
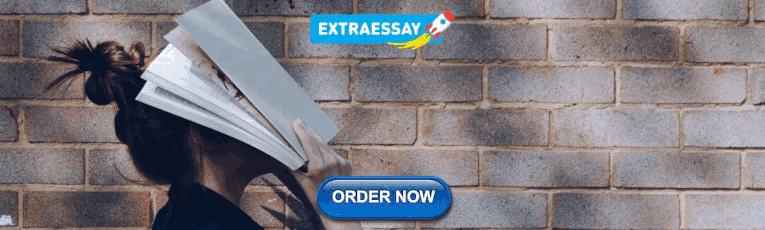
How To Write The Results/Findings Chapter
For quantitative studies (dissertations & theses).
By: Derek Jansen (MBA) | Expert Reviewed By: Kerryn Warren (PhD) | July 2021
So, you’ve completed your quantitative data analysis and it’s time to report on your findings. But where do you start? In this post, we’ll walk you through the results chapter (also called the findings or analysis chapter), step by step, so that you can craft this section of your dissertation or thesis with confidence. If you’re looking for information regarding the results chapter for qualitative studies, you can find that here .
Overview: Quantitative Results Chapter
- What exactly the results chapter is
- What you need to include in your chapter
- How to structure the chapter
- Tips and tricks for writing a top-notch chapter
- Free results chapter template
What exactly is the results chapter?
The results chapter (also referred to as the findings or analysis chapter) is one of the most important chapters of your dissertation or thesis because it shows the reader what you’ve found in terms of the quantitative data you’ve collected. It presents the data using a clear text narrative, supported by tables, graphs and charts. In doing so, it also highlights any potential issues (such as outliers or unusual findings) you’ve come across.
But how’s that different from the discussion chapter?
Well, in the results chapter, you only present your statistical findings. Only the numbers, so to speak – no more, no less. Contrasted to this, in the discussion chapter , you interpret your findings and link them to prior research (i.e. your literature review), as well as your research objectives and research questions . In other words, the results chapter presents and describes the data, while the discussion chapter interprets the data.
Let’s look at an example.
In your results chapter, you may have a plot that shows how respondents to a survey responded: the numbers of respondents per category, for instance. You may also state whether this supports a hypothesis by using a p-value from a statistical test. But it is only in the discussion chapter where you will say why this is relevant or how it compares with the literature or the broader picture. So, in your results chapter, make sure that you don’t present anything other than the hard facts – this is not the place for subjectivity.
It’s worth mentioning that some universities prefer you to combine the results and discussion chapters. Even so, it is good practice to separate the results and discussion elements within the chapter, as this ensures your findings are fully described. Typically, though, the results and discussion chapters are split up in quantitative studies. If you’re unsure, chat with your research supervisor or chair to find out what their preference is.
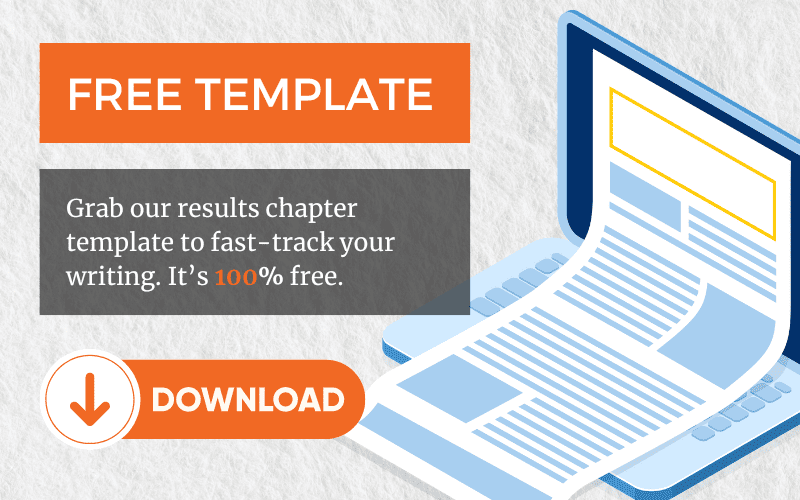
What should you include in the results chapter?
Following your analysis, it’s likely you’ll have far more data than are necessary to include in your chapter. In all likelihood, you’ll have a mountain of SPSS or R output data, and it’s your job to decide what’s most relevant. You’ll need to cut through the noise and focus on the data that matters.
This doesn’t mean that those analyses were a waste of time – on the contrary, those analyses ensure that you have a good understanding of your dataset and how to interpret it. However, that doesn’t mean your reader or examiner needs to see the 165 histograms you created! Relevance is key.
How do I decide what’s relevant?
At this point, it can be difficult to strike a balance between what is and isn’t important. But the most important thing is to ensure your results reflect and align with the purpose of your study . So, you need to revisit your research aims, objectives and research questions and use these as a litmus test for relevance. Make sure that you refer back to these constantly when writing up your chapter so that you stay on track.
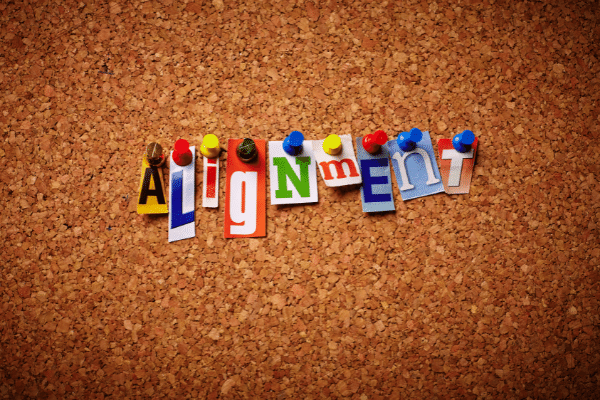
As a general guide, your results chapter will typically include the following:
- Some demographic data about your sample
- Reliability tests (if you used measurement scales)
- Descriptive statistics
- Inferential statistics (if your research objectives and questions require these)
- Hypothesis tests (again, if your research objectives and questions require these)
We’ll discuss each of these points in more detail in the next section.
Importantly, your results chapter needs to lay the foundation for your discussion chapter . This means that, in your results chapter, you need to include all the data that you will use as the basis for your interpretation in the discussion chapter.
For example, if you plan to highlight the strong relationship between Variable X and Variable Y in your discussion chapter, you need to present the respective analysis in your results chapter – perhaps a correlation or regression analysis.
Need a helping hand?
How do I write the results chapter?
There are multiple steps involved in writing up the results chapter for your quantitative research. The exact number of steps applicable to you will vary from study to study and will depend on the nature of the research aims, objectives and research questions . However, we’ll outline the generic steps below.
Step 1 – Revisit your research questions
The first step in writing your results chapter is to revisit your research objectives and research questions . These will be (or at least, should be!) the driving force behind your results and discussion chapters, so you need to review them and then ask yourself which statistical analyses and tests (from your mountain of data) would specifically help you address these . For each research objective and research question, list the specific piece (or pieces) of analysis that address it.
At this stage, it’s also useful to think about the key points that you want to raise in your discussion chapter and note these down so that you have a clear reminder of which data points and analyses you want to highlight in the results chapter. Again, list your points and then list the specific piece of analysis that addresses each point.
Next, you should draw up a rough outline of how you plan to structure your chapter . Which analyses and statistical tests will you present and in what order? We’ll discuss the “standard structure” in more detail later, but it’s worth mentioning now that it’s always useful to draw up a rough outline before you start writing (this advice applies to any chapter).
Step 2 – Craft an overview introduction
As with all chapters in your dissertation or thesis, you should start your quantitative results chapter by providing a brief overview of what you’ll do in the chapter and why . For example, you’d explain that you will start by presenting demographic data to understand the representativeness of the sample, before moving onto X, Y and Z.
This section shouldn’t be lengthy – a paragraph or two maximum. Also, it’s a good idea to weave the research questions into this section so that there’s a golden thread that runs through the document.
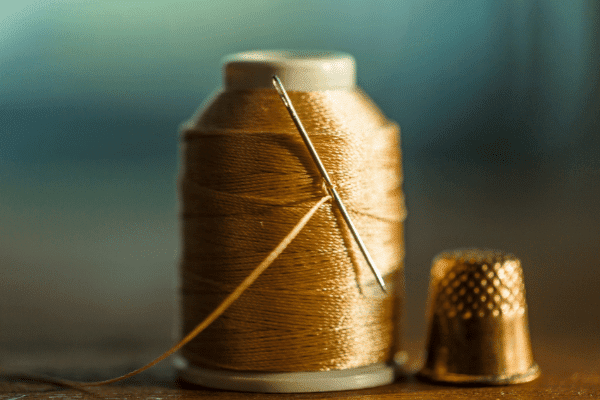
Step 3 – Present the sample demographic data
The first set of data that you’ll present is an overview of the sample demographics – in other words, the demographics of your respondents.
For example:
- What age range are they?
- How is gender distributed?
- How is ethnicity distributed?
- What areas do the participants live in?
The purpose of this is to assess how representative the sample is of the broader population. This is important for the sake of the generalisability of the results. If your sample is not representative of the population, you will not be able to generalise your findings. This is not necessarily the end of the world, but it is a limitation you’ll need to acknowledge.
Of course, to make this representativeness assessment, you’ll need to have a clear view of the demographics of the population. So, make sure that you design your survey to capture the correct demographic information that you will compare your sample to.
But what if I’m not interested in generalisability?
Well, even if your purpose is not necessarily to extrapolate your findings to the broader population, understanding your sample will allow you to interpret your findings appropriately, considering who responded. In other words, it will help you contextualise your findings . For example, if 80% of your sample was aged over 65, this may be a significant contextual factor to consider when interpreting the data. Therefore, it’s important to understand and present the demographic data.
Step 4 – Review composite measures and the data “shape”.
Before you undertake any statistical analysis, you’ll need to do some checks to ensure that your data are suitable for the analysis methods and techniques you plan to use. If you try to analyse data that doesn’t meet the assumptions of a specific statistical technique, your results will be largely meaningless. Therefore, you may need to show that the methods and techniques you’ll use are “allowed”.
Most commonly, there are two areas you need to pay attention to:
#1: Composite measures
The first is when you have multiple scale-based measures that combine to capture one construct – this is called a composite measure . For example, you may have four Likert scale-based measures that (should) all measure the same thing, but in different ways. In other words, in a survey, these four scales should all receive similar ratings. This is called “ internal consistency ”.
Internal consistency is not guaranteed though (especially if you developed the measures yourself), so you need to assess the reliability of each composite measure using a test. Typically, Cronbach’s Alpha is a common test used to assess internal consistency – i.e., to show that the items you’re combining are more or less saying the same thing. A high alpha score means that your measure is internally consistent. A low alpha score means you may need to consider scrapping one or more of the measures.
#2: Data shape
The second matter that you should address early on in your results chapter is data shape. In other words, you need to assess whether the data in your set are symmetrical (i.e. normally distributed) or not, as this will directly impact what type of analyses you can use. For many common inferential tests such as T-tests or ANOVAs (we’ll discuss these a bit later), your data needs to be normally distributed. If it’s not, you’ll need to adjust your strategy and use alternative tests.
To assess the shape of the data, you’ll usually assess a variety of descriptive statistics (such as the mean, median and skewness), which is what we’ll look at next.
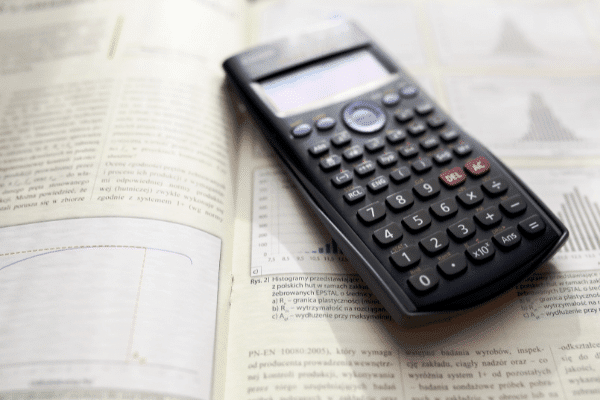
Step 5 – Present the descriptive statistics
Now that you’ve laid the foundation by discussing the representativeness of your sample, as well as the reliability of your measures and the shape of your data, you can get started with the actual statistical analysis. The first step is to present the descriptive statistics for your variables.
For scaled data, this usually includes statistics such as:
- The mean – this is simply the mathematical average of a range of numbers.
- The median – this is the midpoint in a range of numbers when the numbers are arranged in order.
- The mode – this is the most commonly repeated number in the data set.
- Standard deviation – this metric indicates how dispersed a range of numbers is. In other words, how close all the numbers are to the mean (the average).
- Skewness – this indicates how symmetrical a range of numbers is. In other words, do they tend to cluster into a smooth bell curve shape in the middle of the graph (this is called a normal or parametric distribution), or do they lean to the left or right (this is called a non-normal or non-parametric distribution).
- Kurtosis – this metric indicates whether the data are heavily or lightly-tailed, relative to the normal distribution. In other words, how peaked or flat the distribution is.
A large table that indicates all the above for multiple variables can be a very effective way to present your data economically. You can also use colour coding to help make the data more easily digestible.
For categorical data, where you show the percentage of people who chose or fit into a category, for instance, you can either just plain describe the percentages or numbers of people who responded to something or use graphs and charts (such as bar graphs and pie charts) to present your data in this section of the chapter.
When using figures, make sure that you label them simply and clearly , so that your reader can easily understand them. There’s nothing more frustrating than a graph that’s missing axis labels! Keep in mind that although you’ll be presenting charts and graphs, your text content needs to present a clear narrative that can stand on its own. In other words, don’t rely purely on your figures and tables to convey your key points: highlight the crucial trends and values in the text. Figures and tables should complement the writing, not carry it .
Depending on your research aims, objectives and research questions, you may stop your analysis at this point (i.e. descriptive statistics). However, if your study requires inferential statistics, then it’s time to deep dive into those .
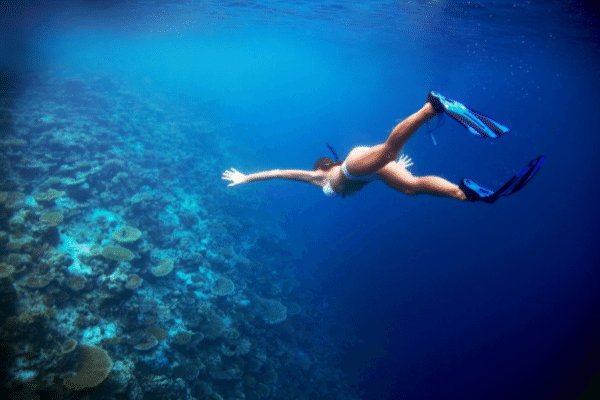
Step 6 – Present the inferential statistics
Inferential statistics are used to make generalisations about a population , whereas descriptive statistics focus purely on the sample . Inferential statistical techniques, broadly speaking, can be broken down into two groups .
First, there are those that compare measurements between groups , such as t-tests (which measure differences between two groups) and ANOVAs (which measure differences between multiple groups). Second, there are techniques that assess the relationships between variables , such as correlation analysis and regression analysis. Within each of these, some tests can be used for normally distributed (parametric) data and some tests are designed specifically for use on non-parametric data.
There are a seemingly endless number of tests that you can use to crunch your data, so it’s easy to run down a rabbit hole and end up with piles of test data. Ultimately, the most important thing is to make sure that you adopt the tests and techniques that allow you to achieve your research objectives and answer your research questions .
In this section of the results chapter, you should try to make use of figures and visual components as effectively as possible. For example, if you present a correlation table, use colour coding to highlight the significance of the correlation values, or scatterplots to visually demonstrate what the trend is. The easier you make it for your reader to digest your findings, the more effectively you’ll be able to make your arguments in the next chapter.
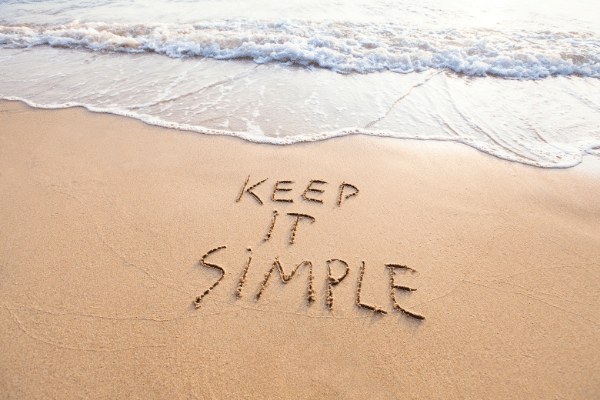
Step 7 – Test your hypotheses
If your study requires it, the next stage is hypothesis testing. A hypothesis is a statement , often indicating a difference between groups or relationship between variables, that can be supported or rejected by a statistical test. However, not all studies will involve hypotheses (again, it depends on the research objectives), so don’t feel like you “must” present and test hypotheses just because you’re undertaking quantitative research.
The basic process for hypothesis testing is as follows:
- Specify your null hypothesis (for example, “The chemical psilocybin has no effect on time perception).
- Specify your alternative hypothesis (e.g., “The chemical psilocybin has an effect on time perception)
- Set your significance level (this is usually 0.05)
- Calculate your statistics and find your p-value (e.g., p=0.01)
- Draw your conclusions (e.g., “The chemical psilocybin does have an effect on time perception”)
Finally, if the aim of your study is to develop and test a conceptual framework , this is the time to present it, following the testing of your hypotheses. While you don’t need to develop or discuss these findings further in the results chapter, indicating whether the tests (and their p-values) support or reject the hypotheses is crucial.
Step 8 – Provide a chapter summary
To wrap up your results chapter and transition to the discussion chapter, you should provide a brief summary of the key findings . “Brief” is the keyword here – much like the chapter introduction, this shouldn’t be lengthy – a paragraph or two maximum. Highlight the findings most relevant to your research objectives and research questions, and wrap it up.
Some final thoughts, tips and tricks
Now that you’ve got the essentials down, here are a few tips and tricks to make your quantitative results chapter shine:
- When writing your results chapter, report your findings in the past tense . You’re talking about what you’ve found in your data, not what you are currently looking for or trying to find.
- Structure your results chapter systematically and sequentially . If you had two experiments where findings from the one generated inputs into the other, report on them in order.
- Make your own tables and graphs rather than copying and pasting them from statistical analysis programmes like SPSS. Check out the DataIsBeautiful reddit for some inspiration.
- Once you’re done writing, review your work to make sure that you have provided enough information to answer your research questions , but also that you didn’t include superfluous information.
If you’ve got any questions about writing up the quantitative results chapter, please leave a comment below. If you’d like 1-on-1 assistance with your quantitative analysis and discussion, check out our hands-on coaching service , or book a free consultation with a friendly coach.
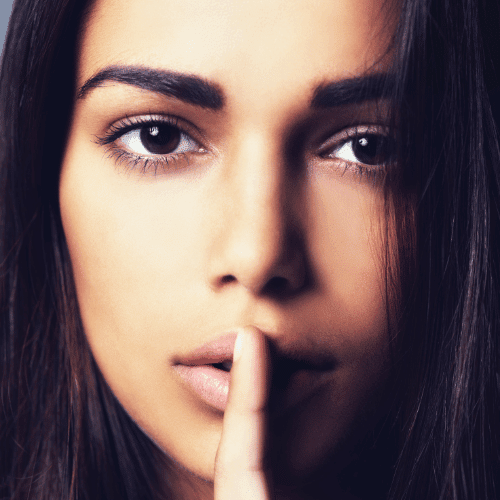
Psst... there’s more!
This post was based on one of our popular Research Bootcamps . If you're working on a research project, you'll definitely want to check this out ...
Thank you. I will try my best to write my results.
Awesome content 👏🏾
this was great explaination
Submit a Comment Cancel reply
Your email address will not be published. Required fields are marked *
Save my name, email, and website in this browser for the next time I comment.
- Print Friendly

- Calculators
- Descriptive Statistics
- Merchandise
- Which Statistics Test?
How to Report Pearson's r (Pearson's Correlation Coefficient) in APA Style
The APA has precise requirements for reporting the results of statistical tests, which means as well as getting the basic format right, you need to pay attention to the placing of brackets, punctuation, italics, and so on.
Happily, the basic format for citing Pearson's r is not too complex, as you can see here (the color red means you substitute in the appropriate value from your study).
r ( degress of freedom ) = the r statistic , p = p value .
Imagine we have conducted a study of 40 students that looked at whether IQ scores and GPA are correlated. We might report the results like this:
IQ and GPA were found to be moderately positively correlated, r (38) = .34, p = .032.
Other Examples
The variables shoe size and height were found to be strongly correlated, r (128) = .89, p < .01.
Among the students of Hogwarts University, the number of hours playing Fortnite per week and midterm exam results were negatively correlated, r (78) = -.45, p < .001.
Here are some things you should watch out for.
1. There are two ways to report p values. The first way is to cite the alpha value as in the second example above. The second way, very much the preferred way in the age of computer aided calculations (and the way recommended by the APA), is to report the exact p value (as in our main example). If you report the exact p value, then you need to state your alpha level early in your results section. The other thing to note here is that if your p value is less than .001, it's conventional simply to state p < .001, rather than give the exact value.
2. The r statistic should be stated at 2 decimal places.
3. Remember to drop the leading 0 from both r and the p value (i.e., not 0.34, but rather .34).
4. You don't need to provide the formula for r .
5. Degrees of freedom for r is N - 2 (the number of data points minus 2).

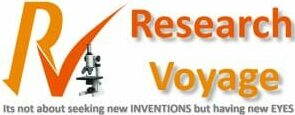
Research Voyage
Research Tips and Infromation
How to Write the Results Section of your Dissertation or Thesis?

