ACM Transactions on Quantum Computing

Volume 5, Issue 3
September 2024

ACM Transactions on Quantum Computing publishes high-impact, original research papers and selected surveys on topics in quantum computing and quantum information science. The journal targets the quantum computer science community with a focus on the theory and practice of quantum computing including but not limited to: models of quantum computing, quantum algorithms and complexity, quantum computing architecture, principles and methods of fault-tolerant quantum computation, design automation for quantum computing, quantum programming languages and systems, distributed quantum computing, quantum networking, quantum security and privacy, and applications (e.g. in machine learning and AI) of quantum computing.

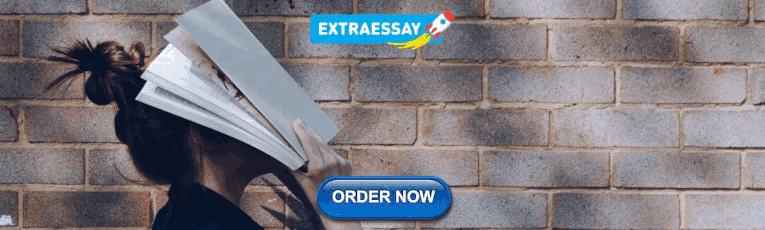
Subject Areas
Announcements.
Editors’ Suggestion
Learning Quantum Processes and Hamiltonians via the Pauli Transfer Matrix , published in issue 2, volume 5, reports new results for efficiently learning the complex processes that characterize quantum systems. The article shows how process learning is possible with linearly many copies of the corresponding Choi state when a quantum memory is available, whereas the case without quantum memory requires exponentially many queries to the unknown process.
TQC Accepted for Scopus and Clarivate ESCI Coverage
ACM Transactions on Quantum Computing (TQC) has been accepted for coverage in Elsevier’s Scopus and Clarivate’s ESCI. Similar to Web of Science, Scopus is an extensive yet selective abstract and citation database that provides comprehensive coverage of peer-reviewed journals, books, conference abstracts, and patents across the natural sciences, social sciences, arts, and humanities. By having its content included in Scopus, TQC's content will be discoverable at 7,000 of the world's top research institutions.
ACM Updates Its Peer Review Policy
ACM is pleased to announce that its Publications Board has approved an updated Peer Review Policy . If you have any questions regarding the update, the associated FAQ addresses topics such as confidentiality, the use of large language models in the peer review process, conflicts of interest, and several other relevant concerns. If there are any issues that are not addressed in the FAQ, please contact ACM’s Director of Publications, Scott Delman .
New ACM Policy on Authorship ACM has a new Policy on Authorship , covering a range of key topics, including the use of generative AI tools. Please familiarize yourself with the new policy and the associated list of Frequently Asked Questions .
Most Frequent Affiliations
Most cited authors, latest issue.
- Volume 5, Issue 3 September 2024 Issue-in-Progress EISSN: 2643-6817 View Table of Contents
An Algorithm for Reversible Logic Circuit Synthesis Based on Tensor Decomposition
The Affiliated Institute of ETRI, Daejeon, Korea (the Republic of)
Optimization Applications as Quantum Performance Benchmarks
Quantum Circuits Inc., New Haven, United States
Advanced Network Science Initiative, Los Alamos National Laboratory, Los Alamos, United States

D-Wave Systems Inc, Burnaby, Canada
Department of Physics and Astronomy, University of California Los Angeles, Los Angeles, United States, Theoretical Division (T-4), Los Alamos National Laboratory, Los Alamos, United States and Research Institute of Advanced Computer Science, Universities Space Research Association, Mountain View, USA
Applied Physics Laboratory, Johns Hopkins University, Baltimore, United States

Research Institute of Advanced Computer Science, Universities Space Research Association, Mountain View, United States, Quantum Artificial Intelligence Laboratory, NASA Ames Research Center, Mountain View, United States and Purdue University System, West Lafayette, United States
Recent Award Winners
Most popular, other acm journals.

Volume 2, Issue 2

Volume 3, Issue 2
April-June 2024

Volume 56, Issue 12
December 2024

Volume 5, Issue 2

Volume 36, Issue 2
Export Citations
- Please download or close your previous search result export first before starting a new bulk export. Preview is not available. By clicking download, a status dialog will open to start the export process. The process may take a few minutes but once it finishes a file will be downloadable from your browser. You may continue to browse the DL while the export process is in progress. Download
- Download citation
- Copy citation
We are preparing your search results for download ...
We will inform you here when the file is ready.
Your file of search results citations is now ready.
Your search export query has expired. Please try again.
Quantum Computing
Quantum Computing merges two great scientific revolutions of the 20th century: computer science and quantum physics. Quantum physics is the theoretical basis of the transistor, the laser, and other technologies which enabled the computing revolution. But on the algorithmic level, today's computing machinery still operates on ""classical"" Boolean logic. Quantum Computing is the design of hardware and software that replaces Boolean logic by quantum law at the algorithmic level. For certain computations such as optimization, sampling, search or quantum simulation this promises dramatic speedups. We are particularly interested in applying quantum computing to artificial intelligence and machine learning. This is because many tasks in these areas rely on solving hard optimization problems or performing efficient sampling.
Recent Publications
Some of our teams.
Applied science
We're always looking for more talented, passionate people.
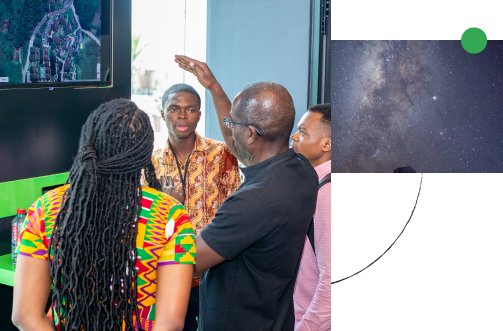
- Quantum computing
- Fundamentals
- Open access
- Published: 05 August 2022
- Volume 32 , pages 2525–2536, ( 2022 )
Cite this article
You have full access to this open access article
- Roman Rietsche 1 ,
- Christian Dremel ORCID: orcid.org/0000-0002-2161-4883 2 ,
- Samuel Bosch 3 ,
- Léa Steinacker 4 ,
- Miriam Meckel 5 &
- Jan-Marco Leimeister 1
19k Accesses
38 Citations
4 Altmetric
Explore all metrics
This article has been updated
Quantum computing promises to be the next disruptive technology, with numerous possible applications and implications for organizations and markets. Quantum computers exploit principles of quantum mechanics, such as superposition and entanglement, to represent data and perform operations on them. Both of these principles enable quantum computers to solve very specific, complex problems significantly faster than standard computers. Against this backdrop, this fundamental gives a brief overview of the three layers of a quantum computer: hardware, system software, and application layer. Furthermore, we introduce potential application areas of quantum computing and possible research directions for the field of information systems.
Similar content being viewed by others
From Quantum Mechanics to Quantum Computing
Quantum Computing and Its Impact
Quantum Computing Meets the Real World
Explore related subjects.
- Artificial Intelligence
- Quantum Computing
Avoid common mistakes on your manuscript.
Introduction
Quantum computing promises to be the next disruptive technology, with numerous possible applications and implications for organizations and markets. A recently published report by McKinsey estimates the global market value of quantum computing to be at USD 1 trillion by 2035, mainly in the financial, chemical, pharmaceutical, and automotive sectors (Hazan et al., 2020 ). Today, the world’s largest technology companies, such as Google, IBM, Microsoft, Amazon, and Alibaba, are already investing billions in research and development of their quantum computing and provide partial access to these quantum computers to the public via cloud infrastructures. However, not only industry players invest but also governments, for example, China is investing USD 10 billion in a national quantum computing laboratory, the U.S. government provided USD 1 billion, and the EU has a budget of overall more than EUR 1 billion (Castelvecchi, 2018; Deicker & Yasiejko, 2018).
Quantum computers exploit principles of quantum mechanics, such as superposition and entanglement, to represent data and perform operations on them (Ding & Chong, 2020 ). Both of these principles enable quantum computers to solve very specific, complex problems significantly faster than standard computers. Additionally, interference plays an important role specifically when reading information from the quantum computer (Aaronson, 2008 ). Quantum computers can calculate and test extensive combinations of hypotheses simultaneously instead of sequentially (S.-S. Li et al., 2001 ). Furthermore, some quantum algorithms can be designed in a way that they can solve problems in much fewer steps than their classical counterparts (their complexity is lower). For this reason, quantum computing could represent a significant breakthrough in modern IT in the next few years and might initiate the transition to the "5th industrial revolution" (Hadda & Schinasi-Halet, 2019 ).
First experiments show promising results, such as the one done by Google in 2019 in which the company claims to have achieved so-called quantum supremacy (IBM “quantum advantage”) (Arute et al., 2019 ). In an artificial experiment, they were able to demonstrate that a programmable quantum device could solve a problem that a classical computer could not solve in a feasible amount of time. However, the task solved by Google ‘s quantum computer was custom tailored to the specific quantum hardware used and has no real-world applications. Nevertheless, it was an important proof of concept. Furthermore, in 2020, Chinese scientists claimed to have built a quantum computer that is able to perform specific computations approximately 100 trillion times faster than the world‘s most advanced supercomputer (Zhong et al., 2020 ).
Given its current state of development, experts anticipate that quantum computing could provide unprecedented advantages, especially in the areas of optimization, artificial intelligence, and simulation (Langione et al., 2019 ; Ménard et al., 2020 ). It is likely that simulations of molecules (for chemical and pharmaceutical industries) will be among the first real-world applications of quantum computers. This is because molecules directly follow the laws of quantum mechanics, so using quantum computers is the most natural way of simulating them. Other industries that could soon benefit include the financial sector, transportation and logistics, the global energy and materials sector but also areas such as meteorology or cybersecurity (Gerbert & Ruess, 2018 ; Langione et al., 2019 ; Ménard et al., 2020 ). However, to date, quantum computing has extensive unsolved challenges in physics and computer science, ranging from hardware architectures and data management to application software and algorithms, which requires fundamental research in all these areas and beyond (Almudever et al., 2017 ).
To inform information systems (IS) research, this Fundamental provides the fundamental concepts of quantum computing and depicts research opportunities. Therefore, we provide in our second section a brief overview of a quantum computer system and its three layers of a quantum computer: hardware, system software, and application layer. The third section introduces potential application areas of quantum computing. Footnote 1 Building upon these and the introduced conceptual layer view on quantum computing, we relate to each layer by detailing potential research opportunities in the context of electronic markets. A whole new ecosystem around quantum computing technology itself is emerging already, provoking questions around the change of (1) business models and process innovation, (2) challenges for IT organizations, or (3) sourcing from start-ups, full-stack providers such as Google, IBM, Microsoft, or Alibaba or individual development.
Quantum computing system
In 1980, Paul Benioff envisioned the concept of a quantum touring machine, i.e., the theoretical concept of a quantum computer (Benioff, 1980 ). In 1982, Richard Feynman proposed the first practical application of a quantum computer: efficient simulations of quantum systems (Feynman, 1982 ). In general, a quantum computer can be defined as a universal computing device that stores information in objects called quantum bits (or qubits) and transforms them by exploiting very specific properties from quantum mechanics (Ding & Chong, 2020 ). The quantum computer performs quantum computing, which is a type of computation that collects the different states of qubits, such as superposition, interference, and entanglement, to perform calculations (Grumbling & Horowitz, 2019 ). Importantly, quantum computers are not intended to become general purpose computers that operate by themselves. They will be highly specialized devices that can solve specific tasks much faster than classical computing. Operating quantum computers will most certainly require a classical computer for loading input/output data, retrieving results from computations as well as controlling the quantum computer’s electronic and internal processes. Thus, quantum computers and classical computers form a quantum computing system that enables quantum computers to perform quantum computing. To depict the different layers of a quantum computing system, we adopt the model of Ding and Chong ( 2020 ) for three reasons. First, it allows us to analytically distinguish the key components of a quantum component system to illustrate the fundamental mechanisms and elements. Second, it builds on an analytics distinction of hardware, system software, and application, which is mirrored in conceptual views on computing architectures, e.g., cloud computing (Infrastructure-as-a-Service, Platform-as-a-Service, and Software-as-a-Service) or the layered modular architecture of digital technologies (Yoo et al., 2010). Third, our expert informants distinguished between similar layers as well in their interview statements to explain the state of the art, the challenges for today’s organizations, and the functioning of quantum computing systems. Figure 1 shows a quantum computing system consisting of a van Neumann architecture for classical computing and a quantum computer with its three layers architecture, which we will explain accordingly.
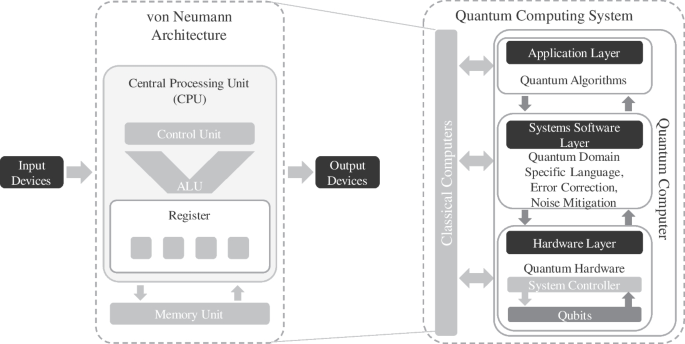
Showing a classical computer (von Neumann architecture) and a quantum computer forming a quantum computing system (adapted from Ding and Chong ( 2020 ))
Hardware layer
One fundamental difference between classical and quantum computers is how information is stored. Whereas classical computers use bits, which can have the value of either zero or one, to store information, quantum computers use quantum bits (or qubits), which can hold any linear combination of zero and one simultaneously (Steane, 1998 ). Qubits leverage the advantage of the properties of quantum mechanics and in particular the effect of superposition (visualization see Fig. 2 ).
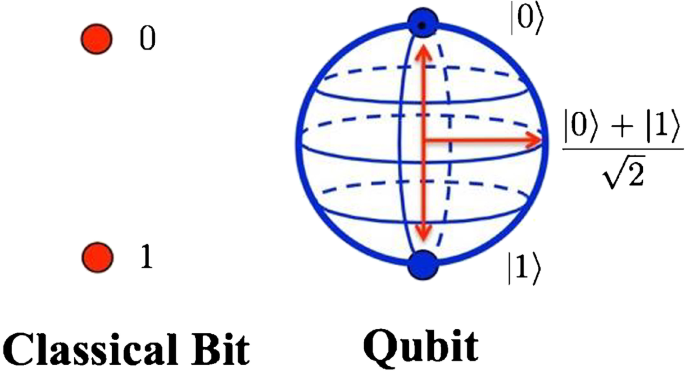
Classical bit and qubit (Superposition)
Superposition
Loosely speaking, a qubit is described by its probability of being either zero or one and not by the distinct value of zero or one. Thus, a qubit can have the probability of being 60% zero and 40% one. Importantly, only at the point of measuring the state of the qubit, it “collapses” to the single classical defined value of either zero or one (Bosch, 2020 ; Ding & Chong, 2020 ). The property of superposition has the advantage that a quantum computer with just four qubits is able to represent 16 four-digit numbers simultaneously. With each further qubit, the number of representable states doubles whereas a classical computer, with a sequence of four bits, can only represent a single four-digit number.
