🚀 Chart your career path in cutting-edge domains.
Talk to a career counsellor for personalised guidance 🤝.

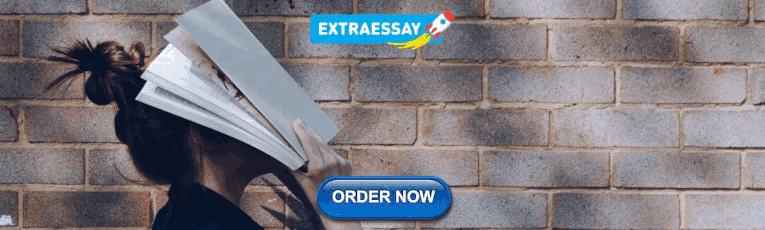
100+ Real-Life Examples of Reinforcement Learning And It's Challenges
The blog features diverse case studies in reinforcement learning, showcasing its practical applications. These studies highlight how reinforcement learning algorithms enable machines to learn and make decisions by interacting with their environment.
From robotics and gaming to recommendation systems, each case study demonstrates the power of reinforcement learning in optimizing actions and achieving desired outcomes. With real-world examples, the blog illustrates the versatility and potential of reinforcement learning in revolutionizing various industries and solving complex problems through intelligent decision-making.
Table of Content
What are machine learning and reinforcement learning.
- Why has the need for Reinforcement Learning rise?
Supply Chain
Agriculture, autonomous vehicles, manufacturing, hospitality, advertising, cybersecurity.
- 100+ Real-Life Applications of Reinforcement Learning
Top 6 Challenges for Reinforcement Learning
- Future of Reinforcement Learning
The Global Machine Learning Market Size is expected to reach $302.62 billion by 2030 at a rate of 38.1%.
The convergence of ample data and powerful computing resources facilitates the widespread adoption and growth of machine learning in industries like healthcare, finance, and autonomous systems.
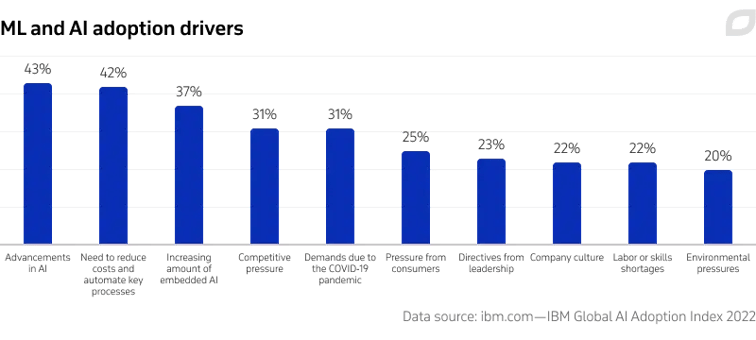
Machine learning (ML) enables computers to carry out certain tasks intelligently by learning from examples or data rather than by following pre-programmed rules, allowing computers to carry out complex procedures. While ML algorithms excel at supervised or unsupervised learning tasks, Reinforcement Learning (RL) is designed to handle sequential decision-making problems where an agent interacts with an environment. RL algorithms learn from trial and error, receiving feedback in the form of rewards or penalties to optimize their behavior over time. Example - Imagine you are playing a game and you want to win. RL is like figuring out the best moves by playing the game over and over again and getting feedback. You learn which actions give you good results (like getting points) and which actions give you bad results (like losing points).
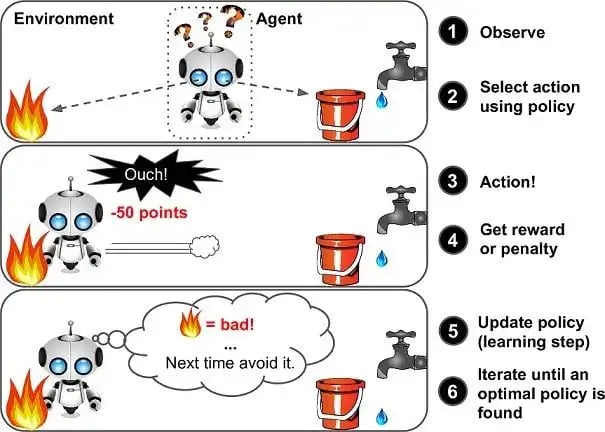
Why is the need for Reinforcement Learning rising?
The need for RL has arisen due to the limitations of traditional machine learning approaches.
While supervised and unsupervised learning techniques are effective in tasks with labeled or unlabeled data, they struggle with problems involving sequential decision-making and dynamic environments. RL addresses these challenges by introducing a framework where an agent learns to make optimal decisions through trial and error interactions with the environment.
RL is particularly valuable in domains where actions have delayed consequences and where an agent must learn to balance short-term rewards with long-term goals. RL empowers machines to adapt and improve their behavior based on feedback, making it a crucial tool for solving complex problems where sequential decision-making and real-time adaptation are necessary.
100+ Real-Life Examples of Reinforcement Learning
Along with RL real world examples, there are perspectives of renowned researchers and experts in the field of Reinforcement Learning. These quotes reflect their insights and expertise on the subject showcasing its potential.
OpenAI Five , learned to play the complex multiplayer online battle game Dota 2 at a high level. It competed against professional human players and showcased advanced strategic decision-making.
DeepMind used RL techniques to train AlphaGo to play the ancient board game Go. By playing millions of games against itself, AlphaGo improved its strategies and went on to defeat world champions, demonstrating the power of RL in mastering complex games.
Project Malmo is an RL platform developed by Microsoft that integrates with the popular game Minecraft. It allows researchers to use RL techniques to train agents within the Minecraft environment. RL agents can learn to navigate, build structures, and interact with the game world, showcasing adaptive and intelligent behavior.
Ubisoft has implemented RL techniques in the development of Assassin's Creed game series. RL algorithms are used to train AI agents that control non-player characters (NPCs) in the game. These AI agents learn to exhibit realistic and diverse behaviors, enhancing the immersion and realism of the game world.
DeepMind 's AI system mastered the real-time strategy game StarCraft II using RL techniques. The RL agent learned to strategize, manage resources, and make tactical decisions to outperform human players.
- Massachusetts General Hospital uses RL to optimize the personalized dosing of blood thinning medications, such as warfarin, for patients. The RL agent learns from patient data to recommend individualized doses, reducing the risk of adverse events and improving treatment outcomes.
- IBM Watson is an RL-based clinical decision support system that assists oncologists in cancer treatment decision-making. It analyzes patient data and medical literature to provide evidence-based treatment recommendations, aiding physicians in creating personalized care plans.
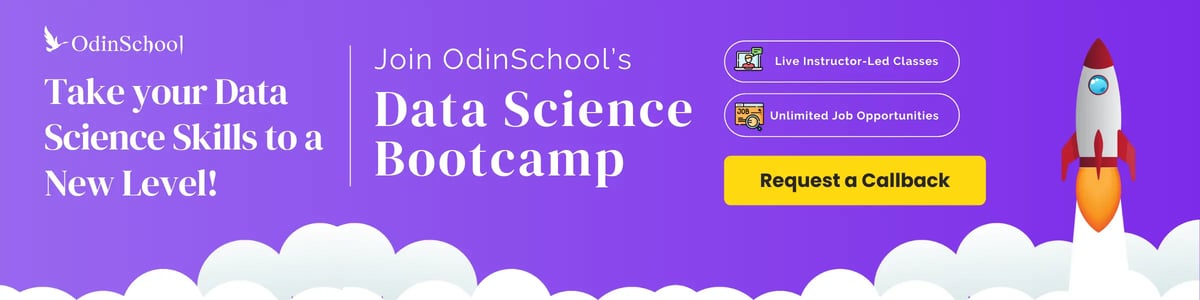
- Google employed RL techniques to develop Flu Trends, a system that uses search queries to monitor and predict flu outbreaks. The RL agent learned from historical flu data to detect patterns and provide real-time estimates of flu activity, assisting in disease monitoring and control efforts.
- Mount Sinai developed an RL-based system to personalize insulin dosing for patients with diabetes. The RL agent learned from patient glucose monitoring data to optimize insulin delivery, resulting in improved glucose control and better management of the disease.
- The da Vinci Surgical System , widely used in robotic-assisted surgeries, employs RL techniques. The RL agent learns from expert surgeon demonstrations to assist surgeons in performing minimally invasive procedures with enhanced precision and dexterity.
Tesco , a multinational retailer, uses RL for assortment planning. RL agents learn from sales data, customer preferences, and market trends to optimize product assortments, ensuring that the right products are available at the right stores, improving customer satisfaction and sales.
Kroger , a grocery store chain, leverages RL to optimize store layouts. The RL agent learns from customer foot traffic patterns, sales data, and product relationships to determine the optimal arrangement of products, improving customer flow and maximizing sales.
Shopify , an e-commerce platform, utilizes RL algorithms for fraud detection. The RL agent learns from historical transaction data and user behavior patterns to identify and prevent fraudulent activities, protecting merchants and customers from financial losses.
Amazon utilizes RL algorithms to dynamically optimize pricing for its products. The RL agent learns from customer behavior, competitor prices, and market conditions to adjust prices in real-time, maximizing revenue and maintaining competitiveness.
Alibaba employs RL techniques to optimize its supply chain operations. RL agents learn from historical data, transportation logistics, and demand forecasts to optimize warehouse operations, inventory allocation, and delivery routes, improving efficiency and reducing costs.
Procter & Gamble (P&G) utilizes RL algorithms to optimize its inventory management. RL agents learn from demand patterns, lead times, and stock levels to determine optimal reorder points and quantities, minimizing stockouts and excess inventory.
UPS utilizes RL algorithms to optimize delivery routes. RL agents learn from real-time traffic data, package volumes, and customer time windows to dynamically adjust route plans, reducing fuel consumption and improving delivery efficiency.
Proximus , a Belgian telecommunications company, uses RL for supplier selection and negotiation. RL agents learn from supplier performance data, pricing models, and contract terms to optimize supplier selection and negotiate favorable agreements.
DHL applies RL techniques in its transportation management operations. RL agents learn from historical shipment data, traffic conditions, and delivery constraints to optimize transport routing, load consolidation, and mode selection, enhancing overall logistics efficiency.
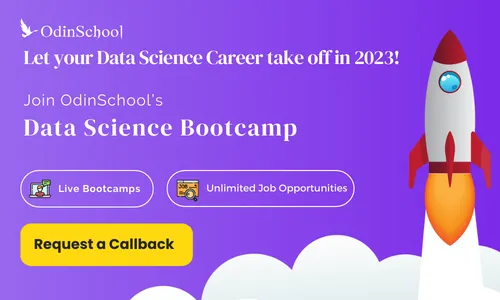
Zara , a global fashion retailer, leverages RL for order fulfillment. RL agents learn from order characteristics, inventory availability, and production capacities to determine optimal sourcing and allocation strategies, ensuring timely order fulfillment.
Cisco employs RL techniques in supply chain risk management. RL agents learn from historical supply chain disruption data, market conditions, and risk indicators to assess and mitigate potential risks, enabling proactive risk management strategies.
Siemens implemented RL algorithms for robotic assembly tasks in manufacturing. RL agents learn to grasp and manipulate objects, perform assembly operations, and adapt to variations in object position and orientation, improving the efficiency and flexibility of robotic assembly lines
Harvard researchers employed RL techniques to coordinate and control a large swarm of small robots called Kilobots. RL agents learn to communicate and collaborate with other Kilobots, self-organizing into desired formations and performing collective tasks.
The ARM-H Robot developed at the University of Cambridge uses RL to adapt to changes in its physical structure. The RL agent learns to control the robot's movements, compensating for changes in joint stiffness or wear, allowing the robot to maintain precise and robust control.
NVIDIA 's Jetson AGX Xavier platform employs RL for autonomous flight control of drones. RL agents learn to navigate and perform complex maneuvers in dynamic environments, such as obstacle avoidance and optimal flight path planning.
OpenAI developed a robotic system called Dactyl that uses RL to learn dexterous manipulation skills. The RL agent learns to control the robot's fingers and manipulate objects through trial and error, achieving impressive levels of object manipulation and fine-grained control.
Fendt's Xaver is a precision fertilizer application system that utilizes RL techniques. RL agents learn from soil nutrient levels, plant growth stages, and field characteristics to optimize fertilizer application rates, reducing fertilizer waste and minimizing environmental impact.
LettUs Grow employs RL techniques for greenhouse climate control. RL agents learn from sensor data, plant growth models, and environmental conditions to optimize factors such as temperature, humidity, and lighting, creating ideal growing conditions and maximizing crop quality.
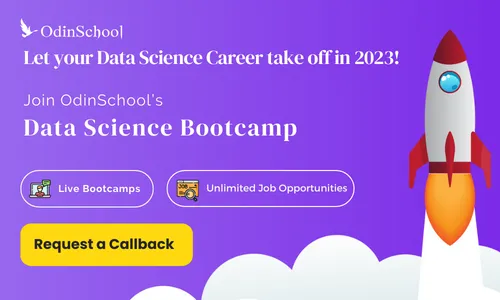
Cargill's Dairy Enteligen platform utilizes RL algorithms for livestock management. RL agents learn from sensor data, animal behavior, and health indicators to optimize feeding schedules, detect anomalies, and improve overall herd health and productivity.
John Deere's GreenON platform utilizes RL algorithms for crop yield optimization. RL agents learn from historical yield data, weather conditions, and field characteristics to generate optimal planting recommendations, maximizing crop yield and profitability.
Bonirob , developed by Deepfield Robotics, utilizes RL algorithms for precision irrigation. RL agents learn from sensor data, crop water requirements, and soil conditions to optimize irrigation scheduling, ensuring efficient water usage and reducing water waste.
American Express employs RL techniques for customer churn prediction. RL agents learn from customer transaction data, usage patterns, and behavior to identify customers at risk of churning, enabling proactive retention strategies and personalized offers.
Uber uses RL algorithms for dynamic pricing of its ride-sharing services. RL agents learn from supply-demand dynamics, traffic conditions, and user behavior to set optimal prices in real-time, maximizing revenue while balancing rider demand and driver availability.
Jump Trading , a proprietary trading firm, utilizes RL in high-frequency trading strategies. RL agents learn from tick-level market data, order book dynamics, and latency considerations to execute trades rapidly and exploit short-term market inefficiencies.
PayPal employs RL algorithms for fraud detection and prevention. RL agents learn from transaction data, user behavior patterns, and fraud indicators to identify suspicious activities, reducing fraudulent transactions and protecting customer accounts.
Citadel Securities , a leading market maker, utilizes RL algorithms in their algorithmic trading strategies. RL agents learn from market data, order book dynamics, and historical trade patterns to make real-time trading decisions, optimizing trade execution and liquidity provision.
LOXM is an RL-based algorithmic trading system developed by JP Morgan. It learns optimal trading strategies, dynamically adjusting trade execution parameters to achieve better performance in stock trading.
- Lemonade an insurance company, uses RL to automate and optimize claims handling processes. The RL agent learns to assess claims, verify information, and process payments efficiently, improving speed and accuracy.
Waymo , a leading autonomous vehicle company, uses RL for self-driving cars. RL agents learn from sensor data, such as cameras and lidar, to make driving decisions like lane keeping, adaptive cruise control, and object detection, improving safety and efficiency.
Tesla 's Autopilot system incorporates RL for collision avoidance. RL agents learn from sensor data and human driver behavior to make real-time decisions, such as emergency braking or evasive maneuvers, to avoid potential collisions on the road.
BMW developed a Remote Valet Parking Assistant using RL. RL agents learn from sensor data, parking lot maps, and vehicle dynamics to autonomously navigate and park the vehicle in tight parking spaces without human intervention.
Lyft employs RL algorithms for optimizing ride-hailing services. RL agents learn from historical demand patterns, traffic conditions, and driver availability to allocate drivers efficiently, reduce wait times, and improve overall service quality.
Roborace is an autonomous racing competition that utilizes RL techniques. RL agents learn from race track data, vehicle dynamics, and optimal racing lines to autonomously control race cars, competing against each other at high speeds.
Wing , a subsidiary of Alphabet, utilizes RL for autonomous delivery drones. RL agents learn from sensor data, airspace regulations, and package delivery requirements to autonomously navigate and deliver packages to specified locations.
Engie , a global energy company, employs RL algorithms for energy trading and pricing. RL agents learn from historical market data, supply-demand dynamics, and price signals to optimize trading strategies, maximize profitability, and manage energy portfolios.
Tesla utilizes RL techniques for energy storage optimization in their Powerpack and Powerwall systems. RL agents learn from electricity price data, demand patterns, and renewable energy generation forecasts to optimize energy storage scheduling, reducing costs and improving grid stability.
Opus One Solutions uses RL algorithms for demand response management. RL agents learn from customer consumption data, grid conditions, and price signals to optimize demand response actions, encouraging customers to adjust their energy usage during peak times and balance grid loads.
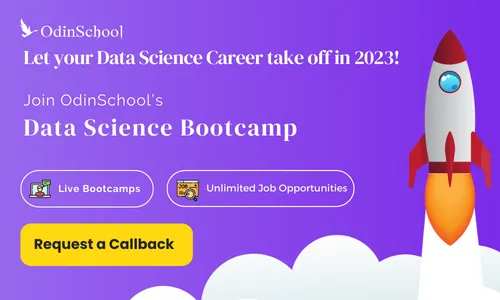
PG&E has implemented RL algorithms for microgrid control. RL agents learn from renewable energy generation, storage capacity, and load profiles to optimize microgrid operations, ensuring efficient energy distribution and minimizing reliance on the main grid.
Vattenfall , a leading European energy company, utilizes RL algorithms for wind farm control. RL agents learn from wind forecasts, turbine characteristics, and grid constraints to optimize turbine operation and power output, maximizing energy generation and grid integration.
ALLEGRO is an RL-based intelligent tutoring system that helps students learn algebra concepts. It adapts to individual student needs, providing personalized instruction, feedback, and exercises based on their performance and learning progress.
ALEKS (Assessment and Learning in Knowledge Spaces): ALEKS is an adaptive learning platform that utilizes RL techniques. It assesses students' knowledge in various subjects, such as math, science, and languages, and provides personalized learning paths based on their strengths and weaknesses. The RL agent continually adjusts the difficulty of the questions and the sequence of topics to optimize the learning experience.
Intelligent Tutoring Systems : RL can be used to develop intelligent tutoring systems that adapt the learning experience based on student performance and progress. The RL agent can adjust the difficulty of the questions, provide personalized hints or feedback, and dynamically generate new learning materials to optimize the student's learning trajectory.
edX , employs its own recommender system to personalize course recommendations for learners. The system considers user preferences, enrollment history, and course interactions to generate relevant suggestions.