Introduction
Organizing your results, providing context, presenting the data in results section, describing statistical analysis, reporting the findings in results section, supporting the findings, visual representation in results section.
As you progress on your journey towards completing your PhD or Post Graduate dissertation, one of the most critical sections that holds immense significance is the results section.
Results section serves as the pinnacle of your research, where you unveil the outcomes of your exhaustive efforts and shed light on the answers to your research questions. In this blog post, we will delve into the intricacies of the results section and explore how to effectively present and interpret your findings to leave a lasting impact.
Whether you’re conducting research in the field of biology, psychology, computer science, or any other discipline, the results section is where your data takes center stage. It is a space where you showcase your meticulous analysis, statistical methods, and the discoveries you’ve made along the way. By understanding the key components and best practices for constructing a compelling results section, you can present your findings in a manner that resonates with both your academic peers and the wider research community.
In this comprehensive guide, we will walk you through the fundamental elements of the results section, from organizing your data to choosing the appropriate visual representations. We will explore the importance of clear and concise reporting, emphasizing the significance of providing contextual information and highlighting any unexpected or groundbreaking discoveries.
Furthermore, we will discuss strategies for effectively interpreting your results, discussing their implications, and connecting them back to your research objectives. By mastering these skills, you will be able to demonstrate the significance of your work, contribute to the existing body of knowledge, and potentially pave the way for further research in your field.
Throughout the blog post, I will provide concrete examples from various disciplines to illustrate the implementation of these techniques. Additionally, I will offer valuable tips on avoiding common pitfalls, ensuring the accuracy and reliability of your results, and seeking feedback from your advisors or peers to enhance the quality of your analysis.
If you are in paucity of time, not confident of your writing skills and in a hurry to complete the writing task then you can think of hiring a research consultant that solves all your problems. Please visit my article on Hiring a Research consultant for your PhD tasks for further details.
Organizing the results of your study in a logical and coherent manner is crucial for effectively communicating your findings. By presenting your results in an organized structure, you enhance the clarity and readability of your dissertation. Here are some key considerations for organizing your results:
- Research studies often involve complex algorithms, software implementations, experimental data, and performance metrics. It is essential to organize these diverse elements in a cohesive manner to make it easier for readers to follow your research. A well-structured results section enables readers to understand the progression of your experiments and the relationship between different findings.
- Begin by reminding readers of the research questions or hypotheses that guided your study. This alignment helps establish a clear connection between the objectives of your research and the subsequent presentation of results. For example, if your research question focuses on evaluating the efficiency of a new sorting algorithm, you would present the experimental data, performance metrics, and comparative analyses specific to that algorithm in relation to the research question.
- Subsubsection 1.1: Experimental Setup
- Subsubsection 1.2: Experimental Results and Analysis
- Subsubsection 2.1: Performance Metrics
- Subsubsection 2.2: Comparative Results and Discussion
Remember to tailor the organization of your results section to the specific requirements of your research. The key is to provide a logical flow and structure that enables readers to easily comprehend and interpret your findings.
Providing context for the results of your study is essential to help readers understand the significance and implications of your findings. By offering background information and study design details, you establish a foundation upon which the results can be properly interpreted. Here are some key considerations for providing context:
- Before delving into the results, it is important to provide readers with relevant background information about the topic or problem being addressed. This may include a literature review of existing research, theories, or methodologies in the field. By doing so, you situate your work within the broader landscape of and demonstrate its relevance. Additionally, explain the design of your study, such as the specific algorithms, software frameworks, datasets, or hardware setups used. This ensures that readers understand the context in which your results were obtained.
- Provide a brief overview of the current state-of-the-art in image recognition algorithms and their limitations.
- Explain the specific challenges or gaps in the existing methods that motivated your research.
- Describe the design of your study, including the choice of machine learning techniques, datasets used for training and evaluation, preprocessing steps, and any hardware or software configurations.
By providing context, you allow readers to understand the background, motivation, and methodology behind your research. This sets the stage for better comprehension and interpretation of your results. Contextualizing your findings, as it helps establish the relevance, novelty, and potential impact of your research within the larger field.
Presenting data in a clear and organized manner is crucial for effectively communicating your results. The way you present your data can greatly impact the reader’s understanding and interpretation of your findings. Here are some key considerations for presenting data:
- Presenting performance metrics of different algorithms using a table to allow for easy comparison.
- Using a line graph to depict the improvement in accuracy over training iterations in a machine learning model.
- Employing a bar chart to compare the execution times of different algorithms on a specific dataset.
- Clear labelling and formatting of your data ensure that readers can easily understand and interpret the information presented. Label each table, figure, chart, or graph with a concise and descriptive title. Ensure that axes, legends, and labels are clearly labelled and units of measurement are specified. Use appropriate fonts, colours, and styles to enhance readability. Consider providing captions or footnotes to provide additional context or explanations where necessary.
- In the text, refer to a specific table presenting the accuracy results of different algorithms and explain how these results support your research hypothesis or contribute to the field.
- Discuss a figure showing the relationship between the number of training examples and the performance of a machine learning model, emphasizing its implications for scalability and generalization.
By presenting data in a visually appealing and well-organized manner, you enhance the clarity and accessibility of your results. Proper labelling, formatting, and referring to each table or figure in the text help readers navigate the information and grasp its significance. Remember to choose the most appropriate format for your data and use visuals to support and reinforce your findings.
The inclusion of statistical analyses in the results section is crucial for providing objective and quantitative evidence to support your findings. Statistical analyses help you draw meaningful conclusions from your data and determine the significance of observed results. Here are some key considerations for describing statistical analyses:
- Statistical analyses play a vital role in determining the reliability and significance of your findings. They provide a systematic and objective framework for interpreting the data and testing hypotheses. Discuss the importance of including statistical analyses in the results section to demonstrate the rigour and validity of your research.
- Describe using a t-test to compare the means of two groups in a user study, as it is appropriate for assessing the statistical significance of differences.
- Explain employing logistic regression to model the relationship between independent variables and a binary outcome in a predictive analytics study.
- Report the p-value as 0.032, indicating a statistically significant difference between the two groups at the 0.05 significance level.
- Interpret an effect size of 0.40 as a medium-sized effect, highlighting its practical importance in the context of the research.
By describing the statistical analyses conducted, explaining the rationale behind the chosen tests, and accurately presenting the statistical values and interpretations, you strengthen the validity and reliability of your findings. Statistical analyses provide an objective framework for drawing conclusions from your data and lend credibility to your research in the computer science domain.
Reporting the findings of your research in an objective, concise, and clear manner is essential for effectively communicating your results. Here are some key considerations for reporting the findings:
- Summarize the key findings of a machine learning study by stating that “the proposed algorithm achieved an average accuracy of 85% on the test dataset, outperforming existing state-of-the-art methods by 10%.”
- For a research question about the impact of different programming languages on software performance, present specific metrics such as execution time or memory usage for each language, along with a comparison and interpretation of the results.
- Instead of using overly technical language, communicate the results in a more accessible way: “The experimental results showed a significant correlation between the number of training samples and the accuracy of the model, indicating that a larger training dataset leads to improved prediction performance.”
By guiding readers on summarizing the results objectively and concisely, addressing each research question or hypothesis, and using clear and concise language, you ensure that your findings are communicated effectively. This approach allows readers to understand the core contributions of your research and how they align with the research questions or hypotheses you set out to investigate.
Providing strong evidence from the data to support your findings, addressing unexpected or contradictory results, and discussing limitations and potential explanations are essential components of reporting research findings. Here are some key considerations for supporting the findings:
- Present empirical evidence from a user study, such as participant feedback or performance metrics, to support the usability and effectiveness of a proposed user interface design.
- If a software system performed unexpectedly poorly in certain scenarios, discuss potential factors such as data bias, implementation issues, or limitations of the evaluation methodology that could have influenced the results.
- Acknowledge limitations such as a small sample size, limited dataset availability, or computational constraints that might affect the generalizability or robustness of the results.
- Discuss potential explanations for unexpected results, such as issues with data quality, algorithmic complexity, or model assumptions.
By providing evidence from the data to support the findings, addressing unexpected or contradictory results, and discussing limitations and potential explanations, you demonstrate a rigorous and reflective approach to your research in the computer science domain. This allows readers to assess the strength and reliability of your findings and gain a deeper understanding of the nuances and implications of your work.
Using visual representations, such as tables, graphs, and figures, alongside the text can greatly enhance the understanding and impact of your findings. Here are some key considerations for visual representation:
Visual representations offer several benefits in presenting research findings. They provide a concise and intuitive way to convey complex information, trends, and patterns. Visuals can help readers grasp key insights at a glance, enhance the overall readability of the document, and make the findings more memorable. Visual representations also facilitate effective comparisons, highlight important relationships, and aid in storytelling. Example:
When creating visual representations, consider the following tips to ensure clarity and effectiveness: a. Choose the appropriate visual format: Select the most suitable format, such as tables, line graphs, scatter plots, or heatmaps, based on the nature of the data and the message you want to convey.
b. Simplify and declutter: Avoid overwhelming the visuals with excessive data points, labels, or unnecessary decorations. Keep the design clean and focused on conveying the essential information.
c. Label and title clearly: Provide descriptive and informative titles for tables, graphs, and figures. Label the axes, data points, or components clearly to facilitate understanding.
d. Use colors and visual cues purposefully: Utilize colors and visual cues to highlight important information or differentiate between categories. Ensure that the chosen colors are distinguishable and accessible. e. Provide legends and captions: Include legends to explain symbols, colors, or abbreviations used in the visuals. Provide informative captions or annotations to guide readers in interpreting the visuals accurately. Example:
By incorporating clear and effective visual representations alongside the text, you enhance the presentation and understanding of your research findings in the computer science domain. Well-designed tables, graphs, and figures can simplify complex information, facilitate comparisons, and enhance the visual appeal of your dissertation. Remember to choose appropriate formats, keep the visuals uncluttered, label clearly, and use colors and visual cues purposefully to maximize their impact.
Writing the results section of a dissertation or thesis is a critical task that requires careful attention to detail, organization, and effective communication. Throughout this blog post, we have explored key elements to consider when crafting this section.
Upcoming Events
- Visit the Upcoming International Conferences at Exotic Travel Destinations with Travel Plan
- Visit for Research Internships Worldwide
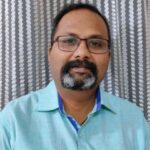
Recent Posts
- Best 5 Journals for Quick Review and High Impact in August 2024
- 05 Quick Review, High Impact, Best Research Journals for Submissions for July 2024
- Top Mistakes to Avoid When Writing a Research Paper
- Average Stipend for Research/Academic Internships
- These Institutes Offer Remote Research/Academic Internships
- All Blog Posts
- Research Career
- Research Conference
- Research Internship
- Research Journal
- Research Tools
- Uncategorized
- Research Conferences
- Research Journals
- Research Grants
- Internships
- Research Internships
- Email Templates
- Conferences
- Blog Partners
- Privacy Policy
Copyright © 2024 Research Voyage
Design by ThemesDNA.com

- Submit a Document

Don't have an account yet? Sign Up!
Already have an account? Log In!
Complete the form below to receive an email with the authorization code needed to reset your password.
- Articles & Writing Help
"The easiest way to get English editing services of a decent quality at a reasonable price in a reliable way." -Jiyeon, United States
Using Correlation Coefficients in Research Papers
Academics have many options when it comes to the research they want to complete. A great option, especially for students, is correlational research. As you'll soon see, this type of analysis is fantastic for conducting quick research on a small or non-existent budget.
In this article, you'll learn about correlational research and correlation coefficients. You will be guided through the steps of gathering, analyzing, and reporting correlational data in your research papers. By the end, you should have a solid understanding of how to find correlation coefficients and use them to present your research findings.
Introduction to Correlation Coefficients
A correlation measures the linear relationship between two variables. The correlation coefficient describes the strength and direction of the relationship between those variables. The correlation coefficient ranges from -1 to +1, where 0 describes no relationship between the variables, and an absolute value of 1 describes a perfect relationship between the variables.
The purpose of correlational research is to pinpoint whether there is some sort of linear relationship between a couple of variables. For example, you may want to know whether the amount of time a student spends studying correlates with the grade they receive. By surveying the students in one class, you could gather data on the number of hours they study per week, along with the final grade they received for that class. Plugging that data into a correlation coefficient formula, you would receive a value ranging from -1 to +1. That value would tell you if grades increase as study hours increase, if they decrease as study hours increase, or if the grades did not have a discernable pattern based on hours studied.
However, it's important to understand that correlation is not equal to causation. If you found, using correlational research, that grades improved as students spent more time studying, you wouldn't be able to conclude that studying more causes students to receive better grades. As you will see later, there can be other explanations for the results you find.
How Is Correlational Research Useful?
If you can't determine causation with correlational research, you may wonder what the point of it is. Why not just conduct experimental research instead? Here are the main reasons someone would consider correlational research:
- It's much quicker than experimental research. With correlational research, you can gather data from natural (non-experimental) settings in a relatively short time.
- It's generally cheaper than experimental research. Correlational research normally involves less time and resources than experimental research, making it a cheaper and easier option.
When Would You Use Correlational Research?
There are a few instances where correlational research is beneficial, even more so than experimental research:
- Investigating non-causal relationships. You won't always expect a causal relationship between two variables, but it can still be helpful to know if they correlate.
- Supporting causal relationship theories. It can sometimes be too expensive, impractical, or even unethical to run experiments that would determine a causal relationship between variables. In those cases, a strong correlational relationship can support the theory of a causal relationship.
- Testing new measurement tools. If a correlational relationship between variables is already well-known, you can conduct correlational research on those variables using new measurement tools to measure their validity and reliability.
Defining Correlation Coefficients
The value of the correlation coefficient details how strong the relationship between those variables is. A value of -1 or +1 is a perfect correlation, meaning that the relationship between the variables is perfectly linear. The closer the value gets to 0, the less linear the relationship is. Whether the value is positive or negative is also important, as that tells you the direction of the relationship. A negative relationship means that if one variable increases, the other variable decreases. A positive relationship means that if one variable increases, the other variable increases as well.
There are multiple different correlation coefficients you can use depending on the data that you've gathered. The correlation coefficient formula also differs depending on the coefficient you're looking for.
Types of Correlation Coefficients
- Pearson's r: The relationship between two continuous, random variables that are both normally distributed. Data must meet these criteria to use Pearson’s accurately.
- Spearman's rho: The relationship between two continuous or ordinal variables. These variables do not need to be normally distributed to use Spearman's rho, and this correlation coefficient is commonly used when the criteria for Pearson's are not met. This coefficient is based on the ranking of the data and not their actual values.
- Kendall's tau: This is an extension of Spearman's rho. You would use this coefficient when using a small dataset and one rank is repeated too many times.
- Phi Coefficient: The strength of the relationship between two categorical variables in a 2x2 contingency table.
- Cramer's V Correlation: The strength of the relationship between two categorical variables in contingency tables larger than 2x2.
Collecting Correlational Data
Just like experimental research, correlational research uses quantitative research methods. The difference is that the variables in correlational research are only observed and not manipulated as they would be in experimental research. There are a few different ways to collect correlational data:
- Surveys: You can use questionnaires to quickly collect data from your population of interest. This can be done in person, but it can more easily be done online, by mail, or by phone.
- Observation: This type of data collection involves observing behavior or phenomena in a natural environment. This often involves recording, counting, and describing the environment, events, and actions you're recording.
- Secondary sources: Not all data needs to be original, and you can find sets of data that have already been collected for several different purposes. This is the fastest and most inexpensive way to collect data, but it can also be unreliable. As you didn't collect it yourself, you don't have any control over the reliability and validity of the data.
Analyzing Correlational Data
Analyzing correlational data begins with plotting the data and calculating the correlation coefficient. As previously mentioned, the correlation coefficient will provide you with a value that represents the strength and direction of the relationship. Plotting the data on the graph will provide you with a visual representation of that relationship.
For determining the strength of the correlation, there are some general guidelines on correlation coefficient interpretations.
The Absolute Value of the Correlation Coefficient | Correlation Coefficient Interpretation |
0.00-0.10 | Negligible |
0.10-0.39 | Weak |
0.40-0.69 | Moderate |
0.70-0.89 | Strong |
0.90-1.00 | Very Strong |
There are a couple of caveats to keep in mind when analyzing and interpreting the results of your correlational analysis. The first is that values near 0 don't necessarily mean that there is no relationship at all between the variables. It only means that there's no linear relationship. However, there could be some other relationship, such as a quadratic relationship. Graphing the data before performing an analysis will allow you to see what the relationship looks like.
The other is that, once again, correlation is not causation. When you discover a correlational relationship, there could be multiple reasons for that result that aren't accounted for in research.
One issue is what's known as the directionality problem. Let's think about the studying and grades example, and we find that as students study more, their grades increase. You could assume that studying more results in better grades, but you could equally assume that getting better grades results in more studying.
Another possible issue is a third variable that wasn't considered in the research. With the studying and grades example, it could be another variable that increases both studying and grades, and that those two don't affect each other in any way. It's entirely possible that students who sleep more tend to study more and get better grades.
Present Both Good Research and Writing
Correlational research is a great option for students, and academics in general, to study relationships between variables. There are a variety of ways to gather, analyze, and interpret correlational data. This information should provide you with a solid foundation to get started on your own correlational research. For more information on conducting research and writing your research papers, take a look at our resources page . When you're ready for rewriting services or proofreading services , our PhD writers and editors are available to help.
Results Section
The results section is where you tell the reader the basic descriptive information about the scales you used (report the mean and standard deviation for each scale). If you have more than 3 or 4 variables in your paper, you might want to put this descriptive information in a table to keep the text from being too choppy and bogged down (see the APA manual for ideas on creating good tables). In the results section, you also tell the reader what statistics you conducted to test your hypothesis (-ses) and what the results indicated. In this paper, you conducted bivariate correlation(s) to test your hypothesis.
Include in Results (include the following in this order in your results section):
Give the descriptive statistics for the relevant variables (mean, standard deviation).
Provide a brief rephrasing of your hypothesis(es) (avoid exact restatement). Then tell the reader what statistical test you used to test your hypothesis and what you found.
Explain which correlations were in the predicted direction, and which were not (if any). Were differences statistically significant (i.e., p < .05 or below)? Don't merely give the statistics without any explanation. Whenever you make a claim that there is (or is not) a significant correlation between X and Y, the reader has to be able to verify it by looking at the appropriate test statistic. For example do not report “The correlation between private self-consciousness and college adjustment was r = - .26, p < .01.” In general, you should not use numbers as part of a sentence in this way. Instead, interpret important data for the reader and use words throughout your sentences: “The negative correlation between private self-consciousness and college adjustment indicated that the more participants felt self-conscious, the worse their adjustment to college, r = - .26, p < .01”
However, don't try to interpret why you got the results you did. Leave that to the Discussion.
Note : Be sure to underline all abbreviations of test statistics (e.g., M for mean and SD for standard deviation). See pages 112-118 of the APA manual for more on reporting statistics in text.
Some specifics:
For each correlation, you need to report the following information either in the text of your paper or in a table: correlation coefficient, significance level (p value).
If you are reporting a single correlation for the whole results section, report it in the text of the paper as follows: r =.26, p < .01 or r = -.11, n.s.
Use n.s. if not significant; or use whichever of the following is most accurate:
p < .05; p < .01; p < .001
If your correlation was non significant, but p < .10 you can still talk about it. You might put the following text in your paper: “While the correlation was not significant relative to the standard alpha level of .05, the p-value was less than .10.” Then provide a rationale for why you should still be able to discuss this non-significant correlation (see your hypothesis testing lecture notes). You may then cautiously interpret such a correlation. Don’t make grand conclusions or use strong language based on the existence of a marginally significant finding. Also, you should indicate that a marginal correlation is non -significant in a table; only refer to the correlation as “approaching significance” in the text of the paper .
If you computed two or more correlations (thus involving at least three variables) provide a table at the end of the paper (ordinarily tables would only be used for even more complex findings, but I'd like you to practice since you have a few correlations to work with). Create a correlation matrix like the example (see Table 1). If you include a correlation matrix table, you should, in the text of the result section, refer readers to your table instead of typing out the r and the p value for each correlation. If you are using Word as your word processor, create the table, then you can adjust the "borders and shading" for each cell/row/column to get the table formatted properly. I can show you how if you have trouble. Other word processors should have similar functions.
This Table is an Example of a Correlation Matrix among Three Variables for an Imaginary Sample of College Students (n = 129).
Variable | 1 | 2 | 3 |
1. name of variable | --- | .56 | -.29 |
2. name of next var. |
| --- | .44 |
3. name of 3 var. |
|
| -- |
* p < .05; **p < .01
=====================================================================
You need to report the statistics in some way in your result section, but regardless of whether you use a table or type the statistics in the text, you should also interpret the correlation for the reader… say exactly what that means:
E.g. “As expected, college adjustment was positively correlated with the amount of contact with friends and family members (see Table 1).”
E.g. “No significant relationship was found between the importance of one's social life and social adjustment to college, r = -.11, n.s.
E.g. “As shown in Table 1, some of my predictions were supported. There was a significant correlation between extroversion and life satisfaction. However, life satisfaction was not significantly related to college adjustment.”
See your text, APA manual, and Sample Paper (“The Title of the Paper”) for more information and suggestions. In general, I would suggest writing the words of the results section first, and then going back to insert the numbers and statistical information.
Discussion section
In your discussion section, relate the results back to your initial hypotheses. Do they support or disconfirm them? Remember: Results do not prove hypotheses right or wrong, they support them or fail to provide support for them.
I suggest the following information in the following order:
Provide a very brief summary of the most important parts of the introduction and then the results sections. In doing so, you should relate the results to the theories you introduced in the Introduction. Your findings are just one piece among many -- resist the tendency to make your results the final story about the phenomenon or theory of interest. Integrate the results and try to make sense of the pattern of the findings.
In the case of a correlational project, be careful to not use causal language to discuss your results – unless you did an experiment you cannot infer causality. However, it would be impossible to fully discuss the implications of your results without making reference to causality. That is fine. Just don't claim that your results themselves are demonstrating causality.
If your findings did not support your hypotheses, speculate why that might be so. You might reconsider the logic of your hypotheses. Or, reconsider whether the variables are adequately measuring the relationship. For example, if you hypothesized a relationship between anger toward the stigmatized and narcissism and didn’t find it – consider whether anger is really the right variable... perhaps "disgust" would better capture the relationship. Alternatively, you might also consider whether the relationship you hypothesized might only show up in certain populations of people or under certain conditions (e.g., self-threat). Where possible, support your speculation with references.
Talk about any qualifications important to your findings (all studies have weaknesses/qualifications). This includes alternative explanations for the results. For example, you might speculate about an unexamined third variable that was not present in you study. However, BE SPECIFIC and back up any assertions you make. For example, if you claim that 3 rd variables might affect your correlations, tell me what they are and how they would affect your correlations.
Speculate about future directions that research could take to further investigate your question. This might relate back to any weaknesses you’ve mentioned above (or reasons why the results didn’t turn out as expected). Future directions may also include interesting next steps in the research.
A discussion section is about “what we have learned so far”; and “where we should go next”; Your final conclusion should talk briefly about the broader significance of your findings. What do they imply about human nature or some aspect of it? (Don't wildly speculate, however!) Leave the reader feeling like this is an important topic... you will likely refer back to your opening paragraph of the introduction here and have partial answers or more specific responses to the questions you posed.
Important Parts of the Paper – Don’t Forget Them!!
Title page - Try to write a title that maximally informs the reader about the topic, without being ridiculously long. Use titles of articles you've read as examples of form. Also provide the RUNNING HEAD and an abbreviated title that appears in the header of each page along with the page number. Provide your name and institutional affiliation (Hanover College). See APA Manual and sample paper.
Abstract - Write the abstract LAST. An abstract is a super-short summary and is difficult to write.
Info on abstracts from APA manual:
An abstract is a brief, comprehensive summary of the contents of an article, allowing readers to survey the contents quickly.
A good abstract is:
accurate : Ensure that your abstract correctly reflects the purpose and content of your paper. Do not include information that does not appear in the body of the paper.
self-contained : Define all abbreviations and acronyms. Spell out names of tests/ questionnaires. Define unique terms. Paraphrase rather than quote.
concise and specific : Make each sentence maximally informative, especially the lead sentence. Begin the abstract with the most important information (your question), but do not repeat the title. Be as brief as possible.
non-evaluative : Report rather than evaluate: do not add to or comment on what is in the body of the manuscript.
coherent and readable : Write in clear and vigorous prose. Use the third person rather than the first person.
In less than 150 words your abstract should describe:
the problem under investigation (an "introduction" type sentence)
the specific variables investigated and the method of doing so (a "method" type sentence)
the results of the study in brief (no numbers, just words)
a hint about the general direction the discussion section takes
References : Use APA style. See your APA manual, textbook and the sample paper for examples of how to cite and how to make a reference list. Make sure that all references mentioned in the text are also mentioned in the reference list and vice versa.
Tables and/or Figures : Use APA style. Tables go at the very end of your paper. Make sure you refer to the table or figure in the text of your paper.
*See APA Manual, textbook and sample paper for information on how to format each section of your paper and how to order the sections.

