
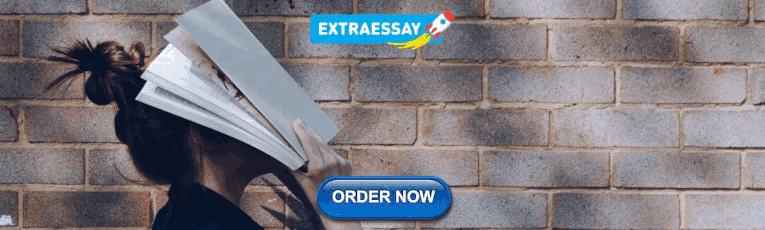
Community Blog
Keep up-to-date on postgraduate related issues with our quick reads written by students, postdocs, professors and industry leaders.
Statistical Treatment of Data – Explained & Example

- By DiscoverPhDs
- September 8, 2020
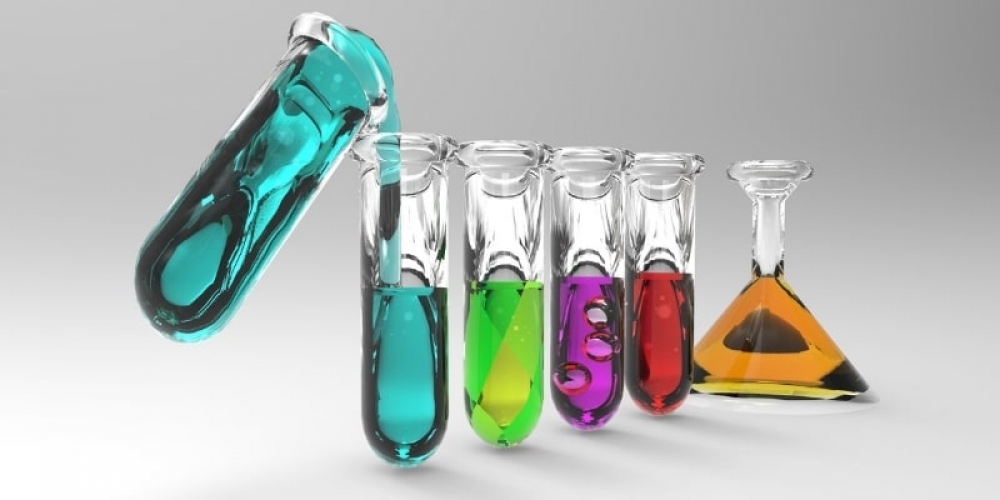
‘Statistical treatment’ is when you apply a statistical method to a data set to draw meaning from it. Statistical treatment can be either descriptive statistics, which describes the relationship between variables in a population, or inferential statistics, which tests a hypothesis by making inferences from the collected data.
Introduction to Statistical Treatment in Research
Every research student, regardless of whether they are a biologist, computer scientist or psychologist, must have a basic understanding of statistical treatment if their study is to be reliable.
This is because designing experiments and collecting data are only a small part of conducting research. The other components, which are often not so well understood by new researchers, are the analysis, interpretation and presentation of the data. This is just as important, if not more important, as this is where meaning is extracted from the study .
What is Statistical Treatment of Data?
Statistical treatment of data is when you apply some form of statistical method to a data set to transform it from a group of meaningless numbers into meaningful output.
Statistical treatment of data involves the use of statistical methods such as:
- regression,
- conditional probability,
- standard deviation and
- distribution range.
These statistical methods allow us to investigate the statistical relationships between the data and identify possible errors in the study.
In addition to being able to identify trends, statistical treatment also allows us to organise and process our data in the first place. This is because when carrying out statistical analysis of our data, it is generally more useful to draw several conclusions for each subgroup within our population than to draw a single, more general conclusion for the whole population. However, to do this, we need to be able to classify the population into different subgroups so that we can later break down our data in the same way before analysing it.
Statistical Treatment Example – Quantitative Research
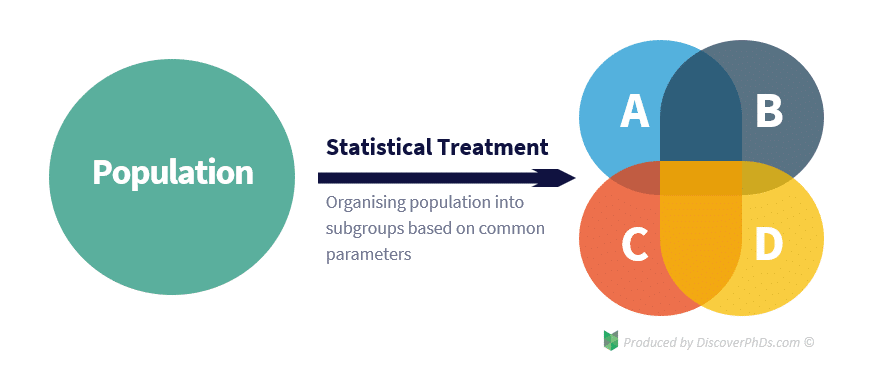
For a statistical treatment of data example, consider a medical study that is investigating the effect of a drug on the human population. As the drug can affect different people in different ways based on parameters such as gender, age and race, the researchers would want to group the data into different subgroups based on these parameters to determine how each one affects the effectiveness of the drug. Categorising the data in this way is an example of performing basic statistical treatment.
Type of Errors
A fundamental part of statistical treatment is using statistical methods to identify possible outliers and errors. No matter how careful we are, all experiments are subject to inaccuracies resulting from two types of errors: systematic errors and random errors.
Systematic errors are errors associated with either the equipment being used to collect the data or with the method in which they are used. Random errors are errors that occur unknowingly or unpredictably in the experimental configuration, such as internal deformations within specimens or small voltage fluctuations in measurement testing instruments.
These experimental errors, in turn, can lead to two types of conclusion errors: type I errors and type II errors . A type I error is a false positive which occurs when a researcher rejects a true null hypothesis. On the other hand, a type II error is a false negative which occurs when a researcher fails to reject a false null hypothesis.
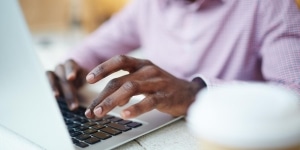
A concept paper is a short document written by a researcher before starting their research project, explaining what the study is about, why it is needed and the methods that will be used.

The title page of your dissertation or thesis conveys all the essential details about your project. This guide helps you format it in the correct way.
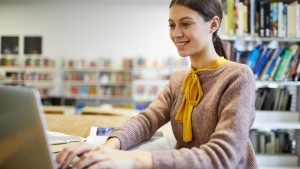
In this post you’ll learn what the significance of the study means, why it’s important, where and how to write one in your paper or thesis with an example.
Join thousands of other students and stay up to date with the latest PhD programmes, funding opportunities and advice.
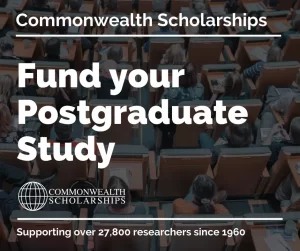
Browse PhDs Now
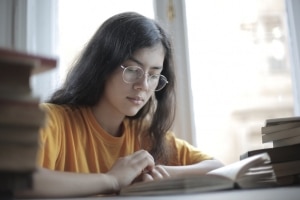
The unit of analysis refers to the main parameter that you’re investigating in your research project or study.
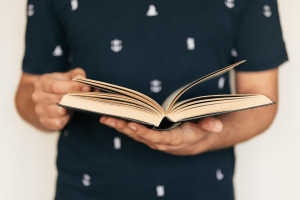
This article will answer common questions about the PhD synopsis, give guidance on how to write one, and provide my thoughts on samples.

Elpida is about to start her third year of PhD research at the University of Leicester. Her research focuses on preventing type 2 diabetes in women who had gestational diabetes, and she an active STEM Ambassador.
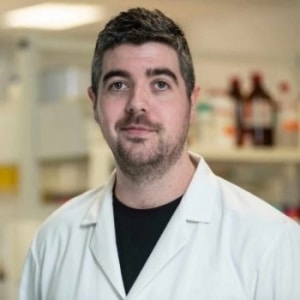
Chris is a third (and final) year PhD student at Ulster University. His project aims to develop a novel method of delivering antibiofilm compounds directly to an infected wound bed in patients.
Join Thousands of Students
Research Paper Statistical Treatment of Data: A Primer
We can all agree that analyzing and presenting data effectively in a research paper is critical, yet often challenging.
This primer on statistical treatment of data will equip you with the key concepts and procedures to accurately analyze and clearly convey research findings.
You'll discover the fundamentals of statistical analysis and data management, the common quantitative and qualitative techniques, how to visually represent data, and best practices for writing the results - all framed specifically for research papers.
If you are curious on how AI can help you with statistica analysis for research, check Hepta AI .
Introduction to Statistical Treatment in Research
Statistical analysis is a crucial component of both quantitative and qualitative research. Properly treating data enables researchers to draw valid conclusions from their studies. This primer provides an introductory guide to fundamental statistical concepts and methods for manuscripts.
Understanding the Importance of Statistical Treatment
Careful statistical treatment demonstrates the reliability of results and ensures findings are grounded in robust quantitative evidence. From determining appropriate sample sizes to selecting accurate analytical tests, statistical rigor adds credibility. Both quantitative and qualitative papers benefit from precise data handling.
Objectives of the Primer
This primer aims to equip researchers with best practices for:
Statistical tools to apply during different research phases
Techniques to manage, analyze, and present data
Methods to demonstrate the validity and reliability of measurements
By covering fundamental concepts ranging from descriptive statistics to measurement validity, it enables both novice and experienced researchers to incorporate proper statistical treatment.
Navigating the Primer: Key Topics and Audience
The primer spans introductory topics including:
Research planning and design
Data collection, management, analysis
Result presentation and interpretation
While useful for researchers at any career stage, earlier-career scientists with limited statistical exposure will find it particularly valuable as they prepare manuscripts.
How do you write a statistical method in a research paper?
Statistical methods are a critical component of research papers, allowing you to analyze, interpret, and draw conclusions from your study data. When writing the statistical methods section, you need to provide enough detail so readers can evaluate the appropriateness of the methods you used.
Here are some key things to include when describing statistical methods in a research paper:
Type of Statistical Tests Used
Specify the types of statistical tests performed on the data, including:
Parametric vs nonparametric tests
Descriptive statistics (means, standard deviations)
Inferential statistics (t-tests, ANOVA, regression, etc.)
Statistical significance level (often p < 0.05)
For example: We used t-tests and one-way ANOVA to compare means across groups, with statistical significance set at p < 0.05.
Analysis of Subgroups
If you examined subgroups or additional variables, describe the methods used for these analyses.
For example: We stratified data by gender and used chi-square tests to analyze differences between subgroups.
Software and Versions
List any statistical software packages used for analysis, including version numbers. Common programs include SPSS, SAS, R, and Stata.
For example: Data were analyzed using SPSS version 25 (IBM Corp, Armonk, NY).
The key is to give readers enough detail to assess the rigor and appropriateness of your statistical methods. The methods should align with your research aims and design. Keep explanations clear and concise using consistent terminology throughout the paper.
What are the 5 statistical treatment in research?
The five most common statistical treatments used in academic research papers include:
The mean, or average, is used to describe the central tendency of a dataset. It provides a singular value that represents the middle of a distribution of numbers. Calculating means allows researchers to characterize typical observations within a sample.
Standard Deviation
Standard deviation measures the amount of variability in a dataset. A low standard deviation indicates observations are clustered closely around the mean, while a high standard deviation signifies the data is more spread out. Reporting standard deviations helps readers contextualize means.
Regression Analysis
Regression analysis models the relationship between independent and dependent variables. It generates an equation that predicts changes in the dependent variable based on changes in the independents. Regressions are useful for hypothesizing causal connections between variables.
Hypothesis Testing
Hypothesis testing evaluates assumptions about population parameters based on statistics calculated from a sample. Common hypothesis tests include t-tests, ANOVA, and chi-squared. These quantify the likelihood of observed differences being due to chance.
Sample Size Determination
Sample size calculations identify the minimum number of observations needed to detect effects of a given size at a desired statistical power. Appropriate sampling ensures studies can uncover true relationships within the constraints of resource limitations.
These five statistical analysis methods form the backbone of most quantitative research processes. Correct application allows researchers to characterize data trends, model predictive relationships, and make probabilistic inferences regarding broader populations. Expertise in these techniques is fundamental for producing valid, reliable, and publishable academic studies.
How do you know what statistical treatment to use in research?
The selection of appropriate statistical methods for the treatment of data in a research paper depends on three key factors:
The Aim and Objective of the Study
The aim and objectives that the study seeks to achieve will determine the type of statistical analysis required.
Descriptive research presenting characteristics of the data may only require descriptive statistics like measures of central tendency (mean, median, mode) and dispersion (range, standard deviation).
Studies aiming to establish relationships or differences between variables need inferential statistics like correlation, t-tests, ANOVA, regression etc.
Predictive modeling research requires methods like regression, discriminant analysis, logistic regression etc.
Thus, clearly identifying the research purpose and objectives is the first step in planning appropriate statistical treatment.
Type and Distribution of Data
The type of data (categorical, numerical) and its distribution (normal, skewed) also guide the choice of statistical techniques.
Parametric tests have assumptions related to normality and homogeneity of variance.
Non-parametric methods are distribution-free and better suited for non-normal or categorical data.
Testing data distribution and characteristics is therefore vital.
Nature of Observations
Statistical methods also differ based on whether the observations are paired or unpaired.
Analyzing changes within one group requires paired tests like paired t-test, Wilcoxon signed-rank test etc.
Comparing between two or more independent groups needs unpaired tests like independent t-test, ANOVA, Kruskal-Wallis test etc.
Thus the nature of observations is pivotal in selecting suitable statistical analyses.
In summary, clearly defining the research objectives, testing the collected data, and understanding the observational units guides proper statistical treatment and interpretation.
What is statistical techniques in research paper?
Statistical methods are essential tools in scientific research papers. They allow researchers to summarize, analyze, interpret and present data in meaningful ways.
Some key statistical techniques used in research papers include:
Descriptive statistics: These provide simple summaries of the sample and the measures. Common examples include measures of central tendency (mean, median, mode), measures of variability (range, standard deviation) and graphs (histograms, pie charts).
Inferential statistics: These help make inferences and predictions about a population from a sample. Common techniques include estimation of parameters, hypothesis testing, correlation and regression analysis.
Analysis of variance (ANOVA): This technique allows researchers to compare means across multiple groups and determine statistical significance.
Factor analysis: This technique identifies underlying relationships between variables and latent constructs. It allows reducing a large set of variables into fewer factors.
Structural equation modeling: This technique estimates causal relationships using both latent and observed factors. It is widely used for testing theoretical models in social sciences.
Proper statistical treatment and presentation of data are crucial for the integrity of any quantitative research paper. Statistical techniques help establish validity, account for errors, test hypotheses, build models and derive meaningful insights from the research.
Fundamental Concepts and Data Management
Exploring basic statistical terms.
Understanding key statistical concepts is essential for effective research design and data analysis. This includes defining key terms like:
Statistics : The science of collecting, organizing, analyzing, and interpreting numerical data to draw conclusions or make predictions.
Variables : Characteristics or attributes of the study participants that can take on different values.
Measurement : The process of assigning numbers to variables based on a set of rules.
Sampling : Selecting a subset of a larger population to estimate characteristics of the whole population.
Data types : Quantitative (numerical) or qualitative (categorical) data.
Descriptive vs. inferential statistics : Descriptive statistics summarize data while inferential statistics allow making conclusions from the sample to the larger population.
Ensuring Validity and Reliability in Measurement
When selecting measurement instruments, it is critical they demonstrate:
Validity : The extent to which the instrument measures what it intends to measure.
Reliability : The consistency of measurement over time and across raters.
Researchers should choose instruments aligned to their research questions and study methodology .
Data Management Essentials
Proper data management requires:
Ethical collection procedures respecting autonomy, justice, beneficence and non-maleficence.
Handling missing data through deletion, imputation or modeling procedures.
Data cleaning by identifying and fixing errors, inconsistencies and duplicates.
Data screening via visual inspection and statistical methods to detect anomalies.
Data Management Techniques and Ethical Considerations
Ethical data management includes:
Obtaining informed consent from all participants.
Anonymization and encryption to protect privacy.
Secure data storage and transfer procedures.
Responsible use of statistical tools free from manipulation or misrepresentation.
Adhering to ethical guidelines preserves public trust in the integrity of research.
Statistical Methods and Procedures
This section provides an introduction to key quantitative analysis techniques and guidance on when to apply them to different types of research questions and data.
Descriptive Statistics and Data Summarization
Descriptive statistics summarize and organize data characteristics such as central tendency, variability, and distributions. Common descriptive statistical methods include:
Measures of central tendency (mean, median, mode)
Measures of variability (range, interquartile range, standard deviation)
Graphical representations (histograms, box plots, scatter plots)
Frequency distributions and percentages
These methods help describe and summarize the sample data so researchers can spot patterns and trends.
Inferential Statistics for Generalizing Findings
While descriptive statistics summarize sample data, inferential statistics help generalize findings to the larger population. Common techniques include:
Hypothesis testing with t-tests, ANOVA
Correlation and regression analysis
Nonparametric tests
These methods allow researchers to draw conclusions and make predictions about the broader population based on the sample data.
Selecting the Right Statistical Tools
Choosing the appropriate analyses involves assessing:
The research design and questions asked
Type of data (categorical, continuous)
Data distributions
Statistical assumptions required
Matching the correct statistical tests to these elements helps ensure accurate results.
Statistical Treatment of Data for Quantitative Research
For quantitative research, common statistical data treatments include:
Testing data reliability and validity
Checking assumptions of statistical tests
Transforming non-normal data
Identifying and handling outliers
Applying appropriate analyses for the research questions and data type
Examples and case studies help demonstrate correct application of statistical tests.
Approaches to Qualitative Data Analysis
Qualitative data is analyzed through methods like:
Thematic analysis
Content analysis
Discourse analysis
Grounded theory
These help researchers discover concepts and patterns within non-numerical data to derive rich insights.
Data Presentation and Research Method
Crafting effective visuals for data presentation.
When presenting analyzed results and statistics in a research paper, well-designed tables, graphs, and charts are key for clearly showcasing patterns in the data to readers. Adhering to formatting standards like APA helps ensure professional data presentation. Consider these best practices:
Choose the appropriate visual type based on the type of data and relationship being depicted. For example, bar charts for comparing categorical data, line graphs to show trends over time.
Label the x-axis, y-axis, legends clearly. Include informative captions.
Use consistent, readable fonts and sizing. Avoid clutter with unnecessary elements. White space can aid readability.
Order data logically. Such as largest to smallest values, or chronologically.
Include clear statistical notations, like error bars, where applicable.
Following academic standards for visuals lends credibility while making interpretation intuitive for readers.
Writing the Results Section with Clarity
When writing the quantitative Results section, aim for clarity by balancing statistical reporting with interpretation of findings. Consider this structure:
Open with an overview of the analysis approach and measurements used.
Break down results by logical subsections for each hypothesis, construct measured etc.
Report exact statistics first, followed by interpretation of their meaning. For example, “Participants exposed to the intervention had significantly higher average scores (M=78, SD=3.2) compared to controls (M=71, SD=4.1), t(115)=3.42, p = 0.001. This suggests the intervention was highly effective for increasing scores.”
Use present verb tense. And scientific, formal language.
Include tables/figures where they aid understanding or visualization.
Writing results clearly gives readers deeper context around statistical findings.
Highlighting Research Method and Design
With a results section full of statistics, it's vital to communicate key aspects of the research method and design. Consider including:
Brief overview of study variables, materials, apparatus used. Helps reproducibility.
Descriptions of study sampling techniques, data collection procedures. Supports transparency.
Explanations around approaches to measurement, data analysis performed. Bolsters methodological rigor.
Noting control variables, attempts to limit biases etc. Demonstrates awareness of limitations.
Covering these methodological details shows readers the care taken in designing the study and analyzing the results obtained.
Acknowledging Limitations and Addressing Biases
Honestly recognizing methodological weaknesses and limitations goes a long way in establishing credibility within the published discussion section. Consider transparently noting:
Measurement errors and biases that may have impacted findings.
Limitations around sampling methods that constrain generalizability.
Caveats related to statistical assumptions, analysis techniques applied.
Attempts made to control/account for biases and directions for future research.
Rather than detracting value, acknowledging limitations demonstrates academic integrity regarding the research performed. It also gives readers deeper insight into interpreting the reported results and findings.
Conclusion: Synthesizing Statistical Treatment Insights
Recap of statistical treatment fundamentals.
Statistical treatment of data is a crucial component of high-quality quantitative research. Proper application of statistical methods and analysis principles enables valid interpretations and inferences from study data. Key fundamentals covered include:
Descriptive statistics to summarize and describe the basic features of study data
Inferential statistics to make judgments of the probability and significance based on the data
Using appropriate statistical tools aligned to the research design and objectives
Following established practices for measurement techniques, data collection, and reporting
Adhering to these core tenets ensures research integrity and allows findings to withstand scientific scrutiny.
Key Takeaways for Research Paper Success
When incorporating statistical treatment into a research paper, keep these best practices in mind:
Clearly state the research hypothesis and variables under examination
Select reliable and valid quantitative measures for assessment
Determine appropriate sample size to achieve statistical power
Apply correct analytical methods suited to the data type and distribution
Comprehensively report methodology procedures and statistical outputs
Interpret results in context of the study limitations and scope
Following these guidelines will bolster confidence in the statistical treatment and strengthen the research quality overall.
Encouraging Continued Learning and Application
As statistical techniques continue advancing, it is imperative for researchers to actively further their statistical literacy. Regularly reviewing new methodological developments and learning advanced tools will augment analytical capabilities. Persistently putting enhanced statistical knowledge into practice through research projects and manuscript preparations will cement competencies. Statistical treatment mastery is a journey requiring persistent effort, but one that pays dividends in research proficiency.

Antonio Carlos Filho @acfilho_dev
Statistical Treatment
Statistics Definitions > Statistical Treatment
What is Statistical Treatment?
Statistical treatment can mean a few different things:
- In Data Analysis : Applying any statistical method — like regression or calculating a mean — to data.
- In Factor Analysis : Any combination of factor levels is called a treatment.
- In a Thesis or Experiment : A summary of the procedure, including statistical methods used.
1. Statistical Treatment in Data Analysis
The term “statistical treatment” is a catch all term which means to apply any statistical method to your data. Treatments are divided into two groups: descriptive statistics , which summarize your data as a graph or summary statistic and inferential statistics , which make predictions and test hypotheses about your data. Treatments could include:
- Finding standard deviations and sample standard errors ,
- Finding T-Scores or Z-Scores .
- Calculating Correlation coefficients .
2. Treatments in Factor Analysis
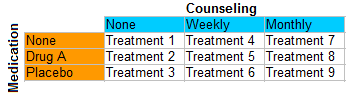
3. Treatments in a Thesis or Experiment
Sometimes you might be asked to include a treatment as part of a thesis. This is asking you to summarize the data and analysis portion of your experiment, including measurements and formulas used. For example, the following experimental summary is from Statistical Treatment in Acta Physiologica Scandinavica. :
Each of the test solutions was injected twice in each subject…30-42 values were obtained for the intensity, and a like number for the duration, of the pain indiced by the solution. The pain values reported in the following are arithmetical means for these 30-42 injections.”
The author goes on to provide formulas for the mean, the standard deviation and the standard error of the mean.
Vogt, W.P. (2005). Dictionary of Statistics & Methodology: A Nontechnical Guide for the Social Sciences . SAGE. Wheelan, C. (2014). Naked Statistics . W. W. Norton & Company Unknown author (1961) Chapter 3: Statistical Treatment. Acta Physiologica Scandinavica. Volume 51, Issue s179 December Pages 16–20.
Loading metrics
Open Access
Ten Simple Rules for Effective Statistical Practice
Affiliation Department of Statistics, Machine Learning Department, and Center for the Neural Basis of Cognition, Carnegie Mellon University, Pittsburgh, Pennsylvania, United States of America
Affiliation Department of Biostatistics, Bloomberg School of Public Health, Johns Hopkins University, Baltimore, Maryland, United States of America
Affiliation Department of Statistics, North Carolina State University, Raleigh, North Carolina, United States of America