The real advantage of quantum computing relies on the fact that one can perform an exponential amount of calculations at the same time. Even though at the end of every program it is possible to read only the solution to one calculation, it is possible to develop a quantum algorithm that makes it very likely that the final result is precisely the one that one is looking for. For example, we might be trying to find out if there exists any possible rarely occurring turbulence that could cause a plane to crash. Instead of simulating billions of combinations of air conditions on a classical computer and checking their individual results, we could simply test almost all possible air conditions at once on a quantum computer and read out only the result that causes the plane to crash.
Entanglement
Not only qubits are unique to quantum computing. Entanglement is also a property of quantum mechanics. Entanglement is when the state of one qubit is dependent on the state of another qubit (Steane, 1998 ). Thus, when two qubits are entangled, making any kind of flip or rotation on one of the qubits would result in the same change happening to the other qubit (Einstein et al., 1935 ; Schrödinger, 1935 ). Furthermore, when the state of either one of the two qubits is measured, the state of both qubits collapses to either one or zero (depending on their probabilities). This is even the case when the qubits are far away from each other. Thus, the advantage of entanglement is that when a qubit influences the other qubits around it, all are working in tandem to arrive at a solution. Therefore, qubits can be correlated in a way that is not possible for bits in traditional computers. This opens up new possibilities and gives the quantum computer the ability to process information in a fundamentally different way than a classical computer (Mooney et al., 2019 ). One example is superdense coding, which is the process of transporting two classical bits of information using one entangled qubit (A. Harrow et al., 2004 ). This process is especially interesting for secure quantum key distribution (Bennett & Brassard, 2014 ). This is a secure communication method that implements a cryptographic protocol relying on quantum entanglement and other quantum phenomena. It enables two parties to produce a shared random secret key (entangled qubit) known only to them, which can then be used to encrypt and decrypt messages (Scarani et al., 2009 ).
Based on the fundamentals of quantum mechanics, we now discuss the approaches to physically represent and manipulate qubits. Broadly speaking, the approaches can be split into two main categories: a) analog quantum computing and b) digital gate-based quantum computing (Ding & Chong, 2020 ).
Analog quantum computing
In analog quantum computing, the quantum state is smoothly changed by quantum operations such that the information encoded in the final system corresponds to the desired answer with high probability. One example of analog quantum computing is adiabatic quantum computers (Albash & Lidar, 2018 ), which refer to the idea of building a type of universal quantum computing. A special form of adiabatic quantum computers is quantum annealing, which is a framework that incorporates algorithms and hardware designed to solve computational problems via quantum evolution towards the ground states (Vinci & Lidar, 2017). Quantum annealing takes advantage of the fact that physical systems strive towards the state with the lowest energy, e.g., hot things cool down over time or objects roll downhill. As such, in quantum annealing the energetically most favorable state then corresponds to the solution of the optimization problem (Albash & Lidar, 2018 ). Using the property of superposition, the quantum annealer is able to calculate all potential solutions at the same time, which speeds up the calculation process drastically in comparison to classical computers (Shin et al., 2014). Quantum annealing is most suitable for optimization problems or probabilistic sampling and is used by companies such as D-Wave. However, to date, it is unclear whether the quantum annealing technique will ever achieve significant quantum speedup (Albash & Lidar, 2018 ).
Digital gate-based quantum computing
In digital gate-based quantum computing, the information encoded in qubits is manipulated through digital gates. In comparison to the analog approach in which you sample the natural evolution of quantum states to find the optimal state of low energy, in digital gate-based quantum computers the evolution of the quantum states is manipulated in terms of activity and controlled to find the optimal solution (Ding & Chong, 2020 ). Thus, the state of qubits is actively manipulated and therefore provides the advantage of being much more flexible, and it can be used to solve large classes of problems, in contrast to quantum annealing. Digital gate-based quantum computing is conceptually very similar to classical computation (Grumbling & Horowitz, 2019 ). A classical algorithm is run on a computer as a series of instructions (gates such as AND, OR, NOT, …). They manipulate individual or pairs of classical bits and flip them between zero and one states according to a set of rules. Quantum gates operate directly on one or multiple qubits by rotating and shifting them between different superpositions of the zero and one states as well as different entangled states. Companies using digital gate-based quantum computing are, for example, IBM, Google and Rigetti.
System software layer
The system software layer builds on top of the hardware layer and orchestrates the system’s processes to leverage the potentials of the qubits (superposition and entanglement). This layer has to cope with challenges of the thermodynamically unstable quantum states. It actively reduces thermal noise within and around the quantum system and performs error correction procedures.
In quantum computing there are many potential sources that can cause noise. For example, quantum computers and especially digital gate-based ones are highly sensitive to changes in the environment, such as vibration, temperature fluctuations, etc. Noise can also be caused by imprecise control of the quantum hardware or manufacturing defects (Ding & Chong, 2020 ). Most quantum computers even require their chips to be cooled down to a hundredth of a degree above absolute zero temperature to operate. Thus, since noise cannot be avoided, the first era of quantum computers is also called noisy Intermediate-Scale Quantum Computer (NISQ, Preskill, 2018). This abbreviation implies that current quantum hardware using dozens of qubits has error rates that are too high, which need to be improved before we can build useful quantum computers with hundreds, or even thousands, of usable qubits.
Noise in the environment can lead to qubit decoherence which is environmental influences causing quantum states to randomly change (Grumbling & Horowitz, 2019 ). This is problematic, as a single error in a calculation usually causes the result to be incorrect, unless the error is corrected during the calculation. Since it is impossible to prevent every kind of noise, error correction is important. Ongoing research on quantum error correction seeks to achieve system-level fault tolerance. Quantum error correction differentiates between physical and logical qubits. Logical qubits are represented by a group of physical qubits, which are needed for error correction. Physical qubits work together on correcting errors on individual physical qubits. A group of physical qubits is less likely to cause an error in a calculation than just one physical qubit. Unfortunately, error-correcting mechanisms can cause errors themselves. Depending on the error-correcting mechanism, the relation is typically five to nine physical qubits to achieve one almost error-free logical qubit (Marinescu & Marinescu, 2012 ; Shor, 1995 ).
One way to do this is by representing every qubit with groups of several physical qubits that, loosely speaking, work together on correcting errors on individual physical qubits. A perfect physical qubit can work as a logical qubit, as it requires no error correction. Today, the biggest challenge is scaling up to thousands of qubits. Even though the computational space that can be used for calculations doubles (Ding & Chong, 2020 ) with the addition of every individual qubit, this advantage presently cannot be exploited in its full capacity due to high error rates. One prominent example for trying to increase the number of qubits is IBM, which states that it wants to achieve over 1,000 qubits by 2023, while currently there are machines with 60–100 available (Gambetta, 2020 ).
Application layer
One of the main challenges of today’s quantum computers is the unsolved problem of efficient quantum memory (Ciliberto et al., 2018 ). There exist several theoretical proposals for building quantum random access memory (QRAM). Even though it may be experimentally difficult to build (just as the quantum computer itself), recent publications demonstrated several possible paths of doing so (Hann et al., 2019 ; Park et al., 2019 ). Thus, currently exists no efficient way to store states of qubits in a memory for a long time for other calculations. Therefore, data needs to be loaded from a classical computer to the targeted quantum computer, and after performing the calculation states need to be read (measured) by the classical computer before the qubits lose their information. Due to the no cloning theorem, we are also not able to make copies of quantum states and use them for calculations. The only way to load a quantum state from quantum memory into a quantum program is by applying a SWAP operation, and thereby removing it from the memory.
When a quantum state is measured, it collapses to either one or zero. Therefore, we have no way of finding out what state a certain qubit is in. The only way we can approximately find out what state a qubit is in is if we have multiple copies of the same qubit and measure them all. In some cases, reading the classical data may dominate the cost of quantum algorithms so that it cannot speed up the whole algorithm at the macro level. Reading out the data exactly may be infeasible, which cannot meet the computing needs in some tasks. This is especially the case for methods that need large data sets, such as machine learning and artificial intelligence.
Finding a useful algorithm for quantum computers is mostly about constructing it in such a way that the probability of measuring the desired outcome is maximized. Even though the output of the quantum computer may be an exponentially large number of solutions, we are usually just interested in a small subset of these solutions. Finding them without having to run the whole algorithm many times is the art of quantum algorithm construction. Here are three of the most important quantum algorithms.
Grover’s algorithm is also known as the quantum search algorithm. Grover’s algorithm is used for searching an unstructured database or an unordered list. Classically, for finding a particular item in a database of size N, we need to go through, on average, N/2 items to find the right one. Using Grover’s algorithm, we can do this in only √N steps, on average. For a large N, this can be remarkably faster. This is called a quadratic speedup (Grover, 1996 ).
Shor’s algorithm , also known as the integer factorization algorithm, can factorize integers almost exponentially faster than the fastest known classical algorithm. Factorizing integers is very difficult computationally and is therefore also the basis of RSA encryption (Shor, 1994 ).
HHL (Harrow Hassidim Lloyd) is also known as the quantum algorithm for linear systems of equations. The algorithm can estimate the result of a function of the solution x of a linear system (Ax = b), where A is a matrix and b a vector (A. W. Harrow et al., 2009 ).
Application areas of quantum computing
Thanks to the enormous progress in hardware, more and more established commercial companies are investing in quantum technology. Examples include Boehringer Ingelheim, who recently announced a research partnership with Google (Boehringer-ingelheim, 2021 ), and Daimler, who announced progress in the field of materials research (Motta et al., 2020 ), or chemistry giants like BASF who aim to stay at the forefront of chemistry research and business (Hartmann & Deppe, 2021 ). Quantum computing has three essential capabilities to address today’s computational problems that current computers are not or only partially capable of and that bear benefits for companies: 1) search and graph, 2) algebraic and 3) simulation (Hoffmann, 2021 ; Li et al., 2020 ). These capabilities determine the potential applications of this technology in numerous industries, such as finance, chemistry and pharma, manufacturing, energy, or cybersecurity (Gerbert & Ruess, 2018 ; Langione et al., 2019 ; Ménard et al., 2020 ). Table 1 provides a summary of the problem types, approaches and potential use cases.
Search and graph
The fact that a qubit can theoretically represent an infinite number of states allows for solving complex combinatorial optimization problems, which is currently one of the major application areas for current quantum computing technologies, such as the solution of D-Wave (Johnson et al., 2011 ). Combinatorial optimization is the process of finding one or more optimal solutions to a problem. Examples of such problems include supply chain optimization, optimizing public transportation schedules and routes, package deliveries, etc. These solutions are searched for in a discrete (finite) but very large configuration space (i.e., a set of states). The set of possible solutions can be defined with several constraints and the goal is to optimize the objective function with the best solution.
Since the problem spaces in certain complex problems are very large, it is extremely difficult to find the optimal or even a single good solution to these problems with classical computers in a reasonable time frame or with sufficient accuracy. Such combinatorial optimization problems often pose a great challenge for the private as well as the public sector. While they are often simple to describe, they turn out to be very difficult to solve. Combinatorial optimization problems may be divided into order, assignment, grouping, and selection problems, and within these classes, subclasses exist, such as the knapsack or the traveling salesman problem. In addition to the property that there can be a lot of qualitatively different solutions for a problem, no known algorithm exists that can easily compute these problems directly. Searching very large problem spaces requires an enormous amount of computing capacity and time.
Respectively, quantum computers are expected to play a decisive role in the financial services industry. Especially players specializing in portfolio optimization and arbitrage could benefit (Egger et al., 2020 ). From a very large pool of existing financial instruments, a subset should be selected so that a certain portfolio volume is achieved, while at the same time a large number of factors must be taken into account to minimize risk and achieve profitability (Chakrabarti et al., 2021 ). Further, Deutsche Börse (a German company offering marketplace organizing for the trading of shares) already experimented with the applicability of quantum computing for a sensitivity analysis on one of their risk models, a computation that is too expensive to be run on classical computers (Braun et al., 2021 ). Due to its suitability to solve optimization problems, another application of quantum computing is the optimization of flow, e.g., of traffic or goods. Collaborating with D-Wave Systems, VW has already shown in a pilot project how to optimize a simplified traffic flow in the city of Lisbon by leveraging quantum annealing technologies (Neukart et al., 2017 ; Yarkoni et al., 2019 ) – a project that started in late 2016 with a proof-of-concept project. It investigated the readiness of quantum computing by building a traffic-flow optimization program that used GPS coordinates of 418 taxis in Beijing to resolve traffic congestion.
Moreover, quantum computers are superior to classical computers regarding certain prime factorization procedures that play an important role in the secure encryption of data. A popular example for this is the aforementioned Shor ( 1994 ) algorithm that factors a number into its prime factors, a process used often in cryptography and cybersecurity. A dataset encrypted with quantum technology would be impossible to decrypt with classical computer technology, or at least not in time periods relevant to human users. Conversely, it would be easy for a quantum computer to crack data encrypted with classical RSA technology – a phenomenon that may be coined as quantum threat (Mone, 2020 ).
The ability of quantum computing to accelerate optimization problems plays a crucial role for narrow AI approaches (Gao et al., 2018 ; Langione et al., 2019 ). Quantum computing can help to calculate complex network architectures and weights for machine learning and artificial intelligence. Quantum computing shows its advantage in transforming and calculating large metrices. For example, in the context of supervised learning, the model aims to minimize the error between the prediction of the model itself compared to the input and adequate output or label given. Quantum computers offer several approaches to solving problems like this, thereby, again, accelerating calculation and allowing for more complex network architectures (DeBenedictis, 2018 ). They may be applicable to all relevant practices or sub-tasks of artificial intelligence, such as image processing and computer vision (Dendukuri & Luu, 2018 ) or natural language processing, as demonstrated in an experiment by Cambridge Quantum Computing (Lorenz et al., 2021 ). Having said that, it is important to note that, so far, no near-term machine learning algorithm with provable speedup has been found.
A quantum computer has a fundamental advantage over classical computers: It can simulate other quantum systems (e.g., a nitrogen molecule) much more efficiently than any computer system available today. For classical computers, even molecules with comparatively low complexity represent an almost unsolvable task. In the 1980s, Richard Feynman theoretically substantiated the possibility of a quantum-based computer for simulating molecules (Feynman, 1982 ). Since then, researchers have attempted to transfer the quantum system of a molecule into another quantum system, i.e., into the quantum computer, in order to simulate it. One new hope in the application of quantum computers is the simulation of more efficient catalysts for ammonia synthesis in the Haber–Bosch process, which today accounts for about 1 to 2 percent of global energy consumption. Better catalysts could reduce energy consumption and thus also help slow global warming. Even quantum computers without full error correction may already be better suited for this application than simulations on classical computers (Budde & Volz, 2019 ).