How to Write an Impressive Thesis Results Section
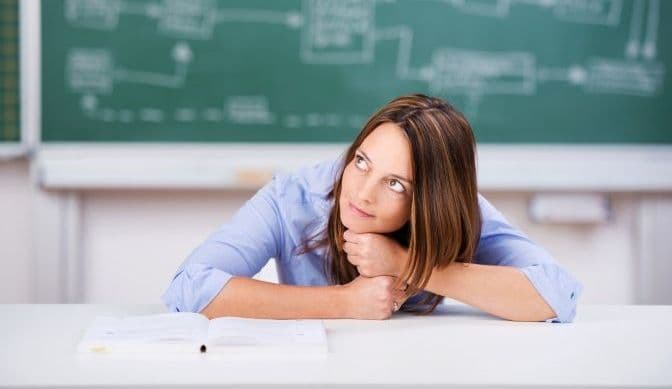
After collecting and analyzing your research data, it’s time to write the results section. This article explains how to write and organize the thesis results section, the differences in reporting qualitative and quantitative data, the differences in the thesis results section across different fields, and the best practices for tables and figures.
What is the thesis results section?
The thesis results section factually and concisely describes what was observed and measured during the study but does not interpret the findings. It presents the findings in a logical order.
What should the thesis results section include?
- Include all relevant results as text, tables, or figures
- Report the results of subject recruitment and data collection
- For qualitative research, present the data from all statistical analyses, whether or not the results are significant
- For quantitative research, present the data by coding or categorizing themes and topics
- Present all secondary findings (e.g., subgroup analyses)
- Include all results, even if they do not fit in with your assumptions or support your hypothesis
What should the thesis results section not include?
- If the study involves the thematic analysis of an interview, don’t include complete transcripts of all interviews. Instead, add these as appendices
- Don’t present raw data. These may be included in appendices
- Don’t include background information (this should be in the introduction section )
- Don’t speculate on the meaning of results that do not support your hypothesis. This will be addressed later in the discussion and conclusion sections.
- Don’t repeat results that have been presented in tables and figures. Only highlight the pertinent points or elaborate on specific aspects
How should the thesis results section be organized?
The opening paragraph of the thesis results section should briefly restate the thesis question. Then, present the results objectively as text, figures, or tables.
Quantitative research presents the results from experiments and statistical tests , usually in the form of tables and figures (graphs, diagrams, and images), with any pertinent findings emphasized in the text. The results are structured around the thesis question. Demographic data are usually presented first in this section.
For each statistical test used, the following information must be mentioned:
- The type of analysis used (e.g., Mann–Whitney U test or multiple regression analysis)
- A concise summary of each result, including descriptive statistics (e.g., means, medians, and modes) and inferential statistics (e.g., correlation, regression, and p values) and whether the results are significant
- Any trends or differences identified through comparisons
- How the findings relate to your research and if they support or contradict your hypothesis
Qualitative research presents results around key themes or topics identified from your data analysis and explains how these themes evolved. The data are usually presented as text because it is hard to present the findings as figures.
For each theme presented, describe:
- General trends or patterns observed
- Significant or representative responses
- Relevant quotations from your study subjects
Relevant characteristics about your study subjects
Differences among the results section in different fields of research
Nevertheless, results should be presented logically across all disciplines and reflect the thesis question and any hypotheses that were tested.
The presentation of results varies considerably across disciplines. For example, a thesis documenting how a particular population interprets a specific event and a thesis investigating customer service may both have collected data using interviews and analyzed it using similar methods. Still, the presentation of the results will vastly differ because they are answering different thesis questions. A science thesis may have used experiments to generate data, and these would be presented differently again, probably involving statistics. Nevertheless, results should be presented logically across all disciplines and reflect the thesis question and any hypotheses that were tested.
Differences between reporting thesis results in the Sciences and the Humanities and Social Sciences (HSS) domains
In the Sciences domain (qualitative and experimental research), the results and discussion sections are considered separate entities, and the results from experiments and statistical tests are presented. In the HSS domain (qualitative research), the results and discussion sections may be combined.
There are two approaches to presenting results in the HSS field:
- If you want to highlight important findings, first present a synopsis of the results and then explain the key findings.
- If you have multiple results of equal significance, present one result and explain it. Then present another result and explain that, and so on. Conclude with an overall synopsis.
Best practices for using tables and figures
The use of figures and tables is highly encouraged because they provide a standalone overview of the research findings that are much easier to understand than wading through dry text mentioning one result after another. The text in the results section should not repeat the information presented in figures and tables. Instead, it should focus on the pertinent findings or elaborate on specific points.
Some popular software programs that can be used for the analysis and presentation of statistical data include Statistical Package for the Social Sciences (SPSS ) , R software , MATLAB , Microsoft Excel, Statistical Analysis Software (SAS) , GraphPad Prism , and Minitab .
The easiest way to construct tables is to use the Table function in Microsoft Word . Microsoft Excel can also be used; however, Word is the easier option.
General guidelines for figures and tables
- Figures and tables must be interpretable independent from the text
- Number tables and figures consecutively (in separate lists) in the order in which they are mentioned in the text
- All tables and figures must be cited in the text
- Provide clear, descriptive titles for all figures and tables
- Include a legend to concisely describe what is presented in the figure or table
Figure guidelines
- Label figures so that the reader can easily understand what is being shown
- Use a consistent font type and font size for all labels in figure panels
- All abbreviations used in the figure artwork should be defined in the figure legend
Table guidelines
- All table columns should have a heading abbreviation used in tables should be defined in the table footnotes
- All numbers and text presented in tables must correlate with the data presented in the manuscript body
Quantitative results example : Figure 3 presents the characteristics of unemployed subjects and their rate of criminal convictions. A statistically significant association was observed between unemployed people <20 years old, the male sex, and no household income.
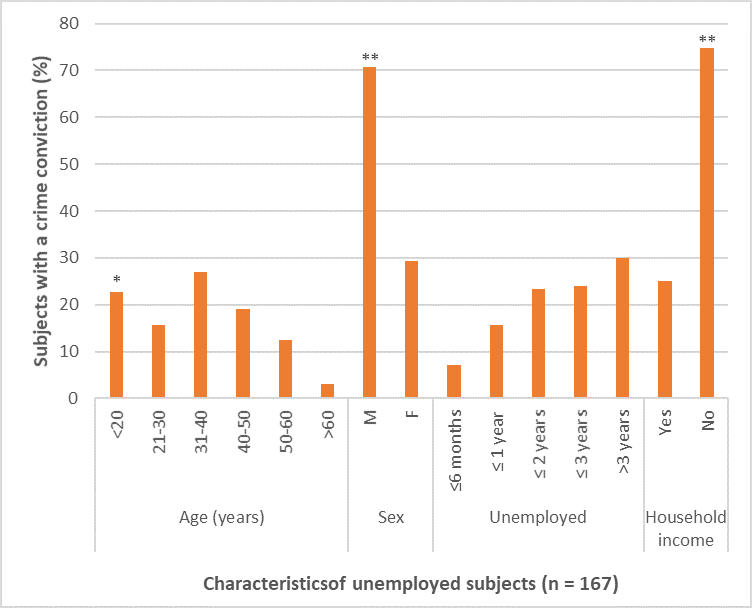
Qualitative results example: Table 5 shows the themes identified during the face-to-face interviews about the application that we developed to anonymously report corruption in the workplace. There was positive feedback on the app layout and ease of use. Concerns that emerged from the interviews included breaches of confidentiality and the inability to report incidents because of unstable cellphone network coverage.
|
|
Ease of use of the app | The app was easy to use, and I did not have to contact the helpdesk |
I wish all apps were so user-friendly! | |
App layout | The screen was not cluttered. The text was easy to read |
The icons on the screen were easy to understand | |
Confidentiality | I am scared that the app developers will disclose my name to my employer |
Unstable network coverage | I was unable to report an incident that occurred at one of our building sites because there was no cellphone reception |
I wanted to report the incident immediately , but I had to wait until I was home, where the cellphone network signal was strong |
Table 5. Themes and selected quotes from the evaluation of our app designed to anonymously report workplace corruption.
Tips for writing the thesis results section
- Do not state that a difference was present between the two groups unless this can be supported by a significant p-value .
- Present the findings only . Do not comment or speculate on their interpretation.
- Every result included must have a corresponding method in the methods section. Conversely, all methods must have associated results presented in the results section.
- Do not explain commonly used methods. Instead, cite a reference.
- Be consistent with the units of measurement used in your thesis study. If you start with kg, then use the same unit all throughout your thesis. Also, be consistent with the capitalization of units of measurement. For example, use either “ml” or “mL” for milliliters, but not both.
- Never manipulate measurement outcomes, even if the result is unexpected. Remain objective.
Results vs. discussion vs. conclusion
Results are presented in three sections of your thesis: the results, discussion, and conclusion.
- In the results section, the data are presented simply and objectively. No speculation or interpretation is given.
- In the discussion section, the meaning of the results is interpreted and put into context (e.g., compared with other findings in the literature ), and its importance is assigned.
- In the conclusion section, the results and the main conclusions are summarized.
A thesis is the most crucial document that you will write during your academic studies. For professional thesis editing and thesis proofreading services , visit Enago Thesis Editing for more information.
Editor’s pick
Get free updates.
Subscribe to our newsletter for regular insights from the research and publishing industry!
Review Checklist
Have you completed all data collection procedures and analyzed all results ?
Have you included all results relevant to your thesis question, even if they do not support your hypothesis?
Have you reported the results objectively , with no interpretation or speculation?
For quantitative research, have you included both descriptive and inferential statistical results and stated whether they support or contradict your hypothesis?
Have you used tables and figures to present all results?
In your thesis body, have you presented only the pertinent results and elaborated on specific aspects that were presented in the tables and figures?
Are all tables and figures correctly labeled and cited in numerical order in the text?
Frequently Asked Questions
What file formats do you accept for plagiarism check service +.
We accept all file formats, including Microsoft Word, Microsoft Excel, PDF, Latex, etc.
What information do i need to provide with my Plagiarism Check order? +
Please upload your research manuscript when you order Plagiarism Check Service . If you want to include the tables, charts, and figure legends in the plagiarism check, please ensure that all content is in editable formats and in one single document.
Is repetition percentage of 25-30% considered acceptable by the journal editors? +
Acceptable repetition rate varies by journal but aim for low percentages (usually <5%). Avoid plagiarism (including self-plagiarism), cite sources, and use detection tools. Plagiarism can lead to rejection, reputation damage, and serious consequences. Consult your institution for guidance on addressing plagiarism concerns.
Do you offer help to rewrite and paraphrase text that has plagiarism concern in my manuscript? +
We can help you rewrite and paraphrase text in your manuscript to ensure it is not plagiarized under our Developmental Content Rewriting Service. You can provide specific passages or sentences that you are concerned about, and we can assist you in rephrasing them or citing the source materials in a proper format.
Which languages does iThenticate support in its database? +
iThenticate searches for content matches in the following 30 languages: Chinese (Simplified and Traditional), Japanese, Thai, Korean, Catalan, Croatian, Czech, Danish, Dutch, Finnish, French, German, Hungarian, Italian, Norwegian (Bokmal, Nynorsk), Polish, Portuguese, Romanian, Serbian, Slovak, Slovenian, Spanish, Swedish, Arabic, Greek, Hebrew, Farsi, Russian, and Turkish. Please note that iThenticate will match your text with text of the same language.
- USC Libraries
- Research Guides
Organizing Your Social Sciences Research Paper
- 7. The Results
- Purpose of Guide
- Design Flaws to Avoid
- Independent and Dependent Variables
- Glossary of Research Terms
- Reading Research Effectively
- Narrowing a Topic Idea
- Broadening a Topic Idea
- Extending the Timeliness of a Topic Idea
- Academic Writing Style
- Applying Critical Thinking
- Choosing a Title
- Making an Outline
- Paragraph Development
- Research Process Video Series
- Executive Summary
- The C.A.R.S. Model
- Background Information
- The Research Problem/Question
- Theoretical Framework
- Citation Tracking
- Content Alert Services
- Evaluating Sources
- Primary Sources
- Secondary Sources
- Tiertiary Sources
- Scholarly vs. Popular Publications
- Qualitative Methods
- Quantitative Methods
- Insiderness
- Using Non-Textual Elements
- Limitations of the Study
- Common Grammar Mistakes
- Writing Concisely
- Avoiding Plagiarism
- Footnotes or Endnotes?
- Further Readings
- Generative AI and Writing
- USC Libraries Tutorials and Other Guides
- Bibliography
The results section is where you report the findings of your study based upon the methodology [or methodologies] you applied to gather information. The results section should state the findings of the research arranged in a logical sequence without bias or interpretation. A section describing results should be particularly detailed if your paper includes data generated from your own research.
Annesley, Thomas M. "Show Your Cards: The Results Section and the Poker Game." Clinical Chemistry 56 (July 2010): 1066-1070.
Importance of a Good Results Section
When formulating the results section, it's important to remember that the results of a study do not prove anything . Findings can only confirm or reject the hypothesis underpinning your study. However, the act of articulating the results helps you to understand the problem from within, to break it into pieces, and to view the research problem from various perspectives.
The page length of this section is set by the amount and types of data to be reported . Be concise. Use non-textual elements appropriately, such as figures and tables, to present findings more effectively. In deciding what data to describe in your results section, you must clearly distinguish information that would normally be included in a research paper from any raw data or other content that could be included as an appendix. In general, raw data that has not been summarized should not be included in the main text of your paper unless requested to do so by your professor.
Avoid providing data that is not critical to answering the research question . The background information you described in the introduction section should provide the reader with any additional context or explanation needed to understand the results. A good strategy is to always re-read the background section of your paper after you have written up your results to ensure that the reader has enough context to understand the results [and, later, how you interpreted the results in the discussion section of your paper that follows].
Bavdekar, Sandeep B. and Sneha Chandak. "Results: Unraveling the Findings." Journal of the Association of Physicians of India 63 (September 2015): 44-46; Brett, Paul. "A Genre Analysis of the Results Section of Sociology Articles." English for Specific Speakers 13 (1994): 47-59; Go to English for Specific Purposes on ScienceDirect;Burton, Neil et al. Doing Your Education Research Project . Los Angeles, CA: SAGE, 2008; Results. The Structure, Format, Content, and Style of a Journal-Style Scientific Paper. Department of Biology. Bates College; Kretchmer, Paul. Twelve Steps to Writing an Effective Results Section. San Francisco Edit; "Reporting Findings." In Making Sense of Social Research Malcolm Williams, editor. (London;: SAGE Publications, 2003) pp. 188-207.
Structure and Writing Style
I. Organization and Approach
For most research papers in the social and behavioral sciences, there are two possible ways of organizing the results . Both approaches are appropriate in how you report your findings, but use only one approach.
- Present a synopsis of the results followed by an explanation of key findings . This approach can be used to highlight important findings. For example, you may have noticed an unusual correlation between two variables during the analysis of your findings. It is appropriate to highlight this finding in the results section. However, speculating as to why this correlation exists and offering a hypothesis about what may be happening belongs in the discussion section of your paper.
- Present a result and then explain it, before presenting the next result then explaining it, and so on, then end with an overall synopsis . This is the preferred approach if you have multiple results of equal significance. It is more common in longer papers because it helps the reader to better understand each finding. In this model, it is helpful to provide a brief conclusion that ties each of the findings together and provides a narrative bridge to the discussion section of the your paper.
NOTE: Just as the literature review should be arranged under conceptual categories rather than systematically describing each source, you should also organize your findings under key themes related to addressing the research problem. This can be done under either format noted above [i.e., a thorough explanation of the key results or a sequential, thematic description and explanation of each finding].
II. Content
In general, the content of your results section should include the following:
- Introductory context for understanding the results by restating the research problem underpinning your study . This is useful in re-orientating the reader's focus back to the research problem after having read a review of the literature and your explanation of the methods used for gathering and analyzing information.
- Inclusion of non-textual elements, such as, figures, charts, photos, maps, tables, etc. to further illustrate key findings, if appropriate . Rather than relying entirely on descriptive text, consider how your findings can be presented visually. This is a helpful way of condensing a lot of data into one place that can then be referred to in the text. Consider referring to appendices if there is a lot of non-textual elements.
- A systematic description of your results, highlighting for the reader observations that are most relevant to the topic under investigation . Not all results that emerge from the methodology used to gather information may be related to answering the " So What? " question. Do not confuse observations with interpretations; observations in this context refers to highlighting important findings you discovered through a process of reviewing prior literature and gathering data.
- The page length of your results section is guided by the amount and types of data to be reported . However, focus on findings that are important and related to addressing the research problem. It is not uncommon to have unanticipated results that are not relevant to answering the research question. This is not to say that you don't acknowledge tangential findings and, in fact, can be referred to as areas for further research in the conclusion of your paper. However, spending time in the results section describing tangential findings clutters your overall results section and distracts the reader.
- A short paragraph that concludes the results section by synthesizing the key findings of the study . Highlight the most important findings you want readers to remember as they transition into the discussion section. This is particularly important if, for example, there are many results to report, the findings are complicated or unanticipated, or they are impactful or actionable in some way [i.e., able to be pursued in a feasible way applied to practice].
NOTE: Always use the past tense when referring to your study's findings. Reference to findings should always be described as having already happened because the method used to gather the information has been completed.
III. Problems to Avoid
When writing the results section, avoid doing the following :
- Discussing or interpreting your results . Save this for the discussion section of your paper, although where appropriate, you should compare or contrast specific results to those found in other studies [e.g., "Similar to the work of Smith [1990], one of the findings of this study is the strong correlation between motivation and academic achievement...."].
- Reporting background information or attempting to explain your findings. This should have been done in your introduction section, but don't panic! Often the results of a study point to the need for additional background information or to explain the topic further, so don't think you did something wrong. Writing up research is rarely a linear process. Always revise your introduction as needed.
- Ignoring negative results . A negative result generally refers to a finding that does not support the underlying assumptions of your study. Do not ignore them. Document these findings and then state in your discussion section why you believe a negative result emerged from your study. Note that negative results, and how you handle them, can give you an opportunity to write a more engaging discussion section, therefore, don't be hesitant to highlight them.
- Including raw data or intermediate calculations . Ask your professor if you need to include any raw data generated by your study, such as transcripts from interviews or data files. If raw data is to be included, place it in an appendix or set of appendices that are referred to in the text.
- Be as factual and concise as possible in reporting your findings . Do not use phrases that are vague or non-specific, such as, "appeared to be greater than other variables..." or "demonstrates promising trends that...." Subjective modifiers should be explained in the discussion section of the paper [i.e., why did one variable appear greater? Or, how does the finding demonstrate a promising trend?].
- Presenting the same data or repeating the same information more than once . If you want to highlight a particular finding, it is appropriate to do so in the results section. However, you should emphasize its significance in relation to addressing the research problem in the discussion section. Do not repeat it in your results section because you can do that in the conclusion of your paper.
- Confusing figures with tables . Be sure to properly label any non-textual elements in your paper. Don't call a chart an illustration or a figure a table. If you are not sure, go here .
Annesley, Thomas M. "Show Your Cards: The Results Section and the Poker Game." Clinical Chemistry 56 (July 2010): 1066-1070; Bavdekar, Sandeep B. and Sneha Chandak. "Results: Unraveling the Findings." Journal of the Association of Physicians of India 63 (September 2015): 44-46; Burton, Neil et al. Doing Your Education Research Project . Los Angeles, CA: SAGE, 2008; Caprette, David R. Writing Research Papers. Experimental Biosciences Resources. Rice University; Hancock, Dawson R. and Bob Algozzine. Doing Case Study Research: A Practical Guide for Beginning Researchers . 2nd ed. New York: Teachers College Press, 2011; Introduction to Nursing Research: Reporting Research Findings. Nursing Research: Open Access Nursing Research and Review Articles. (January 4, 2012); Kretchmer, Paul. Twelve Steps to Writing an Effective Results Section. San Francisco Edit ; Ng, K. H. and W. C. Peh. "Writing the Results." Singapore Medical Journal 49 (2008): 967-968; Reporting Research Findings. Wilder Research, in partnership with the Minnesota Department of Human Services. (February 2009); Results. The Structure, Format, Content, and Style of a Journal-Style Scientific Paper. Department of Biology. Bates College; Schafer, Mickey S. Writing the Results. Thesis Writing in the Sciences. Course Syllabus. University of Florida.
Writing Tip
Why Don't I Just Combine the Results Section with the Discussion Section?
It's not unusual to find articles in scholarly social science journals where the author(s) have combined a description of the findings with a discussion about their significance and implications. You could do this. However, if you are inexperienced writing research papers, consider creating two distinct sections for each section in your paper as a way to better organize your thoughts and, by extension, your paper. Think of the results section as the place where you report what your study found; think of the discussion section as the place where you interpret the information and answer the "So What?" question. As you become more skilled writing research papers, you can consider melding the results of your study with a discussion of its implications.
Driscoll, Dana Lynn and Aleksandra Kasztalska. Writing the Experimental Report: Methods, Results, and Discussion. The Writing Lab and The OWL. Purdue University.
- << Previous: Insiderness
- Next: Using Non-Textual Elements >>
- Last Updated: Jul 30, 2024 10:20 AM
- URL: https://libguides.usc.edu/writingguide
Have a language expert improve your writing
Run a free plagiarism check in 10 minutes, automatically generate references for free.
- Knowledge Base
- Dissertation
- How to Write a Results Section | Tips & Examples
How to Write a Results Section | Tips & Examples
Published on 27 October 2016 by Bas Swaen . Revised on 25 October 2022 by Tegan George.
A results section is where you report the main findings of the data collection and analysis you conducted for your thesis or dissertation . You should report all relevant results concisely and objectively, in a logical order. Don’t include subjective interpretations of why you found these results or what they mean – any evaluation should be saved for the discussion section .
Instantly correct all language mistakes in your text
Be assured that you'll submit flawless writing. Upload your document to correct all your mistakes.
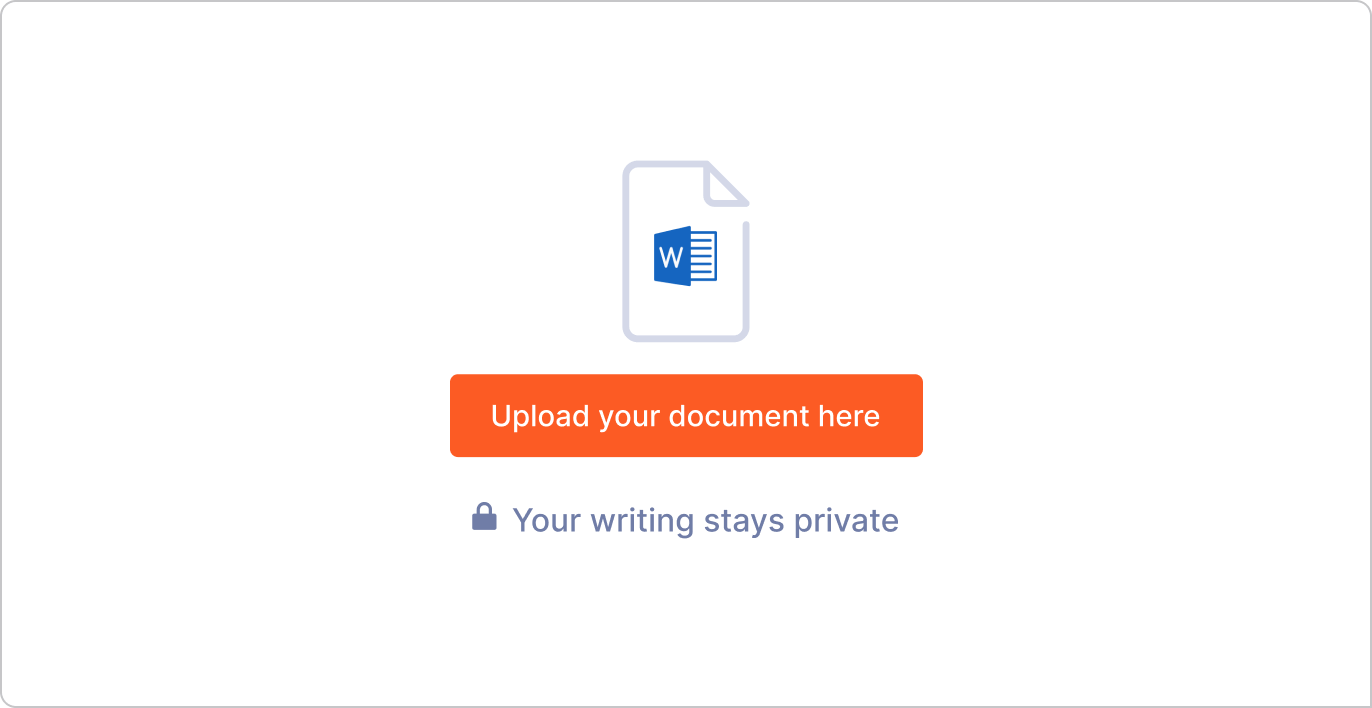
Table of contents
How to write a results section, reporting quantitative research results, reporting qualitative research results, results vs discussion vs conclusion, checklist: research results, frequently asked questions about results sections.
When conducting research, it’s important to report the results of your study prior to discussing your interpretations of it. This gives your reader a clear idea of exactly what you found and keeps the data itself separate from your subjective analysis.
Here are a few best practices:
- Your results should always be written in the past tense.
- While the length of this section depends on how much data you collected and analysed, it should be written as concisely as possible.
- Only include results that are directly relevant to answering your research questions . Avoid speculative or interpretative words like ‘appears’ or ‘implies’.
- If you have other results you’d like to include, consider adding them to an appendix or footnotes.
- Always start out with your broadest results first, and then flow into your more granular (but still relevant) ones. Think of it like a shoe shop: first discuss the shoes as a whole, then the trainers, boots, sandals, etc.
Prevent plagiarism, run a free check.
If you conducted quantitative research , you’ll likely be working with the results of some sort of statistical analysis .
Your results section should report the results of any statistical tests you used to compare groups or assess relationships between variables . It should also state whether or not each hypothesis was supported.
The most logical way to structure quantitative results is to frame them around your research questions or hypotheses. For each question or hypothesis, share:
- A reminder of the type of analysis you used (e.g., a two-sample t test or simple linear regression ). A more detailed description of your analysis should go in your methodology section.
- A concise summary of each relevant result, both positive and negative. This can include any relevant descriptive statistics (e.g., means and standard deviations ) as well as inferential statistics (e.g., t scores, degrees of freedom , and p values ). Remember, these numbers are often placed in parentheses.
- A brief statement of how each result relates to the question, or whether the hypothesis was supported. You can briefly mention any results that didn’t fit with your expectations and assumptions, but save any speculation on their meaning or consequences for your discussion and conclusion.
A note on tables and figures
In quantitative research, it’s often helpful to include visual elements such as graphs, charts, and tables , but only if they are directly relevant to your results. Give these elements clear, descriptive titles and labels so that your reader can easily understand what is being shown. If you want to include any other visual elements that are more tangential in nature, consider adding a figure and table list .
As a rule of thumb:
- Tables are used to communicate exact values, giving a concise overview of various results
- Graphs and charts are used to visualise trends and relationships, giving an at-a-glance illustration of key findings
Don’t forget to also mention any tables and figures you used within the text of your results section. Summarise or elaborate on specific aspects you think your reader should know about rather than merely restating the same numbers already shown.
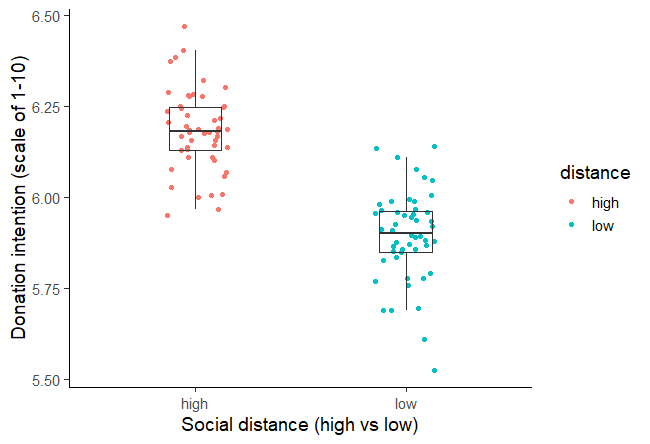
Figure 1: Intention to donate to environmental organisations based on social distance from impact of environmental damage.
In qualitative research , your results might not all be directly related to specific hypotheses. In this case, you can structure your results section around key themes or topics that emerged from your analysis of the data.
For each theme, start with general observations about what the data showed. You can mention:
- Recurring points of agreement or disagreement
- Patterns and trends
- Particularly significant snippets from individual responses
Next, clarify and support these points with direct quotations. Be sure to report any relevant demographic information about participants. Further information (such as full transcripts , if appropriate) can be included in an appendix .
‘I think that in role-playing games, there’s more attention to character design, to world design, because the whole story is important and more attention is paid to certain game elements […] so that perhaps you do need bigger teams of creative experts than in an average shooter or something.’
Responses suggest that video game consumers consider some types of games to have more artistic potential than others.
Your results section should objectively report your findings, presenting only brief observations in relation to each question, hypothesis, or theme.
It should not speculate about the meaning of the results or attempt to answer your main research question . Detailed interpretation of your results is more suitable for your discussion section , while synthesis of your results into an overall answer to your main research question is best left for your conclusion .
I have completed my data collection and analyzed the results.
I have included all results that are relevant to my research questions.
I have concisely and objectively reported each result, including relevant descriptive statistics and inferential statistics .
I have stated whether each hypothesis was supported or refuted.
I have used tables and figures to illustrate my results where appropriate.
All tables and figures are correctly labelled and referred to in the text.
There is no subjective interpretation or speculation on the meaning of the results.
You've finished writing up your results! Use the other checklists to further improve your thesis.
The results chapter of a thesis or dissertation presents your research results concisely and objectively.
In quantitative research , for each question or hypothesis , state:
- The type of analysis used
- Relevant results in the form of descriptive and inferential statistics
- Whether or not the alternative hypothesis was supported
In qualitative research , for each question or theme, describe:
- Recurring patterns
- Significant or representative individual responses
- Relevant quotations from the data
Don’t interpret or speculate in the results chapter.
Results are usually written in the past tense , because they are describing the outcome of completed actions.
The results chapter or section simply and objectively reports what you found, without speculating on why you found these results. The discussion interprets the meaning of the results, puts them in context, and explains why they matter.
In qualitative research , results and discussion are sometimes combined. But in quantitative research , it’s considered important to separate the objective results from your interpretation of them.
Cite this Scribbr article
If you want to cite this source, you can copy and paste the citation or click the ‘Cite this Scribbr article’ button to automatically add the citation to our free Reference Generator.
Swaen, B. (2022, October 25). How to Write a Results Section | Tips & Examples. Scribbr. Retrieved 30 July 2024, from https://www.scribbr.co.uk/thesis-dissertation/results-section/
Is this article helpful?
Other students also liked
What is a research methodology | steps & tips, how to write a discussion section | tips & examples, how to write a thesis or dissertation conclusion.
- +44 (0) 207 391 9032
Recent Posts
- Top 5 Essential Skills You Should Build As An International Student
- How Professional Editing Services Can Take Your Writing to the Next Level
- How to Write an Effective Essay Outline
- How to Write a Law Essay: A Comprehensive Guide with Examples
- What Are the Limitations of ChatGPT?
- How to Properly Write an Essay Outline Using ChatGpt
- Why Presentation Skills Are Important for Students
- Tips on How to Make an Essay Longer
- How to Structure Your Dissertation in 2024
- How to Write a Research Paper Like a Pro
- Academic News
- Custom Essays
- Dissertation Writing
- Essay Marking
- Essay Writing
- Essay Writing Companies
- Model Essays
- Model Exam Answers
- Oxbridge Essays Updates
- PhD Writing
- Significant Academics
- Student News
- Study Skills
- University Applications
- University Essays
- University Life
- Writing Tips