Affiliation Department of Statistics, Harvard University, Cambridge, Massachusetts, United States of America
Affiliation Department of Statistics and Department of Electrical Engineering and Computer Science, University of California Berkeley, Berkeley, California, United States of America
* E-mail: [email protected]
Affiliation Department of Statistical Sciences, University of Toronto, Toronto, Ontario, Canada
- Robert E. Kass,
- Brian S. Caffo,
- Marie Davidian,
- Xiao-Li Meng,
- Bin Yu,
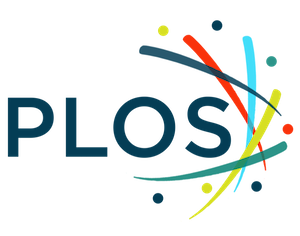
Published: June 9, 2016
- https://doi.org/10.1371/journal.pcbi.1004961
- Reader Comments
Citation: Kass RE, Caffo BS, Davidian M, Meng X-L, Yu B, Reid N (2016) Ten Simple Rules for Effective Statistical Practice. PLoS Comput Biol 12(6): e1004961. https://doi.org/10.1371/journal.pcbi.1004961
Editor: Fran Lewitter, Whitehead Institute, UNITED STATES
Copyright: © 2016 Kass et al. This is an open access article distributed under the terms of the Creative Commons Attribution License , which permits unrestricted use, distribution, and reproduction in any medium, provided the original author and source are credited.
Funding: BSC's research is partially supported by the National Institutes of Health grant EB012547: www.nibib.nih.gov . MD's research is partially supported by the National Institutes of Health grant NIH P01 CA142538: www.nih.gov . REK's research is partially supported by the National Institute of Mental Health R01 MH064537: www.nimh.nih.gov . NR's research is partially supported by the Natural Sciences and Engineering Council of Canada grant RGPIN-2015-06390: www.nserc.ca . BY's research is partially supported by the National Science Foundation grant CCF-0939370: www.nsf.gov . XLM's research is partially supported by the National Science Foundation ( www.nsf.gov ) DMS 1513492. The funders had no role in study design, data collection and analysis, decision to publish, or preparation of the manuscript.
Competing interests: The authors have declared that no competing interests exist.
Introduction
Several months ago, Phil Bourne, the initiator and frequent author of the wildly successful and incredibly useful “Ten Simple Rules” series, suggested that some statisticians put together a Ten Simple Rules article related to statistics. (One of the rules for writing a PLOS Ten Simple Rules article is to be Phil Bourne [ 1 ]. In lieu of that, we hope effusive praise for Phil will suffice.)
Implicit in the guidelines for writing Ten Simple Rules [ 1 ] is “know your audience.” We developed our list of rules with researchers in mind: researchers having some knowledge of statistics, possibly with one or more statisticians available in their building, or possibly with a healthy do-it-yourself attitude and a handful of statistical packages on their laptops. We drew on our experience in both collaborative research and teaching, and, it must be said, from our frustration at being asked, more than once, to “take a quick look at my student’s thesis/my grant application/my referee’s report: it needs some input on the stats, but it should be pretty straightforward.”
There are some outstanding resources available that explain many of these concepts clearly and in much more detail than we have been able to do here: among our favorites are Cox and Donnelly [ 2 ], Leek [ 3 ], Peng [ 4 ], Kass et al. [ 5 ], Tukey [ 6 ], and Yu [ 7 ].
Every article on statistics requires at least one caveat. Here is ours: we refer in this article to “science” as a convenient shorthand for investigations using data to study questions of interest. This includes social science, engineering, digital humanities, finance, and so on. Statisticians are not shy about reminding administrators that statistical science has an impact on nearly every part of almost all organizations.
Rule 1: Statistical Methods Should Enable Data to Answer Scientific Questions
A big difference between inexperienced users of statistics and expert statisticians appears as soon as they contemplate the uses of some data. While it is obvious that experiments generate data to answer scientific questions, inexperienced users of statistics tend to take for granted the link between data and scientific issues and, as a result, may jump directly to a technique based on data structure rather than scientific goal. For example, if the data were in a table, as for microarray gene expression data, they might look for a method by asking, “Which test should I use?” while a more experienced person would, instead, start with the underlying question, such as, “Where are the differentiated genes?” and, from there, would consider multiple ways the data might provide answers. Perhaps a formal statistical test would be useful, but other approaches might be applied as alternatives, such as heat maps or clustering techniques. Similarly, in neuroimaging, understanding brain activity under various experimental conditions is the main goal; illustrating this with nice images is secondary. This shift in perspective from statistical technique to scientific question may change the way one approaches data collection and analysis. After learning about the questions, statistical experts discuss with their scientific collaborators the ways that data might answer these questions and, thus, what kinds of studies might be most useful. Together, they try to identify potential sources of variability and what hidden realities could break the hypothesized links between data and scientific inferences; only then do they develop analytic goals and strategies. This is a major reason why collaborating with statisticians can be helpful, and also why the collaborative process works best when initiated early in an investigation. See Rule 3 .
Rule 2: Signals Always Come with Noise
Grappling with variability is central to the discipline of statistics. Variability comes in many forms. In some cases variability is good, because we need variability in predictors to explain variability in outcomes. For example, to determine if smoking is associated with lung cancer, we need variability in smoking habits; to find genetic associations with diseases, we need genetic variation. Other times variability may be annoying, such as when we get three different numbers when measuring the same thing three times. This latter variability is usually called “noise,” in the sense that it is either not understood or thought to be irrelevant. Statistical analyses aim to assess the signal provided by the data, the interesting variability, in the presence of noise, or irrelevant variability.
A starting point for many statistical procedures is to introduce a mathematical abstraction: outcomes, such as patients being diagnosed with specific diseases or receiving numerical scores on diagnostic tests, will vary across the set of individuals being studied, and statistical formalism describes such variation using probability distributions. Thus, for example, a data histogram might be replaced, in theory, by a probability distribution, thereby shifting attention from the raw data to the numerical parameters that determine the precise features of the probability distribution, such as its shape, its spread, or the location of its center. Probability distributions are used in statistical models, with the model specifying the way signal and noise get combined in producing the data we observe, or would like to observe. This fundamental step makes statistical inferences possible. Without it, every data value would be considered unique, and we would be left trying to figure out all the detailed processes that might cause an instrument to give different values when measuring the same thing several times. Conceptualizing signal and noise in terms of probability within statistical models has proven to be an extremely effective simplification, allowing us to capture the variability in data in order to express uncertainty about quantities we are trying to understand. The formalism can also help by directing us to look for likely sources of systematic error, known as bias.
Big data makes these issues more important, not less. For example, Google Flu Trends debuted to great excitement in 2008, but turned out to overestimate the prevalence of influenza by nearly 50%, largely due to bias caused by the way the data were collected; see Harford [ 8 ], for example.
Rule 3: Plan Ahead, Really Ahead
When substantial effort will be involved in collecting data, statistical issues may not be captured in an isolated statistical question such as, “What should my n be?” As we suggested in Rule 1, rather than focusing on a specific detail in the design of the experiment, someone with a lot of statistical experience is likely to step back and consider many aspects of data collection in the context of overall goals and may start by asking, “What would be the ideal outcome of your experiment, and how would you interpret it?” In trying to determine whether observations of X and Y tend to vary together, as opposed to independently, key issues would involve the way X and Y are measured, the extent to which the measurements represent the underlying conceptual meanings of X and Y, the many factors that could affect the measurements, the ability to control those factors, and whether some of those factors might introduce systematic errors (bias).
In Rule 2 we pointed out that statistical models help link data to goals by shifting attention to theoretical quantities of interest. For example, in making electrophysiological measurements from a pair of neurons, a neurobiologist may take for granted a particular measurement methodology along with the supposition that these two neurons will represent a whole class of similar neurons under similar experimental conditions. On the other hand, a statistician will immediately wonder how the specific measurements get at the issue of co-variation; what the major influences on the measurements are, and whether some of them can be eliminated by clever experimental design; what causes variation among repeated measurements, and how quantitative knowledge about sources of variation might influence data collection; and whether these neurons may be considered to be sampled from a well-defined population, and how the process of picking that pair could influence subsequent statistical analyses. A conversation that covers such basic issues may reveal possibilities an experimenter has not yet considered.
Asking questions at the design stage can save headaches at the analysis stage: careful data collection can greatly simplify analysis and make it more rigorous. Or, as Sir Ronald Fisher put it: “To consult the statistician after an experiment is finished is often merely to ask him to conduct a post mortem examination. He can perhaps say what the experiment died of” [ 9 ]. As a good starting point for reading on planning of investigations, see Chapters 1 through 4 of [ 2 ].
Rule 4: Worry about Data Quality
Well-trained experimenters understand instinctively that, when it comes to data analysis, “garbage in produces garbage out.” However, the complexity of modern data collection requires many assumptions about the function of technology, often including data pre-processing technology. It is highly advisable to approach pre-processing with care, as it can have profound effects that easily go unnoticed.
Even with pre-processed data, further considerable effort may be needed prior to analysis; this is variously called “data cleaning,” “data munging,” or “data carpentry.” Hands-on experience can be extremely useful, as data cleaning often reveals important concerns about data quality, in the best case confirming that what was measured is indeed what was intended to be measured and, in the worst case, ensuring that losses are cut early.
Units of measurement should be understood and recorded consistently. It is important that missing data values can be recognized as such by relevant software. For example, 999 may signify the number 999, or it could be code for “we have no clue.” There should be a defensible rule for handling situations such as “non-detects,” and data should be scanned for anomalies such as variable 27 having half its values equal to 0.00027. Try to understand as much as you can how these data arrived at your desk or disk. Why are some data missing or incomplete? Did they get lost through some substantively relevant mechanism? Understanding such mechanisms can help to avoid some seriously misleading results. For example, in a developmental imaging study of attention deficit hyperactivity disorder, might some data have been lost from children with the most severe hyperactivity because they could not sit still in the MR scanner?
Once the data have been wrestled into a convenient format, have a look! Tinkering around with the data, also known as exploratory data analysis, is often the most informative part of the analysis. Exploratory plots can reveal data quality issues and outliers. Simple summaries, such as means, standard deviations, and quantiles, can help refine thinking and offer face validity checks for hypotheses. Many studies, especially when going in completely new scientific directions, are exploratory by design; the area may be too novel to include clear a priori hypotheses. Working with the data informally can help generate new hypotheses and ideas. However, it is also important to acknowledge the specific ways data are selected prior to formal analyses and to consider how such selection might affect conclusions. And it is important to remember that using a single set of data to both generate and test hypotheses is problematic. See Rule 9 .
Rule 5: Statistical Analysis Is More Than a Set of Computations
Statistical software provides tools to assist analyses, not define them. The scientific context is critical, and the key to principled statistical analysis is to bring analytic methods into close correspondence with scientific questions. See Rule 1 . While it can be helpful to include references to a specific algorithm or piece of software in the Methods section of a paper, this should not be a substitute for an explanation of the choice of statistical method in answering a question. A reader will likely want to consider the fundamental issue of whether the analytic technique is appropriately linked to the substantive questions being answered. Don’t make the reader puzzle over this: spell it out clearly.
At the same time, a structured algorithmic approach to the steps in your analysis can be very helpful in making this analysis reproducible by yourself at a later time, or by others with the same or similar data. See Rule 10 .
Rule 6: Keep it Simple
All else being equal, simplicity trumps complexity. This rule has been rediscovered and enshrined in operating procedures across many domains and variously described as “Occam’s razor,” “KISS,” “less is more,” and “simplicity is the ultimate sophistication.” The principle of parsimony can be a trusted guide: start with simple approaches and only add complexity as needed, and then only add as little as seems essential.
Having said this, scientific data have detailed structure, and simple models can’t always accommodate important intricacies. The common assumption of independence is often incorrect and nearly always needs careful examination. See Rule 8 . Large numbers of measurements, interactions among explanatory variables, nonlinear mechanisms of action, missing data, confounding, sampling biases, and so on, can all require an increase in model complexity.
Keep in mind that good design, implemented well, can often allow simple methods of analysis to produce strong results. See Rule 3 . Simple models help us to create order out of complex phenomena, and simple models are well suited for communication to our colleagues and the wider world.
Rule 7: Provide Assessments of Variability
Nearly all biological measurements, when repeated, exhibit substantial variation, and this creates uncertainty in the result of every calculation based on the data. A basic purpose of statistical analysis is to help assess uncertainty, often in the form of a standard error or confidence interval, and one of the great successes of statistical modeling and inference is that it can provide estimates of standard errors from the same data that produce estimates of the quantity of interest. When reporting results, it is essential to supply some notion of statistical uncertainty. A common mistake is to calculate standard errors without taking into account the dependencies among data or variables, which usually means a substantial underestimate of the real uncertainty. See Rule 8 .
Remember that every number obtained from the data by some computation would change somewhat, even if the measurements were repeated on the same biological material. If you are using new material, you can add to the measurement variability an increase due to the natural variability among samples. If you are collecting data on a different day, in a different lab, or under a slightly changed protocol, there are now three more potential sources of variability to be accounted for. In microarray analysis, batch effects are well known to introduce extra variability, and several methods are available to filter these. Extra variability means extra uncertainty in the conclusions, and this uncertainty needs to be reported. Such reporting is invaluable for planning the next investigation.
It is a very common feature of big data that uncertainty assessments tend to be overly optimistic (Cox [ 10 ], Meng [ 11 ]). For an instructive, and beguilingly simple, quantitative analysis most relevant to surveys, see the “data defect” section of [ 11 ]. Big data is not always as big as it looks: a large number of measurements on a small number of samples requires very careful estimation of the standard error, not least because these measurements are quite likely to be dependent.
Rule 8: Check Your Assumptions
Every statistical inference involves assumptions, which are based on substantive knowledge and some probabilistic representation of data variation—this is what we call a statistical model. Even the so-called “model-free” techniques require assumptions, albeit less restrictive assumptions, so this terminology is somewhat misleading.
The most common statistical methods involve an assumption of linear relationships. For example, the ordinary correlation coefficient, also called the Pearson correlation, is a measure of linear association. Linearity often works well as a first approximation or as a depiction of a general trend, especially when the amount of noise in the data makes it difficult to distinguish between linear and nonlinear relationships. However, for any given set of data, the appropriateness of the linear model is an empirical issue and should be investigated.
In many ways, a more worrisome, and very common, assumption in statistical analysis is that multiple observations in the data are statistically independent. This is worrisome because relatively small deviations from this assumption can have drastic effects. When measurements are made across time, for example, the temporal sequencing may be important; if it is, specialized methods appropriate for time series need to be considered.
In addition to nonlinearity and statistical dependence, missing data, systematic biases in measurements, and a variety of other factors can cause violations of statistical modeling assumptions, even in the best experiments. Widely available statistical software makes it easy to perform analyses without careful attention to inherent assumptions, and this risks inaccurate, or even misleading, results. It is therefore important to understand the assumptions embodied in the methods you are using and to do whatever you can to understand and assess those assumptions. At a minimum, you will want to check how well your statistical model fits the data. Visual displays and plots of data and residuals from fitting are helpful for evaluating the relevance of assumptions and the fit of the model, and some basic techniques for assessing model fit are available in most statistical software. Remember, though, that several models can “pass the fit test” on the same data. See Rule 1 and Rule 6 .
Rule 9: When Possible, Replicate!
Every good analyst examines the data at great length, looking for patterns of many types and searching for predicted and unpredicted results. This process often involves dozens of procedures, including many alternative visualizations and a host of numerical slices through the data. Eventually, some particular features of the data are deemed interesting and important, and these are often the results reported in the resulting publication.
When statistical inferences, such as p -values, follow extensive looks at the data, they no longer have their usual interpretation. Ignoring this reality is dishonest: it is like painting a bull’s eye around the landing spot of your arrow. This is known in some circles as p -hacking, and much has been written about its perils and pitfalls: see, for example, [ 12 ] and [ 13 ].
Recently there has been a great deal of criticism of the use of p -values in science, largely related to the misperception that results can’t be worthy of publication unless “ p is less than 0.05.” The recent statement from the American Statistical Association (ASA) [ 14 ] presents a detailed view of the merits and limitations of the p -value.
Statisticians tend to be aware of the most obvious kinds of data snooping, such as choosing particular variables for a reported analysis, and there are methods that can help adjust results in these cases; the False Discovery Rate method of Benjamini and Hochberg [ 15 ] is the basis for several of these.
For some analyses, there may be a case that some kinds of preliminary data manipulation are likely to be innocuous. In other situations, analysts may build into their work an informal check by trusting only extremely small p -values. For example, in high energy physics, the requirement of a “5-sigma” result is at least partly an approximate correction for what is called the “look-elsewhere effect.”
The only truly reliable solution to the problem posed by data snooping is to record the statistical inference procedures that produced the key results, together with the features of the data to which they were applied, and then to replicate the same analysis using new data. Independent replications of this type often go a step further by introducing modifications to the experimental protocol, so that the replication will also provide some degree of robustness to experimental details.
Ideally, replication is performed by an independent investigator. The scientific results that stand the test of time are those that get confirmed across a variety of different, but closely related, situations. In the absence of experimental replications, appropriate forms of data perturbation can be helpful (Yu [ 16 ]). In many contexts, complete replication is very difficult or impossible, as in large-scale experiments such as multi-center clinical trials. In such cases, a minimum standard would be to follow Rule 10.
Rule 10: Make Your Analysis Reproducible
In our current framework for publication of scientific results, the independent replication discussed in Rule 9 is not practical for most investigators. A different standard, which is easier to achieve, is reproducibility: given the same set of data, together with a complete description of the analysis, it should be possible to reproduce the tables, figures, and statistical inferences. However, even this lower standard can face multiple barriers, such as different computing architectures, software versions, and settings.
One can dramatically improve the ability to reproduce findings by being very systematic about the steps in the analysis (see Rule 5 ), by sharing the data and code used to produce the results, and by following Goodman et al. [ 17 ]. Modern reproducible research tools like Sweave [ 18 ], knitr [ 19 ], and iPython [ 20 ] notebooks take this a step further and combine the research report with the code. Reproducible research is itself an ongoing area of research and a very important area that we all need to pay attention to.
Mark Twain popularized the saying, “There are three kinds of lies: lies, damned lies, and statistics.” It is true that data are frequently used selectively to give arguments a false sense of support. Knowingly misusing data or concealing important information about the way data and data summaries have been obtained is, of course, highly unethical. More insidious, however, are the widespread instances of claims made about scientific hypotheses based on well-intentioned yet faulty statistical reasoning. One of our chief aims here has been to emphasize succinctly many of the origins of such problems and ways to avoid the pitfalls.
A central and common task for us as research investigators is to decipher what our data are able to say about the problems we are trying to solve. Statistics is a language constructed to assist this process, with probability as its grammar. While rudimentary conversations are possible without good command of the language (and are conducted routinely), principled statistical analysis is critical in grappling with many subtle phenomena to ensure that nothing serious will be lost in translation and to increase the likelihood that your research findings will stand the test of time. To achieve full fluency in this mathematically sophisticated language requires years of training and practice, but we hope the Ten Simple Rules laid out here will provide some essential guidelines.
Among the many articles reporting on the ASA’s statement on p- values, we particularly liked a quote from biostatistician Andrew Vickers in [ 21 ]: “Treat statistics as a science, not a recipe.” This is a great candidate for Rule 0.
Acknowledgments
We consulted many colleagues informally about this article, but the opinions expressed here are unique to our small committee of authors. We’d like to give a shout out to xkcd.com for conveying statistical ideas with humor, to the Simply Statistics blog as a reliable source for thoughtful commentary, to FiveThirtyEight for bringing statistics to the world (or at least to the media), to Phil Bourne for suggesting that we put together this article, and to Steve Pierson of the American Statistical Association for getting the effort started.
- View Article
- PubMed/NCBI
- Google Scholar
- 2. Cox DR, Donnelly CA (2011) Principles of Applied Statistics. Cambridge: Cambridge University Press.
- 3. Leek JT (2015) The Elements of Data Analytic Style. Leanpub, https://leanpub.com/artofdatascience .
- 4. Peng R (2014) The Art of Data Science. Leanpub, https://leanpub.com/artofdatascience .
- 5. Kass RE, Eden UT, Brown EN (2014) Analysis of Neural Data. Springer: New York.
- 11. Meng XL (2014) A trio of inference problems that could win you a Nobel prize in statistics (if you help fund it). In: Lin X, Genest C, Banks DL, Molenberghs G, Scott DW, Wang J-L,editors. Past, Present, and Future of Statistical Science, Boca Raton: CRC Press. pp. 537–562.
- 13. Aschwanden C (2015) Science isn’t broken. August 11 2015 http://fivethirtyeight.com/features/science-isnt-broken/
- 16. Yu, B (2015) Data wisdom for data science. April 13 2015 http://www.odbms.org/2015/04/data-wisdom-for-data-science/
- 18. Leisch F (2002) Sweave: Dynamic generation of statistical reports using data analysis. In Härdle W, Rönz H, editors. Compstat: Proceedings in Computational Statistics, Heidelberg: Springer-Verlag, pp. 575–580.
- 19. Xie Y (2014) Dynamic Documents with R and knitr. Boca Raton: CRC Press.
13. Study design and choosing a statistical test
Sample size.