DeepMind developed RL algorithms and the DeepMind Controls Suite to optimize industrial control systems. These algorithms learn to control complex systems like robots and machinery to improve efficiency, reduce energy consumption, and minimize defects.
Baxter , developed by Rethink Robotics, is a collaborative robot that has been trained using RL algorithms. It is designed to perform various tasks in manufacturing environments, such as assembly, packaging, and machine tending.
ABB's YuMi robot is another collaborative robot that has been trained using RL techniques. It is designed for assembly and small parts handling applications in manufacturing industries.
Fanuc , a leading robotics company, has applied RL to their industrial robots to improve their performance in various manufacturing tasks, including welding, material handling, and assembly.
Universal Robots ' collaborative robots, UR3, UR5, and UR10, have been trained using RL algorithms. These robots are designed for a wide range of manufacturing applications, such as pick-and-place operations, machine tending, and quality inspection.
KUKA's iiwa robot , a collaborative robot with sensitive touch capabilities, has been trained using RL techniques. It is used in manufacturing for tasks such as assembly, quality control, and material handling.
RL-based Autonomous Bellhop Robot is a hypothetical autonomous robot designed to assist with luggage transportation within hotels. RL algorithms could enable the robot to learn optimal routes, interact with guests, and navigate through complex environments.
A room service cart equipped with sensors and RL algorithms to optimize the delivery route and timing. The system could learn from historical data and feedback to dynamically adjust the delivery process based on factors like guest preferences, room occupancy, and real-time information.
An HVAC system in hotels that utilizes RL techniques to learn and adapt its temperature and airflow settings based on guest comfort and occupancy patterns. The system could optimize energy consumption while maintaining a comfortable environment for guests.
A food preparation system in hotel kitchens that leverages RL algorithms to optimize ingredient selection, cooking times, and recipes based on guest preferences and nutritional requirements. The system could continuously learn and improve its food preparation techniques.
Booking.com applies RL techniques to dynamically adjust hotel room prices based on factors like demand, seasonality, and competitor prices. The RL agent learns optimal pricing strategies to maximize revenue and occupancy rates.
Google's Duplex is an RL-based virtual assistant developed by Google. It can make phone calls to schedule appointments or make reservations on behalf of users, engaging in natural and human-like conversations to accomplish tasks.
ORION is a routing optimization system that uses RL algorithms to optimize package delivery routes. The RL agent learns to consider factors like traffic patterns, delivery time windows, and package prioritization, minimizing distances and improving efficiency.
Wing (owned by Alphabet Inc.) have been developing and deploying RL-based systems for autonomous drone delivery services. RL can be used to train autonomous delivery drones to optimize their flight paths, navigation, and delivery strategies
Fetch Robotics have developed autonomous mobile robots that utilize RL algorithms to optimize order fulfillment processes in warehouses. These robots learn to navigate the warehouse, locate items, and pick and transport them efficiently.
Celect (acquired by Nike) apply RL techniques to optimize pricing and revenue management in logistics. Their systems use RL algorithms to learn from historical sales data, market conditions, and customer preferences to dynamically adjust prices, promotions, and inventory allocation.
FourKites provide intelligent fleet management solutions that leverage RL algorithms. These solutions optimize logistics operations by learning from real-time data on vehicle locations, traffic conditions, and customer demands to optimize route planning, load balancing, and delivery schedules.
Criteo utilize RL algorithms to optimize bidding strategies in programmatic advertising. Their systems learn from historical data and feedback to dynamically adjust bid amounts based on factors such as user profiles, ad placement, and conversion probabilities.
Google's Smart Display Campaigns leverage RL techniques to optimize ad selection and personalization. RL algorithms learn from user interactions and historical data to dynamically choose the most relevant ad creatives, messages, and targeting options for individual users.
Facebook's Ad Placement Optimization (APO) system utilizes RL algorithms to optimize ad placement decisions across its advertising network. The system learns from user interactions, contextual factors, and historical performance data to dynamically select the most effective ad placements to maximize reach, engagement, and conversions.
Content Recommendation Engines are used by RL techniques advertising platforms such as Taboola. These engines learn from user feedback, engagement data, and contextual signals to dynamically recommend relevant content and advertisements to users, optimizing user experience and ad performance.
Intrusion Detection Systems : Companies like Darktrace utilize RL algorithms in their cybersecurity solutions for real-time intrusion detection. RL agents learn from network traffic patterns and system behavior to detect anomalies, identify potential threats, and take proactive measures to mitigate attacks.
Malware Detection : Cybereason's cybersecurity platform leverages RL techniques for malware detection and prevention. RL algorithms analyze patterns and characteristics of known malware to identify and block emerging threats, even without prior knowledge of specific malware signatures.
Adaptive Firewall Managemen t: Companies like Deep Instinct employ RL algorithms to optimize firewall configurations and rule management. RL agents learn from network traffic and attack patterns to dynamically adjust firewall rules and prioritize security policies for more effective protection against evolving threats.
Vulnerability Assessment and Patch Management : RL techniques can be applied to automate vulnerability assessment and patch management processes. Companies like Tenable utilize RL algorithms to analyze vulnerabilities, prioritize patching efforts, and optimize resource allocation for mitigating security risks.
Adaptive Authentication Systems : Adaptive authentication systems, such as those offered by BioCatch, employ RL algorithms to detect and prevent fraudulent activities by continuously learning and adapting to user behavior patterns. RL agents identify anomalies, unauthorized access attempts, and fraudulent activities to strengthen authentication processes.
RoboCup is an international robotics competition that includes a soccer league where teams of autonomous robots compete against each other. RL algorithms have been used by various teams to train their robotic players, with notable examples including the teams from Carnegie Mellon University and the University of Texas.
IBM's SlamTracker is an RL-based system used in tennis. It analyzes historical tennis match data and player statistics to predict the outcomes of future matches. The system employs RL algorithms to continuously learn and improve its predictions.
Catapult Sports , a sports analytics company, developed OptimEye, a wearable device used in various sports, including soccer, rugby, and basketball. OptimEye uses RL algorithms to analyze player movements, acceleration, and other metrics, providing insights to optimize training regimens and prevent injuries.
STRIVR is a company that uses virtual reality (VR) technology to provide immersive training experiences for athletes. By combining RL techniques with VR, STRIVR enables athletes to simulate game scenarios and make decisions in real-time, helping them improve their skills and decision-making abilities.
Sportlogiq is a sports analytics company that applies RL algorithms to analyze video footage of hockey games. Their system tracks player movements, evaluates game situations, and provides insights to coaches and teams, helping them develop effective strategies and improve performance.
Boeing's Autonomous Aerial Refueling : Boeing has been working on an RL-based system called the Autonomous Aerial Refueling (AAR) system. It uses RL algorithms to enable unmanned aircraft to autonomously perform aerial refueling operations, ensuring precise and safe refueling maneuvers.
NASA's Autonomous Systems : NASA has been actively researching RL for autonomous systems in aviation. They have developed RL algorithms to train autonomous drones and aerial vehicles for tasks such as collision avoidance, path planning, and autonomous landing.
Airbus Skywise Predictive Maintenance : Airbus has implemented RL techniques in their Skywise Predictive Maintenance platform. This platform utilizes RL algorithms to analyze aircraft sensor data, historical maintenance records, and operational data to predict component failures and optimize maintenance schedules, reducing maintenance costs and minimizing disruptions.
Thales Autopilot System : Thales, a global aerospace and defense company, has incorporated RL algorithms into their autopilot system. The RL-based autopilot system learns from pilot inputs and flight data to optimize aircraft control, adjust to different flight conditions, and enhance flight performance.
General Electric's Digital Twin Technology : General Electric (GE) utilizes RL in their digital twin technology for aircraft engines. By creating a virtual replica of the engine and using RL algorithms, GE can optimize engine operation, fuel efficiency, and maintenance schedules, leading to improved performance and reduced costs.
These examples demonstrate the broad applicability of RL in various industries, highlighting its potential for optimizing decision-making, automation, and resource management.
Exploration vs. Exploitation : Balancing exploration and exploitation is a fundamental challenge in RL. Agents must explore the environment to learn optimal policies while also exploiting what they have already learned.
example - Imagine a robot learning to navigate a maze. The robot needs to explore different paths to find the exit (exploration), but it also needs to exploit the known paths to reach the goal quickly. Striking the right balance is crucial because the robot may waste time exploring unnecessary paths or get stuck in suboptimal routes if it only exploits known paths.
Sample Efficiency : RL algorithms often require a substantial number of interactions with the environment to learn effective policies. This high sample complexity can be a significant challenge, especially in real-world applications.
example - Suppose an RL algorithm is used to optimize energy usage in a building. Collecting data on energy consumption and environmental factors can be challenging and time-consuming. With limited data, the algorithm may require a long training period to learn effective energy-saving policies. Improving sample efficiency would involve finding ways to make the algorithm learn faster and make better decisions with fewer data samples.
Generalization : RL algorithms often struggle with generalizing their learned policies to unseen situations or environments. The policies that agents learn in specific settings may not transfer well to different contexts, requiring additional training or adaptation.
example - Consider an RL agent trained to play a specific video game level. If the agent is then tested on a new, unseen level with different obstacles and layouts, it may struggle to perform well. The agent needs to generalize its learned strategies and adapt them to the new level, understanding the underlying principles of the game rather than memorizing specific actions for each level.
Credit Assignment : It is often difficult to attribute the success or failure of an episode to specific actions, making it challenging to learn from past experiences and make effective policy updates.
example - Imagine train ing an RL algorithm to control a robot arm in a manufacturing environment. The algorithm needs to learn to perform tasks like picking and placing objects. Determining which specific actions or arm movements led to successful outcomes (e.g., correctly picking up an object) can be challenging, especially when rewards are sparse or delayed.
Safety and Ethics : In RL applications that involve physical systems or have real-world consequences, ensuring safety and ethical behavior is of paramount importance. Guaranteeing safe and ethical behavior throughout the learning process is a complex challenge that requires careful design and monitoring.
Scalability and Complexity : RL faces challenges when scaling to large-scale or high-dimensional problems. As the complexity of the state and action spaces increases, RL algorithms may struggle to explore and learn effectively. Developing scalable algorithms that can handle complex environments efficiently is an ongoing research area.
Addressing these challenges requires continued research and innovation in RL algorithms, exploration of new techniques such as meta-learning and transfer learning, and collaborations between researchers, practitioners, and policymakers to ensure responsible and beneficial deployment of RL systems.
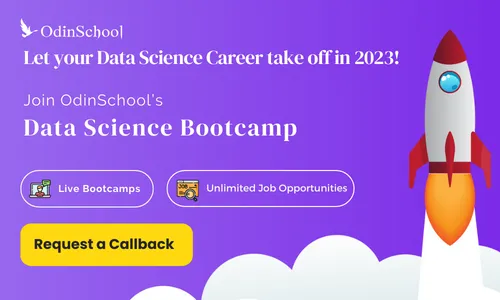
Future of Reinforcement Learning
The future of Reinforcement Learning (RL) holds significant promise as the field continues to advance and find application in various domains. Here are some potential aspects that could shape the future of RL:
Improved Algorithms : Researchers will continue to develop more sophisticated RL algorithms, focusing on areas such as sample efficiency, generalization, and scalability.
Advances in algorithms, such as meta-learning, imitation learning, and hierarchical RL, may enable faster learning, better transferability, and handling of complex problems.
Combination with Other Technologie s: RL will likely be integrated with other emerging technologies, such as deep learning, natural language processing, and computer vision.
Combining RL with these fields can enable more sophisticated and intelligent systems that can understand and interact with the world in a more human-like manner.
Human-AI Collaboration : RL can facilitate human-AI collaboration, where humans and AI systems work together to solve complex problems.
RL algorithms can learn from human demonstrations and feedback, allowing humans to guide and influence the learning process. This collaboration can enhance decision-making, creativity, and problem-solving across multiple domains.
Transfer Learning and Lifelong Learning : RL systems that can transfer knowledge and skills learned in one task to another related task (transfer learning) and adapt to new environments and tasks (lifelong learning) will be of significant interest. These capabilities will enable RL agents to acquire knowledge more efficiently and be adaptable to evolving scenarios.
Multi-Agent RL and Cooperative Systems : The future of RL involves exploring multi-agent settings, where multiple RL agents interact and cooperate to achieve common goals. This can lead to the development of intelligent systems that can collaborate, negotiate, and solve complex tasks in coordination with other agents.
As RL continues to progress, it is expected to have a transformative impact on various aspects of technology, industry, and society, paving the way for intelligent and autonomous systems that can learn, adapt, and make decisions in dynamic and complex environments.
Final Thoughts
Reinforcement Learning is indeed an exciting and valuable area of study, particularly in domains mentioned. With the above examples it is shown how reinforcement learning is evolving every day and is creating endless opportunities. Thus, if one wants to make a career from the reinforcement learning opportunities then it is advisable to join a professional data science course. WHY?
Refer > A Quick Guide to Choosing The Best Data Science Bootcamp for Your Career
Here are a few reasons why joining a data science course can be beneficial:
Comprehensive Skill Development : Data science courses often cover a wide range of topics, including data analysis, machine learning, statistics, and data visualization. These foundational skills are valuable across various domains and provide a well-rounded understanding of data-driven problem-solving.
Diverse Career Opportunities : Data science encompasses various subfields such as machine learning, natural language processing, computer vision, and more. By pursuing a data science course, you gain exposure to these different areas and increase your employability in a broader range of roles.
Fundamental Understanding : Data science courses typically teach the underlying principles and techniques that power RL and other machine learning methods. Having a strong foundation in data science allows you to better understand and apply RL algorithms effectively.
Real-World Applications : While RL has shown promise in areas like robotics and game playing, many real-world applications still rely on other data science techniques. By joining a data science course, you can learn about these techniques and apply them to a wide range of practical problems across industries.
Flexibility and Adaptability : Staying up to date with the latest developments is crucial. By joining a data science course, you can acquire a flexible skill set that allows you to adapt to emerging trends, including RL or other cutting-edge techniques.
If you are looking for a course that helps you achieve your career goals and aspirations, join OdinSchool's Data Science Course .