Writing up the results section of your dissertation
(Last updated: 12 May 2021)
Since 2006, Oxbridge Essays has been the UK’s leading paid essay-writing and dissertation service
We have helped 10,000s of undergraduate, Masters and PhD students to maximise their grades in essays, dissertations, model-exam answers, applications and other materials. If you would like a free chat about your project with one of our UK staff, then please just reach out on one of the methods below.
When asked why doing a dissertation can be such a headache, the typical student usually replies with one of two answers. Either, they simply don't like writing enormous volumes of text, or – and you may relate here – they categorically do not enjoy analysing data. "It's so long and boring!", the typical student wails.
Well, students wail, and we answer. We have put together this very comprehensive, very useful guide on how to write up the results section of your dissertation. To help you further, we've broken the information down into both quantitative and qualitative results, so you can focus on what applies to you most.
Writing up your quantitative results
Understanding the basics of your research.
First, you need to recall what you have assessed – or what your main variables are.
All quantitative research has at least one independent and one dependent variable, and, at this point, you should define them explicitly. An independent variable is one that you control to test its effects on the dependent variable. A dependent variable is thus your outcome variable.
Second, you need to determine if your variables were categorical or continuous.
A categorical variable is one with a fixed number of possible values, and a continuous variable is one where final scores have a wide range. Finally, you need to recall if you have used a so-called covariate or confounder variable. This is a variable that could have influenced the relationship between your independent and dependent variable, and that you controlled in order to accurately estimate the relationship between your main variables.
Let’s explain all this with an example. Suppose that your research was to assess whether height is associated with self-esteem. Here, participants’ height is an independent variable and self-esteem is a dependent variable. Because both height and scores on a measure of self-esteem can have a wide range, you have two continuous variables. You might have also wanted to see if the relationship between height and self-esteem exists after controlling for participants’ weight. In this case, weight is a confounding variable that you need to control for.
Here is another example. You might have assessed whether more females than males want to read a specific romantic novel. Here, your independent variable is gender and your dependent variable is the determination to read the book. Since gender has categories (male and female), this is a categorical variable. If you have assessed the determination to read the book on a scale from 1 to 10 (e.g. 1 = no determination at all to read the book, all the way to 10 = extremely strong determination to read it), then this is a continuous variable; however, if you have asked your participants to say whether they do or do not want to read the book, then this is a categorical variable (since there are two categories: “yes” and “no”).
Lastly, you might have wanted to see if the link between gender and the determination to read the book exists after controlling for participants’ current relationship status. Here, relationship status is your confounding variable.
We will return to these examples throughout this blog post. At this point, it is important to remember that outlining your research in this way helps you to write up your results section in the easiest way possible.
Let’s move on to the next step.
Outlining descriptive and frequencies statistics
In order to report descriptive and/or frequencies statistics, you need to outline all variables that you have used in your research and note whether those variables are continuous or categorical.
For continuous variables, you are using descriptive statistics and reporting the measures of central tendency (mean) and measures of variability or spread (standard deviation). For categorical variables, you are using frequencies statistics and reporting the number (or frequency) of participants per category and associated percentages. Both these statistics require you to make a table, and in both cases you also need to comment upon the statistics.
How does all of this look in practice? Recall the two examples that were outlined above. If you have assessed the association between participants’ height and self-esteem, while controlling for participants’ weight, then your research consists of three continuous variables. You need to make a table, as in TABLE 1 below, which identifies means and standard deviations for all these variables. When commenting upon the results, you can say:
Participants were on average 173.50 cm tall (SD = 5.81) and their mean weight was 65.31 kg (SD = 4.44). On average, participants had moderate levels of self-esteem (M = 5.55, SD = 2.67).
Note that, in this example, you are concluding that participants had moderate self-esteem levels if their self-esteem was assessed on a 1 to 10 scale. Since the value of 5 falls within the middle of this range, you are concluding that the mean value of self-esteem is moderate. If the mean value was higher (e.g., M = 8.33), you would conclude that participants’ self-esteem was, on average, high; and if the mean value was lower (e.g., M = 2.44), you would conclude that average self-esteem scores were low.
M | SD | |
---|---|---|
Height (cm) | 173.50 | 5.81 |
Weight (kg) | 65.31 | 4.44 |
Self-esteem | 5.55 | 2.67 |
Let’s now return to our second research example and say that you want to report the degree to which males and females want to read a romantic novel, where this determination was assessed on a 1-10 (continuous) scale. This would look as shown in TABLE 2.
Males | Females | |
---|---|---|
Determination to read the book | M = 3.20 | M = 6.33 |
Determination to read the book | SD = .43 | SD = 1.36 |
We can see how to report frequencies statistics for different groups by referring to our second example about gender, determination to read a romantic novel, and participants’ relationship status.
Here, you have three categorical variables (if determination to read the novel was assessed by having participants reply with “yes” or “no”). Thus, you are not reporting means and standard deviations, but frequencies and percentages.
To put this another way, you are noting how many males versus females wanted to read the book and how many of them were in a relationship, as shown in TABLE 3. You can report these statistics in this way:
Twenty (40%) male participants wanted to read the book and 35 (70%) female participants wanted to read the book. Moreover, 22 (44%) males and 26 (52%) females indicated that they are currently in a relationship.
Males | Females | |
---|---|---|
Determination to read the book | ||
Yes | 20 (40%) | 35 (70%) |
No | 30 (60%) | 15 (30%) |
Relationship status | ||
Yes | 22 (44%) | 26 (52%) |
No | 28 (56%) | 24 (48%) |
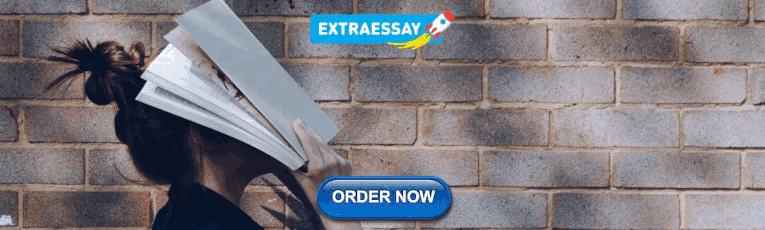
Reporting the results of a correlation analysis
The first of these is correlation, which you use when you want to establish if one or more (continuous, independent) variables relate to another (continuous, dependent) variable. For instance, you may want to see if participants’ height correlates with their self-esteem levels.
The first step here is to report whether your variables are normally distributed. You do this by looking at a histogram that describes your data. If the histogram has a bell-shaped curve (see purple graph below), your data is normally distributed and you need to rely on a Pearson correlation analysis. Here, you need to report the obtained r value (correlation coefficient) and p value (which needs to be lower than .05 in order to establish significance). If you find a correlation, you need to say something like:

One final thing to note, which is important for all analyses, is that when your p value is indicated to be .000, you never report it by saying “ p = .000”, but by noting “ p p = .011”.
If your data is skewed rather than normally distributed (see red graphs), then you need to rely on a Spearman correlation analysis. Here, you report the results by saying:
Spearman correlation analysis revealed a positive relationship between people’s height and their self-esteem (r s = .44, p There has been a significant positive correlation between height and self-esteem after controlling for participants’ weight ( r = .39, p = .034).
You also need to make a table that will summarise your main results. If you didn’t use a covariate, you will have a fairly simple table, such as that shown in TABLE 4. If you have used a covariate, your table is slightly more complex, such as that shown in TABLE 5. Note that both tables use “-” to indicate correlations that have already been noted within the table. Also note how “*”, “**”, and “***” are used to annotate correlations that are significant at different levels.
Height (cm) | Self-esteem | |
---|---|---|
Height (cm) | 1.00 | – |
Self-esteem | .44*** | 1.00 |
*** |
Control variables | Height (cm) | Self-esteem | Weight (kg) | |
---|---|---|---|---|
None | Height (cm) | 1.00 | – | – |
Self-esteem | .44*** | 1.00 | – | |
Weight (kg) | .38** | .32** | 1.00 | |
Weight (kg) | Height (cm) | 1.00 | – | – |
Self-esteem | .39* | 1.00 | -.44 | |
* |
Reporting the results of a regression
These are the specific points that you need to address in order to make sure that all assumptions have been met:
(1) for the assumption of no multicollinearity (i.e., a lack of high correlation between your independent variables), you need to establish that none of your Tolerance statistics are below .01 and none of the VIF statistics are above 10;
(2) for the assumption of no autocorrelation of residuals (i.e., a lack of correlation between the residuals of two observations), you need to look at this table and see whether your Durbin-Watson statistic falls within a desirable range, depending on your number of participants and the number of predictors (independent variables); and,
(3) for the assumptions of linearity (i.e., a linear relationship between independent and dependent variables) and homoscedasticity (i.e., a variance of error terms that should be similar across the independent variables), you need to look at the scatterplot of standardised residual on standardised predicted value and conclude that your graph doesn’t funnel out or curve.
All of this may sound quite complex. But in reality it is not: you just need to look at your results output to note the Tolerance and VIF values, Durbin-Watson value, and the scatterplot. Once you conclude that your assumptions have been met, you write something like:
Since none of the VIF values were below 0.1 and none of the Tolerance values were above 10, the assumption of no multicollinearity has been met. Durbin-Watson statistics fell within an expected range, thus indicating that the assumption of no autocorrelation of residuals has been met as well. Finally, the scatterplot of standardised residual on standardised predicted value did not funnel out or curve, and thus the assumptions of linearity and homoscedasticity have been met as well.
If your assumptions have not been met, you need to dig a bit deeper and understand what this means. A good idea would be to read the chapter on regression (and especially the part about assumptions) written by Andy Field. You can access his book here . This will help you understand all you need to know about the assumptions of a regression analysis, how to test them, and what to do if they have not been met.
Now let’s focus on reporting the results of the actual regression analysis. Let’s say that you wanted to see if participants’ height predicts their self-esteem, after controlling for participants’ weight. You have entered height and weight as predictors in the model and self-esteem as a dependent variable.
First, you need to report whether the model reached significance in predicting self-esteem scores. Look at the results of an ANOVA analysis in your output and note the F value, degrees of freedom for the model and for residuals, and significance level. These values are shown in PICTURE 2.

Significance value tells you if your predictor reached significance – such as whether participants’ height predicted self-esteem scores. You also need to comment upon the β value. This value represents the change in the outcome associated with a unit change in the predictor . Thus, if your β value is .351 for participants’ height (predictor/independent variable), then this means that for every increase in height by 1 cm, self-esteem increases by .35. You need to report the same thing for your other predictor – that is, participants’ weight.
Finally, since your model included both height and weight as predictors, and height acted as a significant predictor, you can conclude that participants’ height influences their self-esteem after controlling for weight.

