3D face recognition: A comprehensive survey in 2022
- Review Article
- Open access
- Published: 05 August 2023
- Volume 9 , pages 657–685, ( 2023 )
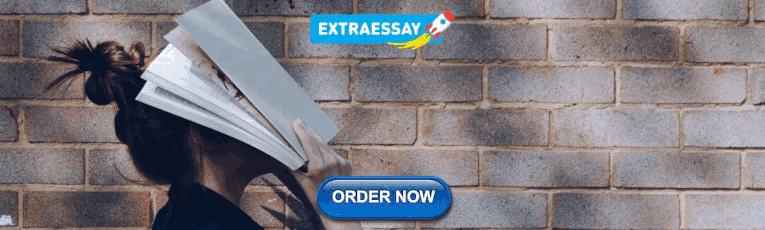
Cite this article
You have full access to this open access article
- Yaping Jing 1 ,
- Xuequan Lu 1 &
- Shang Gao 1
3293 Accesses
7 Citations
4 Altmetric
Explore all metrics
In the past ten years, research on face recognition has shifted to using 3D facial surfaces, as 3D geometric information provides more discriminative features. This comprehensive survey reviews 3D face recognition techniques developed in the past decade, both conventional methods and deep learning methods. These methods are evaluated with detailed descriptions of selected representative works. Their advantages and disadvantages are summarized in terms of accuracy, complexity, and robustness to facial variations (expression, pose, occlusion, etc.). A review of 3D face databases is also provided, and a discussion of future research challenges and directions of the topic.
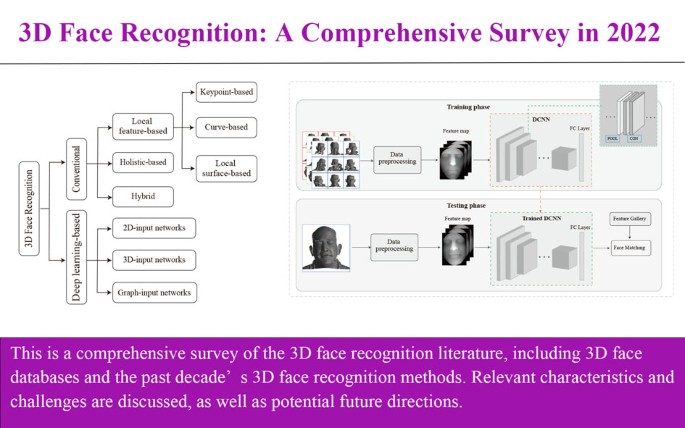
Article PDF
Download to read the full article text
Similar content being viewed by others
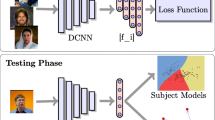
Deep-learned faces: a survey
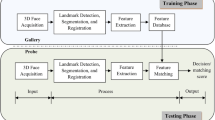
3D face recognition: a survey
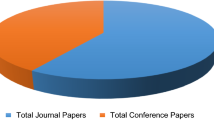
On the frontiers of pose invariant face recognition: a review
Explore related subjects.
- Artificial Intelligence
Avoid common mistakes on your manuscript.
Patil, H.; Kothari, A.; Bhurchandi, K. 3-D face recognition: Features, databases, algorithms and challenges. Artificial Intelligence Review Vol. 44, No. 3, 393–441, 2015.
Article Google Scholar
Zhou, H. L.; Mian, A.; Wei, L.; Creighton, D.; Hossny, M.; Nahavandi, S. Recent advances on singlemodal and multimodal face recognition: A survey. IEEE Transactions on Human-Machine Systems Vol. 44, No. 6, 701–716, 2014.
Bowyer, K. W.; Chang, K.; Flynn, P. A survey of approaches and challenges in 3D and multi-modal 3D + 2D face recognition. Computer Vision and Image Understanding Vol. 101, No. 1, 1–15, 2006.
Huang, G. B.; Mattar, M.; Berg, T.; Learned-Miller, E. Labeled faces in the wild: A database for studying face recognition in unconstrained environments. In: Proceedings of the Workshop on Faces in ‘Real-Life’ Images: Detection, Alignment, and Recognition, 2008.
Cai, Y.; Lei, Y. J.; Yang, M. L.; You, Z. S.; Shan, S. G. A fast and robust 3D face recognition approach based on deeply learned face representation. Neurocomputing Vol. 363, 375–397, 2019.
Zhou, S.; Xiao, S. 3D face recognition: A survey. Human-Centric Computing and Information Sciences Vol. 8, No. 1, 35, 2018.
Blackburn, D. M.; Bone, M.; Phillips, P. J. Face recognition vendor test 2000: Evaluation report. Technical report. Defense Advanced Research Projects Agency Arlington VA, 2001.
Book Google Scholar
Phillips, P. J.; Grother, P.; Micheals, R.; Blackburn, D. M.; Tabassi, E.; Bone, M. Face recognition vendor test 2002. In: Proceedings of the IEEE International SOI Conference, 44, 2003.
Phillips, P. J.; Flynn, P. J.; Scruggs, T.; Bowyer, K. W.; Chang, J.; Hoffman, K.; Marques, J.; Min, J.; Worek, W. Overview of the face recognition grand challenge. In: Proceedings of the IEEE Computer Society Conference on Computer Vision and Pattern Recognition, 947–954, 2005.
Phillips, P. J.; Scruggs, W. T.; O’Toole, A. J.; Flynn, P. J.; Bowyer, K. W.; Schott, C. L.; Sharpe, M. FRVT 2006 and ICE 2006 large-scale experimental results. IEEE Transactions on Pattern Analysis and Machine Intelligence Vol. 32, No. 5, 831–846, 2010.
Abate, A. F.; Nappi, M.; Riccio, D.; Sabatino, G. 2D and 3D face recognition: A survey. Pattern Recognition Letters Vol. 28, No. 14, 1885–1906, 2007.
Smeets, D.; Claes, P.; Hermans, J.; Vandermeulen, D.; Suetens, P. A comparative study of 3-D face recognition under expression variations. IEEE Transactions on Systems, Man, and Cybernetics, Part C (Applications and Reviews) Vol. 42, No. 5, 710–727, 2012.
Soltanpour, S.; Boufama, B.; Jonathan Wu, Q. M. A survey of local feature methods for 3D face recognition. Pattern Recognition Vol. 72, 391–406, 2017.
Guo, G. D.; Zhang, N. A survey on deep learning based face recognition. Computer Vision and Image Understanding Vol. 189, 102805, 2019.
Masi, I.; Wu, Y.; Hassner, T.; Natarajan, P. Deep face recognition: A survey. In: Proceedings of the 31st SIBGRAPI Conference on Graphics, Patterns and Images, 471–478, 2018.
Parkhi, O. M.; Vedaldi, A.; Zisserman, A. Deep face recognition. In: Proceedings of the British Machine Vision Conference, 1–12, 2015.
He, K. M.; Zhang, X. Y.; Ren, S. Q.; Sun, J. Deep residual learning for image recognition. In: Proceedings of the IEEE Conference on Computer Vision and Pattern Recognition, 770–778, 2016.