Furthermore, the development of active ingredients and drugs is often a lengthy and very cost-intensive process. This is due in particular to the fact that a large number of substances have to be tested on a trial-and-error basis in the real world. Yet, building on the same principles of quantum physics, quantum computing may be able to virtually replicate the behaviors such that simulation-based research may sooner or later replace this cost-intensive process.
For instance, BASF, pursuing its high requirements for the accuracy of quantum chemical calculations, investigated, in collaboration with the company HQS, the applicability of quantum computing. Specifically, they aimed to understand the quantum mechanical calculation of the energy course of chemical reactions, as this actually allows for the prediction of the probable course (i.e., how does the reaction proceed, which products, by-products, etc., are formed, how can I accelerate the reaction with the help of catalysts, etc.) of chemical reactions. This application of needed methods reaches the limits of conventional computing methods (Kühn et al., 2019 ). In addition, material research on the functioning of batteries is deemed to inform today’s electromobility and is already targeted by automotive giants such as VW (Neukart, 2021 ; Ziegler & Leonhardt, 2019 ).
Link to the field of information systems
Even though there are high investments in quantum computing, most expert estimations still place the widespread industrial application of quantum computing at least five to ten years in the future. Its exact manifestations in many critical areas remain unclear. Thus, it is the task of today’s research community to creatively conjure up and explore the full potential and the socio-technological consequences of quantum computing. Therefore, based on analyzing existing literature and the conducted interviews with 21 leading experts in industry and research, we propose the following four initial directions for research on quantum computing in information systems (for a summary, see Table 2 ): 1) quantum computing ecosystems as a new networked business, 2) digital understanding as a foundation for use cases and ecosystems, 3) quantum computing as a challenge for IT organizations and IT service providers, and 4) skills needed to leverage quantum computing in the quantum computing field.
All of these directions try to consider the fact that quantum computing despite its disruptive potential will initially be an extension of computing capabilities for established electronic markets, ecosystems, and its participants (see Fig. 3 ), while new ecosystem participants are already establishing themselves (e.g., IonQ or Rigetti).
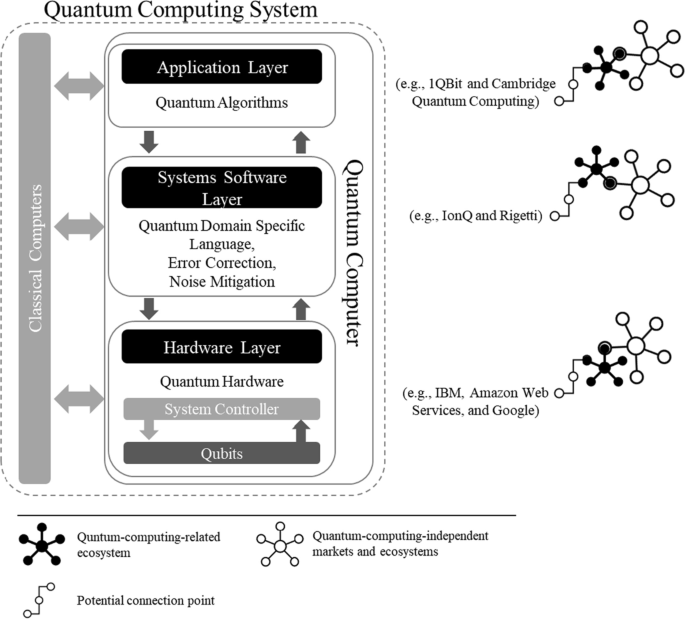
Quantum computing system and relevant point of contacts for established ecosystems
We further try to focus on established research areas and the focus of our research community.
Quantum computing ecosystems as a new networked business
The adoption and diffusion of quantum computing will heavily rely on an emerging ecosystem comprising technology providers, such as IBM, Google, Microsoft, or Amazon Web Services, start-ups with specific playgrounds such as 1Qbit or IonQ as well as consulting firms and academic institutions to support customers in adopting and building applications using quantum computing technologies (Carrel-Billiard et al., 2021 ; IBM, 2019 ). Also, the European Union built their own ecosystem with the “Quantum Flagship”. Footnote 2 Companies, providers, research institutions, and governments ultimately need to engage in such an ecosystem to allow for getting hold of capabilities that transcend their own organizational boundaries or even their entire industry (e.g., building their own computing infrastructures, translating business problems into mathematical and quantum problems, etc.) (Carrel-Billiard et al., 2021 ). Due to this emerging new organizing logic and structure for quantum computing, key aspects need to be considered when pursuing information systems research in this context.
First, the entrance barrier to quantum computation is expected to be very high due to multiple limitations such as the necessity of knowledge in quantum physics, the expensiveness of building quantum computers and the shortage of experts in the labor market. As such, they may enforce divides and limit access. Steps should be taken to reduce a possible quantum divide. Second and consequently, incumbents will need to rely on the capabilities that technology providers, start-ups, consulting firms, or academic institutions may provide, as they might go beyond their domain expertise. As such, prevailing networked businesses and ecosystems need to develop methods and technologies to purposefully connect their way of doing digital business with the emerging quantum computing ecosystem players in the different layers, namely the hardware layer (e.g., Amazon Web Services, IBM, and Google), the system layer (e.g., IonQ and Rigetti), and the application layer (e.g., Cambridge Quantum Computing or 1QBit) (IBM, 2019 ).
Today, the playground is already diverse, with fuzzy boundaries leading to the need for design-science-oriented guidance for incumbents to assess their own business and technology maturity. For instance, IonQ and Rigetti are positioned on both the hardware and the system software layer. Additionally, for companies it is important to mediate the engagement with different players as part of their quantum computing road map. Thus, possible research questions might include the following: Does the access to quantum computing need to be regulated? Does quantum computing need new sourcing strategies? How does the emerging quantum computing ecosystem act as a spoke component to other industries and ecosystems? Which transformation may result from the emergence of a quantum computing networked business?
Digital understanding and representation as a foundation for quantum computing use cases and ecosystems
The proliferation of quantum computing as a generative technology for calculating with an enormous speedup relies on a fundamental premise: The problem which will be solved by a quantum computing approach needs to be replicated in the form of digital data on which basis a calculation becomes possible in the first place. Emerging technologies such as machine learning already challenge today’s organizations. The main reason is that it is complicated to digitally represent business practices and economic behavior to allow for analysis. This phenomenon may be summarized as datafication (Lycett, 2013 ). As such, the dematerialization of the physical world in the form of digital data as a digital representation is an essential prerequisite (Recker et al., 2021 ). Only with this prerequisite, one may use quantum computing when calculating the physical world based on its datafied digital representation.
Achieving an adequate digital representation of the respective quantum computing problem requires a mathematical and conceptual understanding to allow for assessing, understanding, and realizing the value of quantum computing aside from other computing approaches (e.g., high performance computing). Furthermore, quantum computing may also serve as an enabler for process innovation; for example, it could be interesting for research areas around process mining (Mendling et al., 2020 ), such as analyzing and optimizing process configurations or simulating contexts of processes or configurations of processes (vom Brocke et al., 2021 ). Therefore, research on use case analysis and in particular on methods of how to find, describe, and analyze use cases systematically and at scale are highly relevant. Possible research questions could include the following: What approaches could be used or developed to analyze business problems and therefore leverage the potential of quantum computing? How can these problems be described mathematically? What are possible design principles of artifacts to describe use cases? How will QC impact the modeling of a social and economic reality as a transformation from binary to multidimensional quantum states?
Quantum computing as a challenge for IT organizations and IT service providers
IT competencies are increasingly built up in business units using commercial IT services without having the IT department in the loop. Quantum computing drives this change even further, since for the next few decades, the first quantum computers will likely only be available via the cloud for most companies (Carrel-Billiard et al., 2021 ). IT departments are therefore under pressure in terms of how to manage quantum computer usage in companies, especially with regards to transmitting the respective data which is needed for quantum-computing-based calculations. This is of particular interest, since data preparation including data input and output might be the bottleneck for quantum computing in the long run. Furthermore, quantum computing and especially the ability of prime factorization is a threat for current encryption standards and poses huge challenges for the IT organization. Even though new encryption techniques can be used once quantum computers become a real threat to current encryption protocols, past communication and old data can be decrypted retroactively.
Future research questions could include the following: What are possible security approaches to protect legacy IT with old encryption standards? Can quantum computers and AI be used for real-time threat and anomaly detection? How can quantum computers be used to simulate possible intrusions and cyberattacks for calculating risk–cost evaluations? The latter is of special interest due to the hyper-connectivity of digital services, which poses an enormous vulnerability for an infrastructural attack.
Quantum computing skills
Historically, the role of information systems has been to bridge the gap between informatics and business. In the age of quantum computing, this role is becoming more important than ever before. In order to leverage the potential of quantum computing, at least three roles are required (Carrel-Billiard et al., 2021 ; Hughes et al., 2022 ): First, mathematical and quantum physical skills are needed to translate problems into mathematical formulas. Second, domain expertise is needed to integrate the business problem within the mathematical formulation. Third, an intermediary is needed to facilitate between both roles (Gartner, 2019 ). Due to the high complexity and high specialization of the job types (e.g., error correction specialist, quantum algorithm developer), the entrance barrier to the field of quantum computing is significantly higher than for regular "coding". Additionally, for years there has been a shortage of STEM (Science Technology Engineering Mathematics) graduates, which may amplify the war for talents in quantum computing (OECD, 2021 ). Having said that, companies such as IBM, Google, or research institutions such as ETH, are working on developing programming languages and compilers in which a device will decide if the application is suitable for a quantum computer. However, according to experts, this will take years. Future research questions could include the following: How could information systems act in a mediating role for adopting quantum computing technologies? Should quantum computing be included in the information systems curriculum? Since quantum computing knowledge is important on a strategic level, how can future information systems managers be trained to be aware of its potential? How can management leverage the potentials of available techniques, approaches and platforms around quantum computing? How can gaps of knowledge and access to infrastructures be mitigated?
In this Fundamentals article, we provide an overview of the constituting concepts of quantum computing. Against this backdrop, this fundamental gives a brief overview of the three layers of a quantum computer: hardware, system software, and application layer. On this basis and our access to leading experts in quantum computing, we propose several focus areas for studying the socio-technical implications of quantum computing for the emergence of new ecosystems or their extensions as well as for ecosystem participants themselves.
The disruptive nature of quantum computing will lead to various changes in all socio-technical components of organizations and in IS-related ecosystems. As such, we expect a large impact on the IS discipline in academia, practice, and teaching. At the same time, we are aware that quantum computing is in its infancy, both as a field of research for IS research as well as in its development towards an established and well-understood computing approach. Against this backdrop, we hope to inform and inspire research on the socio-technical peculiarities of quantum computing on the ecosystem level or level of electronic markets (e.g., quantum computing ecosystems as a new networked business), the organizational level (e.g., the role of IT organizations and service provider for establishing quantum computing), the individual level (e.g., quantum computing skills) as well as on the crucial role of data (i.e., digital understanding and representation of economic behavior) to allow for quantum computing calculations.
Change history
09 october 2022.
Missing Open Access funding information has been added in the Funding Note.
The Fundamentals article is built on the extent body of knowledge on quantum computing. For our literature review we broadly searched for the term “quantum computing” in libraries, such as EBSCO, ScienceDirect, IEEE, or the AIS eLibrary in computer science and IS research. Both the application areas as well as the research opportunities are informed by the prevailing themes of 21 conducted interviews with technology and academic experts from well-established Fortune 500 companies and prestigious academic institutions.
https://qt.eu/
Aaronson, S. (2008). THE LIMITS OF Quantum. Scientific American , 298 (3), 62–69. http://www.jstor.org/stable/26000518 . Accessed 3 June 2021
Albash, T., & Lidar, D. A. (2018). Adiabatic quantum computation. Reviews of Modern Physics, 90 (1), 015002-1-0150026–4. https://doi.org/10.1103/RevModPhys.90.015002
Article Google Scholar
Almudever, C. G., Lao, L., Fu, X., Khammassi, N., Ashraf, I., Iorga, D., Varsamopoulos, S., Eichler, C., Wallraff, A., Geck, L., Kruth, A., Knoch, J., Bluhm, H., & Bertels, K. (2017). The engineering challenges in quantum computing, Design, Automation & Test in Europe Conference & Exhibition (DATE) , 2017 , 836–845. https://doi.org/10.23919/DATE.2017.7927104
Arute, F., Arya, K., Babbush, R., Bacon, D., Bardin, J. C., Barends, R., Biswas, R., Boixo, S., Brandao, F. G. S. L., Buell, D. A., Burkett, B., Chen, Y., Chen, Z., Chiaro, B., Collins, R., Courtney, W., Dunsworth, A., Farhi, E., Foxen, B., & Martinis, J. M. (2019). Quantum supremacy using a programmable superconducting processor. Nature, 574 (7779), 505–510. https://doi.org/10.1038/s41586-019-1666-5
Benioff, P. (1980). The computer as a physical system: A microscopic quantum mechanical Hamiltonian model of computers as represented by Turing machines. Journal of Statistical Physics, 22 (5), 563–591. https://doi.org/10.1007/BF01011339
Bennett, C. H., & Brassard, G. (2014). Quantum cryptography: Public key distribution and coin tossing. Theoretical Computer Science, 560 , 7–11. https://doi.org/10.1016/j.tcs.2014.05.025
Boehringer-Ingelheim. (2021). Partnership in quantum computing for Pharma R&D | Press . https://www.boehringer-ingelheim.com/press-release/partnering-google-quantum-computing . Accessed 3 June 2021
Bosch, S. (2020). Quantum algorithms for linear algebra and optimization [Master Thesis, École polytechnique fédérale de Lausanne]. Library catalog. https://www.academia.edu/43923193/Quantum_Algorithms_for_Linear_Algebra_and_Optimization?source=swp_share . Accessed 3 June 2021
Braun, M. C., Decker, T., Hegemann, N., Kerstan, S. F., & Schäfer, C. (2021). A quantum algorithm for the sensitivity analysis of business risks . http://arxiv.org/pdf/2103.05475v1 . Accessed 3 June 2021
Budde, F., & Volz, D. (2019). The next big thing? Quantum computing’s potential impact on chemicals . https://www.mckinsey.com/industries/chemicals/our-insights/the-next-big-thing-quantum-computings-potential-impact-on-chemicals . Accessed 3 June 2021
Carrel-Billiard, M., Treat, D., Dukatz, C., & Ramesh, S. (2021). Accenture get ready for the quantum impact . https://www.accenture.com/_acnmedia/PDF-144/Accenture-Get-Ready-for-the-Quantum-Impact.pdf . Accessed 3 June 2021
Chakrabarti, S., Krishnakumar, R., Mazzola, G., Stamatopoulos, N., Woerner, S., & Zeng, W. J. (2021). A threshold for quantum advantage in derivative pricing. Quantum, 5 , 463–504. https://doi.org/10.22331/q-2021-06-01-463
Ciliberto, C., Herbster, M., Ialongo, A. D., Pontil, M., Rocchetto, A., Severini, S., & Wossnig, L. (2018). Quantum machine learning: A classical perspective. Proceedings Mathematical, Physical, and Engineering Sciences, 474 (2209), 20170551. https://doi.org/10.1098/rspa.2017.0551
DeBenedictis, E. P. (2018). A future with quantum machine learning. Computer, 51 (2), 68–71. https://doi.org/10.1109/MC.2018.1451646
Dendukuri, A., & Luu, K. (2018). Image processing in quantum computers . http://arxiv.org/pdf/1812.11042v3 . Accessed 3 June 2021
Ding, Y., & Chong, F. T. (2020). Quantum computer systems: Research for noisy intermediate-scale quantum computers . Synthesis lectures on computer architecture . Morgan &Claypool. https://doi.org/10.2200/S01014ED1V01Y202005CAC051
Egger, D. J., Gambella, C., Marecek, J., McFaddin, S., Mevissen, M., Raymond, R., Simonetto, A., Woerner, S., & Yndurain, E. (2020). Quantum computing for finance: State-of-the-art and future prospects. IEEE Transactions on Quantum Engineering, 1 , 1–24. https://doi.org/10.1109/TQE.2020.3030314
Einstein, A., Podolsky, B., & Rosen, N. (1935). Can quantum-mechanical description of physical reality be considered complete? Physical Review, 47 (10), 777–780. https://doi.org/10.1103/PhysRev.47.777
Feynman, R. P. (1982). Simulating physics with computers. International Journal of Theoretical Physics, 21 (6–7), 467–488. https://doi.org/10.1007/BF02650179
Gambetta, J. (2020). IBM’s Roadmap for scaling quantum technology . https://www.ibm.com/blogs/research/2020/09/ibm-quantum-roadmap/ . Accessed 3 June 2021
Gao, X., Zhang, Z.-Y., & Duan, L.-M. (2018). A quantum machine learning algorithm based on generative models. Science Advances, 4 (12), eaat9004. https://doi.org/10.1126/sciadv.aat9004
Gartner. (2019). The CIO's guide to quantum computing . https://www.gartner.com/smarterwithgartner/the-cios-guide-to-quantum-computing . Accessed 3 June 2021
Gerbert, P., & Ruess, F. (2018). The next decade in quantum computing and how to play . https://www.bcg.com/publications/2018/next-decade-quantum-computing-how-play . Accessed 3 June 2021
Grover, L. K. (1996). A fast quantum mechanical algorithm for database search. In Proceedings of the twenty-eighth annual ACM symposium on Theory of Computing, pp . 212–219. https://doi.org/10.1145/237814.237866
Grumbling, E., & Horowitz, M. (2019). Quantum computing: Progress and prospects (2019) . National Academies Press. https://doi.org/10.17226/25196
Hadda, M., & Schinasi-Halet, G. (2019). Quantum computing: A technology of the future already present . https://www.pwc.fr/fr/assets/files/pdf/2019/11/en-france-pwc-point-of-view-quantum-computing-2019.pdf . Accessed 3 June 2021
Hann, C. T., Zou, C.-L., Zhang, Y., Chu, Y., Schoelkopf, R. J., Girvin, S. M., & Jiang, L. (2019). Hardware-efficient quantum random access memory with hybrid quantum acoustic systems. Physical Review Letters, 123 (25), 250501. https://doi.org/10.1103/PhysRevLett.123.250501
Harrow, A., Hayden, P., & Leung, D. (2004). Superdense coding of quantum states. Physical Review Letters, 92 (18), 187901. https://doi.org/10.1103/PhysRevLett.92.187901
Harrow, A. W., Hassidim, A., & Lloyd, S. (2009). Quantum algorithm for linear systems of equations. Physical Review Letters, 103 (15), 150502.