Reporting the results of a chi-square analysis
For instance, you would do a chi-square analysis when you want to see whether gender (categorical independent variable with two levels: males/females) affects the determination to read a book, when this variable is measured with yes/no answers (categorical dependent variable with two levels: yes/no).
When reporting your results, you should first make a table as shown in TABLE 3 above. Then you need to report the results of a chi-square test, by noting the Pearson chi-square value, degrees of freedom, and significance value. You can see all these in your output.
Finally, you need to report the strength of the association, for which you need to look at the Phi and Cramer’s V values. When each of your variables has only two categories, as in the present example, Phi and Cramer’s V values are identical and it doesn’t matter which one you will report. However, when one of your variables has more than two categories, it is better to report the Cramer’s V value. You report these values by indicating the actual value and the associated significance level.
Note that Cramer’s V value can range from 0 to 1. The closer the value is to 1, the higher the strength of the association. You can report the results of the chi-square analysis in the following way:
Reporting the results of a t-test analysis
Recall that you have previously outlined descriptive statistics for these variables, where you have noted means and standard deviations for males’ and females’ scores on the determination to read the novel (see TABLE 2 above). Now you need to report the obtained t value, degrees of freedom, and significance level – all of which you can see in your results output.
You can say something like:
Reporting the results of one-way ANOVA
In the t-test example, you had two conditions of a categorical independent variable, which corresponded to whether a participant was male or female. You would have three conditions of an independent variable when assessing whether relationship status (independent variable with three levels: single, in a relationship, and divorced) affects the determination to read a romantic novel (as assessed on a 1-10 scale).
Here, you would report the results in a similar manner to that of a t -test. You first report the means and standard deviations on the determination to read the book for all three groups of participants, by saying who had the highest and lowest mean. Then you report the results of the ANOVA test by reporting the F value, degrees of freedom (for within-subjects and between-subjects comparisons), and the significance value.
There are two things to note here. First, before reporting your results, you need to look at your output to see whether the so-called Levene’s test is significant. This test assesses the homogeneity of variance – the assumption being that all comparison groups should have the same variance. If the test is non-significant, the assumption has been met and you are reporting the standard F value.
However, if the test is significant, the assumption has been violated and you need to report instead the Welch statistic, associated degrees of freedom, and significance value (which you will see in your output; for example, see PICTURE 3 above).
The second thing to note is that ANOVA tells you only whether there were significant differences between groups – but if there are differences, it doesn’t tell you where these differences lie. For this, you need to conduct a post-hoc comparison (Tukey’s HSD test). The output will tell you which comparisons are significant.
You can report your results in the following manner:
Reporting the results of ANCOVA
For instance, you will use ANCOVA when you want to test whether relationship status (categorical independent variable with three levels: single, in a relationship, divorced) affects the determination to read a romantic novel (continuous dependent variable, assessed on a 1-10 scale) after controlling for participants’ general interest in books (continuous covariate, assessed on a 1-10 scale).
To report the results, you need to look at the “test of between-subjects effects” table in your output. You need to report the F values, degrees of freedom (for each variable and error), and significance values for both the covariate and the main independent variable. As with ANOVA, a significant ANCOVA doesn’t tell you where the differences lie. For this, you need to conduct planned contrasts and report the associated significance values for different comparisons.
You can report the results in the following manner:
Reporting the results of MANOVA
For instance, you would use MANOVA when testing whether male versus female participants (independent variable) show a different determination to read a romantic novel (dependent variable) and a determination to read a crime novel (dependent variable).
When reporting the results, you first need to notice whether the so-called Box’s test and Levene’s test are significant. These tests assess two assumptions: that there is an equality of covariance matrices (Box’s test) and that there is an equality of variances for each dependent variable (Levene’s test).
Both tests need to be non-significant in order to assess whether your assumptions are met. If the tests are significant, you need to dig deeper and understand what this means. Once again, you may find it helpful to read the chapter by Andy Field on MANOVA, which can be accessed here .
Following this, you need to report your descriptive statistics, as outlined previously. Here, you are reporting the means and standard deviations for each dependent variable, separately for each group of participants. Then you need to look at the results of “multivariate analyses”.
You will notice that you are presented with four statistic values and associated F and significance values. These are labelled as Pillai’s Trace, Wilks’ Lambda, Hotelling’s Trace, and Roy’s Largest Root. These statistics test whether your independent variable has an effect on the dependent variables. The most common practice is to report only the Pillai’s Trace. You report the results in the same manner as reporting ANOVA, by noting the F value, degrees of freedom (for hypothesis and error), and significance value.
However, you also need to report the statistic value of one of the four statistics mentioned above. You can label the Pillai’s Trace statistic with V, the Wilks’ Lambda statistic with A, the Hotelling’s Trace statistic with T, and Roy’s Largest Root statistic with Θ (but you need report only one of them).
Finally, you need to look at the results of the Tests of Between-Subjects Effects (which you will see in your output). These tests tell you how your independent variable affected each dependent variable separately. You report these results in exactly the same way as in ANOVA.
Here’s how you can report all results from MANOVA:
Writing up your qualitative results
Before reporting the results of your qualitative research, you need to recall what type of research you have conducted. The most common types of qualitative research are interviews, observations, and focus groups – and your research is likely to fall into one of these types.
All three types of research are reported in a similar manner. Still, it may be useful if we focus on each of them separately.
Reporting the results of interviews
For example, let’s say that your qualitative research focused on young people’s reasons for smoking. You have asked your participants questions that explored why they started smoking, why they continue to smoke, and why they wish to quit smoking. Since your research was organised in this manner, you already have three major themes: (1) reasons for starting to smoke, (2) reasons for continuing to smoke, and (3) reasons for quitting smoking. You then explore particular reasons why your participants started to smoke, why they continue to smoke, and why they want to quit. Each reason that you identify will act as a subtheme.
When reporting the results, you should organise your text in subsections. Each section should refer to one theme. Then, within each section, you need to discuss the subthemes that you discovered in your data.
Let’s say that you found that young people started smoking because: (1) they thought smoking was cool, (2) they experienced peer pressure, (3) their parents modelled smoking behaviour, (4) they thought smoking reduces stress, and (5) they wanted to try something new. Now you have five subthemes within your “reasons for starting to smoke” theme. What you need to do now is to present the findings for each subtheme, while also reporting quotes that best describe your subtheme. You do that for each theme and subtheme.
It is also good practice to make a table that lists all your themes, subthemes, and associated quotes.
Here’s an example of how to report a quote within a text:
Reporting the results of observations
For instance, you might have noticed that the therapist finds it important to discuss: (1) the origin of the problem, (2) the lack of a patient’s medical difficulties, (3) the experience of stress, (4) the link of stress to the problem, and (5) the new understanding of the problem. You can consider these as themes in your observations.
Accordingly, you will want to report each theme separately. You do this by outlining your observation first (this can be a conversation or a behaviour that you observed), and then commenting upon it.
Here’s an example:
Therapist: Was there something that stressed you out during the last few months?
Patient: Yes, of course. I thought I would lose my job, but that passed. After that, I was breaking up with my girlfriend. But between those things, I was fine.
Therapist: And was there any difference in your symptoms while you were and while you were not stressed?
Patient: Hmmm. Actually yes. Now that I think of it, they were mostly present when I went through those periods.
Therapist: Could it be that stress intensifies your symptoms?
Patient: I never thought of it. I guess it seems logical. Is it?
Reporting the results of focus groups
As an example, let’s say that your focus group dealt with identifying reasons why some people prefer Coca-Cola over Schweppes, and vice versa. You have transcribed your focus group sessions and have extracted themes from the data. You have discovered a wide variety of reasons why people prefer one of the two drinks.
When reporting your results, you should have two sections: one listing reasons for favouring Coca-Cola, and the other listing reasons for favouring Schweppes. Within each section, you need to identify specific reasons for these preferences. You should connect these specific reasons to particular quotes.
Here’s an example of how this may look:
In conclusion…
As we have seen, writing up qualitative results is easier than writing quantitative results. Yet, even reporting statistics is not that hard, especially if you have a good guide to help you.
Hopefully, this guide has reduced your worries and increased your confidence that you can write up the results section of your dissertation without too many difficulties.

How to correctly reference a dissertation

How to finish and format your dissertation

Dissertation writing: publishing a dissertation
- dissertation help
- dissertation research
- dissertation results
- study skills
- writing tips
Writing Services
- Essay Plans
- Critical Reviews
- Literature Reviews
- Presentations
- Dissertation Title Creation
- Dissertation Proposals
- Dissertation Chapters
- PhD Proposals
- Journal Publication
- CV Writing Service
- Business Proofreading Services
Editing Services
- Proofreading Service
- Editing Service
- Academic Editing Service
Additional Services
- Marking Services
- Consultation Calls
- Personal Statements
- Tutoring Services
Our Company
- Frequently Asked Questions
- Become a Writer
Terms & Policies
- Fair Use Policy
- Policy for Students in England
- Privacy Policy
- Terms & Conditions
- [email protected]
- Contact Form
Payment Methods
Cryptocurrency payments.
Have a language expert improve your writing
Run a free plagiarism check in 10 minutes, generate accurate citations for free.
- Knowledge Base
- Correlation Coefficient | Types, Formulas & Examples
Correlation Coefficient | Types, Formulas & Examples
Published on August 2, 2021 by Pritha Bhandari . Revised on June 22, 2023.
A correlation coefficient is a number between -1 and 1 that tells you the strength and direction of a relationship between variables .
In other words, it reflects how similar the measurements of two or more variables are across a dataset.
Correlation coefficient value | Correlation type | Meaning |
---|---|---|
1 | Perfect positive correlation | When one variable changes, the other variables change in the same direction. |
0 | Zero correlation | There is no relationship between the variables. |
-1 | Perfect negative correlation | When one variable changes, the other variables change in the opposite direction. |
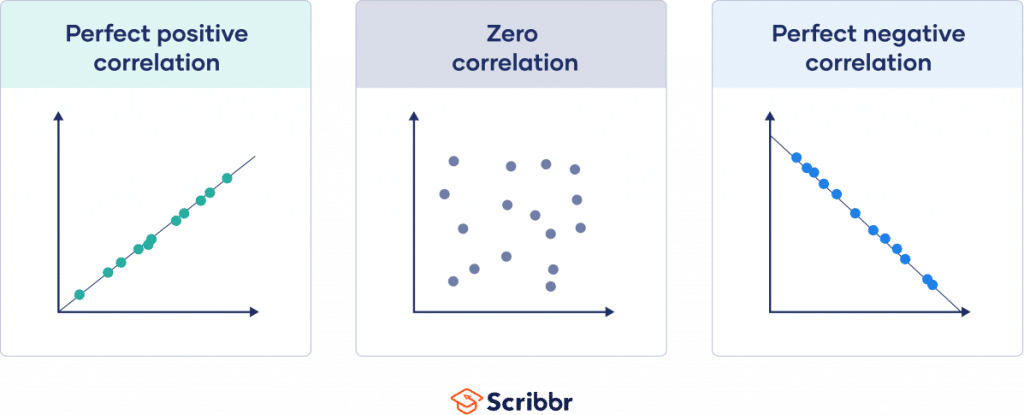
Table of contents
What does a correlation coefficient tell you, using a correlation coefficient, interpreting a correlation coefficient, visualizing linear correlations, types of correlation coefficients, pearson’s r, spearman’s rho, other coefficients, other interesting articles, frequently asked questions about correlation coefficients.
Correlation coefficients summarize data and help you compare results between studies.
Summarizing data
A correlation coefficient is a descriptive statistic . That means that it summarizes sample data without letting you infer anything about the population. A correlation coefficient is a bivariate statistic when it summarizes the relationship between two variables, and it’s a multivariate statistic when you have more than two variables.
If your correlation coefficient is based on sample data, you’ll need an inferential statistic if you want to generalize your results to the population. You can use an F test or a t test to calculate a test statistic that tells you the statistical significance of your finding.
Comparing studies
A correlation coefficient is also an effect size measure, which tells you the practical significance of a result.
Correlation coefficients are unit-free, which makes it possible to directly compare coefficients between studies.
Receive feedback on language, structure, and formatting
Professional editors proofread and edit your paper by focusing on:
- Academic style
- Vague sentences
- Style consistency
See an example
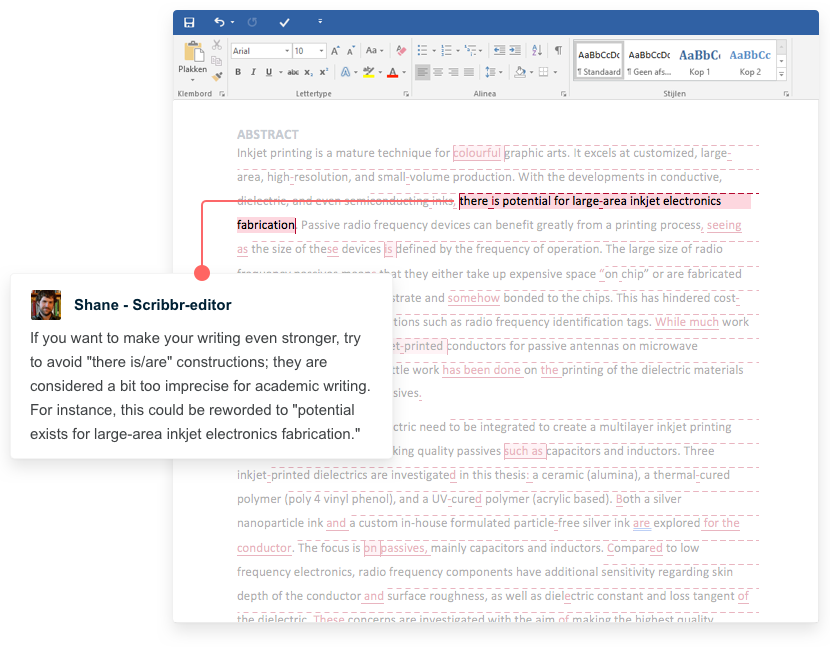
In correlational research , you investigate whether changes in one variable are associated with changes in other variables.
After data collection , you can visualize your data with a scatterplot by plotting one variable on the x-axis and the other on the y-axis. It doesn’t matter which variable you place on either axis.
Visually inspect your plot for a pattern and decide whether there is a linear or non-linear pattern between variables. A linear pattern means you can fit a straight line of best fit between the data points, while a non-linear or curvilinear pattern can take all sorts of different shapes, such as a U-shape or a line with a curve.
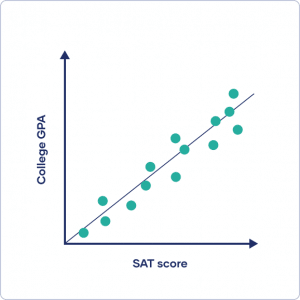
There are many different correlation coefficients that you can calculate. After removing any outliers , select a correlation coefficient that’s appropriate based on the general shape of the scatter plot pattern. Then you can perform a correlation analysis to find the correlation coefficient for your data.
You calculate a correlation coefficient to summarize the relationship between variables without drawing any conclusions about causation .
Both variables are quantitative and normally distributed with no outliers, so you calculate a Pearson’s r correlation coefficient .
The value of the correlation coefficient always ranges between 1 and -1, and you treat it as a general indicator of the strength of the relationship between variables.
The sign of the coefficient reflects whether the variables change in the same or opposite directions: a positive value means the variables change together in the same direction, while a negative value means they change together in opposite directions.
The absolute value of a number is equal to the number without its sign. The absolute value of a correlation coefficient tells you the magnitude of the correlation: the greater the absolute value, the stronger the correlation.
There are many different guidelines for interpreting the correlation coefficient because findings can vary a lot between study fields. You can use the table below as a general guideline for interpreting correlation strength from the value of the correlation coefficient.
While this guideline is helpful in a pinch, it’s much more important to take your research context and purpose into account when forming conclusions. For example, if most studies in your field have correlation coefficients nearing .9, a correlation coefficient of .58 may be low in that context.
Correlation coefficient | Correlation strength | Correlation type |
---|---|---|
-.7 to -1 | Very strong | Negative |
-.5 to -.7 | Strong | Negative |
-.3 to -.5 | Moderate | Negative |
0 to -.3 | Weak | Negative |
0 | None | Zero |
0 to .3 | Weak | Positive |
.3 to .5 | Moderate | Positive |
.5 to .7 | Strong | Positive |
.7 to 1 | Very strong | Positive |
The correlation coefficient tells you how closely your data fit on a line. If you have a linear relationship, you’ll draw a straight line of best fit that takes all of your data points into account on a scatter plot.
The closer your points are to this line, the higher the absolute value of the correlation coefficient and the stronger your linear correlation.
If all points are perfectly on this line, you have a perfect correlation.
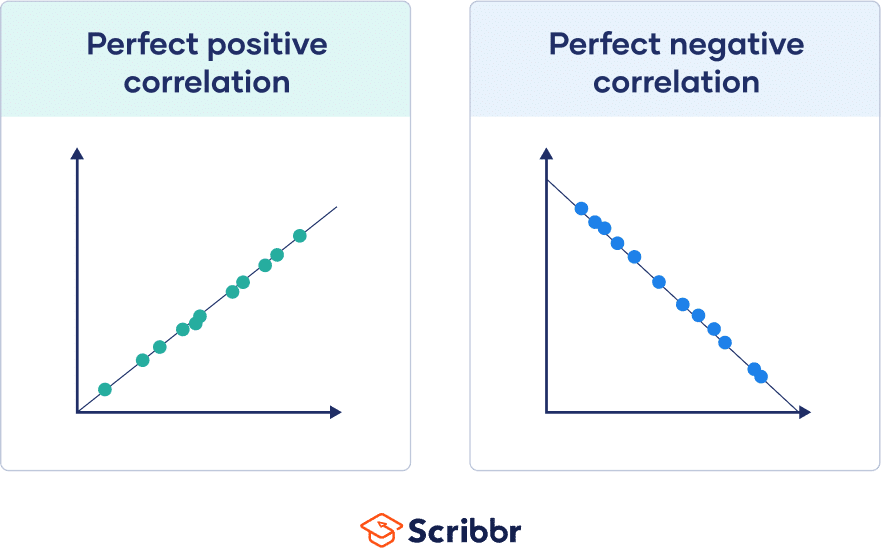
If all points are close to this line, the absolute value of your correlation coefficient is high .
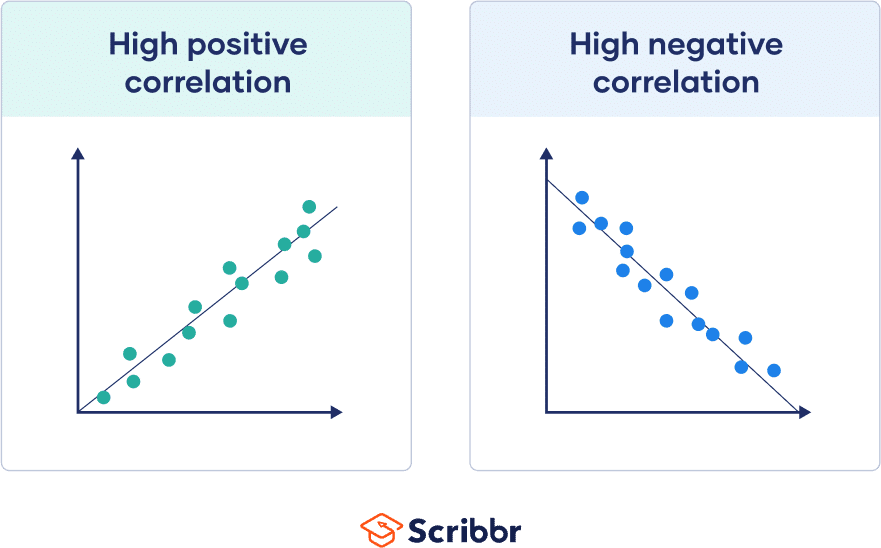
If these points are spread far from this line, the absolute value of your correlation coefficient is low .
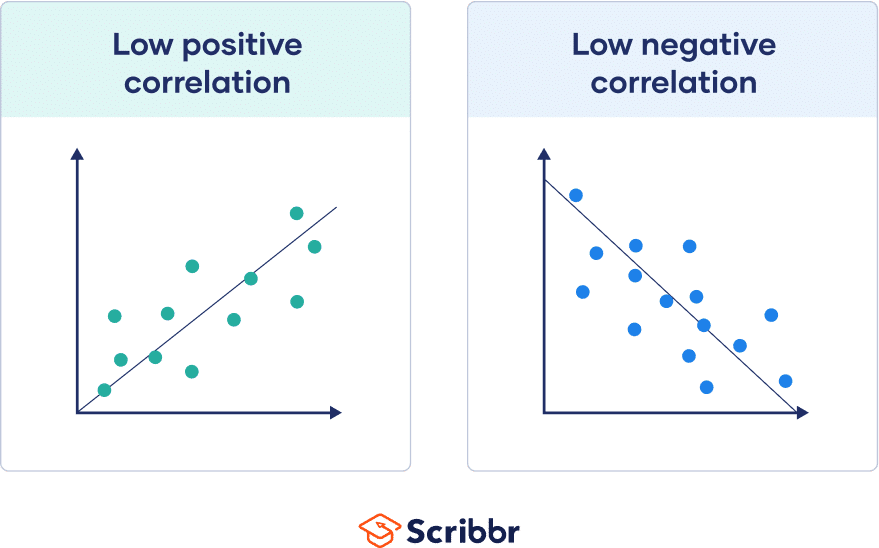
Note that the steepness or slope of the line isn’t related to the correlation coefficient value. The correlation coefficient doesn’t help you predict how much one variable will change based on a given change in the other, because two datasets with the same correlation coefficient value can have lines with very different slopes.
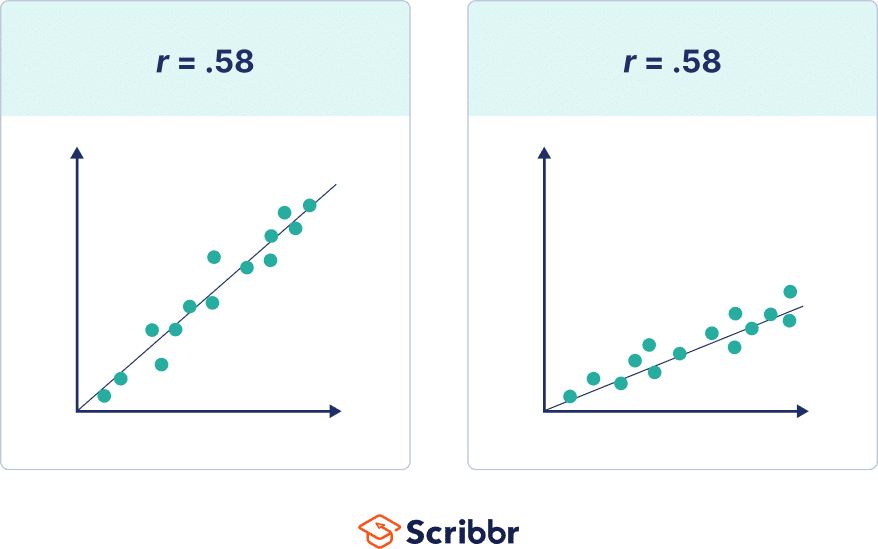
Prevent plagiarism. Run a free check.
You can choose from many different correlation coefficients based on the linearity of the relationship, the level of measurement of your variables, and the distribution of your data.
For high statistical power and accuracy, it’s best to use the correlation coefficient that’s most appropriate for your data.
The most commonly used correlation coefficient is Pearson’s r because it allows for strong inferences. It’s parametric and measures linear relationships. But if your data do not meet all assumptions for this test, you’ll need to use a non-parametric test instead.
Non-parametric tests of rank correlation coefficients summarize non-linear relationships between variables. The Spearman’s rho and Kendall’s tau have the same conditions for use, but Kendall’s tau is generally preferred for smaller samples whereas Spearman’s rho is more widely used.
The table below is a selection of commonly used correlation coefficients, and we’ll cover the two most widely used coefficients in detail in this article.
Correlation coefficient | Type of relationship | Levels of measurement | Data distribution |
---|---|---|---|
Pearson’s r | Linear | Two quantitative (interval or ratio) variables | Normal distribution |
Spearman’s rho | Non-linear | Two , interval or ratio variables | Any distribution |
Point-biserial | Linear | One dichotomous (binary) variable and one quantitative ( or ratio) variable | Normal distribution |
Cramér’s V (Cramér’s φ) | Non-linear | Two | Any distribution |
Kendall’s tau | Non-linear | Two ordinal, interval or | Any distribution |
The Pearson’s product-moment correlation coefficient, also known as Pearson’s r, describes the linear relationship between two quantitative variables.
These are the assumptions your data must meet if you want to use Pearson’s r:
- Both variables are on an interval or ratio level of measurement
- Data from both variables follow normal distributions
- Your data have no outliers
- Your data is from a random or representative sample
- You expect a linear relationship between the two variables
The Pearson’s r is a parametric test, so it has high power. But it’s not a good measure of correlation if your variables have a nonlinear relationship, or if your data have outliers, skewed distributions, or come from categorical variables. If any of these assumptions are violated, you should consider a rank correlation measure.
The formula for the Pearson’s r is complicated, but most computer programs can quickly churn out the correlation coefficient from your data. In a simpler form, the formula divides the covariance between the variables by the product of their standard deviations .
Formula | Explanation |
---|---|
| = strength of the correlation between variables x and y = sample size = sum of what follows… = every x-variable value = every y-variable value = the product of each x-variable score and the corresponding y-variable score |
Pearson sample vs population correlation coefficient formula
When using the Pearson correlation coefficient formula, you’ll need to consider whether you’re dealing with data from a sample or the whole population.
The sample and population formulas differ in their symbols and inputs. A sample correlation coefficient is called r , while a population correlation coefficient is called rho, the Greek letter ρ.
The sample correlation coefficient uses the sample covariance between variables and their sample standard deviations.
Sample correlation coefficient formula | Explanation |
---|---|
| = strength of the correlation between variables x and y ( , ) = covariance of x and y = sample standard deviation of x = sample standard deviation of y |
The population correlation coefficient uses the population covariance between variables and their population standard deviations.
Population correlation coefficient formula | Explanation |
---|---|
| = strength of the correlation between variables X and Y ( , ) = covariance of X and Y = population standard deviation of X = population standard deviation of Y |
Spearman’s rho, or Spearman’s rank correlation coefficient, is the most common alternative to Pearson’s r . It’s a rank correlation coefficient because it uses the rankings of data from each variable (e.g., from lowest to highest) rather than the raw data itself.
You should use Spearman’s rho when your data fail to meet the assumptions of Pearson’s r . This happens when at least one of your variables is on an ordinal level of measurement or when the data from one or both variables do not follow normal distributions.
While the Pearson correlation coefficient measures the linearity of relationships, the Spearman correlation coefficient measures the monotonicity of relationships.
In a linear relationship, each variable changes in one direction at the same rate throughout the data range. In a monotonic relationship, each variable also always changes in only one direction but not necessarily at the same rate.
- Positive monotonic: when one variable increases, the other also increases.
- Negative monotonic: when one variable increases, the other decreases.
Monotonic relationships are less restrictive than linear relationships.
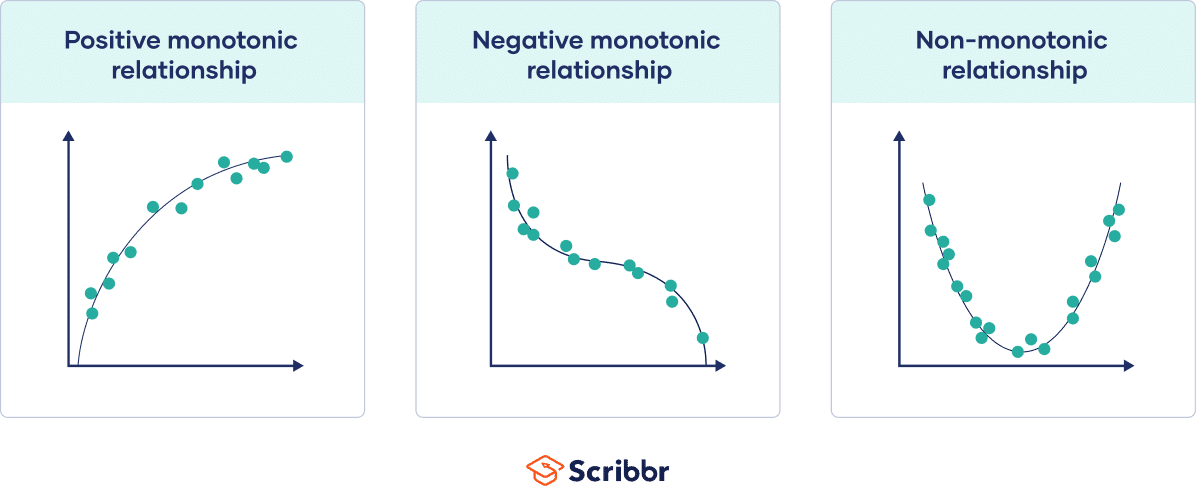
Spearman’s rank correlation coefficient formula
The symbols for Spearman’s rho are ρ for the population coefficient and r s for the sample coefficient. The formula calculates the Pearson’s r correlation coefficient between the rankings of the variable data.
To use this formula, you’ll first rank the data from each variable separately from low to high: every datapoint gets a rank from first, second, or third, etc.
Then, you’ll find the differences (d i ) between the ranks of your variables for each data pair and take that as the main input for the formula.
Spearman’s rank correlation coefficient formula | Explanation |
---|---|
| = strength of the rank correlation between variables = the difference between the x-variable rank and the y-variable rank for each pair of data = sum of the squared differences between x- and y-variable ranks = sample size |
If you have a correlation coefficient of 1, all of the rankings for each variable match up for every data pair. If you have a correlation coefficient of -1, the rankings for one variable are the exact opposite of the ranking of the other variable. A correlation coefficient near zero means that there’s no monotonic relationship between the variable rankings.
The correlation coefficient is related to two other coefficients, and these give you more information about the relationship between variables.
Coefficient of determination
When you square the correlation coefficient, you end up with the correlation of determination ( r 2 ). This is the proportion of common variance between the variables. The coefficient of determination is always between 0 and 1, and it’s often expressed as a percentage.
Coefficient of determination | Explanation |
---|---|
The correlation coefficient multiplied by itself |
The coefficient of determination is used in regression models to measure how much of the variance of one variable is explained by the variance of the other variable.
A regression analysis helps you find the equation for the line of best fit, and you can use it to predict the value of one variable given the value for the other variable.
A high r 2 means that a large amount of variability in one variable is determined by its relationship to the other variable. A low r 2 means that only a small portion of the variability of one variable is explained by its relationship to the other variable; relationships with other variables are more likely to account for the variance in the variable.
The correlation coefficient can often overestimate the relationship between variables, especially in small samples, so the coefficient of determination is often a better indicator of the relationship.
Coefficient of alienation
When you take away the coefficient of determination from unity (one), you’ll get the coefficient of alienation. This is the proportion of common variance not shared between the variables, the unexplained variance between the variables.
Coefficient of alienation | Explanation |
---|---|
1 – | One minus the coefficient of determination |
A high coefficient of alienation indicates that the two variables share very little variance in common. A low coefficient of alienation means that a large amount of variance is accounted for by the relationship between the variables.
If you want to know more about statistics , methodology , or research bias , make sure to check out some of our other articles with explanations and examples.
- Chi square test of independence
- Statistical power
- Descriptive statistics
- Degrees of freedom
- Pearson correlation
- Null hypothesis
Methodology
- Double-blind study
- Case-control study
- Research ethics
- Data collection
- Hypothesis testing
- Structured interviews
Research bias
- Hawthorne effect
- Unconscious bias
- Recall bias
- Halo effect
- Self-serving bias
- Information bias
A correlation reflects the strength and/or direction of the association between two or more variables.
- A positive correlation means that both variables change in the same direction.
- A negative correlation means that the variables change in opposite directions.
- A zero correlation means there’s no relationship between the variables.
A correlation is usually tested for two variables at a time, but you can test correlations between three or more variables.
A correlation coefficient is a single number that describes the strength and direction of the relationship between your variables.
Different types of correlation coefficients might be appropriate for your data based on their levels of measurement and distributions . The Pearson product-moment correlation coefficient (Pearson’s r ) is commonly used to assess a linear relationship between two quantitative variables.
These are the assumptions your data must meet if you want to use Pearson’s r :
Correlation coefficients always range between -1 and 1.
The sign of the coefficient tells you the direction of the relationship: a positive value means the variables change together in the same direction, while a negative value means they change together in opposite directions.
No, the steepness or slope of the line isn’t related to the correlation coefficient value. The correlation coefficient only tells you how closely your data fit on a line, so two datasets with the same correlation coefficient can have very different slopes.
To find the slope of the line, you’ll need to perform a regression analysis .
Cite this Scribbr article
If you want to cite this source, you can copy and paste the citation or click the “Cite this Scribbr article” button to automatically add the citation to our free Citation Generator.
Bhandari, P. (2023, June 22). Correlation Coefficient | Types, Formulas & Examples. Scribbr. Retrieved July 30, 2024, from https://www.scribbr.com/statistics/correlation-coefficient/
Is this article helpful?
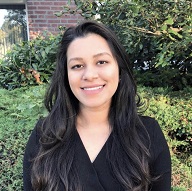
Pritha Bhandari
Other students also liked, correlational research | when & how to use, correlation vs. causation | difference, designs & examples, simple linear regression | an easy introduction & examples, what is your plagiarism score.
Pearson's Product-Moment Correlation using SPSS Statistics
Introduction.
The Pearson product-moment correlation coefficient (Pearson’s correlation, for short) is a measure of the strength and direction of association that exists between two variables measured on at least an interval scale.
For example, you could use a Pearson’s correlation to understand whether there is an association between exam performance and time spent revising. You could also use a Pearson's correlation to understand whether there is an association between depression and length of unemployment.
A Pearson’s correlation attempts to draw a line of best fit through the data of two variables, and the Pearson correlation coefficient, r , indicates how far away all these data points are from this line of best fit (i.e., how well the data points fit this model/line of best fit). You can learn more in our more general guide on Pearson's correlation , which we recommend if you are not familiar with this test.
Note: If one of your two variables is dichotomous you can use a point-biserial correlation instead, or if you have one or more control variables, you can run a Pearson's partial correlation .
This "quick start" guide shows you how to carry out a Pearson's correlation using SPSS Statistics, as well as interpret and report the results from this test. However, before we introduce you to this procedure, you need to understand the different assumptions that your data must meet in order for a Pearson's correlation to give you a valid result. We discuss these assumptions next.
SPSS Statistics
Assumptions.
When you choose to analyse your data using Pearson’s correlation, part of the process involves checking to make sure that the data you want to analyse can actually be analysed using Pearson’s correlation. You need to do this because it is only appropriate to use Pearson’s correlation if your data "passes" four assumptions that are required for Pearson’s correlation to give you a valid result. In practice, checking for these four assumptions just adds a little bit more time to your analysis, requiring you to click of few more buttons in SPSS Statistics when performing your analysis, as well as think a little bit more about your data, but it is not a difficult task.
Before we introduce you to these four assumptions, do not be surprised if, when analysing your own data using SPSS Statistics, one or more of these assumptions is violated (i.e., is not met). This is not uncommon when working with real-world data rather than textbook examples, which often only show you how to carry out Pearson’s correlation when everything goes well! However, don’t worry. Even when your data fails certain assumptions, there is often a solution to overcome this. First, let’s take a look at these four assumptions:
- Assumption #1: Your two variables should be measured at the interval or ratio level (i.e., they are continuous ). Examples of variables that meet this criterion include revision time (measured in hours), intelligence (measured using IQ score), exam performance (measured from 0 to 100), weight (measured in kg), and so forth. You can learn more about interval and ratio variables in our Types of Variable guide.

