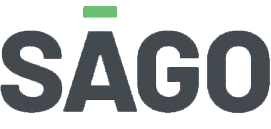
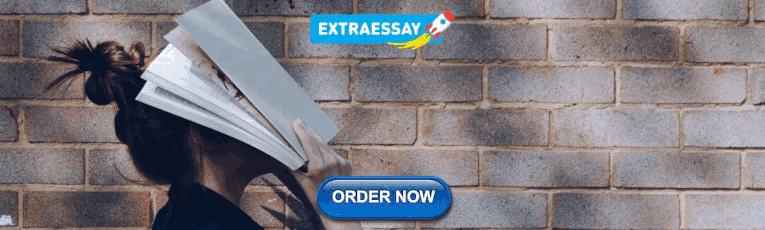
What We Offer
With a comprehensive suite of qualitative and quantitative capabilities and 55 years of experience in the industry, Sago powers insights through adaptive solutions.
- Recruitment
- Communities
- Methodify® Automated research
- QualBoard® Digital Discussions
- QualMeeting® Digital Interviews
- Global Qualitative
- Global Quantitative
- In-Person Facilities
- Healthcare Solutions
- Research Consulting
- Europe Solutions
- Neuromarketing Tools
- Trial & Jury Consulting
Who We Serve
Form deeper customer connections and make the process of answering your business questions easier. Sago delivers unparalleled access to the audiences you need through adaptive solutions and a consultative approach.
- Consumer Packaged Goods
- Financial Services
- Media Technology
- Medical Device Manufacturing
- Marketing Research
With a 55-year legacy of impact, Sago has proven we have what it takes to be a long-standing industry leader and partner. We continually advance our range of expertise to provide our clients with the highest level of confidence.
- Global Offices
- Partnerships & Certifications
- News & Media
- Researcher Events

Steve Schlesinger Inducted Into 2024 Market Research Council Hall of Fame
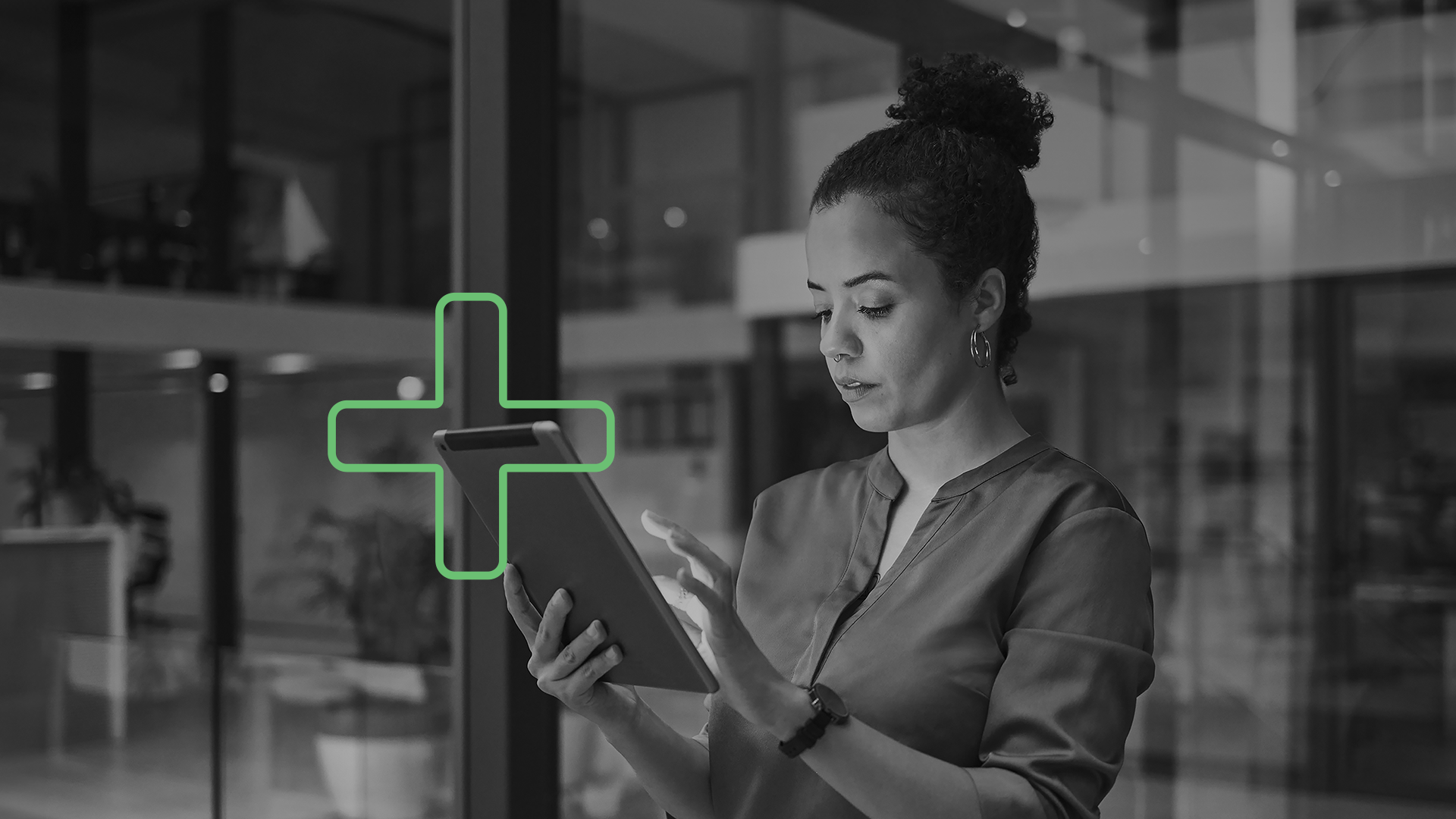
Sago Announces Launch of Sago Health to Elevate Healthcare Research
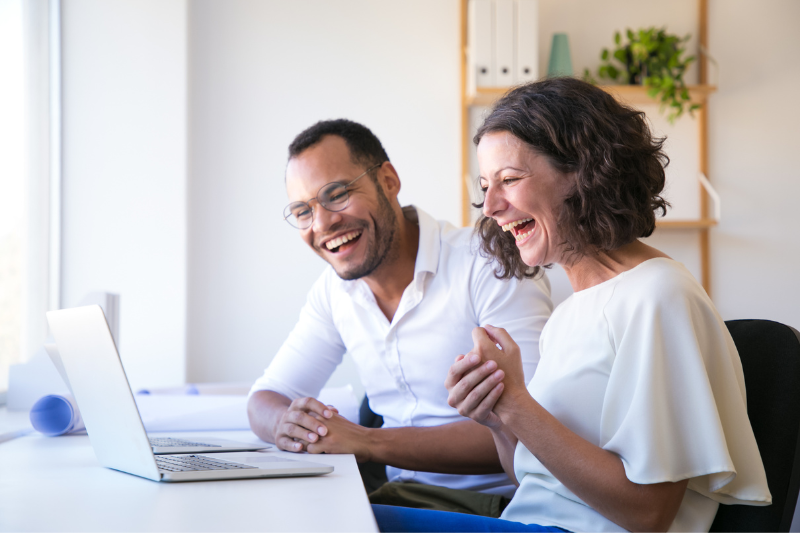
Sago Launches AI Video Summaries on QualBoard to Streamline Data Synthesis
Drop into your new favorite insights rabbit hole and explore content created by the leading minds in market research.
- Case Studies
- Knowledge Kit

Pioneering the Future of Pediatric Health

The Swing Voter Project, July 2024: Florida
- Get in touch

- Account Logins

The Significance of Validity and Reliability in Quantitative Research
- Resources , Blog

Key Takeaways:
- Types of validity to consider during quantitative research include internal, external, construct, and statistical
- Types of reliability that apply to quantitative research include test re-test, inter-rater, internal consistency, and parallel forms
- There are numerous challenges to achieving validity and reliability in quantitative research, but the right techniques can help overcome them
Quantitative research is used to investigate and analyze data to draw meaningful conclusions. Validity and reliability are two critical concepts in quantitative analysis that ensure the accuracy and consistency of the research results. Validity refers to the extent to which the research measures what it intends to measure, while reliability refers to the consistency and reproducibility of the research results over time. Ensuring validity and reliability is crucial in conducting high-quality research, as it increases confidence in the findings and conclusions drawn from the data.
This article aims to provide an in-depth analysis of the significance of validity and reliability in quantitative research. It will explore the different types of validity and reliability, their interrelationships, and the associated challenges and limitations.
In this Article:
The role of validity in quantitative research, the role of reliability in quantitative research, validity and reliability: how they differ and interrelate, challenges and limitations of ensuring validity and reliability, overcoming challenges and limitations to achieve validity and reliability, explore trusted quantitative solutions.
Take the guesswork out of your quant research with solutions that put validity and reliability first. Discover Sago’s quantitative solutions.
Request a consultation
Validity is crucial in maintaining the credibility and reliability of quantitative research outcomes. Therefore, it is critical to establish that the variables being measured in a study align with the research objectives and accurately reflect the phenomenon being investigated.
Several types of validity apply to various study designs; let’s take a deeper look at each one below:
Internal validity is concerned with the extent to which a study establishes a causal relationship between the independent and dependent variables. In other words, internal validity determines whether the changes observed in the conditional variable result from changes in the independent variable or some other factor.
External validity refers to the degree to which the findings of a study can be generalized to other populations and contexts. External validity helps ensure the results of a study are not limited to the specific people or context in which the study was conducted.
Construct validity refers to the degree to which a research study accurately measures the theoretical construct it intends to measure. Construct validity helps provide alignment between the study’s measures and the theoretical concept it aims to investigate.
Finally, statistical validity refers to the accuracy of the statistical tests used to analyze the data. Establishing statistical validity provides confidence that the conclusions drawn from the data are reliable and accurate.
To safeguard the validity of a study, researchers must carefully design their research methodology, select appropriate measures, and control for extraneous variables that may impact the results. Validity is especially crucial in fields such as medicine, where inaccurate research findings can have severe consequences for patients and healthcare practices.
Ensuring the consistency and reproducibility of research outcomes over time is crucial in quantitative research, and this is where the concept of reliability comes into play. Reliability is vital to building trust in the research findings and their ability to be replicated in diverse contexts.
Similar to validity, multiple types of reliability are pertinent to different research designs. Let’s take a closer look at each of these types of reliability below:
Test-retest reliability refers to the consistency of the results obtained when the same test is administered to the same group of participants at different times. This type of reliability is essential when researchers need to administer the same test multiple times to assess changes in behavior or attitudes over time.
Inter-rater reliability refers to the results’ consistency when different raters or observers monitor the same behavior or phenomenon. This type of reliability is vital when researchers are required to rely on different individuals to rate or observe the same behavior or phenomenon.
Internal consistency reliability refers to the degree to which the items or questions in a test or questionnaire measure the same construct. This type of reliability is important in studies where researchers use multiple items or questions to assess a particular construct, such as knowledge or quality of life.
Lastly, parallel forms reliability refers to the consistency of the results obtained when two different versions of the same test are administered to the same group of participants. This type of reliability is important when researchers administer different versions of the same test to assess the consistency of the results.
Reliability in research is like the accuracy and consistency of a medical test. Just as a reliable medical test produces consistent and accurate results that physicians can trust to make informed decisions about patient care, a highly reliable study produces consistent and precise findings that researchers can trust to make knowledgeable conclusions about a particular phenomenon. To ensure reliability in a study, researchers must carefully select appropriate measures and establish protocols for administering the measures consistently. They must also take steps to control for extraneous variables that may impact the results.
Validity and reliability are two critical concepts in quantitative research that significantly determine the quality of research studies. While both terms are often used interchangeably, they refer to different aspects of research. Validity is the extent to which a research study measures what it claims to measure without being affected by extraneous factors or bias. In contrast, reliability is the degree to which the research results are consistent and stable over time and across different samples , methods, and evaluators.
Designing a research study that is both valid and reliable is essential for producing high-quality and trustworthy research findings. Finding this balance requires significant expertise, skill, and attention to detail. Ultimately, the goal is to produce research findings that are valid and reliable but also impactful and influential for the organization requesting them. Achieving this level of excellence requires a deep understanding of the nuances and complexities of research methodology and a commitment to excellence and rigor in all aspects of the research process.
Ensuring validity and reliability in quantitative research is not without its challenges. Some of the factors to consider include:
1. Measuring Complex Constructs or Variables One of the main challenges is the difficulty in accurately measuring complex constructs or variables. For instance, measuring constructs such as intelligence or personality can be complicated due to their multi-dimensional nature, and it can be challenging to capture all aspects accurately.
2. Limitations of Data Collection Instruments In addition, the measures or instruments used to collect data can also be limited in their sensitivity or specificity. This can impact the study’s validity and reliability, as accurate and precise measures can lead to incorrect conclusions and unreliable results. For example, a scale that measures depression but does not include all relevant symptoms may not accurately capture the construct being studied.
3. Sources of Error and Bias in Data Collection The data collection process itself can introduce sources of error or bias, which can impact the validity and reliability of the study. For instance, measurement errors can occur due to the limitations of the measuring instrument or human error during data collection. In addition, response bias can arise when participants provide socially desirable answers, while sampling bias can occur when the sample is not representative of the studied population.
4. The Complexity of Achieving Meaningful and Accurate Research Findings There are also some limitations to validity and reliability in research studies. For example, achieving internal validity by controlling for extraneous variables may only sometimes ensure external validity or the ability to generalize findings to other populations or settings. This can be a limitation for researchers who wish to apply their findings to a larger population or different contexts.
Additionally, while reliability is essential for producing consistent and reproducible results, it does not guarantee the accuracy or truth of the findings. This means that even if a study has reliable results, it may still need to be revised in terms of accuracy. These limitations remind us that research is a complex process, and achieving validity and reliability is just one part of the giant puzzle of producing accurate and meaningful research.
Researchers can adopt various measures and techniques to overcome the challenges and limitations in ensuring validity and reliability in research studies.
One such approach is to use multiple measures or instruments to assess the same construct. In addition, various steps can help identify commonalities and differences across measures, thereby providing a more comprehensive understanding of the construct being studied.
Inter-rater reliability checks can also be conducted to ensure different raters or observers consistently interpret and rate the same data. This can reduce measurement errors and improve the reliability of the results. Additionally, data-cleaning techniques can be used to identify and remove any outliers or errors in the data.
Finally, researchers can use appropriate statistical methods to assess the validity and reliability of their measures. For example, factor analysis identifies the underlying factors contributing to the construct being studied, while test-retest reliability helps evaluate the consistency of results over time. By adopting these measures and techniques, researchers can crease t their findings’ overall quality and usefulness.
The backbone of any quantitative research lies in the validity and reliability of the data collected. These factors ensure the data accurately reflects the intended research objectives and is consistent and reproducible. By carefully balancing the interrelationship between validity and reliability and using appropriate techniques to overcome challenges, researchers protect the credibility and impact of their work. This is essential in producing high-quality research that can withstand scrutiny and drive progress.
Are you seeking a reliable and valid way to collect, analyze, and report your quantitative data? Sago’s comprehensive quantitative solutions provide you the peace of mind to conduct research and draw meaningful conclusions.
Don’t Settle for Subpar Results
Work with a trusted quantitative research partner to deliver quantitative research you can count on. Book a consultation with our team to get started.

Crack the Code: Evolving Panel Expectations

Exploring Travel Trends and Behaviors for Summer 2024

The Deciders, June 24, 2024: Third-Party Georgia Voters

Summer 2024 Insights: The Compass to This Year’s Travel Choices

The Swing Voter Project, June 2024: North Carolina

Making the Case for Virtual Audiences: Unleashing Insights in a Creative Agency

Boost Efficiency with Quantitative Methods Designed for You

The Deciders, June 2024: Hispanic American voters in Arizona

OnDemand: Decoding Gen C: Mastering Engagement with a New Consumer Powerhouse
Take a deep dive into your favorite market research topics
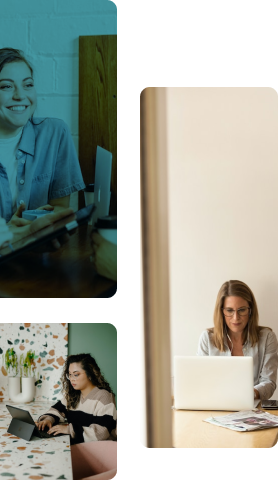
How can we help support you and your research needs?
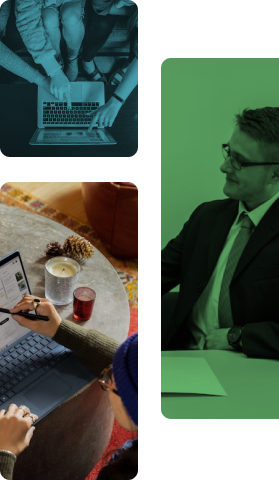
BEFORE YOU GO
Have you considered how to harness AI in your research process? Check out our on-demand webinar for everything you need to know

Have a language expert improve your writing
Run a free plagiarism check in 10 minutes, automatically generate references for free.
- Knowledge Base
- Methodology
- Reliability vs Validity in Research | Differences, Types & Examples
Reliability vs Validity in Research | Differences, Types & Examples
Published on 3 May 2022 by Fiona Middleton . Revised on 10 October 2022.
Reliability and validity are concepts used to evaluate the quality of research. They indicate how well a method , technique, or test measures something. Reliability is about the consistency of a measure, and validity is about the accuracy of a measure.
It’s important to consider reliability and validity when you are creating your research design , planning your methods, and writing up your results, especially in quantitative research .
Reliability | Validity | |
---|---|---|
What does it tell you? | The extent to which the results can be reproduced when the research is repeated under the same conditions. | The extent to which the results really measure what they are supposed to measure. |
How is it assessed? | By checking the consistency of results across time, across different observers, and across parts of the test itself. | By checking how well the results correspond to established theories and other measures of the same concept. |
How do they relate? | A reliable measurement is not always valid: the results might be reproducible, but they’re not necessarily correct. | A valid measurement is generally reliable: if a test produces accurate results, they should be . |
Table of contents
Understanding reliability vs validity, how are reliability and validity assessed, how to ensure validity and reliability in your research, where to write about reliability and validity in a thesis.
Reliability and validity are closely related, but they mean different things. A measurement can be reliable without being valid. However, if a measurement is valid, it is usually also reliable.
What is reliability?
Reliability refers to how consistently a method measures something. If the same result can be consistently achieved by using the same methods under the same circumstances, the measurement is considered reliable.
What is validity?
Validity refers to how accurately a method measures what it is intended to measure. If research has high validity, that means it produces results that correspond to real properties, characteristics, and variations in the physical or social world.
High reliability is one indicator that a measurement is valid. If a method is not reliable, it probably isn’t valid.
However, reliability on its own is not enough to ensure validity. Even if a test is reliable, it may not accurately reflect the real situation.
Validity is harder to assess than reliability, but it is even more important. To obtain useful results, the methods you use to collect your data must be valid: the research must be measuring what it claims to measure. This ensures that your discussion of the data and the conclusions you draw are also valid.
Prevent plagiarism, run a free check.
Reliability can be estimated by comparing different versions of the same measurement. Validity is harder to assess, but it can be estimated by comparing the results to other relevant data or theory. Methods of estimating reliability and validity are usually split up into different types.
Types of reliability
Different types of reliability can be estimated through various statistical methods.
Type of reliability | What does it assess? | Example |
---|---|---|
The consistency of a measure : do you get the same results when you repeat the measurement? | A group of participants complete a designed to measure personality traits. If they repeat the questionnaire days, weeks, or months apart and give the same answers, this indicates high test-retest reliability. | |
The consistency of a measure : do you get the same results when different people conduct the same measurement? | Based on an assessment criteria checklist, five examiners submit substantially different results for the same student project. This indicates that the assessment checklist has low inter-rater reliability (for example, because the criteria are too subjective). | |
The consistency of : do you get the same results from different parts of a test that are designed to measure the same thing? | You design a questionnaire to measure self-esteem. If you randomly split the results into two halves, there should be a between the two sets of results. If the two results are very different, this indicates low internal consistency. |
Types of validity
The validity of a measurement can be estimated based on three main types of evidence. Each type can be evaluated through expert judgement or statistical methods.
Type of validity | What does it assess? | Example |
---|---|---|
The adherence of a measure to of the concept being measured. | A self-esteem questionnaire could be assessed by measuring other traits known or assumed to be related to the concept of self-esteem (such as social skills and optimism). Strong correlation between the scores for self-esteem and associated traits would indicate high construct validity. | |
The extent to which the measurement of the concept being measured. | A test that aims to measure a class of students’ level of Spanish contains reading, writing, and speaking components, but no listening component. Experts agree that listening comprehension is an essential aspect of language ability, so the test lacks content validity for measuring the overall level of ability in Spanish. | |
The extent to which the result of a measure corresponds to of the same concept. | A is conducted to measure the political opinions of voters in a region. If the results accurately predict the later outcome of an election in that region, this indicates that the survey has high criterion validity. |
To assess the validity of a cause-and-effect relationship, you also need to consider internal validity (the design of the experiment ) and external validity (the generalisability of the results).
The reliability and validity of your results depends on creating a strong research design , choosing appropriate methods and samples, and conducting the research carefully and consistently.
Ensuring validity
If you use scores or ratings to measure variations in something (such as psychological traits, levels of ability, or physical properties), it’s important that your results reflect the real variations as accurately as possible. Validity should be considered in the very earliest stages of your research, when you decide how you will collect your data .
- Choose appropriate methods of measurement
Ensure that your method and measurement technique are of high quality and targeted to measure exactly what you want to know. They should be thoroughly researched and based on existing knowledge.
For example, to collect data on a personality trait, you could use a standardised questionnaire that is considered reliable and valid. If you develop your own questionnaire, it should be based on established theory or the findings of previous studies, and the questions should be carefully and precisely worded.
- Use appropriate sampling methods to select your subjects
To produce valid generalisable results, clearly define the population you are researching (e.g., people from a specific age range, geographical location, or profession). Ensure that you have enough participants and that they are representative of the population.
Ensuring reliability
Reliability should be considered throughout the data collection process. When you use a tool or technique to collect data, it’s important that the results are precise, stable, and reproducible.
- Apply your methods consistently
Plan your method carefully to make sure you carry out the same steps in the same way for each measurement. This is especially important if multiple researchers are involved.
For example, if you are conducting interviews or observations, clearly define how specific behaviours or responses will be counted, and make sure questions are phrased the same way each time.
- Standardise the conditions of your research
When you collect your data, keep the circumstances as consistent as possible to reduce the influence of external factors that might create variation in the results.
For example, in an experimental setup, make sure all participants are given the same information and tested under the same conditions.
It’s appropriate to discuss reliability and validity in various sections of your thesis or dissertation or research paper. Showing that you have taken them into account in planning your research and interpreting the results makes your work more credible and trustworthy.
Section | Discuss |
---|---|
What have other researchers done to devise and improve methods that are reliable and valid? | |
How did you plan your research to ensure reliability and validity of the measures used? This includes the chosen sample set and size, sample preparation, external conditions, and measuring techniques. | |
If you calculate reliability and validity, state these values alongside your main results. | |
This is the moment to talk about how reliable and valid your results actually were. Were they consistent, and did they reflect true values? If not, why not? | |
If reliability and validity were a big problem for your findings, it might be helpful to mention this here. |
Cite this Scribbr article
If you want to cite this source, you can copy and paste the citation or click the ‘Cite this Scribbr article’ button to automatically add the citation to our free Reference Generator.
Middleton, F. (2022, October 10). Reliability vs Validity in Research | Differences, Types & Examples. Scribbr. Retrieved 5 August 2024, from https://www.scribbr.co.uk/research-methods/reliability-or-validity/
Is this article helpful?
Fiona Middleton
Other students also liked, the 4 types of validity | types, definitions & examples, a quick guide to experimental design | 5 steps & examples, sampling methods | types, techniques, & examples.
- Skip to secondary menu
- Skip to main content
- Skip to primary sidebar
Statistics By Jim
Making statistics intuitive
Reliability vs Validity: Differences & Examples
By Jim Frost 1 Comment
Reliability and validity are criteria by which researchers assess measurement quality. Measuring a person or item involves assigning scores to represent an attribute. This process creates the data that we analyze. However, to provide meaningful research results, that data must be good. And not all data are good!