Hartmann, M. J., & Deppe, F. (2021). Erste Demonstration von Quantenüberlegenheit . https://doi.org/10.1002/piuz.202001587
Hazan, E., Ménard, A., Patel, M., & Ostojic, I. (2020). The next tech revolution: quantum computing . https://www.mckinsey.com/fr/~/media/McKinsey/Locations/Europe%20and%20Middle%20East/France/Our%20Insights/The%20next%20tech%20revolution%20Quantum%20Computing/Quantum-Computing.ashx . Accessed 3 June 2021
Hoffmann, M. (2021). The quantum speedup will allow completely new applications. Digitale Welt, 5 (2), 10–12. https://doi.org/10.1007/s42354-021-0329-5
Hughes, C., Finke, D., German, D.‑A., Merzbacher, C., Vora, P. M., & Lewandowski, H. J. (2022). Assessing the needs of the quantum industry. IEEE Transactions on Education , 1–10. https://doi.org/10.1109/TE.2022.3153841
IBM. (2019). Building your quantum capability: The case for joining an “ecosystem" . https://www.ibm.com/thought-leadership/institute-business-value/report/quantumeco . Accessed 3 June 2021
Johnson, M. W., Amin, M. H. S., Gildert, S., Lanting, T., Hamze, F., Dickson, N., Harris, R., Berkley, A. J., Johansson, J., Bunyk, P., Chapple, E. M., Enderud, C., Hilton, J. P., Karimi, K., Ladizinsky, E., Ladizinsky, N., Oh, T., Perminov, I., Rich, C., & Rose, G. (2011). Quantum annealing with manufactured spins. Nature, 473 (7346), 194–198. https://doi.org/10.1038/nature10012
Kühn, M., Zanker, S., Deglmann, P., Marthaler, M., & Weiß, H. (2019). Accuracy and resource estimations for quantum chemistry on a near-term quantum computer. Journal of Chemical Theory and Computation, 15 (9), 4764–4780. https://doi.org/10.1021/acs.jctc.9b00236
Langione, M., Tillemann-Dick, C., Kumar, A [Amit], & Taneja, V. (2019). Where will quantum computers create value—and when? https://www.bcg.com/publications/2019/quantum-computers-create-value-when . Accessed 3 June 2021
Li, S.-S., Long, G.-L., Bai, F.-S., Feng, S.-L., & Zheng, H.-Z. (2001). Quantum computing. Proceedings of the National Academy of Sciences of the United States of America, 98 (21), 11847–11848. https://doi.org/10.1073/pnas.191373698
Li, Y [Yangyang], Tian, M., Liu, G., Peng, C., & Jiao, L. (2020). Quantum optimization and quantum learning: A Survey. IEEE Access , 8 , 23568–23593. https://doi.org/10.1109/ACCESS.2020.2970105
Lorenz, R., Pearson, A., Meichanetzidis, K., Kartsaklis, D., & Coecke, B. (2021). QNLP in practice: Running compositional models of meaning on a quantum computer . http://arxiv.org/pdf/2102.12846v1 . Accessed 3 June 2021
Lycett, M. (2013). ‘Datafication’: Making sense of (big) data in a complex world. European Journal of Information Systems, 22 (4), 381–386. https://doi.org/10.1057/ejis.2013.10
Marinescu, D. C., & Marinescu, G. M. (2012). Quantum error-correcting codes. In Classical and Quantum Information (pp. 455–562). Elsevier. https://doi.org/10.1016/B978-0-12-383874-2.00005-9
Ménard, A., Ostojic, I., Patel, M., & Volz, D. (2020). A game plan for quantum computing . https://www.mckinsey.com/business-functions/mckinsey-digital/our-insights/a-game-plan-for-quantum-computing . Accessed 3 June 2021
Mendling, J., Pentland, B. T., & Recker, J. (2020). Building a complementary agenda for business process management and digital innovation. European Journal of Information Systems, 29 (3), 208–219. https://doi.org/10.1080/0960085X.2020.1755207
Mone, G. (2020). The quantum threat. Communications of the ACM, 63 (7), 12–14. https://doi.org/10.1145/3398388
Mooney, G. J., Hill, C. D., & Hollenberg, L. C. L. (2019). Entanglement in a 20-qubit superconducting quantum computer. Scientific Reports, 9 (1), 13465. https://doi.org/10.1038/s41598-019-49805-7
Motta, M., Gujarati, T. P., Rice, J. E., Kumar, A [Ashutosh], Masteran, C., Latone, J. A., Lee, E., Valeev, E. F., & Takeshita, T. Y. (2020). Quantum simulation of electronic structure with a transcorrelated Hamiltonian: Improved accuracy with a smaller footprint on the quantum computer. Physical Chemistry Chemical Physics: PCCP , 22 (42), 24270–24281. https://doi.org/10.1039/d0cp04106h
Neukart, F. (2021). Quantencomputing in der Automobilindustrie. Digitale Welt, 5 (2), 34–37. https://doi.org/10.1007/s42354-021-0334-8
Neukart, F., Compostella, G., Seidel, C., von Dollen, D., Yarkoni, S., & Parney, B. (2017). Traffic flow optimization using a quantum annealer. Frontiers in ICT, 4 , 29. https://doi.org/10.3389/fict.2017.00029
OECD. (2021). Significant shortages exist in ICT and other STEM related knowledge domains. In OECD Economic Surveys: Portugal. OECD Economic Surveys: Portugal 2021. OECD. https://doi.org/10.1787/f1155a36-en
Park, D. K., Petruccione, F., & Rhee, J.-K.K. (2019). Circuit-based quantum random access memory for classical data. Scientific Reports, 9 (1), 3949. https://doi.org/10.1038/s41598-019-40439-3
Recker, J., Lukyanenko, R., Jabbari, M., Samuel, B., & Castellanos, A. (2021). From representation to mediation: A new agenda for conceptual modeling research in a digital world. MIS Quarterly, 45 (1a), 269–300. https://doi.org/10.25300/MISQ/2021/16027
Scarani, V., Bechmann-Pasquinucci, H., Cerf, N. J., Dušek, M., Lütkenhaus, N., & Peev, M. (2009). The security of practical quantum key distribution. Reviews of Modern Physics, 81 (3), 1301–1350. https://doi.org/10.1103/RevModPhys.81.1301
Schrödinger, E. (1935). Discussion of probability relations between separated systems. Mathematical Proceedings of the Cambridge Philosophical Society, 31 (4), 555–563. https://doi.org/10.1017/S0305004100013554
Shor, P. W. (1994). Algorithms for quantum computation: discrete logarithms and factoring. In Proceedings of the 35th Annual Symposium on Foundations of Computer Science. IEEE Computer Society , 124–134. https://doi.org/10.1109/SFCS.1994.365700
Shor, P. W. (1995). Scheme for reducing decoherence in quantum computer memory. Physical Review a, 52 (4), R2493. https://doi.org/10.1103/PhysRevA.52.R2493
Steane, A. (1998). Quantum computing. Reports on Progress in Physics, 61 (2), 117–173. https://doi.org/10.1088/0034-4885/61/2/002
vom Brocke, J., Baier, M.-S., Schmiedel, T., Stelzl, K., Röglinger, M., & Wehking, C. (2021). Context-aware business process management. Business & Information Systems Engineering, 63 (5), 533–550. https://doi.org/10.1007/s12599-021-00685-0
Yarkoni, S., Leib, M., Skolik, A., Streif, M., Neukart, F., & von Dollen, D. (2019). Volkswagen and quantum computing: An industrial perspective. Digitale Welt, 3 (2), 34–37. https://doi.org/10.1007/s42354-019-0166-y
Zhong, H.‑S., Wang, H., Deng, Y.‑H., Chen, M.‑C., Peng, L.‑C., Luo, Y.‑H., Qin, J., Wu, D., Ding, X., Hu, Y., Hu, P., Yang, X.‑Y., Zhang, W.‑J., Li, H., Li, Y [Yuxuan], Jiang, X., Gan, L., Yang, G., You, L., Wang, Z., Li, L., Liu, N.-L., Lu, C.-Y., & Pan, J.‑W. (2020). Quantum computational advantage using photons. Science (New York, N.Y.) , 370 (6523), 1460–1463. https://doi.org/10.1126/science.abe8770
Ziegler, M., & Leonhardt, T. (2019). Quantum computing. Applied now. Digitale Welt, 3 (2), 50–52. https://doi.org/10.1007/s42354-019-0170-2
Download references
Acknowledgements
We would also like to thank all the interviewees for their help and especially Rajiv Krishnakumar for his support in revising this article.
Open access funding provided by NTNU Norwegian University of Science and Technology (incl St. Olavs Hospital - Trondheim University Hospital).
Author information
Authors and affiliations.
Institute of Information Management, University of St.Gallen, Müller-Friedberg-Strasse 8, 9000, St.Gallen, Switzerland
Roman Rietsche & Jan-Marco Leimeister
Norwegian University of Science and Technology, Høgskoleringen 1, 7491, Trondheim, Norway
Christian Dremel
Department of Electrical Engineering and Computer Science, Massachusetts Institute of Technology, 77 Massachusetts Ave., MA, 02139, Cambridge, United States of America
Samuel Bosch
University of St.Gallen, Müller-Friedberg-Strasse 8, 9000, St.Gallen, Switzerland
Léa Steinacker
Institute for Media and Communications Managment, University of St.Gallen, Müller-Friedberg-Srasse 8, 9000, St.Gallen, Switzerland
Miriam Meckel
You can also search for this author in PubMed Google Scholar
Corresponding author
Correspondence to Christian Dremel .
Additional information
Responsible Editor: Rainer Alt
Publisher's note
Springer Nature remains neutral with regard to jurisdictional claims in published maps and institutional affiliations.
Rights and permissions
Open Access This article is licensed under a Creative Commons Attribution 4.0 International License, which permits use, sharing, adaptation, distribution and reproduction in any medium or format, as long as you give appropriate credit to the original author(s) and the source, provide a link to the Creative Commons licence, and indicate if changes were made. The images or other third party material in this article are included in the article's Creative Commons licence, unless indicated otherwise in a credit line to the material. If material is not included in the article's Creative Commons licence and your intended use is not permitted by statutory regulation or exceeds the permitted use, you will need to obtain permission directly from the copyright holder. To view a copy of this licence, visit http://creativecommons.org/licenses/by/4.0/ .
Reprints and permissions
About this article
Rietsche, R., Dremel, C., Bosch, S. et al. Quantum computing. Electron Markets 32 , 2525–2536 (2022). https://doi.org/10.1007/s12525-022-00570-y
Download citation
Received : 08 January 2022
Accepted : 27 June 2022
Published : 05 August 2022
Issue Date : December 2022
DOI : https://doi.org/10.1007/s12525-022-00570-y
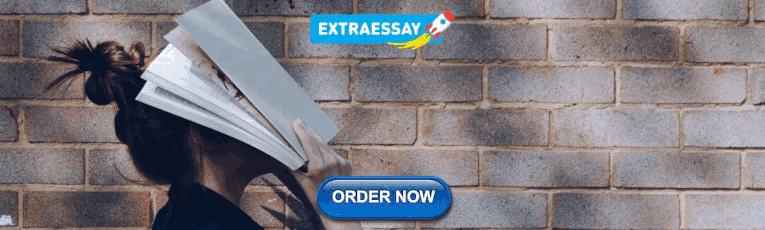
Share this article
Anyone you share the following link with will be able to read this content:
Sorry, a shareable link is not currently available for this article.
Provided by the Springer Nature SharedIt content-sharing initiative
- Quantum physics
- Cloud computing
- Emerging technology
- Information systems
JEL Classification
- Find a journal
- Publish with us
- Track your research
Thank you for visiting nature.com. You are using a browser version with limited support for CSS. To obtain the best experience, we recommend you use a more up to date browser (or turn off compatibility mode in Internet Explorer). In the meantime, to ensure continued support, we are displaying the site without styles and JavaScript.