Note: Pearson's correlation determines the degree to which a relationship is linear. Put another way, it determines whether there is a linear component of association between two continuous variables. As such, linearity is not actually an assumption of Pearson's correlation. However, you would not normally want to pursue a Pearson's correlation to determine the strength and direction of a linear relationship when you already know the relationship between your two variables is not linear. Instead, the relationship between your two variables might be better described by another statistical measure. For this reason, it is not uncommon to view the relationship between your two variables in a scatterplot to see if running a Pearson's correlation is the best choice as a measure of association or whether another measure would be better.

- Assumption #4: Your variables should be approximately normally distributed . In order to assess the statistical significance of the Pearson correlation, you need to have bivariate normality, but this assumption is difficult to assess, so a simpler method is more commonly used. This simpler method involves determining the normality of each variable separately. To test for normality you can use the Shapiro-Wilk test of normality, which is easily tested for using SPSS Statistics. In addition to showing you how to do this in our enhanced Pearson’s correlation guide, we also explain what you can do if your data fails this assumption.
You can check assumptions #2, #3 and #4 using SPSS Statistics. Remember that if you do not test these assumptions correctly, the results you get when running a Pearson's correlation might not be valid. This is why we dedicate a number of sections of our enhanced Pearson's correlation guide to help you get this right. You can find out about our enhanced content on our Features: Overview page, or more specifically, learn how we help with testing assumptions on our Features: Assumptions page.
In the section, Test Procedure in SPSS Statistics , we illustrate the SPSS Statistics procedure to perform a Pearson’s correlation assuming that no assumptions have been violated. First, we set out the example we use to explain the Pearson’s correlation procedure in SPSS Statistics.
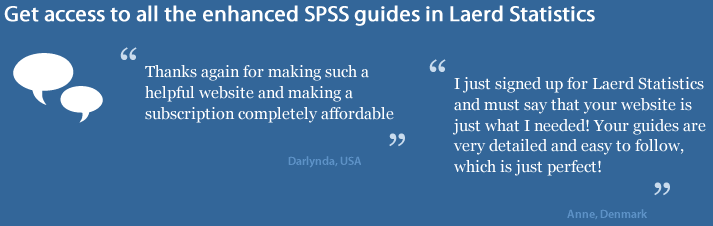
A researcher wants to know whether a person's height is related to how well they perform in a long jump. The researcher recruited untrained individuals from the general population, measured their height and had them perform a long jump. The researcher then investigated whether there was an association between height and long jump performance by running a Pearson's correlation.
Setup in SPSS Statistics
In SPSS Statistics, we created two variables so that we could enter our data: Height (i.e., participants' height) and Jump_Dist (i.e., distance jumped in a long jump). In our enhanced Pearson's correlation guide, we show you how to correctly enter data in SPSS Statistics to run a Pearson's correlation. You can learn about our enhanced data setup content on our Features: Data Setup page. Alternately, see our generic, "quick start" guide: Entering Data in SPSS Statistics .
Test Procedure in SPSS Statistics
The six steps below show you how to analyse your data using Pearson’s correlation in SPSS Statistics when none of the four assumptions in the Assumptions section have been violated. At the end of these six steps, we show you how to interpret the results from this test. If you are looking for help to make sure your data meets assumptions #2, #3 and #4, which are required when using Pearson’s correlations and can be tested using SPSS Statistics, you can learn more about our enhanced guides on our Features: Overview page.

Published with written permission from SPSS Statistics, IBM Corporation.

Note: If you study involves calculating more than one correlation and you want to carry out these correlations at the same time, we show you how to do this in our enhanced Pearson’s correlation guide. We also show you how to write up the results from multiple correlations.
- Make sure that the Pearso n checkbox is selected under the –Correlation Coefficients– area (although it is selected by default in SPSS Statistics).

Output for Pearson's correlation
SPSS Statistics generates a single Correlations table that contains the results of the Pearson’s correlation procedure that you ran in the previous section. If your data passed assumption #2 (linear relationship), assumption #3 (no outliers) and assumption #4 (normality), which we explained earlier in the Assumptions section, you will only need to interpret this one table. However, since you should have tested your data for these assumptions, you will also need to interpret the SPSS Statistics output that was produced when you tested for them (i.e., you will have to interpret: (a) the scatterplot you used to check for a linear relationship between your two variables; (b) the scatterplot that you used to assess whether there were any significant outliers; and (c) the output SPSS Statistics produced for your Shapiro-Wilk test of normality). If you do not know how to do this, we show you in our enhanced Pearson’s correlation guide. Remember that if your data failed any of these assumptions, the output that you get from the Pearson’s correlation procedure (i.e., the table we discuss below) will no longer be correct.
However, in this "quick start" guide, we focus on the results from the Pearson’s correlation procedure only, assuming that your data met all the relevant assumptions. Therefore, when running the Pearson’s correlation procedure, you will be presented with the Correlations table in the IBM SPSS Statistics Output Viewer . The Pearson's correlation result is highlighted below:

The results are presented in a matrix such that, as can be seen above, the correlations are replicated. Nevertheless, the table presents the Pearson correlation coefficient, its significance value and the sample size that the calculation is based on.
In this example, we can see that the Pearson correlation coefficient, r , is 0.706, and that it is statistically significant ( p = 0.005). For interpreting multiple correlations, see our enhanced Pearson’s guide.
Reporting the Output
In our example above, you might report the results as follows:
A Pearson product-moment correlation was run to determine the relationship between height and distance jumped in a long jump. There was a strong, positive correlation between height and distance jumped, which was statistically significant ( r = .706, n = 14, p = .005).
In our enhanced Pearson’s correlation guide, we also show you how to write up the results from your assumptions tests and Pearson’s correlation output if you need to report this in a dissertation, thesis, assignment or research report. We do this using the Harvard and APA styles. We also show you how to write up your results if you have performed multiple Pearson’s correlations. You can learn more about our enhanced content on our Features: Overview page.
- Research Process
- Manuscript Preparation
- Manuscript Review
- Publication Process
- Publication Recognition
- Language Editing Services
- Translation Services
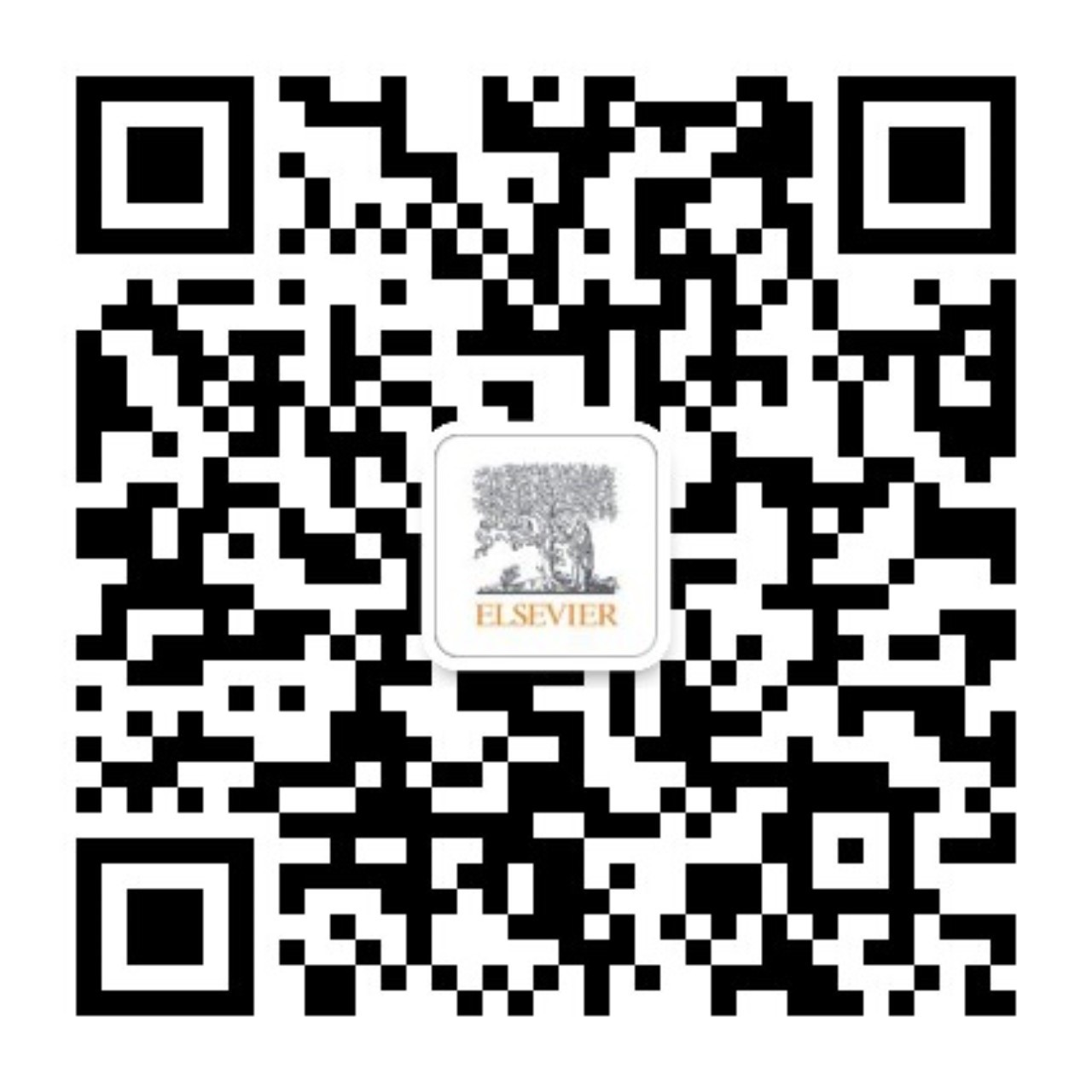
How to write the results section of a research paper
- 3 minute read
- 74.3K views
Table of Contents
At its core, a research paper aims to fill a gap in the research on a given topic. As a result, the results section of the paper, which describes the key findings of the study, is often considered the core of the paper. This is the section that gets the most attention from reviewers, peers, students, and any news organization reporting on your findings. Writing a clear, concise, and logical results section is, therefore, one of the most important parts of preparing your manuscript.
Difference between results and discussion
Before delving into how to write the results section, it is important to first understand the difference between the results and discussion sections. The results section needs to detail the findings of the study. The aim of this section is not to draw connections between the different findings or to compare it to previous findings in literature—that is the purview of the discussion section. Unlike the discussion section, which can touch upon the hypothetical, the results section needs to focus on the purely factual. In some cases, it may even be preferable to club these two sections together into a single section. For example, while writing a review article, it can be worthwhile to club these two sections together, as the main results in this case are the conclusions that can be drawn from the literature.
Structure of the results section
Although the main purpose of the results section in a research paper is to report the findings, it is necessary to present an introduction and repeat the research question. This establishes a connection to the previous section of the paper and creates a smooth flow of information.
Next, the results section needs to communicate the findings of your research in a systematic manner. The section needs to be organized such that the primary research question is addressed first, then the secondary research questions. If the research addresses multiple questions, the results section must individually connect with each of the questions. This ensures clarity and minimizes confusion while reading.
Consider representing your results visually. For example, graphs, tables, and other figures can help illustrate the findings of your paper, especially if there is a large amount of data in the results.
Remember, an appealing results section can help peer reviewers better understand the merits of your research, thereby increasing your chances of publication.
Practical guidance for writing an effective results section for a research paper
- Always use simple and clear language. Avoid the use of uncertain or out-of-focus expressions.
- The findings of the study must be expressed in an objective and unbiased manner. While it is acceptable to correlate certain findings in the discussion section, it is best to avoid overinterpreting the results.
- If the research addresses more than one hypothesis, use sub-sections to describe the results. This prevents confusion and promotes understanding.
- Ensure that negative results are included in this section, even if they do not support the research hypothesis.
- Wherever possible, use illustrations like tables, figures, charts, or other visual representations to showcase the results of your research paper. Mention these illustrations in the text, but do not repeat the information that they convey.
- For statistical data, it is adequate to highlight the tests and explain their results. The initial or raw data should not be mentioned in the results section of a research paper.
The results section of a research paper is usually the most impactful section because it draws the greatest attention. Regardless of the subject of your research paper, a well-written results section is capable of generating interest in your research.
For detailed information and assistance on writing the results of a research paper, refer to Elsevier Author Services.
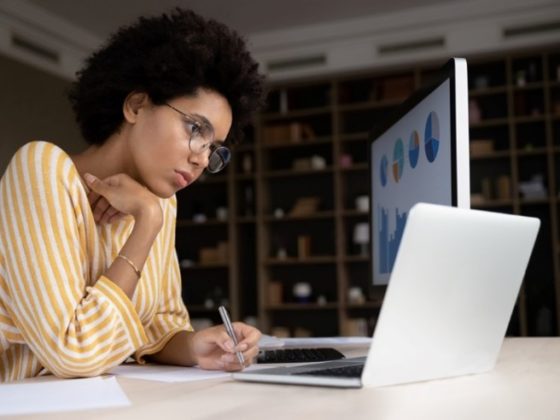
Writing a good review article
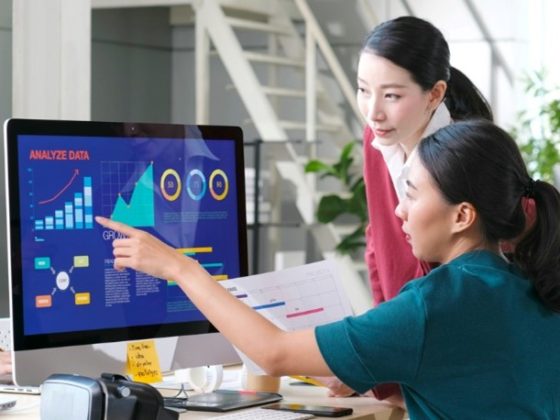
Why is data validation important in research?
You may also like.
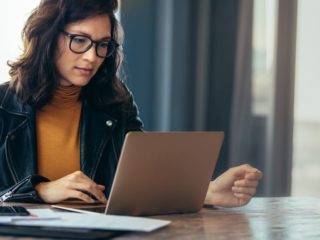
Submission 101: What format should be used for academic papers?
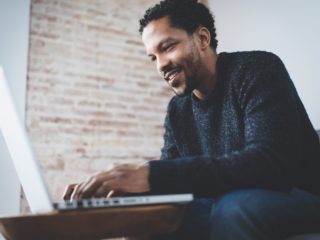
Page-Turner Articles are More Than Just Good Arguments: Be Mindful of Tone and Structure!
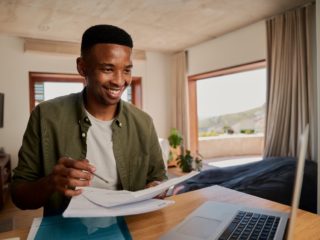
A Must-see for Researchers! How to Ensure Inclusivity in Your Scientific Writing
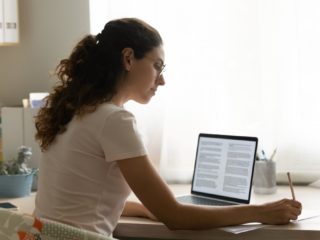
Make Hook, Line, and Sinker: The Art of Crafting Engaging Introductions
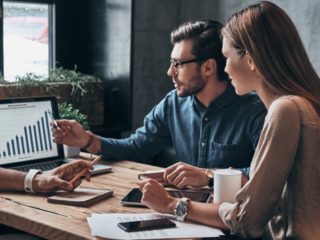
Can Describing Study Limitations Improve the Quality of Your Paper?
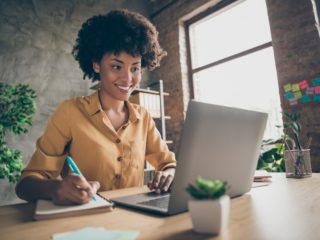
A Guide to Crafting Shorter, Impactful Sentences in Academic Writing
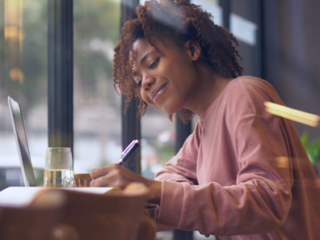
6 Steps to Write an Excellent Discussion in Your Manuscript
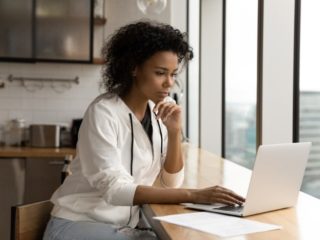
How to Write Clear and Crisp Civil Engineering Papers? Here are 5 Key Tips to Consider
Input your search keywords and press Enter.

