What Is Data Visualization: Brief Theory, Useful Tips and Awesome Examples
- Share on Facebook
- Share on Twitter
By Al Boicheva
in Insights , Inspiration
3 years ago
Viewed 11,647 times
Spread the word about this article:
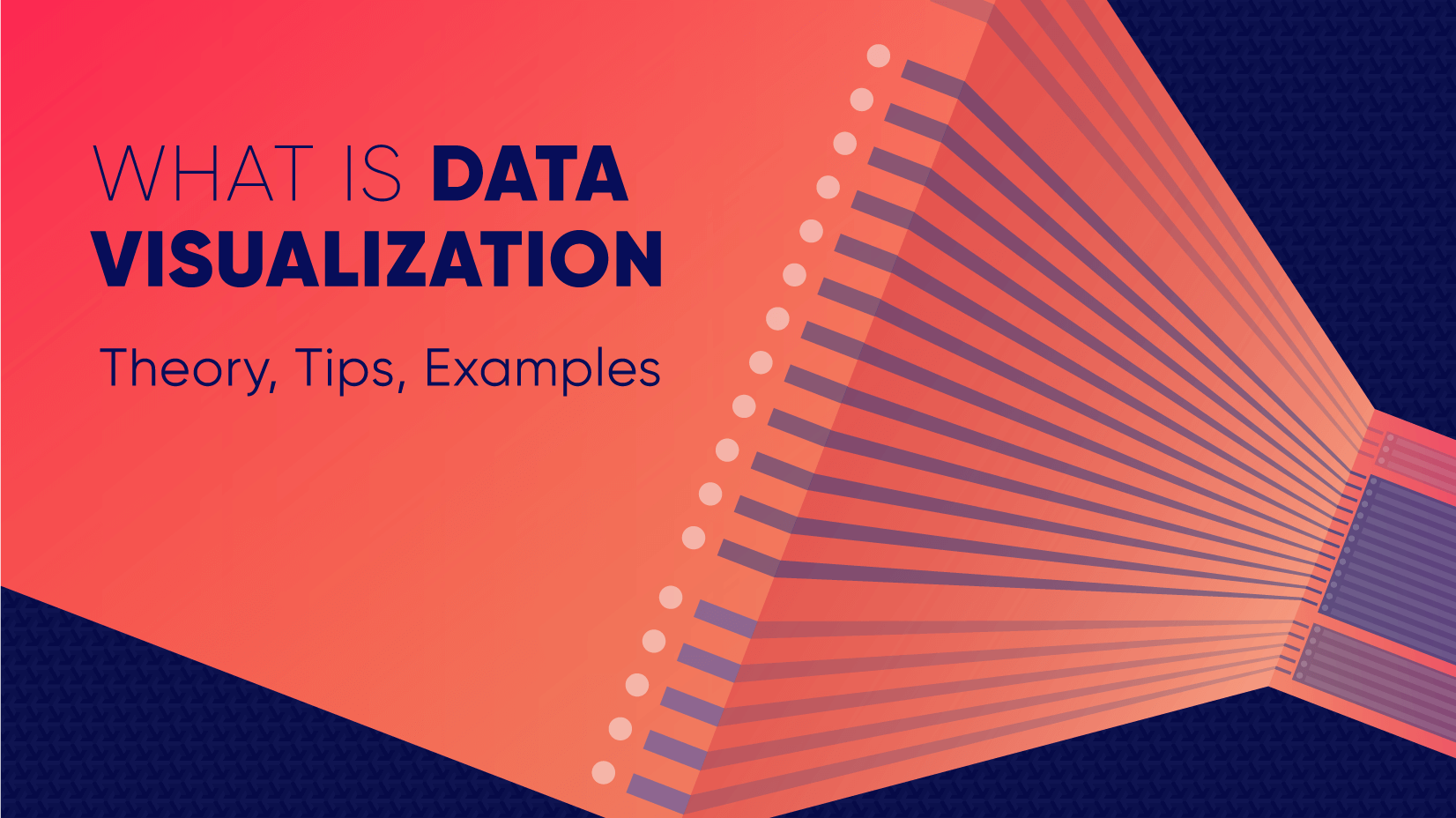
Updated: June 23, 2022
To create data visualization in order to present your data is no longer just a nice to have skill. Now, the skill to effectively sort and communicate your data through charts is a must-have for any business in any field that deals with data. Data visualization helps businesses quickly make sense of complex data and start making decisions based on that data. This is why today we’ll talk about what is data visualization. We’ll discuss how and why does it work, what type of charts to choose in what cases, how to create effective charts, and, of course, end with beautiful examples.
So let’s jump right in. As usual, don’t hesitate to fast-travel to a particular section of your interest.
Article overview: 1. What Does Data Visualization Mean? 2. How Does it Work? 3. When to Use it? 4. Why Use it? 5. Types of Data Visualization 6. Data Visualization VS Infographics: 5 Main Differences 7. How to Create Effective Data Visualization?: 5 Useful Tips 8. Examples of Data Visualization
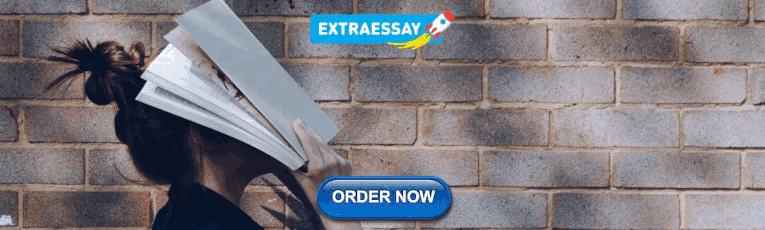
1. What is Data Visualization?
Data Visualization is a graphic representation of data that aims to communicate numerous heavy data in an efficient way that is easier to grasp and understand . In a way, data visualization is the mapping between the original data and graphic elements that determine how the attributes of these elements vary. The visualization is usually made by the use of charts, lines, or points, bars, and maps.
- Data Viz is a branch of Descriptive statistics but it requires both design, computer, and statistical skills.
- Aesthetics and functionality go hand in hand to communicate complex statistics in an intuitive way.
- Data Viz tools and technologies are essential for making data-driven decisions.
- It’s a fine balance between form and functionality.
- Every STEM field benefits from understanding data.
2. How Does it Work?
If we can see it, our brains can internalize and reflect on it. This is why it’s much easier and more effective to make sense of a chart and see trends than to read a massive document that would take a lot of time and focus to rationalize. We wouldn’t want to repeat the cliche that humans are visual creatures, but it’s a fact that visualization is much more effective and comprehensive.
In a way, we can say that data Viz is a form of storytelling with the purpose to help us make decisions based on data. Such data might include:
- Tracking sales
- Identifying trends
- Identifying changes
- Monitoring goals
- Monitoring results
- Combining data
3. When to Use it?
Data visualization is useful for companies that deal with lots of data on a daily basis. It’s essential to have your data and trends instantly visible. Better than scrolling through colossal spreadsheets. When the trends stand out instantly this also helps your clients or viewers to understand them instead of getting lost in the clutter of numbers.
With that being said, Data Viz is suitable for:
- Annual reports
- Presentations
- Social media micronarratives
- Informational brochures
- Trend-trafficking
- Candlestick chart for financial analysis
- Determining routes
Common cases when data visualization sees use are in sales, marketing, healthcare, science, finances, politics, and logistics.
4. Why Use it?
Short answer: decision making. Data Visualization comes with the undeniable benefits of quickly recognizing patterns and interpret data. More specifically, it is an invaluable tool to determine the following cases.
- Identifying correlations between the relationship of variables.
- Getting market insights about audience behavior.
- Determining value vs risk metrics.
- Monitoring trends over time.
- Examining rates and potential through frequency.
- Ability to react to changes.
5. Types of Data Visualization
As you probably already guessed, Data Viz is much more than simple pie charts and graphs styled in a visually appealing way. The methods that this branch uses to visualize statistics include a series of effective types.
Map visualization is a great method to analyze and display geographically related information and present it accurately via maps. This intuitive way aims to distribute data by region. Since maps can be 2D or 3D, static or dynamic, there are numerous combinations one can use in order to create a Data Viz map.
COVID-19 Spending Data Visualization POGO by George Railean
The most common ones, however, are:
- Regional Maps: Classic maps that display countries, cities, or districts. They often represent data in different colors for different characteristics in each region.
- Line Maps: They usually contain space and time and are ideal for routing, especially for driving or taxi routes in the area due to their analysis of specific scenes.
- Point Maps: These maps distribute data of geographic information. They are ideal for businesses to pinpoint the exact locations of their buildings in a region.
- Heat Maps: They indicate the weight of a geographical area based on a specific property. For example, a heat map may distribute the saturation of infected people by area.
Charts present data in the form of graphs, diagrams, and tables. They are often confused with graphs since graphs are indeed a subcategory of charts. However, there is a small difference: graphs show the mathematical relationship between groups of data and is only one of the chart methods to represent data.
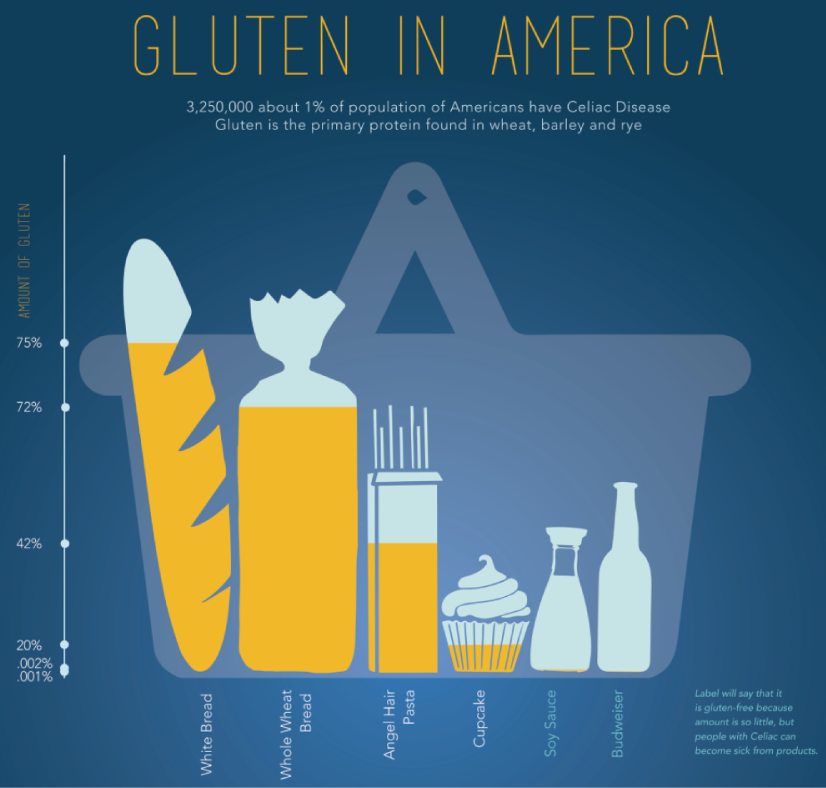
Infographic Data Visualization by Madeline VanRemmen
With that out of the way, let’s talk about the most basic types of charts in data visualization.
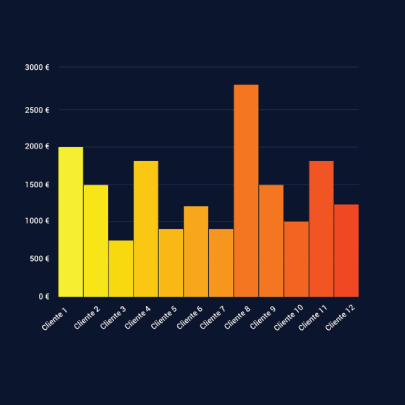
They use a series of bars that illustrate data development. They are ideal for lighter data and follow trends of no more than three variables or else, the bars become cluttered and hard to comprehend. Ideal for year-on-year comparisons and monthly breakdowns.
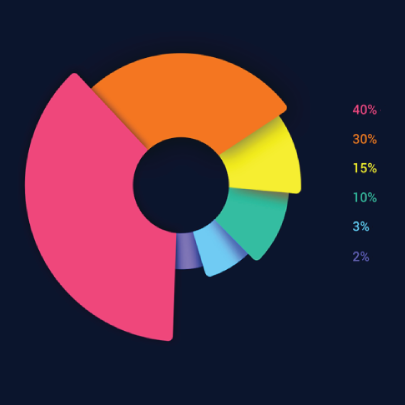
These familiar circular graphs divide data into portions. The bigger the slice, the bigger the portion. They are ideal for depicting sections of a whole and their sum must always be 100%. Avoid pie charts when you need to show data development over time or lack a value for any of the portions. Doughnut charts have the same use as pie charts.
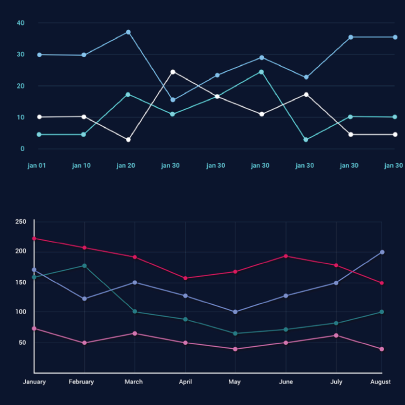
They use a line or more than one lines that show development over time. It allows tracking multiple variables at the same time. A great example is tracking product sales by a brand over the years. Area charts have the same use as line charts.
Scatter Plot
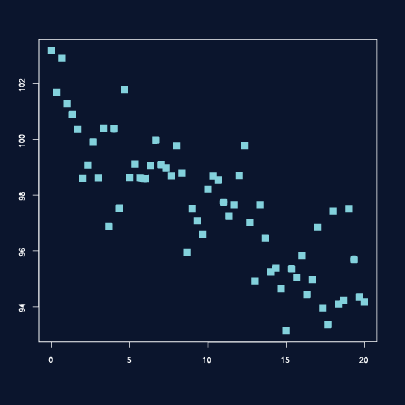
These charts allow you to see patterns through data visualization. They have an x-axis and a y-axis for two different values. For example, if your x-axis contains information about car prices while the y-axis is about salaries, the positive or negative relationship will tell you about what a person’s car tells about their salary.
Unlike the charts we just discussed, tables show data in almost a raw format. They are ideal when your data is hard to present visually and aim to show specific numerical data that one is supposed to read rather than visualize.
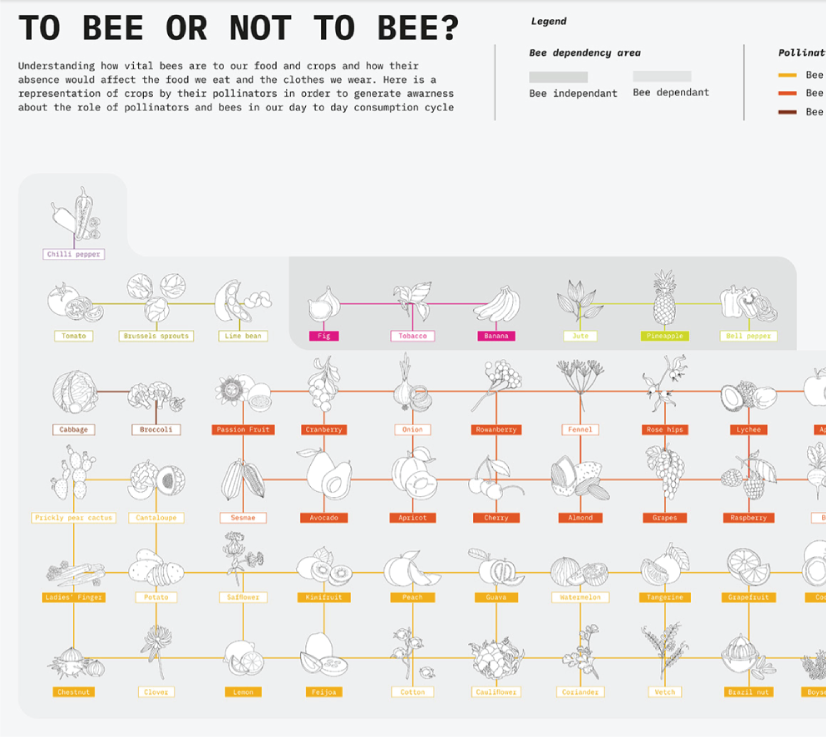
Data Visualisation | To bee or not to bee by Aishwarya Anand Singh
For example, charts are perfect to display data about a particular illness over a time period in a particular area, but a table comes to better use when you also need to understand specifics such as causes, outcomes, relapses, a period of treatment, and so on.
6. Data Visualization VS Infographics
5 main differences.
They are not that different as both visually represent data. It is often you search for infographics and find images titled Data Visualization and the other way around. In many cases, however, these titles aren’t misleading. Why is that?
- Data visualization is made of just one element. It could be a map, a chart, or a table. Infographics , on the other hand, often include multiple Data Viz elements.
- Unlike data visualizations that can be simple or extremely complex and heavy, infographics are simple and target wider audiences. The latter is usually comprehensible even to people outside of the field of research the infographic represents.
- Interestingly enough, data Viz doesn’t offer narratives and conclusions, it’s a tool and basis for reaching those. While infographics, in most cases offer a story and a narrative. For example, a data visualization map may have the title “Air pollution saturation by region”, while an infographic with the same data would go “Areas A and B are the most polluted in Country C”.
- Data visualizations can be made in Excel or use other tools that automatically generate the design unless they are set for presentation or publishing. The aesthetics of infographics , however, are of great importance and the designs must be appealing to wider audiences.
- In terms of interaction, data visualizations often offer interactive charts, especially in an online form. Infographics, on the other hand, rarely have interaction and are usually static images.
While on topic, you could also be interested to check out these 50 engaging infographic examples that make complex data look great.
7. Tips to Create Effective Data Visualization
The process is naturally similar to creating Infographics and it revolves around understanding your data and audience. To be more precise, these are the main steps and best practices when it comes to preparing an effective visualization of data for your viewers to instantly understand.
1. Do Your Homework
Preparation is half the work already done. Before you even start visualizing data, you have to be sure you understand that data to the last detail.
Knowing your audience is undeniable another important part of the homework, as different audiences process information differently. Who are the people you’re visualizing data for? How do they process visual data? Is it enough to hand them a single pie chart or you’ll need a more in-depth visual report?
The third part of preparing is to determine exactly what you want to communicate to the audience. What kind of information you’re visualizing and does it reflect your goal?
And last, think about how much data you’ll be working with and take it into account.
2. Choose the Right Type of Chart
In a previous section, we listed the basic chart types that find use in data visualization. To determine best which one suits your work, there are a few things to consider.
- How many variables will you have in a chart?
- How many items will you place for each of your variables?
- What will be the relation between the values (time period, comparison, distributions, etc.)
With that being said, a pie chart would be ideal if you need to present what portions of a whole takes each item. For example, you can use it to showcase what percent of the market share takes a particular product. Pie charts, however, are unsuitable for distributions, comparisons, and following trends through time periods. Bar graphs, scatter plots,s and line graphs are much more effective in those cases.
Another example is how to use time in your charts. It’s way more accurate to use a horizontal axis because time should run left to right. It’s way more visually intuitive.
3. Sort your Data
Start with removing every piece of data that does not add value and is basically excess for the chart. Sometimes, you have to work with a huge amount of data which will inevitably make your chart pretty complex and hard to read. Don’t hesitate to split your information into two or more charts. If that won’t work for you, you could use highlights or change the entire type of chart with something that would fit better.
Tip: When you use bar charts and columns for comparison, sort the information in an ascending or a descending way by value instead of alphabetical order.
4. Use Colors to Your Advantage
In every form of visualization, colors are your best friend and the most powerful tool. They create contrasts, accents, and emphasis and lead the eye intuitively. Even here, color theory is important.
When you design your chart, make sure you don’t use more than 5 or 6 colors. Anything more than that will make your graph overwhelming and hard to read for your viewers. However, color intensity is a different thing that you can use to your advantage. For example, when you compare the same concept in different periods of time, you could sort your data from the lightest shade of your chosen color to its darker one. It creates a strong visual progression, proper to your timeline.
Things to consider when you choose colors:
- Different colors for different categories.
- A consistent color palette for all charts in a series that you will later compare.
- It’s appropriate to use color blind-friendly palettes.
5. Get Inspired
Always put your inspiration to work when you want to be at the top of your game. Look through examples, infographics, and other people’s work and see what works best for each type of data you need to implement.
This Twitter account Data Visualization Society is a great way to start. In the meantime, we’ll also handpick some amazing examples that will get you in the mood to start creating the visuals for your data.
8. Examples for Data Visualization
As another art form, Data Viz is a fertile ground for some amazing well-designed graphs that prove that data is beautiful. Now let’s check out some.
Dark Souls III Experience Data
We start with Meng Hsiao Wei’s personal project presenting his experience with playing Dark Souls 3. It’s a perfect example that infographics and data visualization are tools for personal designs as well. The research is pretty massive yet very professionally sorted into different types of charts for the different concepts. All data visualizations are made with the same color palette and look great in infographics.
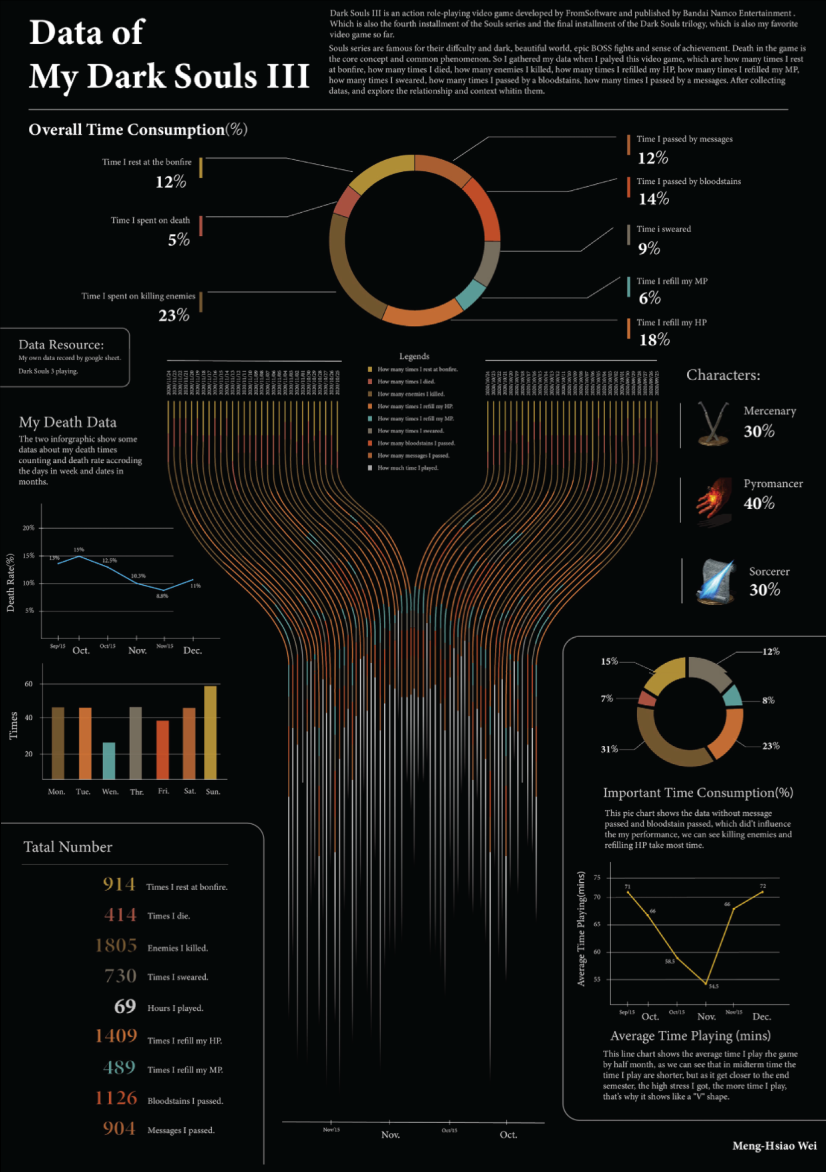
My dark souls 3 playing data by Meng Hsiao Wei
Greatest Movies of all Time
Katie Silver has compiled a list of the 100 greatest movies of all time based on critics and crowd reviews. The visualization shows key data points for every movie such as year of release, oscar nominations and wins, budget, gross, IMDB score, genre, filming location, setting of the film, and production studio. All movies are ordered by the release date.
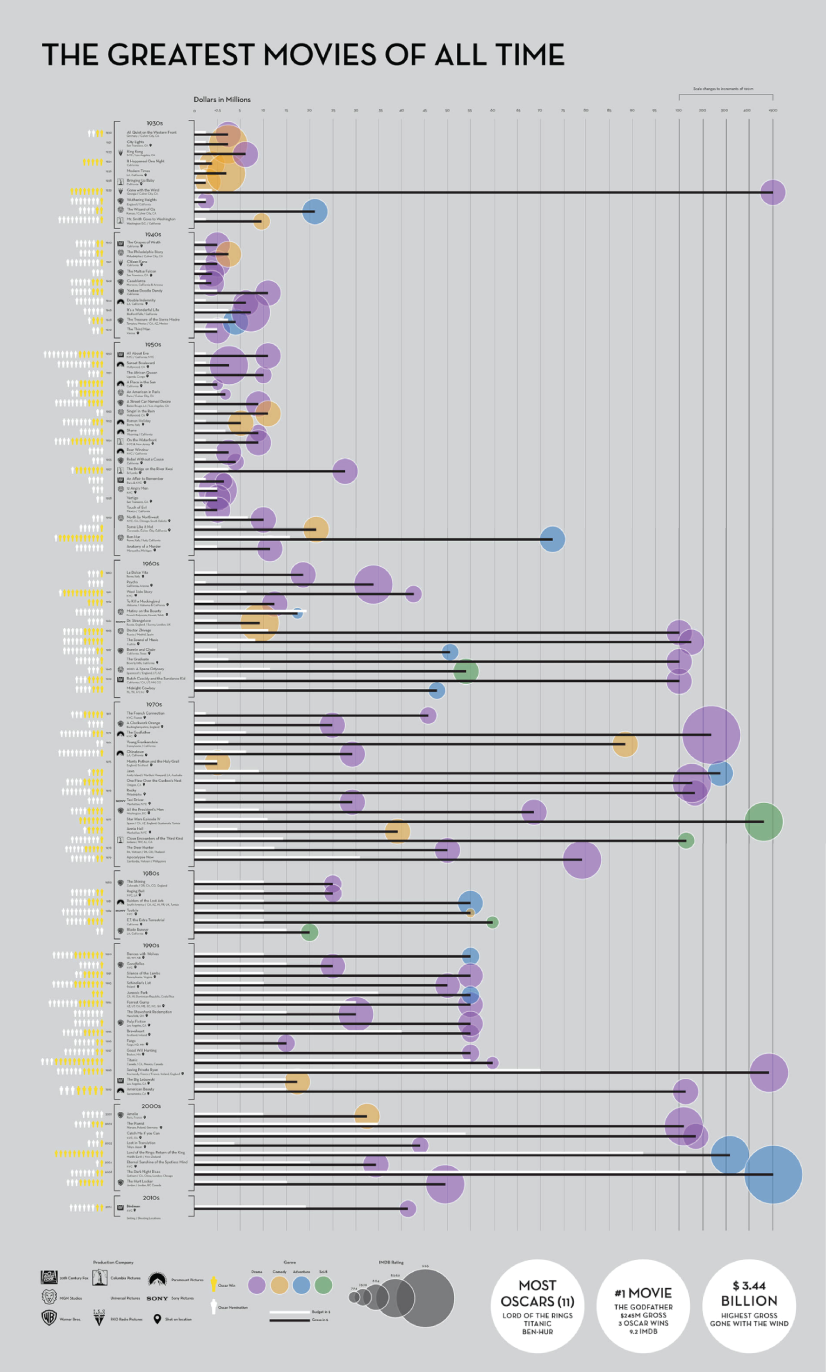
100 Greatest Movies Data Visualization by Katie Silver
The Most Violent Cities
Federica Fragapane shows data for the 50 most violent cities in the world in 2017. The items are arranged on a vertical axis based on population and ordered along the horizontal axis according to the homicide rate.
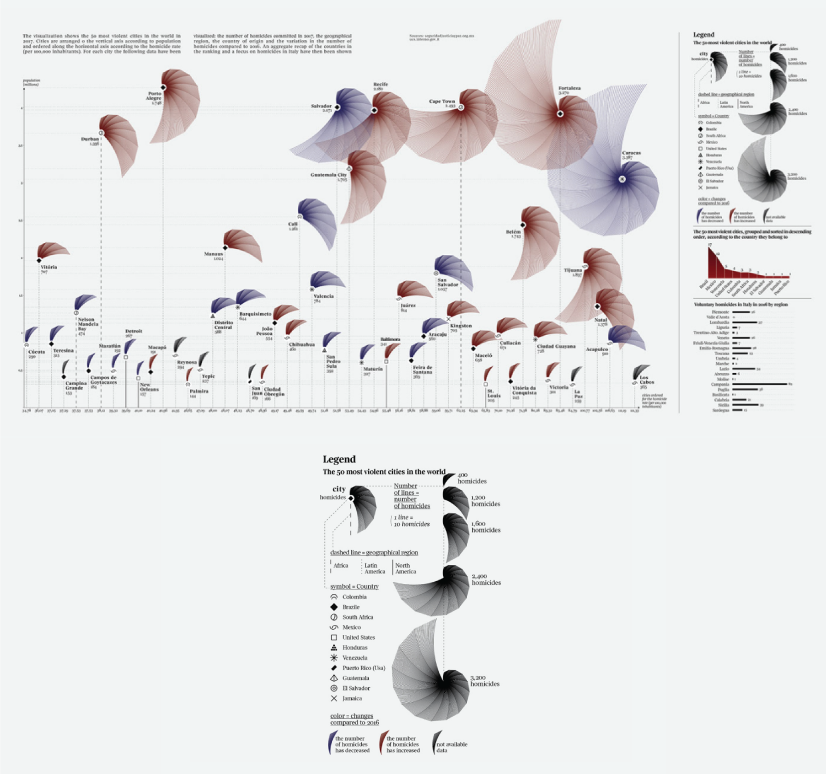
The Most Violent Cities by Federica Fragapane
Family Businesses as Data
These data visualizations and illustrations were made by Valerio Pellegrini for Perspectives Magazine. They show a pie chart with sector breakdown as well as a scatter plot for contribution for employment.
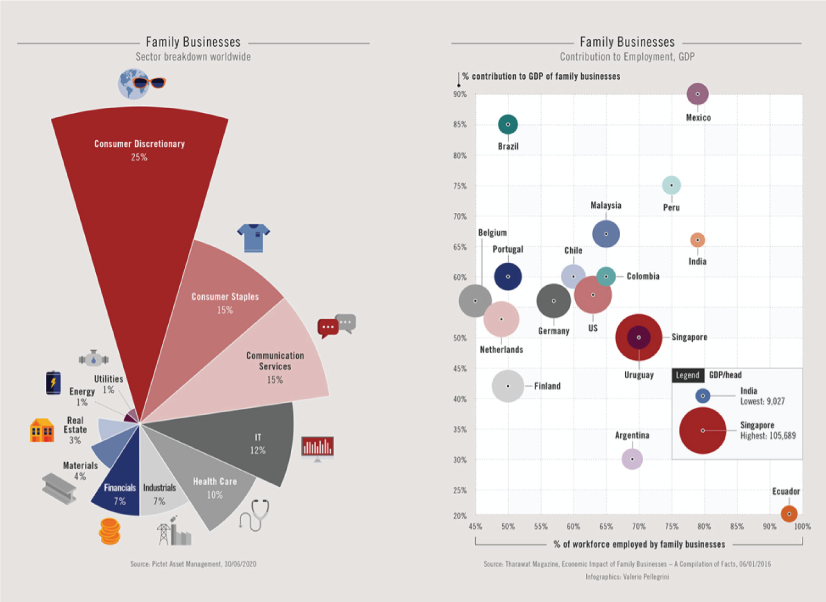
PERSPECTIVES MAGAZINE – Family Businesses by Valerio Pellegrini
Orbit Map of the Solar System
The map shows data on the orbits of more than 18000 asteroids in the solar system. Each asteroid is shown at its position on New Years’ Eve 1999, colored by type of asteroid.
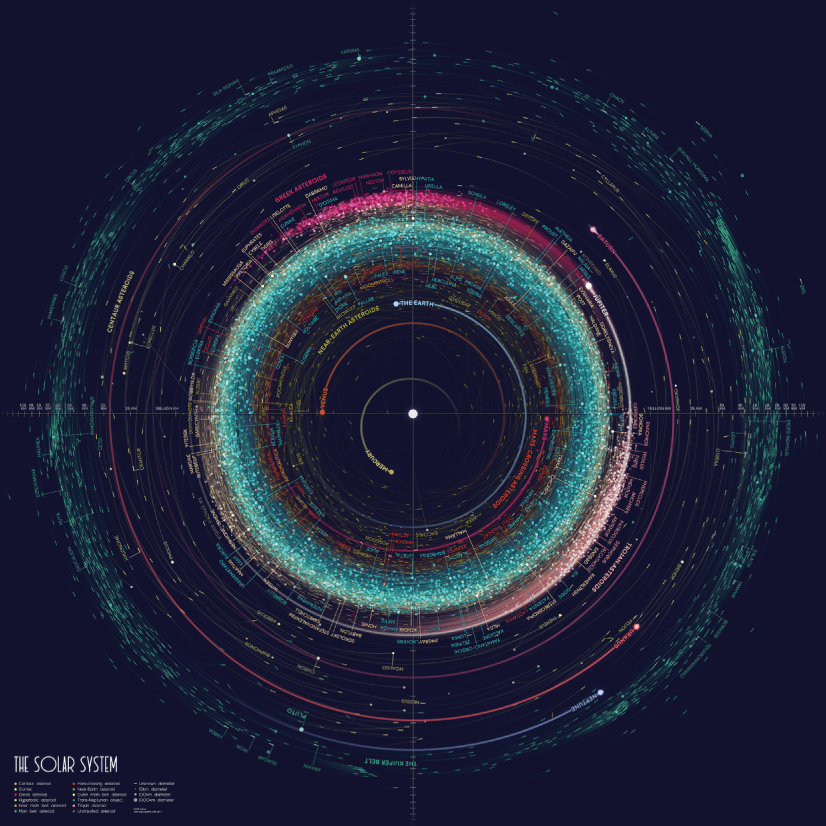
An Orbit Map of the Solar System by Eleanor Lutz
The Semantics Of Headlines
Katja Flükiger has a take on how headlines tell the story. The data visualization aims to communicate how much is the selling influencing the telling. The project was completed at Maryland Institute College of Art to visualize references to immigration and color-coding the value judgments implied by word choice and context.
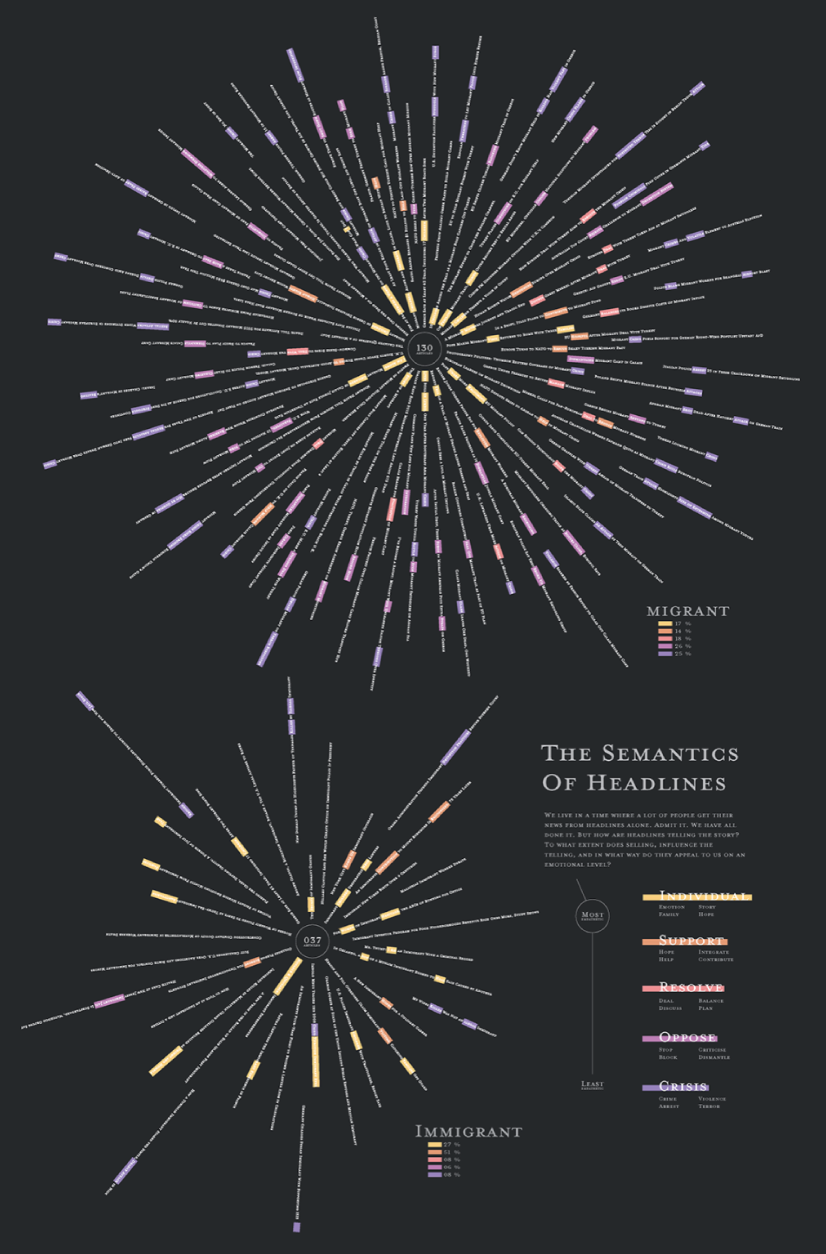
The Semantics of Headlines by Katja Flükiger
Moon and Earthquakes
This data visualization works on answering whether the moon is responsible for earthquakes. The chart features the time and intensity of earthquakes in response to the phase and orbit location of the moon.
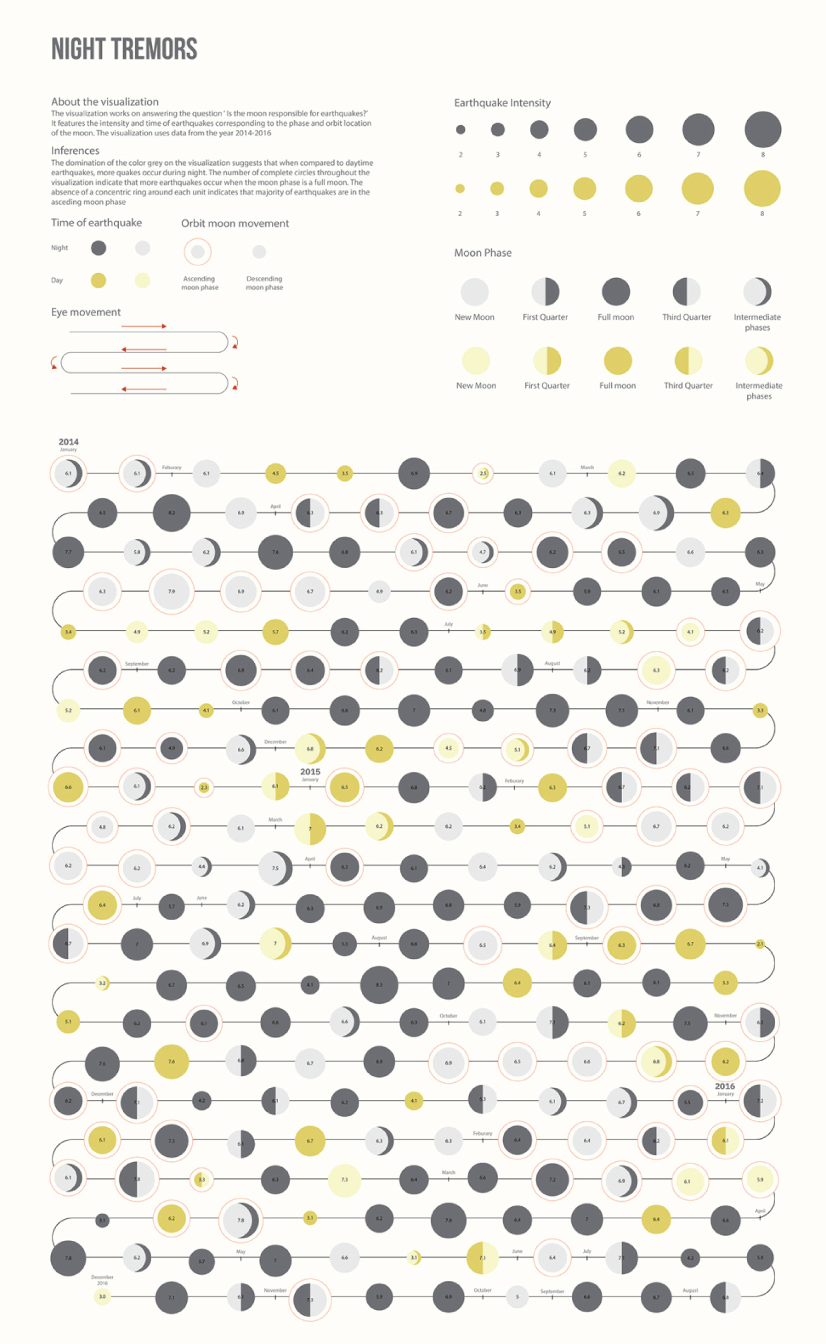
Moon and Earthquakes by Aishwarya Anand Singh
Dawn of the Nanosats
The visualization shows the satellites launched from 2003 to 2015. The graph represents the type of institutions focused on projects as well as the nations that financed them. On the left, it is shown the number of launches per year and satellite applications.
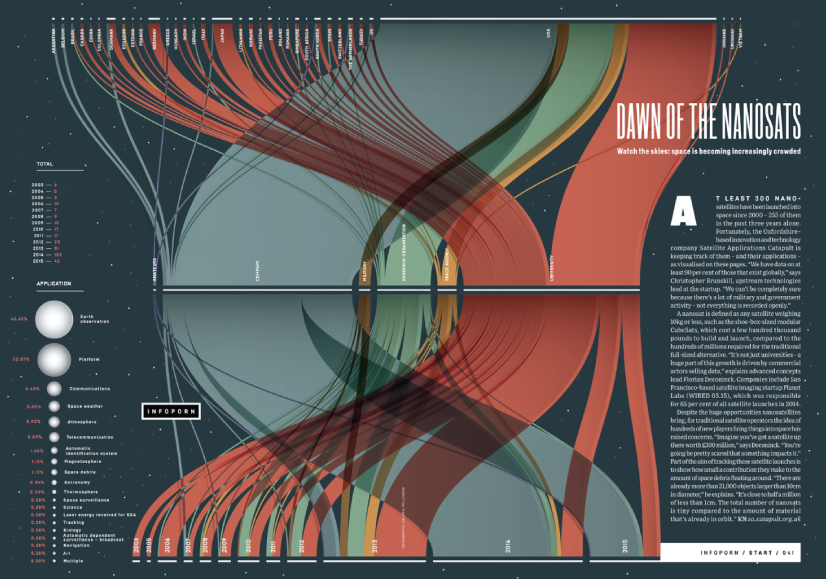
WIRED UK – Dawn of the by Nanosats by Valerio Pellegrini
Final Words
Data visualization is not only a form of science but also a form of art. Its purpose is to help businesses in any field quickly make sense of complex data and start making decisions based on that data. To make your graphs efficient and easy to read, it’s all about knowing your data and audience. This way you’ll be able to choose the right type of chart and use visual techniques to your advantage.
You may also be interested in some of these related articles:
- Infographics for Marketing: How to Grab and Hold the Attention
- 12 Animated Infographics That Will Engage Your Mind from Start to Finish
- 50 Engaging Infographic Examples That Make Complex Ideas Look Great
- Good Color Combinations That Go Beyond Trends: Inspirational Examples and Ideas
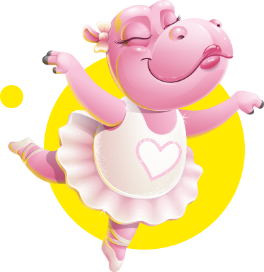
Add some character to your visuals
Cartoon Characters, Design Bundles, Illustrations, Backgrounds and more...
Like us on Facebook
Subscribe to our newsletter
Be the first to know what’s new in the world of graphic design and illustrations.
- [email protected]
Browse High Quality Vector Graphics
E.g.: businessman, lion, girl…
Related Articles
How to use adobe character animator for free in 2022, cartoon yourself today with 10+ tools, tutorials & tips, we can’t wait for these design conferences in 2020, 30+ fantastic 3d website examples with fully immersive designs, amazing black-and-white illustrations that don’t need color to impress, check out our infographics bundle with 500+ infographic templates:, enjoyed this article.
Don’t forget to share!
- Comments (2)
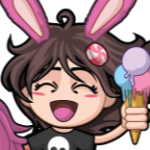
Al Boicheva
Al is an illustrator at GraphicMama with out-of-the-box thinking and a passion for anything creative. In her free time, you will see her drooling over tattoo art, Manga, and horror movies.
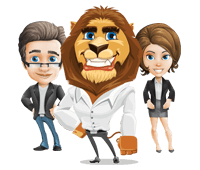
Thousands of vector graphics for your projects.
Hey! You made it all the way to the bottom!
Here are some other articles we think you may like:
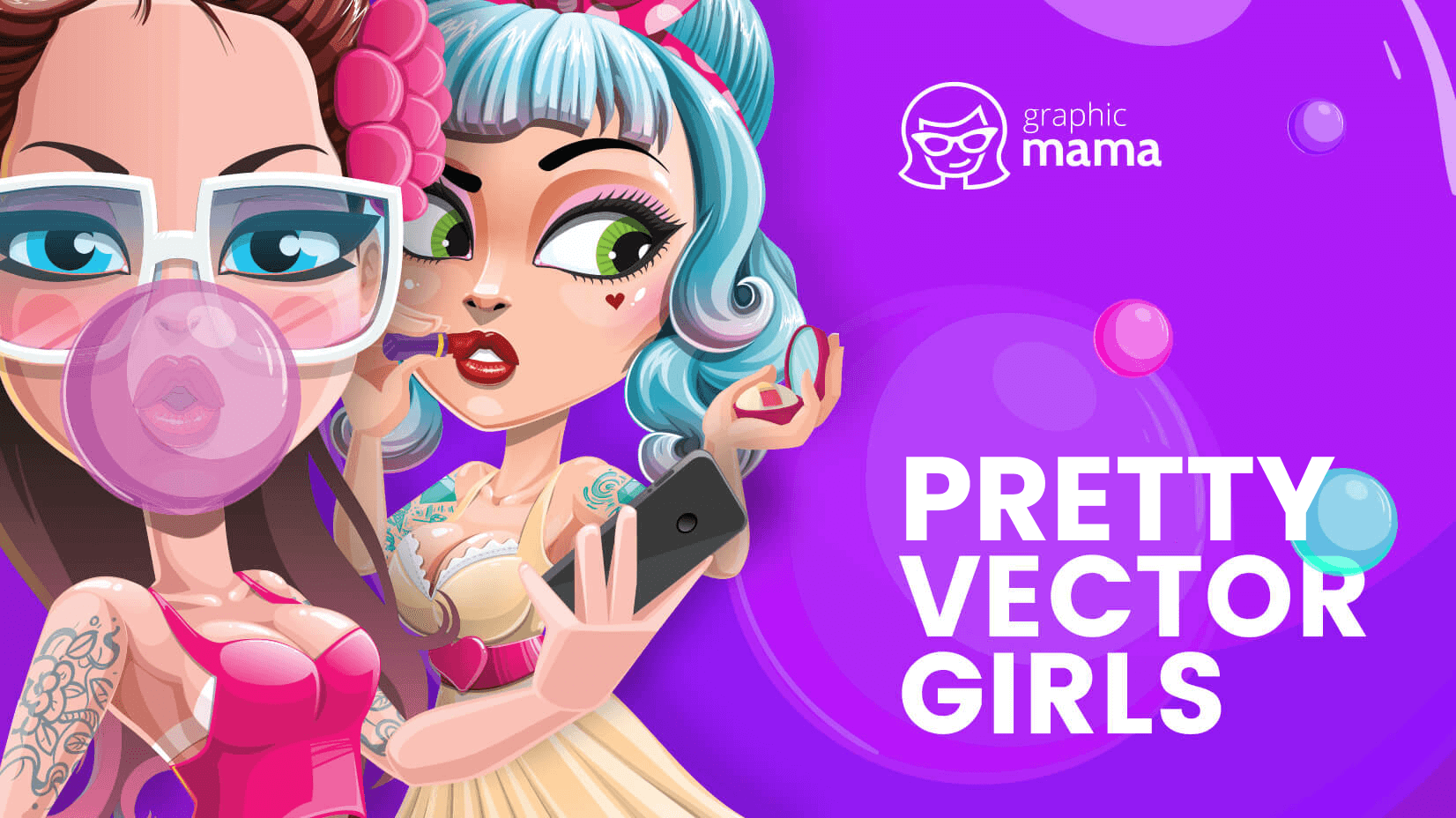
7 Pretty Vector Girls That Will Blow Your Mind
by Iveta Pavlova
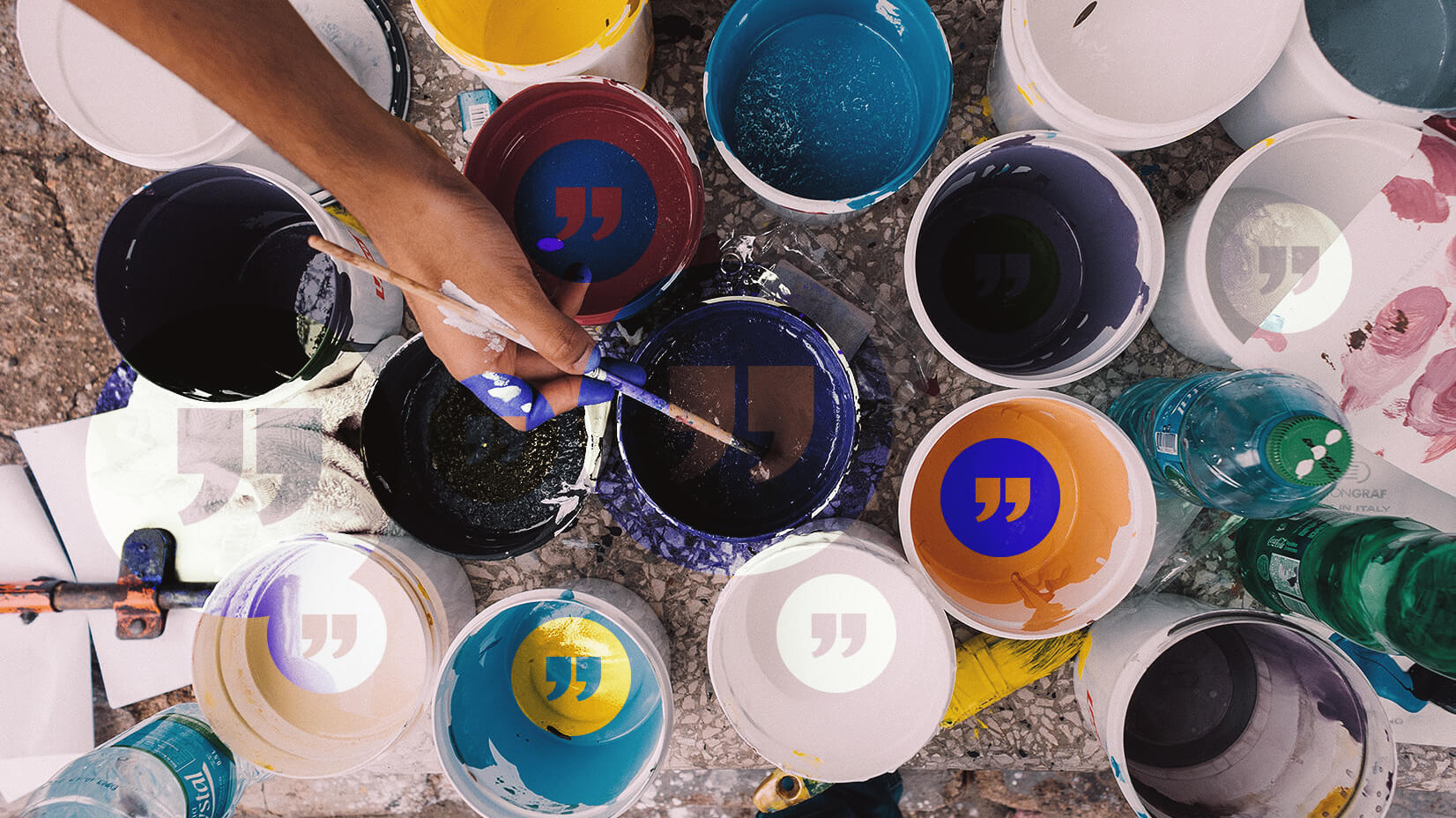
Inspiration
22 rare creativity quotes to awaken the artist in yourself.
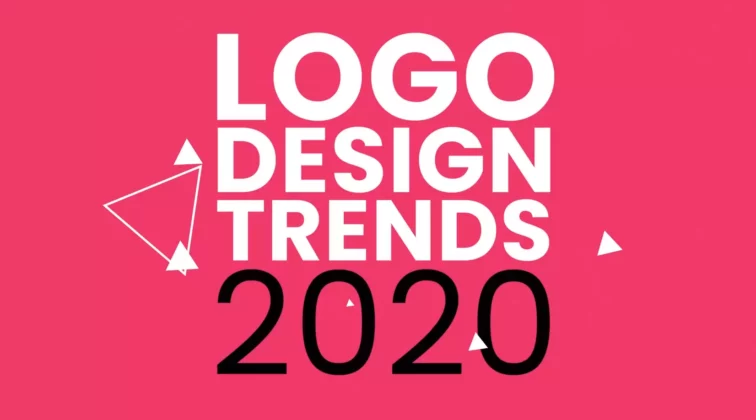
Logo Design Trends 2020: A Blast of Colors and Shapes
by Lyudmil Enchev
Looking for Design Bundles or Cartoon Characters?
A source of high-quality vector graphics offering a huge variety of premade character designs, graphic design bundles, Adobe Character Animator puppets, and more.
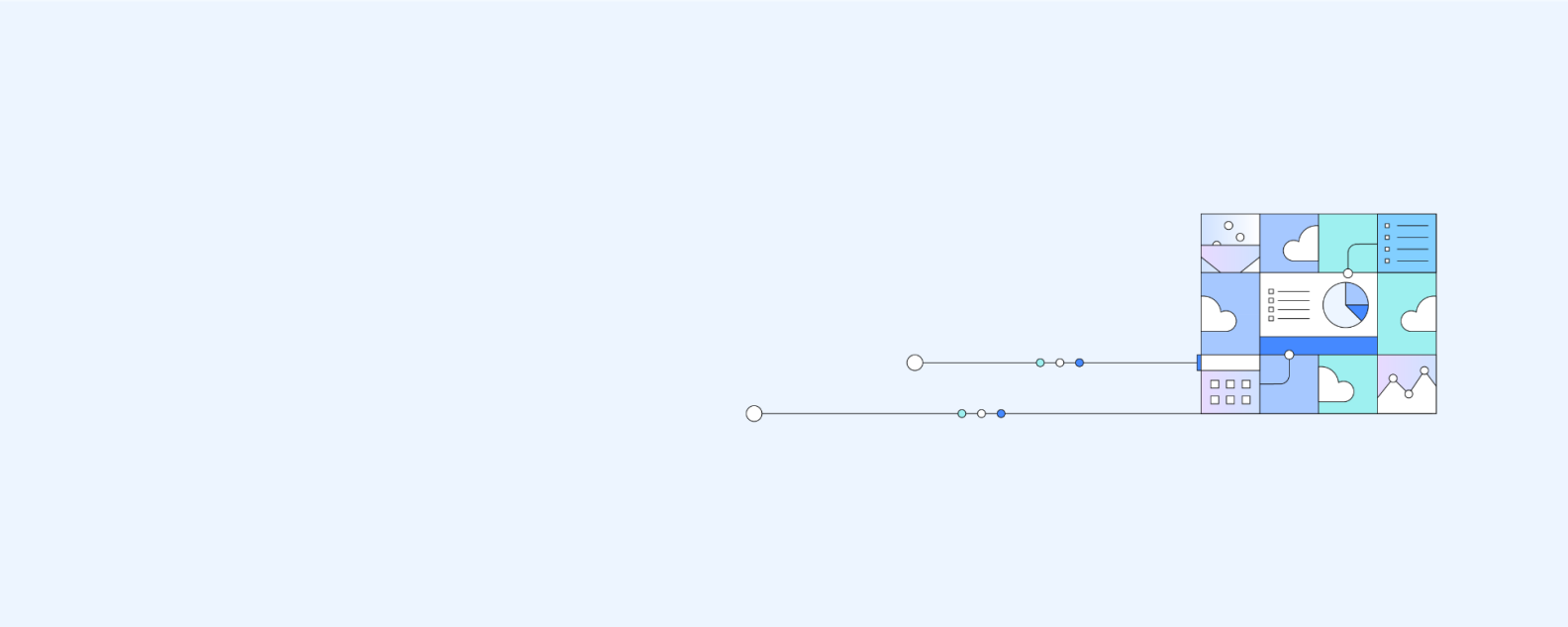
Data visualization is the representation of data through use of common graphics, such as charts, plots, infographics and even animations. These visual displays of information communicate complex data relationships and data-driven insights in a way that is easy to understand.
Data visualization can be utilized for a variety of purposes, and it’s important to note that is not only reserved for use by data teams. Management also leverages it to convey organizational structure and hierarchy while data analysts and data scientists use it to discover and explain patterns and trends. Harvard Business Review (link resides outside ibm.com) categorizes data visualization into four key purposes: idea generation, idea illustration, visual discovery, and everyday dataviz. We’ll delve deeper into these below:
Idea generation
Data visualization is commonly used to spur idea generation across teams. They are frequently leveraged during brainstorming or Design Thinking sessions at the start of a project by supporting the collection of different perspectives and highlighting the common concerns of the collective. While these visualizations are usually unpolished and unrefined, they help set the foundation within the project to ensure that the team is aligned on the problem that they’re looking to address for key stakeholders.
Idea illustration
Data visualization for idea illustration assists in conveying an idea, such as a tactic or process. It is commonly used in learning settings, such as tutorials, certification courses, centers of excellence, but it can also be used to represent organization structures or processes, facilitating communication between the right individuals for specific tasks. Project managers frequently use Gantt charts and waterfall charts to illustrate workflows . Data modeling also uses abstraction to represent and better understand data flow within an enterprise’s information system, making it easier for developers, business analysts, data architects, and others to understand the relationships in a database or data warehouse.
Visual discovery
Visual discovery and every day data viz are more closely aligned with data teams. While visual discovery helps data analysts, data scientists, and other data professionals identify patterns and trends within a dataset, every day data viz supports the subsequent storytelling after a new insight has been found.
Data visualization
Data visualization is a critical step in the data science process, helping teams and individuals convey data more effectively to colleagues and decision makers. Teams that manage reporting systems typically leverage defined template views to monitor performance. However, data visualization isn’t limited to performance dashboards. For example, while text mining an analyst may use a word cloud to to capture key concepts, trends, and hidden relationships within this unstructured data. Alternatively, they may utilize a graph structure to illustrate relationships between entities in a knowledge graph. There are a number of ways to represent different types of data, and it’s important to remember that it is a skillset that should extend beyond your core analytics team.
Use this model selection framework to choose the most appropriate model while balancing your performance requirements with cost, risks and deployment needs.
Register for the ebook on generative AI
The earliest form of data visualization can be traced back the Egyptians in the pre-17th century, largely used to assist in navigation. As time progressed, people leveraged data visualizations for broader applications, such as in economic, social, health disciplines. Perhaps most notably, Edward Tufte published The Visual Display of Quantitative Information (link resides outside ibm.com), which illustrated that individuals could utilize data visualization to present data in a more effective manner. His book continues to stand the test of time, especially as companies turn to dashboards to report their performance metrics in real-time. Dashboards are effective data visualization tools for tracking and visualizing data from multiple data sources, providing visibility into the effects of specific behaviors by a team or an adjacent one on performance. Dashboards include common visualization techniques, such as:
- Tables: This consists of rows and columns used to compare variables. Tables can show a great deal of information in a structured way, but they can also overwhelm users that are simply looking for high-level trends.
- Pie charts and stacked bar charts: These graphs are divided into sections that represent parts of a whole. They provide a simple way to organize data and compare the size of each component to one other.
- Line charts and area charts: These visuals show change in one or more quantities by plotting a series of data points over time and are frequently used within predictive analytics. Line graphs utilize lines to demonstrate these changes while area charts connect data points with line segments, stacking variables on top of one another and using color to distinguish between variables.
- Histograms: This graph plots a distribution of numbers using a bar chart (with no spaces between the bars), representing the quantity of data that falls within a particular range. This visual makes it easy for an end user to identify outliers within a given dataset.
- Scatter plots: These visuals are beneficial in reveling the relationship between two variables, and they are commonly used within regression data analysis. However, these can sometimes be confused with bubble charts, which are used to visualize three variables via the x-axis, the y-axis, and the size of the bubble.
- Heat maps: These graphical representation displays are helpful in visualizing behavioral data by location. This can be a location on a map, or even a webpage.
- Tree maps, which display hierarchical data as a set of nested shapes, typically rectangles. Treemaps are great for comparing the proportions between categories via their area size.
Access to data visualization tools has never been easier. Open source libraries, such as D3.js, provide a way for analysts to present data in an interactive way, allowing them to engage a broader audience with new data. Some of the most popular open source visualization libraries include:
- D3.js: It is a front-end JavaScript library for producing dynamic, interactive data visualizations in web browsers. D3.js (link resides outside ibm.com) uses HTML, CSS, and SVG to create visual representations of data that can be viewed on any browser. It also provides features for interactions and animations.
- ECharts: A powerful charting and visualization library that offers an easy way to add intuitive, interactive, and highly customizable charts to products, research papers, presentations, etc. Echarts (link resides outside ibm.com) is based in JavaScript and ZRender, a lightweight canvas library.
- Vega: Vega (link resides outside ibm.com) defines itself as “visualization grammar,” providing support to customize visualizations across large datasets which are accessible from the web.
- deck.gl: It is part of Uber's open source visualization framework suite. deck.gl (link resides outside ibm.com) is a framework, which is used for exploratory data analysis on big data. It helps build high-performance GPU-powered visualization on the web.
With so many data visualization tools readily available, there has also been a rise in ineffective information visualization. Visual communication should be simple and deliberate to ensure that your data visualization helps your target audience arrive at your intended insight or conclusion. The following best practices can help ensure your data visualization is useful and clear:
Set the context: It’s important to provide general background information to ground the audience around why this particular data point is important. For example, if e-mail open rates were underperforming, we may want to illustrate how a company’s open rate compares to the overall industry, demonstrating that the company has a problem within this marketing channel. To drive an action, the audience needs to understand how current performance compares to something tangible, like a goal, benchmark, or other key performance indicators (KPIs).
Know your audience(s): Think about who your visualization is designed for and then make sure your data visualization fits their needs. What is that person trying to accomplish? What kind of questions do they care about? Does your visualization address their concerns? You’ll want the data that you provide to motivate people to act within their scope of their role. If you’re unsure if the visualization is clear, present it to one or two people within your target audience to get feedback, allowing you to make additional edits prior to a large presentation.
Choose an effective visual: Specific visuals are designed for specific types of datasets. For instance, scatter plots display the relationship between two variables well, while line graphs display time series data well. Ensure that the visual actually assists the audience in understanding your main takeaway. Misalignment of charts and data can result in the opposite, confusing your audience further versus providing clarity.
Keep it simple: Data visualization tools can make it easy to add all sorts of information to your visual. However, just because you can, it doesn’t mean that you should! In data visualization, you want to be very deliberate about the additional information that you add to focus user attention. For example, do you need data labels on every bar in your bar chart? Perhaps you only need one or two to help illustrate your point. Do you need a variety of colors to communicate your idea? Are you using colors that are accessible to a wide range of audiences (e.g. accounting for color blind audiences)? Design your data visualization for maximum impact by eliminating information that may distract your target audience.
An AI-infused integrated planning solution that helps you transcend the limits of manual planning.
Build, run and manage AI models. Prepare data and build models on any cloud using open source code or visual modeling. Predict and optimize your outcomes.
Unlock the value of enterprise data and build an insight-driven organization that delivers business advantage with IBM Consulting.
Your trusted Watson co-pilot for smarter analytics and confident decisions.
Use features within IBM Watson® Studio that help you visualize and gain insights into your data, then cleanse and transform your data to build high-quality predictive models.
Data Refinery makes it easy to explore, prepare, and deliver data that people across your organization can trust.
Learn how to use Apache Superset (a modern, enterprise-ready business intelligence web application) with Netezza database to uncover the story behind the data.
Predict outcomes with flexible AI-infused forecasting and analyze what-if scenarios in real-time. IBM Planning Analytics is an integrated business planning solution that turns raw data into actionable insights. Deploy as you need, on-premises or on cloud.
We use essential cookies to make Venngage work. By clicking “Accept All Cookies”, you agree to the storing of cookies on your device to enhance site navigation, analyze site usage, and assist in our marketing efforts.
Manage Cookies
Cookies and similar technologies collect certain information about how you’re using our website. Some of them are essential, and without them you wouldn’t be able to use Venngage. But others are optional, and you get to choose whether we use them or not.
Strictly Necessary Cookies
These cookies are always on, as they’re essential for making Venngage work, and making it safe. Without these cookies, services you’ve asked for can’t be provided.
Show cookie providers
- Google Login
Functionality Cookies
These cookies help us provide enhanced functionality and personalisation, and remember your settings. They may be set by us or by third party providers.
Performance Cookies
These cookies help us analyze how many people are using Venngage, where they come from and how they're using it. If you opt out of these cookies, we can’t get feedback to make Venngage better for you and all our users.
- Google Analytics
Targeting Cookies
These cookies are set by our advertising partners to track your activity and show you relevant Venngage ads on other sites as you browse the internet.
- Google Tag Manager
Infographics
- Daily Infographics
- Popular Templates
- Accessibility
- Graphic Design
- Graphs and Charts
- Data Visualization
- Human Resources
- Beginner Guides
Blog Graphic Design What is Data Visualization? (Definition, Examples, Best Practices)
What is Data Visualization? (Definition, Examples, Best Practices)
Written by: Midori Nediger Jun 05, 2020
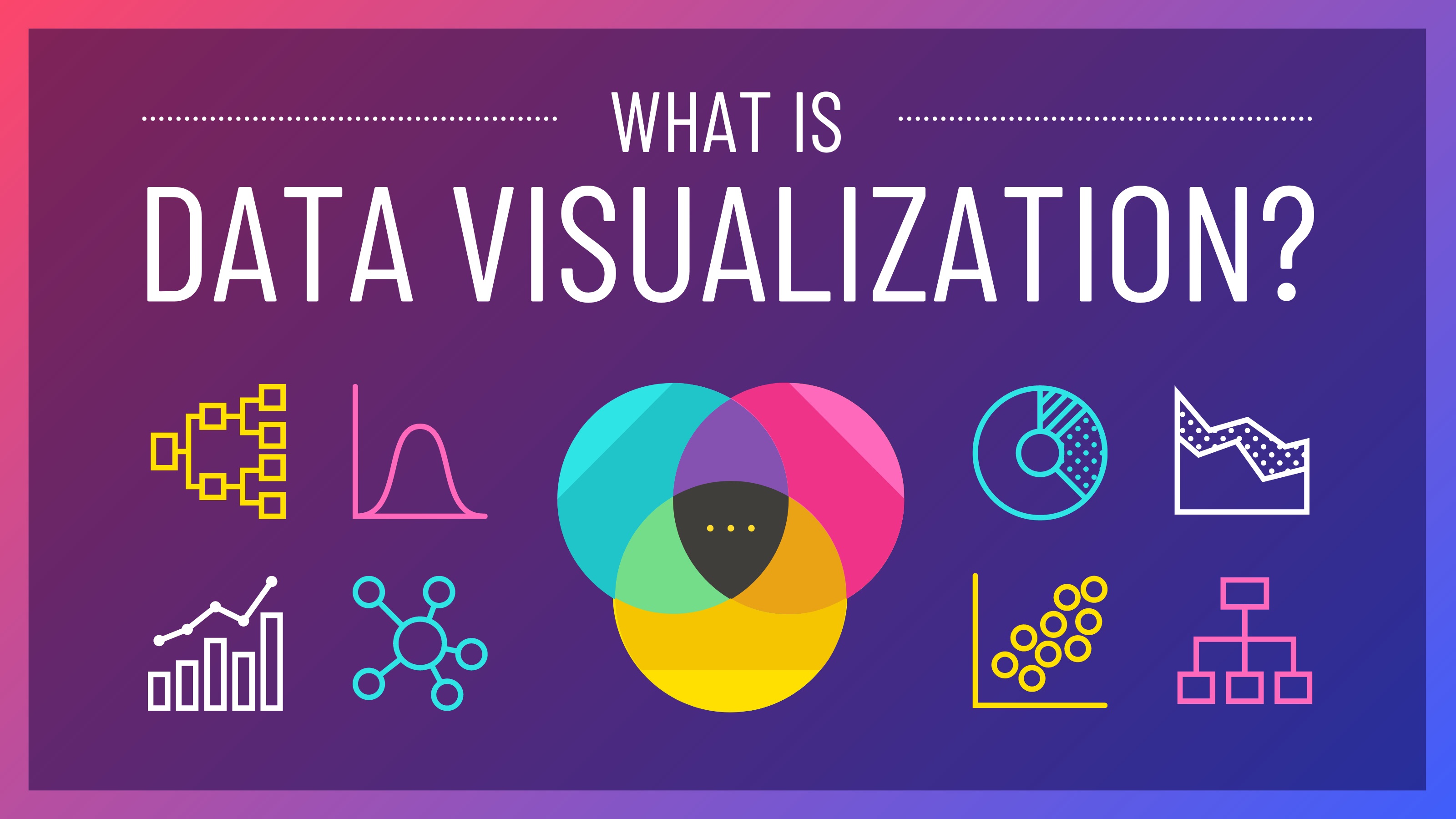
Words don’t always paint the clearest picture. Raw data doesn’t always tell the most compelling story.
The human mind is very receptive to visual information. That’s why data visualization is a powerful tool for communication.
But if “data visualization” sounds tricky and technical don’t worry—it doesn’t have to be.
This guide will explain the fundamentals of data visualization in a way that anyone can understand. Included are a ton of examples of different types of data visualizations and when to use them for your reports, presentations, marketing, and more.
Table of Contents
- What is data visualization?
What is data visualization used for?
Types of data visualizations.
- How to present data visually (for businesses, marketers, nonprofits, and education)
- Data visualization examples
Data visualization is used everywhere.
Businesses use data visualization for reporting, forecasting, and marketing.
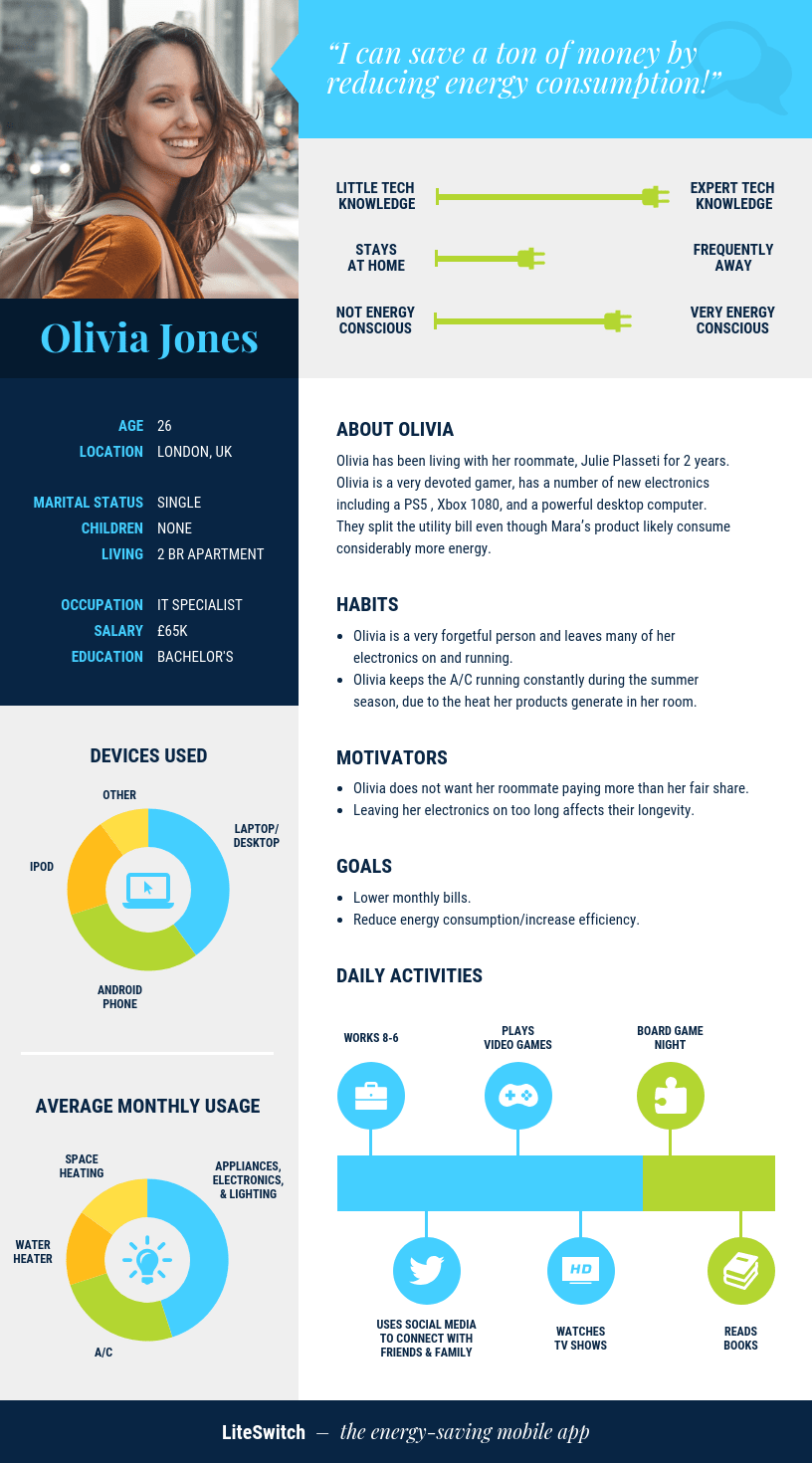
CREATE THIS REPORT TEMPLATE
Nonprofits use data visualizations to put stories and faces to numbers.
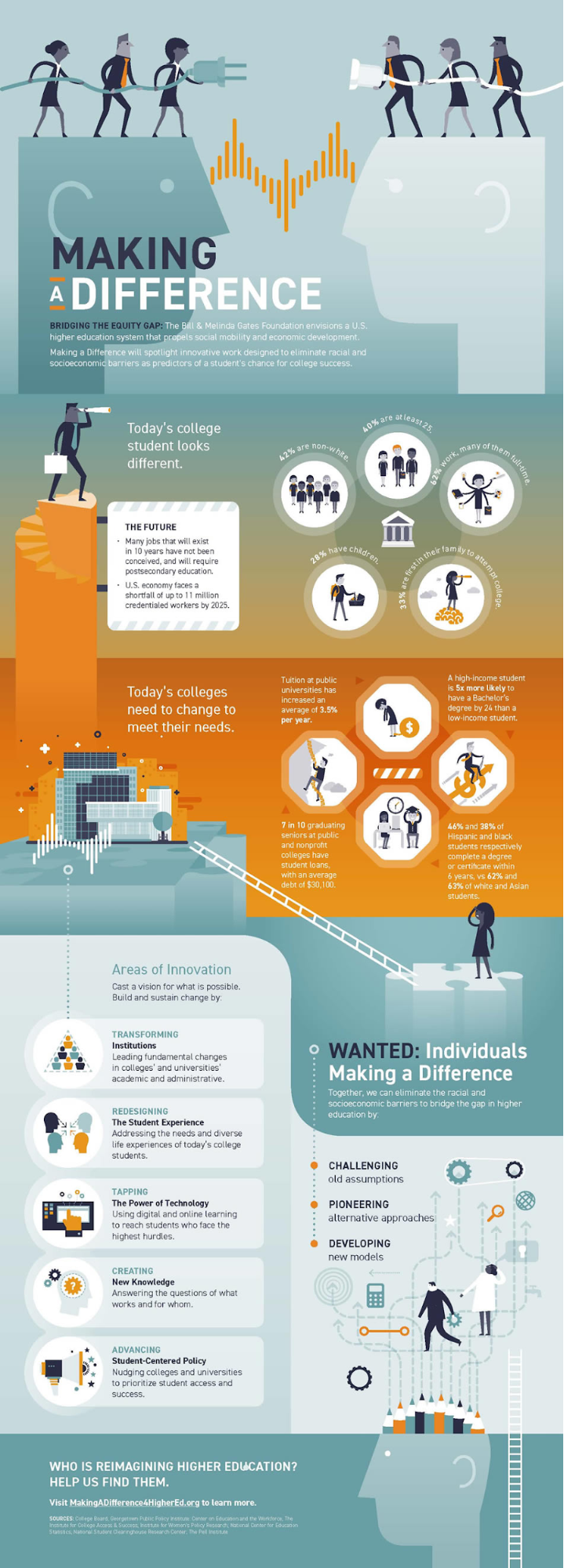
Source: Bill and Melinda Gates Foundation
Scholars and scientists use data visualization to illustrate concepts and reinforce their arguments.
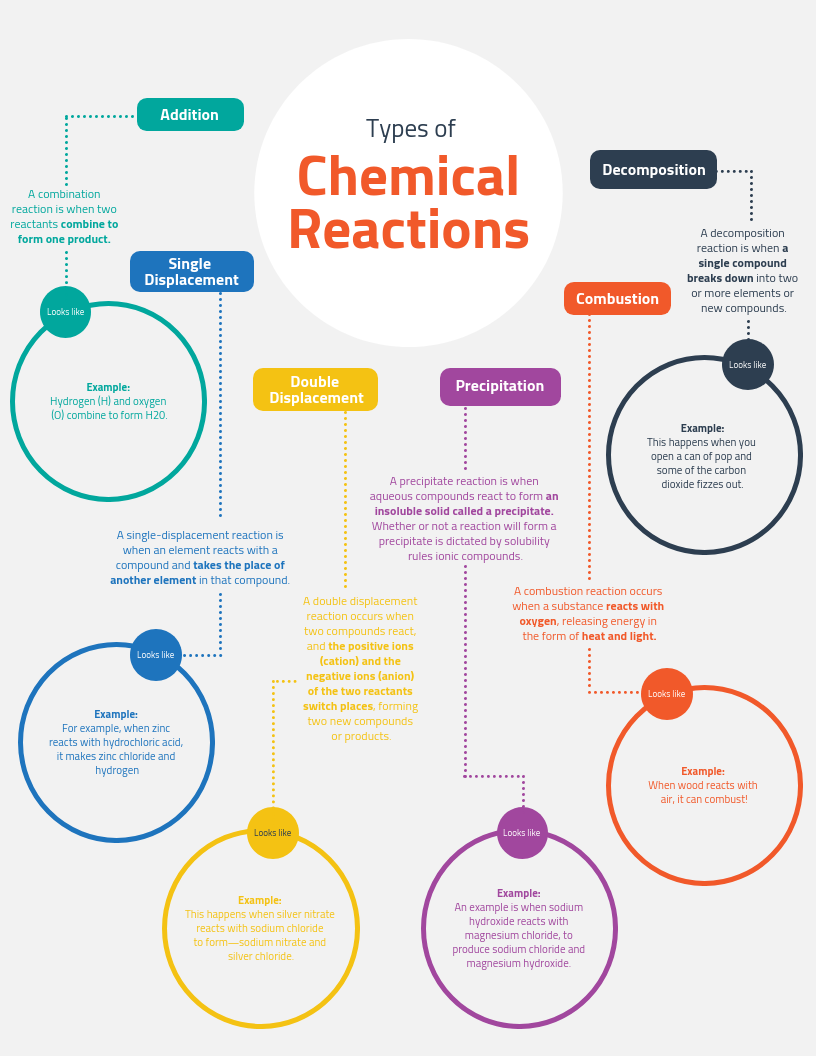
CREATE THIS MIND MAP TEMPLATE
Reporters use data visualization to show trends and contextualize stories.
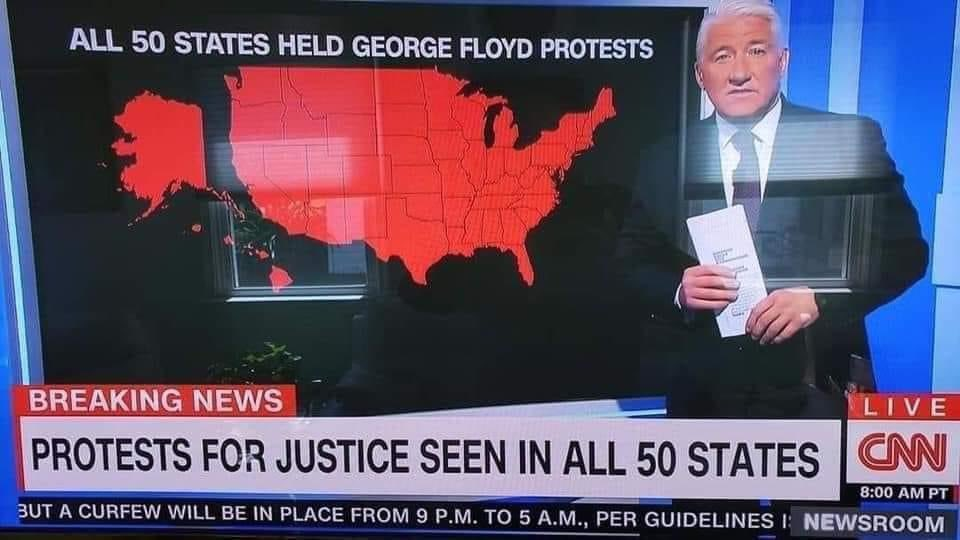
While data visualizations can make your work more professional, they can also be a lot of fun.
What is data visualization? A simple definition of data visualization:
Data visualization is the visual presentation of data or information. The goal of data visualization is to communicate data or information clearly and effectively to readers. Typically, data is visualized in the form of a chart , infographic , diagram or map.
The field of data visualization combines both art and data science. While a data visualization can be creative and pleasing to look at, it should also be functional in its visual communication of the data.
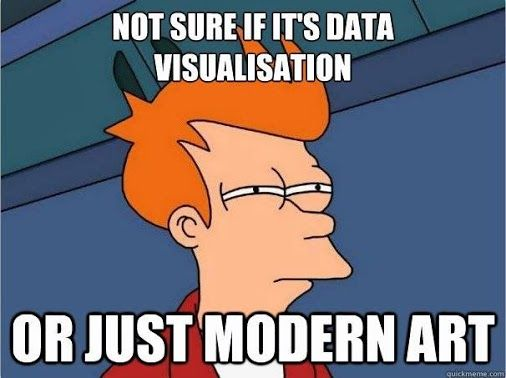
Data, especially a lot of data, can be difficult to wrap your head around. Data visualization can help both you and your audience interpret and understand data.
Data visualizations often use elements of visual storytelling to communicate a message supported by the data.
There are many situations where you would want to present data visually.
Data visualization can be used for:
- Making data engaging and easily digestible
- Identifying trends and outliers within a set of data
- Telling a story found within the data
- Reinforcing an argument or opinion
- Highlighting the important parts of a set of data
Let’s look at some examples for each use case.
1. Make data digestible and easy to understand
Often, a large set of numbers can make us go cross-eyed. It can be difficult to find the significance behind rows of data.
Data visualization allows us to frame the data differently by using illustrations, charts, descriptive text, and engaging design. Visualization also allows us to group and organize data based on categories and themes, which can make it easier to break down into understandable chunks.
Related : How to Use Data Visualization in Your Infographics
For example, this infographic breaks down the concept of neuroplasticity in an approachable way:
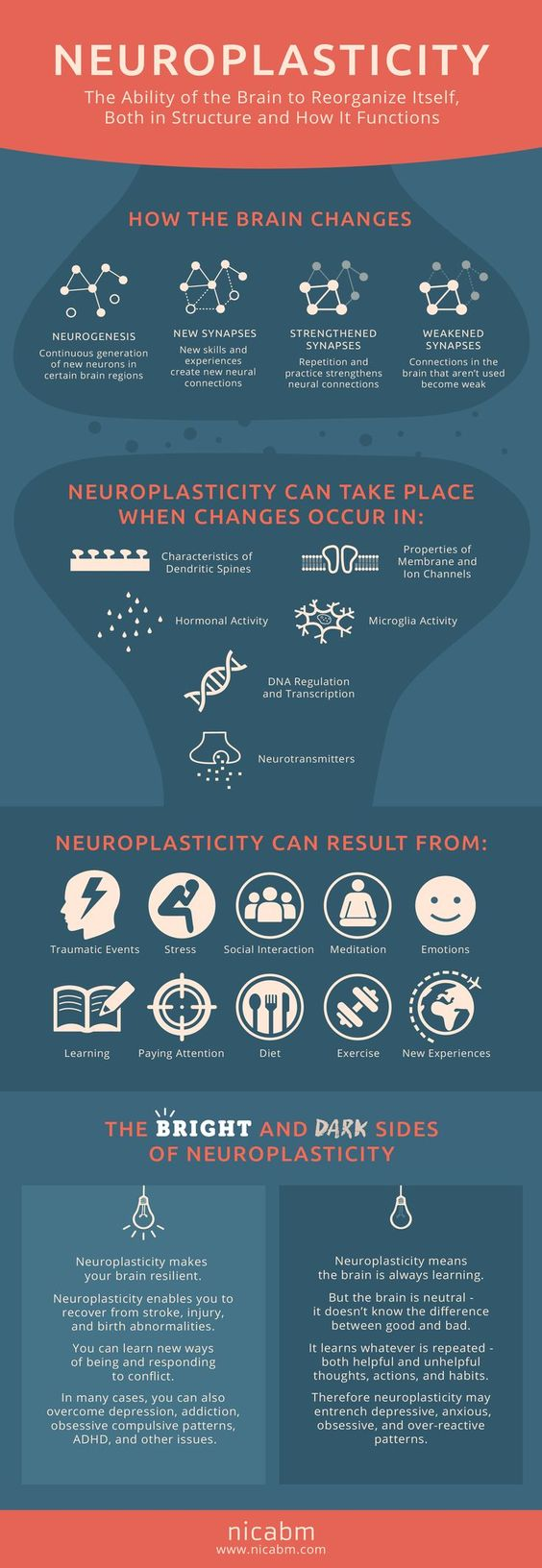
Source: NICABM
The same goes for complex, specialized concepts. It can often be difficult to break down the information in a way that non-specialists will understand. But an infographic that organizes the information, with visuals, can demystify concepts for novice readers.
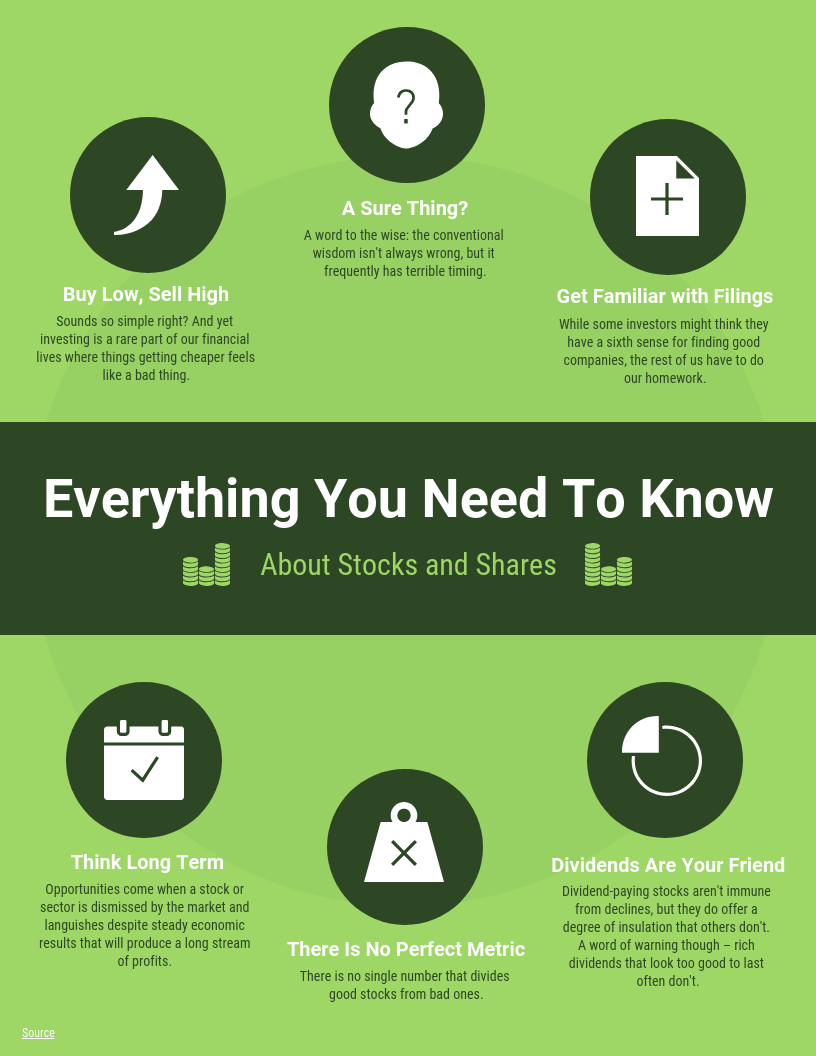
CREATE THIS INFOGRAPHIC TEMPLATE
NEW! Introducing: Marketing Statistics Report 2022
It’s 2022 already. Marketers, are you still using data from pre-COVID times?
Don’t make decisions based on outdated data that no longer applies. It’s time you keep yourself informed of the latest marketing statistics and trends during the past two years, and learn how COVID-19 has affected marketing efforts in different industries — with this FREE marketing statistics report put together by Venngage and HubSpot .
The report uses data gathered from over 100,000 customers of HubSpot CRM. In addition to that, you’ll also know about the trends in using visuals in content marketing and the impacts of the pandemic on visual content, from 200+ marketers all over the world interviewed by Venngage.
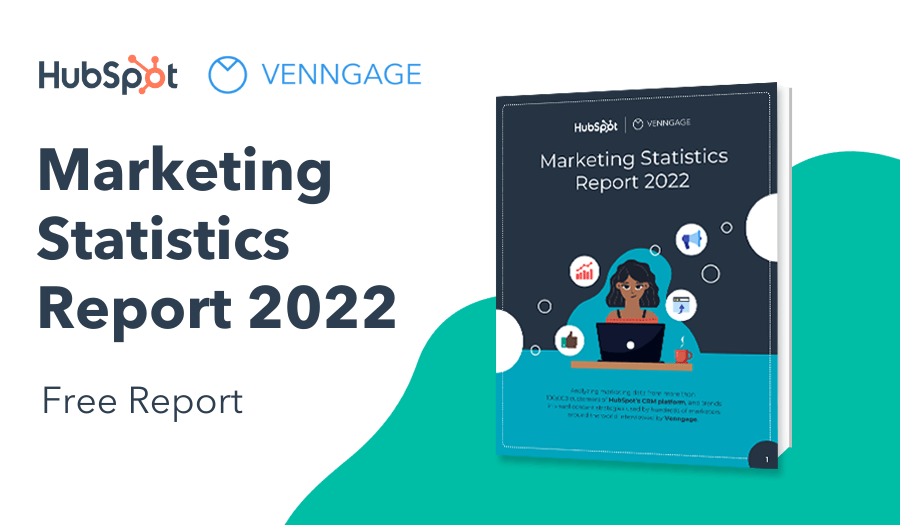
GET YOUR FREE COPY
2. Identify trends and outliers
If you were to sift through raw data manually, it could take ages to notice patterns, trends or outlying data. But by using data visualization tools like charts, you can sort through a lot of data quickly.
Even better, charts enable you to pick up on trends a lot quicker than you would sifting through numbers.
For example, here’s a simple chart generated by Google Search Console that shows the change in Google searches for “toilet paper”. As you can see, in March 2020 there was a huge increase in searches for toilet paper:
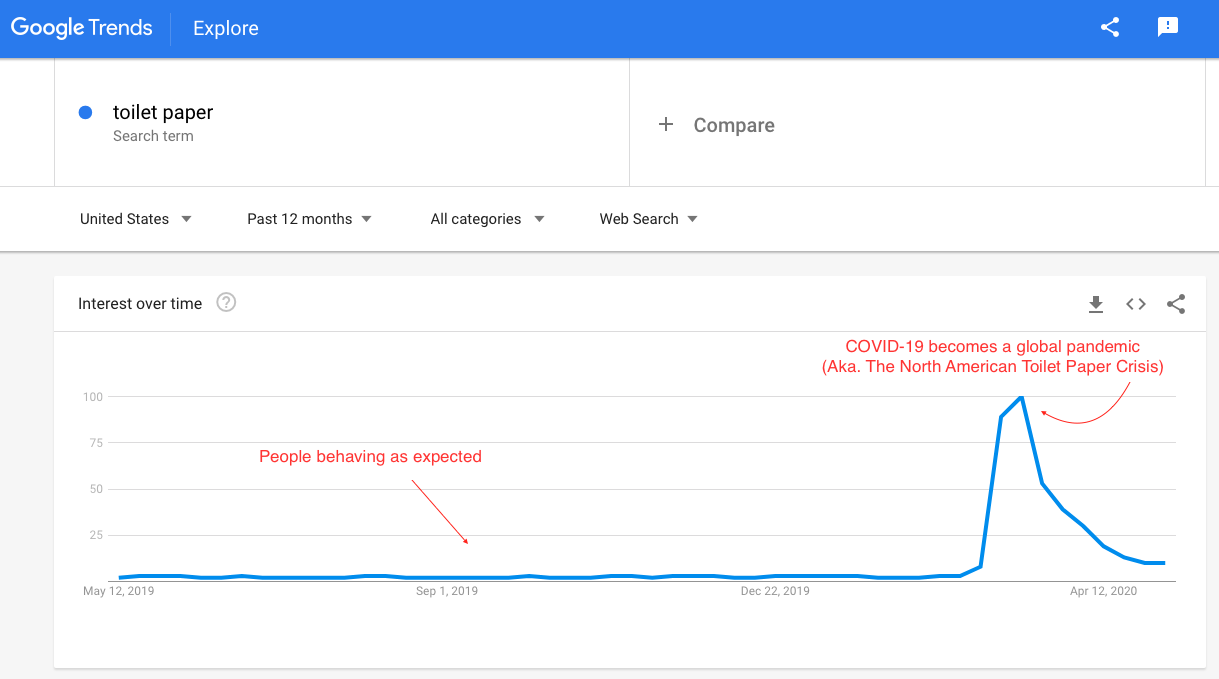
Source: How to Use SEO Data to Fuel Your Content Marketing Strategy in 2020
This chart shows an outlier in the general trend for toilet paper-related Google searches. The reason for the outlier? The outbreak of COVID-19 in North America. With a simple data visualization, we’ve been able to highlight an outlier and hint at a story behind the data.
Uploading your data into charts, to create these kinds of visuals is easy. While working on your design in the editor, select a chart from the left panel. Open the chart and find the green IMPORT button under the DATA tab. Then upload the CSV file and your chart automatically visualizes the information.
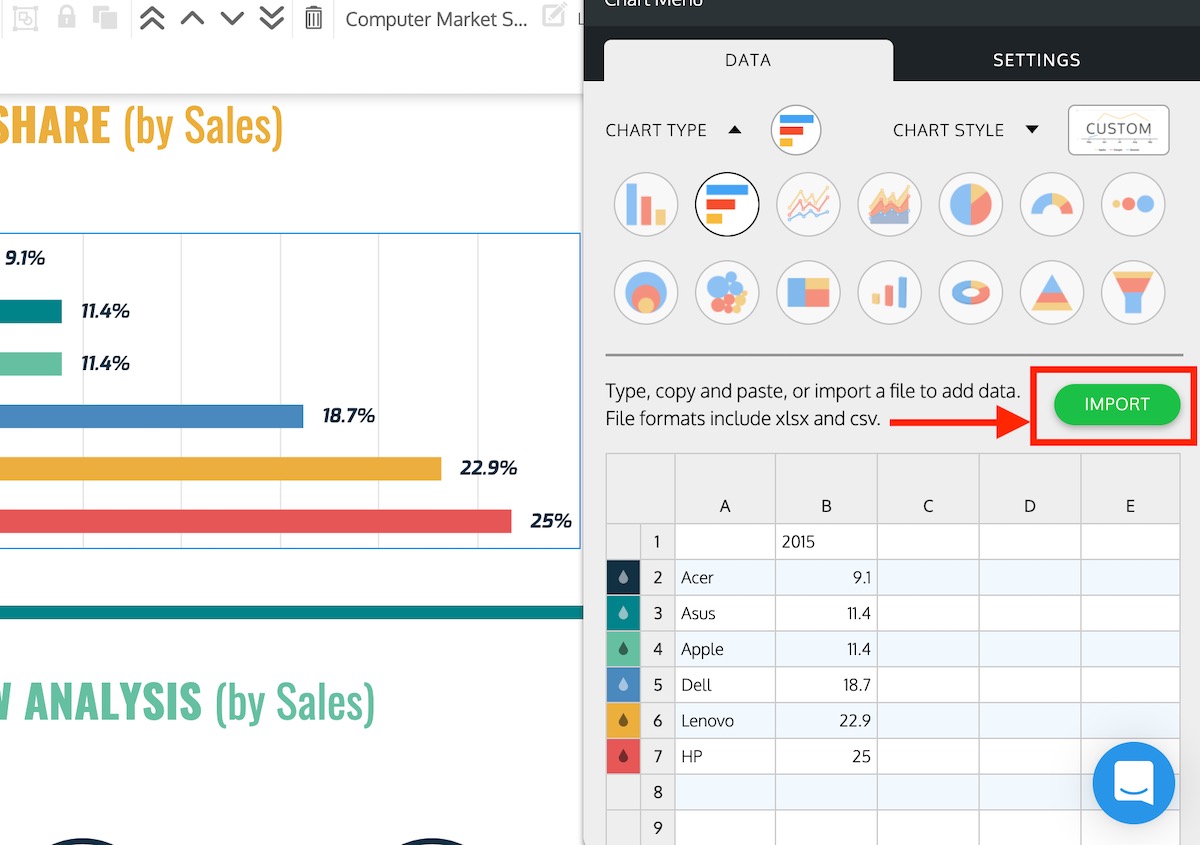
3. Tell a story within the data
Numbers on their own don’t tend to evoke an emotional response. But data visualization can tell a story that gives significance to the data.
Designers use techniques like color theory , illustrations, design style and visual cues to appeal to the emotions of readers, put faces to numbers, and introduce a narrative to the data.
Related : How to Tell a Story With Data (A Guide for Beginners)
For example, here’s an infographic created by World Vision. In the infographics, numbers are visualized using illustrations of cups. While comparing numbers might impress readers, reinforcing those numbers with illustrations helps to make an even greater impact.
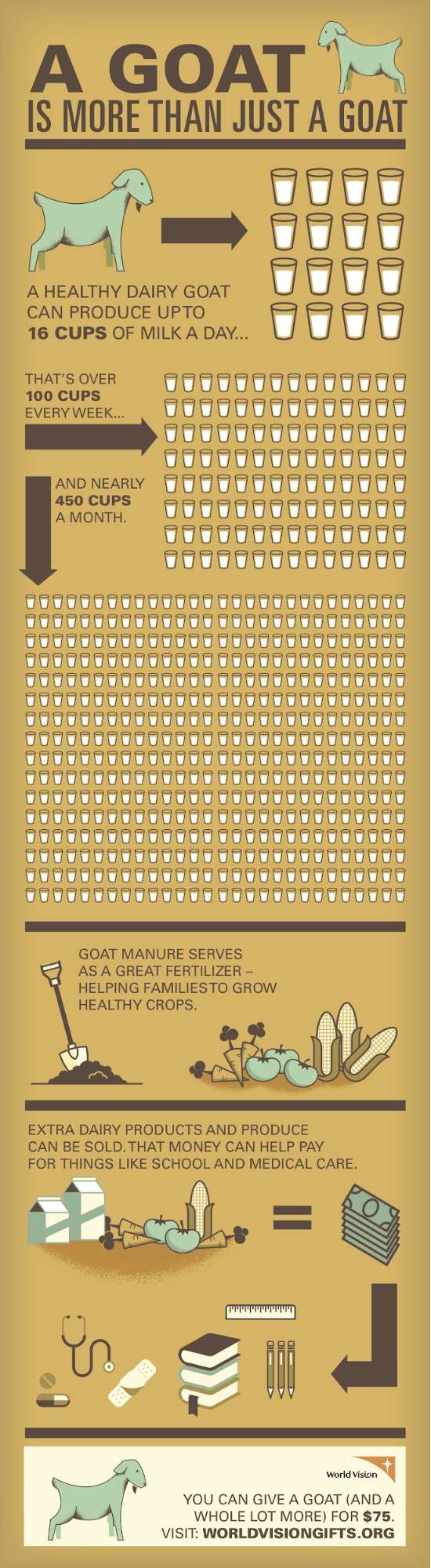
Source: World Vision
Meanwhile, this infographic uses data to draw attention to an often overlooked issue:

