2023 Theses Doctoral
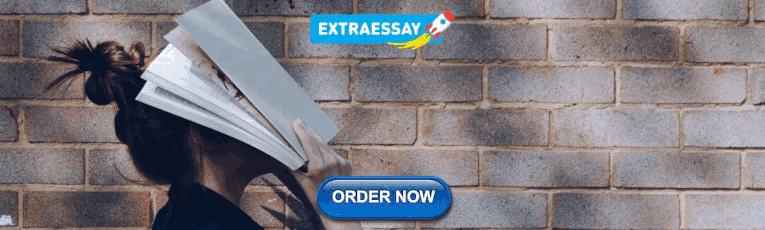
Topological Representational Similarity Analysis in Brains and Beyond
Lin, Baihan
Understanding how the brain represents and processes information is crucial for advancing neuroscience and artificial intelligence. Representational similarity analysis (RSA) has been instrumental in characterizing neural representations by comparing multivariate response patterns elicited by sensory stimuli. However, traditional RSA relies solely on geometric properties, overlooking crucial topological information. This thesis introduces topological RSA (tRSA), a novel framework that combines geometric and topological properties of neural representations. tRSA applies nonlinear monotonic transforms to representational dissimilarities, emphasizing local topology while retaining intermediate-scale geometry. The resulting geo-topological matrices enable model comparisons that are robust to noise and individual idiosyncrasies. This thesis introduces several key methodological advances: (1) Topological RSA (tRSA) identifies computational signatures as accurately as RSA while compressing unnecessary variation with capabilities to test topological hypotheses; (2) Adaptive Geo-Topological Dependence Measure (AGTDM) provides a robust statistical test for detecting complex multivariate relationships; (3) Procrustes-aligned Multidimensional Scaling (pMDS) aligns time-resolved representational geometries to illuminate processing stages in neural computation; (4) Temporal Topological Data Analysis (tTDA) applies spatio-temporal filtration techniques to reveal developmental trajectories in biological systems; and (5) Single-cell Topological Simplicial Analysis (scTSA) characterizes higher-order cell population complexity across different stages of development. Through analyses of neural recordings, biological data, and simulations of neural network models, this thesis demonstrates the power and versatility of these new methods. By advancing RSA with topological techniques, this work provides a powerful new lens for understanding brains, computational models, and complex biological systems. These methods not only offer robust approaches for adjudicating among competing models but also reveal novel theoretical insights into the nature of neural computation. This thesis lays the foundation for future investigations at the intersection of topology, neuroscience, and time series data analysis, promising to deepen our understanding of how information is represented and processed in biological and artificial neural networks. The methods developed here have potential applications in fields ranging from cognitive neuroscience to clinical diagnosis and AI development, paving the way for more nuanced understanding of brain function and dysfunction.
- Neurosciences
- Artificial intelligence
- Bioinformatics
- Neural networks (Neurobiology)
- Brain--Imaging
- Cognitive neuroscience
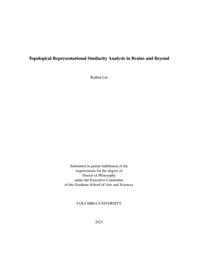
More About This Work
- DOI Copy DOI to clipboard
Convex optimization for neural networks
Abstract/contents, description.
Type of resource | text |
---|---|
Form | electronic resource; remote; computer; online resource |
Extent | 1 online resource. |
Place | California |
Place | [Stanford, California] |
Publisher | [Stanford University] |
Copyright date | 2023; ©2023 |
Publication date | 2023; 2023 |
Issuance | monographic |
Language | English |
Creators/Contributors
Author | Ergen, Tolga |
---|---|
Degree supervisor | Pilanci, Mert |
Thesis advisor | Pilanci, Mert |
Thesis advisor | Boyd, Stephen P |
Thesis advisor | Weissman, Tsachy |
Degree committee member | Boyd, Stephen P |
Degree committee member | Weissman, Tsachy |
Associated with | Stanford University, School of Engineering |
Associated with | Stanford University, Department of Electrical Engineering |
Genre | Theses |
---|---|
Genre | Text |
Bibliographic information
Statement of responsibility | Tolga Ergen. |
---|---|
Note | Submitted to the Department of Electrical Engineering. |
Thesis | Thesis Ph.D. Stanford University 2023. |
Location |
Access conditions
Version 1 | May 9, 2024 | You are viewing this version | |
---|
Each version has a distinct URL, but you can use this PURL to access the latest version. https://purl.stanford.edu/sv935mh9248
Also listed in
Loading usage metrics...
(Stanford users can avoid this Captcha by logging in.)
- Send to text email RefWorks EndNote printer
Convex neural networks
Digital content, item is featured in an exhibit item is featured in exhibits.
Items from this collection are featured in:
More options
- Contributors
Description
Creators/contributors, contents/summary, bibliographic information.

- Stanford Home
- Maps & Directions
- Search Stanford
- Emergency Info
- Terms of Use
- Non-Discrimination
- Accessibility
© Stanford University , Stanford , California 94305 .
Thank you for visiting nature.com. You are using a browser version with limited support for CSS. To obtain the best experience, we recommend you use a more up to date browser (or turn off compatibility mode in Internet Explorer). In the meantime, to ensure continued support, we are displaying the site without styles and JavaScript.
- View all journals
- Explore content
- About the journal
- Publish with us
- Sign up for alerts
- Open access
- Published: 11 September 2024
Video frame interpolation neural network for 3D tomography across different length scales
- Laura Gambini ORCID: orcid.org/0000-0001-6316-7498 1 , 2 ,
- Cian Gabbett ORCID: orcid.org/0000-0003-0957-7714 1 , 2 ,
- Luke Doolan ORCID: orcid.org/0000-0002-2622-3857 1 , 2 ,
- Lewys Jones ORCID: orcid.org/0000-0002-6907-0731 1 , 2 , 3 ,
- Jonathan N. Coleman ORCID: orcid.org/0000-0001-9659-9721 1 , 2 ,
- Paddy Gilligan 4 &
- Stefano Sanvito ORCID: orcid.org/0000-0002-0291-715X 1 , 2
Nature Communications volume 15 , Article number: 7962 ( 2024 ) Cite this article
1 Altmetric
Metrics details
- Materials science
- Medical research
Three-dimensional (3D) tomography is a powerful investigative tool for many scientific domains, going from materials science, to engineering, to medicine. Many factors may limit the 3D resolution, often spatially anisotropic, compromising the precision of the information retrievable. A neural network, designed for video-frame interpolation, is employed to enhance tomographic images, achieving cubic-voxel resolution. The method is applied to distinct domains: the investigation of the morphology of printed graphene nanosheets networks, obtained via focused ion beam-scanning electron microscope (FIB-SEM), magnetic resonance imaging of the human brain, and X-ray computed tomography scans of the abdomen. The accuracy of the 3D tomographic maps can be quantified through computer-vision metrics, but most importantly with the precision on the physical quantities retrievable from the reconstructions, in the case of FIB-SEM the porosity, tortuosity, and effective diffusivity. This work showcases a versatile image-augmentation strategy for optimizing 3D tomography acquisition conditions, while preserving the information content.