About the Author
Mechanical engineer turned wordsmith, Pratyusha, holds an MSIT from IIIT, seamlessly blending technical prowess with creative flair in her content writing. By day, she navigates complex topics with precision; by night, she's a mom on a mission, juggling bedtime stories and brainstorming sessions with equal delight.
Related Posts
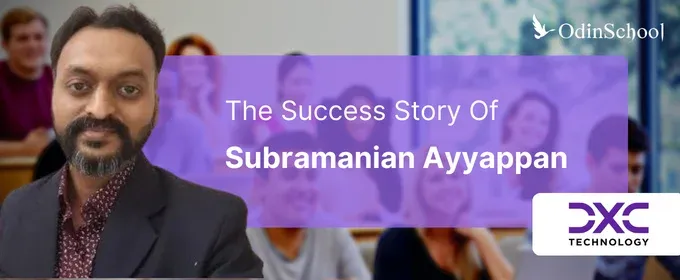
Rebooting at 38: Subramanian's Success Story of Grit and Upskilling
Discover the 15-year career journey filled with courage and adventure of Subramanian Ayyappan!
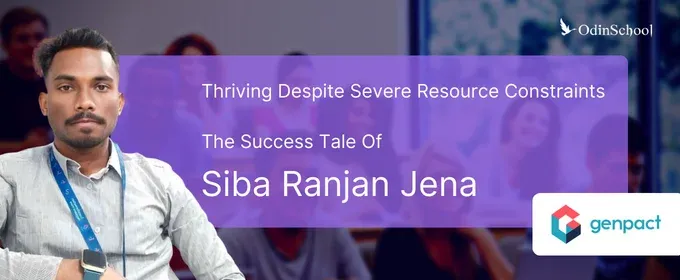
Beyond Expectations: Siba Ranjan's Path to Success at Genpact
Siba Ranjan Jena is now a successful Business Analyst at Genpact .
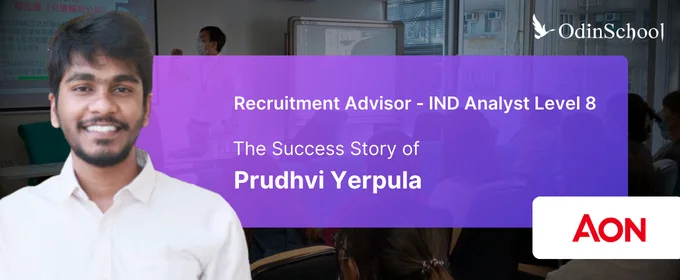
Unlocking Success: AON Analyst's Middle-Class Climb to a 124% Salary Hike!
Discover the importance of structured learning in the pursuit of success in the data science field.
Join OdinSchool's Data Science Bootcamp
With job assistance.