Read More: The Coronavirus Pandemic and the Refugee Crisis
4. Reinforce an argument or opinion
When it comes to convincing people your opinion is right, they often have to see it to believe it. An effective infographic or chart can make your argument more robust and reinforce your creativity.
For example, you can use a comparison infographic to compare sides of an argument, different theories, product/service options, pros and cons, and more. Especially if you’re blending data types.
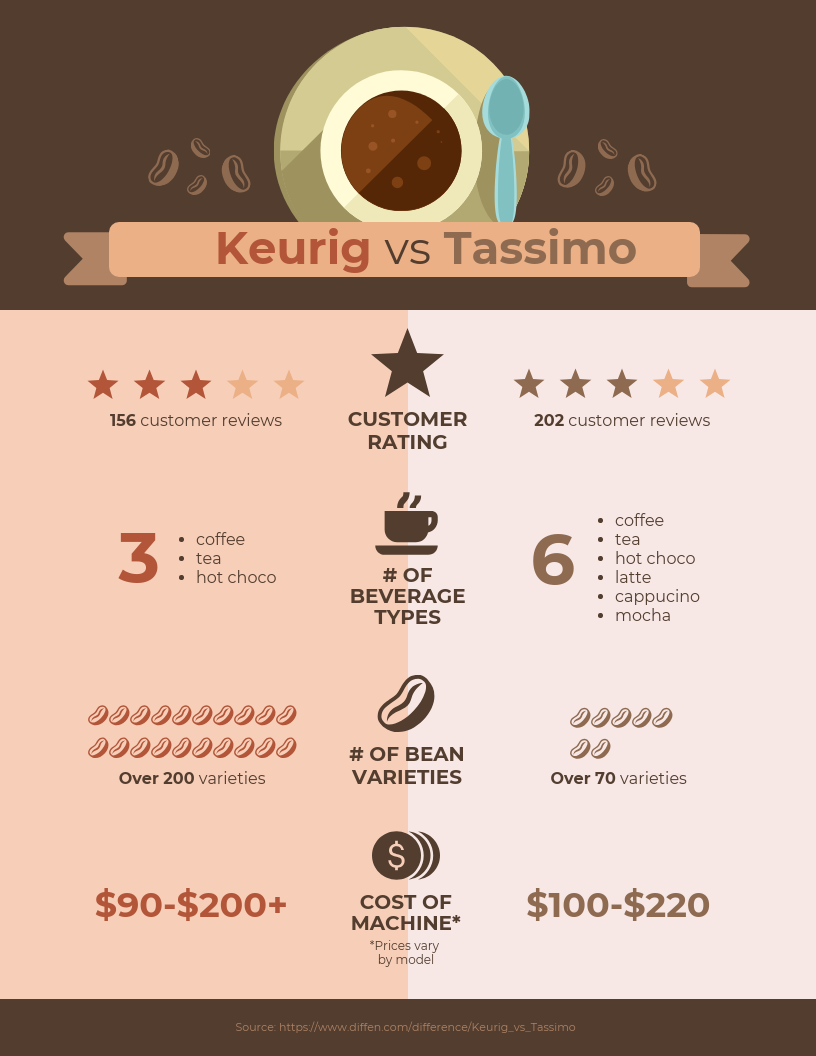
5. Highlight an important point in a set of data
Sometimes we use data visualizations to make it easier for readers to explore the data and come to their own conclusions. But often, we use data visualizations to tell a story, make a particular argument, or encourage readers to come to a specific conclusion.
Designers use visual cues to direct the eye to different places on a page. Visual cues are shapes, symbols, and colors that point to a specific part of the data visualization, or that make a specific part stand out.
For example, in this data visualization, contrasting colors are used to emphasize the difference in the amount of waste sent to landfills versus recycled waste:
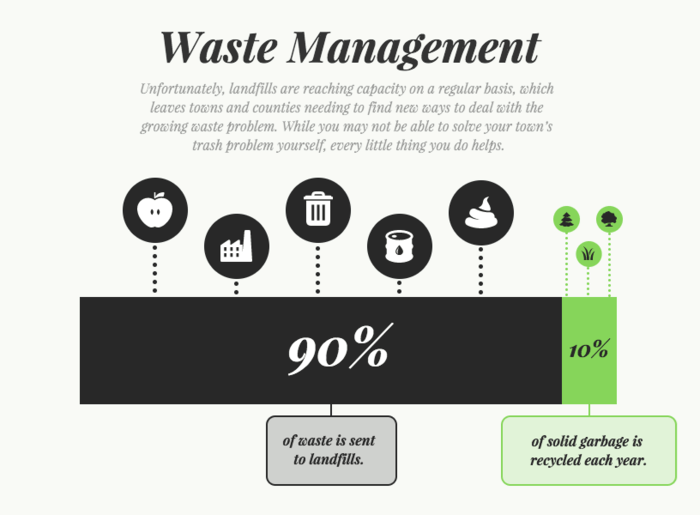
Here’s another example. This time, a red circle and an arrow are used to highlight points on the chart where the numbers show a drop:
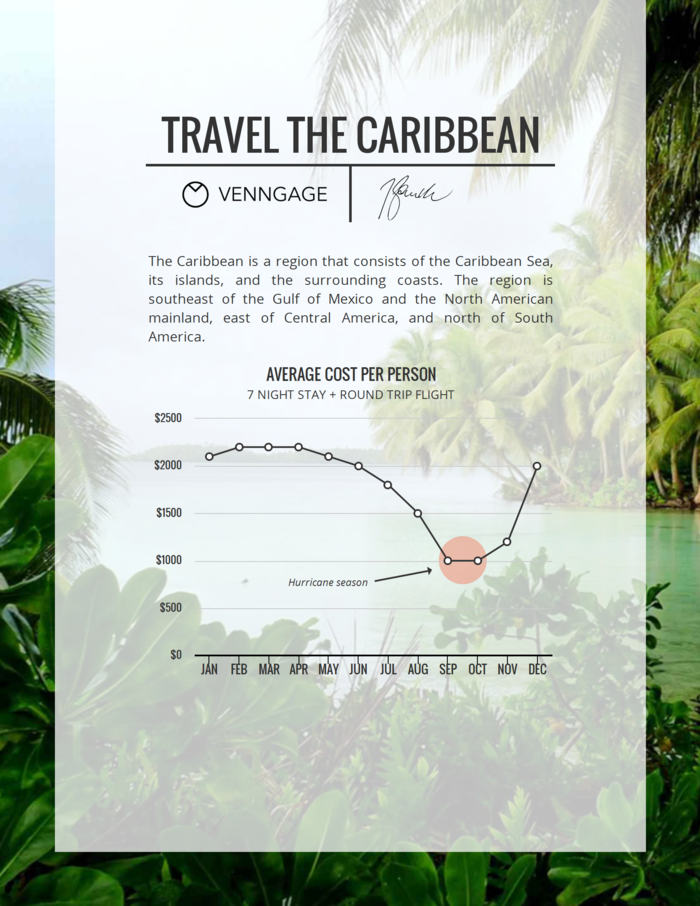
Highlighting specific data points helps your data visualization tell a compelling story.
6. Make books, blog posts, reports and videos more engaging
At Venngage, we use data visualization to make our blog posts more engaging for readers. When we write a blog post or share a post on social media, we like to summarize key points from our content using infographics.
The added benefit of creating engaging visuals like infographics is that it has enabled our site to be featured in publications like The Wall Street Journal , Mashable , Business Insider , The Huffington Post and more.
That’s because data visualizations are different from a lot of other types of content people consume on a daily basis. They make your brain work. They combine concrete facts and numbers with impactful visual elements. They make complex concepts easier to grasp.
Here’s an example of an infographic we made that got a lot of media buzz:
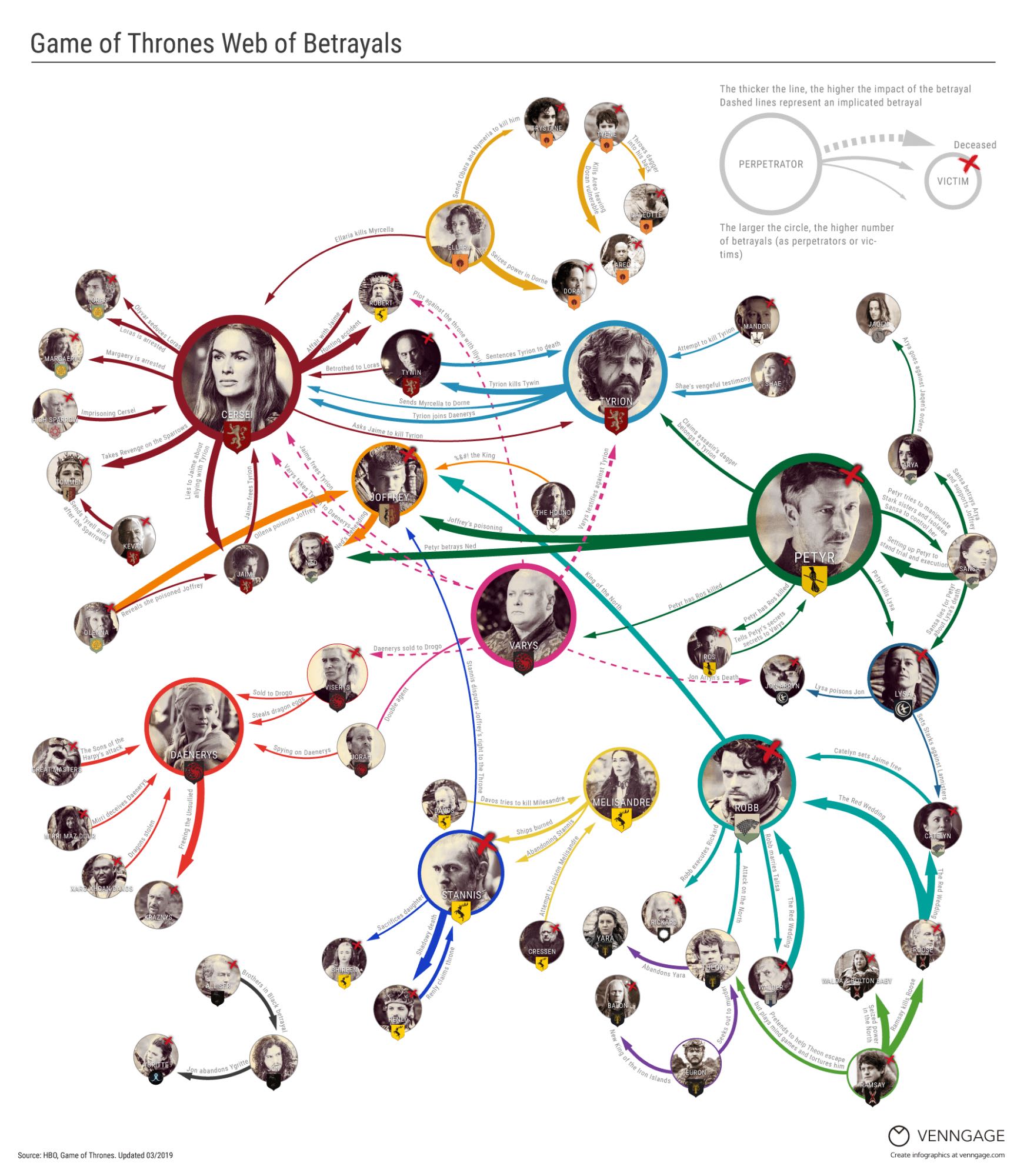
Read the Blog Post: Every Betrayal Ever in Game of Thrones
We created this infographic because a bunch of people on our team are big Game of Thrones fans and we wanted to create a visual that would help other fans follow the show. Because we approached a topic that a lot of people cared about in an original way, the infographic got picked up by a bunch of media sites.
Whether you’re a website looking to promote your content, a journalist looking for an original angle, or a creative building your portfolio, data visualizations can be an effective way to get people’s attention.
Data visualizations can come in many different forms. People are always coming up with new and creative ways to present data visually.
Generally speaking, data visualizations usually fall under these main categories:
An infographic is a collection of imagery, charts, and minimal text that gives an easy-to-understand overview of a topic.
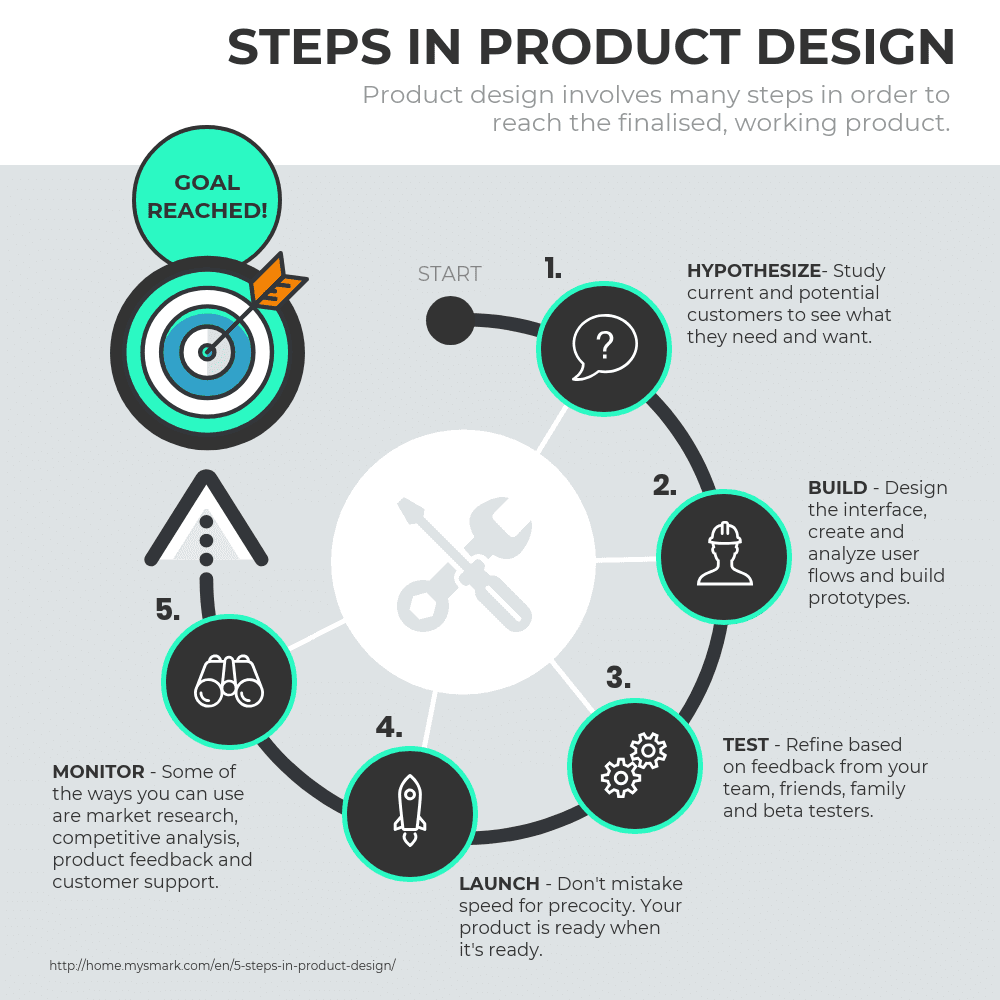
While infographics can take many forms, they can typically be categorized by these infographic types:
- Statistical infographics
- Informational infographics
- Timeline infographics
- Process infographics
- Geographic infographics
- Comparison infographics
- Hierarchical infographics
- List infographics
- Resume infographics
Read More: What is an Infographic? Examples, Templates & Design Tips
Charts
In the simplest terms, a chart is a graphical representation of data. Charts use visual symbols like line, bars, dots, slices, and icons to represent data points.
Some of the most common types of charts are:
- Bar graphs /charts
- Line charts
- Bubble charts
- Stacked bar charts
- Word clouds
- Pictographs
- Area charts
- Scatter plot charts
- Multi-series charts
The question that inevitably follows is: what type of chart should I use to visualize my data? Does it matter?
Short answer: yes, it matters. Choosing a type of chart that doesn’t work with your data can end up misrepresenting and skewing your data.
For example: if you’ve been in the data viz biz for a while, then you may have heard some of the controversy surrounding pie charts. A rookie mistake that people often make is using a pie chart when a bar chart would work better.
Pie charts display portions of a whole. A pie chart works when you want to compare proportions that are substantially different. Like this:
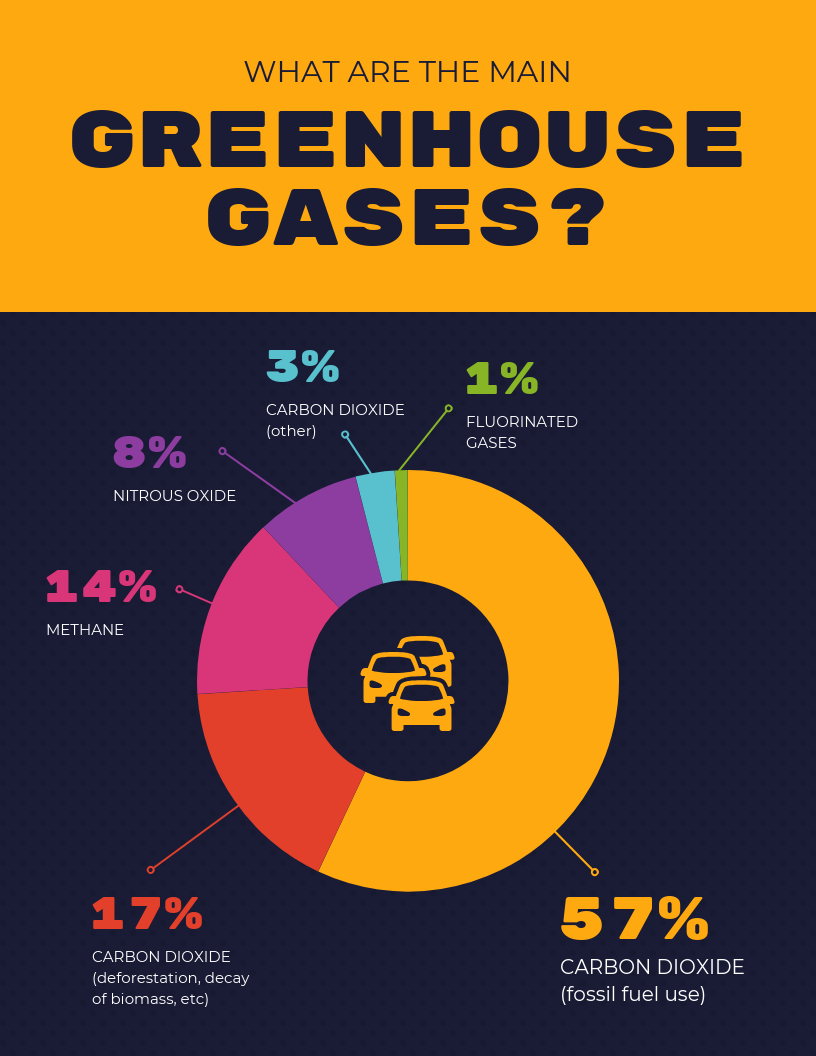
CREATE THIS CHART TEMPLATE
But when your proportions are similar, a pie chart can make it difficult to tell which slice is bigger than the other. That’s why, in most other cases, a bar chart is a safer bet.
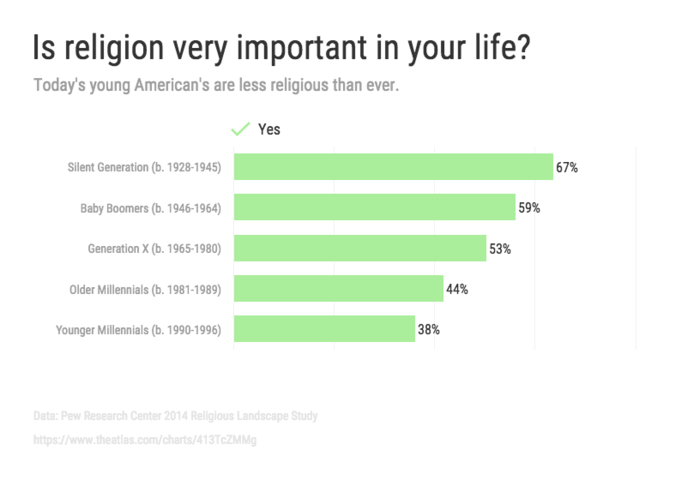
Here is a cheat sheet to help you pick the right type of chart for your data:
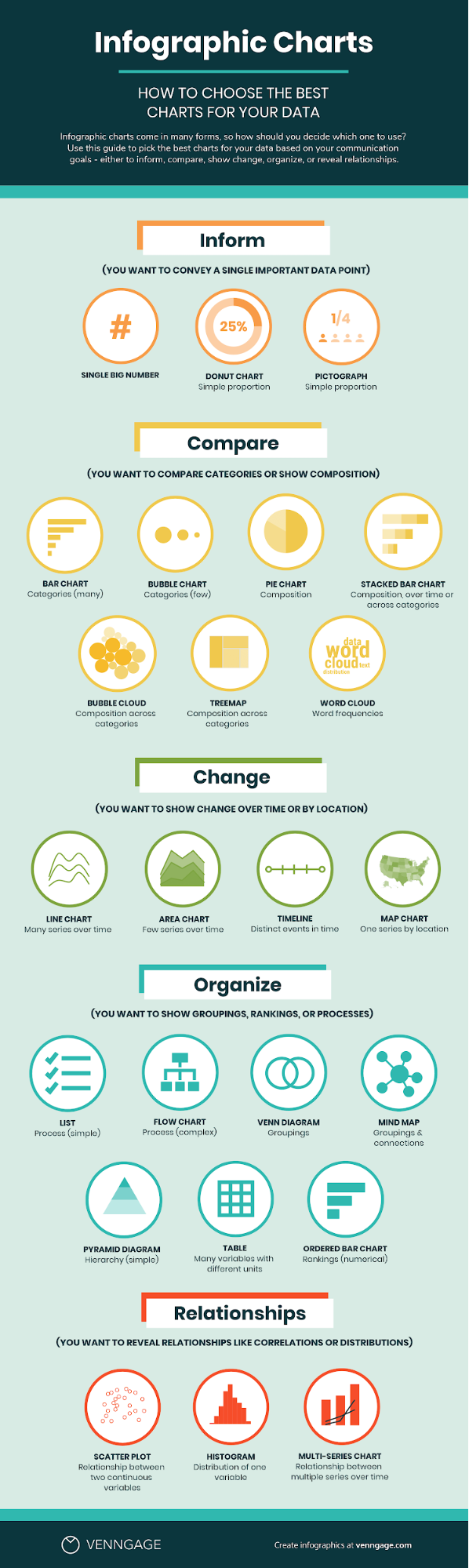
Want to make better charts? Make engaging charts with Venngage’s Chart Maker .
Related : How to Choose the Best Types of Charts For Your Data
Similar to a chart, a diagram is a visual representation of information. Diagrams can be both two-dimensional and three-dimensional.
Some of the most common types of diagrams are:
- Venn diagrams
- Tree diagrams
- SWOT analysis
- Fishbone diagrams
- Use case diagrams
Diagrams are used for mapping out processes, helping with decision making, identifying root causes, connecting ideas, and planning out projects.
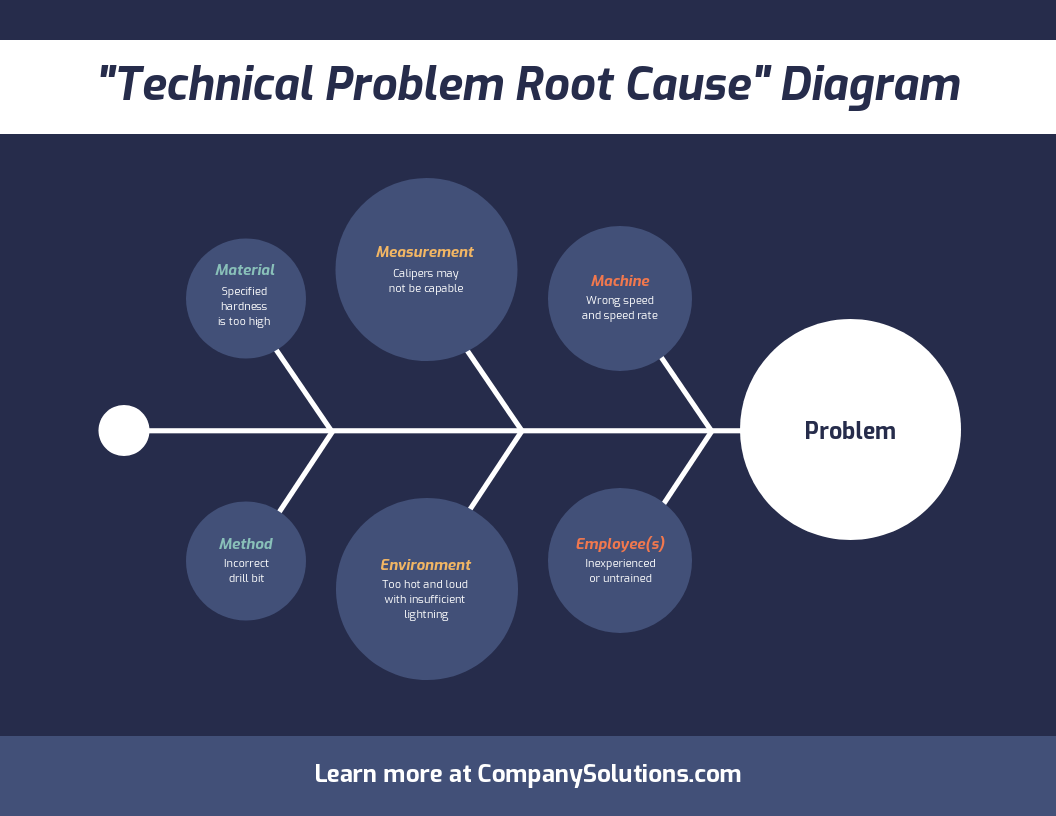
CREATE THIS DIAGRAM TEMPLATE
Want to make a diagram ? Create a Venn diagram and other visuals using our free Venn Diagram Maker .
A map is a visual representation of an area of land. Maps show physical features of land like regions, landscapes, cities, roads, and bodies of water.
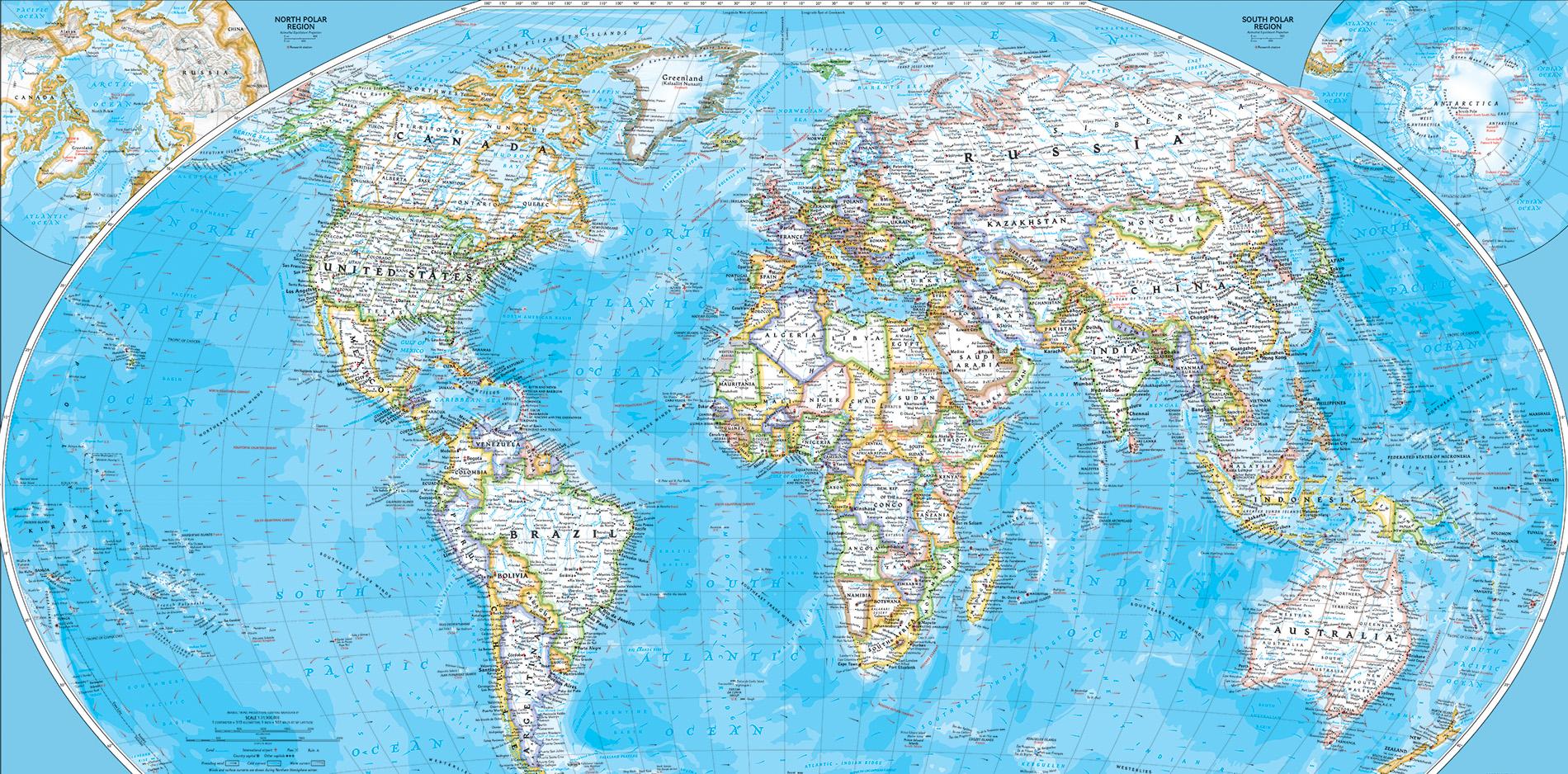
Source: National Geographic
A common type of map you have probably come across in your travels is a choropleth map . Choropleth maps use different shades and colors to indicate average quantities.
For example, a population density map uses varying shades to show the difference in population numbers from region to region:
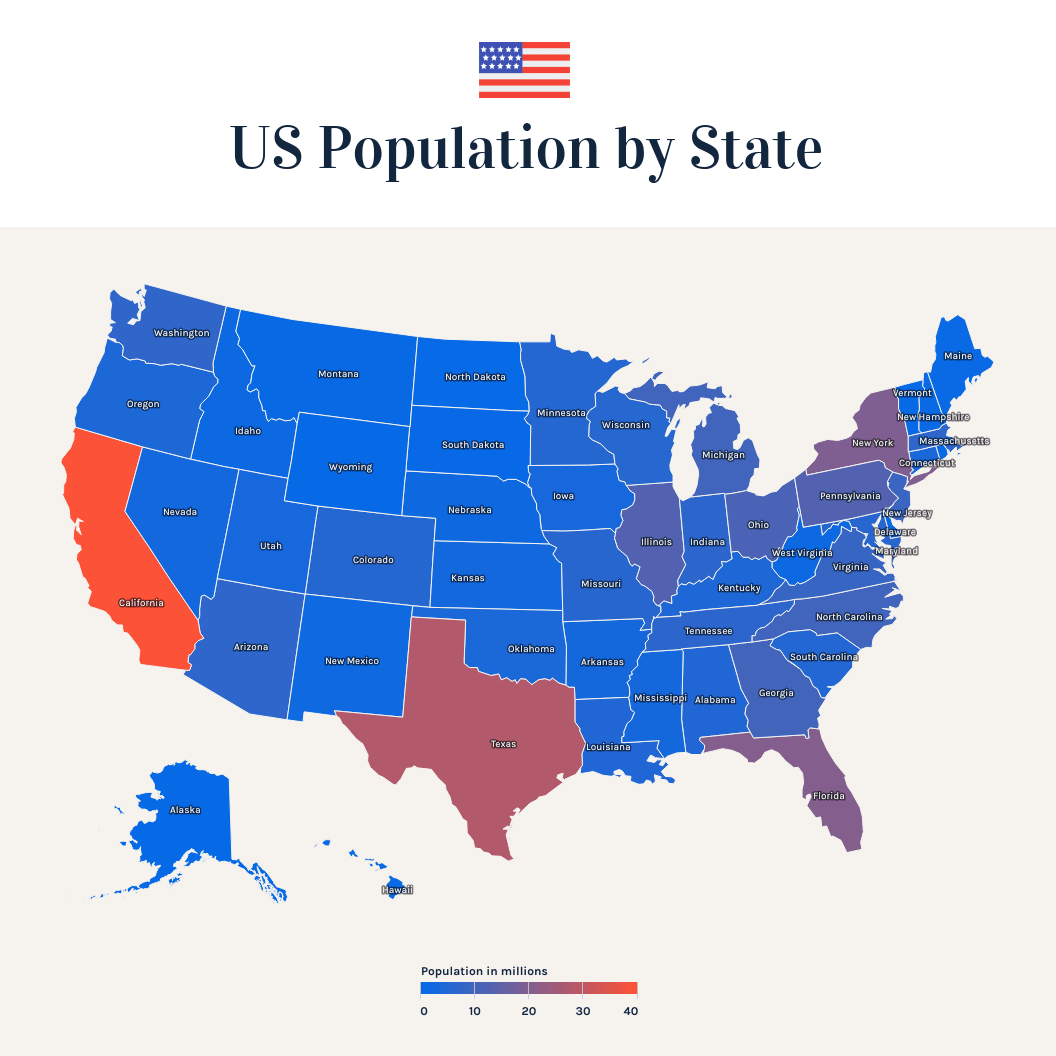
Create your own map for free with Venngage’s Map Maker .
How to present data visually (data visualization best practices)
While good data visualization will communicate data or information clearly and effectively, bad data visualization will do the opposite. Here are some practical tips for how businesses and organizations can use data visualization to communicate information more effectively.
Not a designer? No problem. Venngage’s Graph Maker will help you create better graphs in minutes.
1. Avoid distorting the data
This may be the most important point in this whole blog post. While data visualizations are an opportunity to show off your creative design chops, function should never be sacrificed for fashion.
The chart styles, colors, shapes, and sizing you use all play a role in how the data is interpreted. If you want to present your data accurately and ethically, then you need to take care to ensure that your data visualization does not present the data falsely.
There are a number of different ways data can be distorted in a chart. Some common ways data can be distorted are:
- Making the baselines something other than 0 to make numbers seem bigger or smaller than they are – this is called “truncating” a graph
- Compressing or expanding the scale of the Y-axis to make a line or bar seem bigger or smaller than it should be
- Cherry picking data so that only the data points you want to include are on a graph (i.e. only telling part of the story)
- Using the wrong type of chart, graph or diagram for your data
- Going against standard, expected data visualization conventions
Because people use data visualizations to reinforce their opinions, you should always read data visualizations with a critical eye. Often enough, writers may be using data visualization to skew the data in a way that supports their opinions, but that may not be entirely truthful.

Read More: 5 Ways Writers Use Graphs To Mislead You
Want to create an engaging line graph? Use Venngage’s Line Graph Maker to create your own in minutes.
2. Avoid cluttering up your design with “chartjunk”
When it comes to best practices for data visualization, we should turn to one of the grandfather’s of data visualization: Edward Tufte. He coined the term “ chartjunk ”, which refers to the use of unnecessary or confusing design elements that skews or obscures the data in a chart.
Here’s an example of a data visualization that suffers from chartjunk:
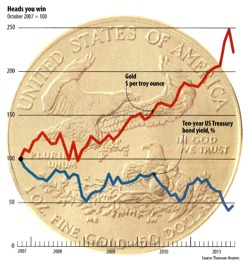
Source: ExcelUser
In this example, the image of the coin is distracting for readers trying to interpret the data. Note how the fonts are tiny – almost unreadable. Mistakes like this are common when a designers tries to put style before function.
Read More : The Worst Infographics of 2020 (With Lessons for 2021)
3. Tell a story with your data
Data visualizations like infographics give you the space to combine data and narrative structure in one page. Visuals like icons and bold fonts let you highlight important statistics and facts.
For example, you could customize this data visualization infographic template to show the benefit of using your product or service (and post it on social media):
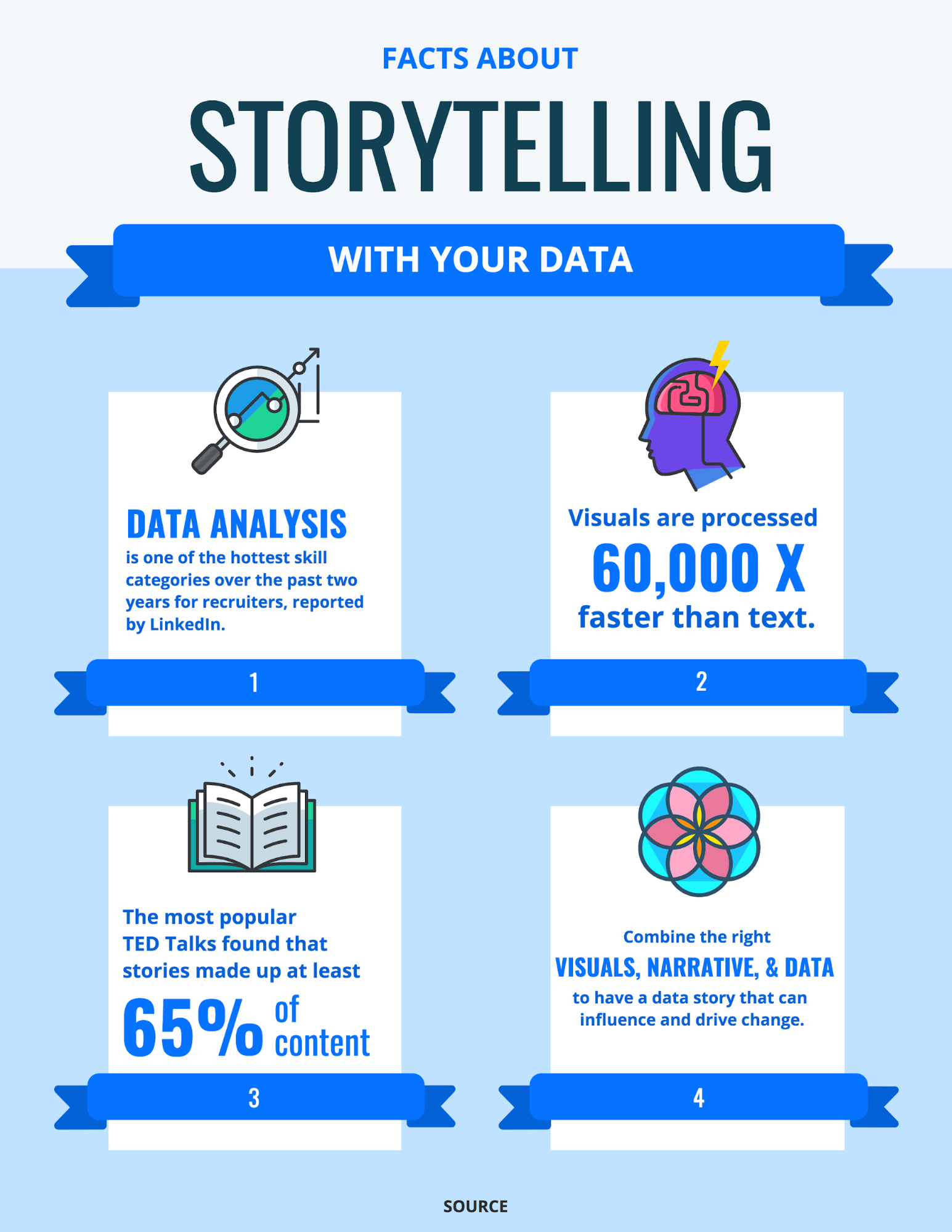
USE THIS TEMPLATE
This data visualization relies heavily on text and icons to tell the story of its data:
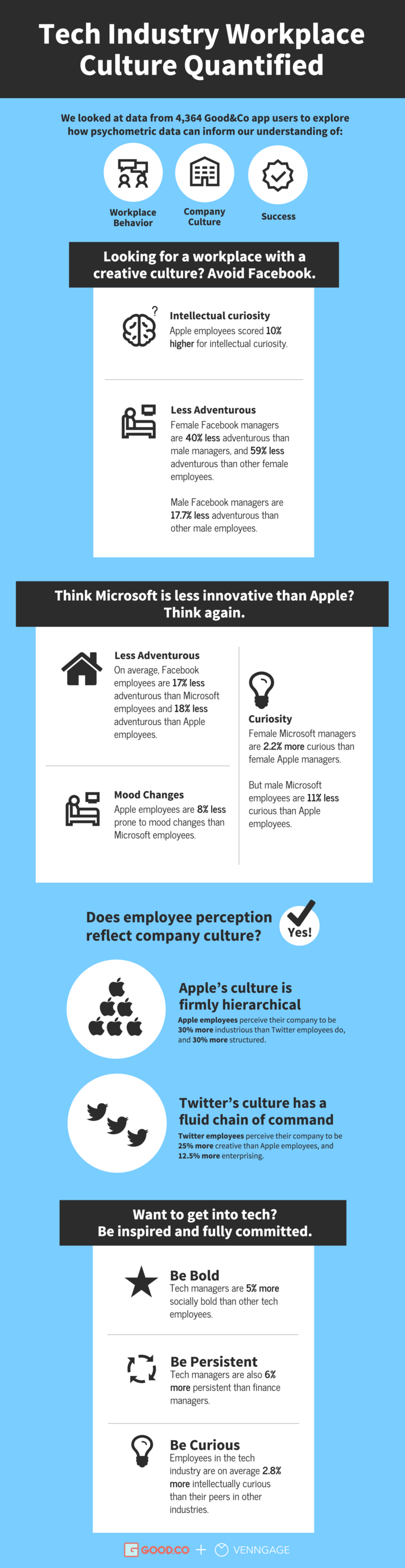
This type of infographic is perfect for those who aren’t as comfortable with charts and graphs. It’s also a great way to showcase original research, get social shares and build brand awareness.
4. Combine different types of data visualizations
While you may choose to keep your data visualization simple, combining multiple types of charts and diagrams can help tell a more rounded story.
Don’t be afraid to combine charts, pictograms and diagrams into one infographic. The result will be a data visualization infographic that is engaging and rich in visual data.
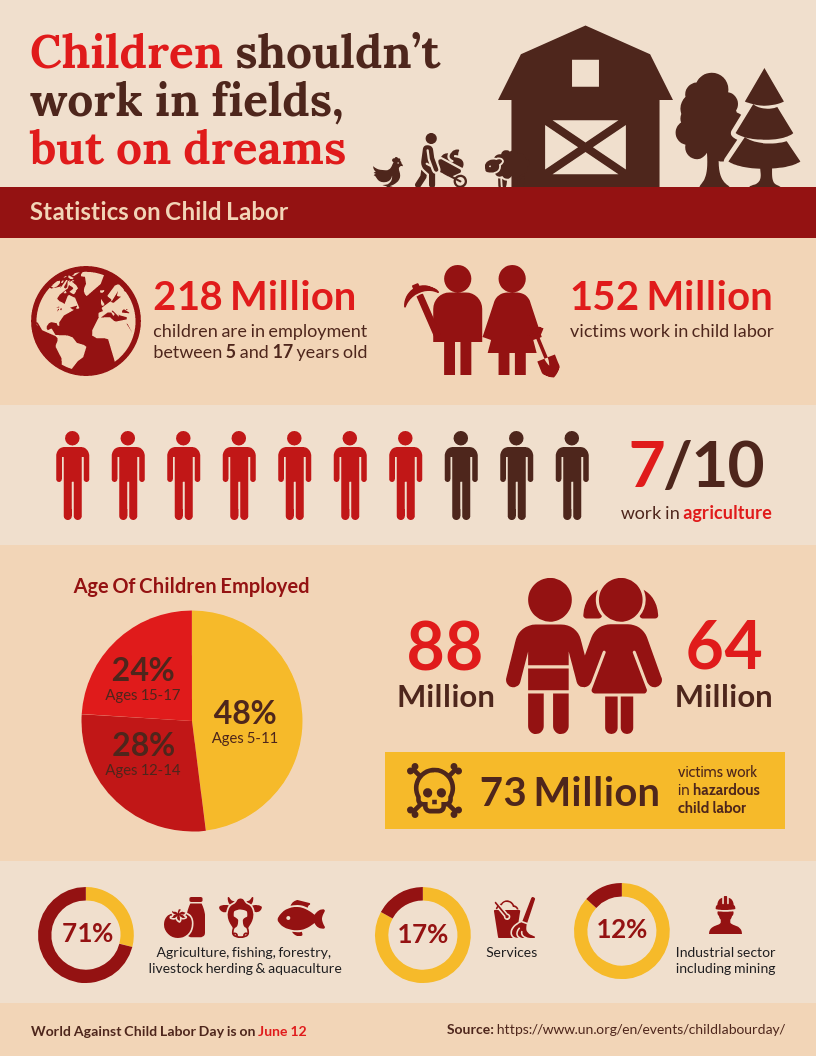
Design Tip: This data visualization infographic would be perfect for nonprofits to customize and include in an email newsletter to increase awareness (and donations).
Or take this data visualization that also combines multiple types of charts, pictograms, and images to engage readers. It could work well in a presentation or report on customer research, customer service scores, quarterly performance and much more:
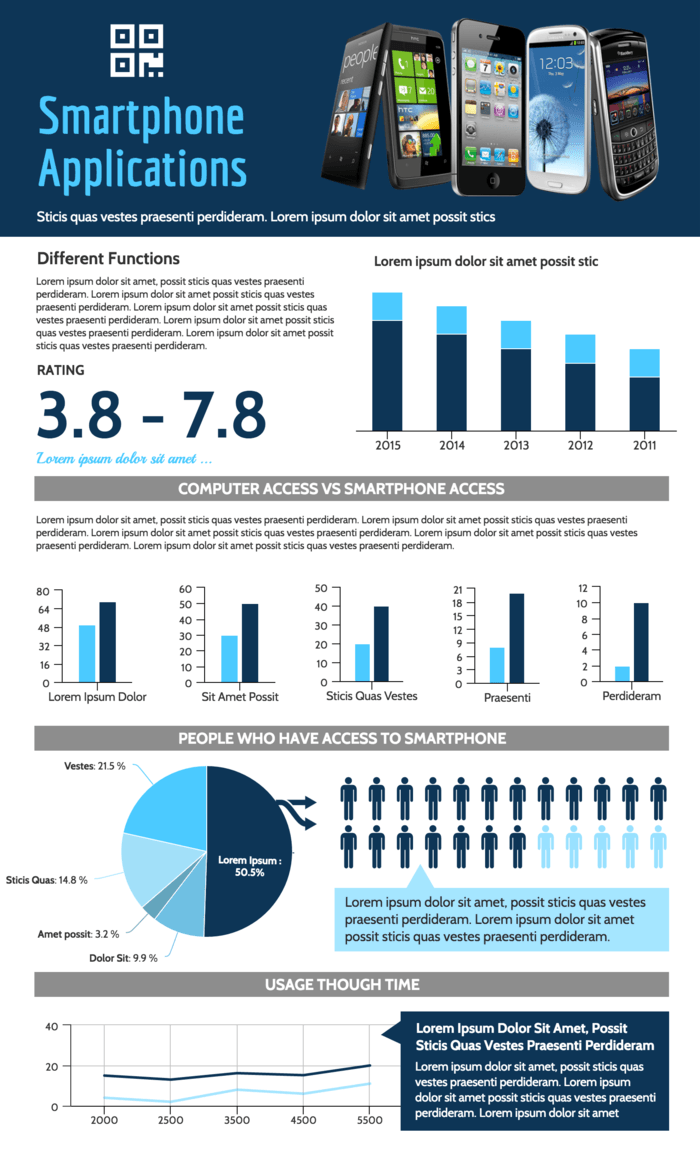
Design Tip: This infographic could work well in a presentation or report on customer research, customer service scores, quarterly performance and much more.
Make your own bar graph in minutes with our free Bar Graph Maker .
5. Use icons to emphasize important points
Icons are perfect for attracting the eye when scanning a page. (Remember: use visual cues!)
If there are specific data points that you want readers to pay attention to, placing an icon beside it will make it more noticeable:

Design Tip: This infographic template would work well on social media to encourage shares and brand awareness.
You can also pair icons with headers to indicate the beginning of a new section.
Meanwhile, this infographic uses icons like bullet points to emphasize and illustrate important points.
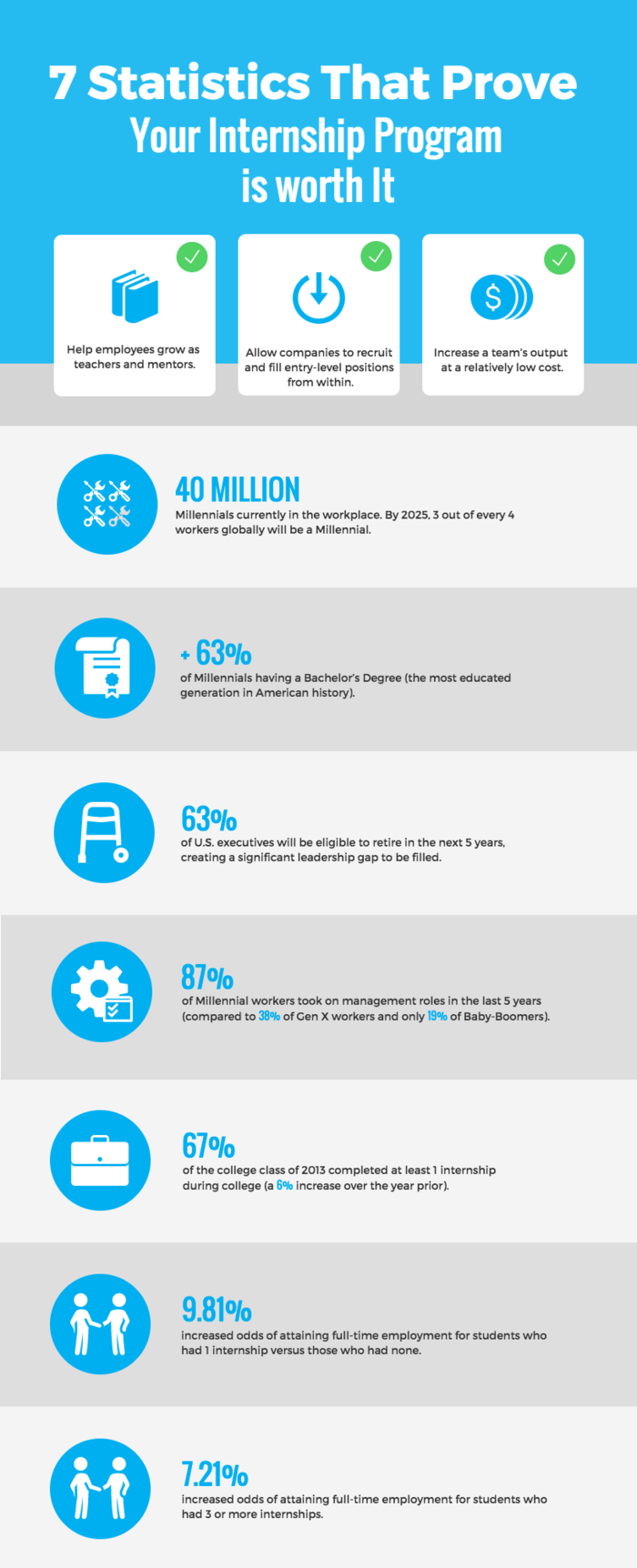
Design Tip: This infographic would make a great sales piece to promote your course or other service.
6. Use bold fonts to make text information engaging
A challenge people often face when setting out to visualize information is knowing how much text to include. After all, the point of data visualization is that it presents information visually, rather than a page of text.
Even if you have a lot of text information, you can still create present data visually. Use bold, interesting fonts to make your data exciting. Just make sure that, above all else, your text is still easy to read.
This data visualization uses different fonts for the headers and body text that are bold but clear. This helps integrate the text into the design and emphasizes particular points:
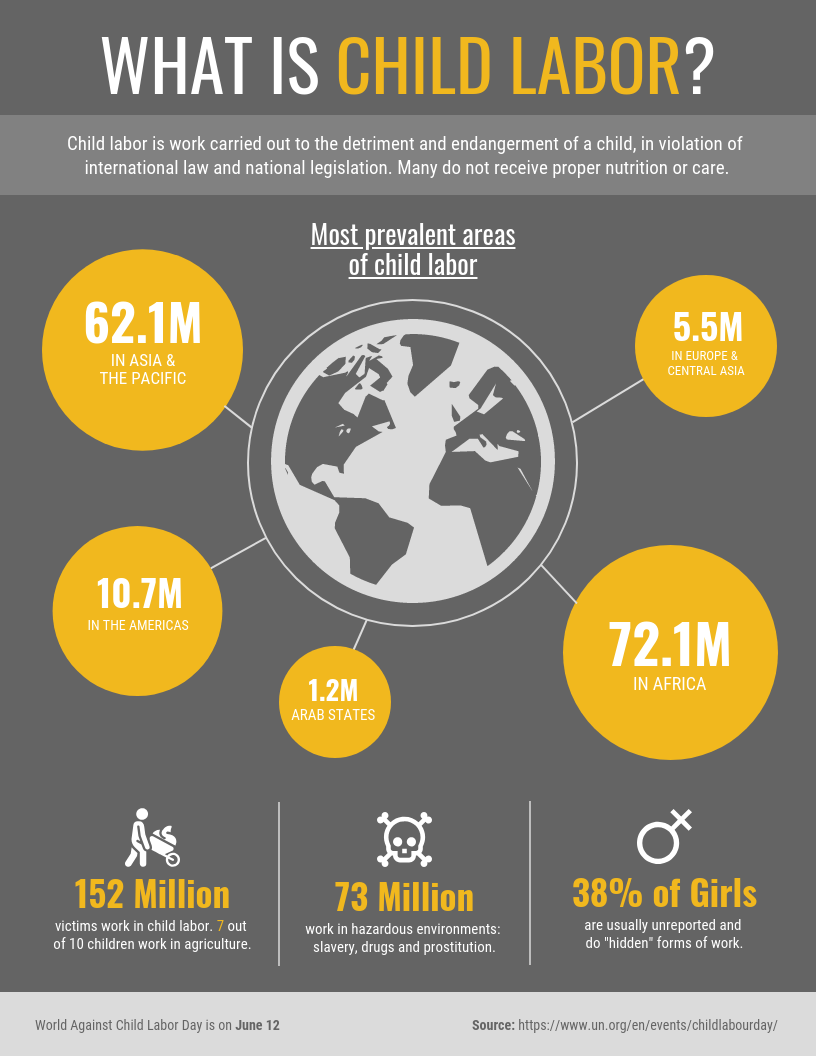
Design Tip: Nonprofits could use this data visualization infographic in a newsletter or on social media to build awareness, but any business could use it to explain the need for their product or service.
As a general rule of thumb, stick to no more than three different font types in one infographic.
This infographic uses one font for headers, another font for body text, and a third font for accent text.
Read More: How to Choose Fonts For Your Designs (With Examples)
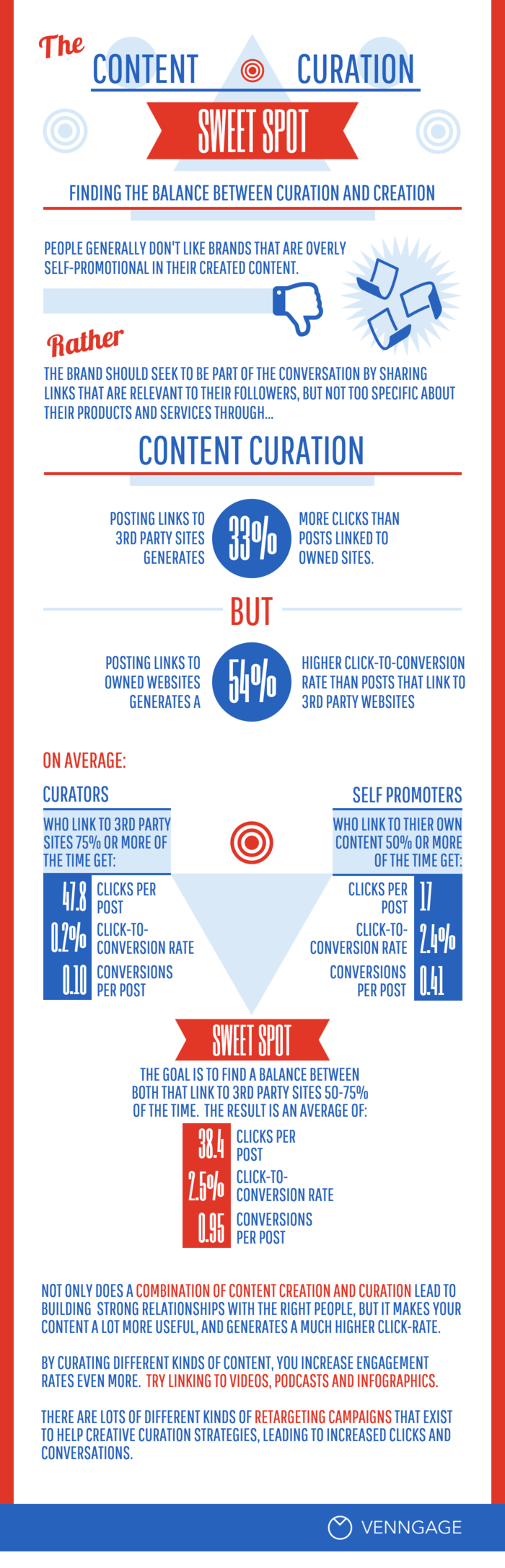
Design Tip: Venngage has a library of fonts to choose from. If you can’t find the icon you’re looking for , you can always request they be added. Our online editor has a chat box with 24/7 customer support.
7. Use colors strategically in your design
In design, colors are as functional as they are fashionable. You can use colors to emphasize points, categorize information, show movement or progression, and more.
For example, this chart uses color to categorize data:
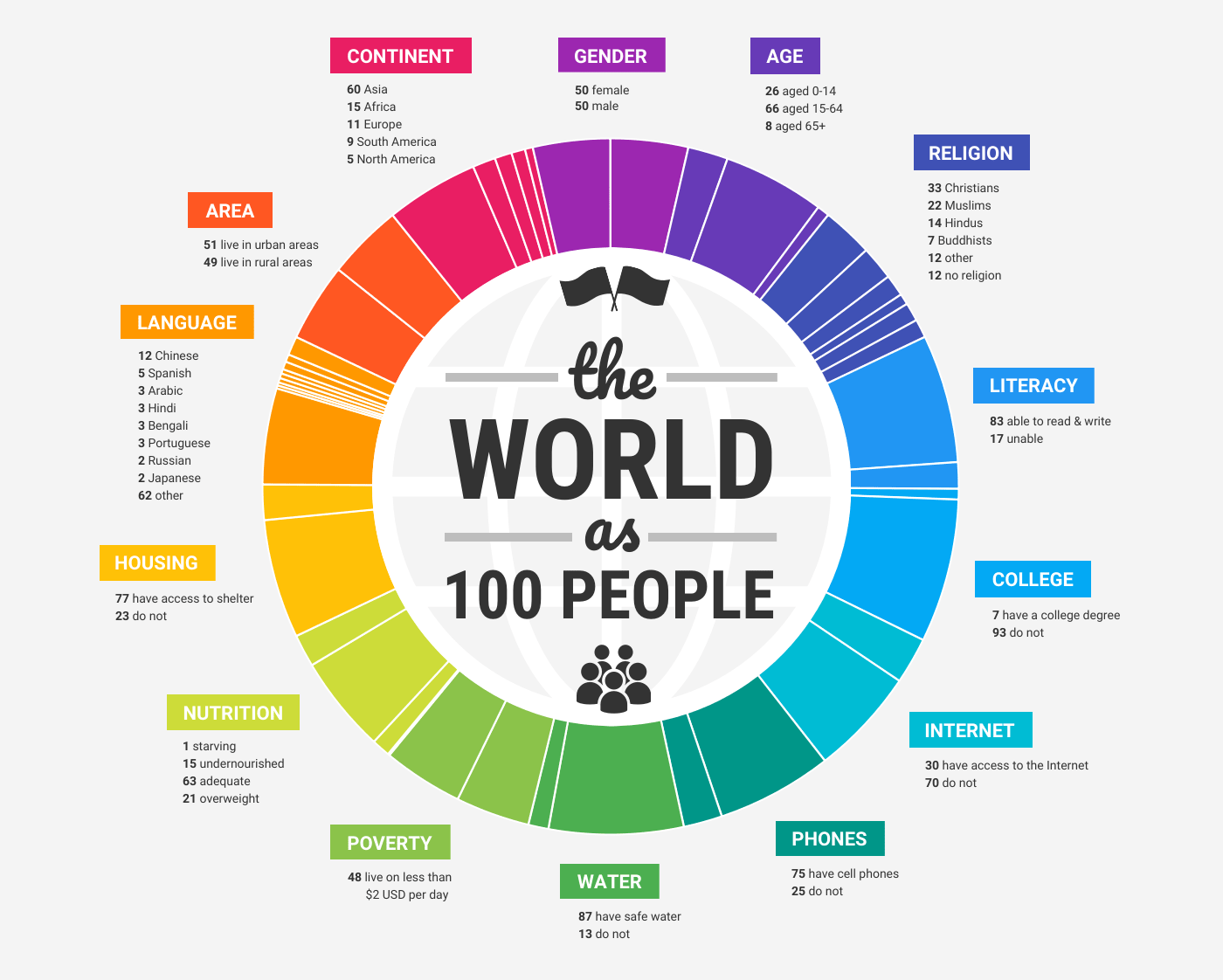
Design Tip : This pie chart can actually be customized in many ways. Human resources could provide a monthly update of people hired by department, nonprofits could show a breakdown of how they spent donations and real estate agents could show the average price of homes sold by neighbourhood.
You can also use light colored text and icons on dark backgrounds to make them stand out. Consider the mood that you want to convey with your infographic and pick colors that will reflect that mood. You can also use contrasting colors from your brand color palette.
This infographic template uses a bold combination of pinks and purples to give the data impact:
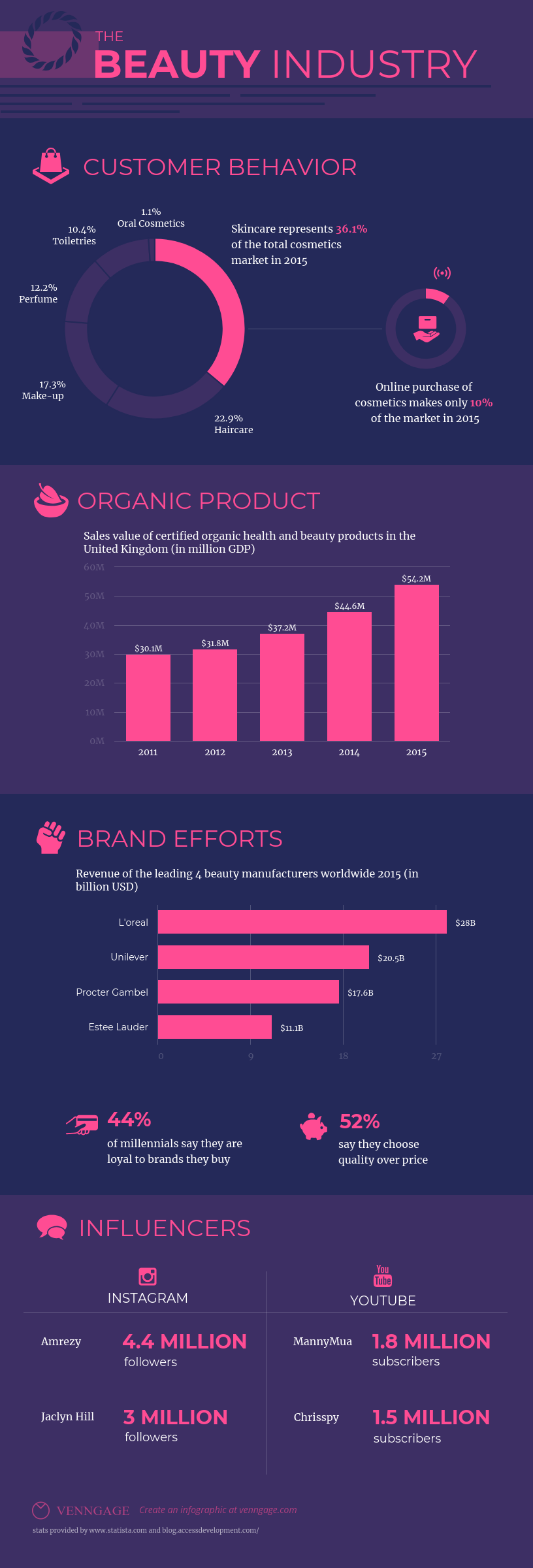
Read More: How to Pick Colors to Captivate Readers and Communicate Effectively
8. Show how parts make up a whole
It can be difficult to break a big topic down into smaller parts. Data visualization can make it a lot easier for people to conceptualize how parts make up a whole.
Using one focus visual, diagram or chart can convey parts of a whole more effectively than a text list can. Look at how this infographic neatly visualizes how marketers use blogging as part of their strategy:
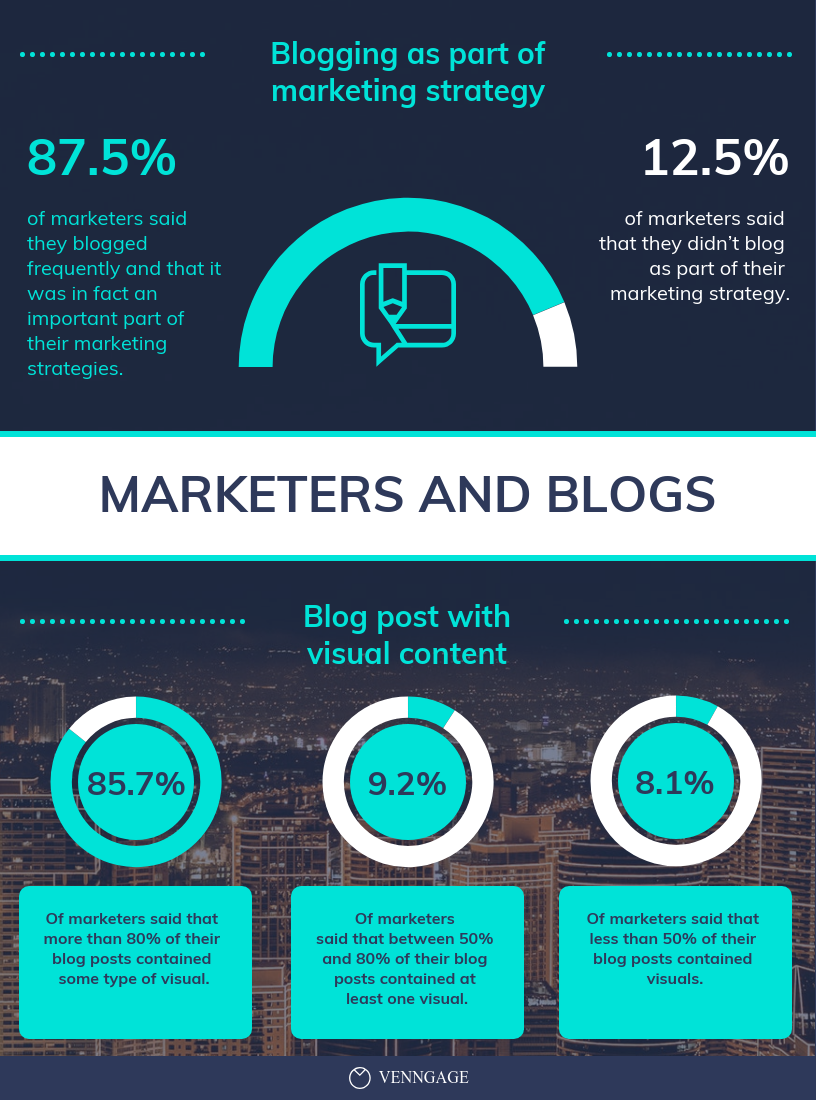
Design Tip: Human resources could use this graphic to show the results of a company survey. Or consultants could promote their services by showing their success rates.
Or look at how this infographic template uses one focus visual to illustrate the nutritional makeup of a banana:
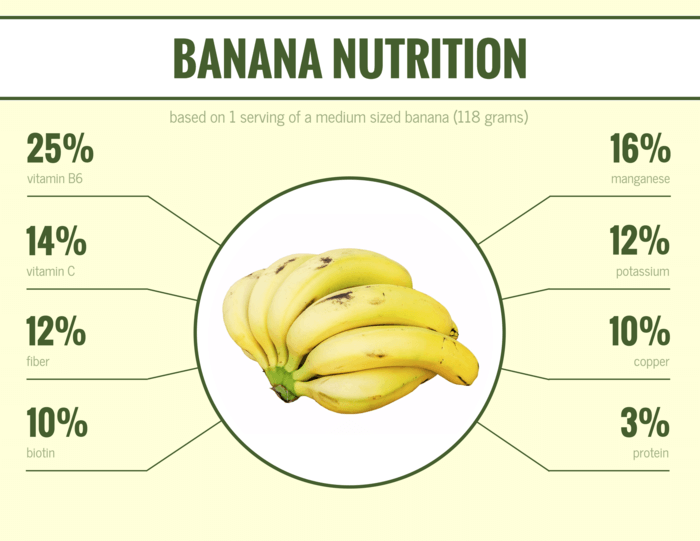
CREATE THIS FLYER TEMPLATE
9. Focus on one amazing statistic
If you are preparing a presentation, it’s best not to try and cram too many visuals into one slide. Instead, focus on one awe-inspiring statistic and make that the focus of your slide.
Use one focus visual to give the statistic even more impact. Smaller visuals like this are ideal for sharing on social media, like in this example:
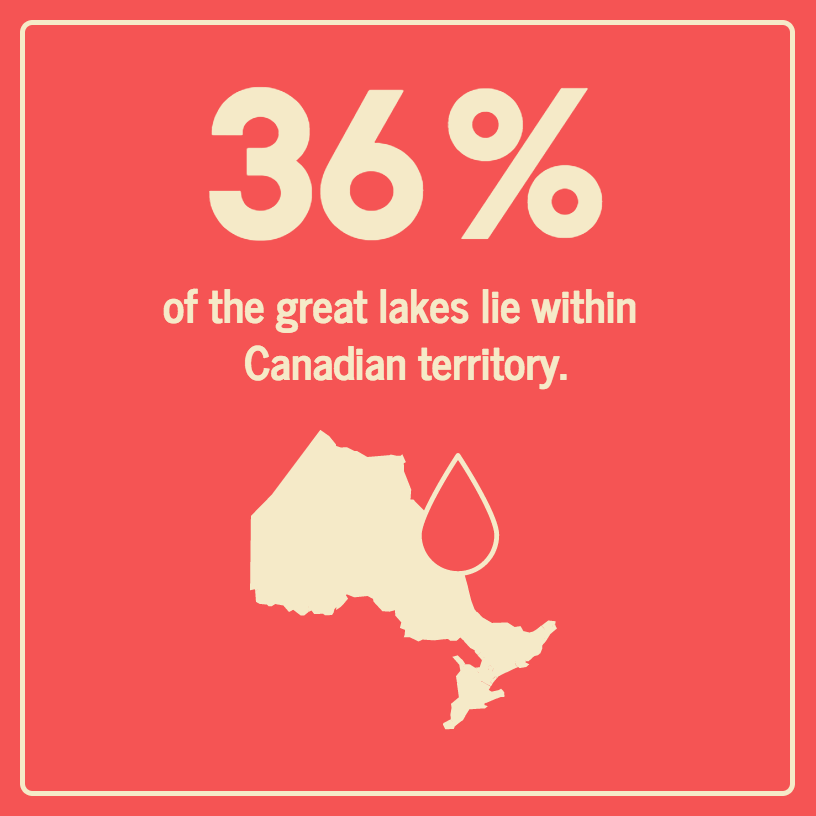
Design Tip: You can easily swap out the icon above (of Ontario, Canada) using Venngage’s drag-and-drop online editor and its in-editor library of icons. Click on the template above to get started.
This template also focuses on one key statistic and offers some supporting information in the bar on the side:
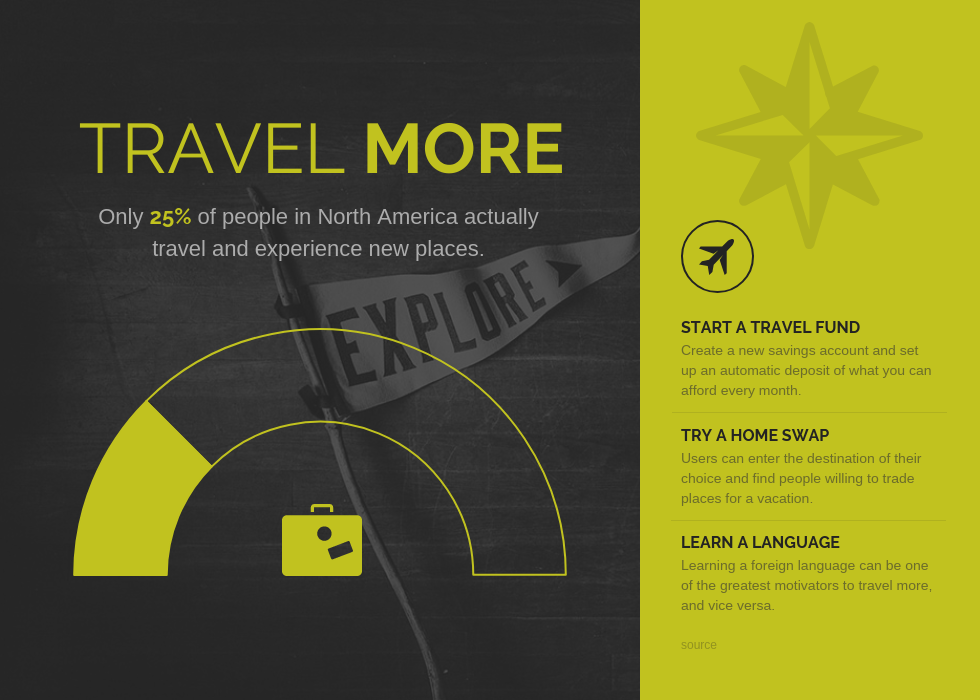
10. Optimize your data visualization for mobile
Complex, information-packed infographics are great for spicing up reports, blog posts, handouts, and more. But they’re not always the best for mobile viewing.
To optimize your data visualization for mobile viewing, use one focus chart or icon and big, legible font. You can create a series of mobile-optimized infographics to share multiple data points in a super original and attention-grabbing way.
For example, this infographic uses concise text and one chart to cut to the core message behind the data:
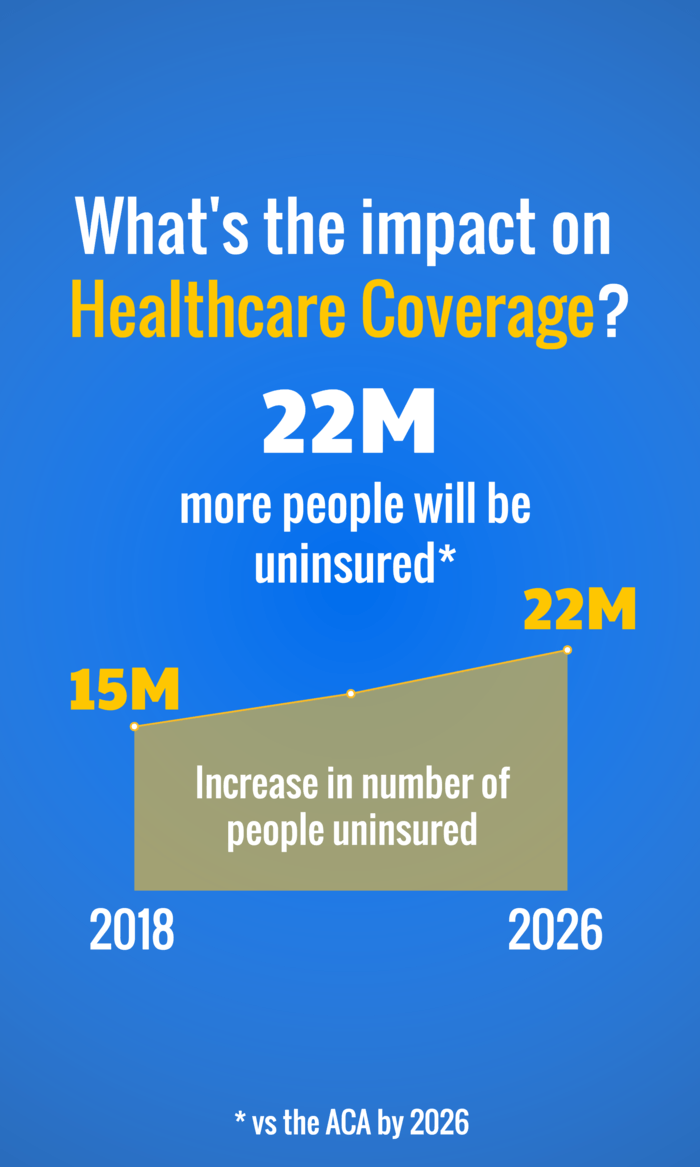
CREATE THIS SOCIAL MEDIA TEMPLATE
Some amazing data visualization examples
Here are some of the best data visualization examples I’ve come across in my years writing about data viz.
Evolution of Marketing Infographic

Graphic Design Trends Infographic
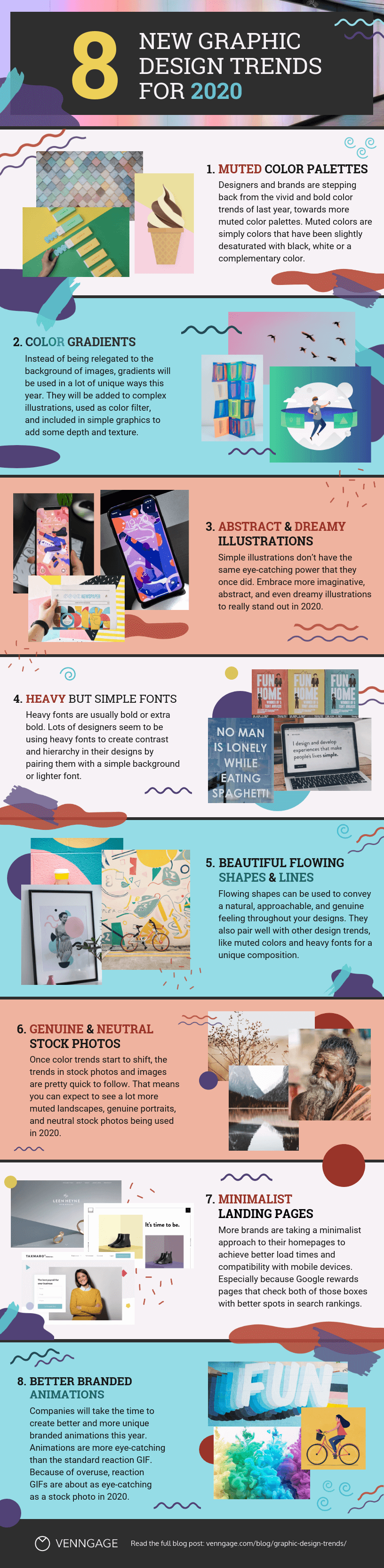
Stop Shark Finning Nonprofit Infographic
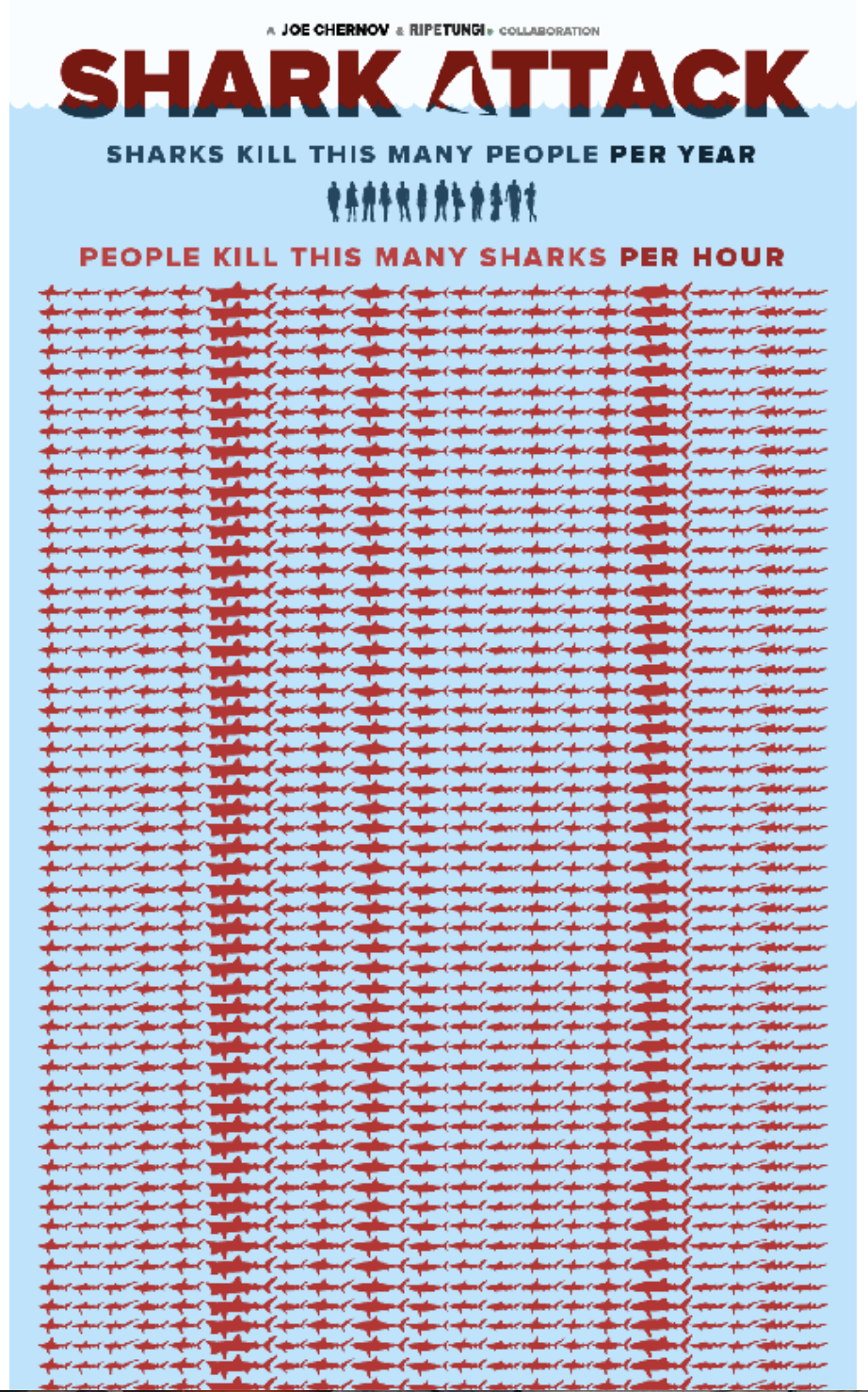
Source: Ripetungi
Coronavirus Impact on Environment Data Visualization

What Disney Characters Tell Us About Color Theory
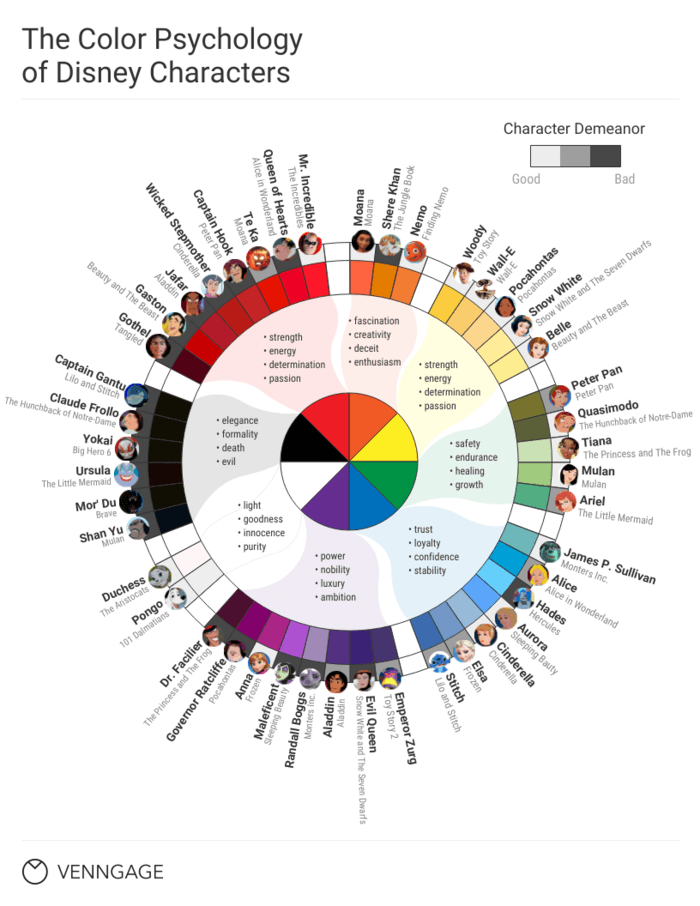
World’s Deadliest Animal Infographic
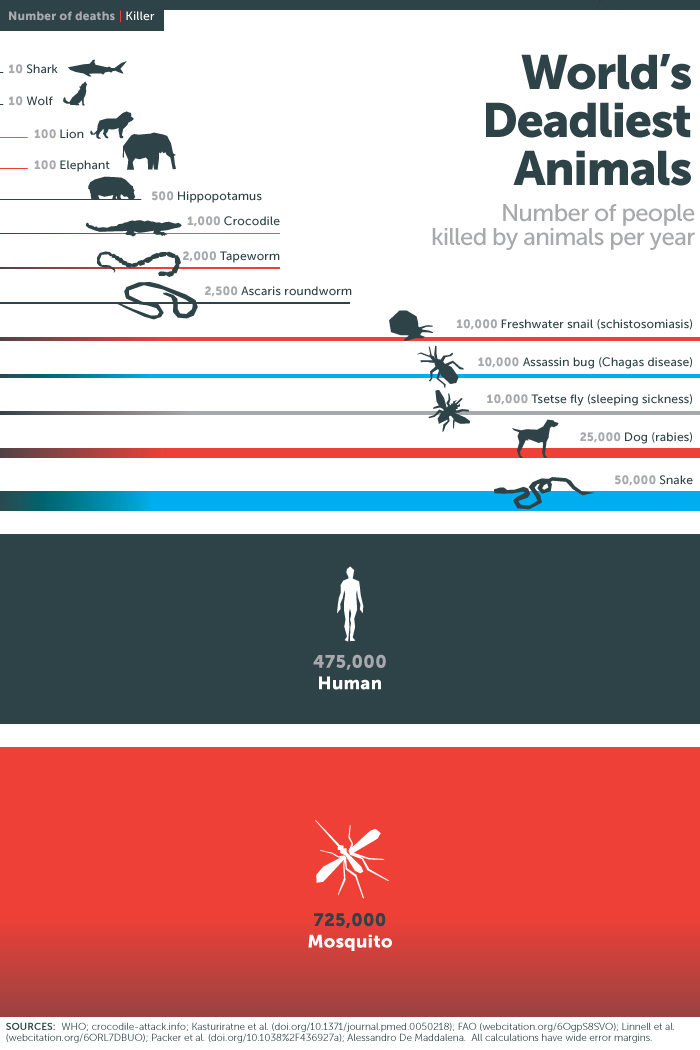
Source: Bill and Melinda Gates Foundation
The Secret Recipe For a Viral Creepypasta

Read More: Creepypasta Study: The Secret Recipe For a Viral Horror Story
The Hero’s Journey Infographic
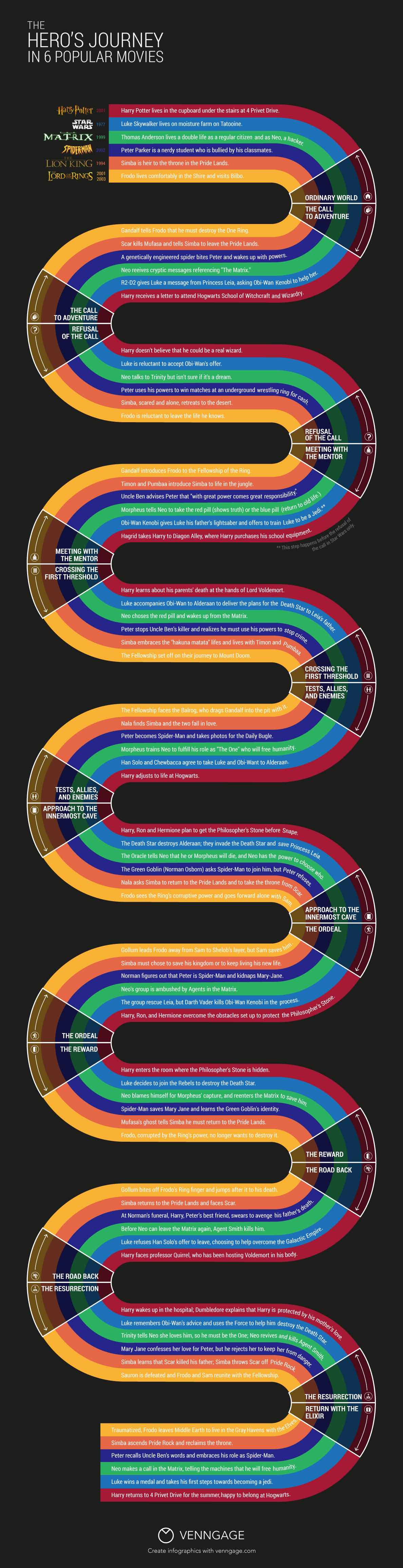
Read More: What Your 6 Favorite Movies Have in Common
Emotional Self Care Guide Infographic
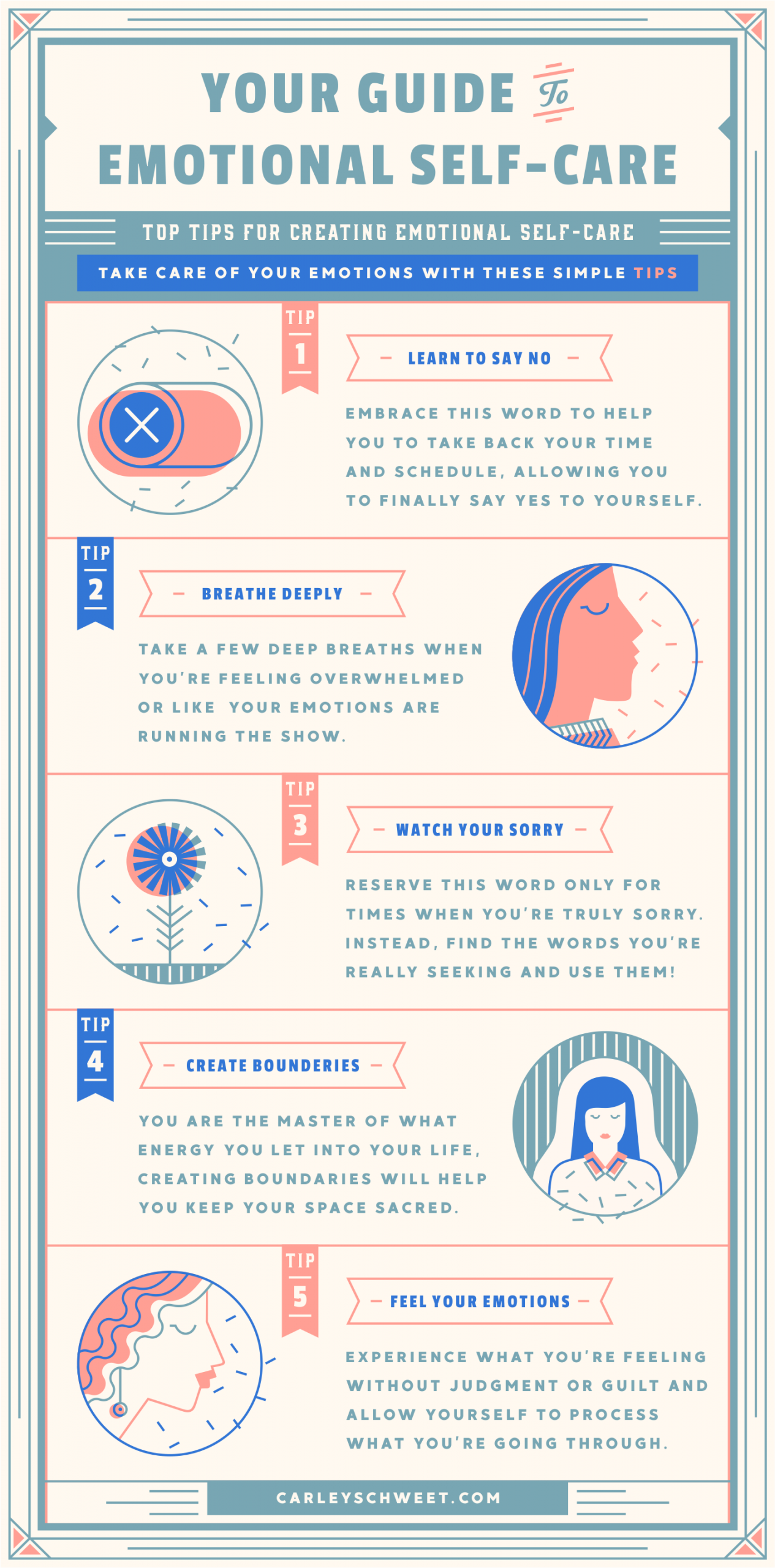
Source: Carley Schweet
Want to look at more amazing data visualization? Read More: 50+ Infographic Ideas, Examples & Templates for 2020 (For Marketers, Nonprofits, Schools, Healthcare Workers, and more)
Discover popular designs
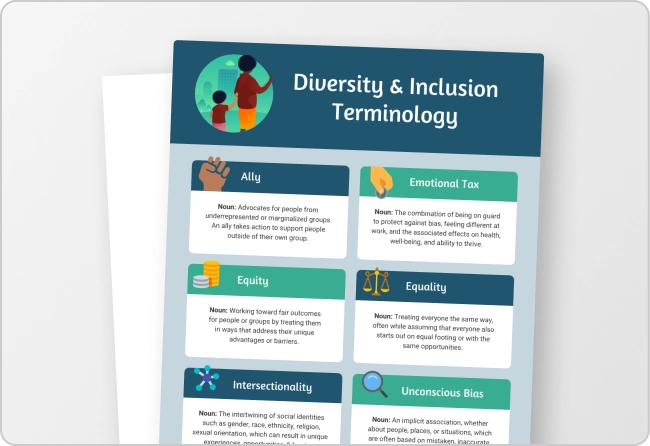
Infographic maker
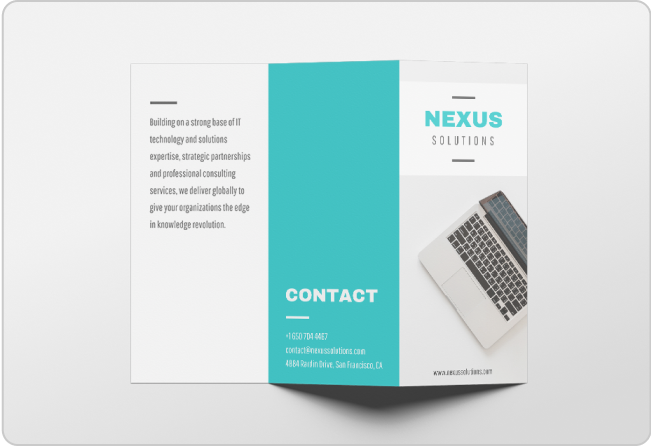
Brochure maker
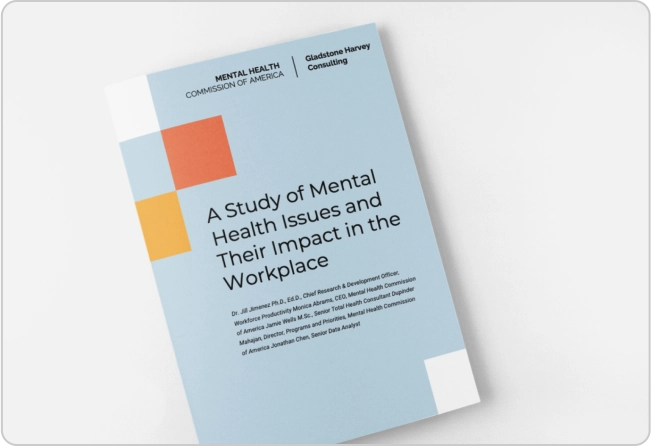
White paper online
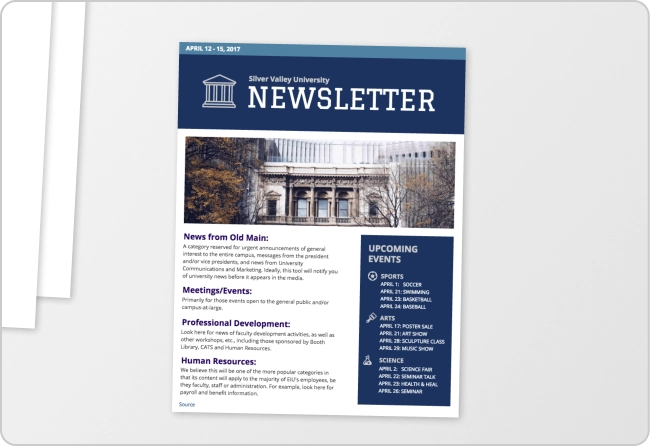
Newsletter creator
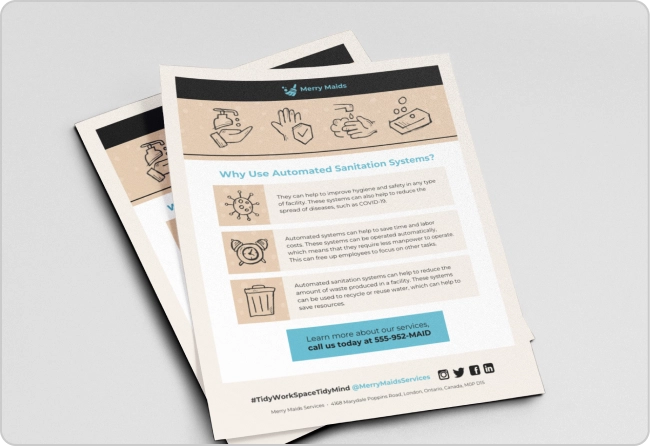
Flyer maker
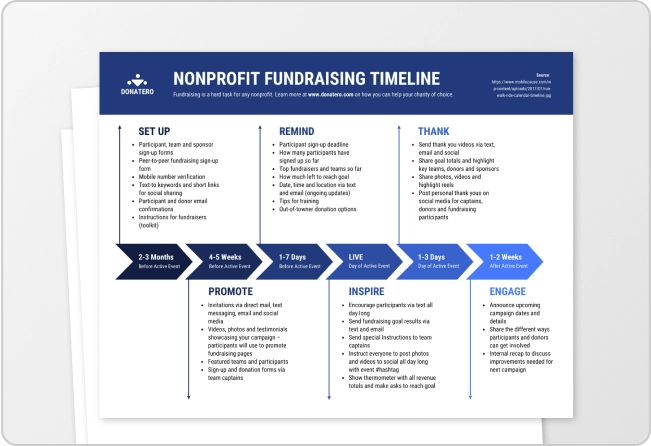
Timeline maker
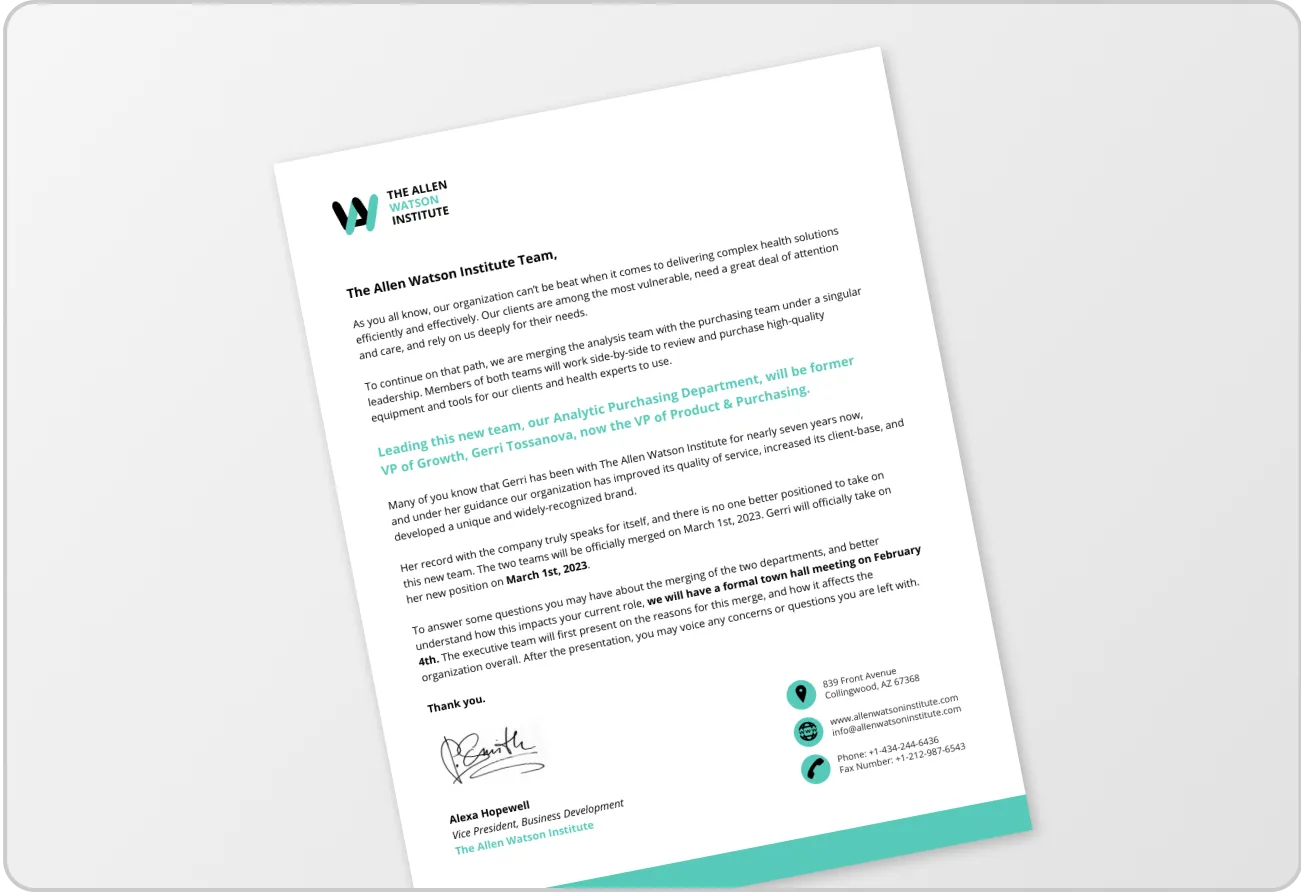
Letterhead maker
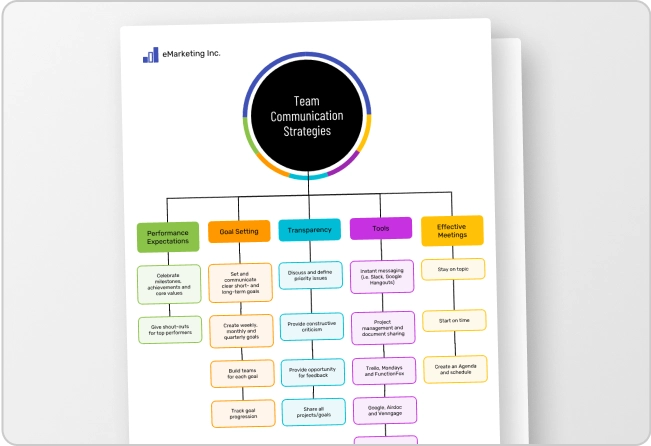
Mind map maker
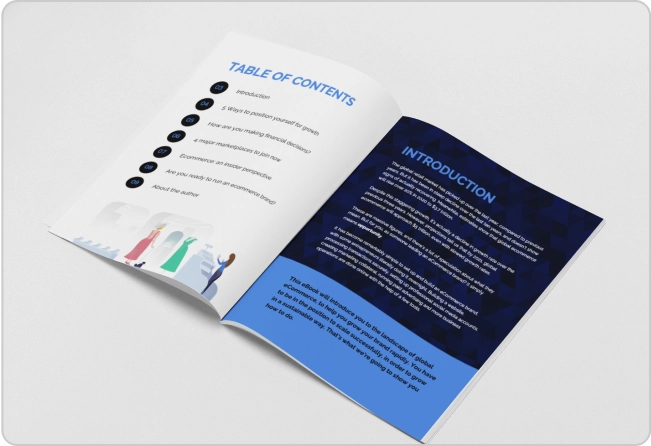
Ebook maker
What Is Data Visualization and Why Is It Important? A Complete Introduction
They say a picture is worth a thousand words, and this is especially true for data analytics.
Data visualization is all about presenting data in a visual format, using charts, graphs, and maps to tell a meaningful story. It’s a crucial step in the data analysis process—and a technique (or art form!) that all areas of business can benefit from.
In this guide, we’ll tell you everything you need to know about data visualization (also known as data viz). We’ll explain what it is, why it matters, some of the most common types, as well as the tools you can use to create them.
This guide is ideal for anyone who wants to present, communicate, and share data-driven insights.
If you’d like to learn more data analytics skills, try this free data short course .
- What is data visualization?
- Why is data visualization important?
- When should you visualize your data?
- Different types of data visualization and when to use them
- Top data visualization tools
- Best practices and principles for effective data visualization
- Getting started with data visualization
So: What is data visualization? Let’s start with a definition.
1. What is data visualization? A definition
Data visualization is the graphical or visual representation of data. It helps to highlight the most useful insights from a dataset, making it easier to spot trends, patterns, outliers, and correlations.
Imagine you’re presented with a spreadsheet containing rows and rows of data. You probably won’t be able to decipher the data without delving into it, and it’s unlikely that you’ll be able to spot trends and patterns at first glance.
Now imagine seeing the same data presented as a bar chart, or on a color-coded map. It’s much easier to see what the data is telling you, right?
That’s the whole point of data visualization. It makes insights visible to the naked eye, so that virtually anyone can see and understand what’s going on. When done well, data visualization tells a story.
This storytelling aspect is crucial as it makes your data actionable. There’s a huge difference between simply having lots of data versus actually understanding how to use it to drive actions and decisions—and data visualization bridges that gap.
There are two broad categories of data visualization: exploration and explanation. Let’s take a look at those now.
What are the two main types of data visualization? Exploration vs. explanation
We’ll look at specific types of data visualization later on, but for now, it’s important to distinguish between exploratory and explanatory data visualization.
In a nutshell, exploratory data visualization helps you figure out what’s in your data, while explanatory visualization helps you to communicate what you’ve found. Exploration takes place while you’re still analyzing the data, while explanation comes towards the end of the process when you’re ready to share your findings.
Exploration
When faced with a new dataset, one of the first things you’ll do is carry out an exploratory data analysis . This is where you investigate the dataset and identify some of its main features, laying the foundation for more thorough analysis.
At this stage, visualizations can make it easier to get a sense of what’s in your dataset and to spot any noteworthy trends or anomalies. Ultimately, you’re getting an initial lay of the land and finding clues as to what the data might be trying to tell you.
Explanation
Once you’ve conducted your analysis and have figured out what the data is telling you, you’ll want to share these insights with others.
These could be key business stakeholders who can take action based on the data, for example, or public audiences who have an interest in your topic area.
Explanatory data visualizations help you tell this story, and it’s up to you to determine which visualizations will help you to do so most effectively. We’ll introduce some of the most common types of data visualization (and when to use them) in section four.
Want to learn more about data visualization, and try your hand at creating visualizations of your own? Give this free introductory tutorial a go. We’ll show you, step by step, how to create bar charts, line graphs, and more for a real dataset in Google Sheets.
2. Why is data visualization important?
The importance of effective data visualization is rooted in the importance of data analytics in general.
We’re living in an increasingly data-rich world; at the start of 2020, the digital universe comprised approximately 44 zettabytes of data . For perspective, one zettabyte is roughly equal to a trillion gigabytes. By 2025, it’s estimated that around 463 exabytes of data will be created every 24 hours across the globe. An exabyte is equivalent to one billion gigabytes. Basically, we’re producing tons and tons of data all the time.
Data analytics allows us to make sense of (at least some of) that data. From a business perspective, it enables companies to learn from the past and plan ahead for the future. In fields like healthcare, it can help to improve patient care and treatment. In finance and insurance, it can help to assess risk and combat fraudulent activity. Essentially, we need data analytics in order to make smart decisions—and data visualization is a crucial part of that.
Data visualization helps us to understand what certain data is telling us, presenting it in a way that’s accessible to a range of audiences—not just data experts. It’s how you bridge the gap between your expertise as a data analyst or data scientist, and those people who can use or act upon the insights you discover.
A line graph and a bar chart taken from the Fitbit app.
The advantages and benefits of effective data visualization at a glance
Data visualization allows you to:
- Get an initial understanding of your data by making trends, patterns, and outliers easily visible to the naked eye
- Comprehend large volumes of data quickly and efficiently
- Communicate insights and findings to non-data experts, making your data accessible and actionable
- Tell a meaningful and impactful story, highlighting only the most relevant information for a given context
Now we know what data visualization is and why it matters, let’s take a look at when and why you might need to visualize your data.
3. When should you visualize your data?
Aside from exploratory data visualization which takes place in the early stages, data visualization usually comprises the final step in the data analysis process . To recap, the data analysis process can be set out as follows:
- Define the question: What problem are you trying to solve?
- Collect the data: Determine what kind of data you need and where you’ll find it.
- Clean the data: Remove errors, duplicates, outliers, and unwanted data points—anything that might skew how your data is interpreted. You can learn more about data cleaning (and how to do it) in this guide .
- Analyze the data: Determine the type of data analysis you need to carry out in order to find the insights you’re looking for.
- Visualize the data and share your findings: Translate your key insights into visual format (e.g. graphs, charts, or heatmaps) and present them to the relevant audience(s).
Essentially, you visualize your data any time you want to summarize and highlight key findings and share them with others. With that in mind, let’s consider what kinds of insights you can convey with data visualizations.
What is data visualization used for?
Within the broader goal of conveying key insights, different visualizations can be used to tell different stories. Data visualizations can be used to:
- Convey changes over time: For example, a line graph could be used to present how the value of Bitcoin changed over a certain time period.
- Determine the frequency of events: You could use a histogram to visualize the frequency distribution of a single event over a certain time period (e.g. number of internet users per year from 2007 to 2021). Learn how to create a histogram in this guide .
- Highlight interesting relationships or correlations between variables: If you wanted to highlight the relationship between two variables (e.g. marketing spend and revenue, or hours of weekly exercise vs. cardiovascular fitness), you could use a scatter plot to see, at a glance, if one increases as the other decreases (or vice versa).
- Examine a network: If you want to understand what’s going on within a certain network (for example, your entire customer base), network visualizations can help you to identify (and depict) meaningful connections and clusters within your network of interest.
- Analyze value and risk: If you want to weigh up value versus risk in order to figure out which opportunities or strategies are worth pursuing, data visualizations—such as a color-coded system—could help you to categorize and identify, at a glance, which items are feasible.
So far, we’ve taken a rather broad, high-level look at data visualization. Now let’s drill down to some specific types of data visualization and when to use them.
An example of data visualization, as seen in the Fitbit app.
4. How to visualize your data: Different types of data visualization (and when to use them)
There are many different options when it comes to visualizing your data. The visualization you choose depends on the type of data you’re working with and what you want to convey or highlight. It’s also important to consider the complexity of your data and how many different variables are involved. Not all types of data visualization lend themselves to elaborate or complex depictions, so it’s important to choose a suitable technique.
Before we explore some of the most common types of data visualization, let’s first introduce five main data visualization categories.
Five data visualization categories
When considering the different types of data viz, it helps to be aware of the different categories that these visualizations may fall into:
- Temporal data visualizations are linear and one-dimensional. Examples include scatterplots, timelines, and line graphs.
- Hierarchical visualizations organize groups within larger groups, and are often used to display clusters of information. Examples include tree diagrams, ring charts, and sunburst diagrams.
- Network visualizations show the relationships and connections between multiple datasets. Examples include matrix charts, word clouds, and node-link diagrams.
- Multidimensional or 3D visualizations are used to depict two or more variables. Examples include pie charts, Venn diagrams, stacked bar graphs, and histograms.
- Geospatial visualizations convey various data points in relation to physical, real-world locations (for example, voting patterns across a certain country). Examples include heat maps, cartograms, and density maps.
With those categories in mind, let’s explore some of the most common types of data visualization.
Five common types of data visualization (and when to use them)
In this section, we’ll introduce some useful types of data visualization. We’ll also point you to our more comprehensive guide where you can learn about additional data visualization methods and how to use them.
1. Scatterplots
Scatterplots (or scatter graphs) visualize the relationship between two variables. One variable is shown on the x-axis, and the other on the y-axis, with each data point depicted as a single “dot” or item on the graph. This creates a “scatter” effect, hence the name.
Source: displayr.com
Scatterplots are best used for large datasets when there’s no temporal element. For example, if you wanted to visualize the relationship between a person’s height and weight, or between how many carats a diamond measures and its monetary value, you could easily visualize this using a scatterplot.
It’s important to bear in mind that scatterplots simply describe the correlation between two variables; they don’t infer any kind of cause-and-effect relationship.
2. Bar charts
Bar charts are used to plot categorical data against discrete values.
Categorical data refers to data that is not numeric, and it’s often used to describe certain traits or characteristics. Some examples of categorical data include things like education level (e.g. high school, undergrad, or post-grad) and age group (e.g. under 30, under 40, under 50, or 50 and over).
Discrete values are those which can only take on certain values—there are no “half measures” or “gray areas.” For example, the number of people attending an event would be a discrete variable, as would the number of sales made in a certain time period (think about it: you can’t make “half a sale” or have “half an event attendee.”)
Source: chartio.com
So, with a bar chart, you have your categorical data on the x-axis plotted against your discrete values on the y-axis.
The height of the bars is directly proportional to the values they represent, making it easy to compare your data at a glance.
3. Pie charts
Just like bar charts, pie charts are used to visualize categorical data.
However, while bar charts represent multiple categories of data, pie charts are used to visualize just one single variable broken down into percentages or proportions. A pie chart is essentially a circle divided into different “slices,” with each slice representing the percentage it contributes to the whole.
Thus, the size of each pie slice is proportional to how much it contributes to the whole “pie.”
Imagine you have a class of thirty students and you want to divide them up based on what color t-shirt they’re wearing on a given day.
The possible “slices” are red, green, blue, and yellow, with each color representing 40%, 30%, 25%, and 5% of the class total respectively. You could easily visualize this using a pie chart—and the yellow slice (5%) would be considerably thinner than the red slice (40%)! Pie charts are best suited for data that can be split into a maximum of five or six categories.
4. Network graphs
Not all data is simple enough to be summarized in a bar or pie chart. For those more complex datasets, there are a range of more elaborate data visualizations at your disposal—network graphs being one of them.
Network graphs show how different elements or entities within a network relate to one another, with each element represented by an individual node. These nodes are connected to other, related nodes via lines.
Source: networkofthrones.wordpress.com
Network graphs are great for spotting and representing clusters within a large network of data.
Let’s imagine you have a huge database filled with customers, and you want to segment them into meaningful clusters for marketing purposes. You could use a network graph to draw connections and parallels between all your customers or customer groups.
With any luck, certain clusters and patterns would emerge, giving you a logical means by which to group your audience.
5. Geographical maps
Geo maps are used to visualize the distribution of data in relation to a physical, geographical area.
For example, you could use a color-coded map to see how natural oil reserves are distributed across the world, or to visualize how different states voted in a political election. Maps are an extremely versatile form of data visualization, and are an excellent way of communicating all kinds of location-related data.
Some other types of maps used in data visualization include dot distribution maps (think scatterplots combined with a map), and cartograms which distort the size of geographical areas to proportionally represent a given variable (population density, for example).
Source: pmfias.com
Here, we’ve introduced just a handful of data visualization types. If you want to learn more, check out our complete guide to different types of data visualization and when to use them .
5. Top data visualization tools
When it comes to creating informative, eye-catching visualizations, there are plenty of tools at your disposal.
When choosing a tool, it’s important to consider your needs in terms of the kinds of visualizations you want to create, as well as your own technical expertise; some tools will require coding knowledge, while others are more suited to non-technical users.
In this section, we’ll briefly introduce some of the most popular data visualization tools. If you’re on the market for a data viz tool and want a more thorough comparison, this guide to the seven best data visualization tools will help you. For now, here are our top three data viz tools to get familiar with:
- Plotly: Open-source software built on Python. Plotly is ideal if you’ve got some coding knowledge and want to create highly customizable visualizations.
- D3.js: A free, open-source data viz library built using JavaScript. As with Plotly, you’ll need some programming knowledge in order to use this data viz tool.
- Tableau: Perhaps one of the most popular data analytics tools , Tableau is known for its user-friendliness—you don’t need any coding knowledge to create beautiful visualizations in Tableau. And, unlike some other BI tools, it’s good at handling large volumes of data.
Before deciding on a tool, it’s worth trying out a few options. The good news is that there are plenty of data viz tools on the market— as well as a number of free tools —allowing you to create beautiful and informative visualizations—even if you’re a newcomer to the field.
What are data dashboards?
Dashboards are another useful tool for data tracking and visualization. A data dashboard essentially allows you to keep track of multiple data sources, visualizing them in one single location for easy viewing.
A common example is the Google Analytics dashboard , which displays a whole host of visualizations on one page—a geo map showing where your website visitors are located, for example, or a pie chart showing what percentage of your users access your website using specific devices.
If you want multiple stakeholders to be able to access and view certain data insights, a dashboard can help you to create a single hub with easy-to-understand visualizations.
A snapshot of a data dashboard, taken from Google Analytics.
6. What are some data visualization best practices?
Data visualization truly is an art form—but the goal is always, first and foremost, to provide valuable information and insights.
If you can do this by way of beautiful visualizations, you’re onto a winner. So, when creating data visualizations, it’s important to adhere to certain best practices.
These will help you strike the right balance, keeping your audience engaged and informed. Here’s how to excel at data visualization.
1. Define a clear purpose
Like any data analytics project, it’s important to define a clear purpose for your data visualizations.
What are the priorities in terms of what you want to convey and communicate? What should your audience take away from your visualization? It’s essential to have this defined from the outset; that way, you can ensure that you’re only presenting the most valuable information—and giving your audience something they can use and act upon.
2. Know your audience
The purpose of data visualization is to communicate insights to a specific audience, so you’ll want to give some thought to who your audience is and how familiar they are with the information you’re presenting.
What kind of context can you provide around your visualizations in order to help your audience understand them? What types of visualization are likely to be most accessible to this particular group of people? Keep your audience in mind at all times.
3. Keep it simple
When creating visualizations, it’s often the case that less is more.
Ultimately, you want your visualizations to be as digestible as possible, and that means trimming away any unnecessary information while presenting key insights clearly and succinctly. The goal is to keep cognitive load to a minimum—that is, the amount of “brainpower” or mental effort it takes to process information.
Even if the data is complex, your visualizations don’t have to be, so strive for simplicity at all times.
4. Avoid distorting the data
You should strive to present your findings as accurately as possible, so avoid any kind of visual “tricks” that could bias how your data is perceived and interpreted.
Think about the labels you use, as well as how you scale your visualizations. For example, things like “blowing up” certain data segments to make them appear more significant, or starting your graph axis on a number other than zero are both bad practices which could mislead your audience. Prioritize integrity and accuracy!
5. Ensure your visualizations are inclusive
Last but by no means least, make sure that your visualizations are accessible and inclusive.
Think about how colors, contrasts, font sizes, and the use of white space affect the readability of your visualization. Is it easy for your users to distinguish between the data and see what’s going on, regardless of whether they have twenty-twenty vision or a visual impairment?
Inclusivity and accessibility are central to good data visualization, so don’t overlook this step.
7. Getting started with data visualization
By now, you hopefully have a good understanding of what data visualization is and why it matters.
Of course, the best way to get to grips with it is to see it in action. Check out our round-up of some of the most beautiful and informative data visualization examples from around the web.
Keen to give it a go yourself? Why not download a free dataset and see what you can do! If you’d like to learn it more, then check out this list of data visualization courses out there to try.
Data visualization is an excellent skill to have, whether you’re forging a career in the data industry or just want to share valuable insights with your colleagues. If you are pursuing a career as a data analyst or data scientist, be sure to include data visualizations in your data portfolio —it’s something that employers will be looking out for.
CareerFoundry’s Data Visualizations with Python course is designed to ease you into this vital area of data analytics. You can take it as a standalone course as well as a specialization within our full Data Analytics Program, you’ll learn and apply the principles of data viz in a real-world project, as well as getting to grips with various data visualization libraries.
Want to learn more? Try your hand at this free, introductory data analytics short course , and check out the following guides:
- What is data quality and why is it important?
- What is web scraping? A beginner’s guide
- An introduction to multivariate analysis
- Business Essentials
- Leadership & Management
- Credential of Leadership, Impact, and Management in Business (CLIMB)
- Entrepreneurship & Innovation
- Digital Transformation
- Finance & Accounting
- Business in Society
- For Organizations
- Support Portal
- Media Coverage
- Founding Donors
- Leadership Team
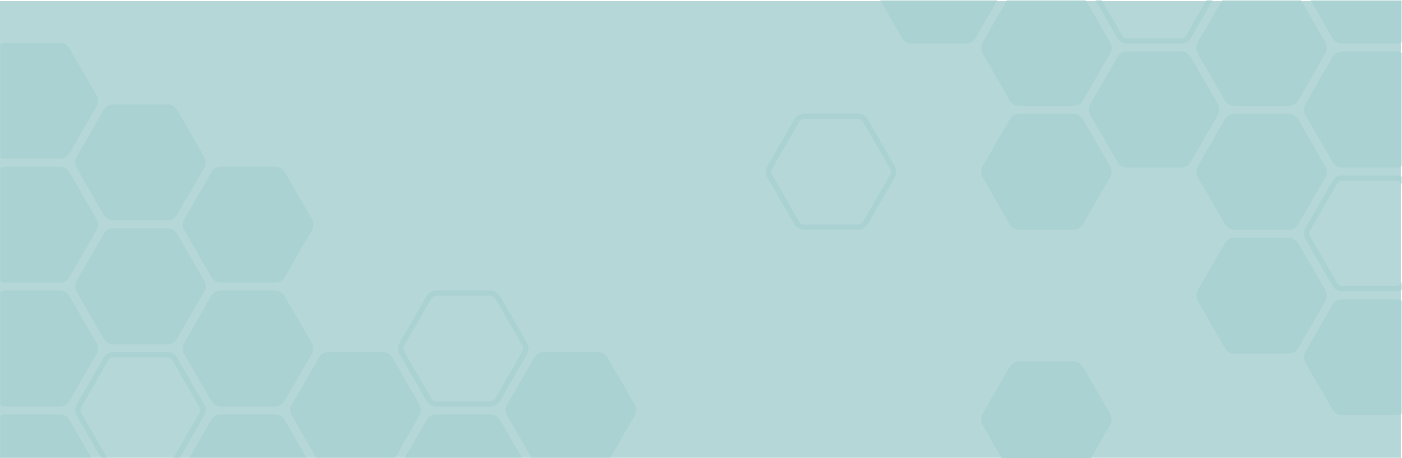
- Harvard Business School →
- HBS Online →
- Business Insights →
Business Insights
Harvard Business School Online's Business Insights Blog provides the career insights you need to achieve your goals and gain confidence in your business skills.
- Career Development
- Communication
- Decision-Making
- Earning Your MBA
- Negotiation
- News & Events
- Productivity
- Staff Spotlight
- Student Profiles
- Work-Life Balance
- AI Essentials for Business
- Alternative Investments
- Business Analytics
- Business Strategy
- Business and Climate Change
- Creating Brand Value
- Design Thinking and Innovation
- Digital Marketing Strategy
- Disruptive Strategy
- Economics for Managers
- Entrepreneurship Essentials
- Financial Accounting
- Global Business
- Launching Tech Ventures
- Leadership Principles
- Leadership, Ethics, and Corporate Accountability
- Leading Change and Organizational Renewal
- Leading with Finance
- Management Essentials
- Negotiation Mastery
- Organizational Leadership
- Power and Influence for Positive Impact
- Strategy Execution
- Sustainable Business Strategy
- Sustainable Investing
- Winning with Digital Platforms
6 Data Visualization Examples To Inspire Your Own
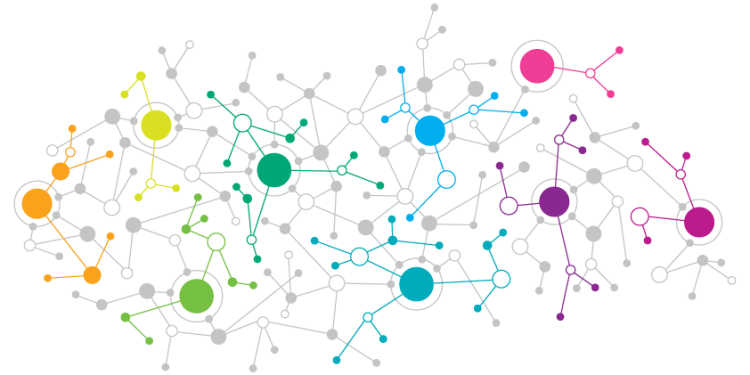
- 12 Jan 2017
Data informs virtually every business decision an organization makes. Because of this, it’s become increasingly important for professionals of all backgrounds to be adept at working with data.
While data can provide immense value, it’s important that professionals are able to effectively communicate the significance of the data to stakeholders. This is where data visualization comes into play. By transforming raw data into engaging visuals using various data visualization tools , it’s much easier to communicate insights gleaned from it.
Here are six real-world examples of data visualization that you can use to inspire your own.
What Is Data Visualization?
Data visualization is the process of turning raw data into graphical representations.
Visualizations make it easy to communicate trends in data and draw conclusions. When presented with a graph or chart, stakeholders can easily visualize the story the data is telling, rather than try to glean insights from raw data.
There are countless data visualization techniques , including:
- Scatter plots
The technique you use will vary based on the type of data you’re handling and what you’re trying to communicate.
6 Real-World Data Visualization Examples
1. the most common jobs by state.

Source: NPR
National Public Radio (NPR) produced a color-coded, interactive display of the most common jobs in each state in each year from 1978 to 2014. By dragging the scroll bar at the bottom of the map, you’re able to visualize occupational changes over time.
If you’re trying to represent geographical data, a map is the best way to go.
2. COVID-19 Hospitalization Rates

Source: CDC
Throughout the COVID-19 pandemic, the Centers for Disease Control and Prevention (CDC) has been transforming raw data into easily digestible visuals. This line graph represents COVID-19 hospitalization rates from March through November 2020.
The CDC tactfully incorporated color to place further emphasis on the stark increase in hospitalization rates, using a darker shade for lower values and a lighter shade for higher values.
3. Forecasted Revenue of Amazon.com

Source: Statista
Data visualizations aren’t limited to historical data. This bar chart created by Statista visualizes the forecasted gross revenue of Amazon.com from 2018 to 2025.
This visualization uses a creative title to summarize the main message that the data is conveying, as well as a darker orange color to spike out the most important data point.
4. Web-Related Statistics

Source: Internet Live Stats
Internet Live Stats has tracked web-related statistics and pioneered methods for visualizing data to show how different digital properties have ebbed and flowed over time.
Simple infographics like this one are particularly effective when your goal is to communicate key statistics rather than visualizing trends or forecasts.
5. Most Popular Food Delivery Items

Source: Eater
Eater, Vox’s food and dining brand, has created this fun take on a “pie” chart, which shows the most common foods ordered for delivery in each of the United States.
To visualize this data, Eater used a specific type of pie chart known as a spie chart. Spie charts are essentially pie charts in which you can vary the height of each segment to further visualize differences in data.
6. Netflix Viewing Patterns

Source: Vox
Vox created this interesting visualization depicting the viewing patterns of Netflix users over time by device type. This Sankey diagram visualizes the tendency of users to switch to streaming via larger device types.

Visualizing Data to Make Business Decisions
The insights and conclusions drawn from data visualizations can guide the decision-making and strategic planning processes for your organization.
To ensure your visualizations are relevant, accurate, and ethical, familiarize yourself with basic data science concepts . With a foundational knowledge in data science, you can maintain confidence in your data and better understand its significance. An online analytics course can help you get started.
Are you interested in improving your data science and analytical skills? Download our Beginner’s Guide to Data & Analytics to learn how you can leverage the power of data for professional and organizational success.
This post was updated on February 26, 2021. It was originally published on January 12, 2017.
17 Best Types of Charts and Graphs for Data Visualization [+ Guide]

Published: May 22, 2024
As a writer for the marketing blog, I frequently use various types of charts and graphs to help readers visualize the data I collect and better understand their significance. And trust me, there's a lot of data to present.
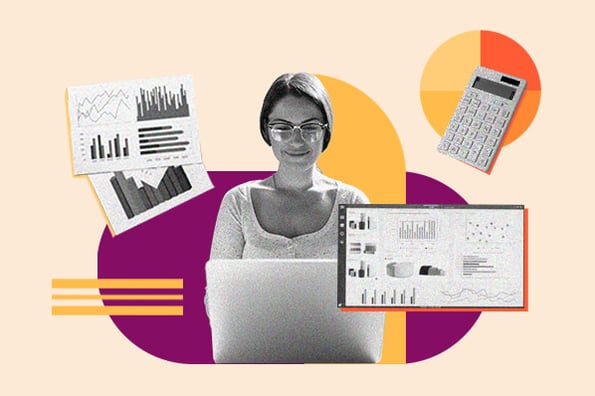
In fact, the volume of data in 2025 will be almost double the data we create, capture, copy, and consume today.

This makes data visualization essential for businesses. Different types of graphs and charts can help you:
- Motivate your team to take action.
- Impress stakeholders with goal progress.
- Show your audience what you value as a business.
Data visualization builds trust and can organize diverse teams around new initiatives. So, I'm going to talk about the types of graphs and charts that you can use to grow your business.
And, if you still need a little more guidance by the end of this post, check out our data visualization guide for more information on how to design visually stunning and engaging charts and graphs.
.png)
Free Excel Graph Templates
Tired of struggling with spreadsheets? These free Microsoft Excel Graph Generator Templates can help.
- Simple, customizable graph designs.
- Data visualization tips & instructions.
- Templates for two, three, four, and five-variable graph templates.
Download Free
All fields are required.
You're all set!
Click this link to access this resource at any time.
Charts vs Graphs: What's the Difference?
A lot of people think charts and graphs are synonymous (I know I did), but they're actually two different things.
Charts visually represent current data in the form of tables and diagrams, but graphs are more numerical in data and show how one variable affects another.
For example, in one of my favorite sitcoms, How I Met Your Mother, Marshall creates a bunch of charts and graphs representing his life. One of these charts is a Venn diagram referencing the song "Cecilia" by Simon and Garfunkle.
Marshall says, "This circle represents people who are breaking my heart, and this circle represents people who are shaking my confidence daily. Where they overlap? Cecilia."
The diagram is a chart and not a graph because it doesn't track how these people make him feel over time or how these variables are influenced by each other.
It may show where the two types of people intersect but not how they influence one another.

Later, Marshall makes a line graph showing how his friends' feelings about his charts have changed in the time since presenting his "Cecilia diagram.
Note: He calls the line graph a chart on the show, but it's acceptable because the nature of line graphs and charts makes the terms interchangeable. I'll explain later, I promise.
The line graph shows how the time since showing his Cecilia chart has influenced his friends' tolerance for his various graphs and charts.

Image source
I can't even begin to tell you all how happy I am to reference my favorite HIMYM joke in this post.
Now, let's dive into the various types of graphs and charts.
Different Types of Graphs for Data Visualization
1. bar graph.
I strongly suggest using a bar graph to avoid clutter when one data label is long or if you have more than 10 items to compare. Also, fun fact: If the example below was vertical it would be a column graph.

Best Use Cases for These Types of Graphs
Bar graphs can help track changes over time. I've found that bar graphs are most useful when there are big changes or to show how one group compares against other groups.
The example above compares the number of customers by business role. It makes it easy to see that there is more than twice the number of customers per role for individual contributors than any other group.
A bar graph also makes it easy to see which group of data is highest or most common.
For example, at the start of the pandemic, online businesses saw a big jump in traffic. So, if you want to look at monthly traffic for an online business, a bar graph would make it easy to see that jump.
Other use cases for bar graphs include:
- Product comparisons.
- Product usage.
- Category comparisons.
- Marketing traffic by month or year.
- Marketing conversions.
Design Best Practices for Bar Graphs
- Use consistent colors throughout the chart, selecting accent colors to highlight meaningful data points or changes over time.
You should also use horizontal labels to improve its readability, and start the y-axis at 0 to appropriately reflect the values in your graph.
2. Line Graph
A line graph reveals trends or progress over time, and you can use it to show many different categories of data. You should use it when you track a continuous data set.
This makes the terms line graphs and line charts interchangeable because the very nature of both is to track how variables impact each other, particularly how something changes over time. Yeah, it confused me, too.
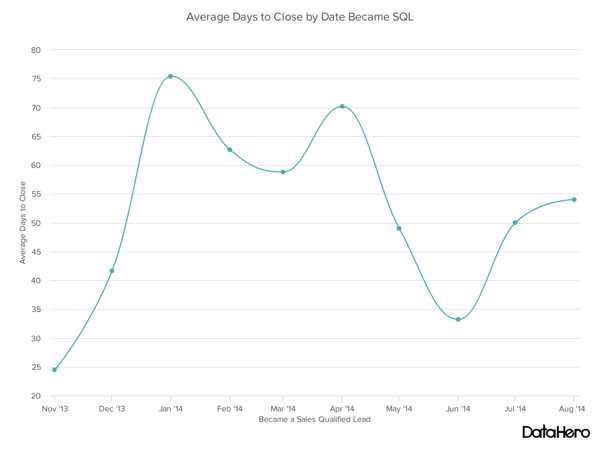
Line graphs help users track changes over short and long periods. Because of this, I find these types of graphs are best for seeing small changes.
Line graphs help me compare changes for more than one group over the same period. They're also helpful for measuring how different groups relate to each other.
A business might use this graph to compare sales rates for different products or services over time.
These charts are also helpful for measuring service channel performance. For example, a line graph that tracks how many chats or emails your team responds to per month.
Design Best Practices for Line Graphs
- Use solid lines only.
- Don't plot more than four lines to avoid visual distractions.
- Use the right height so the lines take up roughly 2/3 of the y-axis' height.
3. Bullet Graph
A bullet graph reveals progress towards a goal, compares this to another measure, and provides context in the form of a rating or performance.
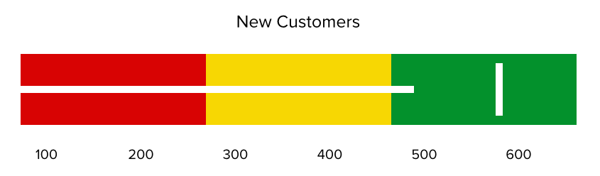
In the example above, the bullet graph shows the number of new customers against a set customer goal. Bullet graphs are great for comparing performance against goals like this.
These types of graphs can also help teams assess possible roadblocks because you can analyze data in a tight visual display.
For example, I could create a series of bullet graphs measuring performance against benchmarks or use a single bullet graph to visualize these KPIs against their goals:
- Customer satisfaction.
- Average order size.
- New customers.
Seeing this data at a glance and alongside each other can help teams make quick decisions.
Bullet graphs are one of the best ways to display year-over-year data analysis. YBullet graphs can also visualize:
- Customer satisfaction scores.
- Customer shopping habits.
- Social media usage by platform.
Design Best Practices for Bullet Graphs
- Use contrasting colors to highlight how the data is progressing.
- Use one color in different shades to gauge progress.
4. Column + Line Graph
Column + line graphs are also called dual-axis charts. They consist of a column and line graph together, with both graphics on the X axis but occupying their own Y axis.
Download our FREE Excel Graph Templates for this graph and more!
Best Use Cases
These graphs are best for comparing two data sets with different measurement units, such as rate and time.
As a marketer, you may want to track two trends at once.
Design Best Practices
Use individual colors for the lines and colors to make the graph more visually appealing and to further differentiate the data.
The Four Basic Types of Charts
Before we get into charts, I want to touch on the four basic chart types that I use the most.
1. Bar Chart
Bar charts are pretty self-explanatory. I use them to indicate values by the length of bars, which can be displayed horizontally or vertically. Vertical bar charts, like the one below, are sometimes called column charts.

2. Line Chart
I use line charts to show changes in values across continuous measurements, such as across time, generations, or categories. For example, the chart below shows the changes in ice cream sales throughout the week.

3. Scatter Plot
A scatter plot uses dotted points to compare values against two different variables on separate axes. It's commonly used to show correlations between values and variables.

4. Pie Chart
Pie charts are charts that represent data in a circular (pie-shaped) graphic, and each slice represents a percentage or portion of the whole.
Notice the example below of a household budget. (Which reminds me that I need to set up my own.)
Notice that the percentage of income going to each expense is represented by a slice.