Lawrence, S.; Giles, C. L.; Tsoi, A. C.; Back, A. D. Face recognition: A convolutional neural-network approach. IEEE Transactions on Neural Networks Vol. 8, No. 1, 98–113, 1997.
Howard, A.; Zhmoginov, A.; Chen, L. C.; Sandler, M.; Zhu, M. Inverted residuals and linear bottlenecks: Mobile networks for classification, detection and segmentation. arXiv preprint arXiv:1801.04381, 2018.
Colombo, A.; Cusano, C.; Schettini, R. UMBDB: A database of partially occluded 3D faces. In: Proceedings of the IEEE International Conference on Computer Vision Workshops, 2113–2119, 2011.
Beumier, C.; Acheroy, M. Automatic 3D face authentication. Image and Vision Computing Vol. 18, No. 4, 315–321, 2000.
Article MATH Google Scholar
Hesher, C.; Srivastava, A.; Erlebacher, G. A novel technique for face recognition using range imaging. In: Proceedings of the 7th International Symposium on Signal Processing and Its Applications, 201–204, 2003.
Moreno, A. GavabDB: A 3D face database. In: Proceedings of the 2nd COST275 Workshop on Biometrics on the Internet, 75–80, 2004.
Chang, K. I.; Bowyer, K. W.; Flynn, P. J. An evaluation of multimodal 2D 3D face biometrics. IEEE Transactions on Pattern Analysis and Machine Intelligence Vol. 27, No. 4, 619–624, 2005.
Wang, Y. M.; Pan, G.; Wu, Z. H.; Wang, Y. G. Exploring facial expression effects in 3D face recognition using partial ICP. In: Computer Vision–ACCV 2006 . Lecture Notes in Computer Science , Vol. 3851 . Narayanan, P. J.; Nayar, S. K.; Shum, H. Y. Eds. Springer Berlin Heidelberg, 581–590, 2006.
Chapter Google Scholar
Yin, L. J.; Wei, X. Z.; Sun, Y.; Wang, J.; Rosato, M. J. A 3D facial expression database for facial behavior research. In: Proceedings of the 7th International Conference on Automatic Face and Gesture Recognition, 211–216, 2006.
Xu, C. H.; Tan, T. N.; Li, S.; Wang, Y. H.; Zhong, C. Learning effective intrinsic features to boost 3D-based face recognition. In: Computer Vision–ECCV 2006 . Lecture Notes in Computer Science , Vol. 3952 . Leonardis, A.; Bischof, H.; Pinz, A. Eds. Springer Berlin Heidelberg, 416–427, 2006.
Conde, C.; Serrano, A.; Cabello, E. Multimodal 2D, 2.5D & 3D face verification. In: Proceedings of the International Conference on Image Processing, 2061–2064, 2006.
Faltemier, T. C.; Bowyer, K. W.; Flynn, P. J. Using a multi-instance enrollment representation to improve 3D face recognition. In: Proceedings of the IEEE International Conference on Biometrics: Theory, Applications, and Systems, 1–6, 2007.
Savran, A.; Alyüz, N.; Dibeklioğlu, H.; Çeliktutan, O.; Gökberk, B.; Sankur, B.; Akarun, L. Bosphorus database for 3D face analysis. In: Biometrics and Identity Management . Lecture Notes in Computer-Science , Vol. 5372 . Schouten, B.; Juul, N. C.; Drygajlo, A.; Tistarelli, M. Eds. Springer Berlin Heidelberg, 47–56, 2008.
Heseltine, T.; Pears, N.; Austin, J. Three-dimensional face recognition using combinations of surface feature map subspace components. Image and Vision Computing Vol. 26, No. 3, 382–396, 2008.
Ter Haar, F. B.; Daoudi, M.; Veltkamp, R. C. SHape REtrieval contest 2008: 3D face scans. In: Proceedings of the IEEE International Conference on Shape Modeling and Applications, 225–226, 2008.
Yin, B. C.; Sun, Y. F.; Wang, C. Z.; Gai, Y. BJUT-3D large scale 3D face database and information processing. Journal of Computer Research and Development Vol. 46, No. 6, 1009–1018, 2009. (in Chinese)
Google Scholar
Gupta, S.; Castleman, K. R.; Markey, M. K.; Bovik, A. C. Texas 3D face recognition database. In: Proceedings of the IEEE Southwest Symposium on Image Analysis & Interpretation, 97–100, 2010.
Vijayan, V.; Bowyer, K. W.; Flynn, P. J.; Huang, D.; Chen, L. M.; Hansen, M.; Ocegueda, O.; Shah, S. K.; Kakadiaris, I. A. Twins 3D face recognition challenge. In: Proceedings of the International Joint Conference on Biometrics, 1–7, 2011.
Veltkamp, R.; van Jole, S.; Drira, H.; Amor, B.; Daoudi, M.; Li, H. B.; Chen, L. M.; Claes, P.; Smeets, D.; Hermans, J.; et al. SHREC’11 track: 3D face models retrieval. In: Proceedings of the 4th Eurographics Conference on 3D Object Retrieval, 89–95, 2011.
Zhang, Y.; Guo, Z.; Lin, Z.; Zhang, H.; Zhang, C. The NPU multi-case Chinese 3D face database and information processing. Chinese Journal of Electronics Vol. 21, No. 2, 283–286, 2012.
Zhang, X.; Yin, L. J.; Cohn, J. F.; Canavan, S.; Reale, M.; Horowitz, A.; Peng, L. A high-resolution spontaneous 3D dynamic facial expression database. In: Proceedings of the 10th IEEE International Conference and Workshops on Automatic Face and Gesture Recognition, 1–6, 2013.
Min, R.; Kose, N.; Dugelay, J. L. KinectFaceDB: A kinect database for face recognition. IEEE Transactions on Systems, Man, and Cybernetics: Systems Vol. 44, No. 11, 1534–1548, 2014.
Zhang, J. J.; Huang, D.; Wang, Y. H.; Sun, J. Lock3DFace: A large-scale database of low-cost Kinect 3D faces. In: Proceedings of the International Conference on Biometrics, 1–8, 2016.
Urbanová, P.; Ferková, Z.; Jandová, M.; Jurda, M.; Černý, D.; Sochor, J. Introducing the FIDENTIS 3D face database. Anthropological Review Vol. 81, No. 2, 202–223, 2018.
Zulqarnain Gilani, S.; Mian, A. Learning from millions of 3D scans for large-scale 3D face recognition. In: Proceedings of the IEEE/CVF Conference on Computer Vision and Pattern Recognition, 1896–1905, 2018.
Cheng, S. Y.; Kotsia, I.; Pantic, M.; Zafeiriou, S. 4DFAB: A large scale 4D database for facial expression analysis and biometric applications. In: Proceedings of the IEEE/CVF Conference on Computer Vision and Pattern Recognition, 5117–5126, 2018.
Jia, S.; Li, X.; Hu, C. B.; Guo, G. D.; Xu, Z. Q. 3D face anti-spoofing with factorized bilinear coding. arXiv preprint arXiv:2005.06514, 2020.