- Foundations
- Write Paper
Search form
- Experiments
- Anthropology
- Self-Esteem
- Social Anxiety
- Statistics >
Statistical Treatment Of Data
Statistical treatment of data is essential in order to make use of the data in the right form. Raw data collection is only one aspect of any experiment; the organization of data is equally important so that appropriate conclusions can be drawn. This is what statistical treatment of data is all about.
This article is a part of the guide:
- Statistics Tutorial
- Branches of Statistics
- Statistical Analysis
- Discrete Variables
Browse Full Outline
- 1 Statistics Tutorial
- 2.1 What is Statistics?
- 2.2 Learn Statistics
- 3 Probability
- 4 Branches of Statistics
- 5 Descriptive Statistics
- 6 Parameters
- 7.1 Data Treatment
- 7.2 Raw Data
- 7.3 Outliers
- 7.4 Data Output
- 8 Statistical Analysis
- 9 Measurement Scales
- 10 Variables and Statistics
- 11 Discrete Variables
There are many techniques involved in statistics that treat data in the required manner. Statistical treatment of data is essential in all experiments, whether social, scientific or any other form. Statistical treatment of data greatly depends on the kind of experiment and the desired result from the experiment.
For example, in a survey regarding the election of a Mayor, parameters like age, gender, occupation, etc. would be important in influencing the person's decision to vote for a particular candidate. Therefore the data needs to be treated in these reference frames.
An important aspect of statistical treatment of data is the handling of errors. All experiments invariably produce errors and noise. Both systematic and random errors need to be taken into consideration.
Depending on the type of experiment being performed, Type-I and Type-II errors also need to be handled. These are the cases of false positives and false negatives that are important to understand and eliminate in order to make sense from the result of the experiment.
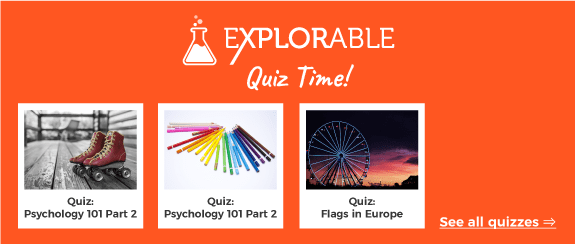
Treatment of Data and Distribution
Trying to classify data into commonly known patterns is a tremendous help and is intricately related to statistical treatment of data. This is because distributions such as the normal probability distribution occur very commonly in nature that they are the underlying distributions in most medical, social and physical experiments.
Therefore if a given sample size is known to be normally distributed, then the statistical treatment of data is made easy for the researcher as he would already have a lot of back up theory in this aspect. Care should always be taken, however, not to assume all data to be normally distributed, and should always be confirmed with appropriate testing.
Statistical treatment of data also involves describing the data. The best way to do this is through the measures of central tendencies like mean , median and mode . These help the researcher explain in short how the data are concentrated. Range, uncertainty and standard deviation help to understand the distribution of the data. Therefore two distributions with the same mean can have wildly different standard deviation, which shows how well the data points are concentrated around the mean.
Statistical treatment of data is an important aspect of all experimentation today and a thorough understanding is necessary to conduct the right experiments with the right inferences from the data obtained.
- Psychology 101
- Flags and Countries
- Capitals and Countries
Siddharth Kalla (Apr 10, 2009). Statistical Treatment Of Data. Retrieved Aug 23, 2024 from Explorable.com: https://explorable.com/statistical-treatment-of-data
You Are Allowed To Copy The Text
The text in this article is licensed under the Creative Commons-License Attribution 4.0 International (CC BY 4.0) .
This means you're free to copy, share and adapt any parts (or all) of the text in the article, as long as you give appropriate credit and provide a link/reference to this page.
That is it. You don't need our permission to copy the article; just include a link/reference back to this page. You can use it freely (with some kind of link), and we're also okay with people reprinting in publications like books, blogs, newsletters, course-material, papers, wikipedia and presentations (with clear attribution).
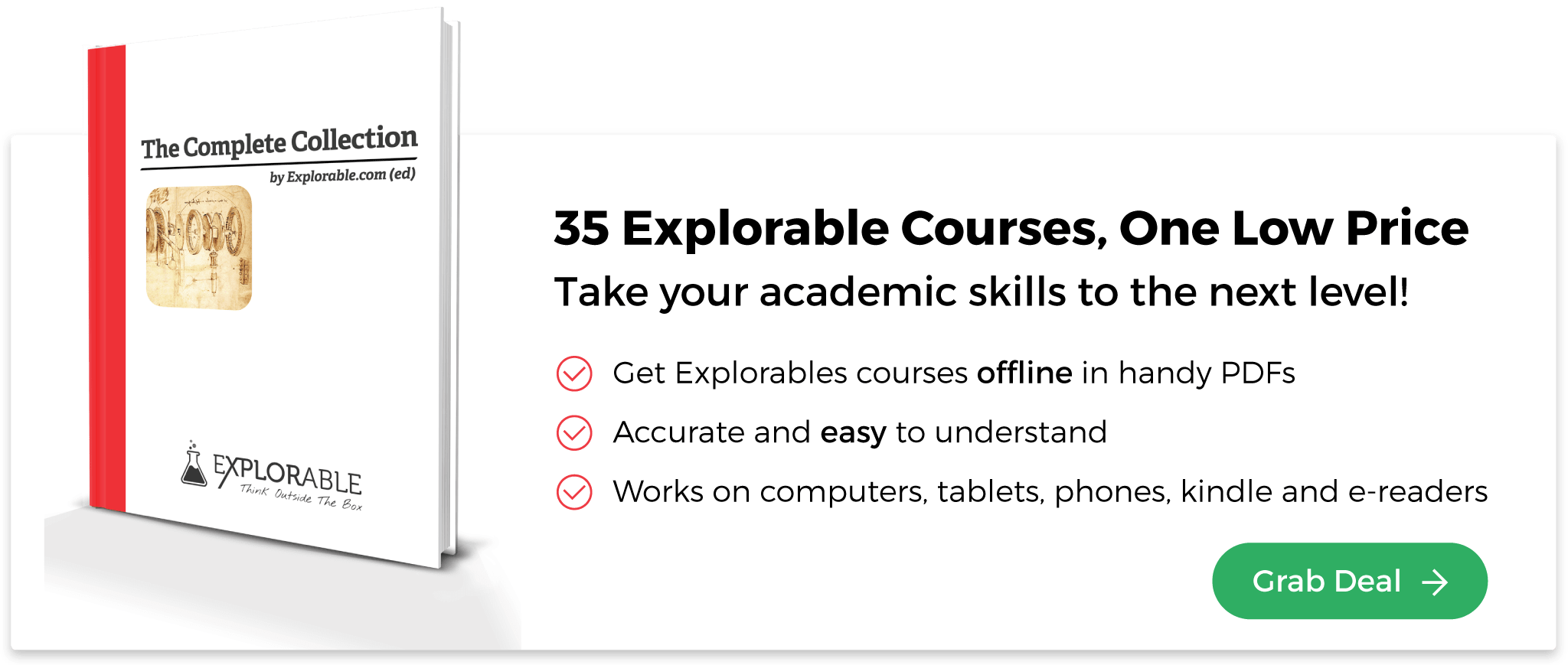
Want to stay up to date? Follow us!
Save this course for later.
Don't have time for it all now? No problem, save it as a course and come back to it later.
Footer bottom
- Privacy Policy
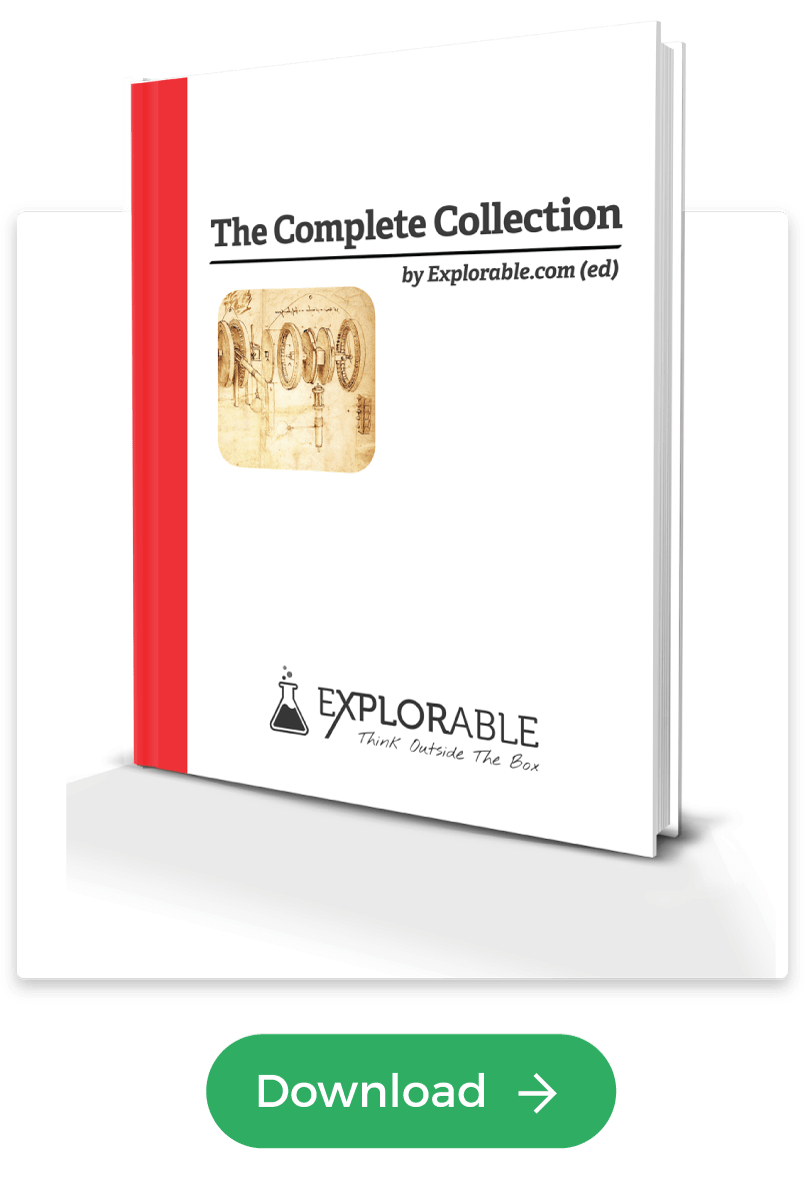
- Subscribe to our RSS Feed
- Like us on Facebook
- Follow us on Twitter
Have a language expert improve your writing
Run a free plagiarism check in 10 minutes, generate accurate citations for free.
- Knowledge Base
Descriptive Statistics | Definitions, Types, Examples
Published on July 9, 2020 by Pritha Bhandari . Revised on June 21, 2023.
Descriptive statistics summarize and organize characteristics of a data set. A data set is a collection of responses or observations from a sample or entire population.
In quantitative research , after collecting data, the first step of statistical analysis is to describe characteristics of the responses, such as the average of one variable (e.g., age), or the relation between two variables (e.g., age and creativity).
The next step is inferential statistics , which help you decide whether your data confirms or refutes your hypothesis and whether it is generalizable to a larger population.
Table of contents
Types of descriptive statistics, frequency distribution, measures of central tendency, measures of variability, univariate descriptive statistics, bivariate descriptive statistics, other interesting articles, frequently asked questions about descriptive statistics.
There are 3 main types of descriptive statistics:
- The distribution concerns the frequency of each value.
- The central tendency concerns the averages of the values.
- The variability or dispersion concerns how spread out the values are.
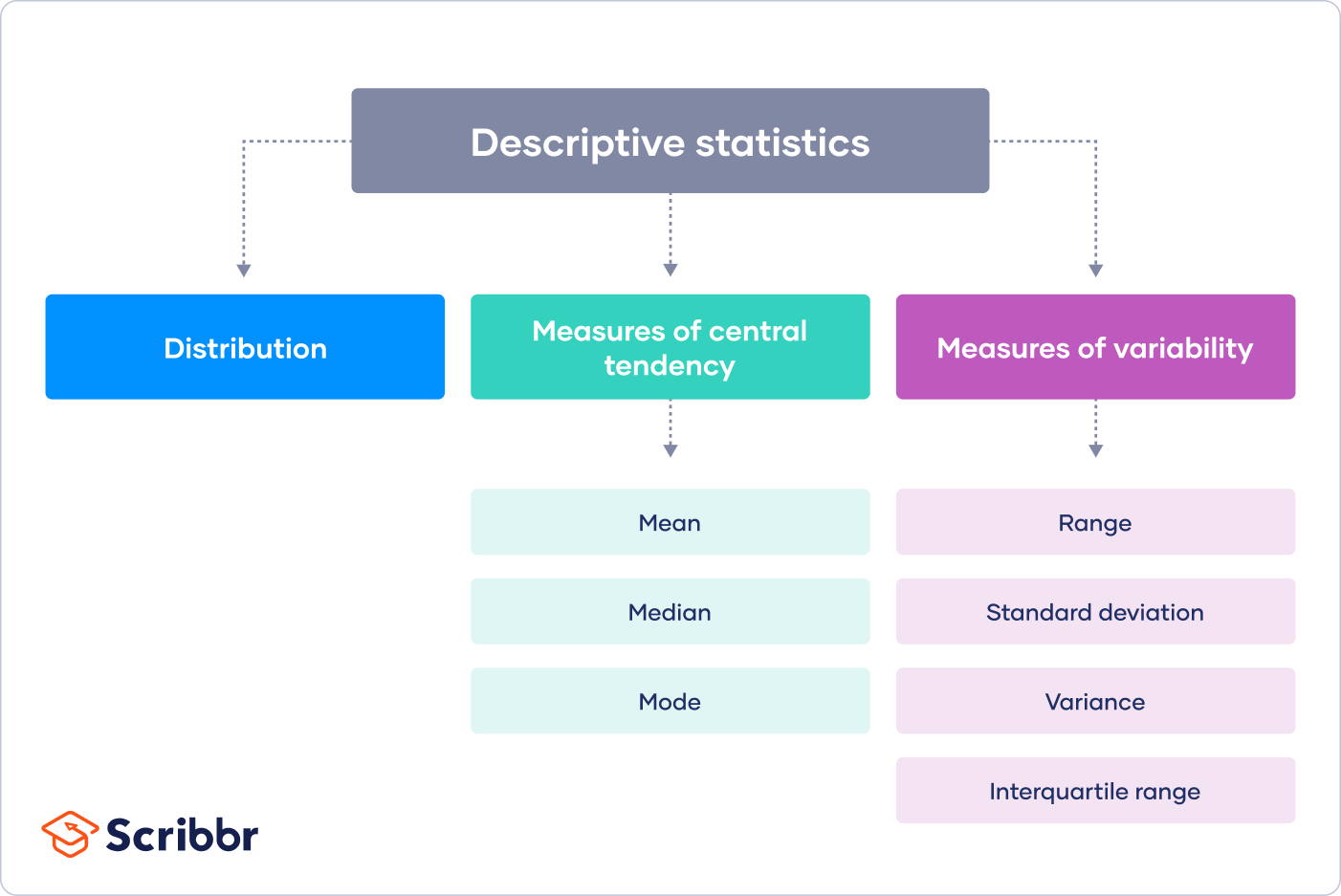
You can apply these to assess only one variable at a time, in univariate analysis, or to compare two or more, in bivariate and multivariate analysis.
- Go to a library
- Watch a movie at a theater
- Visit a national park
Receive feedback on language, structure, and formatting
Professional editors proofread and edit your paper by focusing on:
- Academic style
- Vague sentences
- Style consistency
See an example
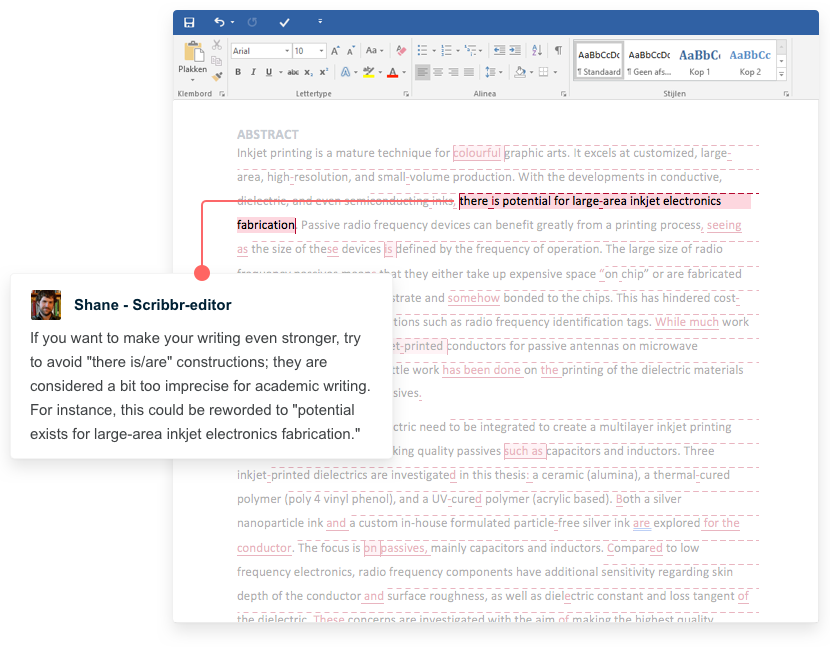
A data set is made up of a distribution of values, or scores. In tables or graphs, you can summarize the frequency of every possible value of a variable in numbers or percentages. This is called a frequency distribution .
- Simple frequency distribution table
- Grouped frequency distribution table
Gender | Number |
---|---|
Male | 182 |
Female | 235 |
Other | 27 |
From this table, you can see that more women than men or people with another gender identity took part in the study. In a grouped frequency distribution, you can group numerical response values and add up the number of responses for each group. You can also convert each of these numbers to percentages.
Library visits in the past year | Percent |
---|---|
0–4 | 6% |
5–8 | 20% |
9–12 | 42% |
13–16 | 24% |
17+ | 8% |
Measures of central tendency estimate the center, or average, of a data set. The mean, median and mode are 3 ways of finding the average.
Here we will demonstrate how to calculate the mean, median, and mode using the first 6 responses of our survey.
The mean , or M , is the most commonly used method for finding the average.
To find the mean, simply add up all response values and divide the sum by the total number of responses. The total number of responses or observations is called N .
Data set | 15, 3, 12, 0, 24, 3 |
---|---|
Sum of all values | 15 + 3 + 12 + 0 + 24 + 3 = 57 |
Total number of responses | = 6 |
Mean | Divide the sum of values by to find : 57/6 = |
The median is the value that’s exactly in the middle of a data set.
To find the median, order each response value from the smallest to the biggest. Then , the median is the number in the middle. If there are two numbers in the middle, find their mean.
Ordered data set | 0, 3, 3, 12, 15, 24 |
---|---|
Middle numbers | 3, 12 |
Median | Find the mean of the two middle numbers: (3 + 12)/2 = |
The mode is the simply the most popular or most frequent response value. A data set can have no mode, one mode, or more than one mode.
To find the mode, order your data set from lowest to highest and find the response that occurs most frequently.
Ordered data set | 0, 3, 3, 12, 15, 24 |
---|---|
Mode | Find the most frequently occurring response: |
Measures of variability give you a sense of how spread out the response values are. The range, standard deviation and variance each reflect different aspects of spread.
The range gives you an idea of how far apart the most extreme response scores are. To find the range , simply subtract the lowest value from the highest value.
Standard deviation
The standard deviation ( s or SD ) is the average amount of variability in your dataset. It tells you, on average, how far each score lies from the mean. The larger the standard deviation, the more variable the data set is.
There are six steps for finding the standard deviation:
- List each score and find their mean.
- Subtract the mean from each score to get the deviation from the mean.
- Square each of these deviations.
- Add up all of the squared deviations.
- Divide the sum of the squared deviations by N – 1.
- Find the square root of the number you found.
Raw data | Deviation from mean | Squared deviation |
---|---|---|
15 | 15 – 9.5 = 5.5 | 30.25 |
3 | 3 – 9.5 = -6.5 | 42.25 |
12 | 12 – 9.5 = 2.5 | 6.25 |
0 | 0 – 9.5 = -9.5 | 90.25 |
24 | 24 – 9.5 = 14.5 | 210.25 |
3 | 3 – 9.5 = -6.5 | 42.25 |
= 9.5 | Sum = 0 | Sum of squares = 421.5 |
Step 5: 421.5/5 = 84.3
Step 6: √84.3 = 9.18
The variance is the average of squared deviations from the mean. Variance reflects the degree of spread in the data set. The more spread the data, the larger the variance is in relation to the mean.
To find the variance, simply square the standard deviation. The symbol for variance is s 2 .
Prevent plagiarism. Run a free check.
Univariate descriptive statistics focus on only one variable at a time. It’s important to examine data from each variable separately using multiple measures of distribution, central tendency and spread. Programs like SPSS and Excel can be used to easily calculate these.
Visits to the library | |
---|---|
6 | |
Mean | 9.5 |
Median | 7.5 |
Mode | 3 |
Standard deviation | 9.18 |
Variance | 84.3 |
Range | 24 |
If you were to only consider the mean as a measure of central tendency, your impression of the “middle” of the data set can be skewed by outliers, unlike the median or mode.
Likewise, while the range is sensitive to outliers , you should also consider the standard deviation and variance to get easily comparable measures of spread.
If you’ve collected data on more than one variable, you can use bivariate or multivariate descriptive statistics to explore whether there are relationships between them.
In bivariate analysis, you simultaneously study the frequency and variability of two variables to see if they vary together. You can also compare the central tendency of the two variables before performing further statistical tests .
Multivariate analysis is the same as bivariate analysis but with more than two variables.
Contingency table
In a contingency table, each cell represents the intersection of two variables. Usually, an independent variable (e.g., gender) appears along the vertical axis and a dependent one appears along the horizontal axis (e.g., activities). You read “across” the table to see how the independent and dependent variables relate to each other.
Number of visits to the library in the past year | |||||
---|---|---|---|---|---|
Group | 0–4 | 5–8 | 9–12 | 13–16 | 17+ |
Children | 32 | 68 | 37 | 23 | 22 |
Adults | 36 | 48 | 43 | 83 | 25 |
Interpreting a contingency table is easier when the raw data is converted to percentages. Percentages make each row comparable to the other by making it seem as if each group had only 100 observations or participants. When creating a percentage-based contingency table, you add the N for each independent variable on the end.
Visits to the library in the past year (Percentages) | ||||||
---|---|---|---|---|---|---|
Group | 0–4 | 5–8 | 9–12 | 13–16 | 17+ | |
Children | 18% | 37% | 20% | 13% | 12% | 182 |
Adults | 15% | 20% | 18% | 35% | 11% | 235 |
From this table, it is more clear that similar proportions of children and adults go to the library over 17 times a year. Additionally, children most commonly went to the library between 5 and 8 times, while for adults, this number was between 13 and 16.
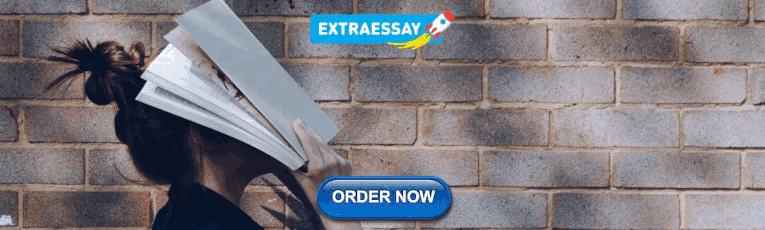
Scatter plots
A scatter plot is a chart that shows you the relationship between two or three variables . It’s a visual representation of the strength of a relationship.
In a scatter plot, you plot one variable along the x-axis and another one along the y-axis. Each data point is represented by a point in the chart.
From your scatter plot, you see that as the number of movies seen at movie theaters increases, the number of visits to the library decreases. Based on your visual assessment of a possible linear relationship, you perform further tests of correlation and regression.
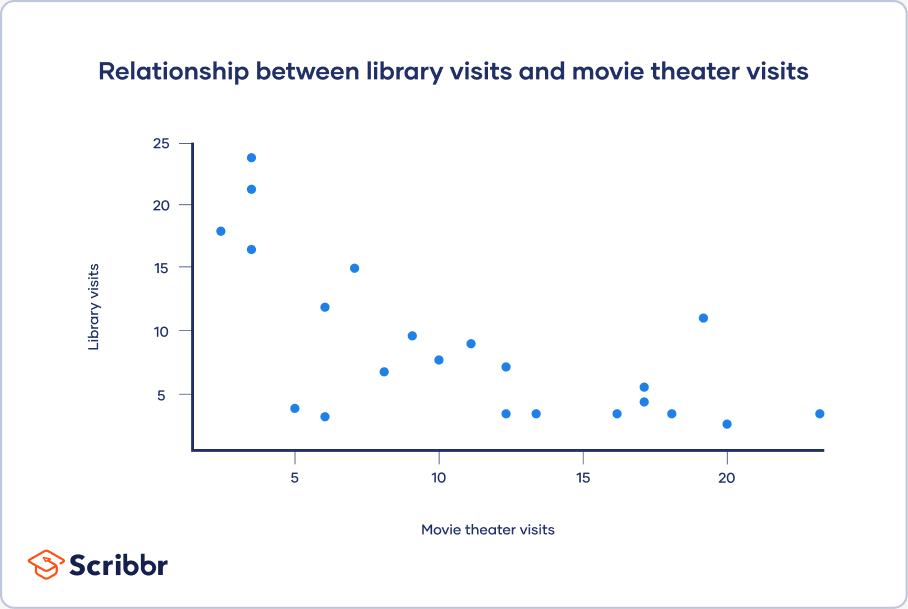
If you want to know more about statistics , methodology , or research bias , make sure to check out some of our other articles with explanations and examples.
- Statistical power
- Pearson correlation
- Degrees of freedom
- Statistical significance
Methodology
- Cluster sampling
- Stratified sampling
- Focus group
- Systematic review
- Ethnography
- Double-Barreled Question
Research bias
- Implicit bias
- Publication bias
- Cognitive bias
- Placebo effect
- Pygmalion effect
- Hindsight bias
- Overconfidence bias
Descriptive statistics summarize the characteristics of a data set. Inferential statistics allow you to test a hypothesis or assess whether your data is generalizable to the broader population.
The 3 main types of descriptive statistics concern the frequency distribution, central tendency, and variability of a dataset.
- Distribution refers to the frequencies of different responses.
- Measures of central tendency give you the average for each response.
- Measures of variability show you the spread or dispersion of your dataset.
- Univariate statistics summarize only one variable at a time.
- Bivariate statistics compare two variables .
- Multivariate statistics compare more than two variables .
Cite this Scribbr article
If you want to cite this source, you can copy and paste the citation or click the “Cite this Scribbr article” button to automatically add the citation to our free Citation Generator.
Bhandari, P. (2023, June 21). Descriptive Statistics | Definitions, Types, Examples. Scribbr. Retrieved August 21, 2024, from https://www.scribbr.com/statistics/descriptive-statistics/
Is this article helpful?
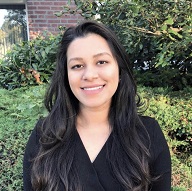
Pritha Bhandari
Other students also liked, central tendency | understanding the mean, median & mode, variability | calculating range, iqr, variance, standard deviation, inferential statistics | an easy introduction & examples, what is your plagiarism score.

Effective Use of Statistics in Research – Methods and Tools for Data Analysis
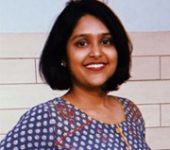
Remember that impending feeling you get when you are asked to analyze your data! Now that you have all the required raw data, you need to statistically prove your hypothesis. Representing your numerical data as part of statistics in research will also help in breaking the stereotype of being a biology student who can’t do math.
Statistical methods are essential for scientific research. In fact, statistical methods dominate the scientific research as they include planning, designing, collecting data, analyzing, drawing meaningful interpretation and reporting of research findings. Furthermore, the results acquired from research project are meaningless raw data unless analyzed with statistical tools. Therefore, determining statistics in research is of utmost necessity to justify research findings. In this article, we will discuss how using statistical methods for biology could help draw meaningful conclusion to analyze biological studies.
Table of Contents
Role of Statistics in Biological Research
Statistics is a branch of science that deals with collection, organization and analysis of data from the sample to the whole population. Moreover, it aids in designing a study more meticulously and also give a logical reasoning in concluding the hypothesis. Furthermore, biology study focuses on study of living organisms and their complex living pathways, which are very dynamic and cannot be explained with logical reasoning. However, statistics is more complex a field of study that defines and explains study patterns based on the sample sizes used. To be precise, statistics provides a trend in the conducted study.
Biological researchers often disregard the use of statistics in their research planning, and mainly use statistical tools at the end of their experiment. Therefore, giving rise to a complicated set of results which are not easily analyzed from statistical tools in research. Statistics in research can help a researcher approach the study in a stepwise manner, wherein the statistical analysis in research follows –
1. Establishing a Sample Size
Usually, a biological experiment starts with choosing samples and selecting the right number of repetitive experiments. Statistics in research deals with basics in statistics that provides statistical randomness and law of using large samples. Statistics teaches how choosing a sample size from a random large pool of sample helps extrapolate statistical findings and reduce experimental bias and errors.
2. Testing of Hypothesis
When conducting a statistical study with large sample pool, biological researchers must make sure that a conclusion is statistically significant. To achieve this, a researcher must create a hypothesis before examining the distribution of data. Furthermore, statistics in research helps interpret the data clustered near the mean of distributed data or spread across the distribution. These trends help analyze the sample and signify the hypothesis.
3. Data Interpretation Through Analysis
When dealing with large data, statistics in research assist in data analysis. This helps researchers to draw an effective conclusion from their experiment and observations. Concluding the study manually or from visual observation may give erroneous results; therefore, thorough statistical analysis will take into consideration all the other statistical measures and variance in the sample to provide a detailed interpretation of the data. Therefore, researchers produce a detailed and important data to support the conclusion.
Types of Statistical Research Methods That Aid in Data Analysis
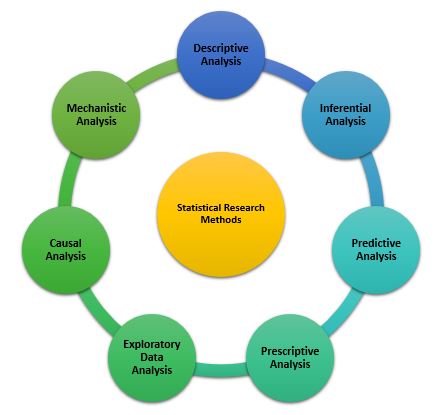
Statistical analysis is the process of analyzing samples of data into patterns or trends that help researchers anticipate situations and make appropriate research conclusions. Based on the type of data, statistical analyses are of the following type:
1. Descriptive Analysis
The descriptive statistical analysis allows organizing and summarizing the large data into graphs and tables . Descriptive analysis involves various processes such as tabulation, measure of central tendency, measure of dispersion or variance, skewness measurements etc.
2. Inferential Analysis
The inferential statistical analysis allows to extrapolate the data acquired from a small sample size to the complete population. This analysis helps draw conclusions and make decisions about the whole population on the basis of sample data. It is a highly recommended statistical method for research projects that work with smaller sample size and meaning to extrapolate conclusion for large population.
3. Predictive Analysis
Predictive analysis is used to make a prediction of future events. This analysis is approached by marketing companies, insurance organizations, online service providers, data-driven marketing, and financial corporations.
4. Prescriptive Analysis
Prescriptive analysis examines data to find out what can be done next. It is widely used in business analysis for finding out the best possible outcome for a situation. It is nearly related to descriptive and predictive analysis. However, prescriptive analysis deals with giving appropriate suggestions among the available preferences.
5. Exploratory Data Analysis
EDA is generally the first step of the data analysis process that is conducted before performing any other statistical analysis technique. It completely focuses on analyzing patterns in the data to recognize potential relationships. EDA is used to discover unknown associations within data, inspect missing data from collected data and obtain maximum insights.
6. Causal Analysis
Causal analysis assists in understanding and determining the reasons behind “why” things happen in a certain way, as they appear. This analysis helps identify root cause of failures or simply find the basic reason why something could happen. For example, causal analysis is used to understand what will happen to the provided variable if another variable changes.
7. Mechanistic Analysis
This is a least common type of statistical analysis. The mechanistic analysis is used in the process of big data analytics and biological science. It uses the concept of understanding individual changes in variables that cause changes in other variables correspondingly while excluding external influences.
Important Statistical Tools In Research
Researchers in the biological field find statistical analysis in research as the scariest aspect of completing research. However, statistical tools in research can help researchers understand what to do with data and how to interpret the results, making this process as easy as possible.
1. Statistical Package for Social Science (SPSS)
It is a widely used software package for human behavior research. SPSS can compile descriptive statistics, as well as graphical depictions of result. Moreover, it includes the option to create scripts that automate analysis or carry out more advanced statistical processing.
2. R Foundation for Statistical Computing
This software package is used among human behavior research and other fields. R is a powerful tool and has a steep learning curve. However, it requires a certain level of coding. Furthermore, it comes with an active community that is engaged in building and enhancing the software and the associated plugins.
3. MATLAB (The Mathworks)
It is an analytical platform and a programming language. Researchers and engineers use this software and create their own code and help answer their research question. While MatLab can be a difficult tool to use for novices, it offers flexibility in terms of what the researcher needs.
4. Microsoft Excel
Not the best solution for statistical analysis in research, but MS Excel offers wide variety of tools for data visualization and simple statistics. It is easy to generate summary and customizable graphs and figures. MS Excel is the most accessible option for those wanting to start with statistics.
5. Statistical Analysis Software (SAS)
It is a statistical platform used in business, healthcare, and human behavior research alike. It can carry out advanced analyzes and produce publication-worthy figures, tables and charts .
6. GraphPad Prism
It is a premium software that is primarily used among biology researchers. But, it offers a range of variety to be used in various other fields. Similar to SPSS, GraphPad gives scripting option to automate analyses to carry out complex statistical calculations.
This software offers basic as well as advanced statistical tools for data analysis. However, similar to GraphPad and SPSS, minitab needs command over coding and can offer automated analyses.
Use of Statistical Tools In Research and Data Analysis
Statistical tools manage the large data. Many biological studies use large data to analyze the trends and patterns in studies. Therefore, using statistical tools becomes essential, as they manage the large data sets, making data processing more convenient.
Following these steps will help biological researchers to showcase the statistics in research in detail, and develop accurate hypothesis and use correct tools for it.
There are a range of statistical tools in research which can help researchers manage their research data and improve the outcome of their research by better interpretation of data. You could use statistics in research by understanding the research question, knowledge of statistics and your personal experience in coding.
Have you faced challenges while using statistics in research? How did you manage it? Did you use any of the statistical tools to help you with your research data? Do write to us or comment below!
Frequently Asked Questions
Statistics in research can help a researcher approach the study in a stepwise manner: 1. Establishing a sample size 2. Testing of hypothesis 3. Data interpretation through analysis
Statistical methods are essential for scientific research. In fact, statistical methods dominate the scientific research as they include planning, designing, collecting data, analyzing, drawing meaningful interpretation and reporting of research findings. Furthermore, the results acquired from research project are meaningless raw data unless analyzed with statistical tools. Therefore, determining statistics in research is of utmost necessity to justify research findings.
Statistical tools in research can help researchers understand what to do with data and how to interpret the results, making this process as easy as possible. They can manage large data sets, making data processing more convenient. A great number of tools are available to carry out statistical analysis of data like SPSS, SAS (Statistical Analysis Software), and Minitab.