Different Types of Charts for Data Visualization
To better understand chart types and how you can use them, here's an overview of each:
1. Column Chart
Use a column chart to show a comparison among different items or to show a comparison of items over time. You could use this format to see the revenue per landing page or customers by close date.
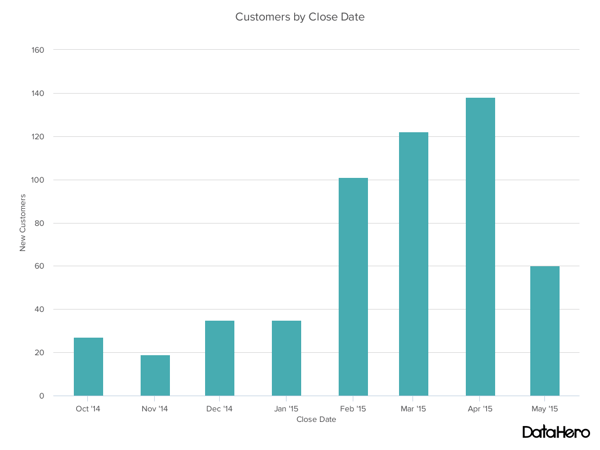
Best Use Cases for This Type of Chart
I use both column charts to display changes in data, but I've noticed column charts are best for negative data. The main difference, of course, is that column charts show information vertically while bar charts show data horizontally.
For example, warehouses often track the number of accidents on the shop floor. When the number of incidents falls below the monthly average, a column chart can make that change easier to see in a presentation.
In the example above, this column chart measures the number of customers by close date. Column charts make it easy to see data changes over a period of time. This means that they have many use cases, including:
- Customer survey data, like showing how many customers prefer a specific product or how much a customer uses a product each day.
- Sales volume, like showing which services are the top sellers each month or the number of sales per week.
- Profit and loss, showing where business investments are growing or falling.
Design Best Practices for Column Charts
- Use horizontal labels to improve readability.
- Start the y-axis at 0 to appropriately reflect the values in your chart .
2. Area Chart
Okay, an area chart is basically a line chart, but I swear there's a meaningful difference.
The space between the x-axis and the line is filled with a color or pattern. It is useful for showing part-to-whole relations, like showing individual sales reps’ contributions to total sales for a year.
It helps me analyze both overall and individual trend information.
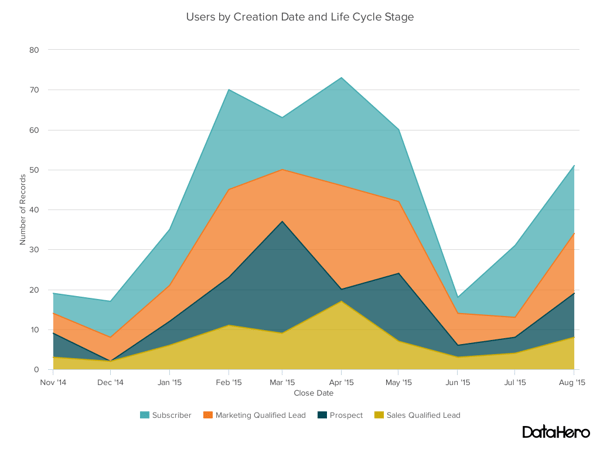
Best Use Cases for These Types of Charts
Area charts help show changes over time. They work best for big differences between data sets and help visualize big trends.
For example, the chart above shows users by creation date and life cycle stage.
A line chart could show more subscribers than marketing qualified leads. But this area chart emphasizes how much bigger the number of subscribers is than any other group.
These charts make the size of a group and how groups relate to each other more visually important than data changes over time.
Area charts can help your business to:
- Visualize which product categories or products within a category are most popular.
- Show key performance indicator (KPI) goals vs. outcomes.
- Spot and analyze industry trends.
Design Best Practices for Area Charts
- Use transparent colors so information isn't obscured in the background.
- Don't display more than four categories to avoid clutter.
- Organize highly variable data at the top of the chart to make it easy to read.
3. Stacked Bar Chart
I suggest using this chart to compare many different items and show the composition of each item you’re comparing.
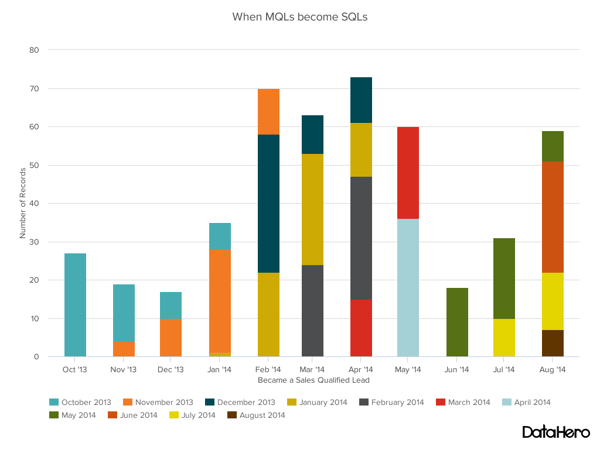
These charts are helpful when a group starts in one column and moves to another over time.
For example, the difference between a marketing qualified lead (MQL) and a sales qualified lead (SQL) is sometimes hard to see. The chart above helps stakeholders see these two lead types from a single point of view — when a lead changes from MQL to SQL.
Stacked bar charts are excellent for marketing. They make it simple to add a lot of data on a single chart or to make a point with limited space.
These charts can show multiple takeaways, so they're also super for quarterly meetings when you have a lot to say but not a lot of time to say it.
Stacked bar charts are also a smart option for planning or strategy meetings. This is because these charts can show a lot of information at once, but they also make it easy to focus on one stack at a time or move data as needed.
You can also use these charts to:
- Show the frequency of survey responses.
- Identify outliers in historical data.
- Compare a part of a strategy to its performance as a whole.
Design Best Practices for Stacked Bar Charts
- Best used to illustrate part-to-whole relationships.
- Use contrasting colors for greater clarity.
- Make the chart scale large enough to view group sizes in relation to one another.
4. Mekko Chart
Also known as a Marimekko chart, this type of chart can compare values, measure each one's composition, and show data distribution across each one.
It's similar to a stacked bar, except the Mekko's x-axis can capture another dimension of your values — instead of time progression, like column charts often do. In the graphic below, the x-axis compares the cities to one another.
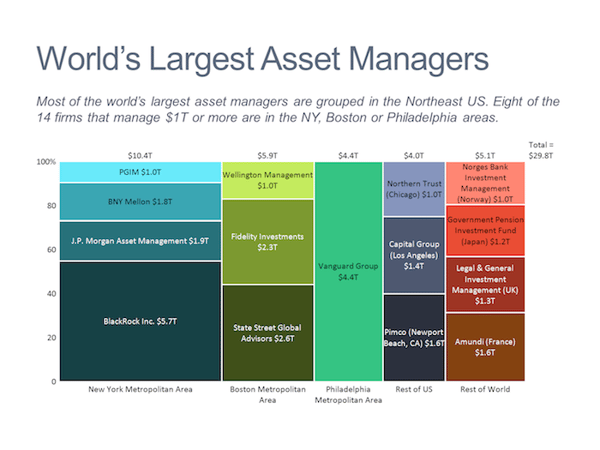
Image Source
I typically use a Mekko chart to show growth, market share, or competitor analysis.
For example, the Mekko chart above shows the market share of asset managers grouped by location and the value of their assets. This chart clarifies which firms manage the most assets in different areas.
It's also easy to see which asset managers are the largest and how they relate to each other.
Mekko charts can seem more complex than other types of charts, so it's best to use these in situations where you want to emphasize scale or differences between groups of data.
Other use cases for Mekko charts include:
- Detailed profit and loss statements.
- Revenue by brand and region.
- Product profitability.
- Share of voice by industry or niche.
Design Best Practices for Mekko Charts
- Vary your bar heights if the portion size is an important point of comparison.
- Don't include too many composite values within each bar. Consider reevaluating your presentation if you have a lot of data.
- Order your bars from left to right in such a way that exposes a relevant trend or message.
5. Pie Chart
Remember, a pie chart represents numbers in percentages, and the total sum of all segments needs to equal 100%.
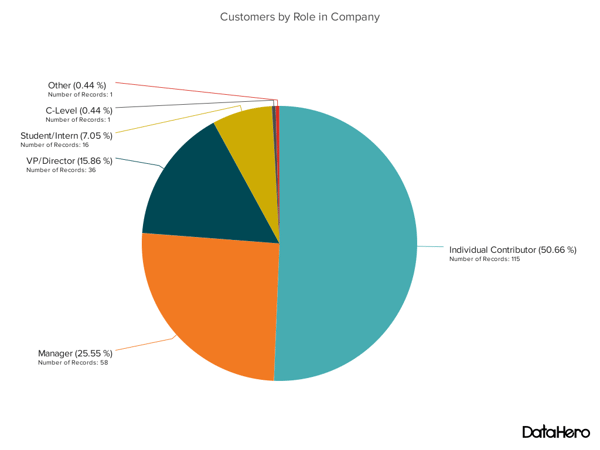
The image above shows another example of customers by role in the company.
The bar chart example shows you that there are more individual contributors than any other role. But this pie chart makes it clear that they make up over 50% of customer roles.
Pie charts make it easy to see a section in relation to the whole, so they are good for showing:
- Customer personas in relation to all customers.
- Revenue from your most popular products or product types in relation to all product sales.
- Percent of total profit from different store locations.
Design Best Practices for Pie Charts
- Don't illustrate too many categories to ensure differentiation between slices.
- Ensure that the slice values add up to 100%.
- Order slices according to their size.
6. Scatter Plot Chart
As I said earlier, a scatter plot or scattergram chart will show the relationship between two different variables or reveal distribution trends.
Use this chart when there are many different data points, and you want to highlight similarities in the data set. This is useful when looking for outliers or understanding your data's distribution.
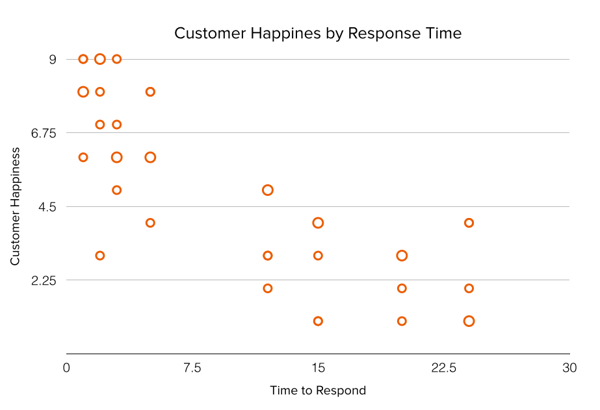
Scatter plots are helpful in situations where you have too much data to see a pattern quickly. They are best when you use them to show relationships between two large data sets.
In the example above, this chart shows how customer happiness relates to the time it takes for them to get a response.
This type of chart makes it easy to compare two data sets. Use cases might include:
- Employment and manufacturing output.
- Retail sales and inflation.
- Visitor numbers and outdoor temperature.
- Sales growth and tax laws.
Try to choose two data sets that already have a positive or negative relationship. That said, this type of chart can also make it easier to see data that falls outside of normal patterns.
Design Best Practices for Scatter Plots
- Include more variables, like different sizes, to incorporate more data.
- Start the y-axis at 0 to represent data accurately.
- If you use trend lines, only use a maximum of two to make your plot easy to understand.
7. Bubble Chart
A bubble chart is similar to a scatter plot in that it can show distribution or relationship. There is a third data set shown by the size of the bubble or circle.
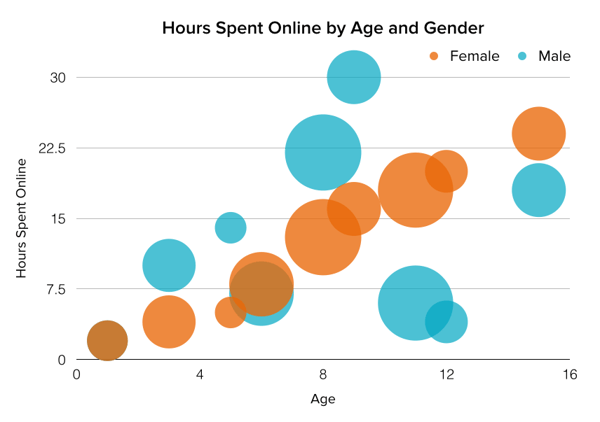
In the example above, the number of hours spent online isn't just compared to the user's age, as it would be on a scatter plot chart.
Instead, you can also see how the gender of the user impacts time spent online.
This makes bubble charts useful for seeing the rise or fall of trends over time. It also lets you add another option when you're trying to understand relationships between different segments or categories.
For example, if you want to launch a new product, this chart could help you quickly see your new product's cost, risk, and value. This can help you focus your energies on a low-risk new product with a high potential return.
You can also use bubble charts for:
- Top sales by month and location.
- Customer satisfaction surveys.
- Store performance tracking.
- Marketing campaign reviews.
Design Best Practices for Bubble Charts
- Scale bubbles according to area, not diameter.
- Make sure labels are clear and visible.
- Use circular shapes only.
8. Waterfall Chart
I sometimes use a waterfall chart to show how an initial value changes with intermediate values — either positive or negative — and results in a final value.
Use this chart to reveal the composition of a number. An example of this would be to showcase how different departments influence overall company revenue and lead to a specific profit number.
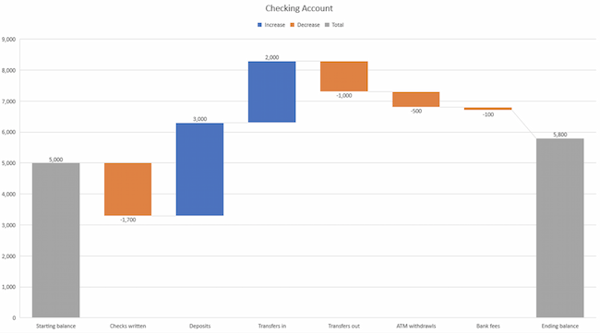
The most common use case for a funnel chart is the marketing or sales funnel. But there are many other ways to use this versatile chart.
If you have at least four stages of sequential data, this chart can help you easily see what inputs or outputs impact the final results.
For example, a funnel chart can help you see how to improve your buyer journey or shopping cart workflow. This is because it can help pinpoint major drop-off points.
Other stellar options for these types of charts include:
- Deal pipelines.
- Conversion and retention analysis.
- Bottlenecks in manufacturing and other multi-step processes.
- Marketing campaign performance.
- Website conversion tracking.
Design Best Practices for Funnel Charts
- Scale the size of each section to accurately reflect the size of the data set.
- Use contrasting colors or one color in graduated hues, from darkest to lightest, as the size of the funnel decreases.
10. Heat Map
A heat map shows the relationship between two items and provides rating information, such as high to low or poor to excellent. This chart displays the rating information using varying colors or saturation.
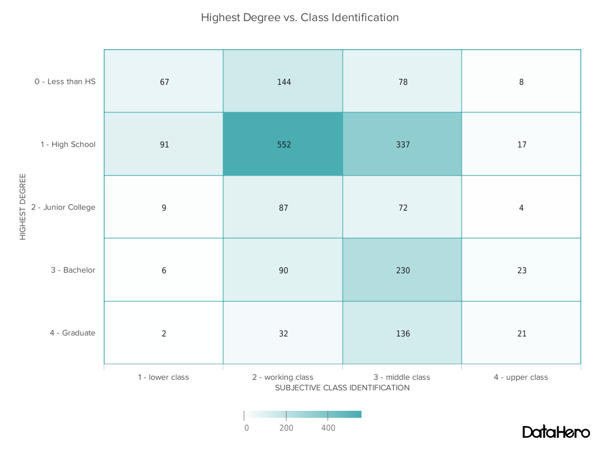
Best Use Cases for Heat Maps
In the example above, the darker the shade of green shows where the majority of people agree.
With enough data, heat maps can make a viewpoint that might seem subjective more concrete. This makes it easier for a business to act on customer sentiment.
There are many uses for these types of charts. In fact, many tech companies use heat map tools to gauge user experience for apps, online tools, and website design .
Another common use for heat map charts is location assessment. If you're trying to find the right location for your new store, these maps can give you an idea of what the area is like in ways that a visit can't communicate.
Heat maps can also help with spotting patterns, so they're good for analyzing trends that change quickly, like ad conversions. They can also help with:
- Competitor research.
- Customer sentiment.
- Sales outreach.
- Campaign impact.
- Customer demographics.
Design Best Practices for Heat Map
- Use a basic and clear map outline to avoid distracting from the data.
- Use a single color in varying shades to show changes in data.
- Avoid using multiple patterns.
11. Gantt Chart
The Gantt chart is a horizontal chart that dates back to 1917. This chart maps the different tasks completed over a period of time.
Gantt charting is one of the most essential tools for project managers. It brings all the completed and uncompleted tasks into one place and tracks the progress of each.
While the left side of the chart displays all the tasks, the right side shows the progress and schedule for each of these tasks.
This chart type allows you to:
- Break projects into tasks.
- Track the start and end of the tasks.
- Set important events, meetings, and announcements.
- Assign tasks to the team and individuals.
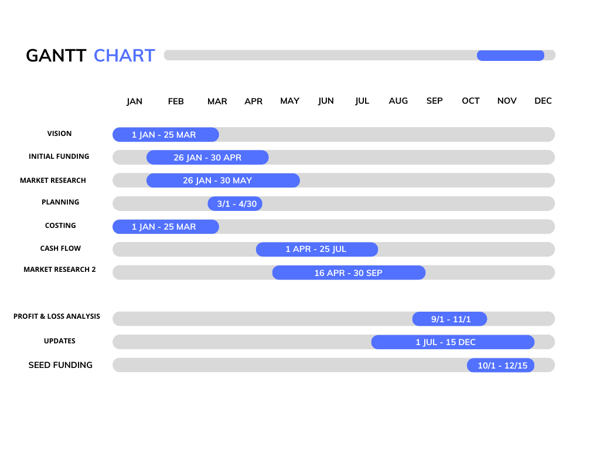
I use donut charts for the same use cases as pie charts, but I tend to prefer the former because of the added benefit that the data is easier to read.
Another benefit to donut charts is that the empty center leaves room for extra layers of data, like in the examples above.
Design Best Practices for Donut Charts
Use varying colors to better differentiate the data being displayed, just make sure the colors are in the same palette so viewers aren't put off by clashing hues.
How to Choose the Right Chart or Graph for Your Data
Channels like social media or blogs have multiple data sources, and managing these complex content assets can get overwhelming. What should you be tracking? What matters most?
How do you visualize and analyze the data so you can extract insights and actionable information?
1. Identify your goals for presenting the data.
Before creating any data-based graphics, I ask myself if I want to convince or clarify a point. Am I trying to visualize data that helped me solve a problem? Or am I trying to communicate a change that's happening?
A chart or graph can help compare different values, understand how different parts impact the whole, or analyze trends. Charts and graphs can also be useful for recognizing data that veers away from what you’re used to or help you see relationships between groups.
So, clarify your goals then use them to guide your chart selection.
2. Figure out what data you need to achieve your goal.
Different types of charts and graphs use different kinds of data. Graphs usually represent numerical data, while charts are visual representations of data that may or may not use numbers.
So, while all graphs are a type of chart, not all charts are graphs. If you don't already have the kind of data you need, you might need to spend some time putting your data together before building your chart.
3. Gather your data.
Most businesses collect numerical data regularly, but you may need to put in some extra time to collect the right data for your chart.
Besides quantitative data tools that measure traffic, revenue, and other user data, you might need some qualitative data.
These are some other ways you can gather data for your data visualization:
- Interviews
- Quizzes and surveys
- Customer reviews
- Reviewing customer documents and records
- Community boards
Fill out the form to get your templates.
4. select the right type of graph or chart..
Choosing the wrong visual aid or defaulting to the most common type of data visualization could confuse your viewer or lead to mistaken data interpretation.
But a chart is only useful to you and your business if it communicates your point clearly and effectively.
Ask yourself the questions below to help find the right chart or graph type.
Download the Excel templates mentioned in the video here.
5 Questions to Ask When Deciding Which Type of Chart to Use
1. do you want to compare values.
Charts and graphs are perfect for comparing one or many value sets, and they can easily show the low and high values in the data sets. To create a comparison chart, use these types of graphs:
- Scatter plot
2. Do you want to show the composition of something?
Use this type of chart to show how individual parts make up the whole of something, like the device type used for mobile visitors to your website or total sales broken down by sales rep.
To show composition, use these charts:
- Stacked bar
3. Do you want to understand the distribution of your data?
Distribution charts help you to understand outliers, the normal tendency, and the range of information in your values.
Use these charts to show distribution:
4. Are you interested in analyzing trends in your data set?
If you want more information about how a data set performed during a specific time, there are specific chart types that do extremely well.
You should choose one of the following:
- Dual-axis line
5. Do you want to better understand the relationship between value sets?
Relationship charts can show how one variable relates to one or many different variables. You could use this to show how something positively affects, has no effect, or negatively affects another variable.
When trying to establish the relationship between things, use these charts:
Featured Resource: The Marketer's Guide to Data Visualization
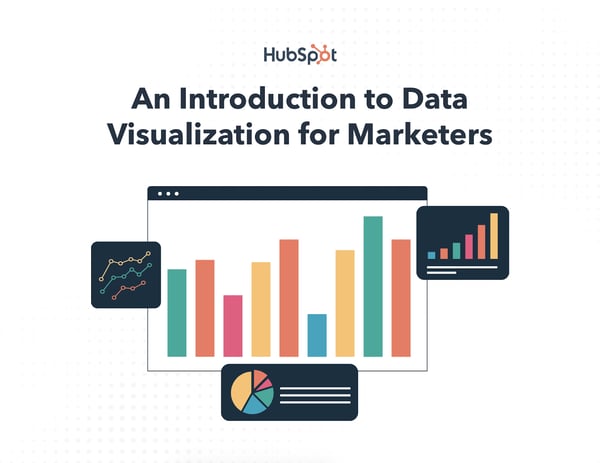
Don't forget to share this post!
Related articles.
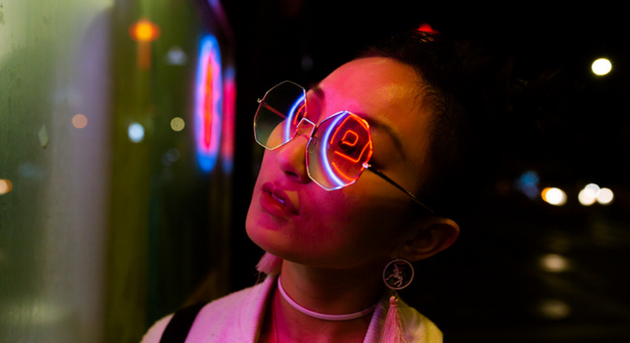
9 Great Ways to Use Data in Content Creation
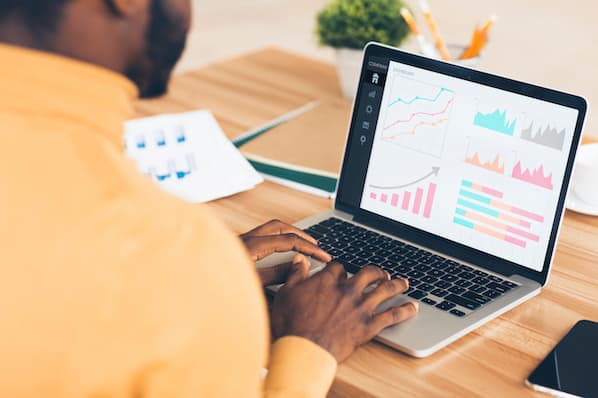
Data Visualization: Tips and Examples to Inspire You
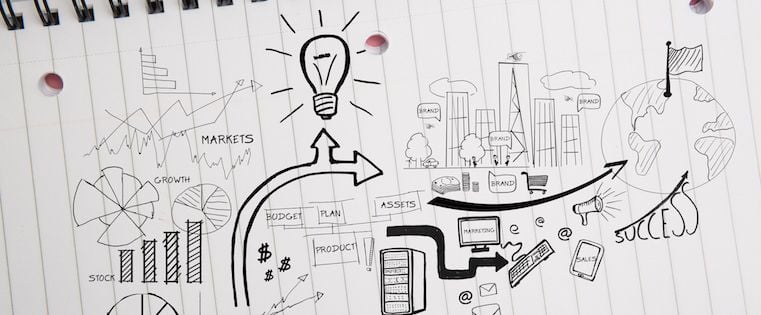
17 Data Visualization Resources You Should Bookmark
![visual representation in data An Introduction to Data Visualization: How to Create Compelling Charts & Graphs [Ebook]](https://53.fs1.hubspotusercontent-na1.net/hubfs/53/data-visualization-guide.jpg)
An Introduction to Data Visualization: How to Create Compelling Charts & Graphs [Ebook]
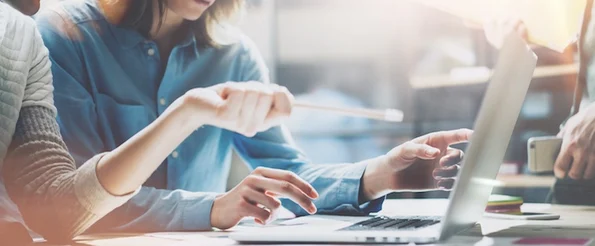
Why Data Is The Real MVP: 7 Examples of Data-Driven Storytelling by Leading Brands
![visual representation in data How to Create an Infographic Using Poll & Survey Data [Infographic]](https://53.fs1.hubspotusercontent-na1.net/hubfs/53/00-Blog_Thinkstock_Images/Survey_Data_Infographic.jpg)
How to Create an Infographic Using Poll & Survey Data [Infographic]
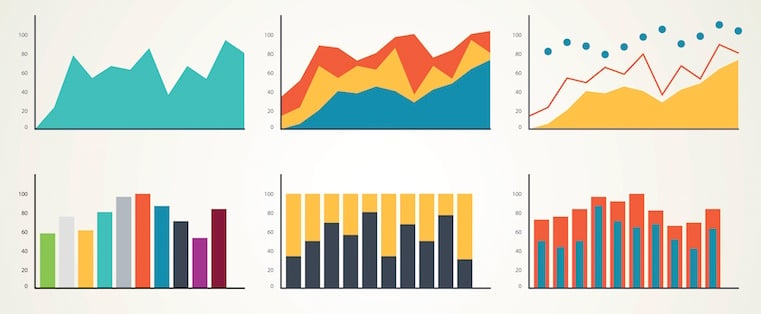
Data Storytelling 101: Helpful Tools for Gathering Ideas, Designing Content & More
Tired of struggling with spreadsheets? These free Microsoft Excel Graph Generator Templates can help
Marketing software that helps you drive revenue, save time and resources, and measure and optimize your investments — all on one easy-to-use platform
How to Visualize Data: 6 Rules, Tips and Best Practices
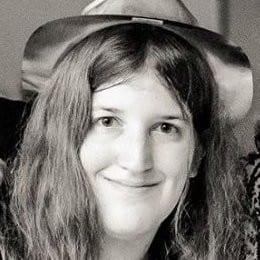
Table of contents
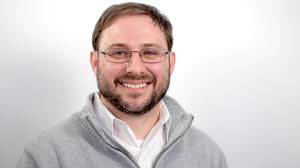
Create interactive data visualizations, unlock data-driven insights, and visualize real-time performance effortlessly with Databox. To discover how, visit this page to learn more.
Creating a great visual report is a lot like being reporting on a news story. Let me explain.
- You start by collecting all of your sources (i.e. data).
- Next, you analyze all of the information/data.
- Then, you put it altogether in a compelling story.
Great visual reporting is all about telling a compelling story through a mix of data visualizations.
In this post, we’re taking a closer looking at what data visualization is along with some best practices.
What is Data Visualization?
Let’s dive in.
Data visualization is the process of turning data into a compelling visual story through the use of graphics, like charts and graphs.
It is one of the most effective ways to show trends and patterns from data analysis.
So, it is no surprise that when we asked 57 data analysts how important data visualization is that 83% said it was very important.
And, 72.2% even confessed to saying that data visualization played a pivotal role in a project’s success.
The two most common formats for visualizing data are dashboards and reports. This allows you to showcase several different images to paint a more compelling story.
In fact, the average dashboard, according to our experts, contains 3-5 charts or graphs. When you use multiple charts in a dashboard, it is important to mix up the format.
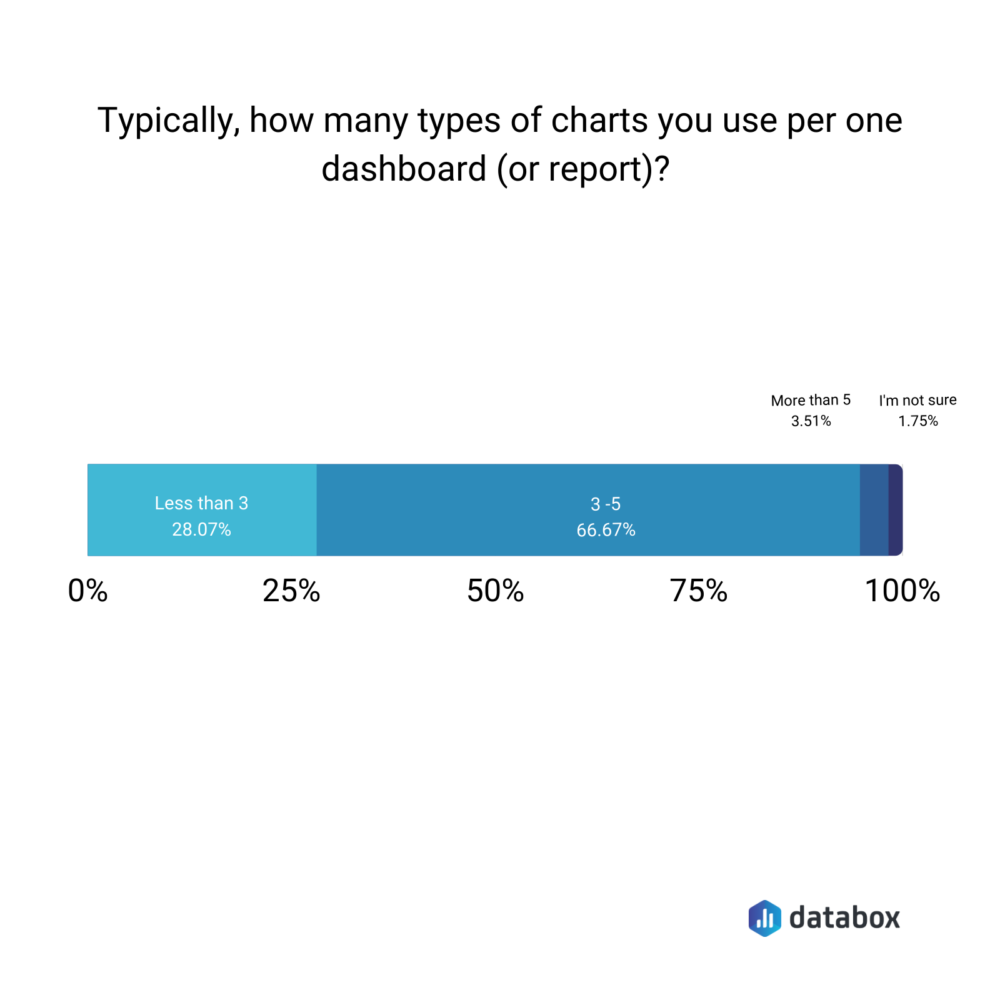
“If you use the same type of visualization every time you present data, it’s as boring as using the same structure in every sentence you speak,” says Melanie Musson of AutoInsureSavings.org . “You’ll lose your audience even if you have great information. Use all the charts but think outside the box. One of my favorite techniques to really make an impact is to use analogy.
For example, if we had a significant improvement toward a goal, I might include a picture of a mouse indicating our small success last year, and an elephant to represent this year. It’s a little silly, but it mixes things up.”
This also doesn’t mean you need to stick to the most popular chart formats – line and bar graphs.
In fact, Eden Cheng of PeopleFinderFree says, “My personal favorite is the bubble chart. It looks attractive and displays information in a very impressive way. Here, the weight of the value is defined by the bubble circumference. So, one can easily spot which factor is important and which is not.
It’s more of storytelling and conveys the idea impactfully. I use it to demonstrate data related to cost and value comparison, highlight the area of constant focus, and streamline activities.
If a bubble chart is not used then my next pick is a customary pie chart. Without making things look clumsy, it conveys the information well. Both these data visualization ways are visually pleasant as multiple colors can be used and information can be conveyed without any mess. But, a pie chart can only be used if you have a few variables or factors to display.”
Angus Chang of Lupilon adds, “We (also) visualize our data with pie charts because it clearly shows proportion and is easy to understand. Pie charts show what percentage each value contributes to the total. The charts are more intuitive than simply listing percentage values that add up to 100%. Using this chart, we illustrate which campaign brings in the biggest share of total leads. A pie chart is our priority because it depicts data accurately and represents percentages.”
Others prefer using funnel charts.
“I personally enjoy funnels and I think they are super helpful for understanding website analytics and just the general user journey as they’re interacting throughout your website,” says Elizabeth Weatherby of Wolf Consulting, LLC, “Funnels can show what page paths users chose, what exit pages users left the site on, and can even give you some solid insight into conversions. No matter what type of visualization you choose, just make sure your date ranges are accurate so you know exactly what data you’re viewing and what you’re comparing it to.”
It is also worth noting that charts and graphs aren’t the only type of visualization. You can also use word clouds to demonstrate trends.
“I use data visualization routinely in the form of word clouds,” explains Janice Wald of Mostly Blogging. “By posting the URL of my published content into a word cloud generator, I show the important results of my data collection since these appear in the biggest letter in the visual. I have just started incorporating line graphs into my content and found I rank higher and quicker. Google likes visuals, readers like visuals. Most people learn by looking at visuals. Incorporating visual data representation is a win-win.”
Pro Tip: Your Go-To Dashboard For Doing a Deeper Dive on Website Traffic and Conversion Sources
Struggling to find an easy yet effective way to gain a comprehensive understanding of your traffic sources, user behavior, and revenue generation?
You can do all that and more with our free plug-and-play GA4 Acquisition dashboard template:
- Understand user acquisition: See where users come from, tailor outreach, and track new user growth;
- Focus on high-performing channels: Identify top channels, optimize resource allocation, and adjust underperformers;
- Track revenue & engagement: Monitor revenue growth, active users, and the effectiveness of your campaigns;
- Go beyond traffic & conversions: Gain deeper insights into demographics, sales, customer journeys, ARPU, and more;
- Optimize marketing & drive results: Make data-driven decisions to improve your marketing strategy and achieve business goals.
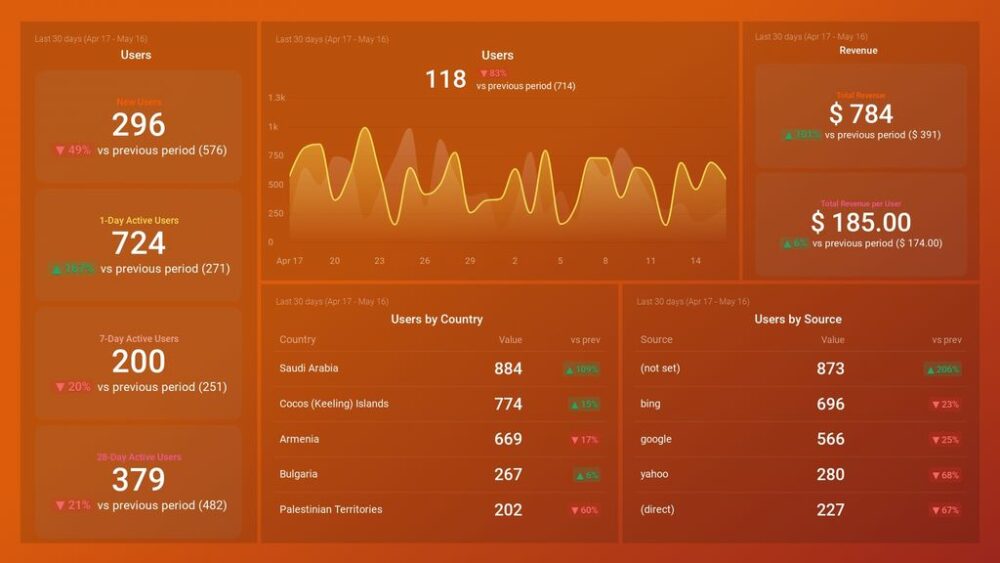
You can easily set it up in just a few clicks – no coding required.
To set up the dashboard, follow these 3 simple steps:
Step 1: Get the template
Step 2: Connect your Google Analytics 4 accounts with Databox.
Step 3: Watch your dashboard populate in seconds.
Here are some of the most important data visualization rules according to the data experts that we surveyed.
- Keep it simple
- Add white space
- Use purposeful design principles
- Focus on these three elements
- Make it easy to compare data
- Blend your data sources
1. Keep it simple
There is a tendency to overcomplicate it and add all of the charts, graphs, and data into your
dashboards and reports. This makes it hard to see what’s going on unless you are using dedicated marketing reporting software . Being concise and telling your story through the fewest, but most impactful charts are almost always the way to go.
“The main reason to visualize data is to get a point across,” says Amber Theurer of ivee. “For example, if your sales have increased within the past month, it would make sense for there to be a graph showing an incline of sales going in an upward motion from the left to the right side of the screen.
You don’t need to show any extra data that does not pertain to this topic, unless that data is covered on another page within another topic. Especially if you are presenting this data to your teammates at work, you don’t want to bore people with every single minute detail from your data to the point where they might lose interest.
Keep each data visualization clear and simple so that others will be able to easily understand what the data conveys.”
2. Add white space
A good rule of thumb is when in doubt, add more white space to your data dashboard .
“Well, I believe that the No. 1 rule for good data visualization is to let your data breathe,” says David Wurst of Webcitz. “When it comes to data visualization, one of the most common mistakes people make is trying to cram too much visual information into a single design. Whether it’s to “spice up” a design or to convey too much data, the end result is always the same: a visually cluttered, unintelligible design that asks more questions than it answers.”
Will Ward of Translation Equipment HQ adds, “Our number one rule with data visualization is to avoid ‘chartjunk’. This refers to both design elements such as borders, highlights, shading, as well as unnecessary labels, legends, and text. More often than not elements such as these detract from the comprehensibility of visual data, whilst adding very little substantial information in return.
Taking a hard anti-chartjunk stance has vastly reduced the amount of time we spend analyzing and discussing visual data. We found we were losing time discussing the pros and cons of design elements rather than the actual take-away from the data itself.
So, whilst chartjunk might make visual data look more pleasing, in some cases, most of the time it simply distracts. We’ve been able to reach conclusions and identify next steps easier and faster by keeping a strict philosophy of design simplicity regarding visual data.”
3. Use purposeful design principles
Another way to avoid cluttering your data reports and dashboards is to start with your end goal in mind.
“Have a clear goal before you choose the data you want to visualize,” explains Mile Zivkovic of Better Proposals. “Otherwise, you’ll create visualizations, charts, and graphs just for the sake of creating them.”
This applies both to the dashboard design as well as the individual chart level.
“Picking the best way to visualize your data is a lot like picking out the right outfit – you wouldn’t wear a tuxedo to go hiking and you shouldn’t use a pie chart to analyze a trend,” says Alex Kus of Buddy. “You’ve got to ensure your visual is fit to purpose, keeping your audience in mind and it should have one clear message that you want to be conveyed per graphic. Keep your visualization simple – if you’re like me and have seen one overly complex area chart too many, you’ll know this is important because it makes your data actually possible to parse.
Also, be aware that data visualization does not mean use no text – this is a common mistake of the overly enthusiastic designer, but you should really include labels with some text to bring attention to key points where necessary.”
Brad Touesnard of SpinupWP says, ”If you want to compare values, the best format is to use column charts which allow side-by-side comparisons of different values. Column charts are useful for data, such as website views and sessions, that don’t change much on a day-to-day basis. If you want to analyze a trend, line charts can illustrate how values in one or more categories change over the same period of time.
For example, you can use a line chart to visualize the monthly sales of two different product lines over a year. If you want to show proportions, pie charts are your best choice. For example, if you want to illustrate which campaigns generate the greatest share of your total leads, you can create a pie chart with slices for PPC, SEO, social media, and blogging.”
Ed Cravo of Groundbreaker adds, “It’s often challenging to choose the right visualization for the data you want to show. Before jumping to specifics, think about what you want to accomplish with the visualization, which helps you decide what data to include.
One size does not fit all so carefully consider and choose the right format for your visualization that will best tell the story and answer key questions generated by data—all of it connecting with your main purpose.
Once you’ve determined what types of visualizations work best for the data you have collected, it’s time to choose what delivery method makes sense.
If you need to get information out to customers, stakeholders, investors , or employees, an emailed report can be a good method of distribution, especially if this data is being tracked over a period of time where regular updates would be beneficial for your audience.
If you are using data analysis or a business intelligence platform, there are often in-app visualization options, such as templates or custom dashboards , email or PDF report delivery, and even scheduled ongoing reports.
If they’re done well, visualizations tell an interesting story. They can also shine a light on hidden information and details that you wouldn’t uncover in a bar chart, or pie graph or stacked bar chart in Google Sheet .”
4. Focus on these three elements
“I find it useful to keep in mind these three steps when I’m designing information-rich graphics: data, design, and feel,” explains Natasha Rei of Explainerd. “First, the data points need to add up logically and accurately. Check your math! Make sure that your story matches up with what you’re trying to show – if there’s a discrepancy between the data and the caption or scale’s title, then you’ve done something wrong.
Then step back from just purely making a pretty picture and think about how clear a chart will make a point or tell a story most effectively without too much clutter–are your labels readable? Appropriate headings? A simple graph with plenty of room for viewers’ notes might be better than one overloaded by too many numbers.”
5. Make it easy to compare data
Data isn’t just pretty charts. It serves a purpose. When designing for executives or investors, that purpose is usually to make it easier to spot trends, patterns, and see correlations. The easier you can make it compare your data, the better.
“Data visualization is vital to founders in assessing and analyzing company data, making it more valuable and extracting more insights,” says Anton Giuroiu of Homesthetics.net. “One essential advantage of data visualization is determining correlations easier than just plain data. Simple it may sound but column data are appropriate with comparing items and comparing data over time. In highlighting the peaks and trends, you can combine the column chart with the line chart. You can easily say which is higher and which is the highest, without looking at the numerical data.”
For example, Andrew Johnson of Prime Seamless says, “As a business owner, I use data visualization aided by a custom dashboard software to help identify sales trends and be able to compare certain aspects pertaining to my products. I have found that different forms of visual charts serve different purposes. A combination of line and column charts is best suited to help identify trends and to compare items/data over time. This is great for sales reports .
If you need to compare specific items, then a bar chart might be what you need. Personally, my business uses this form of visual data to compare the sales trends of different products and services for multiple age groups. Last but not least, pie charts are a game-changer when it comes to displaying proportional data or percentages. It gives meaningful context to the data and helps quantify the relationship between the data being compared.”
6. Blend your data sources
You also want to make sure your data is accurate, clean, and in a form that makes it compatible for comparing apples to apples.
“Good data visualization starts with blending all of your data sources in one place, and then display this data in a way that allows you to compare values and analyze trends over time,” says Rod Austin of Localize. “By organizing all of your key metrics with the flexibility to dial in views across multiple sources, you’re able to more effectively address real issues and elevate meaningful priorities from a more comprehensive vantage point.”
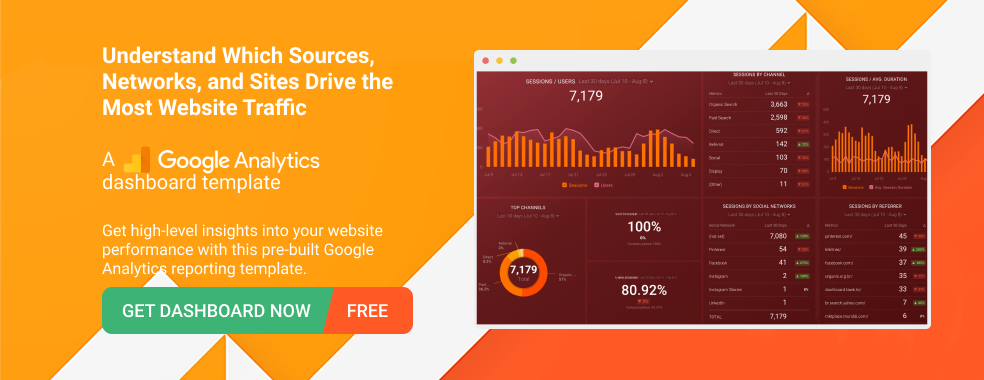
Visualize Your Data with Databox
Great data visualizations tell a compelling story. This starts by having a goal in mind and then working backwards to identify the number of charts and different formats you need. The final version can be shared as a report or a dashboard.
If you are looking for a simple way to share your data visualizations in a dashboard format, get started with Databox for free .
Dashboard Templates
- Marketing Dashboards
- Social Media Dashboards
- Paid Ads Dashboards
- SEO Dashboards
- Content Marketing Dashboards
- Lead Generation Dashboards
Want to create dashboards in minutes , without code?
Dashboards have never been easier with Databox. Join 20K+ customers and create beautiful dashboards easily by connecting your favorite data sources - all from GA4, Ads, and Social, to SEO, CRM, and Finance tools.

Jessica Malnik is a content strategist and copywriter for SaaS and productized service businesses. Her writing has appeared on The Next Web, Social Media Examiner, SEMRush, CMX, Help Scout, Convince & Convert, and many other sites.
Marketing Reporting: The KPIs, Reports, & Dashboard Templates You Need to Get Started
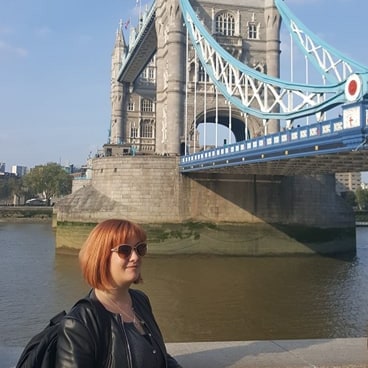
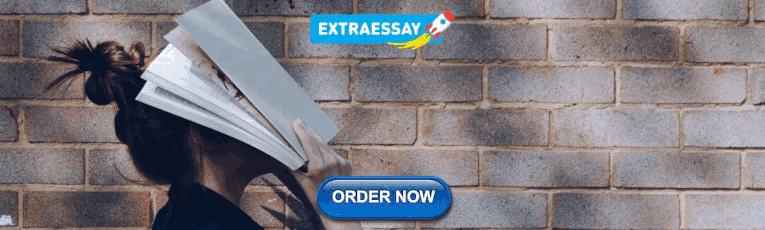
12 Tips for Developing a Successful Data Analytics Strategy
What is data reporting and how to create data reports for your business.
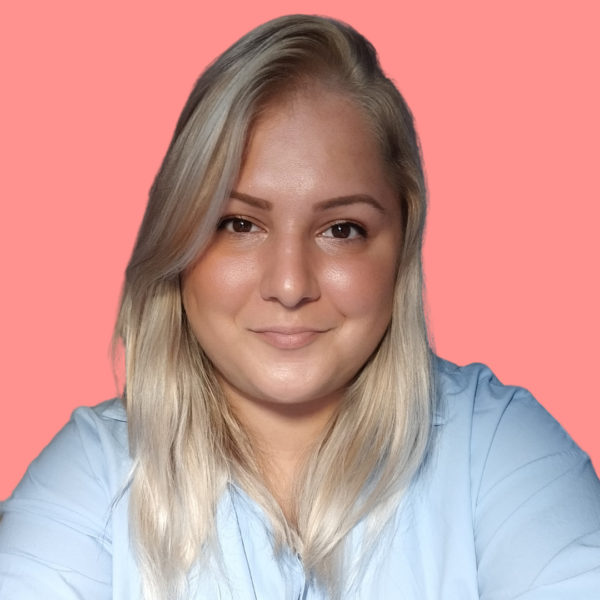
Get Started with Databox
Build your first dashboard in 5 minutes or less
Latest from our blog
- How Does Website Age Impact Performance? Insights from 145+ Companies August 8, 2024
- When and Why Should SMEs Upgrade Their Email Service Provider (ESP)? August 2, 2024
- Metrics & KPIs
- vs. Tableau
- vs. Looker Studio
- vs. Klipfolio
- vs. Power BI
- vs. Whatagraph
- vs. AgencyAnalytics
- Product & Engineering
- Inside Databox
- Terms of Service
- Privacy Policy
- Talent Resources
- We're Hiring!
- Help Center
- API Documentation
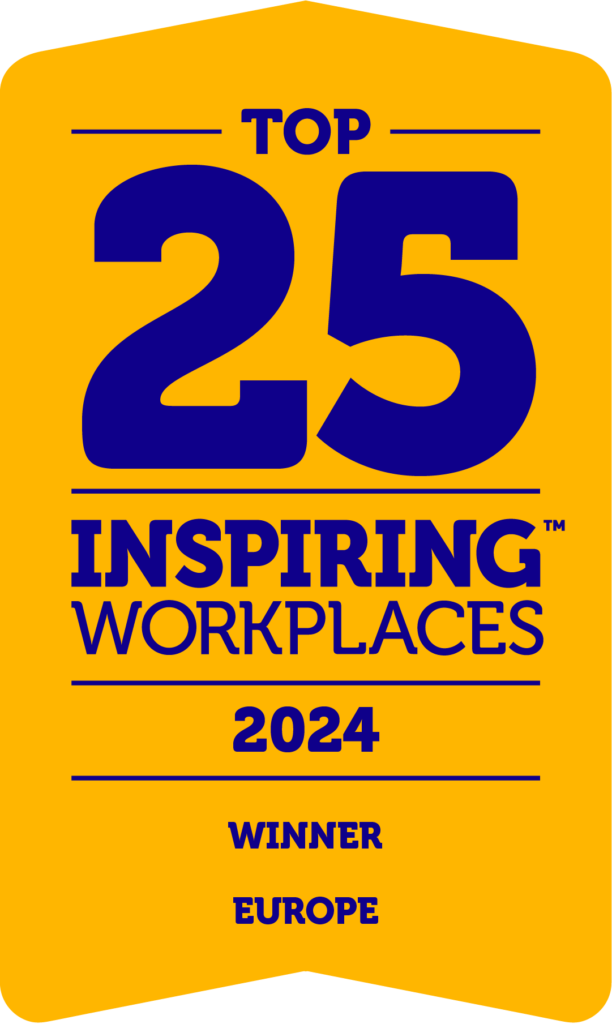
- ODSC EUROPE
- AI+ Training
- Speak at ODSC

- Data Analytics
- Data Engineering
- Data Visualization
- Deep Learning
- Generative AI
- Machine Learning
- NLP and LLMs
- Business & Use Cases
- Career Advice
- Write for us
- ODSC Community Slack Channel
- Upcoming Webinars

Mastering the Art of Data Visualization: Tips & Techniques
Data Visualization Modeling posted by ODSC Community October 5, 2023 ODSC Community
In the digital era, data visualization stands as an indispensable tool in the realm of business intelligence . It represents the graphical display of data and information, transforming complex datasets into intuitive and understandable visuals.
By implementing data visualization, businesses can reap multifaceted benefits:
- Simplified Data Interpretation : Complex data are converted into easily comprehensible visuals, enabling quick interpretation and understanding
- Enhanced Decision-making Process : Visual data representation aids in identifying patterns, trends, and outliers, fostering informed decision-making
- Improved Information Retention : Visuals increase information retention and recall, promoting long-term strategic planning
Remember that effective data visualization acts not only as a summary of your data but also as a guide towards informed decisions. By harnessing the power of visual grammar rules and frameworks like the McCandless Method or Kaiser Fung’s Junk Charts Trifecta Checkup, you can elevate your business intelligence strategy to new heights.
Key Principles and Best Practices for Data Visualization
Understanding the key principles and best practices for data visualization can significantly improve your ability to present data in a meaningful, digestible way.
Visual Grammar Rules
Visual grammar rules are fundamental to creating effective visual presentations. These include clarity, simplicity, and emphasis on the information rather than the graphic design itself.
- Clarity: The purpose of your visualization should be immediately clear to your audience. Avoid unnecessary complexity in charts, graphs, and diagrams.
- Simplicity: Keep designs as simple as possible while still conveying the necessary information. Extraneous elements can distract from the data you’re presenting.
- Emphasis on Information: The main focus should always be on the data, not on the aesthetics of the presentation. Avoid using overly flashy designs or effects that could detract from your message.
Consider these points when designing your own visualizations.
Consider a bar chart showing annual sales figures for your company’s product range. Making each bar a different color might make the chart look more appealing, but it can confuse the audience if there’s no clear reason for the color variation. Instead, use one color for all bars and differentiate them by labeling each one with the product name and sales figure.
This approach applies clarity , simplicity , and an emphasis on information , demonstrating how these visual grammar rules enhance data visualization best practices.
Incorporate these design principles into your own work to ensure your visualizations communicate effectively, keeping your audience engaged and informed without overwhelming them with unnecessary detail or confusing graphics.
Moving forward, let’s delve deeper into organizing visualization through popular frameworks that help structure data effectively. Using such frameworks will enable you to deliver insights more coherently, making it easier for your audience to understand complex datasets.
Frameworks for Organizing Visualization
Organizing data visualization is an art as much as it is a science. It’s where design principles meet visual grammar rules, creating effective and engaging visuals. Two popular frameworks that encapsulate data visualization best practices are the McCandless Method and Kaiser Fung’s Junk Charts Trifecta Checkup .
The McCandless Method, coined by David McCandless, advocates for a balance between information and design. This involves considering aspects like:
- Ensuring data accuracy
- Emphasizing clarity and precision
- Incorporating a meaningful color palette
- Utilizing pre-attentive attributes effectively to guide viewer attention
Meanwhile, Kaiser Fung’s Junk Charts Trifecta Checkup takes a more analytical approach to visualization. It encourages us to ask three key questions:
- What does the chart show?
- What does the data say?
- What relevant factor is missing?
By following these frameworks, you can create striking visuals that not only look good but also communicate your message effectively.
Effective Techniques for Data Visualization
Let’s dive into the world of Power BI to explore its features and learn some useful data visualization tips and tricks for data visualization. Power BI, a business analytics tool developed by Microsoft, offers interactive visualizations with self-service business intelligence capabilities.
Using Power BI Tips and Tricks
To begin with, Power BI stands out in the crowd due to its ability to produce beautiful reports with interactive visualizations. It allows sharing these reports directly within the platform or embedding them in an app or website. The tool’s drag-and-drop feature simplifies creating complex dashboards, making it user-friendly even for beginners.
One of the most potent features of Power BI is Quick Insights . This function finds patterns, trends, and correlations in the data automatically. To use it effectively:
- Select a dataset
- Click ‘Get Insights’
- Wait for Power BI to do its magic!
Another valuable feature is Natural Language Querying . With this feature, you can type in questions about your data in natural language and get immediate answers. For example, if you have an e-commerce business and want to know your best-selling product last month, just type “What was my top-selling product last month?” into the query box.
The Q&A Visual takes this one step further by allowing users to ask questions directly on the report page and presenting answers in a visual format. To harness this feature:
- Drag the Q&A button onto your report page
- Start asking questions!
Don’t forget about Bookmarking . This tool allows you to save a customized view of a report (filters and slicers) and return to it at any point. This is particularly useful when dealing with large datasets.
Lastly, consider using Data Drill Down for hierarchical data visualization. This technique helps users navigate from general overviews down to specific details in just a few clicks.
In the realm of data visualization, Power BI is a game-changer with its advanced features and user-friendly interface. Harness these data visualization tips and techniques to create compelling visualizations and gain valuable insights from your data. Up next, we’ll delve into more intriguing aspects of data visualization: keyboard shortcuts and custom themes. Stay tuned!
Utilizing Keyboard Shortcuts and Custom Themes
Efficiency in data visualization is crucial. One way to boost your productivity is through keyboard shortcuts . They provide a fast, seamless way to navigate your workspace, execute commands, and manipulate data. For instance, in Power BI, you can use Ctrl + M to start a new measure or Alt + Shift + F10 to access the context menu.
Complementing the use of shortcuts, custom themes are essential for enhancing visual appeal. They not only add color and style but also consistency across your visuals. You can create custom themes within Power BI by going to View > Themes > Customize current theme . From there, you can tweak colors, text properties, and visual elements to match your brand or preference.
Remember: The right combination of keyboard efficiency and design aesthetics elevates the impact of your data visualization techniques.
Data Modeling and Drill-Through Techniques
Data modeling plays a crucial role in data visualization. It’s the process of creating a visual representation of data, which can help to understand complex patterns and relationships. Using data modeling effectively allows you to uncover insights that would be difficult to grasp in raw, unprocessed data.
To illustrate, it’s like taking a jumbled pile of puzzle pieces and organizing them into an understandable image. When you organize your data in this way, it becomes easier for everyone to understand.
One of the most powerful techniques in data modeling is drill-through. This technique allows users to navigate from a summary view into detailed data. For instance, if you’re viewing sales data by region, a drill-through could allow you to click on one region and see the individual sales by city or even by store.
Here are some tips for implementing drill-through techniques:
- Plan Ahead : Define what detailed information would be useful before setting up your drill-throughs.
- Limit Your Layers : Too many layers can confuse users. Stick to a few key details.
- Use Clear Labels : Make sure it’s obvious what each layer represents.
These techniques, when used correctly, can dramatically enhance your ability to communicate complex information through your visualizations.
Real-Time Dashboards and Explaining Data
Access to real-time dashboards in data visualization provides a game-changing advantage. These dynamic tools compile and display data as it enters the system, allowing for immediate analysis and action. Benefits of utilizing real-time dashboards include:
- Keeping stakeholders informed with up-to-the-minute data
- Enabling rapid response to emerging trends or issues
- Facilitating ongoing optimization of strategies based on live data
To extract maximum value from your real-time dashboards, it’s essential to adequately explain the data they present. Densely packed data or complex graphs can be overwhelming without clear, concise explanations. Here are some techniques to effectively communicate complex data:
- Simplicity : Distil complex ideas into simple, understandable terms. Avoid jargon where possible.
- Context : Provide relevant background information to help readers understand why the data matters.
- Visual Aids : Use charts, graphs, and infographics to represent data visually, making it easier to digest.
- Narrative : Weave a story around the data to make it more engaging and relatable.
By employing these techniques, you can ensure that your audience not only sees the numbers but also comprehends their implications. Remember that balancing real-time insight with effective explanations can greatly improve your decision-making process in any online business venture.
Through this article, we’ve unlocked the potential of data visualization , from understanding its benefits to adopting best practices. We’ve dived into Visual Grammar Rules and explored frameworks like the McCandless Method and Kaiser Fung’s Junk Charts Trifecta Checkup . Power BI has been our companion, guiding us through data visualization tips, tricks, and techniques such as keyboard shortcuts, custom themes, data modeling, and drill-through techniques.
We encourage you to leverage these insights in your quest for effective data visualization. Remember, the key is to keep experimenting and learning. Let’s transform complex data into understandable visuals together. After all, a picture is worth a thousand words.
About the author on data visualization tips:

ODSC Community
The Open Data Science community is passionate and diverse, and we always welcome contributions from data science professionals! All of the articles under this profile are from our community, with individual authors mentioned in the text itself.

3 Ways to Boost Your Prompt Engineering for AI-Assisted Programming
West 2024 Generative AI posted by ODSC Community Aug 13, 2024 Editor’s note: Tom Taulli is a speaker for ODSC West 2024 this October 29th-31st. Be sure...

Domain-Driven Design in Practice: Crafting an AI Assistant Step-by-Step
Europe 2024 Modeling posted by ODSC Community Aug 13, 2024 Editor’s note: Shawn Kyzer is a speaker for ODSC Europe this September 5th-6th. Be sure to...

States Take the Lead in Regulating AI in Healthcare Amid Federal Inaction
AI and Data Science News posted by ODSC Team Aug 12, 2024 Due to the absence of comprehensive federal regulations on AI in health care, state governments are...


What Is Data Visualization and Why Is It Important?
The sheer amount of data generated today means we need new ways to understand what’s happening in order to take action faster. Every click, transaction, subscription, loyalty card swipe, and social media interaction contributes to a digital footprint that continues to grow exponentially. The result? A massive explosion of data that is revolutionizing the way we live and work. Data visualization, in particular, plays a critical role in presenting data in a meaningful and understandable format. By using a visual representation of data , it’s much easier to identify patterns, trends, and relationships that may not be immediately apparent when sifting through large data sets.
Here’s what we’ll cover in this guide to data visualization:
- Data Visualization Definition
Benefits of Data Visualization
Why data visualization is important .
- Types of Data Visualization and Examples
- Evaluating Data Visualization Tools
- Take the Next Step and Start Analyzing With Data Visualization
Data Visualization Definition
Data visualization is the process of transforming raw data into visual formats, such as charts, graphs, or maps, to help identify patterns, trends, and insights that might not be apparent from numerical data alone.
Additionally, it enables data to be more accessible, understandable, and impactful, especially when communicating with stakeholders, investors, or team members who may not be familiar with the data.
For example, data visualization could help:
- In retail, gaining insights into customer behavior, purchase patterns, and product performance.
- In finance, monitoring market trends, tracking portfolio performance, and conducting risk analysis.
- In public health, showing the geographical distribution of outbreaks and helping track the spread of infectious diseases.
- In supply chain industries, tracking inventory levels, monitoring logistics operations, and optimizing resource allocation.
- In sports, evaluating player performance, game strategies, and match statistics.
- In education, tracking student performance, analyzing learning outcomes, and identifying areas for improvement.
Data visualization has several benefits for businesses including: the ability to process information faster, identify trends at scale, and make data more digestible. Companies regularly use data to make decisions, and through data visualization, can find insights quickly and move to action. Data visualization specifically helps with the following:
- Visualizing patterns and relationships
- Storytelling, including specifically data storytelling
- Accessibility to information
Exploration
Let’s take a look at each of these benefits in detail.
Visualize patterns and relationships
Data visualization constitutes an excellent method for the discernment of interconnections and patterns amidst vast collections of information. For example, a scatter plot can be used to display the relationship between two variables, such as the correlation between temperature and sales. This enables users to understand the relationship and identify trends and outliers more quickly and easily.
Read a guide of Sigma’s visual library.

Storytelling
Your audience, whether it's coworkers or clients, want to hear a coherent story from your data. Storytelling with data cannot be done successfully without visualizations. Colorful charting and dynamic pivots are just as important as characters and plots are in a traditional story, so using them to communicate information makes data that much more engaging and memorable for audiences. Data can be complex and convoluted for some audiences, so data storytelling is an approach to convey important information effectively through a captivating narrative. Good visualizations are a vital part of that narrative.
For example, if an analyst is investigating the performance of e-commerce sales for their retail company over time, they may leverage several data sources such as spreadsheets, calculations, code, etc. to do so. However, when they report these new insights to their stakeholders, the analyst will need to summarize and communicate their findings in a digestible way.
An easy way the analyst could do this is by using the data to create a map of the U.S. with a color gradient overlaying every state that is lighter or darker based on its total sales volume. This visual story tells the least and most successful retail locations at a glance.

Accessibility / Easily Share Information
Data visualization serves as an invaluable mechanism for the facilitation of accessibility, allowing for the communication of information amongst individuals, even for those who may not usually engage with data , which broadens the audience.
Visualizations help simplify complex information by leveraging people’s ability to naturally recognize patterns. A viewer typically does not have to be taught that bigger means more and that smaller means less. In a case where an analyst wants to highlight the difference in scale between one product’s profitability vs. another, a bar chart can clearly show the user which product is more profitable and by how much, making it easy for even non-technical team members to understand and compare the performance of different products.
Exploration is a key component of successful data visualization. The more flexible charting and dashboarding is, the more follow-up questions end users can ask directly of their data. For example, an interactive dashboard can be used to explore retail sales data over time, enabling users to filter and drill down into the data to identify trends and patterns.
Data visualization exploration is often associated with the concept of “drill downs.” Drill downs in data visualization refer to the process of starting with an overview of data and then narrowing the focus to more specific aspects of it. As an example, one might start with a visualization of global climate data and drill down to data about a specific country, a specific state, a specific city, or even a specific neighborhood within that city. Each drill down reveals more precise, detailed, and nuanced information.
The main goal of data visualization is that it helps unify and bring teams onto the same page. The human mind is wired to grasp visual information more effortlessly than raw data in spreadsheets or detailed reports. Thus, graphical representation of voluminous and intricate data is more user-friendly. Data visualization offers a swift and straightforward method to communicate ideas in a universally understood format, with the added benefit of enabling scenario testing through minor modifications.
By translating information into visual form, it ensures everyone, irrespective of the complexity of the data or the depth of the analysis, can share a unified understanding. Any industry can benefit from using data visualization, because pretty much every industry relies on data to power it. That includes finance, marketing, consumer goods, education, government, sports, history, and many more. Another thing to keep in mind is that data visualization can be a double-edged sword. For example, charts can be manipulated and skewed to force a desired outcome. Ungoverned, static, desktop tools can become the wild west in suggesting an inaccurate outcome “proven by data.” Even in the cases where the visualization builder is acting in good faith, there are still pitfalls to watch out for. Always be considerate of:
- Individual outliers having an outsized impact, skewing the visual direction of a chart
- The need for for business users to see the underlying data
- Allowing for transparency down to row-level detail in data sets

Types of Data Visualizations & Examples
There is a long list of types of data visualization techniques and methods that can be used to represent data. While no type of data visualization is perfect, we’ll walk through different examples and when to apply each one.
We’ll be looking at:
- Line charts and area charts
- Scatter plots
- Pivot tables
- Box-and-whisker plots
- Sankey charts
Tables, although more commonly thought of as a data source, can also be considered a type of data visualization. Especially when conditional formatting is applied to the table’s rows and columns, the data within the table becomes more visually engaging and informative. With conditional formatting, important insights and patterns can be highlighted, making it easier for viewers to identify trends and outliers at a glance. Additionally, tables offer a structured and organized way to present information, allowing for a comprehensive comparison of data points, which further enhances data understanding and analysis. For example, Sigma’s UI is based on a spreadsheet-like interface, which means almost everything in Sigma begins in a table format. That said, you can also create visual tables that display a smaller amount of data in order to tell a clearer story. In data visualization, tables are a simplified way of representing this interface.
When to use tables:
- For detailed numeric comparisons, or when precision of data is key
- For displaying multidimensional data; tables can handle this complexity quite well
When to avoid tables:
- When patterns, trends, or relationships need to be highlighted at a glance
- When dealing with large amounts of data

Pie charts —similar to stacked bar charts—are useful for displaying categorical data, such as market share or customer demographics. Pie charts are often used to display data that can be divided into categories or subgroups, and to show how each category or subgroup contributes to the whole. For example, a pie chart could be used to show the proportion of sales for different product categories in a given period of time, or the percent of a company's revenue broken down by various regions.
When to use pie charts:
- You want to display a proportion or percentage of a whole
- You’re visualizing only seven categories or less
When to avoid pie charts:
- You’re visualizing more than seven categories
- You want to compare something with more details, rather than just proportion
- You want to display and pinpoint exact values
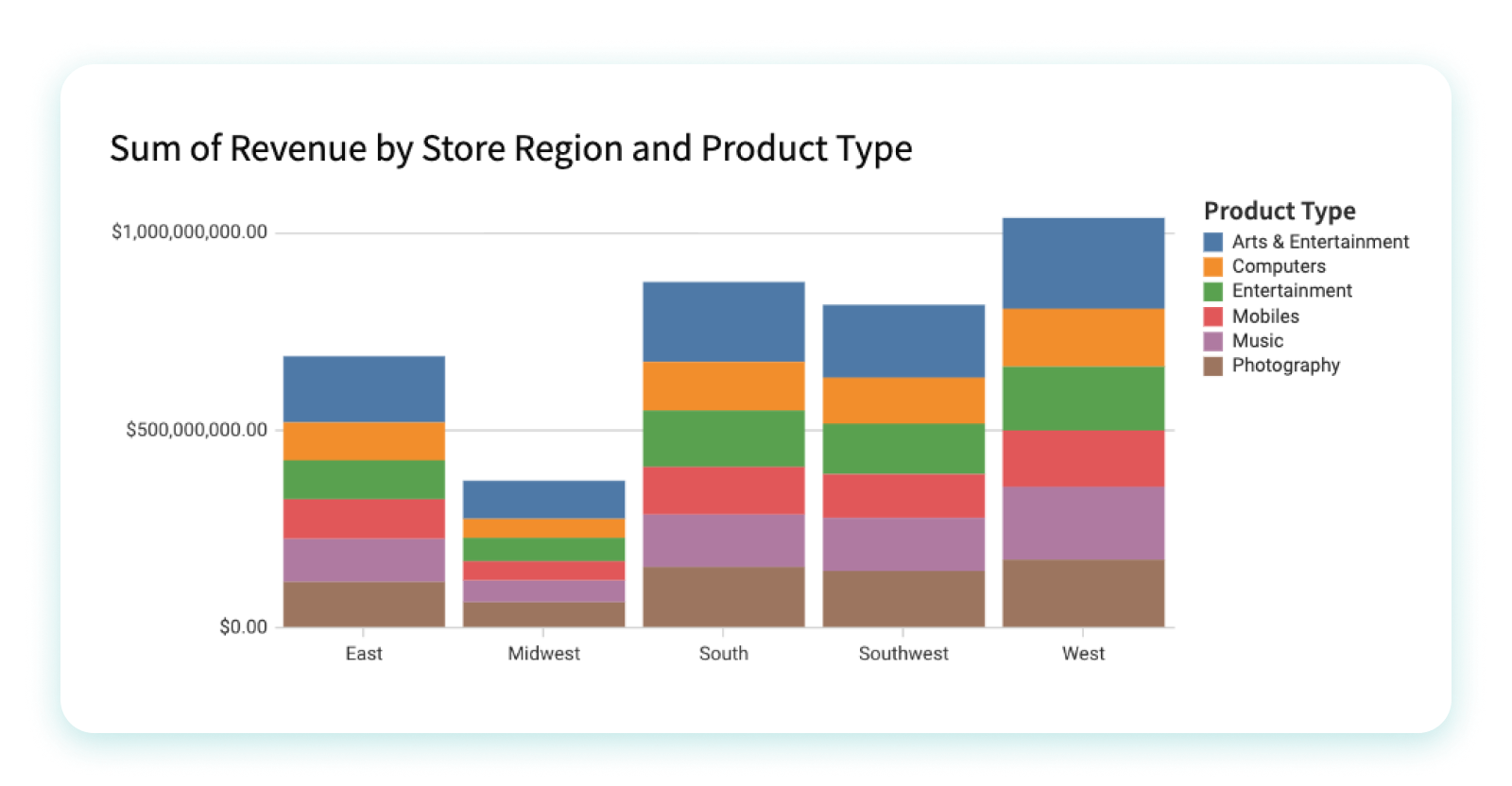
A bar chart, or bar graph, constitutes a variety of graphs that employ rectangular bars to depict data. These bars can be oriented either horizontally or vertically, with their extent being directly proportional to the numerical values they are intended to embody. Predominantly utilized for juxtaposing data across disparate categories or illustrating shifts in data over temporal progressions, bar charts offer a straightforward, yet potent means of conveying information visually. They frequently function as the initial tool in the exploratory process of data investigation.
When to use bar charts:
- Emphasizing and contrasting different sets of data, making the disparities or similarities between categories clear
- To display a subset of a larger dataset
When to avoid bar charts:
- When a particular field encompasses an overwhelming variety of data types
- When the differences between fields are too subtle, or when these differences exist on different scales, as it could lead to confusion or misinterpretation
Line Charts & Area Charts

Line charts and area charts are two types of charts that are commonly used to visualize data trends over time. A line chart, also called a line graph, is a distinct type of graphical representation that exhibits information in the form of a multitude of data points, which are interconnected by unbroken lines. These line charts are typically employed to demonstrate transformations in data over a certain duration, where the horizontal axis symbolizes time, and the vertical axis signifies the values under scrutiny. Furthermore, they can serve to juxtapose several series of data within the same chart, or to graphically illustrate predicted time periods.
For example, a line chart can be used to visualize a company's stock prices over the course of a year. Similarly, an area chart can be used to visualize the temperature changes over a day.
When to use line charts:
- When you’re displaying time-based continuous data
- When you have multiple series or larger datasets
When to avoid line charts:
- When you have smaller datasets, bar charts are likely a better way to present the information
- Avoid when you need to compare multiple categories at once

When to use area charts:
- When you want to display the volume of the data you have
- When comparing data across more than one time period
When to avoid area charts:
- Avoid if you need to compare multiple categories, as well as when you need to examine the specific data value
Scatter Plots
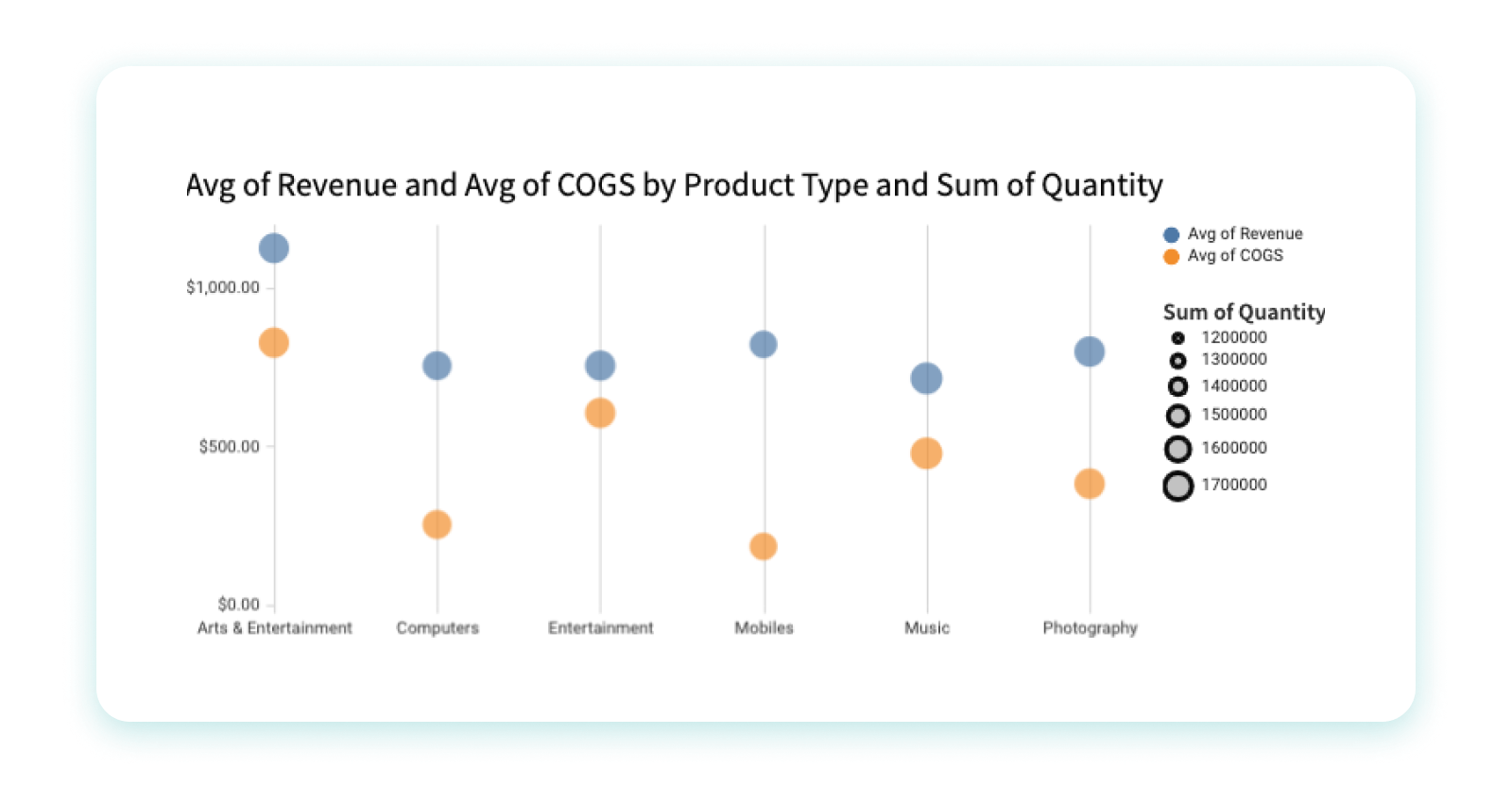
A scatter plot , also called a scatter chart or scatter graph, is a specialized form of chart that demonstrates the correlation between two distinct variables by mapping them as a succession of individual data points. Each data point denotes a combined value of the two variables, with its specific placement within the chart dictated by these values.
Scatter charts prove instrumental in discerning patterns and trends within data, and they also help us understand how strong and in what direction the relationship is between two variables. They also serve as effective tools for identifying outliers, or those data points that deviate significantly from anticipated values based on the pattern displayed by other data points. These charts find widespread use across a range of fields including, but not limited to, statistics, engineering, and social sciences, for the purpose of analyzing and visualizing intricate data sets. In the realm of business, they are frequently utilized to identify correlations between different variables, for instance, examining the relationship between marketing outlays and resultant sales revenue. For example, a scatter plot might be used to visualize the relationship between the age and income of a group of people. Another example would be to plot the correlation between the amount of rainfall and the crop yield for a particular region.
When to use scatter plots:
- Highlight correlations within your data
- They are useful tools for statistical investigations
- Consider scatter plots to reveal underlying patterns or trends
When to avoid scatter plots:
- For smaller datasets, scatter plots may not be optimal
- Avoid scatter plots for excessively large datasets to prevent unintelligible data clustering
- If your data lacks correlations, scatter plots may not be the best choice
Pivot Tables
While pivot tables may not be what first comes to mind for data visualization, they can give important context with hard numbers and provide strong visual indicators through formatting. Pivot tables can also be enhanced with conditional formatting to provide color scales that make performance trends more visible. Data bars can also be added to cells to run either red or green for positive and negative values.
When to Use Pivot Tables:
- Cohort analysis performance trends or portfolio analysis with a mix of positive and negative values
What Not to Use Pivot Tables:
- When your dataset is too large to get a good understanding of the whole
- When data can easily be summarized with a bar chart instead

An example of a pivot table, where colors are used to show positive or negative progress on a company’s portfolio. The user can pivot the table to show multiple categories in different ways.
A heat map is a type of chart that uses color to represent data values. It is often used to visualize data that is organized in a matrix or table format. The color of each cell in the matrix is determined by the value of the corresponding data point. Heat maps are best used when analyzing data that is organized in a two-dimensional grid or matrix.
For example, a heat map can be used to visualize a company's website traffic, where the rows represent different pages on the website, and the columns represent different periods of time.
When to use heat maps:
- When you need to visualize the density or intensity of variables
- When you want to display patterns or trends over time or space
When to avoid heat maps:
- When precise values are needed; heat maps are better at showing relative differences rather than precise values
- When working with small data sets
A tree map is a type of chart that is used to visualize hierarchical data. It consists of a series of nested rectangles, where the size and color of each rectangle represent a different variable. Tree maps are best used when analyzing data that has a hierarchical structure.
For example, a tree map can be used to visualize the market share of different companies in an industry. The largest rectangle would represent the entire industry, with smaller rectangles representing the market share of each individual company.
When to use tree maps:
- When you want to visualize hierarchical data
- When you need to illustrate the proportion of different categories within a whole
When to avoid tree maps:
- When exact values are important
- When there are too many categories
Box-and-Whisker Plots