- View all journals
- Explore content
- About the journal
- Publish with us
- Sign up for alerts
- Open access
- Published: 11 May 2021
Power of data in quantum machine learning
- Hsin-Yuan Huang 1 , 2 , 3 ,
- Michael Broughton 1 ,
- Masoud Mohseni 1 ,
- Ryan Babbush 1 ,
- Sergio Boixo ORCID: orcid.org/0000-0002-1090-7584 1 ,
- Hartmut Neven 1 &
- Jarrod R. McClean ORCID: orcid.org/0000-0002-2809-0509 1
Nature Communications volume 12 , Article number: 2631 ( 2021 ) Cite this article
62k Accesses
272 Citations
137 Altmetric
Metrics details
- Computer science
- Quantum information
The use of quantum computing for machine learning is among the most exciting prospective applications of quantum technologies. However, machine learning tasks where data is provided can be considerably different than commonly studied computational tasks. In this work, we show that some problems that are classically hard to compute can be easily predicted by classical machines learning from data. Using rigorous prediction error bounds as a foundation, we develop a methodology for assessing potential quantum advantage in learning tasks. The bounds are tight asymptotically and empirically predictive for a wide range of learning models. These constructions explain numerical results showing that with the help of data, classical machine learning models can be competitive with quantum models even if they are tailored to quantum problems. We then propose a projected quantum model that provides a simple and rigorous quantum speed-up for a learning problem in the fault-tolerant regime. For near-term implementations, we demonstrate a significant prediction advantage over some classical models on engineered data sets designed to demonstrate a maximal quantum advantage in one of the largest numerical tests for gate-based quantum machine learning to date, up to 30 qubits.
Similar content being viewed by others
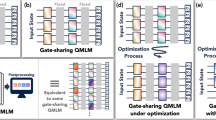
Generalization in quantum machine learning from few training data
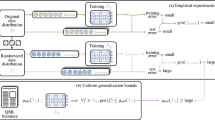
Understanding quantum machine learning also requires rethinking generalization
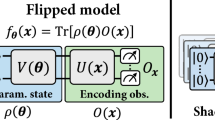
Shadows of quantum machine learning
Introduction.
As quantum technologies continue to rapidly advance, it becomes increasingly important to understand which applications can benefit from the power of these devices. At the same time, machine learning on classical computers has made great strides, revolutionizing applications in image recognition, text translation, and even physics applications, with more computational power leading to ever increasing performance 1 . As such, if quantum computers could accelerate machine learning, the potential for impact is enormous.
At least two paths towards quantum enhancement of machine learning have been considered. First, motivated by quantum applications in optimization 2 , 3 , 4 , the power of quantum computing could, in principle, be used to help improve the training process of existing classical models 5 , 6 , or enhance inference in graphical models 7 . This could include finding better optima in a training landscape or finding optima with fewer queries. However, without more structure known in the problem, the advantage along these lines may be limited to quadratic or small polynomial speedups 8 , 9 .
The second vein of interest is the possibility of using quantum models to generate correlations between variables that are inefficient to represent through classical computation. The recent success both theoretically and experimentally for demonstrating quantum computations beyond classical tractability can be taken as evidence that quantum computers can sample from probability distributions that are exponentially difficult to sample from classically 10 , 11 . If these distributions were to coincide with real-world distributions, this would suggest the potential for significant advantage. This is typically the type of advantage that has been sought in recent work on both quantum neural networks 12 , 13 , 14 , which seek to parameterize a distribution through some set of adjustable parameters, and quantum kernel methods 15 that use quantum computers to define a feature map that maps classical data into the quantum Hilbert space. The justification for the capability of these methods to exceed classical models often follows similar lines as refs. 10 , 11 or quantum simulation results. That is, if the model leverages a quantum circuit that is hard to sample results from classically, then there is potential for a quantum advantage.
In this work, we show quantitatively how this picture is incomplete in machine learning (ML) problems where some training data are provided. The provided data can elevate classical models to rival quantum models, even when the quantum circuits generating the data are hard to compute classically. We begin with a motivating example and complexity-theoretic argument showing how classical algorithms with data can match quantum output. Following this, we provide rigorous prediction error bounds for training classical and quantum ML methods based on kernel functions 15 , 16 , 17 , 18 , 19 , 20 , 21 , 22 , 23 , 24 to learn quantum mechanical models. We focus on kernel methods, as they not only provide provable guarantees, but are also very flexible in the functions they can learn. For example, recent advancements in theoretical machine learning show that training neural networks with large hidden layers is equivalent to training an ML model with a particular kernel, known as the neural tangent kernel 19 , 20 , 21 . Throughout, when we refer to classical ML models related to our theoretical developments, we will be referring to ML models that can be easily associated with a kernel, either explicitly as in kernel methods, or implicitly as in the neural tangent kernels. However, in the numerical section, we will also include performance comparisons to methods where direct association of a kernel is challenging, such as random forest methods. In the quantum case, we will also show how quantum ML based on kernels can be made equivalent to training an infinite depth quantum neural network.
We use our prediction error bounds to devise a flowchart for testing potential quantum prediction advantage, the separation between prediction errors of quantum and classical ML models for a fixed amount of training data. The most important test is a geometric difference between kernel functions defined by classical and quantum ML. Formally, the geometric difference is defined by the closest efficient classical ML model. In practice, one should consider the geometric difference with respect to a suite of optimized classical ML models. If the geometric difference is small, then a classical ML method is guaranteed to provide similar or better performance in prediction on the dataset, independent of the function values or labels. Hence this represents a powerful, function independent prescreening that allows one to evaluate if there is any possibility of better performance. On the other hand, if the geometry differs greatly, we show both the existence of a dataset that exhibits large prediction advantage using the quantum ML model and how one can construct it efficiently. While the tools we develop could be used to compare and construct hard classical models like hash functions, we enforce restrictions that allow us to say something about a quantum separation. In particular, the feature map will be white box, in that a quantum circuit specification is available for the ideal feature map, and that feature map can be made computationally hard to evaluate classically. A constructive example of this is a discrete log feature map, where a provable separation for our kernel is given in Supplementary Section 11 . Additionally, the minimum over classical models means that classical hash functions are reproduced formally by definition.
Moreover, application of these tools to existing models in the literature rules many of them out immediately, providing a powerful sieve for focusing development of new data encodings. Following these constructions, in numerical experiments, we find that a variety of common quantum models in the literature perform similarly or worse than classical ML on both classical and quantum datasets due to a small geometric difference. The small geometric difference is a consequence of the exponentially large Hilbert space employed by existing quantum models, where all inputs are too far apart. To circumvent the setback, we propose an improvement, which enlarges the geometric difference by projecting quantum states embedded from classical data back to approximate classical representation 25 , 26 , 27 . With the large geometric difference endowed by the projected quantum model, we are able to construct engineered datasets to demonstrate large prediction advantage over common classical ML models in numerical experiments up to 30 qubits. Despite our constructions being based on methods with associated kernels, we find empirically that the prediction advantage remains robust across tested classical methods, including those without an easily determined kernel. This opens the possibility to use a small quantum computer to generate efficiently verifiable machine learning problems that could be challenging for classical ML models.
Setup and motivating example
We begin by setting up the problems and methods of interest for classical and quantum models, and then provide a simple motivating example for studying how data can increase the power of classical models on quantum data. The focus will be a supervised learning task with a collection of N training examples {( x i , y i )}, where x i is the input data and y i is an associated label or value. We assume that x i are sampled independently from a data distribution \({\mathcal{D}}\) .
In our theoretical analysis, we will consider \({y}_{i}\in {\mathbb{R}}\) to be generated by some quantum model. In particular, we consider a continuous encoding unitary that maps a classical input data x i into quantum state \(\left|{x}_{i}\right\rangle ={U}_{\text{enc}}({x}_{i}){\left|0\right\rangle }^{\otimes n}\) and refer to the corresponding density matrix as ρ ( x i ). The expressive power of these embeddings have been investigated from a functional analysis point of view 28 , 29 ; however, the setting where data are provided requires special attention. The encoding unitary is followed by a unitary U QNN ( θ ). We then measure an observable O after the quantum neural network. This produces the label/value for input x i given as \({y}_{i}=f({x}_{i})=\left\langle {x}_{i}\right|{U}_{\,\text{QNN}}^{\dagger }O{U}_{\text{QNN}}\left|{x}_{i}\right\rangle \) . The quantum model considered here is also referred to as a quantum neural network (QNN) in the literature 14 , 30 . The goal is to understand when it is easy to predict the function f ( x ) by training classical/quantum machine learning models.
With notation in place, we turn to a simple motivating example to understand how the availability of data in machine learning tasks can change computational hardness. Consider data points \({\{{{\bf{x}}}_{i}\}}_{i = 1}^{N}\) that are p -dimensional classical vectors with ∣ ∣ x i ∣ ∣ 2 = 1, and use amplitude encoding 31 , 32 , 33 to encode the data into an n -qubit state \(\left|{{\bf{x}}}_{i}\right\rangle =\mathop{\sum }\nolimits_{k = 1}^{p}{x}_{i}^{k}\left|k\right\rangle \) , where \({x}_{i}^{k}\) is the individual coordinate of the vector x i . If U QNN is a time-evolution under a many-body Hamiltonian, then the function \(f({\bf{x}})=\left\langle {\bf{x}}\right|{U}_{\,\text{QNN}}^{\dagger }O{U}_{\text{QNN}}\left|{\bf{x}}\right\rangle \) is in general hard to compute classically 34 , even for a single input state. In particular, we have the following proposition showing that if a classical algorithm can compute f ( x ) efficiently, then quantum computers will be no more powerful than classical computers; see Supplementary Section 1 for a proof.
Proposition 1
If a classical algorithm without training data can compute f ( x ) efficiently for any U QNN and O , then BPP = BQP.
Nevertheless, it is incorrect to conclude that training a classical model from data to learn this evolution is hard. To see this, we write out the expectation value as
which is a quadratic function with p 2 coefficients \({B}_{kl}=\left\langle k\right|{U}_{\,\text{QNN}}^{\dagger }O{U}_{\text{QNN}}\left|l\right\rangle \) . Using the theory developed later in this work, we can show that, for any U QNN and O , training a specific classical ML model on a collection of N training examples {( x i , y i = f ( x i ))} would give rise to a prediction model h ( x i ) with
for a constant c > 0. We refer to Supplementary Section 1 for the proof of this result. Hence, with N ∝ p 2 / ϵ 2 training data, one can train a classical ML model to predict the function f ( x ) up to an additive prediction error ϵ . This elevation of classical models through some training samples is illustrative of the power of data. In Supplementary Section 2 , we give a rigorous complexity-theoretic argument on the computational power provided by data. A cartoon depiction of the complexity separation induced by data is provided in Fig. 1 (a).
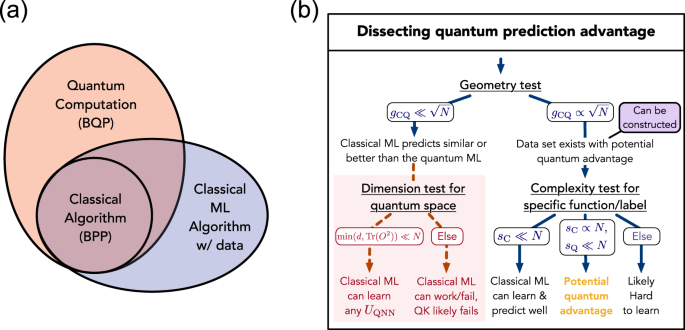
a We cartoon the separation between problem complexities that are created by the addition of data to a problem. Classical algorithms that can learn from data define a complexity class that can solve problems beyond classical computation (BPP), but it is still expected that quantum computation can efficiently solve problems that classical ML algorithms with data cannot. A rigorous definition and proof for the separation between classical algorithms that can learn from data and BPP/BQP is given in Supplementary Section 2 . b The flowchart we develop for understanding the potential for quantum prediction advantage. N samples of data from a potentially infinite depth QNN made with encoding and function circuits U enc and U QNN are provided as input along with quantum and classical methods with associated kernels. Tests are given as functions of N to emphasize the role of data in the possibility of a prediction advantage. One can first evaluate a geometric quantity g CQ that measures the possibility of an advantageous quantum/classical prediction separation without yet considering the actual function to learn. We show how one can efficiently construct an adversarial function that saturates this limit if the test is passed, otherwise the classical approach is guaranteed to match performance for any function of the data. To subsequently consider the actual function provided, a label/function-specific test may be run using the model complexities s C and s Q . If one specifically uses the quantum kernel (QK) method, the red dashed arrows can evaluate if all possible choices of U QNN lead to an easy classical function for the chosen encoding of the data.
While this simple example makes the basic point that sufficient data can change complexity considerations, it perhaps opens more questions than it answers. For example, it uses a rather weak encoding into amplitudes and assumes one has access to an amount of data that is on par with the dimension of the model. The more interesting cases occur if we strengthen the data encoding, include modern classical ML models, and consider the number of data N much less than the dimension of the model. These more interesting cases are the ones we quantitatively answer.
Our primary interest will be ML algorithms that are much stronger than fitting a quadratic function and the input data are provided in more interesting ways than an amplitude encoding. In this work, we focus on both classical and quantum ML models based on kernel functions k ( x i , x j ). At a high level, a kernel function can be seen as a measure of similarity, if k ( x i , x j ) is large when x i and x j are close. When considered for finite input data, a kernel function may be represented as a matrix K i j = k ( x i , x j ) and the conditions required for kernel methods are satisfied when the matrix representation is Hermitian and positive semi-definite.
A given kernel function corresponds to a nonlinear feature mapping ϕ ( x ) that maps x to a possibly infinite-dimensional feature space, such that \(k({x}_{i},{x}_{j})=\phi {({x}_{i})}^{\dagger }\phi ({x}_{j})\) . This is the basis of the so-called “kernel trick” where intricate and powerful maps ϕ ( x i ) can be implemented through the evaluation of relatively simple kernel functions k . As a simple case, in the example above, using a kernel of k ( x i , x j ) = ∣ 〈 x i ∣ x j 〉 ∣ 2 corresponds to a feature map \(\phi ({x}_{i})={\sum }_{kl}{x}_{i}^{k* }{x}_{i}^{l}\left|k\right\rangle \otimes \left|l\right\rangle \) which is capable of learning quadratic functions in the amplitudes. In kernel based ML algorithms, the trained model can always be written as h ( x ) = w † ϕ ( x ) where w is a vector in the feature space defined by the kernel. For example, training a convolutional neural network with large hidden layers 19 , 35 is equivalent to using a corresponding neural tangent kernel k CNN . The feature map ϕ CNN for the kernel k CNN is a nonlinear mapping that extracts all local properties of x 35 . In quantum mechanics, similarly a kernel function can be defined using the native geometry of the quantum state space \(\left|x\right\rangle \) . For example, we can define the kernel function as 〈 x i ∣ x j 〉 or ∣ 〈 x i ∣ x j 〉 ∣ 2 . Using the output from this kernel in a method like a classical support vector machine 16 defines the quantum kernel method.
