables them to take a persistent rather than a helpless approach to schoolwork, and subsequently perform well. In Chinese culture, academic achievement has been traditionally regarded as the passport to social success and reputation, and a way to enhance the family's social status (Hau & Ho, 2010 ). Therefore, parents dedicate a large part of their family resources to their children's education, a practice that is still prevalent in Taiwan today (Hsieh, 2020 ). Parental control aimed at better academic achievement is exerted within the behavioral and psychological domains. For instance, Taiwan parents tightly schedule and control their children’s time, planning private tutoring after school and on weekends. Parental control thus refers to “parental intrusiveness, pressure, or domination, with the inverse being parental support of autonomy” (Grolnick & Pomerantz, 2009 ). There are two types of parental control: behavioral and psychological. Behavioral control, which includes parental regulation and monitoring over what children do (Steinberg et al., 1992 ), predict positive psychosocial outcomes for children. Outcomes include low externalizing problems, high academic achievement (Stice & Barrera, 1995 ), and low depression. In contrast, psychological control, which is exerted over the children’s psychological world, is known to be problematic (Stolz et al., 2005 ). Psychological control involves strategies such as guilt induction and love withdrawal (Steinberg et al., 1992 ) and is related with disregard for children’s emotional autonomy and needs (Steinberg et al., 1992 ). Therefore, it is very important to discuss the type of parental control.
Troll et al. ( 2021 ) suggested that it is not the objective amount of smartphone use but the effective handling of smartphones that helps students with higher trait self-control to fare better academically. Heo and Lee ( 2021 ) discussed the mediating effect of self-control. They found that self-control was partially mediated by those who were not at risk for smartphone addiction. That is to say, smartphone addiction could be managed by strengthening self-control to promote healthy use. In an earlier study Hsieh and Lin ( 2021 ), we collected 41 international journal papers involving 136,491students across 15 countries, for meta-analysis. We found that the average and majority of the correlations were both negative. The short conclusion here was that smartphone addiction /reliance may have had a negative impact on learning performance. Clearly, it is very important to investigate the effect of self-control on learning effectiveness with regard to academic performance.
Smartphone use and its effects on learning effectiveness and academic performance
The impact of new technologies on learning or academic performance has been investigated in the literature. Kates et al. ( 2018 ) conducted a meta-analysis of 39 studies published over a 10-year period (2007–2018) to examine potential relationships between smartphone use and academic achievement. The effect of smartphone use on learning outcomes can be summarized as follows: r = − 0.16 with a 95% confidence interval of − 0.20 to − 0.13. In other words, smartphone use and academic achievement were negatively correlated. Amez and Beart ( 2020 ) systematically reviewed the literature on smartphone use and academic performance, observing the predominance of empirical findings supporting a negative correlation. However, they advised caution in interpreting this result because this negative correlation was less often observed in studies analyzing data collected through paper-and-pencil questionnaires than in studies on data collected through online surveys. Furthermore, this correlation was less often noted in studies in which the analyses were based on self-reported grade point averages than in studies in which actual grades were used. Salvation ( 2017 ) revealed that the type of smartphone applications and the method of use determined students’ level of knowledge and overall grades. However, this impact was mediated by the amount of time spent using such applications; that is, when more time is spent on educational smartphone applications, the likelihood of enhancement in knowledge and academic performance is higher. This is because smartphones in this context are used as tools to obtain the information necessary for assignments and tests or examinations. Lin et al. ( 2021 ) provided robust evidence that smartphones can promote improvements in academic performance if used appropriately.
In summary, the findings of empirical investigations into the effects of smartphone use have been inconsistent—positive, negative, or none. Thus, we explore the correlation between elementary school students’ smartphone use and learning effectiveness with regard to academic performance through the following hypotheses:
- Hypothesis 3: Smartphone use is associated with learning effectiveness with regard to academic performance.
- Hypothesis 4: Differences in smartphone use correspond to differences in learning effectiveness with regard to academic performance.
Hypotheses 1 to 4 are aimed at understanding the mediating effect of smartphone behavior; see Fig. 1 . It is assumed that smartphone behavior is the mediating variable, parental control and self-control are independent variables, and academic performance is the dependent variable. We want to understand the mediation effect of this model.

Model 1: Model to test the impact of parental control and students’ self-control on academic performance
Thus, the following hypotheses are presented.
- Hypothesis 5: Smartphone behaviors are the mediating variable to impact the academic performance.
Effects of the COVID-19 pandemic on smartphone use for online learning
According to 2020 statistics from the United Nations Educational, Scientific and Cultural Organization (UNESCO), since the start of the COVID-19 pandemic, full or partial school closures have affected approximately 800 million learners worldwide, more than half of the global student population. Schools worldwide have been closed for 14 to 22 weeks on average, equivalent to two thirds of an academic year (UNESCO, 2021 ). Because of the pandemic, instructors have been compelled to transition to online teaching (Carrillo & Flores, 2020 ). According to Tang et al. ( 2020 ), online learning is among the most effective responses to the COVID-19 pandemic. However, the effectiveness of online learning for young children is limited by their parents’ technological literacy in terms of their ability to navigate learning platforms and use the relevant resources. Parents’ time availability constitutes another constraint (Dong et al., 2020 ). Furthermore, a fast and stable Internet connection, as well as access to devices such as desktops, laptops, or tablet computers, definitively affects equity in online education. For example, in 2018, 14% of households in the United States lacked Internet access (Morgan, 2020 ). In addition, the availability and stability of network connections cannot be guaranteed in relatively remote areas, including some parts of Australia (Park et al., 2021 ). In Japan, more than 50% of 3-year-old children and 68% of 6-year-old children used the Internet in their studies, but only 21% of households in Thailand have computer equipment (Park et al., 2021 ).
In short, the COVID-19 pandemic has led to changes in educational practices. With advances in Internet technology and computer hardware, online education has become the norm amid. However, the process and effectiveness of learning in this context is affected by multiple factors. Aside from the parents’ financial ability, knowledge of educational concepts, and technological literacy, the availability of computer equipment and Internet connectivity also exert impacts. This is especially true for elementary school students, who rely on their parents in online learning more than do middle or high school students, because of their short attention spans and undeveloped computer skills. Therefore, this study focuses on the use of smartphones by elementary school students during the COVID-19 pandemic and its impact on learning effectiveness.
Participants
Participants were recruited through stratified random sampling. They comprised 499 Taiwanese elementary school students (in grades 5 and 6) who had used smartphones for at least 12 months. Specifically, the students advanced to grades 5 or 6 at the beginning of the 2018–2019 school year. Boys and girls accounted for 47.7% and 52.3% ( n = 238 and 261, respectively) of the sample.
Data collection and measurement
In 2020, a questionnaire survey was conducted to collect relevant data. Of the 620 questionnaires distributed, 575 (92.7%) completed questionnaires were returned. After 64 participants were excluded because they had not used their smartphones continually over the past 12 months and 14 participants were excluded for providing invalid responses, 499 individuals remained. The questionnaire was developed by one of the authors on the basis of a literature review. The questionnaire content can be categorized as follows: (1) students’ demographic characteristics, (2) smartphone use, (3) smartphone behavior, and (4) learning effectiveness. The questionnaire was modified according to evaluation feedback provided by six experts. Exploratory and confirmatory factor analyses were conducted to test the structural validity of the questionnaire. Factor analysis was performed using principal component analysis and oblique rotation. From the exploratory factor analysis, 25 items (15 and 10 items on smartphone behavior and academic performance as constructs, respectively) were extracted and confirmed. According to the results of the exploratory factor analysis, smartphone behavior can be classified into three dimensions: interpersonal communication, leisure and entertainment, and searching for information. Interpersonal communication is defined as when students use smartphones to communicate with classmates or friends, such as in response to questions like ‘I often use my smartphone to call or text my friends’. Leisure and entertainment mean that students spend a lot of their time using their smartphones for leisure and entertainment, e.g. ‘I often use my smartphone to listen to music’ or ‘I often play media games with my smartphone’. Searching for information means that students spend a lot of their time using their smartphones to search for information that will help them learn, such as in response to questions like this ‘I often use my smartphone to search for information online, such as looking up words in a dictionary’ or ‘I will use my smartphone to read e-books and newspapers online’.