Box plots are useful for quickly summarizing the distribution of a dataset, particularly its central tendency and variability. For example, a box-and-whisker plot can be used to visualize the test scores of a group of students.
Colloquially recognized as a box-and-whisker plot, a box plot is a distinct form of chart that showcases the distribution of a collection of numerical data through its quartile divisions. Box plots serve as efficient tools for rapidly encapsulating the distribution of a dataset, specifically its central propensity and variability.
A box-and-whisker plot consists of a rectangle (the "box") and a pair of "whiskers" that extend from it. The box embodies the middle 50% of the data, with the lower boundary of the box signaling the first quartile (25th percentile) and the upper boundary of the box indicating the third quartile (75th percentile). The line situated within the box signifies the median value of the data. The whiskers project from the box to the minimum and maximum values of the data, or to a designated distance from the box referred to as the "fences." Any data points that reside outside the whiskers or fences are categorized as outliers and are plotted as individual points. When to use box plot charts:
- When you want to display data spread and skewness
- When showcasing the distribution of data, including the range, quartiles, and potential outliers
- When comparing multiple groups or categories side-by-side; they allow for easy comparison of different distributions.
When to avoid box plot charts:
- If you need to show more detail, since box plots focus on a high-level summary
- When individual data points are important to the story you’re telling
- When your audience isn’t familiar with them, since they can sometimes be less intuitive than other types of visualizations
A histogram is a type of chart that displays the distribution of a dataset. It consists of a series of vertical bars, where the height of each bar represents the number of observations in a particular range. Histograms are best used when analyzing continuous data. It’s used the most when you want to understand the frequency distribution of a numerical variable, like height, weight, or age. For example, a histogram can be used to visualize the distribution of heights in a population. Read more about building histograms in Sigma here.
When to Use a Histogram:
- When understanding the shape of a distribution; for example, whether it’s symmetric, skewed to the left or right, or bimodal
- When identifying outliers, like which data points are significantly different from the rest of the data
- When comparing distribution of a variable across different groups, such as males and females, or different age groups.
- To set boundaries for data ranges; for example, you might use a histogram to determine what constitutes a "normal" or "abnormal" value for a particular variable
When to Avoid a Histogram:
- When you need to look at multiple dimensions at the same time
- If your data isn’t all on the same scale
Sankey Charts
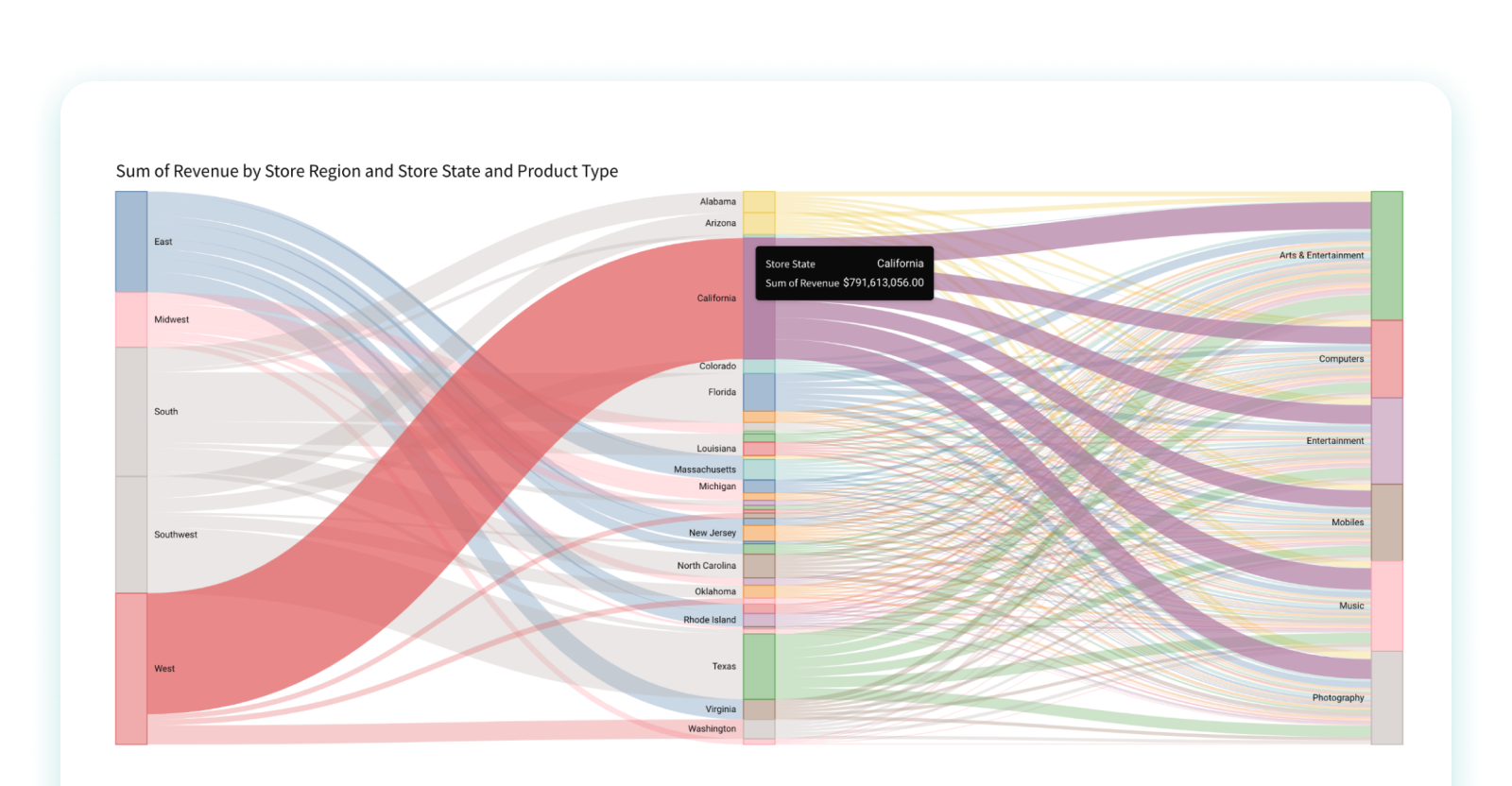
We end our guide with the controversial Sankey chart. A Sankey chart is a type of diagram that illustrates the movement or transfer of data, resources, or quantities through various stages of a system or process. Common applications of Sankey charts include visualizing complex sequences like energy usage, material distribution, or even a website's user journey. The structure of the chart includes nodes and links—with nodes representing the starting points, endpoints, or intermediate steps, and links depicting the transition of quantities or data between these nodes.
The thickness of the links in a Sankey chart directly corresponds to the volume of data or resources being moved, offering an intuitive comparison of the relative sizes of these transfers. They can be invaluable for recognizing inefficiencies, bottlenecks, or potential areas for enhancement in a system or process. These charts serve as a powerful tool for communicating complex information in a straightforward and comprehensible way. However, if there are too many nodes or links, Sankey charts can become cluttered and challenging to interpret, hence their use should be considerate and targeted.
When to use Sankey charts:
- When you want to show the data as part of a process
When to avoid Sankey charts:
- When it starts to feel too confusing, which can quickly happen when there are too many nodes or links
- When you need to see exact values, it might not be the most intuitive option.
Evaluating Data Visualization Tools
Data visualization tools have become increasingly popular in recent years, with a wide variety of options available to choose from. However, determining which tool best suits your needs can be challenging with so many options. When evaluating data visualization tools, there are several key questions to consider:
- What are your goals and needs? It's crucial to clearly understand your goals and needs before selecting a data visualization tool. Are you looking to explore your data, communicate a specific message, or both? Understanding your objectives will help you choose the right tool for your project.
- What features do you require? Different data visualization tools come with different features. Before selecting a tool, you should consider what features you need to achieve your goals. For example, do you require interactive capabilities or the ability to create custom visualizations?
- Where will your data come from? The source of your data is another critical factor to consider when selecting a data visualization tool. Some tools are better suited for specific types of data, such as structured or unstructured data, while others may require specific file formats or data storage solutions.
- Where will you need to see your data? Different data visualization tools may be more suitable for specific platforms or devices. For example, some tools may be optimized for mobile devices, while others are designed for desktop computers or specific web browsers. You may also be interested in embedding visualizations elsewhere , such as internal applications or external portals.
- Where would you like to publish your visualization? Finally, consider where you would like to publish your visualization. Some tools may provide built-in publishing capabilities, while others may require you to export your visualization to a separate platform. Selecting a tool that supports your publishing needs is important to ensure your visualization reaches your intended audience.
By considering these key questions, you can evaluate different data visualization tools and select the one that best meets your needs.
Read a side-by-side comparison of Sigma against similar BI tools.
Take the Next Step & Start Analyzing With Data Visualization
Data visualization is a powerful tool for understanding and communicating complex data. While there are many data visualization tools on the market, Sigma offers an intuitive and familiar spreadsheet interface that allows users to easily explore, analyze, and collaborate on their data.
Explore Sigma’s capabilities and start transforming your data today via a free trial of Sigma .
Related resources
.webp)
This is the default text value
Watch on-DEMAND DEMOS
ATTEND A LIVE LAB
Get a free trial
SEE WORKBOOK EXAMPLES
JOIN THE COMMUNITY
SCHEDULE A CALL
JOIN THE SIGMA COMMUNITY
21 Best Data Visualization Types: Examples of Graphs and Charts Uses
Those who master different data visualization types and techniques (such as graphs, charts, diagrams, and maps) are gaining the most value from data.
Why? Because they can analyze data and make the best-informed decisions.
Whether you work in business, marketing, sales, statistics, or anything else, you need data visualization techniques and skills.
Graphs and charts make data much more understandable for the human brain.
On this page:
- What are data visualization techniques? Definition, benefits, and importance.
- 21 top data visualization types. Examples of graphs and charts with an explanation.
- When to use different data visualization graphs, charts, diagrams, and maps?
- How to create effective data visualization?
- 10 best data visualization tools for creating compelling graphs and charts.
What Are Data V isualization T echniques? Definition And Benefits.
Data visualization techniques are visual elements (like a line graph, bar chart, pie chart, etc.) that are used to represent information and data.
Big data hides a story (like a trend and pattern).
By using different types of graphs and charts, you can easily see and understand trends, outliers, and patterns in data.
They allow you to get the meaning behind figures and numbers and make important decisions or conclusions.
Data visualization techniques can benefit you in several ways to improve decision making.
Key benefits:
- Data is processed faster Visualized data is processed faster than text and table reports. Our brains can easily recognize images and make sense of them.
- Better analysis Help you analyze better reports in sales, marketing, product management, etc. Thus, you can focus on the areas that require attention such as areas for improvement, errors or high-performing spots.
- Faster decision making Businesses who can understand and quickly act on their data will gain more competitive advantages because they can make informed decisions sooner than the competitors.
- You can easily identify relationships, trends, patterns Visuals are especially helpful when you’re trying to find trends, patterns or relationships among hundreds or thousands of variables. Data is presented in ways that are easy to consume while allowing exploration. Therefore, people across all levels in your company can dive deeper into data and use the insights for faster and smarter decisions.
- No need for coding or data science skills There are many advanced tools that allow you to create beautiful charts and graphs without the need for data scientist skills . Thereby, a broad range of business users can create, visually explore, and discover important insights into data.
How Do Data Visualization Techniques work?
Data visualization techniques convert tons of data into meaningful visuals using software tools.
The tools can operate various types of data and present them in visual elements like charts, diagrams, and maps.
They allow you to easily analyze massive amounts of information, discover trends and patterns in data and then make data-driven decisions .
Why data visualization is very important for any job?
Each professional industry benefits from making data easier to understand. Government, marketing, finance, sales, science, consumer goods, education, sports, and so on.
As all types of organizations become more and more data-driven, the ability to work with data isn’t a good plus, it’s essential.
Whether you’re in sales and need to present your products to prospects or a manager trying to optimize employee performance – everything is measurable and needs to be scored against different KPI s.
We need to constantly analyze and share data with our team or customers.
Having data visualization skills will allow you to understand what is happening in your company and to make the right decisions for the good of the organization.
Before start using visuals, you must know…
Data visualization is one of the most important skills for the modern-day worker.
However, it’s not enough to see your data in easily digestible visuals to get real insights and make the right decisions.
- First : to define the information you need to present
- Second: to find the best possible visual to show that information
Don’t start with “I need a bar chart/pie chart/map here. Let’s make one that looks cool” . This is how you can end up with misleading visualizations that, while beautiful, don’t help for smart decision making.
Regardless of the type of data visualization, its purpose is to help you see a pattern or trend in the data being analyzed.
The goal is not to come up with complex descriptions such as: “ A’s sales were more than B by 5.8% in 2018, and despite a sales growth of 30% in 2019, A’s sales became less than B by 6.2% in 2019. ”
A good data visualization summarizes and presents information in a way that enables you to focus on the most important points.
Let’s go through 21 data visualization types with examples, outline their features, and explain how and when to use them for the best results.
21 Best Types Of Data Visualization With Examples And Uses
1. Line Graph
The line graph is the most popular type of graph with many business applications because they show an overall trend clearly and concisely.
What is a line graph?
A line graph (also known as a line chart) is a graph used to visualize the values of something over a specified period of time.
For example, your sales department may plot the change in the number of sales your company has on hand over time.
Data points that display the values are connected by straight lines.
When to use line graphs?
- When you want to display trends.
- When you want to represent trends for different categories over the same period of time and thus to show comparison.
For example, the above line graph shows the total units of a company sales of Product A, Product B, and Product C from 2012 to 2019.
Here, you can see at a glance that the top-performing product over the years is product C, followed by Product B.
2. Bar Chart
At some point or another, you’ve interacted with a bar chart before. Bar charts are very popular data visualization types as they allow you to easily scan them for valuable insights.
And they are great for comparing several different categories of data.
What is a bar chart?
A bar chart (also called bar graph) is a chart that represents data using bars of different heights.
The bars can be two types – vertical or horizontal. It doesn’t matter which type you use.
The bar chart can easily compare the data for each variable at each moment in time.
For example, a bar chart could compare your company’s sales from this year to last year.
When to use a bar chart?
- When you need to compare several different categories.
- When you need to show how large data changes over time.
The above bar graph visualizes revenue by age group for three different product lines – A, B, and C.
You can see more granular differences between revenue for each product within each age group.
As different product lines are groups by age group, you can easily see that the group of 34-45-year-old buyers are the most valuable to your business as they are your biggest customers.
3. Column Chart
If you want to make side-by-side comparisons of different values, the column chart is your answer.
What is a column chart?
A column chart is a type of bar chart that uses vertical bars to show a comparison between categories.
If something can be counted, it can be displayed in a column chart.
Column charts work best for showing the situation at a point in time (for example, the number of products sold on a website).
Their main purpose is to draw attention to total numbers rather than the trend (trends are more suitable for a line chart).
When to use a column chart?
- When you need to show a side-by-side comparison of different values.
- When you want to emphasize the difference between values.
- When you want to highlight the total figures rather than the trends.
For example, the column chart above shows the traffic sources of a website. It illustrates direct traffic vs search traffic vs social media traffic on a series of dates.
The numbers don’t change much from day to day, so a line graph isn’t appropriate as it wouldn’t reveal anything important in terms of trends.
The important information here is the concrete number of visitors coming from different sources to the website each day.
4. Pie Chart
Pie charts are attractive data visualization types. At a high-level, they’re easy to read and used for representing relative sizes.
What is a pie chart?
A Pie Chart is a circular graph that uses “pie slices” to display relative sizes of data.
A pie chart is a perfect choice for visualizing percentages because it shows each element as part of a whole.
The entire pie represents 100 percent of a whole. The pie slices represent portions of the whole.
When to use a pie chart?
- When you want to represent the share each value has of the whole.
- When you want to show how a group is broken down into smaller pieces.
The above pie chart shows which traffic sources bring in the biggest share of total visitors.
You see that Searches is the most effective source, followed by Social Media, and then Links.
At a glance, your marketing team can spot what’s working best, helping them to concentrate their efforts to maximize the number of visitors.
5. Area Chart
If you need to present data that depicts a time-series relationship, an area chart is a great option.
What is an area chart?
An area chart is a type of chart that represents the change in one or more quantities over time. It is similar to a line graph.
In both area charts and line graphs, data points are connected by a line to show the value of a quantity at different times. They are both good for showing trends.
However, the area chart is different from the line graph, because the area between the x-axis and the line is filled in with color. Thus, area charts give a sense of the overall volume.
Area charts emphasize a trend over time. They aren’t so focused on showing exact values.
Also, area charts are perfect for indicating the change among different data groups.
When to use an area chart?
- When you want to use multiple lines to make a comparison between groups (aka series).
- When you want to track not only the whole value but also want to understand the breakdown of that total by groups.
In the area chart above, you can see how much revenue is overlapped by cost.
Moreover, you see at once where the pink sliver of profit is at its thinnest.
Thus, you can spot where cash flow really is tightest, rather than where in the year your company simply has the most cash.
Area charts can help you with things like resource planning, financial management, defining appropriate storage space, and more.
6. Scatter Plot
The scatter plot is also among the popular data visualization types and has other names such as a scatter diagram, scatter graph, and correlation chart.
Scatter plot helps in many areas of today’s world – business, biology, social statistics, data science and etc.
What is a Scatter plot?
Scatter plot is a graph that represents a relationship between two variables . The purpose is to show how much one variable affects another.
Usually, when there is a relationship between 2 variables, the first one is called independent. The second variable is called dependent because its values depend on the first variable.
But it is also possible to have no relationship between 2 variables at all.
When to use a Scatter plot?
- When you need to observe and show relationships between two numeric variables.
- When just want to visualize the correlation between 2 large datasets without regard to time.
The above scatter plot illustrates the relationship between monthly e-commerce sales and online advertising costs of a company.
At a glance, you can see that online advertising costs affect monthly e-commerce sales.
When online advertising costs increase, e-commerce sales also increase.
Scatter plots also show if there are unexpected gaps in the data or if there are any outlier points.
7. Bubble chart
If you want to display 3 related dimensions of data in one elegant visualization, a bubble chart will help you.
What is a bubble chart?
A bubble chart is like an extension of the scatter plot used to display relationships between three variables.
The variables’ values for each point are shown by horizontal position, vertical position, and dot size.
In a bubble chart, we can make three different pairwise comparisons (X vs. Y, Y vs. Z, X vs. Z).
When to use a bubble chart?
- When you want to depict and show relationships between three variables.
The bubble chart above illustrates the relationship between 3 dimensions of data:
- Cost (X-Axis)
- Profit (Y-Axis)
- Probability of Success (%) (Bubble Size).
Bubbles are proportional to the third dimension – the probability of success. The larger the bubble, the greater the probability of success.
It is obvious that Product A has the highest probability of success.
8. Pyramid Graph
Pyramid graphs are very interesting and visually appealing graphs. Moreover, they are one of the most easy-to-read data visualization types and techniques.
What is a pyramid graph?
It is a graph in the shape of a triangle or pyramid. It is best used when you want to show some kind of hierarchy. The pyramid levels display some kind of progressive order, such as:
- More important to least important. For example, CEOs at the top and temporary employees on the bottom level.
- Specific to least specific. For example, expert fields at the top, general fields at the bottom.
- Older to newer.
When to use a pyramid graph?
- When you need to illustrate some kind of hierarchy or progressive order
Image Source: Conceptdraw
The above is a 5 Level Pyramid of information system types that is based on the hierarchy in an organization.
It shows progressive order from tacit knowledge to more basic knowledge. Executive information system at the top and transaction processing system on the bottom level.
The levels are displayed in different colors. It’s very easy to read and understand.
9. Treemaps
Treemaps also show a hierarchical structure like the pyramid graph, however in a completely different way.
What is a treemap?
Treemap is a type of data visualization technique that is used to display a hierarchical structure using nested rectangles.
Data is organized as branches and sub-branches. Treemaps display quantities for each category and sub-category via a rectangle area size.
Treemaps are a compact and space-efficient option for showing hierarchies.
They are also great at comparing the proportions between categories via their area size. Thus, they provide an instant sense of which data categories are the most important overall.
When to use a treemap?
- When you want to illustrate hierarchies and comparative value between categories and subcategories.
Image source: Power BI
For example, let’s say you work in a company that sells clothing categories: Urban, Rural, Youth, and Mix.
The above treemap depicts the sales of different clothing categories, which are then broken down by clothing manufacturers.
You see at a glance that Urban is your most successful clothing category, but that the Quibus is your most valuable clothing manufacturer, across all categories.
10. Funnel chart
Funnel charts are used to illustrate optimizations, specifically to see which stages most impact drop-off.
Illustrating the drop-offs helps to show the importance of each stage.
What is a funnel chart?
A funnel chart is a popular data visualization type that shows the flow of users through a sales or other business process.
It looks like a funnel that starts from a large head and ends in a smaller neck. The number of users at each step of the process is visualized from the funnel width as it narrows.
A funnel chart is very useful for identifying potential problem areas in the sales process.
When to use a funnel chart?
- When you need to represent stages in a sales or other business process and show the amount of revenue for each stage.
Image Source: DevExpress
This funnel chart shows the conversion rate of a website.
The conversion rate shows what percentage of all visitors completed a specific desired action (such as subscription or purchase).
The chart starts with the people that visited the website and goes through every touchpoint until the final desired action – renewal of the subscription.
You can see easily where visitors are dropping out of the process.
11. Venn Diagram
Venn diagrams are great data visualization types for representing relationships between items and highlighting how the items are similar and different.
What is a Venn diagram?
A Venn Diagram is an illustration that shows logical relationships between two or more data groups. Typically, the Venn diagram uses circles (both overlapping and nonoverlapping).
Venn diagrams can clearly show how given items are similar and different.
Venn diagram with 2 and 3 circles are the most common types. Diagrams with a larger number of circles (5,6,7,8,10…) become extremely complicated.
When to use a Venn diagram?
- When you want to compare two or more options and see what they have in common.
- When you need to show how given items are similar or different.
- To display logical relationships from various datasets.
The above Venn chart clearly shows the core customers of a product – the people who like eating fast foods but don’t want to gain weight.
The Venn chart gives you an instant understanding of who you will need to sell.
Then, you can plan how to attract the target segment with advertising and promotions.
12. Decision Tree
As graphical representations of complex or simple problems and questions, decision trees have an important role in business, finance, marketing, and in any other areas.
What is a decision tree?
A decision tree is a diagram that shows possible solutions to a decision.
It displays different outcomes from a set of decisions. The diagram is a widely used decision-making tool for analysis and planning.
The diagram starts with a box (or root), which branches off into several solutions. That’s why it is called a decision tree.
Decision trees are helpful for a variety of reasons. Not only they are easy-to-understand diagrams that support you ‘see’ your thoughts, but also because they provide a framework for estimating all possible alternatives.
When to use a decision tree?
- When you need help in making decisions and want to display several possible solutions.
Imagine you are an IT project manager and you need to decide whether to start a particular project or not.
You need to take into account important possible outcomes and consequences.
The decision tree, in this case, might look like the diagram above.
13. Fishbone Diagram
Fishbone diagram is a key tool for root cause analysis that has important uses in almost any business area.
It is recognized as one of the best graphical methods to understand and solve problems because it takes into consideration all the possible causes.
What is a fishbone diagram?
A fishbone diagram (also known as a cause and effect diagram, Ishikawa diagram or herringbone diagram) is a data visualization technique for categorizing the potential causes of a problem.
The main purpose is to find the root cause.
It combines brainstorming with a kind of mind mapping and makes you think about all potential causes of a given problem, rather than just the one or two.
It also helps you see the relationships between the causes in an easy to understand way.
When to use a fishbone diagram?
- When you want to display all the possible causes of a problem in a simple, easy to read graphical way.
Let’s say you are an online marketing specialist working for a company witch experience low website traffic.
You have the task to find the main reasons. Above is a fishbone diagram example that displays the possible reasons and can help you resolve the situation.
14. Process Flow Diagram
If you need to visualize a specific process, the process flow diagram will help you a lot.
What is the process flow diagram?
As the name suggests, it is a graphical way of describing a process, its elements (steps), and their sequence.
Process flow diagrams show how a large complex process is broken down into smaller steps or tasks and how these go together.
As a data visualization technique, it can help your team see the bigger picture while illustrating the stages of a process.
When to use a process flow diagram?
- When you need to display steps in a process and want to show their sequences clearly.
The above process flow diagram shows clearly the relationship between tasks in a customer ordering process.
The large ordering process is broken down into smaller functions and steps.
15. Spider/Radar Chart
Imagine, you need to rank your favorite beer on 8 aspects (Bitterness, Sweetness, Sourness, Saltiness, Hop, Malt, Yeast, and Special Grain) and then show them graphically. You can use a radar chart.
What is a radar chart?
Radar chart (also called spider, web, and polar bar) is a popular data visualization technique that displays multivariate data.
In can compare several items with many metrics of characteristics.
To be effective and clear, the radar chart should have more than 2 but no more than 6 items that are judged.
When to use a radar chart?
- When you need to compare several items with more than 5 metrics of characteristics.
The above radar chart compares employee’s performance with a scale of 1-5 on skills such as Communications, Problem-solving, Meeting deadlines, Technical knowledge, Teamwork.
A point that is closer to the center on an axis shows a lower value and a worse performance.
It is obvious that Mary has a better performance than Linda.
16. Mind Map
Mind maps are beautiful data visuals that represent complex relationships in a very digestible way.
What is a mind map?
A mind map is a popular diagram that represents ideas and concepts.
It can help you structure your information and analyze, recall, and generate new ideas.
It is called a mind map because it is structured in a way that resembles how the human brain works.
And, best of all, it is a fun and artistic data visualization technique that engages your brain in a much richer way.
When to use a mind map?
- When you want to visualize and connect ideas in an easy to digest way.
- When you want to capture your thoughts/ideas and bring them to life in visual form.
Image source: Lucidchart
The above example of a mind map illustrates the key elements for running a successful digital marketing campaign.
It can help you prepare and organize your marketing efforts more effectively.
17. Gantt Chart
A well-structured Gantt chart aids you to manage your project successfully against time.
What is a Gantt chart?
Gantt charts are data visualization types used to schedule projects by splitting them into tasks and subtasks and putting them on a timeline.
Each task is listed on one side of the chart. This task also has a horizontal line opposite it representing the length of the task.
By displaying tasks with the Gantt chart, you can see how long each task will take and which tasks will overlap.
Gantt charts are super useful for scheduling and planning projects.
They help you estimate how long a project should take and determine the resources needed.
They also help you plan the order in which you’ll complete tasks and manage the dependencies between tasks.
When to use a Gantt chart?
- When you need to plan and track the tasks in project schedules.
Image Source: Aha.io
The above example is a portfolio planning Gantt Chart Template that illustrates very well how Gantt Charts work.
It visualizes the release timeline for multiple products for an entire year.
It shows also dependencies between releases.
You can use it to help team members understand the release schedule for the upcoming year, the duration of each release, and the time for delivering.
This helps you in resource planning and allows teams to coordinate implementation plans.
18. Organizational Charts
Organizational charts are data visualization types widely used for management and planning.
What is an organizational chart?
An organizational chart (also called an org chart) is a diagram that illustrates a relationship hierarchy.
The most common application of an org chart is to display the structure of a business or other organization.
Org charts are very useful for showing work responsibilities and reporting relationships.
They help leaders effectively manage growth or change.
Moreover, they show employees how their work fits into the company’s overall structure.
When to use the org chart?
- When you want to display a hierarchical structure of a department, company or other types of organization.
Image Source: Organimi
The above hierarchical org chart illustrates the chain of command that goes from the top (e.g., the CEOs) down (e.g., entry-level and low-level employees) and each person has a supervisor.
It clearly shows levels of authority and responsibility and who each person reports to.
It also shows employees the career paths and chances for promotion.
19. Area Map
Most business data has a location. Revenue, sales, customers, or population are often displayed with a dimensional variable on a map.
What is an area map?
It is a map that visualizes location data.
They allow you to see immediately which geographical locations are most important to your brand and business.
Image Source: Infogram
The map above depicts sales by location and the color indicates the level of sales (the darker the blue, the higher the sales).
These data visualization types are very useful as they show where in the world most of your sales are from and where your most valuable sales are from.
Insights like these illustrate weaknesses in a sales and marketing strategy in seconds.
20. Infographics
In recent years, the use of infographics has exploded in almost every industry.
From sales and marketing to science and healthcare, infographics are applied everywhere to present information in a visually appealing way.
What is an infographic?
Infographics are specific data visualization types that combine images, charts, graphs, and text. The purpose is to represent an easy-to-understand overview of a topic.
However, the main goal of an infographic is not only to provide information but also to make the viewing experience fun and engaging for readers.
It makes data beautiful—and easy to digest.
When you want to represent and share information, there are many data visualization types to do that – spreadsheets, graphs, charts, emails, etc.
But when you need to show data in a visually impactful way, the infographic is the most effective choice.
When to use infographics?
- When you need to present complex data in a concise, highly visually-pleasing way.
Image Source: Venngage
The above statistical infographic represents an overview of Social Buzz’s biggest social platforms by age and geography.
For example, we see that 75% of active Facebook users are 18-29 years old and 48% of active users live in North America.
21. T-Chart
If you want to compare and contrast items in a table form, T-Chart can be your solution.
What is a T-Chart?
A T-Chart is a type of graphic organizer in the shape of the English letter “T”. It is used for comparison by separating information into two or more columns.
You can use T-Chart to compare ideas, concepts or solutions clearly and effectively.
T-Charts are often used for comparison of pros and cons, facts and opinions.
By using T-Chart, you can list points side by side, achieve a quick, at-a-glance overview of the facts, and arrive at conclusions quickly and easily.
When to use a T-Chart?
- When you need to compare and contrast two or more items.
- When you want to evaluate the pros and cons of a decision.
The above T-Chart example clearly outlines the cons and pros of hiring a social media manager in a company.
10 Best Data Visualization Tools
There is a broad range of data visualization tools that allow you to make fascinating graphs, charts, diagrams, maps, and dashboards in no time.
They vary from BI (Business Intelligence) tools with robust features and comprehensive dashboards to more simple software for just creating graphs and charts.
Here we’ve collected some of the most popular solutions. They can help you present your data in a way that facilitates understanding and decision making.
1. Visme is a data presentation and visualization tool that allows you to create stunning data reports. It provides a great variety of presentation tools and templates for a unique design.
2. Infogram is a chart software tool that provides robust diagram-making capabilities. It comes with an intuitive drag-and-drop editor and ready-made templates for reports. You can also add images for your reports, icons, GIFs, photos, etc.
3. Venngage is an infographic maker. But it also is a great chart software for small businesses because of its ease of use, intuitive design, and great templates.
4. SmartDraw is best for those that have someone graphic design skills. It has a slightly more advanced design and complexity than Venngage, Visme, and Infogram, … so having some design skills is an advantage. It’s a drawing tool with a wide range of charts, diagrams, maps, and well-designed templates.
5. Creately is a dynamic diagramming tool that offers the best free version. It can be deployed from the cloud or on the desktop and allows you to create your graphs, charts, diagrams, and maps without any tech skills.
6. Edraw Max is an all-in-one diagramming software tool that allows you to create different data visualization types at a high speed. These include process flow charts, line graphs, org charts, mind maps, infographics, floor plans, network diagrams, and many others. Edraw Max has a wide selection of templates and symbols, letting you to rapidly produce the visuals you need for any purpose.
7. Chartio is an efficient business intelligence tool that can help you make sense of your company data. Chartio is simple to use and allows you to explore all sorts of information in real-time.
8. Sisense – a business intelligence platform with a full range of data visualizations. You can create dashboards and graphical representations with a drag and drop user interface.
9. Tableau – a business intelligence system that lets you quickly create, connect, visualize, and share data seamlessly.
10. Domo is a cloud business intelligence platform that helps you examine data using graphs and charts. You can conduct advanced analysis and create great interactive visualization.
Data visualization techniques are vital components of data analysis, as they can summarize large amounts of data effectively in an easy to understand graphical form.
There are countless data visualization types, each with different pros, cons, and use cases.
The trickiest part is to choose the right visual to represent your data.
Your choice depends on several factors – the kind of conclusion you want to draw, your audience, the key metrics, etc.
I hope the above article helps you understand better the basic graphs and their uses.
When you create your graph or diagram, always remember this:
A good graph is the one reduced to its simplest and most elegant form without sacrificing what matters most – the purpose of the visual.
About The Author

Silvia Valcheva
Silvia Valcheva is a digital marketer with over a decade of experience creating content for the tech industry. She has a strong passion for writing about emerging software and technologies such as big data, AI (Artificial Intelligence), IoT (Internet of Things), process automation, etc.
Leave a Reply Cancel Reply
This site uses Akismet to reduce spam. Learn how your comment data is processed .

- SUGGESTED TOPICS
- The Magazine
- Newsletters
- Managing Yourself
- Managing Teams
- Work-life Balance
- The Big Idea
- Data & Visuals
- Reading Lists
- Case Selections
- HBR Learning
- Topic Feeds
- Account Settings
- Email Preferences
Visualizations That Really Work
- Scott Berinato

Not long ago, the ability to create smart data visualizations (or dataviz) was a nice-to-have skill for design- and data-minded managers. But now it’s a must-have skill for all managers, because it’s often the only way to make sense of the work they do. Decision making increasingly relies on data, which arrives with such overwhelming velocity, and in such volume, that some level of abstraction is crucial. Thanks to the internet and a growing number of affordable tools, visualization is accessible for everyone—but that convenience can lead to charts that are merely adequate or even ineffective.
By answering just two questions, Berinato writes, you can set yourself up to succeed: Is the information conceptual or data-driven? and Am I declaring something or exploring something? He leads readers through a simple process of identifying which of the four types of visualization they might use to achieve their goals most effectively: idea illustration, idea generation, visual discovery, or everyday dataviz.
This article is adapted from the author’s just-published book, Good Charts: The HBR Guide to Making Smarter, More Persuasive Data Visualizations.
Know what message you’re trying to communicate before you get down in the weeds.
Idea in Brief
Knowledge workers need greater visual literacy than they used to, because so much data—and so many ideas—are now presented graphically. But few of us have been taught data-visualization skills.
Tools Are Fine…
Inexpensive tools allow anyone to perform simple tasks such as importing spreadsheet data into a bar chart. But that means it’s easy to create terrible charts. Visualization can be so much more: It’s an agile, powerful way to explore ideas and communicate information.
…But Strategy Is Key
Don’t jump straight to execution. Instead, first think about what you’re representing—ideas or data? Then consider your purpose: Do you want to inform, persuade, or explore? The answers will suggest what tools and resources you need.
Not long ago, the ability to create smart data visualizations, or dataviz, was a nice-to-have skill. For the most part, it benefited design- and data-minded managers who made a deliberate decision to invest in acquiring it. That’s changed. Now visual communication is a must-have skill for all managers, because more and more often, it’s the only way to make sense of the work they do.
- Scott Berinato is a senior editor at Harvard Business Review and the author of Good Charts Workbook: Tips Tools, and Exercises for Making Better Data Visualizations and Good Charts: The HBR Guide to Making Smarter, More Persuasive Data Visualizations .

Partner Center
- Data Visualizations
- Most Recent
- Presentations
- Infographics
- Forms and Surveys
- Video & Animation
- Case Studies
- Design for Business
- Digital Marketing
- Design Inspiration
- Visual Thinking
- Product Updates
- Visme Webinars
- Artificial Intelligence
The 30 Best Data Visualizations of 2024 [Examples]
![visual representation in data The 30 Best Data Visualizations of 2024 [Examples]](https://visme.co/blog/wp-content/uploads/2021/08/Data-Visualization-Header.jpg)
Written by: Anna Glivinska
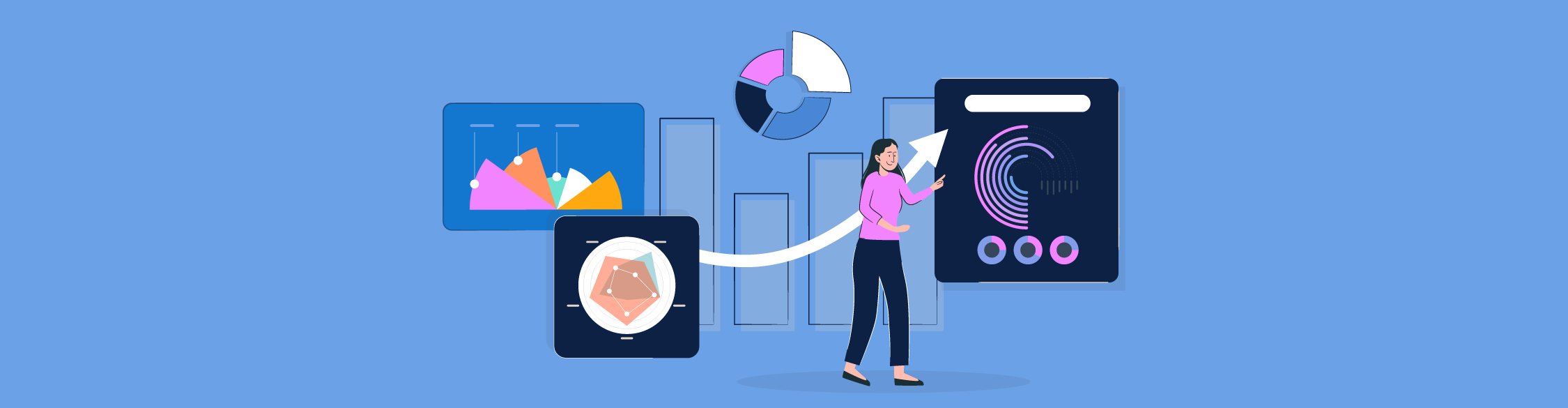
Data is beautiful; it can inspire, improve lives and bring out the best in people. To keep you inspired, we’ve gathered the best data visualizations of 2024.
The chosen works cover a variety of topics from NASA asteroids in space to environmental issue statistics and futuristic LIDAR data graphs.
With over 4.54 billion people using the Internet in 2020, we’re sure to witness even more amazing data visualizations every year. For now, get ready to dive into 2024’s best data visualization examples. Enjoy your flight of imagination!
- NASA's Eyes on Asteroids is a good data visualization example that provides a great user experience. The design is simple and intuitive, making it easy for users to navigate the site and find what they're looking for.
- The History of Pandemics is an infographic that presents a visual timeline of every known pandemic and includes information on how many people were affected, where it spread and what caused it.
- Void of the Memories is the rarest data visualization on this list. It's a great combination of calligraphy and data visualization that tells the story of human memory and experience.
- The search for dark matter is one of the most important scientific questions in physics today, and this infographic, “The Search for Dark Matter,” serves as a great introduction to the subject.
- Enhance your data storytelling skills and creatively showcase your data by signing up for Visme's data visualization tools .
1 Nasa’s Eyes on Asteroids
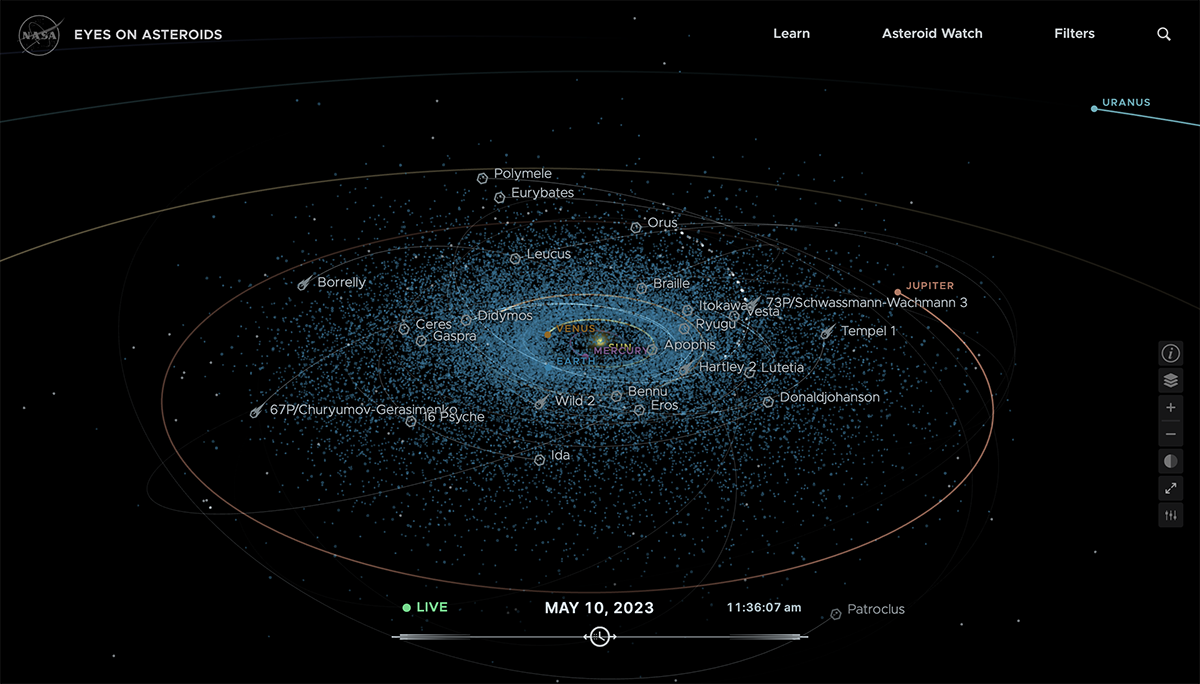
Image Source
If you are interested in exploring data visualization topics in space exploration, check out this striking data visualization created by NASA.
NASA's Eyes on Asteroids is one of the best data visualizations due to its exceptional design and functionality. This interactive visualization allows users to explore the asteroid belt and see the real-time positions of asteroids in our solar system.
The design of this visualization is highly engaging and visually stunning, with a sleek and modern interface that is easy to use. The visualization features a 3D solar system model, allowing users to zoom in and out to explore asteroids and other celestial bodies.
One of the key features of NASA's Eyes on Asteroids visualization is its real-time data feed, which provides up-to-date information on the positions and trajectories of asteroids. This feature makes the visualization highly informative and relevant to current events, allowing users to track potentially hazardous asteroids and see their projected paths over time.
Design your own space exploration infographic using Visme. Allowing you to create data visualizations easier and faster.
Get inspired by one of our loyal Visme users, MacKenzie Stonis , Economic Research Analyst at Greater Memphis Chamber, who said:
"I have enough complications in life; I don’t need my report-building tool to add any fuel to the fire,” she laughs. “I personally had experience with similar applications before Visme and found their tools weren’t as user-friendly as Visme, and their tools didn’t handle data very well. They didn’t provide the solution I really wanted."
2 Selfiecity – The Science of Selfies
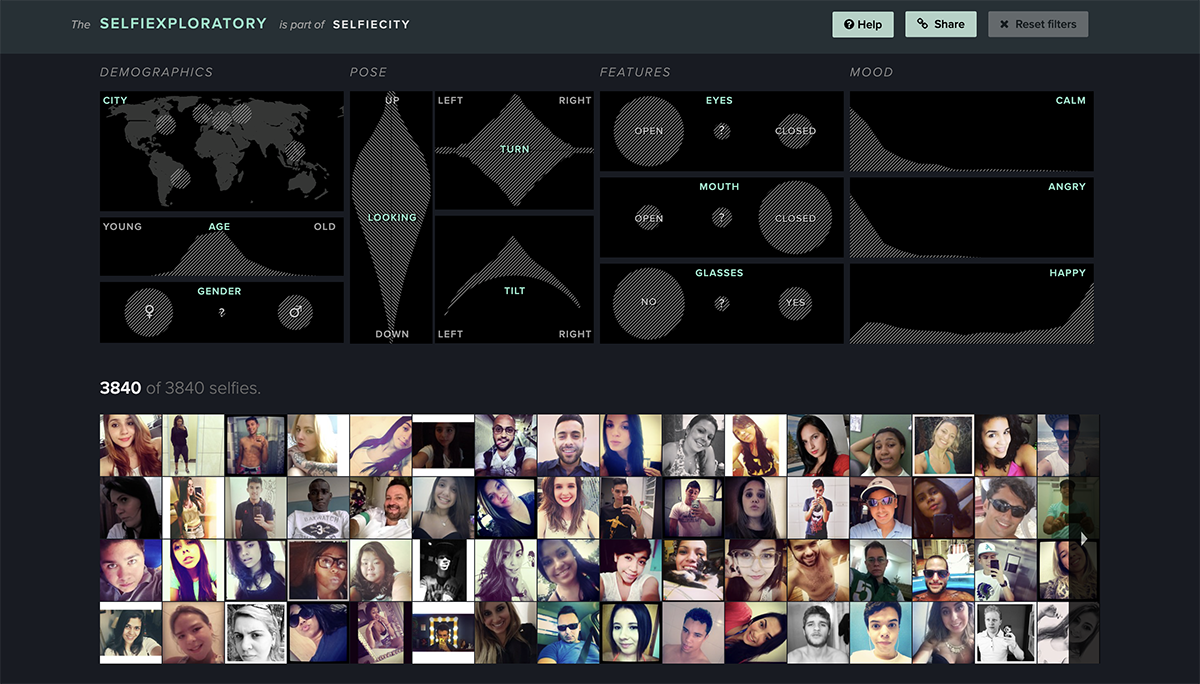
Selfiecity is an innovative and engaging data visualization project exploring the selfies world. It uses a variety of visualizations to analyze selfies from five cities around the world.
They collected over 120,000 selfies from the five cities and selected nearly 1,000 photos from each town. After collecting the images, they analyzed various metrics such as demographics, poses, moods and features.
The project then revealed exciting insights into the culture and social behavior of the people who take selfies. For example, the project shows that women take more selfies than men and that people tend to take selfies in public places rather than private spaces.
The study was quite complex and yielded valuable insights, which presented a challenge when it came to sharing the results . However, the team did an excellent job creating visually appealing data visualizations to present the information.
3 The Ancient Seven Wonders of the World
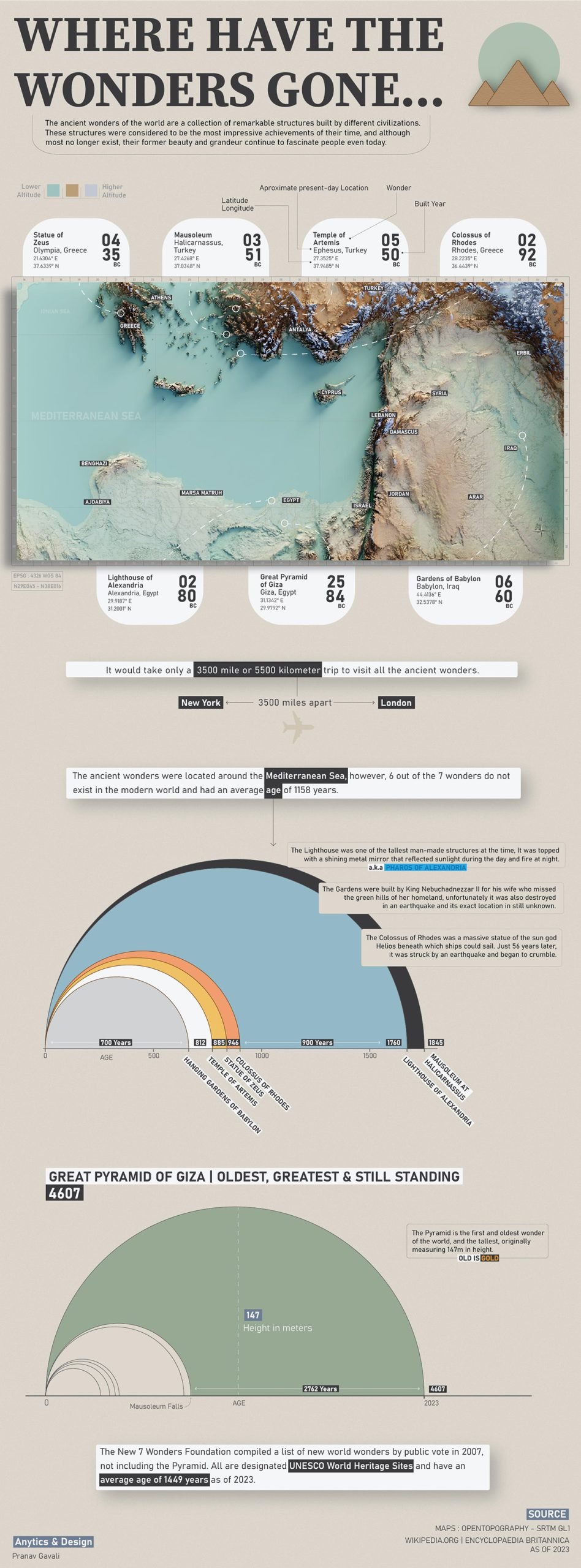
The civil engineering feats of humankind have reached the highest peaks of the mountains and deep into the ocean, and we have built pyramids, temples and statues that are still standing today.
The seven wonders of the ancient world are a collection of man-made structures that are considered to be remarkable feats at the time they were built.
Pranav Gavali, a Data Scientist, created this graphic using data from Encyclopedia Britannica and Wikipedia to visualize the world's seven ancient wonders along with their features and modern-day locations.
The graphic perfectly illustrates how the seven wonders were built and why they are considered a wonder of the world. The Great Pyramid of Giza is the only one of the seven wonders that still stands today.
Design an infographic like this one using Visme’s pre-designed content blocks and infographic templates . Include live data visualizations by connecting to your Google or Excel spreadsheets. When connecting your Visme charts to Excel Online, select full sheets or only a specific range. Plus, when values change in your linked sheet, the chart is This is a prime example of how creative design can bring data to life
4 The World’s Population at 8 Billion
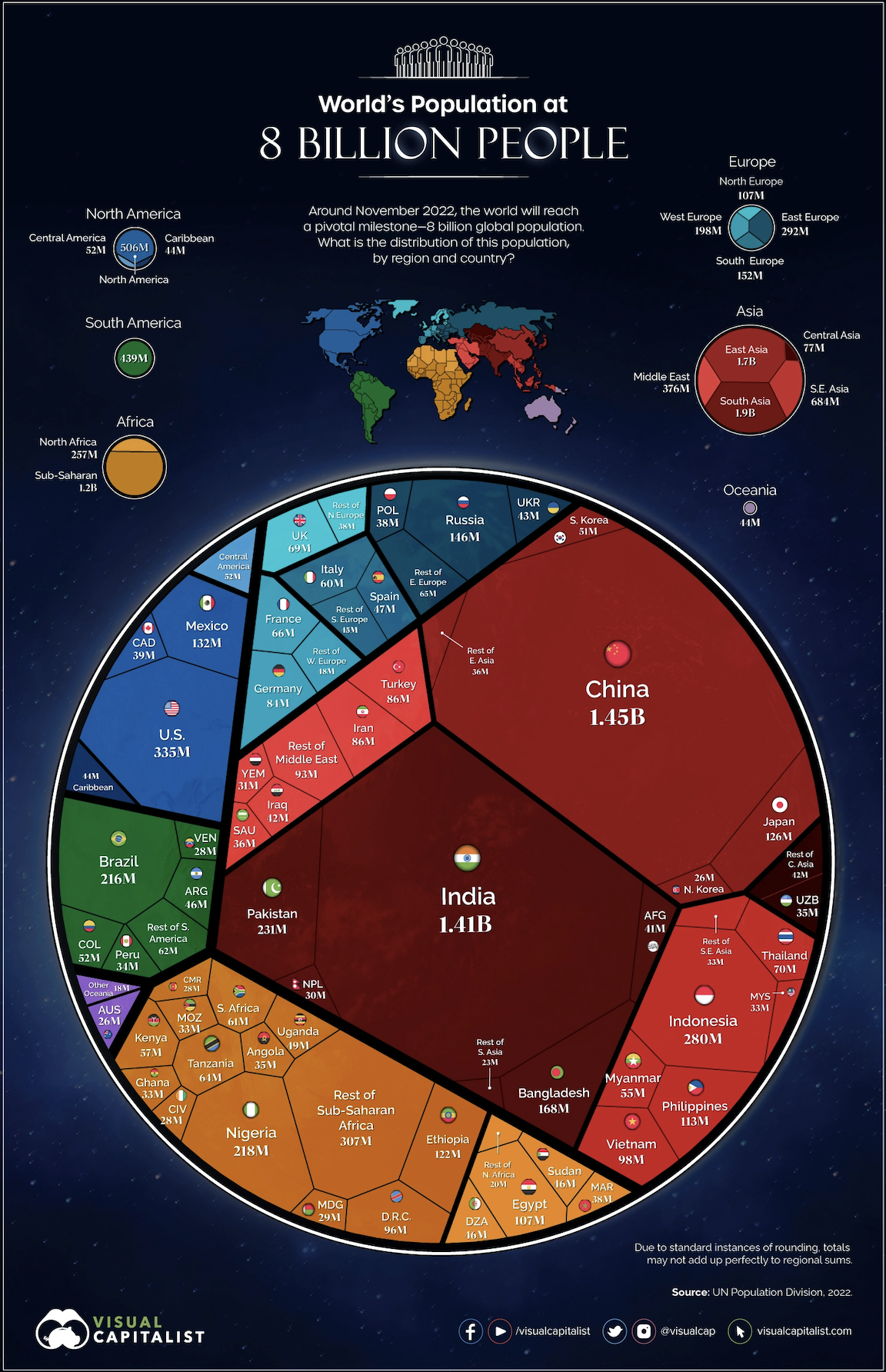
On November 15, 2022, the world’s population reached 8 billion. This is the first time in history that there have been this many people on Earth. And there can't be a more straightforward and visually appealing way to present this data than this visualization.
What makes this big data visualization stand out is its simplicity and effectiveness in conveying the message. Using a circle to represent the earth is a powerful symbol that makes the visualization easy to understand and remember.
By using colors to represent continents and lines to separate countries, the visualization effectively conveys the complexity of the world's population in a simple and visually appealing way.
5 The Top 10 Largest Nuclear Explosions
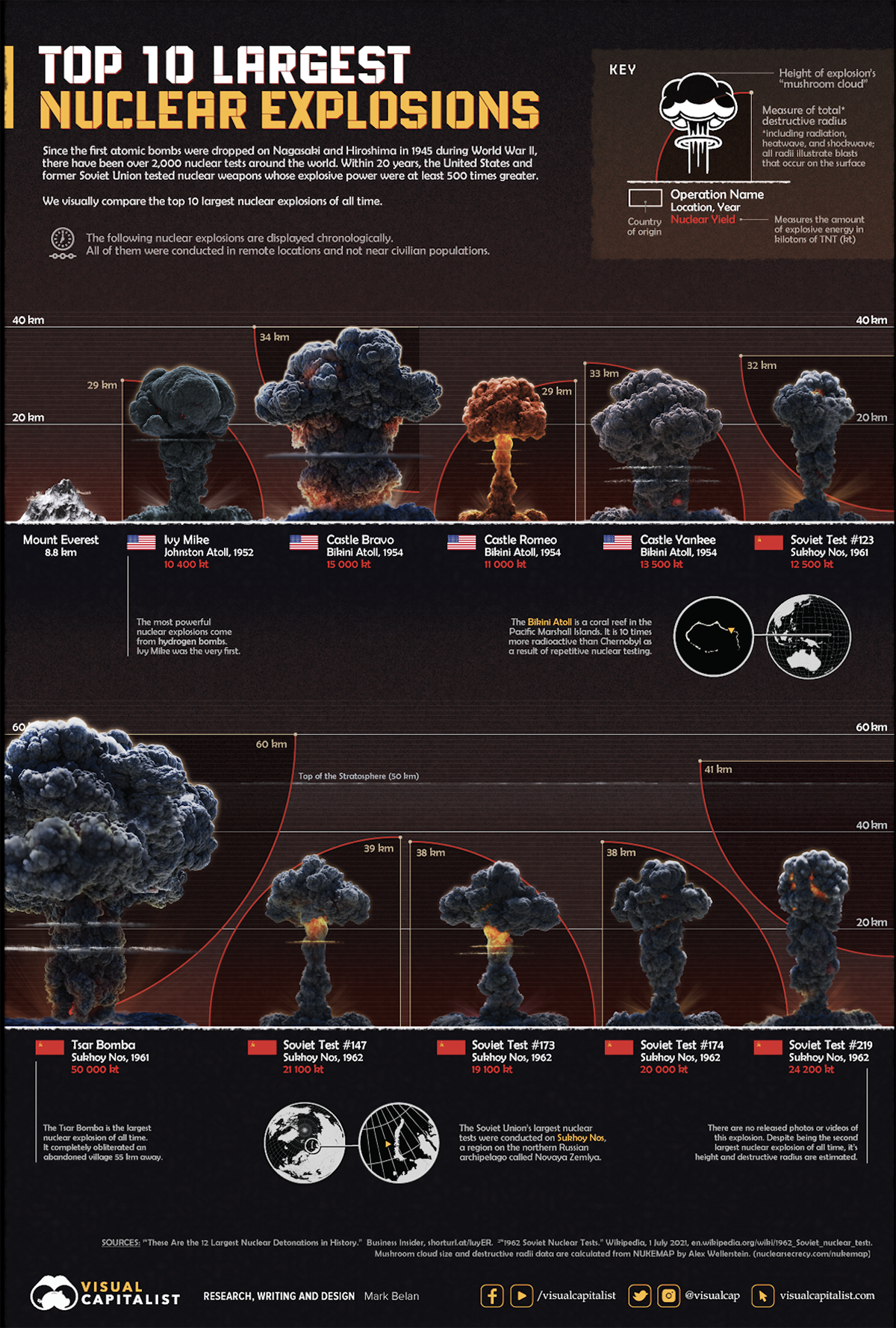
This is a prime example of how creative design can bring data to life. Beyond the interesting data visualization, it uses a unique approach, similar to an infographic, to showcase the impact and size of the largest nuclear explosions ever detonated.
It features a series of explosion image examples that help visualize each explosion's scale and impact. The use of images effectively conveys the destructive power of each blast in a way that is easy to understand and remember.
The data is presented clearly and concisely, with each explosion listed along with its country of origin.
6 Visualizing the History of Pandemics
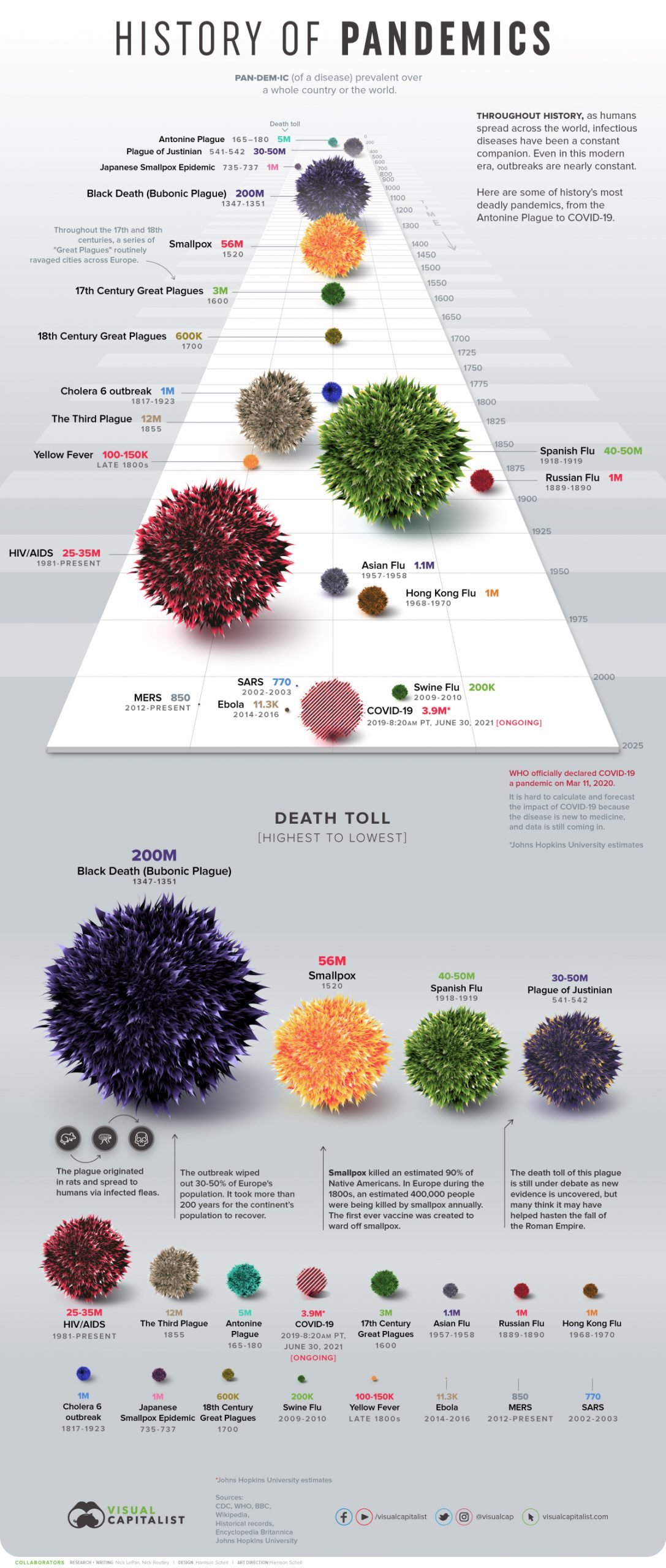
This is an informative graphic named Visualizing the History of Pandemics by Nicholas LePan. It tells the story of all the known pandemics in the history of mankind, including the name of the disease, death toll and the approximate date the pandemic occurred.
While the exact number of victims of every disease is still under question, we can still learn from this graphic that super-spreading infections happened across all history of mankind. Statistical data of this infographic shows some diseases scaling with the growth of the population.
Striking 3D illustrations of diseases are combined with the research data from CDC, WHO, BBC, Wikipedia, Historical records, Encyclopedia Britannica and John Hopkins University. The illustrations scale according to the recorded death toll to allow scanning and recognizing data easily.
7 It Fell From the Sky
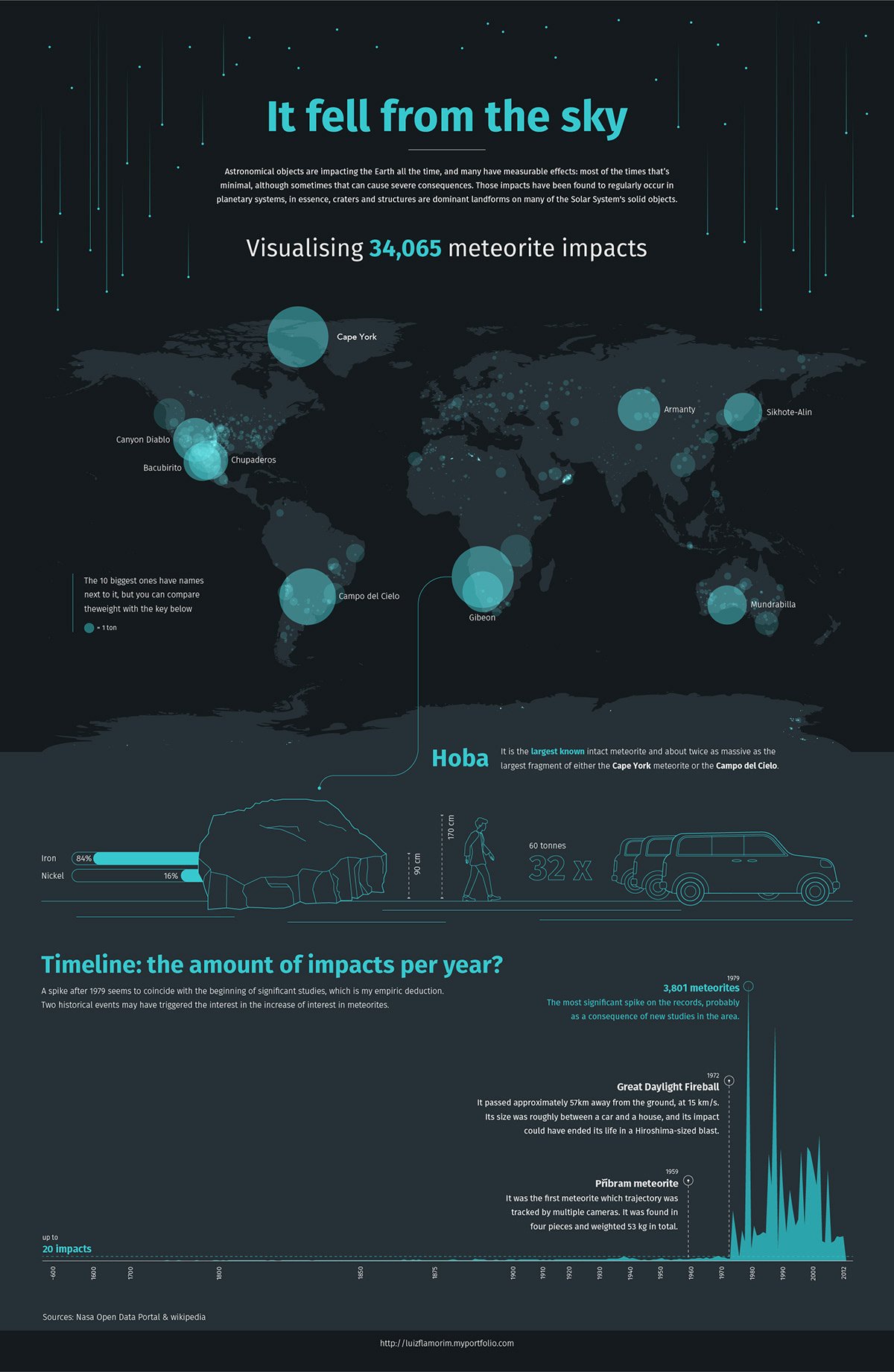
Created by a UK-based designer, this infographic highlights beautiful data visualization of 34,000 meteorites that have fallen on the Earth. You will discover the map and timeline of the impacts per year, wrapped up in clean, stylish graphics. The visualization also shows spikes on the records and comparing the size of the biggest meteorites recorded.
Meteorites hit almost all of Earth’s surface, but some areas seem untouched; this phenomenon could be connected with Earth’s magnetic fields. And who knows – the future may bring us even more meteorites to explore!
If you’re a fan of space and astronomy, you can learn more about meteorites from NASA website or check out this database of the Meteoritical Society.
Try Visme, our all-in-one design for creating stunning visualizations on meteorites in space or other research topics you’re working on.
Get the most out of Visme’s seamless integration with Google Sheets to create visualizations of live, easy-to-update data.
Link to your Google Sheets account or import through a link. Select the page and data range and connect them to your Visme chart. When the data changes in the Google Sheet, it automatically applies to the live project. Simply press the refresh button.
Sign up to Visme for free.
8 Mars Mission 2024 Promo Reel
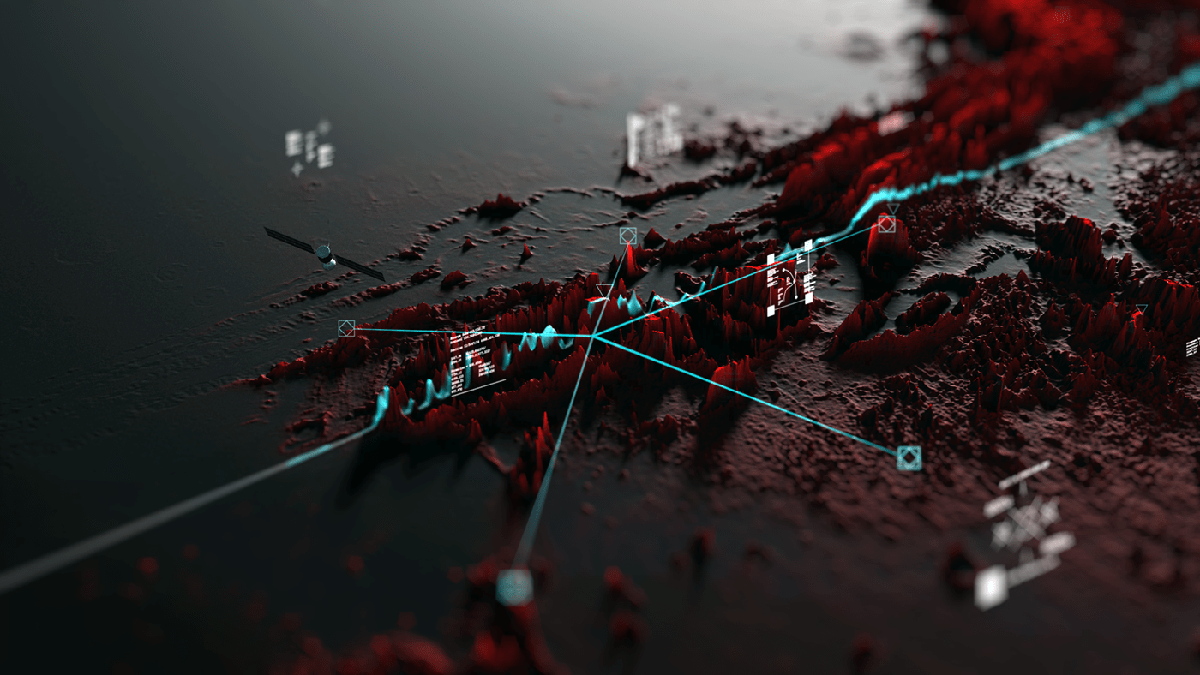
Vivid, rich in details. This 3D graphic uses beautiful data visualizations to share the vision of the future. Space missions and sending people into space are shown in an eye-catching red-grey palette.
The complicated animation of terrain exploration, space module flight and surface graphics are breathtaking. For a moment, you feel like a Mars mission crew member with your eyes on the stars.
9 Void of the Memories
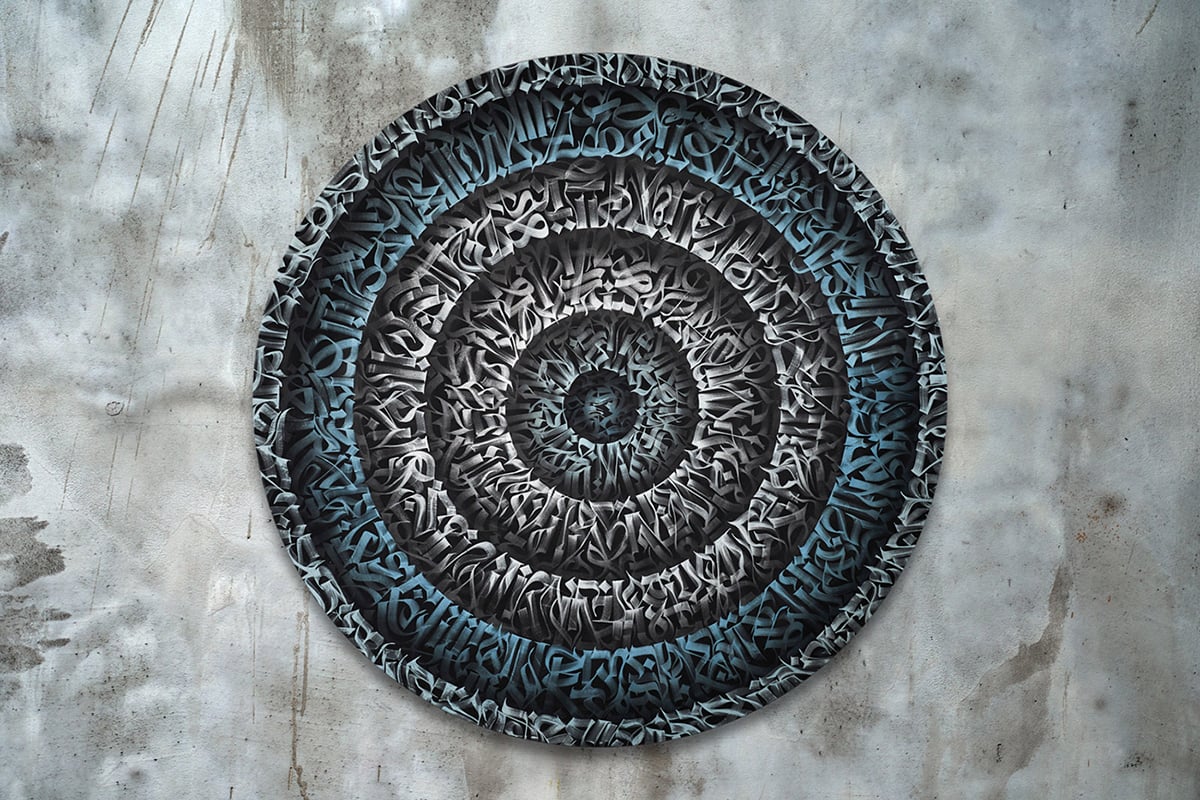
These mesmerizing circles were brought to you by one of the best-in-class street art and calligraphy authors, Pokras Lampas. Whether you would like to decipher this canvas or refer to it as a pure visual object, the unique gothic Calligrafuturism style is an eye magnet for anyone.
The project is focused on the human consciousness and the theme of dreams in the context of human memory and experience. According to the author, the future is for global unity and harmony of cultures – and it’s visible in the fusion of styles, techniques and systems used in the project graphics.
10 Plastic Waste Pollution
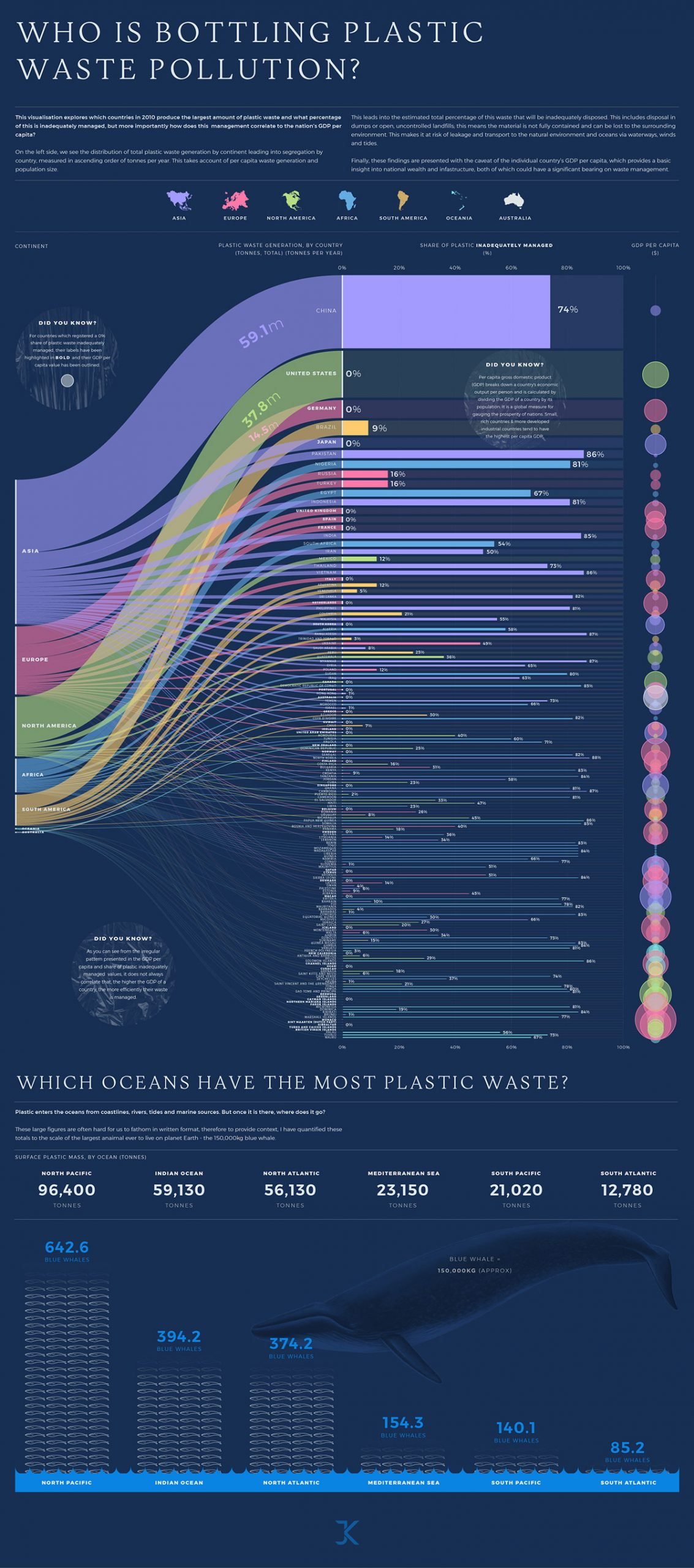
Based on data on the distribution of total plastic waste generation by continent, Jamie Kettle created this personal project to estimate the percentage of plastic waste that was inadequately disposed of.
The infographic provides a clear and precise picture of current surface plastic mass by ocean, measuring it in a creative way. We can see plastic waste management for every country in a colored bar chart. The names of the countries that report 100% of all their plastic waste handled properly are highlighted in bold.
One of the major findings here is that the country's GDP and efficient plastic waste management aren’t always correlated—you can see this by the irregular patterns shown in the infographic.
If you are curious about plastic waste, here are some resources for you: a guide on plastic waste, detailed info on plastic waste pollution from the UN Environment Program and Impacts of Mismanaged Trash by the United States Environmental Protection Agency.
If you’re working on a research topic like waste management, use Visme’s charts and graphs templates to highlight your findings and statistical analysis. Incorporate vertical bar graphs and align the values to the left, right or center to match your overall design.
11 Fossil Fuels
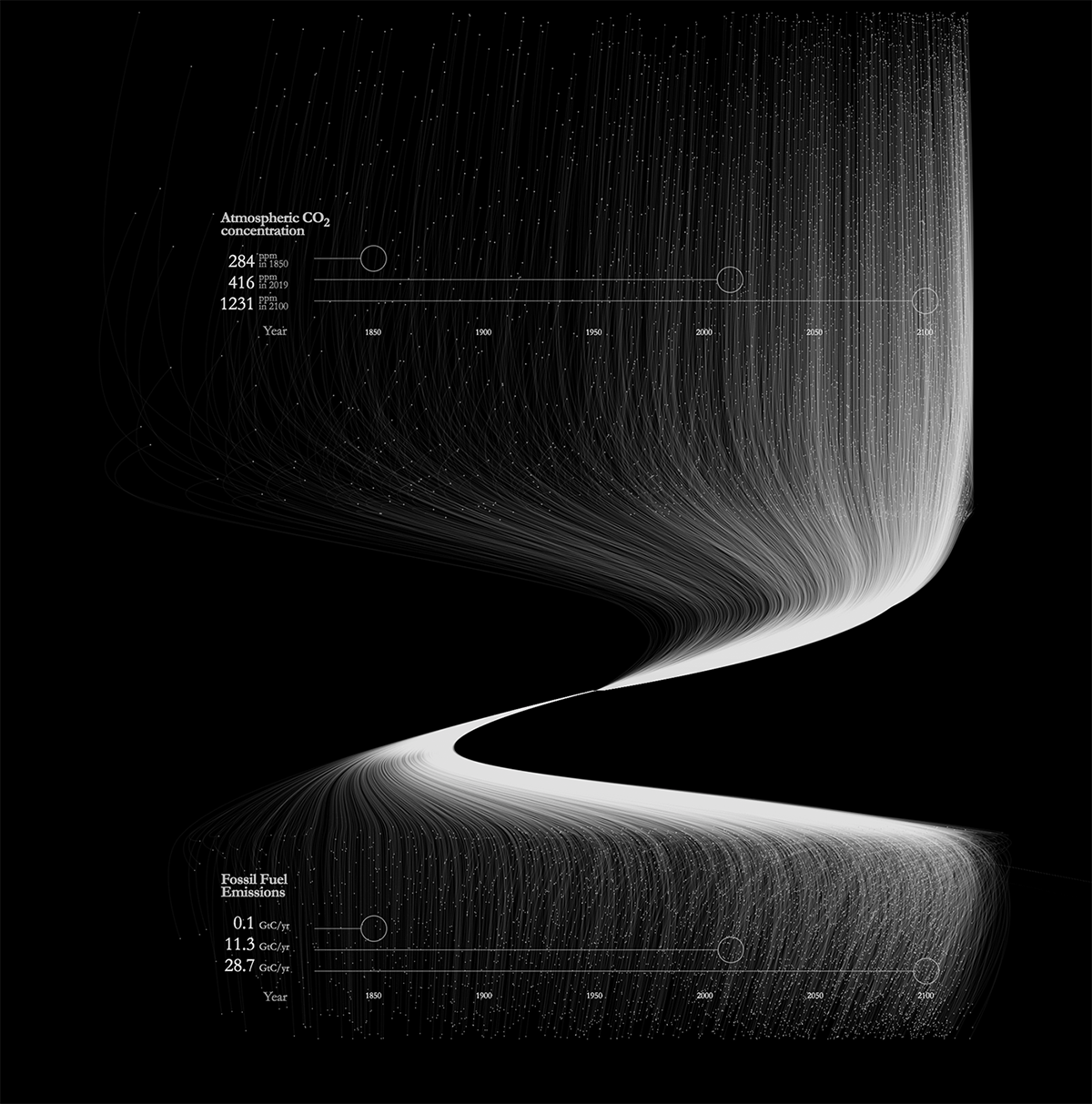
This profound and complex visualization tells us about one of the most pressing environmental issues – the increasing amount of carbon dioxide in the Earth's atmosphere.
While CO 2 buildup is responsible for climate change, the trend is projected to continue, and the infographic provides insight into when this could happen. It’s easy to notice a steady increase in fossil fuel emissions since the Industrial Revolution and the projected sharp rise in the concentration of carbon dioxide until 2100.
Find more data on CO 2 emissions in the Our World in Data research, EPA website and Worldometer stats.
12 Price of a Pandemic: Poverty Spreads Around the Globe
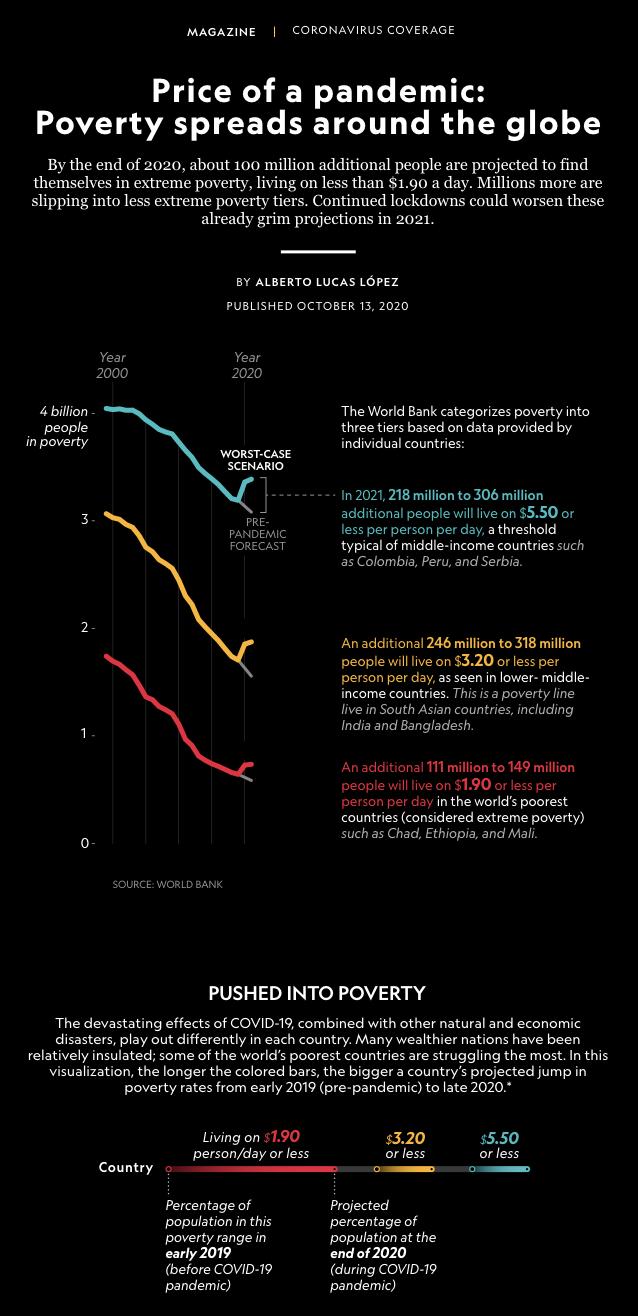
In this classic data visualization by National Geographic, data is placed against the dark background for better contrast and readability. Simple, comprehensive charts show us the effect of the pandemic on the income of people in various countries.
The authors distributed three levels of income range for countries with low and middle class income to provide a clear picture of the current situation. Core findings of the report were that the pandemic pushed a tremendous amount of people to extreme poverty – projected data is 100 million of people living on $1.90 per person/day.
Based on the World Bank data, the infographic provides a wide view of the exact factors influencing people’s wellbeing – from travel restrictions and job loss to wars, displacements and higher food costs. Highlights at the beginning reveal rapid shrinking of income in examined countries across all continents on a mass scale.
13 Water Consumption
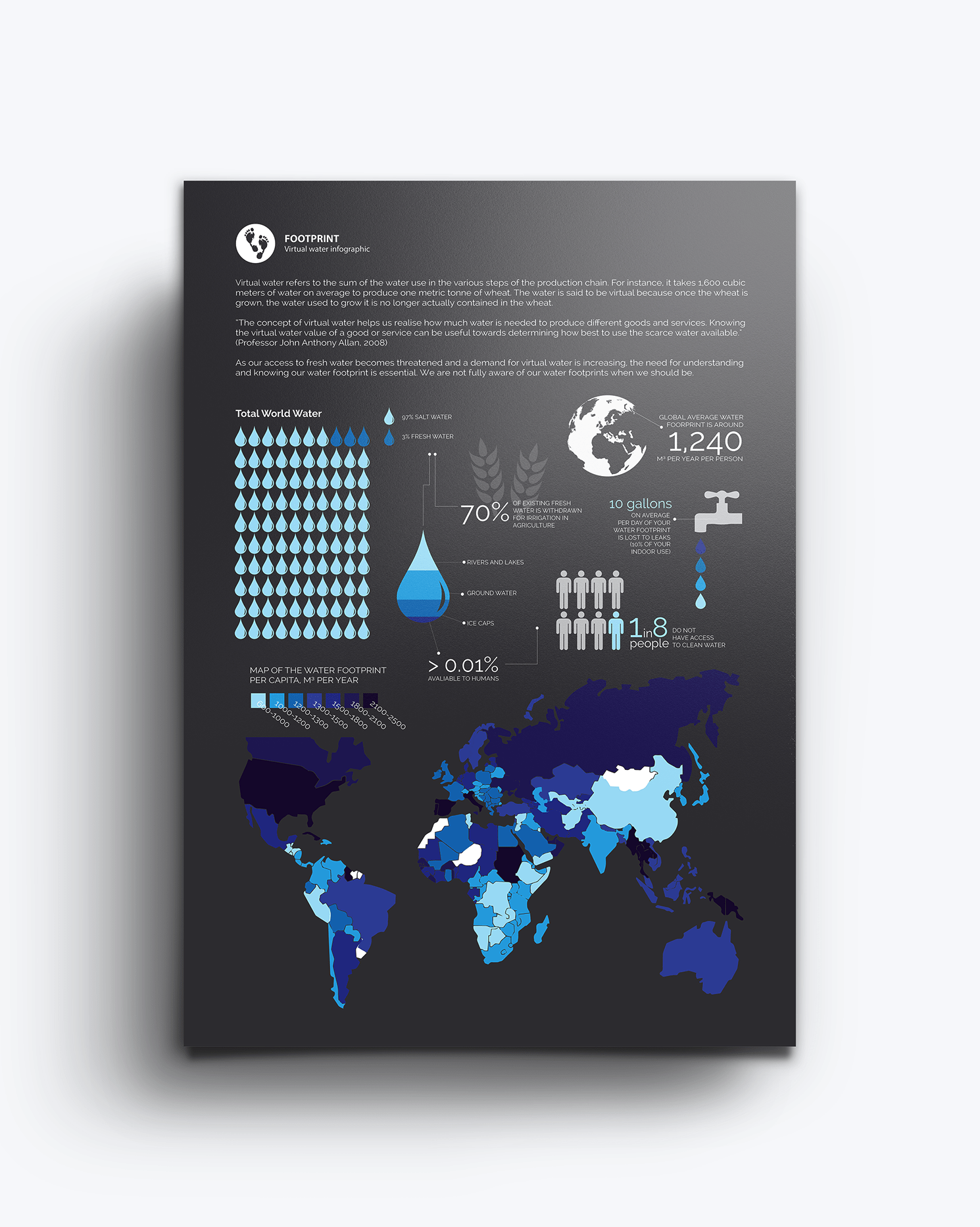
Hidden food production costs involve a great amount of freshwater. This stunning example of visualization created by Chesca Kirkland unfolds a story of water consumption required to produce certain kinds of food.
From chocolate to cheese, coffee and beer, every product requires a certain amount of freshwater to grow or be produced. The second part of the infographic is centered on the water resources available, including the map of the water footprint per capita per year and general availability of clean water to people.
Nominated for two C-Change Environmental and Sustainability Awards, the project won First Class Honours in Final Design Futures. Raising awareness about water sustainability is vital as we move forward to a more intelligent, AI-driven future.
We at Visme are inviting you to take up the challenge and create informative infographics that can invite change to various industry branches. Use our amazing free infographic library to create graphics for your personal projects as well as corporate or brand presentations.
For more detailed info on the infographic creation, watch this video on the 13 major types of infographics .