nice article to read
Holistic but delineating. A very good read.
Rate this article Cancel Reply
Your email address will not be published.
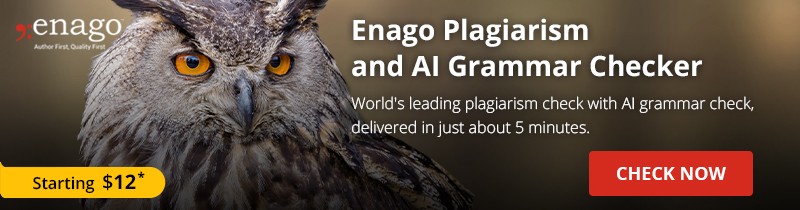
Enago Academy's Most Popular Articles
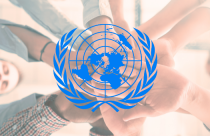
- Promoting Research
- Thought Leadership
- Trending Now
How Enago Academy Contributes to Sustainable Development Goals (SDGs) Through Empowering Researchers
The United Nations Sustainable Development Goals (SDGs) are a universal call to action to end…
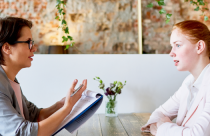
- Reporting Research
Research Interviews: An effective and insightful way of data collection
Research interviews play a pivotal role in collecting data for various academic, scientific, and professional…
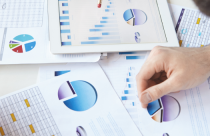
Planning Your Data Collection: Designing methods for effective research
Planning your research is very important to obtain desirable results. In research, the relevance of…
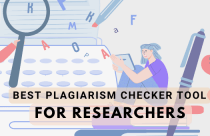
- Language & Grammar
Best Plagiarism Checker Tool for Researchers — Top 4 to choose from!
While common writing issues like language enhancement, punctuation errors, grammatical errors, etc. can be dealt…
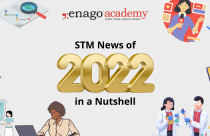
- Industry News
- Publishing News
2022 in a Nutshell — Reminiscing the year when opportunities were seized and feats were achieved!
It’s beginning to look a lot like success! Some of the greatest opportunities to research…
2022 in a Nutshell — Reminiscing the year when opportunities were seized and feats…

Sign-up to read more
Subscribe for free to get unrestricted access to all our resources on research writing and academic publishing including:
- 2000+ blog articles
- 50+ Webinars
- 10+ Expert podcasts
- 50+ Infographics
- 10+ Checklists
- Research Guides
We hate spam too. We promise to protect your privacy and never spam you.
- Publishing Research
- AI in Academia
- Career Corner
- Diversity and Inclusion
- Infographics
- Expert Video Library
- Other Resources
- Enago Learn
- Upcoming & On-Demand Webinars
- Peer Review Week 2024
- Open Access Week 2023
- Conference Videos
- Enago Report
- Journal Finder
- Enago Plagiarism & AI Grammar Check
- Editing Services
- Publication Support Services
- Research Impact
- Translation Services
- Publication solutions
- AI-Based Solutions
- Call for Articles
- Call for Speakers
- Author Training
- Edit Profile
I am looking for Editing/ Proofreading services for my manuscript Tentative date of next journal submission:
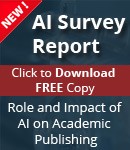
In your opinion, what is the most effective way to improve integrity in the peer review process?

An official website of the United States government
The .gov means it’s official. Federal government websites often end in .gov or .mil. Before sharing sensitive information, make sure you’re on a federal government site.
The site is secure. The https:// ensures that you are connecting to the official website and that any information you provide is encrypted and transmitted securely.
- Publications
- Account settings
- My Bibliography
- Collections
- Citation manager
Save citation to file
Email citation, add to collections.
- Create a new collection
- Add to an existing collection
Add to My Bibliography
Your saved search, create a file for external citation management software, your rss feed.
- Search in PubMed
- Search in NLM Catalog
- Add to Search
An overview of commonly used statistical methods in clinical research
Affiliations.
- 1 Center for Surgical Outcomes Research, The Research Institute at Nationwide Children's Hospital, Columbus, OH, USA.
- 2 Department of Surgery, Children's Mercy Hospital, 2401 Gillham Road, Kansas City, MO 64108, USA. Electronic address: [email protected].
- PMID: 30473041
- DOI: 10.1053/j.sempedsurg.2018.10.008
Statistics plays an essential role in clinical research by providing a framework for making inferences about a population of interest. In order to interpret research datasets, clinicians involved in clinical research should have an understanding of statistical methodology. This article provides a brief overview of statistical methods that are frequently used in clinical research studies. Descriptive and inferential methods, including regression modeling and propensity scores, are discussed, with focus on the rationale, assumptions, strengths, and limitations to their application.
Keywords: Descriptive statistics; Inferential statistics; Propensity scores; Regression analysis; Survival analysis.
Copyright © 2018 Elsevier Inc. All rights reserved.
PubMed Disclaimer
Similar articles
- Study design: the basics. Lim HJ, Hoffmann RG. Lim HJ, et al. Methods Mol Biol. 2007;404:1-17. doi: 10.1007/978-1-59745-530-5_1. Methods Mol Biol. 2007. PMID: 18450042 Review.
- Evaluating statistics in clinical trials: making the unintelligible intelligible. Gilmore SJ. Gilmore SJ. Australas J Dermatol. 2008 Nov;49(4):177-84; quiz 185-6. doi: 10.1111/j.1440-0960.2008.00465_1.x. Australas J Dermatol. 2008. PMID: 18855776 Review.
- Biostatistics primer: part 2. Overholser BR, Sowinski KM. Overholser BR, et al. Nutr Clin Pract. 2008 Feb;23(1):76-84. doi: 10.1177/011542650802300176. Nutr Clin Pract. 2008. PMID: 18203967
- Statistical problems in medical research. Okeh UM. Okeh UM. East Afr J Public Health. 2009 Apr;6 Suppl(1):1-7. doi: 10.4314/eajph.v6i3.45762. East Afr J Public Health. 2009. PMID: 20088069
- Biostatistics primer: part I. Overholser BR, Sowinski KM. Overholser BR, et al. Nutr Clin Pract. 2007 Dec;22(6):629-35. doi: 10.1177/0115426507022006629. Nutr Clin Pract. 2007. PMID: 18042950 Review.
- Type and approach of hysterectomy and oncological survival of women with stage II cancer of endometrium: a large retrospective cohort study. Shuai X, Xiao D, Han B, Du Y. Shuai X, et al. Front Oncol. 2024 May 13;14:1404831. doi: 10.3389/fonc.2024.1404831. eCollection 2024. Front Oncol. 2024. PMID: 38803540 Free PMC article.
- Say farewell to bland regression reporting: Three forest plot variations for visualizing linear models. Fries J, Oberleiter S, Pietschnig J. Fries J, et al. PLoS One. 2024 Feb 2;19(2):e0297033. doi: 10.1371/journal.pone.0297033. eCollection 2024. PLoS One. 2024. PMID: 38306346 Free PMC article.
- Correlation between hypertensive retinopathy and fetal outcomes in patients with preeclampsia in a tertiary care hospital: A prospective cohort study. Jacob N, Kasturi N, Deb AK, Maurya DK. Jacob N, et al. Indian J Ophthalmol. 2023 Mar;71(3):910-915. doi: 10.4103/IJO.IJO_2995_22. Indian J Ophthalmol. 2023. PMID: 36872708 Free PMC article.
- Genotyping Helicobacter pylori and fgf7 gene expression in gastric cancer. Hedayati MA, Khani D, Bashiri H. Hedayati MA, et al. Mol Biol Rep. 2022 Sep;49(9):8827-8834. doi: 10.1007/s11033-022-07732-3. Epub 2022 Jul 7. Mol Biol Rep. 2022. PMID: 35799083
- A systematic review and meta-analysis of the effect of transitional care interventions on the prognosis of patients with heart failure. Lin X, Ji R, Wang X, Xin R, Chen Q. Lin X, et al. J Thorac Dis. 2022 Apr;14(4):1164-1171. doi: 10.21037/jtd-22-102. J Thorac Dis. 2022. PMID: 35572902 Free PMC article.
Publication types
- Search in MeSH
LinkOut - more resources
Full text sources.
- Elsevier Science
- MedlinePlus Health Information
- Citation Manager
NCBI Literature Resources
MeSH PMC Bookshelf Disclaimer
The PubMed wordmark and PubMed logo are registered trademarks of the U.S. Department of Health and Human Services (HHS). Unauthorized use of these marks is strictly prohibited.

An official website of the United States government
The .gov means it’s official. Federal government websites often end in .gov or .mil. Before sharing sensitive information, make sure you’re on a federal government site.
The site is secure. The https:// ensures that you are connecting to the official website and that any information you provide is encrypted and transmitted securely.
- Publications
- Account settings
Preview improvements coming to the PMC website in October 2024. Learn More or Try it out now .
- Advanced Search
- Journal List
- Indian J Anaesth
- v.60(9); 2016 Sep
Basic statistical tools in research and data analysis
Zulfiqar ali.
Department of Anaesthesiology, Division of Neuroanaesthesiology, Sheri Kashmir Institute of Medical Sciences, Soura, Srinagar, Jammu and Kashmir, India
S Bala Bhaskar
1 Department of Anaesthesiology and Critical Care, Vijayanagar Institute of Medical Sciences, Bellary, Karnataka, India
Statistical methods involved in carrying out a study include planning, designing, collecting data, analysing, drawing meaningful interpretation and reporting of the research findings. The statistical analysis gives meaning to the meaningless numbers, thereby breathing life into a lifeless data. The results and inferences are precise only if proper statistical tests are used. This article will try to acquaint the reader with the basic research tools that are utilised while conducting various studies. The article covers a brief outline of the variables, an understanding of quantitative and qualitative variables and the measures of central tendency. An idea of the sample size estimation, power analysis and the statistical errors is given. Finally, there is a summary of parametric and non-parametric tests used for data analysis.
INTRODUCTION
Statistics is a branch of science that deals with the collection, organisation, analysis of data and drawing of inferences from the samples to the whole population.[ 1 ] This requires a proper design of the study, an appropriate selection of the study sample and choice of a suitable statistical test. An adequate knowledge of statistics is necessary for proper designing of an epidemiological study or a clinical trial. Improper statistical methods may result in erroneous conclusions which may lead to unethical practice.[ 2 ]
Variable is a characteristic that varies from one individual member of population to another individual.[ 3 ] Variables such as height and weight are measured by some type of scale, convey quantitative information and are called as quantitative variables. Sex and eye colour give qualitative information and are called as qualitative variables[ 3 ] [ Figure 1 ].

Classification of variables
Quantitative variables
Quantitative or numerical data are subdivided into discrete and continuous measurements. Discrete numerical data are recorded as a whole number such as 0, 1, 2, 3,… (integer), whereas continuous data can assume any value. Observations that can be counted constitute the discrete data and observations that can be measured constitute the continuous data. Examples of discrete data are number of episodes of respiratory arrests or the number of re-intubations in an intensive care unit. Similarly, examples of continuous data are the serial serum glucose levels, partial pressure of oxygen in arterial blood and the oesophageal temperature.
A hierarchical scale of increasing precision can be used for observing and recording the data which is based on categorical, ordinal, interval and ratio scales [ Figure 1 ].
Categorical or nominal variables are unordered. The data are merely classified into categories and cannot be arranged in any particular order. If only two categories exist (as in gender male and female), it is called as a dichotomous (or binary) data. The various causes of re-intubation in an intensive care unit due to upper airway obstruction, impaired clearance of secretions, hypoxemia, hypercapnia, pulmonary oedema and neurological impairment are examples of categorical variables.
Ordinal variables have a clear ordering between the variables. However, the ordered data may not have equal intervals. Examples are the American Society of Anesthesiologists status or Richmond agitation-sedation scale.
Interval variables are similar to an ordinal variable, except that the intervals between the values of the interval variable are equally spaced. A good example of an interval scale is the Fahrenheit degree scale used to measure temperature. With the Fahrenheit scale, the difference between 70° and 75° is equal to the difference between 80° and 85°: The units of measurement are equal throughout the full range of the scale.
Ratio scales are similar to interval scales, in that equal differences between scale values have equal quantitative meaning. However, ratio scales also have a true zero point, which gives them an additional property. For example, the system of centimetres is an example of a ratio scale. There is a true zero point and the value of 0 cm means a complete absence of length. The thyromental distance of 6 cm in an adult may be twice that of a child in whom it may be 3 cm.
STATISTICS: DESCRIPTIVE AND INFERENTIAL STATISTICS
Descriptive statistics[ 4 ] try to describe the relationship between variables in a sample or population. Descriptive statistics provide a summary of data in the form of mean, median and mode. Inferential statistics[ 4 ] use a random sample of data taken from a population to describe and make inferences about the whole population. It is valuable when it is not possible to examine each member of an entire population. The examples if descriptive and inferential statistics are illustrated in Table 1 .
Example of descriptive and inferential statistics

Descriptive statistics
The extent to which the observations cluster around a central location is described by the central tendency and the spread towards the extremes is described by the degree of dispersion.
Measures of central tendency
The measures of central tendency are mean, median and mode.[ 6 ] Mean (or the arithmetic average) is the sum of all the scores divided by the number of scores. Mean may be influenced profoundly by the extreme variables. For example, the average stay of organophosphorus poisoning patients in ICU may be influenced by a single patient who stays in ICU for around 5 months because of septicaemia. The extreme values are called outliers. The formula for the mean is

where x = each observation and n = number of observations. Median[ 6 ] is defined as the middle of a distribution in a ranked data (with half of the variables in the sample above and half below the median value) while mode is the most frequently occurring variable in a distribution. Range defines the spread, or variability, of a sample.[ 7 ] It is described by the minimum and maximum values of the variables. If we rank the data and after ranking, group the observations into percentiles, we can get better information of the pattern of spread of the variables. In percentiles, we rank the observations into 100 equal parts. We can then describe 25%, 50%, 75% or any other percentile amount. The median is the 50 th percentile. The interquartile range will be the observations in the middle 50% of the observations about the median (25 th -75 th percentile). Variance[ 7 ] is a measure of how spread out is the distribution. It gives an indication of how close an individual observation clusters about the mean value. The variance of a population is defined by the following formula:

where σ 2 is the population variance, X is the population mean, X i is the i th element from the population and N is the number of elements in the population. The variance of a sample is defined by slightly different formula:

where s 2 is the sample variance, x is the sample mean, x i is the i th element from the sample and n is the number of elements in the sample. The formula for the variance of a population has the value ‘ n ’ as the denominator. The expression ‘ n −1’ is known as the degrees of freedom and is one less than the number of parameters. Each observation is free to vary, except the last one which must be a defined value. The variance is measured in squared units. To make the interpretation of the data simple and to retain the basic unit of observation, the square root of variance is used. The square root of the variance is the standard deviation (SD).[ 8 ] The SD of a population is defined by the following formula:

where σ is the population SD, X is the population mean, X i is the i th element from the population and N is the number of elements in the population. The SD of a sample is defined by slightly different formula:

where s is the sample SD, x is the sample mean, x i is the i th element from the sample and n is the number of elements in the sample. An example for calculation of variation and SD is illustrated in Table 2 .
Example of mean, variance, standard deviation

Normal distribution or Gaussian distribution
Most of the biological variables usually cluster around a central value, with symmetrical positive and negative deviations about this point.[ 1 ] The standard normal distribution curve is a symmetrical bell-shaped. In a normal distribution curve, about 68% of the scores are within 1 SD of the mean. Around 95% of the scores are within 2 SDs of the mean and 99% within 3 SDs of the mean [ Figure 2 ].

Normal distribution curve
Skewed distribution
It is a distribution with an asymmetry of the variables about its mean. In a negatively skewed distribution [ Figure 3 ], the mass of the distribution is concentrated on the right of Figure 1 . In a positively skewed distribution [ Figure 3 ], the mass of the distribution is concentrated on the left of the figure leading to a longer right tail.

Curves showing negatively skewed and positively skewed distribution
Inferential statistics
In inferential statistics, data are analysed from a sample to make inferences in the larger collection of the population. The purpose is to answer or test the hypotheses. A hypothesis (plural hypotheses) is a proposed explanation for a phenomenon. Hypothesis tests are thus procedures for making rational decisions about the reality of observed effects.
Probability is the measure of the likelihood that an event will occur. Probability is quantified as a number between 0 and 1 (where 0 indicates impossibility and 1 indicates certainty).
In inferential statistics, the term ‘null hypothesis’ ( H 0 ‘ H-naught ,’ ‘ H-null ’) denotes that there is no relationship (difference) between the population variables in question.[ 9 ]
Alternative hypothesis ( H 1 and H a ) denotes that a statement between the variables is expected to be true.[ 9 ]
The P value (or the calculated probability) is the probability of the event occurring by chance if the null hypothesis is true. The P value is a numerical between 0 and 1 and is interpreted by researchers in deciding whether to reject or retain the null hypothesis [ Table 3 ].
P values with interpretation

If P value is less than the arbitrarily chosen value (known as α or the significance level), the null hypothesis (H0) is rejected [ Table 4 ]. However, if null hypotheses (H0) is incorrectly rejected, this is known as a Type I error.[ 11 ] Further details regarding alpha error, beta error and sample size calculation and factors influencing them are dealt with in another section of this issue by Das S et al .[ 12 ]
Illustration for null hypothesis

PARAMETRIC AND NON-PARAMETRIC TESTS
Numerical data (quantitative variables) that are normally distributed are analysed with parametric tests.[ 13 ]
Two most basic prerequisites for parametric statistical analysis are:
- The assumption of normality which specifies that the means of the sample group are normally distributed
- The assumption of equal variance which specifies that the variances of the samples and of their corresponding population are equal.
However, if the distribution of the sample is skewed towards one side or the distribution is unknown due to the small sample size, non-parametric[ 14 ] statistical techniques are used. Non-parametric tests are used to analyse ordinal and categorical data.
Parametric tests
The parametric tests assume that the data are on a quantitative (numerical) scale, with a normal distribution of the underlying population. The samples have the same variance (homogeneity of variances). The samples are randomly drawn from the population, and the observations within a group are independent of each other. The commonly used parametric tests are the Student's t -test, analysis of variance (ANOVA) and repeated measures ANOVA.
Student's t -test
Student's t -test is used to test the null hypothesis that there is no difference between the means of the two groups. It is used in three circumstances:

where X = sample mean, u = population mean and SE = standard error of mean

where X 1 − X 2 is the difference between the means of the two groups and SE denotes the standard error of the difference.
- To test if the population means estimated by two dependent samples differ significantly (the paired t -test). A usual setting for paired t -test is when measurements are made on the same subjects before and after a treatment.
The formula for paired t -test is:

where d is the mean difference and SE denotes the standard error of this difference.
The group variances can be compared using the F -test. The F -test is the ratio of variances (var l/var 2). If F differs significantly from 1.0, then it is concluded that the group variances differ significantly.
Analysis of variance
The Student's t -test cannot be used for comparison of three or more groups. The purpose of ANOVA is to test if there is any significant difference between the means of two or more groups.
In ANOVA, we study two variances – (a) between-group variability and (b) within-group variability. The within-group variability (error variance) is the variation that cannot be accounted for in the study design. It is based on random differences present in our samples.
However, the between-group (or effect variance) is the result of our treatment. These two estimates of variances are compared using the F-test.
A simplified formula for the F statistic is:

where MS b is the mean squares between the groups and MS w is the mean squares within groups.
Repeated measures analysis of variance
As with ANOVA, repeated measures ANOVA analyses the equality of means of three or more groups. However, a repeated measure ANOVA is used when all variables of a sample are measured under different conditions or at different points in time.
As the variables are measured from a sample at different points of time, the measurement of the dependent variable is repeated. Using a standard ANOVA in this case is not appropriate because it fails to model the correlation between the repeated measures: The data violate the ANOVA assumption of independence. Hence, in the measurement of repeated dependent variables, repeated measures ANOVA should be used.
Non-parametric tests
When the assumptions of normality are not met, and the sample means are not normally, distributed parametric tests can lead to erroneous results. Non-parametric tests (distribution-free test) are used in such situation as they do not require the normality assumption.[ 15 ] Non-parametric tests may fail to detect a significant difference when compared with a parametric test. That is, they usually have less power.
As is done for the parametric tests, the test statistic is compared with known values for the sampling distribution of that statistic and the null hypothesis is accepted or rejected. The types of non-parametric analysis techniques and the corresponding parametric analysis techniques are delineated in Table 5 .
Analogue of parametric and non-parametric tests

Median test for one sample: The sign test and Wilcoxon's signed rank test
The sign test and Wilcoxon's signed rank test are used for median tests of one sample. These tests examine whether one instance of sample data is greater or smaller than the median reference value.
This test examines the hypothesis about the median θ0 of a population. It tests the null hypothesis H0 = θ0. When the observed value (Xi) is greater than the reference value (θ0), it is marked as+. If the observed value is smaller than the reference value, it is marked as − sign. If the observed value is equal to the reference value (θ0), it is eliminated from the sample.
If the null hypothesis is true, there will be an equal number of + signs and − signs.
The sign test ignores the actual values of the data and only uses + or − signs. Therefore, it is useful when it is difficult to measure the values.
Wilcoxon's signed rank test
There is a major limitation of sign test as we lose the quantitative information of the given data and merely use the + or – signs. Wilcoxon's signed rank test not only examines the observed values in comparison with θ0 but also takes into consideration the relative sizes, adding more statistical power to the test. As in the sign test, if there is an observed value that is equal to the reference value θ0, this observed value is eliminated from the sample.
Wilcoxon's rank sum test ranks all data points in order, calculates the rank sum of each sample and compares the difference in the rank sums.
Mann-Whitney test
It is used to test the null hypothesis that two samples have the same median or, alternatively, whether observations in one sample tend to be larger than observations in the other.
Mann–Whitney test compares all data (xi) belonging to the X group and all data (yi) belonging to the Y group and calculates the probability of xi being greater than yi: P (xi > yi). The null hypothesis states that P (xi > yi) = P (xi < yi) =1/2 while the alternative hypothesis states that P (xi > yi) ≠1/2.
Kolmogorov-Smirnov test
The two-sample Kolmogorov-Smirnov (KS) test was designed as a generic method to test whether two random samples are drawn from the same distribution. The null hypothesis of the KS test is that both distributions are identical. The statistic of the KS test is a distance between the two empirical distributions, computed as the maximum absolute difference between their cumulative curves.
Kruskal-Wallis test
The Kruskal–Wallis test is a non-parametric test to analyse the variance.[ 14 ] It analyses if there is any difference in the median values of three or more independent samples. The data values are ranked in an increasing order, and the rank sums calculated followed by calculation of the test statistic.
Jonckheere test
In contrast to Kruskal–Wallis test, in Jonckheere test, there is an a priori ordering that gives it a more statistical power than the Kruskal–Wallis test.[ 14 ]
Friedman test
The Friedman test is a non-parametric test for testing the difference between several related samples. The Friedman test is an alternative for repeated measures ANOVAs which is used when the same parameter has been measured under different conditions on the same subjects.[ 13 ]
Tests to analyse the categorical data
Chi-square test, Fischer's exact test and McNemar's test are used to analyse the categorical or nominal variables. The Chi-square test compares the frequencies and tests whether the observed data differ significantly from that of the expected data if there were no differences between groups (i.e., the null hypothesis). It is calculated by the sum of the squared difference between observed ( O ) and the expected ( E ) data (or the deviation, d ) divided by the expected data by the following formula:

A Yates correction factor is used when the sample size is small. Fischer's exact test is used to determine if there are non-random associations between two categorical variables. It does not assume random sampling, and instead of referring a calculated statistic to a sampling distribution, it calculates an exact probability. McNemar's test is used for paired nominal data. It is applied to 2 × 2 table with paired-dependent samples. It is used to determine whether the row and column frequencies are equal (that is, whether there is ‘marginal homogeneity’). The null hypothesis is that the paired proportions are equal. The Mantel-Haenszel Chi-square test is a multivariate test as it analyses multiple grouping variables. It stratifies according to the nominated confounding variables and identifies any that affects the primary outcome variable. If the outcome variable is dichotomous, then logistic regression is used.
SOFTWARES AVAILABLE FOR STATISTICS, SAMPLE SIZE CALCULATION AND POWER ANALYSIS
Numerous statistical software systems are available currently. The commonly used software systems are Statistical Package for the Social Sciences (SPSS – manufactured by IBM corporation), Statistical Analysis System ((SAS – developed by SAS Institute North Carolina, United States of America), R (designed by Ross Ihaka and Robert Gentleman from R core team), Minitab (developed by Minitab Inc), Stata (developed by StataCorp) and the MS Excel (developed by Microsoft).
There are a number of web resources which are related to statistical power analyses. A few are:
- StatPages.net – provides links to a number of online power calculators
- G-Power – provides a downloadable power analysis program that runs under DOS
- Power analysis for ANOVA designs an interactive site that calculates power or sample size needed to attain a given power for one effect in a factorial ANOVA design
- SPSS makes a program called SamplePower. It gives an output of a complete report on the computer screen which can be cut and paste into another document.
It is important that a researcher knows the concepts of the basic statistical methods used for conduct of a research study. This will help to conduct an appropriately well-designed study leading to valid and reliable results. Inappropriate use of statistical techniques may lead to faulty conclusions, inducing errors and undermining the significance of the article. Bad statistics may lead to bad research, and bad research may lead to unethical practice. Hence, an adequate knowledge of statistics and the appropriate use of statistical tests are important. An appropriate knowledge about the basic statistical methods will go a long way in improving the research designs and producing quality medical research which can be utilised for formulating the evidence-based guidelines.
Financial support and sponsorship
Conflicts of interest.
There are no conflicts of interest.
Statistical Treatment Rules for Heterogeneous Populations: With Application to Randomized Experiments
This paper uses Wald's concept of the risk of a statistical decision function to address the question: How should sample data on treatment response be used to guide treatment choices in a heterogeneous population? Statistical treatment rules (STRs) are statistical decision functions that map observed covariates of population members and sample data on treatment response into treatment choices. I propose evaluation of STRs by their expected welfare (negative risk in Wald's terms), and I apply this criterion to compare two STRs when the sample data are generated by a classical randomized experiment. The rules compared both embody the reasonable idea that persons should be assigned the treatment with the best empirical success rate, but they differ in their use of covariate information. The conditional success (CS) rule selects treatments with the best empirical success rates conditional on specified covariates and the unconditional success (US) rule selects a treatment with the best unconditional empirical success rate. The main finding is a proposition giving finite-sample bounds on expected welfare under the two rules. The bounds, which rest on a large-deviations theorem of Hoeffding, yield explicit sample-size and distributional conditions under which the CS Rule is superior to the US rule.
- Acknowledgements and Disclosures
MARC RIS BibTeΧ
Download Citation Data
Published Versions
Manski, Charles F. "Statistical Treatment Rules For Heterogeneous Populations," Econometrica, 2004, v72(4,Jul), 1221-1246.
More from NBER
In addition to working papers , the NBER disseminates affiliates’ latest findings through a range of free periodicals — the NBER Reporter , the NBER Digest , the Bulletin on Retirement and Disability , the Bulletin on Health , and the Bulletin on Entrepreneurship — as well as online conference reports , video lectures , and interviews .
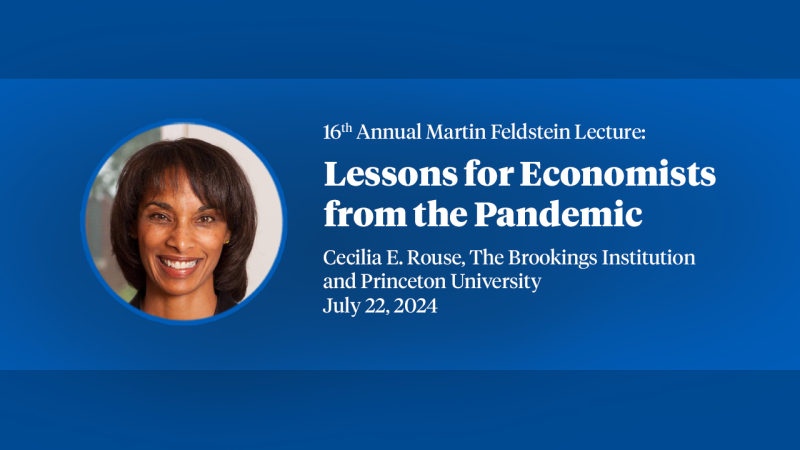
Thank you for visiting nature.com. You are using a browser version with limited support for CSS. To obtain the best experience, we recommend you use a more up to date browser (or turn off compatibility mode in Internet Explorer). In the meantime, to ensure continued support, we are displaying the site without styles and JavaScript.
- View all journals
- Explore content
- About the journal
- Publish with us
- Sign up for alerts
- Open access
- Published: 24 August 2024
The impact of the European Union emissions trading system on carbon dioxide emissions: a matrix completion analysis
- Francesco Biancalani 1 ,
- Giorgio Gnecco 1 ,
- Rodolfo Metulini 2 &
- Massimo Riccaboni 1 , 3
Scientific Reports volume 14 , Article number: 19676 ( 2024 ) Cite this article
Metrics details
- Environmental economics
- Environmental impact
Despite the negative externalities on the environment and human health, today’s economies still produce excessive carbon dioxide emissions. As a result, governments are trying to shift production and consumption to more sustainable models that reduce the environmental impact of carbon dioxide emissions. The European Union, in particular, has implemented an innovative policy to reduce carbon dioxide emissions by creating a market for emission rights, the emissions trading system. The objective of this paper is to perform a counterfactual analysis to measure the impact of the emissions trading system on the reduction of carbon dioxide emissions. For this purpose, a recently-developed statistical machine learning method called matrix completion with fixed effects estimation is used and compared to traditional econometric techniques. We apply matrix completion with fixed effects estimation to the prediction of missing counterfactual entries of a carbon dioxide emissions matrix whose elements (indexed row-wise by country and column-wise by year) represent emissions without the emissions trading system for country-year pairs. The results obtained, confirmed by robust diagnostic tests, show a significant effect of the emissions trading system on the reduction of carbon dioxide emissions: the majority of European Union countries included in our analysis reduced their total carbon dioxide emissions (associated with selected industries) by about 15.4% during the emissions trading system treatment period 2005–2020, compared to the total carbon dioxide emissions (associated with the same industries) that would have been achieved in the absence of the emissions trading system policy. Finally, several managerial/practical implications of the study are discussed, together with its possible extensions.
Introduction
Global warming is mainly a consequence of human activities and the use of fuels in an economic system. Currently, there is an extensive literature that examines various aspects related to global warming associated with \(\hbox {CO}_{2}\) emissions 1 , 2 , 3 , 4 . Limiting yearly carbon dioxide ( \(\hbox {CO}_{2}\) ) emissions can be an effective way to reduce the effects of global warming (e.g., by slowing the rise in temperature). In fact, increases in such production lead, for example, to sea level rise (and thus a reduction in available dry land). Global warming is a hot research topic because, among other things, it causes natural disasters such as hurricanes and floods. They all can cause persistent damage to agriculture and more generally to the whole economic system. For a debate on these issues, the reader is referred to 5 , 6 , 7 , 8 , 9 , 10 .
In recent years, some possible measures to mitigate climate change were proposed by governments, international organizations, and associations. But not all countries took significant action. One notable example of a policy to reduce \(\hbox {CO}_{2}\) emissions is the Emissions Trading System (ETS), which was introduced by the European Union (EU) in 2005 and came into effect in various stages. The ETS sets an annual cap on \(\hbox {CO}_{2}\) emissions for companies in certain industries. The basic idea behind this policy is that \(\hbox {CO}_{2}\) emissions are the main cause of current global warming and that reducing \(\hbox {CO}_{2}\) emissions can lead to stopping or slowing climate change. A significant portion of new \(\hbox {CO}_{2}\) emissions is caused by human impacts on the environment during manufacturing, transportation, and energy production (from fossil sources) that use large quantities of hydrocarbons. Since the amount of (EU) allowances is set by the authorities and a fine per ton is imposed if emissions are exceeded, the EU can effectively curb \(\hbox {CO}_{2}\) emissions. Up to 2020, this policy has come into force in different phases (the first was in 2005, the second in 2008, and the third in 2013). The EU ETS policy is consistent with the 2016 Paris Agreement and the EU Nationally Determined Contributions (NDC). The latter calls for a 55% reduction in \(\hbox {CO}_{2}\) emissions by 2030, using 1990 levels as the basis for calculation 11 . On the other hand, some non-EU countries implemented their \(\hbox {CO}_{2}\) reduction policies later and in a softer way.
Although some effects of the EU ETS policy were already studied (see the literature review in the “ Empirical review ” section), an examination of the literature reveals the following gaps: (i) few studies analyzed the impact of the EU ETS policy at the European level, while the rest of the analyses focused on specific EU countries; (ii) the results of the analyses conducted in different papers were often contradictory; (iii) few studies used a rigorous counterfactual analysis; (iv) typically, only the first phase of the EU ETS policy was analyzed (by considering quite a short period of time), not its long-term impact.
In this work, we have addressed the above gaps by using a state-of-the-art machine learning method (namely, Matrix Completion with Fixed Effects estimation, or MCFE, hereafter), which was recently shown by 12 to be a more effective method for evaluating policies than other, more traditional econometric methods used for panel data analysis. From the policy-making perspective, our study has several managerial/practical implications (also discussed in the “ Conclusions, policy implications, and possible future research developments ” section). First, the study highlights the need to use more reliable and general methods to estimate the impact of policies, such as EU ETS, on pollution reduction. This issue is particularly important given the current prominence of climate change and, more generally, environmental issues and the lessons we can draw from the EU experience for policymakers in developing countries. Second, a similar analysis as ours could be used to suggest the adoption of policies similar to the EU ETS one to other countries. Third, the methodology used in this study could be used as one of the tools to assess the economic, social, and environmental impact of policies, as established by recent EU legislation. In this context, a possible motivation for the application of such methodology would be a sequential (rather than simultaneous) data availability.
The work is organized as follows: the “ Literature review ” section provides a literature review; the “ Idea of the work and its original contributions ” section illustrates the idea of the work and its original contributions; the “ Description of the data set ” section describes the available data set, used for our study; the “ Methodology ” section details the methodology adopted; the “ Results ” section shows the results obtained by applying this methodology to a suitable pre-processed \(\hbox {CO}_{2}\) emission matrix; the “ Conclusions, policy implications, and possible future research developments ” section concludes the work and sheds light on possible future developments. Some technical appendices provide additional analyses, and comparisons with other methods.
Literature review
In the following, we provide a literature review, which is divided into two parts: (1) a methodological review, mainly focused on the description of some methodologies commonly adopted in the literature for policy evaluation; (2) an empirical review, mainly focused on the state of the art on the evaluation of the EU ETS policy. These two parts are connected to the present work in the following way: our study deals with policy evaluation, in which the set of treated units is a subset of EU countries, and the set of untreated (control) units is a subset of non-EU countries; the study applies a recently developed methodology (Matrix Completion with Fixed Effects estimation, or MCFE) for policy evaluation, which overcomes most of the limitations of previous methods presented in the methodological review; MCFE is used in our study to investigate the effects of the EU ETS policy on total yearly \(\hbox {CO}_{2}\) emissions of (treated) industries in EU countries.
Methodological review
When doing policy evaluation, it is often the case that the ideal situation of a randomized sample in which treated subjects have ex-ante the same characteristics as untreated subjects cannot be achieved. Therefore, one should use techniques that can provide good counterfactual data for the elements of the treated group.
In the case in which randomized controls are not available (which occurs in most policy impact analyses), various techniques, such as Instrumental Variables (IVs), are often used in the literature to evaluate interventions. A relevant example of the use of IVs in the context of ecological economics comes from 13 , whose authors studied the effects of voluntary adoption of green programs on mayoral elections, using as IV the existence of a Covenant Territorial Coordinator. They found that participation in non-mandatory green programs at the local level was not a barrier to re-election. Another typical IV application was considered by 14 , where the authors examined the impact of environmental Non-Governmental Organisations (NGOs) on air quality. In this case, IVs were represented by the number of international NGOs per capita and membership density of international NGOs.
Another technique is Regression Discontinuity Design (RDD), which can be applied when there is at least one specific threshold that separates treated and untreated units. For example, water conservation in California was examined by 15 using three discontinuity points in the timeline: June 2015, February 2016, and November 2016 (i.e., the dates of key legislative events). Similarly, a spatial regression discontinuity design was used by 16 to examine whether the Forest Stewardship Council (FSC) has changed (or not) the standard of living of indigenous people in Congo.
The use of Difference-in-Differences (DiD) ( Remark 1 : A brief introduction to the DiD method is provided in the “ Supplementary Material ”.) may provide a good alternative approach 17 , but its application requires the so-called parallel trend assumption, which is often difficult to be met. Adjustments to the control group such as Propensity Score Matching (or PSM) 18 and Mahalanobis distance matching and entropy (or Hainmueller) balancing 19 are often not conclusive in case of a large heterogeneity and small number of units available. These problems (especially the comparison between a small number of states or regions) can be partially solved with the Synthetic Control Method (SCM) ( Remark 2 : A non-technical introduction to the SCM is provided in the “ Supplementary Material ”.). This method was applied by 20 to analyze the impact of oil production in Basilicata (a small region in southern Italy) on socioeconomic indicators.
Empirical review
The topic of the implementation of markets for emission rights, and in particular the implementation of the one designed by the EU (ETS), was already studied in the literature from various points of view 21 , 22 . One of them is the empirical analysis of the effective reduction of total \(\hbox {CO}_{2}\) emissions in the countries of the old continent. However, there is no consensus in the current literature on the impact of the EU ETS policy 23 . A relevant literature review 24 reports, among other things, on the following effects of policies such as the EU ETS: they tend to increase prices of emission-intensive goods 25 , thus generating incentives to reduce such emissions (in the case of the EU ETS and other policies, such a reduction was highlighted by several studies (i.e., 21 , 26 , 27 , 28 , 29 , 30 , 31 , 32 , 33 , 34 , 35 , 36 ) reviewed in Chapter 13 of 24 ; they can stimulate technological change by participants and even other actors not involved in such policies 30 , 37 , 38 ; they may impact other countries in various ways, including changes in their emissions (leakage effects, see 39 ), although no (or not significant) evidence of such changes was observed for the case of the EU ETS 23 , 27 , 30 , 40 , 41 , 42 , 43 , 44 , 45 . Still, the study of the impact of ETS policies is complicated by the possible simultaneous presence of other similar policies and exogenous factors (e.g., fossil fuel price changes and fluctuating economic conditions) 30 , 31 , 46 , and by the fact that they apply to emissions associated with a subset of industries, possibly with different shares of emissions in different countries 47 .
In more detail, focusing on the specific techniques of analysis, one very relevant paper on the environmental impact of the ETS is certainly 48 , which combined a nearest-neighbor matching approach, between treated large plants and untreated small plants, with DiD and Difference-in-Means (DiM) estimators. However, in that work, whose analysis was focused on the United Kingdom from 2000 to 2012, no significant effect of the ETS on \(\hbox {CO}_{2}\) emissions reductions was found for that country. The impact of the EU ETS on Lithuanian companies was studied by 49 , analyzing data from 2003 to 2010 ( Remark 3 : Lithuania joined the EU in 2004, so 2004 was considered the pre-treatment period for all its observed firms.). In their analysis, the authors combined nearest neighbor matching with DiD and then applied kernel matching as a robustness check. They concluded that the ETS did not significantly reduce \(\hbox {CO}_{2}\) emissions in Lithuania (in some treated years, only minor effects were achieved as the old plants of the large polluters were released). A similar methodological approach was used by 50 for the Norwegian case ( Remark 4 : Although Norway is not an EU member, it adopted the EU ETS policy in 2008.). In that work, the authors used a fixed-effects DiD approach and selected a control group through nearest-neighbor matching, specifically assuming exact industry-level matching between treated and untreated firms. However, the results obtained related to emissions were not statistically significant.
Another stream of literature showed that the EU ETS had a positive impact on reducing \(\hbox {CO}_{2}\) emissions of selected European countries. For example, a relevant reduction in \(\hbox {CO}_{2}\) production in Germany was found by 51 , motivated by an increase in the energy efficiency of plants. Their econometric methodology used PSM to weigh treated and non-treated firms. Similarly, a significant reduction in \(\hbox {CO}_{2}\) production in France was observed by 52 . In our opinion, these approaches may hide a problem in obtaining a fair evaluation of the policy, since the treated plants were quite large, while the ones in the control group were small. As a result, there may be economies of scale in \(\hbox {CO}_{2}\) emissions that were not captured by the models. In other words, if the control group has different characteristics (i.e., in particular, a different order of magnitude in size) than the treated group, approaches based on (classical) matching cannot produce an adequate control group because the control group obtained cannot be entirely similar to the treated group.
A very recent article 53 found a reduction of about 10% in \(\hbox {CO}_{2}\) emissions between 2005 and 2012 in four countries studied (i.e., France, the Netherlands, Norway, and the United Kingdom). However, in their one-to-one matching approach, it was necessary to exclude a number of companies for which it was not possible to find a good match (e.g., large electricity production companies). This might have biased the results of their analysis due to the possible exclusion of some of the most important examples of potential \(\hbox {CO}_{2}\) emissions reductions.
Finally, a significant methodological improvement in studying the performance of the EU ETS in reducing pollution was made by 31 , where researchers applied the SCM at the industry level and concluded that the presence of the EU ETS policy significantly reduced \(\hbox {CO}_{2}\) production in the EU by 3.8% between 2008 and 2016, compared to its absence. However, SCM may fail under various circumstances, especially if the period of pre-treatment observations is not long enough 54 . The same method was also used by 55 , where the scholars concentrated their analysis on estimating the effects on emissions for Australia, if Australia had adopted the EU ETS scheme. They found a statistically significant reduction in the \(\hbox {CO}_{2}\) emissions per capita.
Idea of the work and its original contributions
Considering the limitations of the methods presented in the “ Methodological review ” section and reviewed in their application to the EU ETS policy analysis in the “ Empirical review ” section, in our analysis we have chosen to use another recently developed method coming from the Statistical Machine Learning literature (SML), namely Matrix Completion (MC), in order to verify whether the results found by 31 are confirmed or not with this novel approach. Moreover, our use of MC allows us to fill the four gaps in the literature highlighted at the end of the “ Introduction ” section, namely: (i) the opportunity of focusing the analysis on a larger set of countries; (ii) the necessity of using reliable estimation methods; (iii) the requirement of performing a rigorous counterfactual analysis; (iv) the need of analyzing a period covering various phases of the EU ETS policy.
The following is a non-technical introduction to MC. In essence, MC deals with the challenge of reconstructing a data matrix with missing entries (see Chapter 4 in 56 ). A typical example is given by predicting missing elements in a recommender system’s rating matrix, which reflects users’ preferences for various items (such as movies). Imagine a partially filled matrix in which each entry represents a user’s rating for a movie (if the user has seen that movie). MC aims to predict the missing entries (users’ ratings for movies that they have not watched, but that have been seen by other users). In this application, the final goal could be, e.g., to suggest unseen movies that users might enjoy. To achieve this, MC leverages user and item similarities. The idea is that users who give similar ratings to movies likely share similar tastes for new ones. The resulting underlying pattern of the rating matrix, known as its low-rank structure, allows MC to make predictions for unseen entries in that matrix. For this reason, MC typically includes a regularization term in its mathematical formulation, whose goal is to enforce a low-rank structure to the reconstructed matrix. In its application to counterfactual analysis, detailed by 12 and also considered in the present work, the goal of MC is, instead, to predict counterfactual values of an outcome variable for treated units during the treatment period, starting from observations of the outcome variable for the treated units in the pre-treatment period, and the observations of that variable during the whole (pre-treatment and treatment) period for the control units.
In more detail, from an optimization point of view, the main idea of (low-rank) MC is to minimize a proper tradeoff between a suitably-defined approximation error on a set of observed entries of a matrix (training set) and a proxy for the rank of the reconstructed matrix, e.g., its nuclear norm (i.e., the summation of its singular values). More technical details are reported in the “ Methodology ” section. MC is a state-of-the-art quantitative method particularly suited for counterfactual analyses, as recently demonstrated by 12 , where it was successfully compared with other commonly-adopted econometric methods such as DiD and SCM. Other effective applications of MC were made by 57 , in which MC was exploited in the context of international trade for the reconstruction of World Input–Output Database (WIOD) subtables 58 , by 59 , 60 , in which MC was used for the analysis of economic complexity, and by 61 , 62 , where MC was exploited for job analysis.
Non-technical overview of the original contributions of the work
According to the framework detailed above, our main research question is to investigate, by means of the application of a specific MC method (suitable for the prediction of counterfactuals), whether EU countries—through the EU ETS policy—reduced \(\hbox {CO}_{2}\) production significantly during a particular treatment period (2005–2020), which is equivalent to assessing the effectiveness of the EU ETS policy in reducing \(\hbox {CO}_{2}\) emissions in that period. Our goal is not limited to assessing whether or not reductions occurred but also includes quantifying (through a robust SML approach) the reduction in \(\hbox {CO}_{2}\) emissions due to the presence of the EU ETS policy. Specifically, we perform a counterfactual analysis based on MC to estimate the (unobserved) \(\hbox {CO}_{2}\) emissions of EU countries in the years of treatment in the absence of the EU ETS policy.
In this work, we aim to contribute to the academic debate by examining the impact of the EU ETS policy on reducing \(\hbox {CO}_{2}\) emissions. This work can be viewed as a development of the research made by 63 and of our earlier conference article 64 on the application of MC to the prediction of \(\hbox {CO}_{2}\) emissions, each based on two different data sets ( Remark 5 : The analysis made by 64 was further extended recently by 65 , showing that the predictive accuracy of MC, applied to a matrix of \(\hbox {CO}_{2}\) emissions, can be improved by combining it with a baseline estimate (e.g., an estimate of fixed effects). In that work, an ensemble machine-learning approach was followed, in which first the baseline estimate was generated, then MC was applied to the residual. The MC approach by 12 , used in the present work, is based on a similar but more sophisticated idea, in which fixed-effects estimation and MC are performed simultaneously.). In contrast to these papers (in which only the predictive accuracy of MC was evaluated), here we perform a counterfactual analysis, based on MC. Moreover, this analysis is based on a different choice of the matrix to which MC is applied, as well as an appropriate choice of matrix elements provided as inputs to MC. We also use a different MC method that is more appropriate for estimating causal effects, perform robustness checks for the application of that MC method, and compare it with several other methods for counterfactual prediction ( Remark 6 : To improve the readability of the work, details on this comparison are reported in the “ Supplementary Material ”.). To our knowledge, no other previous work analyzed the effects of the EU ETS policy using MC. Finally, in our analysis, we apply MC to a data set related to total \(\hbox {CO}_{2}\) emissions coming from various EU and non-EU countries during a period of several years in the past.
With the aforementioned goal in mind, in the present paper we propose to perform a counterfactual analysis for the EU ETS policy by referring to the approach used by 12 (MC with Fixed Effects estimation, or MCFE, hereafter), based on a nuclear norm MC optimization problem, which is an extension of the optimization problem introduced by 66 (baseline MC or MCB, hereafter, i.e., MC without fixed effects estimation) and solved numerically by applying the soft-impute algorithm developed in the latter work. The MCFE method is specifically designed for panel data analysis (where the rows and columns of the matrix may refer to individuals and time points, for example). It introduces a two-way (individual and time) fixed effects component to the MCB optimization problem considered by 66 , with the aim to increase the performance of matrix completion (or matrix reconstruction) ( Remark 7 : The performance of MCFE compared to MCB was recently evaluated in our conference paper 67 using a simulation study for \(\hbox {CO}_{2}\) emission data. Therein, we found that the inclusion of individual and time fixed effects in the MC optimization problem, as well as an appropriate pre-processing of the original data achieved by applying a suitable \(l_1\) row-normalization, increases the predictive performance of the MC method. In particular, the latter normalization filters out the possible side effect of differences in \(\hbox {CO}_{2}\) emission levels between countries. Therefore, also in the present work, we apply \(l_1\) normalization by row (i.e., by country) as an appropriate pre-processing of the matrix.). One important reason for its use in the present work is that the MCFE method was recently found to achieve better performance than alternative traditional methods used for causal panel data analysis (such as DiD and SCM), in several problems for which a ground truth is available 12 ( Remark 8 : As a side note, just to highlight the significance of MCFE as a state-of-the-art methodology for policy evaluation, it is worth remarking here that the work by 12 in which MCFE was developed reached a huge number of citations since its recent publication (more than 630 citations at the time of writing the present study), and that it was co-authored by a recent recipient (in 2021) of the Nobel prize in Economics, namely Prof. Guido Imbens.). More technical motivations for which MCFE is preferable, for the study of the impact of the EU ETS policy on \(\hbox {CO}_{2}\) emissions, to MCB, DiD, and SCM, are reported, respectively, in Supplementary Sections A , B , and C of the Supplementary Material. It is also worth observing that the MCFE method does not suffer from multicollinearity issues, since it works directly on a matrix of (partially) observed realizations of the outcome variable ( Remark 9 : Actually, the presence of multicollinearity could even support adopting a low-rank approximation of the matrix to be reconstructed, which is enforced by the presence of the nuclear-norm regularization term in the objective function of the MC optimization problem ( 1 ), which is reported later in the “ Methodology ” section.). In other words, its goal is not to estimate the coefficients associated with covariates in a linear regression model, but just to generate predictions of counterfactual values of the outcome variable ( Remark 10 : This remark is valid also for the extension of the MC method to the inclusion of covariates in that method, which was examined by 12 . Indeed, also in that case focus is on prediction of counterfactual values of the outcome variable, not on the estimation of the coefficients associated with the covariates.). Moreover, the MCFE method does not rely on matrix inversion, which would suffer from numerical issues in case of approximate multicollinearity ( Remark 11 : These last two remarks hold also for the MCB method but, as already discussed, the MCFE method is more suitable for policy evaluation.).
We conclude this subsection by providing, in Fig. 1 , a broad overview of the conceptual framework of the work, which is detailed in the following sections. We anticipate here that, in summary, as described in the next sections, our analysis finds that the EU ETS policy reduced \(\hbox {CO}_{2}\) emissions by about 15.4% in the European countries studied during the 2005–2020 period, ranging from almost no impact for Austria to a reduction of about 35% for Denmark.
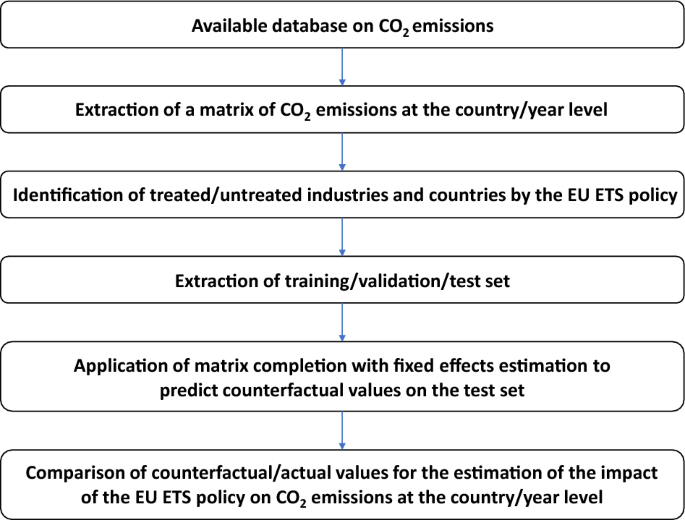
Conceptual framework of the work.
Detailed overview of the original contributions of the work
The main original contributions of the work are related to an extensive evaluation of the MCFE application to assess the impact of the EU ETS policy on \(\hbox {CO}_{2}\) emissions on EU countries. For a better reading, the results of such an analysis are reported in the following subsections of the “ Results ” section, whose titles provide a more detailed overview of the original contributions of this work: application of statistical tests about the presence of individual and time fixed effects (“ Application of statistical tests about the presence of individual and time fixed effects ” section); evaluation of the MCFE reconstruction accuracy in the pre-treatment period (“ Evaluation of the MCFE reconstruction accuracy in the pre-treatment period ” section); additional comparisons with other methods (“ Additional comparisons with other methods ” section); comparison between counterfactual/actual values in the treatment period (“ Comparison between counterfactual/actual values in the treatment period ” section); application of statistical tests about the comparison between estimated and actual data (“ Application of statistical tests about the comparison between estimated and actual data ” section); robustness checks (“ Robustness checks ” section); analysis of the effects of the EU ETS policy on \(\hbox {CO}_{2}\) emissions (“ Analysis of the effects of the EU ETS policy on CO 2 emissions ” section).
Additional more technical original contributions are reported in the “ Supplementary Material ”. They refer, respectively, to: a comparison between MC with/without fixed effects estimation (Supplementary Section A ); a comparison with the DiD model (Supplementary Section B ); a comparison with the SCM (Supplementary Section C ); the presentation of additional tables related to the results obtained in the work (Supplementary Section D ).
Description of the data set
In this paper, we use data on \(\hbox {CO}_{2}\) emissions by country. In our analysis of the causal effects of the EU ETS policy (covering the period from 2000 to 2020, being this policy introduced in 2005), we can consider EU countries as “treated” and selected high-income non-EU countries as “untreated”, since for the latter the potential treatment (before 2020) is limited compared to that of the EU countries 31 . The data set used for our analysis is extracted from the Emissions Database for Global Atmospheric Research (EDGAR), which covers all countries and can be accessed for free at https://edgar.jrc.ec.europa.eu/ ( Remark 12 : This hyperlink was accessed in July 2024.). The reader is referred to 68 for details about how missing data for specific sectors and years are handled in the construction of the EDGAR database (basically, suitable interpolation procedures are used therein). The issue of missing data is discussed also at the end of this section.