A wide class of functions can be learned with a sufficiently large amount of data by using the right kernel function k . For example, in contrast to the perhaps more natural kernel, 〈 x i ∣ x j 〉, the quantum kernel k Q ( x i , x j ) = ∣ 〈 x i ∣ x j 〉 ∣ 2 = Tr( ρ ( x i ) ρ ( x j )) can learn arbitrarily deep quantum neural network U QNN that measures any observable O (shown in Supplementary Section 3 ), and the Gaussian kernel, \({k}^{\gamma }({x}_{i},{x}_{j})=\exp (-\gamma | | {x}_{i}-{x}_{j}| {| }^{2})\) with hyperparameter γ , can learn any continuous function in a compact space 36 , which includes learning any QNN. Nevertheless, the required amount of data N to achieve a small prediction error could be very large in the worst case. Although we will work with other kernels defined through a quantum space, due both to this expressive property and terminology of past work, we will refer to \({k}^{{\rm{Q}}}({x}_{i},{x}_{j})=\,\text{Tr}\,\big[\rho ({x}_{i})\rho ({x}_{j})\big]\) as the quantum kernel method throughout this work, which is also the definition given in 15 .
Testing quantum advantage
We now construct our more general framework for assessing the potential for quantum prediction advantage in a machine learning task. Beginning from a general result, we build both intuition and practical tests based on the geometry of the learning spaces. This framework is summarized in Fig. 1 .
Our foundation is a general prediction error bound for training classical/quantum ML models to predict some quantum model defined by f ( x ) = Tr( O U ρ ( x )) derived from concentration inequalities, where \({O}^{U}={U}_{\,\text{QNN}}^{\dagger }O{U}_{\text{QNN}}\) . Suppose we have obtained N training examples {( x i , y i = f ( x i ))}. After training on this data, there exists an ML algorithm that outputs h ( x ) = w † ϕ ( x ) using kernel \(k({x}_{i},{x}_{j})={K}_{ij}=\phi {({x}_{i})}^{\dagger }\phi ({x}_{j})\) , which has a simplified prediction error bounded by
for a constant c > 0 and N independent samples from the data distribution \({\mathcal{D}}\) . We note here that this and all subsequent bounds have a key dependence on the quantity of data N , reflecting the role of data to improve prediction performance. Due to a scaling freedom between αϕ ( x ) and w / α , we have assumed \(\mathop{\sum }\nolimits_{i = 1}^{N}\phi {({x}_{i})}^{\dagger }\phi ({x}_{i})=\,\text{Tr}\,(K)=N\) . A derivation of this result is given in Supplementary Section 4 .
Given this core prediction error bound, we now seek to understand its implications. The main quantity that determines the prediction error is
The quantity s K ( N ) is equal to the model complexity of the trained function h ( x ) = w † ϕ ( x ), where s K ( N ) = ∣ ∣ w ∣ ∣ 2 = w † w after training. A smaller value of s K ( N ) implies better generalization to new data x sampled from the distribution \({\mathcal{D}}\) . Intuitively, s K ( N ) measures whether the closeness between x i , x j defined by the kernel function k ( x i , x j ) matches well with the closeness of the observable expectation for the quantum states ρ ( x i ), ρ ( x j ), recalling that a larger kernel value indicates two points are closer. The computation of s K ( N ) can be performed efficiently on a classical computer by inverting an N × N matrix K after obtaining the N values Tr( O U ρ ( x i )) by performing order N experiments on a physical quantum device. The time complexity scales at most as order N 3 . Due to the connection between w † w and the model complexity, a regularization term w † w is often added to the optimization problem during the training of h ( x ) = w † ϕ ( x ), see e.g., refs. 16 , 37 , 38 . Regularization prevents s K ( N ) from becoming too large at the expense of not completely fitting the training data. A detailed discussion and proof under regularization is given in Supplementary Section 4 and 6 .
The prediction error upper bound can often be shown to be asymptotically tight by proving a matching lower bound. As an example, when k ( x i , x j ) is the quantum kernel Tr( ρ ( x i ) ρ ( x j )), we can deduce that s K ( N ) ≤ Tr( O 2 ) hence one would need a number of data N scaling as Tr( O 2 ). In Supplementary Section 8 , we give a matching lower bound showing that a scaling of Tr( O 2 ) is unavoidable if we assume a large Hilbert space dimension. This lower bound holds for any learning algorithm and not only for quantum kernel methods. The lower bound proof uses mutual information analysis and could easily extend to other kernels. This proof strategy is also employed extensively in a follow-up work 39 to devise upper and lower bounds for classical and quantum ML in learning quantum models. Furthermore, not only are the bounds asymptotically tight, in numerical experiments given in Supplementary Section 13 we find that the prediction error bound also captures the performance of other classical ML models not based on kernels where the constant factors are observed to be quite modest.
Given some set of data, if s K ( N ) is found to be small relative to N after training for a classical ML model, this quantum model f ( x ) can be predicted accurately even if f ( x ) is hard to compute classically for any given x . In order to formally evaluate the potential for quantum prediction advantage generally, one must take s K ( N ) to be the minimal over efficient classical models. However, we will be more focused on minimally attainable values over a reasonable set of classical methods with tuned hyperparameters. This prescribes an effective method for evaluating potential quantum advantage in practice, and already rules out a considerable number of examples from the literature.
From the bound, we can see that the potential advantage for one ML algorithm defined by K 1 to predict better than another ML algorithm defined by K 2 depends on the largest possible separation between \({s}_{{K}^{1}}\) and \({s}_{{K}^{2}}\) for a dataset. The separation can be characterized by defining an asymmetric geometric difference that depends on the dataset, but is independent of the function values or labels. Hence evaluating this quantity is a good first step in understanding if there is a potential for quantum advantage, as shown in Fig. 1 . This quantity is defined by
where ∣ ∣ . ∣ ∣ ∞ is the spectral norm of the resulting matrix and we assume Tr( K 1 ) = Tr( K 2 ) = N . One can show that \({s}_{{K}^{1}}\le {g}_{12}^{2}{s}_{{K}^{2}}\) , which implies the prediction error bound \(c\sqrt{{s}_{{K}^{1}}/N}\le c{g}_{12}\sqrt{{s}_{{K}^{2}}/N}\) . A detailed derivation is given in Supplementary Section C and an illustration of g 12 can be found in Fig. 2 . The geometric difference g ( K 1 ∣ ∣ K 2 ) can be computed on a classical computer by performing a singular value decomposition of the N × N matrices K 1 and K 2 . Standard numerical analysis packages 40 provide highly efficient computation of a singular value decomposition in time at most order N 3 . Intuitively, if K 1 ( x i , x j ) is small/large when K 2 ( x i , x j ) is small/large, then the geometric difference g 12 is a small value ~1, where g 12 grows as the kernels deviate.
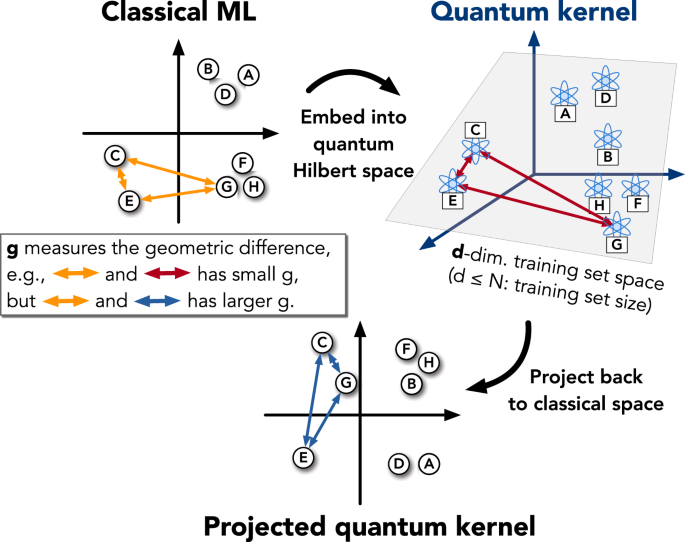
The letters A, B, ... represent data points { x i } in different spaces with arrows representing the similarity measure (kernel function) between data. The geometric difference g is a difference between similarity measures (arrows) in different ML models and d is an effective dimension of the dataset in the quantum Hilbert space.
To see more explicitly how the geometric difference allows one to make statements about the possibility for one ML model to make different predictions from another, consider the geometric difference g CQ = g ( K C ∣ ∣ K Q ) between a classical ML model with kernel k C ( x i , x j ) and a quantum ML model, e.g., with k Q ( x i , x j ) = Tr( ρ ( x i ) ρ ( x j )). If g CQ is small, because
the classical ML model will always have a similar or better model complexity s K ( N ) compared to the quantum ML model. This implies that the prediction performance for the classical ML will likely be competitive or better than the quantum ML model, and one is likely to prefer using the classical model. This is captured in the first step of our flowchart in Fig. 1 .
In contrast, if g CQ is large we show that there exists a dataset with \({s}_{{\rm{C}}}={g}_{{\rm{CQ}}}^{2}{s}_{{\rm{Q}}}\) with the quantum model exhibiting superior prediction performance. An efficient method to explicitly construct such a maximally divergent dataset is given in Supplementary Section 7 and a numerical demonstration of the stability of this separation is provided in the next section. While a formal statement about classical methods generally requires defining it overall efficient classical methods, in practice, we consider g CQ to be the minimum geometric difference among a suite of optimized classical ML models. Our engineered approach minimizes this value as a hyperparameter search to find the best classical adversary, and shows remarkable robustness across classical methods including those without an associated kernel, such as random forests 41 .
In the specific case of the quantum kernel method with \({K}_{ij}^{Q}={k}^{{\rm{Q}}}({x}_{i},{x}_{j})=\,\text{Tr}\,(\rho ({x}_{i})\rho ({x}_{j}))\) , we can gain additional insights into the model complexity s K , and sometimes make conclusions about classically learnability for all possible U QNN for the given encoding of the data. Let us define vec( X ) for a Hermitian matrix X to be a vector containing the real and imaginary part of each entry in X . In this case, we find \({s}_{Q}={\rm{vec}}{({O}^{U})}^{T}{P}_{Q}{\rm{vec}}({O}^{U})\) , where P Q is the projector onto the subspace formed by {vec( ρ ( x 1 )), …, vec( ρ ( x N ))}. We highlight
which defines the effective dimension of the quantum state space spanned by the training data. An illustration of the dimension d can be found in Fig. 1 . Because P Q is a projector and has eigenvalues 0 or 1, \({s}_{{\rm{Q}}}\le \min (d,{\rm{vec}}{({O}^{U})}^{T}{\rm{vec}}({O}^{U}))=\min (d,\,\text{Tr}\,({O}^{2}))\) assuming ∣ ∣ O ∣ ∣ ∞ ≤ 1. Hence in the case of the quantum kernel method, the prediction error bound may be written as
A detailed derivation is given in Supplementary Section A. We can also consider the approximate dimension d , where small eigenvalues in K Q are truncated, by incurring a small training error. After obtaining K Q from a quantum device, the dimension d can be computed efficiently on a classical machine by performing a singular value decomposition on the N × N matrix K Q . Estimation of Tr( O 2 ) can be performed by sampling random states \(\left|\psi \right\rangle \) from a quantum 2-design, measuring O on \(\left|\psi \right\rangle \) , and performing statistical analysis on the measurement data 25 . This prediction error bound shows that a quantum kernel method can learn any U QNN when the dimension of the training set space d or the squared Frobenius norm of observable Tr( O 2 ) is much smaller than the amount of data N . In Supplementary Section 8 , we show that quantum kernel methods are optimal for learning quantum models with bounded Tr( O 2 ) as they saturate the fundamental lower bound. However, in practice, most observables, such as Pauli operators, will have exponentially large Tr( O 2 ), so the central quantity is the dimension d . Using the prediction error bound for the quantum kernel method, if both g CQ and \(\min (d,\,\text{Tr}\,({O}^{2}))\) are small, then a classical ML would also be able to learn any U QNN . In such a case, one must conclude that the given encoding of the data is classically easy, and this cannot be affected by an arbitrarily deep U QNN . This constitutes the bottom left part of our flowchart in Fig. 1 .
Ultimately, to see a prediction advantage in a particular dataset with specific function values/labels, we need a large separation between s C and s Q . This happens when the inputs x i , x j considered close in a quantum ML model are actually close in the target function f ( x ), but are far in classical ML. This is represented as the final test in Fig. 1 and the methodology here outlines how this result can be achieved in terms of its more essential components.
Projected quantum kernels
In addition to analyzing existing quantum models, the analysis approach introduced also provides suggestions for new quantum models with improved properties, which we now address here. For example, if we start with the original quantum kernel, when the effective dimension d is large, kernel Tr( ρ ( x i ) ρ ( x j )), which is based on a fidelity-type metric, will regard all data to be far from each other and the kernel matrix K Q will be close to identity. This results in a small geometric difference g CQ leading to classical ML models being competitive or outperforming the quantum kernel method. In Supplementary Section 9 , we present a simple quantum model that requires an exponential amount of samples to learn using the quantum kernel Tr( ρ ( x i ) ρ ( x j )), but only needs a linear number of samples to learn using a classical ML model.
To circumvent this setback, we propose a family of projected quantum kernels as a solution. These kernels work by projecting the quantum states to an approximate classical representation, e.g., using reduced physical observables or classical shadows 25 , 27 , 42 , 43 , 44 . Even if the training set space has a large dimension d ~ N , the projection allows us to reduce to a low-dimensional classical space that can generalize better. Furthermore, by going through the exponentially large quantum Hilbert space, the projected quantum kernel can be challenging to evaluate without a quantum computer. In numerical experiments, we find that the classical projection increases rather than decreases the geometric difference with classical ML models. These constructions will be the foundation of our best performing quantum method later.
One of the simplest forms of projected quantum kernel is to measure the one-particle reduced density matrix (1-RDM) on all qubits for the encoded state, ρ k ( x i ) = Tr j ≠ k [ ρ ( x i )], then define the kernel as
This kernel defines a feature map function in the 1-RDM space that is capable of expressing arbitrary functions of powers of the 1-RDMs of the quantum state. From nonintuitive results in density functional theory, we know even one body densities can be sufficient for determining exact ground state 45 and time-dependent 46 properties of many-body systems under modest assumptions. In Supplementary Section 10 , we provide examples of other projected quantum kernels. This includes an efficient method for computing a kernel function that contains all orders of RDMs using local randomized measurements and the formalism of classical shadows 25 . The classical shadow formalism allows efficient construction of RDMs from very few measurements. In Supplementary Section 11 , we show that projected versions of quantum kernels lead to a simple and rigorous quantum speed-up in a recently proposed learning problem based on discrete logarithms 24 .