Similar content being viewed by others
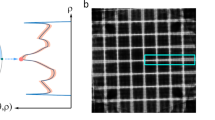
Sub-10 second fly-scan nano-tomography using machine learning
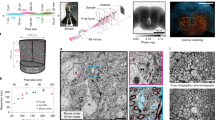
Dense neuronal reconstruction through X-ray holographic nano-tomography
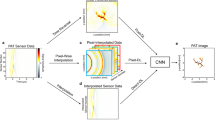
Limited-View and Sparse Photoacoustic Tomography for Neuroimaging with Deep Learning
Introduction.
Three-dimensional (3D) tomography refers to a collection of methods used for producing 3D representations of the internal structure of a solid object. This practice is commonly used for the study of specimens, where the material properties are strongly related to their internal structure. The morphology of a sample can be practically defined over many different length scales. A good example in materials science is that of networks of solution-processed nanomaterials, widely employed in electronics, energy, and sensing 1 , 2 . For these, the charge transport is determined by the morphology of the contacts between nanosheets, which are defined at a few tens of nanometers length scale. At the opposite side of the length-scale spectrum, one finds medical imaging techniques 3 , such as magnetic resonance imaging (MRI) and X-ray computed tomography (CT), where the relevant information is typically available with millimeter resolution.
There are several limitations to 3D tomography, common to many experimental techniques and length scales. Firstly, the resolution achieved must be sufficient for extracting the desired information, but it is often limited by the measuring technique and the necessity to keep the acquisition time short. For instance, in a CT scan, one wants to have enough details to inform a medical decision but limit the radiation dose the patient is exposed to 4 . Furthermore, in several cases, the measurement is destructive, meaning that the specimen being imaged is destroyed during the measurement process 5 , 6 . In this situation the 3D resolution is often anisotropic, meaning that cubic-voxel definition in the three dimensions is not achieved. In addition, in a destructive experiment one cannot go back and take a second measurement, should the first have not achieved enough resolution. The crucial question is then whether or not an image augmentation method may help in enhancing the image quality, in terms of resolution, thus enabling the extraction of more precise information. Should this be possible, one can also revert the question and establish which experimental conditions will be enabled by an image-augmentation method achieving the same information content as the non-corrected measurement. In order to understand better these issues, let us consider the specific case of FIB-SEM nanotomography (FIB-SEM-NT), where the term nano refers to the length scale involved 5 . This is a destructive imaging technique, where a focused-ion beam (FIB) mills away slices of a specimen, often a composite, while a scanning electron microscope (SEM) takes images of the exposed planes. The typical outcome is a stack of hundreds of 2D images, used to produce a high-fidelity 3D reconstruction. The resolution of the resulting 3D volume is often anisotropic, especially when working at high resolution. In fact, while the cross-section (also referred to as the xy-plane) is imaged at the SEM resolution, about 5 nm in our case, the resolution along the milling direction (the z -direction) corresponds to the slice thickness, and it is usually around 10–20 nm. As a consequence, the reconstructed 3D volume will not be characterized by cubic voxels. Note that cutting thinner slices is hindered by the instrumentation, the nature of the specimen, and economic constraints. Moreover, a reduction of the slice thickness implies a detriment of the resolution in the xy-plane, since the damage produced during one cut can propagate to the following one. This limitation is linked to another problem of FIB-SEM instruments, namely the slow imaging speed 7 . One would then desire a method to interpolate images, which preserves and possibly enhances the information quality and ideally reduces the number of milling steps to perform.
The simplest solution is linear interpolation 8 . However, this is reliable only when one can safely assume that the structural variations across consecutive cross-sections are smooth. Unfortunately, when this condition is only approximately met, linear interpolation tends to blur feature edges. This can be partially improved 9 with interpolation strategies that account for feature changes among consecutive images by using optical flow 10 , but the performance remains poor at the image borders. As a consequence, such portions of the frame must be discarded, with a consequent loss of valuable information. Alternative solutions involve deep-learning algorithms. For instance, Hagita et al. 11 proposed a deep-learning-based method for super-resolution of 3D images with asymmetric sampling. The model was trained on images obtained from the cross-section and applied to frames co-planar to the milling direction and obtained from the 3D reconstruction. Unfortunately, this strategy works only when it is possible to assume that the three directions have the same morphology, but does not provide unbiased reconstruction. In a different effort, Dahari et al. 12 developed a generative adversarial network (GAN) trained on pairs of high-resolution 2D images and low-resolution 3D data, aiming at generating a super-resolved 3D volume. The scheme showed success on a variety of datasets. However, generative models are ambiguous to use in this context since they do not allow one to find a unique solution, due to the nature of this deep-learning architecture.
Here, we propose an alternative solution that relies on the use of a deep-learning model trained for video-frame interpolation. This is a process, where the frame per second of a video is increased by generating additional frames between the existing ones, thus creating a more visually fluid motion 13 . Common applications include the generation of slow-motion videos 14 and video predictions 15 . Video-frame interpolation can be considered as a combination of both enhancement and reconstruction technology. In fact, it enhances the perceptual quality of a video by providing smoother and more natural motion. At the same time, it can be designated as a form of video reconstruction, since it aims at reconstructing the temporal evolution of a scene. Several deep-learning frameworks have been proposed for this task. The state-of-the-art algorithm is the Real-Time Intermediate Flow Estimation ( rife ) 16 , which will be extensively used here. The selected video-frame interpolation method, like any neural network developed for this purpose, is inherently bound by certain limitations. It is important to note that such networks are constrained by the information contained in the frames provided. The limitations associated with the chosen video-frame interpolation method have been studied in the original paper 16 and are anticipated to be transferable to any dataset under investigation.
We begin by considering a dataset made of printed graphene-nanosheet images, obtained with FIB-SEM, where the milling direction is taken as correspondent to the video time direction. The resolution of this dataset is then improved by the application of rife , and quantitatively validated using several approaches. In particular, together with standard computer-vision metrics, we evaluate physical quantities, which can be extracted from the final 3D reconstructions, after appropriate image binarization with standard software such as F iji 17 or D ragonfly 18 . These are the porosity, tortuosity, and effective diffusivity, and their precise evaluation allows us to understand what information content is preserved during the interpolation. Then, the same scheme is applied, at a completely different length scale, to both MRI and CT scans. In the first case, the 3D mapping is already isotropic, so that our reconstructed images can be compared to an available ground truth, as in the case of FIB-SEM. Instead, for CT scans, we show a significant enhancement of the picture quality, a result that may enable us to reduce the scanning rate and, therefore, the radiation dose for the patient.