9 Reinforcement Learning Real-Life Applications

“Most human and animal learning can be said to fall into unsupervised learning. It has been wisely said that if intelligence was a cake, unsupervised learning could be the cake, supervised learning would be the icing on the cake, and reinforcement learning would be the cherry on the top.”
It seems intriguing, right?
Reinforcement Learning is the closest to human learning.
Just like we humans learn from the dynamic environment we live in and our actions determine whether we are rewarded or punished, so do Reinforcement Learning agents whose ultimate aim is to maximize the rewards.
Isn’t it what we are looking?
We want the AI agents to be as intelligent and decisive as us.
Reinforcement Learning techniques are the base of all the solutions, from self-driving cars to surgeons being replaced by medical AI bots. It has become the main driver of emerging technologies and, quite frankly, that’s just the tip of the iceberg.
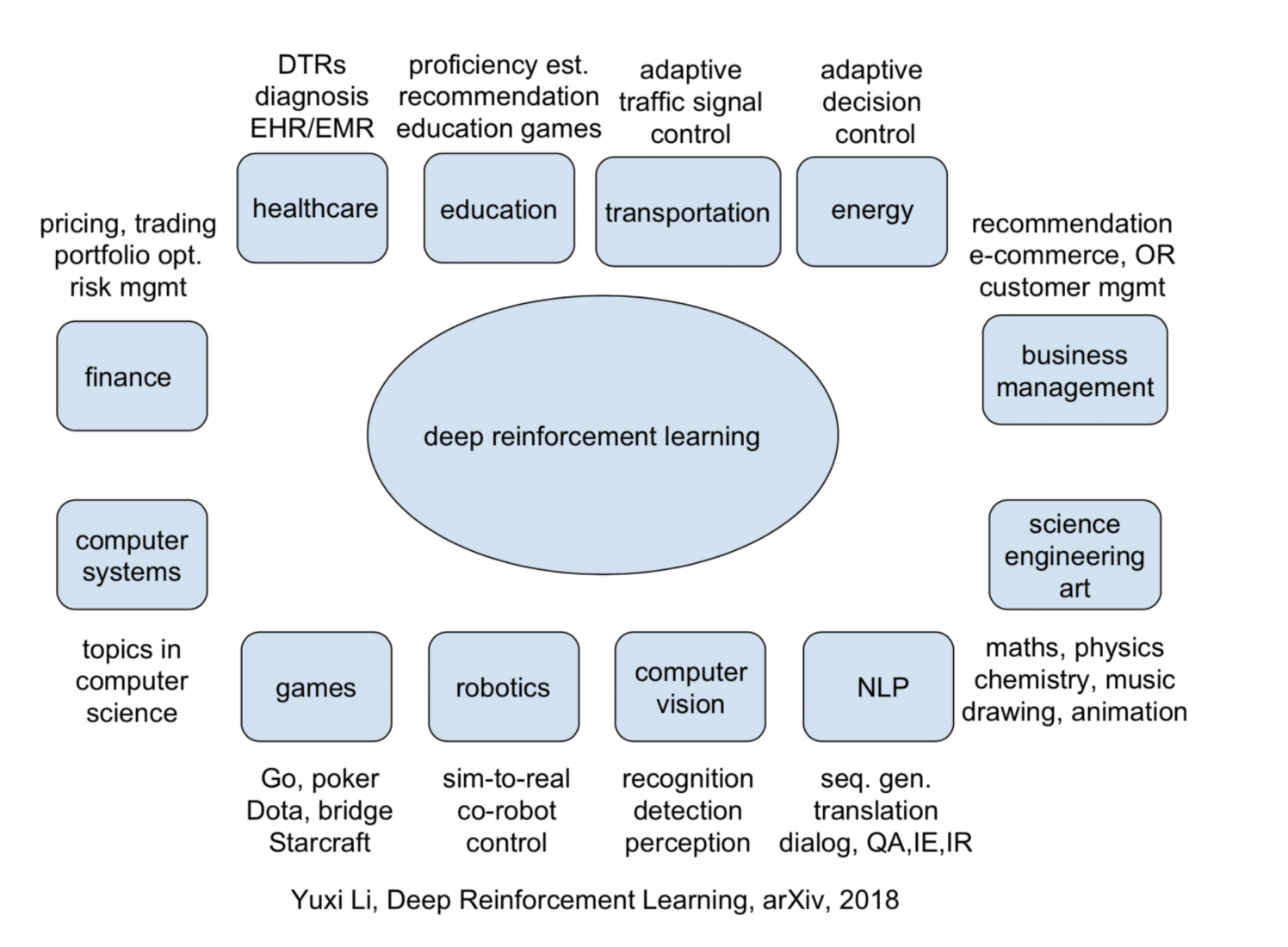
💡 Pro Tip: Read more on Neural Network architecture, which is a major governing factor of the Deep Reinforcement Learning algorithms.
In this article, we’ll discuss ten different Reinforcement Learning applications and learn how they are shaping the future of AI across all industries.
Here’s what we’ll cover:
- Autonomous cars
Datacenters cooling
Traffic light control, image processing.
Use computer vision and LLMs for quality control automation
Ready to streamline AI product deployment right away? Check out:
- V7 Model Training
- V7 Workflows
- V7 Auto Annotation
- V7 Dataset Management
Autonomous cars
Vehicle driving in an open context environment should be backed by the machine learning model trained with all possible scenes and scenarios in the real world.
The collection of these varieties of scenes is a complicated problem to solve. How can we ensure that a self-driving car has already learned all possible scenarios and safely masters every situation?
The answer to this is Reinforcement Learning .
Reinforcement Learning models are trained in a dynamic environment by learning a policy from its own experiences following the principles of exploration and exploitation that minimize disruption to traffic. Self-driving cars have many aspects to consider depending on which it makes optimal decisions.
Driving zones, traffic handling, maintaining the speed limit, avoiding collisions are significant factors.
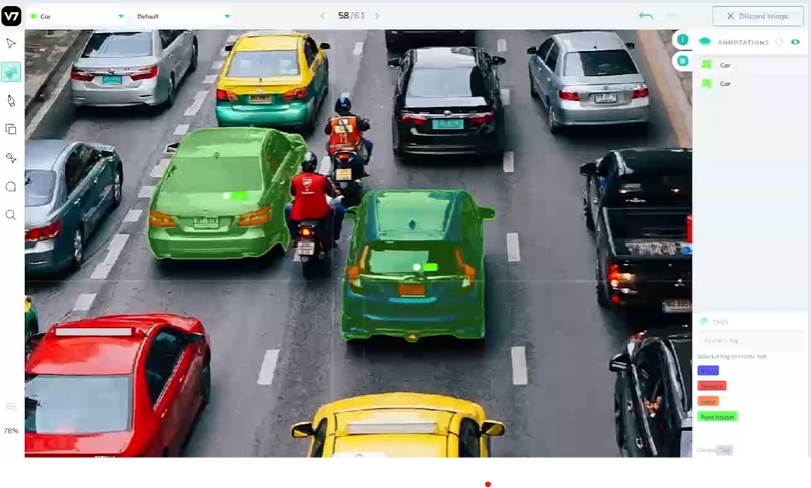
💡 Pro Tip: Have a look at our Open Datasets repository or upload your own multimodal traffic data to V7, annotate it , and train deep Neural Networks in less than an hour!
Many simulation environments are available for testing Reinforcement Learning models for autonomous vehicle technologies.
DeepTraffic is an open-source environment that combines the powers of Reinforcement Learning, Deep Learning , and Computer Vision to build algorithms used for autonomous driving launched by MIT. It simulates autonomous vehicles such as drones, cars, etc.
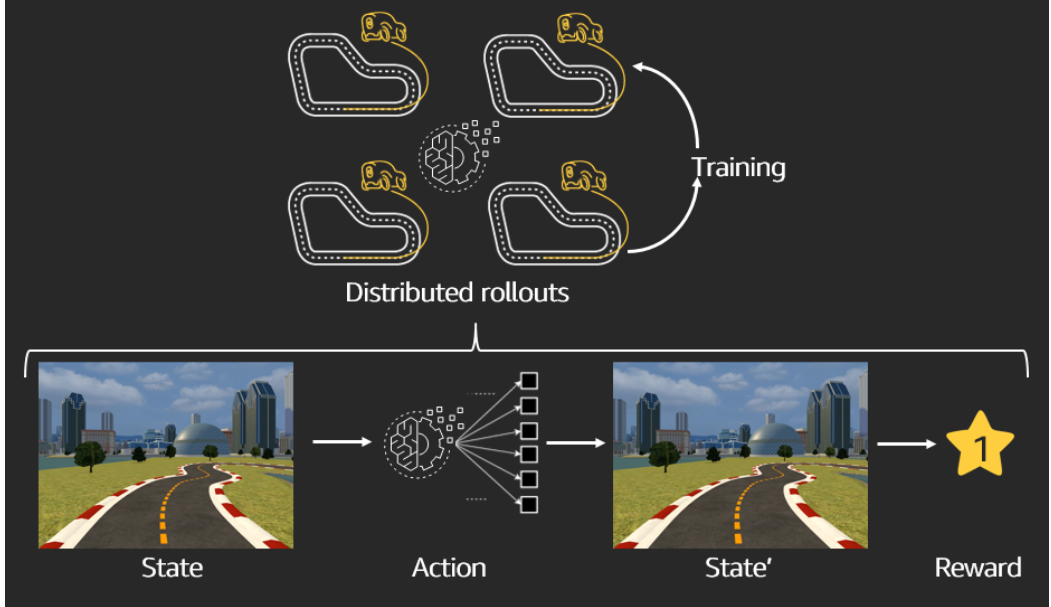
Carla is another excellent alternative that has been developed to support the development, training and validation of autonomous driving systems. It replicates the urban layouts, buildings, vehicles to train the self-driving cars in real-time simulated environments very close to reality.
💡 Pro-tip: Have a look at 27+ Most Popular Computer Vision Applications and Use Cases and start your first Reinforcement learning project.
Autonomous driving uses Reinforcement Learning with the help of these synthetic environments to target the significant problems of Trajectory optimization and Dynamic pathing.
Reinforcement Learning agents are trained in these dynamic environments to optimize trajectories. The agents learn motion planning, route changing, decision and position of parking and speed control, etc.
A paper on Confidence based Reinforcement Learning proposes an effective solution to use Reinforcement Learning with a baseline rule-based policy with a high confidence score.
We are in this era where AI can help us tackle some of the world’s most challenging physical problems—such as energy consumption. With the entire world at the edge of virtualization and cloud-based applications, large-scale commercial and industrial systems like data centers have a large energy consumption to keep the servers running.
Interesting Fact: Google data centers using machine learning algorithms have reduced the amount of energy for cooling by up to 40 percent.
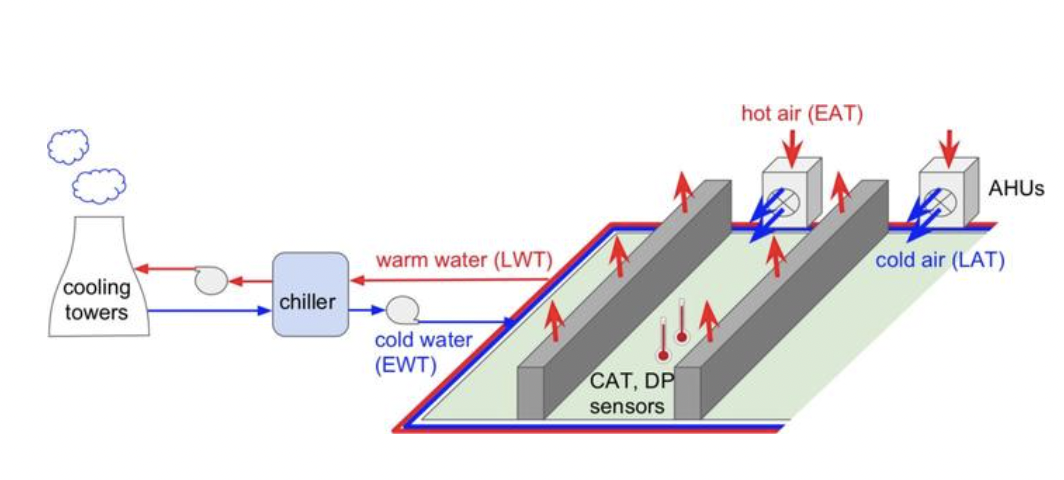
Researchers in this domain have proved that a few hours of exploration enables data-driven, model-based learning.
This approach of a Reinforcement Learning agent with little or no prior knowledge can effectively and safely regulate conditions on a server floor efficiently compared to the existing PID controllers. The data collected by thousands of sensors within the data centers have attributes like temperatures, power, setpoints, etc.—that are fed to be used to train the deep neural networks for datacentre cooling.
Due to the difficulty of directly solving this problem through conventional machine learning algorithms due to the lack of varied datasets, deep Q-learning Network (DQN)- based methods are broadly used to conquer this challenge.
With the increase of urbanization and the increase in the number of cars per household, traffic congestion has become an enormous problem, especially in metropolitan areas.
Reinforcement Learning is a trending data-driven approach for adaptive traffic signal control. These models are trained with the objective of learning a policy using a value function that optimally controls the traffic light based on the current status of the traffic.
The decision-making needs to be dynamic depending upon the arrival rate of traffic from different directions, which ought to vary at different times of the day. The conventional way of handling traffic seems to be limited due to this non-stationary behavior. Also, the policy π trained for an intersection with x lanes cannot be re-used in an intersection with y lanes.
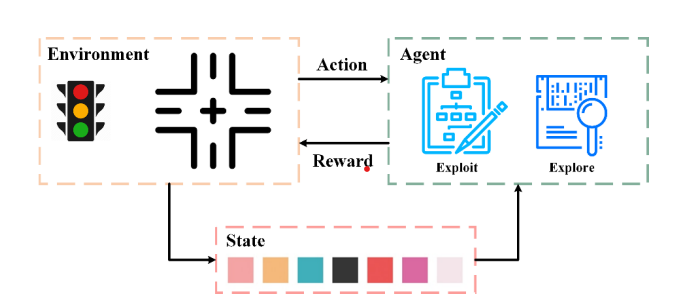
Reinforcement Learning (RL) is a trending approach due to its data-driven nature for adaptive traffic signal control in complex urban traffic networks.
There are some limitations in applying deep Reinforcement Learning algorithms to transportation networks, like an exploration-exploitation dilemma, multi-agent training schemes, continuous action spaces, signal coordination, etc.
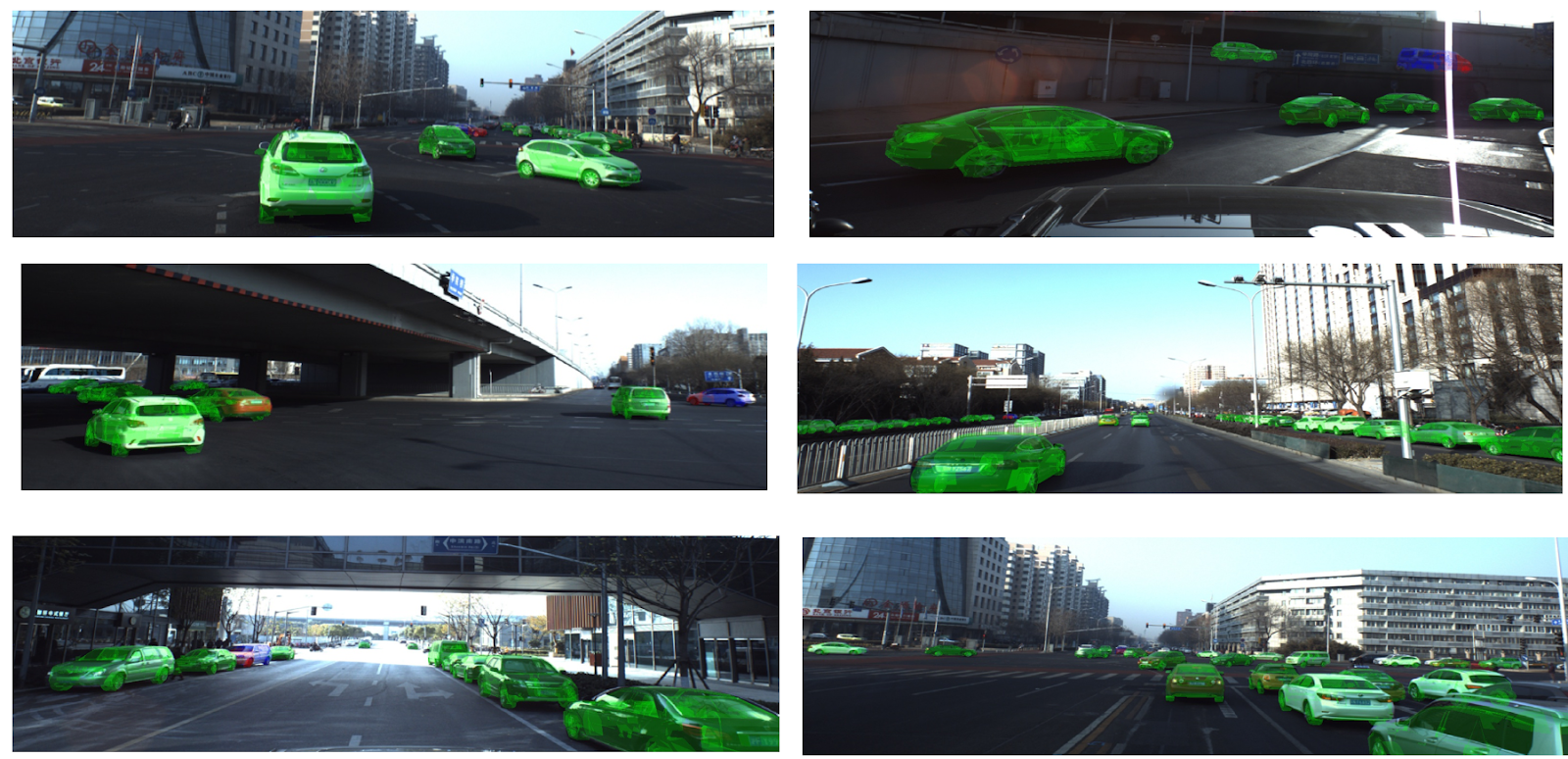
💡 Pro tip: Take a step back and revise the concepts of quality training data to improve your model’s accuracy.
Choosing medicines is hard. It is even more challenging when the patient has been on medication for years, and no improvements have been seen.
Recent research shows that a patient suffering from chronic disease tries different medicines before giving up. We must find the right treatments and map them to the right person.
The healthcare sector has always been an early adopter and a significant beneficiary of technological advancements. This industry has seen a significant tilt towards Reinforcement Learning in the past few years, especially in implementing dynamic treatment regimes ( DTRs ) for patients suffering from long-term illnesses.
It has also found its application in automated medical diagnosis, health resource scheduling, drug discovery and development, and health management.
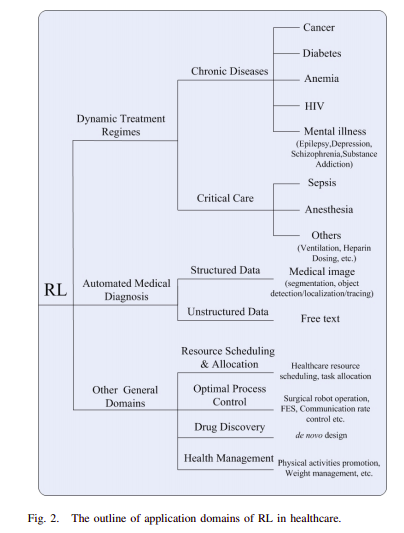
Automated medical diagnosis
Deep Reinforcement Learning (DRL) augments the Reinforcement Learning framework, which learns a sequence of actions that maximizes the expected reward, using deep neural networks' representative power.
Reinforcement Learning has taken over medical report generation, identification of nodules/tumors and blood vessel blockage, analysis of these reports, etc. Refer to this paper for more insights into this problem space and the solutions offered by the Reinforcement Learning approach.
💡 Pro-tip: Have a look at our healthcare datasets for computer vision and start annotating medical data today.
Dtrs (dynamic treatment regimes).
DTRs involve sequential healthcare decisions – including treatment type, drug dosages, and appointment timing – tailored to an individual patient based on their medical history and conditions over time. This input data is fed to the algorithm outputting treatment options to provide the patient’s most desirable environmental state.
The tricky thing is that patients suffering from chronic long-term diseases like HIV develop resistance to drugs, so the drugs need to be switched over time, making the treatment sequence important. When physicians need to adapt treatment for individual patients, they may refer to past trials, systematic reviews, and analyses. However, the specific use-case data may not be available for many ICU conditions.
Many patients admitted to ICUs might also be too ill for inclusion in clinical trials. We need other methods to aid ICU clinical decisions, including sizeable observational data sets. Given the dynamic nature of critically ill patients, one machine learning method called reinforcement learning (RL) is particularly suitable for ICU settings.
Robotic surgeries
A powerful Reinforcement Learning application in decision-making is the use of surgical bots that can minimize errors and any variations and will eventually help increase the surgeons' efficiency. One such robot is Da Vinci , which allows surgeons to perform complex procedures with greater flexibility and control than conventional approaches.
The critical features served are aiding surgeons with advanced instruments, translating hand movements of the surgeons in real-time, and delivering a 3D high-definition view of the surgical area.
Reinforcement Learning is data-intensive and is well-versed in interacting with a dynamic and initially unknown environment. The current solutions offered in Image Processing by supervised and unsupervised neural networks focus more on the classification of the objects identified. However, they do not acknowledge the interdependency among different entities and the deviation from the human perception procedure.
It is used in the following subfields of Image Processing.
Object detection and Localization
The RL approach learns multiple searching policies by maximizing the long-term reward, starting with the entire image as a proposal, allowing the agent to discover multiple objects sequentially.
It offers more diversity in search paths and can find multiple objects in a single feed and generate bounding boxes or polygons. This paper on Active Object Localization with Deep Reinforcement Learning validates its effectiveness.
💡 Pro tip: Check out our guide to YOLO: Real-Time Object Detection.
Scene understanding.
Artificial vision systems based on deep convolutional neural networks consume large, labeled datasets to learn functions that map the sequence of images to human-generated scene descriptions. Reinforcement Learning offers rich and generalizable simulation engines for physical scene understanding.
This paper shows a new model based on pixel-wise rewards (pixelRL) for image processing. In pixelRL, an agent is attached to each pixel responsible for changing the pixel value by taking action. It is an effective learning method that significantly improves the performance by considering the future states of the own pixel and neighbor pixels.
Reinforcement learning is one of the most modern machine learning technologies in which learning is carried out through interaction with the environment. It is used in computer vision tasks like feature detection, image segmentation , object recognition , and tracking .
Here are some other examples where Reinforcement Learning is used in image processing:-
- Robots equipped with visual sensors from which they learn the state of the surrounding environment
- Scanners to understand the text
- Image pre-processing and segmentation of medical images like CT Scans
- Traffic analysis and real-time road processing by video segmentation and frame-by-frame image processing
- CCTV cameras for traffic and crowd analytics etc.
Robots operate in a highly dynamic and ever-changing environment, making it impossible to predict what will happen next. Reinforcement Learning provides a considerable advantage in these scenarios to make the robots robust enough and help acquire complex behaviors adaptively in different scenarios.
It aims to remove the need for time-consuming and tedious checks and replaces them with computer vision systems ensuring higher levels of quality control on the production assembly line.
💡 Pro tip: Read these guides on data cleaning and data preprocessing.
Robots are used in warehouse navigation mainly for part supplies, quality testing, packaging, automizing the complete process in the environment where other humans, vehicles, and devices are also involved.
All these scenarios are complex to handle by the traditional machine learning paradigm. The robot should be intelligent and responsive enough to walk through these complex environments. It is trained to have object manipulation knowledge for grasping objects of different sizes and shapes depending upon the texture and mass of the object embedded with the power of image processing and computer vision.
Let us quickly walk through some of the use-cases in this field of robotics that Reinforcement Learning offers solutions for.
Product assembly
Computer vision is used by multiple manufacturers to help improve their product assembly process and to completely automate this and remove the manual intervention from this entire flow. One central area in the product assembly is object detection and object tracking.
Defect Inspection
A deep Reinforcement learning model is trained using multimodal data to easily identify missing pieces, dents, cracks, scratches, and overall damage, with the images spanning millions of data points .
Using V7’s software, you can train object detection, instance segmentation , and image classification models to spot defects and anomalies.
💡 Pro tip: Learn more about training defect inspection models with V7
Inventory management.
The inventory management in big companies and warehouses has become automated with the inventions in the field of computer vision to track stock in real-time. Deep reinforcement learning agents can locate empty containers, and ensure that restocking is fully optimised.