Correlation Hypothesis
Ai generator.
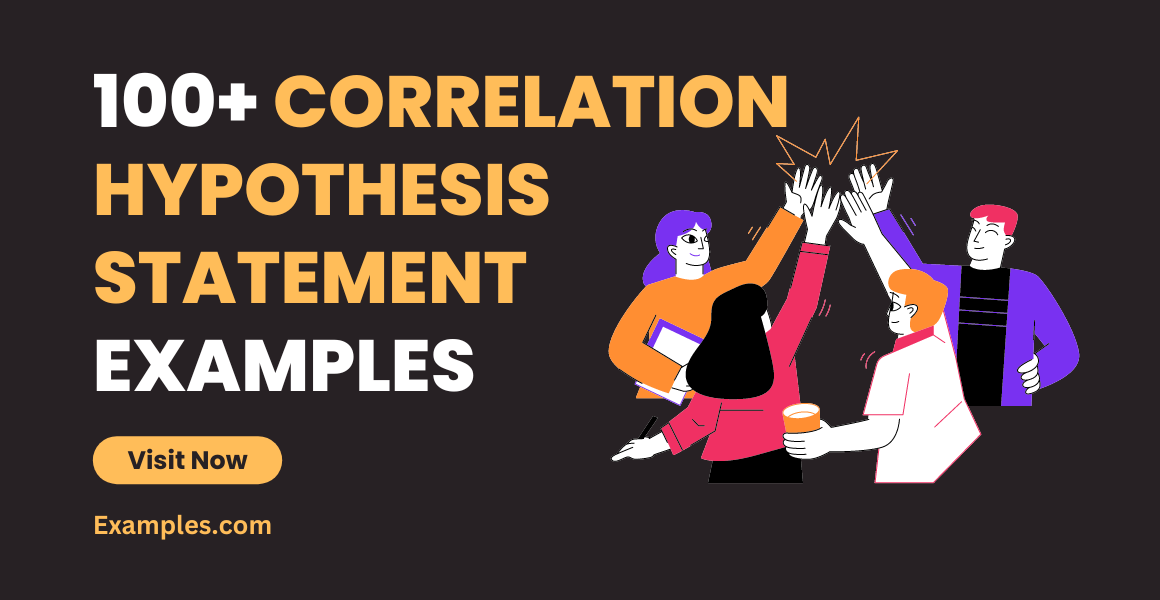
Understanding the relationships between variables is pivotal in research. Correlation hypotheses delve into the degree of association between two or more variables. In this guide, delve into an array of correlation hypothesis examples that explore connections, followed by a step-by-step tutorial on crafting these thesis statement hypothesis effectively. Enhance your research prowess with valuable tips tailored to unravel the intricate world of correlations.
What is Correlation Hypothesis?
A correlation hypothesis is a statement that predicts a specific relationship between two or more variables based on the assumption that changes in one variable are associated with changes in another variable. It suggests that there is a correlation or statistical relationship between the variables, meaning that when one variable changes, the other variable is likely to change in a consistent manner.
What is an example of a Correlation Hypothesis Statement?
Example: “If the amount of exercise increases, then the level of physical fitness will also increase.”
In this example, the correlation hypothesis suggests that there is a positive correlation between the amount of exercise a person engages in and their level of physical fitness. As exercise increases, the hypothesis predicts that physical fitness will increase as well. This hypothesis can be tested by collecting data on exercise levels and physical fitness levels and analyzing the relationship between the two variables using statistical methods.
100 Correlation Hypothesis Statement Examples
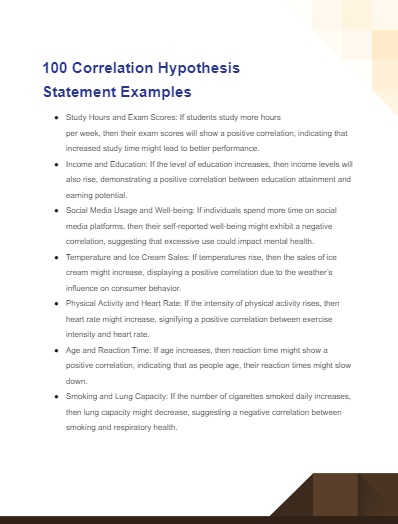
Size: 277 KB
Discover the intriguing world of correlation through a collection of examples that illustrate how variables can be linked in research. Explore diverse scenarios where changes in one variable may correspond to changes in another, forming the basis of correlation hypotheses. These real-world instances shed light on the essence of correlation analysis and its role in uncovering connections between different aspects of data.
- Study Hours and Exam Scores : If students study more hours per week, then their exam scores will show a positive correlation, indicating that increased study time might lead to better performance.
- Income and Education : If the level of education increases, then income levels will also rise, demonstrating a positive correlation between education attainment and earning potential.
- Social Media Usage and Well-being : If individuals spend more time on social media platforms, then their self-reported well-being might exhibit a negative correlation, suggesting that excessive use could impact mental health.
- Temperature and Ice Cream Sales : If temperatures rise, then the sales of ice cream might increase, displaying a positive correlation due to the weather’s influence on consumer behavior.
- Physical Activity and Heart Rate : If the intensity of physical activity rises, then heart rate might increase, signifying a positive correlation between exercise intensity and heart rate.
- Age and Reaction Time : If age increases, then reaction time might show a positive correlation, indicating that as people age, their reaction times might slow down.
- Smoking and Lung Capacity : If the number of cigarettes smoked daily increases, then lung capacity might decrease, suggesting a negative correlation between smoking and respiratory health.
- Stress and Sleep Quality : If stress levels elevate, then sleep quality might decline, reflecting a negative correlation between psychological stress and restorative sleep.
- Rainfall and Crop Yield : If the amount of rainfall decreases, then crop yield might also decrease, illustrating a negative correlation between precipitation and agricultural productivity.
- Screen Time and Academic Performance : If screen time usage increases among students, then academic performance might show a negative correlation, suggesting that excessive screen time could be detrimental to studies.
- Exercise and Body Weight : If individuals engage in regular exercise, then their body weight might exhibit a negative correlation, implying that physical activity can contribute to weight management.
- Income and Crime Rates : If income levels decrease in a neighborhood, then crime rates might show a positive correlation, indicating a potential link between socio-economic factors and crime.
- Social Support and Mental Health : If the level of social support increases, then individuals’ mental health scores may exhibit a positive correlation, highlighting the potential positive impact of strong social networks on psychological well-being.
- Study Time and GPA : If students spend more time studying, then their Grade Point Average (GPA) might display a positive correlation, suggesting that increased study efforts may lead to higher academic achievement.
- Parental Involvement and Academic Success : If parents are more involved in their child’s education, then the child’s academic success may show a positive correlation, emphasizing the role of parental support in shaping student outcomes.
- Alcohol Consumption and Reaction Time : If alcohol consumption increases, then reaction time might slow down, indicating a negative correlation between alcohol intake and cognitive performance.
- Social Media Engagement and Loneliness : If time spent on social media platforms increases, then feelings of loneliness might show a positive correlation, suggesting a potential connection between excessive online interaction and emotional well-being.
- Temperature and Insect Activity : If temperatures rise, then the activity of certain insects might increase, demonstrating a potential positive correlation between temperature and insect behavior.
- Education Level and Voting Participation : If education levels rise, then voter participation rates may also increase, showcasing a positive correlation between education and civic engagement.
- Work Commute Time and Job Satisfaction : If work commute time decreases, then job satisfaction might show a positive correlation, indicating that shorter commutes could contribute to higher job satisfaction.
- Sleep Duration and Cognitive Performance : If sleep duration increases, then cognitive performance scores might also rise, suggesting a potential positive correlation between adequate sleep and cognitive functioning.
- Healthcare Access and Mortality Rate : If access to healthcare services improves, then the mortality rate might decrease, highlighting a potential negative correlation between healthcare accessibility and mortality.
- Exercise and Blood Pressure : If individuals engage in regular exercise, then their blood pressure levels might exhibit a negative correlation, indicating that physical activity can contribute to maintaining healthy blood pressure.
- Social Media Use and Academic Distraction : If students spend more time on social media during study sessions, then their academic focus might show a negative correlation, suggesting that excessive online engagement can hinder concentration.
- Age and Technological Adaptation : If age increases, then the speed of adapting to new technologies might exhibit a negative correlation, suggesting that younger individuals tend to adapt more quickly.
- Temperature and Plant Growth : If temperatures rise, then the rate of plant growth might increase, indicating a potential positive correlation between temperature and biological processes.
- Music Exposure and Mood : If individuals listen to upbeat music, then their reported mood might show a positive correlation, suggesting that music can influence emotional states.
- Income and Healthcare Utilization : If income levels increase, then the frequency of healthcare utilization might decrease, suggesting a potential negative correlation between income and healthcare needs.
- Distance and Communication Frequency : If physical distance between individuals increases, then their communication frequency might show a negative correlation, indicating that proximity tends to facilitate communication.
- Study Group Attendance and Exam Scores : If students regularly attend study groups, then their exam scores might exhibit a positive correlation, suggesting that collaborative study efforts could enhance performance.
- Temperature and Disease Transmission : If temperatures rise, then the transmission of certain diseases might increase, pointing to a potential positive correlation between temperature and disease spread.
- Interest Rates and Consumer Spending : If interest rates decrease, then consumer spending might show a positive correlation, suggesting that lower interest rates encourage increased economic activity.
- Digital Device Use and Eye Strain : If individuals spend more time on digital devices, then the occurrence of eye strain might show a positive correlation, suggesting that prolonged screen time can impact eye health.
- Parental Education and Children’s Educational Attainment : If parents have higher levels of education, then their children’s educational attainment might display a positive correlation, highlighting the intergenerational impact of education.
- Social Interaction and Happiness : If individuals engage in frequent social interactions, then their reported happiness levels might show a positive correlation, indicating that social connections contribute to well-being.
- Temperature and Energy Consumption : If temperatures decrease, then energy consumption for heating might increase, suggesting a potential positive correlation between temperature and energy usage.
- Physical Activity and Stress Reduction : If individuals engage in regular physical activity, then their reported stress levels might display a negative correlation, indicating that exercise can help alleviate stress.
- Diet Quality and Chronic Diseases : If diet quality improves, then the prevalence of chronic diseases might decrease, suggesting a potential negative correlation between healthy eating habits and disease risk.
- Social Media Use and Body Image Dissatisfaction : If time spent on social media increases, then feelings of body image dissatisfaction might show a positive correlation, suggesting that online platforms can influence self-perception.
- Income and Access to Quality Education : If household income increases, then access to quality education for children might improve, suggesting a potential positive correlation between financial resources and educational opportunities.
- Workplace Diversity and Innovation : If workplace diversity increases, then the rate of innovation might show a positive correlation, indicating that diverse teams often generate more creative solutions.
- Physical Activity and Bone Density : If individuals engage in weight-bearing exercises, then their bone density might exhibit a positive correlation, suggesting that exercise contributes to bone health.
- Screen Time and Attention Span : If screen time increases, then attention span might show a negative correlation, indicating that excessive screen exposure can impact sustained focus.
- Social Support and Resilience : If individuals have strong social support networks, then their resilience levels might display a positive correlation, suggesting that social connections contribute to coping abilities.
- Weather Conditions and Mood : If sunny weather persists, then individuals’ reported mood might exhibit a positive correlation, reflecting the potential impact of weather on emotional states.
- Nutrition Education and Healthy Eating : If individuals receive nutrition education, then their consumption of fruits and vegetables might show a positive correlation, suggesting that knowledge influences dietary choices.
- Physical Activity and Cognitive Aging : If adults engage in regular physical activity, then their cognitive decline with aging might show a slower rate, indicating a potential negative correlation between exercise and cognitive aging.
- Air Quality and Respiratory Illnesses : If air quality deteriorates, then the incidence of respiratory illnesses might increase, suggesting a potential positive correlation between air pollutants and health impacts.
- Reading Habits and Vocabulary Growth : If individuals read regularly, then their vocabulary size might exhibit a positive correlation, suggesting that reading contributes to language development.
- Sleep Quality and Stress Levels : If sleep quality improves, then reported stress levels might display a negative correlation, indicating that sleep can impact psychological well-being.
- Social Media Engagement and Academic Performance : If students spend more time on social media, then their academic performance might exhibit a negative correlation, suggesting that excessive online engagement can impact studies.
- Exercise and Blood Sugar Levels : If individuals engage in regular exercise, then their blood sugar levels might display a negative correlation, indicating that physical activity can influence glucose regulation.
- Screen Time and Sleep Duration : If screen time before bedtime increases, then sleep duration might show a negative correlation, suggesting that screen exposure can affect sleep patterns.
- Environmental Pollution and Health Outcomes : If exposure to environmental pollutants increases, then the occurrence of health issues might show a positive correlation, suggesting that pollution can impact well-being.
- Time Management and Academic Achievement : If students improve time management skills, then their academic achievement might exhibit a positive correlation, indicating that effective planning contributes to success.
- Physical Fitness and Heart Health : If individuals improve their physical fitness, then their heart health indicators might display a positive correlation, indicating that exercise benefits cardiovascular well-being.
- Weather Conditions and Outdoor Activities : If weather is sunny, then outdoor activities might show a positive correlation, suggesting that favorable weather encourages outdoor engagement.
- Media Exposure and Body Image Perception : If exposure to media images increases, then body image dissatisfaction might show a positive correlation, indicating media’s potential influence on self-perception.
- Community Engagement and Civic Participation : If individuals engage in community activities, then their civic participation might exhibit a positive correlation, indicating an active citizenry.
- Social Media Use and Productivity : If individuals spend more time on social media, then their productivity levels might exhibit a negative correlation, suggesting that online distractions can affect work efficiency.
- Income and Stress Levels : If income levels increase, then reported stress levels might exhibit a negative correlation, suggesting that financial stability can impact psychological well-being.
- Social Media Use and Interpersonal Skills : If individuals spend more time on social media, then their interpersonal skills might show a negative correlation, indicating potential effects on face-to-face interactions.
- Parental Involvement and Academic Motivation : If parents are more involved in their child’s education, then the child’s academic motivation may exhibit a positive correlation, highlighting the role of parental support.
- Technology Use and Sleep Quality : If screen time increases before bedtime, then sleep quality might show a negative correlation, suggesting that technology use can impact sleep.
- Outdoor Activity and Mood Enhancement : If individuals engage in outdoor activities, then their reported mood might display a positive correlation, suggesting the potential emotional benefits of nature exposure.
- Income Inequality and Social Mobility : If income inequality increases, then social mobility might exhibit a negative correlation, suggesting that higher inequality can hinder upward mobility.
- Vegetable Consumption and Heart Health : If individuals increase their vegetable consumption, then heart health indicators might show a positive correlation, indicating the potential benefits of a nutritious diet.
- Online Learning and Academic Achievement : If students engage in online learning, then their academic achievement might display a positive correlation, highlighting the effectiveness of digital education.
- Emotional Intelligence and Workplace Performance : If emotional intelligence improves, then workplace performance might exhibit a positive correlation, indicating the relevance of emotional skills.
- Community Engagement and Mental Well-being : If individuals engage in community activities, then their reported mental well-being might show a positive correlation, emphasizing social connections’ impact.
- Rainfall and Agriculture Productivity : If rainfall levels increase, then agricultural productivity might exhibit a positive correlation, indicating the importance of water for crops.
- Social Media Use and Body Posture : If screen time increases, then poor body posture might show a positive correlation, suggesting that screen use can influence physical habits.
- Marital Satisfaction and Relationship Length : If marital satisfaction decreases, then relationship length might show a negative correlation, indicating potential challenges over time.
- Exercise and Anxiety Levels : If individuals engage in regular exercise, then reported anxiety levels might exhibit a negative correlation, indicating the potential benefits of physical activity on mental health.
- Music Listening and Concentration : If individuals listen to instrumental music, then their concentration levels might display a positive correlation, suggesting music’s impact on focus.
- Internet Usage and Attention Deficits : If screen time increases, then attention deficits might show a positive correlation, implying that excessive internet use can affect concentration.
- Financial Literacy and Debt Levels : If financial literacy improves, then personal debt levels might exhibit a negative correlation, suggesting better financial decision-making.
- Time Spent Outdoors and Vitamin D Levels : If time spent outdoors increases, then vitamin D levels might show a positive correlation, indicating sun exposure’s role in vitamin synthesis.
- Family Meal Frequency and Nutrition : If families eat meals together frequently, then nutrition quality might display a positive correlation, emphasizing family dining’s impact on health.
- Temperature and Allergy Symptoms : If temperatures rise, then allergy symptoms might increase, suggesting a potential positive correlation between temperature and allergen exposure.
- Social Media Use and Academic Distraction : If students spend more time on social media, then their academic focus might exhibit a negative correlation, indicating that online engagement can hinder studies.
- Financial Stress and Health Outcomes : If financial stress increases, then the occurrence of health issues might show a positive correlation, suggesting potential health impacts of economic strain.
- Study Hours and Test Anxiety : If students study more hours, then test anxiety might show a negative correlation, suggesting that increased preparation can reduce anxiety.
- Music Tempo and Exercise Intensity : If music tempo increases, then exercise intensity might display a positive correlation, indicating music’s potential to influence workout vigor.
- Green Space Accessibility and Stress Reduction : If access to green spaces improves, then reported stress levels might exhibit a negative correlation, highlighting nature’s stress-reducing effects.
- Parenting Style and Child Behavior : If authoritative parenting increases, then positive child behaviors might display a positive correlation, suggesting parenting’s influence on behavior.
- Sleep Quality and Productivity : If sleep quality improves, then work productivity might show a positive correlation, emphasizing the connection between rest and efficiency.
- Media Consumption and Political Beliefs : If media consumption increases, then alignment with specific political beliefs might exhibit a positive correlation, suggesting media’s influence on ideology.
- Workplace Satisfaction and Employee Retention : If workplace satisfaction increases, then employee retention rates might show a positive correlation, indicating the link between job satisfaction and tenure.
- Digital Device Use and Eye Discomfort : If screen time increases, then reported eye discomfort might show a positive correlation, indicating potential impacts of screen exposure.
- Age and Adaptability to Technology : If age increases, then adaptability to new technologies might exhibit a negative correlation, indicating generational differences in tech adoption.
- Physical Activity and Mental Health : If individuals engage in regular physical activity, then reported mental health scores might exhibit a positive correlation, showcasing exercise’s impact.
- Video Gaming and Attention Span : If time spent on video games increases, then attention span might display a negative correlation, indicating potential effects on focus.
- Social Media Use and Empathy Levels : If social media use increases, then reported empathy levels might show a negative correlation, suggesting possible effects on emotional understanding.
- Reading Habits and Creativity : If individuals read diverse genres, then their creative thinking might exhibit a positive correlation, emphasizing reading’s cognitive benefits.
- Weather Conditions and Outdoor Exercise : If weather is pleasant, then outdoor exercise might show a positive correlation, suggesting weather’s influence on physical activity.
- Parental Involvement and Bullying Prevention : If parents are actively involved, then instances of bullying might exhibit a negative correlation, emphasizing parental impact on behavior.
- Digital Device Use and Sleep Disruption : If screen time before bedtime increases, then sleep disruption might show a positive correlation, indicating technology’s influence on sleep.
- Friendship Quality and Psychological Well-being : If friendship quality increases, then reported psychological well-being might show a positive correlation, highlighting social support’s impact.
- Income and Environmental Consciousness : If income levels increase, then environmental consciousness might also rise, indicating potential links between affluence and sustainability awareness.
Correlational Hypothesis Interpretation Statement Examples
Explore the art of interpreting correlation hypotheses with these illustrative examples. Understand the implications of positive, negative, and zero correlations, and learn how to deduce meaningful insights from data relationships.
- Relationship Between Exercise and Mood : A positive correlation between exercise frequency and mood scores suggests that increased physical activity might contribute to enhanced emotional well-being.
- Association Between Screen Time and Sleep Quality : A negative correlation between screen time before bedtime and sleep quality indicates that higher screen exposure could lead to poorer sleep outcomes.
- Connection Between Study Hours and Exam Performance : A positive correlation between study hours and exam scores implies that increased study time might correspond to better academic results.
- Link Between Stress Levels and Meditation Practice : A negative correlation between stress levels and meditation frequency suggests that engaging in meditation could be associated with lower perceived stress.
- Relationship Between Social Media Use and Loneliness : A positive correlation between social media engagement and feelings of loneliness implies that excessive online interaction might contribute to increased loneliness.
- Association Between Income and Happiness : A positive correlation between income and self-reported happiness indicates that higher income levels might be linked to greater subjective well-being.
- Connection Between Parental Involvement and Academic Performance : A positive correlation between parental involvement and students’ grades suggests that active parental engagement might contribute to better academic outcomes.
- Link Between Time Management and Stress Levels : A negative correlation between effective time management and reported stress levels implies that better time management skills could lead to lower stress.
- Relationship Between Outdoor Activities and Vitamin D Levels : A positive correlation between time spent outdoors and vitamin D levels suggests that increased outdoor engagement might be associated with higher vitamin D concentrations.
- Association Between Water Consumption and Skin Hydration : A positive correlation between water intake and skin hydration indicates that higher fluid consumption might lead to improved skin moisture levels.
Alternative Correlational Hypothesis Statement Examples
Explore alternative scenarios and potential correlations in these examples. Learn to articulate different hypotheses that could explain data relationships beyond the conventional assumptions.