Importantly, these are only some illustrative applications of the proposed strategy, which could be useful in many other fields. Some examples include in-situ electron microscopy 19 , such as transmission electron microscopy (TEM) and scanning TEM. In this context, a reduction of recorded frames is crucial to minimize the beam dose and, therefore, the possible beam-induced damage to the specimen, and may allow to reduce the number of tilt images taken to obtain a tomographic TEM reconstruction. In this work, we demonstrate the applicability of a neural network developed for video frame interpolation, in contexts different from its original purpose. In particular, we employ this neural network to ameliorate the acquisition conditions of 3D tomography across different length scales. Throughout this work, we compare our algorithm to alternative interpolation strategies, demonstrating its quantitative advantage.
The first test was conducted on a dataset made of printed nanostructured graphene networks, described in the Methods section. In order to prove the method’s efficacy, some frames are removed from the dataset and used as ground truth for results assessment. We consider different scenarios where one, three and seven consecutive frames are removed from the image sequence, although the seven-frame error suggests that it is not advisable to reduce so drastically the image density along the milling direction. A simple visual comparison offers a qualitative overview of the efficacy of the various interpolation methods. This is shown in Fig. 1 for the case where three consecutive frames are removed from our FIB-SEM sequence and then reconstructed by the different models. The first column shows the ground-truth image, namely that removed from the original dataset, while the remaining ones contain the pictures reconstructed with various methods. In order to appreciate better the quality of the reconstructions, we also provide the difference between the ground truth and the reconstructed images (second row), the magnification of a 120 × 120-pixel portion of each picture (third row), and again the difference from their ground truth (fourth row). The differences are obtained by simply subtracting the greyscale bitmap of each pixel. Blue (red) regions mean that the reconstructed image appears lighter (darker) than the original one.
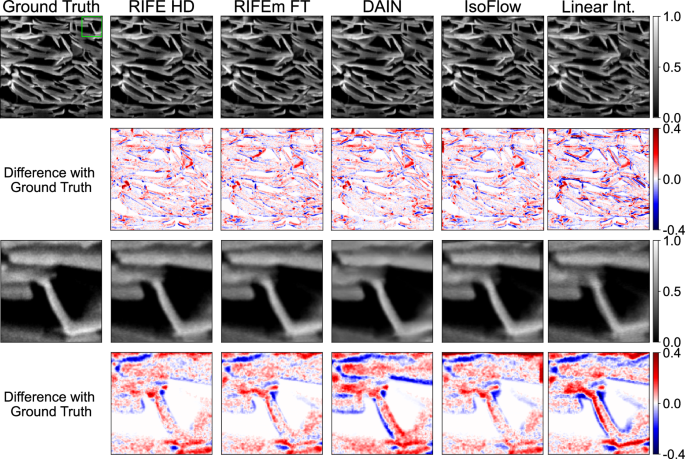
Images from the FIB-SEM dataset are augmented using different approaches. In particular, three additional frames are generated every two consecutive frames in the sequence. From left to right we show: the ground truth image (original FIB-SEM), and those reconstructed by rife hd , the fine-tuned rife m , dain , IsoFlow , and linear interpolation. The second row displays the difference between the ground truth and the reconstructions. A 120 × 120-pixel portion of each image (see green box in the upper left panel) is magnified and shown in the third row, while the differences from the original image are in the fourth row.
The inspection of the figure leads to some qualitative considerations on the different methods, and the comparison is particularly clear for the magnified images. The most notable feature is the loss of sharpness brought by the linear interpolation, which is not motion-aware. In fact, instead of tracing the motion of the border between a graphene nanosheet and a pore, namely the border region between dark and bright pixels, linear interpolation simply fills the space with an average grayscale. As a result, the image differences (e.g., see the rightmost lower panel) present some dipolar distribution, which, as we will show below, causes information loss. A similar, although less pronounced, drawback is found for images reconstructed by dain , which also tends to over-smooth the graphene borders. In contrast, I so F low appears to generate generally good-quality pictures, in particular in the middle of the frame. However, one can clearly notice a significant error appearing at the image border, which is not well reproduced and whose information thus needs to be discarded. Finally, the two rife models are clearly the best-performing ones. Of similar quality, they are able to maintain the original image sharpness across the entire field of view and do not seem to show any systematic failure. Although instructive, visual inspection just provides a qualitative understanding, more quantitative metrics need to be evaluated in order to determine what image content is preserved by the various reconstructions.
Quantitative assessment of the extracted information content
A full quantitative analysis is better performed on segmented images, where the pore and nanosheet components are well separated 5 . This can be obtained by using the trainable weka segmentation tool 20 available in F iji 17 . The procedure to produce binarised data is demonstrated in Fig. 2 . A set of images from the original dataset is used to train a model, whose goal is to classify each pixel of the image either as pore or as nanosheet. The training set is automatically built by weka following the manual identification of pore and nanosheet areas from the user. This is shown in Fig. 2 a, where the red circles identify pixels that are labeled as pores, and the green circles represent pixels labeled as nanosheets. Panel (b) of the same figure displays the outcome of the application of the trained model, where the grayscale expresses the probability of each pixel being labeled as a pore or nanosheet. This is called a probability map. Finally, a threshold is applied to obtain a binary classification, as shown in Fig. 2 c. In particular, here, the I sodata algorithm 21 , available in F iji , is used to select an appropriate threshold.
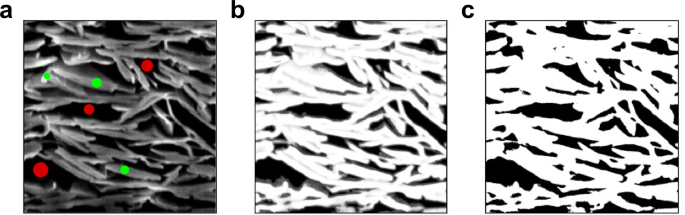
Different phases of the procedure used to segment each frame into pore and nanosheet components. This generates binarised data by using the trainable weka segmentation plugin of Fiji 5 , 20 . In panel (a ) the user first manually assigns the label of pore (red circles) and nanosheet (green circles) to some areas of the original image. This information is used to build the dataset for training a classifier, whose output is the probability map displayed in panel ( b ). Here the probability of each pixel being either pore or nanosheet is displayed using pixel intensity values. Panel ( c ) shows the final output of the procedure, namely the binarised image, obtained by applying a threshold on the probability map.
Once the classifier is trained and the threshold is established, all the datasets obtained from the different interpolation strategies can be binarised. It should be noted that the performance of the classifier depends on the manual selection performed by the user. However, using the same classifier for all the analyzed datasets guarantees consistency in the segmentation process and, consequently, in the quantitative assessment of the various reconstruction methods.