Academic performance can be classified into three dimensions: learning activities, learning applications, and learning attitudes. Learning activities are when students use their smartphones to help them with learning, such as in response to a question like ‘I often use some online resources from my smartphone to help with my coursework’. Learning applications are defined as when students apply smartphone software to help them with their learning activities, e.g. ‘With a smartphone, I am more accustomed to using multimedia software’. Learning attitudes define the students’ attitudes toward using the smartphone, with questions like ‘Since I have had a smartphone, I often find class boring; using a smartphone is more fun’ (This is a reverse coded item). The factor analysis results are shown in the appendix (Appendix Tables Tables10, 10 , ,11, 11 , ,12, 12 , ,13 13 and and14). 14 ). It can be seen that the KMO value is higher than 0.75, and the Bartlett’s test is also significant. The total variance explained for smartphone behavior is 53.47% and for academic performance it is 59.81%. These results demonstrate the validity of the research tool.
KMO and Bartlett's Test
Construct name | KMO | Bartlett's Test of Sphericity | df | Value |
---|---|---|---|---|
Smartphone behavior | .846 | 2198.32*** | 105 | < .001 |
Academic performance | .731 | 1128.61*** | 45 | < .001 |
Total variance explained of smartphone behavior
Construct name | Total Variance Explained | ||
---|---|---|---|
Eigenvalues | % of Variance | Cumulative % | |
Interpersonal communication | 4.40 | 29.33 | 29.33 |
Leisure and entertainment | 2.27 | 15.13 | 44.47 |
Information searches | 1.35 | 9.00 | 53.47 |
Total variance explained of academic performance
Construct name | Total Variance Explained | ||
---|---|---|---|
Eigenvalues | % of Variance | Cumulative % | |
Learning activities | 2.93 | 29.30 | 29.30 |
Learning applications | 2.94 | 19.41 | 47.71 |
Learning attitudes | 1.11 | 11.09 | 59.81 |
Factor loading of smartphone behavior
Item No | Communalities | factor | ||
---|---|---|---|---|
1 | 2 | 3 | ||
1 | .466 | .695 | ||
2 | .527 | .739 | ||
3 | .357 | .561 | ||
4 | .631 | .693 | ||
5 | .504 | .666 | ||
6 | .718 | .513 | ||
7 | .490 | 610 | ||
8 | .540 | .601 | ||
9 | .383 | .618 | ||
10 | .358 | .522 | ||
11 | .557 | .766 | ||
12 | .512 | .768 | ||
13 | .740 | .805 | ||
14 | .671 | .792 | ||
15 | .568 | .748 |
Factor loading of academic performance
Item No | Communalities | factor | ||
---|---|---|---|---|
1 | 2 | 3 | ||
1 | .568 | .522 | ||
2 | .651 | .731 | ||
3 | .491 | .575 | ||
4 | .675 | .842 | ||
5 | .726 | .873 | ||
6 | .535 | .663 | ||
7 | .585 | .588 | ||
8 | .653 | .730 | ||
9 | .505 | .832 |
In this study, students were defined as "proactive" if they had asked their parents to buy a smartphone for their own use and "reactive" if their parents gave them a smartphone unsolicited (i.e. they had not asked for it). According to Heo and Lee ( 2021 ), students who proactively asked their parents to buy them a smartphone gave the assurance that they could control themselves and not become addicted, but if they had been given a smartphone (without having to ask for it), they did not need to offer their parents any such guarantees. They defined user addiction (meaning low self-control) as more than four hours of smartphone use per day (Peng et al., 2022 ).
A cross-tabulation of self-control results is presented in Table Table2, 2 , with the columns representing “proactive” and “reactive”, and the rows showing “high self-control” and “low self-control”. There are four variables in this cross-tabulation, “Proactive high self-control” (students promised parents they would not become smartphone addicts and were successful), “Proactive low self-control” (assured their parents they would not become smartphone addicts, but were unsuccessful), “Reactive high self-control”, and “Reactive low self-control”.
Cross-tabulation of self-control ability
Students who asked their parents to buy them a smartphone | Students whose parents gave them a smartphone without being asked | |
---|---|---|
Using a smartphone for less than 4 h a day | Proactive high self-control | Reactive high self-control |
Using a smartphone for more than 4 h a day | Proactive low self-control | Reactive low self-control |
Regarding internal consistency among the constructs, the Cronbach's α values ranged from 0.850 to 0.884. According to the guidelines established by George and Mallery ( 2010 ), these values were acceptable because they exceeded 0.7. The overall Cronbach's α for the constructs was 0.922. The Cronbach's α value of the smartphone behavior construct was 0.850, whereas that of the academic performance construct was 0.884.
Data analysis
The participants’ demographic characteristics and smartphone use (expressed as frequencies and percentages) were subjected to a descriptive analysis. To examine hypotheses 1 and 2, an independent samples t test (for gender and grade) and one-way analysis of variance (ANOVA) were performed to test the differences in smartphone use and learning effectiveness with respect to academic performance among elementary school students under various background variables. To test hypothesis 3, Pearson’s correlation analysis was conducted to analyze the association between smartphone behavior and academic performance. To test hypothesis 4, one-way multivariate ANOVA (MANOVA) was employed to examine differences in smartphone behavior and its impacts on learning effectiveness. To test Hypothesis 5, structural equation modeling (SEM) was used to test whether smartphone behavior is a mediator of academic performance.
Descriptive analysis
The descriptive analysis (Table (Table1) 1 ) revealed that the parents of 71.1% of the participants ( n = 499) conditionally controlled their smartphone use. Moreover, 42.5% of the participants noted that they started using smartphones in grade 3 or 4. Notably, 43.3% reported that they used their parents’ old smartphones; in other words, almost half of the students used secondhand smartphones. Overall, 79% of the participants indicated that they most frequently used their smartphones after school. Regarding smartphone use on weekends, 54.1% and 44.1% used their smartphones during the daytime and nighttime, respectively. Family members and classmates (45.1% and 43.3%, respectively) were the people that the participants communicated with the most on their smartphones. Regarding bringing their smartphones to school, 53.1% of the participants indicated that they were most concerned about losing their phones. As for smartphone use duration, 28.3% of the participants indicated that they used their smartphones for less than 1 h a day, whereas 24.4% reported using them for 1 to 2 h a day.
Descriptive analysis results
Demographic variable | Variable | Number | Percentage (%) |
---|---|---|---|
Gender | Male | 238 | 47.7 |
Female | 261 | 52.3 | |
Grade | Fifth | 244 | 48.9 |
Sixth | 255 | 51.1 | |
What are your parents' attitudes toward controlling your smartphone use (Parental control) | Strict | 46 | 9.2 |
Conditional | 355 | 71.1 | |
None | 98 | 19.6 | |
When did you first start using a smartphone? | Before elementary school | 96 | 19.2 |
Grades 1 to 2 | 118 | 23.6 | |
Grades 3 to 4 | 212 | 42.5 | |
Grades 5 to 6 | 73 | 14.6 | |
How did you obtain your smartphone? | My parents bought it for me | 184 | 36.9 |
My parents gave me their old smartphone | 216 | 43.3 | |
I asked my parents to buy it for me | 35 | 7 | |
I asked my parents, and they gave me their old smartphone | 64 | 12.8 | |
When do you use your smartphone the most often? | Before school | 8 | 1.6 |
After school | 394 | 79 | |
At school | 97 | 19.4 | |
At what time of day do you most frequently use your smartphone on weekends? | Daytime | 270 | 54.1 |
Nighttime | 220 | 44.1 | |
All day | 9 | 1.8 | |
Who do you contact the most often on your smartphone? | Family member(s) | 225 | 45.1 |
Teacher(s) | 2 | 0.4 | |
Classmate(s) | 221 | 44.3 | |
Others | 51 | 10.2 | |
Do you usually bring your smartphone to school? | Yes | 26 | 5.2 |
No | 393 | 78.8 | |
Depends on the situation | 80 | 16 | |
What is your greatest concern about bringing your smartphone to school? | I’m not concerned about this | 73 | 14.6 |
That I’ll lose it | 265 | 53.1 | |
That it’ll be confiscated | 64 | 12.8 | |
That it’ll be compared with my classmates’ smartphones | 6 | 1.2 | |
That my classmates will use it | 36 | 7.2 | |
Other | 55 | 11 | |
What is your greatest concern about smartphone use? | I’m not concerned about this | 212 | 42.5 |
Signal interference | 124 | 24.8 | |
An increase in my phone bill | 16 | 3.2 | |
Effects of electromagnetic radiation | 32 | 6.4 | |
Negative effects on my studies | 78 | 15.6 | |
Other | 37 | 7.4 | |
How much time do you spend on your smartphone in a day? | Less than 1 h | 141 | 28.3 |
1 to 2 h | 122 | 24.4 | |
2 to 3 h | 80 | 16 | |
3 to 4 h | 58 | 11.6 | |
More than 4 h | 98 | 19.6 | |
Self-control | Proactive high self-control | 278 | 55.7 |
Reactive high self-control | 66 | 13 | |
Proactive low self-control | 122 | 24.4 | |
Reactive low self-control | 34 | 6.8 |
Smartphone behavior varies with parental control and based on students' self-control
We used the question ‘How did you obtain your smartphone?’ (to investigate proactivity), and ‘How much time do you spend on your smartphone in a day?’ (to investigate the effects of students' self-control). According to the Hsieh and Lin ( 2021 ), and Peng et al. ( 2022 ), addition is defined more than 4 h a day are defined as smartphone addiction (meaning that students have low self-control).