- Alternative to Exercise and Mood : An alternative hypothesis could suggest a non-linear relationship between exercise and mood, indicating that moderate exercise might have the most positive impact on emotional well-being.
- Alternative to Screen Time and Sleep Quality : An alternative hypothesis might propose that screen time has a curvilinear relationship with sleep quality, suggesting that moderate screen exposure leads to optimal sleep outcomes.
- Alternative to Study Hours and Exam Performance : An alternative hypothesis could propose that there’s an interaction effect between study hours and study method, influencing the relationship between study time and exam scores.
- Alternative to Stress Levels and Meditation Practice : An alternative hypothesis might consider that the relationship between stress levels and meditation practice is moderated by personality traits, resulting in varying effects.
- Alternative to Social Media Use and Loneliness : An alternative hypothesis could posit that the relationship between social media use and loneliness depends on the quality of online interactions and content consumption.
- Alternative to Income and Happiness : An alternative hypothesis might propose that the relationship between income and happiness differs based on cultural factors, leading to varying happiness levels at different income ranges.
- Alternative to Parental Involvement and Academic Performance : An alternative hypothesis could suggest that the relationship between parental involvement and academic performance varies based on students’ learning styles and preferences.
- Alternative to Time Management and Stress Levels : An alternative hypothesis might explore the possibility of a curvilinear relationship between time management and stress levels, indicating that extreme time management efforts might elevate stress.
- Alternative to Outdoor Activities and Vitamin D Levels : An alternative hypothesis could consider that the relationship between outdoor activities and vitamin D levels is moderated by sunscreen usage, influencing vitamin synthesis.
- Alternative to Water Consumption and Skin Hydration : An alternative hypothesis might propose that the relationship between water consumption and skin hydration is mediated by dietary factors, influencing fluid retention and skin health.
Correlational Hypothesis Pearson Interpretation Statement Examples
Discover how the Pearson correlation coefficient enhances your understanding of data relationships with these examples. Learn to interpret correlation strength and direction using this valuable statistical measure.
- Strong Positive Correlation : A Pearson correlation coefficient of +0.85 between study time and exam scores indicates a strong positive relationship, suggesting that increased study time is strongly associated with higher grades.
- Moderate Negative Correlation : A Pearson correlation coefficient of -0.45 between screen time and sleep quality reflects a moderate negative correlation, implying that higher screen exposure is moderately linked to poorer sleep outcomes.
- Weak Positive Correlation : A Pearson correlation coefficient of +0.25 between social media use and loneliness suggests a weak positive correlation, indicating that increased online engagement is weakly related to higher loneliness.
- Strong Negative Correlation : A Pearson correlation coefficient of -0.75 between stress levels and meditation practice indicates a strong negative relationship, implying that engaging in meditation is strongly associated with lower stress.
- Moderate Positive Correlation : A Pearson correlation coefficient of +0.60 between income and happiness signifies a moderate positive correlation, suggesting that higher income is moderately linked to greater happiness.
- Weak Negative Correlation : A Pearson correlation coefficient of -0.30 between parental involvement and academic performance represents a weak negative correlation, indicating that higher parental involvement is weakly associated with lower academic performance.
- Strong Positive Correlation : A Pearson correlation coefficient of +0.80 between time management and stress levels reveals a strong positive relationship, suggesting that effective time management is strongly linked to lower stress.
- Weak Negative Correlation : A Pearson correlation coefficient of -0.20 between outdoor activities and vitamin D levels signifies a weak negative correlation, implying that higher outdoor engagement is weakly related to lower vitamin D levels.
- Moderate Positive Correlation : A Pearson correlation coefficient of +0.50 between water consumption and skin hydration denotes a moderate positive correlation, suggesting that increased fluid intake is moderately linked to better skin hydration.
- Strong Negative Correlation : A Pearson correlation coefficient of -0.70 between screen time and attention span indicates a strong negative relationship, implying that higher screen exposure is strongly associated with shorter attention spans.
Correlational Hypothesis Statement Examples in Psychology
Explore how correlation hypotheses apply to psychological research with these examples. Understand how psychologists investigate relationships between variables to gain insights into human behavior.
- Sleep Patterns and Cognitive Performance : There is a positive correlation between consistent sleep patterns and cognitive performance, suggesting that individuals with regular sleep schedules exhibit better cognitive functioning.
- Anxiety Levels and Social Media Use : There is a positive correlation between anxiety levels and excessive social media use, indicating that individuals who spend more time on social media might experience higher anxiety.
- Self-Esteem and Body Image Satisfaction : There is a negative correlation between self-esteem and body image satisfaction, implying that individuals with higher self-esteem tend to be more satisfied with their physical appearance.
- Parenting Styles and Child Aggression : There is a negative correlation between authoritative parenting styles and child aggression, suggesting that children raised by authoritative parents might exhibit lower levels of aggression.
- Emotional Intelligence and Conflict Resolution : There is a positive correlation between emotional intelligence and effective conflict resolution, indicating that individuals with higher emotional intelligence tend to resolve conflicts more successfully.
- Personality Traits and Career Satisfaction : There is a positive correlation between certain personality traits (e.g., extraversion, openness) and career satisfaction, suggesting that individuals with specific traits experience higher job contentment.
- Stress Levels and Coping Mechanisms : There is a negative correlation between stress levels and adaptive coping mechanisms, indicating that individuals with lower stress levels are more likely to employ effective coping strategies.
- Attachment Styles and Romantic Relationship Quality : There is a positive correlation between secure attachment styles and higher romantic relationship quality, suggesting that individuals with secure attachments tend to have healthier relationships.
- Social Support and Mental Health : There is a negative correlation between perceived social support and mental health issues, indicating that individuals with strong social support networks tend to experience fewer mental health challenges.
- Motivation and Academic Achievement : There is a positive correlation between intrinsic motivation and academic achievement, implying that students who are internally motivated tend to perform better academically.
Does Correlational Research Have Hypothesis?
Correlational research involves examining the relationship between two or more variables to determine whether they are related and how they change together. While correlational studies do not establish causation, they still utilize hypotheses to formulate expectations about the relationships between variables. These good hypotheses predict the presence, direction, and strength of correlations. However, in correlational research, the focus is on measuring and analyzing the degree of association rather than establishing cause-and-effect relationships.
How Do You Write a Null-Hypothesis for a Correlational Study?
The null hypothesis in a correlational study states that there is no significant correlation between the variables being studied. It assumes that any observed correlation is due to chance and lacks meaningful association. When writing a null hypothesis for a correlational study, follow these steps:
- Identify the Variables: Clearly define the variables you are studying and their relationship (e.g., “There is no significant correlation between X and Y”).
- Specify the Population: Indicate the population from which the data is drawn (e.g., “In the population of [target population]…”).
- Include the Direction of Correlation: If relevant, specify the direction of correlation (positive, negative, or zero) that you are testing (e.g., “…there is no significant positive/negative correlation…”).
- State the Hypothesis: Write the null hypothesis as a clear statement that there is no significant correlation between the variables (e.g., “…there is no significant correlation between X and Y”).
What Is Correlation Hypothesis Formula?
The correlation hypothesis is often expressed in the form of a statement that predicts the presence and nature of a relationship between two variables. It typically follows the “If-Then” structure, indicating the expected change in one variable based on changes in another. The correlation hypothesis formula can be written as:
“If [Variable X] changes, then [Variable Y] will also change [in a specified direction] because [rationale for the expected correlation].”
For example, “If the amount of exercise increases, then mood scores will improve because physical activity has been linked to better emotional well-being.”
What Is a Correlational Hypothesis in Research Methodology?
A correlational hypothesis in research methodology is a testable hypothesis statement that predicts the presence and nature of a relationship between two or more variables. It forms the basis for conducting a correlational study, where the goal is to measure and analyze the degree of association between variables. Correlational hypotheses are essential in guiding the research process, collecting relevant data, and assessing whether the observed correlations are statistically significant.
How Do You Write a Hypothesis for Correlation? – A Step by Step Guide
Writing a hypothesis for correlation involves crafting a clear and testable statement about the expected relationship between variables. Here’s a step-by-step guide:
- Identify Variables : Clearly define the variables you are studying and their nature (e.g., “There is a relationship between X and Y…”).
- Specify Direction : Indicate the expected direction of correlation (positive, negative, or zero) based on your understanding of the variables and existing literature.
- Formulate the If-Then Statement : Write an “If-Then” statement that predicts the change in one variable based on changes in the other variable (e.g., “If [Variable X] changes, then [Variable Y] will also change [in a specified direction]…”).
- Provide Rationale : Explain why you expect the correlation to exist, referencing existing theories, research, or logical reasoning.
- Quantitative Prediction (Optional) : If applicable, provide a quantitative prediction about the strength of the correlation (e.g., “…for every one unit increase in [Variable X], [Variable Y] is predicted to increase by [numerical value].”).
- Specify Population : Indicate the population to which your hypothesis applies (e.g., “In a sample of [target population]…”).
Tips for Writing Correlational Hypothesis
- Base on Existing Knowledge : Ground your hypothesis in existing literature, theories, or empirical evidence to ensure it’s well-informed.
- Be Specific : Clearly define the variables and direction of correlation you’re predicting to avoid ambiguity.
- Avoid Causation Claims : Remember that correlational hypotheses do not imply causation. Focus on predicting relationships, not causes.
- Use Clear Language : Write in clear and concise language, avoiding jargon that may confuse readers.
- Consider Alternative Explanations : Acknowledge potential confounding variables or alternative explanations that could affect the observed correlation.
- Be Open to Results : Correlation results can be unexpected. Be prepared to interpret findings even if they don’t align with your initial hypothesis.
- Test Statistically : Once you collect data, use appropriate statistical tests to determine if the observed correlation is statistically significant.
- Revise as Needed : If your findings don’t support your hypothesis, revise it based on the data and insights gained.
Crafting a well-structured correlational hypothesis is crucial for guiding your research, conducting meaningful analysis, and contributing to the understanding of relationships between variables.
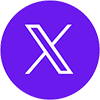
Text prompt
- Instructive
- Professional
10 Examples of Public speaking
20 Examples of Gas lighting
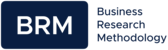
Correlation Analysis
Methods of correlation and regression can be used in order to analyze the extent and the nature of relationships between different variables. Correlation analysis is used to understand the nature of relationships between two individual variables. For example, if we aim to study the impact of foreign direct investment (FDI) on the level of economic growth in Vietnam, then two variables can be specified as the amounts of FDI and GDP for the same period.
Correlation coefficient ‘r’ is calculated through the following formula:
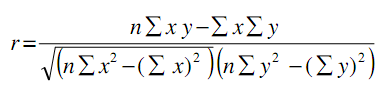
Where, x and y are values of variables, and n is size of the sample.
The value of correlation coefficient can be interpreted in the following manner:
If ‘r’ is equal to 1, then there is perfect positive correlation between two values;
If ‘r’ is equal to -1, then there is perfect negative correlation between two values;
If ‘r’ is equal to zero, then there is no correlation between the two values.
In practical terms, the closer the value of ‘r’ to 1, the higher positive impact of FDI on GDP growth in Vietnam. Similarly, if the value of ‘r’ is less than 0, the closer it is to – 1, the greater the negative impact of FDI on GDP growth in Vietnam. If ‘r’ is equal to zero, then FDI is perceived to have no impact on GDP change in Vietnama within the given sample.
The most popular forms of correlation analysis used in business studies include Pearson product-moment correlation , Spearman Rank correlation and Autocorrelation.
The Pearson product-moment correlation is calculated by taking the ratio of the sample of the two variables to the product of the two standard deviations and illustrates the strength of linear relationships. In Pearson product-moment correlation the correlation coefficient is not robust due to the fact that strong linear relationships between the variables are not recognized. The correlation coefficient is sensitive to outlying points therefore the correlation coefficient is not resistant.
Spearman Rank correlation requires the data to be sorted and the value to be assigned a specific rank with 1 to be assigned as the lowest value. Moreover, in case of data value appearing more than once, equal values will be specified their average rank.
Autocorrelation (serial correlation) implies the correlation among the values of the same variables but at various times. Autocorrelation coefficient is calculated by changing lagged data with the formula for the Pearson product-moment correlation coefficient. Also, because a series of unshifted data will express perfect correlation, the function begins with the coefficient of 1.
Correlation coefficient ‘r’ illustrated above is just a mathematical formula and you don’t have to calculate correlation coefficient manually. For a bachelor’s degree dissertation most supervisors accept correlation tests that have been run on a simple Excel spreadsheet. For master’s or PhD level studies, on the other hand, you will have to use more advanced statistical software such as SPSS or NCSS for your correlation analysis.
Correlation analysis as a research method offers a range of advantages. This method allows data analysis from many subjects simultaneously. Moreover, correlation analysis can study a wide range of variables and their interrelations. On the negative side, findings of correlation does not indicate causations i.e. cause and effect relationships.
My e-book, The Ultimate Guide to Writing a Dissertation in Business Studies: a step by step assistance offers practical assistance to complete a dissertation with minimum or no stress. The e-book covers all stages of writing a dissertation starting from the selection to the research area to submitting the completed version of the work within the deadline. John Dudovskiy
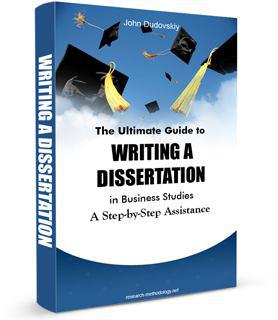
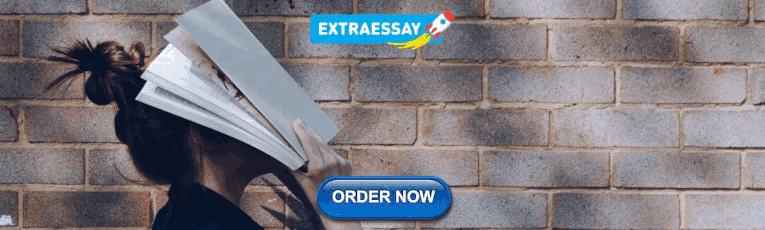
IMAGES
VIDEO
COMMENTS
There was a [negative or positive] correlation between the two variables, r (df) = [r value], p = [p-value]. Keep in mind the following when reporting Pearson's r in APA format: Round the p-value to three decimal places. Round the value for r to two decimal places. Drop the leading 0 for the p-value and r (e.g. use .77, not 0.77)
Here are a few best practices: Your results should always be written in the past tense. While the length of this section depends on how much data you collected and analyzed, it should be written as concisely as possible. Only include results that are directly relevant to answering your research questions.
Report value of Pearson's r to provide an understanding of the strength and direction of the relationship between the two variables. Also report whether the relationship is significant. Example: "There was a weak, positive correlation between the two variables, r = .047, N = 21; however, the relationship was not significant (p = .839).". 3.
It is a number between -1 and 1 that measures the strength and direction of the relationship between two variables. Pearson correlation coefficient ( r) Correlation type. Interpretation. Example. Between 0 and 1. Positive correlation. When one variable changes, the other variable changes in the same direction. Baby length & weight:
This video explains how to do the write up of a correlation in the results section of a thesis.
The results chapter (also referred to as the findings or analysis chapter) is one of the most important chapters of your dissertation or thesis because it shows the reader what you've found in terms of the quantitative data you've collected. It presents the data using a clear text narrative, supported by tables, graphs and charts.
1. There are two ways to report p values. The first way is to cite the alpha value as in the second example above. The second way, very much the preferred way in the age of computer aided calculations (and the way recommended by the APA), is to report the exact p value (as in our main example). If you report the exact p value, then you need to ...
A well-structured results section enables readers to understand the progression of your experiments and the relationship between different findings. Begin by reminding readers of the research questions or hypotheses that guided your study. This alignment helps establish a clear connection between the objectives of your research and the ...
Revised on June 22, 2023. A correlational research design investigates relationships between variables without the researcher controlling or manipulating any of them. A correlation reflects the strength and/or direction of the relationship between two (or more) variables. The direction of a correlation can be either positive or negative.
Defining Correlation Coefficients. The value of the correlation coefficient details how strong the relationship between those variables is. A value of -1 or +1 is a perfect correlation, meaning that the relationship between the variables is perfectly linear. The closer the value gets to 0, the less linear the relationship is.
For each correlation, you need to report the following information either in the text of your paper or in a table: correlation coefficient, significance level (p value). If you are reporting a single correlation for the whole results section, report it in the text of the paper as follows: r =.26, p < .01 or r = -.11, n.s.
Include all relevant results as text, tables, or figures. Report the results of subject recruitment and data collection. For qualitative research, present the data from all statistical analyses, whether or not the results are significant. For quantitative research, present the data by coding or categorizing themes and topics.
Table of contents. What not to include in your discussion section. Step 1: Summarise your key findings. Step 2: Give your interpretations. Step 3: Discuss the implications. Step 4: Acknowledge the limitations. Step 5: Share your recommendations. Discussion section example.
For example, you may have noticed an unusual correlation between two variables during the analysis of your findings. It is appropriate to highlight this finding in the results section. ... Department of Biology. Bates College; Schafer, Mickey S. Writing the Results. Thesis Writing in the Sciences. Course Syllabus. University of Florida.
Here are a few best practices: Your results should always be written in the past tense. While the length of this section depends on how much data you collected and analysed, it should be written as concisely as possible. Only include results that are directly relevant to answering your research questions.
PICTURE 2. Results of ANOVA for regression: Now you need to report the value of R 2 (see PICTURE 3), which tells you the degree to which your model predicted self-esteem scores. You need to multiply this value by 100 to get a percentage. Thus, if your R 2 value is .335, the percentage becomes 33.5%.
Self-concept results Correlations were computed among five self-concept scales on data for 80 men. The results suggest that 7 out of 10 correlations were statistically significant and were greater or equal to r(78) = +.35, p < .05, two-tailed. The correlations of competence rating of scholarly knowledge with other self-concept measures were not significant, with the
Address the primary and secondary research questions by reporting the detailed results of your main analyses. Results of subgroup or exploratory analyses, if applicable. Place detailed results in supplementary materials. Write up the results in the past tense because you're describing the outcomes of a completed research study.
What does a correlation coefficient tell you? Correlation coefficients summarize data and help you compare results between studies.. Summarizing data. A correlation coefficient is a descriptive statistic.That means that it summarizes sample data without letting you infer anything about the population. A correlation coefficient is a bivariate statistic when it summarizes the relationship ...
In our enhanced Pearson's correlation guide, we also show you how to write up the results from your assumptions tests and Pearson's correlation output if you need to report this in a dissertation, thesis, assignment or research report. We do this using the Harvard and APA styles.
Next, the results section needs to communicate the findings of your research in a systematic manner. The section needs to be organized such that the primary research question is addressed first, then the secondary research questions. If the research addresses multiple questions, the results section must individually connect with each of the ...
A correlational hypothesis in research methodology is a testable hypothesis statement that predicts the presence and nature of a relationship between two or more variables. It forms the basis for conducting a correlational study, where the goal is to measure and analyze the degree of association between variables.
Correlation coefficients (''r'') are a numerical value that ranges from -1 to 1 that represents the directionality and the strength or weakness of the linear relationship between two quantifiable ...
On the negative side, findings of correlation does not indicate causations i.e. cause and effect relationships. My e-book, The Ultimate Guide to Writing a Dissertation in Business Studies: a step by step assistance offers practical assistance to complete a dissertation with minimum or no stress. The e-book covers all stages of writing a ...
assignment that demonstrates your writing abilities and style needs to be uploaded to the Application portal. The sample must include in-text citations and references of scholarly work that informed your paper. The sample helps faculty advisors to determine the degree of assistance the student may need with thesis writing.