Numerical studies
We now provide numerical evidence up to 30 qubits that supports our theory on the relation between the dimension d , the geometric difference g , and the prediction performance. Using the projected quantum kernel, the geometric difference g is much larger and we see the strongest empirical advantage of a scalable quantum model on quantum datasets to date. These are the largest combined simulation and analysis in digital quantum machine learning that we are aware of, and make use of the TensorFlow and TensorFlow-Quantum package 47 , reaching a peak throughput of up to 1.1 quadrillion floating point operations per second (petaflop/s). Trends of ~300 teraflop/s for quantum simulation and 800 teraflop/s for classical analysis were observed up to the maximum experiment size with the overall floating point operations across all experiments totalling ~2 quintillion (exaflop).
In order to mimic a data distribution that pertains to real-world data, we conduct our experiments around the fashion-MNIST dataset 48 , which is an image classification for distinguishing clothing items, and is more challenging than the original digit-based MNIST source 49 . We preprocess the data using principal component analysis 50 to transform each image into an n -dimensional vector. The same data are provided to the quantum and classical models, where in the classical case the data is the n -dimensional input vector, and the quantum case uses a given circuit to embed the n -dimensional vector into the space of n qubits. For quantum embeddings, we explore three options, E1 is a separable rotation circuit 32 , 51 , 52 , E2 is an IQP-type embedding circuit 15 , and E3 is a Hamiltonian evolution circuit, with explicit constructions in Supplementary Section 12 .
For the classical ML task (C), the goal is to correctly identify the images as shirts or dresses from the original dataset. For the quantum ML tasks, we use the same fashion-MINST source data and embeddings as above, but take as function values the expectation value of a local observable that has been evolved under a quantum neural network resembling the Trotter evolution of 1D-Heisenberg model with random couplings. In these cases, the embedding is taken as part of the ground truth, so the resulting function will be different depending on the quantum embedding. For these ML tasks, we compare against the best performing model from a list of standard classical ML algorithms with properly tuned hyperparameters (see Supplementary Section 12 for details).
In Fig. 3 , we give a comparison between the prediction performance of classical and quantum ML models. One can see that not only do classical ML models perform best on the original classical dataset, the prediction performance for the classical methods on the quantum datasets is also very competitive and can even outperform existing quantum ML models despite the quantum ML models having access to the training embedding while the classical methods do not. The performance of the classical ML model is especially strong on Dataset (Q, E1) and Dataset (Q, E2). This elevation of the classical performance is evidence of the power of data. Moreover, this intriguing behavior and the lack of quantum advantage may be explained by considering the effective dimension d and the geometric difference g following our theoretical constructions. From Fig. 3 a, we can see that the dimension d of the original quantum state space grows rather quickly, and the geometric difference g becomes small as the dimension becomes too large ( d ∝ N ) for the standard quantum kernel. The saturation of the dimension coincides with the decreasing and statistical fluctuations in performance seen in Fig. 4 . Moreover, given poor ML performance a natural instinct is to throw more resources at the problem, e.g., more qubits, but as demonstrated here, doing this for naïve quantum kernel methods is likely to lead to tiny inner products and even worse performance. In contrast, the projected quantum space has a low dimension even when d grows, and yields a higher geometric difference g for all embeddings and system sizes. Our methodology predicts that, when g is small, classical ML model will be competitive or outperform the quantum ML model. This is verified in Fig. 3 b for both the original and projected quantum kernel, where a small geometric difference g leads to a very good performance of classical ML models and no large quantum advantage can be seen. Only when the geometric difference g is large (projected kernel method with embedding E3) can we see some mild advantage over the best classical method. This result holds disregarding any detail of the quantum evolution we are trying to learn, even for ones that are hard to simulate classically.
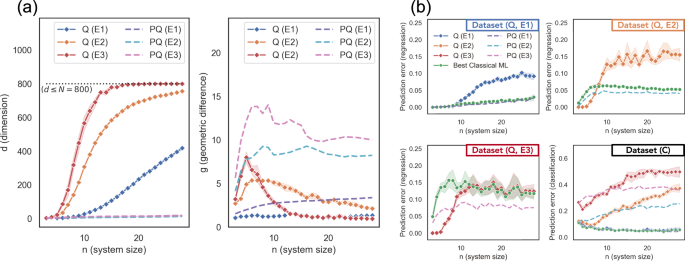
The shaded regions are the standard deviation over 10 independent runs and n is the number of qubits in the quantum encoding and dimension of the input for the classical encoding. a The approximate dimension d and the geometric difference g with classical ML models for quantum kernel (Q) and projected quantum kernel (PQ) under different embeddings and system sizes n . b Prediction error (lower is better) of the quantum kernel method (Q), projected quantum kernel method (PQ), and classical ML models on classical (C) and quantum (Q) datasets with number of data N = 600. As d grows too large, the geometric difference g for quantum kernel becomes small. We see that small geometric difference g always results in classical ML being competitive or outperforming the quantum ML model. When g is large, there is a potential for improvement over classical ML. For example, projected quantum kernel improves upon the best classical ML in Dataset (Q, E3).
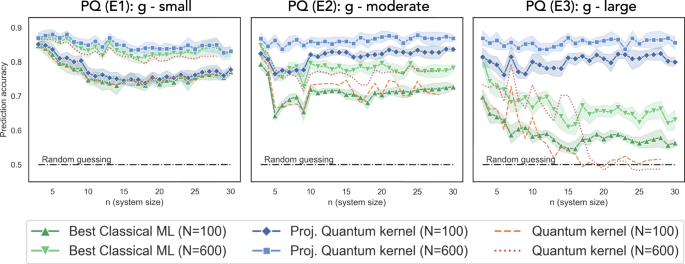
A label function is engineered to match the geometric difference g (C ∣ ∣ PQ) between projected quantum kernel and classical approaches, demonstrating a significant gap between quantum and the best classical models up to 30 qubits when g is large. We consider the best performing classical ML models among Gaussian SVM, linear SVM, Adaboost, random forest, neural networks, and gradient boosting. We only report the accuracy of the quantum kernel method up to system size n = 28 due to the high simulation cost and the inferior performance.
In order to push the limits of separation between quantum and classical approaches in a learning setting, we now consider a set of engineered datasets with function values designed to saturate the geometric inequality \({s}_{{\rm{C}}}\le g{({K}^{{\rm{C}}}| | {K}^{{\rm{PQ}}})}^{2}{s}_{{\rm{PQ}}}\) between classical ML models with associated kernels and the projected quantum kernel method. In particular, we design the dataset such that s PQ = 1 and \({s}_{{\rm{C}}}=g{({K}^{{\rm{C}}}| | {K}^{{\rm{PQ}}})}^{2}\) . Recall from Eq. ( 3 ), this dataset will hence show the largest separation in the prediction error bound \(\sqrt{s(N)/N}\) . The engineered dataset is constructed via a simple eigenvalue problem with the exact procedure described in Supplementary Section 7 and the results are shown in Fig. 4 . As the quantum nature of the encoding increases from E1 to E3, corresponding to increasing g , the performance of both the best classical methods and the original quantum kernel decline precipitously. The advantage of the projected quantum kernel closely follows the geometric difference g and reaches more than 20% for large sizes. Despite the optimization of g only being possible for classical methods with an associated kernel, the performance advantage remains stable across other common classical methods. Note that we also constructed engineered datasets saturating the geometric inequality between classical ML and the original quantum kernel, but the small geometric difference g presented no empirical advantage at large system size (see Supplementary Section 13 ).
In keeping with our arguments about the role of data, when we increase the number of training data N , all methods improve, and the advantage will gradually diminish. While this dataset is engineered, it shows the strongest empirical separation on the largest system size to date. We conjecture that this procedure could be used with a quantum computer to create challenging datasets that are easy to learn with a quantum device, hard to learn classically, while still being easy to verify classically given the correct labels. Moreover, the size of the margin implies that this separation may even persist under moderate amounts of noise in a quantum device.
The use of quantum computing in machine learning remains an exciting prospect, but quantifying quantum advantage for such applications has some subtle issues that one must approach carefully. Here, we constructed a foundation for understanding opportunities for quantum advantage in a learning setting. We showed quantitatively how classical ML algorithms with data can become computationally more powerful, and a prediction advantage for quantum models is not guaranteed even if the data come from a quantum process that is challenging to independently simulate. Motivated by these tests, we introduced projected quantum kernels. On engineered datasets, projected quantum kernels outperform all tested classical models in prediction error. To the authors’ knowledge, this is the first empirical demonstration of such a large separation between quantum and classical ML models.
This work suggests a simple guidebook for generating ML problems which give a large separation between quantum and classical models, even at a modest number of qubits. The size of this separation and trend up to 30 qubits suggests the existence of learning tasks that may be easy to verify, but hard to model classically, requiring just a modest number of qubits and allowing for device noise. Claims of true advantage in a quantum machine learning setting require not only benchmarking classical machine learning models, but also classical approximations of quantum models. Additional work will be needed to identify embeddings that satisfy the sometimes conflicting requirements of being hard to approximate classically and exhibiting meaningful signal on local observables for very large numbers of qubits. Further research will be required to find use cases on datasets closer to practical interest and evaluate potential claims of advantage, but we believe the tools developed in this work will help to pave the way for this exciting frontier.
Data availability
All other data that support the plots within this paper and other findings of this study are available upon reasonable request. Source data are provided with this paper.
Code availability
A tutorial for reproducing smaller numerical experiments is available at https://www.tensorflow.org/quantum/tutorials/quantum_data .
Halevy, A., Norvig, P. & Pereira, F. The unreasonable effectiveness of data. IEEE Intell. Syst. 24 , 8 (2009).
Article Google Scholar
Grover, L. K. A fast quantum mechanical algorithm for database search. in Proc. twenty-eighth annual ACM symposium on Theory of computing (1996).
Durr, C. & Hoyer, P. A quantum algorithm for finding the minimum . https://arxiv.org/abs/quant-ph/9607014 (1996).
Farhi, E. et al. A quantum adiabatic evolution algorithm applied to random instances of an np-complete problem. Science 292 , 472 (2001).
Article ADS MathSciNet CAS Google Scholar
Neven, H., Denchev, V. S., Rose, G. & Macready, W. G. Training a large scale classifier with the quantum adiabatic algorithm . https://arxiv.org/abs/0912.0779 (2009).
Rebentrost, P., Mohseni, M. & Lloyd, S. Quantum support vector machine for big data classification. Phys. Rev. Lett. 113 , 130503 (2014).
Article ADS Google Scholar
Leifer, M. S. & Poulin, D. Quantum graphical models and belief propagation. Ann. Phys. 323 , 1899 (2008).
Aaronson, S. & Ambainis, A. The need for structure in quantum speedups . https://arxiv.org/abs/0911.0996 (2009).
McClean, J. R. et al. Low depth mechanisms for quantum optimization . https://arxiv.org/abs/2008.08615 (2020).
Boixo, S. et al. Characterizing quantum supremacy in near-term devices. Nat. Phys. 14 , 595 (2018).
Article CAS Google Scholar
Arute, F. et al. Quantum supremacy using a programmable superconducting processor. Nature 574 , 505 (2019).
ADS CAS PubMed Google Scholar
Peruzzo, A. et al. A variational eigenvalue solver on a photonic quantum processor. Nat. Commun. 5 , 4213 (2014).
Article ADS CAS Google Scholar
McClean, J. R., Romero, J., Babbush, R. & Aspuru-Guzik, A. The theory of variational hybrid quantum-classical algorithms. N. J. Phys. 18 , 023023 (2016).
Farhi, E. & Neven, H. Classification with quantum neural networks on near term processors. arXiv preprint arXiv:1802.06002 https://arxiv.org/abs/1802.06002 (2018).
Havlíček, V. et al. Supervised learning with quantum-enhanced feature spaces. Nature 567 , 209 (2019).
Cortes, C. & Vapnik, V. Support-vector networks. Mach. Learn. 20 , 273 (1995).
MATH Google Scholar
Schölkopf, B. et al. Learning with kernels: support vector machines, regularization, optimization, and beyond. https://mitpress.mit.edu/books/learning-kernels (2002).
Mohri, M., Rostamizadeh, A. & Talwalkar, A. Foundations of machine learning. https://mitpress.mit.edu/books/foundations-machine-learning-second-edition (2018).
Jacot, A., Gabriel, F. & Hongler, C. Neural tangent kernel: Convergence and generalization in neural networks. NIPS’18: Proceedings of the 32nd International Conference on Neural Information Processing Systems https://dl.acm.org/doi/abs/10.5555/3327757.3327948 pp. 8580–8589 (2018).
Novak, R. et al. Neural tangents: Fast and easy infinite neural networks in python. arXiv preprint arXiv:1912.02803 https://openreview.net/pdf?id=SklD9yrFPS (2019).
Arora, S. et al. On exact computation with an infinitely wide neural net. in Advances in Neural Information Processing Systems (2019).
Blank, C., Park, D. K., Rhee, J.-K. K. & Petruccione, F. Quantum classifier with tailored quantum kernel. npj Quantum Inf. 6 , 1 (2020).
Bartkiewicz, K. Experimental kernel-based quantum machine learning in finite feature space. Sci. Rep. 10 , 1 (2020).
Liu, Y., Arunachalam, S. & Temme, K. A rigorous and robust quantum speed-up in supervised machine learning. arXiv preprint arXiv:2010.02174 https://arxiv.org/abs/2010.02174 (2020).
Huang, H.-Y., Kueng, R. & Preskill, J. Predicting many properties of a quantum system from very few measurements. Nat. Phys . https://doi.org/10.1038/s41567-020-0932-7 (2020).
Cotler, J. & Wilczek, F. Quantum overlapping tomography. Phys. Rev. Lett. 124 , 100401 (2020).
Paini, M. and Kalev, A. An approximate description of quantum states. arXiv preprint arXiv:1910.10543 https://arxiv.org/abs/1910.10543 (2019).
Lloyd, S., Schuld, M., Ijaz, A., Izaac, J. & Killoran, N. Quantum embeddings for machine learning. arXiv preprint arXiv:2001.03622 https://arxiv.org/abs/2008.08605 (2020).
Schuld, M., Sweke, R. & Meyer, J. J. The effect of data encoding on the expressive power of variational quantum machine learning models. Phys. Rev. A 103 , 032430 (2021).
McClean, J. R., Boixo, S., Smelyanskiy, V. N., Babbush, R. & Neven, H. Barren plateaus in quantum neural network training landscapes. Nat. Commun. 9 , 1 (2018).
Grant, E., Wossnig, L., Ostaszewski, M. & Benedetti, M. An initialization strategy for addressing barren plateaus in parametrized quantum circuits. Quantum 3 , 214 (2019).
Schuld, M., Bocharov, A., Svore, K. M. & Wiebe, N. Circuit-centric quantum classifiers. Phys. Rev. A 101 , 032308 (2020b).
LaRose, R. & Coyle, B. Robust data encodings for quantum classifiers. Phys. Rev. A 102 , 032420 (2020).
Harrow, A. W. & Montanaro, A. Quantum computational supremacy. Nature 549 , 203 (2017).
Li, Z. et al. Enhanced convolutional neural tangent kernels. arXiv preprint arXiv:1911.00809 https://arxiv.org/abs/1911.00809 (2019).