Table Table2 2 gives the cross-tabulation results for self-control ability. Students who asked their parents to buy a smartphone, but use it for less than 4 h a day are defined as having ‘Proactive high self-control’; students using a smartphone for more than 4 h a day are defined as having ‘Proactive low self-control’. Students whose parents gave them a smartphone but use them for less than 4 h a day are defined as having ‘Reactive high self-control’; students given smart phones and using them for more than 4 h a day are defined as having ‘Reactive low self-control’; others, we define as having moderate levels of self-control.
Tables Tables3 3 – 5 present the results of the t test and analysis of covariance (ANCOVA) on differences in the smartphone behaviors based on parental control and students' self-control. As mentioned, smartphone behavior can be classified into three dimensions: interpersonal communication, leisure and entertainment, and information searches. Table Table3 3 lists the significant independent variables in the first dimension of smartphone behavior based on parental control and students' self-control. Among the students using their smartphones for the purpose of communication, the proportion of parents enforcing no control over smartphone use was significantly higher than the proportions of parents enforcing strict or conditional control ( F = 11.828, p < 0.001). This indicates that the lack of parental control over smartphone use leads to the participants spending more time using their smartphones for interpersonal communication.
Significant independent variables (Parental control and Self-control) in the first dimension (interpersonal communication) of smartphone use
Independent variable | Variable | Number | Mean | SD | F value | A posteriori comparison |
---|---|---|---|---|---|---|
Parental control | (1) Strict | 46 | 3.13 | 1.17 | 11.82*** | 3 > 1,2 |
(2) Conditional | 355 | 3.51 | 0.99 | |||
(3) None | 98 | 3.93 | 0.84 | |||
Self-control | (1) Proactive high self-control | 278 | 3.47 | 0.05 | 18.88*** | 1,3,4 > 2 |
(2) Reactive high self-control | 66 | 2.95 | 0.11 | |||
(3) Proactive low self-control | 122 | 3.98 | 0.08 | |||
(4) Reactive low self-control | 34 | 3.91 | 1.16 |
*** p < .001
Independent variables (Parental control and Self-control) in the third dimension (information searches) of smartphone behavior
Independent variable | Variable | Number | Mean | SD | value/F value | A posteriori comparison |
---|---|---|---|---|---|---|
Gender | Male (boys) | 238 | 3.52 | 0.96 | − 3.979*** | girls > boys |
Female (girls) | 261 | 3.74 | 0.88 | |||
Parental control | (1) Strict | 46 | 3.77 | 1.05 | 0.72 | |
(2) Conditional | 355 | 3.60 | 0.92 | |||
(3) None | 98 | 3.67 | 0.88 | |||
Self-control | (1) Proactive high self-control | 278 | 3.63 | 0.94 | 0.35 | |
(2) Reactive high self-control | 66 | 3.62 | 1.00 | |||
(3) Proactive low self-control | 122 | 3.62 | 0.86 | |||
(4) Reactive low self-control | 34 | 3.67 | 0.91 |
SD standard deviation
For the independent variable of self-control, regardless of whether students had proactive high self-control, proactive low self-control or reactive low self-control, significantly higher levels of interpersonal communication than reactive high self-control were reported ( F = 18.88, p < 0.001). This means that students effectively able to control themselves, who had not asked their parents to buy them smartphones, spent less time using their smartphones for interpersonal communication. However, students with high self-control but who had asked their parents to buy them smartphones, would spend more time on interpersonal communication (meaning that while they may not spend a lot of time on their smartphones each day, the time spent on interpersonal communication is no different than for the other groups). Those without effective self-control, regardless of whether they had actively asked their parents to buy them a smartphone or not, would spend more time using their smartphones for interpersonal communication.
Table Table4 4 displays the independent variables (parental control and students' self-control) significant in the dimension of leisure and entertainment. Among the students using their smartphones for this purpose, the proportion of parents enforcing no control over smartphone use was significantly higher than the proportions of parents enforcing strict or conditional control ( F = 8.539, p < 0.001). This indicates that the lack of parental control over smartphone use leads to the participants spending more time using their smartphones for leisure and entertainment.
Significant independent variables (Parental control and Self-control) in the second dimension (leisure and entertainment) of smartphone behavior
Independent variable | Variable | Number | Mean | SD | F value | A posteriori comparison |
---|---|---|---|---|---|---|
Parental control | (1) Strict | 46 | 3.11 | 0.87 | 8.53*** | 3 > 1,2 |
(2) Conditional | 355 | 3.37 | 0.65 | |||
(3) None | 98 | 3.59 | 0.67 | |||
Self-control | (1) Proactive high self-control | 278 | 3.31 | 0.69 | 8.77*** | 3,4 > 1,2 |
(2) Reactive high self-control | 66 | 3.19 | 0.76 | |||
(3) Proactive low self-control | 122 | 3.62 | 0.57 | |||
(4) Reactive low self-control | 34 | 3.59 | 0.59 |
For the independent variable of self-control, students with proactive low self-control and reactive low self-control reported significantly higher use of smartphones for leisure and entertainment than did students with proactive high self-control and reactive high self-control ( F = 8.77, p < 0.001). This means that students who cannot control themselves, whether proactive or passive in terms of asking their parents to buy them a smartphone, will spend more time using their smartphones for leisure and entertainment.
Table Table5 5 presents the significant independent variables in the dimension of information searching. Significant differences were observed only for gender, with a significantly higher proportion of girls using their smartphones to search for information ( t = − 3.979, p < 0.001). Parental control and students' self-control had no significance in the dimension of information searching. This means that the parents' attitudes towards control did not affect the students' use of smartphones for information searches. This is conceivable, as Asian parents generally discourage their children from using their smartphones for non-study related activities (such as entertainment or making friends), but not for learning-related activities. It is also worth noting that student self-control was not significant in relation to searching for information. This means that it makes no difference whether or not students have self-control in their search for learning-related information.
Four notable results are presented as follows.
First, a significantly higher proportion of girls used their smartphones to search for information. Second, if smartphone use was not subject to parental control, the participants spent more time using their smartphones for interpersonal communication and for leisure and entertainment rather than for information searches. This means that if parents make the effort to control their children's smartphone use, this will reduce their children's use of smartphones for interpersonal communication and entertainment. Third, student self-control affects smartphone use behavior for interpersonal communication and entertainment (but not searching for information). This does not mean that they spend more time on their smartphones in their daily lives, it means that they spend the most time interacting with people while using their smartphones (For example, they may only spend 2–3 h a day using their smartphone. During those 2–3 h, they spend more than 90% of their time interacting with people and only 10% doing other things), which is the fourth result.
These results support hypotheses 1 and 2.
Pearson’s correlation analysis of smartphone behavior and academic performance
Table Table6 6 presents the results of Pearson’s correlation analysis of smartphone behavior and academic performance. Except for information searches and learning attitudes, all variables exhibited significant and positively correlations. In short, there was a positive correlation between smartphone behavior and academic performance. Thus, hypothesis 3 is supported.
Pearson’s correlation analysis of smartphone use and academic performance
Variable | Interpersonal communication | Leisure and entertainment | Information searches | Smartphone behavior |
---|---|---|---|---|
Learning activities | .369** | .342** | 176** | .382** |
Learning applications | .436** | .435** | .472** | .565** |
Learning attitudes | .286** | .330** | .027 | .286** |
Academic performance | .486** | .493** | .321** | .557** |
** p < .01
Analysis of differences in the academic performance of students with different smartphone behaviors
Differences in smartphone behavior and its impacts on learning effectiveness with regard to academic performance were examined through. In step 1, cluster analysis was conducted to convert continuous variables into discrete variables. In step 2, a one-way MANOVA was performed to analyze differences in the academic performance of students with varying smartphone behavior. Regarding the cluster analysis results (Table (Table7), 7 ), the value of the change in the Bayesian information criterion in the second cluster was − 271.954, indicating that it would be appropriate to group the data. Specifically, we assigned the participants into either the high smartphone use group or the low smartphone use group, comprised of 230 and 269 participants (46.1% and 53.9%), respectively.