For data to be good enough to allow you to draw meaningful conclusions from a research study, they must be reliable and valid. What are the properties of good measurements? In a nutshell, reliability relates to the consistency of measures, and validity addresses whether the measurements are quantifying the correct attribute.
In this post, learn about reliability vs. validity, their relationship, and the various ways to assess them.
Learn more about Experimental Design: Definition, Types, and Examples .
Reliability
Reliability refers to the consistency of the measure. High reliability indicates that the measurement system produces similar results under the same conditions. If you measure the same item or person multiple times, you want to obtain comparable values. They are reproducible.
If you take measurements multiple times and obtain very different values, your data are unreliable. Numbers are meaningless if repeated measures do not produce similar values. What’s the correct value? No one knows! This inconsistency hampers your ability to draw conclusions and understand relationships.
Suppose you have a bathroom scale that displays very inconsistent results from one time to the next. It’s very unreliable. It would be hard to use your scale to determine your correct weight and to know whether you are losing weight.
Inadequate data collection procedures and low-quality or defective data collection tools can produce unreliable data. Additionally, some characteristics are more challenging to measure reliably. For example, the length of an object is concrete. On the other hand, a psychological construct, such as conscientiousness, depression, and self-esteem, can be trickier to measure reliably.
When assessing studies, evaluate data collection methodologies and consider whether any issues undermine their reliability.
Validity refers to whether the measurements reflect what they’re supposed to measure. This concept is a broader issue than reliability. Researchers need to consider whether they’re measuring what they think they’re measuring. Or do the measurements reflect something else? Does the instrument measure what it says it measures? It’s a question that addresses the appropriateness of the data rather than whether measurements are repeatable.
Validity is a smaller concern for tangible measurements like height and weight. You might have a biased bathroom scale if it tends to read too high or too low—but it still measures weight. Validity is a bigger concern in the social sciences, where you can measure elusive concepts such as positive outlook and self-esteem. If you’re assessing the psychological construct of conscientiousness, you need to confirm that the instrument poses questions that appraise this attribute rather than, say, obedience.
Reliability vs Validity
A measurement must be reliable first before it has a chance of being valid. After all, if you don’t obtain consistent measurements for the same object or person under similar conditions, it can’t be valid. If your scale displays a different weight every time you step on it, it’s unreliable, and it is also invalid.
So, having reliable measurements is the first step towards having valid measures. Validity is necessary for reliability, but it is insufficient by itself.
Suppose you have a reliable measurement. You step on your scale a few times in a short period, and it displays very similar weights. It’s reliable. But the weight might be incorrect.
Just because you can measure the same object multiple times and get consistent values, it does not necessarily indicate that the measurements reflect the desired characteristic.
How can you determine whether measurements are both valid and reliable? Assessing reliability vs. validity is the topic for the rest of this post!
Similar measurements for the same person/item under the same conditions. | Measurements reflect what they’re supposed to measure. | |
Stability of results across time, between observers, within the test. | Measures have appropriate relationships to theories, similar measures, and different measures. | |
Unreliable measurements typically cannot be valid. | Valid measurements are also reliable. |
How to Assess Reliability
Reliability relates to measurement consistency. To evaluate reliability, analysts assess consistency over time, within the measurement instrument, and between different observers. These types of consistency are also known as—test-retest, internal, and inter-rater reliability. Typically, appraising these forms of reliability involves taking multiple measures of the same person, object, or construct and assessing scatterplots and correlations of the measurements. Reliable measurements have high correlations because the scores are similar.
Test-Retest Reliability
Analysts often assume that measurements should be consistent across a short time. If you measure your height twice over a couple of days, you should obtain roughly the same measurements.
To assess test-retest reliability, the experimenters typically measure a group of participants on two occasions within a few days. Usually, you’ll evaluate the reliability of the repeated measures using scatterplots and correlation coefficients . You expect to see high correlations and tight lines on the scatterplot when the characteristic you measure is consistent over a short period, and you have a reliable measurement system.
This type of reliability establishes the degree to which a test can produce stable, consistent scores across time. However, in practice, measurement instruments are never entirely consistent.
Keep in mind that some characteristics should not be consistent across time. A good example is your mood, which can change from moment to moment. A test-retest assessment of mood is not likely to produce a high correlation even though it might be a useful measurement instrument.
Internal Reliability
This type of reliability assesses consistency across items within a single instrument. Researchers evaluate internal reliability when they’re using instruments such as a survey or personality inventories. In these instruments, multiple items relate to a single construct. Questions that measure the same characteristic should have a high correlation. People who indicate they are risk-takers should also note that they participate in dangerous activities. If items that supposedly measure the same underlying construct have a low correlation, they are not consistent with each other and might not measure the same thing.
Inter-Rater Reliability
This type of reliability assesses consistency across different observers, judges, or evaluators. When various observers produce similar measurements for the same item or person, their scores are highly correlated. Inter-rater reliability is essential when the subjectivity or skill of the evaluator plays a role. For example, assessing the quality of a writing sample involves subjectivity. Researchers can employ rating guidelines to reduce subjectivity. Comparing the scores from different evaluators for the same writing sample helps establish the measure’s reliability. Learn more about inter-rater reliability .
Related post : Interpreting Correlation
Cronbach’s Alpha
Cronbach’s alpha measures the internal consistency, or reliability, of a set of survey items. Use this statistic to help determine whether a collection of items consistently measures the same characteristic. Learn more about Cronbach’s Alpha .
Gage R&R Studies
These studies evaluation a measurement systems reliability and identifies sources of variation that can help you target improvement efforts effectively. Learn more about Gage R&R Studies .
How to Assess Validity
Validity is more difficult to evaluate than reliability. After all, with reliability, you only assess whether the measures are consistent across time, within the instrument, and between observers. On the other hand, evaluating validity involves determining whether the instrument measures the correct characteristic. This process frequently requires examining relationships between these measurements, other data, and theory. Validating a measurement instrument requires you to use a wide range of subject-area knowledge and different types of constructs to determine whether the measurements from your instrument fit in with the bigger picture!
An instrument with high validity produces measurements that correctly fit the larger picture with other constructs. Validity assesses whether the web of empirical relationships aligns with the theoretical relationships.
The measurements must have a positive relationship with other measures of the same construct. Additionally, they need to correlate in the correct direction (positively or negatively) with the theoretically correct constructs. Finally, the measures should have no relationship with unrelated constructs.
If you need more detailed information, read my post that focuses on Measurement Validity . In that post, I cover the various types, how to evaluate them, and provide examples.
Experimental validity relates to experimental designs and methods. To learn about that topic, read my post about Internal and External Validity .
Whew, that’s a lot of information about reliability vs. validity. Using these concepts, you can determine whether a measurement instrument produces good data!
Share this:

Reader Interactions
August 17, 2022 at 3:53 am
Good way of expressing what validity and reliabiliy with building examples.
Comments and Questions Cancel reply

Validity vs. Reliability in Research: What's the Difference?