Ye, Y. P.; Song, Z.; Guo, J. G.; Qiao, Y. SIAT-3DFE: A high-resolution 3D facial expression dataset. IEEE Access Vol. 8, 48205–48211, 2020.
Yang, H. T.; Zhu, H.; Wang, Y. R.; Huang, M. K.; Shen, Q.; Yang, R. G.; Cao, X. FaceScape: A large-scale high quality 3D face dataset and detailed riggable 3D face prediction. In: Proceedings of the IEEE/CVF Conference on Computer Vision and Pattern Recognition, 598–607, 2020.
Li, Q.; Dong, X. X.; Wang, W. N.; Shan, C. F. CAS-AIR-3D face: A low-quality, multi-modal and multi-pose 3D face database. In: Proceedings of the IEEE International Joint Conference on Biometrics, 1–8, 2021.
Gilani, S. Z.; Mian, A. Towards large-scale 3D face recognition. In: Proceedings of the International Conference on Digital Image Computing: Techniques and Applications, 1–8, 2016.
Farkas, L. G. Anthropometry of the Head and Face . Raven Press, 1994.
Blanz, V.; Vetter, T. A morphable model for the synthesis of 3D faces. In: Proceedings of the 26th Annual Conference on Computer Graphics and Interactive Techniques, 187–194, 1999.
Kim, D.; Hernandez, M.; Choi, J.; Medioni, G. Deep 3D face identification. In: Proceedings of the IEEE International Joint Conference on Biometrics, 133–142, 2017.
Zhang, Z. Y.; Da, F. P.; Yu, Y. Data-free point cloud network for 3D face recognition. arXiv preprint arXiv:1911.04731, 2019.
Deng, J. K.; Cheng, S. Y.; Xue, N. N.; Zhou, Y. X.; Zafeiriou, S. UV-GAN: Adversarial facial UV map completion for pose-invariant face recognition. In: Proceedings of the IEEE/CVF Conference on Computer Vision and Pattern Recognition, 7093–7102, 2018.
Zhao, J.; Xiong, L.; Cheng, Y.; Cheng, Y.; Li, J.; Zhou, L.; Xu, Y.; Karlekar, J.; Pranata, S.; Shen, S.; et al. 3D-aided deep pose-invariant face recognition. In: Proceedings of the 27th International Joint Conference on Artificial Intelligence, 1184–1190, 2018.
Shen, Y. J.; Luo, P.; Yan, J. J.; Wang, X. G.; Tang, X. O. FaceID-GAN: Learning a symmetry three-player GAN for identity-preserving face synthesis. In: Proceedings of the IEEE/CVF Conference on Computer Vision and Pattern Recognition, 821–830, 2018.
Zhang, X. Y.; Zhao, Y.; Zhang, H. Dual-discriminator GAN: A GAN way of profile face recognition. In: Proceedings of the IEEE International Conference on Artificial Intelligence and Computer Applications, 162–166, 2020.
Marriott, R. T.; Romdhani, S.; Chen, L. M. A 3D GAN for improved large-pose facial recognition. In: Proceedings of the IEEE/CVF Conference on Computer Vision and Pattern Recognition, 13440–13450, 2021.
Luo, M. D.; Cao, J.; Ma, X.; Zhang, X. Y.; He, R. FA-GAN: Face augmentation GAN for deformation-invariant face recognition. IEEE Transactions on Information Forensics and Security Vol. 16, 2341–2355, 2021.
Bruna, J.; Zaremba, W.; Szlam, A.; LeCun, Y. Spectral networks and locally connected networks on graphs. arXiv preprint arXiv:1312.6203, 2013.
Zhao, W.; Chellappa, R.; Phillips, P. J.; Rosenfeld, A. Face recognition. ACM Computing Surveys Vol. 35, No. 4, 399–458, 2003.
Berretti, S.; del Bimbo, A.; Pala, P. 3D partial face matching using local shape descriptors. In: Proceedings of the Joint ACM Workshop on Human Gesture and Behavior Understanding, 65–71, 2011.
Li, H. B.; Huang, D.; Lemaire, P.; Morvan, J. M.; Chen, L. M. Expression robust 3D face recognition via mesh-based histograms of multiple order surface differential quantities. In: Proceedings of the 18th IEEE International Conference on Image Processing, 3053–3056, 2011.
Creusot, C.; Pears, N.; Austin, J. Automatic keypoint detection on 3D faces using a dictionary of local shapes. In: Proceedings of the International Conference on 3D Imaging, Modeling, Processing, Visualization and Transmission, 204–211, 2011.
Zhang, G. P.; Wang, Y. H. Robust 3D face recognition based on resolution invariant features. Pattern Recognition Letters Vol. 32, No. 7, 1009–1019, 2011.
Inan, T.; Halici, U. 3-D face recognition with local shape descriptors. IEEE Transactions on Information Forensics and Security Vol. 7, No. 2, 577–587, 2012.
Berretti, S.; del Bimbo, A.; Pala, P. Sparse matching of salient facial curves for recognition of 3-D faces with missing parts. IEEE Transactions on Information Forensics and Security Vol. 8, No. 2, 374–389, 2013.
Li, X. L.; Da, F. P. Efficient 3D face recognition handling facial expression and hair occlusion. Image and Vision Computing Vol. 30, No. 9, 668–679, 2012.
Ballihi, L.; Ben Amor, B.; Daoudi, M.; Srivastava, A.; Aboutajdine, D. Boosting 3-D-geometric features for efficient face recognition and gender classification. IEEE Transactions on Information Forensics and Security Vol. 7, No. 6, 1766–1779, 2012.
Berretti, S.; Werghi, N.; del Bimbo, A.; Pala, P. Matching 3D face scans using interest points and local histogram descriptors. Computers & Graphics Vol. 37, No. 5, 509–525, 2013.
Smeets, D.; Keustermans, J.; Vandermeulen, D.; Suetens, P. meshSIFT: Local surface features for 3D face recognition under expression variations and partial data. Computer Vision and Image Understanding Vol. 117, No. 2, 158–169, 2013.
Creusot, C.; Pears, N.; Austin, J. A machine-learning approach to keypoint detection and landmarking on 3D meshes. International Journal of Computer Vision Vol. 102, Nos. 1–3, 146–179, 2013.
Tang, H. L.; Yin, B. C.; Sun, Y. F.; Hu, Y. L. 3D face recognition using local binary patterns. Signal Processing Vol. 93, No. 8, 2190–2198, 2013.
Lei, Y. J.; Bennamoun, M.; El-Sallam, A. A. An efficient 3D face recognition approach based on the fusion of novel local low-level features. Pattern Recognition Vol. 46, No. 1, 24–37, 2013.
Elaiwat, S.; Bennamoun, M.; Boussaid, F.; El-Sallam, A. 3-D face recognition using curvelet local features. IEEE Signal Processing Letters Vol. 21, No. 2, 172–175, 2014.
Drira, H.; Ben Amor, B.; Srivastava, A.; Daoudi, M.; Slama, R. 3D face recognition under expressions, occlusions, and pose variations. IEEE Transactions on Pattern Analysis and Machine Intelligence Vol. 35, No. 9, 2270–2283, 2013.