Micchelli, C. A., Xu, Y. & Zhang, H. Universal kernels. J. Mach. Learn. Res. 7 , 2651 (2006).
MathSciNet MATH Google Scholar
Krogh, A. & Hertz, J. A. A simple weight decay can improve generalization . Adv. Neural Inf. Process. Syst. 950–957 (1992).
Suykens, J. A. & Vandewalle, J. Least squares support vector machine classifiers. Neural Process. Lett. 9 , 293 (1999).
Huang, H.-Y., Kueng, R. & Preskill, J. Information-theoretic bounds on quantum advantage in machine learning. arXiv preprint arXiv:2101.02464 https://arxiv.org/abs/2101.02464 (2021).
Anderson, E. et al. LAPACK Users’ Guide , 3rd edn. (Society for Industrial and Applied Mathematics, 1999).
Breiman, L. Random forests. Mach. Learn. 45 , 5 (2001).
Gosset, D. & Smolin, J. A compressed classical description of quantum states. arXiv preprint arXiv:1801.05721 https://arxiv.org/abs/1801.05721 (2018).
Aaronson, S. Shadow tomography of quantum states. SIAM J. Comput. https://dl.acm.org/doi/abs/10.1145/3188745.3188802 (2020).
Aaronson, S. and Rothblum, G. N. Gentle measurement of quantum states and differential privacy. in Proc. 51st Annual ACM SIGACT Symposium on Theory of Computing (2019).
Hohenberg, P. & Kohn, W. Inhomogeneous electron gas. Phys. Rev. 136 , B864 (1964).
Article ADS MathSciNet Google Scholar
Runge, E. & Gross, E. K. Density-functional theory for time-dependent systems. Phys. Rev. Lett. 52 , 997 (1984).
Broughton, M. et al. Tensorflow quantum: A software framework for quantum machine learning. arXiv preprint arXiv:2003.02989 https://arxiv.org/abs/2003.02989 (2020).
Xiao, H., Rasul, K. & Vollgraf, R. Fashion-mnist: a novel image dataset for benchmarking machine learning algorithms. arXiv preprint arXiv:1708.07747 https://arxiv.org/abs/1708.07747 (2017).
LeCun, Y., Cortes, C. & Burges, C. Mnist handwritten digit database . http://yann.lecun.com/exdb/mnist (2010).
Jolliffe, I. T. in Principal component analysis 129–155 (Springer, 1986).
Schuld, M. & Killoran, N. Quantum machine learning in feature hilbert spaces. Phys. Rev. Lett. 122 , 040504 (2019).
Skolik, A., McClean, J. R., Mohseni, M., van der Smagt, P. & Leib, M. Layerwise learning for quantum neural networks. Quantum Machine Intelligence 3 , 5 (2021).
Download references
Acknowledgements
We want to thank Richard Kueng, John Platt, John Preskill, Thomas Vidick, Nathan Wiebe, and Chun-Ju Wu for valuable inputs and inspiring discussions. We thank Bálint Pató for crucial contributions in setting up simulations.
Author information
Authors and affiliations.
Google Quantum AI, Venice, CA, USA
Hsin-Yuan Huang, Michael Broughton, Masoud Mohseni, Ryan Babbush, Sergio Boixo, Hartmut Neven & Jarrod R. McClean
Institute for Quantum Information and Matter, Caltech, Pasadena, CA, USA
Hsin-Yuan Huang
Department of Computing and Mathematical Sciences, Caltech, Pasadena, CA, USA
You can also search for this author in PubMed Google Scholar
Contributions
H.H. and J.M. developed the theoretical aspects of this work. H.H. and M.B. conducted the numerical experiments and wrote the open source code. H.H., M.M., R.B., S.B., H.N., and J.M. contributed to technical discussions and writing of the manuscript.
Corresponding author
Correspondence to Jarrod R. McClean .
Ethics declarations
Competing interests.
The authors declare no competing interests.
Additional information
Peer review information Nature Communications thanks Nana Liu and the other, anonymous, reviewer(s) for their contribution to the peer review of this work.
Publisher’s note Springer Nature remains neutral with regard to jurisdictional claims in published maps and institutional affiliations.
Supplementary information
Supplementary information, source data, source data, rights and permissions.
Open Access This article is licensed under a Creative Commons Attribution 4.0 International License, which permits use, sharing, adaptation, distribution and reproduction in any medium or format, as long as you give appropriate credit to the original author(s) and the source, provide a link to the Creative Commons license, and indicate if changes were made. The images or other third party material in this article are included in the article’s Creative Commons license, unless indicated otherwise in a credit line to the material. If material is not included in the article’s Creative Commons license and your intended use is not permitted by statutory regulation or exceeds the permitted use, you will need to obtain permission directly from the copyright holder. To view a copy of this license, visit http://creativecommons.org/licenses/by/4.0/ .
Reprints and permissions
About this article
Cite this article.
Huang, HY., Broughton, M., Mohseni, M. et al. Power of data in quantum machine learning. Nat Commun 12 , 2631 (2021). https://doi.org/10.1038/s41467-021-22539-9
Download citation
Received : 18 November 2020
Accepted : 16 March 2021
Published : 11 May 2021
DOI : https://doi.org/10.1038/s41467-021-22539-9
Share this article
Anyone you share the following link with will be able to read this content:
Sorry, a shareable link is not currently available for this article.
Provided by the Springer Nature SharedIt content-sharing initiative
This article is cited by
Quantum-parallel vectorized data encodings and computations on trapped-ion and transmon qpus.
- Jan Balewski
- Mercy G. Amankwah
Scientific Reports (2024)
Drug design on quantum computers
- Raffaele Santagati
- Alan Aspuru-Guzik
- Clemens Utschig-Utschig
Nature Physics (2024)
Scalable parameterized quantum circuits classifier
- Xiaodong Ding
- Zhihui Song
Covariant quantum kernels for data with group structure
- Jennifer R. Glick
- Tanvi P. Gujarati
- Kristan Temme
Towards provably efficient quantum algorithms for large-scale machine-learning models
- Minzhao Liu
- Liang Jiang
Nature Communications (2024)
By submitting a comment you agree to abide by our Terms and Community Guidelines . If you find something abusive or that does not comply with our terms or guidelines please flag it as inappropriate.
Quick links
- Explore articles by subject
- Guide to authors
- Editorial policies
Sign up for the Nature Briefing: AI and Robotics newsletter — what matters in AI and robotics research, free to your inbox weekly.

arXiv's Accessibility Forum starts next month!
Help | Advanced Search
Quantum Physics
Title: quantum computing 2022.
Abstract: Quantum technology is full of figurative and literal noise obscuring its promise. In this overview, we will attempt to provide a sober assessment of the promise of quantum technology with a focus on computing. We provide a tour of quantum computing and quantum technology that is aimed to be comprehensible to scientists and engineers without becoming a popular account. The goal is not a comprehensive review nor a superficial introduction but rather to serve as a useful map to navigate the hype, the scientific literature, and upcoming press releases about quantum technology and quantum computing. We have aimed to cite the most recent topical reviews, key results, and guide the reader away from fallacies and towards active discussions in the current quantum computing literature. The goal of this article was to be pedantic and introductory without compromising on the science.
Comments: | 14 pages. Comments are welcome. Updated with additional quantum primacy results |
Subjects: | Quantum Physics (quant-ph) |
Cite as: | [quant-ph] |
(or [quant-ph] for this version) | |
Focus to learn more arXiv-issued DOI via DataCite |
Submission history
Access paper:.
- Other Formats

References & Citations
- INSPIRE HEP
- Google Scholar
- Semantic Scholar
BibTeX formatted citation

Bibliographic and Citation Tools
Code, data and media associated with this article, recommenders and search tools.
- Institution
arXivLabs: experimental projects with community collaborators
arXivLabs is a framework that allows collaborators to develop and share new arXiv features directly on our website.
Both individuals and organizations that work with arXivLabs have embraced and accepted our values of openness, community, excellence, and user data privacy. arXiv is committed to these values and only works with partners that adhere to them.
Have an idea for a project that will add value for arXiv's community? Learn more about arXivLabs .
IEEE Account
- Change Username/Password
- Update Address
Purchase Details
- Payment Options
- Order History
- View Purchased Documents
Profile Information
- Communications Preferences
- Profession and Education
- Technical Interests
- US & Canada: +1 800 678 4333
- Worldwide: +1 732 981 0060
- Contact & Support
- About IEEE Xplore
- Accessibility
- Terms of Use
- Nondiscrimination Policy
- Privacy & Opting Out of Cookies
A not-for-profit organization, IEEE is the world's largest technical professional organization dedicated to advancing technology for the benefit of humanity. © Copyright 2024 IEEE - All rights reserved. Use of this web site signifies your agreement to the terms and conditions.
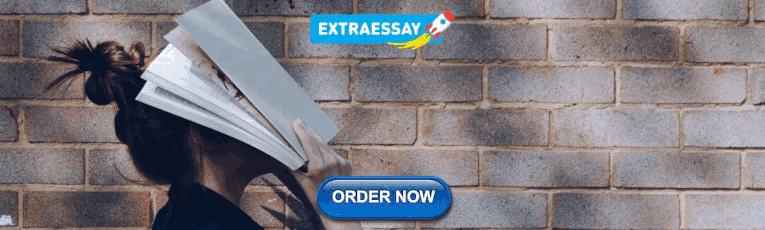
IMAGES
COMMENTS
Quantum Computing Review: A Decade of Research. Abstract: Quantum computing (QC) has the potential to be the next abstruse technology, with a wide range of possible applications and ramifications for organizations and markets. QC provides an exponential speedup by employing quantum mechanics principles, including superposition and entanglement.
Quantum computing, a transformative field that emerged from quantum mechanics and computer science, has gained immense attention for its potential to revolutionize computation. This paper aims to address the fundamentals of quantum computing and provide a comprehensive guide for both novices and experts in the field of quantum computing. Beginning with the foundational principles of quantum ...
Nature Reviews Physics 4 , 1 ( 2022) Cite this article. This year we celebrate four decades of quantum computing by looking back at the milestones of the field and forward to the challenges and ...
ACM Transactions on Quantum Computing publishes high-impact, original research papers and selected surveys on topics in quantum computing and quantum information science. The journal targets the quantum computer science community with a focus on the theory and practice of quantum computing including but not limited to: models of quantum computing, quantum algorithms and complexity, quantum ...
To better understand quantum computing, this paper examines the foundations and vision based on current research in this area. We discuss cutting-edge developments in quantum computer hardware advancement and subsequent advances in quantum cryptography, quantum software, and high-scalability quantum computers. Many potential challenges and ...
Quantum computers, therefore, can access an exponentially large Hilbert space (or computational space), where "n" qubits could be in a superposition state of 2 n possible outcomes at any given time. This will allow quantum computers to tackle large scale space problems. Developing a large-scale quantum computer has its own challenges.
Quantum computers can calculate and test extensive combinations of hypotheses simultaneously instead of sequentially (S.‐S. Li et al., 2001). Furthermore, some quantum algorithms can be designed in a way that they can solve problems in much fewer steps than their classical counterparts (their complex‐ ity is lower).
The fast advancement of computational quantum research has enormous promise for reshaping the computing landscape (Feynman, 2018).Compared to today's computing machines, although comparable on simple calculations, quantum technology is faster, giving it advantages across multiple sectors useful for society (Gill et al., 2022).In the coming decade, it has the potential to transform defence ...
Quantum computers store data in quantum binary digits called quantum bits, or qubits, that can be made using various technologies, including superconducting rings; optical traps; and photons of light.
This paper attempts to cover as many current state-of-the-art models of quantum computing in various domains and to contribute vital knowledge to the quantum field, readers, and researchers. ... Tom Garlinghouse for the office of the Dean for Research: Quantum computing: Opening new realms of possibilities. Jan. 21, 2020. https://www.princeton ...
pursued at the industrial level, NMR quantum computing has important conceptual significance. Many of the key quantum computing concepts were first uncovered in this context and many of the first experimental implementations were done using NMR quantum computing [29], [28]. While light sources are important for manipulating super-
Quantum computing is a revolutionary concept among emerging technologies visioned by researchers. The interdisciplinary nature of quantum computing evolves as cross-pollination of ideas, techniques, and methodologies. Henceforth, a comprehensive analysis of the literature is conducted to insight the progression of quantum computing research. Our study unfurls the intellectual landscape of ...
Read the paper: Quantum supremacy using a programmable superconducting processor Arute and colleagues chose a task that is related to random-number generation: namely, sampling the output of a ...
Abstract. Quantum computing hardware technologies have advanced during the past two decades, with the goal of building systems that can solve problems that are intractable on classical computers. The ability to realize large-scale systems depends on major advances in materials science, materials engineering, and new fabrication techniques.
Quantum Computing merges two great scientific revolutions of the 20th century: computer science and quantum physics. Quantum physics is the theoretical basis of the transistor, the laser, and other technologies which enabled the computing revolution. But on the algorithmic level, today's computing machinery still operates on ""classical ...
2.2 Limitations of Classical Computers and birth of art of Quantum Computing 2.2.1 Public key Cryptography and Classical factoring of big integers. 2.2.2 Quantum Factoring 2.2.3 Searching of an item with desired property. 2.2.4 Simulation of quantum system by classical computer. 2.3 Quantum Computing: A whole new concept in Parallelism
Initialization is an important challenge for nuclear magnetic resonance quantum computers. The first proposals employed pseudo-pure-state techniques, which isolate the signal of an initialized ...
Quantum computing promises to be the next disruptive technology, with numerous possible applications and implications for organizations and markets. Quantum computers exploit principles of quantum mechanics, such as superposition and entanglement, to represent data and perform operations on them. Both of these principles enable quantum computers to solve very specific, complex problems ...
Quantum computers are the new emerging and exciting field of computer science. The Quantum computer technology is based on the laws of quantum physics which hav ... This research paper will give an overview of the Quantum computers, the introduction of the Quantum Computers, description that how it works, brief knowledge about it's features and ...
View a PDF of the paper titled Systematic Literature Review: Quantum Machine Learning and its applications, by David Peral Garc\'ia and 1 other authors. Quantum computing is the process of performing calculations using quantum mechanics. This field studies the quantum behavior of certain subatomic particles for subsequent use in performing ...
1. INTRODUCTION. As of 2016, actual quantum computers are yet to be. developed, but using sma ll number of bits several. experiments a re carri ed out. Research in the field of. Quantum Computing ...
First, motivated by quantum applications in optimization 2,3,4, the power of quantum computing could, in principle, be used to help improve the training process of existing classical models 5,6 ...
View a PDF of the paper titled Quantum Computing 2022, by James D. Whitfield and 4 other authors. Quantum technology is full of figurative and literal noise obscuring its promise. In this overview, we will attempt to provide a sober assessment of the promise of quantum technology with a focus on computing. We provide a tour of quantum computing ...
In this paper, I will be discussing quantum computing in cybersecurity. Quantum computing is in development and will be released to the public sooner rather than later. With how quickly technology evolves, it will get here faster than we know it. However, this opens the door for cyberattacks with strength never seen before, possibly breaching the privacy of millions of individuals and ...