💡 Pro tip: Want to learn more? Check out AI in manufacturing.
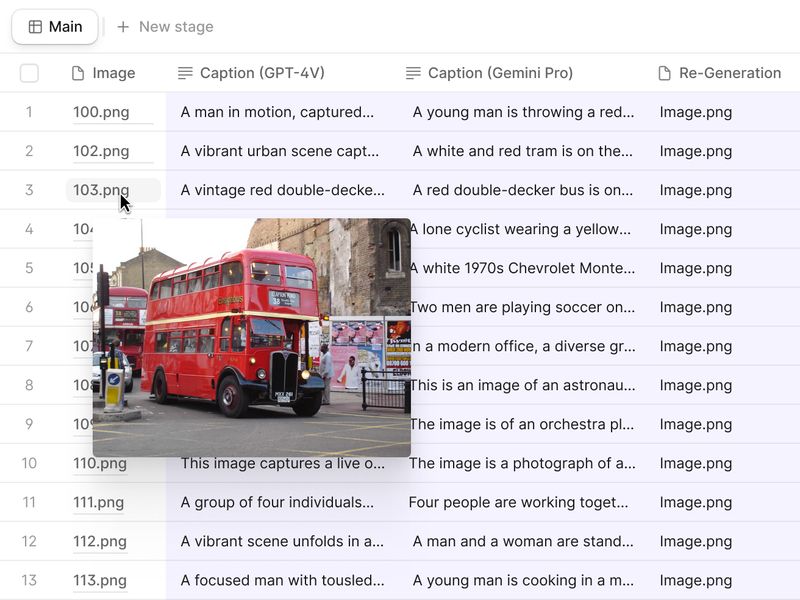
Automate repetitive tasks and complex processes with AI
Language understanding uses Reinforcement Learning because of its inherent nature of decision making. The agent tries to understand the state of the sentence and tries to form an action set maximizing the value it would add.
The problem is complex because the state space is huge; the action space is vast too. Reinforcement Learning is used in multiple areas of NLP like text summarization, question answering, translation, dialogue generation, machine translation etc.
Reinforcement Learning agents can be trained to understand a few sentences of the document and use it to answer the corresponding questions. Reinforcement Learning with a combination of RNN is used to generate the answers for those questions as shown in this paper.
💡 Pro tip: Don't forget to have a look at Supervised Learning vs. Unsupervised Learning .
Research led by Salesforce introduced a new training method that combines standard supervised word prediction and reinforcement learning (RL), showing improvement over previous state-of-the-art models for summarization as shown here in this paper .

Robots in industries or healthcare working towards reducing manual intervention use reinforcement learning to map natural language instructions to sequences of executable actions.
During training, the learner repeatedly constructs action sequences, executes those actions, and observes the resulting rewards. A reward function works in the backend that defines the quality of these executed actions. This paper demonstrates that this method can rival supervised learning techniques while requiring only a few annotated training examples.

💡 Pro-tip: Read this guide on test, train and validation split for better results.
Another interesting research in this area is led by the researchers of Stanford University, Ohio State University, and Microsoft Research on Deep RL for dialogue generation .
The deep RL finds application in a chatbot dialogue . Conversations are simulated using two virtual agents and the quality is improved in progressive iterations.
Reinforcement Learning is used in various marketing spheres to develop techniques that maximize customer growth and strive for a balance between long-term and short-term rewards.
Let us go through the various scenarios where real-time bidding via Reinforcement Learning is used in the marketing space.
Customized Recommendations for customers
Personalized product suggestions give customers what they want. The Reinforcement Learning bot is trained to handle situations where challenging barriers like reputation, limited customer data, and consumers evolving mindset are dealt.
It dynamically learns the customer's requirements and analyses the behavior to serve high-quality recommendations. This increases the ROI and profit margins for the company.
Creating the most beneficial content for advertisement
Coming up with the best marketing pitch that attracts a broader audience is challenging. Models based on Q-Learning are trained on a reward basis and develop an inherent knowledge of positive actions and the desired results. The Reinforcement Learning model will find the advertisement that the users are more likely to click on, thus increasing the customer footprint.
Identifying interest areas of customers with store’s CCTV to deliver better advertisements and offers.
Reinforcement Learning For Consumers And Brands
Without the power of AI, there is a big hurdle in optimizing the reach of advertisements to the customers.
Analyzing which advertisement would suit the need at a given scenario is very hard by naive methods; it paves the way for Reinforcement Learning models. The algorithm meets associated user preferences and dynamically chooses the perfect frequency for buyers.
As a result, increased online conversions are transforming browsing into business.
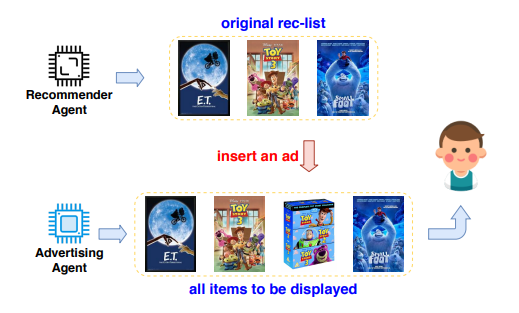
Reinforcement Learning has taken over the traditional methods of creating video games.
As compared to traditional video games where we need to have a complex behavioral tree to craft the logic of game, training a Reinforcement Learning model is much simpler. Here, the agent is set to learn by itself in the simulated game environment by performing the necessary sequence of actions to achieve the desired behavior.
💡Pro-Tip: Looking to speed up your annotation process? Check out V7—Automated Image Annotation.
In Reinforcement Learning, the agent should be trained for all the aspects of the game like path finding, defense, attack and creating situation based strategies to make the game interesting for the opponent.
Depending upon the intelligence the bot has obtained, levels of the game are set.
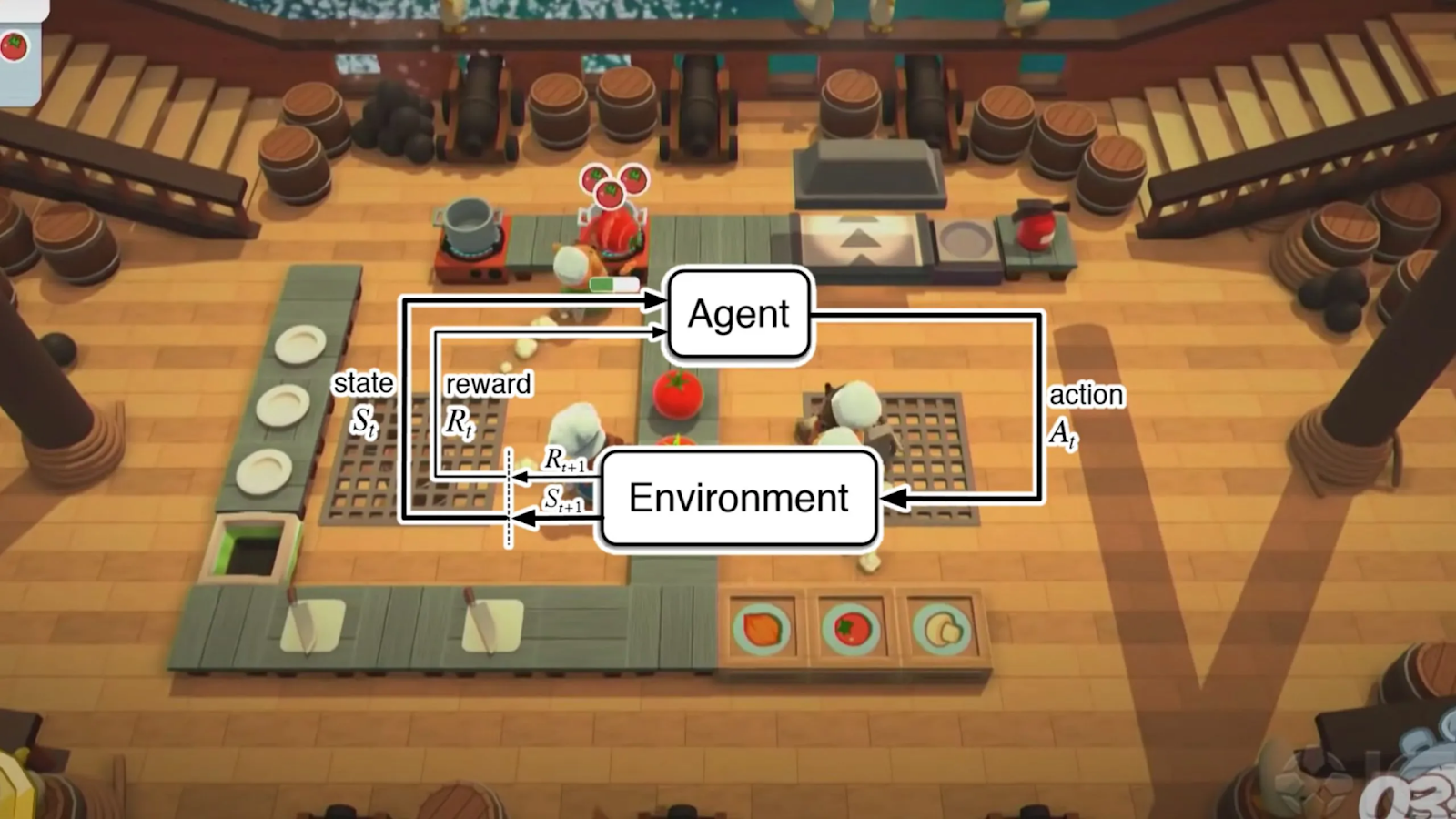
Google DeepMind is a live example of Game Optimization.
We have seen in AlphaGo, a RL trained agent beat the strongest Go player in history scoring a goal that was considered impossible at that time. It is known to be a very challenging game for Artifical Intelligence.
AlphaGo, a computer program, created by DeepMind a Google company, uses an amalgamation of the advanced search tree and deep neural networks. These neural networks take the Go board as an input derive features through different network layers containing millions of neuron-like connections.
Reinforcement Learning agents are also used in bug detection and game testing. This is due to its ability to run a ton of iterations without human input, stress testing, and creating situations for potential bugs.
Newer games companies such as Ubisoft have recently utilized Reinforcement Learning to decrease the number of active bugs found within the game. RL agents are trained in the game environment using exploration and exploitation techniques to test some of its game mechanics in an attempt to fix them.
Reinforcement Learning Applications: Key Takeaways
Finally, here's a quick recap of everything we've learned:
- Reinforcement Learning involves training a model so that they produce a sequence of decisions. It is either trained using a positive mechanism where the models are rewarded for actions to be more likely to generate it in the future. On the other hand, negative Reinforcement Learning adds punishment so that they don't produce the current sequence of results again.
- Reinforcement Learning has changed the dynamics of various sectors like Healthcare, Robotics, Gaming, Retail, Marketing, and many more.
- Various companies have started managing the marketing campaigns digitally with Reinforcement Learning due to its fundamental ability to increase the profit margins by predicting the choices and behavior of customers towards the products/services.
- Healthcare is another sector where Reinforcement Learning is used to help doctors discover the treatment type, suggest appropriate doses of drugs and timings for taking such doses.
- Reinforcement Learning approaches are used in the field of Game Optimization and simulating synthetic environments for game creation.
- Reinforcement Learning also finds application in self-driving cars to train an agent for optimizing trajectories and dynamically planning the most efficient path.
- RL can be used for NLP use cases such as text summarization, question & answers, machine translation.
💡 Read next:
A Step-by-Step Guide to Text Annotation [+Free OCR Tool]
The Complete Guide to CVAT—Pros & Cons
5 Alternatives to Scale AI
The Ultimate Guide to Semi-Supervised Learning
9 Essential Features for a Bounding Box Annotation Tool
Mean Average Precision (mAP) Explained: Everything You Need to Know
The Complete Guide to Ensemble Learning
The Beginner’s Guide to Contrastive Learning

Pragati is a software developer at Microsoft, and a deep learning enthusiast. She writes about the fundamental mathematics behind deep neural networks.
“Collecting user feedback and using human-in-the-loop methods for quality control are crucial for improving Al models over time and ensuring their reliability and safety. Capturing data on the inputs, outputs, user actions, and corrections can help filter and refine the dataset for fine-tuning and developing secure ML solutions.”

Related articles

Explore Neptune Scale: tracker for foundation models -> Tour a live project 📈
Model-Based and Model-Free Reinforcement Learning: Pytennis Case Study
Reinforcement learning is a field of Artificial Intelligence in which you build an intelligent system that learns from its environment through interaction and evaluates what it learns in real-time.
A good example of this is self-driving cars, or when DeepMind built what we know today as AlphaGo, AlphaStar, and AlphaZero.
AlphaZero is a program built to master the games of chess, shogi and go (AlphaGo is the first program that beat a human Go master). AlphaStar plays the video game StarCraft II.
In this article, we’ll compare model-free vs model-based reinforcement learning. Along the way, we will explore:
- Fundamental concepts of Reinforcement Learning a) Markov decision processes / Q-Value / Q-Learning / Deep Q Network
- Difference between model-based and model-free reinforcement learning.
- Discrete mathematical approach to playing tennis – model-free reinforcement learning.
- Tennis game using Deep Q Network – model-based reinforcement learning.
Comparison/Evaluation
- References to learn more
SEE RELATED ARTICLES
7 Applications of Reinforcement Learning in Finance and Trading 10 Real-Life Applications of Reinforcement Learning Best Reinforcement Learning Tutorials, Examples, Projects, and Courses
Fundamental concepts of Reinforcement Learning
Any reinforcement learning problem includes the following elements:
- Agent – the program controlling the object of concern (for instance, a robot).
- Environment – this defines the outside world programmatically. Everything the agent(s) interacts with is part of the environment. It’s built for the agent to make it seem like a real-world case. It’s needed to prove the performance of an agent, meaning if it will do well once implemented in a real world application.
- Rewards – this gives us a score of how the algorithm performs with respect to the environment. It’s represented as 1 or 0. ‘1’ means that the policy network made the right move, ‘0’ means wrong move. In other words, rewards represent gains and losses.
- Policy – the algorithm used by the agent to decide its actions. This is the part that can be model-based or model-free.
Every problem that needs an RL solution starts with simulating an environment for the agent. Next, you build a policy network that guides the actions of the agent. The agent can then evaluate the policy if its corresponding action resulted in a gain or a loss.
The policy is our main discussion point for this article. Policy can be model-based or model-free. When building, our concern is how to optimize the policy network via policy gradient (PG).
PG algorithms directly try to optimize the policy to increase rewards. To understand these algorithms, we must take a look at Markov decision processes (MDP).
Markov decision processes / Q-Value / Q-Learning / Deep Q Network
MDP is a process with a fixed number of states, and it randomly evolves from one state to another at each step. The probability for it to evolve from state A to state B is fixed.
A lot of Reinforcement Learning problems with discrete actions are modeled as Markov decision processes , with the agent having no initial clue on the next transition state. The agent also has no idea on the rewarding principle, so it has to explore all possible states to begin to decode how to adjust to a perfect rewarding system. This will lead us to what we call Q Learning.
The Q-Learning algorithm is adapted from the Q-Value Iteration algorithm, in a situation where the agent has no prior knowledge of preferred states and rewarding principles. Q-Values can be defined as an optimal estimate of a state-action value in an MDP.
It is often said that Q-Learning doesn’t scale well to large (or even medium) MDPs with many states and actions. The solution is to approximate the Q-Value of any state-action pair (s,a). This is called Approximate Q-Learning.
DeepMind proposed the use of deep neural networks, which work much better, especially for complex problems – without the use of any feature engineering. A deep neural network used to estimate Q-Values is called a deep Q-network (DQN). Using DQN for approximated Q-learning is called Deep Q-Learning.
Difference between model-based and model-free Reinforcement Learning
RL algorithms can be mainly divided into two categories – model-based and model-free .
Model-based , as it sounds, has an agent trying to understand its environment and creating a model for it based on its interactions with this environment. In such a system, preferences take priority over the consequences of the actions i.e. the greedy agent will always try to perform an action that will get the maximum reward irrespective of what that action may cause.
On the other hand, model-free algorithms seek to learn the consequences of their actions through experience via algorithms such as Policy Gradient, Q-Learning, etc. In other words, such an algorithm will carry out an action multiple times and will adjust the policy (the strategy behind its actions) for optimal rewards, based on the outcomes.
Think of it this way, if the agent can predict the reward for some action before actually performing it thereby planning what it should do, the algorithm is model-based. While if it actually needs to carry out the action to see what happens and learn from it, it is model-free.
This results in different applications for these two classes, for e.g. a model-based approach may be the perfect fit for playing chess or for a robotic arm in the assembly line of a product, where the environment is static and getting the task done most efficiently is our main concern. However, in the case of real-world applications such as self-driving cars, a model-based approach might prompt the car to run over a pedestrian to reach its destination in less time (maximum reward), but a model-free approach would make the car wait till the road is clear (optimal way out).
To better understand this, we will explain everything with an example. In the example, we’ll build model-free and model-based RL for tennis games . To build the model, we need an environment for the policy to get implemented. However we won’t build the environment in this article, we’ll import one to use for our program.
Pytennis environment
We’ll use the Pytennis environment to build a model-free and model-based RL system.
A tennis game requires the following:
- 2 players which implies 2 agents.
- A tennis lawn – main environment.
- A single tennis ball.
- Movement of the agents left-right (or right-left direction).
The Pytennis environment specifications are:
- There are 2 agents (2 players) with a ball.
- There’s a tennis field of dimension (x, y) – (300, 500)
- The ball was designed to move on a straight line, such that agent A decides a target point between x1 (0) and x2 (300) of side B (Agent B side), therefore it displays the ball 50 different times with respect to an FPS of 20. This makes the ball move in a straight line from source to destination. This also applies to agent B.
- Movement of Agent A and Agent B is bound between (x1= 100, to x2 = 600).
- Movement of the ball is bound along the y-axis (y1 = 100 to y2 = 600).
- Movement of the ball is bound along the x-axis (x1 = 100, to x2 = 600).
Pytennis is an environment that mimics real-life tennis situations. As shown below, the image on the left is a model-free Pytennis game, and the one on the right is model-based .