Li, H. B.; Huang, D.; Morvan, J. M.; Chen, L. M.; Wang, Y. H. Expression-robust 3D face recognition via weighted sparse representation of multi-scale and multi-component local normal patterns. Neurocomputing Vol. 133, 179–193, 2014.
Berretti, S.; Werghi, N.; Bimbo, A.; Pala, P. Selecting stable keypoints and local descriptors for person identification using 3D face scans. The Visual Computer Vol. 30, No. 11, 1275–1292, 2014.
Lei, Y. J.; Bennamoun, M.; Hayat, M.; Guo, Y. L. An efficient 3D face recognition approach using local geometrical signatures. Pattern Recognition Vol. 47, No. 2, 509–524, 2014.
Tabia, H.; Laga, H.; Picard, D.; Gosselin, P. H. Covariance descriptors for 3D shape matching and retrieval. In: Proceedings of the IEEE Conference on Computer Vision and Pattern Recognition, 4185–4192, 2014.
Vezzetti, E.; Marcolin, F.; Fracastoro, G. 3D face recognition: An automatic strategy based on geometrical descriptors and landmarks. Robotics and Autonomous Systems Vol. 62, No. 12, 1768–1776, 2014.
Li, H. B.; Huang, D.; Morvan, J. M.; Wang, Y. H.; Chen, L. M. Towards 3D face recognition in the real: A registration-free approach using fine-grained matching of 3D keypoint descriptors. International Journal of Computer Vision Vol. 113, No. 2, 128–142, 2015.
Article MathSciNet Google Scholar
Elaiwat, S.; Bennamoun, M.; Boussaid, F.; El-Sallam, A. A curvelet-based approach for textured 3D face recognition. Pattern Recognition Vol. 48, No. 4, 1235–1246, 2015.
Al-Osaimi, F. R. A novel multi-purpose matching representation of local 3D surfaces: A rotationally invariant, efficient, and highly discriminative approach with an adjustable sensitivity. IEEE Transactions on Image Processing Vol. 25, No. 2, 658–672, 2016.
Article MathSciNet MATH Google Scholar
Ming, Y. Robust regional bounding spherical descriptor for 3D face recognition and emotion analysis. Image and Vision Computing Vol. 35, 14–22, 2015.
Guo, Y. L.; Lei, Y. J.; Liu, L.; Wang, Y.; Bennamoun, M.; Sohel, F. EI3D: Expression-invariant 3D face recognition based on feature and shape matching. Pattern Recognition Letters Vol. 83, 403–412, 2016.
Soltanpour, S.; Wu, Q. J. Multimodal 2D–3D face recognition using local descriptors: Pyramidal shape map and structural context. IET Biometrics Vol. 6, No. 1, 27–35, 2017.
Lei, Y. J.; Guo, Y. L.; Hayat, M.; Bennamoun, M.; Zhou, X. Z. A Two-Phase Weighted Collaborative Representation for 3D partial face recognition with single sample. Pattern Recognition Vol. 52, 218–237, 2016.
Emambakhsh, M.; Evans, A. Nasal patches and curves for expression-robust 3D face recognition. IEEE Transactions on Pattern Analysis and Machine Intelligence Vol. 39, No. 5, 995–1007, 2017.
Werghi, N.; Tortorici, C.; Berretti, S.; Del Bimbo, A. Boosting 3D LBP-based face recognition by fusing shape and texture descriptors on the mesh. IEEE Transactions on Information Forensics and Security Vol. 11, No. 5, 964–979, 2016.
Hariri, W.; Tabia, H.; Farah, N.; Benouareth, A.; Declercq, D. 3D face recognition using covariance based descriptors. Pattern Recognition Letters Vol. 78, 1–7, 2016.
Soltanpour, S.; Jonathan Wu, Q. M. High-order local normal derivative pattern (LNDP) for 3D face recognition. In: Proceedings of the IEEE International Conference on Image Processing, 2811–2815, 2017.
Deng, X.; Da, F. P.; Shao, H. J. Efficient 3D face recognition using local covariance descriptor and Riemannian kernel sparse coding. Computers & Electrical Engineering Vol. 62, 81–91, 2017.
Abbad, A.; Abbad, K.; Tairi, H. 3D face recognition: Multi-scale strategy based on geometric and local descriptors. Computers & Electrical Engineering Vol. 70, 525–537, 2018.
Soltanpour, S.; Wu, Q. M. J. Weighted extreme sparse classifier and local derivative pattern for 3D face recognition. IEEE Transactions on Image Processing Vol. 28, No. 6, 3020–3033, 2019.
Shi, L. L.; Wang, X.; Shen, Y. L. Research on 3D face recognition method based on LBP and SVM. Optik Vol. 220, 165157, 2020.
Samir, C.; Srivastava, A.; Daoudi, M.; Klassen, E. An intrinsic framework for analysis of facial surfaces. International Journal of Computer Vision Vol. 82, No. 1, 80–95, 2009.
Samir, C.; Srivastava, A.; Daoudi, M. Three-dimensional face recognition using shapes of facial curves. IEEE Transactions on Pattern Analysis and Machine Intelligence Vol. 28, No. 11, 1858–1863, 2006.
Lowe, D. G. Distinctive image features from scale-invariant keypoints. International Journal of Computer Vision Vol. 60, No. 2, 91–110, 2004.
Deng, X.; Da, F.; Shao, H. J.; Jiang, Y. T. A multi-scale three-dimensional face recognition approach with sparse representation-based classifier and fusion of local covariance descriptors. Computers & Electrical Engineering Vol. 85, 106700, 2020.
Vezzetti, E.; Marcolin, F.; Tornincasa, S.; Ulrich, L.; Dagnes, N. 3D geometry-based automatic landmark localization in presence of facial occlusions. Multimedia Tools and Applications Vol. 77, No. 11, 14177–14205, 2018.
Drira, H.; Benamor, B.; Daoudi, M.; Srivastava, A. Pose and expression-invariant 3D face recognition using elastic radial curves. In: Proceedings of the British Machine Vision Conference, 1–11, 2010.
Freund, Y.; Schapire, R. E. A short introduction to boosting. Journal of Japanese Society for Artificial Intelligence Vol. 14, No. 5, 771–780, 1999.
Aubry, M.; Schlickewei, U.; Cremers, D. The wave kernel signature: A quantum mechanical approach to shape analysis. In: Proceedings of the IEEE International Conference on Computer Vision Workshops, 1626–1633, 2011.
Ojala, T.; Pietikainen, M.; Maenpaa, T. Multiresolution gray-scale and rotation invariant texture classification with local binary patterns. IEEE Transactions on Pattern Analysis and Machine Intelligence Vol. 24, No. 7, 971–987, 2002.
Yu, Y.; Da, F. P.; Guo, Y. F. Sparse ICP with resampling and denoising for 3D face verification. IEEE Transactions on Information Forensics and Security Vol. 14, No. 7, 1917–1927, 2019.
Spreeuwers, L. Fast and accurate 3D face recognition. International Journal of Computer Vision Vol. 93, No. 3, 389–414, 2011.