Cluster analysis results
Number of Clusters | BIC | BIC change | Ratio of BIC change | Ratio of distance measures |
---|---|---|---|---|
1 | 1073.416 | |||
2 | 801.463 | − 271.954 | 1.000 | 2.397 |
3 | 709.753 | − 91.710 | .337 | 1.880 |
4 | 678.418 | − 31.335 | .115 | 1.378 |
5 | 665.887 | − 12.531 | .046 | 1.120 |
6 | 658.695 | − 7.192 | .026 | 1.052 |
7 | 653.697 | − 4.998 | .018 | 1.142 |
8 | 653.969 | .272 | − .001 | 1.674 |
9 | 669.141 | 15.172 | − .056 | 1.179 |
10 | 687.669 | 18.528 | − .068 | 1.178 |
11 | 709.026 | 21.357 | − .079 | 1.018 |
12 | 730.666 | 21.640 | − .080 | 1.088 |
13 | 753.570 | 22.904 | − .084 | 1.140 |
14 | 778.241 | 24.671 | − .091 | 1.009 |
15 | 803.025 | 24.785 | − .091 | 1.057 |
BIC Bayesian information criterion
The MANOVA was preceded by the Levene test for the equality of variance, which revealed nonsignificant results, F (6, 167,784.219) = 1.285, p > 0.05. Thus, we proceeded to use MANOVA to examine differences in the academic performance of students with differing smartphone behaviors (Table (Table8). 8 ). Between-group differences in academic performance were significant, F (3, 495) = 44.083, p < 0.001, Λ = 0.789, η 2 = 0.211, power = 0.999. Subsequently, because academic performance consists of three dimensions, we performed univariate tests and an a posteriori comparison.
Multivariate analysis of variance results
Effect | Λ | F | Hypothesis df | Error df | value | η | Observed power |
---|---|---|---|---|---|---|---|
Intercept | .041 | 3893.541 | 3 | 495 | < .001*** | .959 | .999 |
Group | .789 | 44.083 | 3 | 495 | < .001*** | .211 | .999 |
Df degrees of freedom
Table Table9 9 presents the results of the univariate tests. Between-group differences in learning activities were significant, ( F [1, 497] = 40.8, p < 0.001, η 2 = 0.076, power = 0.999). Between-group differences in learning applications were also significant ( F [1, 497] = 117.98, p < 0.001, η 2 = 0.192, power = 0.999). Finally, differences between the groups in learning attitudes were significant ( F [1, 497] = 23.22, p < 0.001, η 2 = 0.045, power = 0.998). The a posteriori comparison demonstrated that the high smartphone use group significantly outperformed the low smartphone use group in all dependent variables with regard to academic performance. Thus, hypothesis 4 is supported.
Univariate analysis results
Dependent variable | SS | df | MS | F | value | η | Observed power | A posteriori comparisons | |
---|---|---|---|---|---|---|---|---|---|
Learning activities | Contrast | 27.53 | 1 | 27.53 | 40.80 | < .001*** | .076 | 1.000 | |
Error | 335.31 | 497 | .675 | High group > Low group | |||||
Learning applications | Contrast | 73.95 | 1 | 73.95 | 117.98 | < .001*** | .192 | 1.000 | |
Error | 311.53 | 497 | .627 | ||||||
Learning attitudes | Contrast | 20.93 | 1 | 20.93 | 23.22 | < .001*** | .045 | .998 | |
Error | 448.08 | 497 | .902 |
SS sum of squares; df degrees of freedom; MS mean square
Smartphone behavior as the mediating variable impacting academic performance
As suggested by Baron and Kenny ( 1986 ), smartphone behavior is a mediating variable affecting academic performance. We examined the impact through the following four-step process:
- Step 1. The independent variable (parental control and students' self-control) must have a significant effect on the dependent variable (academic performance), as in model 1 (please see Fig. 1 ).

Model 2: Model to test the impact of parental control and students’ self-control on smartphone behavior

Model 3: Both independent variables (parental control and student self-control) and mediators (smartphone behavior) were used as predictors to predict dependent variables
- Step 4. In model 3, the regression coefficient of the independent variables (parental control and student self-control) on the dependent variables must be less than in mode 1 or become insignificant.
As can be seen in Fig. 1 , parental control and student self-control are observed variables, and smartphone behavior is a latent variable. "Strict" is set to 0, which means "Conditional", with "None" compared to "Strict". “Proactive high self-control” is also set to 0. From Fig. 1 we find that the independent variables have a significant effect on the dependent variable. The regression coefficient of parental control is 0.176, t = 3.45 ( p < 0.01); the regression coefficient of students’ self-control is 0.218, t = 4.12 ( p < 0.001), proving the fit of the model (Chi Square = 13.96**, df = 4, GFI = 0.989, AGFI = 0.959, CFI = 0.996, TLI = 0.915, RMSEA = 0.051, SRMR = 0.031). Therefore, the test results for Model 1 are in line with the recommendations of Baron and Kenny ( 1986 ).
As can be seen in Fig. 2 , the independent variables have a significant effect on smartphone behaviors. The regression coefficient of parental control is 0.166, t = 3.11 ( p < 0.01); the regression coefficient of students’ self-control is 0.149, t = 2.85 ( p < 0.01). The coefficients of the model fit are: Chi Square = 15.10**, df = 4, GFI = 0.988, AGFI = 0.954, CFI = 0.973, TLI = 0.932, RMSEA = 0.052, SRMR = 0.039. Therefore, the results of the test of Model 2 are in line with the recommendations of Baron and Kenny ( 1986 ).
As can be seen in Fig. 3 , smartphone behaviors have a significant effect on the dependent variable. The regression coefficient is 0.664, t = 10.2 ( p < 0.001). The coefficients of the model fit are: Chi Square = 91.04**, df = 16, GFI = 0.958, AGFI = 0.905, CFI = 0.918, TLI = 0.900, RMSEA = 0.077, SRMR = 0.063. Therefore, the results of the test of Model 3 are in line with the recommendations of Baron and Kenny ( 1986 ).
As can be seen in Fig. 4 , the regression coefficient of the independent variables (parental control and student self-control) on the dependent variables is less than in model 1, and the parental control variable becomes insignificant. The regression coefficient of parental control is 0.013, t = 0.226 ( p > 0.05); the path coefficient of students’ self-control is 0.155, t = 3.07 ( p < 0.01).

Model 4: Model three’s regression coefficient of the independent variables (parental control and student self-control) on the dependent variables
To sum up, we prove that smartphone behavior is the mediating variable to impact the academic performance. Thus, hypothesis 5 is supported.
This study investigated differences in the smartphone behavior of fifth and sixth graders in Taiwan with different background variables (focus on parental control and students’ self-control) and their effects on academic performance. The correlation between smartphone behavior and academic performance was also examined. Although smartphones are being used in elementary school learning activities, relatively few studies have explored their effects on academic performance. In this study, the proportion of girls who used smartphones to search for information was significantly higher than that of boys. Past studies have been inconclusive about gender differences in smartphone use. Lee and Kim ( 2018 ) observed no gender differences in smartphone use, but did note that boys engaged in more smartphone use if their parents set fewer restrictions. Kim et al. ( 2019 ) found that boys exhibited higher levels of smartphone dependency than girls. By contrast, Kim ( 2017 ) reported that girls had higher levels of smartphone dependency than boys did. Most relevant studies have focused on smartphone dependency; comparatively little attention has been devoted to smartphone behavior. The present study contributes to the literature in this regard.
Notably, this study found that parental control affected smartphone use. If the participants’ parents imposed no restrictions, students spent more time on leisure and entertainment and on interpersonal communication rather than on information searches. This is conceivable, as Asian parents generally discourage their children from using their smartphones for non-study related activities (such as entertainment or making friends) but not for learning-related activities. If Asian parents believe that using a smartphone can improve their child's academic performance, they will encourage their child to use it. Parents in Taiwan attach great importance to their children's academic performance (Lee et al., 2016 ). A considerable amount of research has been conducted on parental attitudes or control in this context. Hwang and Jeong ( 2015 ) suggested that parental attitudes mediated their children’s smartphone use. Similarly, Chang et al. ( 2019 ) observed that parental attitudes mediated the smartphone use of children in Taiwan. Our results are consistent with extant evidence in this regard. Lee and Ogbolu ( 2018 ) demonstrated that the stronger children’s perception was of parental control over their smartphone use, the more frequently they used their smartphones. The study did not further explain the activities the children engaged in on their smartphones after they increased their frequency of use. In the present study, the participants spent more time on their smartphones for leisure and entertainment and for interpersonal communication than for information searches.
Notably, this study also found that students’ self-control affected smartphone use.