Since the ETS is mandatory for EU countries, we consider the following 17 countries as “treated” for our study: Austria (AUT), Belgium (BEL), Czech Republic (CZE), Germany (DEU), Denmark (DNK), Spain (ESP), Finland (FIN), France (FRA), Hungary (HUN), United Kingdom (GBR), Greece (GRC), Ireland (IRL), Italy (ITA), Poland (POL), Portugal (PRT), Slovakia (SVK), and Sweden (SWE). All these countries were members of the EU between 2005 (the beginning of the ETS policy) and 2020 ( Remark 13 : Brexit came into effect in 2021, so the United Kingdom was a member of the EU during the study period. Indeed, although the United Kingdom left the EU at the end of January 2020, it continued to participate in many EU institutions, during a transition period that covered 11 months. Then, starting from January 2021, the EU ETS policy in the United Kingdom was replaced by the UK ETS policy.). Among the 25 countries that were part of the EU in 2005, we do not consider in our analysis small countries having less than 5 million inhabitants at the time (i.e., Cyprus, Estonia, Latvia, Lithuania, Luxembourg, Malta, Slovenia), as their data are expected to be highly sensitive even to small shocks (since even the cessation of production of a single plant could have had a large impact on their respective total \(\hbox {CO}_{2}\) emissions). In the case of the Netherlands, only partial data are available in the EDGAR database for the period considered for our study. Hence, also that country is excluded from the analysis. Additionally, data related to other 6 non-EU countries (as of 2005) influenced by the ETS policy, i.e., Bulgaria, Croatia, Iceland, Liechtenstein, Norway, and Romania are not included in our analysis partly because of the small-country issue mentioned above, and partly because their emissions in the period of analysis could have been significantly influenced by other ad-hoc policies beside the EU ETS. Altogether, the selected 17 EU countries represent, respectively, about 87% of the population in 2005 of the 25 EU countries at the time and of the 6 non-EU countries influenced by the ETS policy reported above, and 92% of total \(\hbox {CO}_{2}\) emissions of the same set of countries.
As a control group (non-treated countries, without ETS), we consider countries that were not members of the EU between 2000 and 2020 and that were not highly related to the EU (e.g., as a result of very relevant special agreements) during this period (i.e., Iceland, Liechtenstein, Norway, and Switzerland are excluded) ( Remark 14 : Iceland, Liechtenstein, and Norway introduced the EU ETS in 2008 (i.e., during its phase 2), while Switzerland has several bilateral agreements with EU countries. A second reason to exclude from the control group countries such as Iceland, Liechtenstein, and Switzerland is the small-country issue just mentioned in the main text.). Our control group for this work consists of large non-EU high-income countries in the available database that are almost in the same phase of the so-called environmental Kuznets curve ( Remark 15 : Emerging economies are commonly regarded as the world’s biggest polluters. Conversely, developed countries are generally considered cleaner. This perception is theoretically supported by the concept of environmental Kuznets curve (see, e.g., 69 ), according to which there is an inverted “U” relationship between Gross Domestic Product (GDP) per capita and environmental degradation, measured as \(\hbox {CO}_{2}\) emissions per capita.) as the selected EU countries, and that are far from such countries geographically. Indeed, to obtain a valid control group, one needs to exclude as much as possible spillover effects of the EU ETS policy on its countries (due, e.g., to possible delocalization induced by the policy, which is unlikely to occur for the selected countries in the control group). Specifically, we include the following seven industrialized countries in the control group: Australia (AUS), Canada (CAN), Israel (ISR), Japan (JPN), New Zealand (NZL), South Korea (KOR), and the United States of America (USA). Although some non-EU countries also took specific measures to reduce \(\hbox {CO}_{2}\) emissions, the impact of these measures was relatively negligible compared to the EU ETS policy at least until 2016 28 . For instance, Australia adopted a policy similar to the EU ETS one only some years later (in 2011), and ratified the Paris agreement only in November 2016 55 .
In our analysis, we aggregate to the country-year level the values of \(\hbox {CO}_{2}\) emissions originally available at the country-industry-year level in the EDGAR database. Instead of aggregating \(\hbox {CO}_{2}\) emissions coming from all the industries, we consider only those associated with a subset of industries that, according to 70 , are potentially influenced by the EU ETS policy. Specifically, for both the treated and untreated countries, we aggregate only \(\hbox {CO}_{2}\) emissions coming from the following industries (represented here by their EDGAR codes): 1.A.1.a (Main Activity Electricity and Heat Production), 1.A.1.bc (Petroleum Refining—Manufacture of Solid Fuels and Other Energy Industries), 1.A.2 (Manufacturing Industries and Construction), 2.A.1 (Cement Production), 2.A.2 (Lime Production), 2.A.3 (Glass Production), 2.A.4 (Other Process Uses of Carbonates), 2.B (Chemical Industry), 2.C (Metal Industry) ( Remark 16 : This partial aggregation has the advantage of limiting the potential influence of other policies similar to the EU ETS on our counterfactual estimates of \(\hbox {CO}_{2}\) emissions of treated countries.). Emission data related to these industries are available for all the countries considered in our analysis (which motivates the exclusion of the Netherlands, for which this does not hold). In this way, the resulting \(24 \times 21\) \(\hbox {CO}_{2}\) emission matrix represents the (total) amount of \(\hbox {CO}_{2}\) emissions for each country and year, restricted to the selected industries. The portions of this matrix that are associated, respectively, with the pre-treatment period of EU countries (2000–2004) and with their treatment period (2005–2020) are reported in Tables 1 and 2 . For illustrative reasons, rows corresponding to treated/untreated countries appear separately in the two tables.
The goal of this data aggregation is threefold. First, we reduce the computational burden of repeatedly applying MC(FE) by using a smaller matrix as input. Second, we simplify the analysis by focusing on the aggregate level for each country. Comparing countries, industries, and years (i.e., using three dimensions in the analysis) would make the approach to completing the matrix much more complex, possibly calling for its extension to the tensor case 71 . Third, by aggregating emissions patterns at the national level, our pre-processing provides an effective way to reduce the noise possibly due to missing disaggregated data.
Methodology
In this paper, we opt for an innovative methodological approach in the field of policy evaluation. Clearly, with the available data set, it is not possible to conduct a randomized control trial analysis, because we have data on sovereign countries, and the ideal case of a randomized sample in which treated/untreated units have ex-ante the same characteristics is not realized. Some possible methods to perform policy evaluation in this situation (and their limitations) have been discussed in the “ Methodological review ” section ( Remark 17 : The application of some of the methods presented in the “ Methodological review ” section was excluded immediately, taking into account the peculiarities of the available data set: for instance, in our case, it was not possible to find at least one valid IV among the available variables; also RDD did not appear to be applicable because there was no sharp temporal or spatial threshold between treated and untreated countries; adjustments to the control group such as PSM and Mahalanobis distance matching and entropy balancing were not conclusive in our case, given the large heterogeneity and quite small number of countries in our sample.).
Given the peculiarities of our specific problem and data structure, in our analysis we prefer to adopt the MCFE approach for the following reasons: (i) the numerical results reported by 12 show that their proposed MCFE method for policy evaluation generally outperforms other alternative methods, such as the SCM and elastic net estimators; (ii) the MCFE approach can also be interpreted as a generalization of earlier approaches such as the SCM. Indeed, these approaches share the same objective function (based on the Fröbenius norm of a suitable projection of the difference between a latent matrix and the observed matrix), but have different constraints (which are less stringent in the case of the MCFE method).
For the application considered in this paper, the use of the MCFE method is justified by the fact that the counterfactual \(\hbox {CO}_{2}\) emission levels for the treated countries (namely, the selected EU countries) are not known in the years of treatment when the EU ETS policy was in force. Therefore, we use the MCFE method to generate estimates of such counterfactual values and compare them to the actual \(\hbox {CO}_{2}\) emission values, with the ultimate goal of estimating the effect of the treatment on \(\hbox {CO}_{2}\) emission values through the EU ETS policy.
The main idea is to consider the treated values (i.e., the \(\hbox {CO}_{2}\) emission values of EU countries in the years of treatment) as missing values and the other entries of the \(\hbox {CO}_{2}\) emission matrix as given data. Specifically, in this paper, we apply the following formulation of the MC optimization problem, namely, the MCFE optimization problem proposed by 12 :
\(\Omega ^{\rm{tr}}\) is a subset of pairs of indices ( i , j ) corresponding to the positions of known entries of a matrix \(\textbf{M} \in \mathbb {R}^{m \times n}\) (using a machine learning expression, \(\Omega^{\rm{tr}}\) can be called a training set of pairs of indices);
\(\textbf{1}_{n }\) and \(\textbf{1}_{m }\) are column vectors consisting of n entries and m entries, respectively, all equal to 1;
\({\hat{\textbf{M}}}\) is the completed matrix decomposed as
(where \({\hat{\textbf{L}}}\) , \({\varvec{\hat{\Gamma }}}\) and \({\varvec{\hat{\Delta }}}\) must be chosen to solve the above optimization problem);
\(\lambda \ge 0\) is a regularization constant;
\(\Vert {\hat{\textbf{L}}}\Vert _*\) is the nuclear norm of the matrix \({\hat{\textbf{L}}}\) , i.e., the summation of all its singular values.
In the above, the two terms \({\varvec{\hat{\Gamma }}} \textbf{1}_{n }^\top\) and \(\textbf{1}_{m } {\varvec{\hat{\Delta }}}^\top\) model, respectively, estimates of row-fixed effects (e.g., of unit-fixed effects) and of column-fixed effects (e.g., of time-fixed effects) in the reconstruction \({\hat{\textbf{M}}}\) of \(\textbf{M}\) according to Eq. ( 2 ). The regularization constant \(\lambda\) controls the tradeoff between adequately fitting the known entries of the matrix \(\textbf{M}\) and achieving a small nuclear norm of the first term \({\hat{\textbf{L}}}\) of its reconstruction. Here, the nuclear norm plays a similar role as the \(l_1\) -norm regularization term used in the well-known and widely used Least Absolute Shrinkage and Selection Operator (LASSO) regularization method 56 . It is worth noting that, in contrast to earlier formulations of the MC optimization problem—see, e.g., 66 —the nuclear norm \(\Vert {\hat{\textbf{L}}}\Vert _*\) is used in the optimization problem ( 1 ) instead of the nuclear norm \(\Vert {\hat{\textbf{M}}}\Vert _*\) . In other words, in the case of the MCFE method, the estimated fixed effects \({\varvec{\hat{\Gamma }}} \textbf{1}_{n }^\top\) and \(\textbf{1}_{m } {\varvec{\hat{\Delta }}}^\top\) are not regularized. In the present context, this is an important issue because otherwise, by using the alternative regularization term \(\lambda \Vert {\hat{\textbf{M}}}\Vert _*\) instead of \(\lambda \Vert {\hat{\textbf{L}}}\Vert _*\) (i.e., by regularizing the entire reconstructed matrix \({\hat{\textbf{M}}}\) , which is the case of the MCB method) ( Remark 18 : Or, equivalently, by further constraining the optimization vectors \({\varvec{\hat{\Gamma }}}\) and \({\varvec{\hat{\Delta }}}\) to be equal to \(\textbf{0}\) .), one could obtain biased estimates that might underestimate the actual values 72 . In other words, any estimated element \(\hat{M}_{i,j}\) could be a systematic underestimate of the corresponding element \({M}_{i,j}\) for the optimal choice of \(\lambda\) , making it difficult to obtain reliable estimated counterfactual values ( Remark 19 : The issue of underestimation under MC is investigated in more detail in Supplementary Section A of the Supplementary Material, where it is shown by means of one representative example that MC without fixed effects estimation (i.e., MCB) indeed underestimates the missing elements systematically, when it is applied to the specific data set considered in the present work.). As described in the literature, this is a common problem when using regularization methods. For instance, it is well-known that the LASSO regularization method may be affected by underestimation problems 73 , 74 .
In this paper, the optimization problem ( 1 ) is solved numerically by applying the soft-impute algorithm developed by 66 and adapted by 12 to the case of the optimization problem ( 1 ) ( Remark 20 : Specifically, we apply the function “mcnnm” contained in the R package “MCPanel”, which is freely available at the following hyperlink: https://rdrr.io/github/susanathey/MCPanel/man/mcnnm.html . This function allows the possibility of either estimating or not the row-fixed and column-fixed effects.). It is worth noting that the MCFE estimator used in this work was demonstrated by 12 —using two applications to smoker data and stock market data—to outperform several alternative methods such as DiD, SCM, vertical regression with elastic net regularization, and horizontal regression with elastic net regularization 12 .
The soft-impute algorithm for calculating the MCFE estimator goes as follows. Let the projection operator \(\textbf{P}_{\Omega^{\rm{tr}}}: \mathbb {R}^{m \times n} \rightarrow \mathbb {R}^{m \times n}\) be defined as \([\textbf{P}_{\Omega^{\rm{tr}}}(\textbf{M})]_{i,j}:= M_{i,j} \, \, \text {if} \,\, (i,j) \in \Omega^{\rm{tr}}, \, \, 0 \, \, \text {otherwise}\) . Similarly, let the projection operator \(\textbf{P}^{\perp }_{\Omega^{\rm{tr}}}: \mathbb {R}^{m \times n} \rightarrow \mathbb {R}^{m \times n}\) be defined as \([\textbf{P}^{\perp }_{\Omega^{\rm{tr}}}(\textbf{M})]_{i,j}:= M_{i,j} \, \, \text {if} \,\, (i,j) \notin \Omega^{\rm{tr}}, \, \, 0 \, \, \text {otherwise}\) . For a matrix \(\textbf{M}\in \mathbb {R}^{m \times n}\) with rank r , let its Singular Value Decomposition (SVD) be
where \({\textbf {D}}_r \in \mathbb {R}^{r \times r}\) is a diagonal matrix, which collects the r singular values \(d_1,\ldots,d_r\) of \({\textbf {M}}\) . Then, the soft-thresholded version of the SVD of \({\textbf {M}}\) reads as
and the subscript “+” stands for the non-negative part of a real number.
According to the soft-impute algorithm, we first initialize \({\hat{\textbf{L}}}\) as \({\hat{\textbf{L}}}^{\rm{old}}=\textbf{P}_{\Omega^{\rm{tr}}}(\textbf{M}) \in \mathbb {R}^{m \times n}\) and generate an increasing sequence of K values ( \(\lambda _1< \ldots < \lambda _K\) ) for the regularization constant \(\lambda \ge 0\) . Let \(\varepsilon > 0\) denote a selected tolerance, and \(\Vert \cdot \Vert _F\) the Fröbenius norm. Then, for each \(k = 1,\ldots ,K\) , set \(\lambda = \lambda _k\) and
Iterate until convergence the following:
Given the current \({ \hat{\textbf{L}}}={\hat{\textbf{L}}}^{\rm{old}}\) , get \({\varvec{\hat{\Gamma }}}\) and \({\varvec{\hat{\Delta }}}\) by imposing the first-order optimality conditions in the optimization problem ( 1 );
Compute \({\hat{\textbf{L}}}^{\rm{new}}\leftarrow \textbf{S}_{\frac{\lambda |\Omega^{\rm{tr}}|}{2}}\left( \textbf{P}_{\Omega^{\rm{tr}}}(\textbf{M}-{\varvec{\hat{\Gamma }}} \textbf{1}_{n }^\top -\textbf{1}_{m } {\varvec{\hat{\Delta }}}^\top )+\textbf{P}_{\Omega^{\rm{tr}}}^{\perp }({\hat{\textbf{L}}}^{\rm{old}}) \right)\) ;
If \(\frac{\Vert {\hat{\textbf{L}}}^{\rm{new}}-{\hat{\textbf{L}}}^{\rm{old}} \Vert _F^2}{\Vert {\hat{\textbf{L}}}^{\rm{old}}\Vert _F^2} \le \varepsilon\) , go to Step 2;
Set \({\hat{\textbf{L}}}^{\rm{old}}\leftarrow {\hat{\textbf{L}}}^{\rm{new}}\) ;
Set \({\hat{\textbf{L}}}_\lambda \leftarrow {\hat{\textbf{L}}}^{\rm{new}}\) and \({\hat{\textbf{M}}}_\lambda \leftarrow {\hat{\textbf{L}}}_\lambda +{\varvec{\hat{\Gamma }}} \textbf{1}_{n }^\top +\textbf{1}_{m } {\varvec{\hat{\Delta }}}^\top\) .
Since previous investigations showed that MC performs better when the elements of the matrix to which it is applied have similar magnitudes (e.g., when they are row-normalized, as in the cases considered by 65 , 67 ), in our application the original matrix of annual \(\hbox {CO}_{2}\) emissions is pre-processed by dividing each row (country) by the \(l_1\) -norm of that row restricted to the training set ( Remark 21 : This restriction is applied to avoid any use of the validation and test sets in the pre-processing phase.), and multiplied by the fraction of observed entries in that row. Then, MCFE is actually applied to the resulting matrix \({\textbf {M}}\) (then, in a post-processing phase, a row de-normalization is performed, to go back to the original scale of the data).
In our application, where \(\textbf{M}\) is derived from the \(24 \times 21\) true \(\hbox {CO}_{2}\) emission matrix, with rows referring to countries and columns to years, the tolerance parameter \(\varepsilon\) is chosen as \(\varepsilon =10^{-30}\) , in order to avoid early stopping of the algorithm. If convergence is not achieved, the soft-impute algorithm is stopped after \(N^{\rm{it}}=10^4\) iterations to reduce the total computational time.
In the present application, as shown in Fig. 2 :
the training set \(\Omega^{\rm{tr}}\) corresponds to the union of the positions of all entries for the years 2000–2004 (pre-treatment period) and 75% (randomly selected) of the positions of entries belonging to non-EU countries in the years 2005–2020 (treatment period covered by the data set);
the validation set \(\Omega^{\rm{val}}\) corresponds to the other 25% of the items of the entries belonging to non-EU countries in 2005–2020 that are not part of the training set;
the test set \(\Omega^{\rm{test}}\) corresponds to the items belonging to EU countries in the treatment period covered by the data set (2005–2020).
It is noteworthy that while ground truth without treatment is available for the validation set \(\Omega^{\rm{val}}\) (which refers to untreated non-EU countries), this is not true for the test set \(\Omega^{\rm{test}}\) (which refers to treated EU countries). To generate confidence intervals and represent the best/worst scenarios for the estimates for each treated country, MC is applied 100 times ( Remark 22 : This number was chosen as a tradeoff between reducing machine processing time and achieving a satisfactory number of generations.), each time randomly selecting the training and validation sets as described above.
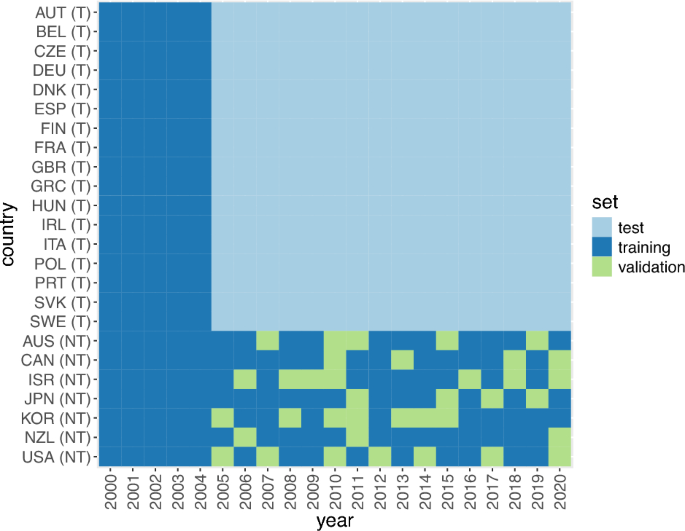
Partition of the considered matrix into training, validation and test sets. Countries are indicated on the y axis, while years are indicated on the x axis. T stands for “Treated” and NT stands for “Non Treated” (untreated). The training set \(\Omega^{\rm{tr}}\) corresponds to the union of the positions of all entries for the years 2000–2004 and 75% (randomly selected) of the positions of entries belonging to industrialized non-EU countries in the years 2005–2020. The validation set \(\Omega^{\rm{val}}\) corresponds to the other 25% of the positions of the entries belonging to industrialized non-EU countries in 2005–2020 that are not part of the training set. The test set \(\Omega^{\rm{test}}\) corresponds to the positions of the items belonging to the 17 considered EU countries in 2005–2020.
In each application of MCFE, the regularization constant \(\lambda\) is selected via an approach similar to that proposed by 12 . In particular, the optimization problem ( 1 ) is solved for multiple choices \(\lambda _k\) for \(\lambda\) . To explore different scales, these values are exponentially distributed as \(\lambda _k=2^{k/2-25}\) , for \(k=1,\ldots ,K=100\) . For each \(\lambda _k\) , the Root Mean Square Error (RMSE) of the matrix reconstruction on the validation set is calculated as follows:
then the choice \(\lambda _k^\circ\) that minimizes the \(RMSE_{\lambda _k}^{\rm{val}}\) for \(k=1,\ldots ,100\) is found. For each \(\lambda _k\) , the RMSE of the matrix reconstruction on the training set ( \(RMSE_{\lambda _k}^{\rm{tr}}\) ) is defined in a similar way, as
In particular, the focus is on the values of \(RMSE_{\lambda _k}^{\rm{val}}\) and \(RMSE_{\lambda _k}^{\rm{tr}}\) calculated for \(\lambda =\lambda _k^\circ\) . Since there is no ground truth for the counterfactual values in the test set (i.e., the values without treatment), the RMSE for the test set is not calculated in this application of the MCFE method.
The MCFE method described in the “ Methodology ” section was applied starting from the \(24 \times 21\) country-year level \(\hbox {CO}_{2}\) emissions matrix where selected industries are aggregated (see the “ Description of the data set ” section), and then pre-processed according to the methodology described in the “ Methodology ” section.
Application of statistical tests about the presence of individual and time fixed effects
To further motivate the adoption of the MCFE method described in the “ Methodology ” section (which also includes individual and time fixed effects), we performed some statistical tests for the presence of significant individual and time effects in our data. More specifically, we conducted an F -test for the null hypothesis of the absence of such effects in the context of a within regression model for panel data 75 where the 1-year lagged term is the only model covariate. We did not find a significant departure from the null hypothesis of absence of individual effects ( F = 0.610, p- value = 0.923). On the contrary, time-fixed effects, conditional to allowing for individual fixed effects, were found to be statistically significant ( F = 2.946, p- value = 0.000). The null hypothesis of the F -test for the joint absence of time and individual effects was also rejected ( F = 1.694, p- value = 0.006). In addition, it should be noted that, as mentioned by 12 , the inclusion of the individual and temporal components aims to improve the quality of the imputation by penalizing only the residual component of the completed matrix in the optimization problem ( 1 ) (i.e., the one obtained by filtering out the individual and temporal components). Thus, it would have made sense to include them even in the case in which the F -test had not rejected the null hypothesis. As mentioned in the “ Methodology ” section, not including such components would lead to systematic underestimates.
Evaluation of the MCFE reconstruction accuracy in the pre-treatment period
It is worth noting that a necessary condition for obtaining credible counterfactual results is that the MCFE method achieves satisfactory performance in reconstructing the original matrix without any treatment. In the specific analysis, underestimation of the predicted values would not be recommended, as it could lead to incorrect conclusions about the impact of the ETS policy on different EU countries. In other words, in this context, it is crucial to avoid systematically underestimating the predicted values. It is worth mentioning that the performance of nuclear norm-based MC methods was assessed through a simulation study by 67 by applying such methods to a total \(\hbox {CO}_{2}\) emission matrix, limited to the period 2000–2005, and generated based on the World Input–Output Database (WIOD) environmental accounts, 2016 release 76 . In that work, we found that the MCFE method proposed by 12 outperformed the MCB method developed by 66 in terms of goodness of fit (Mean Absolute Percentage Error, or MAPE, was used in that study). In particular, the MAPE of MCFE was very low even with a rather large number of unobserved entries in the matrix.
Additional comparisons with other methods
An additional comparison between MC with/without fixed effects estimation (i.e., between MCFE and MCB) is reported in Supplementary Section A of the Supplementary Material, which shows that MCFE turns out to be more suitable than MCB for assessing the impact of the EU ETS policy on \(\hbox {CO}_{2}\) emissions. Moreover, Supplementary Sections B and C of the Supplementary Material compare MCFE with two other econometric methods commonly used to estimate counterfactuals (respectively, DiD and SCM), highlighting the advantages of MCFE for the specific case of assessing the impact of the EU ETS policy on \(\hbox {CO}_{2}\) emissions. To ease the reading, these three comparisons are reported in the “ Supplementary Material ” rather than in the main text.
Comparison between counterfactual/actual values in the treatment period
The following results refer to the comparison of the counterfactual values (without treatment) with the actual values (with treatment). To summarize the results of the analysis, we report our main findings in Fig. 3 . The estimated \(\hbox {CO}_{2}\) emission values of the treated countries were obtained by applying MCFE. In other words, we used MCFE to estimate \(\hbox {CO}_{2}\) emissions in the years of treatment for EU countries (i.e., treated countries) as if they had not received the treatment. We repeated the estimation process 100 times, each time randomly splitting the untreated portion of the matrix into training and validation sets, as described in the “ Methodology ” section. Then, Fig. 3 shows, for the elements of the test set related to the treated countries, the actual values of \(\hbox {CO}_{2}\) emissions (i.e., those obtained in the case of treatment) against the appropriate statistics of the corresponding estimated values obtained by MCFE in the case of no treatment. Points are used for the medians (black), 10th percentiles (red), and 90th percentiles (blue) of the distributions of estimated values (obtained in the no-treatment case) in the 100 repetitions (one distribution for each treated country). The actual values (corresponding to the case of treatment) are shown through dark green points. It is worth noting that the actual and estimated values presented in Fig. 3 and in the successive figures of the main text have been de-normalized by row, since the application of the MCFE method was done after performing the \(l_1\) -norm row-normalization, then it was necessary to invert that process to go back to the original scale of the data. At first glance, we can see that the estimated values are, at a different extent, higher than the actual values for all treated countries in our analysis. In other words, according to our results, the ETS policy generally reduced \(\hbox {CO}_{2}\) emissions of treated countries, particularly in the years after the second year of the second treatment phase (i.e., from 2009 onwards), as intended by the policy itself. It is worth noting that to obtain such a result, it was necessary to use the MCFE method by 12 instead of the MCB method (without fixed effects estimation) by 66 , as explained in the “ Methodology ” section.
Application of statistical tests about the comparison between estimated and actual data
A parametric t -student test for the difference between means in independent populations (paired data t -test) was also performed to test whether the difference between actual values and estimated values was statistically significant under the hypothesis of no treatment. The test was performed for both the raw data and their natural logarithmic transformation (to more easily satisfy the normality assumption). To perform this statistical test, we considered two samples ( \(S_1\) , with the actual values, and \(S_2\) , with the imputed values) with the same sample size of \(n_1=n_2=272\) , where 272 is the product of the number of countries treated (17) and the number of years of treatment (16).
For all 100 simulations performed (with both raw and log normalized data), we rejected the null hypothesis of equal means. This simple evidence combined with Fig. 3 might suggest that the introduction of the EU ETS policy had a significant effect on reducing \(\hbox {CO}_{2}\) emissions. At the same time, without a further check, we cannot rule out that this preliminary result is the consequence of using a positively biased estimator, i.e., the method used might tend to yield higher values than the true (unknown) counterfactual values.
To verify that this was not the case, we compared, as a diagnostic test, the actual values and the values estimated by the MCFE method for both the training and validation sets in the case of the untreated countries (this comparison was not possible for the test set because the counterfactual values were not available as ground truth). If the MCFE method we used were robust (i.e., if there were no significant overestimates or underestimates), then the true and estimated values for these countries would be essentially indistinguishable (especially in the case of the training set). This would be particularly important in the case of the validation set because it would rule out the overfitting of the training set.
Figures 4 and 5 —referring respectively to the training set (restricted to the untreated countries after the start of treatment for treated countries) and the validation set (which, by construction, refers only to the untreated countries),—show that the differences between the actual values and the values estimated by MCFE were, as expected, quite negligible with respect to the corresponding differences obtained, in the original analysis, on the elements of the test set related to the treated countries.
Robustness checks
To further verify that our main results, related to the significant reduction of \(\hbox {CO}_{2}\) emissions by the treatment, were not affected by a systematic overestimation, we decided to perform a counter-proof as a robustness test. To this end, we repeated the counterfactual analysis by reversing the roles of treated and untreated countries. In other words, this time we considered the EU countries as untreated and the non-EU countries as treated. So, for this second analysis, the (modified) test set was for the non-EU countries over the period 2005–2020. As can be seen in Fig. 6 , the treatment effects (artificial this time) remained very strong (though reversed in sign, as expected) and, in particular, it was possible to rule out the problem of systematic overestimation of the MCFE method used, since the predicted values on the new test set looked much lower than the observed values.
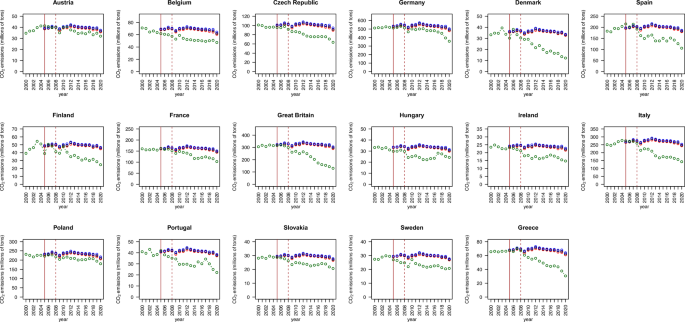
\(\hbox {CO}_{2}\) emissions of treated countries. Actual values (dark green points) compared to values calculated by MCFE for the no-treatment hypothesis (test set). Medians (black points), 10th percentiles (red points), and 90th percentiles (blue points) considering the 100 MCFE random simulations. The solid vertical red line divides the period into pre-treatment and treatment periods. The dashed vertical red line represents the start of the second phase of ETS. The reader is referred to the “ Comparison between counterfactual/actual values in the treatment period ”–“ Analysis of the effects of the EU ETS policy on CO 2 emissions ” sections for an additional discussion of this figure.
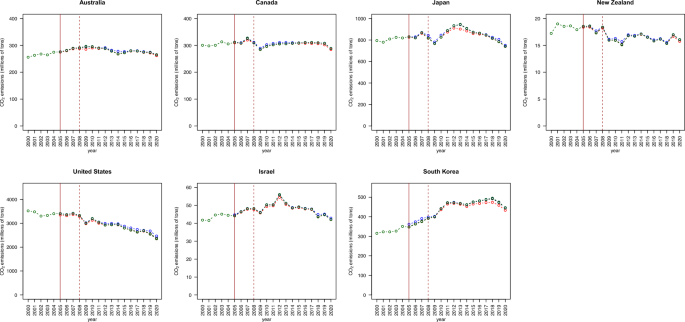
\(\hbox {CO}_{2}\) emissions of untreated countries. Actual values (dark green points) compared to values calculated by MCFE for the no-treatment hypothesis (training set only, after the start of treatment of treated countries). Medians (black points), 10th percentiles (red points), and 90th percentiles (blue points) considering the 100 MCFE random simulations. The solid vertical red line divides the period into pre-treatment and treatment periods. The dashed vertical red line represents the start of the second phase of ETS. The reader is referred to the “ Application of statistical tests about the comparison between estimated and actual data ” section for an additional discussion of this figure.
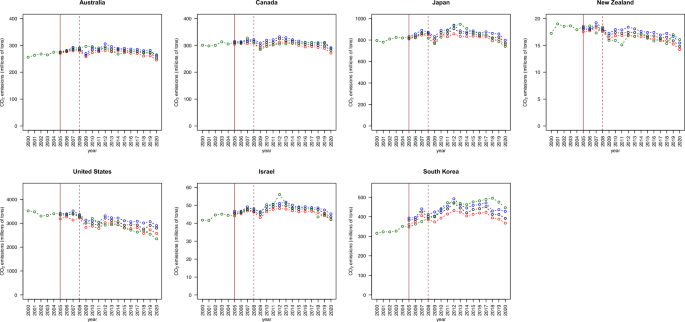
\(\hbox {CO}_{2}\) emissions of untreated countries. Actual values (dark green points) compared to values calculated by MCFE for the no-treatment hypothesis (validation set only). Medians (black points), 10th percentiles (red points), and 90th percentiles (blue points) considering the 100 MCFE random simulations. The solid vertical red line divides the period into pre-treatment and treatment periods. The dashed vertical red line represents the start of the second phase of ETS. The reader is referred to the “ Application of statistical tests about the comparison between estimated and actual data ” section for an additional discussion of this figure.
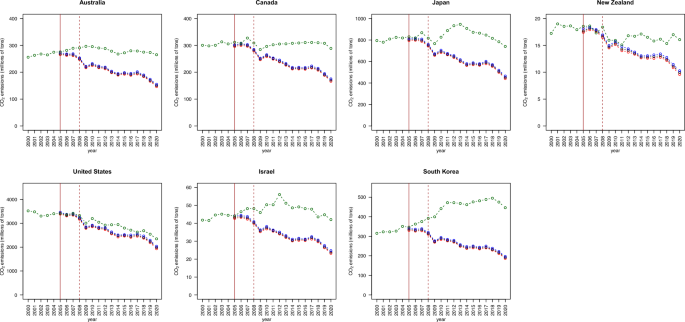
Inversion of treated and untreated countries in MCFE analysis. \(\hbox {CO}_{2}\) emissions of untreated countries. Actual values (dark green points) versus values calculated by MCFE in the treatment hypothesis (modified test set). Medians (black points), 10th percentiles (red points), and 90th percentiles (blue points) considering the 100 MCFE random simulations. The solid vertical red line divides the period into pre-treatment and treatment periods. The dashed vertical red line represents the start of the second phase of ETS. The reader is referred to the “ Robustness checks ” section for an additional discussion of this figure.
Analysis of the effects of the EU ETS policy on \(\hbox {CO}_{2}\) emissions
To return to the original analysis presented in the “ Comparison between counterfactual/actual values in the treatment period ” section, as can be seen in Fig. 3 , when comparing the actual values of treated countries in the years of treatment with the medians of the corresponding counterfactual estimates ( Remark 23 : For simplicity, we consider here and below the medians of the estimated values instead of the estimated values themselves, since these are random variables.) obtained (in the case of no treatment) by the MCFE simulations, we can conclude that the EU ETS policy was effective in reducing \(\hbox {CO}_{2}\) emissions. This is in line with other literature such as 31 , 51 .
As shown in the “ Supplementary Material ”, during the whole treatment period covered in the database (2005–2020), the majority of the EU countries included in our analysis achieved a ratio between the sum of the observed values and the sum of the medians of the estimated values (expressed as a percentage) of about 75–80%. The smallest value (65.01%) was obtained in the case of Denmark (i.e., Denmark’s \(\hbox {CO}_{2}\) emissions were reduced to more than 3/10 of the sum of the medians of the estimated counterfactual values associated with no treatment throughout the analysis period). The largest value (93.32%) was found in the case of Austria (this means that the amount of \(\hbox {CO}_{2}\) emissions of Austria decreased at a minimal extent during the whole treatment period covered in the database, i.e., 2005–2020, due to the EU ETS policy). In general, however, we can conclude that the EU ETS policy did not have an irrelevant impact on \(\hbox {CO}_{2}\) emissions in the EU. According to the results of our analysis, the reduction was even larger than that estimated by 31 . In that paper, the authors estimated a reduction in \(\hbox {CO}_{2}\) emissions (with respect to the case of the absence of the EU ETS policy) of about 3.8% using SCM across the EU for the period 2008–2016. According to the results of our analysis (based on the medians of the estimates), the reduction in \(\hbox {CO}_{2}\) emissions for all EU countries included in our analysis ( Remark 24 : This reduction was calculated by comparing the sum of \(\hbox {CO}_{2}\) emissions for each period for all EU countries included in the analysis with the sum of the medians of the estimated counterfactual \(\hbox {CO}_{2}\) emissions for the same period and the same EU countries.) was approximately 19.0% in the same period 2008–2016 which was considered by 31 ; 15.4% in the entire 2005–2020 treatment period (these results were obtained from the comparison of the sums of actual and counterfactual median values included in Fig. 7 and also in the Supplementary Section D of the Supplementary Material) ( Remark 25 : Still, we point out that the vertical distance between the various 10th and 90th percentile curves reported in Fig. 3 tends to increase when moving towards the end of the time horizon of analysis, so the results obtained at the end of the period turn out to be less reliable than, e.g., the ones related to the first year in the treatment period.).