The Mean Square Error (MSE) and the Structure Similarity Index Method (SSIM) are some of the standard metrics used in computer vision to evaluate results 22 . Both are full-reference metrics, meaning that the ground truth is required to assess their value. The MSE focuses on the pixel-by-pixel comparison and not on the structure of the image, while SSIM performs better in discriminating the structural information of the frames. Here the MSE is calculated between each of the generated frames and the corresponding image removed from the original dataset. The average of these values is then computed for each case of study (one, three, and seven replaced frames) and for each technique ( rife hd , rife m , dain , I so F low and linear interpolation), for a set of 100 images. The same procedure is followed for the evaluation of the SSIM, and our results are available in panels a and b of Fig. 3 . As expected, all models perform better when the number of removed frames remains limited, and in general, there is a significant loss in performance for the case of seven replaced frames. In more detail, rife -type schemes are always the top performer, with linear interpolation and also dain , remaining the most problematic. Interestingly, I so F low appears quite accurate according to these computer-vision metrics, which clearly do not emphasize the loss of resolution at the frame boundary. However, we will now see that this does not necessarily translate into the ability to preserve information.
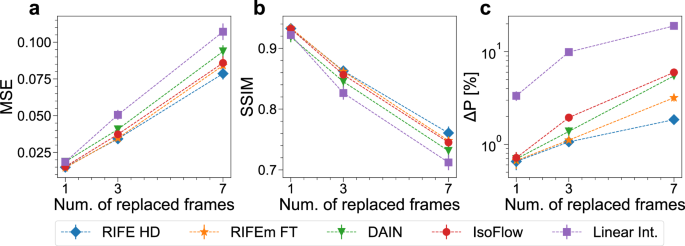
Mean Squared Error (MSE - panel a ), Structural Similarity Index Method (SSIM - panel b ), and Porosity (P - panel c ) were evaluated for each test case (one, three, and seven replaced frames), and each interpolation method, for 100 images. MSE and SSIM are evaluated for each frame against the ground truth and they are expressed as an average over the 100 frames. P is also evaluated for each frame against the ground truth, and it is expressed in terms of Δ P [see Eq. ( 1 )], namely as a percentage deviation from the ground-truth value. For each panel, the average value is shown with the associated variance.
Ultimately, the quality of a reconstruction procedure must be measured with the quality of the information that is able to be transferred/retrieved. In the case of printed graphene-nanosheet ensembles, the morphological properties can be measured and compared. The so-called network porosity, P , defined as the percentage of the total volume occupied by the pores, is one of the most important features measured in 2D networks and it affects the material electrical properties 23 . In our image-segmented 3D reconstruction this translates into the fraction of pores surface in each image, a quantity that can be evaluated from the binarised images by the conventional image-processing software fiji 17 . Such analysis is performed here for each case of study and technique. In particular, from the binarised data, the porosity of each frame is computed as P = (pores pixels)/(total pixels).
The results are then expressed in terms of delta porosity, Δ P , which is the fractional difference between the porosity computed from images reconstructed with a particular method m, P m , and that of the ground truth, P GT , namely
In our case, the Δ P of 100 images is computed, and the average of these values is presented in panel c of Fig. 3 . From the figure, the advantage of using rife is quite clear. In fact, although all methods, except for the linear interpolation, give a faithful approximation of P when one frame is removed from the sequence, differences start to emerge already at three replaced frames, where rife significantly outperforms all other schemes. The difference becomes even more evident for seven replaced frames, for which the rife error remains surprisingly below 2%. Also, it is interesting to note that, in contrast to what is suggested by the computer-vision metrics, I so F low is not capable of accurately returning a precise porosity, mainly because of the poor description of the image borders. In contrast, the weak performance of linear interpolation has to be attributed to its inability to describe sharp borders between nanosheets and pores.
A second important structural feature that can be retrieved from nanostructured networks is the network tortuosity, τ , which can be evaluated on binarised data using the T au F actor software 24 . This quantity describes the effect that a convolution in the geometry of heterogeneous media has on diffusive transport and can be measured for both the nanosheet and pore volumes. The nanosheet network tortuosity factor influences charge transport through the film. Pore tortuosity affects performance in nanosheet-based battery electrodes, while in gas sensing applications, pore tortuosity is directly linked to gas diffusion. The tortuosity, τ , and volume fraction, ε , of a phase are used to relate the reduction in the diffusive flux through that phase by comparing its effective diffusivity, D eff , to the intrinsic diffusivity, D :
The T au F actor software calculates the tortuosity from stacks of segmented images. Specifically, it employs an over-relaxed iterative method to determine the tortuosity. This approach is utilized for solving the steady-state diffusion equation governing the movement of species in an infinitely dilute solution within a porous medium confined by fixed-value boundaries. It has been proved that for the evaluation of the tortuosity and diffusivity, the sample volume needs to be adequately large, in order to be representative of the bulk and to reduce the effect of microscopic heterogeneities 25 . For this reason, we consider ten randomly selected volumes, ranging from 55% to 60% of the original one. Such fraction has been chosen after performing a scaling analysis, namely by computing the tortuosity factor for different volumes and by noticing that the bulk limit was obtained at around 55% (see Supplementary Information ). As the size of the input sample highly affects the computation speed and memory requirement, not all methods are considered for this comparison. In particular, only rife hd and dain results are used as input for the tortuosity and diffusivity study. These two methods are chosen since they provide the best evaluation of the porosity. Then, the Python version of T au F actor 26 is run on Quadro RTX 8000 GPUs.
Also in this case, we compute the fractional change of any given quantity from the ground truth, and the results are displayed in Fig. 4 . Confirming the results obtained for Δ P also in this case rife is the best-performing method, with errors remaining below 2% at three replaced frames for both τ and D eff . In contrast, dain displays significant errors, exceeding 10%, already for a single replaced frame, an error that suggested analysis at other replaced-frame rates was unnecessary.
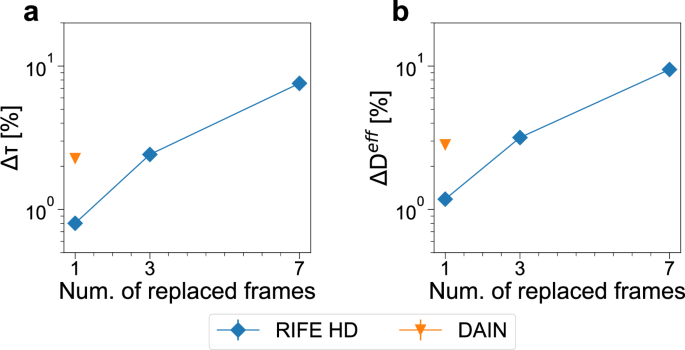
Delta tortuosity, Δ τ (panel a), and delta effective diffusivity, Δ D eff (panel b ), were evaluated for each test case (one, three, and seven replaced frames) for rife hd , and for one replaced frame for the dain interpolation. The metrics are evaluated for each frame against the ground truth and averaged over the sequence. The variance is also displayed, and in the case of rife, it is smaller than the symbol size.