Regarding the Pearson’s correlation analysis of smartphone behavior and academic performance, except for information searches and learning attitudes, all the variables were significantly positively correlated. In other words, there was a positive correlation between smartphone behavior and academic performance. In their systematic review, Amez and Beart ( 2020 ) determined that most empirical results provided evidence of a negative correlation between smartphone behavior and academic performance, playing a more considerable role in that relationship than the theoretical mechanisms or empirical methods in the studies they examined. The discrepancy between our results and theirs can be explained by the between-study variations in the definitions of learning achievement or performance.
Regarding the present results on the differences in the academic performance of students with varying smartphone behaviors, we carried out a cluster analysis, dividing the participants into a high smartphone use group and a low smartphone use group. Subsequent MANOVA revealed that the high smartphone use group academically outperformed the low smartphone use group; significant differences were noted in the academic performance of students with different smartphone behaviors. Given the observed correlation between smartphone behavior and academic performance, this result is not unexpected. The findings on the relationship between smartphone behavior and academic performance can be applied to smartphone use in the context of education.
Finally, in a discussion of whether smartphone behavior is a mediator of academic performance, it is proved that smartphone behavior is the mediating variable impacting academic performance. Our findings show that parental control and students’ self-control can affect academic performance. However, the role of the mediating variable (smartphone use behavior) means that changes in parental control have no effect on academic achievement at all. This means that smartphone use behaviors have a full mediating effect on parental control. It is also found that students’ self-control has a partial mediating effect. Our findings suggest that parental attitudes towards the control of smartphone use and students' self-control do affect academic performance, but smartphone use behavior has a significant mediating effect on this. In other words, it is more important to understand the children's smartphone behavior than to control their smartphone usage. There have been many studies in the past exploring the mediator variables for smartphone use addiction and academic performance. For instance, Ahmed et al. ( 2020 ) found that the mediating variables of electronic word of mouth (eWOM) and attitude have a significant and positive influence in the relationship between smartphone functions. Cho and Lee ( 2017 ) found that parental attitude is the mediating variable for smartphone use addiction. Cho et al. ( 2017 ) indicated that stress had a significant influence on smartphone addiction, while self-control mediates that influence. In conclusion, the outcomes demonstrate that parental control and students’ self-control do influence student academic performance in primary school. Previous studies have offered mixed results as to whether smartphone usage has an adverse or affirmative influence on student academic performance. This study points out a new direction, thinking of smartphone use behavior as a mediator.
In brief, the participants spent more smartphone time on leisure and entertainment and interpersonal communication, but the academic performance of the high smartphone use group surpassed that of the low smartphone use group. This result may clarify the role of students’ communication skills in their smartphone use. As Kang and Jung ( 2014 ) noted, conventional communication methods have been largely replaced by mobile technologies. This suggests that students’ conventional communication skills are also shifting to accommodate smartphone use. Elementary students are relatively confident in communicating with others through smartphones; thus, they likely have greater self‐efficacy in this regard and in turn may be better able to improve their academic performance by leveraging mobile technologies. This premise requires verification through further research. Notably, high smartphone use suggests the greater availability of time and opportunity in this regard. Conversely, low smartphone use suggests the relative lack of such time and opportunity. The finding that the high smartphone use group academically outperformed the low smartphone use group also indicates that smartphone accessibility constitutes a potential inequality in the learning opportunities of elementary school students. Therefore, elementary school teachers must be aware of this issue, especially in view of the shift to online learning triggered by the COVID-19 pandemic, when many students are dependent on smartphones and computers for online learning.
Conclusions and implications
This study examined the relationship between smartphone behavior and academic performance for fifth and sixth graders in Taiwan. Various background variables (parental control and students’ self-control) were also considered. The findings provide new insights into student attitudes toward smartphone use and into the impacts of smartphone use on academic performance. Smartphone behavior and academic performance were correlated. The students in the high smartphone use group academically outperformed the low smartphone use group. This result indicates that smartphone use constitutes a potential inequality in elementary school students’ learning opportunities. This can be explained as follows: high smartphone use suggests that the participants had sufficient time and opportunity to access and use smartphones. Conversely, low smartphone use suggests that the participants did not have sufficient time and opportunity for this purpose. Students’ academic performance may be adversely affected by fewer opportunities for access. Disparities between their performance and that of their peers with ready access to smartphones may widen amid the prevalent class suspension and school closure during the ongoing COVID-19 pandemic.
This study has laid down the basic foundations for future studies concerning the influence of smartphones on student academic performance in primary school as the outcome variable. This model can be replicated and applied to other social science variables which can influence the academic performance of primary school students as the outcome variable. Moreover, the outcomes of this study can also provide guidelines to teachers, parents, and policymakers on how smartphones can be most effectively used to derive the maximum benefits in relation to academic performance in primary school as the outcome variable. Finally, the discussion of the mediating variable can also be used as the basis for the future projects.
Limitations and areas of future research
This research is significant in the field of smartphone functions and the student academic performance for primary school students. However, certain limitations remain. The small number of students sampled is the main problem in this study. For more generalized results, the sample data may be taken across countries within the region and increased in number (rather than limited to certain cities and countries). For more robust results, data might also be obtained from both rural and urban centers. In this study, only one mediating variable was incorporated, but in future studies, several other psychological and behavioral variables might be included for more comprehensive outcomes. We used the SEM-based multivariate approach which does not address the cause and effect between the variables, therefore, in future work, more robust models could be employed for cause-and-effect investigation amongst the variables.
Acknowledgements
The authors would like to express their gratitude to the school participants in the study.
Appendix 1 Factor analysis results
Author contributions.
Kung and Wang conceived of the presented idea. Kung, Wang and Hsieh developed the theory and performed the computations. Kung and Hsieh verified the analytical methods. Wang encouraged Kung and Hsieh to verify the numerical checklist and supervised the findings of this work. All authors discussed the results and contributed to the final manuscript.
The work done for this study was financially supported by the Ministry of Science and Technology of Taiwan under project No. MOST 109–2511-H-017–005.
Data availability
Declarations.
The authors declare that they have no known competing financial interests or personal relationships that could have appeared to influence the work reported in this paper.
Publisher's note
Springer Nature remains neutral with regard to jurisdictional claims in published maps and institutional affiliations.
Contributor Information
Jen Chun Wang, Email: wt.ude.unkn@gnawcj .
Chia-Yen Hsieh, Email: wt.ude.utpn@anudnab .
Shih-Hao Kung, Email: wt.moc.oohay@1-hsg .