14 Icebergs and Climate Change
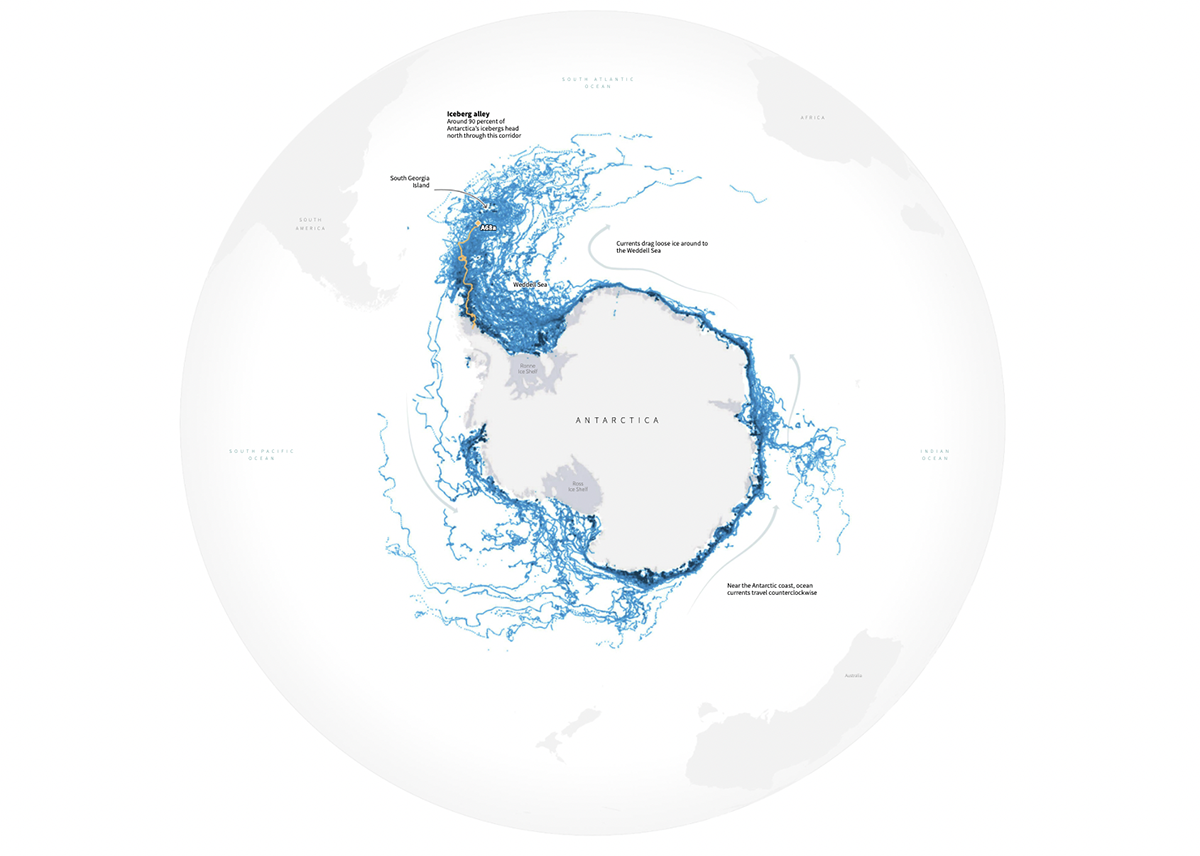
Dedicated to “travel adventures” of this 4,200-square-kilometer iceberg, this infographic alerts people to climate change. A giant chunk of ice the length of Puerto Rico broke off the Antarctic peninsula coast to wander into the wild – and dangerously close to South Georgia Island, packed with wildlife.
The graphic compares the size of the berg with 66 countries or territories and cites that the ice mass is so large that it cannot be captured in one photograph. Besides, we can also see impressive geodata on the wildlife from the IUCN Red List of Threatened Species inhabiting the endangered South Georgia Island.
15 Cell Towers Map of the World
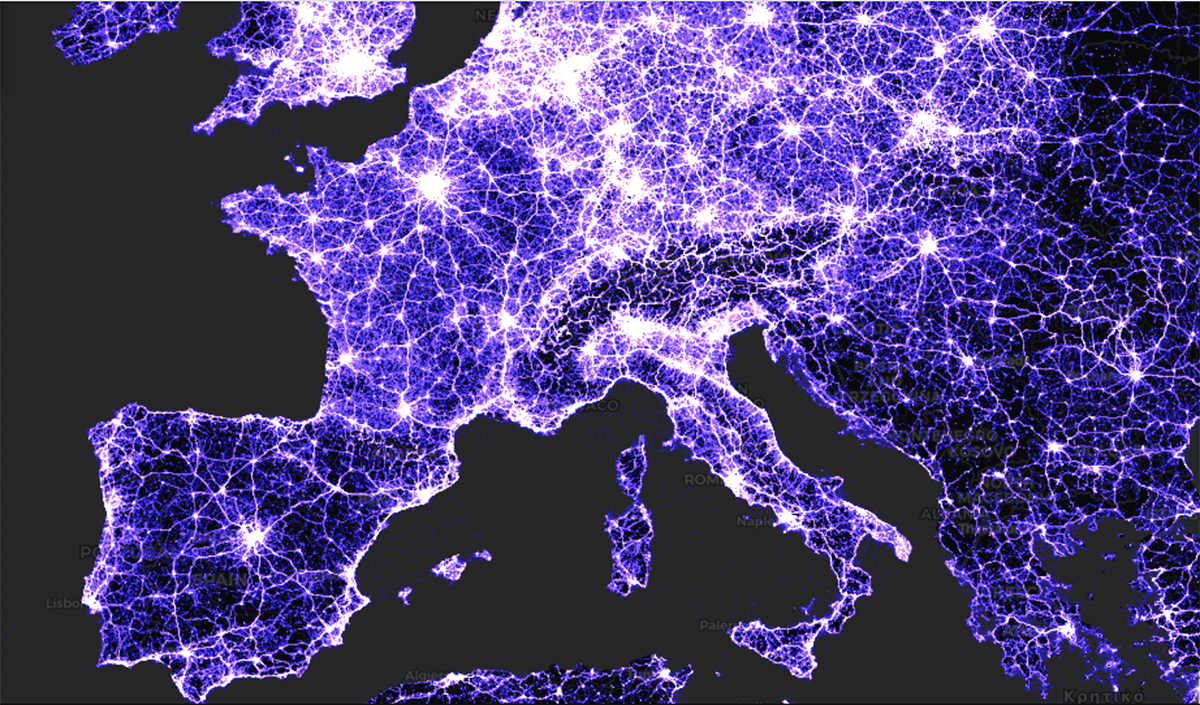
This stunning, elegant and creative visualization of 40 million cell towers is surely an unforgettable view. Based on OpenCelliD, the world's largest open database of cell towers, this interactive map is so far one of the most precise publicly available data sources for telecom-related projects.
We can see how the cell tower network lights up Europe and other big cities of the world; simultaneously, vast areas of “wilderness” are still present on the map. Harsh climate and low population density in the northern regions of Russia and Canada, along with central areas of Africa and Mongolia result in low quantity of cell towers in these areas.
Closeup view of this cell tower map resembles the brain structure. Similar to the neurons, axons and dendrites that create the communication network of the human body – cell towers keep humanity connected.
16 Active Satellites in Space
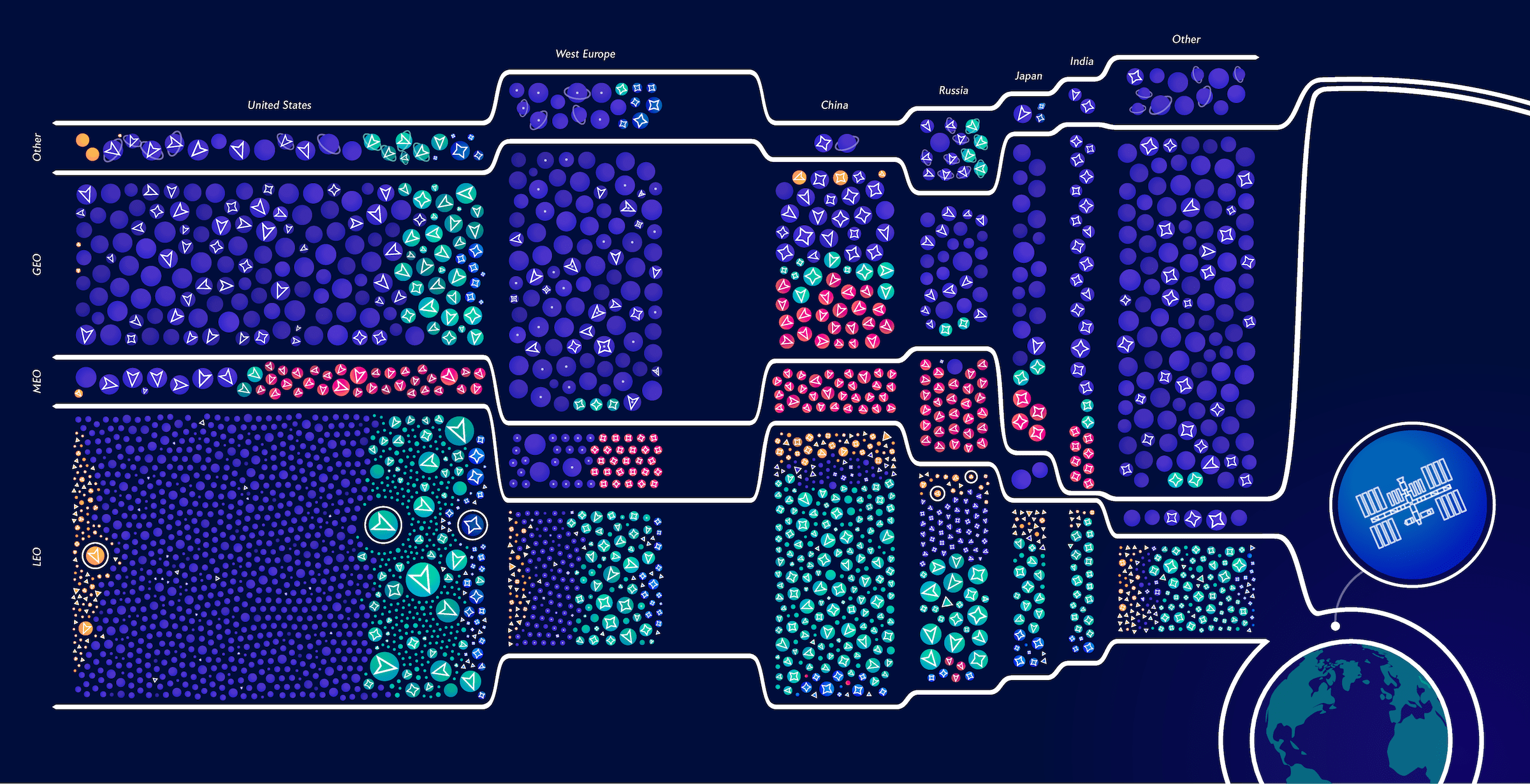
Created for Scientific American, this colorful and bright data visualization displays satellites in an original way. Neat and stylish satellite cluster grids sort them by country, orbit and class – business/commercial, civil, amateur/academic or defense.
The graphic details the mass of the satellites (100 kgs - 5,000 kgs), category (Test and Training, Communications, Images, Surveillance and Meteorology, Navigation and Research) and the launch date, from Nov 1974 till Aug 2020.
According to the graphic, six countries of the world control the largest amount of the satellites in orbit, and the US owns the largest share so far.
17 Covid Vaccination Tracker
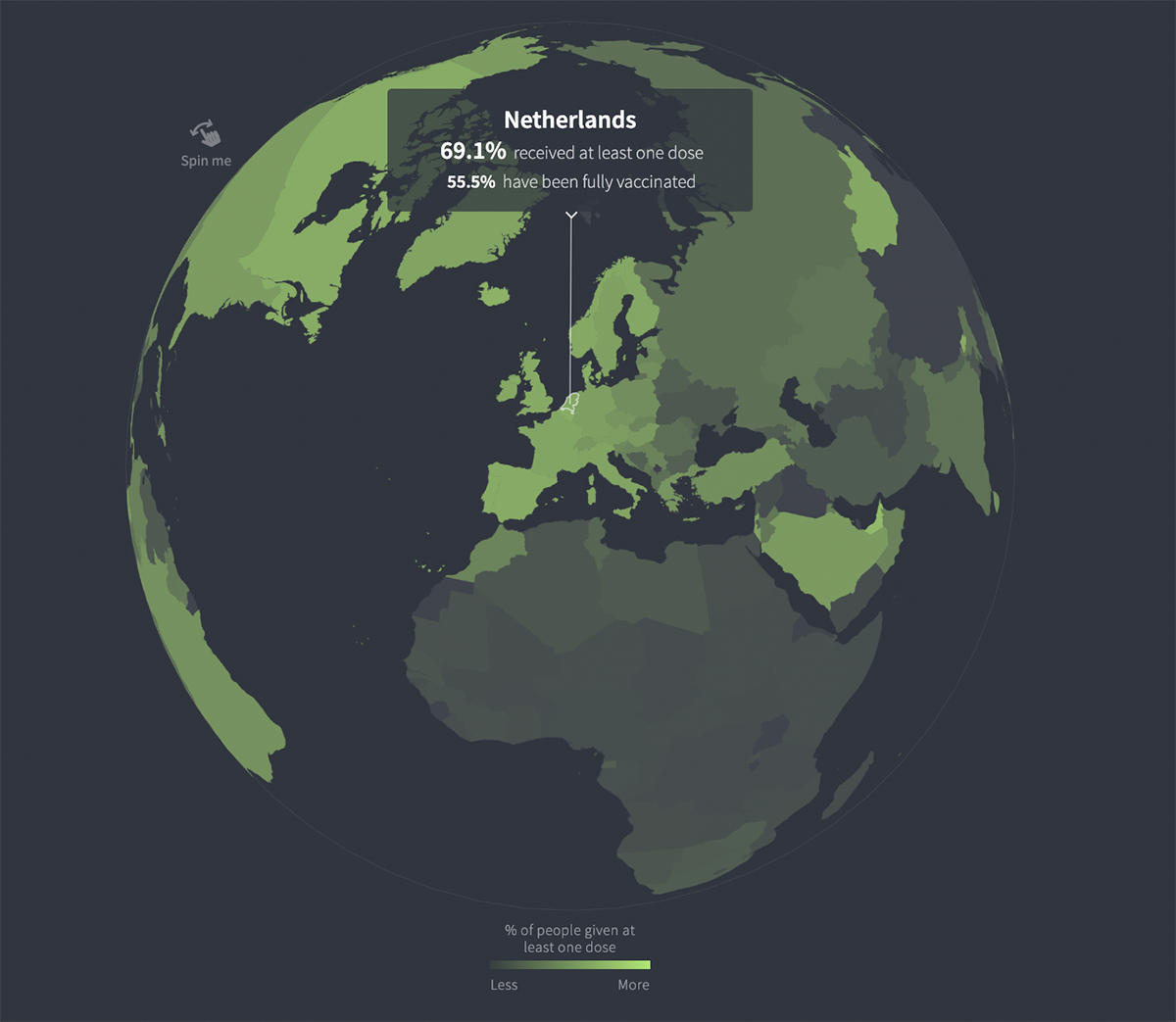
Updated until July 15, 2022, this animated Covid vaccination tracker shows the percentage of people in the world given at least one dose. The infographic and data illustration displays data on the vaccination rollout plan in over 80 countries and 50 US states.
Data presented in this data visualization is sourced from the Our World in Data project at the University of Oxford. Uncluttered, simple graphs show the 7-day Covid vaccination rolling average as well. The interactive charts allow you to sort the percent of population given at least one dose by country or income.
At the bottom of the page we can see the detailed, in-depth Covid-19 vaccination statistics, with type of vaccines offered (Pfizer-BioNTech, Moderna, Sinopharm, CanSino, Oxford-AstraZeneca, Johnson & Johnson, Covishield, Sputnik V, etc.) and vaccination priority groups for various countries separately.
If you’re working on an infographic that includes map data, like this example, try Visme’s map data visualization tool . It comes equipped with a handy hover tooltip that labels country names and square footage. If you don’t need to show this data, you can hide it in the Map settings.
Create demographic visualization easily with Visme’s map templates . If you need to edit your map infographic on the go, you can do so from the mobile app on Android and iOS.
18 Blindsight
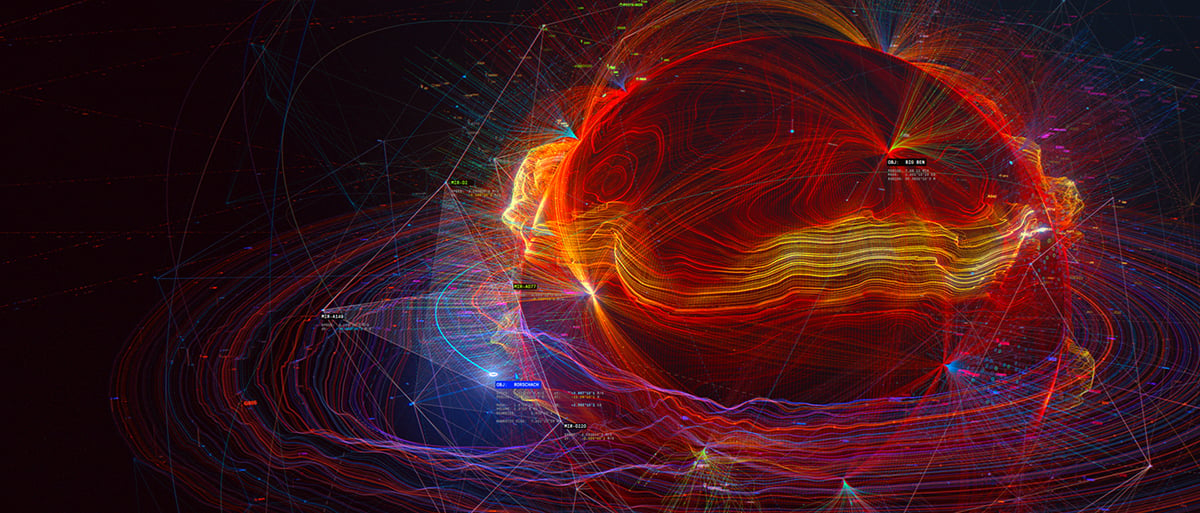
It took 4 years to create this non-commercial self-funded project. Based on the eponymous sci-fi novel by Peter Watts, this visualization row includes breathtaking renders of the solar system, four-dimensional objects as a system of data visualization and manipulation, spacesuit interface renders, cryo capsule graphics and nonhuman species concepts.
The visualization received over a dozen awards and nominations such as Best VFX Screen Power Film Festival 2020, Outstanding Achievement Award (Sci-fi Short) Indie Short Fest LA 2020, Winner Best Sound & Music Fantasy/Sci-fi film Festival 2021, Award Winner Flickfair 2020, Official selection Miami International Sci-fi Film Festival 2021 and so on.
Space mysteries have always tempted mankind. With the outstanding talent of the team behind the project, we hope to enjoy the related movie one day.
19 Gravitational Waves
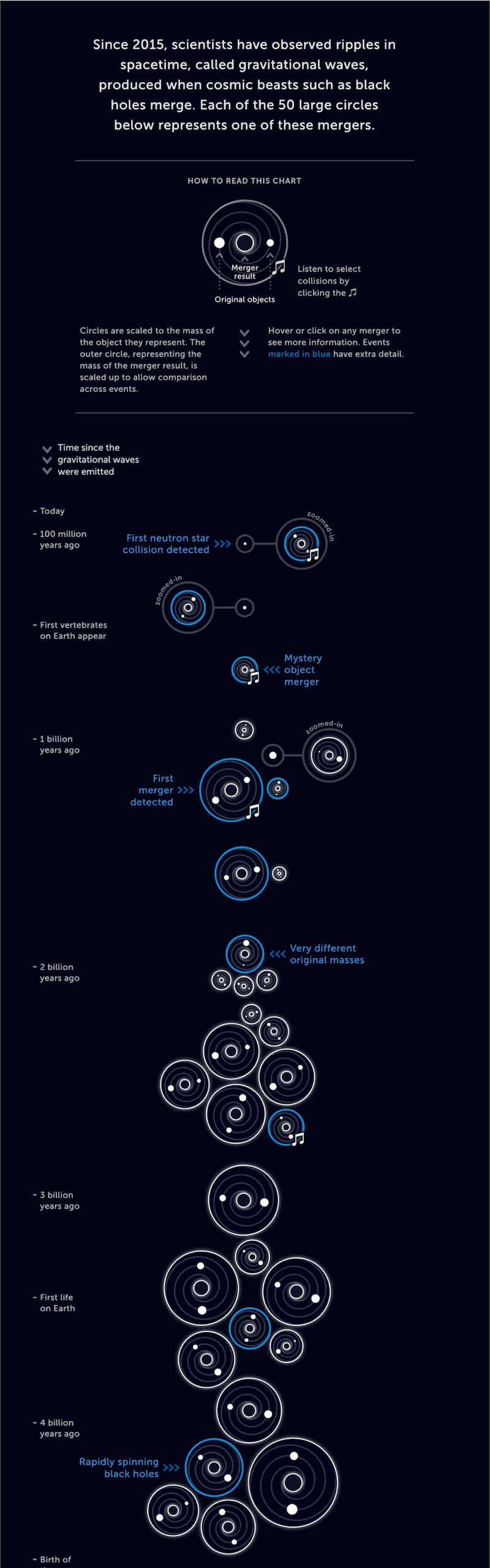
Introducing to you another captivating space-themed project – the interactive visualization of gravitational wave events. Created for Science News, this space-time ripples design is amazingly minimalistic, slick and informative.
This enchanting spiral animation is saturated with useful data about black hole mergers or cosmic smashups. You can learn about the original and final mass of the mergers, total merger size and other details of gravitational wave events.
20 Map of the Lighthouses of Ireland
Updated my map of the lighthouses of Ireland from the #30DayMapChallenge - now with the correct timings/flash patterns etc. Thanks to @IrishLights for providing additional information pic.twitter.com/eLlicP8fw5 — Neil Southall (@neilcfd1) December 8, 2020
This great animation was created as a part of 30 Day Map Challenge and it depicts all lighthouses in Ireland according to their timing and flash patterns. Here, the author visualizes data from the IrishLights – the maritime organization delivering the safety service around the coast of Ireland.
Aside from being a vital part of the water safety of coastal waterways, lighthouses are a symbol of hope and undying light even through the toughest circumstances. That’s one of the reasons why this minimalistic graphic is so appealing.
21 Together [Hierarchical Positions of Employees in a Corporation]
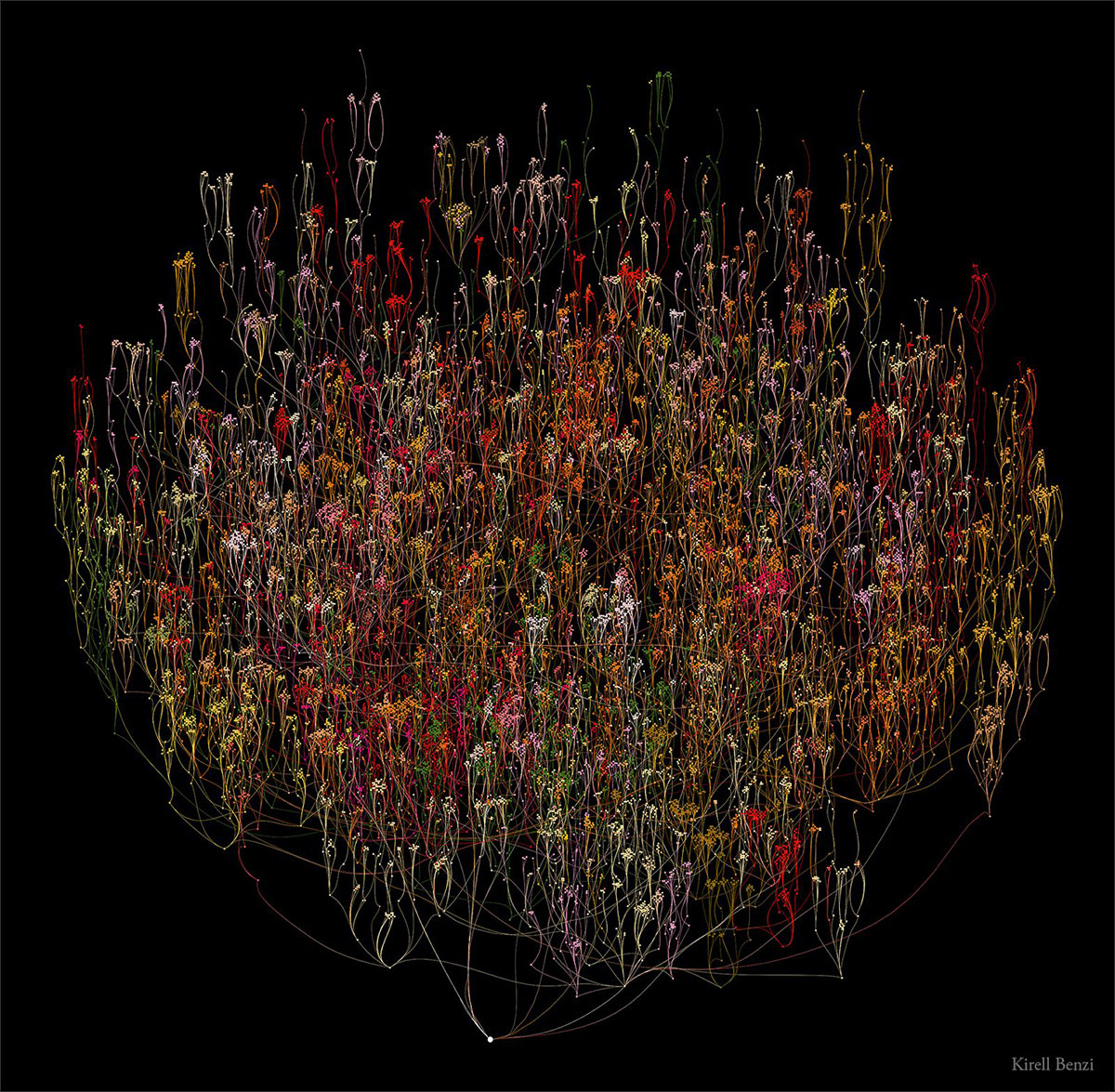
Good data visualizations are essential for conveying complex information in an easily understandable way. Look at this creative way of displaying the hierarchical organization structure in a large corporation with a presence in over 100 countries. This creative data visualization example looks fun and a bit otherworldly, with muffled but contrasting colors.
Linking C-level executives to their subordinates in every branch revealed an intricate and complex corporation structure. It’s suggested that in most cases, flat patterns would fail to represent company structures correctly because of the flexibility of human relations.
22 The Search for Dark Matter
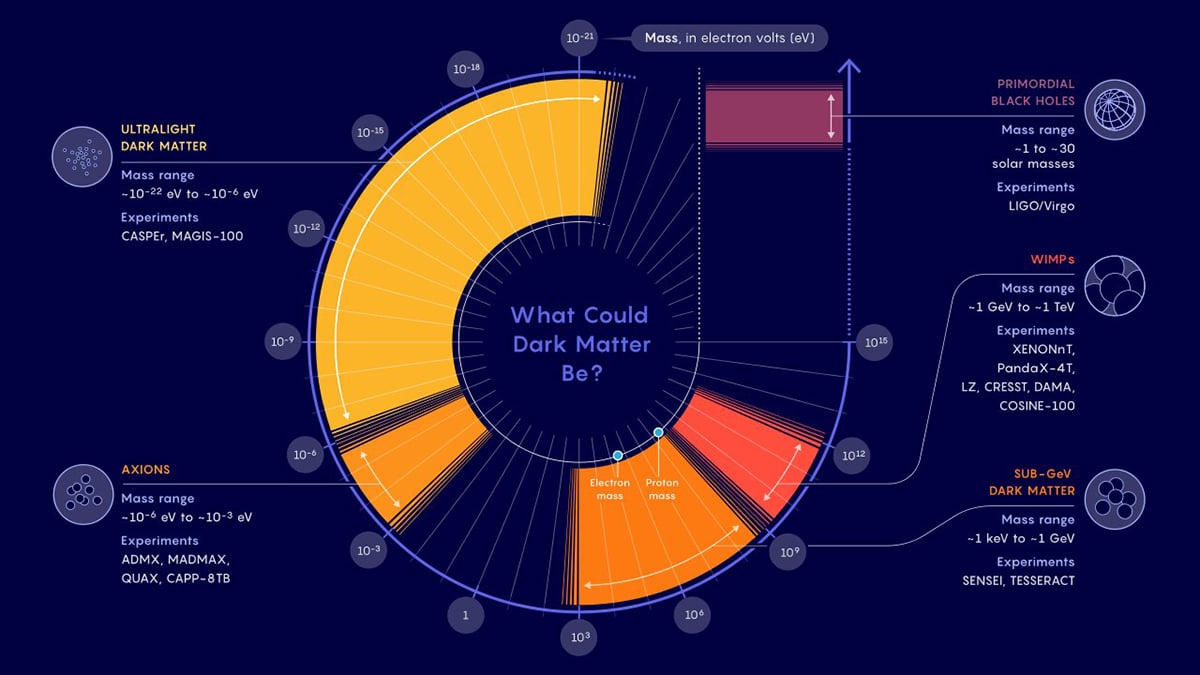
The search for the ever elusive and intriguing dark matter continues. The problem isn’t likely to get solved any time soon – but here is a striking infographic for you to follow the lead.
Quanta Magazine created this interesting data visualization to represent the types of particles that dark matter could be made of. Axions, WIMPs, ultralight dark matter or primordial black holes – any of these could be a star candidate.
Distributing every particle type along the scale according to their mass, the visualization also provides clear, concise descriptions for every type. Additionally, you can dive into the experiments’ data. Are you the one to solve the new puzzle in particle physics?
23 2020 Autonomous Vehicle Technology Report
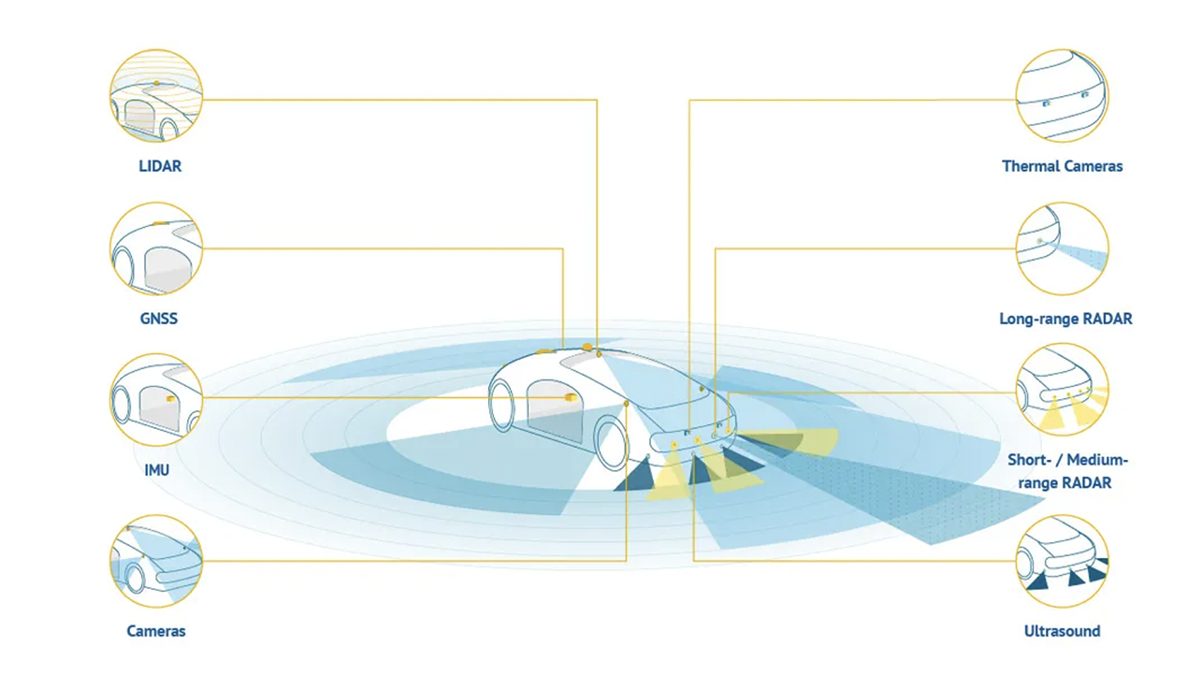
Concise and lean, this comprehensive report draws focus to autonomous vehicle technology and provides an insight into the hardware & software market for self-driving vehicles.
The report starts from the visualization explaining levels of autonomous vehicle capabilities in context of the environment. We learn that the greatest challenge for Google (Waymo), Uber and other companies building self-driving vehicles is to enable the vehicle to adjust to all driving scenarios.
Sensory technology is an essential part of autonomous vehicles, and they’re designed to build an environment map and localize themselves inside that map at the same time. This requires huge computational technologies – maps created by AI systems and humans are of great help here.
Further in the report, we see the visualization of the electromagnetic spectrum and its usage for perception sensors, graphics of the time-of-flight (ToF) principle of environment sensing and various object detection sensor types such as radars, cameras, LIDARs, MEMS, etc. The next visualization covers different sets of sensors used for autonomy by Tesla, Volvo-Uber and Waymo.
Short, clean-cut schemes of the AI architecture of autonomous vehicles, the computation/decision making environment of an autonomous vehicle and the concept of vehicle-to-everything (V2X) communication complete the report.
24 The U.S. Election Twitter Network Graph Tool
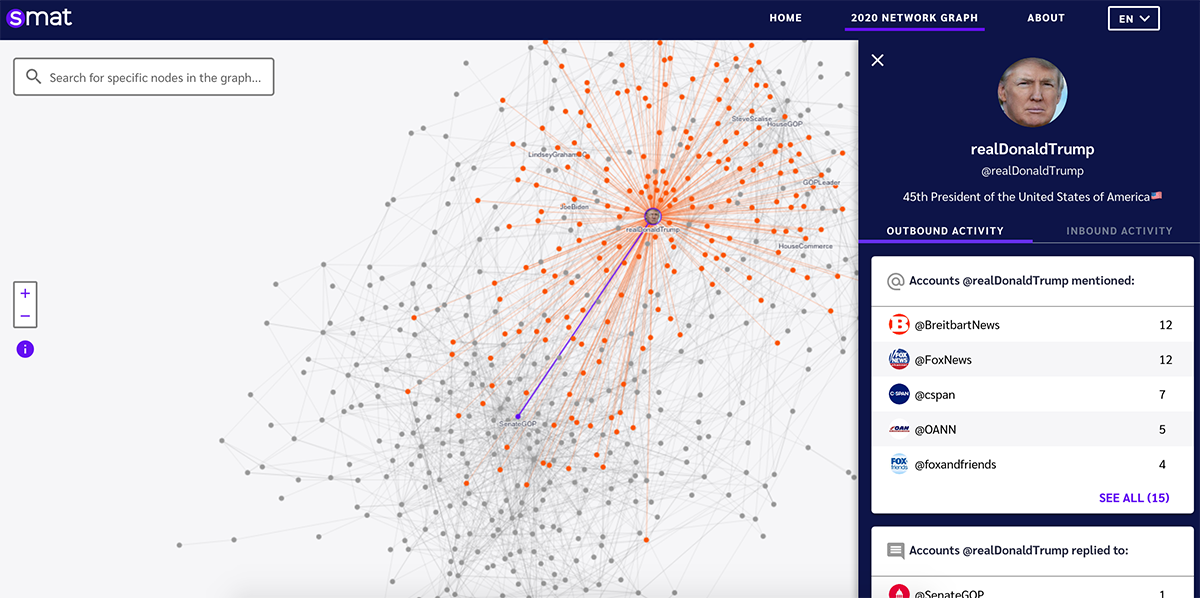
These cutting edge visuals from the U.S. Election Twitter Network Graph Tool enables a viewer to analyze social media interactions that define the online political landscape. In this case, we’re tracking the influence and connections between various political figures.
It’s clearly visible which accounts the target account is most likely to mention or reply to. The network graphs clearly show the potential of certain accounts to generate new connections and influence their followers.
You can search for specific nodes in the interactive map. All information flow between nodes is reflected in the color of the node edges. Working together with other open-source investigation tools, this graph is meant to increase transparency and help fight misinformation in social networks.
25 Map of a Fly Brain
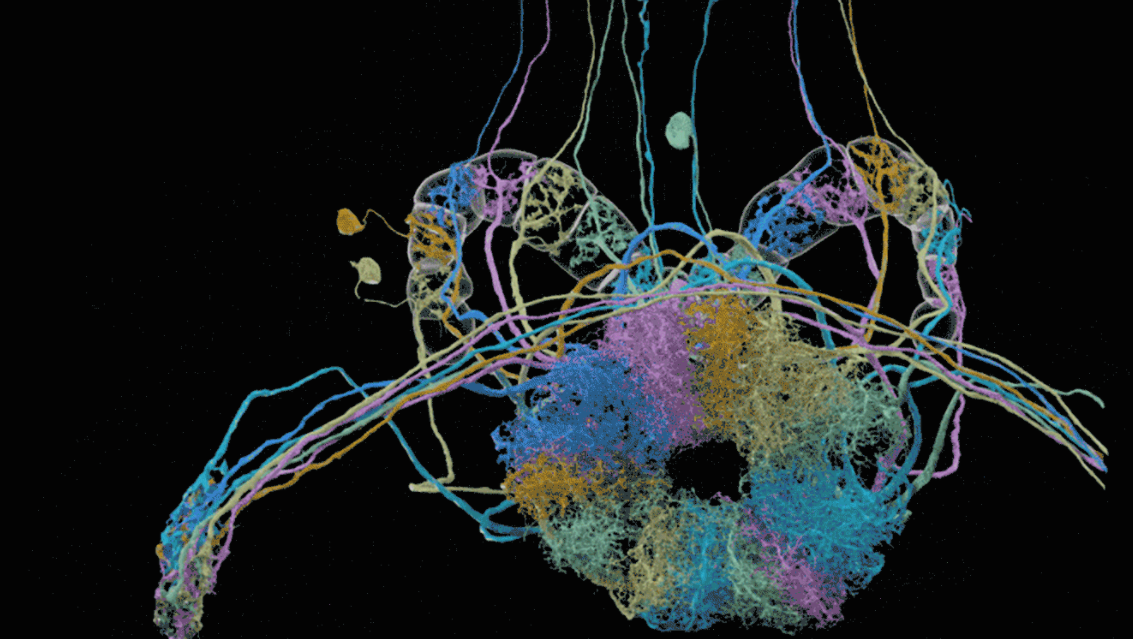
The high-resolution nervous system map represented in the above graphic is a part of the fruit-fly’s brain – yet the complexity and harmony of the structure is astounding.
Millions of connections between 25,000 neurons create a wiring diagram, or connectome, of connections in various parts of a fruit fly’s brain.
It’s estimated that tracking all neuron connections in the fruit fly’s brain manually would need 250 people working for 20 years at least. Google’s computational power has helped to speed up this research, and scientists are aiming to create a full fruit fly brain visualization by 2022.
26 Freight Rail Works
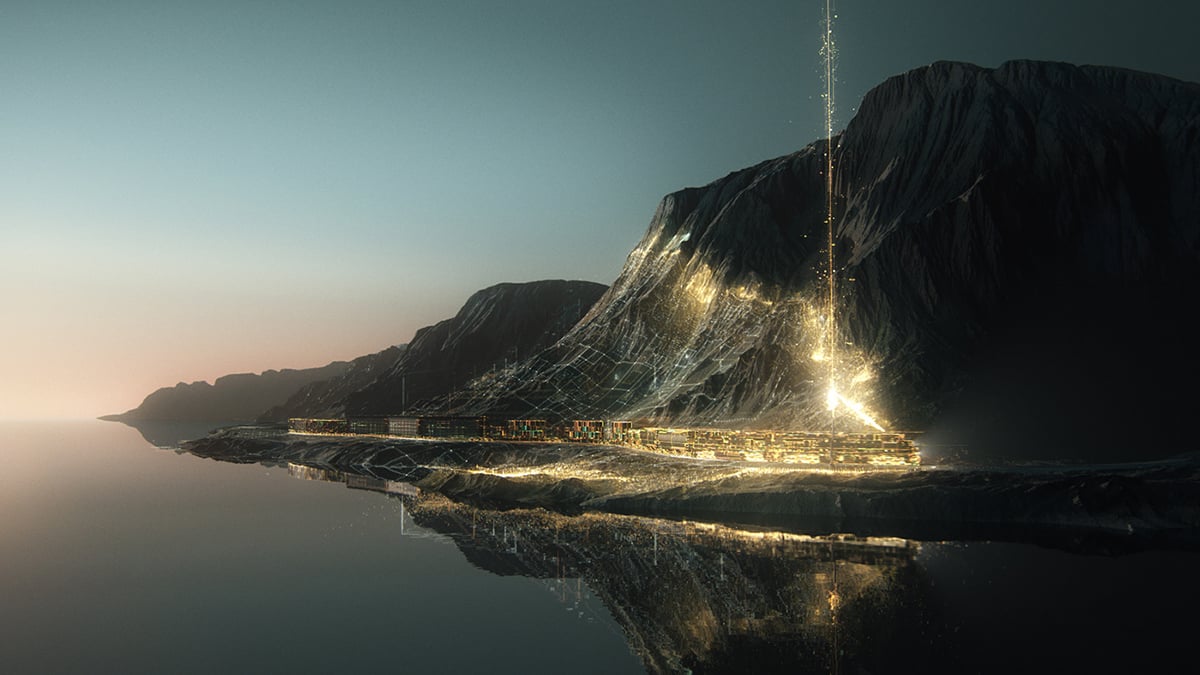
Our next interesting visualization highlights the advanced layers of technology Freight Rail Works uses across its infrastructure. Talented Danil Krivoruchko & Aggressive/Loop teams produced a futuristic and dynamic animation of the data-world around a train in motion.
Magnificent waves of data light up outlines of the objects and then vanish in waves as the train moves forward to the smart city. Graphics of the giant city cluster zoom out to reveal the continent routes and the beauty of a simple railway communications network.
In the era of semi-autonomous aircrafts and drones, the simple, down-to-earth railway system looks stable but innovative in this graphic.
27 The Korean Clusters
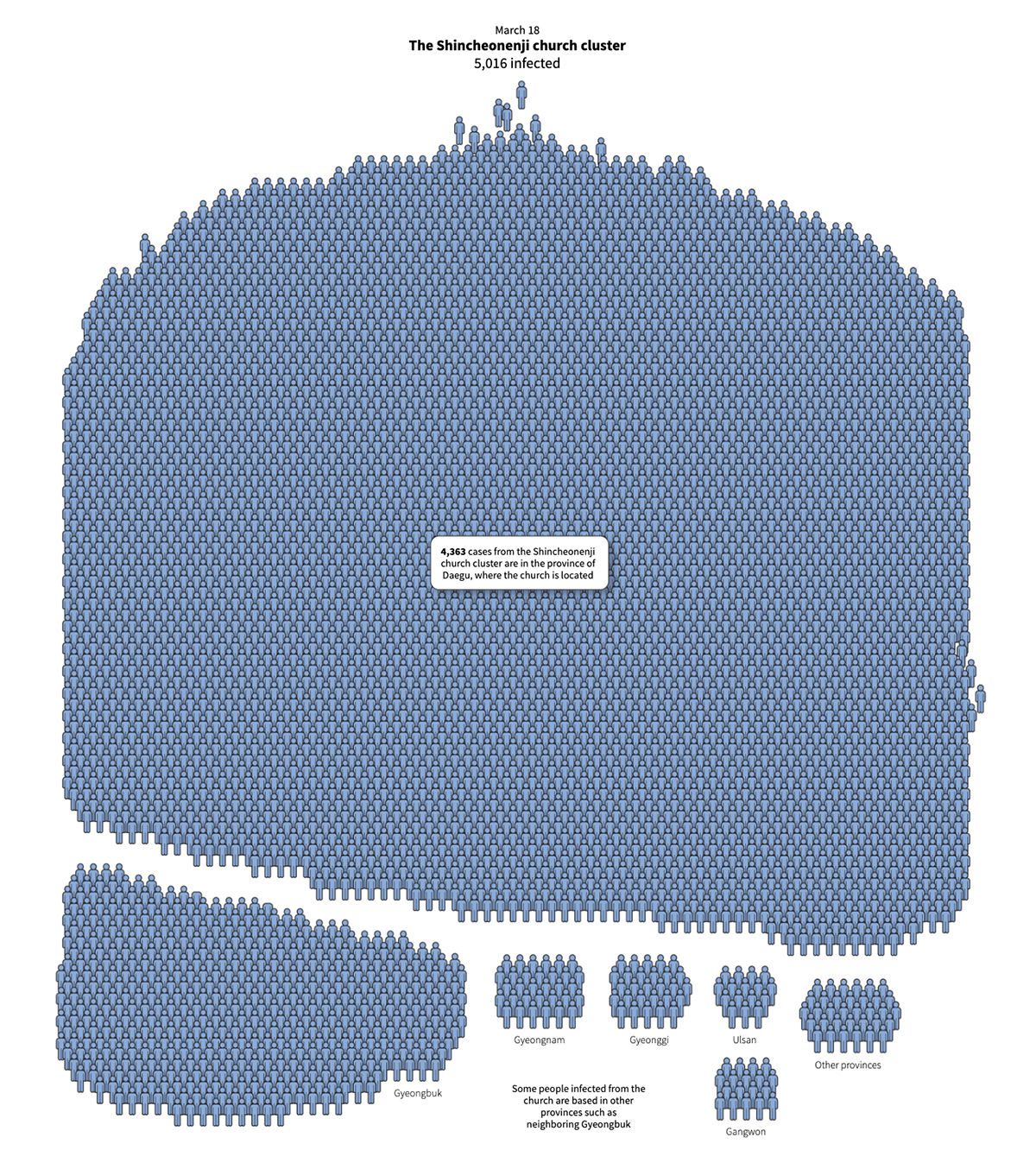
Korean hospitals and churches experienced a burst of Covid infections among their visitors in January 2020. Having linked connections between the confirmed cases, scientists were able to trace back the first case and build a tree of contacts between the affected people.
Tracking the timeline of the first patient’s actions revealed that this person caused thousands of infections. Wandering sick for a few days resulted in over 30 more people infected. Subsequently, the Shincheonji Church cluster with 5,016 infected people accounted for at least 60% of all cases in South Korea at that time.
28 2020’s Biggest Tech Mergers and Acquisitions
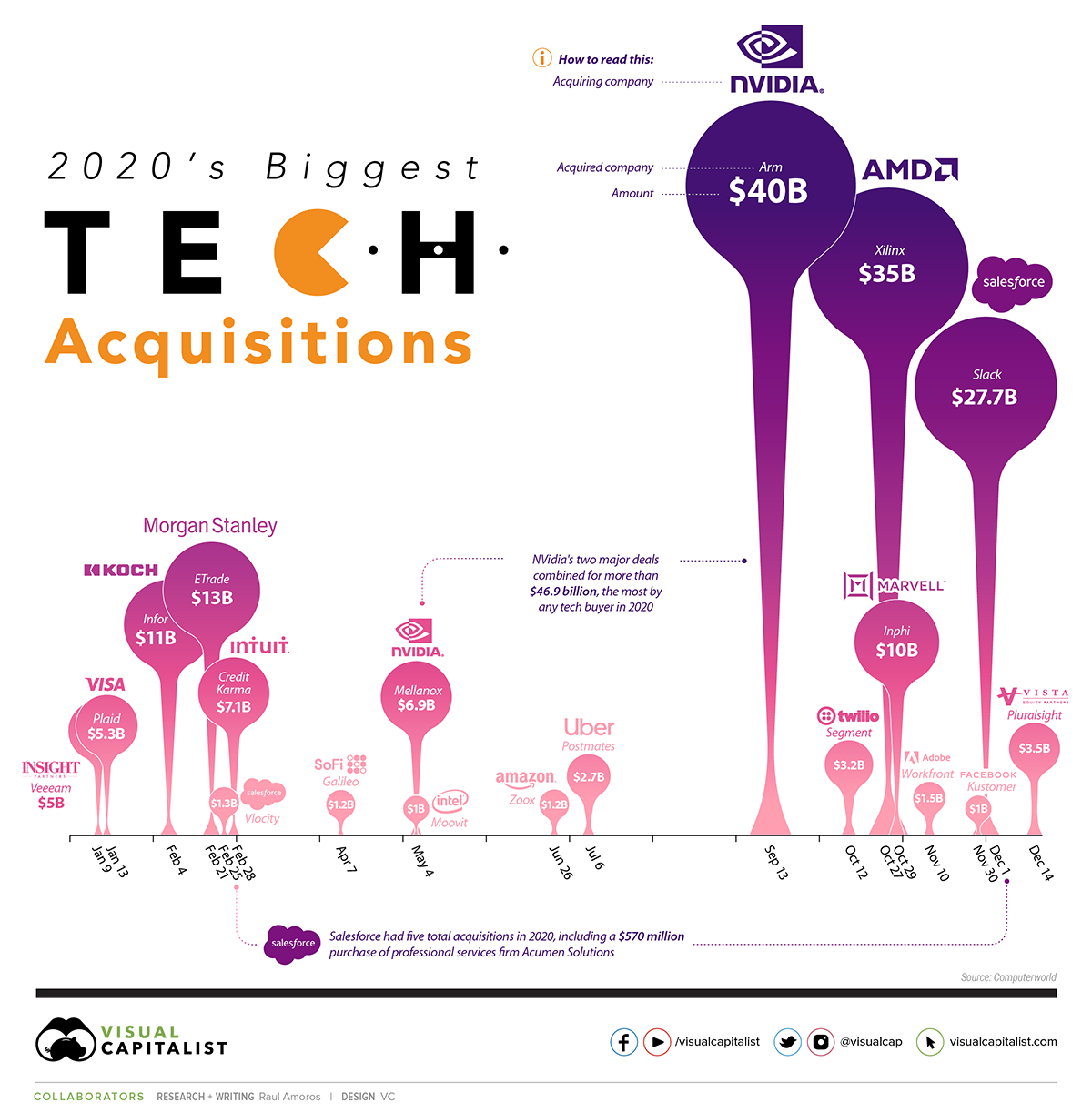
Despite the fact that for most businesses 2020 was a devastating year with grim outcomes, this data visualization shows that Big Tech experienced a growth boost. It’s not surprising that people working remotely increasingly need digital services of all kinds.
The graphic shows the biggest tech mergers and acquisitions closed in 2020, together with the short description of the acquired company, acquiring company, deal amount and deal date. While the chart is visually busy, it’s also innovative and visually appealing.
If you need a market report from your industry area, grab the data from Crunchbase and build your own custom branded infographic via our data visualization tool quickly and easily. Sign up free .
29 Stolen Paintings
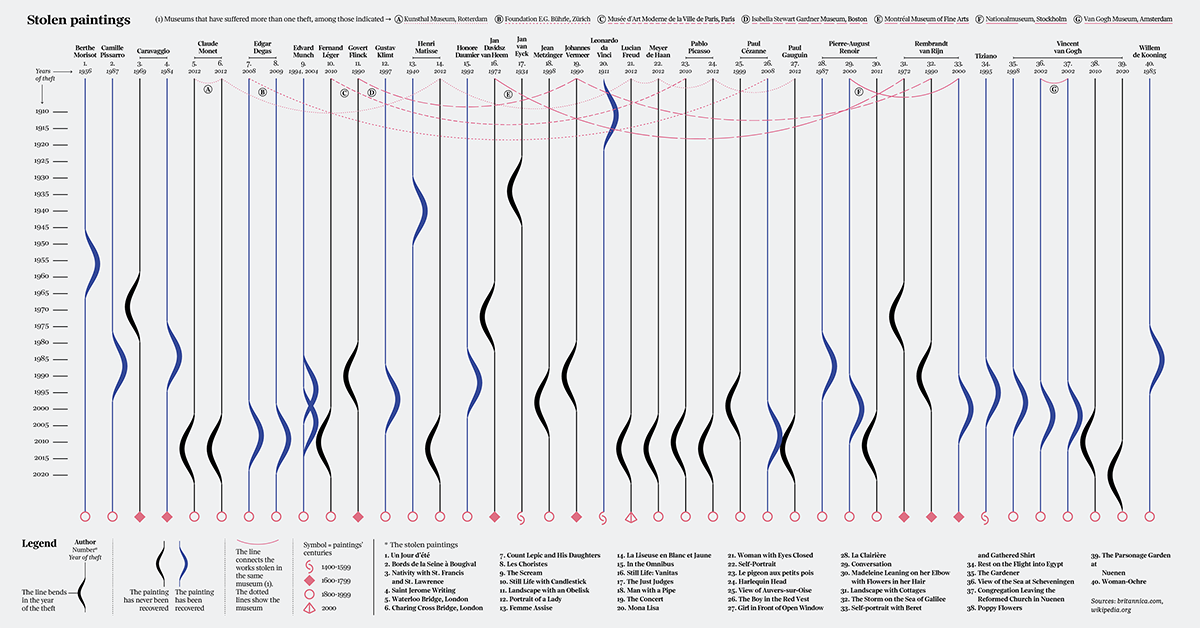
This wonderful visualization was created for Visual Data, a column on "La Lettura," the cultural supplement of "Corriere Della Sera."
From 1900 to the present day, the infographic reveals the details of 40 stolen paintings. Neutral, minimalistic visuals highlight the painting’s artist, the year when the painting was created and the year of theft.
It was shocking to find out that the majority of thefts took place during the last 20 years (2000-2020) – and most of the art works have never been recovered.
30 House Of Cards LIDAR
House of Cards from Brendan Dawes on Vimeo .
Take a look at the last cool data visualization in this list – the rework of Radiohead's House of Cards video. This astonishing art was created on the basis of around one minute of the LIDAR data.
Motion graphics of particles scattered around a person’s face create an unforgettable image. The hero of the story in the video is clearly emotional – but we can’t tell anymore whether this person is even human.
AI generated data can be beautiful, but how can you take control?
Data Visualization FAQs
What is the most popular form of data visualization.
Bar graphs, bar charts or column charts are the most popular type of data visualization.
Bar charts are best for comparing numerical values across categories using rectangles (or bars) of equal width and variable height. You can use bar graphs to compare items between different groups, measure changes over time and identify patterns or trends.
Other popular forms of data visualization include pie charts , line graphs , area charts , histograms , pivot tables, boxplots, scatter plots , radar charts and choropleth maps.
What Are the Benefits of Data Visualization?
Here’s how data visualization helps users to make the most of their data.
- Data visualizations make data clear, concise and easy to understand. Users can easily unlock key values from massive data sets, interpret them and draw conclusions.
- Visualization allows business users to identify relationships, patterns and trends between data, giving it greater meaning. You can easily uncover fresh insights and focus areas that require more attention.
- Creative data visualization is about creating compelling narratives through the use of graphics, diagrams and visual analytics. Visualizing data helps users tell better stories and convey messages in an engaging manner.
- Data visualization can significantly increase the pace of decision-making processes since it makes it simple for us to understand visual data. It’s no surprise, as The Wharton School of Business says that data visualization can cut down on meeting time by up to 24% .
Visualizing data helps quickly spot any errors so they can be removed. If you still doubt the importance of data visualization, this article about 50 data visualization statistics might change your thought process.
What are the Best Practices of Data Visualization?
Below are data visualization best practices to help you present data in an engaging and appealing way.
- Specify the audience and their unique needs. Your data visualization should be crafted to communicate, provide real value and meet the needs of the target audience.
- Define a Clear Purpose. Specify what questions you want your data visualizations to answer or the problems you want them to solve.
- Keep your data clean. Before visualizing your data, make sure to fix or remove incomplete, duplicate, incorrect, corrupted and incorrectly formatted data within your dataset.
- Use the right visuals. With so many charts available, identify the best type for presenting the particular data type you’re working on.
- Keep your data organized. At a glance, your audience should be able to view and digest information quickly.
- Use the right color combination.
Read our article to learn more about data visualization best practices.
Create Your Own Data Visualizations
If you are feeling inspired by these cool data visualizations, use our data visualization software to convert disparate data into clean, comprehensive visuals using the best data visualization techniques . You'll find an extensive library of customizable charts and graphs including bubble charts, bar graphs , line charts , scatter plots, and much more.
Wondering if Visme's data visualization tools are right for you? Take a look at what one of our satisfied customers, Cassandra C. | Owner, has to say:
“I also appreciate the wide range of features, including charts, graphs, and other visuals that can be used to present data in a clear and concise way. Overall, I'm very happy with Visme and would highly recommend it to anyone looking for a fun, user-friendly tool to create visuals.”
To learn more about creating your own data visualizations, check out our detailed guide on data visualization types and the introduction to data viz on our blog.
Create beautiful charts, graphs and data visualizations with ease.
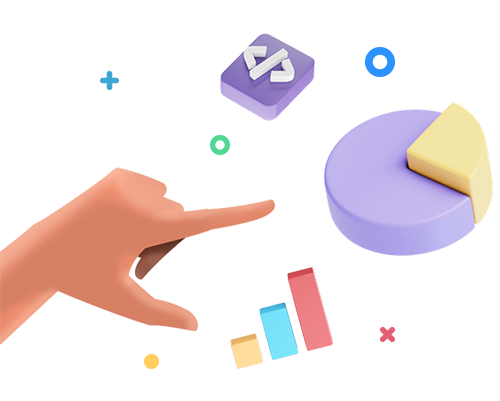
Trusted by leading brands
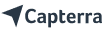
Recommended content for you:
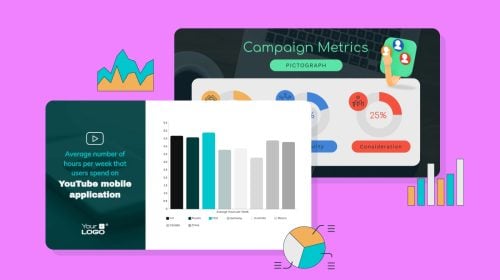
Create Stunning Content!
Design visual brand experiences for your business whether you are a seasoned designer or a total novice.
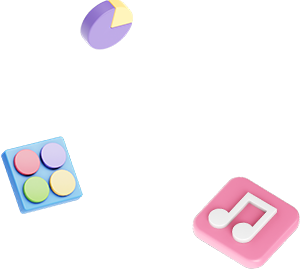
About the Author
Anna enjoys hot weather, collecting shells, and solving challenges in B2B marketing. She delights in thinking about abstract ideas and analyzing complex information to choose the best solution.
👀 Turn any prompt into captivating visuals in seconds with our AI-powered design generator ✨ Try Piktochart AI!
18 Types of Diagrams You Can Use to Visualize Data (Templates Included)
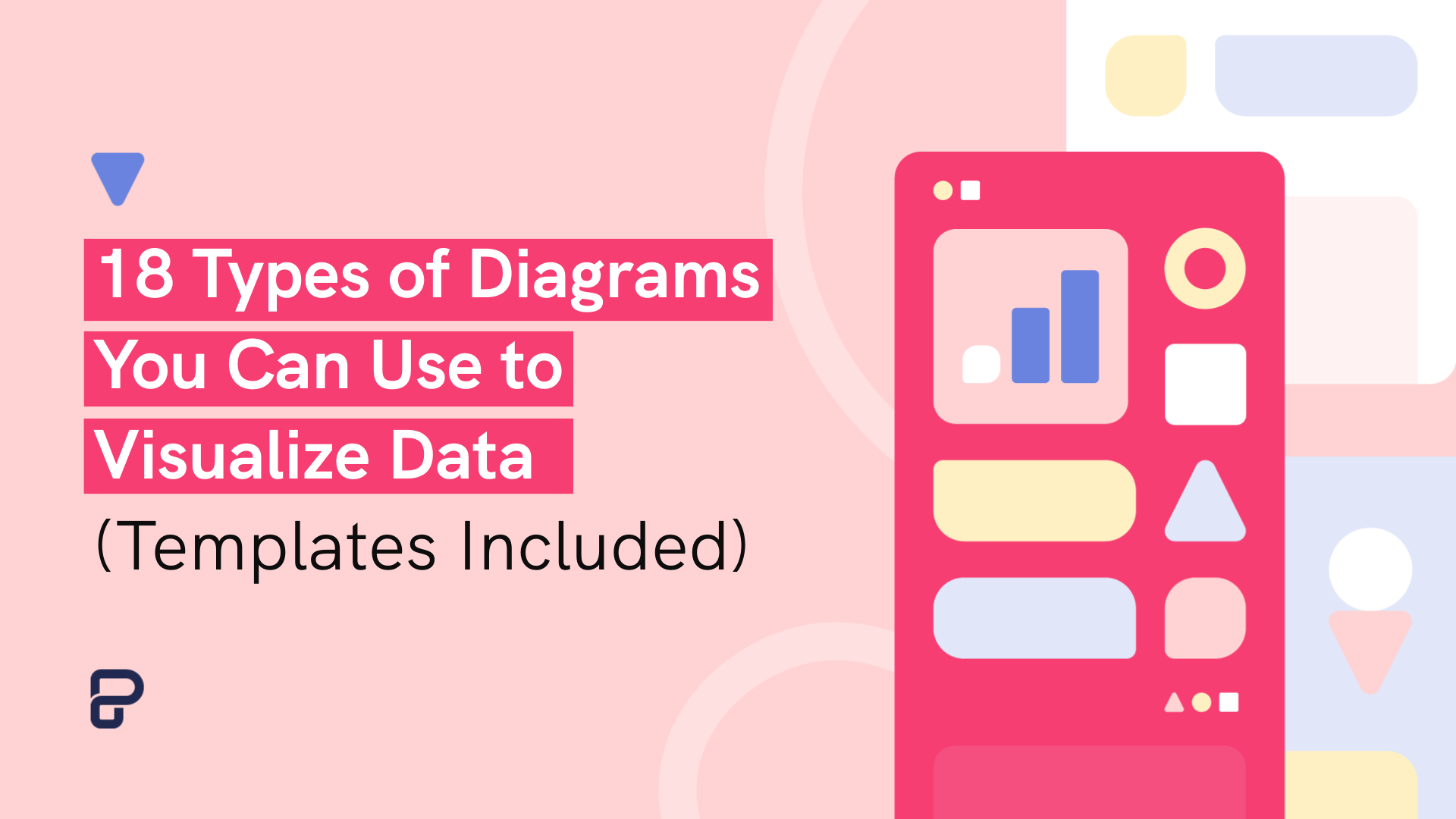
Have you ever found yourself stuck while trying to explain a complex concept to someone? Or struggling to put your idea into words?
This is where diagrams come in.
While simple text is best for highlighting figures or information, diagrams are handy for conveying complex ideas and loads of information without overwhelming your audience. They can visualize almost anything, from numerical data to qualitative relationships, making them versatile tools in numerous fields.
Whether you’re in the academe or enterprise setting, this guide is for you. We’ll explore the different types of diagrams with a brief explanation for each type, the best time to use a diagram type, and how you can use them to be a better visual storyteller and communicator. You’ll also find examples and templates for each type of diagram.
Let’s get on with it.
You can also follow along by creating a free account . Select a template to get started.
What exactly is a diagram?
A diagram is a visual snapshot of information. Think of diagrams as visual representations of data or information that communicate a concept, idea, or process in a simplified and easily understandable way. You can also use them to illustrate relationships, hierarchies, cycles, or workflows.
Diagrams aren’t just used to show quantitative data, such as sales earnings or satisfaction ratings with a diagram. They’re equally helpful if you want to share qualitative data. For example, a diagram could be used to illustrate the life cycle of a butterfly, showcasing each transformation stage.
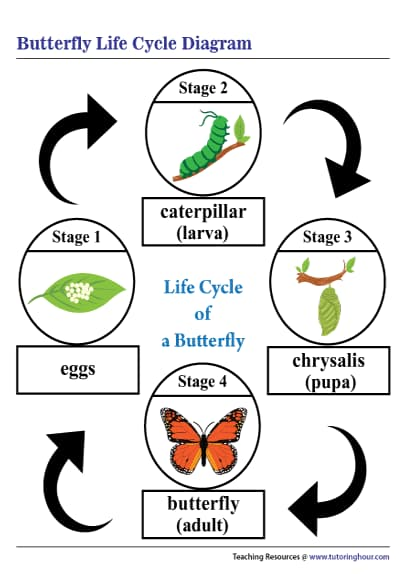
Now, let’s jump into the various types of diagrams, ranging from simple flow charts to the more complex Unified Modeling Language (UML) diagrams.
18 diagram types and when to use each type
Whether you’re doing data analysis or need a simple visual representation of data, there is a wide array of diagrams at your fingertips. If you’re having a hard time choosing the right diagram for your data visualization needs, use the list below as a quick guide.
1. Flowchart
A flowchart is a type of diagram that acts as a roadmap for a process or workflow. It uses shapes and arrows to guide you through each step, making complex procedures simple to understand.
Flowcharts are best for : Simplifying complex processes into understandable stages, making it easier for your readers to follow along and see the ‘big picture”.
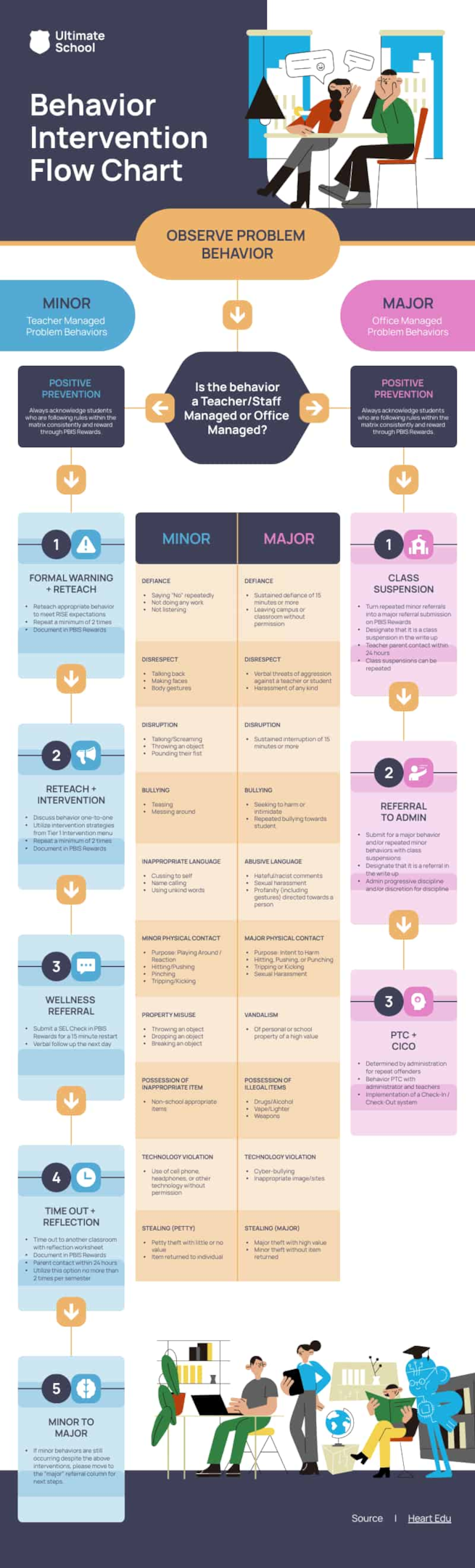
2. Line graph
Line graphs , sometimes called line charts, visualizes numerical data points connected by straight lines. In a line graph or line chart, data points representing different time periods are plotted and connected by a line. This helps with easy visualization of trends and patterns.
Line graphs are best for: Representing the change of one or more quantities over time, making them excellent for tracking the progression of data points.

3. Bar chart
A bar chart , often interchangeable with bar graphs, is a type of diagram used primarily to display and compare data. For this diagram type, rectangular bars of varying lengths represent data of different categories or groups. Each bar represents a category, and the length or height of the bar corresponds to the numeric data or quantity.
Variations of bar charts include stacked bar charts, grouped bar charts, and horizontal bar charts.
Bar charts are best for : Comparing the frequency, count, or other measures (such as average) for different categories or groups. A bar chart is particularly useful if you want to display data sets that can be grouped into categories.
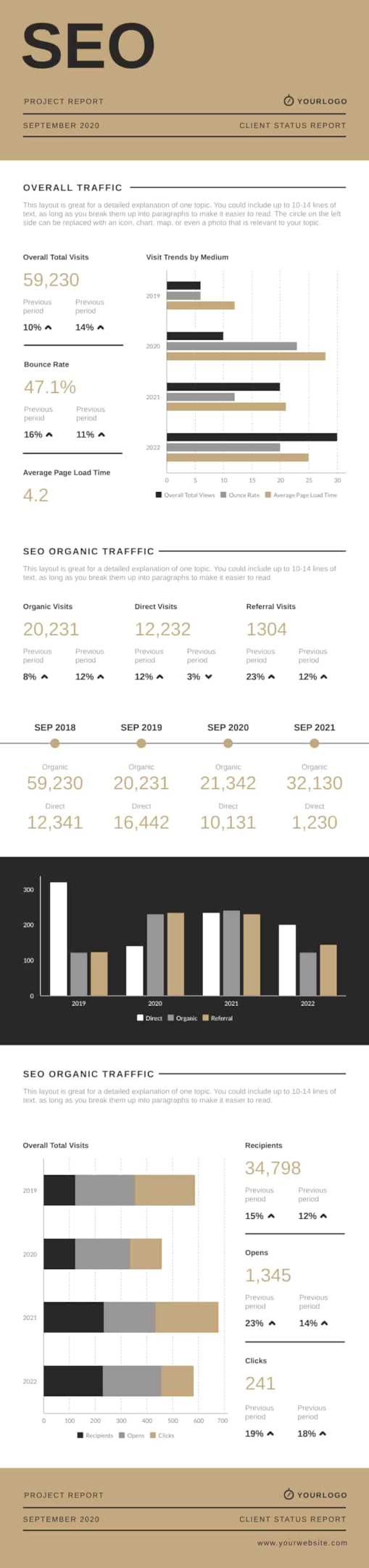
4. Circle diagram or pie chart
A pie chart is a circular diagram that represents data in slices. Each slice of the pie chart represents a different category and its proportion to the whole.
Pie charts are best for: Displaying categorical data where you want to highlight each category’s percentage of the total.
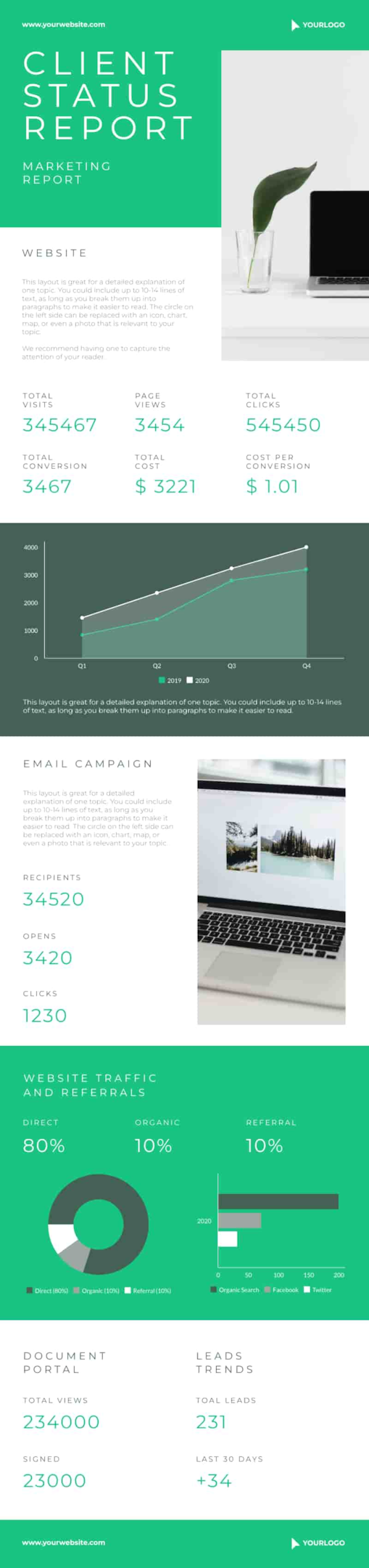
5.Venn diagrams
A Venn diagram compares the differences and similarities of groups of things. As a diagram based on overlapping circles, each circle in a Venn diagram represents a different set, and their overlap represents the intersection of the data sets.
Venn diagrams are best for : Visualizing the relationships between different groups of things. They are helpful when you want to show areas of overlap between elements. A good example is if you want to compare the features of different products or two overlapping concepts, like in the Ikigai Venn diagram template below. Easily create your Venn diagram with Piktochart’s online Venn diagram maker .
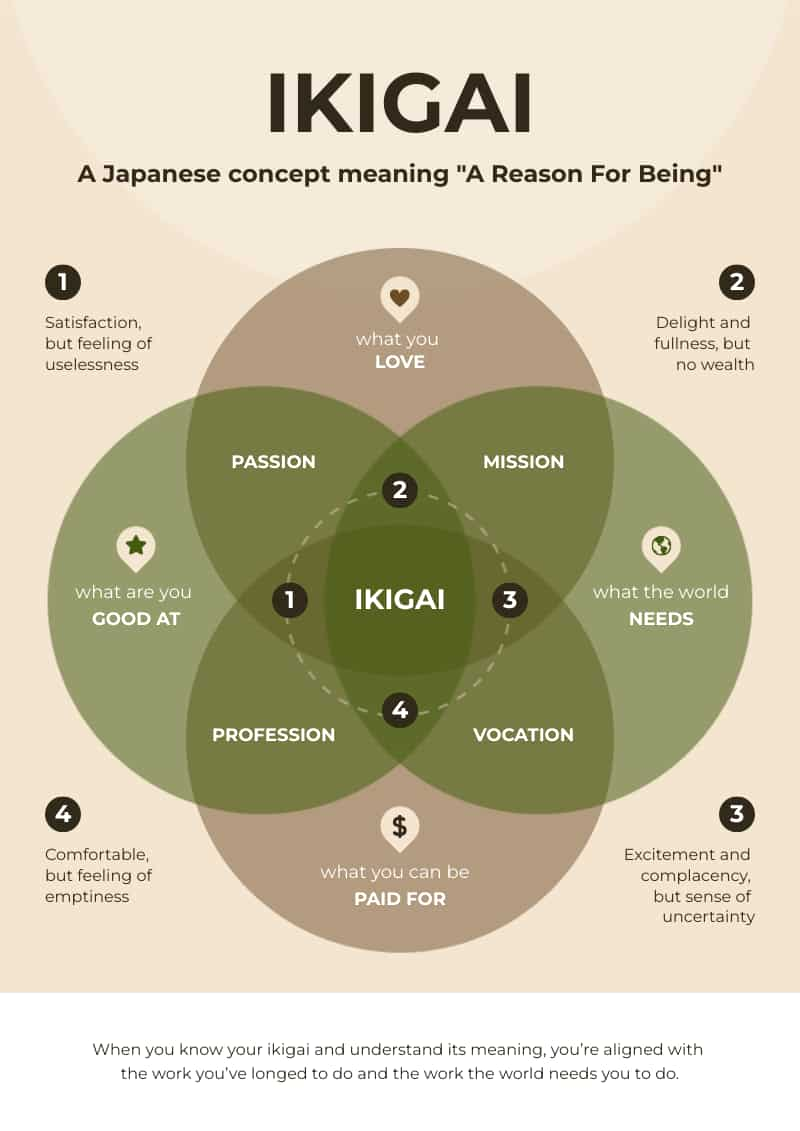
6. Tree diagrams
A tree diagram is a diagram that starts with one central idea and expands with branching lines to show multiple paths, all possible outcomes, decisions, or steps. Each ‘branch’ represents a possible outcome or decision in a tree diagram, moving from left to right. Tree diagrams are best for : Representing hierarchy like organizational roles, evolutionary relationships, or possible outcomes of events like when a company launches a product.
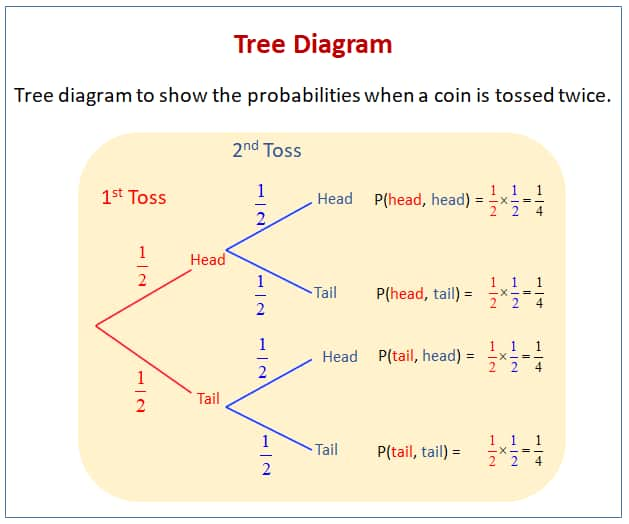
7. Organizational chart
Organizational charts are diagrams used to display the structure of an organization. In an organizational chart, each box or node represents a different role or department, and lines connecting the boxes illustrate the lines of authority, communication, and responsibility. The chart typically starts with the highest-ranking individual or body (like a CEO or Board of Directors) at the top and branches downwards to various levels of management and individual employees.
Organizational charts are best for : Showing relationships between different members and departments in a company or organization.
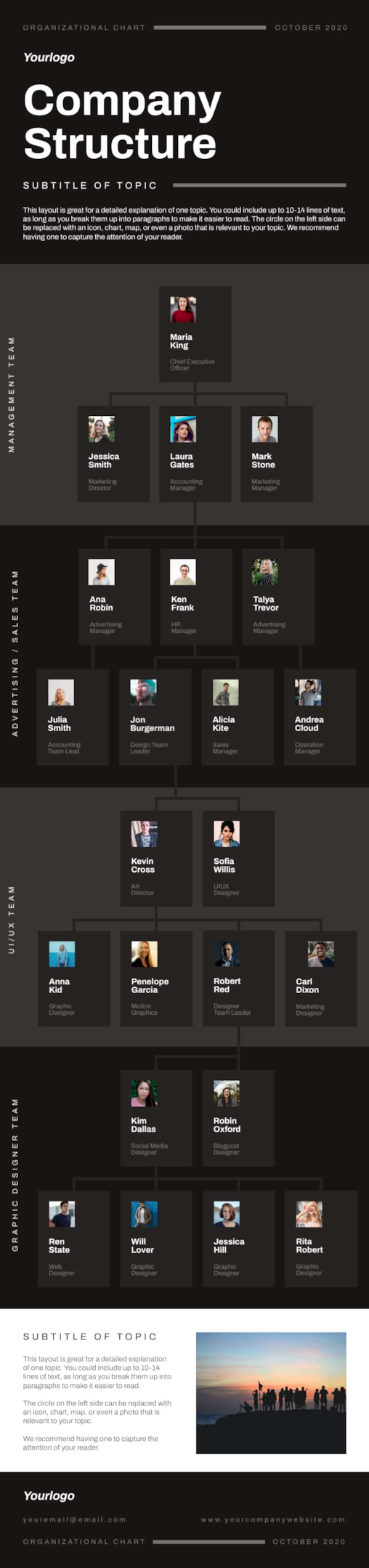
8. Gantt charts
Gantt charts are typically used in project management to represent the timeline of a project. They consist of horizontal bars, with each bar representing a task or activity.
For this type of diagram, each chart is represented by a horizontal bar spanning from its start date to its end date. The length of the bar corresponds to the duration of the task. Tasks are listed vertically, often in the order they need to be completed. In some projects, tasks are grouped under larger, overarching activities or phases.
Gantt charts are best for : Projects where you need to manage multiple tasks that occur over time, often in a specific sequence, and may depend on each other.