Introduction
What is the difference between reliability and validity in a study, what is an example of reliability and validity, how to ensure validity and reliability in your research, critiques of reliability and validity.
In research, validity and reliability are crucial for producing robust findings. They provide a foundation that assures scholars, practitioners, and readers alike that the research's insights are both accurate and consistent. However, the nuanced nature of qualitative data often blurs the lines between these concepts, making it imperative for researchers to discern their distinct roles.
This article seeks to illuminate the intricacies of reliability and validity, highlighting their significance and distinguishing their unique attributes. By understanding these critical facets, qualitative researchers can ensure their work not only resonates with authenticity but also trustworthiness.
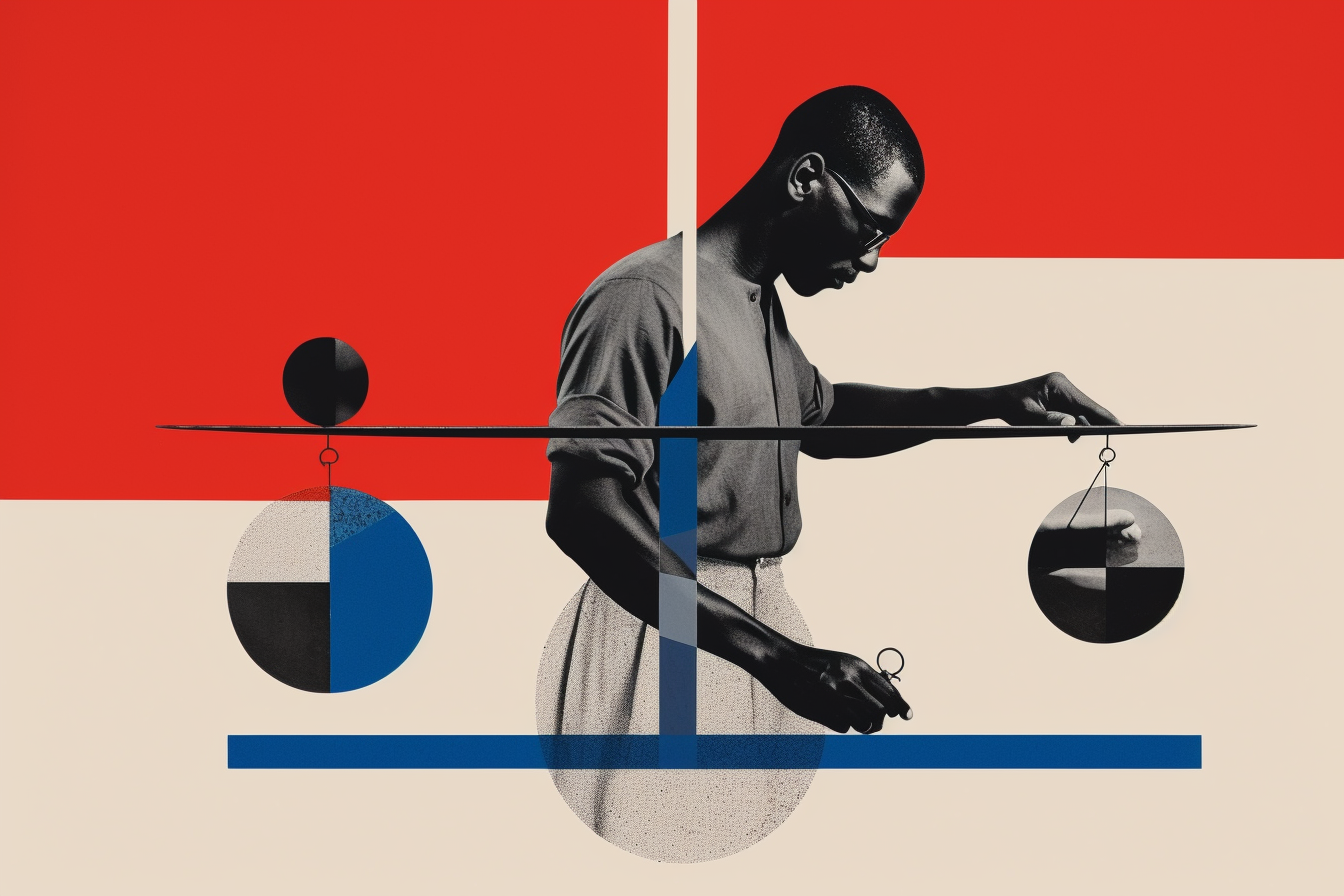
In the domain of research, whether qualitative or quantitative , two concepts often arise when discussing the quality and rigor of a study: reliability and validity . These two terms, while interconnected, have distinct meanings that hold significant weight in the world of research.
Reliability, at its core, speaks to the consistency of a study. If a study or test measures the same concept repeatedly and yields the same results, it demonstrates a high degree of reliability. A common method for assessing reliability is through internal consistency reliability, which checks if multiple items that measure the same concept produce similar scores.
Another method often used is inter-rater reliability , which gauges the consistency of scores given by different raters. This approach is especially amenable to qualitative research , and it can help researchers assess the clarity of their code system and the consistency of their codings . For a study to be more dependable, it's imperative to ensure a sufficient measurement of reliability is achieved.
On the other hand, validity is concerned with accuracy. It looks at whether a study truly measures what it claims to. Within the realm of validity, several types exist. Construct validity, for instance, verifies that a study measures the intended abstract concept or underlying construct. If a research aims to measure self-esteem and accurately captures this abstract trait, it demonstrates strong construct validity.
Content validity ensures that a test or study comprehensively represents the entire domain of the concept it seeks to measure. For instance, if a test aims to assess mathematical ability, it should cover arithmetic, algebra, geometry, and more to showcase strong content validity.
Criterion validity is another form of validity that ensures that the scores from a test correlate well with a measure from a related outcome. A subset of this is predictive validity, which checks if the test can predict future outcomes. For instance, if an aptitude test can predict future job performance, it can be said to have high predictive validity.
The distinction between reliability and validity becomes clear when one considers the nature of their focus. While reliability is concerned with consistency and reproducibility, validity zeroes in on accuracy and truthfulness.
A research tool can be reliable without being valid. For instance, faulty instrument measures might consistently give bad readings (reliable but not valid). Conversely, in discussions about test reliability, the same test measure administered multiple times could sometimes hit the mark and at other times miss it entirely, producing different test scores each time. This would make it valid in some instances but not reliable.
For a study to be robust, it must achieve both reliability and validity. Reliability ensures the study's findings are reproducible while validity confirms that it accurately represents the phenomena it claims to. Ensuring both in a study means the results are both dependable and accurate, forming a cornerstone for high-quality research.
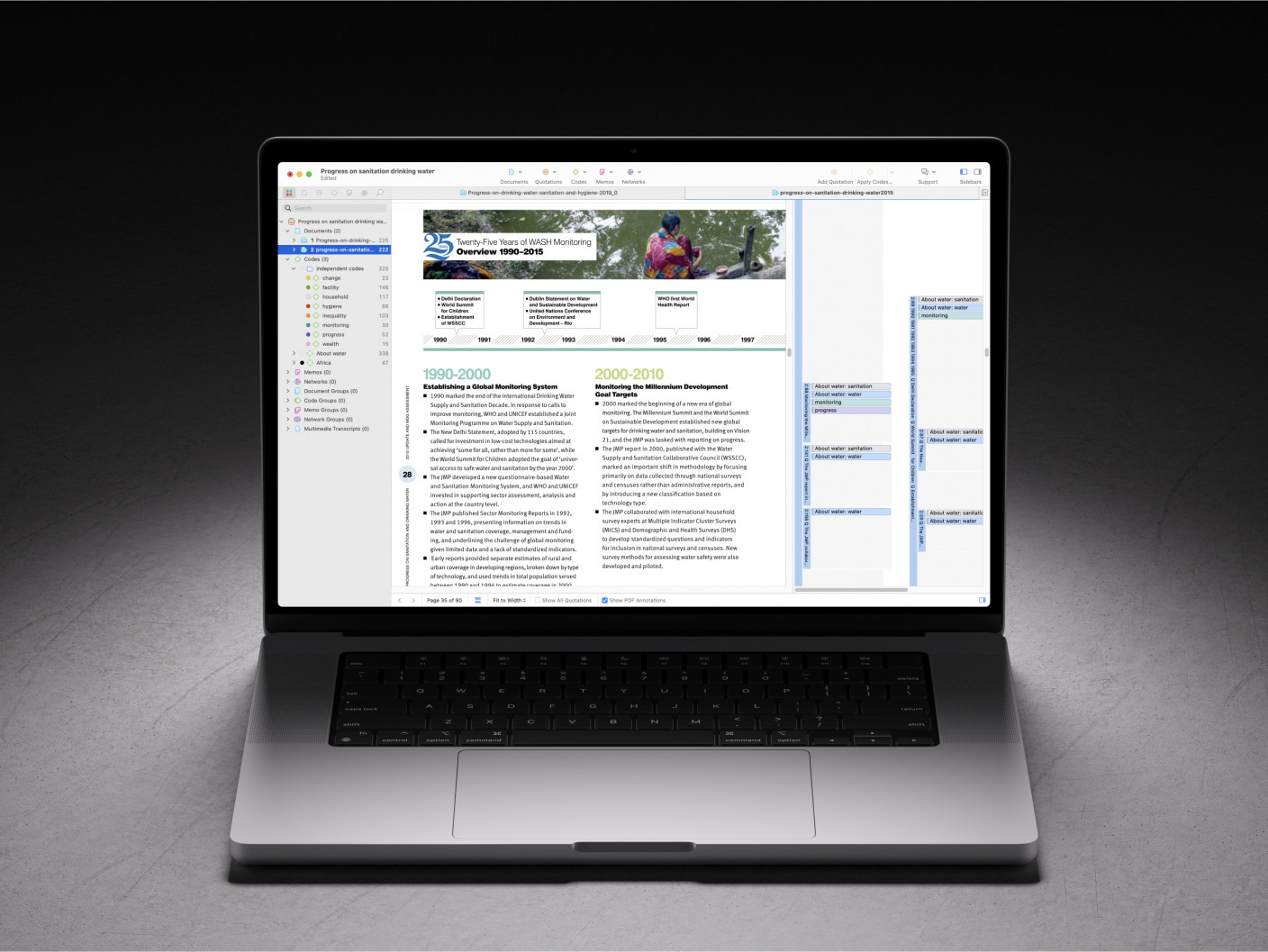
Efficient, easy data analysis with ATLAS.ti
Start analyzing data quickly and more deeply with ATLAS.ti. Download a free trial today.
Understanding the nuances of reliability and validity becomes clearer when contextualized within a real-world research setting. Imagine a qualitative study where a researcher aims to explore the experiences of teachers in urban schools concerning classroom management. The primary method of data collection is semi-structured interviews .
To ensure the reliability of this qualitative study, the researcher crafts a consistent list of open-ended questions for the interview. This ensures that, while each conversation might meander based on the individual’s experiences, there remains a core set of topics related to classroom management that every participant addresses.
The essence of reliability in this context isn't necessarily about garnering identical responses but rather about achieving a consistent approach to data collection and subsequent interpretation . As part of this commitment to reliability, two researchers might independently transcribe and analyze a subset of these interviews. If they identify similar themes and patterns in their independent analyses, it suggests a consistent interpretation of the data, showcasing inter-rater reliability .
Validity , on the other hand, is anchored in ensuring that the research genuinely captures and represents the lived experiences and sentiments of teachers concerning classroom management. To establish content validity, the list of interview questions is thoroughly reviewed by a panel of educational experts. Their feedback ensures that the questions encompass the breadth of issues and concerns related to classroom management in urban school settings.
As the interviews are conducted, the researcher pays close attention to the depth and authenticity of responses. After the interviews, member checking could be employed, where participants review the researcher's interpretation of their responses to ensure that their experiences and perspectives have been accurately captured. This strategy helps in affirming the study's construct validity, ensuring that the abstract concept of "experiences with classroom management" has been truthfully and adequately represented.
In this example, we can see that while the interview study is rooted in qualitative methods and subjective experiences, the principles of reliability and validity can still meaningfully inform the research process. They serve as guides to ensure the research's findings are both dependable and genuinely reflective of the participants' experiences.
Ensuring validity and reliability in research, irrespective of its qualitative or quantitative nature, is pivotal to producing results that are both trustworthy and robust. Here's how you can integrate these concepts into your study to ensure its rigor:
Reliability is about consistency. One of the most straightforward ways to gauge it in quantitative research is using test-retest reliability. It involves administering the same test to the same group of participants on two separate occasions and then comparing the results.
A high degree of similarity between the two sets of results indicates good reliability. This can often be measured using a correlation coefficient, where a value closer to 1 indicates a strong positive consistency between the two test iterations.
Validity, on the other hand, ensures that the research genuinely measures what it intends to. There are various forms of validity to consider. Convergent validity ensures that two measures of the same construct or those that should theoretically be related, are indeed correlated. For example, two different measures assessing self-esteem should show similar results for the same group, highlighting that they are measuring the same underlying construct.
Face validity is the most basic form of validity and is gauged by the sheer appearance of the measurement tool. If, at face value, a test seems like it measures what it claims to, it has face validity. This is often the first step and is usually followed by more rigorous forms of validity testing.
Criterion-related validity, a subtype of the previously discussed criterion validity, evaluates how well the outcomes of a particular test or measurement correlate with another related measure. For example, if a new tool is developed to measure reading comprehension, its results can be compared with those of an established reading comprehension test to assess its criterion-related validity. If the results show a strong correlation, it's a sign that the new tool is valid.
Ensuring both validity and reliability requires deliberate planning, meticulous testing, and constant reflection on the study's methods and results. This might involve using established scales or measures with proven validity and reliability, conducting pilot studies to refine measurement tools, and always staying cognizant of the fact that these two concepts are important considerations for research robustness.
While reliability and validity are foundational concepts in many traditional research paradigms, they have not escaped scrutiny, especially from critical and poststructuralist perspectives. These critiques often arise from the fundamental philosophical differences in how knowledge, truth, and reality are perceived and constructed.
From a poststructuralist viewpoint, the very pursuit of a singular "truth" or an objective reality is questionable. In such a perspective, multiple truths exist, each shaped by its own socio-cultural, historical, and individual contexts.
Reliability, with its emphasis on consistent replication, might then seem at odds with this understanding. If truths are multiple and shifting, how can consistency across repeated measures or observations be a valid measure of anything other than the research instrument's stability?
Validity, too, faces critique. In seeking to ensure that a study measures what it purports to measure, there's an implicit assumption of an observable, knowable reality. Poststructuralist critiques question this foundation, arguing that reality is too fluid, multifaceted, and influenced by power dynamics to be pinned down by any singular measurement or representation.
Moreover, the very act of determining "validity" often requires an external benchmark or "gold standard." This brings up the issue of who determines this standard and the power dynamics and potential biases inherent in such decisions.
Another point of contention is the way these concepts can inadvertently prioritize certain forms of knowledge over others. For instance, privileging research that meets stringent reliability and validity criteria might marginalize more exploratory, interpretive, or indigenous research methods. These methods, while offering deep insights, might not align neatly with traditional understandings of reliability and validity, potentially relegating them to the periphery of "accepted" knowledge production.
To be sure, reliability and validity serve as guiding principles in many research approaches. However, it's essential to recognize their limitations and the critiques posed by alternative epistemologies. Engaging with these critiques doesn't diminish the value of reliability and validity but rather enriches our understanding of the multifaceted nature of knowledge and the complexities of its pursuit.
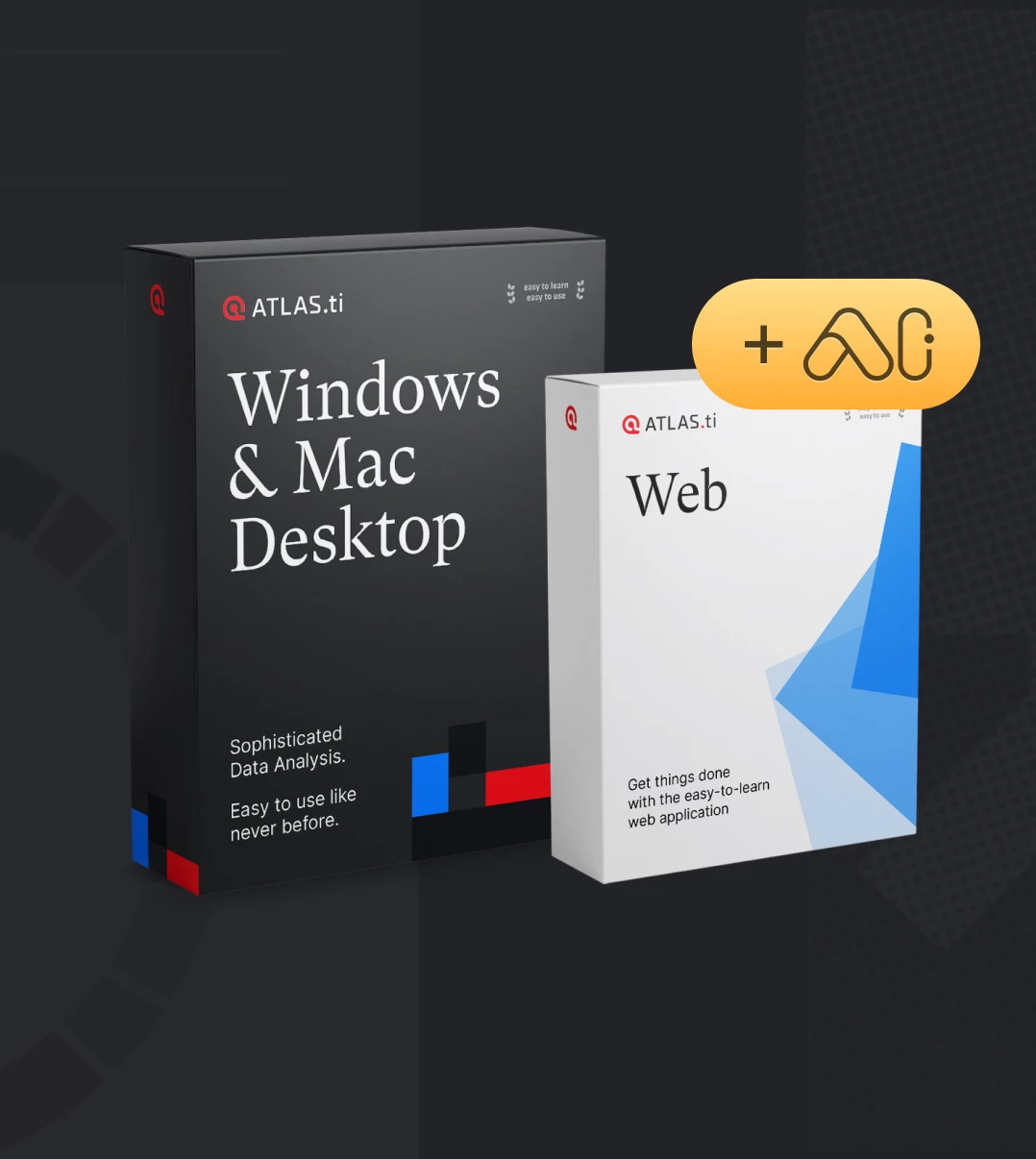
A rigorous research process begins with ATLAS.ti
Download a free trial of our powerful data analysis software to make the most of your research.
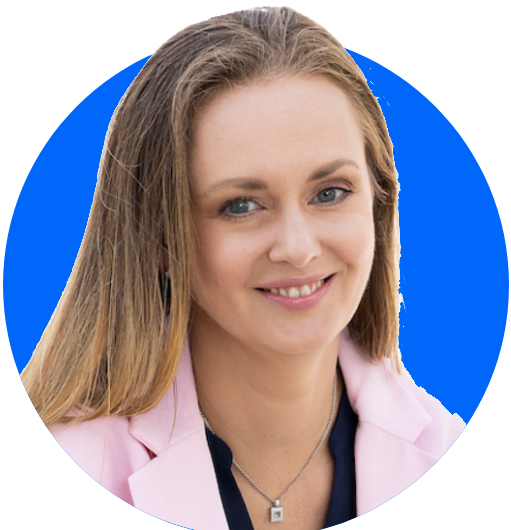
Have a language expert improve your writing
Run a free plagiarism check in 10 minutes, generate accurate citations for free.
- Knowledge Base
Methodology
- The 4 Types of Reliability in Research | Definitions & Examples
The 4 Types of Reliability in Research | Definitions & Examples
Published on August 8, 2019 by Fiona Middleton . Revised on June 22, 2023.
Reliability tells you how consistently a method measures something. When you apply the same method to the same sample under the same conditions, you should get the same results. If not, the method of measurement may be unreliable or bias may have crept into your research.
There are four main types of reliability. Each can be estimated by comparing different sets of results produced by the same method.
Type of reliability | Measures the consistency of… |
---|---|
The same test over . | |
The same test conducted by different . | |
of a test which are designed to be equivalent. | |
The of a test. | |
Table of contents
Test-retest reliability, interrater reliability, parallel forms reliability, internal consistency, which type of reliability applies to my research, other interesting articles, frequently asked questions about types of reliability.
Test-retest reliability measures the consistency of results when you repeat the same test on the same sample at a different point in time. You use it when you are measuring something that you expect to stay constant in your sample.
Why it’s important
Many factors can influence your results at different points in time: for example, respondents might experience different moods, or external conditions might affect their ability to respond accurately.
Test-retest reliability can be used to assess how well a method resists these factors over time. The smaller the difference between the two sets of results, the higher the test-retest reliability.
How to measure it
To measure test-retest reliability, you conduct the same test on the same group of people at two different points in time. Then you calculate the correlation between the two sets of results.
Test-retest reliability example
You devise a questionnaire to measure the IQ of a group of participants (a property that is unlikely to change significantly over time).You administer the test two months apart to the same group of people, but the results are significantly different, so the test-retest reliability of the IQ questionnaire is low.
Improving test-retest reliability
- When designing tests or questionnaires , try to formulate questions, statements, and tasks in a way that won’t be influenced by the mood or concentration of participants.
- When planning your methods of data collection , try to minimize the influence of external factors, and make sure all samples are tested under the same conditions.
- Remember that changes or recall bias can be expected to occur in the participants over time, and take these into account.
Receive feedback on language, structure, and formatting
Professional editors proofread and edit your paper by focusing on:
- Academic style
- Vague sentences
- Style consistency
See an example
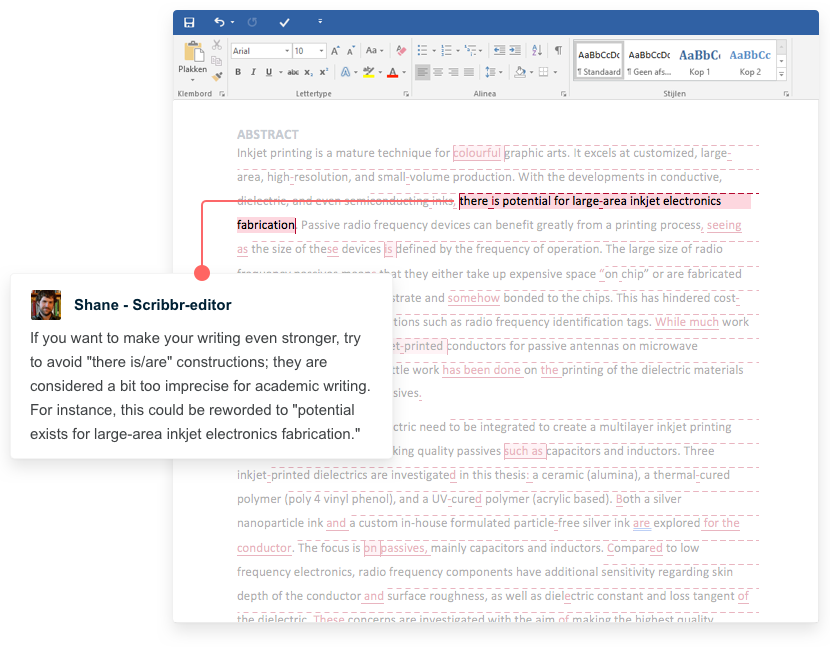
Interrater reliability (also called interobserver reliability) measures the degree of agreement between different people observing or assessing the same thing. You use it when data is collected by researchers assigning ratings, scores or categories to one or more variables , and it can help mitigate observer bias .
People are subjective, so different observers’ perceptions of situations and phenomena naturally differ. Reliable research aims to minimize subjectivity as much as possible so that a different researcher could replicate the same results.
When designing the scale and criteria for data collection, it’s important to make sure that different people will rate the same variable consistently with minimal bias . This is especially important when there are multiple researchers involved in data collection or analysis.
To measure interrater reliability, different researchers conduct the same measurement or observation on the same sample. Then you calculate the correlation between their different sets of results. If all the researchers give similar ratings, the test has high interrater reliability.
Interrater reliability example
A team of researchers observe the progress of wound healing in patients. To record the stages of healing, rating scales are used, with a set of criteria to assess various aspects of wounds. The results of different researchers assessing the same set of patients are compared, and there is a strong correlation between all sets of results, so the test has high interrater reliability.
Improving interrater reliability
- Clearly define your variables and the methods that will be used to measure them.
- Develop detailed, objective criteria for how the variables will be rated, counted or categorized.
- If multiple researchers are involved, ensure that they all have exactly the same information and training.
Parallel forms reliability measures the correlation between two equivalent versions of a test. You use it when you have two different assessment tools or sets of questions designed to measure the same thing.
If you want to use multiple different versions of a test (for example, to avoid respondents repeating the same answers from memory), you first need to make sure that all the sets of questions or measurements give reliable results.
The most common way to measure parallel forms reliability is to produce a large set of questions to evaluate the same thing, then divide these randomly into two question sets.
The same group of respondents answers both sets, and you calculate the correlation between the results. High correlation between the two indicates high parallel forms reliability.
Parallel forms reliability example
A set of questions is formulated to measure financial risk aversion in a group of respondents. The questions are randomly divided into two sets, and the respondents are randomly divided into two groups. Both groups take both tests: group A takes test A first, and group B takes test B first. The results of the two tests are compared, and the results are almost identical, indicating high parallel forms reliability.
Improving parallel forms reliability
- Ensure that all questions or test items are based on the same theory and formulated to measure the same thing.
Internal consistency assesses the correlation between multiple items in a test that are intended to measure the same construct.
You can calculate internal consistency without repeating the test or involving other researchers, so it’s a good way of assessing reliability when you only have one data set.
When you devise a set of questions or ratings that will be combined into an overall score, you have to make sure that all of the items really do reflect the same thing. If responses to different items contradict one another, the test might be unreliable.
Two common methods are used to measure internal consistency.
- Average inter-item correlation : For a set of measures designed to assess the same construct, you calculate the correlation between the results of all possible pairs of items and then calculate the average.
- Split-half reliability : You randomly split a set of measures into two sets. After testing the entire set on the respondents, you calculate the correlation between the two sets of responses.
Internal consistency example
A group of respondents are presented with a set of statements designed to measure optimistic and pessimistic mindsets. They must rate their agreement with each statement on a scale from 1 to 5. If the test is internally consistent, an optimistic respondent should generally give high ratings to optimism indicators and low ratings to pessimism indicators. The correlation is calculated between all the responses to the “optimistic” statements, but the correlation is very weak. This suggests that the test has low internal consistency.
Improving internal consistency
- Take care when devising questions or measures: those intended to reflect the same concept should be based on the same theory and carefully formulated.
Prevent plagiarism. Run a free check.
It’s important to consider reliability when planning your research design , collecting and analyzing your data, and writing up your research. The type of reliability you should calculate depends on the type of research and your methodology .
What is my methodology? | Which form of reliability is relevant? |
---|---|
Measuring a property that you expect to stay the same over time. | Test-retest |
Multiple researchers making observations or ratings about the same topic. | Interrater |
Using two different tests to measure the same thing. | Parallel forms |
Using a multi-item test where all the items are intended to measure the same variable. | Internal consistency |
If possible and relevant, you should statistically calculate reliability and state this alongside your results .
If you want to know more about statistics , methodology , or research bias , make sure to check out some of our other articles with explanations and examples.
- Normal distribution
- Degrees of freedom
- Null hypothesis
- Discourse analysis
- Control groups
- Mixed methods research
- Non-probability sampling
- Quantitative research
- Ecological validity
Research bias
- Rosenthal effect
- Implicit bias
- Cognitive bias
- Selection bias
- Negativity bias
- Status quo bias
Reliability and validity are both about how well a method measures something:
- Reliability refers to the consistency of a measure (whether the results can be reproduced under the same conditions).
- Validity refers to the accuracy of a measure (whether the results really do represent what they are supposed to measure).
If you are doing experimental research, you also have to consider the internal and external validity of your experiment.
You can use several tactics to minimize observer bias .
- Use masking (blinding) to hide the purpose of your study from all observers.
- Triangulate your data with different data collection methods or sources.
- Use multiple observers and ensure interrater reliability.
- Train your observers to make sure data is consistently recorded between them.
- Standardize your observation procedures to make sure they are structured and clear.
Reproducibility and replicability are related terms.
- A successful reproduction shows that the data analyses were conducted in a fair and honest manner.
- A successful replication shows that the reliability of the results is high.
Research bias affects the validity and reliability of your research findings , leading to false conclusions and a misinterpretation of the truth. This can have serious implications in areas like medical research where, for example, a new form of treatment may be evaluated.
Cite this Scribbr article
If you want to cite this source, you can copy and paste the citation or click the “Cite this Scribbr article” button to automatically add the citation to our free Citation Generator.
Middleton, F. (2023, June 22). The 4 Types of Reliability in Research | Definitions & Examples. Scribbr. Retrieved August 5, 2024, from https://www.scribbr.com/methodology/types-of-reliability/
Is this article helpful?
Fiona Middleton
Other students also liked, reliability vs. validity in research | difference, types and examples, what is quantitative research | definition, uses & methods, data collection | definition, methods & examples, what is your plagiarism score.
- How it works
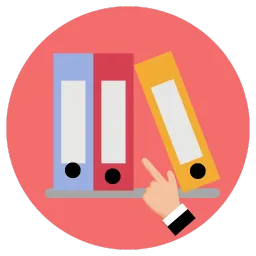
Reliability and Validity – Definitions, Types & Examples
Published by Alvin Nicolas at August 16th, 2021 , Revised On October 26, 2023
A researcher must test the collected data before making any conclusion. Every research design needs to be concerned with reliability and validity to measure the quality of the research.
What is Reliability?
Reliability refers to the consistency of the measurement. Reliability shows how trustworthy is the score of the test. If the collected data shows the same results after being tested using various methods and sample groups, the information is reliable. If your method has reliability, the results will be valid.
Example: If you weigh yourself on a weighing scale throughout the day, you’ll get the same results. These are considered reliable results obtained through repeated measures.
Example: If a teacher conducts the same math test of students and repeats it next week with the same questions. If she gets the same score, then the reliability of the test is high.
What is the Validity?
Validity refers to the accuracy of the measurement. Validity shows how a specific test is suitable for a particular situation. If the results are accurate according to the researcher’s situation, explanation, and prediction, then the research is valid.
If the method of measuring is accurate, then it’ll produce accurate results. If a method is reliable, then it’s valid. In contrast, if a method is not reliable, it’s not valid.
Example: Your weighing scale shows different results each time you weigh yourself within a day even after handling it carefully, and weighing before and after meals. Your weighing machine might be malfunctioning. It means your method had low reliability. Hence you are getting inaccurate or inconsistent results that are not valid.
Example: Suppose a questionnaire is distributed among a group of people to check the quality of a skincare product and repeated the same questionnaire with many groups. If you get the same response from various participants, it means the validity of the questionnaire and product is high as it has high reliability.
Most of the time, validity is difficult to measure even though the process of measurement is reliable. It isn’t easy to interpret the real situation.
Example: If the weighing scale shows the same result, let’s say 70 kg each time, even if your actual weight is 55 kg, then it means the weighing scale is malfunctioning. However, it was showing consistent results, but it cannot be considered as reliable. It means the method has low reliability.
Internal Vs. External Validity
One of the key features of randomised designs is that they have significantly high internal and external validity.