- Ahmed, R. R., Salman, F., Malik, S. A., Streimikiene, D., Soomro, R. H., & Pahi, M. H. (2020). Smartphone Use and Academic Performance of University Students: A Mediation and Moderation Analysis. Sustainability, 12 (1), 439. MDPI AG. Retrieved from 10.3390/su12010439
- Amez S, Beart S. Smartphone use and academic performance: A literature review. International Journal of Educational Research. 2020; 103 :101618. doi: 10.1016/j.ijer.2020.101618. [ CrossRef ] [ Google Scholar ]
- Anshari M, Almunawar MN, Shahrill M, Wicaksono DK, Huda M. Smartphones usage in the classrooms: Learning aid or interference? Education and Information Technologies. 2017; 22 :3063–3079. doi: 10.1007/s10639-017-9572-7. [ CrossRef ] [ Google Scholar ]
- Bae SM. The relationships between perceived parenting style, learning motivation, friendship satisfaction, and the addictive use of smartphones with elementary school students of South Korea: Using multivariate latent growth modeling. School Psychology International. 2015; 36 (5):513–531. doi: 10.1177/0143034315604017. [ CrossRef ] [ Google Scholar ]
- Baron RM, Kenny DA. The moderator–mediator variable distinction in social psychological research: Conceptual, strategic, and statistical considerations. Journal of Personality and Social Psychology. 1986; 51 (6):1173–1182. doi: 10.1037/0022-3514.51.6.1173. [ PubMed ] [ CrossRef ] [ Google Scholar ]
- Bluestein SA, Kim T. Expectations and fulfillment of course engagement, gained skills, and non-academic usage of college students utilizing tablets in an undergraduate skills course. Education and Information Technologies. 2017; 22 (4):1757–1770. doi: 10.1007/s10639-016-9515-8. [ CrossRef ] [ Google Scholar ]
- Carrillo C, Flores MA. COVID-19 and teacher education: A literature review of online teaching and learning practices. European Journal of Teacher Education. 2020; 43 (4):466–487. doi: 10.1080/02619768.2020.1821184. [ CrossRef ] [ Google Scholar ]
- Chang FC, Chiu CH, Chen PH, Chiang JT, Miao NF, Chuang HT, Liu S. Children's use of mobile devices, smartphone addiction and parental mediation in Taiwan. Computers in Human Behavior. 2019; 93 :25–32. doi: 10.1016/j.chb.2018.11.048. [ CrossRef ] [ Google Scholar ]
- Chen RS, Ji CH. Investigating the relationship between thinking style and personal electronic device use and its implications for academic performance. Computers in Human Behavior. 2015; 52 :177–183. doi: 10.1016/j.chb.2015.05.042. [ CrossRef ] [ Google Scholar ]
- Chen C, Chen S, Wen P, Snow CE. Are screen devices soothing children or soothing parents?Investigating the relationships among children's exposure to different types of screen media, parental efficacy and home literacy practices. Computers in Human Behavior. 2020; 112 :106462. doi: 10.1016/j.chb.2020.106462. [ CrossRef ] [ Google Scholar ]
- Cheng YM, Kuo SH, Lou SJ, Shih RC. The development and implementation of u-msg for college students' English learning. International Journal of Distance Education Technologies. 2016; 14 (2):17–29. doi: 10.4018/IJDET.2016040102. [ CrossRef ] [ Google Scholar ]
- Cho KS, Lee JM. Influence of smartphone addiction proneness of young children on problematic behaviors and emotional intelligence: Mediating self-assessment effects of parents using smartphones. Computers in Human Behavior. 2017; 66 :303–311. doi: 10.1016/j.chb.2016.09.063. [ PMC free article ] [ PubMed ] [ CrossRef ] [ Google Scholar ]
- Cho H-Y, Kim KJ, Park JW. Stress and adult smartphone addiction: Mediation by self-control, neuroticism, and extraversion. Stress and Heath. 2017; 33 :624–630. doi: 10.1002/smi.2749. [ PubMed ] [ CrossRef ] [ Google Scholar ]
- Clayton, K., & Murphy, A. (2016). Smartphone apps in education: Students create videos to teach smartphone use as tool for learning. Journal of Media Literacy Education , 8 , 99–109. Retrieved October 13, 2021. Review from https://files.eric.ed.gov/fulltext/EJ1125609.pdf
- Daems K, Pelsmacker PD, Moons I. The effect of ad integration and interactivity on young teenagers’ memory, brand attitude and personal data sharing. Computers in Human Behavior. 2019; 99 :245–259. doi: 10.1016/j.chb.2019.05.031. [ CrossRef ] [ Google Scholar ]
- Deci EL, Ryan RM. Facilitating optimal motivation and psychological well-being across life’s domains. Canadian Psychology. 2008; 49 (1):14–23. doi: 10.1037/0708-5591.49.1.14. [ CrossRef ] [ Google Scholar ]
- Deci EL, Koestner R, Ryan RM. A meta-analytic review of experiments examining the effects of extrinsic rewards on intrinsic motivation. Psychological Bulletin. 1999; 125 :627–668. doi: 10.1037//0033-2909.125.6.627. [ PubMed ] [ CrossRef ] [ Google Scholar ]
- Dong C, Cao S, Li H. Young children’s online learning during COVID-19 pandemic: Chinese parents’ beliefs and attitudes. Children and Youth Services Review. 2020; 118 :105440. doi: 10.1016/j.childyouth.2020.105440. [ PMC free article ] [ PubMed ] [ CrossRef ] [ Google Scholar ]
- Du J, van Koningsbruggen GM, Kerkhof P. A brief measure of social media self-control failure. Computers in Human Behavior. 2018; 84 :68–75. doi: 10.1016/j.chb.2018.02.002. [ CrossRef ] [ Google Scholar ]
- Firmansyah, R. O., Hamdani, R. A., & Kuswardhana, D. (2020). IOP conference series: Materials science and engineering. The use of smartphone on learning activities: Systematic review . In International Symposium on Materials and Electrical Engineering 2019 (ISMEE 2019), Bandung, Indonesia. 10.1088/1757-899X/850/1/012006
- Garbe A, Ogurlu U, Logan N, Cook P. COVID-19 and remote learning: Experiences of parents with children during the pandemic. American Journal of Qualitative Research. 2020; 4 (3):45–65. doi: 10.29333/ajqr/8471. [ CrossRef ] [ Google Scholar ]
- George D, Mallery P. SPSS for windows step by step: A simple guide and reference. 17.0 update. 10. Pearson; 2010. [ Google Scholar ]
- Grant M, Hsu YC. Making personal and professional learning mobile: Blending mobile devices, social media, social networks, and mobile apps to support PLEs, PLNs, & ProLNs. Advances in Communications and Media Research Series. 2014; 10 :27–46. [ Google Scholar ]
- Grolnick WS, Pomerantz EM. Issues and challenges in studying parental control: Toward a new conceptualization. Child Development Perspectives. 2009; 3 :165–170. doi: 10.1111/j.1750-8606.2009.00099.x. [ PMC free article ] [ PubMed ] [ CrossRef ] [ Google Scholar ]
- Guo Z, Lu X, Li Y, Li Y. A framework of students' reasons for using CMC media in learning contexts: A structural approach. Journal of the Association for Information Science and Technology. 2011; 62 (11):2182–2200. doi: 10.1002/asi.21631. [ CrossRef ] [ Google Scholar ]
- Hadad S, Meishar-Tal H, Blau I. The parents' tale: Why parents resist the educational use of smartphones at schools? Computers & Education. 2020; 157 :103984. doi: 10.1016/j.compedu.2020.103984. [ CrossRef ] [ Google Scholar ]
- Hau K-T, Ho IT. Chinese students’ motivation and achievement. In: Bond MH, editor. Oxford handbook of Chinese psychology. Oxford University Press; 2010. pp. 187–204. [ Google Scholar ]
- Hawi NS, Samaha M. To excel or not to excel: Strong evidence on the adverse effect of smartphone addiction on academic performance. Computers & Education. 2016; 98 :81–89. doi: 10.1016/j.compedu.2016.03.007. [ CrossRef ] [ Google Scholar ]
- Heo YJ, Lee K. Smartphone addiction and school life adjustment among high school students: The mediating effect of self-control. Journal of Psychosocial Nursing and Mental Health Services. 2021; 56 (11):28–36. doi: 10.3928/02793695-20180503-06. [ PubMed ] [ CrossRef ] [ Google Scholar ]
- Hofmann W, Friese M, Strack F. Impulse and self-control from a dual-systems perspective. Perspectives on Psychological Science. 2009; 4 (2):162–176. doi: 10.1111/j.1745-6924.2009.01116.x. [ PubMed ] [ CrossRef ] [ Google Scholar ]
- Hsieh CY. Predictive analysis of instruction in science to students’ declining interest in science-An analysis of gifted students of sixth - and seventh-grade in Taiwan. International Journal of Engineering Education. 2020; 2 (1):33–51. doi: 10.14710/ijee.2.1.33-51. [ CrossRef ] [ Google Scholar ]
- Hsieh, C. Y., Lin, C. H. (2021). Other important issues. Meta-analysis: the relationship between smartphone addiction and college students’ academic performance . 2021 TERA International Conference on Education. IN National Sun Yat-sen University (NSYSU), Kaohsiung.