9. Unified Modeling Language (UML) diagram
Software engineers use Unified Modeling Language (UML) diagrams to create standardized diagrams that illustrate the building blocks of a software system.
UML diagrams, such as class diagrams, sequence diagrams, and state diagrams, provide different perspectives on complex systems. Class diagrams depict a system’s static structure, displaying classes, attributes, and relationships. Meanwhile, sequence diagrams illustrate interactions and communication between system entities, providing insight into system functionality.
UML diagrams are best for : Visualizing a software system’s architecture in software engineering.
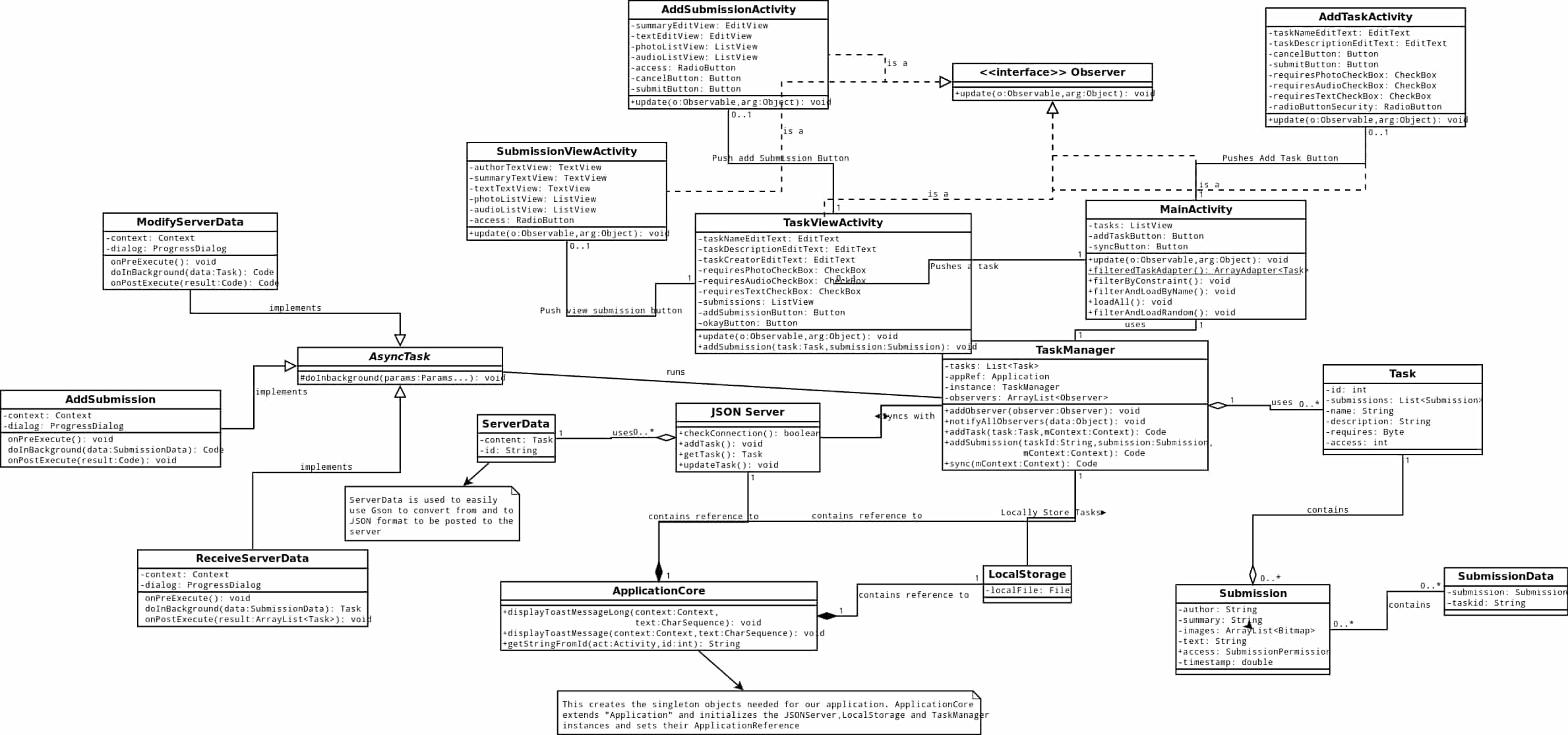
10. SWOT analysis diagrams
A SWOT analysis diagram is used in business strategy for evaluating internal and external factors affecting the organization. The acronym stands for Strengths, Weaknesses, Opportunities, and Threats. Each category is represented in a quadrant chart, providing a comprehensive view of the business landscape.
SWOT diagrams are best for : Strategic planning and decision-making. They represent data that can help identify areas of competitive advantage and inform strategy development.
Piktochart offers professionally-designed templates to create diagrams , reports , presentations , brochures , and more. Sign up for a free account today to create impressive visuals within minutes.
11. Fishbone diagram
Fishbone diagrams, sometimes called cause-and-effect diagrams, are used to represent the causes of a problem. They consist of a central idea, with different diagrams or branches representing the factors contributing to the problem.
Fishbone diagrams are best for : Brainstorming and problem-solving sessions.
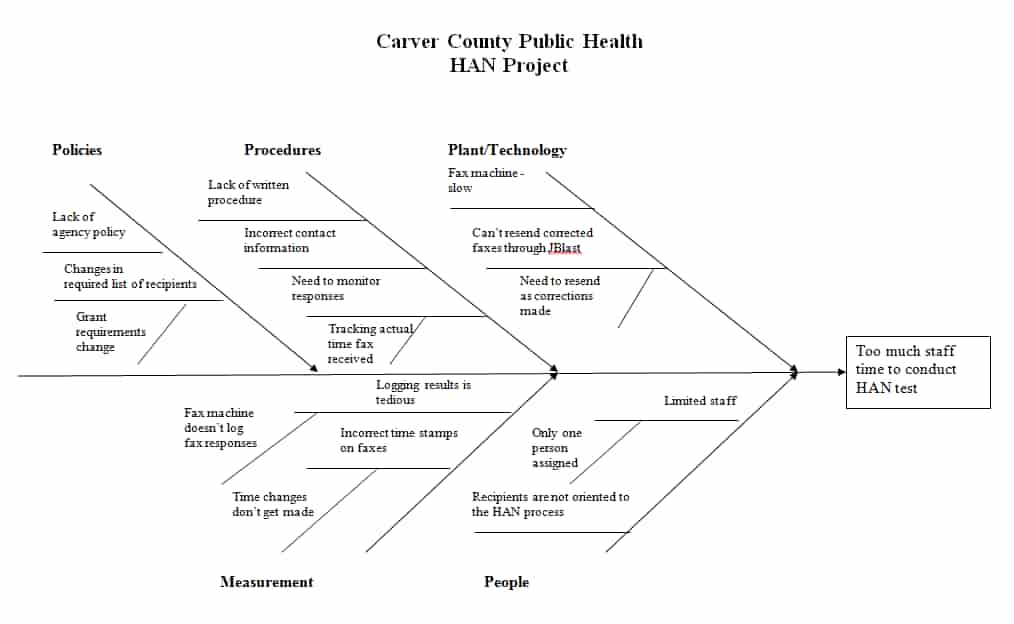
12. Funnel chart
A funnel chart is a type of diagram used to represent stages or progress. In a funnel chart, each stage is represented by a horizontal bar, and the length of the bar corresponds to the quantity or value at that stage. The chart is widest at the top, where the quantity or value is greatest, and narrows down to represent the decrease at each subsequent stage.
Funnel charts are best for: Visual representation of the sales pipeline or data visualization of how a broad market is narrowed down into potential leads and a select group of customers.
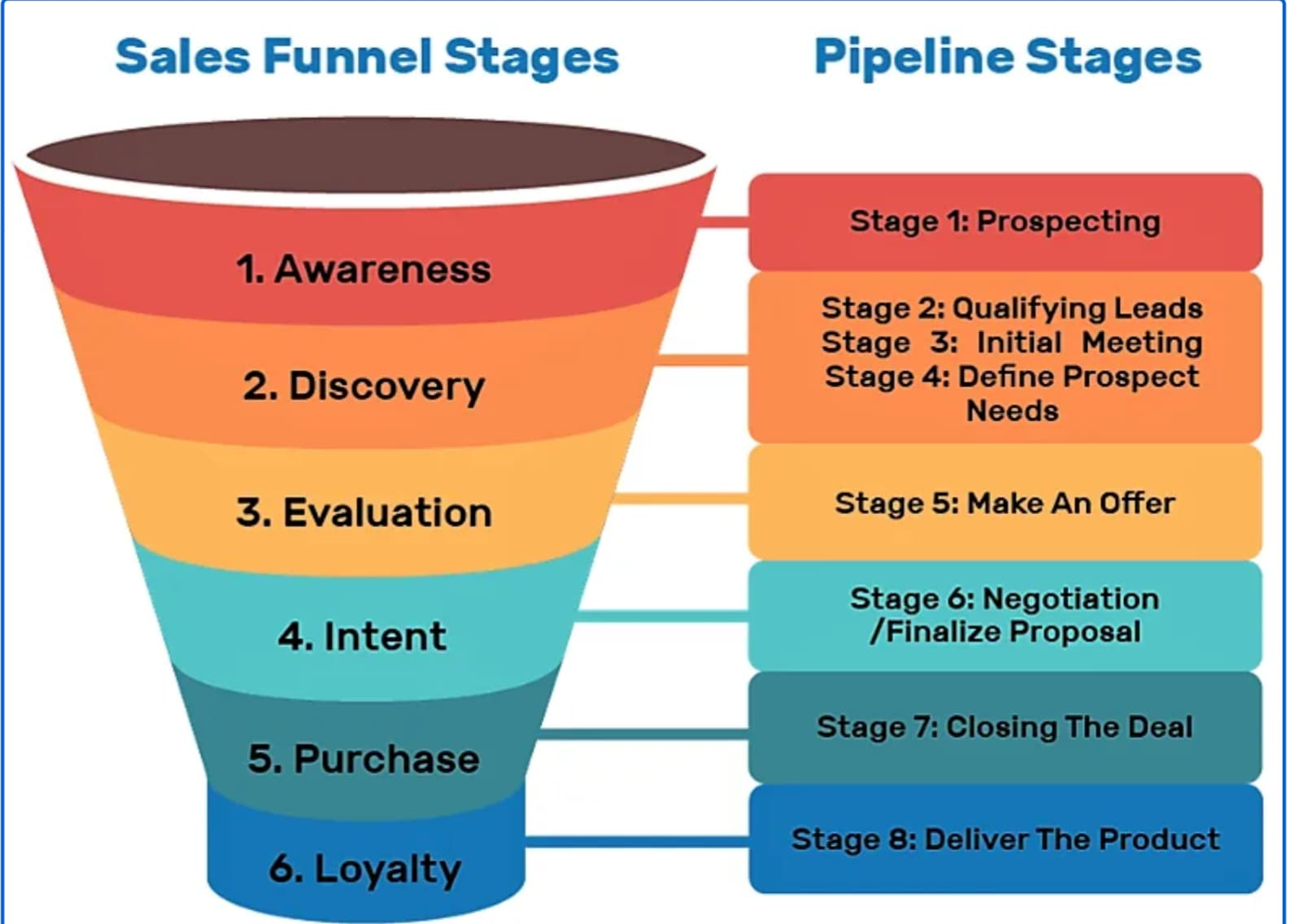
13. SIPOC diagrams
A SIPOC diagram is used in process improvement to represent the different components of a process. The acronym stands for Suppliers, Inputs, Process, Outputs, and Customers.
SIPOC diagrams are best for: Providing a high-level view of a process which helps visualize the sequence of events and their interconnections.
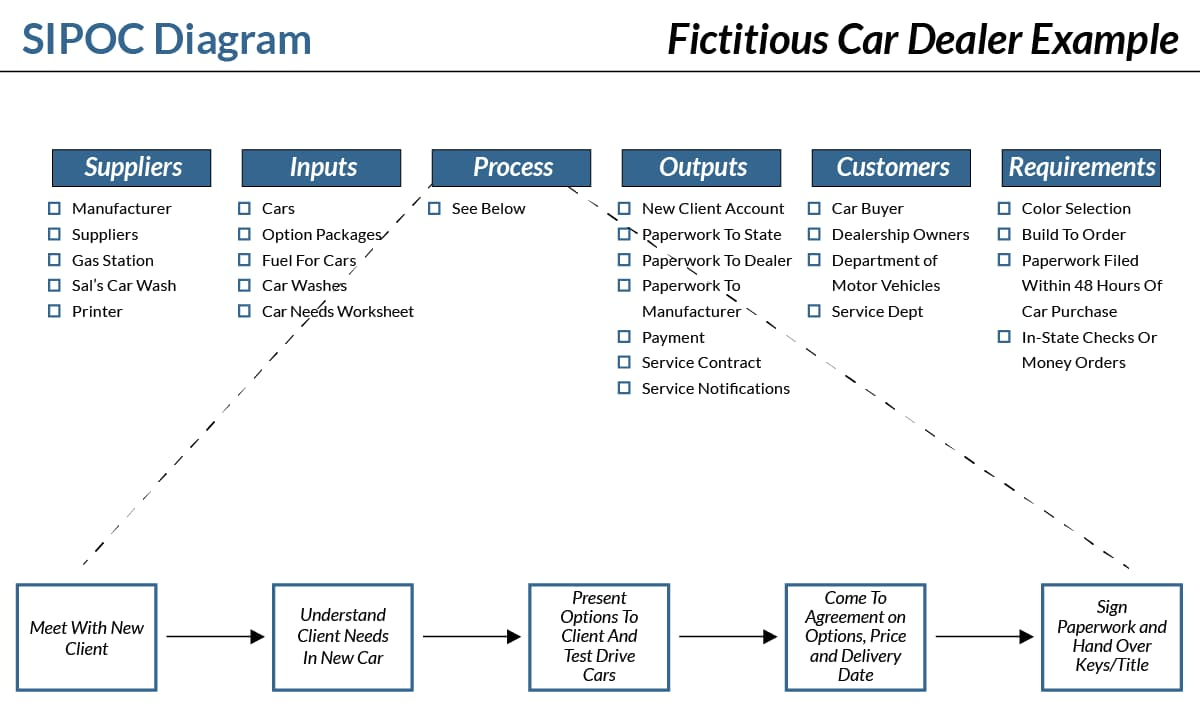
14. Swimlane diagrams
Swimlane diagrams are best for mapping out complex processes that involve multiple participants or groups.
Keep in mind that each lane (which can be either horizontal or vertical) in a swimlane diagram represents a different participant or group involved in the process. The steps or activities carried out by each participant are plotted within their respective lanes. This helps clarify roles and responsibilities as well as the sequence of events and points of interaction.
Swimlane diagrams are best for : Visualizing how different roles or departments interact and collaborate throughout a workflow or process.
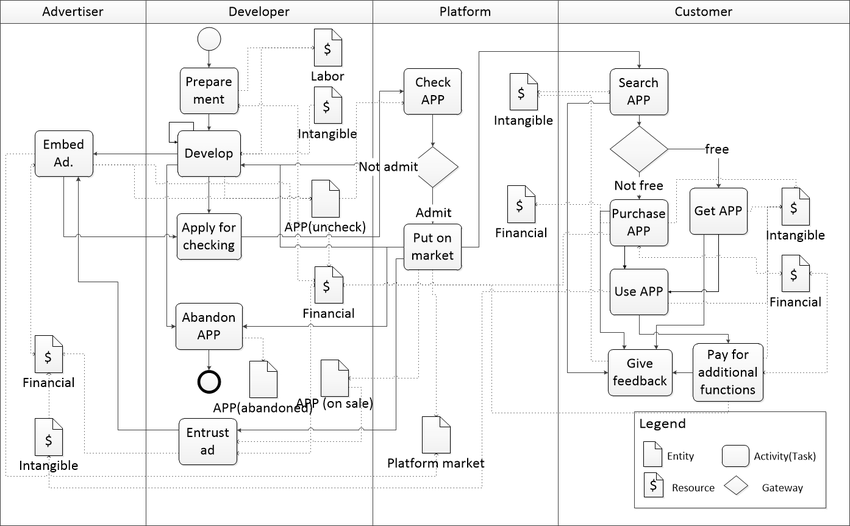
15. Mind maps
A mind map starts with a central idea and expands outward to include supporting ideas, related subtopics, concepts, or tasks, which can be further subdivided as needed. The branches radiating out from the central idea represent hierarchical relationships and connections between the different pieces of information in a mind map.
Mind maps are best for : Brainstorming, taking notes, organizing information, and visualizing complex concepts in a digestible format.
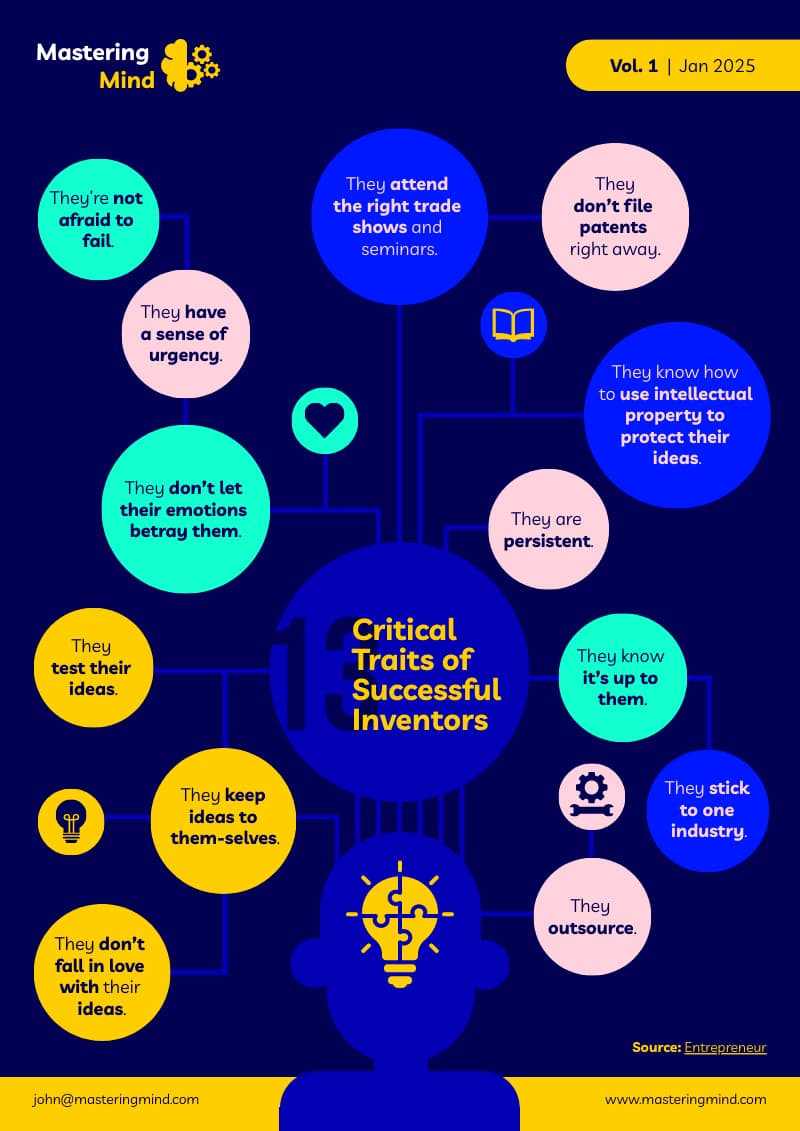
16. Scatter Plots
Scatter plots are used to compare data and represent the relationship between two variables. In a scatter plot, each dot represents a data point with its position along the x and y axes representing the values of two variables.
Scatter plots are best for : Observing relationships and trends between the two variables. These scatter plots are useful for regression analysis, hypothesis testing, and data exploration in various fields such as statistics, economics, and natural sciences.
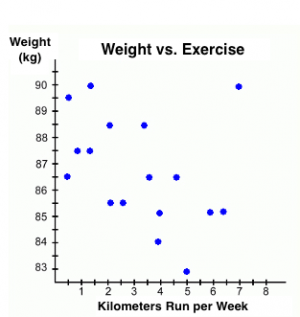
17. PERT chart
PERT (Project Evaluation Review Technique) charts are project management tools used to schedule tasks. Each node or arrow represents each task, while lines represent dependencies between tasks. The chart includes task duration and earliest/latest start/end times.
Construction project managers often use PERT charts to schedule tasks like design, site prep, construction, and inspection. Identifying the critical path helps focus resources on tasks that impact the project timeline.
PERT charts are best for : Visualizing the sequence of tasks, the time required for each task, and project timelines.
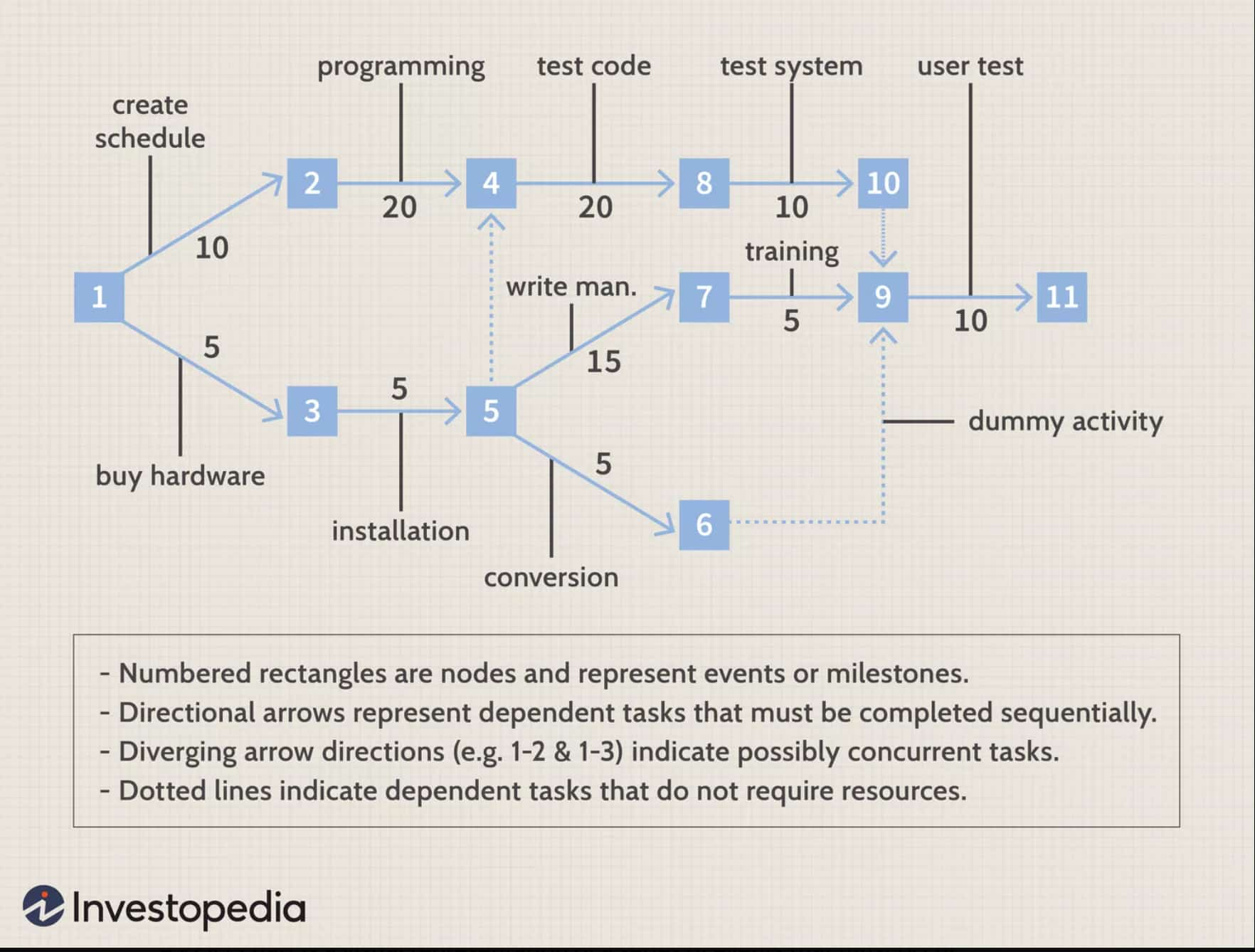
18. Network diagrams
A network diagram visually represents the relationships between elements in a system or project. In network diagrams, each node represents an element, such as a device in a computer network or a task in a project. The lines or arrows connecting the nodes represent the relationships or interactions between these elements.
Network diagrams are best for: Visually representing the relationships or connections between different elements in a system or a project. They are often used in telecommunications, computer networking, project management, and organization planning.
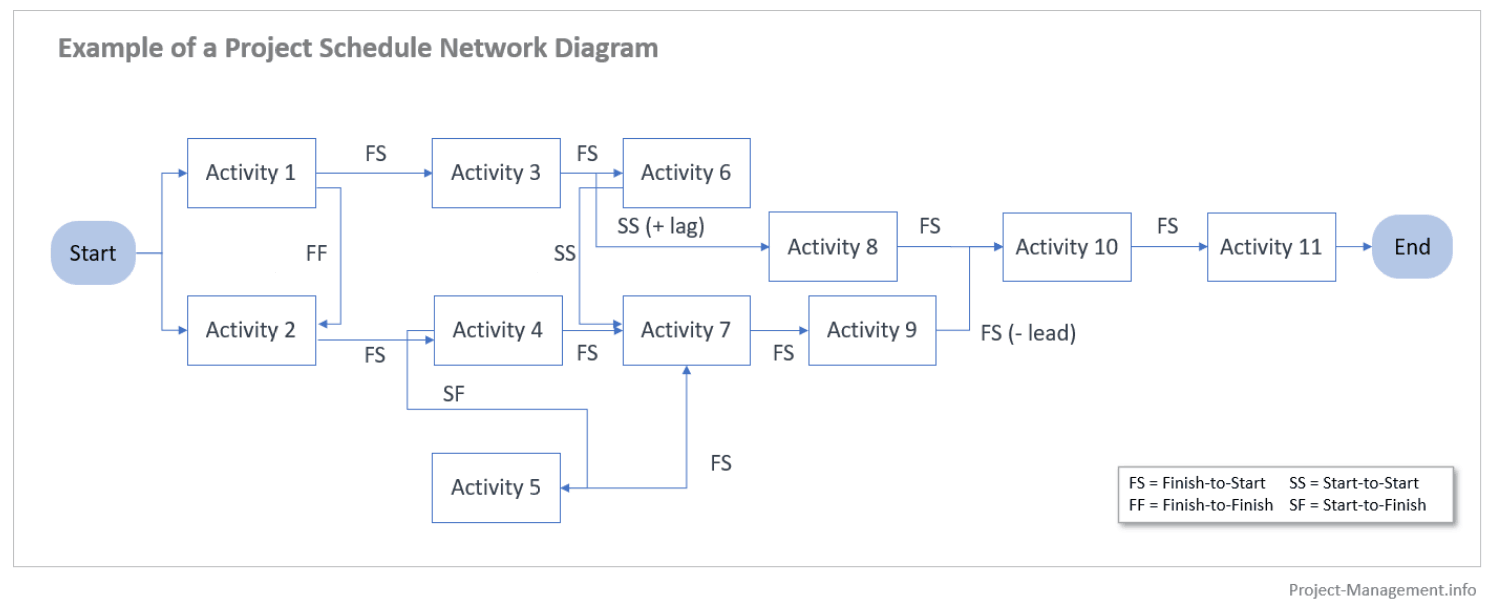
Choosing the right diagram starts with a good understanding of your audience
Understanding your audience’s needs, expectations, and context is necessary before designing diagrams. The best diagram is not the one that looks the most impressive but the one that communicates complex information most clearly and effectively to your intended audience.
Make professional diagrams for free with no design experience with Piktochart’s online diagram maker . Sign up for free .

Other Posts
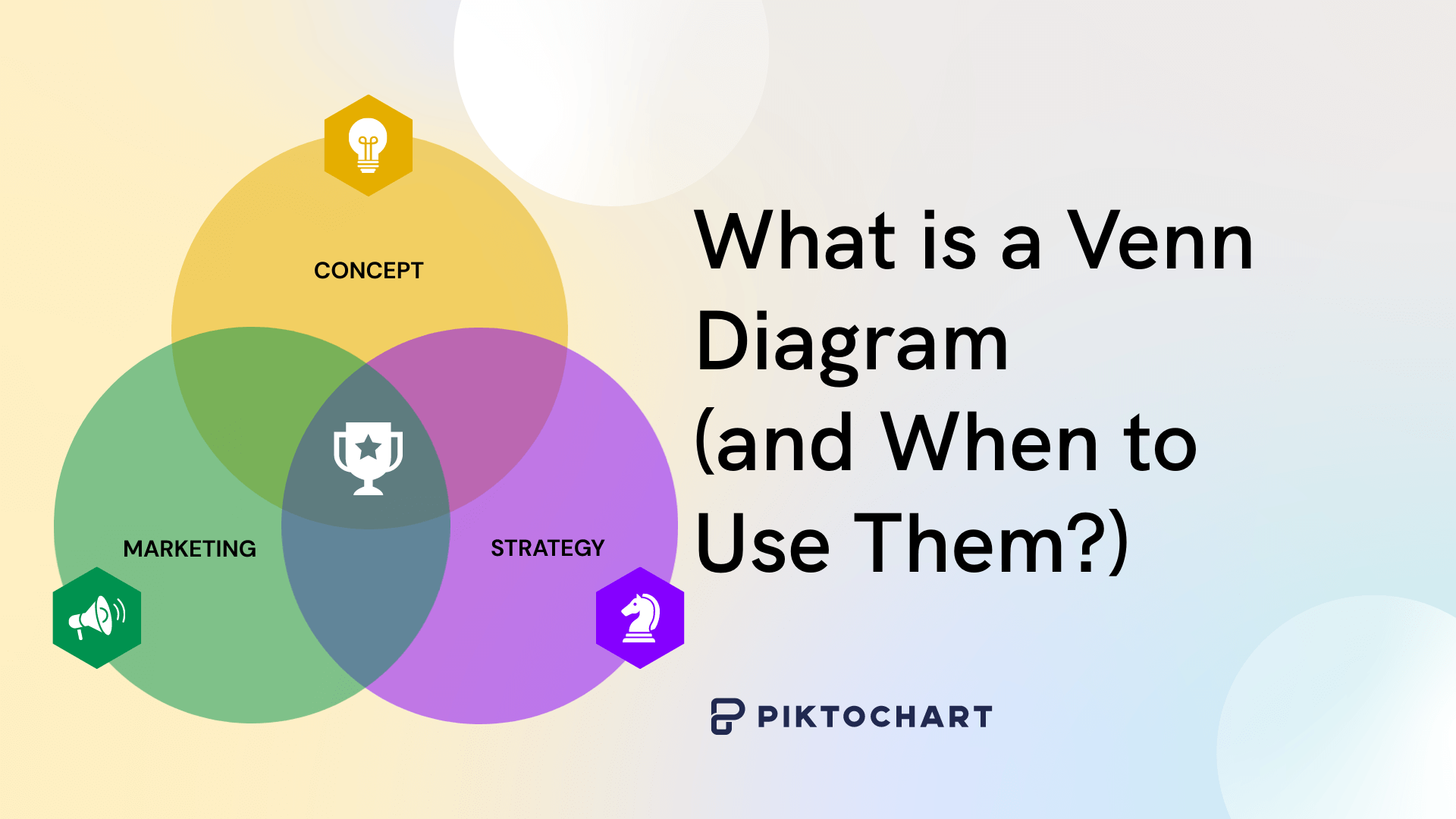
What is a Venn Diagram and When to Use Them?
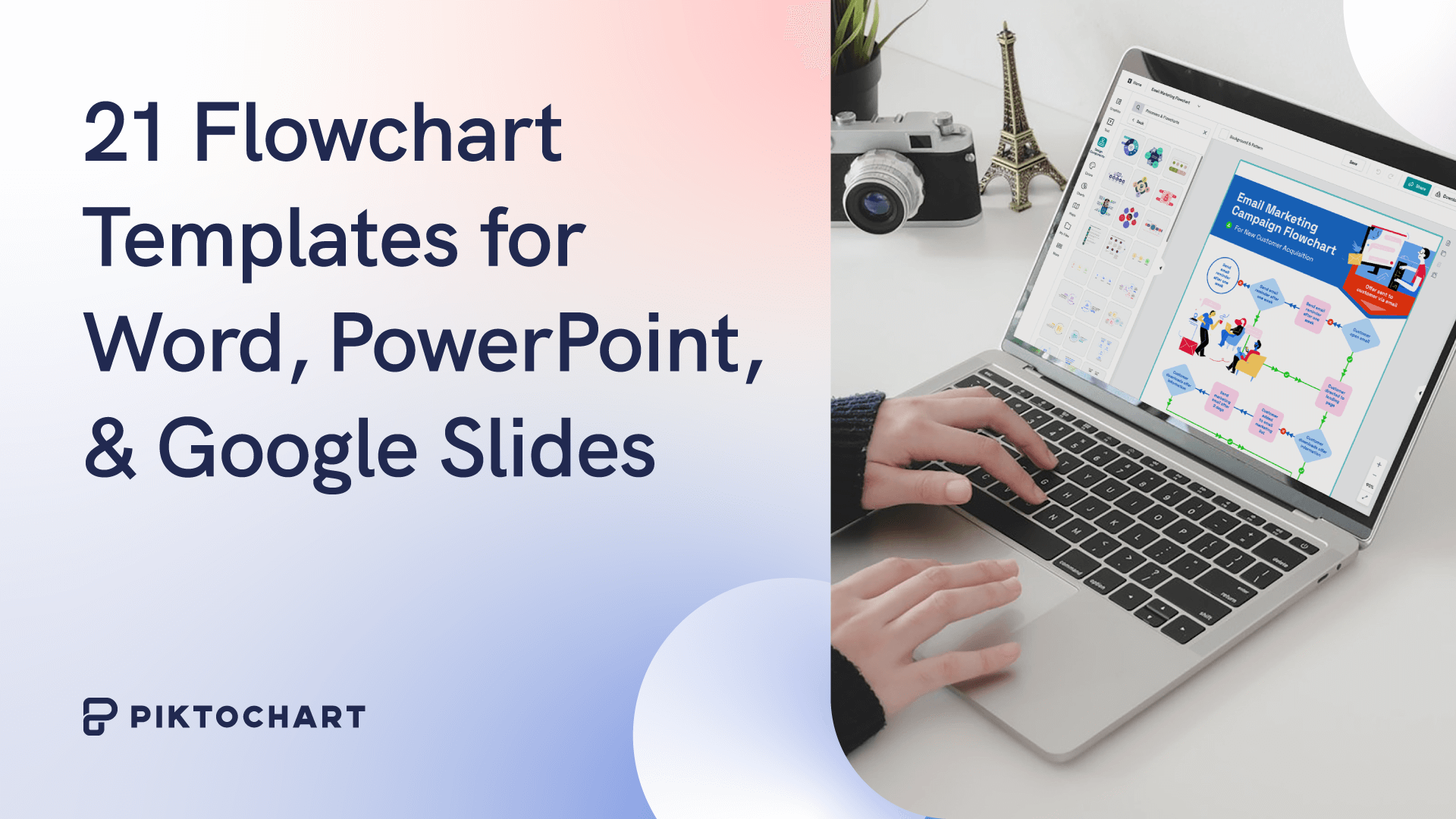
21 Flowchart Templates for Word, PowerPoint, and Google Slides
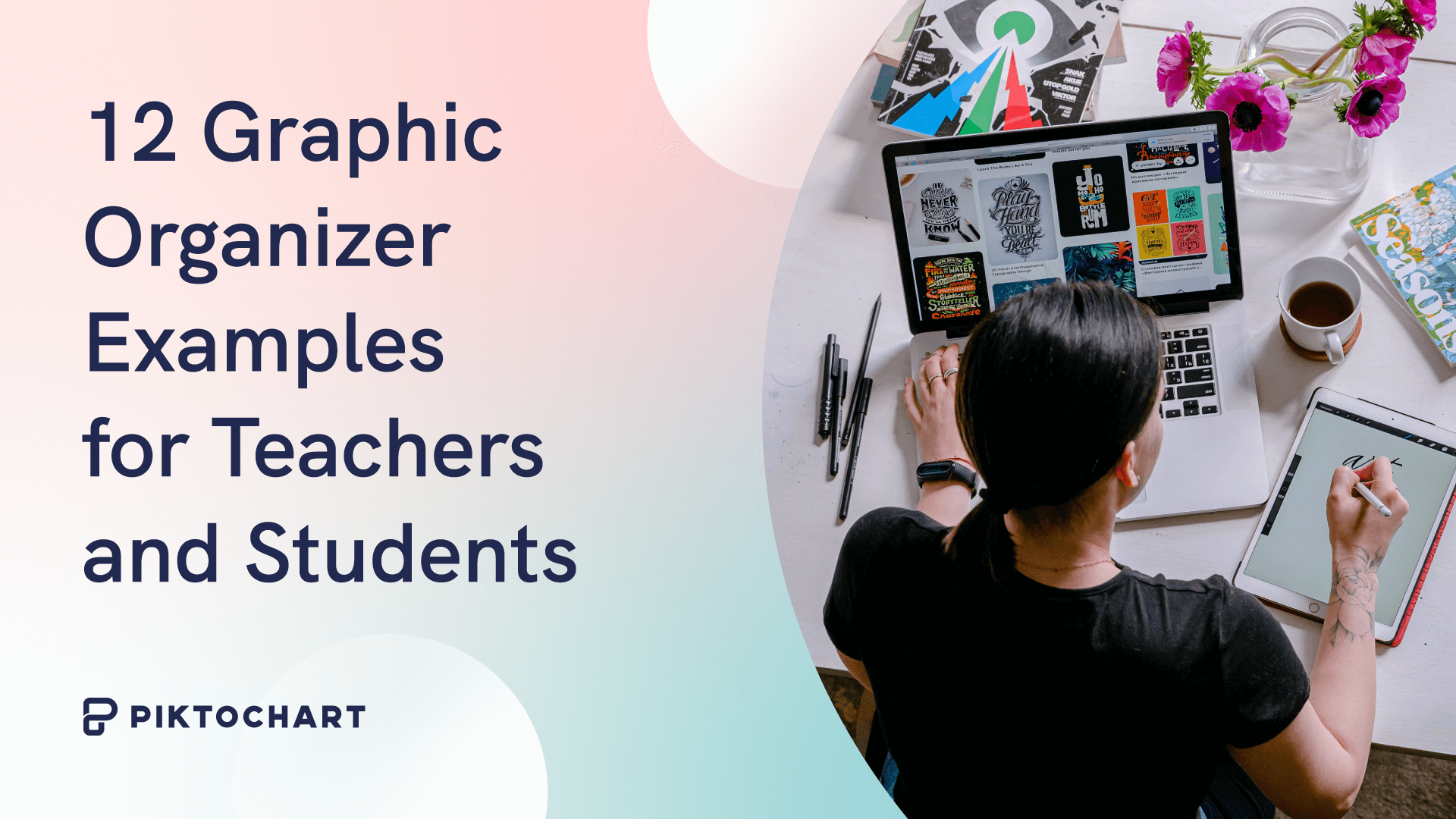
12 Graphic Organizer Examples for Teachers and Students
More From Forbes
Irrational decision making from data: nine mistakes your business could be making.

- Share to Facebook
- Share to Twitter
- Share to Linkedin
As a cofounder at Innowise Group with 20-year experience in IT, Pavel loves helping businesses grow through data analytics .
Data analytics has become a common thing for both large-scale corporations and local businesses that want to succeed at designing and promoting their products. It can help perform the magic of defining the needs and wishes of a target audience, show the path to optimized production and distribution and much more. When used incorrectly, though, data analytics can lead to poor decision making based on wrong assumptions.
Data analytics is a powerful tool, but there are several major mistakes businesses can make while using it that can lead to severe failures instead of overwhelming success. Here are nine things to watch out for while making data-based decisions.
1. Refusing To Create Data Lakes
Data lakes are the type of storage used for keeping raw, totally unprocessed data. Keeping such information allows businesses to build precise and retrospective predictive models based on historical data. It also enables the use of the original data with new processing and analytical tools. Without raw data stored, businesses have to rely on third-party data brokers that may share unsuitable data or the needed data at incommensurable costs.
2. Ignoring Visual Representations
Data represented in such vivid forms as graphs and dashboards empower decision makers to quickly and more effectively make conclusions without the need for assistance from data analysts. Using specialized dashboards, businesses can make decisions based on the visualized data, comparing it with other valuable insights acting like context indicators. This can lead to faster and more successful decision making and valuable advantages over competitors.
3. Forgetting About AI And ML
Machine learning (ML) and artificial intelligence (AI) are the key modern tools for data analytics. They can automatically process incoming data in real time at a pace unavailable to a team of professionals. Moreover, such tools often turn the tables on competitors by revealing overlooked trends and insights that a human can miss out on.
4. Lack Of Data Quality Control
Data quality control is a process that ensures you get representative and helpful data suitable for further analysis. Businesses that don’t keep track of data quality usually end up using unreliable data in their inner processes. This often leads to poor and uninformed decisions, which can be devastating. It’s vital to ensure your data is accurate, relatable and representative before using it for any purpose.
5. Ignoring Data Context
Certain events can lead to dramatic changes in acquired data. Particular events, even small ones like Elon Musk’s tweets about DogeCoin, can dramatically increase attention and demand for certain products. Data should be used with caution and always paired with its context to determine which events impact certain results. Sometimes, it’s a good idea to cut such influencing events out of the general data analytics model and work with them separately.
6. Neglecting Data Security
Data security is another important aspect of data management and analytics. Keeping data safe means securing business strategies and proprietary knowledge from being used by competitors. If businesses don’t take measures to secure their data, it’s like spending resources on something that they’ll give away to everyone around them.
7. Ignoring Data Ethics, Privacy And Legal Concerns
Despite being a powerful tool, data analytics brings a sufficient amount of risks. All the collected data must be obtained ethically, with user information kept in a depersonalized form and in compliance with local and global regulations concerning data gathering and analytics. Without it, a business can incur such damage as fines and reputational loss and even get shut down.
8. Not Controlling Confounding Variables
Confounding variables are the ones that impact both dependent and independent variables. When they occur, they can spoil the outcome of data analysis by bringing fake correlations and results to the table. If such cases aren’t tracked and managed, the resulting information can be inaccurate, and the decisions it’s based on are unlikely to be sound.
9. Not Being Transparent About Data Analytics And Decision Making
Data analytics and decision-making processes should be transparent for several reasons. First, it shows how ethical and secure data analytic processes are. Second, if there’s a flaw in the pipelines, employees and other stakeholders will be able to suggest fixes. Moreover, during decision making, if there’s a flaw in data analytics, it can also be pointed out and help prevent a business from taking poorly planned actions.
Final Thoughts
Despite being a powerful tool for decision making and planning, data and analytics tools should be approached very carefully. It can help a business to collaborate with a team of professionals that are highly experienced in the field.
Today’s businesses need data analytics to help gain a competitive advantage, but tomorrow, it might become a core survival point. Take it as it is but keep these considerations that can affect decisions in mind.
Forbes Technology Council is an invitation-only community for world-class CIOs, CTOs and technology executives. Do I qualify?
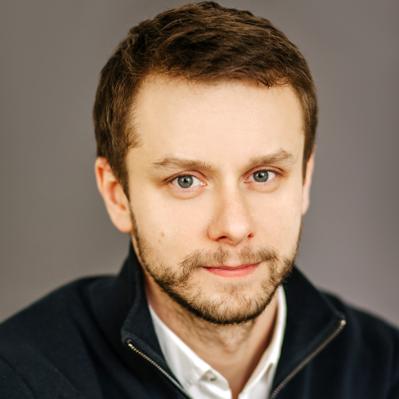
- Editorial Standards
- Reprints & Permissions
Thank you for visiting nature.com. You are using a browser version with limited support for CSS. To obtain the best experience, we recommend you use a more up to date browser (or turn off compatibility mode in Internet Explorer). In the meantime, to ensure continued support, we are displaying the site without styles and JavaScript.
- View all journals
- Explore content
- About the journal
- Publish with us
- Sign up for alerts
- Open access
- Published: 18 July 2024
Automated construction of cognitive maps with visual predictive coding
- James Gornet 1 &
- Matt Thomson ORCID: orcid.org/0000-0003-1021-1234 1
Nature Machine Intelligence volume 6 , pages 820–833 ( 2024 ) Cite this article
7189 Accesses
1 Citations
81 Altmetric
Metrics details
- Computational science
- Learning algorithms
A preprint version of the article is available at bioRxiv.
Humans construct internal cognitive maps of their environment directly from sensory inputs without access to a system of explicit coordinates or distance measurements. Although machine learning algorithms like simultaneous localization and mapping utilize specialized inference procedures to identify visual features and construct spatial maps from visual and odometry data, the general nature of cognitive maps in the brain suggests a unified mapping algorithmic strategy that can generalize to auditory, tactile and linguistic inputs. Here we demonstrate that predictive coding provides a natural and versatile neural network algorithm for constructing spatial maps using sensory data. We introduce a framework in which an agent navigates a virtual environment while engaging in visual predictive coding using a self-attention-equipped convolutional neural network. While learning a next-image prediction task, the agent automatically constructs an internal representation of the environment that quantitatively reflects spatial distances. The internal map enables the agent to pinpoint its location relative to landmarks using only visual information.The predictive coding network generates a vectorized encoding of the environment that supports vector navigation, where individual latent space units delineate localized, overlapping neighbourhoods in the environment. Broadly, our work introduces predictive coding as a unified algorithmic framework for constructing cognitive maps that can naturally extend to the mapping of auditory, sensorimotor and linguistic inputs.
Similar content being viewed by others
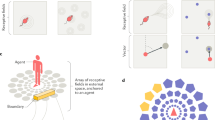
Neuronal vector coding in spatial cognition
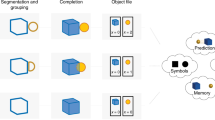
Capturing the objects of vision with neural networks
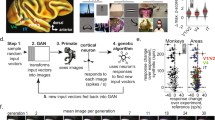
Visual prototypes in the ventral stream are attuned to complexity and gaze behavior
Space and time are fundamental physical structures in the natural world, and all organisms have evolved strategies for navigating space to forage, mate and escape predation 1 , 2 , 3 . In humans and other mammals, the concept of a spatial or cognitive map has been postulated to underlie spatial reasoning tasks 4 , 5 , 6 . A spatial map is an internal, neural representation of an animal’s environment that marks the location of landmarks, food, water and shelter, which can be queried for navigation and planning. The neural algorithms underlying spatial mapping are thought to generalize to other sensory modes to provide cognitive representations of auditory and somatosensory data 7 as well as to construct internal maps of more abstract information including concepts 8 , 9 , tasks 10 , semantic information 11 , 12 , 13 and memories 14 . Empirical evidence suggests that the brain uses common cognitive mapping strategies for spatial and non-spatial sensory information so that common mapping algorithms might exist that can map and navigate over not only visual but also semantic information and logical rules inferred from experience 7 , 8 , 15 . In such a paradigm, reasoning itself could be implemented as a form of navigation within a cognitive map of concepts, facts and ideas.
After the notion of a spatial or cognitive map emerged, the question of how environments are represented within the brain and how the maps can be learned from experience has been a central question in neuroscience 16 . Place cells in the hippocampus are neurons that are active when an animal transits through a specific location in an environment 16 . Grid cells in the entorhinal cortex fire in regular spatial intervals and likely track an organism’s displacement in the environment 17 , 18 . Yet, even with the identification of a substrate for the representation of space, the question of how a spatial map can be learned from sensory data has remained, and the neural algorithms that enable the construction of spatial and other cognitive maps remain poorly understood.
Empirical work in machine learning has demonstrated that deep neural networks can solve spatial navigation tasks as well as perform path prediction and grid cell formation 19 , 20 . Two studies 19 , 20 demonstrate that neural networks can learn to perform path prediction and that networks generate firing patterns that resemble the firing patterns of grid cells in the entorhinal cortex. Other studies 20 , 21 , 22 demonstrate navigation algorithms that require the environment’s map or that use firing patterns resembllng place cells in the hippocampus. These studies allow an agent to access environmental coordinates explicitly 19 or initialize a model with place cells that represent specific locations in an arena 20 . In machine learning and autonomous navigation, a variety of algorithms have been developed to perform mapping tasks, including simultaneous location and mapping (SLAM) and monocular SLAM algorithms 23 , 24 , 25 , 26 , as well as neural network implementations 27 , 28 , 29 . Yet, SLAM algorithms contain many specific inference strategies, like visual feature and object detection, that are specifically engineered for map building, wayfinding and pose estimation based on visual information. Whereas extensive research in computer vision and machine learning use video frames, these studies do not extract representations of the environment’s map 30 , 31 . A unified theoretical and mathematical framework for understanding the mapping of spaces based on sensory information remains incomplete.
Predictive coding has been proposed as a unifying theory of neural function where the fundamental goal of a neural system is to predict future observations given past data 32 , 33 , 34 . When an agent explores a physical environment, temporal correlations in sensory observations reflect the structure of the physical environment. Landmarks nearby one another in space will also be observed in temporal sequence. In this way, predicting observations in a temporal series of sensory observations requires an agent to internalize some implicit information about a spatial domain. Historically, Poincaré motivated the possibility of spatial mapping through a predictive coding strategy, where an agent assembles a global representation of an environment by gluing together information gathered through local exploration 35 , 36 . The exploratory paths together contain information that could, in principle, enable the assembly of a spatial map for both flat and curved manifolds. Indeed, extended Kalman filters 25 , 37 for SLAM perform a form of predictive coding by directly mapping visual changes and movement to spatial changes. However, extended Kalman filters, as well as other SLAM approaches, require intricate strategies for landmark size calibration, image feature extraction and models of the camera’s distortion, whereas biological systems can solve flexible mapping and navigation issues that engineered systems cannot. Yet, while the concept of predictive coding for spatial mapping is intuitively attractive, a major challenge is the development of algorithms that can glue together local sensory information gathered by an agent into a global, internally consistent environmental map. Connections between mapping and predictive coding in the literature have primarily focused on situations where an agent has explicit access to its spatial location as a state variable 38 , 39 , 40 . The problem of building spatial maps de novo from sensory data remains poorly understood.
Here we demonstrate that a neural network trained on a sensory predictive coding task can construct an implicit spatial map of an environment by assembling observations acquired along local exploratory paths into a global representation of a physical space within the network’s latent space. We analyse sensory predictive coding theoretically and demonstrate mathematically that solutions to the predictive sensory inference problem have a mathematical structure that can naturally be implemented by a neural network trained using backpropagation and comprising a ‘path encoder’, an internal spatial map and a ‘sensory decoder’. In such a paradigm, a network learns an internal map of its environment by inferring an internal geometric representation that supports predictive sensory inference. We implement sensory predictive coding within an agent that explores a virtual environment while performing visual predictive coding using a convolutional neural network with self-attention. Following network training during exploration, we find that the encoder network embeds images collected by an agent exploring an environment into an internal representation of space. Within the embedding, the distances between images reflect their relative spatial position, not object-level similarity between images. During exploratory training, the network implicitly assembles information from local paths into a global representation of space as it performs a next-image inference problem. Fundamentally, we connect predictive coding and mapping tasks, demonstrating a computational and mathematical strategy for integrating information from local measurements into a global self-consistent environmental model.
Mathematical formulation of spatial mapping as sensory predictive coding
In this Article, we aim to understand how a spatial map can be assembled by an agent that is making sensory observations while exploring an environment. Papers in the literature that study connections between predictive coding and mapping have primarily focused on situations where an agent has access to its ‘state’ or location in the environment 38 , 39 , 40 . Here we develop a theoretical model and neural network implementation of sensory predictive coding that illustrates why and how an internal spatial map can emerge naturally as a solution to sensory inference problems. The neural network is a feedforward deep neural network trained using backpropagation, or gradient descent, rather than Helmholtz machines 41 , 42 , which are commonly used in predictive coding. We first formulate a theoretical model of visual predictive coding and demonstrate that the predictive coding problem can be solved by an inference procedure that constructs an implicit representation of an agent’s environment to predict future sensory observations. The theoretical analysis also suggests that the underlying inference problem can be solved by an encoder–decoder neural network that infers spatial position based upon observed image sequences.
We consider an agent exploring an environment \({{\varOmega }}\subset {{\mathbb{R}}}^{2}\) , while acquiring visual information in the form of pixel valued image vectors \({{I}}_{x}\in {{\mathbb{R}}}^{m\times n}\) given an x ∈ Ω . The agent’s environment Ω is a bounded subset of \({{\mathbb{R}}}^{2}\) that could contain obstructions and holes. In general, at any given time t , the agent’s state can be characterized by a position x ( t ) and orientation θ ( t ) where x ( t ) and θ ( t ) are coordinates within a global coordinate system unknown to the agent.
The agent’s environment comes equipped with a visual scene, and the agent makes observations by acquiring image vectors \({{I}}_{{x}_{k}}\in {{\mathbb{R}}}^{m\times n}\) as it moves along a sequence of points x k . At every position x and orientation θ , the agent acquires an image by effectively sampling from an image the conditional probability distribution P ( I ∣ x k , θ k ) which encodes the probability of observing a specific image vector I when the agent is positioned at position x k and orientation θ k . The distribution P ( I ∣ x , θ ) has a deterministic and stochastic component where the deterministic component is set by landmarks in the environment while stochastic effects can emerge due to changes in lighting, background and scene dynamics. Mathematically, we can view P ( I ∣ x , θ ) as a function on a vector bundle with base space Ω and total space Ω × I (ref. 43 ). The function assigns an observation probability to every possible image vector for an agent positioned at a point ( x , θ ). Intuitively, the agent’s observations preserve the geometric structure of the environment: the spatial structure influences temporal correlations.
In the predictive coding problem, the agent moves along a series of points ( x 0 , θ 0 ), ( x 1 , θ 1 ), …, ( x k , θ k ) while acquiring images I 0 , I 1 , … , I k . The motion of the agent in Ω is generated by a Markov process with transition probabilities P ( x i +1 , θ i +1 ∣ x i , θ i ). Note that the agent has access to the image observations I i but not the spatial coordinates ( x i , θ i ). Given the set { I 0 , … , I k } the agent aims to predict I k +1 . Mathematically, the image prediction problem can be solved theoretically through statistical inference by (1) inferring the posterior probability distribution P ( I k +1 ∣ I 0 , I 1 . …, I k ) from observations. Then, (2) given a specific sequence of observed images { I 0 , … , I k }, the agent can predict the next image I k +1 by finding the image I k +1 that maximizes the posterior probability distribution P ( I k +1 ∣ I 0 , I 1 , …, I k ).
The posterior probability distribution P ( I k +1 ∣ I 0 , I 1 , …, I k ) is by definition
If we consider P ( I 0 , I 1 , … , I k , I k +1 ) to be a function of an implicit set of spatial coordinates ( x i , θ i ) where the ( x i , θ i ) provide an internal representation of the spatial environment, then we can express the posterior probability P ( I k +1 ∣ I 0 , I 1 , …, I k ) in terms of the implicit spatial representation
where in equation ( 1 ) the integration is over all possible paths {( x 0 , θ 0 ), …, ( x k , θ k )} in the domain Ω , for differentials d x = d x 0 , …, d x k and d θ = dθ 0 , …, d θ k . Equation ( 1 ) can be interpreted as a path integral over the domain Ω . The path integral assigns a probability to every possible path in the domain and then computes the probability that the agent will observe a next image I k given an inferred location ( x k +1 , θ k +1 ). In detail, term 1 assigns a probability to every discrete path {( x 0 , θ 0 ), …, ( x k , θ k )} ∈ Ω as the conditional likelihood of the path given the observed sequences of images { I 0 , … , I k }. Term 2 computes the probability that an agent at a terminal position x k moves to the position ( x k +1 , θ k +1 ), given the Markov transition function P ( x k +1 , θ k +1 ∣ x k , θ k ). Term 3 is the conditional probability that image I k +1 is observed, given that the agent is at position ( x k +1 , θ k +1 ).
Conceptually, the product of terms solves the next-image prediction problem in three steps. First, estimating the probability that an agent has traversed a particular sequence of points given the observed images; second, estimating the next position of the agent ( x k +1 , θ k +1 ) for each potential path; and third, computing the probability of observing a next image I k +1 given the inferred terminal location x k +1 of the agent. Critically, an algorithm that implements the inference procedure encoded in the equation would construct an internal but implicit representation of the environment as a coordinate system x , θ that is learned by the agent and used during the next-image inference procedure. The coordinate system provides an internal, inferred representation of the agent’s environment that is used to estimate future image observation probabilities. Thus, our theoretical framework demonstrates how an agent might construct an implicit representation of its spatial environment by solving the predictive coding problem.
The three-step inference procedure represented in the equation for P ( I k +1 ∣ I 0 , I 1 , … , I k ) can be directly implemented in a neural network architecture, as demonstrated in the Supplementary Information . The first term acts as an ‘encoder’ network that computes the probability that the agent has traversed a path {( x 0 , θ 0 ), …, ( x k , θ k )} given an observed image sequence I 0 , …, I k that has been observed by the network (Fig. 1b ). The network can then estimate the next position ( x k +1 , θ k +1 ) of the agent given an inferred location ( x k , θ k ) and apply a decoding network to compute P ( I k +1 ∣ x k +1 , θ k +1 ), while outputting the prediction I k +1 using a decoder. A network trained through visual experience must learn an internal coordinate system and representation x , θ that not only offers an environmental representation but also establishes a connection between observed images I j and inferred locations ( x j , θ j ).
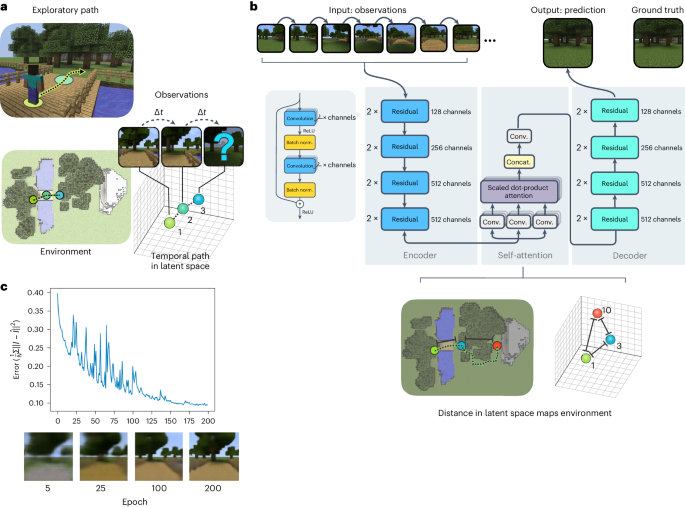
In predictive coding, a model predicts observations and updates its parameters using the prediction error. a , An agent traverses its environment by taking the most direct path to random positions. b , A self-attention-based encoder–decoder neural network architecture learns to perform predictive coding. A ResNet-18 convolutional neural network acts as an encoder; self-attention is performed with eight heads, and a corresponding ResNet-18 convolutional neural network performs decoding to the predicted image. c , The neural network learns to perform predictive coding effectively, achieving a mean-squared error of 0.094 between the actual and predicted images. Conv., convolution; concat., concatenation; norm., normalization.
A neural network performs predictive coding
Motivated by the implicit representation of space contained in the predictive coding inference problem, we developed a computational implementation of a predictive coding agent and studied the representation of space learned by that agent as it explored a virtual environment. We first create an environment with the Malmo environment in Minecraft 44 . The physical environment measures 40 × 65 lattice units and encapsulates three aspects of visual scenes: a cave provides a global visual landmark, a forest provides degeneracy between visual scenes, and a river with a bridge constrains how an agent traverses the environment (Fig. 1a ). An agent follows paths (Supplementary Fig. 5b,c ), determined by A * search to find the shortest path between randomly sampled positions, and receives visual images along every path.
To perform predictive coding, we construct an encoder–decoder convolutional neural network with a ResNet-18 architecture 45 for the encoder and a corresponding ResNet-18 architecture with transposed convolutions in the decoder (Fig. 1b ). The encoder–decoder architecture uses the U-Net architecture 46 to pass the encoded latent units into the decoder. Multi-headed attention 47 processes the sequence of encoded latent units to encode the history of past visual observations. The multi-headed attention has h = 8 heads. For the encoded latent units with dimension D = C × H × W , the dimension d of a single head is d = C × H × W / h for height H , width W and channels C .
The predictive coder approximates predictive coding by minimizing the mean-squared error between the actual observation and its predicted observation. The predictive coder trains on 82,630 samples for 200 epochs with gradient descent optimization with Nesterov momentum 48 , a weight decay of 5 × 10 −6 and a learning rate of 10 −1 adjusted by OneCycle learning-rate scheduling 49 . The optimized predictive coder has a mean-squared error between the predicted and actual images of 0.094 and a good visual fidelity (Fig. 1c ).
Predictive coding network constructs an implicit spatial map
We show that the predictive coder creates an implicit spatial map by demonstrating it recovers the environment’s spatial position and distance. We encode the image sequences using the predictive coder’s encoder to analyse the encoded sequence as the predictive coder’s latent units. To measure the positional information in the predictive coder, we train a neural network to predict the agent’s position from the predictive coder’s latent units (Fig. 1a ). The neural network’s prediction error
indirectly measures the predictive coder’s positional information. To provide comparative baselines, we construct a position prediction model. To provide a lower bound for the prediction error, we construct a model that gives the agent’s actual position with small additive Gaussian noise:
such that ε ∼ 𝒩 (0, σ ) indicates noise ε that is distributed from a Gaussian distribution with zero mean and standard deviation σ . To compare the predictive coder to the baselines, we compare the prediction error histograms (Fig. 2b ).
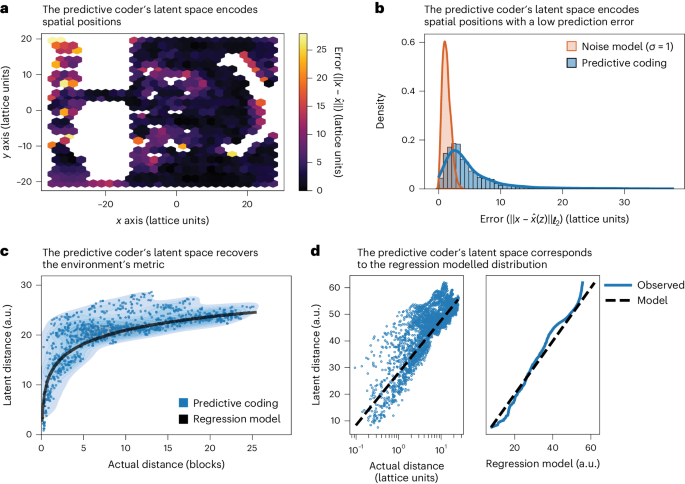
a , The predictive coder’s latent space encodes accurate spatial positions. A neural network predicts the spatial location from the predictive coding’s latent space. A heatmap of the prediction errors between the actual position and the predictive coder’s predicted positions show a low prediction error. b , The histogram of prediction errors of positions from the predictive coder’s latent space show a low prediction error. As a baseline (Noise model ( σ = 1 lattice unit)), actual positions with a small noise displacement give an error model. c , Predictive coding’s latent distances recover the environment’s spatial metric. Sequential visual images are mapped to the neural network’s latent space, and the latent space distances ( ℓ 2 ) are plotted with physical distances onto a joint density plot. A nonlinear regression model \(\left\Vert z-z^{\prime} \right\Vert =\alpha \log \left\Vert x-x^{\prime} \right\Vert +\beta\) is shown as a baseline. d , A correlation plot and a quantile–quantile plot show the overlap between the empirical and model distributions.
The predictive coder encodes the environment’s spatial position to a low prediction error (Fig. 2d ). The predictive coder has a mean error of 5.04 lattice units and >80% of samples have an error <7.3 lattice units. The additive Gaussian model with σ = 4 has a mean error of 4.98 lattice units and >80% of samples with an error <7.12 lattice units.
We show the predictive coder’s latent space recovers the local distances between the environment’s physical positions. For every path that the agent traverses, we calculate the local pairwise distances in physical space and in the predictive coder’s latent space with a neighbourhood of 100 time points. To determine whether latent space distances correspond to physical distances, we calculate the joint density between latent space distances and physical distances (Fig. 2c ). We model the latent distances by fitting the physical distances with additive Gaussian noise to a logarithmic function:
The modelled distribution is concentrated with the predictive coder’s distribution (Fig. 2d ) with a Pearson correlation coefficient of 0.827 and a Kullback–Leibler divergence \(({{\mathbb{D}}}_{{{{\rm{KL}}}}}(\,{p}_{{{{\rm{PC}}}}}\parallel {p}_{{{{\rm{model}}}}}))\) of 0.429 bits.
Predictive coding network learns spatial proximity not image similarity
In the previous section, we show that a neural network that performs predictive coding learns an internal representation of its physical environment within its latent space. Here we demonstrate that the prediction task itself is essential for spatial mapping. Prediction forces a network to learn spatial proximity and not merely image similarity. Many frameworks, including principal components analysis, IsoMap 50 and auto-encoder neural networks can collocate images by visual similarity. While similar scenes might be proximate in space, similar scenes can also be spatially divergent. For example, the virtual environment we constructed has two different ‘forest’ regions that are separated by a lake. Thus, the two forest environments might generate similar images but are actually each closer to the lake region than to one another (Fig. 1a ).
To demonstrate the central role for prediction in mapping, we compared the latent representation of images generated by the predictive coding network to a representation learned by an auto-encoder. The auto-encoder network has a similar architecture to the predictive encoder but encodes a single image observation in a latent space and decodes the same observations. As the auto-encoder only operates on a single image, rather than a sequence, the auto-encoder learns an embedding based on image proximity not underlying spatial relationships. As with the predictive coder, the auto-encoder (Fig. 3a ) trains to minimize the mean-squared error between the actual image and the predicted image on 82,630 samples for 200 epochs with gradient descent optimization with Nesterov momentum, a weight decay of 5 × 10 −6 and a learning rate of 10 −1 adjusted by the OneCycle learning-rate scheduler. The auto-encoder has a mean-squared error of 0.039 and a high visual fidelity.
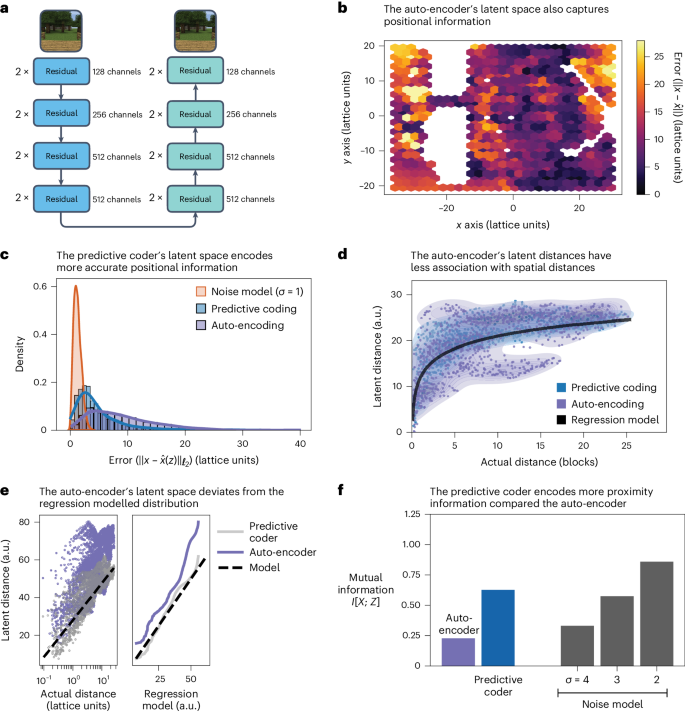
a , An auto-encoding neural network compresses visual images into a low-dimensional latent vector and reconstructs the image from the latent space. The auto-encoder trains on visual images from the environment without any sequential order. b , c , Auto-encoding encodes lower resolution in positional information. A neural network predicts the spatial location from the auto-encoding’s latent space ( b ). A heatmap of the prediction errors between the actual position and the auto-encoder’s predicted positions show a higher prediction error compared to the predictive coder. Auto-encoding captures less positional information compared to predictive coding ( c ). The histogram shows the prediction errors of positions from the latent space of both the auto-encoder and the predictive coder. d , Latent distances, however, show a weaker relationship with physical distances, as the joint histogram between physical and latent distances is less concentrated. e , A correlation plot and a quantile–quantile plot show a lower correlation and a lower density overlap between the empirical and model distributions. f , Predictive coding’s latent units communicate more fine-grained spatial distances, whereas auto-encoding communicates broad spatial regions. Joint density plots show the association between latent distances and physical distances for both predictive coding and auto-encoding. Predictive coding’s latent distances increase with spatial distances, with a higher concentration compared to auto-encoding.
The predictive coder encodes a higher resolution and a more accurate spatial map in its latent space than the auto-encoder. As with the predictive coder, we train an auxiliary neural network to predict the agent’s position from the auto-encoder’s latent units (Fig. 3b ). The neural network’s prediction error indirectly measures the auto-encoder positional information. For greater than 80% of the auto-encoder’s points, its prediction error is less than 13.1 lattice units, as compared to the predictive coder that has >80% of its samples below a prediction error of 7.3 lattice units (Fig. 3c ).
We also show that the predictive coder recovers the environment’s spatial distances with finer resolution compared to the auto-encoder. As with the predictive coder, we calculate the local pairwise distances in physical space and in the auto-encoder’s latent space, and we generate the joint density between the physical and latent distances (Fig. 3d ). Compared to the predictive coder’s joint density, the auto-encoder’s latent distances increase with the agent’s physical distance. The auto-encoder’s joint density shows a larger dispersion compared to the predictive coder’s joint density, indicating that the auto-encoder encodes spatial distances with higher uncertainty.
We can quantitatively measure the dispersion in the auto-encoder’s joint density by calculating mutual information of the joint density (Fig. 3e )
The auto-encoder has a mutual information of 0.227 bits, while the predictive coder has a mutual information of 0.627 bits. As a comparison, positions with additive Gaussian noise having a standard deviation σ of 2 lattice units have a mutual information of 0.911 bits. The predictive coder encodes 0.400 additional bits of distance information to the auto-encoder. The predictive coder’s additional distance information of 0.400 bits exceeds the auto-encoder’s distance information of 0.227 bits, which indicates the temporal dependencies encoded by the predictive coder capture more spatial information compared to visual similarity.
Predictive coding network maps visual degenerate environments, whereas auto-encoding cannot
The sequential prediction task is beneficial for spatial mapping: the predictive coder captures more accurate spatial information compared to the auto-encoder, and the predictive coder’s latent distances have a stronger correspondence to the environment’s metric. However, it is unclear whether predictive coding is necessary (as opposed to beneficial) to recover an environment’s map; an auto-encoder may still recover the environment’s map. In this section, we demonstrate that predictive coding is necessary for recovering an environment’s map. First, we show empirically that there exist environments that auto-encoding cannot recover. Second, we provide insight into why the auto-encoder fails with a theorem showing that auto-encoding cannot recover many environments—specifically, environments with visually similar yet spatially different locations.
In the previous sections, the agent explores a natural environment with forest, river and cave landmarks. While this environment models exploration in outdoor environments, the lack of controlled visual scenes complicates the interpretation of the operation of the predictive coder and auto-encoder. We introduce a circular corridor (Fig. 4a ) to introduce visual scenes that are visually identical—rather than visually similar—yet spatially different. Specifically, the rooms appear clockwise as red, green, red, blue and yellow; there exist two distinct red rooms. The two distinct red rooms permit answering two questions: (1) Can the predictive coder and auto-encoder recover the map for environments with visual symmetry? (2) Does the predictive coder recover a global map or a relative map? In other words, does the predictive coder recover the circular corridor’s geometry, or does it learn a linear hallway?
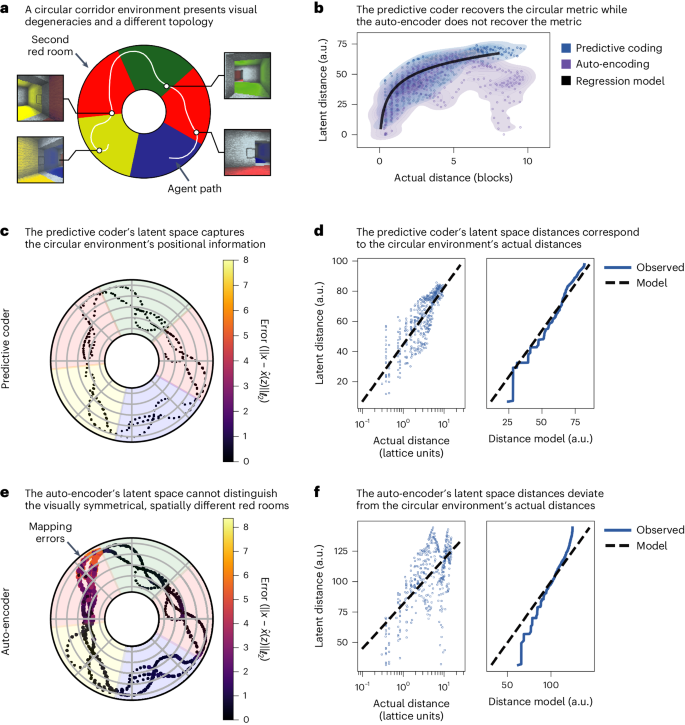
a , An agent traverses a circular environment with two visually identical red rooms; this provides visually similar yet spatially different locations. b , The predictive coder’s latent distances show a correspondence with the circular environment’s metric, while the auto-encoder’s latent distances show little correlation. c , Similar to Figs. 2 and 3 , a different neural network measures the predictive coder’s spatial information by predicting the agent’s location from the predictive coder’s latent space. The predictive coder’s latent space demonstrates a low prediction error. d , Similar to Figs. 2 and 3 , the nonlinear regression measures the correspondence between the latent distances \(\parallel z-z^{\prime}\parallel\) and the actual distances \(\parallel {x}-{x}^{\prime}\parallel\) with the model \(\parallel{z-z}^{\prime}\parallel=\alpha\log\parallel{x-x}^{\prime}\parallel+\beta .\) The correlation plot (left) with the nonlinear regression model show a strong correlation between the predictive coder’s latent distances and the environment’s actual distances ( r = 0.827). The quantile–quantile plot (right) between the predictive coder’s latent distances and the regression model show high overlap ( \({{\mathbb{D}}}_{{{{\rm{KL}}}}}({p}_{{{{\rm{PC}}}}}\parallel {p}_{{{{\rm{model}}}}})=0.250\) ). e , Without any past information, the auto-encoder cannot distinguish the two different red rooms and produces a high prediction error in these locations. f , The correlation plot (left) with the nonlinear regression model show little correlation between the auto-encoder’s latent distances and the environment’s actual distances ( r = 0.288). The quantile–quantile plot (right) between the auto-encoder’s latent distances and the regression model show little overlap ( \({{\mathbb{D}}}_{{{{\rm{KL}}}}}({p}_{{{{\rm{PC}}}}}\parallel {p}_{{{{\rm{model}}}}})=3.806\) ).
Similar to previous sections, we train a neural network (or a predictive coder) to perform predictive coding while traversing the circular corridor. In addition, we train a neural network (or an auto-encoder) to perform auto-encoding. The auto-encoder fails to recover spatial information in areas with visual degeneracy: it maps the two distinct red rooms to the same location (Fig. 4e ). In Fig. 4e , the auto-encoder predicts the images in the left red room to locations in the right red room—whereas locations with distinct visual scenes (such as the yellow and blue rooms) show a low prediction error (mean error \({\left\Vert x-\hat{x}\right\Vert }_{{\ell }_{2}}=5.004\) lattice units). In addition, the auto-encoder’s latent distances do not separate the different red rooms in latent space—whereas the predictive coder separates the two red rooms (Fig. 4b ). Moreover, the predictive coder demonstrates a low prediction error throughout, including the two visually degenerate red rooms (mean error \({\left\Vert x-\hat{x}\right\Vert }_{{\ell }_{2}}=0.071\) lattice units) (Fig. 4c ).
Moreover, we measure the relationship between the predictive coder’s (and auto-encoder’s) metric and the environment’s metric by fitting a regression model (Fig. 4b ),
between the predictive coder’s (and auto-encoder’s) latent distances ( \(\left\Vert z-z^{\prime} \right\Vert\) ) and the environment’s physical distances ( \(\left\Vert x-x^{\prime} \right\Vert\) ). Compared to the natural environment, the auto-encoder’s latent distances show more deviation from the environment’s spatial distances, whereas the predictive coder’s latent distances maintain a correspondence with spatial distances. For the predictive coder, the latent metric recovers spatial metric quantitatively: the correlation plot (Fig. 4d , left) shows a high correlation ( r = 0.827) between the latent and spatial distances, and the quantile–quantile plot (Fig. 4d , right) shows a high overlap between the regression model and the observed latent distances ( \({{\mathbb{D}}}_{{{{\rm{KL}}}}}({p}_{{{{\rm{PC}}}}}\parallel {p}_{{{{\rm{model}}}}})=0.250\) ). The auto-encoder’s latent metric, conversely, does not recover the spatial metric: the correlation plot (Fig. 4f , left) shows a low correlation ( r = 0.288) between the latent and spatial distances, and the quantile–quantile plot (Fig. 4f , right)) shows a low overlap between the regression model and the observed latent distances ( \({{\mathbb{D}}}_{{{{\rm{KL}}}}}({p}_{{{{\rm{PC}}}}}\parallel {p}_{{{{\rm{model}}}}})=3.806\) ).
As shown in Fig. 4 , the auto-encoder cannot recover the spatial map of the circular corridor—whereas the predictive coder can recover the map. Here we show that auto-encoders cannot recover the environment’s map for any environment with visual degeneracy, not just the circular corridor. To show that the auto-encoder cannot learn the environment’s map, we show that any statistical estimator cannot learn the environment’s map from stationary observations. For clarity and brevity, we will provide a proof sketch on a lattice environment X , a closed subset of \({{\mathbb{Z}}}^{2}\) .
Consider an environment X , a closed subset of the lattice \({{\mathbb{Z}}}^{2}\) with a function \(x \,\stackrel{f}{\mapsto} \,I\) that gives an image \({I}_{x}=f(x)\subset {{\mathbb{R}}}^{D}\) for the image dimension D and for each position x ∈ X . Let the environment’s observations be degenerate such that
There exists no decoder \(I\,\stackrel{d}{\mapsto}\,x\) that satisfies
The proof proceeds as a consequence that a function has no left inverse if and only if it is not one-to-one. Suppose there exists a decoder \(I\,\stackrel{d}{\mapsto}\,x\) that satisfies
which is a contradiction, as required.
Because Theorem 1 demonstrates there exists no decoder for a visually degenerate environment with stationary observations, an auto-encoder cannot recover a visually degenerate environment; the auto-encoder’s failure arises because two locations with the same observation cannot be discriminated.
Corollary 1
Consider an auto-encoder \({g\,=\,{\mathrm{dec}}\,\circ}\) enc with an encoder \(I \,\stackrel{\rm{enc}}{\mapsto}\, z\) and decoder \(z \,\stackrel{{{dec}}}{\mapsto}\, I\) that compresses images into a latent space \(z\in {{\mathbb{R}}}^{L}\) for the latent dimension L . There exists no decoder \(z \,\stackrel{h}{\mapsto}\, x\) that satisfies
Consider the decoder \({d\,=\,{h}\,\circ}\,{\mathrm{enc}}:\,{I}\rightarrow{x}\) . By Theorem 1, this decoder cannot satisfy
as required.
Predictive coding generates units with localized receptive fields that support vector navigation
In the previous section, we demonstrate that the predictive coding neural network captures spatial relationships within an environment containing more internal spatial information than can be captured by an auto-encoder network that encodes image similarity. Here we analyse the structure of the spatial code learned by the predictive coding network. We demonstrate that each unit in the neural network’s latent space activates at distinct, localized regions—akin to place fields in the mammalian brain—in the environment’s physical space (Fig. 5a ). These place fields overlap, and their aggregate covers the entire physical space. Each physical location is represented by a unique combination of overlapping regions encoded by the latent units. This combination of overlapping regions recovers the agent’s current physical position. Furthermore, given two physical locations, there now exist two distinct combinations of overlapping regions in latent space. Vector navigation is the representation of the vector heading to a goal location from a current location 51 . We show that overlapping regions (or place fields) can give a heading from a current location to a goal location. Specifically, a linear decoder recovers the vector to a goal location from a starting location by taking the difference in place fields, which supports vector navigation (Supplementary Fig. 1) . Traditionally, other studies 51 consider grid cell-supported vector navigation, whereas we only consider vector navigation using place cells.
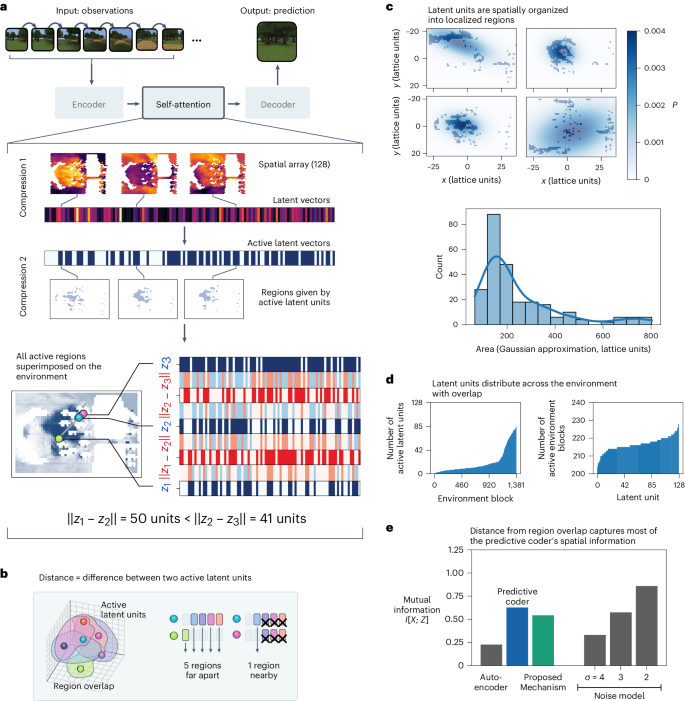
a , When encoding past images for predictive coding, the self-attention module generates latent vectors. Each continuous unit in these latent vectors activates in concentrated, localized regions in physical space. These continuous units can be thresholded to generate a binary vector determining whether each unit is active. Each latent unit covers a unique region, and each physical location gives a unique combination of these overlapping regions. As an agent moves away from its original location, the combination of overlapping regions gradually deviates from its original combinations. This deviation, as measured by Hamming distance, correlates with physical distance. b , Distance is given by the difference in the latent units’ overlapping regions. Two nearby locations have small deviations in overlap (right) while two distant locations have large deviations (middle). c , Latent units are spatially organized into localized regions. The active latent units are approximated by a two-dimensional Gaussian distribution (bottom) to measure the latent unit’s localization (top). The latent units’ Gaussian approximations are highly localized, with a mean area of 254.6 for densities P ≥ 0.0005. d , Latent units distributed across the environment. The number of latent units was calculated as each lattice block in the environment (left), and the number of lattice blocks was calculated for each active unit (right). The latent units provide a unique combination for 87.6% of the environment, and their aggregate covers the entire environment. e , distance from the region overlap captures most of the predictive coder’s spatial information. We calculate the distance for every pair of active latent vectors and their respective physical Euclidean distances as a joint distribution. The proposed mechanism captures a majority of the predictive coder’s spatial information, as the proposed mechanism’s mutual information (0.542 bits) compares to the predictive coder’s mutual information (0.627 bits).
To support this proposed mechanism, we first demonstrate the neural network generates place fields. In other words, units from the neural network’s latent space produce localized regions in physical space. To determine whether a latent unit is active, we threshold the continuous value with its 90th-percentile value. The agent has a head direction that varies to ensure the regions are stable across all head directions. To measure a latent unit’s localization in physical space, we fit each latent unit distribution, with respect to physical space, to a two-dimensional Gaussian distribution (Fig. 5c , top), defined by
for the covariance matrix Σ and the mean vector μ . We measure the area of the ellipsoid given by the Gaussian approximation where P ≥ 0.0005 (Fig. 5c , bottom). The area of the latent unit approximation measures how localized a unit is compared to the environment’s area, which measures 40 × 65 = 2,600 lattice units. The latent unit approximations have a mean area of 254.6 lattice units (9.79% of the environment) and 80% of the areas are <352.6 lattice units (13.6% of the environment).
The units in the neural network’s latent space provide a unique combinatorial code for each spatial position. The aggregate of latent units covers the environment’s entire physical space. At each lattice block in the environment, we calculate the number of active latent units (Fig. 5d , left). The number of active latent units is different in 87.6% of the lattice blocks. Every lattice block has at least one active latent unit, which indicates the aggregate of the latent units covers the environment’s physical space. Moreover, to ensure the regions remain stable across shifting landmarks, the environment’s trees were removed and randomly redistributed in the environment (Supplementary Fig. 5a,b ). The regions remain stable after changing the tree landmarks, with a Jaccard index ( ∣ S new ∩ S old ∣ / ∣ S new ∪ S old ∣ ) (or the intersection over union of new regions S new and old regions S old ) of 0.828.
Lastly, we demonstrate that the neural network can measure physical distances and could perform vector navigation—representing the vector heading from a current location to a goal location—by comparing the combinations of overlapping regions in its latent space. We first determine the active latent units by thresholding each continuous value by its 90th-percentile value. At each position, we have a 128-dimensional binary vector that gives the overlap of 128 latent units. We take the bitwise difference z 1 − z 2 between the overlapping codes z 1 and z 2 at two varying positions x 1 and x 2 with the vector displacement x 1 − x 2 (Supplementary Fig. 1a ). We then fit a linear decoder from the code z 1 − z 2 to the vector displacement x 1 − x 2 ,
for weight W and bias b . The predicted distance error \(\left\Vert r-\hat{r}\right\Vert\) and the predicted direction error \(\Vert \theta -\hat{\theta }\Vert\) are decomposed from the predicted displacement \({\hat{x}}_{1}-{\hat{x}}_{2}\) . The linear decoder has a low prediction error for distance (<80%, 12.49 lattice units; mean 7.89 lattice units) and direction (<80%, 48.04°; mean 30.6°) (Supplementary Fig. 1b,c ). The code z 1 − z 2 is highly correlated with direction θ and distance r with Pearson correlation coefficients 0.924 and 0.718, respectively (Supplementary Fig. 1d ).
We can measure the correspondence between the bitwise distance ∣ z 1 − z 2 ∣ and the physical distances \({\left\Vert {x}_{1}-{x}_{2}\right\Vert }_{{\ell }_{2}}\) , which use the Euclidean distance \(\vert\vert x {{\vert \vert_{\ell}}_{2}} = {\sqrt {\sum^{D}_{i=1} x^{2}_{i}}}\) for dimension D . For the bitwise distance, we threshold the latent units to its 90th-percentile then compute the L 1 -norm ( \(\vert\vert x {{\vert \vert_{\ell}}_{1}} = \sum^{D}_{i=1} \vert x_{i} \vert\) for dimension D ) between the units. Similar to the previous sections, we compute the joint densities of the binary vectors’ bitwise distances and the physical positions’ Euclidean distances. We then calculate their mutual information to measure how much spatial information the bitwise distance captures. The proposed mechanism for the neural network’s distance measurement—the binary vector’s Hamming distance—gives a mutual information of 0.542 bits, compared to the predictive coder’s mutual information of 0.627 bits and the auto-encoder’s mutual information of 0.227 bits (Fig. 5e ). The code from the overlapping regions captures a majority amount of the predictive coder’s spatial information.
Mapping is a general mechanism for generating an internal representation of sensory information. While spatial maps facilitate navigation and planning within an environment, mapping is a ubiquitous neural function that extends to representations beyond visual–spatial mapping. The primary sensory cortex, for example, maps tactile events topographically. Physical touches that occur in proximity are mapped in proximity for both the neural representations and the anatomical brain regions 52 . Similarly, the cortex maps natural speech by tiling regions with different words and their relationships, which shows that topographic maps in the brain extend to higher-order cognition. The similar representation of non-spatial and spatial maps in the brain suggests a common mechanism for charting cognitive maps 53 . However, it is unclear how a single mechanism can generate both spatial and non-spatial maps.
Here we show that predictive coding provides a basic, general mechanism for charting spatial maps by predicting sensory data from past sensory experiences—including environments with degenerate observations. Our theoretical framework applies to any vector-valued sensory data and could be extended to auditory data, tactile data or tokenized representations of language. We demonstrate a neural network that performs predictive coding and can construct an implicit spatial map of an environment by assembling information from local paths into a global frame within the neural network’s latent space. The implicit spatial map depends specifically on the sequential task of predicting future visual images. Neural networks trained as auto-encoders do not reconstruct a faithful geometric representation in the presence of physically distant yet visually similar landmarks.
Moreover, we study the predictive coding neural network’s representation in latent space. Each unit in the network’s latent space activates at distinct, localized regions—called place fields—with respect to physical space. At each physical location, there exists a unique combination of overlapping place fields. At two locations, the differences in the combinations of overlapping place fields provide the distance between the two physical locations. The existence of place fields in both the neural network and the hippocampus 16 suggests that predictive coding is a universal mechanism for mapping. In addition, vector navigation emerges naturally from predictive coding by computing distances from overlapping place field units. Predictive coding may provide a model for understanding how place cells emerge, change and function.
Predictive coding can be performed over any sensory modality that has some temporal sequence. As natural speech forms a cognitive map, predictive coding may underlie the geometry of human language. Intriguingly, large language models train on causal word prediction—a form of predictive coding—build internal maps that support generalized reasoning, answer questions and mimic other forms of higher-order reasoning 54 . Similarities in spatial and non-spatial maps in the brain suggest that large language models organize language into a cognitive map and chart concepts geometrically. These results all suggest that predictive coding might provide a unified theory for building representations of information-connecting disparate theories including place cell formation in the hippocampus, somatosensory maps in the cortex and human language.
Environment simulation
Forest–cave–river environment.
These experiments leverage the Malmo framework 44 to construct a controlled environment within Minecraft. This environment is a rectangular space measuring 40 by 65 lattice units and incorporates three key visual features: a prominent cave serving as a global landmark, a forest area introducing some visual ambiguity between scenes and a river with a bridge that restricts agent movement options. Within this environment, an agent traverses paths between randomly chosen waypoints. These paths are determined using the A * search algorithm to ensure obstacles did not block the agent’s path. The agent varies its speed and direction to traverse the generated paths. During its exploration, the agent captures visual observations at regular intervals along each path.
Circular environment
To explore the model’s ability to differentiate between visually identical but spatially distinct scenes, these experiments used a circular corridor environment. This environment consists of an infinitely repeating sequence of rooms, specifically coloured red, green, red, blue and yellow in a clockwise direction. Notably, there are two distinct red rooms despite their identical appearance. Technically, the environment is an infinitely long hallway segmented into these coloured rooms. Similar to the previous experiment, an agent navigates between randomly chosen waypoints within this environment. The paths are determined using the A * search algorithm, and the agent captures visual observations at regular intervals along its journey.
Predictive coder
Architecture.
The proposed neural network follows an encoder–decoder architecture, employing a U-Net structure to process input image sequences and predict future images. The encoder and decoder components are both based on ResNet-18 convolutional neural networks.
The encoding module utilizes a ResNet-18 model to extract hierarchical features from the input image sequence. Each image in the sequence is processed independently through the ResNet-18 encoder, generating a sequence of latent vectors. The encoder consists of residual blocks, each containing convolutional layers, batch normalization and rectified linear unit (ReLU) activations. The downsampling is achieved via strided convolutions within the residual blocks.
The self-attention module utilizes multi-headed attention, which processes the sequence of encoded latent units to encode the history of past visual observations. The network consists of one layer of multi-headed attention. The multi-headed attention has h = 8 heads. For the encoded latent units with dimension D = C × H × W , the dimension d of a single head is d = C × H × W / h .
The latent vectors output by the encoder are concatenated to form an ordered sequence. This sequence is then processed by a self-attention layer to capture temporal dependencies and relationships among the image sequence. The self-attention mechanism enables the model to weigh the importance of each latent vector in the context of the entire sequence, facilitating improved temporal feature representation.
The decoding module mirrors the encoder’s architecture, utilizing a ResNet-18 model adapted for upsampling. The decoder reconstructs the future images from the transformed latent vectors, employing transposed convolutions and residual blocks analogous to those in the encoder.
The predictive coder is trained for 200 epochs using stochastic gradient descent as the optimization algorithm. The training parameters include a learning rate of 0.1, Nesterov momentum of 0.9 and a weight decay of 5 × 10 −6 . To optimize the learning process, the learning rate is scheduled using the OneCycle learning-rate policy. This policy adjusts the learning rate cyclically between a lower and upper bound, facilitating efficient convergence and improved performance. The OneCycle learning-rate schedule is characterized by an initial increase in the learning rate, followed by a subsequent decrease.
Latent units
The predictive coder’s encoding and self-attention modules were used to analyse the encoded sequences as the predictive coder’s latent units. The image sequence first undergoes processing through the encoder, which extracts a compressed representation capturing the key features within each image. Subsequently, this encoded sequence is fed into the self-attention module. This module specifically focuses on the inherent temporal order of the images within the sequence. The self-attention module’s processed output forms the predictive coder’s latent units.
Auto-encoder
Unlike the predictive coder architecture, the auto-encoder architecture transforms the current images (rather than the past images for the predictive coder) into a low-dimensional latent vector. The proposed neural network follows an encoder–decoder architecture employing a U-Net structure to process input image sequences into a low-dimensional latent vector and to reconstruct the initial image. The encoder and decoder components are both based on ResNet-18 convolutional neural networks. However, the auto-encoder architecture does not utilize any self-attention layers to integrate past observations of images.
The encoding module utilizes a ResNet-18 model to extract hierarchical features from the input image sequence. Each image in the sequence is processed independently through the ResNet-18 encoder, generating a sequence of latent vectors. The encoder consists of residual blocks, each containing convolutional layers, batch normalization and ReLU activations. The downsampling is achieved via strided convolutions within the residual blocks.
Unlike the predictive coder, the latent vectors output by the encoder are directly processed by the decoder. Whereas the predictive coder predicts the future images within an image sequence, the auto-encoder predicts the current images, given the low-dimensional latent vector generated by the encoder.
The auto-encoder’s encoding module was used to analyse the encoded images as the auto-encoder’s latent units. The image sequence first undergoes processing through the encoder, which extracts a compressed representation capturing the key features within each image. The encoder’s processed output forms the auto-encoder’s latent units.
Positional decoder
To assess the effectiveness of the predictive coder in capturing positional information within the encoded sequences, this analysis employed an auxiliary neural network for position prediction. This network, referred to as the positional decoder, takes the latent units generated by the predictive coder—or auto-encoder—as input. The decoder architecture consists of several layers designed to extract this positional information: a convolutional layer transforms the input to a higher dimension (256), followed by a ReLU activation for non-linearity. A max pooling layer then reduces the spatial resolution while maintaining relevant features. Subsequently, two fully connected (affine) layers with ReLU activations project the data to a lower dimension (64) and finally to a 2-dimensional output, corresponding to the agent’s predicted position ( x and y coordinates).
During training, the mean-squared error between the agent’s actual position and the predicted position served as the loss function
To optimize this loss, the AdamW optimizer was employed with a two-stage learning-rate schedule. The initial stage utilized a learning rate of 10 −4 for 1,000 epochs, followed by a fine-tuning stage with a reduced learning rate of 10 −5 for an additional 1,000 epochs.
Modelling the correspondence between latent and physical distances
This analysis evaluated the ability of the predictive coder’s latent space to encode local positional information. For each path traversed by the agent, we computed the pairwise distances between positions in physical space and the corresponding latent space distances within a neighbourhood of 100 time steps. To assess the correspondence between these two distance measures, we analysed the joint distribution of physical and latent space distances. We modelled the relationship between latent distances and their corresponding physical distances using a logarithmic function with additive Gaussian noise
The goodness-of-fit between the model and the actual data was evaluated using two metrics: the Pearson correlation coefficient, which measures the dependence between the physical and latent distances, and the Kullback–Leibler divergence
which quantifies the difference between the two modelled regression distribution and the observed empirical distribution.
Mutual information of the predictive coder and auto-encoder
The spatial information encoded within the latent representations of both the predictive coder and the auto-encoder was evaluated. To achieve this, this analysis computed the joint densities between the latent distances in each model and the corresponding actual physical distances within the environment. By analysing these joint densities, we were able to quantify the physical information within each model’s latent space. Mutual information
was employed as a metric to assess this physical information. Higher mutual information indicates that the latent distances in a model encode a greater amount of spatial information, signifying a stronger correlation between the distances in the latent space and the actual physical separations between locations in the environment. This comparison allows us to gauge the relative effectiveness of each model in capturing and representing spatial relationships within their respective latent spaces.
Place field analysis
Place field calculation.
This analysis investigated the spatial localization of individual units within the neural network’s latent space. First, this analysis computed the histogram of the distribution of the 128-dimensional latent vectors. To identify active units, this analysis employed a thresholding technique based on the 90th-percentile value of the continuous latent unit values. This ensured a focus on units with notable activation levels. The agent’s head direction was varied during data collection to ensure the identified localized regions remained stable regardless of the agent’s orientation.
Place field statistical fitting
To quantify the degree of localization for each active unit, this analysis fitted a two-dimensional Gaussian distribution
to its corresponding distribution in physical space. The area of the resulting ellipsoid, defined by the Gaussian approximation and exceeding a probability threshold of P ≥ 0.0005, served as our localization metric. This area reflects the spatial extent of the unit’s activation within the environment, relative to the overall environment size of 40 × 65 lattice units (2,600 units). Units with smaller ellipsoid areas indicate a more concentrated activation pattern in physical space, suggesting a higher degree of localization.
Vector navigation analysis
This analysis investigated the ability of the neural network’s latent space to not only encode positional information but also represent the vector heading from a current location to a goal location—called vector navigation. To assess this, we compared the combinations of overlapping regions in the latent space representations of two distinct positions x 1 and x 2 . This analysis achieved this by computing the bitwise difference z 1 − z 2 between the corresponding latent codes z 1 , z 2 for these positions. Subsequently, we examined the relationship between this difference vector and the actual physical displacement vector x 1 − x 2 using a linear decoder
This decoder was trained to predict the displacement vector based solely on the latent code difference. The predicted displacement was then decomposed into its distance and directional components, to calculate the specific errors associated with predicting both the distance and direction to the goal location. This analysis computed the Pearson correlation coefficient between the predicted distance, predicted direction and the predicted displacement vector.
Mutual information calculation
This analysis employed a complementary approach to evaluate the spatial information encoded within the binary vectors derived from the latent space. Here the joint densities were computed between the bitwise distances of these binary vectors and the Euclidean distances between corresponding physical positions. The mutual information
was then computed to quantify the amount of spatial information captured by the bitwise distances. This metric essentially reflects how well the bitwise distance between latent codes reflects the actual physical separation between locations in the environment. Finally, to provide context for the obtained value, the mutual information of the binary vectors’ bitwise distance was compared with the mutual information derived from the latent distances of both the predictive coder and the auto-encoder. This comparison assesses the relative effectiveness of each model in capturing spatial information within their respective latent representations.
Place field stability with shifting landmarks
To assess the stability of the identified localized regions within the latent space, this analysis investigated their resilience to changes in the environment’s landmarks. The environment was manipulated: the trees, originally serving as landmarks, were removed and then randomly redistributed throughout the space. Subsequently, the Jaccard index
was employed to quantify the overlap between the latent units identified in the original environment and those found in the environment with shifted landmarks. The Jaccard index ranges from 0 to 1, where a value of 1 indicates a perfect overlap between the sets of latent units, and 0 signifies no overlap. This analysis allowed us to evaluate how well the latent units maintain their spatial correspondence despite alterations to the environment’s visual features.
Reporting summary
Further information on research design is available in the Nature Portfolio Reporting Summary linked to this article.
Data availability
All datasets supporting the findings of this study, including the latent variables for the auto-encoding and predictive coding neural networks, as well as the training and validation datasets, are available on GitHub at https://github.com/jgornet/predictive-coding-recovers-maps and via Zenodo at https://doi.org/10.5281/zenodo.11287439 (ref. 55 ).
Code availability
The code supporting the conclusions of this study is available on GitHub at https://github.com/jgornet/predictive-coding-recovers-maps and via Zenodo at https://doi.org/10.5281/zenodo.11287439 (ref. 55 ). The repository contains the Project Malmo environment code, training scripts for both the predictive coding and auto-encoding neural networks, as well as code for the analysis of predictive coding and auto-encoding results.
Epstein, R. A., Patai, E. Z., Julian, J. B. & Spiers, H. J. The cognitive map in humans: spatial navigation and beyond. Nat. Neurosci. 20 , 1504–1513 (2017).
Article Google Scholar
Wang, Z. J. & Thomson, M. Localization of signaling receptors maximizes cellular information acquisition in spatially structured natural environments. Cell Syst. 13 , 530–546 (2022).
Sivak, D. A. & Thomson, M. Environmental statistics and optimal regulation. PLoS Comput. Biol. 10 , e1003826 (2014).
Anderson, J. Cognitive Psychology and Its Implications 9th edn (Worth Publishers, 2020).
Rescorla, M. Cognitive maps and the language of thought. Br. J. Philos. Sci. 60 , 377–407 (2009).
Whittington, J. C., McCaffary, D., Bakermans, J. J. & Behrens, T. E. How to build a cognitive map. Nat. Neurosci. 25 , 1257–1272 (2022).
Aronov, D., Nevers, R. & Tank, D. W. Mapping of a non-spatial dimension by the hippocampal–entorhinal circuit. Nature 543 , 719–722 (2017).
Nieh, E. H. et al. Geometry of abstract learned knowledge in the hippocampus. Nature 595 , 80–84 (2021).
Whittington, J. C. et al. The Tolman-Eichenbaum machine: unifying space and relational memory through generalization in the hippocampal formation. Cell 183 , 1249–1263 (2020).
Wilson, R. C., Takahashi, Y. K., Schoenbaum, G. & Niv, Y. Orbitofrontal cortex as a cognitive map of task space. Neuron 81 , 267–279 (2014).
Constantinescu, A. O., O’Reilly, J. X. & Behrens, T. E. J. Organizing conceptual knowledge in humans with a gridlike code. Science 352 , 1464–1468 (2016).
Garvert, M. M., Dolan, R. J. & Behrens, T. E. A map of abstract relational knowledge in the human hippocampal–entorhinal cortex. eLife 6 , e17086 (2017).
Huth, A. G., de Heer, W. A., Griffiths, T. L., Theunissen, F. E. & Gallant, J. L. Natural speech reveals the semantic maps that tile human cerebral cortex. Nature 532 , 453–458 (2016).
Corkin, S. Lasting consequences of bilateral medial temporal lobectomy: clinical course and experimental findings in H.M. Semin. Neurol. 4 , 249–259 (1984).
Behrens, T. E. et al. What is a cognitive map? Organizing knowledge for flexible behavior. Neuron 100 , 490–509 (2018).
O’Keefe, J. Place units in the hippocampus of the freely moving rat. Exp. Neurol. 51 , 78–109 (1976).
Hafting, T., Fyhn, M., Molden, S., Moser, M.-B. & Moser, E. I. Microstructure of a spatial map in the entorhinal cortex. Nature 436 , 801–806 (2005).
Amaral, D. G., Ishizuka, N. & Claiborne, B. in Understanding the Brain Through the Hippocampus: the Hippocampal Region as a Model for Studying Brain Structure and Function (eds Storm-Mathisen, J. et al.) Ch 1 (1990).
Cueva, C. J. & Wei, X.-X. Emergence of grid-like representations by training recurrent neural networks to perform spatial localization. In Proc. 6th International Conference on Learning Representations (ICLR) 1512–1530 (Curran Associates, Inc., 2018).
Banino, A. et al. Vector-based navigation using grid-like representations in artificial agents. Nature 557 , 429–433 (2018).
Crane, K., Weischedel, C. & Wardetzky, M. The heat method for distance computation. Commun. ACM 60 , 90–99 (2017).
Zhang, T., Rosenberg, M., Jing, Z., Perona, P. & Meister, M. Endotaxis: A neuromorphic algorithm for mapping, goal-learning, navigation, and patrolling. eLife 12 , RP84141 (2023).
Thrun, S. & Montemerlo, M. The Graph SLAM algorithm with applications to large-scale mapping of urban structures. Int. J. Robot. Res. 25 , 403–429 (2006).
Mur-Artal, R. & Tardós, J. D. Visual-inertial monocular SLAM with map reuse. IEEE Robot. Autom. Lett. 2 , 796–803 (2017).
Mourikis, A. I. & Roumeliotis, S. I. A multi-state constraint Kalman filter for vision-aided inertial navigation. In Proc. 2007 IEEE International Conference on Robotics and Automation 3565–3572 (IEEE, 2007).
Lynen, S. et al. Get out of my lab: large-scale, real-tme visual-inertial localization. In Proc. Robotics: Science and System XI (eds Kavraki, L. E., Hsu, D. & Buchli, J.) (RSS, 2015); https://doi.org/10.15607/RSS.2015.XI.037
Gupta, S. et al. Cognitive mapping and planning for visual navigation. In Proc. 2017 IEEE Conference on Computer Vision and Pattern Recognition (CVPR) 7272–7281 (IEEE, 2017).
Mirowski, P. et al. Learning to navigate in cities without a map. In Proc. 32nd International Conference on Neural Information Processing Systems (eds Bengio, S. & Wallach, H.M.) 2424–2435 (Curran Associates, Inc., 2018).
Duan, Y. et al. RL 2 : fast reinforcement learning via slow reinforcement learning. Preprint at https://doi.org/10.48550/arXiv.1611.02779 (2016).
Higgins, I. et al. DARLA: improving zero-shot transfer in reinforcement learning. In Proc. 34th International Conference on Machine Learning (eds Precup, D. & Teb, Y. W.) 1480–1490 (PMLR, 2017); https://proceedings.mlr.press/v70/higgins17a.html
Seo, Y., Lee, K., James, S. L. & Abbeel, P. Reinforcement learning with action-free pre-training from videos. In Proc. 39th International Conference on Machine Learning (eds Chaudhuri, K. et al.) 19561–19579 (PMLR, 2022); https://proceedings.mlr.press/v162/seo22a.html
Lee, T. S. & Mumford, D. Hierarchical Bayesian inference in the visual cortex. JOSA A 20 , 1434–1448 (2003).
Mumford, D. in First European Congress of Mathematics. Progress in Mathematics Vol. 3 (eds Joseph, A. et al.) 187–224 (Springer, 1994).
Rao, R. P. N. & Ballard, D. H. Predictive coding in the visual cortex: a functional interpretation of some extra-classical receptive-field effects. Nat. Neurosci. 2 , 79–87 (1999).
Poincaré, H. The Foundations of Science: Science and Hypothesis, the Value of Science, Science and Method (Cambridge Univ. Press, 2015).
O’Keefe, J. & Nadel, L. The Hippocampus as a Cognitive Map (Clarendon Press, Oxford Univ. Press, 1978).
Thrun, S., Burgard, W. & Fox, D. Probabilistic Robotics (MIT Press, 2005).
Stachenfeld, K. L., Botvinick, M. M. & Gershman, S. J. The hippocampus as a predictive map. Nat. Neurosci. 20 , 1643–1653 (2017).
Recanatesi, S. et al. Predictive learning as a network mechanism for extracting low-dimensional latent space representations. Nat. Commun. 12 , 1417 (2021).
Fang, C., Aronov, D., Abbott, L. & Mackevicius, E. L. Neural learning rules for generating flexible predictions and computing the successor representation. eLife 12 , e80680 (2023).
Dayan, P., Hinton, G. E., Neal, R. M. & Zemel, R. S. The Helmholtz machine. Neural Comput. 7 , 889–904 (1995).
Luttrell, S. P. A Bayesian analysis of self-organizing maps. Neural Comput. 6 , 767–794 (1994).
Tu, L. W. Differential Geometry: Connections, Curvature, and Characteristic Classes 1st edn (Springer, 2017).
Johnson, M., Hofmann, K., Hutton, T. & Bignell, D. The Malmo platform for artificial intelligence experimentation. In Proc. Twenty-Fifth International Joint Conference on Artificial Intelligence (ed. Brewka, G.) 4246–4247 (AAAI Press, 2016).
He, K., Zhang, X., Ren, S. & Sun, J. Deep residual learning for image recognition. In Proc. 2016 IEEE Conference on Computer Vision and Pattern Recognition (CVPR) 770–778 (IEEE, 2016).
Ronneberger, O., Fischer, P. & Brox, T. U-Net: convolutional networks for biomedical image segmentation. In Medical Image Computing and Computer-Assisted Intervention (MICCAI 2015) (eds Navab, N. et al.) 234–241 (Springer International Publishing, 2015).
Vaswani, A. et al. Attention is all you need. In Proc. 31st International Conference on Neural Information Processing Systems (eds Von Luxburg, U. et al.) 5999–6009 (Curran Associates, Inc., 2017).
Sutskever, I., Martens, J., Dahl, G. & Hinton, G. On the importance of initialization and momentum in deep learning. In Proc. 30th International Conference on Machine Learning (eds Dasgupta, S. & McAllester, D.) 1139–1147 (PMLR, 2013); https://proceedings.mlr.press/v28/sutskever13.html
Smith, L. N. & Topin, N. Super-convergence: very fast training of neural networks using large learning rates. Preprint at https://doi.org/10.48550/arXiv.1708.07120 (2018).
Tenenbaum, J. B., de Silva, V. & Langford, J. C. A global geometric framework for nonlinear dimensionality reduction. Science 290 , 2319–2323 (2000).
Bush, D., Barry, C., Manson, D. & Burgess, N. Using grid cells for navigation. Neuron 87 , 507–520 (2015).
Rosenthal, I. A. et al. S1 represents multisensory contexts and somatotopic locations within and outside the bounds of the cortical homunculus. Cell Rep. 42 , 112312 (2023).
Behrens, T. E. J. et al. What is a cognitive map? Organizing knowledge for flexible behavior. Neuron 100 , 490–509 (2018).
Brown, T. et al. Language models are few-shot learners. In Proc. 33rd International Conference on Neural Information Processing Systems (eds Larochelle, H. et al.) 1877–1901 (Curran Associates, Inc., 2020).
Gornet, J. jgornet/predictive-coding-recovers-maps: Nature Machine Intelligence pre-release. Zenodo https://doi.org/10.5281/zenodo.11287439 (2024).
Download references
Acknowledgements
We appreciate I. Strazhnik for her contributions to the scientific visualizations and figure illustrations. Her expertise in translating our research into clear visuals has significantly elevated the clarity and impact of our paper. We are grateful to T. Siapas, E. Lubenov, D. Mobbs and M. Rosenberg for their invaluable and insightful discussions. Their expertise and feedback have been instrumental in the development and realization of this research. Additionally, we appreciate the insights provided by L. Xu, M. Wang and J. Zheng, which played a crucial role in refining various aspects of our study. We are thankful for the support provided by The David and Lucile Packard Foundation under grant no. 2019-69662, as well as the Chen Institute at Caltech, the Heritage Medical Research Institute and the Chan Zuckerberg Initiative (M.T. and J.G.).
Author information
Authors and affiliations.
Division of Biology and Biological Engineering, California Institute of Technology, Los Angeles, CA, USA
James Gornet & Matt Thomson
You can also search for this author in PubMed Google Scholar
Contributions
J.G. and M.T. contributed equally to all stages of the work. J.G. and M.T. conceived the study. M.T. and J.G. jointly derived the mathematical framework, J.G. and M.T. built the environment simulation and the corresponding dataset. J.G. and M.T. constructed the neural network architecture and oversaw its training. J.G. and M.T. performed the analysis of the results.
Corresponding author
Correspondence to Matt Thomson .
Ethics declarations
Competing interests.
The authors declare no competing interests.
Peer review
Peer review information.
Nature Machine Intelligence thanks the anonymous reviewers for their contribution to the peer review of this work.
Additional information
Publisher’s note Springer Nature remains neutral with regard to jurisdictional claims in published maps and institutional affiliations.
Supplementary information
Supplementary information.
Supplementary text and Figs. 1–6.
Reporting Summary
Rights and permissions.
Open Access This article is licensed under a Creative Commons Attribution 4.0 International License, which permits use, sharing, adaptation, distribution and reproduction in any medium or format, as long as you give appropriate credit to the original author(s) and the source, provide a link to the Creative Commons licence, and indicate if changes were made. The images or other third party material in this article are included in the article’s Creative Commons licence, unless indicated otherwise in a credit line to the material. If material is not included in the article’s Creative Commons licence and your intended use is not permitted by statutory regulation or exceeds the permitted use, you will need to obtain permission directly from the copyright holder. To view a copy of this licence, visit http://creativecommons.org/licenses/by/4.0/ .
Reprints and permissions
About this article
Cite this article.
Gornet, J., Thomson, M. Automated construction of cognitive maps with visual predictive coding. Nat Mach Intell 6 , 820–833 (2024). https://doi.org/10.1038/s42256-024-00863-1
Download citation
Received : 26 September 2023
Accepted : 11 June 2024
Published : 18 July 2024
Issue Date : July 2024
DOI : https://doi.org/10.1038/s42256-024-00863-1
Share this article
Anyone you share the following link with will be able to read this content:
Sorry, a shareable link is not currently available for this article.
Provided by the Springer Nature SharedIt content-sharing initiative
This article is cited by
Cognitive maps from predictive vision.
- Margaret C. von Ebers
- Xue-Xin Wei
Nature Machine Intelligence (2024)
Quick links
- Explore articles by subject
- Guide to authors
- Editorial policies
Sign up for the Nature Briefing: AI and Robotics newsletter — what matters in AI and robotics research, free to your inbox weekly.