Analyzing the properties outlined in this section is an essential aspect for all applications involving transport in heterogeneous media, influenced by geometry. For instance, in the field of catalysis, the mentioned properties are used to determine the extent of accessibility to the nanosheet surface area.
Dependence on the features size
As demonstrated in the previous section, our method performs well at reconstructing the frames of the FIB-SEM sequence, with errors on physically relevant quantities remaining below 10% even for seven replaced frames. This is equivalent to having a milling thickness of about 100 nm, indeed a very favorable experimental condition. In this section, we discuss the limitations of our approach in relation to the type of sample to investigate. A relevant problem with image interpolation techniques concerns the level of continuity between consecutive frames. In fact, it is well understood that rapid changes between the images in a sequence can reduce the quality of the interpolated frames 13 . The same issue may arise when considering FIB-SEM measurements of graphene nanosheets of different lengths. In this case, shorter nanosheets will result in FIB-SEM images with more abrupt changes between consecutive frames. For instance, if the average nanosheet length is L and the milling distance \({L}^{{\prime} }\) , for \(L \sim {L}^{{\prime} }\) one will often encounter a situation where a nanosheet present in one image will not appear in the next one.
In order to explore how the proposed model works with increasingly challenging datasets, we investigate the case networks made of shorter graphene nanosheets, namely 80 nm and 298 nm in length (these are the average lengths). Examples of such networks, together with the original one of 695 nm, can be found in Fig. 5 . In this case, we use Δ P as an evaluation criterion and consider three replaced frames, together with the original rife hd model. Our results can be found in Fig. 6 , where we show Δ P against the average nanosheet length. For this comparison, the data are binarised following the procedure described in the previous section, and Δ P is computed as an average over 100 images. It is evident from the figure, as expected, that the performance of our model indeed deteriorates when reducing the nanosheet’s length. However, even for the smallest sample, 80 nm, the error remains below a very acceptable 2%. Note that in these conditions (nanosheet length 80 nm and three replaced frames, equivalent to ~ 50 nm milling distance) the milling distance is about half of the average feature size of the sample. Since networks made of small flakes are certainly structurally more fragile than those made with larger ones, the fact that the milling frequency can be reduced significantly without a sensible loss in the accuracy of the morphology determination establishes a possible new experimental condition, where the milling effects on the final morphology are strongly minimized.
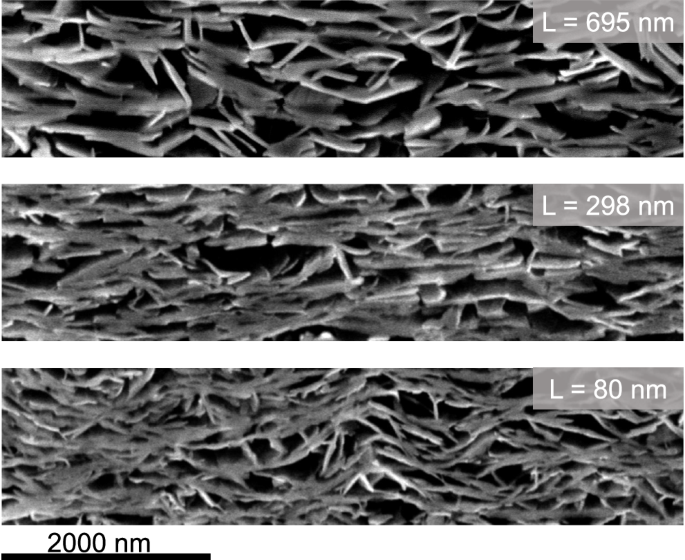
Cross-sections of printed graphene nanosheets of different lengths (L). The nanosheet length decreases going from the top panel to the bottom one. In each case, the image width shown is 6510 nm.
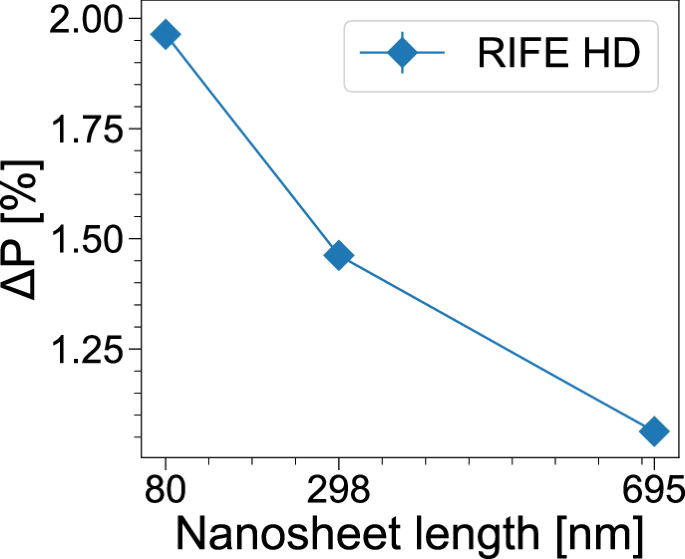
Porosity was evaluated for the three replaced frame cases for different nanosheet lengths (80 nm, 298 nm, and 695 nm). The porosity is evaluated for each frame generated by rife hd against the ground truth, and it is expressed in terms of Δ P [see Eq. ( 1 )]. Here we show the average Δ P over 100 images and the associated variance.
Application to medical datasets
The strategy proposed in this work is not limited to FIB-SEM generated data but can be employed to increase the through-plane resolution of datasets of materials at different scales, obtained by using different imaging instruments. The purpose of this section is exactly to show such transfer across scales, and for this reason, we did not perform any additional training or fine-tuning of the original model. As such, we now consider only rife hd . The first example is an application of rife to human brain MRI scans. For this dataset, the voxels of the reconstructed volume are already cubic with a 1 mm 3 resolution. However, this is a useful case study, since it is possible to remove frames from the scan sequence and use them as ground truth for the validation, as in the case of FIB-SEM. In particular, we remove every other frame from the original dataset, which has been downloaded from the Brainstorm repository 27 , 28 . This is well documented and freely available online for download under the GNU general public license. For this study, we consider brain scans in the sagittal view as input for hd rife .
A visual comparison of one original and the corresponding rife -generated slice in the sagittal view is shown in the first row of Fig. 7 (panels a, b, c), where the patient was defaced to fulfill privacy requirements. The third column (panels c and f) of the mentioned figure shows the difference between the original and the generated image. Clearly, the visual inspection of the reconstructed image appears very positive with a difference from the ground truth (see top right panel), which presents an error similar to that of the FIB-SEM data, despite the rather different length scale, and little structure in its distribution.