Ocegueda, O.; Passalis, G.; Theoharis, T.; Shah, S. K.; Kakadiaris, I. A. UR3D-C: Linear dimensionality reduction for efficient 3D face recognition. In: Proceedings of the International Joint Conference on Biometrics, 1–6, 2011.
Ming, Y.; Ruan, Q. Q. Robust sparse bounding sphere for 3D face recognition. Image and Vision Computing Vol. 30, No. 8, 524–534, 2012.
Liu, P. J.; Wang, Y. H.; Huang, D.; Zhang, Z. X.; Chen, L. M. Learning the spherical harmonic features for 3-D face recognition. IEEE Transactions on Image Processing Vol. 22, No. 3, 914–925, 2013.
Taghizadegan, Y.; Ghassemian, H.; Naser-Moghaddasi, M. 3D face recognition method using 2DPCA-Euclidean distance classification. ACEEE International Journal on Control System and Instrumentation Vol. 3, No. 1, 1–5, 2012.
Mohammadzade, H.; Hatzinakos, D. Iterative closest normal point for 3D face recognition. IEEE Transactions on Pattern Analysis and Machine Intelligence Vol. 35, No. 2, 381–397, 2013.
Ming, Y. Rigid-area orthogonal spectral regression for efficient 3D face recognition. Neurocomputing Vol. 129, 445–457, 2014.
Ratyal, N. I.; Ahmad Taj, I.; Bajwa, U. I.; Sajid, M. 3D face recognition based on pose and expression invariant alignment. Computers & Electrical Engineering Vol. 46, 241–255, 2015.
Tang, Y. H.; Sun, X.; Huang, D.; Morvan, J. M.; Wang, Y. H.; Chen, L. M. 3D face recognition with asymptotic cones based principal curvatures. In: Proceedings of the International Conference on Biometrics, 466–472, 2015.
Gilani, S. Z.; Mian, A.; Eastwood, P. Deep, dense and accurate 3D face correspondence for generating population specific deformable models. Pattern Recognition Vol. 69, 238–250, 2017.
Peter, M.; Minoi, J. L.; Hipiny, I. H. M. 3D face recognition using kernel-based PCA approach. In: Computational Science and Technology . Lecture Notes in Electrical Engineering , Vol. 481 . Alfred, R.; Lim, Y.; Ibrahim, A.; Anthony, P. Eds. Springer Singapore, 77–86, 2019.
Passalis, G.; Perakis, P.; Theoharis, T.; Kakadiaris, I. A. Using facial symmetry to handle pose variations in real-world 3D face recognition. IEEE Transactions on Pattern Analysis and Machine Intelligence Vol. 33, No. 10, 1938–1951, 2011.
Huang, D.; Ardabilian, M.; Wang, Y. H.; Chen, L. M. 3-D face recognition using eLBP-based facial description and local feature hybrid matching. IEEE Transactions on Information Forensics and Security Vol. 7, No. 5, 1551–1565, 2012.
Alyüz, N.; Gökberk, B.; Spreeuwers, L.; Veldhuis, R.; Akarun, L. Robust 3D face recognition in the presence of realistic occlusions. In: Proceedings of the 5th IAPR International Conference on Biometrics, 111–118, 2012.
Fadaifard, H.; Wolberg, G.; Haralick, R. Multiscale 3D feature extraction and matching with an application to 3D face recognition. Graphical Models Vol. 75, No. 4, 157–176, 2013.
Alyuz, N.; Gokberk, B.; Akarun, L. 3-D face recognition under occlusion using masked projection. IEEE Transactions on Information Forensics and Security Vol. 8, No. 5, 789–802, 2013.
Bagchi, P.; Bhattacharjee, D.; Nasipuri, M. Robust 3D face recognition in presence of pose and partial occlusions or missing parts. arXiv preprint arXiv:1408.3709, 2014.
Bagchi, P.; Bhattacharjee, D.; Nasipuri, M. 3D Face Recognition using surface normals. In: Proceedings of the TENCON 2015 - 2015 IEEE Region 10 Conference, 1–6, 2015.
Liang, Y.; Zhang, Y.; Zeng, X. X. Pose-invariant 3D face recognition using half face. Signal Processing: Image Communication Vol. 57, 84–90, 2017.
LeCun, Y.; Bengio, Y.; Hinton, G. Deep learning. Nature Vol. 521, No. 7553, 436–444, 2015.
Taigman, Y.; Yang, M.; Ranzato, M.; Wolf, L. DeepFace: Closing the gap to human-level performance in face verification. In: Proceedings of the IEEE Conference on Computer Vision and Pattern Recognition, 1701–1708, 2014.
Sun, Y.; Chen, Y. H.; Wang, X. G.; Tang, X. O. Deep learning face representation by joint identification-verification. In: Proceedings of the 27th International Conference on Neural Information Processing Systems, Vol. 2, 1988–1996, 2014.
Sun, Y.; Wang, X. G.; Tang, X. O. Deep learning face representation from predicting 10,000 classes. In: Proceedings of the IEEE Conference on Computer Vision and Pattern Recognition, 1891–1898, 2014.
Sun, Y.; Wang, X. G.; Tang, X. O. Deeply learned face representations are sparse, selective, and robust. In: Proceedings of the IEEE Conference on Computer Vision and Pattern Recognition, 2892–2900, 2015.
Sun, Y.; Liang, D.; Wang, X. G.; Tang, X. O. DeepID3: Face recognition with very deep neural networks. arXiv preprint arXiv:1502.00873, 2015.
Schroff, F.; Kalenichenko, D.; Philbin, J. FaceNet: A unified embedding for face recognition and clustering. In: Proceedings of the IEEE Conference on Computer Vision and Pattern Recognition, 815–823, 2015.
Charles, R. Q.; Hao, S.; Mo, K. C.; Guibas, L. J. PointNet: Deep learning on point sets for 3D classification and segmentation. In: Proceedings of the IEEE Conference on Computer Vision and Pattern Recognition, 77–85, 2017.
Qi, C. R.; Yi, L.; Su, H.; Guibas, L. J. PointNet++: Deep hierarchical feature learning on point sets in a metric space. In: Proceedings of the 31st International Conference on Neural Information Processing System, 5105–5114, 2017.
Ding, Y. Q.; Li, N. Y.; Young, S. S.; Ye, J. W. Efficient 3D face recognition in uncontrolled environment. In: Advances in Visual Computing . Lecture Notes in Computer Science , Vol. 11844 . Springer Cham, 430–443, 2019.
Lin, S. S.; Liu, F.; Liu, Y. H.; Shen, L. L. Local feature tensor based deep learning for 3D face recognition. In: Proceedings of the 14th IEEE International Conference on Automatic Face & Gesture Recognition, 1–5, 2019.
Tan, Y.; Lin, H. X.; Xiao, Z. L.; Ding, S. Y.; Chao, H. Y. Face recognition from sequential sparse 3D data via deep registration. In: Proceedings of the International Conference on Biometrics, 1–8, 2019.