Our results are consistent with the large increase in \(\hbox {CO}_{2}\) emissions compared to the pre-treatment period, as shown in Fig. 5 . Although both our analysis and that presented by 31 show positive effects of the EU ETS policy, some differences are observed in the magnitude of the effects achieved. This result could be explained not only by the different selection of EU countries considered in the two analyses ( Remark 26 : Supplementary Sections B and C of the Supplementary Material show that a similar magnitude of the estimated effects was obtained by replacing MCFE with either DiD or SCM (thus, providing even stronger support to the results obtained by MCFE). Nevertheless, MCFE still presented several advantages over these two other methods, as detailed in those appendices.), but also by the fact that the work 31 , neglecting possible transmission effects, derived all their control and treated units within the same group of EU countries (i.e., their control and treated units were, respectively, economic sectors of EU countries directly affected by EU ETS policy and other economic sectors of the same EU countries not directly affected by the EU ETS policy). Instead, our analysis is done at a more aggregate level (i.e., EU countries are treated as a whole, limiting to industries potentially affected by that policy, while the control units are other countries outside the EU).
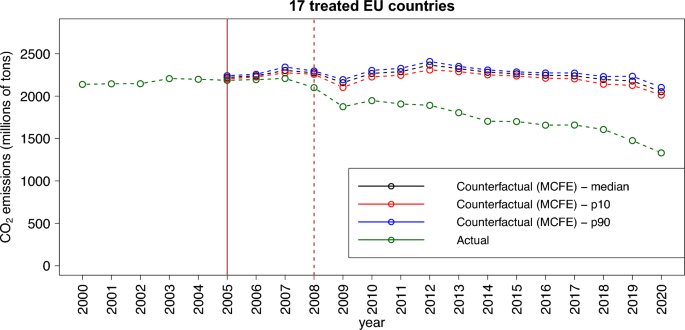
Sums of (observed and estimated) \(\hbox {CO}_{2}\) emissions of the entire group of the 17 treated EU countries. Actual values (dark green points) compared to values calculated by MCFE for the hypothesis without EU ETS treatment (test set). Sum of medians across treated countries (black points), sum of 10th percentiles across treated countries (red points), and sum of 90th percentiles across treated countries (blue points) considering the 100 MCFE random simulations. The solid vertical red line divides the period into the pre-treatment and treatment periods. The dashed vertical red line represents the start of the second phase of ETS. The reader is referred to the “ Analysis of the effects of the EU ETS policy on CO 2 emissions ” section for an additional discussion of this figure.
It is worth noting that our results change somewhat if we consider \(\hbox {CO}_{2}\) emissions reductions only in the first phase of the EU ETS policy (2005–2007), as indicated in the “ Supplementary Material ”. In this case, the reduction in \(\hbox {CO}_{2}\) emissions from the EU ETS policy was much smaller. In particular, looking at the median estimates, the reduction in \(\hbox {CO}_{2}\) emissions across the entire group of EU countries included in the analysis was about 2.5% in 2005–2007 ( Remark 27 : It is worth noting that the results obtained by our analysis, at the level of the whole set of treated countries, turned out to be quite robust with respect to variations in the data set. Indeed, we performed a similar analysis using \(\hbox {CO}_{2}\) emissions data from a different source (the WIOD database environmental accounts, see 76 ). Two smaller subsets of treated and untreated countries were selected, a shorter period of analysis was considered, and the aggregation of \(\hbox {CO}_{2}\) emissions was made by considering all the industries. In spite of these changes, our estimated reductions of \(\hbox {CO}_{2}\) emissions in the whole set of treated countries in the periods 2005–2007 and 2008–2016 ( \(3.1\%\) and \(19.4\%\) , respectively) were quite similar to the ones obtained in the present analysis, based on the EDGAR database (i.e., \(2.5\%\) and \(19.0\%\) , respectively). This issue, combined with the extremely high coverage of \(\hbox {CO}_{2}\) emissions by the data set considered in the present analysis, leads us to believe that the impacts estimated by our analysis are quite robust to different approaches to identify treated and control units of analysis.). Moreover, in three countries (Austria, Italy and Spain) the sums of the medians of the estimated counterfactuals were even higher than the corresponding sums of the observed values. In the first phase of the policy, the penalty for \(\hbox {CO}_{2}\) emissions exceeding the quota was indeed small. This fact could be a possible explanation for the lower reduction in the first years of the policy.
Conclusions, policy implications, and possible future research developments
\(\hbox {CO}_{2}\) emissions represent a growing problem closely related to pollution and climate change. Economic systems produce large amounts of \(\hbox {CO}_{2}\) through the use of fossil energy. Therefore, governments are trying to shift production to new systems in order to reduce emissions 77 . In this context, the EU has introduced a market for emission rights, called the Emissions Trading Scheme (ETS), which was launched in 2005 and further expanded in subsequent years, as the second phase began in 2008. The impact of EU ETS on reducing \(\hbox {CO}_{2}\) emissions is still debated in the literature.
In this paper, we present a new approach to quantify the impact of EU ETS policy on \(\hbox {CO}_{2}\) emissions reductions. A counterfactual analysis allows us to quantify the reduction in \(\hbox {CO}_{2}\) emissions from the ETS policy. The novelty of our work is that we apply, for the evaluation of the EU ETS policy, a state-of-the-art Statistical Machine Learning (SML) method based on Matrix Completion with Fixed Effects estimation (MCFE) for counterfactual analysis. The importance of using MCFE for this task becomes clear when one considers that conventional policy evaluation methods such as matching techniques—e.g., Propensity Score Matching (PSM), Mahalanobis and Hainmueller balancing—and the Synthetic Control Method (SCM) are not always suitable for performing true policy evaluation, since in some applications it may be nearly impossible to identify an appropriate control group for these methods, or small-sample issues may arise ( Remark 28 : See Supplementary Section C of the Supplementary Material for a more detailed discussion on these issues for the SCM case.). Applying the MCFE method to the \(\hbox {CO}_{2}\) emissions matrix at the country-year level allowed us to quantitatively assess the impact of EU ETS on reducing emissions.
Using robust statistical tests and diagnostic controls, the effect of the EU ETS was found to be statistically significant, in line with some recent contributions. Based on our analysis, the \(\hbox {CO}_{2}\) reduction from this policy appears to be higher than that found in the previous literature. We believe that the previous literature tends to underestimate the \(\hbox {CO}_{2}\) reduction because it focused on the first phase of the policy and selected countries using less sophisticated methods to establish valid counterfactual estimates. We believe that overcoming such drawbacks through the adoption of MCFE is a significant result in terms of policy evaluation.
From the policy-making perspective, a first managerial/practical implication of this study concerns suggesting the adoption of policies similar to the EU ETS one to other similar countries (e.g., in the same phase of the environmental Kuznets curve) ( Remark 29 : This kind of application would be similar to the counter-proof reported in the “ Robustness checks ” section.), in the spirit of the classical Matrix Completion (MC) application to recommender systems, which has been outlined at the beginning of the “ Non-technical overview of the original contributions of the work ” section. A second managerial/practical implication of our study concerns following the same pipeline as in the “ Results ” section for the evaluation of the environmental impact of other policies different from the EU ETS one. As a third important managerial/practical implication, the methodology adopted in the study could be employed as one of the tools to assess the economic, social, and environmental impact of policies, as established by recent EU legislation ( Remark 30 : See https://commission.europa.eu/law/law-making-process/planning-and-proposing-law/impact-assessments_en .). In this context, MC could be used to do a more rigorous impact analysis of various policies, also with predictive aims: for instance, when one was given timely information for a group of countries but less recent information for a second group of countries, then one aimed to estimate trends for the countries in the latter group. This situation would likely arise when data related to different countries were not available simultaneously, but sequentially, because of the presence of heterogeneous data sources ( Remark 31 : A similar argument was used to motivate the recent MC application made by 57 in the context of input–output analysis.).
The results of our analysis make it possible in a rather simple way to express the reduction in environmental damage resulting from the introduction of the EU ETS policy in monetary terms. As this issue is not the focus of the present work, we plan to address it in a future extension of this research. Other possible future research developments concern overcoming some of the limitations of the current MCFE application, which are listed below along with possible ways to overcome these limitations.
First, the assumption that EU countries represent the treated group while the selected non-EU high-income countries are untreated by the EU ETS policy might oversimplify the complexity of that policy implementation and of its environmental impact. In particular, the possible presence of confounding factors—such as heterogeneous population growth and Gross Domestic Product (GDP) growth—could influence the estimates ( Remark 32 : It is also worth noting that the MC methodology adopted in our work already deals partially with these issues, because, differently from other econometric methods such as the Difference-in-Differences (DiD), it does not require the parallel trend assumption in the pre-treatment phase, implying the possibility to extrapolate time series to the treatment period even in the presence of heterogeneous trends in such time series during the pre-treatment period.) and would deserve further investigation in a future development of the present study ( Remark 33 : The possible presence of such factors is one additional reason for the exclusion of small countries from the data set used for the analysis made in the present study, in view of their expected high sensitivity to small shocks (see “ Description of the data set ” section).). Still remaining in the context of a MCFE analysis, the validity of the assumption above could be verified in the following way. First, one could include additional covariates in the MCFE optimization problem, either by inserting them at the beginning of each row of the matrix to be reconstructed (as is done in some applications of SCM 78 ), or by applying the extension to the presence of covariates, still proposed by 12 , of the MCFE optimization problem considered in this study. Then, one would compare the resulting estimates with the ones achieved in the present work, assessing if only minor changes were obtained. Such additional analyses are expected to require an extensive integration of the available data set, with the aim of including a sufficiently large number of potential confounders. An alternative way to address this issue could consist in replacing MC(FE) with either collective MC 79 or tensor completion 80 (possibly still with the insertion of covariates in the model). This could have the advantage of making it possible to consider different levels of treatment. However, both the latter extensions are expected to be much more computationally expensive than the MCFE approach adopted in this work.
Second, the MCFE application made in the present study works at quite an aggregate level, since the entries of the matrix to be reconstructed refer to \(\hbox {CO}_{2}\) emissions at the country-year level, by restricting the aggregation to \(\hbox {CO}_{2}\) emissions coming from selected industries. On one hand, a first possible future development could consist in a further data aggregation in each country by considering \(\hbox {CO}_{2}\) emissions coming from all the industries, with the aim of verifying the absence of leakage effects of the EU ETS policy with respect to other industries in the same country. On the other hand, as a second possible future development, one could further disaggregate the data. For instance, after estimating the yearly impact of the ETS policy for a specific EU country (as made in the present work), one could distinguish between treated/untreated industries in that country, and perform the analysis at the industry-year level (focusing on the specific country). This would help assessing possible heterogeneous impacts of the ETS policy on different industries of the selected country. It is worth mentioning that, differently from the MCFE application considered in the present article, at this less aggregate level the average effect of the ETS policy of a given EU country on \(\hbox {CO}_{2}\) emissions related to the activity of specific industries could be even negative, while at the level of the whole country, the effect of the policy on \(\hbox {CO}_{2}\) emissions of the activity of all the industries in that country is expected to be positive, at least after some years since the adoption of the policy. So, identifying industries for which a negative average effect is obtained could help policymakers to investigate improvements of the policy and/or the possible presence of confounding factors that have influenced negatively the policy implementation.
Third, the EU ETS policy is a rather complex policy, which actually covers several phases of actuation. In our work, we have simplified the analysis of the treated countries by including information for them only on the fact they were treated/untreated in a specific period. Indeed, a direct application to causal inference of the matrix completion methodology developed by 12 allows one only to distinguish, for treated countries, between periods of treatment and periods of no treatment, but does not allow to include (at least in a straightforward manner) information about the occurrence of a specific phase of treatment. Further developments of this methodology would make it more feasible to extend the present MC analysis to the estimation of phase-dependent \(\hbox {CO}_{2}\) counterfactual emission levels.
Fourth, for future research, we also consider developing a more sophisticated model that examines (in monetary terms) whether or not the decrease in output due to the price of \(\hbox {CO}_{2}\) emissions outweighs the reduced environmental damage (this would require an appropriate definition of green gross domestic product). In addition, the whole analysis could be extended to a less aggregate level (with larger matrices) after accelerating/parallelizing the MCFE implementation, as was done recently by 60 for another application of the related baseline MC (MCB) method. This is important for the potential use of MCFE with three-dimensional data set of countries, industries, and years, possibly achieved by associating country-industry pairs with a single index, to avoid replacing MC with tensor completion. Finally, more sophisticated methods could be applied to obtain an estimate of the possible indirect impact of the EU ETS policy (e.g., related to carbon leakage to other non-EU countries) to better estimate the overall impact of the EU ETS policy.
Fifth, another possible research development concerns the investigation of potential adverse economic effects of the EU ETS policy. This would require replacing/combining the data set used for the current analysis with one related to the yearly level of economic activities of each country. Beside MCFE, other possible methods of analysis could be the already-mentioned collective MC and tensor completion.
Finally, the recent Machine Learning (ML) literature points out other methods for time-series prediction, such as multivariate Gaussian processes regression 81 , and deep learning techniques 82 , which could be also considered as possible alternative methods for counterfactual prediction in a future extension of this research.
Data availibility
The database used (i.e., the Emissions Database for Global Atmospheric Research, or EDGAR) can be accessed for free at https://edgar.jrc.ec.europa.eu/ . Population data are taken from the World Bank’s free database, available at https://data.worldbank.org/ . The MCFE analysis performed in this work is based on the application of the function “mcnnm” contained in the R package “MCPanel”, which is freely available at the following hyperlink: https://rdrr.io/github/susanathey/MCPanel/man/mcnnm.html . This function allows the possibility of either estimating or not the row-fixed and column-fixed effects (hence, to apply also the MCB method). The SCM application is based on the MATLAB package “Synth”, which is freely available at the following hyperlink: https://web.stanford.edu/~jhain/Synth_Matlab/Synth_MATLAB.zip .
El Ouardighi, F., Kogan, K., Gnecco, G. & Sanguineti, M. Transboundary pollution control and environmental absorption efficiency. Ann. Oper. Res. 287 , 653–681 (2020).
Article MathSciNet Google Scholar
Machado, F. L. V., Halmenschlager, V., Abdallah, P. R., da Silva Teixeira, G. & Sumaila, U. R. The relation between fishing subsidies and CO 2 emissions in the fisheries sector. Ecol. Econ. 185 , 107057 (2021).
Article Google Scholar
Sun, X., Dong, Y., Wang, Y. & Ren, J. Sources of greenhouse gas emission reductions in OECD countries: Composition or technique effects. Ecol. Econ. 193 , 107288 (2022).
Zhang, Y., Wang, F. & Zhang, B. The impacts of household structure transitions on household carbon emissions in China. Ecol. Econ. 206 , 107734 (2023).
Van Aalst, M. K. The impacts of climate change on the risk of natural disasters. Disasters 30 , 5–18 (2006).
Article PubMed Google Scholar
Tol, R. S. J. The economic effects of climate change. J. Econ. Perspect. 23 , 29–51 (2009).
Ackerman, F. & Munitz, C. Climate damages in the FUND model: A disaggregated analysis. Ecol. Econ. 77 , 219–224 (2012).
Burke, M., Hsiang, S. M. & Miguel, E. Global non-linear effect of temperature on economic productions. Nature 527 , 235–239 (2015).
Article ADS CAS PubMed Google Scholar
Burke, M., Davis, W. M. & Diffenbaugh, N. S. Large potential reductions in economic damages under UN mitigation targets. Nature 557 , 549–53 (2018).
Burke, M. & Tanutama, V. Climatic Constraints on Aggregate Economic Output NBER working paper 25779 (2019).
Martin, R., Muûls, M. & Wagner, U. J. The impact of the European Union emissions trading scheme on regulated firms: What is the evidence after ten years? Rev. Environ. Econ. Policy 10 , 129–148 (2016).
Athey, S., Bayati, M., Doudchenko, N., Imbens, G. & Khosravi, K. Matrix completion methods for causal panel data models. J. Am. Stat. Assoc. 116 , 1716–1730 (2021).
Article MathSciNet CAS Google Scholar
Martelli, S. et al. Do voters support local commitments for climate change mitigation in Italy? Ecol. Econ. 144 , 27–35 (2018).
Binder, S. & Neumayer, E. Environmental pressure group strength and air pollution: An empirical analysis. Ecol. Econ. 55 , 527–538 (2005).
Soliman, A. Prescriptive drought policy and water supplier compliance. Ecol. Econ. 197 , 107429 (2022).
Doremus, J. Unintended impacts from forest certification: Evidence from indigenous Aka households in Congo. Ecol. Econ. 166 , 106378 (2019).
Koch, N. & Themann, M. Catching up and falling behind: Cross-country evidence on the impact of the EU ETS on firm productivity. Resour. Energy Econ. 69 , 101315 (2022).
Heckman, J. J., Ichimura, H. & Todd, P. E. Matching as an econometric evaluation estimator: Evidence from evaluating a job training programme. Rev. Econ. Stud. 64 , 605–654 (1997).
Hainmueller, J. Entropy balancing for causal effects: A multivariate reweighting method to produce balanced samples in observational studies. Polit. Anal. 20 , 25–46 (2012).
Pellegrini, L., Tasciotti, L. & Spartaco, A. A regional resource curse? A synthetic-control approach to oil extraction in Basilicata, Italy. Ecol. Econ. 185 , 107041 (2021).
Green, J. F. Does carbon pricing reduce emissions? A review of ex-post analyses. Environ. Res. Lett. 16 , 043004 (2021).
Article ADS CAS Google Scholar
Teixidó, J., Verde, S. F. & Nicolli, F. The impact of the EU emissions trading system on low-carbon technological change: The empirical evidence. Ecol. Econ. 164 , 106347 (2019).
Verde, S. F. The impact of the EU emissions trading system on competitiveness and carbon leakage: The econometric evidence. J. Econ. Surv. 34 , 320–343 (2020).
IPCC. Climate Change 2022: Mitigation of Climate Change (Cambridge University Press, 2022). Contribution of Working Group III to the Sixth Assessment Report of the Intergovernmental Panel on Climate Change (eds Shukla, P. R., Skea, J., Slade, R., Al Khourdajie, A., van Diemen, R., McCollum, D., Pathak, M., Some, S., Vyas, P., Fradera, R., Belkacemi, M., Hasija, A., Lisboa, G., Luz, S., Malley, J.).
Stavins, R. N. The Future of U.S. Carbon-Pricing Policy Vol. 56 (Harvard Environmental Economics Program, Cambridge, 2019).
Book Google Scholar
Aydin, C. & Esen, O. Reducing CO 2 emissions in the EU member states: Do environmental taxes work? J. Environ. Plan. Manag. 61 , 2396 (2018).
Haites, E. et al. Experience with carbon taxes and greenhouse gas emissions trading systems. Duke Environ. Law Policy Forum 29 , 109–182 (2018).
Google Scholar
Narassimhan, E., Gallagher, K. S., Koester, S. & Alejo, J. R. Carbon pricing in practice: A review of existing emissions trading systems. Clim. Policy 18 , 967–991 (2018).
Andersson, J. J. Carbon taxes and CO 2 emissions: Sweden as a case study. Am. Econ. J. Econ. Pol. 11 , 1–30 (2019).
FSR Climate. A literature-based assessment of the EU ETS. Florence School of Regulation 224 (European University Institute, 2019).
Bayer, P. & Aklin, M. The European Union emissions trading system reduced CO 2 emissions despite low prices. Proc. Natl. Acad. Sci. 117 , 8804–8812 (2020).
Article ADS CAS PubMed PubMed Central Google Scholar
Diaz, G., Munoz, F. D. & Moreno, R. Equilibrium analysis of a tax on carbon emissions with pass-through restrictions and side-payment rules. Energy J. 41 , 93–122 (2020).
Arimura, T. H. & Abe, T. The impact of the Tokyo emissions trading scheme on office buildings: What factor contributed to the emission reduction? Environ. Econ. Policy Stud. 23 , 517–533 (2021).
Pretis, F. Does a carbon tax reduce CO 2 emissions? Evidence from British Columbia. Environ. Resour. Econ. 83 , 115–144 (2022).
Metcalf, G. E. & Stock, J. H. The macroeconomic impact of Europe’s carbon taxes. Am. Econ. J. Macroecon. 15 , 265–286 (2023).
Rafaty, R., Dolphin, G. & Pretis, F. Carbon pricing and the elasticity of CO 2 emissions . Resources for the Future Working Paper 21–23 (2023). https://media.rff.org/documents/wp_21-33_tUca3j8.pdf .
Calel, R. & Dechezleprêtre, A. Environmental policy and directed technological change: Evidence from the European carbon market. Rev. Econ. Stat. 98 , 173–191 (2016).
van den Bergh, J. & Savin, I. Impact of carbon pricing on low-carbon innovation and deep decarbonisation: Controversies and path forward. Environ. Resour. Econ. 80 , 705–715 (2021).
Zhang, Z. & Zhang, Z. Intermediate input linkage and carbon leakage. Environ. Dev. Econ. 22 , 725–746 (2017).
Article CAS Google Scholar
Branger, F., Quirion, P. & Chevallier, J. Carbon leakage and competitiveness of cement and steel industries under the EU ETS: Much ado about nothing. Energy J. 37 , 109–135 (2016).
Koch, N. & Basse Mama, H. Does the EU emissions trading system induce investment leakage? Evidence from German multinational firms. Energy Econ. 81 , 479–492 (2019).
aus dem Moore, N., Grokurth, P. & Themann, M. Multinational corporations and the EU emissions trading system: The specter of asset erosion and creeping deindustrialization. J. Environ. Econ. Manag. 94 , 1–26 (2019).
Borghesi, S., Franco, C. & Marin, G. Outward foreign direct investment patterns of Italian firms in the European Union’s emission trading scheme. Scand. J. Econ. 122 , 219–256 (2020).
Kuusi, T., et al. Carbon Border Adjustment Mechanisms and Their Economic Impact on Finland and the EU (ETLA Economic Research, 2020). https://www.etla.fi/en/publications/carbon-border-adjustment- mechanisms-and-their-economic-impact-on-finland-and-the-eu/ .
Venmans, F., Ellis, J. & Nachtigall, D. Carbon pricing and competitiveness: Are they at odds? Clim. Policy 20 , 1070–1091 (2020).
Chéze, B., Chevallier, J., Berghmans, N. & Alberola, E. On the CO 2 emissions determinants during the EU ETS phases I and II: A plant-level analysis merging the EUTL and Platts power data. Energy J. 41 , 153–183 (2020).
World Bank. State and Trends of Carbon Pricing 2021 87 (World Bank Group, 2021).
Calel, R. Adopt or innovate: Understanding technological responses to cap-and-trade. Am. Econ. J. Econ. Pol. 12 , 170–201 (2020).
Jaraitė, J. & Maria, C. D. Did the EU ETS make a difference? An empirical assessment using Lithuanian firm-level data. Energy J. 37 , 1–23 (2016).
Klemetsen, M., Rosendahl, K. E. & Jakobsen, A. L. The impacts of the EU ETS on Norwegian plants’ environmental and economic performance. Clim. Change Econ. 11 , 2050006 (2020).
Petrick, S. & Wagner, U. J. The impact of carbon trading on industry: Evidence from German manufacturing firms Kiel Working Paper No. 1912 (2014).
Wagner, U. J., Muûls, M., Martin, R. & Colmer, J. The causal effect of the European Union emissions trading scheme: Evidence from French manufacturing plants. In Proceedings of the IZA Workshop: Labor Market Effects of Environmental Policies 33 (2014).
Dechezleprêtre, A., Nachtigall, D. & Venmans, F. The joint impact of the European Union emissions trading system on carbon emissions and economic performance. J. Environ. Econ. Manag. 118 , 102758 (2023).
Abadie, A. Using synthetic controls: Feasibility, data requirements, and methodological aspects. J. Econ. Lit. 59 , 391–425 (2021).
Anderson, H. M., Gao, J., Turnip, G., Vahid, F. & Wei, W. Estimating the effect of an EU-ETS type scheme in Australia using a synthetic treatment approach. Energy Econ. 125 , 106798 (2023).
Hastie, T., Tibshirani, R. & Wainwright, M. Statistical Learning with Sparsity Vol. 143 (CRC Press, 2015).
Metulini, R., Gnecco, G., Biancalani, F. & Riccaboni, M. Hierarchical clustering and matrix completion for the reconstruction of world input–output tables. Adv. Stat. Anal. 107 , 575–620 (2023).
Timmer, M. P., Dietzenbacher, E., Los, B., Stehrer, R. & De Vries, G. J. An illustrated user guide to the world input–output database: The case of global automotive production. Rev. Int. Econ. 23 , 575–605 (2015).
Gnecco, G., Nutarelli, F. & Riccaboni, M. A machine learning approach to economic complexity based on matrix completion. Sci. Rep. 12 , 9639 (2022).
Gnecco, G., Nutarelli, F. & Riccaboni, M. Matrix completion of world trade: An analysis of interpretability through Shapley values. World Econ. 46 , 2707–2731 (2023).
Gnecco, G., Landi, S. & Riccaboni, M. Can machines learn creativity needs? An approach based on matrix completion. Ital. Econ. J. 9 , 1111–1151 (2023).
Gnecco, G., Landi, S. & Riccaboni, M. The emergence of social soft skill needs in the post COVID-19 era. Qual. Quant. 58 , 647–680 (2024).
Huang, W., Wei, D., Wang, C. & Lin, C. Matrix completion-based prediction analysis in carbon emissions. Int. J. Embed. Syst. 14 , 143–148 (2021).
Biancalani, F., Gnecco, G., Metulini, R. & Riccaboni, M. Matrix completion for the prediction of yearly country and industry-level CO 2 emissions. In Machine Learning, Optimization, and Data Science, 8th International Workshop, LOD 2022, Certosa di Pontignano, Italy, September 19–22, 2022, Revised Selected Papers, Part I 14–19 (2023).
Biancalani, F., Gnecco, G., Metulini, R. & Riccaboni, M. Prediction of annual CO 2 emissions at the country and sector levels, based on a matrix completion optimization problem. Optim. Lett. https://doi.org/10.1007/s11590-023-02052-2 (2023).
Mazumder, R., Hastie, T. & Tibshirani, R. Spectral regularization algorithms for learning large incomplete matrices. J. Mach. Learn. Res. 11 , 2287–2322 (2010).
MathSciNet PubMed PubMed Central Google Scholar
Metulini, R., Biancalani, F., Gnecco, G. & Riccaboni, M. Assessing the performance of nuclear norm-based matrix completion methods on CO 2 emissions data. In Book of the Short Papers—SIS 2023—Statistical Learning, Sustainability and Impact Evaluation (eds. Chelli, F. M., Ciommi, M., Ingrassia, S., Mariani, F., Recchioni, M. C.) 776–781 (Pearson, 2023).
Crippa, M., et al. GHG emissions of all world countries Publications Office of the European Union, Luxembourg, JRC134504. https://doi.org/10.2760/953322 (2023).
Grossman, G. M. & Krueger, A. B. Economic growth and the environment. Q. J. Econ. 110 , 353–377 (1995).
Raude, M., Mazzarano, M. & Borghesi, S. EU emissions trading system sectoral environmental and economic indicators. EUI, RSC, Research Data, 2024, Florence School of Regulation, LIFE COASE—Collaborative Observatory for Assessment of the EU ETS [Climate]. https://hdl.handle.net/1814/76817 .
Song, Q., Ge, H., Caverlee, J. & Hu, X. Tensor completion algorithms in big data analytics. ACM Trans. Knowl. Discove. Data 13 , 1–48 (2019).
Wang, L., Ma, S. & Han, Q. Enhanced sparse low-rank representation via nonconvex regularization for rotating machinery early fault feature extraction. IEEE/ASME Trans. Mechatron. 27 , 3570–3578 (2022).
An, B., Zhao, Z., Wang, S., Chen, S. & Chen, X. Sparsity-assisted bearing fault diagnosis using multiscale period group lasso. ISA Trans. 98 , 338–348 (2020).
Feng, Z. Z., Yang, X., Subedi, S. & McNicholas, P. D. The LASSO and sparse least squares regression methods for SNP selection in predicting quantitative traits. IEEE/ACM Trans. Comput. Biol. Bioinf. 9 , 629–636 (2012).
Baltagi, B. H. Econometric Analysis of Panel Data 4th edn. (Springer, 2008).
Corsatea, T. D., et al. World Input–Output Database Environmental Accounts. Update 2000–2016 . JRC Technical Report (2019). https://op.europa.eu/en/publication-detail/-/publication/df9c194b-81ba-11e9-9f05-01aa75ed71a1/language-en .
Sgarciu, S., Scholz, D. & Müsgens, F. How CO 2 prices accelerate decarbonisation—The case of coal-fired generation in Germany. Energy Policy 173 , 113375 (2023).
Abadie, A., Diamond, A. & Hainmueller, J. Synthetic control methods for comparative case studies: Estimating the effect of California’s tobacco control program. J. Am. Stat. Assoc. 105 , 493–505 (2010).
Alaya, M. Z. & Klopp, O. Collective matrix completion. J. Mach. Learn. Res. 20 , 1–43 (2019).
MathSciNet Google Scholar
Liu, A. & Moitra, A. Tensor completion made practical. In Proceeding of the 34th Conference on Neural Information Processing Systems (NeurIPS 2020), Vancouver, Canada , 18905–18916 (ACM, 2020).
Chen, Z., Fan, J. & Wang, K. Multivariate Gaussian processes: Definitions, examples and applications. Metron 81 , 181–91 (2023).
Masti, D. & Bemporad, A. Learning nonlinear state-space models using autoencoders. Automatica 129 (1–9), 109666 (2021).
Download references
Acknowledgements
The work was supported in part by the “Contributi Liberali 2022” project “Application of Matrix Completion Techniques to the Definition of Economic and Financial Recommender Systems”, granted by the Bank of Italy, by the project “Aspetti Socioeconomici e di Sostenibilitá—Green Economy e Startup” (project code: PO232, CUP code: D65F21001600001), granted by MUR, by the PRIN 2022 project “MAHATMA” (CUP: D53D23008790006), funded by the European Union - Next Generation EU program, by the PRIN PNRR 2022 project “MOTUS” (CUP: D53D23017470001), funded by the European Union - Next Generation EU program, and by the PRIN PNRR 2022 project “SIGNUM” (CUP: F53D23010910001), funded by the European Union - Next Generation EU program.
Author information
Authors and affiliations.
AXES – IMT School for Advanced Studies, Lucca, Italy
Francesco Biancalani, Giorgio Gnecco & Massimo Riccaboni
Department of Economics, University of Bergamo, Bergamo, Italy
Rodolfo Metulini
Istituto Universitario di Studi Superiori, Pavia, Italy
Massimo Riccaboni
You can also search for this author in PubMed Google Scholar
Contributions
The authors equally contributed to the work.
Corresponding author
Correspondence to Giorgio Gnecco .
Ethics declarations
Competing interests.
The authors declare no competing interests.
Additional information
Publisher's note.
Springer Nature remains neutral with regard to jurisdictional claims in published maps and institutional affiliations.
Supplementary Information
Supplementary information., rights and permissions.
Open Access This article is licensed under a Creative Commons Attribution-NonCommercial-NoDerivatives 4.0 International License, which permits any non-commercial use, sharing, distribution and reproduction in any medium or format, as long as you give appropriate credit to the original author(s) and the source, provide a link to the Creative Commons licence, and indicate if you modified the licensed material. You do not have permission under this licence to share adapted material derived from this article or parts of it. The images or other third party material in this article are included in the article’s Creative Commons licence, unless indicated otherwise in a credit line to the material. If material is not included in the article’s Creative Commons licence and your intended use is not permitted by statutory regulation or exceeds the permitted use, you will need to obtain permission directly from the copyright holder. To view a copy of this licence, visit http://creativecommons.org/licenses/by-nc-nd/4.0/ .
Reprints and permissions
About this article
Cite this article.
Biancalani, F., Gnecco, G., Metulini, R. et al. The impact of the European Union emissions trading system on carbon dioxide emissions: a matrix completion analysis. Sci Rep 14 , 19676 (2024). https://doi.org/10.1038/s41598-024-70260-6
Download citation
Received : 12 April 2024
Accepted : 14 August 2024
Published : 24 August 2024
DOI : https://doi.org/10.1038/s41598-024-70260-6
Share this article
Anyone you share the following link with will be able to read this content:
Sorry, a shareable link is not currently available for this article.
Provided by the Springer Nature SharedIt content-sharing initiative
- Matrix completion with fixed effects estimation
- Counterfactual analysis
- Policy impact analysis
- Green economy
By submitting a comment you agree to abide by our Terms and Community Guidelines . If you find something abusive or that does not comply with our terms or guidelines please flag it as inappropriate.
Quick links
- Explore articles by subject
- Guide to authors
- Editorial policies
Sign up for the Nature Briefing: Anthropocene newsletter — what matters in anthropocene research, free to your inbox weekly.