- Hwang Y, Jeong SH. Predictors of parental mediation regarding children's smartphone use. Cyberpsychology, Behavior, and Social Networking. 2015; 18 (12):737–743. doi: 10.1089/cyber.2015.0286. [ PubMed ] [ CrossRef ] [ Google Scholar ]
- Jeong S-H, Kim HJ, Yum J-Y, Hwang Y. What type of content are smartphone users addicted to? SNS vs. games. Computers in Human Behavior. 2016; 54 :10–17. doi: 10.1016/j.chb.2015.07.035. [ CrossRef ] [ Google Scholar ]
- Judd T. Making sense of multitasking: The role of facebook. Computers & Education. 2014; 70 :194–202. doi: 10.1016/j.compedu.2013.08.013. [ CrossRef ] [ Google Scholar ]
- Junco R, Cotton SR. No A 4 U: The relationship between multitasking and academic performance. Computers & Education. 2012; 59 :505–514. doi: 10.1016/j.compedu.2011.12.023. [ CrossRef ] [ Google Scholar ]
- Kang S, Jung J. Mobile communication for human needs: A comparison of smartphone use between the US and Korea. Computers in Human Behavior. 2014; 35 :376–387. doi: 10.1016/j.chb.2014.03.024. [ CrossRef ] [ Google Scholar ]
- Karikoski J, Soikkeli T. Contextual usage patterns in smartphone communication services. Personal and Ubiquitous Computing. 2013; 17 (3):491–502. doi: 10.1007/s00779-011-0503-0. [ CrossRef ] [ Google Scholar ]
- Karpinski AC, Kirschner PA, Ozer I, Mellott JA, Ochwo P. An exploration of social networking site use, multitasking, and academic performance among United States and European university students. Computers in Human Behavior. 2013; 29 :1182–1192. doi: 10.1016/j.chb.2012.10.011. [ CrossRef ] [ Google Scholar ]
- Kates AW, Wu H, Coryn CLS. The effects of mobile phone use on academic performance: A meta-analysis. Computers & Education. 2018; 127 :107–112. doi: 10.1016/j.compedu.2018.08.012. [ CrossRef ] [ Google Scholar ]
- Kim K. Smartphone addiction and the current status of smartphone usage among Korean adolescents. Studies in Humanities and Social Sciences. 2017; 2017 (56):115–142. doi: 10.17939/hushss.2017.56.006. [ CrossRef ] [ Google Scholar ]
- Kim D, Chun H, Lee H. Determining the factors that influence college students' adoption of smartphones. Journal of the Association for Information Science and Technology. 2014; 65 (3):578–588. doi: 10.1002/asi.22987. [ CrossRef ] [ Google Scholar ]
- Kim B, Jahng KE, Oh H. The moderating effect of elementary school students' perception of open communication with their parents in the relationship between smartphone dependency and school adjustment. Korean Journal of Childcare and Education. 2019; 15 (1):54–73. doi: 10.14698/jkcce.2019.15.01.057. [ CrossRef ] [ Google Scholar ]
- Lee J, Cho B. Effects of self-control and school adjustment on smartphone addiction among elementary school students. Korea Science. 2015; 11 (3):1–6. doi: 10.5392/IJoC.2015.11.3.001. [ CrossRef ] [ Google Scholar ]
- Lee EJ, Kim HS. Gender differences in smartphone addiction behaviors associated with parent-child bonding, parent-child communication, and parental mediation among Korean elementary school students. Journal of Addictions Nursing. 2018; 29 (4):244–254. doi: 10.1097/JAN.0000000000000254. [ PubMed ] [ CrossRef ] [ Google Scholar ]
- Lee SJ, Moon HJ. Effects of self-Control, parent- adolescent communication, and school life satisfaction on smart-phone addiction for middle school students. Korean Journal of Human Ecology. 2013; 22 (6):87–598. doi: 10.5934/kjhe.2013.22.6.587. [ CrossRef ] [ Google Scholar ]
- Lee EJ, Ogbolu Y. Does parental control work with smartphone addiction? Journal of Addictions Nursing. 2018; 29 (2):128–138. doi: 10.1097/JAN.0000000000000222. [ PubMed ] [ CrossRef ] [ Google Scholar ]
- Lee J, Cho B, Kim Y, Noh J. Smartphone addiction in university students and its implication for learning. In: Chen G, Kinshuk VK, Huang R, Kong SC, editors. Emerging issues in smart learning. Springer; 2015. pp. 297–305. [ Google Scholar ]
- Lee S, Lee K, Yi SH, Park HJ, Hong YJ, Cho H. Effects of Parental Psychological Control on Child’s School Life: Mobile Phone Dependency as Mediator. Journal of Child and Family Studies. 2016; 25 :407–418. doi: 10.1007/s10826-015-0251-2. [ CrossRef ] [ Google Scholar ]
- Lepp A, Barkley JE, Karpinski AC. The relationship between cell phone use, academic performance, anxiety, and satisfaction with life in college students. Computers in Human Behavior. 2014; 31 :343–350. doi: 10.1016/j.chb.2013.10.049. [ CrossRef ] [ Google Scholar ]
- Lin YQ, Liu Y, Fan WJ, Tuunainen VK, Deng SG. Revisiting the relationship between smartphone use and academic performance: A large-scale study. Computers in Human Behavior. 2021; 122 :106835. doi: 10.1016/j.chb.2021.106835. [ CrossRef ] [ Google Scholar ]
- Looi CK, Lim KF, Pang J, Koh ALH, Seow P, Sun D, Boticki I, Norris C, Soloway E. Bridging formal and informal learning with the use of mobile technology. In: Chai CS, Lim CP, Tan CM, editors. Future learning in primary schools. Springer; 2016. pp. 79–96. [ Google Scholar ]
- Martin F, Ertzberger J. Here and now mobile learning: An experimental study on the use of mobile technology. Computers & Education. 2013; 68 :76–85. doi: 10.1016/j.compedu.2013.04.021. [ CrossRef ] [ Google Scholar ]
- Meier, A. (2017). Neither pleasurable nor virtuous: Procrastination links smartphone habits and messenger checking behavior to decreased hedonic as well as eudaimonic well-being . Paper presented at the 67th Annual Conference of the International Communication Association (ICA), San Diego, CA.
- Morgan H. Best practices for implementing remote learning during a pandemic. The Clearing House: A Journal of Educational Strategies, Issues and Ideas. 2020; 93 (3):135–141. doi: 10.1080/00098655.2020.1751480. [ CrossRef ] [ Google Scholar ]
- Park JH. Smartphone use patterns of smartphone-dependent children. Korean Academy of Child Health Nursing. 2020; 26 (1):47–54. doi: 10.4094/chnr.2020.26.1.47. [ PMC free article ] [ PubMed ] [ CrossRef ] [ Google Scholar ]
- Park N, Lee H. Social implications of smartphone use: Korean college students' smartphone use and psychological well-being. Cyberpsychology, Behavior, and Social Networking. 2012; 15 (9):491–497. doi: 10.1089/cyber.2011.0580. [ PubMed ] [ CrossRef ] [ Google Scholar ]
- Park E, Logan H, Zhang L, Kamigaichi N, Kulapichitr U. Responses to coronavirus pandemic in early childhood services across five countries in the Asia-Pacific region: OMEP Policy Forum. International Journal of Early Childhood. 2021; 2021 :1–18. doi: 10.1007/s13158-020-00278-0. [ PMC free article ] [ PubMed ] [ CrossRef ] [ Google Scholar ]
- Peng Y, Zhou H, Zhang B, Mao H, Hu R, Jiang H. Perceived stress and mobile phone addiction among college students during the 2019 coronavirus disease: The mediating roles of rumination and the moderating role of self-control. Personality and Individual Differences. 2022; 185 :111222. doi: 10.1016/j.paid.2021.111222. [ PMC free article ] [ PubMed ] [ CrossRef ] [ Google Scholar ]
- Reinecke L, Aufenanger S, Beutel ME, Dreier M, Quiring O, Stark B, Müller KW. Digital stress over the life span: The effects of communication load and Internet multitasking on perceived stress and psychological health impairments in a German probability sample. Media Psychology. 2017; 20 (1):90–115. doi: 10.1080/15213269.2015.1121832. [ CrossRef ] [ Google Scholar ]
- Ryan RM, Deci EL. Self-determination theory and the facilitation of intrinsic motivation, social development, and well-being. American Psychologist. 2000; 55 (1):68–78. doi: 10.1037//0003-066x.55.1.68. [ PubMed ] [ CrossRef ] [ Google Scholar ]
- Ryan RM, Deci EL. Self-regulation and the problem of human autonomy: Does psychology need choice, self-determination, and will? Journal of Personality. 2006; 74 :1557–1585. doi: 10.1111/j.1467-6494.2006.00420.x. [ PubMed ] [ CrossRef ] [ Google Scholar ]
- Salvation, M. D. (2017). The relationship between smartphone applications usage and students’ academic performance. Computational Methods in Social Sciences , 5 (2), 26–39. Retrieved October 13, 2021. Review from http://cmss.univnt.ro/wp-content/uploads/vol/split/vol_V_issue_2/CMSS_vol_V_issue_2_art.003.pdf
- Sarker IH, Kayes ASM, Watters P. Effectiveness analysis of machine learning classification models for predicting personalized context-aware smartphone usage. Journal of Big Data. 2019; 6 :57. doi: 10.1186/s40537-019-0219-y. [ CrossRef ] [ Google Scholar ]
- Sarwar M, Soomro TR. Impact of smartphone's on society. European Journal of Scientific Research. 2013; 98 (2):219–226. [ Google Scholar ]
- Sepulveda-Escobar P, Morrison A. Online teaching placement during the COVID-19 pandemic in Chile: Challenges and opportunities. European Journal of Teacher Education. 2020; 43 (4):587–607. doi: 10.1080/02619768.2020.1820981. [ CrossRef ] [ Google Scholar ]
- Sklar, A., Rim, S. Y. & Fujita, K. (2017). Proactive and reactive self-control. In D., de Ridder, M., Adriaanse, & K. Fujita (Eds). The Routledge International Handbook of Self-Control in Health and Well-Being (p. 11). Routledge. 10.4324/9781315648576