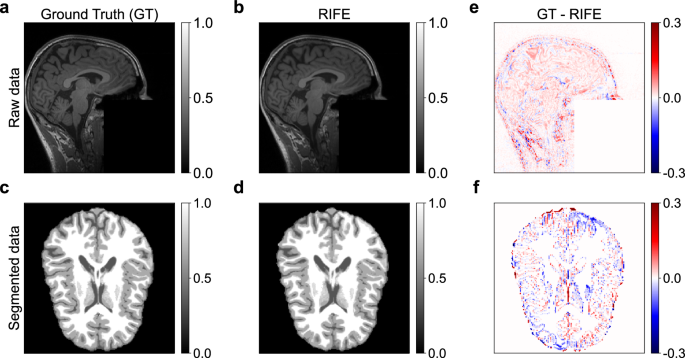
An example of the original and the corresponding rife -generated frame in the sagittal view is shown in panels ( a ) and ( b ), respectively. Panel ( c ) displays the intensity difference between the ground truth and the rife -generated images. The two datasets are segmented by using the Anatomical pipeline of the BrainsSuite software, as displayed in the second row. The original and corresponding rife -generated data are shown in panels ( d ) and ( e) , respectively, while panel ( f ) presents the difference between them. Although the results are here presented in axial view only, the segmentation is performed on the full volume.
The B rain S uite software 29 is then used to quantitatively compare the original and the rife -generated volumes, again trying to understand whether the information content is preserved. More specifically, the Anatomical pipeline is performed on both stacks to obtain the full brain segmentation, whose output is shown in the axial view in panels d and e of Fig. 7 . Also, in this case, the visual inspection is similar to that made for the original images, with similar error characteristics. These segmentations are then used to evaluate the gray-matter volume variation (GMV), a widely used metric for the investigation of brain disorders such as Alzheimer’s disease 30 , 31 . This is defined as,
where p gray and p white indicate the number of gray and white pixels, respectively. The percentage error between the GMV of the two datasets is computed to be 0.5 %, again very low.
The final medical application investigated here refers to CT scans. Note that this measurement technique is not limited to the medical space, but it is also widely used in industrial settings and in general as a research tool across materials science 32 . The use of interpolation methods for medical CT could be really transformative since a reduction of the collected frames translates into a reduction of the radiation dose delivered to the patient. As a consequence, the potential risk of radiation-induced cancer will diminish 33 . Alternatively, one may have the possibility to perform more frequent scans for close monitoring of particular diseases. The dataset used for this investigation is downloaded from the Cancer Imaging Archive 34 , 35 and is provided as a set of 152 frames in the axial view, with a pixel size of 0.74 × 0.74 × 2.49 mm. For this example, the voxels in the reconstructed volume are not cubic, and no ground truth is available. rife is then used to generate three additional frames between every two existing ones. The results can be seen in Figs. 8 and 9 . Figure 8 shows the data in axial view. Panel a shows one of the original frames of the CT dataset, while panel b presents a frame generated with rife , using the former image as an input for the interpolation model. It is important to note that in this case, the two panels do not represent the same section of the body, therefore they can only be compared from a qualitative point of view. From this figure, we can only observe that rife is able to generate realistic representations of CT scans in the axial view. Figure 9 displays the original and rife -augmented datasets in coronal view on panels a and b, respectively. The original-sized data are shown in the first row, while the second row (panels c and d) presents 150 × 150-pixel magnified portions of the data (indicated by green boxes in the first row). The red and blue boxes are used for the noise power spectrum analysis that will be described shortly. For the coronal view, the comparative figure helps assess the improvement introduced by rife . This visual comparison appears quite favorable, with the rife reconstruction being, in general, smoother than the original image. This is, of course, the result of having more frames added to the sequence.
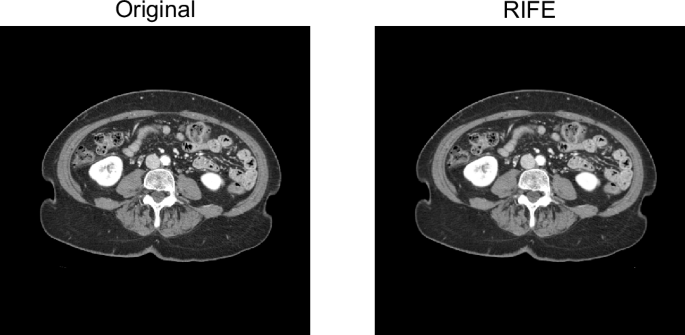
Here the results are presented in the axial view, namely the view in which the original sequence, used as input for rife , is depicted. On the left-hand-side panel, there is one of the original frames from the CT dataset, while the right-hand-side panel displays a frame created using rife , with the former image serving as an input for the interpolation model. It is essential to emphasize that these two panels depict different sections of the body, allowing for only a qualitative comparison. This figure enables us to discern that rife effectively produces reasonable representations of CT scans in the axial view.
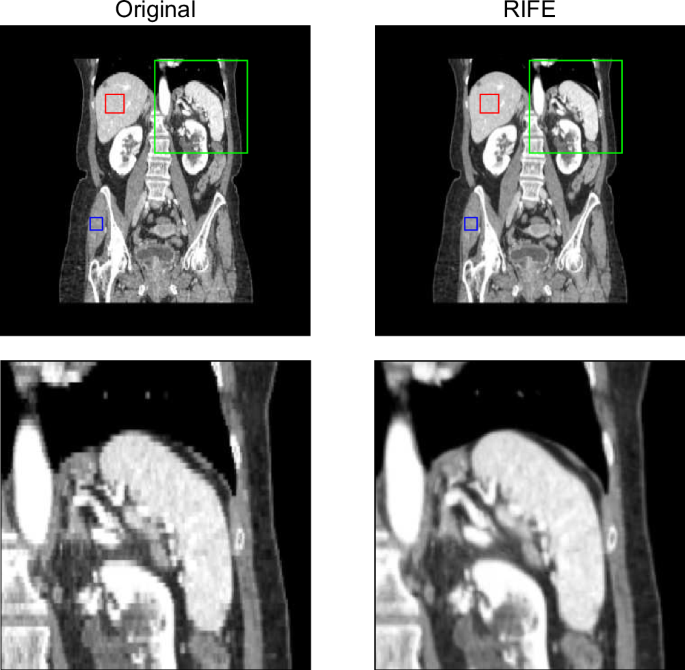
Here the results are presented in the coronal view. Panel ( a ) displays the original dataset, while panel ( b ) shows the rife -augmented dataset, where three additional frames have been added between every two consecutive frames. The green boxes in the first row of the figure indicate a 150 × 150-pixel portion of the original-sized data that is magnified in the second row. The red (Case 1) and blue (Case 2) boxes represent the uniform regions used for the noise power spectrum analysis, whose results are presented in Fig. 10 .