Olivetti, E. C.; Ferretti, J.; Cirrincione, G.; Nonis, F.; Tornincasa, S.; Marcolin, F. Deep CNN for 3D face recognition. In: Design Tools and Methods in Industrial Engineering . Lecture Notes in Mechanical Engineering . Rizzi, C.; Andrisano, A. O.; Leali, F.; Gherardini, F.; Pini, F.; Vergnano, A. Eds. Springer Cham, 665–674, 2020.
Xu, K. M.; Wang, X. M.; Hu, Z. H.; Zhang, Z. H. 3D face recognition based on twin neural network combining deep map and texture. In: Proceedings of the IEEE 19th International Conference on Communication Technology, 1665–1668, 2019.
Feng, J. Y.; Guo, Q.; Guan, Y. D.; Wu, M. D.; Zhang, X. R.; Ti, C. L. 3D face recognition method based on deep convolutional neural network In: Smart Innovations in Communication and Computational Sciences . Advances in Intelligent Systems and Computing , Vol. 670 . Panigrahi, B.; Trivedi, M.; Mishra, K.; Tiwari, S.; Singh, P. Eds. Springer Singapore, 123–130, 2019.
Mu, G. D.; Huang, D.; Hu, G. S.; Sun, J.; Wang, Y. H. Led3D: A lightweight and efficient deep approach to recognizing low-quality 3D faces. In: Proceedings of the IEEE/CVF Conference on Computer Vision and Pattern Recognition, 5766–5775, 2019.
Dutta, K.; Bhattacharjee, D.; Nasipuri, M. SpPCANet: A simple deep learning-based feature extraction approach for 3D face recognition. Multimedia Tools and Applications Vol. 79, Nos. 41–42, 31329–31352, 2020.
Cao, C. Q.; Swash, M. R.; Meng, H. Y. Reliable holoscopic 3D face recognition. In: Proceedings of the 7th International Conference on Signal Processing and Integrated Networks, 696–701, 2020.
Lin, S. S.; Jiang, C. Y.; Liu, F.; Shen, L. L. High quality facial data synthesis and fusion for 3D low-quality face recognition. In: Proceedings of the IEEE International Joint Conference on Biometrics, 1–8, 2021.
Chiu, M. T.; Cheng, H. Y.; Wang, C. Y.; Lai, S. H. High-accuracy RGB-D face recognition via segmentation-aware face depth estimation and mask-guided attention network. In: Proceedings of the 16th IEEE International Conference on Automatic Face and Gesture Recognition, 1–8, 2021.
Zhang, Z. Y.; Da, F. P.; Yu, Y. Learning directly from synthetic point clouds for “in-the-wild” 3D face recognition. Pattern Recognition Vol. 123, 108394, 2022.
Bhople, A. R.; Shrivastava, A. M.; Prakash, S. Point cloud based deep convolutional neural network for 3D face recognition. Multimedia Tools and Applications Vol. 80, No. 20, 30237–30259, 2021.
Bhople, A. R.; Prakash, S. Learning similarity and dissimilarity in 3D faces with triplet network. Multimedia Tools and Applications Vol. 80, Nos. 28–29, 35973–35991, 2021.
Jiang, C. Y.; Lin, S. S.; Chen, W.; Liu, F.; Shen, L. L. PointFace: Point set based feature learning for 3D face recognition. In: Proceedings of the IEEE International Joint Conference on Biometrics, 1–8, 2021.
Papadopoulos, K.; Kacem, A.; Shabayek, A.; Aouada, D. Face-GCN: A graph convolutional network for 3D dynamic face identification/recognition. arXiv preprint arXiv:2104.09145, 2021.
Li, B. Y. L.; Mian, A. S.; Liu, W. Q.; Krishna, A. Using Kinect for face recognition under varying poses, expressions, illumination and disguise. In: Proceedings of the IEEE Workshop on Applications of Computer Vision, 186–192, 2013.
Wang, F.; Cheng, J.; Liu, W. Y.; Liu, H. J. Additive margin softmax for face verification. IEEE Signal Processing Letters Vol. 25, No. 7, 926–930, 2018.
Cortes, C.; Vapnik, V. Support-vector networks. Machine Learning Vol. 20, No. 3, 273–297, 1995.
Isola, P.; Zhu, J. Y.; Zhou, T. H.; Efros, A. A. Image-to-image translation with conditional adversarial networks. In: Proceedings of the IEEE Conference on Computer Vision and Pattern Recognition, 5967–5976, 2017.
Jiang, L.; Zhang, J. Y.; Deng, B. L. Robust RGB-D face recognition using attribute-aware loss. IEEE Transactions on Pattern Analysis and Machine Intelligence Vol. 42, No. 10, 2552–2566, 2020.
Paysan, P.; Knothe, R.; Amberg, B.; Romdhani, S.; Vetter, T. A 3D face model for pose and illumination invariant face recognition. In: Proceedings of the 6th IEEE International Conference on Advanced Video and Signal Based Surveillance, 296–301, 2009.
Cao, C.; Weng, Y. L.; Zhou, S.; Tong, Y. Y.; Zhou, K. FaceWarehouse: A 3D facial expression database for visual computing. IEEE Transactions on Visualization and Computer Graphics Vol. 20, No. 3, 413–425, 2014.
Castellani, U.; Bartoli, A. 3D shape registration. In: 3D Imaging , Analysis and Applications . Liu, Y.; Pears, N.; Rosin, P. L.; Huber, P. Eds. Springer Cham, 353–411, 2020.
D’Errico, J. Surface fitting using gridfit. MATLAB Central File Exchange. 2005. Available at https://www.mathworks.com/matlabcentral/fileexchange/8998-surface-fitting-using-gridfit .
Ciravegna, G.; Cirrincione, G.; Marcolin, F.; Barbiero, P.; Dagnes, N.; Piccolo, E. Assessing discriminating capability of geometrical descriptors for 3D face recognition by using the GH-EXIN neural network. In: Neural Approaches to Dynamics of Signal Exchanges . Smart Innovation, Systems and Technologies , Vol. 151 . Esposito, A.; Faundez-Zanuy, M.; Morabito, F.; Pasero, E. Eds. Springer Singapore, 223–233, 2020.
Lüthi, M.; Gerig, T.; Jud, C.; Vetter, T. Gaussian process morphable models. IEEE Transactions on Pattern Analysis and Machine Intelligence Vol. 40, No. 8, 1860–1873, 2017.
Gilani, S. Z.; Mian, A.; Shafait, F.; Reid, I. Dense 3D face correspondence. IEEE Transactions on Pattern Analysis and Machine Intelligence Vol. 40, No. 7, 1584–1598, 2018.
El Rahman Shabayek, A.; Aouada, D.; Cherenkova, K.; Gusev, G.; Ottersten, B. 3D deformation signature for dynamic face recognition. In: Proceedings of the IEEE International Conference on Acoustics, Speech and Signal Processing, 2138–2142, 2020.
Smith, M.; Smith, L.; Huang, N.; Hansen, M.; Smith, M. Deep 3D face recognition using 3D data augmentation and transfer learning. In: Proceedings of the 16th International Conference on Machine Learning and Data Mining, 209–218, 2020.