- Steinberg L, Lamborn SD, Dornbusch SM, Darling N. Impact of parenting practices on adolescent achievement: Authoritative parenting, school involvement, and encouragement to succeed. Child Development. 1992; 63 :1266–1281. doi: 10.1111/j.1467-8624.1992.tb01694.x. [ PubMed ] [ CrossRef ] [ Google Scholar ]
- Stice E, Barrera M. A longitudinal examination of the reciprocal relations between perceived parenting and adolescents’ substance use and externalizing behaviors. Developmental Psychology. 1995; 31 :322–334. doi: 10.1037/0012-1649.31.2.322. [ CrossRef ] [ Google Scholar ]
- Stolz HE, Barber BK, Olsen JA. Toward disentangling fathering and mothering: An assessment of relative importance. Journal of Marriage and Family. 2005; 67 :1076–1092. doi: 10.1111/j.1741-3737.2005.00195.x. [ CrossRef ] [ Google Scholar ]
- Tang T, Abuhmaid AM, Olaimat M, Oudat DM, Aldhaeebi M, Bamanger E. Efficiency of flipped classroom with online-based teaching under COVID-19. Interactive Learning Environments. 2020 doi: 10.1080/10494820.2020.1817761. [ CrossRef ] [ Google Scholar ]
- Troll ES, Friese M, Loschelder DD. How students’ self-control and smartphone-use explain their academic performance. Computers in Human Behavior. 2021; 117 :106624. doi: 10.1016/j.chb.2020.106624. [ CrossRef ] [ Google Scholar ]
- UNESCO. (2021). UNESCO figures show two thirds of an academic year lost on average worldwide due to Covid-19 school closures . Retrieved July 23, 2021. Retrieved, from https://en.unesco.org/news/unesco-figures-show-two-thirds-academic-year-lost-average-worldwide-due-covid-19-school
- Vanderloo LM. Screen-viewing among preschoolers in childcare: A systematic review. BMC Pediatric. 2014; 14 :205. doi: 10.1186/1471-2431-14-205. [ PMC free article ] [ PubMed ] [ CrossRef ] [ Google Scholar ]
- Wang TH, Cheng HY. Problematic Internet use among elementary school students: Prevalence and risk factors. Information, Communication & Society. 2019; 24 (2):108–134. doi: 10.1080/1369118X.2019.1645192. [ CrossRef ] [ Google Scholar ]
- Wang CJ, Chang FC, Chiu CH. Smartphone addiction and related factors among elementary school students in New Taipei City. Research of Educational Communications and Technology. 2017; 117 :67–87. doi: 10.6137/RECT.201712_117.0005. [ CrossRef ] [ Google Scholar ]
- Yi YJ, You S, Bae BJ. The influence of smartphones on academic performance: The development of the technology-to-performance chain model. Library Hi Tech. 2016; 34 (3):480–499. doi: 10.1108/LHT-04-2016-0038. [ CrossRef ] [ Google Scholar ]
- Zhang MWB, Ho CSH, Ho CM. Methodology of development and students’ perceptions of a psychiatry educational smartphone application. Technology and Health Care. 2014; 22 :847–855. doi: 10.3233/THC-140861. [ PubMed ] [ CrossRef ] [ Google Scholar ]
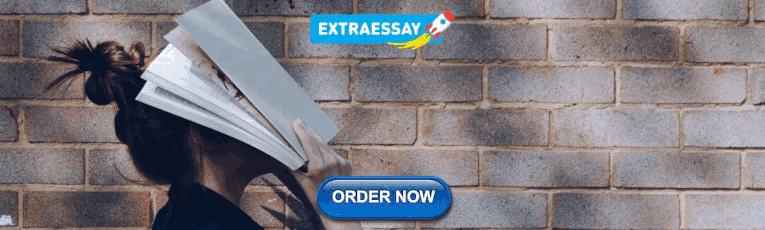
IMAGES
VIDEO
COMMENTS
The mobile phone is stimulating one of the most important technological revolutions in human history. This article first presents impacts, challenges, and predictions of mobile phone use. It first ...
The mobile phone is stimulating one of the most important technological revolutions in human history. This article first presents impacts, challenges, and predictions of mobile phone use. It first in...
We present the first systematic review of the scientific literature on smartphone use and academic success. We synthesise the theoretical mechanisms, empirical approaches, and empirical findings described in the multidisciplinary literature to date. Our analysis of the literature reveals a predominance of empirical results supporting a negative ...
The purpose of this study was to carry out a review of observational studies that consider links between mobile phone use and mental health from a psychological or behavioral perspective. Systematic literature searches in PubMed and PsycINFO for articles ...
Growing use of mobiles phones (MP) and other wireless devices (WD) has raised concerns about their possible effects on children and adolescents' wellbeing. Understanding whether these technologies affect children and adolescents' mental health in positive or detrimental ways has become more urgent following further increase in use since the COVID-19 outbreak. To review the empirical ...
Phone conscious thought significantly predicted memory accuracy. We found that the presence of a smartphone and high phone conscious thought affects one's memory learning and recall, indicating the negative effect of a smartphone proximity to our learning and memory.
The first mobile phone by Motorola in 1983 5 was so big and heavy that it was nicknamed "the brick." Current phones are significantly more lightweight and compact and have the capability to text, email, access social media, access the Internet, and much more.
Our aim was to examine the effect of a smartphone's presence on learning and memory among undergraduates. A total of 119 undergraduates completed a memory task and the Smartphone Addiction Scale (SAS). As predicted, those without smartphones had higher recall accuracy compared to those with smartphones. Results showed a significant negative ...
Abstract Due to the ubiquity of mobile phones throughout the world, there have been extensive scholarly research on their impact within and across areas.
We will talk about the use of mobile phones for research and data collection, crowdsourcing and science education. In short, does the lab have a place for the mobile phone?
In the field of mobile media research, extant research supported this argument by showing that people's mobile phone usage and dependency were associated with their psychological attributes (e.g., Butt & Phillips, 2008; Chittaranjan, Blom, & Gatica-Perez, 2011; Delevi & Weisskirch, 2013; Lane & Manner, 2011; Takao, 2014; Tosun & Lajunen, 2010 ).
Research design A meta-analysis of research conducted on the relationship between mobile phone use and student educational outcomes over a 10-year period (2008-2017) was conducted.
This article reports on the findings from a field study of mobile phone use among dyads in public. Replicating an originally published field study from 2005, this study highlights how mobile phones and use have changed in the last 15 years and demonstrates the ways that mobile phones are used to both detract and enhance social interactions.
Smartphones are now nearly ubiquitous; their numerous built-in sensors enable continuous measurement of activities of daily living, making them especially well-suited for health research.
Abstract A review of the impact of mobile phone and base station radiation on human health and the environment has been presented here. Cell phone is an important invention in human history that has revolutionized people's lifestyles. As mobile phones have become an integral part of human daily routine, the quality of life around the world has improved significantly. However, concerns about ...
Of the 82 responses to this open-ended question, 40 indicated mobile phones are used for in-class research, whether to quickly look up information pertaining to that day's topic or to locate sources for speeches or papers.
Mobile phone use in the community and in professional sectors including health care settings is a potential source of microbial dissemination.
The behavior of consumers towards smartphones is increasingly a focus of marketing research. In particular, consumer behavior in the smartphone industry, from adoption motivation to post-usage ...
18 Health Systems Research Unit, South African Medical Research Council, Cape Town, South Africa. 19 Centre for Epidemic Interventions Research (CEIR), Norwegian Institute of Public Health, ... Most described mobile phone use as opposed to other mobile devices, such as tablets. We have moderate to high confidence in the statements in the ...
Thus, this paper aims to unveil the effects and challenges of Information Communication Technology (ICT) on agricultural development in Africa, focusing on mobile phone usage. This paper uses a systematic literature review approach for identifying and screening relevant studies.
Research on smartphone use among college students is extensive. Although numerous studies have examined the relationships between mobile phone use and…
In addition, multiple studies have found that mobile phone use is inversely related to academic performance (Judd, 2014; Karpinski et al., 2013). Most research on smartphone use and academic performance has focused on college students. There have few studies focused on elementary school students.
In this study, we examined the impact of mobile phone usage, during class lecture, on student learning. Participants in three different study groups (control, low-distraction, and high-distraction)...
his technology but also explain the dark side of Mobile phone usage. The research paper titled as Mobile-phone addiction in adolescence: The Test of Mobile Phone Dependence (TMD) by Chóliz M. (2012) Published in Prog Health Sci (Vol. 2, No. 1) includes the information related to the physical and psychological motivations behind the use of ...