Discrete mathematical approach to playing tennis – model-free Reinforcement Learning
Why “discrete mathematical approach to playing tennis”? Because this method is a logical implementation of the Pytennis environment.
The code below shows us the implementation of the ball movement on the lawn. You can find the source code here .
Here is how this works once the networks are initialized (Network A for Agent A and Network B for Agent B):
Each network is bounded by the directions of ball movement. Network A represents Agent A, which defines the movement of the ball from Agent A to any position between 100 and 300 along the x-axis at Agent B. This also applies to Network B (Agent B).
When the network is started, the .network method discretely generates 50 y-points (between y1 = 100 and y2 = 600), and corresponding x-points (between x1 which happens to be the location of the ball from Agent A to a randomly selected point x2 on Agent B side) for network A. This also applies to Network B (Agent B).
To automate the movement of each agent, the opposing agent has to move in a corresponding direction with respect to the ball. This can only be done by setting the x position of the ball to be the x position of the opposing agent, as in the code below.
Meanwhile the source agent has to move back to its default position from its current position. The code below illustrates this.
Now, to make the agents play with each other recursively, this has to run in a loop. After every 50 counts (50 frame display of the ball), the opposing player is made the next player. The code below puts all of it together in a loop.
And this is basic model-free reinforcement learning. It’s model-free because you need no form of learning or modelling for the 2 agents to play simultaneously and accurately.
Tennis game using Deep Q Network – model-based Reinforcement Learning
A typical example of model-based reinforcement learning is the Deep Q Network. Source code to this work is available here .
The code below illustrates the Deep Q Network, which is the model architecture for this work.
In this case, we need a policy network to control the movement of each agent as they move along the x-axis. Since the values are continuous, that is from (x1 = 100 to x2 = 300), we can’t have a model that predicts or works with 200 states.
To simplify this problem, we can split x1 and x2 into 10 states / 10 actions, and define an upper and lower bound for each state.
Note that we have 10 actions, because from a state there are 10 possibilities.
The code below illustrates the definition of both upper and lower bounds for each state.
The Deep Neural Network (DNN) used experimentally for this work is a network of 1 input (which represents the previous state), 2 hidden layers of 64 neurons each, and an output layer of 10 neurons (binary selection from 10 different states). This is shown below:
Now that we have a DQN model that predicts the next state/action of the model, and the Pytennis environment already sorted out the ball movement in a straight line, let’s go ahead and write a function that carries out an action by an agent, based on the DQN model prediction regarding it’s next state.
The detailed code below illustrates how agent A makes a decision on where to direct the ball (on Agent B’s side and vice-versa). This code also evaluates agent B, if it was able to receive the ball.
From the code above, function stepA gets executed when AgentA has to play. While playing, AgentA uses the next action predicted by DQN to estimate the target (x2 position, at Agent B, from the current position of the ball, x1, which is on it’s own side), by using the ball trajectory network developed by the Pytennis environment to make its own move.
Agent A, for example, is able to get a precise point x2 on Agent’s B side by using the function randomVal , as shown above, to randomly select a coordinate x2 bounded by the action given by DQN.
Finally, function stepA evaluates the response of AgentB to target point x2 by using the function evaluate_action . The function evaluate_action defines if AgentB should be penalized or rewarded. Just as this is described for AgentA to AgentB, it applies for AgentB to AgentA (same code by different variable names).
Now that we have the policy, reward, environment, states and actions correctly defined, we can go ahead and recursively make the two agents play the game with each other.
The code below shows how turns are taken by each agent after 50 ball displays. Note that for each ball display, the DQN is making a decision on where to toss the ball for the next agent to play.
Having played this game model-free and model-based, here are some differences that we need to be aware of:
If you’re interested, the videos below show these two techniques in action playing tennis games:
1. Model-free
2. Model-based
Tennis might be simple compared to self-driving cars, but hopefully this example showed you a few things about RL that you didn’t know.
The main difference between model-free and model-based RL is the policy network, which is required for model-based RL and unnecessary in model-free.
It’s worth noting that oftentimes, model-based RL takes a massive amount of time for the DNN to learn the states perfectly without getting it wrong.
But every technique has its drawbacks and advantages, choosing the right one depends on what exactly you need your program to do.
Thanks for reading, I left a few additional references for you to follow if you want to explore this topic more.
- AlphaGo documentary: https://www.youtube.com/watch?v=WXuK6gekU1Y
- List of reinforcement learning environments: https://medium.com/@mauriciofadelargerich/reinforcement-learning-environments-cff767bc241f
- Create your own reinforcement learning environment: https://towardsdatascience.com/create-your-own-reinforcement-learning-environment-beb12f4151ef
- Types of RL Environments: https://subscription.packtpub.com/book/big_data_and_business_intelligence/9781838649777/1/ch01lvl1sec14/types-of-rl-environment
- Model-based Deep Q Network: https://github.com/elishatofunmi/pytennis-Deep-Q-Network-DQN
- Discrete mathematics approach youtube video: https://youtu.be/iUYxZ2tYKHw
- Deep Q Network approach YouTube video: https://youtu.be/FCwGNRiq9SY
- Model-free discrete mathematics implementation: https://github.com/elishatofunmi/pytennis-Discrete-Mathematics-Approach-
- Hands-on Machine Learning with scikit-learn and TensorFlow: https://www.amazon.com/Hands-Machine-Learning-Scikit-Learn-TensorFlow/dp/1491962291
Was the article useful?
More about model-based and model-free reinforcement learning: pytennis case study, check out our product resources and related articles below:, observability in llmops: different levels of scale, llm observability: fundamentals, practices, and tools, 3 takes on end-to-end for the mlops stack: was it worth it, adversarial machine learning: defense strategies, explore more content topics:, manage your model metadata in a single place.
Join 50,000+ ML Engineers & Data Scientists using Neptune to easily log, compare, register, and share ML metadata.
Exploring Reinforcement Learning: A Case Study Applied to the Popular Snake Game
- Conference paper
- First Online: 28 February 2022
- Cite this conference paper
- Russell Sammut Bonnici 14 ,
- Chantelle Saliba 14 ,
- Giulia Elena Caligari 14 &
- Mark Bugeja 14
Part of the book series: Lecture Notes in Networks and Systems ((LNNS,volume 382))
Included in the following conference series:
- The International Conference on Intelligent Systems & Networks
Reinforcement Learning is a machine learning approach in which an agent interacts with their environment to gather information, and make an informed decision based on the accumulated information. In this research, we investigate the applicability of various reinforcement learning techniques for Snake, a video game popular on the Nokia 3310 mobile phone. Q-Learning (Quality-Learning), SARSA (State-Action Reward State-Action) and PPO (Proximal Policy Optimization), were implemented and evaluated for Snake. Q-Learning and SARSA did not generate optimal results due to the large environment of the game. Meanwhile, PPO was implemented with three varying approaches for input; a vector, CNN and raycasting based approach. PPO, in conjunction with raycasting, resulted in the best performance, with the snake agent learning for both collecting food and avoiding obstacles. Furthermore, A* Pathfinding was tested and it achieved a performance better than Q-Learning and SARSA but not better than PPO as it was less adaptable to large environments. In the future, agents in large dynamic game environments, may benefit further from utilizing PPO.
This is a preview of subscription content, log in via an institution to check access.
Access this chapter
Subscribe and save.
- Get 10 units per month
- Download Article/Chapter or eBook
- 1 Unit = 1 Article or 1 Chapter
- Cancel anytime
- Available as PDF
- Read on any device
- Instant download
- Own it forever
- Available as EPUB and PDF
- Compact, lightweight edition
- Dispatched in 3 to 5 business days
- Free shipping worldwide - see info
Tax calculation will be finalised at checkout
Purchases are for personal use only
Institutional subscriptions
Similar content being viewed by others
Leaving the NavMesh: An Ablative Analysis of Deep Reinforcement Learning for Complex Navigation in 3D Virtual Environments
Evaluating Human-like Behaviors of Video-Game Agents Autonomously Acquired with Biological Constraints
Mario Fast Learner: Fast and Efficient Solutions for Super Mario Bros
Anunpattana, P., Panumate, C., Iida, H.: Finding comfortable settings of snake game using game refinement measurement. In: Advances in Computer Science and Ubiquitous Computing, pp. 66–73 (2016)
Google Scholar
Jost, J.,, Li, W.: Reinforcement learning in complementarity game and population dynamics. Phys. Rev. E, Stat. Nonlinear Soft Matter Phys. 89 (2), 022113 (2014). ISSN: 15393755. http://search.proquest.com/docview/1639976188/
Kaelbling, L.P., Littman, M.L., Moore, A.W.: Reinforcement learning: a survey. In: CoRR cs.AI/9605103 (1996). https://arxiv.org/abs/cs/9605103
Sutton, R.S., Barto, A.G.: Reinforcement learning: an introduction. IEEE Trans. Neural Netw. 16 , 285–286 (1988)
MATH Google Scholar
Amiri, R., Mehrpouyan, H., Fridman, L., Mallik, R.K., Nallanathan, A., Matolak, D.: A machine learning approach for power allocation in HetNets considering QoS. In: 2018 IEEE International Conference on Communications (ICC), pp 1–7, May 2018. IEEE. https://ieeexplore.ieee.org/abstract/document/8422864
Zheng, Y.: Reinforcement learning and video games (2019). arXiv: 1909.04751 [cs.LG]
Silver, D., et al.: Mastering Chess and Shogi by self-play with a general reinforcement learning algorithm (2017). arXiv: 1712.01815 [cs.AI]
Mnih, V., et al.: Playing Atari with Deep Reinforcement Learning (2013). arXiv: 1312.5602 [cs.LG]
Ma, B., Tang, M.X., Zhang, J.: Exploration of reinforcement learning to SNAKE (2016)
Wei, Z., et al.: Autonomous agents in Snake Game via Deep Reinforcement Learning. In: 2018 IEEE International conference on Agents (ICA), pp 20–25, July 2018. https://doi.org/10.1109/AGENTS.2018.8460004.
Watkins, CJCH.: Learning from delayed rewards (1989)
Manju, S., Punithavalli, M.: An analysis of Q-learning algorithms with strategies of reward function. Int. J. Comput. Sci. Eng. 3 (2011)
Watkins, C.J.C.H., Dayan, P.: Q-learning. In: Machine learning 8 (3), 279–292 (1992). ISSN: 1573-0565. https://doi.org/10.1007/BF00992698
Xu, D., et al.: Path planning method combining depth learning and Sarsa Algorithm’. In: 2017 10th International Symposium on Computational Intelligence and Design (ISCID), vol. 2. pp. 77–82, Dec 2017. https://doi.org/10.1109/ISCID.2017.145
Chettibi, S., Chikhi, S.: An adaptive energy-aware routing protocol for MANETs using the SARSA reinforcement learning algorithm. In: 2012 IEEE Conference on Evolving and Adaptive Intelligent Systems. pp. 84-89, May 2012. https://doi.org/10.1109/EAIS.2012.6232810
Iima, H., Kuroe, Y.: Swarm reinforcement learning algorithms based on Sarsa method. In: 2008 SICE Annual Conference, Aug 2008, pp 2045–2049. https://doi.org/10.1109/SICE.2008.4654998
Schulman, J., et al.: Proximal policy optimization algorithms (2017). arXiv: 1707.06347 [cs.LG]
Juliani, A., et al.: Unity: a general platform for Intelligent Agents (2018). arXiv: 1809.02627 [cs.LG]
Aron Granberg’s Pathfinding Project. https://arongranberg.com/astar/ . Accessed: 2020-02-05
Download references
Author information
Authors and affiliations.
University of Malta, Msida, MSD 2080, Malta
Russell Sammut Bonnici, Chantelle Saliba, Giulia Elena Caligari & Mark Bugeja
You can also search for this author in PubMed Google Scholar
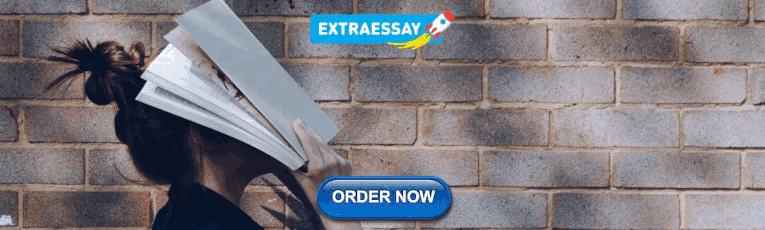
Corresponding author
Correspondence to Russell Sammut Bonnici .
Editor information
Editors and affiliations.
Department of Artificial Intelligence, Faculty of ICT, University of Malta, Msida, Malta
Alexiei Dingli
Emergent Technologies Lab, Danube-University Krems, Vienna, Austria
Alexander Pfeiffer
School of Marketing and Communication, University of Vaasa, Vaasa, Finland
Alesha Serada
Department of Artificial Intelligence, Institute of Climate Change and Sustainable Development, University of Malta, Msida, Malta
Mark Bugeja
Stephen Bezzina
Rights and permissions
Reprints and permissions
Copyright information
© 2022 The Author(s), under exclusive license to Springer Nature Switzerland AG
About this paper
Cite this paper.
Bonnici, R.S., Saliba, C., Caligari, G.E., Bugeja, M. (2022). Exploring Reinforcement Learning: A Case Study Applied to the Popular Snake Game. In: Dingli, A., Pfeiffer, A., Serada, A., Bugeja, M., Bezzina, S. (eds) Disruptive Technologies in Media, Arts and Design. ICISN 2021. Lecture Notes in Networks and Systems, vol 382. Springer, Cham. https://doi.org/10.1007/978-3-030-93780-5_12
Download citation
DOI : https://doi.org/10.1007/978-3-030-93780-5_12
Published : 28 February 2022
Publisher Name : Springer, Cham
Print ISBN : 978-3-030-93779-9
Online ISBN : 978-3-030-93780-5
eBook Packages : Intelligent Technologies and Robotics Intelligent Technologies and Robotics (R0)
Share this paper
Anyone you share the following link with will be able to read this content:
Sorry, a shareable link is not currently available for this article.
Provided by the Springer Nature SharedIt content-sharing initiative
- Publish with us
Policies and ethics
- Find a journal
- Track your research

- SVP Experience
- Ethical Business Decisions Playbook
- Silicon Valley Hiring Guide
- Tuition and Financial Aid
- GMAT/GRE Waiver
- Admissions Requirements
- Application Deadlines
- How to Apply
- Networking Opportunities
- Student Success
9 Real-Life Examples of Reinforcement Learning
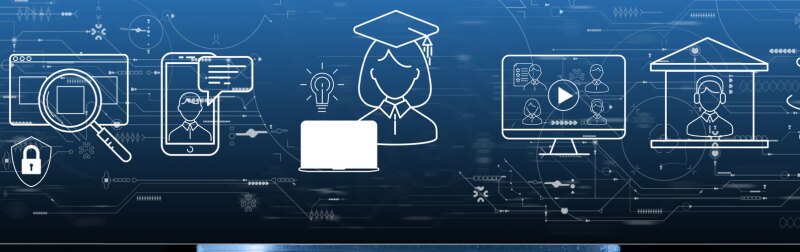
Most people follow a similar process for learning new things: receive the information, process it, try it out yourself, and receive feedback on how it went. A lot of this process is also bolstered by rewards and punishment: if you answer correctly, you get a gold star, an extra point, or a higher grade. If you answer incorrectly, you lose points, leave the competition, or must repeat the exercise.
As artificial intelligence becomes increasingly prevalent and competent, programmers are using the same processes in a popular form of machine learning called reinforcement learning (RL). With this technology, businesses are able to optimize, control, and monitor their workflows with a previously impossible level of accuracy and finesse. 1 As reinforcement learning evolves, its potential and benefits only grow stronger.
Keep reading to find out more about the origins and applications of RL, many of which you might have already experienced today.
What is reinforcement learning?
Reinforcement learning is the closest to human learning as digital systems and machines can get. Through this training, machine learning models can be taught to follow instructions, conduct tests, operate equipment, and much more. 2
Reinforcement learning is centered around a digital agent who is put in a specific environment to learn. Similar to the way that we learn new things, the agent faces a game-like situation and must make a series of decisions to try to achieve the correct outcome. 3 Through trial and error, the agent will learn what to do (and what not to do) and is rewarded and punished accordingly. Every time it receives a reward, it reinforces the behavior and signals the agent to employ the same tactics again next time.
History & Background
The foundations for reinforcement learning were laid over 100 years ago, and it is actually said to have a two-pronged origin. The first is rooted in animal learning and the “Law of Effect,” coined by Edward Thorndike. Thorndike described the Law of Effect in 1911 as the notion that an animal will repeat actions if they produce satisfaction, and it will be deterred from actions that produce discomfort. Furthermore, the greater the level of pleasure or pain, the greater the pursuit or deterrence from the action. 4 The Law of Effect combines both selectional and associative learning; with selectional learning, the animal will try to try a few different options and routes and select among them based on how they went. In associative learning, the animal chooses its options based on what situations they associate them with, and whether they’re positive or negative.
Although Thorndike established the essence of reinforcement learning, the term “reinforcement” wasn’t formally used until 1927 by Ivan Pavlov. He described reinforcement as “the strengthening of a pattern of behavior due to an animal receiving a stimulus—a reinforcer— in a time-dependent relationship with another stimulus or with a response.” 4 In other words, when animals receive a reaction to something they’ve done shortly after they’ve done it, it affects whether or not they’ll do it again, in the same way, in the future.
The second origin, optimal control, is more rooted in mathematics and algorithms than animal learning. Starting in the 1950s, researchers began to define optimization methods to derive control policies in continuous time control problems. Building on this, Richard Bellman developed programming that defines a functional equation using a dynamic system’s state and returns an optimal value function (commonly referred to as the Bellman equation). Bellman then went on to introduce the Markovian Decision Process (MDP), which he defines as “a discrete stochastic version of the optimal control problem”. 4,1 MDPs helped create solution methods that gradually reach the correct answer to something through successive guesses, much like modern reinforcement learning.
Applications of Reinforcement Learning
Reinforcement learning is on the rise and its future is just as vibrant. Here, we’ll take a look at some of the current ways RL is working in the real world.
1. Automated Robots
While most robots don’t look like pop culture has led us to believe, their capabilities are just as impressive. The more robots learn using RL, the more accurate they become, and the quicker they can complete a previously arduous task. They can also perform duties that would be dangerous for people with far less consequences. For these reasons, aside from requiring some oversight and regular maintenance, robots are a cost-effective and efficient alternative to manual labor.
For example, some restaurants use robots to deliver food to tables. Grocery stores are using robots to identify where shelves are low and order more product. In common settings, automated robots have been used thus far to assemble products; inspect for defects; count, track, and manage inventory; deliver goods; travel long and short distances; input, organize, and report on data; and grasp and handle objects of all different shapes and sizes. As we continue to test robotic abilities, new features are being introduced to expand their potential.
2. Natural Language Processing
Predictive text, text summarization, question answering, and machine translation are all examples of natural language processing (NLP) that uses reinforcement learning. By studying typical language patterns, RL agents can mimic and predict how people speak to each other every day. This includes the actual language used, as well as syntax, (the arrangement of words and phrases) and diction (the choice of words).
In 2016, researchers from Stanford University, Ohio State University, and Microsoft Research used this learning to generate dialogue, like what’s used for chatbots. Using two virtual agents, they simulated conversations and used policy gradient methods to reward important attributes such as coherence, informativity, and ease of answering. 5 This research was unique in that it didn’t only focus on the question at hand, but also on how an answer could influence future outcomes. This approach to reinforcement learning in NLP is now widely adopted and used by customer service departments in many major organizations.
3. Marketing and Advertising
Both brands and consumers can use reinforcement learning to their benefit. For brands selling to target audiences, they can use real-time bidding platforms, A/B testing, and automatic ad optimization. This means that they can place a series of advertisements in the marketplace and the host will automatically serve the best-performing ads in the best spots for the lowest prices. 2,5 Although brands post and set up the campaigns themselves, marketing and advertising platforms are also learning which types of ads are resonating with audiences and will display those ads more frequently and prominently.
From a consumer perspective, you might notice that the ads you receive are usually from companies whose websites you’ve visited before, whom you have bought from before, or are in the same industry as a company from which you’ve made a purchase. That’s because marketing and advertising platforms can use reinforcement learning to associate similar companies, products, and services to prioritize for certain customers. If they try certain options and receive a click or other engagement, it signals that they were ‘correct’ and should employ the same strategy again. 2
4. Image Processing
Have you ever taken a security test that asked you to identify objects in frames, such as “Click on the photos that have a street sign in them”? This is similar to what learning machines can do, although they approach it in a different way.
When asked to process an image, RL agents will search an entire image as their starting point, then identify objects sequentially until everything is registered. Artificial vision systems also use deep convolutional neural networks, made up of large, labeled datasets, to map images to human-generated scene descriptions from simulation engines. 2
Some more examples of reinforcement learning in image processing include: 2
- Robots equipped with visual sensors from to learn their surrounding environment
- Scanners to understand and interpret text
- Image pre-processing and segmentation of medical images, like CT Scans
- Traffic analysis and real-time road processing by video segmentation and frame-by-frame image processing
- CCTV cameras for traffic and crowd analytics
5. Recommendation Systems
The “Frequently Bought Together” section on Amazon, a “Customers Also Liked” tab online at Target, and the “Recommended Reading” articles from news outlets all utilize learning machines to generate recommendations. Specifically for news reading, RL agents can track the types of stories, topics, and even author names someone prefers so that the system can queue the next story they think they would enjoy. That includes the details of exactly how they interact with the content, e.g., clicks and shares, and aspects such as timing and freshness of the news. A reward is then defined based on these user behaviors. 5
Recommendation systems also analyze past behaviors to try to predict future ones. So if, for example, a hundred people who bought ski pants then went on to buy ski boots, a company’s system learns to send ads for ski boots to anyone who just bought ski pants. If the ads are unsuccessful, they might try to display ads for ski jackets, instead, and see how the results compare.
From creating a new game, to testing its bugs, to defeating its levels, RL is an efficient and relatively easy resource on which programmers can rely. Compared to traditional video games that require complex behavioral trees to craft the logic of the game, training an RL model is much simpler. Here, the agent will learn by itself in the simulated game environment through navigation, defense, attack, and strategizing. 2 Through trial and error, they’ll begin to perform the necessary actions to reach the desired goal.
RL agents are also used in bug detection and game testing. This is due to its ability to run a large number of iterations without human input, stress testing, and creating situations for potential bugs. 2
7. Energy Conservation
As much of the world works to lower their effects on the climate, reducing energy consumption is at the top of the list. A prime example is the partnership between Deepmind and Google to cool massive and essential Google Data Centers. With a fully-functioning AI system, the centers saw a 40% reduction in energy spending without the need for human intervention—though there is still some supervision from data center experts. 5,6
The system works in the following way: 5
- Taking snapshots of data from the data centers every five minutes and feeding this to deep neural networks
- Predicting how different combinations will affect future energy consumptions
- Identifying actions that will lead to minimal power consumption while maintaining a set standard of safety criteria
- Sending and implementing these actions at the data center
- Verifying the actions by the local control system
Another example may be an Eco setting on your thermostat, or motion-activated lights that offer different settings based on the level of light already in the room.
8. Traffic Control
Civil engineers have been struggling with traffic for centuries, but reinforcement learning is working to help solve that. Continuous traffic monitoring in complex urban networks helps build a literal and figurative “map” of traffic patterns and vehicle behavior. Due to its data-driven nature, the RL agents can start to learn when traffic is heaviest, which directions it’s coming from, and how quickly cars are moving through each light color. 2 Then, they adapt accordingly and continue to test and learn across times, climates, and seasons.
9. Healthcare
Healthcare employs machine learning and artificial intelligence in much of its work, and RL is no exception. It has been used in automated medical diagnosis, resource scheduling, drug discovery and development, and health management. 5
One important avenue for deploying reinforcement learning is in dynamic treatment regimes (DTRs). To create a DTR, someone must input a set of clinical observations and assessments of a patient. Using previous outcomes and patient medical history, the learning system will then output a suggestion on treatment type, drug dosages, and appointment timing for every stage of the patient’s journey. This is extremely beneficial for making time-dependent decisions for the best treatment for a patient at a specific time without expending much time, energy, or effort to consult with multiple parties. 2
Learn RL & More to Advance in Analytics
Behind every successful reinforcement learning scenario is a team of data scientists, programmers, and business analysts who make it all possible. But RL requires a specific set of skills, one that a Master’s in Business Analytics or Data Science is guaranteed to give you.
The Online MSBA program at Santa Clara University’s Leavey School of Business offers courses on RL Algorithms, Temporal Difference Learning, Q-Learning, Deep Learning Neural Networks, and much more. The popularity of and demand for these skills is certainly apparent: jobs for professionals trained in data science and analytics increased by 50% across a number of sectors in the past few years, and the U.S. Bureau of Labor Statistics has listed data science as one of the top 20 fastest growing occupations.*,*If you're already a student of business analytics, or a prospective student looking to enhance your career, consider how an MSBA degree could enhance your career.
- Retrieved on September 19, 2022, from towardsdatascience.com/reinforcement-learning-fda8ff535bb6#757c
- Retrieved on September 19, 2022, from v7labs.com/blog/reinforcement-learning-applications#h8
- Retrieved on September 19, 2022, from deepsense.ai/what-is-reinforcement-learning-the-complete-guide/
- Retrieved on September 19, 2022, from researchdatapod.com/history-reinforcement-learning/
- Retrieved on September 20, 2022, from neptune.ai/blog/reinforcement-learning-applications
- Retrieved on September 20, 2022, from deepmind.com/blog/safety-first-ai-for-autonomous-data-centre-cooling-and-industrial-control
Return to Media
Santa Clara University has engaged Everspring , a leading provider of education and technology services, to support select aspects of program delivery.
Interested in one of our online programs? Receive a program brochure.
IEEE Account
- Change Username/Password
- Update Address
Purchase Details
- Payment Options
- Order History
- View Purchased Documents
Profile Information
- Communications Preferences
- Profession and Education
- Technical Interests
- US & Canada: +1 800 678 4333
- Worldwide: +1 732 981 0060
- Contact & Support
- About IEEE Xplore
- Accessibility
- Terms of Use
- Nondiscrimination Policy
- Privacy & Opting Out of Cookies
A not-for-profit organization, IEEE is the world's largest technical professional organization dedicated to advancing technology for the benefit of humanity. © Copyright 2024 IEEE - All rights reserved. Use of this web site signifies your agreement to the terms and conditions.
Grab your spot at the free arXiv Accessibility Forum
Help | Advanced Search
Computer Science > Machine Learning
Title: adaptive user journeys in pharma e-commerce with reinforcement learning: insights from swiperx.
Abstract: This paper introduces a reinforcement learning (RL) platform that enhances end-to-end user journeys in healthcare digital tools through personalization. We explore a case study with SwipeRx, the most popular all-in-one app for pharmacists in Southeast Asia, demonstrating how the platform can be used to personalize and adapt user experiences. Our RL framework is tested through a series of experiments with product recommendations tailored to each pharmacy based on real-time information on their purchasing history and in-app engagement, showing a significant increase in basket size. By integrating adaptive interventions into existing mobile health solutions and enriching user journeys, our platform offers a scalable solution to improve pharmaceutical supply chain management, health worker capacity building, and clinical decision and patient care, ultimately contributing to better healthcare outcomes.
Comments: | Presented at the Third Workshop on End-to-End Customer Journey Optimization at KDD 2024 (KDD CJ Workshop '24), August 26, Barcelona, Spain |
Subjects: | Machine Learning (cs.LG); Artificial Intelligence (cs.AI); Machine Learning (stat.ML) |
Cite as: | [cs.LG] |
(or [cs.LG] for this version) | |
Focus to learn more arXiv-issued DOI via DataCite |
Submission history
Access paper:.
- HTML (experimental)
- Other Formats