Grab your spot at the free arXiv Accessibility Forum
Help | Advanced Search
Computer Science > Computation and Language
Title: logogramnlp: comparing visual and textual representations of ancient logographic writing systems for nlp.
Abstract: Standard natural language processing (NLP) pipelines operate on symbolic representations of language, which typically consist of sequences of discrete tokens. However, creating an analogous representation for ancient logographic writing systems is an extremely labor intensive process that requires expert knowledge. At present, a large portion of logographic data persists in a purely visual form due to the absence of transcription -- this issue poses a bottleneck for researchers seeking to apply NLP toolkits to study ancient logographic languages: most of the relevant data are images of writing. This paper investigates whether direct processing of visual representations of language offers a potential solution. We introduce LogogramNLP, the first benchmark enabling NLP analysis of ancient logographic languages, featuring both transcribed and visual datasets for four writing systems along with annotations for tasks like classification, translation, and parsing. Our experiments compare systems that employ recent visual and text encoding strategies as backbones. The results demonstrate that visual representations outperform textual representations for some investigated tasks, suggesting that visual processing pipelines may unlock a large amount of cultural heritage data of logographic languages for NLP-based analyses.
Subjects: | Computation and Language (cs.CL); Artificial Intelligence (cs.AI); Computer Vision and Pattern Recognition (cs.CV) |
Cite as: | [cs.CL] |
(or [cs.CL] for this version) | |
Focus to learn more arXiv-issued DOI via DataCite | |
Journal reference: | ACL 2024, long paper |
Submission history
Access paper:.
- HTML (experimental)
- Other Formats
References & Citations
- Google Scholar
- Semantic Scholar
BibTeX formatted citation

Bibliographic and Citation Tools
Code, data and media associated with this article, recommenders and search tools.
- Institution
arXivLabs: experimental projects with community collaborators
arXivLabs is a framework that allows collaborators to develop and share new arXiv features directly on our website.
Both individuals and organizations that work with arXivLabs have embraced and accepted our values of openness, community, excellence, and user data privacy. arXiv is committed to these values and only works with partners that adhere to them.
Have an idea for a project that will add value for arXiv's community? Learn more about arXivLabs .

The Code Compass
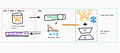
What is QLoRA?: A Visual Guide to Efficient Finetuning of Quantized LLMs
Sometimes smaller is better. how qlora combines efficiency and performance..

Get a list of personally curated and freely accessible ML, NLP, and computer vision resources for FREE on newsletter sign-up.
Consider sharing this with someone who wants to know more about machine learning.
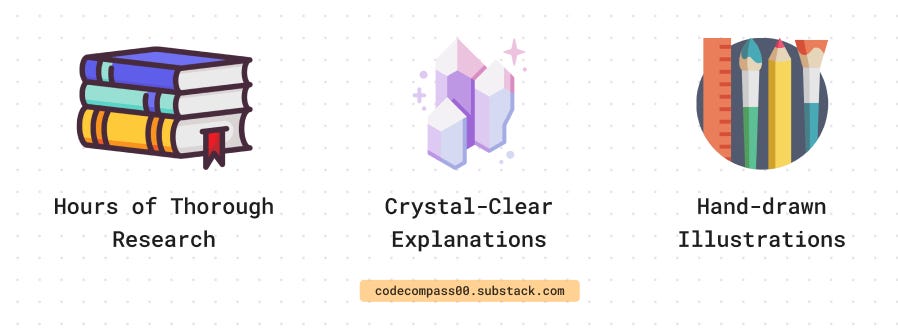
In a previous article , we discussed how full fine-tuning large models such as GPT-4, Gemini, Claude, and LLAMA [2, 3, 4, 5, 6] for specific tasks is extremely resource (GPU and data) and capital-intensive.
LoRA or Low-Rank Adaptation came to the rescue as one of the most well-known methods for PEFT (Parameter-Efficient Fine-Tuning).
Can we get better than LoRA? Indeed QLoRA improves upon LoRA. A quote from the QLoRA paper:
“Our results show that QLoRA finetuning on a small high-quality dataset leads to state-of-the-art results, even when using smaller models than the previous SoTA.” [16]
In this visual guide, we discuss the technical details behind QLoRA, which is how it has become the default parameter-efficient fine-tuning method for LLMs.
Apple’s WWDC 2024 announcement [12] talks about quantization and LoRA being used for running large models (LLMs and MLMs [7]) directly on Apple devices to power their AI features (dubbed as “Apple Intelligence”).
“For on-device inference, we use low-bit palletization, a critical optimization technique that achieves the necessary memory, power, and performance requirements. To maintain model quality, we developed a new framework using LoRA adapters that incorporates a mixed 2-bit and 4-bit configuration strategy — averaging 3.7 bits-per-weight — to achieve the same accuracy as the uncompressed models. More aggressively, the model can be compressed to 3.5 bits-per-weight without significant quality loss.” — Apple, June 2024
Today, we take a deep dive into QLoRA:
Why should we care about methods such as LoRA and QLoRA?
QLoRA vs. LoRA
Primers on Floating Point Representations and Blockwise Quantization
The NormalFloat4 Format
Putting It All Together To Get QLoRA
Final Thoughts and Takeaways
Would like to read other related pieces? Here you can read more about the Transformers series and LLMs series .
![visual representation in data [Jupyter Notebook] Build Your Own Open-source RAG Using LangChain, LLAMA 3 and Chroma](https://substackcdn.com/image/fetch/w_140,h_140,c_fill,f_auto,q_auto:good,fl_progressive:steep,g_auto/https%3A%2F%2Fsubstack-post-media.s3.amazonaws.com%2Fpublic%2Fimages%2F87ac01f1-0169-4922-8b67-cbcaaba60fae_1117x574.png)
[Jupyter Notebook] Build Your Own Open-source RAG Using LangChain, LLAMA 3 and Chroma
![visual representation in data "Attention, Please!": A Visual Guide To The Attention Mechanism [Transformers Series]](https://substackcdn.com/image/fetch/w_140,h_140,c_fill,f_auto,q_auto:good,fl_progressive:steep,g_auto/https%3A%2F%2Fsubstack-post-media.s3.amazonaws.com%2Fpublic%2Fimages%2F375dc525-fa19-4e6f-81f2-68820bfd36a1_1903x856.png)
"Attention, Please!": A Visual Guide To The Attention Mechanism [Transformers Series]
1. why should we care about methods such as lora and qlora.
By now, we know the basis of performant LLMs: pre-training.
The idea is to get similar performance without performing pre-training or full fine-tuning on large models.
Putting such a large volume of data with a model with trillions of parameters can cost over $100 million to train it (yes, this is ChatGPT4) across a cluster of 100-1000s of GPUs over a couple of weeks. Such pre-training runs are expensive and would only be performed every quarter or even annually. — What is LoRA?: A Visual Guide to Low-Rank Approximation for Fine-Tuning LLMs Efficiently
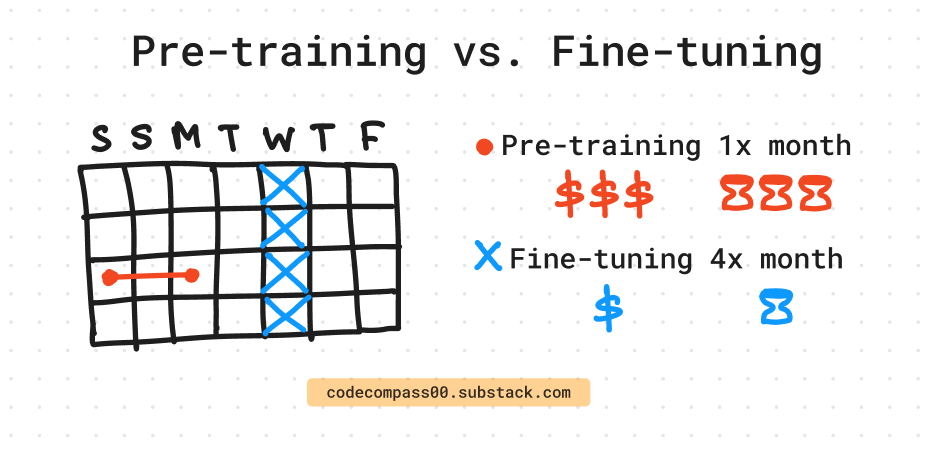
2. QLoRA: Smaller Is Better
“We present QLoRA, an efficient finetuning approach that reduces memory usage enough to finetune a 65B parameter model on a single 48GB GPU while preserving full 16-bit finetuning task performance. QLoRA backpropagates gradients through a frozen, 4-bit quantized pretrained language model into Low Rank Adapters (LoRA)” [16]
QLoRA is weight quantization combined with LoRA. With the help of QLoRA models can be fine-tuned with the following benefits:
Lower Memory Requirements: The first thing that comes out of the box with quantization is a reduced memory footprint. Thanks to the quantization in QLoRA you can fine-tune bigger models than it would be possible with just regular fine-tuning (models with 33B and 65B parameters). The base model is quantized to store weights from 16 bits to 4 bits.
Competitive Performance: Fine-tuning with QLoRA results in performance competitive to that of a full-finetuning or LoRA fine-tuning.
Smaller is Better: QLoRA + smaller model + high-quality data performs better than previous SoTA that use larger models.
Of course, it also brings the benefits of the base LoRA which we mentioned in a previous post . Here is a summary but you can read all of this in more detail here :
Memory efficiency with <1% memory footprint: …
Converges to the performance of a fully fine-tuned model: …
No overhead during model inference: …
Time and cost-efficient fine-tuning: …
Before we jump into the technical details of the QLoRA, let’s first do a primer on how floating point numbers (weights in our case) are represented and stored.
Continue reading more:
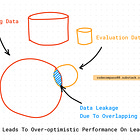
The Challenges of Building Effective LLM Benchmarks
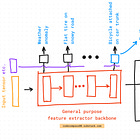
How Tesla Continuously and Automatically Improves Autopilot and Full Self-Driving Capability On 5M+ Cars
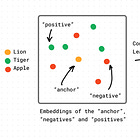
How Apple's iPhone Uses Private, On-Device Machine Learning To Recognize People In Photos
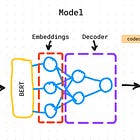
How Netflix Uses Machine Learning To Decide What Content To Create Next For Its 260M Users
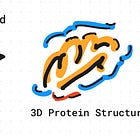
Inside AlphaFold: DeepMind’s Recipe For Success
3. floating point representations: a quick primer.
To represent a floating point number in a binary format (FP32, FP16, TF32, etc.) one requires 3 pieces of information:
Sign : Is the number positive or negative? This is represented by a sign bit.
Range : How large of a number can this format support?
Precision : How finely can the format distinguish different values?
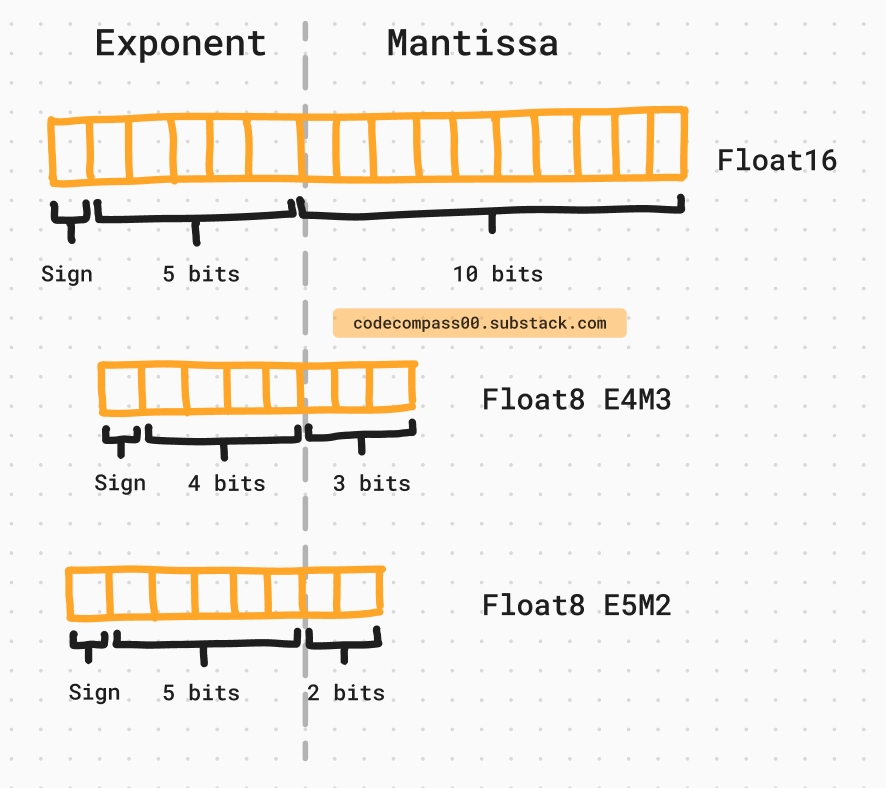
The memory required to store each format depends on the number of bits the format needs to represent a number. Moreover, with the same number of bits, one can change the number of bits storing the range and the number of bits storing the precision.
Ideally, a good balance between range and precision should lead us to the ideal floating point format for machine learning. There has been empirical analysis on which floating point representation works best for machine learning:
“It has been empirically proven that the E4M3 is best suited for the forward pass, and the second version [E5M2] is best suited for the backward computation.” [19]
Let’s look at an example number and how it would be represented using 1 bit for the sign, 4 bits for the range, and 3 bits for the mantissa (the part after the decimal point).
-3.75 would be written as follows:
Range : 0011
Precision : 110
Putting it together -3.75 is written as 10011.110
4. Blockwise Quantization: A Quick Primer
The paper mentions quantizing a 32-bit Floating Point (FP32) number to an Int8 which has a range [-127, 127]. Let’s go through this more concretely.
Define the 32-bit float tensor.
Chunk the tensor into blocks. We use 2 blocks in this example.
Calculate the quantization constant for each block.
For block 1:
For block 2:
Quantize each block
Combine quantized blocks
Here are the quantization constants:
What happens if we don’t use blockwise quantization?
Without blockwise quantization, information may be lost when large values are present in the input.
If the tensor being quantized has a large outlier value it will increase the absmax value. It is now possible that two values that are close but different can become indistinguishable after the quantization.
For simplicity, let’s say we have 3 numbers: [0.5, 3.0, 1000.0]. The absmax is now 1000.
Now let’s try to quantize them to Int8 (like above) with range [-127, 127].
Here, we can see that 0.5 and 3.0 both ended up being mapped to the same value i.e. 0 because of such a large quantization constant thanks to the outlier 1000.
5. The Need for A New Format: NormalFloat4 (NF4)
Standard quantization works well when the values are uniformly distributed in a range. If it is not that case standard quantization has a drawback.
“Since pretrained neural network weights usually have a zero-centered normal distribution” [16]
NormalFloat4 tackles this by assuming that the values to be quantized i.e. the values in X come from a normal distribution. The is taken and normalized by the absmax (max of the absolute values) so that the values now all fall between [-1, 1]. Now that we have our range of input values, let’s divide them into bins for quantization.
NormalFloat4 has 4-bits so we have 2^4 = 16 different bins available for quantization i.e. [0000, 0001, 0010, …, 1111]. Using standard quantization we could divide the range [-1, 1] into 16 equal-sized bins but we know that this is not ideal when values come from a normal distribution.
NF4 exploits the knowledge of the values following a normal distribution where a bulk of the values are around the center of the bell curve and then it flattens out at either extreme. With this QLoRA design NF4 creates bins based on the probability of finding points in that bin. Ideally, each bin has the same number of points falling in it assuring an optimal quantization.
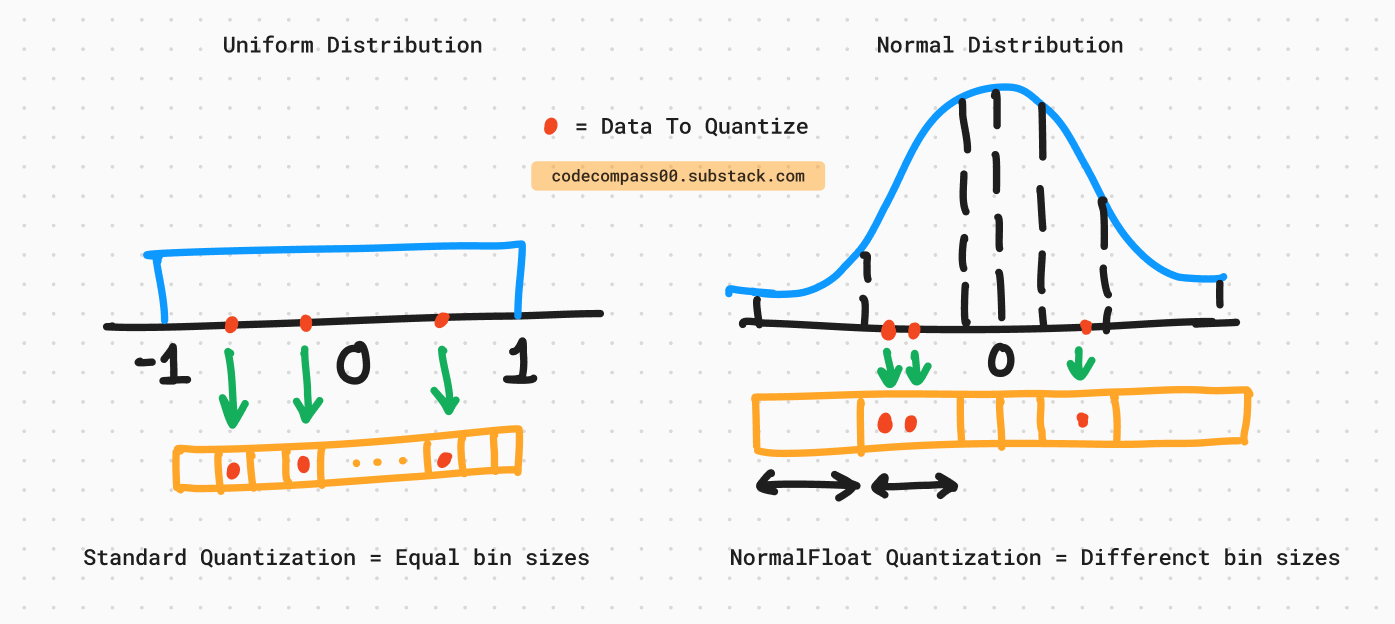
Quantile Quantization
Quantile Quantization is a technique used in data quantization where the goal is to ensure that each quantization bin has an equal number of values assigned from the input tensor. This is achieved by using the quantiles of the input data distribution.
Quantiles: Quantiles are points taken at regular intervals from the cumulative distribution function (CDF) of a random variable. For instance, the median is the 0.5 quantile, meaning 50% of the data is below this value.
Equal Bins: In quantile quantization, the input data is divided into bins in such a way that each bin contains an equal number of data points. This contrasts with standard (uniform) quantization, where bins have equal widths but might contain varying numbers of data points.
Optimal Data Type: Quantile quantization is considered information-theoretically optimal because it minimizes the quantization error by ensuring that the bins are populated equally, making efficient use of the available quantization levels.
Why is NF4 Optimal?
Error Minimization And Efficient Use of Bins: By ensuring an equal population of bins, NF4 minimizes quantization error, especially important for data with a normal distribution. Since each bin is equally populated, the NF4 data type makes efficient use of the available quantization levels, leading to better preservation of the original data's statistical properties.
Information-Theoretic Optimality: The process of quantizing based on quantiles is theoretically optimal because it distributes the quantization error evenly across the data range, rather than clustering errors in certain regions.
6. Intuition Behind QLoRA: Quantization + LoRA
QLoRA can fine-tune a quantized model without performance degradations.
Here is a step-by-step breakdown of what QLoRA does:
Quantize the model weights to their proposed NormalFloat4 (NF4) float format.
Train low-rank adapters on top of this.
“QLoRA reduces the average memory requirements of finetuning a 65B parameter model from >780GB of GPU memory to <48GB without degrading the runtime or predictive performance compared to a 16- bit fully finetuned baseline.” [16]
QLoRA is a success due to 3 main innovations that work in tandem to make it more efficient and equally performant to SoTA:
4-bit NormalFloat Quantization : We converted this in the section above.
Double Quantization : This is the quantization of the quantization constant itself. For N blocks, there are N quantization constants. This step helps optimize memory footprint by quantizing these N values.
“On average, for a blocksize of 64, this quantization reduces the memory footprint per parameter from 32/64 = 0.5 bits, to 8/64 + 32/(64 · 256) = 0.127 bits, a reduction of 0.373 bits per parameter.” [16]
Paged Optimizers : When sequence lengths are extremely long, the GPU can run out of memory. To prevent this, optimizer states are moved from the GPU to the CPU. The optimizer state is moved back to the GPU for the optimizer’s update step.
“… transfers between the CPU and GPU for error-free GPU processing in the scenario where the GPU occasionally runs out-of-memory” [16]
Quantization + LoRA : Tie the above together with low-rank adapters (see LoRA post for details).
These all tied together with low-rank adapters (LoRA) give a significant reduction in GPU memory consumption while maintaining performance.
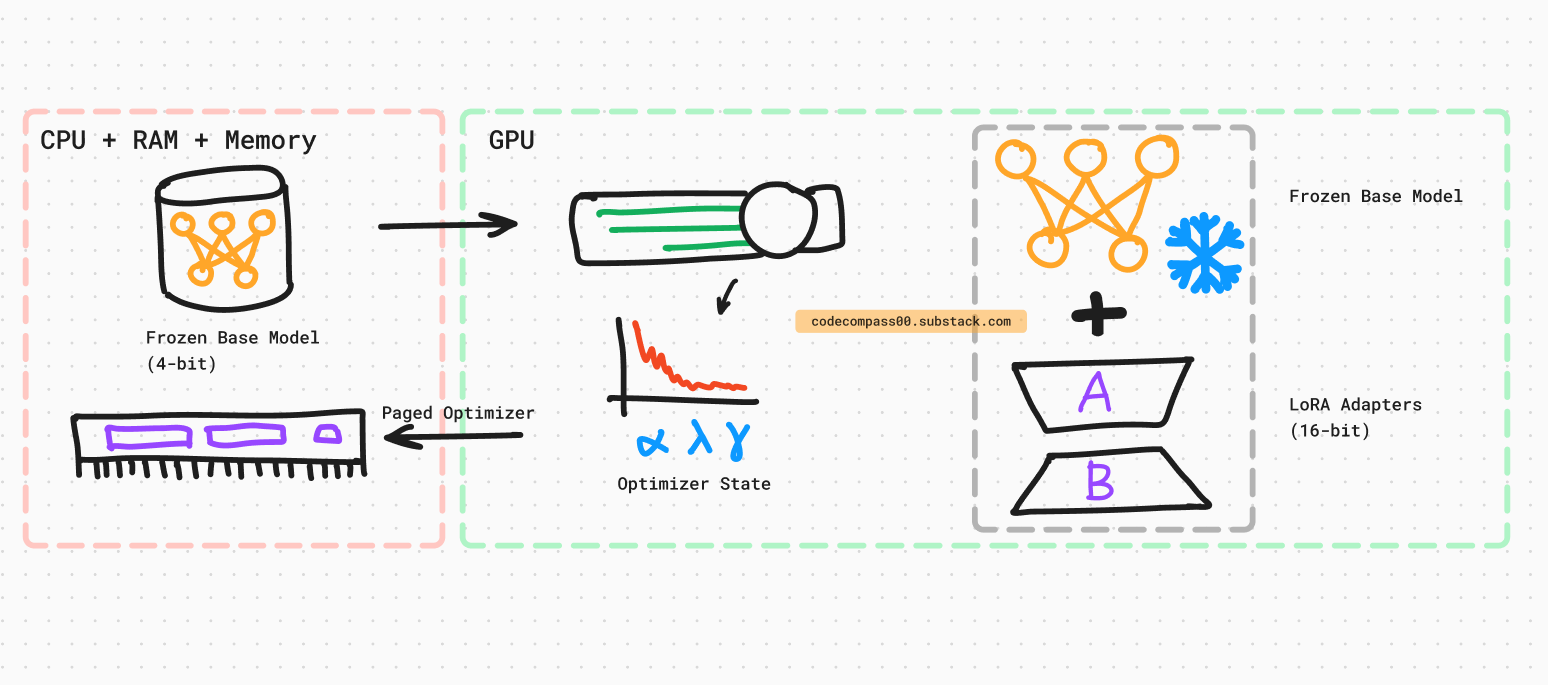
Here is a quick summary. You can read more details about LoRA in the dedicated post.
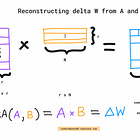
What is LoRA?: A Visual Guide to Low-Rank Approximation for Fine-Tuning LLMs Efficiently
What is a low-rank adapter.
A low-rank adapter consists of two smaller matrices, A and B , which are inserted into the model to capture task-specific variations. During training, only these matrices are updated, leaving the majority of the pre-trained model parameters unchanged.
Assume a matrix of the form MxN, with M=100 and N=100. LoRA “breaks” the MxN matrix down such that instead of updating 100*100 weights, only a small fraction of 10,000 parameters are involved in the process.
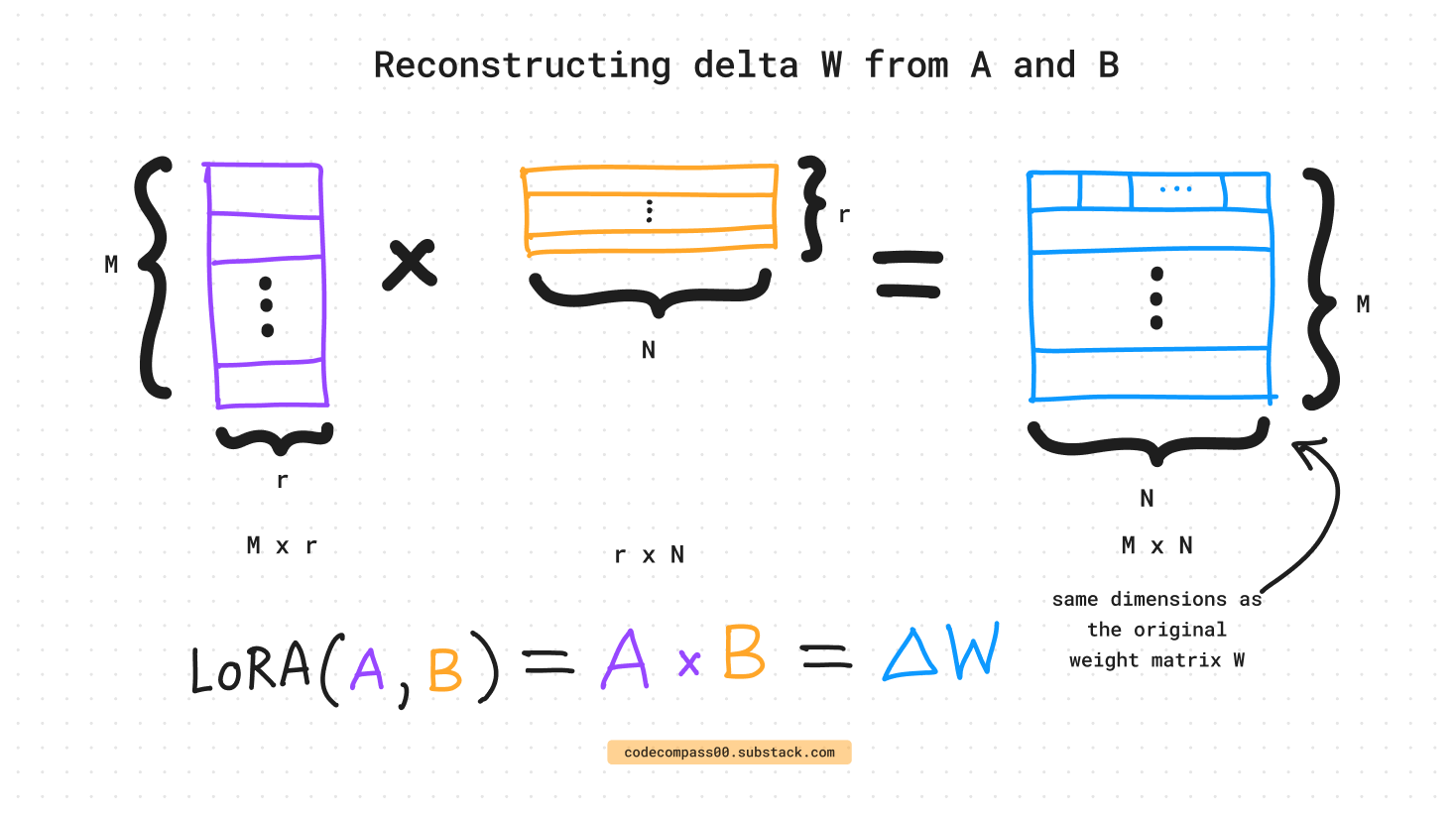
Thanks to the quantization which reduced the memory footprint. With QLoRA, the number of low-rank adapters can be increased without increasing the memory significantly as most of the memory usage comes from storing the original (frozen) model weights and not so much from the LoRA parameters (weights) or their gradients.
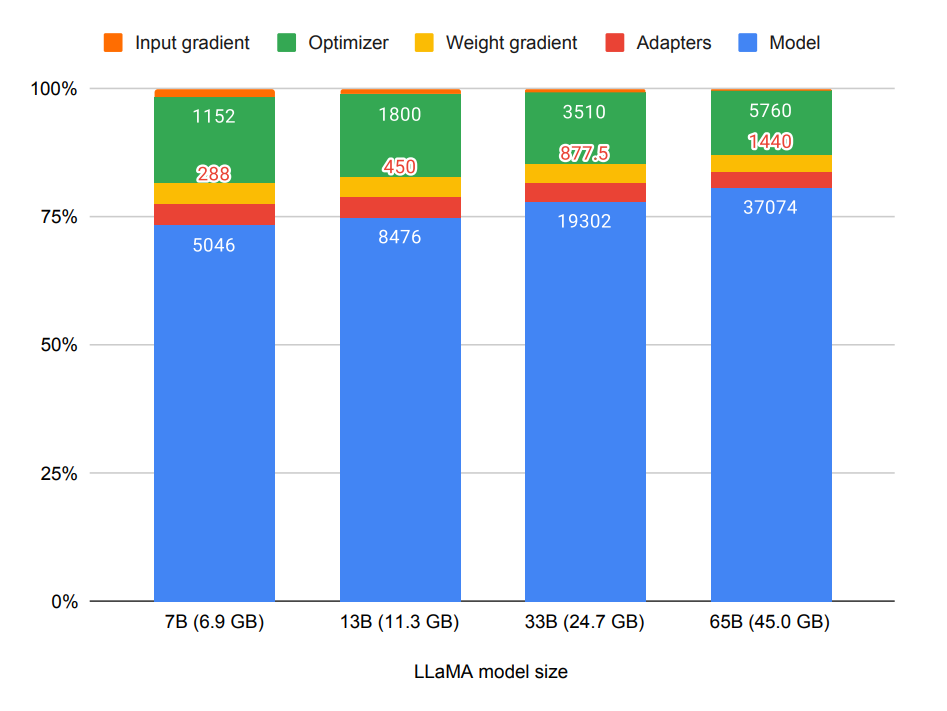
“For a 7B LLaMA model trained on FLAN v2 with a batch size of 1, with LoRA weights equivalent to commonly used 0.2% of the original model weights, the LoRA input gradients have a memory footprint of 567 MB while the LoRA parameters take up only 26 MB.” [16]
What happens during training and inference?
QLoRA can be seen as using 2 data types:
Storage Data Type : 4-bit NormalFloat (NF4). This is for the base model being fine-tuned which has its frozen weights quantized to NF4.
Computation Data Type : 16-bit BrainFloat (BF16). When performing forward and backward passes, the storage data type is de-quantized (reverse of quantization) to 16-bit format. The 16-bit format is then used for computation.
Gradients are computed and applied only to the LoRA adapter parameters, which are also in 16-bit BrainFloat (BF16). The low-bit quantized weights are not updated directly during training. It is important to note that only LoRA parameters are updated.
7. Key Takeaways
1. 4-bit qlora with nf4 >> 16-bit lora.
Using the NF4 format for quantization with QLoRA outperforms standard 16-bit finetuning as well as 16-bit LoRA.
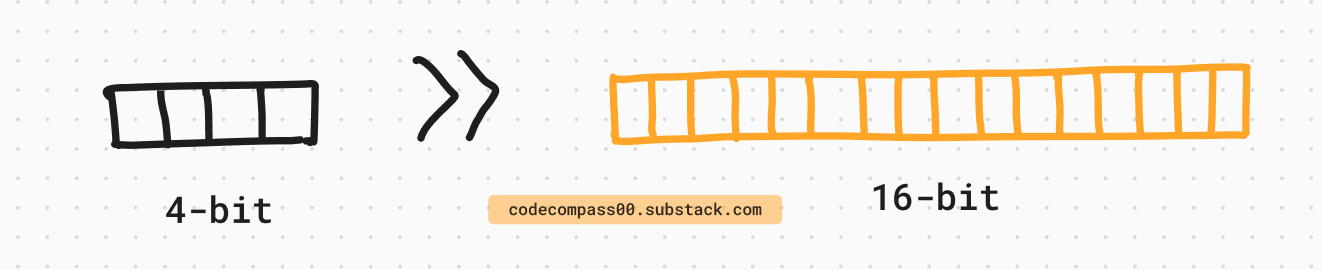
2. NormalFloat4 Format >> FloatingPoint4 Format
NF4 is more performant than the standard FP4 format. Double dequantization leads to minor performance gains but reduces memory footprint to fit larger models.
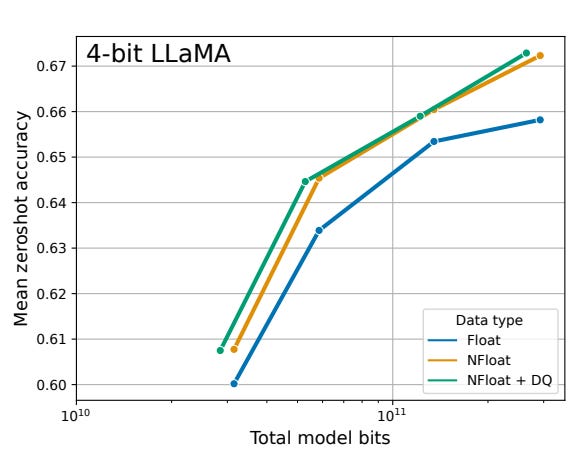
3. Increase Params + Reduce Precision
Given a constant budget, it is better to increase the number of parameters and decrease their precision.
“… with a given finetuning and inference resource budget it is beneficial to increase the number of parameters in the base model while decreasing their precision” [16]
4. Data Quality >> Data Size
One of the most important takeaways from the paper is that data quality >> data size!
“… we find that data quality is far more important than dataset size, e.g., a 9k sample dataset (OASST1) outperformed a 450k sample dataset (FLAN v2, subsampled) on chatbot performance, even when both are meant to support instruction following generalization.” [16]
See you in the next edition of the Code Compass.
Read more on the Transformers series , LLMs series , or Tesla’s data engine:
Consider subscribing to get it straight into your mailbox:
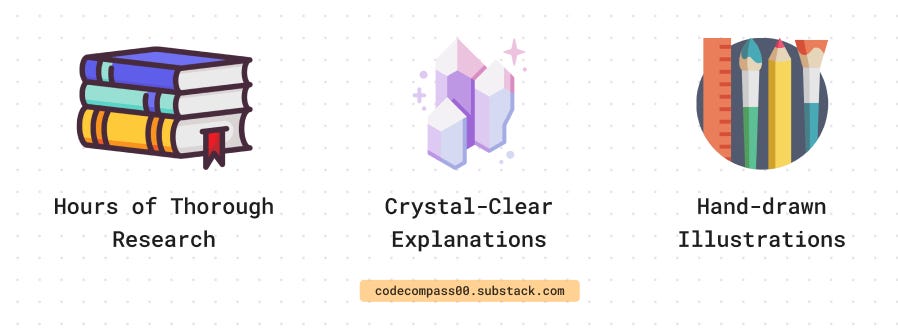
[1] Attention Is All You Need: https://arxiv.org/abs/1706.03762
[2] GPT-4 Technical Report: https://arxiv.org/abs/2303.08774
[3] Gemini: A Family of Highly Capable Multimodal Models: https://arxiv.org/abs/2312.11805
[4] Gemini 1.5: https://arxiv.org/abs/2403.05530
[5] Claude 3: https://www.anthropic.com/news/claude-3-family
[6] LLAMA: https://arxiv.org/abs/2302.13971
[7] Multimodal Large Language Models: A Survey
[8] An Image is Worth 16x16 Words: Transformers for Image Recognition at Scale: https://arxiv.org/abs/2010.11929
[9] Intro to Large Language Models: youtube.com/watch?v=zjkBMFhNj_g
[10] Hugging Face LoRA: https://huggingface.co/docs/diffusers/training/lora
[11] LoRA: Low-Rank Adaptation of Large Language Models: https://arxiv.org/abs/2106.09685
[12] Apple WWDC 24: https://developer.apple.com/wwdc24/
[13] Mistral: https://mistral.ai/
[14] Matrix rank: https://en.wikipedia.org/wiki/Rank_(linear_algebra)
[15] Pytorch: https://pytorch.org/
[16] QLoRA: Efficient Finetuning of Quantized LLMs: https://arxiv.org/abs/2305.14314
[17] Floating point representation: Wikibook
[18] Transformers: QLoRA + bitsandbytes
[19] Transformers: bitsandbytes

Ready for more?

Correlation and Matching Representations of Binocular Disparity across the Human Visual Cortex
- Find this author on Google Scholar
- Find this author on PubMed
- Search for this author on this site
- For correspondence: [email protected]
- Info/History
- Preview PDF
Seeing three-dimensional objects requires multiple stages of representational transformation, beginning in the primary visual cortex (V1). Here, neurons compute binocular disparity from the left and right retinal inputs through a mechanism similar to local cross-correlation. However, correlation-based representation is ambiguous because it is sensitive to disparities in both similar and dissimilar features between the eyes. Along the visual pathways, the representation transforms to a cross-matching basis, eliminating responses to falsely matched disparities. We investigated this transformation across the human visual areas using functional magnetic resonance imaging (fMRI) and computational modeling. By fitting a linear weighted sum of cross-correlation and cross-matching model representations to the brain′s representational structure of disparity, we found that areas V1-V3 exhibited stronger cross-correlation components, V3A/B, V7, and hV4 were slightly inclined towards cross-matching, and hMT+ was strongly engaged in cross-matching. To explore the underlying mechanism, we identified a deep neural network optimized for estimating disparity in natural scenes that matched human depth judgment in the random-dot stereograms used in the fMRI experiments. Despite not being constrained to match fMRI data, the network units' responses progressed from cross-correlation to cross-matching across layers. Activation maximization analysis on the network suggests that the transformation incorporates three phases, each emphasizing different aspects of binocular similarity and dissimilarity for depth extraction. Our findings suggest a systematic distribution of both components throughout the visual cortex, with cross-matching playing a greater role in areas anterior to V3, and that the transformation exploits responses to false matches rather than discarding them.
Competing Interest Statement
The authors have declared no competing interest.
https://github.com/wundari/CMM_model
View the discussion thread.
Thank you for your interest in spreading the word about bioRxiv.
NOTE: Your email address is requested solely to identify you as the sender of this article.

Citation Manager Formats
- EndNote (tagged)
- EndNote 8 (xml)
- RefWorks Tagged
- Ref Manager
- Tweet Widget
- Facebook Like
- Google Plus One
Subject Area
- Neuroscience
- Animal Behavior and Cognition (5516)
- Biochemistry (12543)
- Bioengineering (9415)
- Bioinformatics (30784)
- Biophysics (15831)
- Cancer Biology (12898)
- Cell Biology (18479)
- Clinical Trials (138)
- Developmental Biology (9988)
- Ecology (14945)
- Epidemiology (2067)
- Evolutionary Biology (19140)
- Genetics (12724)
- Genomics (17520)
- Immunology (12659)
- Microbiology (29670)
- Molecular Biology (12354)
- Neuroscience (64635)
- Paleontology (479)
- Pathology (1999)
- Pharmacology and Toxicology (3449)
- Physiology (5322)
- Plant Biology (11068)
- Scientific Communication and Education (1728)
- Synthetic Biology (3060)
- Systems Biology (7680)
- Zoology (1728)
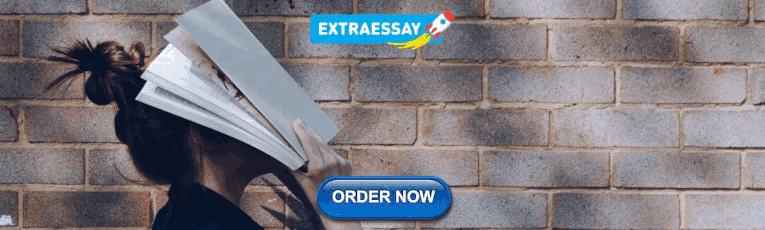
IMAGES
COMMENTS
The Power of Good Data Visualization. Data visualization involves the use of graphical representations of data, such as graphs, charts, and maps. Compared to descriptive statistics or tables, visuals provide a more effective way to analyze data, including identifying patterns, distributions, and correlations and spotting outliers in complex ...
Some data visualization tools, however, allow you to add interactivity to your map so the exact values are accessible. 15. Word Cloud. A word cloud, or tag cloud, is a visual representation of text data in which the size of the word is proportional to its frequency. The more often a specific word appears in a dataset, the larger it appears in ...
Data visualisation is the graphical representation of information and data. By using visual elements like charts, graphs and maps, data visualisation tools provide an accessible way to see and understand trends, outliers and patterns in data. In the world of big data, data visualisation tools and technologies are essential for analysing massive ...
What is data visualization? Data visualization is the graphical representation of information and data. By using visual elements like charts, graphs, and maps, data visualization tools provide an accessible way to see and understand trends, outliers, and patterns in data.Additionally, it provides an excellent way for employees or business owners to present data to non-technical audiences ...
Data visualization is the representation of information and data using charts, graphs, maps, and other visual tools. These visualizations allow us to easily understand any patterns, trends, or outliers in a data set. Data visualization also presents data to the general public or specific audiences without technical knowledge in an accessible ...
Data visualization is important because it breaks down complex data and extracts meaningful insights in a more digestible way. Displaying the data in a more engaging way helps audiences make sense of the information with a higher chance of retention. ... A histogram is a visual representation of the distribution of data. The graph itself ...
Data Visualization is a graphic representation of data that aims to communicate numerous heavy data in an efficient way that is easier to grasp and understand. In a way, data visualization is the mapping between the original data and graphic elements that determine how the attributes of these elements vary. The visualization is usually made by ...
Data visualization is the representation of data through use of common graphics, such as charts, plots, infographics and even animations. These visual displays of information communicate complex data relationships and data-driven insights in a way that is easy to understand.
Data visualization may be described as graphically representing data. It is the act of translating data into a visual context, which can be done using charts, plots, animations, infographics, etc. The idea behind it is to make it easier for us (humans) to identify trends, outliers, and patterns in data.
A simple definition of data visualization: Data visualization is the visual presentation of data or information. The goal of data visualization is to communicate data or information clearly and effectively to readers. Typically, data is visualized in the form of a chart, infographic, diagram or map. The field of data visualization combines both ...
What is Data Visualization? Data visualization is the graphical representation of different pieces of information or data, using visual elements such as charts, graphs, or maps. Data visualization tools provide the ability to see and understand data trends, outliers, and patterns in an easy, intuitive way. Learn more about data visualization.
A definition. Data visualization is the graphical or visual representation of data. It helps to highlight the most useful insights from a dataset, making it easier to spot trends, patterns, outliers, and correlations. Imagine you're presented with a spreadsheet containing rows and rows of data.
6 Real-World Data Visualization Examples. 1. The Most Common Jobs by State. Source: NPR. National Public Radio (NPR) produced a color-coded, interactive display of the most common jobs in each state in each year from 1978 to 2014. By dragging the scroll bar at the bottom of the map, you're able to visualize occupational changes over time.
2. Figure out what data you need to achieve your goal. Different types of charts and graphs use different kinds of data. Graphs usually represent numerical data, while charts are visual representations of data that may or may not use numbers. So, while all graphs are a type of chart, not all charts are graphs.
Incorporating visual data representation is a win-win." ... Personally, my business uses this form of visual data to compare the sales trends of different products and services for multiple age groups. Last but not least, pie charts are a game-changer when it comes to displaying proportional data or percentages. It gives meaningful context to ...
Data Modeling and Drill-Through Techniques. Data modeling plays a crucial role in data visualization. It's the process of creating a visual representation of data, which can help to understand complex patterns and relationships. Using data modeling effectively allows you to uncover insights that would be difficult to grasp in raw, unprocessed ...
The main goal of data visualization is that it helps unify and bring teams onto the same page. The human mind is wired to grasp visual information more effortlessly than raw data in spreadsheets or detailed reports. Thus, graphical representation of voluminous and intricate data is more user-friendly.
6. Scatter Plot. The scatter plot is also among the popular data visualization types and has other names such as a scatter diagram, scatter graph, and correlation chart. Scatter plot helps in many areas of today's world - business, biology, social statistics, data science and etc.
Summary. Not long ago, the ability to create smart data visualizations (or dataviz) was a nice-to-have skill for design- and data-minded managers. But now it's a must-have skill for all managers ...
v. t. e. Data and information visualization ( data viz/vis or info viz/vis) [ 2] is the practice of designing and creating easy-to-communicate and easy-to-understand graphic or visual representations of a large amount [ 3] of complex quantitative and qualitative data and information with the help of static, dynamic or interactive visual items.
Technique #1: Consider Your Audience. Technique #2: Choose the Right Data Visualization Tools. Technique #3: Choose Appropriate Charts and Graphs. Technique #4: Use Multiple Charts to Visualize Big Data. Technique #5: Use Color to Convey Meaning. Technique #6: Use 3D Assets. Technique #7: Incorporate Thematic Design.
1 Nasa's Eyes on Asteroids. Image Source. If you are interested in exploring data visualization topics in space exploration, check out this striking data visualization created by NASA. NASA's Eyes on Asteroids is one of the best data visualizations due to its exceptional design and functionality.
A diagram is a visual snapshot of information. Think of diagrams as visual representations of data or information that communicate a concept, idea, or process in a simplified and easily understandable way. You can also use them to illustrate relationships, hierarchies, cycles, or workflows.
2. Ignoring Visual Representations. Data represented in such vivid forms as graphs and dashboards empower decision makers to quickly and more effectively make conclusions without the need for ...
A network trained through visual experience must learn an internal coordinate system and representation x, θ that not only offers an environmental representation but also establishes a connection ...
Standard natural language processing (NLP) pipelines operate on symbolic representations of language, which typically consist of sequences of discrete tokens. However, creating an analogous representation for ancient logographic writing systems is an extremely labor intensive process that requires expert knowledge. At present, a large portion of logographic data persists in a purely visual ...
Storage Data Type: 4-bit NormalFloat (NF4). This is for the base model being fine-tuned which has its frozen weights quantized to NF4. Computation Data Type: 16-bit BrainFloat (BF16). When performing forward and backward passes, the storage data type is de-quantized (reverse of quantization) to 16-bit format.
Seeing three-dimensional objects requires multiple stages of representational transformation, beginning in the primary visual cortex (V1). Here, neurons compute binocular disparity from the left and right retinal inputs through a mechanism similar to local cross-correlation. However, correlation-based representation is ambiguous because it is sensitive to disparities in both similar and ...
It was the finest margins which determined the outcome, as Lyles covered the distance between 80-90 metres in 0.84 and the final 10 metres in 0.86 - compared to 0.85 and 0.87 for Thompson.