Dou, P. F.; Shah, S. K.; Kakadiaris, I. A. End-to-end 3D face reconstruction with deep neural networks. In: Proceedings of the IEEE Conference on Computer Vision and Pattern Recognition, 1503–1512, 2017.
Richardson, E.; Sela, M. T.; Kimmel, R. 3D face reconstruction by learning from synthetic data. In: Proceedings of the 4th International Conference on 3D Vision, 460–469, 2016.
Download references
Author information
Authors and affiliations.
The School of Information Technology, Deakin University, Waurn Ponds, VIC, Australia
Yaping Jing, Xuequan Lu & Shang Gao
You can also search for this author in PubMed Google Scholar
Corresponding author
Correspondence to Xuequan Lu .
Ethics declarations
The authors have no competing interests to declare that are relevant to the content of this article.
Additional information
Yaping Jing received her bachelor degree (Hons) in information technology from Deakin University, Australia in 2016. She is currently a Ph.D. candidate in the School of Information Technology at Deakin University. Her research interests include 3D face recognition, 3D data processing, and machine learning.
Xuequan Lu is a lecturer (assistant professor) in Deakin University, Australia. He spent more than two years working research fellow in Singapore. Prior to that, he received his Ph.D. degree from Zhejiang University, China in 2016. His research interests mainly fall into the category of visual data computing, for example, geometry modeling, processing and analysis, animation, simulation, 2D data processing and analysis.
Shang Gao received her Ph.D. degree in computer science from Northeastern University, China in 2000. She is currently a senior lecturer in the School of Information Technology, Deakin University. Her current research interests include cybersecurity, cloud computing, and machine learning.
Rights and permissions
This article is licensed under a Creative Commons Attribution 4.0 International License, which permits use, sharing, adaptation, distribution and reproduction in any medium or format, as long as you give appropriate credit to the original author(s) and the source, provide a link to the Creative Commons licence, and indicate if changes were made.
The images or other third party material in this article are included in the article’s Creative Commons licence, unless indicated otherwise in a credit line to the material. If material is not included in the article’s Creative Commons licence and your intended use is not permitted by statutory regulation or exceeds the permitted use, you will need to obtain permission directly from the copyright holder.
To view a copy of this licence, visit http://creativecommons.org/licenses/by/4.0/ .
Other papers from this open access journal are available free of charge from http://www.springer.com/journal/41095 . To submit a manuscript, please go to https://www.editorialmanager.com/cvmj .
Reprints and permissions
About this article
Jing, Y., Lu, X. & Gao, S. 3D face recognition: A comprehensive survey in 2022. Comp. Visual Media 9 , 657–685 (2023). https://doi.org/10.1007/s41095-022-0317-1
Download citation
Received : 14 April 2022
Accepted : 29 September 2022
Published : 05 August 2023
Issue Date : December 2023
DOI : https://doi.org/10.1007/s41095-022-0317-1
Share this article
Anyone you share the following link with will be able to read this content:
Sorry, a shareable link is not currently available for this article.
Provided by the Springer Nature SharedIt content-sharing initiative
- 3D face recognition
- 3D face databases
- deep learning
- local features
- global features
- Find a journal
- Publish with us
- Track your research
Grab your spot at the free arXiv Accessibility Forum
Help | Advanced Search
Computer Science > Computer Vision and Pattern Recognition
Title: eight years of face recognition research: reproducibility, achievements and open issues.
Abstract: Automatic face recognition is a research area with high popularity. Many different face recognition algorithms have been proposed in the last thirty years of intensive research in the field. With the popularity of deep learning and its capability to solve a huge variety of different problems, face recognition researchers have concentrated effort on creating better models under this paradigm. From the year 2015, state-of-the-art face recognition has been rooted in deep learning models. Despite the availability of large-scale and diverse datasets for evaluating the performance of face recognition algorithms, many of the modern datasets just combine different factors that influence face recognition, such as face pose, occlusion, illumination, facial expression and image quality. When algorithms produce errors on these datasets, it is not clear which of the factors has caused this error and, hence, there is no guidance in which direction more research is required. This work is a followup from our previous works developed in 2014 and eventually published in 2016, showing the impact of various facial aspects on face recognition algorithms. By comparing the current state-of-the-art with the best systems from the past, we demonstrate that faces under strong occlusions, some types of illumination, and strong expressions are problems mastered by deep learning algorithms, whereas recognition with low-resolution images, extreme pose variations, and open-set recognition is still an open problem. To show this, we run a sequence of experiments using six different datasets and five different face recognition algorithms in an open-source and reproducible manner. We provide the source code to run all of our experiments, which is easily extensible so that utilizing your own deep network in our evaluation is just a few minutes away.
Subjects: | Computer Vision and Pattern Recognition (cs.CV) |
Cite as: | [cs.CV] |
(or [cs.CV] for this version) | |
Focus to learn more arXiv-issued DOI via DataCite |
Submission history
Access paper:.
- Other Formats

References & Citations
- Google Scholar
- Semantic Scholar
BibTeX formatted citation

Bibliographic and Citation Tools
Code, data and media associated with this article, recommenders and search tools.
- Institution
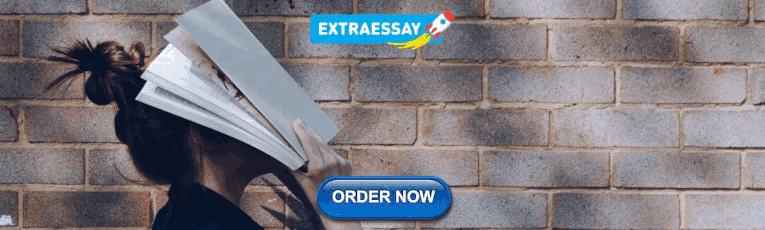
arXivLabs: experimental projects with community collaborators
arXivLabs is a framework that allows collaborators to develop and share new arXiv features directly on our website.
Both individuals and organizations that work with arXivLabs have embraced and accepted our values of openness, community, excellence, and user data privacy. arXiv is committed to these values and only works with partners that adhere to them.
Have an idea for a project that will add value for arXiv's community? Learn more about arXivLabs .
IEEE Account
- Change Username/Password
- Update Address
Purchase Details
- Payment Options
- Order History
- View Purchased Documents
Profile Information
- Communications Preferences
- Profession and Education
- Technical Interests
- US & Canada: +1 800 678 4333
- Worldwide: +1 732 981 0060
- Contact & Support
- About IEEE Xplore
- Accessibility
- Terms of Use
- Nondiscrimination Policy
- Privacy & Opting Out of Cookies
A not-for-profit organization, IEEE is the world's largest technical professional organization dedicated to advancing technology for the benefit of humanity. © Copyright 2024 IEEE - All rights reserved. Use of this web site signifies your agreement to the terms and conditions.
Subscribe to the PwC Newsletter
Join the community, add a new evaluation result row, face recognition.
606 papers with code • 23 benchmarks • 64 datasets
Facial Recognition is the task of making a positive identification of a face in a photo or video image against a pre-existing database of faces. It begins with detection - distinguishing human faces from other objects in the image - and then works on identification of those detected faces.
The state of the art tables for this task are contained mainly in the consistent parts of the task : the face verification and face identification tasks.