Internal validity is the ability to draw a causal link between your treatment and the dependent variable of interest. It means the observed changes should be due to the experiment conducted, and any external factor should not influence the variables .
Example: age, level, height, and grade.
External validity is the ability to identify and generalise your study outcomes to the population at large. The relationship between the study’s situation and the situations outside the study is considered external validity.
Also, read about Inductive vs Deductive reasoning in this article.
Looking for reliable dissertation support?
We hear you.
- Whether you want a full dissertation written or need help forming a dissertation proposal, we can help you with both.
- Get different dissertation services at ResearchProspect and score amazing grades!
Threats to Interval Validity
Threat | Definition | Example |
---|---|---|
Confounding factors | Unexpected events during the experiment that are not a part of treatment. | If you feel the increased weight of your experiment participants is due to lack of physical activity, but it was actually due to the consumption of coffee with sugar. |
Maturation | The influence on the independent variable due to passage of time. | During a long-term experiment, subjects may feel tired, bored, and hungry. |
Testing | The results of one test affect the results of another test. | Participants of the first experiment may react differently during the second experiment. |
Instrumentation | Changes in the instrument’s collaboration | Change in the may give different results instead of the expected results. |
Statistical regression | Groups selected depending on the extreme scores are not as extreme on subsequent testing. | Students who failed in the pre-final exam are likely to get passed in the final exams; they might be more confident and conscious than earlier. |
Selection bias | Choosing comparison groups without randomisation. | A group of trained and efficient teachers is selected to teach children communication skills instead of randomly selecting them. |
Experimental mortality | Due to the extension of the time of the experiment, participants may leave the experiment. | Due to multi-tasking and various competition levels, the participants may leave the competition because they are dissatisfied with the time-extension even if they were doing well. |
Threats of External Validity
Threat | Definition | Example |
---|---|---|
Reactive/interactive effects of testing | The participants of the pre-test may get awareness about the next experiment. The treatment may not be effective without the pre-test. | Students who got failed in the pre-final exam are likely to get passed in the final exams; they might be more confident and conscious than earlier. |
Selection of participants | A group of participants selected with specific characteristics and the treatment of the experiment may work only on the participants possessing those characteristics | If an experiment is conducted specifically on the health issues of pregnant women, the same treatment cannot be given to male participants. |
How to Assess Reliability and Validity?
Reliability can be measured by comparing the consistency of the procedure and its results. There are various methods to measure validity and reliability. Reliability can be measured through various statistical methods depending on the types of validity, as explained below:
Types of Reliability
Type of reliability | What does it measure? | Example |
---|---|---|
Test-Retests | It measures the consistency of the results at different points of time. It identifies whether the results are the same after repeated measures. | Suppose a questionnaire is distributed among a group of people to check the quality of a skincare product and repeated the same questionnaire with many groups. If you get the same response from a various group of participants, it means the validity of the questionnaire and product is high as it has high test-retest reliability. |
Inter-Rater | It measures the consistency of the results at the same time by different raters (researchers) | Suppose five researchers measure the academic performance of the same student by incorporating various questions from all the academic subjects and submit various results. It shows that the questionnaire has low inter-rater reliability. |
Parallel Forms | It measures Equivalence. It includes different forms of the same test performed on the same participants. | Suppose the same researcher conducts the two different forms of tests on the same topic and the same students. The tests could be written and oral tests on the same topic. If results are the same, then the parallel-forms reliability of the test is high; otherwise, it’ll be low if the results are different. |
Inter-Term | It measures the consistency of the measurement. | The results of the same tests are split into two halves and compared with each other. If there is a lot of difference in results, then the inter-term reliability of the test is low. |
Types of Validity
As we discussed above, the reliability of the measurement alone cannot determine its validity. Validity is difficult to be measured even if the method is reliable. The following type of tests is conducted for measuring validity.
Type of reliability | What does it measure? | Example |
---|---|---|
Content validity | It shows whether all the aspects of the test/measurement are covered. | A language test is designed to measure the writing and reading skills, listening, and speaking skills. It indicates that a test has high content validity. |
Face validity | It is about the validity of the appearance of a test or procedure of the test. | The type of included in the question paper, time, and marks allotted. The number of questions and their categories. Is it a good question paper to measure the academic performance of students? |
Construct validity | It shows whether the test is measuring the correct construct (ability/attribute, trait, skill) | Is the test conducted to measure communication skills is actually measuring communication skills? |
Criterion validity | It shows whether the test scores obtained are similar to other measures of the same concept. | The results obtained from a prefinal exam of graduate accurately predict the results of the later final exam. It shows that the test has high criterion validity. |
Does your Research Methodology Have the Following?
- Great Research/Sources
- Perfect Language
- Accurate Sources
If not, we can help. Our panel of experts makes sure to keep the 3 pillars of Research Methodology strong.
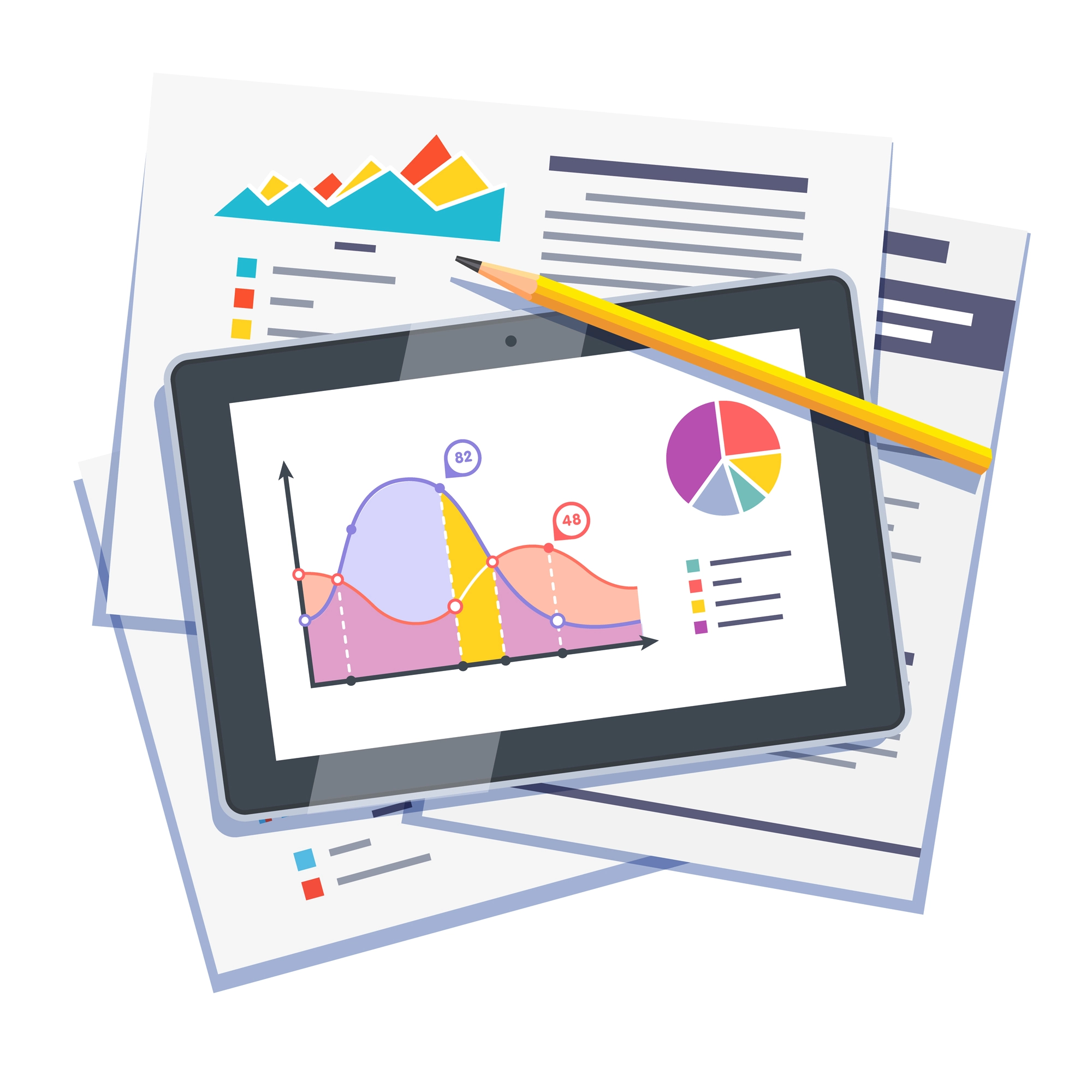
How to Increase Reliability?
- Use an appropriate questionnaire to measure the competency level.
- Ensure a consistent environment for participants
- Make the participants familiar with the criteria of assessment.
- Train the participants appropriately.
- Analyse the research items regularly to avoid poor performance.
How to Increase Validity?
Ensuring Validity is also not an easy job. A proper functioning method to ensure validity is given below:
- The reactivity should be minimised at the first concern.
- The Hawthorne effect should be reduced.
- The respondents should be motivated.
- The intervals between the pre-test and post-test should not be lengthy.
- Dropout rates should be avoided.
- The inter-rater reliability should be ensured.
- Control and experimental groups should be matched with each other.
How to Implement Reliability and Validity in your Thesis?
According to the experts, it is helpful if to implement the concept of reliability and Validity. Especially, in the thesis and the dissertation, these concepts are adopted much. The method for implementation given below:
Segments | Explanation | |||||||||||||||||||
---|---|---|---|---|---|---|---|---|---|---|---|---|---|---|---|---|---|---|---|---|
All the planning about reliability and validity will be discussed here, including the chosen samples and size and the techniques used to measure reliability and validity. | ||||||||||||||||||||
Please talk about the level of reliability and validity of your results and their influence on values. | ||||||||||||||||||||
Frequently Asked QuestionsWhat is reliability and validity in research. Reliability in research refers to the consistency and stability of measurements or findings. Validity relates to the accuracy and truthfulness of results, measuring what the study intends to. Both are crucial for trustworthy and credible research outcomes. What is validity?Validity in research refers to the extent to which a study accurately measures what it intends to measure. It ensures that the results are truly representative of the phenomena under investigation. Without validity, research findings may be irrelevant, misleading, or incorrect, limiting their applicability and credibility. What is reliability?Reliability in research refers to the consistency and stability of measurements over time. If a study is reliable, repeating the experiment or test under the same conditions should produce similar results. Without reliability, findings become unpredictable and lack dependability, potentially undermining the study’s credibility and generalisability. ![]() What is reliability in psychology?In psychology, reliability refers to the consistency of a measurement tool or test. A reliable psychological assessment produces stable and consistent results across different times, situations, or raters. It ensures that an instrument’s scores are not due to random error, making the findings dependable and reproducible in similar conditions. What is test retest reliability?Test-retest reliability assesses the consistency of measurements taken by a test over time. It involves administering the same test to the same participants at two different points in time and comparing the results. A high correlation between the scores indicates that the test produces stable and consistent results over time. How to improve reliability of an experiment?
What is the difference between reliability and validity?Reliability refers to the consistency and repeatability of measurements, ensuring results are stable over time. Validity indicates how well an instrument measures what it’s intended to measure, ensuring accuracy and relevance. While a test can be reliable without being valid, a valid test must inherently be reliable. Both are essential for credible research. Are interviews reliable and valid?Interviews can be both reliable and valid, but they are susceptible to biases. The reliability and validity depend on the design, structure, and execution of the interview. Structured interviews with standardised questions improve reliability. Validity is enhanced when questions accurately capture the intended construct and when interviewer biases are minimised. Are IQ tests valid and reliable?IQ tests are generally considered reliable, producing consistent scores over time. Their validity, however, is a subject of debate. While they effectively measure certain cognitive skills, whether they capture the entirety of “intelligence” or predict success in all life areas is contested. Cultural bias and over-reliance on tests are also concerns. Are questionnaires reliable and valid?Questionnaires can be both reliable and valid if well-designed. Reliability is achieved when they produce consistent results over time or across similar populations. Validity is ensured when questions accurately measure the intended construct. However, factors like poorly phrased questions, respondent bias, and lack of standardisation can compromise their reliability and validity. You May Also LikeDiscourse analysis is an essential aspect of studying a language. It is used in various disciplines of social science and humanities such as linguistic, sociolinguistics, and psycholinguistic. Inductive and deductive reasoning takes into account assumptions and incidents. Here is all you need to know about inductive vs deductive reasoning. A survey includes questions relevant to the research topic. The participants are selected, and the questionnaire is distributed to collect the data. USEFUL LINKS LEARNING RESOURCES ![]() COMPANY DETAILS ![]()
Breadcrumbs Section. Click here to navigate to respective pages. ![]() Validity and Reliability DOI link for Validity and Reliability Click here to navigate to parent product. This chapter provides an in-depth look into the concepts of reliability and validity. The inherent lack of total reliability in planning research gets further explained by specific challenges. Several different ways to measure reliability—inter-rater reliability, equivalency reliability, and internal consistency—and validity—face, construct, internal, and external validities—are presented. The chapter concludes with two examples of planning research studies that exemplify the concepts of reliability and validity.
Connect with us Registered in England & Wales No. 3099067 5 Howick Place | London | SW1P 1WG © 2024 Informa UK Limited
![]() Home » Reliability Vs Validity Reliability Vs ValidityTable of Contents ![]() Reliability and validity are two important concepts in research that are used to evaluate the quality of measurement instruments or research studies. ReliabilityReliability refers to the degree to which a measurement instrument or research study produces consistent and stable results over time, across different observers or raters, or under different conditions. In other words, reliability is the extent to which a measurement instrument or research study produces results that are free from random error. A reliable measurement instrument or research study should produce similar results each time it is used or conducted, regardless of who is using it or conducting it. Validity, on the other hand, refers to the degree to which a measurement instrument or research study accurately measures what it is supposed to measure or tests what it is supposed to test. In other words, validity is the extent to which a measurement instrument or research study measures or tests what it claims to measure or test. A valid measurement instrument or research study should produce results that accurately reflect the concept or construct being measured or tested. Difference Between Reliability Vs ValidityHere’s a comparison table that highlights the differences between reliability and validity:
Also see Research Methods About the authorMuhammad HassanResearcher, Academic Writer, Web developer You may also like![]() Primary Vs Secondary Research![]() Research Hypothesis Vs Null Hypothesis![]() Market Research Vs Marketing Research![]() Generative Vs Evaluative Research![]() Criterion Validity – Methods, Examples and...![]() Descriptive vs Inferential Statistics – All Key...![]() An official website of the United States government The .gov means it’s official. Federal government websites often end in .gov or .mil. Before sharing sensitive information, make sure you’re on a federal government site. The site is secure. The https:// ensures that you are connecting to the official website and that any information you provide is encrypted and transmitted securely.
Save citation to fileEmail citation, add to collections.
Add to My BibliographyYour saved search, create a file for external citation management software, your rss feed.
Reliability and validity in researchAffiliation.
This article examines reliability and validity as ways to demonstrate the rigour and trustworthiness of quantitative and qualitative research. The authors discuss the basic principles of reliability and validity for readers who are new to research. PubMed Disclaimer Similar articles
Publication types
LinkOut - more resourcesFull text sources.
NCBI Literature Resources MeSH PMC Bookshelf Disclaimer The PubMed wordmark and PubMed logo are registered trademarks of the U.S. Department of Health and Human Services (HHS). Unauthorized use of these marks is strictly prohibited. Qualitative vs. Quantitative Data: 7 Key DifferencesQualitative data is information you can describe with words rather than numbers. Quantitative data is information represented in a measurable way using numbers. One type of data isn’t better than the other. To conduct thorough research, you need both. But knowing the difference between them is important if you want to harness the full power of both qualitative and quantitative data. In this post, we’ll explore seven key differences between these two types of data. #1. The Type of DataThe single biggest difference between quantitative and qualitative data is that one deals with numbers, and the other deals with concepts and ideas. The words “qualitative” and “quantitative” are really similar, which can make it hard to keep track of which one is which. I like to think of them this way:
Qualitative data—the descriptive one—usually involves written or spoken words, images, or even objects. It’s collected in all sorts of ways: video recordings, interviews, open-ended survey responses, and field notes, for example. I like how researcher James W. Crick defines qualitative research in a 2021 issue of the Journal of Strategic Marketing : “Qualitative research is designed to generate in-depth and subjective findings to build theory.” In other words, qualitative research helps you learn more about a topic—usually from a primary, or firsthand, source—so you can form ideas about what it means. This type of data is often rich in detail, and its interpretation can vary depending on who’s analyzing it. Here’s what I mean: if you ask five different people to observe how 60 kittens behave when presented with a hamster wheel, you’ll get five different versions of the same event. Quantitative data, on the other hand, is all about numbers and statistics. There’s no wiggle room when it comes to interpretation. In our kitten scenario, quantitative data might show us that of the 60 kittens presented with a hamster wheel, 40 pawed at it, 5 jumped inside and started spinning, and 15 ignored it completely. There’s no ifs, ands, or buts about the numbers. They just are. #2. When to Use Each Type of DataYou should use both quantitative and quantitative data to make decisions for your business. Quantitative data helps you get to the what . Qualitative data unearths the why . Quantitative data collects surface information, like numbers. Qualitative data dives deep beneath these same numbers and fleshes out the nuances there. Research projects can often benefit from both types of data, which is why you’ll see the term “mixed-method” research in peer-reviewed journals. The term “mixed-method” refers to using both quantitative and qualitative methods in a study. So, maybe you’re diving into original research. Or maybe you’re looking at other peoples’ studies to make an important business decision. In either case, you can use both quantitative and qualitative data to guide you. Imagine you want to start a company that makes hamster wheels for cats. You run that kitten experiment, only to learn that most kittens aren’t all that interested in the hamster wheel. That’s what your quantitative data seems to say. Of the 60 kittens who participated in the study, only 5 hopped into the wheel. But 40 of the kittens pawed at the wheel. According to your quantitative data, these 40 kittens touched the wheel but did not get inside. This is where your qualitative data comes into play. Why did these 40 kittens touch the wheel but stop exploring it? You turn to the researchers’ observations. Since there were five different researchers, you have five sets of detailed notes to study. From these observations, you learn that many of the kittens seemed frightened when the wheel moved after they pawed it. They grew suspicious of the structure, meowing and circling it, agitated. One researcher noted that the kittens seemed desperate to enjoy the wheel, but they didn’t seem to feel it was safe. So your idea isn’t a flop, exactly. It just needs tweaking. According to your quantitative data, 75% of the kittens studied either touched or actively participated in the hamster wheel. Your qualitative data suggests more kittens would have jumped into the wheel if it hadn’t moved so easily when they pawed at it. You decide to make your kitten wheel sturdier and try the whole test again with a new set of kittens. Hopefully, this time a higher percentage of your feline participants will hop in and enjoy the fun. This is a very simplistic and fictional example of how a mixed-method approach can help you make important choices for your business. #3. Data You Have Access ToWhen you can swing it, you should look at both qualitative and quantitative data before you make any big decisions. But this is where we come to another big difference between quantitative vs. qualitative data: it’s a lot easier to source qualitative data than quantitative data. Why? Because it’s easy to run a survey, host a focus group, or conduct a round of interviews. All you have to do is hop on SurveyMonkey or Zoom and you’re on your way to gathering original qualitative data. And yes, you can get some quantitative data here. If you run a survey and 45 customers respond, you can collect demographic data and yes/no answers for that pool of 45 respondents. But this is a relatively small sample size. (More on why this matters in a moment.) To tell you anything meaningful, quantitative data must achieve statistical significance. If it’s been a while since your college statistics class, here’s a refresh: statistical significance is a measuring stick. It tells you whether the results you get are due to a specific cause or if they can be attributed to random chance. To achieve statistical significance in a study, you have to be really careful to set the study up the right way and with a meaningful sample size. This doesn’t mean it’s impossible to get quantitative data. But unless you have someone on your team who knows all about null hypotheses and p-values and statistical analysis, you might need to outsource quantitative research. Plenty of businesses do this, but it’s pricey. When you’re just starting out or you’re strapped for cash, qualitative data can get you valuable information—quickly and without gouging your wallet. #4. Big vs. Small Sample SizeAnother reason qualitative data is more accessible? It requires a smaller sample size to achieve meaningful results. Even one person’s perspective brings value to a research project—ever heard of a case study? The sweet spot depends on the purpose of the study, but for qualitative market research, somewhere between 10-40 respondents is a good number. Any more than that and you risk reaching saturation. That’s when you keep getting results that echo each other and add nothing new to the research. Quantitative data needs enough respondents to reach statistical significance without veering into saturation territory. The ideal sample size number is usually higher than it is for qualitative data. But as with qualitative data, there’s no single, magic number. It all depends on statistical values like confidence level, population size, and margin of error. Because it often requires a larger sample size, quantitative research can be more difficult for the average person to do on their own. #5. Methods of AnalysisRunning a study is just the first part of conducting qualitative and quantitative research. After you’ve collected data, you have to study it. Find themes, patterns, consistencies, inconsistencies. Interpret and organize the numbers or survey responses or interview recordings. Tidy it all up into something you can draw conclusions from and apply to various situations. This is called data analysis, and it’s done in completely different ways for qualitative vs. quantitative data. For qualitative data, analysis includes:
You can often do qualitative data analysis manually or with tools like NVivo and ATLAS.ti. These tools help you organize, code, and analyze your subjective qualitative data. Quantitative data analysis is a lot less subjective. Here’s how it generally goes:
Researchers generally use sophisticated data analysis tools like RapidMiner and Tableau to help them do this work. #6. FlexibilityQuantitative research tends to be less flexible than qualitative research. It relies on structured data collection methods, which researchers must set up well before the study begins. This rigid structure is part of what makes quantitative data so reliable. But the downside here is that once you start the study, it’s hard to change anything without negatively affecting the results. If something unexpected comes up—or if new questions arise—researchers can’t easily change the scope of the study. Qualitative research is a lot more flexible. This is why qualitative data can go deeper than quantitative data. If you’re interviewing someone and an interesting, unexpected topic comes up, you can immediately explore it. Other qualitative research methods offer flexibility, too. Most big survey software brands allow you to build flexible surveys using branching and skip logic. These features let you customize which questions respondents see based on the answers they give. This flexibility is unheard of in quantitative research. But even though it’s as flexible as an Olympic gymnast, qualitative data can be less reliable—and harder to validate. #7. Reliability and ValidityQuantitative data is more reliable than qualitative data. Numbers can’t be massaged to fit a certain bias. If you replicate the study—in other words, run the exact same quantitative study two or more times—you should get nearly identical results each time. The same goes if another set of researchers runs the same study using the same methods. This is what gives quantitative data that reliability factor. There are a few key benefits here. First, reliable data means you can confidently make generalizations that apply to a larger population. It also means the data is valid and accurately measures whatever it is you’re trying to measure. And finally, reliable data is trustworthy. Big industries like healthcare, marketing, and education frequently use quantitative data to make life-or-death decisions. The more reliable and trustworthy the data, the more confident these decision-makers can be when it’s time to make critical choices. Unlike quantitative data, qualitative data isn’t overtly reliable. It’s not easy to replicate. If you send out the same qualitative survey on two separate occasions, you’ll get a new mix of responses. Your interpretations of the data might look different, too. There’s still incredible value in qualitative data, of course—and there are ways to make sure the data is valid. These include:
Whether you’re dealing with qualitative or quantitative data, transparency, accuracy, and validity are crucial. Focus on sourcing (or conducting) quantitative research that’s easy to replicate and qualitative research that’s been peer-reviewed. With rock-solid data like this, you can make critical business decisions with confidence. Make your website better. Instantly.Keep reading about user experience. ![]() 7 Qualitative Data Examples and Why They WorkQualitative data presents information using descriptive language, images, and videos instead of numbers. To help make sense of this type of data—as opposed to quantitative… ![]() The 5 Best Usability Testing Tools ComparedUsability testing helps designers, product managers, and other teams figure out how easily users can use a website, app, or product. With these tools, user… ![]() 5 Qualitative Data Analysis Methods + When To Use EachQualitative data analysis is the work of organizing and interpreting descriptive data. Interview recordings, open-ended survey responses, and focus group observations all yield descriptive—qualitative—information. This… ![]() The 5 Best UX Research Tools ComparedUX research tools help designers, product managers, and other teams understand users and how they interact with a company’s products and services. The tools provide… ![]() Qualitative data is information you can describe with words rather than numbers. Quantitative data is information represented in a measurable way using numbers. One type… ![]() 6 Real Ways AI Has Improved the User ExperienceIt seems like every other company is bragging about their AI-enhanced user experiences. Consumers and the UX professionals responsible for designing great user experiences are… ![]() 12 Key UX Metrics: What They Mean + How To Calculate EachUX metrics help identify where users struggle when using an app or website and where they are successful. The data collected helps designers, developers, and… ![]() 5 Key Principles Of Good Website UsabilityEase of use is a common expectation for a site to be considered well designed. Over the past few years, we have been used to… ![]() 20 Ways to Speed Up Your Website and Improve Conversion in 2024Think that speeding up your website isn’t important? Big mistake. A one-second delay in page load time yields: Your site taking a few extra seconds to… ![]() How to Do Usability Testing RightUser experience is one of the most important aspects of having a successful website, app, piece of software, or any other product that you’ve built. … ![]() Website Navigation: Tips, Examples and Best PracticesYour website’s navigation structure has a huge impact on conversions, sales, and bounce rates. If visitors can’t figure out where to find what they want,… ![]() How to Create a Heatmap Online for Free in Less Than 15 MinutesA heatmap is an extremely valuable tool for anyone with a website. Heatmaps are a visual representation of crucial website data. With just a simple… ![]() Website Analysis: Our 4-Step Process Plus the Tools and Examples You Need to Get StartedThe problem with a lot of the content that covers website analysis is that the term “website analysis” can refer to a lot of different things—and… ![]() How to Improve My Website: Grow Engagement and Conversions by Fixing 3 Common Website ProblemsHere, we show you how to use Google Analytics together with Crazy Egg’s heatmap reports to easily identify and fix 3 common website problems. ![]() Comprehensive Guide to Website Usability Testing (With Tools and Software to Help)We share the 3-step process for the website usability testing we recommend to our customers, plus the tools to pull actionable insights out of the process. Over 300,000 websites use Crazy Egg to improve what's working, fix what isn't and test new ideas. Last Updated on September 14, 2021 Ask an Academic ![]() What are validity and reliability in Quantitative research?how validity and reliability are achieved in quantitative research? Quantitative research is the process of a systematic investigation, primarily using numerical techniques (statistical, mathematical or computational), to test hypothetical generalisations. As a way of measuring the likelihood of the researcher’s result being misleading, statisticians developed procedures for expressing the likelihoods and accuracy of the results. These procedures help demonstrate the rigour and usefulness of the researcher’s work. Rigour, in quantitative studies, refers to the extent the researchers worked to enhance the quality of the study; this is achieved through measurement of reliability and validity. Reliability refers to the consistency of the measurements or the degree to which an instrument measures the same with every use under the exact same conditions. Reliability is usually estimated using internal consistency – the relationship/correlation between different results of a test, or instrument. These correlations are most commonly measured using Cronbach’s α coefficient; a statistical test that ‘splits’ all the results in half and calculates the correlations between the two sets. From this, a single value between 0-1 is generated and the closer the coefficient generated is to 1, the higher the reliability estimate of your instrument/test. Validity is defined as the extent to which a measure or concept is accurately measured in a study. In essence, it is how well a test or piece of research measures what it is intended to measure. In quantitative studies, there are two broad measurements of validity – internal and external. Internal validity is an estimate of the degree to which conclusions about causal relationships can be made based on the research design. Internal validity utilises three approaches (content validity, criterion-related validity and construct validity) to address the reasons for the outcome of the study. External validity is the extent the results of a study can be generalised to other populations, settings or situations; commonly applied to laboratory research studies. This validity can usually be divided into population validity and ecological validity; essential elements in judging the strength of an experimental design. Privacy OverviewUnderstanding validity and reliability from qualitative and quantitative research traditions
![]()
Discover the world's research
![]()
![]()
![]()
![]()
![]()
![]()
![]()
![]()
![]()
ORIGINAL RESEARCH articlePredictive and concurrent validity of pain sensitivity phenotype, neuropeptidomics and neuroepigenetics in the mi-rat osteoarthritic surgical model in rats.
Background: Micro-RNAs could provide great insights about the neuropathological mechanisms associated with osteoarthritis (OA) pain processing. Using the validated M ontreal I nduction of R at A rthritis T esting (MI-RAT) model, this study aimed to characterize neuroepigenetic markers susceptible to correlate with innovative pain functional phenotype and targeted neuropeptide alterations. Methods: Functional biomechanical, somatosensory sensitization (peripheral–via tactile paw withdrawal threshold; central–via response to mechanical temporal summation), and diffuse noxious inhibitory control (via conditioned pain modulation) alterations were assessed sequentially in OA ( n = 12) and Naïve ( n = 12) rats. Joint structural, targeted spinal neuropeptides and differential expression of spinal cord micro-RNAs analyses were conducted at the sacrifice (day (D) 56). Results: The MI-RAT model caused important structural damages (reaching 35.77% of cartilage surface) compared to the Naïve group ( P < 0.001). This was concomitantly associated with nociceptive sensitization: ipsilateral weight shift to the contralateral hind limb (asymmetry index) from −55.61% ± 8.50% (D7) to −26.29% ± 8.50% (D35) ( P < 0.0001); mechanical pain hypersensitivity was present as soon as D7 and persisting until D56 ( P < 0.008); central sensitization was evident at D21 ( P = 0.038); pain endogenous inhibitory control was distinguished with higher conditioned pain modulation rate ( P < 0.05) at D7, D21, and D35 as a reflect of filtrated pain perception. Somatosensory profile alterations of OA rats were translated in a persistent elevation of pro-nociceptive neuropeptides substance P and bradykinin, along with an increased expression of spinal miR-181b ( P = 0.029) at D56. Conclusion: The MI-RAT OA model is associated, not only with structural lesions and static weight-bearing alterations, but also with a somatosensory profile that encompasses pain centralized sensitization, associated to active endogenous inhibitory/facilitatory controls, and corresponding neuropeptidomic and neuroepigenetic alterations. This preliminary neuroepigenetic research confirms the crucial role of pain endogenous inhibitory control in the development of OA chronic pain (not only hypersensitivity) and validates the MI-RAT model for its study. 1 IntroductionOsteoarthritis (OA) affects more than 25% of the population in Western countries, ranking it as the most common degenerative joint disease ( GBD, 2018 ), with its prevalence rising yearly due to global aging and obesity. This complex disease, involving joint structural damage and evolving pain, challenges therapeutic development. Biochemical changes contribute to nociceptive peripheral sensitization ( Malfait and Schnitzer, 2013 ). Increased nociceptive inputs may lead to centralized sensitization, an extended hyperexcitability of central nervous system (CNS) pain circuits, and an adaptive CNS response ( Malfait and Schnitzer, 2013 ; Eitner et al., 2017 ). Individuals with advanced OA may experience pain from nociceptive, inflammatory mechanisms, excessive (neuropathic) excitability, and/or deficient endogenous inhibitory control in pain processes ( Fu et al., 2018 ). Classically characterized by local cartilage degeneration, bone remodeling, synovium inflammation, and soft-tissue alterations ( Dobson et al., 2018 ), OA establishes a complex pain process described as nociplastic ( Fitzcharles et al., 2021 ; Buldys et al., 2023 ), involving chronic pain with increased sensitivity due to altered function in pain-related sensory pathways ( Chimenti et al., 2018 ). The translation from experimental pain animal models to effective clinical treatment for chronic pain faces significant challenges, with some attributing these translation failures to certain shortcomings in clinical trials, or the lack of validity in animal models and/or chronic pain assessment methods, which might hinder the translation of promising interventions ( Mogil, 2017 ). The chemical intra-articular injection of monosodium iodoacetate (MIA) is the most common OA pain model in rats, and in this model, central sensitization may be associated with an up-regulation of spinal neuropeptides, indicating the activation of peripheral nociceptors on peptidergic afferent C-fibers ( Im et al., 2010 ; Eitner et al., 2017 ). However, criticism has emerged regarding the etiopathogenesis, acute occurrence, and temporal transience of this OA pain model, which may not necessarily be related to the OA disease, classifying it more as inflammatory and nociceptive ( Barve et al., 2007 ; Hummel and Whiteside, 2017 ; Otis et al., 2017 ). Various surgical models of OA have been tested in rats ( Gervais et al., 2019 ). The one that combines cranial cruciate ligament transection (CCLT) and destabilization of the medial meniscus (DMM) associated with an exercise protocol, known as the M ontreal I nduction of R at A rthritis T esting (MI-RAT) model, has been demonstrated to allow the progressive development of structural OA over time and indicates persistent chronic pain changes ( Otis et al., 2023 ). These changes include behavioral biomechanical alterations, sensory mechanical hypersensitivity, and spinal neuropeptide changes ( Gervais et al., 2019 ; Keita-Alassane et al., 2022 ; Otis et al., 2023 ). In a recent study leading to the refinement of the surgical CCLT – DMM OA model, it was observed that gender dimorphism must be carefully considered when evaluating OA pain, as 17β-estradiol supplementation influenced central sensitization development ( Keita-Alassane et al., 2022 ). Interestingly, associating calibrated slight exercise (on a treadmill) with stifle instability surgical induction in the MI-RAT model resulted in major benefits, including 1) homogenization of structural alterations and 2) persistence of the pain and sensory sensitization profile over time, resembling the human OA condition more closely ( Otis et al., 2023 ). The sensitization level was lower than in sedentary CCLT – DMM group and implied the involvement of endogenous inhibitory control (EIC), as supported by spinal neuropeptidomics. In continuation of the previous studies, this work aims to advance the development of quantitative sensory testing (QST) of pain in association with neuropeptidomics and neuroepigenetics. Validated in human patients, QST is a psychophysical test method investigating the functional state of the somatosensory system ( Mucke et al., 2021 ). It assesses sensory (pain) loss (hypoalgesia, reinforcement of EIC) and sensory (pain) gain (hyperalgesia/allodynia). Static QST was previously validated concurrently with neuropeptidomics, to be related to somatosensory hypersensitivity, using the MIA rat chemical OA model ( Otis et al., 2016 ; Otis et al., 2017 ; Gervais et al., 2019 ; Otis et al., 2019 ), the surgical CCLT and/or DMM OA models ( Gervais et al., 2019 ; Keita-Alassane et al., 2022 ), as well as in the MI-RAT OA model ( Otis et al., 2023 ). Dynamic QST, allowing exploration of the altered function of pain-related sensory pathways in the periphery and CNS, i.e., facilitation or inhibition of pain signals, were adapted, in the current study, for testing in the MI-RAT model. Epigenetic mechanisms regulate gene expression without altering the primary DNA sequence. Endogenous small non-coding single-stranded RNA, defined as micro-RNAs (miRNAs), plays pivotal role in post-transcriptional gene regulation of a wide range of biological processes ( McDonald and Ajit, 2015 ). They modulate gene expression by binding to target mRNAs, affecting translation ( Lu, 2023 ), or degradation, and are involved in diverse cellular processes, including development, differentiation, disease pathways, and are suspecting to play an important role in chronic pain ( Lutz et al., 2014 ; Golmakani et al., 2024 ). Considering that alterations in protein expression play a crucial role in the development of long-term hyperexcitability in nociceptive neurons and contribute to chronic pain establishment, miRNAs hold great potential for elucidating nociceptive sensitization processes ( Gold and Gebhart, 2010 ). Understanding miRNA involvement could unravel novel targets for managing chronic pain and mitigating nociceptive sensitization. To our knowledge, only one study has demonstrated altered expression of spinal miR-146a and the miR-183 cluster, linked to OA pain in the stifle joint, in a surgically induced OA rat model ( Li et al., 2013 ). The hypothesis of this preliminary study was that neuroepigenetics might be modulated by somatosensory sensitization development (or vice-versa) in an animal model of chronic OA pain. Taken together, the expression of neuroepigenetics, neuropeptidomics, and pain phenotype, particularly QST, would highlight pathophysiological mechanisms at the peripheral, spinal, and/or supraspinal levels, recognized to be involved in nociplastic pain ( Buldys et al., 2023 ). In a prospective, randomized, blinded, and controlled study, the objectives were to document parallel changes induced in the MI-RAT OA model on spinal neuroepigenetics and neuropeptidomics in the non-evoked expression of musculoskeletal pain, as well as on evoked QST. This would pursue the determination of the MI-RAT as a predictive and concurrently validated translatable OA model. 2.1 Functional pain outcomes2.1.1 static weight-bearing (swb). The contralateral weight shift from the right (OA-induced) to the left hind limb is represented as the SWB asymmetry index in Figure 1 . Statistical analysis (general linear mixed model) revealed significant effects of group ( P < 0.001), time ( P < 0.001), and time × group interaction ( P < 0.001). Rats in the OA group exerted a markedly higher SWB report to the left hind limb following OA induction. From day (D)7 to D35 inclusively, the SWB report in the OA group was statistically significant and different in comparison to the Naïve group ( P < 0.001), indicating that OA rats applied less weight on their affected limb (right hind limb) from the first timepoint of assessment and reported it on their contralateral limb (left hind limb). The SWB asymmetry index (least square means ± 95% confidence intervals) in the OA group ranged from −55.61% ± 8.50% at D7 to −26.29% ± 8.50% at D35, demonstrating a statistically significant temporal change ( P < 0.001). Over time, results in Figure 1 suggest that OA rats gradually reduced the weight applied from the contralateral to the ipsilateral hind limb, leading to a normal SWB distribution at D49 ( P = 0.438) and D56 ( P = 0.283) with no significant difference compared to the Naïve group. ![]() Figure 1 . Temporal evolution of static weight-bearing (SWB) asymmetry index in the osteoarthritis (OA) and the Naïve rat groups. Temporal evolution of SWB asymmetry index (%) from the ipsilateral (right) to the contralateral (left) hind limb (least square means ± 95% confidence intervals). The OA group presented a marked contralateral report of SWB from D7 to D35 compared to the Naïve group ( P < 0.001). The contralateral transfer then disappeared at D49 and D56 ( P > 0.280). *Inter-group significant difference at each day ( P < 0.050). 2.1.2 Static QST: tactile paw withdrawal threshold (PWT)Statistical analysis (general linear mixed model) of right hind PWT values revealed significant effects of group ( P < 0.001), time ( P = 0.009), although there was no time × group interaction effect ( P = 0.617). Hence, the OA rats presented lower PWT ( i.e. , evoked mechanical pain hypersensitivity) in the ipsilateral (right) paw ( Figure 2 ) throughout the entire study period (D7 to D56) compared to the Naïve group ( P < 0.008). The PWT for the OA group remained with a significant ( P < 0.021) lower threshold than baseline up to the end of the experiment. ![]() Figure 2 . Temporal evolution of the paw withdrawal threshold (PWT) of ipsilateral (right) hind paw in the osteoarthritis (OA) and the Naïve rat groups. Temporal evolution of the ipsilateral PWT in grams (least square means ± 95% confidence intervals). A significant mechanical pain sensitivity in ipsilateral paw was present as soon as D7 in OA group and maintained up to D56 ( P < 0.008) supported by lower PWT. *Inter-group significant difference at each day ( P < 0.050). 2.1.3 Dynamic QST: response to mechanical temporal summation (RMTS) facilitation and conditioned pain modulation (CPM) inhibitionThe RMTS number of stimuli required to induce a behavioral pain/discomfort-expressive response related to central sensitization development after repeated mechanical stimulation is illustrated in Figure 3 . Statistical analysis (general linear mixed model) demonstrated only a significant effect of time ( P = 0.045), with no significant impact observed for the group ( P = 0.298) or the time × group interaction ( P = 0.231). However, a significant decrease in the number of stimuli necessary to trigger a behavioral response was noted in the OA group (24.27 ± 2.53) at D21 ( P = 0.038) compared to the Naïve group (28.64 ± 2.53). No significant inter-group difference was observed at D35 ( P = 0.590) and D56 ( P = 0.448) between both experimental groups. ![]() Figure 3 . Dynamic quantitative sensory testing evolution overtime of response to mechanical temporal summation as number of stimuli (NS) required to induce a response in the Naïve and osteoarthritis (OA) groups. Dynamic QST is expressed in number of stimuli (NS) needed to induce a pain behavioral response (least square means ± 95% confidence intervals), with a cut-off of 30 NS. Central sensitization was noted at D21 ( P = 0.038) in the OA group by a significant decrease in NS to induce a withdrawal reactive behavior. *Inter-group significant difference at each day ( P < 0.050). The measure of EIC activation following a dynamic conditioning stimulus (CS) is represented by the CPM functional rate in Figure 4 for both the OA and Naïve rat groups. The percentage of CPM positive responders in each group at all timepoints is presented in Table 1 . Compared to baseline, there was a trend from D14 up to D56 of the number of CPM positive responders to increase in the OA group, with a statistically significant inter-group difference (Fisher’s exact test) at D21 ( P = 0.037). In addition, the ipsilateral right hind CPM PWT functional rate (general linear mixed model) exhibited significant group ( P < 0.001) and time effects ( P = 0.001), without a time × group interaction ( P = 0.088). A substantial increase in the CPM functional rate for OA rats ( Figure 4 ) was present throughout the entire follow-up period (group effect). Specifically, significant increases were observed at D7 (32.43%, P = 0.050), D21 (90.37%, P < 0.001), and D35 (35.37%, P = 0.050) compared to the CPM functional rate of Naïve rats. ![]() Figure 4 . Temporal evolution of conditioned pain modulation (CPM) in the osteoarthritis (OA) and the Naïve rat groups. The temporal evolution of CPM rate (in percentage of increase in delta CPM post- minus pre-CS PWT) for each group (least square means ± 95% confidence intervals) was determined by including positive responders of CPM in the ipsilateral right hind paw. An increase in CPM rate is a measure of nociceptive endogenous inhibitory control activation. CPM rate of OA rats was higher at D7, D21 and D35 ( P < 0.050) and significantly different than Naïve rats ( P < 0.001). *Inter-group significant difference at each day ( P < 0.050). ![]() Table 1 . Percentage of positive responders to conditioned pain modulation (CPM) . 2.2 Molecular analysis2.2.1 comparison of spinal neuropeptides revealed an increase in the concentration of pain-related neuropeptides in the oa group compared to the naïve group. At D56, in comparison (two-sided Mann-Whitney-Wilcoxon test) to Naïve-ovariectomized rats, the OA group exhibited a significant ( P = 0.002) increase in spinal concentration (mean (standard deviation); median [min-max]) of substance P (SP) (102.59 (8.72); 102.40 [91.90–115.25] fmol/mg) versus (79.16 (3.20); 79.90 [74.96–82.99] fmol/mg) and bradykinin (BK) (312.61 (26.28); 312.74 [272.95–354.31] fmol/mg versus 235.04 (25.60); 237.88 [187.27–262.30] fmol/mg), as illustrated in Figure 5 (data values are represented as mean (standard deviation)). The other two neuropeptides, calcitonin gene-related peptide (CGRP) and somatostatin (SST), displayed a similar trend of increase in OA rats (516.33 (75.84); 497.99 [447.37–664.80] and 402.73 (34.28); 419.90 [345.86–429.36] fmol/mg, respectively) compared to Naïve-ovariectomized rats (452.82 (54.31); 451.69 [370.31–532.39] and 342.47 (62.47); 324.55 [295.46–466.14] fmol/mg, respectively), although the difference was not statistically significant ( P = 0.093 and P = 0.065, respectively). ![]() Figure 5 . Spinal neuropeptides concentration (fmol/mg) of substance P (SP), calcitonin gene-related peptide (CGRP), bradykinin (BK) and somatostatin (SST) 56 days after induction of the MI-RAT model. The MI-RAT OA model induced a significant increase at D56 in SP and BK spinal cord (mean ± standard deviation) of OA rats ( P = 0.002). CGRP and SST were also higher in OA rats, however, not at a statistically significant level ( P = 0.093 and 0.065, respectively). *Inter-group significant difference for each neuropeptide ( P < 0.050). 2.2.2 Comparison between the OA and Naïve groups for the expression level of fourteen selected pain-related miRNAs showed a difference in the expression of one miRNAAnalysis (two-sided Mann-Whitney-Wilcoxon test) of the data revealed that 56 days after the OA induction (surgical joint instability) and development (through regular calibrated slight exercise), miRNA expression in the OA rat spinal cord differed for one of the fourteen analyzed miRNAs ( Table 2 ). Indeed, only miR-181b-5p showed a statistically significant alteration in its expression ( P = 0.029) compared to the Naïve group. ![]() Table 2 . Fold change (OA/Naïve) in expression of selected miRNAs for RT-qPCR screening in spinal cord 56 days after OA induction and development. 