References & Citations
- Google Scholar
- Semantic Scholar
BibTeX formatted citation

Bibliographic and Citation Tools
Code, data and media associated with this article, recommenders and search tools.
- Institution
arXivLabs: experimental projects with community collaborators
arXivLabs is a framework that allows collaborators to develop and share new arXiv features directly on our website.
Both individuals and organizations that work with arXivLabs have embraced and accepted our values of openness, community, excellence, and user data privacy. arXiv is committed to these values and only works with partners that adhere to them.
Have an idea for a project that will add value for arXiv's community? Learn more about arXivLabs .

An official website of the United States government
The .gov means it’s official. Federal government websites often end in .gov or .mil. Before sharing sensitive information, make sure you’re on a federal government site.
The site is secure. The https:// ensures that you are connecting to the official website and that any information you provide is encrypted and transmitted securely.
- Publications
- Account settings
Preview improvements coming to the PMC website in October 2024. Learn More or Try it out now .
- Advanced Search
- Journal List
- Front Pharmacol
Reinforcement learning as an innovative model-based approach: Examples from precision dosing, digital health and computational psychiatry
Associated data.
The original contributions presented in the study are included in the article/ Supplementary Material , further inquiries can be directed to the corresponding author.
Model-based approaches are instrumental for successful drug development and use. Anchored within pharmacological principles, through mathematical modeling they contribute to the quantification of drug response variability and enables precision dosing. Reinforcement learning (RL)—a set of computational methods addressing optimization problems as a continuous learning process—shows relevance for precision dosing with high flexibility for dosing rule adaptation and for coping with high dimensional efficacy and/or safety markers, constituting a relevant approach to take advantage of data from digital health technologies. RL can also support contributions to the successful development of digital health applications, recognized as key players of the future healthcare systems, in particular for reducing the burden of non-communicable diseases to society. RL is also pivotal in computational psychiatry—a way to characterize mental dysfunctions in terms of aberrant brain computations—and represents an innovative modeling approach forpsychiatric indications such as depression or substance abuse disorders for which digital therapeutics are foreseen as promising modalities.
1 Reinforcement learning for precision dosing
Precision dosing, or the ability to identify and deliver the right dose and schedule (i.e. the dose and schedule with highest likelihood of maximizing efficacy and minimizing toxicity), is critical for public health and society. Precision dosing is not only important for marketed drugs to reduce the consequences of imprecise dosing in terms of costs and adverse events; but also for therapeutics in development to reduce attrition, often related to the challenge of precisely characterizing the therapeutic window due to a suboptimal understanding of drug-response variability. Achieving the benefit to society of precision dosing requires the identification of the main drivers of response variability, as early as possible in the drug development process, and the deployment into clinical practice through an infrastructure designed for real-time dosing decisions in patients ( Maxfield and Zineh, 2021 ; Peck, 2021 ).
Model-based approaches to clinical pharmacology, also known as clinical pharmacometrics (PMX) play a critical role in precision dosing. First, they contribute to the identification of the determinants of response variability through quantitative analysis of pharmacokinetic (PK) and pharmacodynamics (PD) relationships, and second, they constitute a central part of the infrastructure providing a simulation engine, predicting individual patient’s response to a dose, and from which optimal dosing is identified through reverse engineering. Often this reverse engineering comprises two steps: first the PMX model’s individual parameters are calculated through Bayesian inference, i.e. through the calculation of the mode of posterior distribution (maximum a posteriori or MAP); second, an optimal dosing scheduling is calculated, often via an heuristic approach through simulating various feasible dosing scenarios on inferred individuals model’s instances.
Many examples exist in literature describing relevant PKPD models for precision dosing. For instance, in oncology, a model describing the time course of neutrophils following chemotherapy treatment is an ideal candidate for optimizing chemotherapy delivery (see ( Friberg et al., 2002 ) as an example). Studies have also reported clinical investigations of model-based precision dosing approaches. For instance, the clinical study “MODEL1” was a phase I/II trial and a clear clinical attempt of a personalized dosing regimen of docetaxel and epirubicin patients with metastatic breast cancer and was shown to lead to improved efficacy-toxicity balance ( Henin et al., 2016 ).
Reinforcement learning (RL) was also used for precision dosing. Still in oncology, Maier et al. extended the classical framework of model-driven precision dosing with RL coupled or not with data assimilation techniques ( Maier et al., 2021 ). Previously, RL applications—although without clinical confirmation—were developed for brain tumors ( Yauney and Shah, 2018 ) based on a model of tumor size response to chemotherapy ( Ribba et al., 2012 ). We have recently evaluated the performance of RL algorithms for precision dosing of propofol for general anesthesia and for which a meta-analysis showed that the monitoring of the bispectral index (BIS)—a PD endpoint—contributes to reduce the amount of propofol given and the incidence of adverse reactions ( Wang et al., 2021 ). In ( Ribba et al., 2022 ), we performed a theoretical analysis of propofol precision dosing confronting RL to hallmarks of clinical pharmacology problems during drug development, i.e. the low number of patients and tested dosing regimen, the incomplete understanding of the drivers of response and the presence of high variability in the data.
While RL does not present as a universal solution for all types of precision dosing problems, it is an interesting modeling paradigm worth exploring. In comparison to the way PMX traditionally addresses precision dosing, RL presents several advantages. First, the possibility to take into account high dimensional PKPD variables while classical model-based approaches are often limited to a low number of variables (plasma concentration and one endpoint). In doing so, it represents an opportunity for the integration of digital health data such as from wearable devices or digital health technologies in general. Second, the definition of the precision dosing policy in a dynamic and adaptable manner through the continuous learning of the algorithm through real and simulated experience (data). RL is an approach by which both the underlying model and the optimal dosing rules are learnt simultaneously while for classical approaches, these represent two sequential steps: in other words, the consequence of the dose does not influence the model structure. Recently, studies have been published illustrating methodologies for adapting PKPD model structures through data assimilation ( Lu et al., 2021 ; Bram et al., 2022 ). While high dosing frequency is not a prerequisite condition for the applicability of RL to precision dosing, this approach is well suited when the solution space of dosing is large, making heuristic approaches to find optimal dosing solutions inadequate. In our example on propofol, dosing could happen every 5 s so over a short period of 2 min, the space of solutions to explore when considering dichotomous dosing even is greater than 16 million possibilities.
RL is at the crossroads between two scientific fields. First, the field of learning by trial and error that started with the study of the psychology of animal learning and second, the field of optimal control ( Sutton and Barto, 2018 ). RL are often formally described with Markov Decision Process or MDP which includes all important features a learning agent should have, namely, being able to sense the environment, being able to take action and have clarity on the goal. In RL, a learning agent takes an action and, as a result, transitions from one state to another. After each action taken, the interaction between the agent and its environment produces a reward. The goal of the RL problem is to map actions to situations (state), i.e. knowing which actions to take in each state to maximize the accumulated reward. As long as the optimization problem can be formulated within the MDP framework, RL can be applied and its efficiency explored.
For precision dosing of propofol, the state can be represented by a table, an approach also called tabular solution methods. In the next two sections, the state will be defined by a continuous function. The reward was determined based on the value reached by the BIS as a direct consequence of the action taken: the closer the BIS to the target, the higher the reward. Finally, given the theoretical study, the true PKPD model (linking the dose application to BIS) was used as an experience (data) generator. The left column of Table 1 summarizes the characteristics of the application of RL to the propofol precision dosing problem.
Main characteristics of RL algorithm implementation to the precision dosing of pharmacological interventions (left column); the precision dosing of digital intervention (middle column); and computational psychiatry (right column). While there are multiple similarities between the precision dosing of pharmacological and digital interventions, the application of RL in computational psychiatry shows as a paradigm shift. RL computational machinery is not deployed as a technical approach to address the optimal control problem of precision dosing but is fitted to (cognitive task) data assuming the algorithm itself presents mechanistic similarities with how brain’s participants functioned during the task.
Precision dosing of a pharmacological intervention | Precision dosing of a digital intervention | Computation psychiatry | |
---|---|---|---|
Study case [References] | Optimal dosing of propofol administration ( ) | Just-in-time-adaptive-intervention for HeartSteps, mobile app aimed at reducing physical inactivity ( ) | Population analysis of signal-detection task in anhedonic subjects ( ) |
Type of RL solution | Tabular | Continuous | |
State | |||
PK drivers and/or PD endpoint such as the BIS | Contextual drivers (e.g. weather conditions, time of the day) and patient-related status derived from wearable device equipment | Belief of the correctness (weight) of each stimuli present in the task | |
Action | Dose or not | Dose (walking suggestion message) or not | Participant’s answer choice |
Reward | |||
Simple function of BIS leading to high reward when actual BIS is close to its target | Step count in the 30 min window after each decision time | Automatically derived from the answer as per task design and setup | |
Use of simulated experience? | |||
The true underlying PKPD model is used | Linear model assimilating real data | No need for simulated experience, RL algorithm is mapped to the trial-by-trial data | |
Algorithm | Temporal difference Q-learning | Thomson Sampling | Temporal difference Q-learning |
Free parameters | |||
Parameters of the PKPD model | Parameters of the linear model for reward prediction under alternative dosing scenarios | Learning rate and reward sensitivity parameter |
The minimal set of RL characteristics makes it a very flexible paradigm, suitable for a large variety of problems. Herein, we will in fact illustrate this flexibility by illustrating how this framework can be viewed as a bridge between a priori distinct areas such as precision dosing of pharmacological drugs, digital health and computational psychiatry.
In the appendix, we propose to demystify how RL algorithms—such as temporal difference Q-learning, repeatedly mentioned here—work, taking a simple illustration from video gaming.
2 Reinforcement learning in digital health
For several years, many reports have indicated the key importance of digital health for reducing the burden to society of non-communicable diseases such as cardiovascular, diabetes, cancer or psychiatric diseases, in part due to the aging of the population and—paradoxically—the success of pharmacologically-based interventions in increasing life expectancy while being affected by pathological conditions ( Fleisch et al., 2021 ). Prevention and interventions targeting lifestyle are essential tools to address this societal challenge of ever-growing importance as our healthcare systems risk collapse under cost pressure.
In 2008, it was estimated that physical inactivity causes 6% of the burden of coronary heart disease, 7% of type II diabetes, 10% of breast cancer and 10% of colon cancer and overall the cause of more than 5.3 million of the 57 million deaths which occurred that year ( Lee et al., 2012 ). In that study, the authors also estimated that with 25% reduction of physical inactivity, 1.3 million of deaths could be averted every year. Given the constant increase of smartphone coverage worldwide, it is natural to think of mobile health technologies to support healthy lifestyle habits and prevention. The thinktank Metaforum from KU Leuven dedicated its position paper 17 on the use of wearables and mobile technologies for collecting information on individual behavior and physical status—combined with data from individual’s environment—to personalize recommendations (interventions) bringing the subject to adopt a healthier lifestyle ( Claes, 2022 ).
When the intervention is intended to have a therapeutic benefit, it falls in the field of digital therapeutics when associated with demonstration of clinical effectiveness and approved by regulatory bodies ( Sverdlov et al., 2018 ). This point of junction between digital health applications and pharmacological drugs represents a ground for attempting to reframe PMX—a recognized key player in the development of the latter—as a key support to the development of the former, in particular when it comes to precision dosing for digital health.
The precision dosing of digital therapeutics overlaps with the concept of just-in-time adaptive intervention or JITAI ( Nahum-Shani et al., 2018 ). In the mobile technology literature, JITAI has been primarily considered as a critical topic for increasing adherence and retention of users; but within a therapeutic perspective, it should encompass both the topic of adherence and retention to the therapeutic modality and the topic of its optimal dosing in order to maximize clinical benefit. For clarity, these two different learning problems should be distinguished as many existing applications focus primarily on the first one. For example, a growing number of mobile applications developed under the concept of virtual coaching aim to optimize the design of the interventions (time and content, e.g. messages sent by the app to the users with the form of a prompt appearing on a locked screen) to incite the user to take actions. HeartSteps was designed to encourage user to increase their physical activity and where content delivery, such as tailored walking suggestion messages, is optimized with an RL algorithm ( Liao et al., 2020 ). Here, RL is used to address the first learning problem: How to deliver the content so that the user is doing what is recommended. We each need different forms of prompting and potentially different forms of exercise to increase our physical activity. Overall, this problem is similar to that of adherence to a pharmacological regimen. But a second problem is: what is the right dose of the desired intervention? In other words: How many steps is optimal for each patient? This is the usual precision dosing problem for drugs and there is a clear opportunity for digital health applications to extend the domain of application of JITAIs to that problem as well.
One of the particularly interesting aspects of the research on RL algorithms for HeartSteps is that, beyond the innovative nature of the work purely related to the design of personalized interventions, it also includes ways to objectively evaluate its efficiency. An experimental design called micro-randomized trial (MRT) is proposed as a framework to evaluate the effectiveness of personalized versus non-personalized interventions ( Klasnja et al., 2015 ; Qian et al., 2022 ). The principle of MRT is to randomize the interventions multiple times for each subject. Statistical approaches have been studied to leverage MRT-derived data in order to inform treatment effects and the response variability ( Qian et al., 2020 ). In the theoretical propofol example described in the previous section, we used the true PKPD model to simulate experience. In the real-life RL application of HeartSteps, the authors had the objective to design a method for learning quickly and for accommodating noisy data ( Liao et al., 2020 ). To address these points, the authors used a simulation engine to enhance data collected from real experience and this simulation engine was built with simple linear models. Precisely, the authors modeled the difference in reward function under alternative dosing options with low dimensional linear models, which features were selected based on retrospective analysis of previous HeartSteps data and based on experts’ guidance. The precision dosing problem was addressed using posterior sampling via Thompson-Sampling, identified as performant in balancing exploration and exploitation ( Russo and Van Roy, 2014 ; Russo et al., 2018 ). The definition of the state was based on several individual’s features including contextual information or sensor data from wearable devices while the reward was defined as the step counts within 30 min after the “dosing” event. The middle column of Table 1 summarizes the main characteristic of RL application to this problem.
3 Reinforcement learning in computational psychiatry
Like mechanistic modelling, computational psychiatry refers to a systems approach aimed at integrating underlying pathophysiological processes. However, while mechanistic modelling efforts typically use multiscale biological processes as building blocks, some models that fall within the remit of computational psychiatry (such as RL) use different types of building blocks, and in particular brain cognitive processes.
Model-based approaches have shown relevance for addressing major challenges in neuroscience (see ( Conrado et al., 2020 ) for an example for Alzheimer disease). Quantitative systems pharmacology and mechanistic-based multiscale modelling are, in particular, associated with major hopes while acknowledging significant challenges such as the lack of quantitative and validated biomarkers, the subjective nature of clinical endpoints and the high selectivity of drug candidates not reflecting the complex interactions of different brain circuits ( Geerts et al., 2020 ; Bloomingdale et al., 2021 ). These challenges are equally valid for attempting to address psychiatric conditions. This can partly explain the efficiency of non-pharmacological interventions, such as targeted psychotherapy approaches, recognized as one of the most precise and powerful approaches ( Insel and Cuthbert, 2015 ).
The efficiency of such interventions is a testimony of how the brain’s intrinsic plasticity can alter neural circuits. Some (discursive) disease models—with a focus on systems dimensions–propose new perspectives in the understanding of such conditions. For instance, it has been reported that emotion-cognition interactions gone awry can lead to anxiety and depression conditions; with anxious individuals displaying attentional-bias toward threatening stimuli and have difficulty disengaging from it ( Crocker et al., 2013 ). Further data-driven understanding—at the systems level—is key to increase the likelihood of success of such non-pharmacological interventions, as it is equally the case for research and development of pharmaceutical compounds ( Pao and Nagel, 2022 ). Such data-driven understanding can be integrated in the design of relevant non-pharmacological interventions, with some of them known to be amenable to digital delivery through, for instance, digital therapeutics ( Jacobson et al., 2022 ).
A precision medicine initiative—precision psychiatry—has been initiated for psychiatric indications, such as major depression or substance abuse disorder, constituting a major part of non-communicable diseases ( Insel and Cuthbert, 2015 ). The core idea of precision psychiatry lies in the reframing the diagnosis and care of affected subjects by moving away from a symptom-based to a data-driven categorization through a focus on system dimension via integration of data from cognitive, affective and social neuroscience, overall shifting the way to characterize these conditions in terms of brain circuits (dys-)functioning. This concept materialized in proposing the Research Domain Criteria (RDoc) in 2010 ( Insel et al., 2010 ) as a framework for research in pathophysiology of psychiatric conditions.
Integrating into a multiscale modelling framework, data from cognitive, affective and social neuroscience is an objective of computational psychiatry, defined as a way to characterize mental dysfunction in terms of aberrant computation in the brain ( Montague et al., 2012 ). Not surprisingly, by its mimicking of human and animal learning processes, RL plays a key role in computational psychiatry. RL in computational psychiatry proposes to map brain functioning in an algorithmic language offering then the possibility to explore, through simulations, the dysfunctioning of these processes as well as the theoretical benefit of interventional strategies. Two examples will be further developed here and the readers can refer to ( Seriès, 2020 ) for an overview of more computational psychiatry methods, models and study cases.
In a RL framework, actions by the learner are chosen according to their value function, which holds the expected accumulated reward. The value function is updated through experience using feedback from the environment to the action taken. This update is also called temporal difference. An analogy has been drawn between this temporal difference and reward-error signals carried by dopamine in decision-making. Temporal difference reinforcement learning algorithms learn by estimating a value function based on temporal differences. The learning stops as this different converges to zero (see Supplementary Material for further details). Such a framework can be used to reframe addiction as a decision-making process gone awry. Based on the observation that addictive drugs produce a transient increase in dopamine through neuropharmacological mechanisms, the proposed model assumes that an addictive drug produces a positive temporal difference independent of the value function so that the action of taking drug will be always preferred over other actions ( Redish, 2004 ). This model provides a tool to explore the efficiency of public health strategies. For instance, the model proposes some hypotheses to explain the incomplete success of strategies based on offering money as an alternate choice from drug intake.
RL models are used for the analysis of data of cognitive tasks and in particular tasks related to decision-making. Instead of focusing on the summary statistics of such tests (e.g, total number of errors), RL-based approaches allow for the integration of trial-by-trial data similarly to what model-based approaches typically do—with longitudinal data analysis—to better decipher response variability via the characterization of PK and PD processes. In the same way, trial-by-trial data can be leveraged to estimate RL-model based parameters which, in turn, can be compared to clinical endpoints such as measures of symptom severity to disentangle the role of brain circuit mechanisms overall contributing to a better understanding of response variability. RL for cognitive testing data in psychiatric populations is a complete paradigm change with respect to its application for precision dosing problems. While–in the two previous examples—RL was used to solve the problem of optimal dosing, now the RL algorithm is mapped to neuro-cognitive processes. Quantitatively characterizing these processes for each patient (estimating parameters from RL algorithms) is proposed as a methodology for extracting relevant information towards disease characterization and thus, response variability.
In ( Huys et al., 2013 ), the authors use RL models to analyse population data of a behavioural test (signal-detection task) to study aspects of anhedonia—a core symptom of depression—related to reward learning. The authors proposed a RL model based on Q-learning update integrating two parameters: the classical learning rate and a parameter related to reward sensitivity modulating the percentage of the reward value actually contributes to the update of the Q value function. By performing a correlation analysis of the inferred parameters with anhedonic depression questionnaire, the authors found a negative correlation between the reward sensitivity but no correlation with the learning rate. Overall, these results led to the conclusion that the sensitivity to the reward and not the learning rate could be the main driver explaining why in anhedonic individuals, reward has less impact than in non-anhedonic individuals. Unravelling these two mechanisms is important for the planning of successful digital, behavioural and pharmacological strategies. The right column in Table 1 depicts the summary characteristics of RL applied to that study.
4 Conclusion
In this perspective, we have illustrated the flexibility of RL framework throughout the described applications in precision dosing, digital health and computational psychiatry and with that have demonstrated the benefit for the modeling community to become familiar with these approaches. The contrary is also true, and the field of precision digital therapeutics and computational psychiatry can benefit much from a proximity to the PMX community.
First, PMX methods could make RL even better. The field of computational psychiatry could benefit from input from the PMX community when it comes to statistical aspects related to parameters inference and clinical endpoint modelling. Two areas for which PMX has adopted as its state-of-the-art, population approach (with powerful algorithms such as stochastic approximation expectation-maximization algorithm ( Lavielle, 2014 )) and joint modelling respectively.
Second, the field of digital health should benefit from what constitutes one of the essential objectives of model-based drug development approaches, namely: elucidating response variability. It is particularly important for the successful development of digital therapeutic interventions to know how to characterize the efficacy and safety profiles and to know how to develop personalization strategies based on this understanding. The fact that it is about digital interventions should not prevent developers from prioritizing research in understanding underlying causal biological and (patho)-physiological processes of response, which will always be a key factor of successful therapy development, either pharmacological or not. Figure 1 proposes an illustration of these mutual benefits.