( Image credit: Face Verification )

Benchmarks Add a Result
Trend | Dataset | Best Model | -->Paper | Code | Compare |
---|---|---|---|---|---|
GhostFaceNetV2-1 (MS1MV3) | -->|||||
GhostFaceNetV2-1 | -->|||||
MS1MV2, R100, SFace | -->|||||
Fine-tuned ArcFace | -->|||||
Fine-tuned ArcFace | -->|||||
ArcFace+CSFM | -->|||||
PIC - QMagFace | -->|||||
Prodpoly | -->|||||
Prodpoly | -->|||||
PIC - MagFace | -->|||||
PIC - ArcFace | -->|||||
FaceNet+Adaptive Threshold | -->|||||
FaceNet+Adaptive Threshold | -->|||||
FaceNet+Adaptive Threshold | -->|||||
Model with Up Convolution + DoG Filter (Aligned) | -->|||||
Model with Up Convolution + DoG Filter | -->|||||
GhostFaceNetV2-1 | -->|||||
Model with Up Convolution + DoG Filter | -->|||||
GhostFaceNetV2-1 | -->|||||
Multi-task | -->|||||
FaceTransformer+OctupletLoss | -->|||||
Partial FC | -->|||||
MCN | -->

Most implemented papers
Facenet: a unified embedding for face recognition and clustering.
On the widely used Labeled Faces in the Wild (LFW) dataset, our system achieves a new record accuracy of 99. 63%.
ArcFace: Additive Angular Margin Loss for Deep Face Recognition

Recently, a popular line of research in face recognition is adopting margins in the well-established softmax loss function to maximize class separability.
VGGFace2: A dataset for recognising faces across pose and age
The dataset was collected with three goals in mind: (i) to have both a large number of identities and also a large number of images for each identity; (ii) to cover a large range of pose, age and ethnicity; and (iii) to minimize the label noise.
SphereFace: Deep Hypersphere Embedding for Face Recognition
This paper addresses deep face recognition (FR) problem under open-set protocol, where ideal face features are expected to have smaller maximal intra-class distance than minimal inter-class distance under a suitably chosen metric space.
A Light CNN for Deep Face Representation with Noisy Labels
This paper presents a Light CNN framework to learn a compact embedding on the large-scale face data with massive noisy labels.
Learning Face Representation from Scratch
The current situation in the field of face recognition is that data is more important than algorithm.
Circle Loss: A Unified Perspective of Pair Similarity Optimization
This paper provides a pair similarity optimization viewpoint on deep feature learning, aiming to maximize the within-class similarity $s_p$ and minimize the between-class similarity $s_n$.
MS-Celeb-1M: A Dataset and Benchmark for Large-Scale Face Recognition
In this paper, we design a benchmark task and provide the associated datasets for recognizing face images and link them to corresponding entity keys in a knowledge base.
DeepID3: Face Recognition with Very Deep Neural Networks
Very deep neural networks recently achieved great success on general object recognition because of their superb learning capacity.
Can we still avoid automatic face detection?
Recognito-Vision/Linux-FaceRecognition-FaceLivenessDetection • 14 Feb 2016
In this setting, is it still possible for privacy-conscientious users to avoid automatic face detection and recognition?
- DOI: 10.1007/s41095-022-0317-1
- Corpus ID: 260645664
3D face recognition: A comprehensive survey in 2022
- Yaping Jing , Xuequan Lu , Shang Gao
- Published in Computational Visual Media 5 August 2023
- Computer Science
9 Citations
3d face recognition: two decades of progress and prospects, face recognition: a literature review, deep learning-powered face detection and recognition for challenging environments, novel and effective approach for multiview biometric object detection using deep learning based cutting edge techniques, exploration on facial image recognition and processing algorithms under hadoop, mart: masked affective representation learning via masked temporal distribution distillation, an improved machine learning method by applying cloud forensic meta-model to enhance the data collection process in cloud environments, veintr: robust end-to-end full-hand vein identification with transformer, study on the applications of artificial intelligence in image recognition, 171 references, 3d face recognition: a survey, a fast and robust 3d face recognition approach based on deeply learned face representation.
- Highly Influential
3-D face recognition: features, databases, algorithms and challenges
3-d face recognition using curvelet local features, 3d partial face matching using local shape descriptors, efficient 3d face recognition in uncontrolled environment, a survey of local feature methods for 3d face recognition, pose-invariant 3d face recognition using half face, deep 3d face identification, 3d face recognition using surface normals, related papers.
Showing 1 through 3 of 0 Related Papers
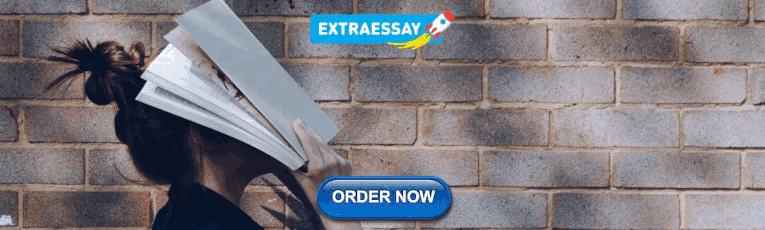
IMAGES
COMMENTS
This comprehensive survey reviews 3D face recognition techniques developed in the past decade, both conventional methods and deep learning methods. These methods are evaluated with detailed descriptions of selected representative works.
In this paper, some of the most impactful face recognition systems were surveyed. Firstly, the paper gives an overview of a general face recognition system. Secondly, the survey covers various network architectures and training losses that have had a substantial impact.
This paper provides an introduction to face recognition, including its history, pipeline, algorithms based on conventional manually designed features or deep learning, mainstream training, evaluation datasets, and related applications.
The fields of image processing, pattern recognition, and computer vision are all crucial to face recognition. Almost 20 papers from 2011 to 2021 were analyzed and it was observed that a framework called Deep Learning includes a number of significant algorithms.
Eight Years of Face Recognition Research: Reproducibility, Achievements and Open Issues. Automatic face recognition is a research area with high popularity. Many different face recognition algorithms have been proposed in the last thirty years of intensive research in the field.
Compared with traditional machine learning algorithms, convolutional neural networks (CNN) can yield better performance and higher efficiency in face recognition. In this paper, we illustrate the mechanism of CNN methods, followed by discussions on the latest research progress of face recognition using CNN methods and comparisons between ...
606 papers with code • 23 benchmarks • 64 datasets. Facial Recognition is the task of making a positive identification of a face in a photo or video image against a pre-existing database of faces.
This comprehensive survey reviews 3D face recognition techniques developed in the past decade, both conventional methods and deep learning methods, and summarizes their advantages and disadvantages in terms of accuracy, complexity, and robustness to facial variations.
Illustration of the proposed 3D bypass enhanced repre-sentation learning (3D-BERL). Inspired by the physical model of image formation, 3D-BERL incorporates a self-supervised 3D re-construction bypass to improve the face recognition.
This comprehensive survey reviews 3D face recognition techniques developed in the past decade, both conventional methods and deep learning methods. These methods are evaluated with detailed ...