Since no ground truth is available in this case, we need to resource computer-vision metrics to provide a quantitative assessment of the reconstruction procedure. Therefore, the two image stacks, the original and the rife -reconstructed one are compared by estimating the noise power spectrum on the coronal plane, computed over uniform regions of a sequence of ten images. This metric is generally used for the assessment of the image quality of CT scanners and it is evaluated over uniform regions of interest in water-filled phantoms 36 . As such, in order to adapt this metric to clinical datasets, it is necessary to select uniform areas of the image. For instance, the areas in Fig. 9 marked with the red and blue squares represent two possible regions of interest, here called ‘case 1’ and ‘case 2’, respectively. The noise power spectrum of these areas, evaluated over a stack of ten frames, is shown in Fig. 10 . From the analysis of both cases, it is evident that this time augmenting the number of frames using rife hd is associated with a reduction of the noise in the data, a reduction visible across the entire frequency range. This quantifies the original observation that rife -augmented images look smoother. The same consideration holds for other uniform regions that have been investigated.
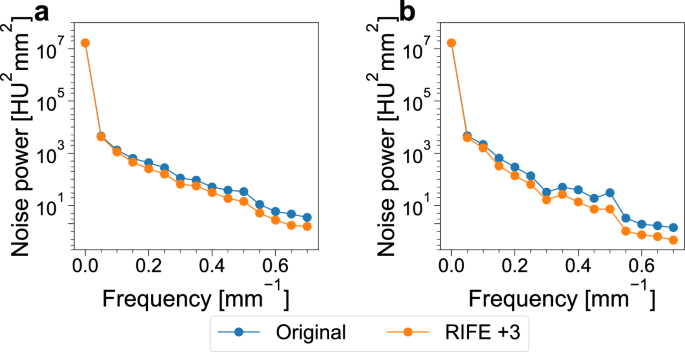
Analysis of the noise power spectrum conducted over two uniform regions in the coronal view (see Fig. 9 for definition). The comparison is performed between the original and the rife -augmented dataset. The use of rife results in noise reduction for both the selected regions and across the entire frequency range. The first case is displayed in panel ( a ), and the second case is displayed in panel ( b ).
It is worth mentioning that medical images undergo significant processing, which impacts how noise and resolution appear in the final results. This is influenced by several factors, such as image acquisition techniques, reconstruction methodologies, and any additional post-processing steps. Further improvements, achievable with different approaches, were not investigated in this work.
We wish to conclude this section by making two considerations concerning the augmentation of sequences of images in the medical domain. In general, this has two main issues. Firstly, one has to ensure that the quality of the reconstructed images in a sequence is very close to the quality of the physically acquired images so that the information content in a sequence is preserved. Such a test has been performed here for the FIB-SEM case, for which ground truth was available, but only partially for the medical examples. In fact, for the medical examples, we were able just to evaluate computer-graphic metrics but not the information content, since no ground truth was accessible to us. Such a test may certainly become possible in the future, initially, mostly likely, through real images taken with dummies. The second issue is significantly more complex, as one has to evaluate whether the enhanced tomography helps the practitioners to make better decisions. This is an issue that must be resolved through a tight collaboration with medical experts, who will provide their human judgment on the usefulness of the augmentation. The format of such evaluation has still to be developed, but it is certainly desirable to formulate a protocol for both the research and the regulatory environments. In the absence of this, the deployment of AI-technology in the medical field will certainly remain at large.
We have demonstrated that a state-of-the-art neural network developed for video-frame interpolation can be used to increase the resolution of image sequences in 3D tomography. This can be applied, without further training, across different length scales, going from a few nanometers to millimeters, and to the most diverse types of samples. As the main benchmark, we have considered a dataset of images of printed graphene nanostructured networks, obtained with the destructive FIB-SEM technique. For this, we have carefully evaluated computer-vision metrics, but most importantly, the quality of the information content that can be extracted from the 3D reconstruction. In particular, we have computed the porosity, tortuosity, and effective diffusivity of the original dataset. This was then compared to datasets where an increasing number of images were removed and replaced with computer-generated ones.
In general, we have found that motion-aware video-frame interpolation outperforms the other interpolation strategies we tested. In particular, we have shown that it is not prone to image blurring, typical of simple linear interpolation, or to resolution loss at the image boundaries, as shown by some hybrid optical-flow algorithms. This is due to the coarse-to-fine approach implemented within the rife model, which allows one to make accurate predictions both in terms of intermediate flow estimation and level of detail, as demonstrated in the original paper for several datasets 16 . Most importantly, the error on the determination of morphological observables remains below 2% as long as the milling thickness is less than approximately half of the nanosheet length. This suggests a very favorable experimental condition, where the effects of the milling on the measured morphology are significantly mitigated.
Then, we have moved our analysis to datasets taken from the medical field. These include a 3D tomography of the human brain volume, acquired with magnetic resonance imaging, and the X-ray computed tomography of the human torso. In the first case a ground truth was available, and we have been able to show that the estimate of the gray-matter volume variation is only affected by 0.5%, when half of the images in a scan are replaced with video-frame interpolated ones. This suggests that the scan rate can be actually increased, saving in acquisition time. In contrast, for the CT scan, no ground truth was available, so we limited ourselves to estimating computer-vision metrics. In particular, we have shown that the augmentation of data with computer-interpolated images, in order to reach cubic-voxel resolution, improves the power spectrum of the tomographic reconstruction, confirming the visual impression of smoother images. This result may be potentially transformative, since it can pave the way for reduced scan rates, with the consequent reduction in the radiation dose to be administrated to the patient.
In summary, we have shown that video-frame interpolation techniques can be successfully applied to 3D tomography regardless of the acquisition experimental technique and the nature of the specimen to the image. This can improve practices when radiation-dose damage or the acquisition time are issues limiting the applicability of the method.
Printed graphene network dataset
The main dataset used in this work contains 801 images, generated with FIB-SEM, of printed nanostructured graphene networks, with a nanosheet length of approximately 700 nm. Each image, made of 4041 × 510 pixels, has a 5 nm resolution in the cross-section, while the slice thickness is 15 nm. Therefore, the voxel size in the resulting reconstructed volume is 5 × 5 × 15 = 375 nm 3 . Note that the voxel size achievable with conventional micro CT scanners is 10–1000 times larger 37 , 38 . Therefore, FIB-SEM nanotomography is more suitable than CT for the quantitative characterization of the graphene network morphology. Further details on sample preparation and data acquisition can be found in ref. 5 .