2.3 Structural joint evaluation2.3.1 induced-oa model in rats using the mi-rat protocol involves macroscopic and histological lesions in the stifle structures. The CCLT – DMM joint instability surgery and calibrated slight exercise induced significant ( P < 0.001) cartilage damages in the right stifle (35.77% ± 9.00% cartilage lesion score) compared (two-sided Mann-Whitney-Wilcoxon test) to the Naïve group ( Table 3 ). Histological alterations of cartilage in OA rat stifle joints appeared to be mainly attributed to increased chondral lesions, matrix proteoglycan loss, and enhanced cluster formation ( Figure 6 ). ![]() Table 3 . Macroscopic assessment of cartilage lesions and histological modified Mankin score (mMs) in percentage (%) of cartilage alterations of the tibial and femoral (medial and lateral) right stifle at sacrifice (D56). ![]() Figure 6 . Representative cartilage of Naïve and osteoarthritis (OA) rats. Photomicrographs of representative histological sections (stained with hematoxylin and eosin, and Safranin-O/Fast green) of tibial plateaus of Naïve (A) and OA (B) rats. Arrows indicate cartilage erosion, black arrowheads the lost of proteoglycans and white arrowheads the presence of cell clusters. Original magnification ×40. Scale bar: 100 µm. 3 DiscussionThe reproducibility crisis in scientific research, particularly in the pain field, has raised doubts about the translational reliability of efficacy data from animal disease models ( Klinck et al., 2017 ). It is crucial for animal models to closely mimic clinical conditions, including subjects used, disease induction methods, and the validity and limitations of outcome measurements ( Klinck et al., 2017 ), yet there remains a lack of standardization design in animal model. The ideal model should be reliable, valid, and highly translational ( Little and Smith, 2008 ), but how can the reliability and translational relevance of OA pain models be enhanced to better reflect clinical outcomes? To address this issue, our lab focused on OA rat models over the past decade, assessing psychometric validity (repeatability and inter-rater reliability) of various functional outcomes, including biomechanical measures, operant behaviors, and sensory sensitization. Different acclimatization protocols and environmental influences (gender, observer experience, circadian cycle, exercise) were tested ( Otis et al., 2016 ). Using the intra-articular MIA model, specificity and sensitivity of functional outcomes like PWT-assessed sensory hypersensitivity and spinal neuropeptides were evaluated, along with treatment responsiveness to pharmacological treatments ( Otis et al., 2016 ; 2017 ; 2019 ). Recognizing limitations in the MIA model, surgical OA pain models, particularly the CCLT–DMM model, were explored for their effectiveness in inducing structural and functional alterations and spinal neuropeptidomics ( Gervais et al., 2019 ). Our recent studies demonstrated the relevance of using ovariectomized animals in the MI-RAT model for studying central sensitization process, showing significant analgesic effects with 17β-estradiol supplementation ( Keita-Alassane et al., 2022 ). Adding a calibrated exercise program post-OA induction standardized structural alterations, resulting in a pain and sensory profile closer to human OA ( Otis et al., 2023 ). The exercise MI-RAT group showed reduced mechanical pain hypersensitivity and lower levels of pro-nociceptive spinal peptides compared to the sedentary group. This effect may involve reinforced descending EIC, supported by increased spinal concentration in SST, Met-ENK, and Leu-ENK. Additionally, exercise increased BK, possibly due to joint manipulation ( Meini and Maggi, 2008 ; Otis et al., 2023 ). These findings confirmed the effectiveness of incorporating slight exercise into the surgical OA model, enhancing its translatability and responsiveness to multimodal pharmacological treatments, closer to clinical OA ( Otis et al., 2023 ). Therefore, the aim of this study was to pursue the refinement of the MI-RAT model by validating a panel of pain assessment methods, including functional neuropeptidomics, neuroepigenetics, and innovative QST applied to the experimental MI-RAT model, in order to characterize OA pain with great validity and reliability. The results obtained provided valuable insights: neuroepigenetics appears to be activated by the development of somatosensory sensitization and its complex facilitatory/inhibitory endogenous control in the MI-RAT OA model. Therefore, the MI-RAT model induced significant structural damage, coinciding with nociceptive sensitization, as evidenced by several factors: ipsilateral weight shift towards the contralateral hind limb (asymmetry index) at D7 and D35; mechanical pain hypersensitivity observed from D7 and persisting until D56; central sensitization becoming apparent at D21; and enhanced EIC noted with a higher CPM rate at D7, D21, and D35. The somatosensory profile alterations observed in OA rats were characterized, even at D56, by an increase in pro-nociceptive neuropeptides SP and BK, alongside augmented expression of spinal miR-181b at D56. First, functional pain outcome evaluations indicated that the MI-RAT OA model induced biomechanical (SWB) and sensory sensitization (static and dynamic QST) alterations associated with OA development. Significant ipsilateral to contralateral weight shift on SWB was observed in OA rats from the initial assessment (D7) post-OA induction, lasting until D35. However, during the subsequent 3 weeks (D49 and D56 timepoints), MI-RAT rats concealed the biomechanical pain phenotype, suggesting an attenuated perception of discomfort of the OA-induced limb, whereas in the previous timepoints, they alleviated the noxious sensation by reducing applied weight ( Gervais et al., 2019 ). The standing on hind limbs, especially with an OA-induced stifle, appeared painful or uncomfortable for the rats. Therefore, the observed spontaneous (non-evoked) pain in SWB assessment can be interpreted as biomechanical allodynia, indicating initial sensory hypersensitivity countered by reinforced EIC, ultimately leading to condition normalization 7 weeks post-OA induction. Secondly, the persistent decrease in the right PWT, alongside lower static QST values, throughout the whole follow-up indicated the presence of a centralized sensitization. Mechanical pain hypersensitivity in the plantar region of the ipsilateral hind limb is secondary to the damaged stifle joint, suggesting referred pain, similar to observations in OA patients ( Harvey and Dickenson, 2009 ). Dynamic QST results revealed a significantly lower number of RMTS for OA rats at D21, representing a phenotypical form of central sensory hypersensitivity ( Woolf, 2011 ; Guillot et al., 2014 ). In naturally affected OA cats, RMTS assessment has shown specificity to the OA condition and sensitivity to anti-nociceptive tramadol, but not NSAID meloxicam ( Monteiro et al., 2016 ; Monteiro et al., 2017 ). Temporal summation, widely used to explore spinal cord excitability, reflects the early phase of neuronal windup, considered intrinsic to CNS changes in pathological pain. Described as activity-dependent facilitation, temporal summation evaluates conscious perception of centralized sensitization, contrasting with PWT (static QST), which assesses sensory-reflexive hypersensitivity ( Guillot et al., 2014 ). Central sensitization mechanisms include various biochemical processes such as increased spinal release of pro-nociceptive neurotransmitters and neuromodulators, and increased excitability of postsynaptic neurons ( Woolf, 1996 ). Neurophysiologically, temporal summation occurs when a presynaptic neuron releases neurotransmitter(s) multiple times, exceeding the postsynaptic neuron’s threshold and inducing excitability ( Woolf, 1996 ; Woolf, 2011 ). To the authors knowledge, this is the first report of a dynamic QST method to an experimental OA rat model, revealing a spinal windup phenomenon likely to manifest around 3 weeks after OA induction by CCLT – DMM surgery. This is promising avenue as the authors consider bringing technological modifications to the RMTS methodology to improve the metrological properties (specificity, sensitivity, and reliability) of the method in future assessments of the MI-RAT OA model. Finally, pain endogenous inhibitory modulation was evaluated by applying a painful CS before conducting a second static QST, based on the concept of “pain inhibiting pain” as a measure of pain perception ( Yarnitsky et al., 2010 ; Youssef et al., 2016 ). This concept is based on activation of diffuse noxious inhibitory control resulting in higher pain threshold (lower sensitivity) as a functional CPM process ( Le Bars et al., 1979 ; Yarnitsky et al., 2010 ). Our results indicated that CPM resulted in a 15.94% increase in post-CS PWT at baseline (unpainful) and revealed a group effect, with a more functional CPM rate observed in the OA rats throughout the entire follow-up. Moreover, at D7, D21, and D35, pain perception in OA rats was significantly modulated by a higher CPM rate, reaching a peak at D21 with a 90.37% increase in CPM intensity. At the same time (D21), there was also a significantly higher rate of positive CPM responders in OA group, aligning with the observed central sensitization noted by RMTS in the MI-RAT model. This is further explained and supported by previous QST assessments in this study, indicating that OA rats from the MI-RAT model are more painful at those timepoints, necessitating the activation of diffuse noxious inhibitory control. The CPM paradigm is currently an effective and validated somatosensory test used to identify humans suffering from chronic pain related to OA ( Arant et al., 2022 ). All four functional pain outcome assessments indicated nociceptive centralized sensitization and hyperexcitability processes in OA rats, predominantly observed from D7 to D35. However, at D49 and D56, only PWT, a more sensitive outcome, demonstrated these effects. During this period, hyperexcitability was progressively counteracted by efficient EIC, reducing the demand on CPM functionality, allowing OA-affected rats to conceal their biomechanical imbalance. The decline in centralized sensory sensitization, associated with reinforced EIC, was demonstrated previously by calibrated slight exercise post-OA induction in rats, compared to sedentary rats with the same surgical (CCLT – DMM) model, with a reduction, at D56, in pro-nociceptive tachykinins and an increase in anti-nociceptive SST and enkephalins ( Otis et al., 2023 ). Neuropeptide analysis 56 days post MI-RAT induction supports functional pain outcomes. Glutamate and aspartate act as excitatory neurotransmitters in the somatosensory system, persistently activating post-synaptic receptors and sensitizing dorsal horn neurons, leading to increased receptive field size, decreased activation threshold, and prolonged depolarization ( Guillot et al., 2014 ; Nouri et al., 2018 ). Conversely, glycine and γ-aminobutyric acid (GABA) serve as chief inhibitory neurotransmitters in the somatosensory system ( Ferland et al., 2011 ; Nouri et al., 2018 ). Norepinephrine’s inhibitory effects in the descending brainstem to dorsal horn pathway have a dual impact: direct activation of inhibitory GABAergic interneurons and inhibition of excitatory interneurons. Serotonin plays a key role in descending inhibitory controls, primarily from midbrain raphe magnus nuclei . Neuropeptides, classically considered as modulators of sensory transmission, are categorized as either excitatory or inhibitory compounds. Neurokinin A, SP and CGRP are found in intrinsic neurons of the spinal dorsal horn and are released only in specific response to noxious stimuli, sufficient to elicit sustained discharges of unmyelinated C-fibers in the superficial layers ( Nouri et al., 2018 ). Vesicles containing mature neuropeptides undergo exocytosis at the synaptic cleft, in response to noxious stimuli, facilitatory/inhibitory controls, influencing neurotransmission and modulating neuronal signals. These peptides, rather than acting as synaptic transmitters, diffuse in the dorsal horn, potentially influencing multiple synapses distant from their release point, contributing to final sensory sensitization signal ( Nouri et al., 2018 ). Spinal inhibitory neuropeptides include SST, enkephalins, and possibly dynorphin, found in intrinsic neurons of the dorsal horn (local circuit) and fibers descending from various brainstem nuclei . Sensory sensitization may result from long-term potentiation, reduced GABAergic or glycinergic inhibitory neurotransmission (disinhibition), intrinsic plasticity in dorsal horn neurons, and changes in low threshold mechanoreceptive Aβ afferents. Many excitatory interneurons contain SST, and the resulting increase in the release of this peptide may hyperpolarize nearby inhibitory interneurons, exerting a disinhibitory effect ( Prescott, 2015 ). At D56, CGRP concentration was lower than expected, indicating possible establishment and fluctuation of nociceptive sensitization, partly influenced by SP. In a dose-response comparative study of the chemical MIA model in rats ( Otis et al., 2017 ), SP, CGRP, BK, SST, and transthyretin exhibited a correlation with cartilage lesions and functional assessments. These markers were also highly sensitive in our original neuropeptidomics screening across various pain animal models ( Rialland et al., 2014a ; Rialland et al., 2014b ). Indeed, SST uniquely demonstrated a dose-effect with MIA intra-articular injection ( Otis et al., 2017 ) and paralleled structural alterations induced by different surgical OA models ( Gervais et al., 2019 ), highlighting its heightened sensitivity in pain detection. Moreover, SP, SST, and transthyretin mimicked the beneficial effects observed on functional assessments for different analgesics, namely intra-articular lidocaine ( Otis et al., 2016 ), systemic pregabalin, morphine and carprofen ( Otis et al., 2019 ), suggesting a higher sensitivity of these biomarkers in treatment responsiveness. Somatostatin exhibits anti-inflammatory and anti-(or pro-) nociceptive effects through interactions with other neuropeptides, including SP and CGRP ( Pinter et al., 2006 ). The increased SST release in inflammatory conditions, along with other neuropeptides, indicates the potential for hyperexcitability induction ( Gervais et al., 2019 ). Although SST levels were elevated in the OA group, the difference with the Naïve group was not statistically significant ( P = 0.065). Another noteworthy neuropeptide in OA pain is BK, a kinin family peptide released during tissue injury and inflammation, acting on B 2 and injury-induced B 1 G-protein-coupled receptors expressed on peripheral terminals of primary neurons ( Ferreira et al., 2002 ; Wang et al., 2005 ). Interaction of BK with other pro-inflammatory and hyperalgesic mediators, such as ion channels, prostaglandins, CGRP and other neuropeptides, highlights its pro-inflammatory and nociceptive properties ( Ferreira et al., 2002 ; Aldossary et al., 2024 ). Despite being primarily considered a peripheral inflammatory mediator, BK is also involved in central pain transmission, with identified B 1 and B 2 receptors in the dorsal root ganglion and spinal dorsal horn modulating glutamatergic transmission ( Ferreira et al., 2002 ; Wang et al., 2005 ; Kohno et al., 2008 ). These findings suggest that, by the end of the study, nociceptive processes were gradually coming under control, explaining the limited differences in functional pain outcomes observed at D49 and D56 (statistically significant only for PWT). Additionally, neuroepigenetic changes likely play a regulatory role in nociceptive processes, as supported by miRNA expression levels correlating with neuropeptidomic data analysis. Circulating miRNAs hold increasing importance in clinical medicine for diagnostic, prognostic, and therapeutic stratification ( Tramullas et al., 2018 ). Their stability, specificity, and ease of detection make them promising tools for personalized medicine, aiding in the management of chronic pain and guiding therapeutic decisions. Our objective was to identify potential differences in spinal miRNA expression between a Naïve group and an OA group (MI-RAT model) in rat spinal cord tissue. Our expectations, supported by the validated OA model ( Gervais et al., 2019 ; Keita-Alassane et al., 2022 ; Otis et al., 2023 ), were partially met, revealing limited differences 56 days post-OA induction. With functional alterations more persisting up to D35, would they be linked to higher neuropeptidomics and neuroepigenetics changes at this time? However, by D56, observed neuropeptidomic changes were potentially declining. In the few past years, studies have explored miRNA expression in chronic, neuropathic, and inflammatory pain conditions. Most studies utilized chemical models like intra-articular MIA injection ( Li et al., 2011 ), inflammatory models (complete Freund’s adjuvant ( Bai et al., 2007 ; Pan et al., 2014 ), formalin ( Kynast et al., 2013 ), interleukin-1β ( Willemen et al., 2012 ), bone-cancer pain ( Bali et al., 2013 ), or neuropathic models ( Aldrich et al., 2009 ; Nakanishi et al., 2010 ; Kusuda et al., 2011 ; von Schack et al., 2011 ; Yu et al., 2011 ; Bhalala et al., 2012 ; Sakai et al., 2013 ; Sakai and Suzuki, 2013 ; Shi et al., 2013 ), to reveal neural miRNA expression alterations. In a neuropathic pain study ( Tramullas et al., 2018 ), miR-30c-5p showed upregulation in the spinal dorsal horn 2 weeks post sciatic nerve injury. Intriguingly, this miRNA was the only one significantly dysregulated following qPCR validation, with a modest fold change from next-generation sequencing. Specifically for OA pain in rats, a surgical model (medial meniscus transection) was tested for behavioral (secondary tactile allodynia via static QST), structural, and neuroepigenetic alterations over 8 weeks ( Li et al., 2013 ). This study identified downregulation of miR-146a and miR-183 clusters in the dorsal root ganglia and spinal cord at weeks 4 and 8 (but not 2) post-OA induction, while sensory sensitivity persisted throughout the follow-up ( Li et al., 2013 ). Moreover, OA establishment in human patients or mice models has correlated with miRNA dysregulation in tissues other than the dorsal root ganglia or spinal cord, such as joint tissues (cartilage, bone, synovium) ( Iliopoulos et al., 2008 ; Yamasaki et al., 2009 ; Song et al., 2013 ; Nakamura et al., 2016 ; Tao et al., 2017 ; Nakamura et al., 2019 ). Using a DMM model in mice, it was not possible to demonstrate serum miRNA dysregulation between the OA group and the control group, even though DMM mice showed significant histological signs of cartilage degradation ( Kung et al., 2017 ). In the current study, only one potential neuroepigenetic biomarker demonstrated a different expression between the OA and Naïve groups. Circulating miR181b-5p, identified as elevated in patients with myalgic encephalomyelitis/chronic fatigue syndrome ( Nepotchatykh et al., 2020 ), showed increased expression in the spinal cord of OA rats. Inversely, repressed miR-181b-5p has been reported to participate in the pathogenesis of inflammation and neurological diseases ( Lu et al., 2019 ). The present finding is noteworthy for various reasons in OA research. Structurally, miR-181b and its closely related family member, miR-181a, have been implicated as potential mediators of cartilage degeneration in OA facet and knee joints ( Song et al., 2013 ; Nakamura et al., 2016 ; Nakamura et al., 2019 ). Members of the miR-181 family are associated with the upregulation of catabolic matrix metalloproteinase-13, release of inflammatory mediators, and cartilage degradation ( Nakamura et al., 2016 ). Functionally, the miR-181 family is of interest in OA, as it has been linked to GABAergic regulation ( Zhao et al., 2012 ; Sengupta et al., 2013 ). The overexpression of miR-181a and miR-181b in the spinal cord of a visceral inflammatory model has been demonstrated, associating the upregulation of miR-181b with a downregulation of GABA Aα-1 receptor subunit mRNA and protein ( Sengupta et al., 2013 ). The majority of neurons (>95%) in the spinal dorsal horn are local circuit interneurons releasing neuromodulatory substances such as enkephalin, glycine, and GABA ( Prescott, 2015 ; Nouri et al., 2018 ), and it could be hypothesized that miR181b participates in the spinal dorsal horn inhibitory/facilitatory balance in nociceptive neuromodulation, as suggested by our neuropeptidomics results, specifically concerning SST and transthyretin, both involved in inhibiting neuronal activity. Few years ago, transthyretin knockdown has been associated with decreased GABA A receptor expression ( Zhou et al., 2019 ), where higher level of functional pain and mechanical pain sensitivity were observed in different pain models associated with spinally decreased, and increased, transthyretin, and SST, respectively ( Rialland et al., 2014a ; Rialland et al., 2014b ; Otis et al., 2016 ; Otis et al., 2017 ; Gervais et al., 2019 ; Otis et al., 2019 ; Otis et al., 2023 ). Moreover, SST interneurons in the brain were shown to inhibit excitatory transmission through GABA B receptor astrocytic and presynaptic activation ( Shen et al., 2022 ). Thus, these small molecules hold promise as pain assessment biomarkers, reflecting dysfunction in pain processing at different levels (transmission, modulation, perception, etc.), therefore enhancing our comprehension of various chronic pain states and facilitating the development of novel analgesics. The present discovery of miR-181b-5p in OA pain modulation (inhibition of GABAergic transmission) and the recognized involvement of the miR-181 family in OA structural alterations will require further clarification in the future. Research into the specific mechanisms by which miR-101b-5p influences pain pathways could provide valuable insights into the pathophysiology of chronic pain, and lead to the development of novel therapeutic strategies. Macroscopic and histological assessments demonstrated moderate lesions in the right stifle of rats in the OA group. From a histological standpoint, chondral lesions, loss of proteoglycans, and cluster formation were the most significant injuries observed in the damaged joint. Hence, MI-RAT model achieved to change the hyaline cartilage to the point where 35.77% of the structure showed irregularities and erosion. Increased cluster formation leads to a dysregulation of cartilage homeostasis, that affects other joint structures, and is more pronounced in mature alterations ( Lotz et al., 2010 ). These alterations, along with the significant loss of proteoglycans–crucial hydrophilic substances enabling the absorption of mechanical impacts–and chondrocytes, typically initiate substantial changes in all articular components, triggering processes associated with OA pain ( Xia et al., 2014 ). Notably, there is a remarkable consistency in stifle histological alterations, static QST, and targeted neuropeptides spinal concentration between the current study and our previous one ( Otis et al., 2023 ), employing the same MI-RAT OA model. This underscores its excellent reproducibility and validity. The differences in miRNA expression found in OA subjects do not necessarily reflect the extent of cartilage degeneration, but perhaps some other aspects of joint pathology ( Kung et al., 2017 ). Thus, the structure/function (including pain) correlation in OA would require further investigations, particularly for epigenetics considering that miR-181a appears to be involved in OA cartilage degradation ( Song et al., 2013 ; Nakamura et al., 2016 ; Nakamura et al., 2019 ). A serial assessment of joint structural alteration developed in this MI-RAT model in relation to pain functional behaviors and measures of spinal targeted pain neuropeptides as well as joint and spinal epigenetics would precise the structure/function inter-relationship. Too often, experimental rodent models use focused on molecular and pathophysiological joint structural changes as indicators of successful intervention, but without investigating pain behavior. The refinement of the MI-RAT OA model, including standardized procedures (peri-operative analgesia, anesthesia, surgical procedure, enrichment, calibrated exercise, behavioral outcome measures) with the emergence of QST applications in animals, as well as neuropeptidomic and epigenetic biomarkers bonify the validity of MI-RAT for studying both structural (joint) and neurophysiological changes associated with the OA model. This study demonstrated that OA pain development in the MI-RAT model is strongly linked to centralized sensitization and enhanced EIC activation, evidenced by concurrent changes in pain phenotype, neuropeptidomic and neuroepigenetics biomarkers. Of utmost importance, homogenous joint structural lesions were observed, permitting us to compare other outcomes measures. Functional pain assessments and neuropeptidomic analysis at D56 indicated the development of centralized sensitization, with the CNS gradually gaining control over (hyper)nociceptive inputs despite heightened inflammation signals. The complete functional pain platform included non-evoked mechanical sensitivity (through SWB) waning at D35, as well as evoked static QST (through PWT) persisting up to D56, whereas dynamic QST highlighted the involvement of endogenous facilitatory (through RMTS) and inhibitory (through CPM) controls during the initial timepoints, reaching a peak at D21 and fading at D35. Neuroepigenetic analysis showed elevated spinal expression of miR-181b-5p following inflammatory and nociceptive inputs from stifle joint lesions. Overexpression of spinal miR-181b can repress the GABAergic central inhibitory system. This repression disrupts the balance between excitatory and inhibitory neurotransmission in the spinal cord, potentially contributing to hyperexcitability and enhanced nociceptive signaling, thus exacerbating pain perception and chronic pain conditions. This preliminary study had limitations, including potential interference from the exercise protocol, a limited sample size effect, and the need for additional molecular analysis validation at various time points and tissues in future studies. Nonetheless, the present study highlights the potential use of neuroepigenetic analysis, combined with pain phenotype changes and functional targeted neuropeptide outcomes, to enhance our understanding of constituents in OA pain mechanisms and advance translational research. 