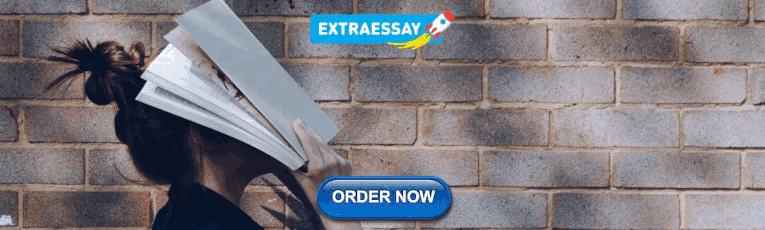
IMAGES
COMMENTS
Statistical Treatment Example - Quantitative Research. For a statistical treatment of data example, consider a medical study that is investigating the effect of a drug on the human population. As the drug can affect different people in different ways based on parameters such as gender, age and race, the researchers would want to group the ...
Research Paper Statistical Treatment of Data: A Primer. March 11, 2024. We can all agree that analyzing and presenting data effectively in a research paper is critical, yet often challenging. This primer on statistical treatment of data will equip you with the key concepts and procedures to accurately analyze and clearly convey research findings.
Table of contents. Step 1: Write your hypotheses and plan your research design. Step 2: Collect data from a sample. Step 3: Summarize your data with descriptive statistics. Step 4: Test hypotheses or make estimates with inferential statistics.
Practice of wrong or inappropriate statistical method is a common phenomenon in the published articles in biomedical research. Incorrect statistical methods can be seen in many conditions like use of unpaired t-test on paired data ... (mean ± SD) of the control (126.45 ± 8.85, n 1 =20) and treatment (121.85 ± 5.96, n 2 =20) group was ...
Introduction. Statistical analysis is necessary for any research project seeking to make quantitative conclusions. The following is a primer for research-based statistical analysis. It is intended to be a high-level overview of appropriate statistical testing, while not diving too deep into any specific methodology.
The SAMBR checklists have three parts as follows: Part (A) focuses on the reporting elements of common biomedical research designs, part (B) shows the essential steps to be followed in common studies for quality assessment of statistical analysis, and part (C) comprises the steps for data analysis and related essential and preferred choices of ...
1. Statistical Treatment in Data Analysis. The term "statistical treatment" is a catch all term which means to apply any statistical method to your data. Treatments are divided into two groups: descriptive statistics, which summarize your data as a graph or summary statistic and inferential statistics, which make predictions and test ...
Rule 5: Statistical Analysis Is More Than a Set of Computations. Statistical software provides tools to assist analyses, not define them. The scientific context is critical, and the key to principled statistical analysis is to bring analytic methods into close correspondence with scientific questions. See Rule 1. While it can be helpful to ...
Some of these methods and tools are used within specific fields of research, such as statistical tests of significance, double-blind trials, and proper phrasing of questions on surveys. Others apply across all research fields, such as describing to others what one has done so that research data and results can be verified and extended.
The new guidelines discuss many aspects of the reporting of studies in the Journal, including a requirement to replace P values with estimates of effects or association and 95% confidence ...
Now, if we divide the frequency with which a given mean was obtained by the total number of sample means (36), we obtain the probability of selecting that mean (last column in Table 10.5). Thus, eight different samples of n = 2 would yield a mean equal to 3.0. The probability of selecting that mean is 8/36 = 0.222.
Research Design and Methodology. Chapter 3 consists of three parts: (1) Purpose of the. study and research design, (2) Methods, and (3) Statistical. Data analysis procedure. Part one, Purpose of ...
Always Active. Design In many ways the design of a study is more important than the analysis. A badly designed study can never be retrieved, whereas a poorly analysed one can usually be reanalysed. (1) Consideration of design is also important because the design of a study will govern how the data are to be analys.
Categorical variables represent groupings of things (e.g. the different tree species in a forest). Types of categorical variables include: Ordinal: represent data with an order (e.g. rankings). Nominal: represent group names (e.g. brands or species names). Binary: represent data with a yes/no or 1/0 outcome (e.g. win or lose).
Statistical treatment of data also involves describing the data. The best way to do this is through the measures of central tendencies like mean, median and mode. These help the researcher explain in short how the data are concentrated. Range, uncertainty and standard deviation help to understand the distribution of the data.
In a previous article in this series, we looked at different types of data and ways to summarise them. 1 At the end of the research study, statistical analyses are performed to test the hypothesis and either prove or disprove it. The choice of statistical test needs to be carefully performed since the use of incorrect tests could lead to misleading conclusions.
Types of descriptive statistics. There are 3 main types of descriptive statistics: The distribution concerns the frequency of each value. The central tendency concerns the averages of the values. The variability or dispersion concerns how spread out the values are. You can apply these to assess only one variable at a time, in univariate ...
[email protected]. August 21, 2019. Abstract. The use of statistical software in academia and enterprises has been evolving over the last. years. More often than not, students, professors ...
The descriptive statistical analysis allows organizing and summarizing the large data into graphs and tables. Descriptive analysis involves various processes such as tabulation, measure of central tendency, measure of dispersion or variance, skewness measurements etc. 2. Inferential Analysis.
Abstract. Statistical methods involved in carrying out a study include planning, designing, collecting data, analysing, drawing meaningful interpretation and reporting of the research findings ...
In order to interpret research datasets, clinicians involved in clinical research should have an understanding of statistical methodology. This article provides a brief overview of statistical methods that are frequently used in clinical research studies. Descriptive and inferential methods, including regression modeling and propensity scores ...
Abstract. Statistical methods involved in carrying out a study include planning, designing, collecting data, analysing, drawing meaningful interpretation and reporting of the research findings. The statistical analysis gives meaning to the meaningless numbers, thereby breathing life into a lifeless data. The results and inferences are precise ...
Statistical treatment rules (STRs) are statistical decision functions that map observed covariates of population members and sample data on treatment response into treatment choices. I propose evaluation of STRs by their expected welfare (negative risk in Wald's terms), and I apply this criterion to compare two STRs when the sample data are ...
Jun 1992. POLYM COMPOSITE. A. Cervenka. P. Sheard. PDF | On Nov 1, 1979, James W. Dally published Statistical Treatment of Experimental Data | Find, read and cite all the research you need on ...
Despite the negative externalities on the environment and human health, today's economies still produce excessive carbon dioxide emissions. As a result, governments are trying to shift ...