Illustration of the mutual benefits of increased permeability between model-based approaches to precision dosing and digital health, on one hand, and computational psychiatry on the other hand.
Table 1 : Main characteristics of RL algorithm implementation to the precision dosing of pharmacological interventions (left column); the precision dosing of digital intervention (middle column); and computational psychiatry (right column). While there are multiple similarities between the precision dosing of pharmacological and digital interventions, the application of RL in computational psychiatry shows as a paradigm shift. RL computational machinery is not deployed as a technical approach to address the optimal control problem of precision dosing but is fitted to (cognitive task) data assuming the algorithm itself present mechanistic similarities with how brain’s participants functioned during the task.
Acknowledgments
The author wishes to acknowledge Lucy Hutchinson, Richard Peck and Denis Engelmann for providing inputs on the drat manuscript.
Data availability statement
Author contributions.
BR: manuscript writing.
Conflict of interest
The author is employed by F. Hoffmann La Roche Ltd.
Publisher’s note
All claims expressed in this article are solely those of the authors and do not necessarily represent those of their affiliated organizations, or those of the publisher, the editors and the reviewers. Any product that may be evaluated in this article, or claim that may be made by its manufacturer, is not guaranteed or endorsed by the publisher.
Supplementary material
The Supplementary Material for this article can be found online at: https://www.frontiersin.org/articles/10.3389/fphar.2022.1094281/full#supplementary-material
- Bloomingdale P., Karelina T., Cirit M., Muldoon S. F., Baker J., McCarty W. J., et al. (2021). Quantitative systems pharmacology in neuroscience: Novel methodologies and technologies . CPT Pharmacometrics Syst. Pharmacol. 10 ( 5 ), 412–419. 10.1002/psp4.12607 [ PMC free article ] [ PubMed ] [ CrossRef ] [ Google Scholar ]
- Bram D. S., Parrott N., Hutchinson L., Steiert B. (2022). Introduction of an artificial neural network-based method for concentration-time predictions . CPT Pharmacometrics Syst. Pharmacol. 11 ( 6 ), 745–754. 10.1002/psp4.12786 [ PMC free article ] [ PubMed ] [ CrossRef ] [ Google Scholar ]
- Claes S. (2022), Mobile health revolution in healthcare: Are we ready? Metaforum position paper 17 2019 [cited 2022 October 10]; Available at: https://www.kuleuven.be/metaforum/visie-en-debatteksten/2019-mobile-health-revolution-in-healthcare .
- Conrado D. J., Duvvuri S., Geerts H., Burton J., Biesdorf C., Ahamadi M., et al. (2020). Challenges in alzheimer's disease drug discovery and development: The role of modeling, simulation, and open data . Clin. Pharmacol. Ther. 107 ( 4 ), 796–805. 10.1002/cpt.1782 [ PubMed ] [ CrossRef ] [ Google Scholar ]
- Crocker L. D., Heller W., Warren S. L., O'Hare A. J., Infantolino Z. P., Miller G. A. (2013). Relationships among cognition, emotion, and motivation: Implications for intervention and neuroplasticity in psychopathology . Front. Hum. Neurosci. 7 , 261. 10.3389/fnhum.2013.00261 [ PMC free article ] [ PubMed ] [ CrossRef ] [ Google Scholar ]
- Fleisch E., Franz C., Herrmann A. (2021). The digital pill . [ Google Scholar ]
- Friberg L. E., Henningsson A., Maas H., Nguyen L., Karlsson M. O. (2002). Model of chemotherapy-induced myelosuppression with parameter consistency across drugs . J. Clin. Oncol. 20 ( 24 ), 4713–4721. 10.1200/JCO.2002.02.140 [ PubMed ] [ CrossRef ] [ Google Scholar ]
- Geerts H., Wikswo J., van der Graaf P. H., Bai J. P. F., Gaiteri C., Bennett D., et al. (2020). Quantitative systems pharmacology for neuroscience drug discovery and development: Current status, opportunities, and challenges . CPT Pharmacometrics Syst. Pharmacol. 9 ( 1 ), 5–20. 10.1002/psp4.12478 [ PMC free article ] [ PubMed ] [ CrossRef ] [ Google Scholar ]
- Henin E., Meille C., Barbolosi D., You B., Guitton J., Iliadis A., et al. (2016). Revisiting dosing regimen using PK/PD modeling: The MODEL1 phase I/II trial of docetaxel plus epirubicin in metastatic breast cancer patients . Breast Cancer Res. Treat. 156 ( 2 ), 331–341. 10.1007/s10549-016-3760-9 [ PubMed ] [ CrossRef ] [ Google Scholar ]
- Huys Q. J., Pizzagalli D. A., Bogdan R., Dayan P. (2013). Mapping anhedonia onto reinforcement learning: A behavioural meta-analysis . Biol. Mood Anxiety Disord. 3 ( 1 ), 12. 10.1186/2045-5380-3-12 [ PMC free article ] [ PubMed ] [ CrossRef ] [ Google Scholar ]
- Insel T., Cuthbert B., Garvey M., Heinssen R., Pine D. S., Quinn K., et al. (2010). Research domain criteria (RDoC): Toward a new classification framework for research on mental disorders . Am. J. Psychiatry 167 ( 7 ), 748–751. 10.1176/appi.ajp.2010.09091379 [ PubMed ] [ CrossRef ] [ Google Scholar ]
- Insel T. R., Cuthbert B. N. (2015). Medicine. Brain disorders? Precisely . Science 348 ( 6234 ), 499–500. 10.1126/science.aab2358 [ PubMed ] [ CrossRef ] [ Google Scholar ]
- Jacobson N. C., Kowatsch T., Marsch L. A. (2022). Digital therapeutics for mental health and addiction: The state of the science and vision for the future . San Diego, CA: Academic Press, 270. [ Google Scholar ]
- Klasnja P., Hekler E. B., Shiffman S., Boruvka A., Almirall D., Tewari A., et al. (2015). Microrandomized trials: An experimental design for developing just-in-time adaptive interventions . Health Psychol. 34S , 1220–1228. 10.1037/hea0000305 [ PMC free article ] [ PubMed ] [ CrossRef ] [ Google Scholar ]
- Lavielle M. (2014). Mixed effects models for the population approach: Models, tasks, methods and tools . 1st edition. Chapman and Hall/CRC. [ Google Scholar ]
- Lee I. M., Shiroma E. J., Lobelo F., Puska P., Blair S. N., Katzmarzyk P. T., et al. (2012). Effect of physical inactivity on major non-communicable diseases worldwide: An analysis of burden of disease and life expectancy . Lancet 380 ( 9838 ), 219–229. 10.1016/S0140-6736(12)61031-9 [ PMC free article ] [ PubMed ] [ CrossRef ] [ Google Scholar ]
- Liao P., Greenewald K., Klasnja P., Murphy S. (2020). Personalized HeartSteps: A reinforcement learning algorithm for optimizing physical activity . Proc. ACM Interact. Mob. Wearable Ubiquitous Technol. 4 ( 1 ), 18. 10.1145/3381007 [ PMC free article ] [ PubMed ] [ CrossRef ] [ Google Scholar ]
- Lu J., Deng K., Zhang X., Liu G., Guan Y. (2021). Neural-ODE for pharmacokinetics modeling and its advantage to alternative machine learning models in predicting new dosing regimens . iScience 24 ( 7 ), 102804. 10.1016/j.isci.2021.102804 [ PMC free article ] [ PubMed ] [ CrossRef ] [ Google Scholar ]
- Maier C., Hartung N., Kloft C., Huisinga W., de Wiljes J. (2021). Reinforcement learning and Bayesian data assimilation for model-informed precision dosing in oncology . CPT Pharmacometrics Syst. Pharmacol. 10 ( 3 ), 241–254. 10.1002/psp4.12588 [ PMC free article ] [ PubMed ] [ CrossRef ] [ Google Scholar ]
- Maxfield K., Zineh I. (2021). Precision dosing: A clinical and public health imperative . JAMA 325 ( 15 ), 1505–1506. 10.1001/jama.2021.1004 [ PubMed ] [ CrossRef ] [ Google Scholar ]
- Montague P. R., Dolan R. J., Friston K. J., Dayan P. (2012). Computational psychiatry . Trends Cogn. Sci. 16 ( 1 ), 72–80. 10.1016/j.tics.2011.11.018 [ PMC free article ] [ PubMed ] [ CrossRef ] [ Google Scholar ]
- Nahum-Shani I., Smith S. N., Spring B. J., Collins L. M., Witkiewitz K., Tewari A., et al. (2018). Just-in-Time adaptive interventions (JITAIs) in mobile health: Key components and design principles for ongoing health behavior support . Ann. Behav. Med. 52 ( 6 ), 446–462. 10.1007/s12160-016-9830-8 [ PMC free article ] [ PubMed ] [ CrossRef ] [ Google Scholar ]
- Pao W., Nagel Y. A. (2022). Paradigms for the development of transformative medicines-lessons from the EGFR story . Ann. Oncol. 33 ( 5 ), 556–560. 10.1016/j.annonc.2022.02.005 [ PubMed ] [ CrossRef ] [ Google Scholar ]
- Peck R. W. (2021). Precision dosing: An industry perspective . Clin. Pharmacol. Ther. 109 ( 1 ), 47–50. [ PMC free article ] [ PubMed ] [ Google Scholar ]
- Qian T., Klasnja P., Murphy S. A. (2020). Linear mixed models with endogenous covariates: Modeling sequential treatment effects with application to a mobile health study . Stat. Sci. 35 ( 3 ), 375–390. 10.1214/19-sts720 [ PMC free article ] [ PubMed ] [ CrossRef ] [ Google Scholar ]
- Qian T., Walton A. E., Collins L. M., Klasnja P., Lanza S. T., Nahum-Shani I., et al. (2022). The microrandomized trial for developing digital interventions: Experimental design and data analysis considerations . Psychol. Methods 27 , 874–894. 10.1037/met0000283 [ PMC free article ] [ PubMed ] [ CrossRef ] [ Google Scholar ]
- Redish A. D. (2004). Addiction as a computational process gone awry . Science 306 ( 5703 ), 1944–1947. 10.1126/science.1102384 [ PubMed ] [ CrossRef ] [ Google Scholar ]
- Ribba B., et al. (2022). Model enhanced reinforcement learning to enable precision dosing: A theoretical case study with dosing of propofol . CPT Pharmacometrics Syst Pharmacol. [ PMC free article ] [ PubMed ] [ Google Scholar ]
- Ribba B., Kaloshi G., Peyre M., Ricard D., Calvez V., Tod M., et al. (2012). A tumor growth inhibition model for low-grade glioma treated with chemotherapy or radiotherapy . Clin. Cancer Res. 18 ( 18 ), 5071–5080. 10.1158/1078-0432.CCR-12-0084 [ PubMed ] [ CrossRef ] [ Google Scholar ]
- Russo D. J., Van Roy B., Kazerouni A., Osband I., Wen Z. (2018). A tutorial on Thompson sampling . Found. Trends® Mach. Learn. 11 ( 1 ), 1–96. 10.1561/2200000070 [ CrossRef ] [ Google Scholar ]
- Russo D., Van Roy B. (2014). Learning to optimize via posterior sampling . Math. Operations Res. 39 ( 4 ), 1221–1243. 10.1287/moor.2014.0650 [ CrossRef ] [ Google Scholar ]
- Seriès P. E. (2020). Computational psychiatry . The MIT Press. [ Google Scholar ]
- Sutton R., Barto A. (2018). Reinforcement learning: An introduction . Second edition. [ Google Scholar ]
- Sverdlov O., van Dam J., Hannesdottir K., Thornton-Wells T. (2018). Digital therapeutics: An integral component of digital innovation in drug development . Clin. Pharmacol. Ther. 104 ( 1 ), 72–80. 10.1002/cpt.1036 [ PubMed ] [ CrossRef ] [ Google Scholar ]
- Wang D., Song Z., Zhang C., Chen P. (2021). Bispectral index monitoring of the clinical effects of propofol closed-loop target-controlled infusion: Systematic review and meta-analysis of randomized controlled trials . Med. Baltim. 100 ( 4 ), e23930. 10.1097/MD.0000000000023930 [ PMC free article ] [ PubMed ] [ CrossRef ] [ Google Scholar ]
- Yauney G., Shah P. (2018). “ Reinforcement learning with action-derived rewards for chemotherapy and clinical trial dosing regimen selection ,” in Proceedings of the 3rd Machine Learning for Healthcare Conference (PMLR: Proceedings of Machine Learning Research; ), 161–226. D.-V. Finale, et al., Editors. [ Google Scholar ]
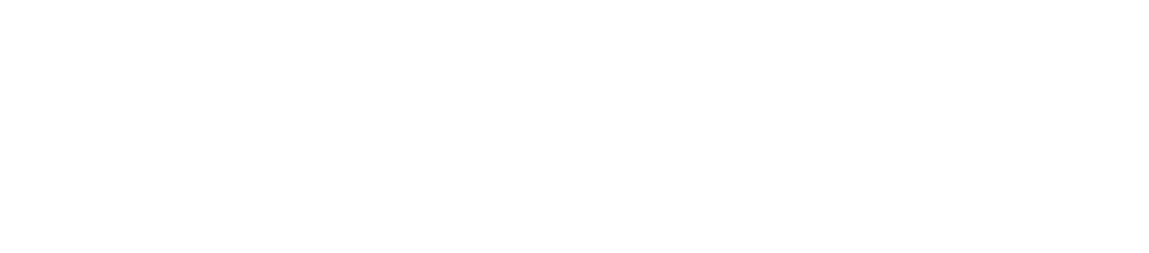
Departments
- Applied Physics
- Biomedical Engineering
- Center for Urban Science and Progress
- Chemical and Biomolecular Engineering
- Civil and Urban Engineering
- Computer Science and Engineering
- Electrical and Computer Engineering
- Finance and Risk Engineering
- Mathematics
- Mechanical and Aerospace Engineering
- Technology, Culture and Society
- Technology Management and Innovation
Degrees & Programs
- Bachelor of Science
- Master of Science
- Doctor of Philosophy
- Digital Learning
- Certificate Programs
- NYU Tandon Bridge
- Undergraduate
- Records & Registration
- Digital Learning Services
- Teaching Innovation
- Explore NYU Tandon
- Year in Review
- Strategic Plan
- Diversity & Inclusion
News & Events
- Social Media
Looking for News or Events ?
Robust reinforcement learning: A case study in linear quadratic regulation
This research, whose principal author is Ph.D. student Bo Pang, was directed by Zhong-Ping Jiang, professor in the Department of Electrical and Computer Engineering.
As an important and popular method in reinforcement learning (RL), policy iteration has been widely studied by researchers and utilized in different kinds of real-life applications by practitioners.
Policy iteration involves two steps: policy evaluation and policy improvement. In policy evaluation, a given policy is evaluated based on a scalar performance index. Then this performance index is utilized to generate a new control policy in policy improvement. These two steps are iterated in turn, to find the solution of the RL problem at hand. When all the information involved in this process is exactly known, the convergence to the optimal solution can be provably guaranteed, by exploiting the monotonicity property of the policy improvement step. That is, the performance of the newly generated policy is no worse than that of the given policy in each iteration.
However, in practice policy evaluation or policy improvement can hardly be implemented precisely, because of the existence of various errors, which may be induced by function approximation, state estimation, sensor noise, external disturbance and so on. Therefore, a natural question to ask is: when is a policy iteration algorithm robust to the errors in the learning process? In other words, under what conditions on the errors does the policy iteration still converge to (a neighborhood of) the optimal solution? And how to quantify the size of this neighbourhood?
This paper studies the robustness of reinforcement learning algorithms to errors in the learning process. Specifically, they revisit the benchmark problem of discrete-time linear quadratic regulation (LQR) and study the long-standing open question: Under what conditions is the policy iteration method robustly stable from a dynamical systems perspective?
Using advanced stability results in control theory, they show that policy iteration for LQR is inherently robust to small errors in the learning process and enjoys small-disturbance input-to-state stability: whenever the error in each iteration is bounded and small, the solutions of the policy iteration algorithm are also bounded, and, moreover, enter and stay in a small neighbourhood of the optimal LQR solution. As an application, a novel off-policy optimistic least-squares policy iteration for the LQR problem is proposed, when the system dynamics are subjected to additive stochastic disturbances. The proposed new results in robust reinforcement learning are validated by a numerical example.
This work was supported in part by the U.S. National Science Foundation.
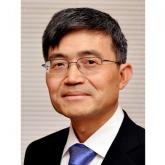
Zhong-Ping Jiang
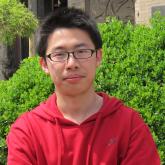
More to Read
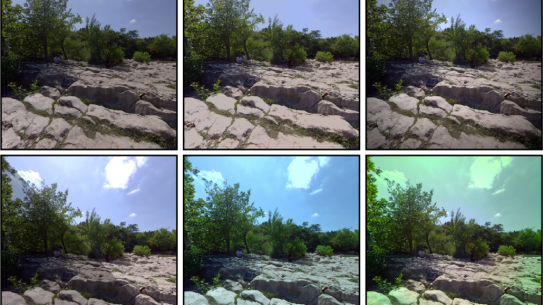
Immersive Computing Lab teams up with Meta to uncover how energy-saving tactics affect perceived quality of XR experiences
Stakeholders in the national artificial intelligence research resource (nairr) convene for high-level discussions at nyu, towards open and standardized human mobility data: nyu tandon researchers address key challenges and solutions.
- Data Science
- Data Analysis
- Data Visualization
- Machine Learning
- Deep Learning
- Computer Vision
- Artificial Intelligence
- AI ML DS Interview Series
- AI ML DS Projects series
- Data Engineering
- Web Scrapping
Reinforcement learning
Reinforcement learning is an area of Machine Learning. It is about taking suitable action to maximize reward in a particular situation. It is employed by various software and machines to find the best possible behavior or path it should take in a specific situation. Reinforcement learning differs from supervised learning in a way that in supervised learning the training data has the answer key with it so the model is trained with the correct answer itself whereas in reinforcement learning, there is no answer but the reinforcement agent decides what to do to perform the given task. In the absence of a training dataset, it is bound to learn from its experience.
Reinforcement Learning (RL) is the science of decision making. It is about learning the optimal behavior in an environment to obtain maximum reward. In RL, the data is accumulated from machine learning systems that use a trial-and-error method. Data is not part of the input that we would find in supervised or unsupervised machine learning.
Reinforcement learning uses algorithms that learn from outcomes and decide which action to take next. After each action, the algorithm receives feedback that helps it determine whether the choice it made was correct, neutral or incorrect. It is a good technique to use for automated systems that have to make a lot of small decisions without human guidance.
Reinforcement learning is an autonomous, self-teaching system that essentially learns by trial and error. It performs actions with the aim of maximizing rewards, or in other words, it is learning by doing in order to achieve the best outcomes.
The problem is as follows: We have an agent and a reward, with many hurdles in between. The agent is supposed to find the best possible path to reach the reward. The following problem explains the problem more easily.
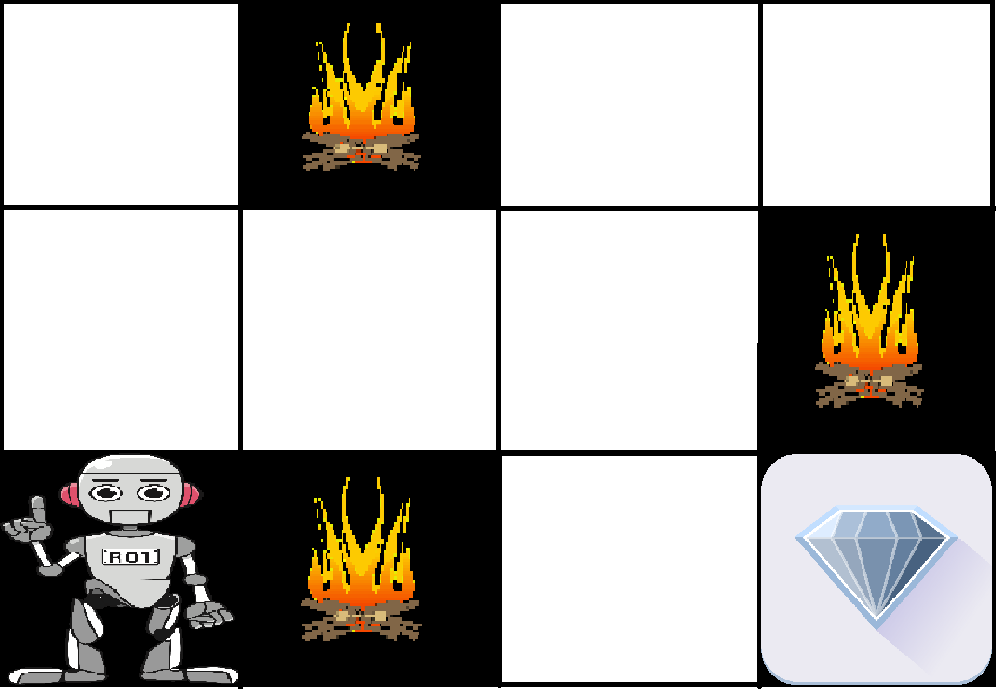
The above image shows the robot, diamond, and fire. The goal of the robot is to get the reward that is the diamond and avoid the hurdles that are fired. The robot learns by trying all the possible paths and then choosing the path which gives him the reward with the least hurdles. Each right step will give the robot a reward and each wrong step will subtract the reward of the robot. The total reward will be calculated when it reaches the final reward that is the diamond. Main points in Reinforcement learning –
- Input: The input should be an initial state from which the model will start
- Output: There are many possible outputs as there are a variety of solutions to a particular problem
- Training: The training is based upon the input, The model will return a state and the user will decide to reward or punish the model based on its output.
- The model keeps continues to learn.
- The best solution is decided based on the maximum reward.
Difference between Reinforcement learning and Supervised learning:
Reinforcement learning | Supervised learning |
---|---|
Reinforcement learning is all about making decisions sequentially. In simple words, we can say that the output depends on the state of the current input and the next input depends on the output of the previous input | In Supervised learning, the decision is made on the initial input or the input given at the start |
In Reinforcement learning decision is dependent, So we give labels to sequences of dependent decisions | In supervised learning the decisions are independent of each other so labels are given to each decision. |
Example: Chess game,text summarization | Example: Object recognition,spam detetction |
Types of Reinforcement:
There are two types of Reinforcement:
- Maximizes Performance
- Sustain Change for a long period of time
- Too much Reinforcement can lead to an overload of states which can diminish the results
- Increases Behavior
- Provide defiance to a minimum standard of performance
- It Only provides enough to meet up the minimum behavior
Elements of Reinforcement Learning
Reinforcement learning elements are as follows:
- Reward function
- Value function
- Model of the environment
Policy: Policy defines the learning agent behavior for given time period. It is a mapping from perceived states of the environment to actions to be taken when in those states.
Reward function: Reward function is used to define a goal in a reinforcement learning problem.A reward function is a function that provides a numerical score based on the state of the environment
Value function: Value functions specify what is good in the long run. The value of a state is the total amount of reward an agent can expect to accumulate over the future, starting from that state.
Model of the environment: Models are used for planning.
- Credit assignment problem: Reinforcement learning algorithms learn to generate an internal value for the intermediate states as to how good they are in leading to the goal. The learning decision maker is called the agent. The agent interacts with the environment that includes everything outside the agent.
The agent has sensors to decide on its state in the environment and takes action that modifies its state.
- The reinforcement learning problem model is an agent continuously interacting with an environment. The agent and the environment interact in a sequence of time steps. At each time step t, the agent receives the state of the environment and a scalar numerical reward for the previous action, and then the agent then selects an action.
Reinforcement learning is a technique for solving Markov decision problems.
- Reinforcement learning uses a formal framework defining the interaction between a learning agent and its environment in terms of states, actions, and rewards. This framework is intended to be a simple way of representing essential features of the artificial intelligence problem.
Various Practical Applications of Reinforcement Learning –
- RL can be used in robotics for industrial automation.
- RL can be used in machine learning and data processing
- RL can be used to create training systems that provide custom instruction and materials according to the requirement of students.
Application of Reinforcement Learnings
1. Robotics: Robots with pre-programmed behavior are useful in structured environments, such as the assembly line of an automobile manufacturing plant, where the task is repetitive in nature.
2. A master chess player makes a move. The choice is informed both by planning, anticipating possible replies and counter replies.
3. An adaptive controller adjusts parameters of a petroleum refinery’s operation in real time.
RL can be used in large environments in the following situations:
- A model of the environment is known, but an analytic solution is not available;
- Only a simulation model of the environment is given (the subject of simulation-based optimization)
- The only way to collect information about the environment is to interact with it.
Advantages and Disadvantages of Reinforcement Learning
Advantages of Reinforcement learning
1. Reinforcement learning can be used to solve very complex problems that cannot be solved by conventional techniques.
2. The model can correct the errors that occurred during the training process.
3. In RL, training data is obtained via the direct interaction of the agent with the environment
4. Reinforcement learning can handle environments that are non-deterministic, meaning that the outcomes of actions are not always predictable. This is useful in real-world applications where the environment may change over time or is uncertain.
5. Reinforcement learning can be used to solve a wide range of problems, including those that involve decision making, control, and optimization.
6. Reinforcement learning is a flexible approach that can be combined with other machine learning techniques, such as deep learning, to improve performance.
Disadvantages of Reinforcement learning
1. Reinforcement learning is not preferable to use for solving simple problems.
2. Reinforcement learning needs a lot of data and a lot of computation
3. Reinforcement learning is highly dependent on the quality of the reward function. If the reward function is poorly designed, the agent may not learn the desired behavior.
4. Reinforcement learning can be difficult to debug and interpret. It is not always clear why the agent is behaving in a certain way, which can make it difficult to diagnose and fix problems.
You can also read our recent article on Implementation – Reinforcement Learning Algorithm
Please Login to comment...
Similar reads, improve your coding skills with practice.
What kind of Experience do you want to share?
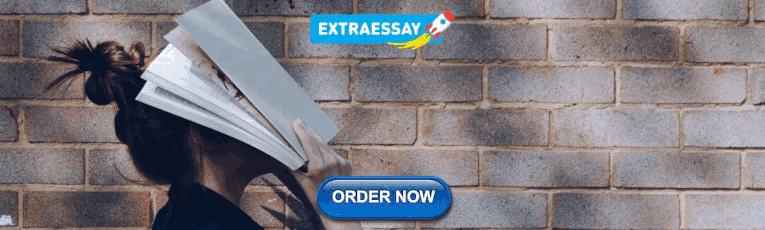
COMMENTS
Case Study: Playing Atari Games 42 Objective: Complete the game with the highest score State: Raw pixel inputs of the game state Action: Game controls e.g. Left, Right, Up, Down Reward: Score increase/decrease at each time step ... - Mix of supervised learning and reinforcement learning.
These studies highlight how reinforcement learning algorithms enable machines to learn and make decisions by interacting with their environment. From robotics and gaming to recommendation systems, each case study demonstrates the power of reinforcement learning in optimizing actions and achieving desired outcomes. With real-world examples, the ...
Reinforcement learning tutorials. 1. RL with Mario Bros - Learn about reinforcement learning in this unique tutorial based on one of the most popular arcade games of all time - Super Mario. 2. Machine Learning for Humans: Reinforcement Learning - This tutorial is part of an ebook titled 'Machine Learning for Humans'.
DeepTraffic is an open-source environment that combines the powers of Reinforcement Learning, Deep Learning, and Computer Vision to build algorithms used for autonomous driving launched by MIT. It simulates autonomous vehicles such as drones, cars, etc. Deep reinforcement learning in self-driving cars.
The computational study of reinforcement learning is now a large eld, with hundreds of active researchers around the world in di- ... In this case, it may be desirable to cover only a subset of the material. We recommend covering Chapter 1 for a brief overview, Chapter 2 through Section 2.2, Chapter 3 except Sections 3.4,
Reinforcement Learning Case Studies Case Study 1: Autonomous Vehicle Navigation. One of the most prominent applications of reinforcement learning in data science is autonomous vehicle navigation ...
Model-Based and Model-Free Reinforcement Learning: Pytennis Case Study. Reinforcement learning is a field of Artificial Intelligence in which you build an intelligent system that learns from its environment through interaction and evaluates what it learns in real-time. A good example of this is self-driving cars, or when DeepMind built what we ...
IBM Watson's application of reinforcement learning in health care has led to advances in treatment personalization and diagnosis accuracy. This cognitive computing system broke major ground in medical technology, with the potential to save lives and improve health outcomes significantly. 3. Tesla's Autopilot System.
Reinforcement Learning is a machine learning approach in which an agent interacts with their environment to gather information, and make an informed decision based on the accumulated information. ... Exploring Reinforcement Learning: A Case Study Applied to the Popular Snake Game. In: Dingli, A., Pfeiffer, A., Serada, A., Bugeja, M., Bezzina, S ...
Next, I try to put RL and control techniques on the same footing through a case study of the linear quadratic regulator (LQR) with unknown dynamics. This baseline will illuminate the var- ... 2 What is reinforcement learning? Reinforcement learning is the study of how to use past data to enhance the future manipulation of a dynamical system ...
2. Natural Language Processing. Predictive text, text summarization, question answering, and machine translation are all examples of natural language processing (NLP) that uses reinforcement learning. By studying typical language patterns, RL agents can mimic and predict how people speak to each other every day.
In this paper, we presented a case study of a novel RL-based approach to lateral control. Input to the agent is a trajectory consisting of several waypoints ahead, whereas the output consists of steering commands. In addition to waypoint information, the state-space uses several ego vehicle parameters such as the wheel speed, and engine rpm.
Reinforcement learning (RL) algorithms have recently seen rapid advancement and adoption in the field of electronic design automation (EDA) in both academia and industry. In this paper, we first give an overview of RL and its applications in EDA. In particular, we discuss three case studies: chip macro placement, analog transistor sizing, and logic synthesis. In collaboration with Google Brain ...
Abstract—This paper studies the robustness aspect of reinforcement learning algorithms in the presence of errors. Specifically, we revisit the benchmark problem of discrete-time linear quadratic regulation (LQR) and study the long-standing open question: Under what conditions is the policy iteration method robustly stable for dynamical ...
increased ROI, profit margins. predicting the choices, reactions, and behavior of customers towards your products/services. 2. RL in Broadcast Journalism. Through different types of Reinforcement Learning, attracting likes and views along with tracking the reader's behavior is much simpler.
This paper introduces a reinforcement learning (RL) platform that enhances end-to-end user journeys in healthcare digital tools through personalization. We explore a case study with SwipeRx, the most popular all-in-one app for pharmacists in Southeast Asia, demonstrating how the platform can be used to personalize and adapt user experiences. Our RL framework is tested through a series of ...
A Reinforcement learning model consists of an agent which infers an action which then acts on the environment to make a change, and the significance of the action is reflected using a reward function.
For instance, the clinical study "MODEL1" was a phase I/II trial and a clear clinical attempt of a personalized dosing regimen of docetaxel and epirubicin patients with metastatic breast cancer and was shown to lead to improved efficacy-toxicity balance (Henin et al., 2016). Reinforcement learning (RL) was also used for precision dosing.
This paper studies the robustness of reinforcement learning algorithms to errors in the learning process. Specifically, they revisit the benchmark problem of discrete-time linear quadratic regulation (LQR) and study the long-standing open question: Under what conditions is the policy iteration method robustly stable from a dynamical systems ...
To address this problem, this study introduces a novel safe reinforcement learning algorithm that satisfies joint chance constraints with a high probability for multi-constraint gold cyanide leaching processes. In particular, the proposed algorithm employs chance control barrier functions to maintain the state within the desired safe set with ...
5. Reinforcement learning can be used to solve a wide range of problems, including those that involve decision making, control, and optimization. 6. Reinforcement learning is a flexible approach that can be combined with other machine learning techniques, such as deep learning, to improve performance. Disadvantages of Reinforcement learning. 1.
Combining global reinforcement learning with local heuristic algorithms, HDRL improves decision-making speed and efficiency in economic dispatch under uncertain conditions. ... The case study ...