4 Materials and methods4.1 ethics statement. Institutional Animal Care and Use Committee of Université de Montréal approved the protocol (#Rech-1766) which was conducted in accordance with principles outlined in the current Guide to the Care and Use of Experimental Animals published by the Canadian Council on Animal Care and the Guide for the Care and Use of Laboratory Animals published by the US National Institutes of Health. 4.2 AnimalsThe study was conducted on adult ovariectomized female ( n = 24) Sprague-Dawley rats (Charles-River Laboratories, Saint-Constant, QC, Canada), as previously validated ( Keita-Alassane et al., 2022 ), weighing between 230 and 250 g at the beginning of the study. Rats were housed under regular laboratory conditions and maintained under a 12-h light-dark cycle with food and water provided ad libitum . Animals were randomly divided in two groups: Naïve ( n = 12) and surgically induced OA ( n = 12). Subjects belonging to the same group were paired and caged together. Body weight (g) was obtained weekly. 4.3 Montreal Induction of Rat Arthritis Testing (MI-RAT) model4.3.1 anesthesia and analgesia. On the day of the surgical intervention (D0), rats were placed in an induction box and anesthetized with an isoflurane-O 2 mixture (IsoFlo ® , Abbott Animal Health, Montreal, Québec, Canada). Anesthesia was maintained with a 2% isoflurane-O 2 mixture via a face mask and a non-rebreathing Bain system. After anesthesia induction, a single intramuscular premedication injection of 1.0 mg/kg of Buprenorphine SR™ (Chiron Compounding Pharmacy Inc., Guelph, ON, Canada) was administered to provide approximately 72 h of analgesic coverage ( Gervais et al., 2019 ; Keita-Alassane et al., 2022 ; Otis et al., 2023 ). A periarticular block of 0.25% bupivacaine (Marcaine ® , McKesson Canada, St.-Laurent, Québec, Canada) at a dose of 0.05–0.1 mL per stifle (<1 mg/kg) was given at the end of the surgical procedure ( Gervais et al., 2019 ; Keita-Alassane et al., 2022 ; Otis et al., 2023 ). 4.3.2 Surgical OA-inductionAnimals were placed in dorsal recumbency, and their right hind limb was prepared using aseptic techniques. The surgical CCLT – DMM procedure was performed as previously described ( Gervais et al., 2019 ) and validated ( Keita-Alassane et al., 2022 ; Otis et al., 2023 ): skin incision, medial parapatellar arthrotomy, lateral patella luxation, DMM (by transection of the cranio-medial meniscotibial ligament) and CCLT, patellar reduction, and surgical site closure in sequential planes. All animals successfully completed the study, and there was no complication following the surgical procedure. 4.3.3 Exercise protocolThe MI-RAT model included a regular exercise protocol, a 10-min running period on a motor-driven treadmill (IITC Life Science Inc., Woodland Hills, CA, United States) for rodents, at a constant speed of 18.3 cm/s, on three non-consecutive days a week for 8 weeks ( Otis et al., 2023 ). This protocol was associated with surgical joint instability, aiming to minimize variability in functional OA pain outcomes and structural joint OA alterations, as observed in the MIA rat model ( Otis et al., 2016 ). 4.4 Functional pain assessmentIt involved biomechanical distribution through static weight-bearing (SWB), a non-evoked behavioral measure of musculoskeletal pain, and a somatosensory profile using a QST protocol recognized as a behavioral expression of nociceptive sensitization ( Cruz-Almeida and Fillingim, 2013 ). Rats were acclimatized to the evaluation environment at D–14, D–7, D–5, and D–3, as per previous rat validation studies ( Otis et al., 2016 ; Keita-Alassane et al., 2022 ; Otis et al., 2023 ). One day before OA induction, baseline values for functional assessments were established. Assessments were repeated at D7, D14, D21, D35, D49, D56. Observers (female) remained completely blinded to OA induction and the experimental design and operated during daylight ( Otis et al., 2016 ). 4.4.1 Static weight-bearing (SWB)An Incapacitance Meter ® (IITC Life Science Inc., Woodland Hills, CA, United States) was employed to assess SWB distribution between the right and left hind limbs ( Otis et al., 2016 ; Otis et al., 2017 ; Otis et al., 2023 ). The weight (force) applied by the animal for each hind limb was measured in grams but expressed as a percentage of total body weight (%BW) to normalize the data for each animal before calculating the asymmetry index value. Measurements were obtained over a 3-s period simultaneously for each limb, and triplicate readings were taken at each timepoint. Contralateral report (SWB asymmetry index) was assessed by calculating the weight report from the ipsilateral to the contralateral hind limb, as follows: 4.4.2 Quantitative sensory testing (QST)Static QST assesses the sensory threshold or the rating of a single stimulus ( Arendt-Nielsen and Yarnitsky, 2009 ). In this study, it involved secondary mechanical pain sensitivity using an electronic von Frey Esthesiometer ® with a propylene probe Rigid Tip ® of 0.7 mm 2 surface, 28G (IITC Life Sciences Inc., Woodland Hills, CA, United States) to determine the paw withdrawal threshold (PWT) of each hind paw. Static QST was performed as described previously ( Otis et al., 2016 ; Otis et al., 2017 ; Otis et al., 2023 ). The peak force was recorded in grams, and a cut-off value was set at 100 g. Both hind paws were evaluated alternately, and triplicate measures were taken for each, with 60-s intervals between stimuli for each animal. Dynamic QST assesses the response to a number of stimuli ( Arendt-Nielsen and Yarnitsky, 2009 ; Guillot et al., 2014 ; Mackey et al., 2017 ), providing the opportunity to investigate the central processing of incoming nociceptive signals ( Guillot et al., 2014 ; Mackey et al., 2017 ). In this experiment, dynamic QST was evaluated by measuring the response to mechanical temporal summation (RMTS) and conditioned pain modulation (CPM). The RMTS was assessed by inducing repeated sub-threshold intensity mechanical stimuli (TopCat Metrology Ltd., Cambs, United Kingdom) previously validated in OA cats ( Guillot et al., 2014 ). The mechanical stimulation, set at a predetermined and steady 2N intensity (0.4 Hz), was applied through a hemispherical-ended metallic pin (2.5 mm diameter, 10 mm length) mounted on a rolling diaphragm actuator, adapted from a validated mechanical threshold testing system ( Dixon et al., 2010 ). The mechanical stimulator was positioned on the rat’s back and secured by a narrow strap passing under the thorax just behind the front limbs. Animals had freedom of movement in the cage before each session and during testing. Normal behavior of the rat wearing the device in the cage was observed for 5 min. Sessions were stopped by the evaluator as soon as clear disagreeable reaction was observed (e.g., vocalization, agitation, biting at the band) or when the cut-off number ( n = 30) was reached and noted as the number of stimuli. Each assessment included a description of the rat’s behavior. Due to its preliminary validation, RMTS was measured at a limited number of timepoints (Baseline, D21, D35, and D56). The CPM paradigm serves as a psychophysical experimental measure of the pain endogenous inhibitory pathway ( Yarnitsky et al., 2010 ), associated with diffuse noxious inhibitory control (DNIC) in humans ( Youssef et al., 2016 ), originally demonstrated in rats ( Le Bars et al., 1979 ). It involves the application of a conditioning stimulus (CS) to decrease pain perception following an initial noxious stimulus ( Mackey et al., 2017 ). Dysfunction of the descending EIC was shown in dogs with primary bone cancer using CPM ( Monteiro et al., 2018 ). In rats, the functional CPM PWT rate was measured with a dynamic CS induced by clipping the left ear with a curved Bulldog serrifine clamp (50 mm in length, duration of 1 min) before performing a second static QST (post-CS). The difference (delta) of post-CS minus pre-CS was used to calculate the CPM rate. Functionality of the CPM response was determined as looking at positive responders to CS. Individual CPM rate was calculated as follows: A rat was considered as a positive responder if its CPM rate in ipsilateral hind PWT was higher than 100% (considered as no change) during the follow-up (post-OA induction) at each timepoint. 4.5 Molecular analysis4.5.1 euthanasia and spinal cord collection. After the final functional evaluation day (D56), euthanasia was carried out by transection of the cervical spine using a guillotine following a 4%–5% isoflurane overdose. Immediately after decapitation, the entire spinal cord was collected using a saline flush technique ( Ferland et al., 2011 ; Otis et al., 2016 ; Otis et al., 2017 ; Gervais et al., 2019 ; Otis et al., 2019 ; Keita-Alassane et al., 2022 ; Otis et al., 2023 ). Samples were quickly snap-frozen in cold hexane, stored individually, and kept at −80°C for subsequent neuroepigenetic (half samples) and neuropeptidomic (half samples) analyses. 4.5.2 Neuropeptidomic analysisAll chemicals were obtained from Sigma-Aldrich (Oakville, ON, Canada), unless specifically indicated. Tissue processing is a crucial step in preserving neuropeptides from in situ degradation ( Beaudry, 2010 ). Rat spinal cords ( n = 6 in each group) were individually weighed precisely, homogenized, and processed as previously described ( Otis et al., 2019 ; Keita-Alassane et al., 2022 ; Otis et al., 2023 ). Peptides were then extracted using a standard C18 solid-phase extraction protocol as also published formerly ( Otis et al., 2019 ). Quantification of extracted neuropeptides from spinal cord homogenate was achieved by mass spectrometry coupled with a liquid chromatography system. Chromatography was performed using a gradient mobile phase along with a microbore column Thermo Biobasic C18 100 × 1 mm, with a particle size of 5 μm (Vanquish FLEX UHPLC ® system, Thermo Scientific, San Jose, CA, United States) as described previously ( Otis et al., 2019 ; Otis et al., 2023 ). Mass spectrometry detection was performed using a Q-Exactive Orbitrap Mass Spectrometer ® (Thermo Scientific, San Jose, CA, United States) interfaced with an UltiMate 3000 ® Rapid Separation UHPLC system using a pneumatic-assisted heated electrospray ion source. Peptide quantification for SP, CGRP, BK, and SST was determined using stable isotope labelled internal standard peptides and expressed in fmol/mg of spinal cord homogenate, as previously described ( Ferland et al., 2011 ; Otis et al., 2016 ; Otis et al., 2017 ; Otis et al., 2019 ; Keita-Alassane et al., 2022 ; Otis et al., 2023 ). 4.5.3 miRNA analysis: RNA extraction, miRNA screening and real-time quantitative polymerase chain reaction (RT-qPCR) assaysTotal RNA was extracted from (30 mg lumbar portion) rat spinal cord samples (L5-S1) using miRCURY™ RNA isolation kit for tissues (#300115, Exiqon Inc., Woburn, MA, United States). Manufacturer protocol was followed except for the elution step that was collected in 35 μL. Quantification and quality control for total RNA samples was acquired using the total RNA Nanochip assay on an Agilent 210 ® Bioanalyzer (Agilent Technologies Inc., Santa Clara, CA, United States). MiRNA selection was based on a literature review englobing original studies about (miRNA OR microRNA) AND (chronic pain OR osteoarthritis OR osteoarthrosis OR degenerative joint disease) AND (chronic pain OR nociceptive OR inflammatory OR neuropathic OR cancer OR cancerous) AND (spinal cord OR central nervous system). Fourteen miRNAs were chosen, based on their yet existing or potential role in pain, for expression screening in all spinal cord samples ( n = 6 in each group). Conditions and tissues in which selected miRNA has been described are briefly presented in Table 2 with respective references. TaqMan ® MicroRNA Reverse Transcription Kit (#4366596, Applied Biosystems, Carlsbad, CA, United States) was used for reverse transcription of total RNA samples and TaqMan ® MicroRNA Assays kit (#4427975) was used with TaqMan ® Fast Advanced Master Mix (#4444556) for RT-qPCR amplification following manufacturer’s instructions. Expression levels were normalized using miR-191 as reference, as suggested by TaqMan ® technical guide, since its expression has been reported as consistent across several tissues ( Peltier and Latham, 2008 ; Schwarzenbach et al., 2015 ), and found as not different in both groups. Fold change of miRNA expression was calculated on obtained comparative cycle threshold (Ct) RT-qPCR ratios which were calculated with an efficiency correction using the Pfaffl Method ( Pfaffl, 2001 ). 4.6 Structural joint evaluationRight stifle joints from OA ( n = 12) and Naïve ( n = 6) rats were collected and dissected free of muscle immediately following sacrifice at D56. The stifle joints were fixed in 10% formaldehyde solution (pH 7.4) for at least 3 days. 4.6.1 Evaluation of macroscopic lesionsExamination of the right stifle for morphological changes was performed by two independent observers under blinded conditions as previously described ( Fernandes et al., 1995 ). Macroscopic lesions of medial and lateral aspects of femoral condyles and tibial plateaus were characterized based on the surface area (size) of articular surface changes which were measured (ImageJ, U. S. National Institutes of Health, Bethesda, Maryland, United States), and expressed in mm 2 . For each compartment, the macroscopic cartilage lesions size was reported on the total compartment size and expressed in percentage of cartilage alteration. The total score was emitted as the mean (standard error of the mean), median (min-max) of the four compartments for both groups. 4.6.2 Histological analysisJoint tissues were decalcified and embedded in paraffin for histological evaluation. Serial sections were cut with a thickness of 5 μm for each stifle after a hematoxylin and eosin, or Safranin-O/Fast Green staining. Medial and lateral femoral condyles, as well as medial and lateral tibial plateaus were analyzed. Articular lesions were graded on a scale using a table modified from Mankin’s score (mMs) ( Colombo et al., 1983 ; Gerwin et al., 2010 ; Otis et al., 2023 ) by an independent observer blinded to the study. Severity of lesions ranged from 0 to 25 for each of the four compartments of the stifle. Structural changes were scored from 0 (normal) to 10 (highest surface irregularities) to assess chondral lesions; Safranin-O staining was evaluated to identify proteoglycan loss with a scale from 0 (no loss of staining) to 6 (loss of staining in all the articular cartilage by more than 50%); clusters formation was evaluated from a range of 0 (no cluster formation) to 3 (more than 8 clusters); and loss of chondrocytes was scored on 0 (normal) to 6 (diffuse loss of chondrocytes) scale. The sum of all four compartment scores was calculated (maximum 100) and was expressed in percentage of cartilage alterations ( Otis et al., 2023 ). 4.7 Statistical analysisAll statistical analyses were conducted using IBM ® SPSS ® Statistics Server version 26.0 (New York City, NY, United States), with a threshold alpha set at 5% for inferential analysis. First, the data averages of the three trials for SWB and PWT were calculated for each subject. Then, data from functional pain outcomes (SWB, static and dynamic QST) were analyzed using general linear mixed models for repeated measures ( Otis et al., 2016 ; Otis et al., 2017 ; Otis et al., 2019 ; Keita-Alassane et al., 2022 ; Otis et al., 2023 ), and the normality of the outcomes residuals was verified using the Shapiro-Wilk test. The groups, days and their interactions (day x group) were considered as fixed effects, with baseline measurement as covariate and using type-3 regressive covariance structure. Data are presented as the estimate mean (least square mean (LSM)) with 95% confidence limits (inferior and superior). The number of CPM positive responders was analysed by using a Fisher’s exact test. Neuropeptides, epigenetic, macroscopic and histological joint data were analyzed using the nonparametric two-sided Mann-Whitney-Wilcoxon test. Data availability statementThe datasets presented in this study can be found in an online repository: https://data.mendeley.com/datasets/j9ky4cpdrn/12 . Ethics statementThe animal study was approved by Comité d’éthique et d’utilisation des animaux (CEUA), Faculty of veterinary medicine, Université de Montréal. The study was conducted in accordance with the local legislation and institutional requirements. Author contributionsCO: Conceptualization, Data curation, Formal Analysis, Investigation, Methodology, Software, Validation, Writing–original draft, Writing–review and editing. K-AC: Data curation, Formal Analysis, Investigation, Methodology, Software, Validation, Visualization, Writing–original draft, Writing–review and editing. MF: Investigation, Writing–review and editing. AD: Investigation, Writing–review and editing. JM-P: Conceptualization, Writing–review and editing. J-PP: Conceptualization, Funding acquisition, Writing–review and editing. FB: Data curation, Methodology, Writing–review and editing. BL: Data curation, Methodology, Writing–review and editing. AB: Conceptualization, Formal Analysis, Methodology, Validation, Writing–review and editing. ET: Conceptualization, Formal Analysis, Funding acquisition, Methodology, Project administration, Resources, Supervision, Validation, Writing–original draft, Writing–review and editing. The author(s) declare that financial support was received for the research, authorship, and/or publication of this article. This work was sponsored, in part, by Discovery grants (#RGPIN 441651–2013; #RGPIN 05512–2020 ET – #RGPIN 386637–2010; #RGPIN-2015–05071 FB) supporting salaries, and a Collaborative Research and Development grant (#RDCPJ 491953–2016; ET, in partnership with ArthroLab Inc.) supporting operations and salaries, from the Natural Sciences and Engineering Research Council of Canada, as well as by an ongoing New Opportunities Fund grant (#9483; ET), a Leader Opportunity Fund grant (#24601; ET), supporting pain/function equipment from the Canada Foundation for Innovation, and the Chair in Osteoarthritis of the Université de Montréal (JM-P and J-PP). Francis Beaudry laboratory equipment was funded by the Canadian Foundation for Innovation (Leader Opportunity Fund grant #36706) and the Fonds de Recherche du Québec (FRQ). Francis Beaudry is the holder of the Canada Research Chair in metrology of bioactive molecules and target discovery (grant #CRC-2021-00160). AcknowledgmentsThe authors would like to thank the FANI of Université de Montréal personnel for their professionalism and support. Conflict of interestThe authors declare that the research was conducted in the absence of any commercial or financial relationships that could be construed as a potential conflict of interest. The Reviewer NA declared a shared committee group ( Non-Human Pain Special Interest Group of the IASP) with the author ET to the handling Editor. Publisher’s noteAll claims expressed in this article are solely those of the authors and do not necessarily represent those of their affiliated organizations, or those of the publisher, the editors and the reviewers. Any product that may be evaluated in this article, or claim that may be made by its manufacturer, is not guaranteed or endorsed by the publisher. Aldossary, S. A., Alsalem, M., and Grubb, B. D. (2024). Role of bradykinin and prostaglandin EP4 receptors in regulating TRPV1 channel sensitization in rat dorsal root ganglion neurons. Basic Clin. Pharmacol. Toxicol. 134 (3), 345–360. doi:10.1111/bcpt.13967 PubMed Abstract | CrossRef Full Text | Google Scholar Aldrich, B. T., Frakes, E. P., Kasuya, J., Hammond, D. L., and Kitamoto, T. (2009). Changes in expression of sensory organ-specific microRNAs in rat dorsal root ganglia in association with mechanical hypersensitivity induced by spinal nerve ligation. Neuroscience 164 (2), 711–723. doi:10.1016/j.neuroscience.2009.08.033 Arant, K. R., Katz, J. N., and Neogi, T. (2022). Quantitative sensory testing: identifying pain characteristics in patients with osteoarthritis. Osteoarthr. Cartil. 30 (1), 17–31. doi:10.1016/j.joca.2021.09.011 CrossRef Full Text | Google Scholar Arendt-Nielsen, L., and Yarnitsky, D. (2009). Experimental and clinical applications of quantitative sensory testing applied to skin, muscles and viscera. J. Pain 10 (6), 556–572. doi:10.1016/j.jpain.2009.02.002 Bai, G., Ambalavanar, R., Wei, D., and Dessem, D. (2007). Downregulation of selective microRNAs in trigeminal ganglion neurons following inflammatory muscle pain. Mol. Pain 3, 15. doi:10.1186/1744-8069-3-15 Bali, K. K., Selvaraj, D., Satagopam, V. P., Lu, J., Schneider, R., and Kuner, R. (2013). Genome-wide identification and functional analyses of microRNA signatures associated with cancer pain. EMBO Mol. Med. 5 (11), 1740–1758. doi:10.1002/emmm.201302797 Barve, R. A., Minnerly, J. C., Weiss, D. J., Meyer, D. M., Aguiar, D. J., Sullivan, P. M., et al. (2007). Transcriptional profiling and pathway analysis of monosodium iodoacetate-induced experimental osteoarthritis in rats: relevance to human disease. Osteoarthr. Cartil. 15 (10), 1190–1198. doi:10.1016/j.joca.2007.03.014 Beaudry, F. (2010). Stability comparison between sample preparation procedures for mass spectrometry-based targeted or shotgun peptidomic analysis. Anal. Biochem. 407 (2), 290–292. doi:10.1016/j.ab.2010.08.017 Bhalala, O. G., Pan, L., Sahni, V., McGuire, T. L., Gruner, K., Tourtellotte, W. G., et al. (2012). microRNA-21 regulates astrocytic response following spinal cord injury. J. Neurosci. 32 (50), 17935–17947. doi:10.1523/JNEUROSCI.3860-12.2012 Buldys, K., Gornicki, T., Kalka, D., Szuster, E., Biernikiewicz, M., Markuszewski, L., et al. (2023). What do we know about nociplastic pain? Healthc. (Basel) 11 (12), 1794. doi:10.3390/healthcare11121794 Chimenti, R. L., Frey-Law, L. A., and Sluka, K. A. (2018). A mechanism-based approach to physical therapist management of pain. Phys. Ther. 98 (5), 302–314. doi:10.1093/ptj/pzy030 Colombo, C., Butler, M., Hickman, L., Selwyn, M., Chart, J., and Steinetz, B. (1983). A new model of osteoarthritis in rabbits. II. Evaluation of anti-osteoarthritic effects of selected antirheumatic drugs administered systemically. Arthritis Rheum. 26 (9), 1132–1139. doi:10.1002/art.1780260911 Cruz-Almeida, Y., and Fillingim, R. B. (2013). Can quantitative sensory testing move us closer to mechanism-based pain management? Pain Med. 15 (5), 61–72. doi:10.1111/pme.12230 Dixon, M. J., Taylor, P. M., Slingsby, L., Hoffmann, M. V., Kastner, S. B. R., and Murrell, J. (2010). A small, silent, low friction, linear actuator for mechanical nociceptive testing in veterinary research. Lab. Anim. 44, 247–253. doi:10.1258/la.2010.009080 Dobson, G. P., Letson, H. L., Grant, A., McEwen, P., Hazratwala, K., Wilkinson, M., et al. (2018). Defining the osteoarthritis patient: back to the future. Osteoarthr. Cartil. 26 (8), 1003–1007. doi:10.1016/j.joca.2018.04.018 Dou, C., Zhang, C., Kang, F., Yang, X., Jiang, H., Bai, Y., et al. (2014). MiR-7b directly targets DC-STAMP causing suppression of NFATc1 and c-Fos signaling during osteoclast fusion and differentiation. Biochim. Biophys. Acta 1839 (11), 1084–1096. doi:10.1016/j.bbagrm.2014.08.002 Eitner, A., Hofmann, G. O., and Schaible, H. G. (2017). Mechanisms of osteoarthritic pain. Studies in humans and experimental models. Front. Mol. Neurosci. 10, 349. doi:10.3389/fnmol.2017.00349 Ferland, C. E., Pailleux, F., Vachon, P., and Beaudry, F. (2011). Determination of specific neuropeptides modulation time course in a rat model of osteoarthritis pain by liquid chromatography ion trap mass spectrometry. Neuropeptides 45 (6), 423–429. doi:10.1016/j.npep.2011.07.007 Fernandes, J. C., Martel-Pelletier, J., Otterness, I. G., Lopez-Anaya, A., Mineau, F., Tardif, G., et al. (1995). Effects of tenidap on canine experimental osteoarthritis. I. Morphologic and metalloprotease analysis. Arthritis and Rheumatism 38 (9), 1290–1303. doi:10.1002/art.1780380918 Ferreira, J., Campos, M. M., Araújo, R., Bader, M., Pesquero, J. B., and Calixto, J. B. (2002). The use of kinin B1 and B2 receptor knockout mice and selective antagonists to characterize the nociceptive responses caused by kinins at the spinal level. Neuropharmacology 43 (7), 1188–1197. doi:10.1016/s0028-3908(02)00311-8 Fitzcharles, M. A., Cohen, S. P., Clauw, D. J., Littlejohn, G., Usui, C., and Hauser, W. (2021). Nociplastic pain: towards an understanding of prevalent pain conditions. Lancet 397 (10289), 2098–2110. doi:10.1016/S0140-6736(21)00392-5 Fu, K., Robbins, S. R., and McDougall, J. J. (2018). Osteoarthritis: the genesis of pain. Rheumatol. Oxf. 57 (Suppl. 4), iv43–iv50. doi:10.1093/rheumatology/kex419 GBD (2018). Global, regional, and national incidence, prevalence, and years lived with disability for 354 diseases and injuries for 195 countries and territories, 1990-2017: a systematic analysis for the Global Burden of Disease Study 2017. Lancet 392, 1789–1858. doi:10.1016/S0140-6736(18)32279-7 Gervais, J. A., Otis, C., Lussier, B., Guillot, M., Martel-Pelletier, J., Pelletier, J. P., et al. (2019). Osteoarthritic pain model influences functional outcomes and spinal neuropeptidomics: a pilot study in female rats. Can. J. Veterinary Res. 83 (2), 133–141. PubMed Abstract | Google Scholar Gerwin, N., Bendele, A. M., Glasson, S. S., and Carlson, C. S. (2010). The OARSI histopathology initiative - recommendations for histological assessments of osteoarthritis in the rat. Osteoarthr. Cartil. 18 (Suppl. 3), S24–S34. doi:10.1016/j.joca.2010.05.030 Gold, M. S., and Gebhart, G. F. (2010). Nociceptor sensitization in pain pathogenesis. Nat. Med. 16 (11), 1248–1257. doi:10.1038/nm.2235 Golmakani, H., Azimian, A., and Golmakani, E. (2024). Newly discovered functions of miRNAs in neuropathic pain: transitioning from recent discoveries to innovative underlying mechanisms. Mol. Pain 20, 17448069231225845. doi:10.1177/17448069231225845 Guillot, M., Taylor, P. M., Rialland, P., Klinck, M. P., Martel-Pelletier, J., Pelletier, J.-P., et al. (2014). Evoked temporal summation in cats to highlight central sensitization related to osteoarthritis-associated chronic pain: a preliminary study. PLoS ONE 9 (5), e97347. doi:10.1371/journal.pone.0097347 Harvey, V. L., and Dickenson, A. H. (2009). Behavioural and electrophysiological characterisation of experimentally induced osteoarthritis and neuropathy in C57Bl/6 mice. Mol. Pain 5 (18), 18. doi:10.1186/1744-8069-5-18 Hummel, M., and Whiteside, G. T. (2017). Measuring and realizing the translational significance of preclinical in vivo studies of painful osteoarthritis. Osteoarthr. Cartil. 25 (3), 376–384. doi:10.1016/j.joca.2016.08.007 Iliopoulos, D., Malizos, K. N., Oikonomou, P., and Tsezou, A. (2008). Integrative microRNA and proteomic approaches identify novel osteoarthritis genes and their collaborative metabolic and inflammatory networks. PLoS ONE 3 (11), e3740. doi:10.1371/journal.pone.0003740 Im, H. J., Kim, J. S., Li, X., Kotwal, N., Sumner, D. R., van Wijnen, A. J., et al. (2010). Alteration of sensory neurons and spinal response to an experimental osteoarthritis pain model. Arthritis Rheum. 62 (10), 2995–3005. doi:10.1002/art.27608 Keita-Alassane, S., Otis, C., Bouet, E., Guillot, M., Frezier, M., Delsart, A., et al. (2022). Estrogenic impregnation alters pain expression: analysis through functional neuropeptidomics in a surgical rat model of osteoarthritis. Naunyn Schmiedeb. Arch. Pharmacol. 395 (6), 703–715. doi:10.1007/s00210-022-02231-5 Klinck, M. P., Mogil, J. S., Moreau, M., Lascelles, B. D. X., Flecknell, P. A., Poitte, T., et al. (2017). Translational pain assessment: could natural animal models be the missing link? Pain 158 (9), 1633–1646. doi:10.1097/j.pain.0000000000000978 Kohno, T., Wang, H., Amaya, F., Brenner, G. J., Cheng, J. K., Ji, R. R., et al. (2008). Bradykinin enhances AMPA and NMDA receptor activity in spinal cord dorsal horn neurons by activating multiple kinases to produce pain hypersensitivity. J. Neurosci. 28 (17), 4533–4540. doi:10.1523/JNEUROSCI.5349-07.2008 Kung, L. H. W., Zaki, S., Ravi, V., Rowley, L., Smith, M. M., Bell, K. M., et al. (2017). Utility of circulating serum miRNAs as biomarkers of early cartilage degeneration in animal models of post-traumatic osteoarthritis and inflammatory arthritis. Osteoarthr. Cartil. 25 (3), 426–434. doi:10.1016/j.joca.2016.09.002 Kusuda, R., Cadetti, F., Ravanelli, M. I., Sousa, T. A., Zanon, S., De Lucca, F. L., et al. (2011). Differential expression of microRNAs in mouse pain models. Mol. Pain 7, 17. doi:10.1186/1744-8069-7-17 Kynast, K. L., Russe, O. Q., Moser, C. V., Geisslinger, G., and Niederberger, E. (2013). Modulation of central nervous system-specific microRNA-124a alters the inflammatory response in the formalin test in mice. Pain 154 (3), 368–376. doi:10.1016/j.pain.2012.11.010 Le Bars, D., Dickenson, A. H., and Besson, J. M. (1979). Diffuse noxious inhibitory controls (DNIC). I. Effects on dorsal horn convergent neurones in the rat. Pain 6 (3), 283–304. doi:10.1016/0304-3959(79)90049-6 Li, X., Gibson, G., Kim, J. S., Kroin, J., Xu, S., van Wijnen, A. J., et al. (2011). MicroRNA-146a is linked to pain-related pathophysiology of osteoarthritis. Gene 480 (1–2), 34–41. doi:10.1016/j.gene.2011.03.003 Li, X., Kroin, J. S., Kc, R., Gibson, G., Chen, D., Corbett, G. T., et al. (2013). Altered spinal microRNA-146a and the microRNA-183 cluster contribute to osteoarthritic pain in knee joints. J. Bone Min. Res. 28 (12), 2512–2522. doi:10.1002/jbmr.2002 Little, C. B., and Smith, M. M. (2008). Animal models of osteoarthritis. Curr. Rheumatol. Rev. 4 (3), 175–182. doi:10.2174/157339708785133523 Lotz, M. K., Otsuki, S., Grogan, S. P., Sah, R., Terkeltaub, R., and D'Lima, D. (2010). Cartilage cell clusters. Arthritis Rheum. 62 (8), 2206–2218. doi:10.1002/art.27528 Lu, M. C. (2023). Regulatory RNAs in rheumatology: from pathogenesis to potential therapy. Int. J. Rheum. Dis. 26 (4), 605–606. doi:10.1111/1756-185X.14615 Lu, Y., Xu, X., Dong, R., Sun, L., Chen, L., Zhang, Z., et al. (2019). MicroRNA-181b-5p attenuates early postoperative cognitive dysfunction by suppressing hippocampal neuroinflammation in mice. Cytokine 120, 41–53. doi:10.1016/j.cyto.2019.04.005 Lutz, B. M., Bekker, A., and Tao, Y. X. (2014). Noncoding RNAs: new players in chronic pain. Anesthesiology 121 (2), 409–417. doi:10.1097/ALN.0000000000000265 Mackey, I. G., Dixon, E. A., Johnson, K., and Kong, J. T. (2017). Dynamic quantitative sensory testing to characterize central pain processing. J. Vis. Exp. 120, e54452. doi:10.3791/54452 Malfait, A. M., and Schnitzer, T. J. (2013). Towards a mechanism-based approach to pain management in osteoarthritis. Nat. Rev. Rheumatol. 9 (11), 654–664. doi:10.1038/nrrheum.2013.138 McDonald, M. K., and Ajit, S. K. (2015). MicroRNA biology and pain. Prog. Mol. Biol. Transl. Sci. 131, 215–249. doi:10.1016/bs.pmbts.2014.11.015 Meini, S., and Maggi, C. A. (2008). Knee osteoarthritis: a role for bradykinin? Inflamm. Res. 57 (8), 351–361. doi:10.1007/s00011-007-7204-1 Mogil, J. S. (2017). Laboratory environmental factors and pain behavior: the relevance of unknown unknowns to reproducibility and translation. Lab. Anim. (NY) 46 (4), 136–141. doi:10.1038/laban.1223 Monteiro, B. P., de Lorimier, L. P., Moreau, M., Beauchamp, G., Blair, J., Lussier, B., et al. (2018). Pain characterization and response to palliative care in dogs with naturally-occurring appendicular osteosarcoma: an open label clinical trial. PLoS ONE 13 (12), e0207200. doi:10.1371/journal.pone.0207200 Monteiro, B. P., Klinck, M. P., Moreau, M., Guillot, M., Steagall, P. V., Edge, D. K., et al. (2016). Analgesic efficacy of an oral transmucosal spray formulation of meloxicam alone or in combination with tramadol in cats with naturally occurring osteoarthritis. Vet. Anaesth. Analg. 43 (6), 643–651. doi:10.1111/vaa.12360 Monteiro, B. P., Klinck, M. P., Moreau, M., Guillot, M., Steagall, P. V., Pelletier, J. P., et al. (2017). Analgesic efficacy of tramadol in cats with naturally occurring osteoarthritis. PLoS ONE 12 (4), e0175565. doi:10.1371/journal.pone.0175565 Mucke, M., Cuhls, H., Radbruch, L., Baron, R., Maier, C., Tolle, T., et al. (2021). Quantitative sensory testing (QST). English version. Schmerz 35 (Suppl. 3), 153–160. doi:10.1007/s00482-015-0093-2 Nakamura, A., Rampersaud, Y. R., Nakamura, S., Sharma, A., Zeng, F., Rossomacha, E., et al. (2019). microRNA-181a-5p antisense oligonucleotides attenuate osteoarthritis in facet and knee joints. Ann. Rheum. Dis. 78 (1), 111–121. doi:10.1136/annrheumdis-2018-213629 Nakamura, A., Rampersaud, Y. R., Sharma, A., Lewis, S. J., Wu, B., Datta, P., et al. (2016). Identification of microRNA-181a-5p and microRNA-4454 as mediators of facet cartilage degeneration. JCI Insight 1 (12), e86820. doi:10.1172/jci.insight.86820 Nakanishi, K., Nakasa, T., Tanaka, N., Ishikawa, M., Yamada, K., Yamasaki, K., et al. (2010). Responses of microRNAs 124a and 223 following spinal cord injury in mice. Spinal Cord. 48 (3), 192–196. doi:10.1038/sc.2009.89 Nepotchatykh, E., Elremaly, W., Caraus, I., Godbout, C., Leveau, C., Chalder, L., et al. (2020). Profile of circulating microRNAs in myalgic encephalomyelitis and their relation to symptom severity, and disease pathophysiology. Sci. Rep. 10 (1), 19620. doi:10.1038/s41598-020-76438-y Nouri, K. H., Osuagwu, U., Boyette-Davis, J., Ringkamp, M., Raja, S. N., and Dougherty, P. M. (2018). “Neurochemistry of somatosensory and pain processing,” in Essentials of pain medicine . Editors H. Benzon, S. N. Raja, S. M. Fishman, S. S. Liu, and S. P. Cohen ( Elsevier Inc ). Orlova, I., Alexander, G. M., Qureshi, R., Sacan, A., Graziano, A., Barrett, J. E., et al. (2011). MicroRNA modulation in complex regional pain syndrome. J. Transl. Med. 9, 195. doi:10.1186/1479-5876-9-195 Otis, C., Bouet, E., Keita-Alassane, S., Frezier, M., Delsart, A., Guillot, M., et al. (2023). Face and predictive validity of MI-RAT ( m ontreal i nduction of r at a rthritis t esting), a surgical model of osteoarthritis pain in rodents combined with calibrated exercise. Int. J. Mol. Sci. 24 (2), 16341. doi:10.3390/ijms242216341 Otis, C., Gervais, J., Guillot, M., Gervais, J. A., Gauvin, D., Pethel, C., et al. (2016). Concurrent validity of different functional and neuroproteomic pain assessment methods in the rat osteoarthritis monosodium iodoacetate (MIA) model. Arthritis Res. Ther. 18, 150. doi:10.1186/s13075-016-1047-5 Otis, C., Guillot, M., Moreau, M., Martel-Pelletier, J., Pelletier, J. P., Beaudry, F., et al. (2017). Spinal neuropeptide modulation, functional assessment and cartilage lesions in a monosodium iodoacetate rat model of osteoarthritis. Neuropeptides 65, 56–62. doi:10.1016/j.npep.2017.04.009 Otis, C., Guillot, M., Moreau, M., Pelletier, J. P., Beaudry, F., and Troncy, E. (2019). Sensitivity of functional targeted neuropeptide evaluation in testing pregabalin analgesic efficacy in a rat model of osteoarthritis pain. Clin. Exp. Pharmacol. Physiol. 46 (8), 723–733. doi:10.1111/1440-1681.13100 Pan, Z., Zhu, L. J., Li, Y. Q., Hao, L. Y., Yin, C., Yang, J. X., et al. (2014). Epigenetic modification of spinal miR-219 expression regulates chronic inflammation pain by targeting CaMKIIγ. J. Neurosci. 34 (29), 9476–9483. doi:10.1523/JNEUROSCI.5346-13.2014 Peltier, H. J., and Latham, G. J. (2008). Normalization of microRNA expression levels in quantitative RT-PCR assays: identification of suitable reference RNA targets in normal and cancerous human solid tissues. RNA 14 (5), 844–852. doi:10.1261/rna.939908 Pfaffl, M. W. (2001). A new mathematical model for relative quantification in real-time RT–PCR. Nucleic Acids Res. 29 (9), e45. doi:10.1093/nar.29.9.e45 Pinter, E., Helyes, Z., and Szolcsanyi, J. (2006). Inhibitory effect of somatostatin on inflammation and nociception. Pharmacol. Ther. 112 (2), 440–456. doi:10.1016/j.pharmthera.2006.04.010 Prescott, S. A. (2015). Synaptic inhibition and disinhibition in the spinal dorsal horn. Prog. Mol. Biol. Transl. Sci. 131, 359–383. doi:10.1016/bs.pmbts.2014.11.008 Recchiuti, A., Krishnamoorthy, S., Fredman, G., Chiang, N., and Serhan, C. N. (2011). MicroRNAs in resolution of acute inflammation: identification of novel resolvin D1-miRNA circuits. FASEB J. 25 (2), 544–560. doi:10.1096/fj.10-169599 Rialland, P., Otis, C., de Courval, M. L., Mulon, P. Y., Harvey, D., Bichot, S., et al. (2014a). Assessing experimental visceral pain in dairy cattle: a pilot, prospective, blinded, randomized, and controlled study focusing on spinal pain proteomics. J. Dairy Sci. 97 (4), 2118–2134. doi:10.3168/jds.2013-7142 Rialland, P., Otis, C., Moreau, M., Pelletier, J. P., Martel-Pelletier, J., Beaudry, F., et al. (2014b). Association between sensitisation and pain-related behaviours in an experimental canine model of osteoarthritis. Pain 155 (10), 2071–2079. doi:10.1016/j.pain.2014.07.017 Sakai, A., Saitow, F., Miyake, N., Miyake, K., Shimada, T., and Suzuki, H. (2013). miR-7a alleviates the maintenance of neuropathic pain through regulation of neuronal excitability. Brain 136 (Pt 9), 2738–2750. doi:10.1093/brain/awt191 Sakai, A., and Suzuki, H. (2013). Nerve injury-induced upregulation of miR-21 in the primary sensory neurons contributes to neuropathic pain in rats. Biochem. Biophys. Res. Commun. 435 (2), 176–181. doi:10.1016/j.bbrc.2013.04.089 Schwarzenbach, H., da Silva, A. M., Calin, G., and Pantel, K. (2015). Data normalization strategies for MicroRNA quantification. Clin. Chem. 61 (11), 1333–1342. doi:10.1373/clinchem.2015.239459 Sengupta, J. N., Pochiraju, S., Kannampalli, P., Bruckert, M., Addya, S., Yadav, P., et al. (2013). MicroRNA-mediated GABA Aα-1 receptor subunit down-regulation in adult spinal cord following neonatal cystitis-induced chronic visceral pain in rats. Pain 154 (1), 59–70. doi:10.1016/j.pain.2012.09.002 Shen, W., Li, Z., Tang, Y., Han, P., Zhu, F., Dong, J., et al. (2022). Somatostatin interneurons inhibit excitatory transmission mediated by astrocytic GABA(B) and presynaptic GABA(B) and adenosine A(1) receptors in the hippocampus. J. Neurochem. 163 (4), 310–326. doi:10.1111/jnc.15662 Shi, G., Shi, J., Liu, K., Liu, N., Wang, Y., Fu, Z., et al. (2013). Increased miR-195 aggravates neuropathic pain by inhibiting autophagy following peripheral nerve injury. Glia 61 (4), 504–512. doi:10.1002/glia.22451 Song, J., Lee, M., Kim, D., Han, J., Chun, C. H., and Jin, E. J. (2013). MicroRNA-181b regulates articular chondrocytes differentiation and cartilage integrity. Biochem. Biophys. Res. Commun. 431 (2), 210–214. doi:10.1016/j.bbrc.2012.12.133 Tao, Y., Wang, Z., Wang, L., Shi, J., Guo, X., Zhou, W., et al. (2017). Downregulation of miR-106b attenuates inflammatory responses and joint damage in collagen-induced arthritis. Rheumatol. Oxf. 56 (10), 1804–1813. doi:10.1093/rheumatology/kex233 Tramullas, M., Francés, R., de la Fuente, R., Velategui, S., Carcelén, M., García, R., et al. (2018). MicroRNA-30c-5p modulates neuropathic pain in rodents. Sci. Transl. Med. 10 (453), eaao6299. doi:10.1126/scitranslmed.aao6299 von Schack, D., Agostino, M. J., Murray, B. S., Li, Y., Reddy, P. S., Chen, J., et al. (2011). Dynamic changes in the microRNA expression profile reveal multiple regulatory mechanisms in the spinal nerve ligation model of neuropathic pain. PLoS ONE 6 (3), e17670. doi:10.1371/journal.pone.0017670 Wang, H., Kohno, T., Amaya, F., Brenner, G. J., Ito, N., Allchorne, A., et al. (2005). Bradykinin produces pain hypersensitivity by potentiating spinal cord glutamatergic synaptic transmission. J. Neurosci. 25 (35), 7986–7992. doi:10.1523/JNEUROSCI.2393-05.2005 Willemen, H., Huo, X.-J., Mao-Ying, Q.-L., Zijlstra, J., Heijnen, C. J., and Kavelaars, A. (2012). MicroRNA-124 as a novel treatment for persistent hyperalgesia. J. Neuroinflammation 9 (143), 143–210. doi:10.1186/1742-2094-9-143 Woolf, C. J. (1996). Windup and central sensitization are not equivalent. Pain 66 (2–3), 105–108. doi:10.1097/00006396-199608000-00001 Woolf, C. J. (2011). Central sensitization: implications for the diagnosis and treatment of pain. Pain 152 (Suppl. 3), S2–S15. doi:10.1016/j.pain.2010.09.030 Xia, B., Di, C., Zhang, J., Hu, S., Jin, H., and Tong, P. (2014). Osteoarthritis pathogenesis: a review of molecular mechanisms. Calcif. Tissue Int. 95 (6), 495–505. doi:10.1007/s00223-014-9917-9 Yamasaki, K., Nakasa, T., Miyaki, S., Ishikawa, M., Deie, M., Adachi, N., et al. (2009). Expression of MicroRNA-146a in osteoarthritis cartilage. Arthritis Rheum. 60 (4), 1035–1041. doi:10.1002/art.24404 Yarnitsky, D., Arendt-Nielsen, L., Bouhassira, D., Edwards, R. R., Fillingim, R. B., Granot, M., et al. (2010). Recommendations on terminology and practice of psychophysical DNIC testing. Eur. J. Pain 14 (4), 339. doi:10.1016/j.ejpain.2010.02.004 Youssef, A. M., Macefield, V. G., and Henderson, L. A. (2016). Pain inhibits pain; human brainstem mechanisms. Neuroimage 124 (Pt A), 54–62. doi:10.1016/j.neuroimage.2015.08.060 Yu, B., Zhou, S., Wang, Y., Ding, G., Ding, F., and Gu, X. (2011). Profile of microRNAs following rat sciatic nerve injury by deep sequencing: implication for mechanisms of nerve regeneration. PLoS ONE 6 (9), e24612. doi:10.1371/journal.pone.0024612 Zhao, C., Huang, C., Weng, T., Xiao, X., Ma, H., and Liu, L. (2012). Computational prediction of MicroRNAs targeting GABA receptors and experimental verification of miR-181, miR-216 and miR-203 targets in GABA-A receptor. BMC Res. Notes 5 (91), 91–98. doi:10.1186/1756-0500-5-91 Zhou, L., Tang, X., Li, X., Bai, Y., Buxbaum, J. N., and Chen, G. (2019). Identification of transthyretin as a novel interacting partner for the delta subunit of GABAA receptors. PLoS ONE 14 (1), e0210094. doi:10.1371/journal.pone.0210094 Keywords: miRNA, epigenetic, musculoskeletal, chronic nociplastic pain, quantitative sensory testing Citation: Otis C, Cristofanilli K-A, Frezier M, Delsart A, Martel-Pelletier J, Pelletier J-P, Beaudry F, Lussier B, Boyer A and Troncy E (2024) Predictive and concurrent validity of pain sensitivity phenotype, neuropeptidomics and neuroepigenetics in the MI-RAT osteoarthritic surgical model in rats. Front. Cell Dev. Biol. 12:1400650. doi: 10.3389/fcell.2024.1400650 Received: 13 March 2024; Accepted: 23 July 2024; Published: 08 August 2024. Reviewed by: Copyright © 2024 Otis, Cristofanilli, Frezier, Delsart, Martel-Pelletier, Pelletier, Beaudry, Lussier, Boyer and Troncy. This is an open-access article distributed under the terms of the Creative Commons Attribution License (CC BY). The use, distribution or reproduction in other forums is permitted, provided the original author(s) and the copyright owner(s) are credited and that the original publication in this journal is cited, in accordance with accepted academic practice. No use, distribution or reproduction is permitted which does not comply with these terms. *Correspondence: Eric Troncy, [email protected] Disclaimer: All claims expressed in this article are solely those of the authors and do not necessarily represent those of their affiliated organizations, or those of the publisher, the editors and the reviewers. Any product that may be evaluated in this article or claim that may be made by its manufacturer is not guaranteed or endorsed by the publisher. ![]() Evaluation of the Reliability and Validity of the Retirement Knowledge Scale (RKS)Luisa r. blanco ron d. hays.
Share this article
Cite this article
Latest issueLog in to access this content or Request a Demo Related Topics
![]() |
IMAGES
VIDEO
COMMENTS
Reliability is about the consistency of a measure, and validity is about the accuracy of a measure.opt. It's important to consider reliability and validity when you are creating your research design, planning your methods, and writing up your results, especially in quantitative research. Failing to do so can lead to several types of research ...
Validity. Validity is defined as the extent to which a concept is accurately measured in a quantitative study. For example, a survey designed to explore depression but which actually measures anxiety would not be considered valid. The second measure of quality in a quantitative study is reliability, or the accuracy of an instrument.In other words, the extent to which a research instrument ...
Quantitative research is used to investigate and analyze data to draw meaningful conclusions. Validity and reliability are two critical concepts in quantitative analysis that ensure the accuracy and consistency of the research results. Validity refers to the extent to which the research measures what it intends to measure, while reliability ...
validity and reliability in quantitative r esearch 2707 make their research healthier and mor e meaningful, it would be beneficial for researchers to state experts ' comments on the specified ...
3 Predictive validity —means that the instrument. should have high correlations with futur e criterions. For example, a score of high self-ef ficacy related to. performing a task should predict ...
Revised on 10 October 2022. Reliability and validity are concepts used to evaluate the quality of research. They indicate how well a method, technique, or test measures something. Reliability is about the consistency of a measure, and validity is about the accuracy of a measure. It's important to consider reliability and validity when you are ...
As with validity, reliability is an attribute of a measurement instrument - for example, a survey, a weight scale or even a blood pressure monitor. But while validity is concerned with whether the instrument is measuring the "thing" it's supposed to be measuring, reliability is concerned with consistency and stability.
Critical Analysis of Reliability and Validity in Literature Reviews. Go to citation Crossref Google Scholar Pub Med. ... Quantitative Research for the Qualitative Researcher. 2014. SAGE Knowledge. Book chapter . Issues in Validity and Reliability. Show details Hide details. Daniel J. Boudah.
Reliability and validity are criteria by which researchers assess measurement quality. Measuring a person or item involves assigning scores to represent an attribute. This process creates the data that we analyze. However, to provide meaningful research results, that data must be good.
In quantitative research, you have to consider the reliability and validity of your methods and measurements. ... Reliability vs. Validity in Research | Difference, Types and Examples Reliability is about a method's consistency, and validity is about its accuracy. You can assess both using various types of evidence.
In an era of data-driven decision-making, a comprehensive understanding of quantitative research is indispensable. Current guides often provide fragmented insights, failing to offer a holistic view, while more comprehensive sources remain lengthy and less accessible, hindered by physical and proprietary barriers.
Reliability and validity are among the most important and fundamental domains in the assessment of any measuring methodology for data-collection in a good research. Validity is about what an instrument measures and how well it does so, whereas reliability concerns the truthfulness in the data obtained and the degree to which any measuring tool ...
the studies. In quantitative research, this is achieved through measurement of the validity and reliability.1 Validity Validity is defined as the extent to which a concept is accurately measured in a quantitative study. For example, a survey designed to explore depression but which actually measures anxiety would not be consid-ered valid.
What is the difference between reliability and validity in a study? In the domain of research, whether qualitative or quantitative, two concepts often arise when discussing the quality and rigor of a study: reliability and validity.These two terms, while interconnected, have distinct meanings that hold significant weight in the world of research.
Validity is a test that shows an instrument's accuracy, logic, and relevance for measuring variables in a quantitative study (Cypress, 2017; Heale & Twycross, 2015). Based on this background, the ...
Reliability is a key concept in research that measures how consistent and trustworthy the results are. In this article, you will learn about the four types of reliability in research: test-retest, inter-rater, parallel forms, and internal consistency. You will also find definitions and examples of each type, as well as tips on how to improve reliability in your own research.
Reliability refers to the consistency of the measurement. Reliability shows how trustworthy is the score of the test. If the collected data shows the same results after being tested using various methods and sample groups, the information is reliable. If your method has reliability, the results will be valid. Example: If you weigh yourself on a ...
Validity and reliability in quantitative studies Evid Based Nurs. 2015 Jul;18(3):66-7. doi: 10.1136/eb-2015-102129. Epub 2015 May 15. Authors Roberta Heale 1 , Alison Twycross 2 Affiliations 1 School of Nursing, Laurentian University, Sudbury, Ontario ...
Several different ways to measure reliability—inter-rater reliability, equivalency reliability, and internal consistency—and validity—face, construct, internal, and external validities—are presented. The chapter concludes with two examples of planning research studies that exemplify the concepts of reliability and validity.
Test-retest reliability, inter-rater reliability, internal consistency reliability: Content validity, criterion validity, construct validity: Measure: Degree of agreement or correlation between repeated measures or observers: Degree of association between a measure and an external criterion, or degree to which a measure assesses the intended ...
Statistical conclusion validity is an issue whenever statistical tests are used to test hypotheses. The research design can address threats to validity through. considerations of statistical power. alpha reduction procedures (e.g., Bonferoni technique) when multiple tests are used. use of reliable instruments.
Reproducibility of Results. Research Design / standards*. Research Personnel / psychology. This article examines reliability and validity as ways to demonstrate the rigour and trustworthiness of quantitative and qualitative research. The authors discuss the basic principles of reliability and validity for readers who are new to research.
This flexibility is unheard of in quantitative research. But even though it's as flexible as an Olympic gymnast, qualitative data can be less reliable—and harder to validate. #7. Reliability and Validity. Quantitative data is more reliable than qualitative data. Numbers can't be massaged to fit a certain bias.
Validity is defined as the extent to which a measure or concept is accurately measured in a study. In essence, it is how well a test or piece of research measures what it is intended to measure. In quantitative studies, there are two broad measurements of validity - internal and external. Internal validity is an estimate of the degree to ...
49) and given, in addition. to generalization, "the status of a scientific. holy trinity" (Kvale, 2002, p. 300). Validity. and reliability originated from quantitative. research, which follows ...
1 Research Group in Animal Pharmacology of Quebec (GREPAQ), Université de Montréal, Saint-Hyacinthe, ... this work aims to advance the development of quantitative sensory testing (QST) of pain in association with neuropeptidomics and neuroepigenetics. ... in order to characterize OA pain with great validity and reliability. The results ...
Quantitative Finance; Regulation, Taxation, Governance, and Compliance; Economics and Financial History; Asset Classes; Journals. All Journals; The Journal of Portfolio Management ; The Journal of Investing; The Journal of Alternative Investments; The Journal of Financial Data Science; The Journal of Impact and ESG Investing ; The Journal of ...