A fraction of the original dataset is considered for the majority of the analysis, so as to reduce the computational effort and to inspect the images in more detail. Specifically, for the computer-vision metrics and porosity analysis, 100 images of the original dataset are considered. Each image of this subset is cut to a 510 × 510 pixels size. In contrast, for the tortuosity and effective diffusivity study, ten randomly selected volumes are considered, ranging from 55% to 60% of the original volume. It should be noted that in all cases, the resolution is not altered.
Neural network
Five main methodologies are usually employed for video-frame interpolation, namely flow-based methods, convolutional neural networks (CNN), phase-based approaches, GANs, and hybrid schemes. These typically differ from each other because of the network architecture and their mathematical foundation 39 . rife 16 belongs to the flow-based category, whose focus is the determination of the nature of the flow between corresponding elements in consecutive frames. When compared to other popular algorithms 40 , 41 , 42 , rife performs better both in terms of accuracy and computational speed. Models belonging to this class usually involve a two-phase process: the warping of input frames in accordance with the approximated optical flow and the use of CNNs to combine and refine the warped frames. The outcome of the intermediate flow estimation often requires the presence of additional components, such as depth-estimation 40 and flow-refinement models 41 , so to mitigate potential inaccuracy. Unlike other methods, rife does not require supplementary networks, a feature that significantly impacts the model speed. In fact, the intermediate flow is learned end-to-end by a CNN. As demonstrated in the original rife paper 16 , learning the intermediate flow end-to-end can reduce motion-modeling related inaccuracies. rife adopts a neural-network architecture, IFNet, which directly estimates the intermediate flow adopting a coarse-to-fine approach with progressively increased resolution. In particular, the first step is an approximated prediction of the intermediate flow on low resolution. This allows one to capture large motions between consecutive frames. Subsequently, the prediction is refined iteratively, by considering frames with gradually increased resolution, a procedure that allows the model to retain fine details in the interpolated frame. This approach guarantees accurate results, both in terms of flow estimation and level of detail in the generated frame. Moreover, a privileged distillation scheme is introduced to train the model. Specifically, a teacher model with access to the ground-truth intermediate frame refines the student model performance. The input of the rife model can either be a video or a sequence of two images. For this project, the most straightforward solution is to use images. Therefore, we have adapted the model to accept a series of any number of images, instead of only two at a time. The new version of the inference file of the code can be found at the link provided in this manuscript. Although the model is trained on RGB data, it can seamlessly handle grayscale images, as this functionality is inherently embedded in the original code. Specifically, the images are loaded using an O pen CV 43 flag, which ensures that they are loaded as grayscale data. As with any large machine-learning model, rife updates regularly. At the current moment in time, the best version available is the HD model v4.6, referred to as rife hd 44 . This is trained on the Vimeo90K dataset, which covers a large variety of scenes and actions, involving people, animals, and objects 45 . As the training set is remarkably different from the application cases of this work, we perform fine-tuning of the available pre-trained model on Quadro RTX 8000 GPUs, provided by Nvidia. Since fine-tuning of rife hd is currently not possible, the second-best model is here considered, namely rife m 46 . The fine-tuning is then performed on a subset of the graphene dataset, made of 1000 portions of the original images, cropped to a 510 × 510-pixel size, and not used for testing. The instructions provided by the rife code developers are followed to perform the fine-tuning, with some small code modifications. The modified training file can be found in the provided repository. The results of both the original and the fine-tuned model are presented in this work and compared throughout. Furthermore, we benchmark our scheme against another flow-based deep-learning algorithm, dain 40 , and against non-deep-learning methods. In particular, we consider the simple but widely used linear interpolation and the I so F low algorithm 9 , an interpolation technique that takes into consideration the variation among slices by using optical flow 10 .
In closing, we note that here we have explored the current state of the art in video-frame interpolation. Certainly, one can also expect that our results will further improve as newer and more accurate machine-learning architectures for video-frame interpolation become available.
Reporting summary
Further information on research design is available in the Nature Portfolio Reporting Summary linked to this article.
Data availability
The data that support the findings of this study are available from the corresponding author upon request. Source data are provided in this paper.
Code availability
The main code used for this project, developed by Huang et al., can be found at ref. 44 , 46 . Some minor changes were made to this code, the modified version is available at: https://github.com/StefanoSanvitoGroup/RIFE-3D-tom 47 .
Tan, D., Jiang, C., Li, Q., Bi, S. & Song, J. Silver nanowire networks with preparations and applications: a review. J. Mater. Sci. Mater. Electron. 31 , 15669–15696 (2020).
Article CAS Google Scholar
Carey, T. et al. Inkjet printed circuits with 2d semiconductor inks for high-performance electronics. Adv. Electron. Mater. 7 , 2100112 (2021).
Zhou, L., Fan, M., Hansen, C., Johnson, C. R. & Weiskopf, D. A review of three-dimensional medical image visualization. Health Data Sci. 2022 , 840519 (2022).
Article Google Scholar
Verdun, F. R. et al. Quality initiatives radiation risk: what you should know to tell your patient. Radiographics 28 , 1807–1816 (2008).
Article PubMed Google Scholar
Gabbett, C. et al. Quantitative analysis of printed nanostructured networks using high-resolution 3D FIB-SEM nanotomography. Nat. Commun. 15 , 278 (2024).
González-Solares, E. A. et al. Imaging and molecular annotation of xenographs and tumours (imaxt): High throughput data and analysis infrastructure. Biological Imaging 3 , 11 (2023).
Xu, C. S. et al. Enhanced fib-sem systems for large-volume 3d imaging. Elife 6 , 25916 (2017).
Roldán, D., Redenbach, C., Schladitz, K., Klingele, M. & Godehardt, M. Reconstructing porous structures from fib-sem image data: Optimizing sampling scheme and image processing. Ultramicroscopy 226 , 113291 (2021).
González-Ruiz, V., García-Ortiz, J. P., Fernández-Fernández, M. & Fernández, J. J. Optical flow driven interpolation for isotropic fib-sem reconstructions. Comput. Methods Programs Biomed. 221 , 106856 (2022).
Nixon, M. & Aguado, A. Feature Extraction and Image Processing for Computer Vision . (Academic Press, London, 2019).
Google Scholar
Hagita, K., Higuchi, T. & Jinnai, H. Super-resolution for asymmetric resolution of fib-sem 3d imaging using ai with deep learning. Sci. Rep. 8 , 1–8 (2018).
Article ADS Google Scholar
Dahari, A., Kench, S., Squires, I. & Cooper, S. J. Fusion of complementary 2d and 3d mesostructural datasets using generative adversarial networks. Adv. Energy Mater. 13 , 2202407 (2023).
Parihar, A.S., Varshney, D., Pandya, K., Aggarwal, A. A comprehensive survey on video frame interpolation techniques. Vis. Comput. 38 , 295–319 (2021).
Jin, M., Hu, Z., Favaro, P. Learning to extract flawless slow motion from blurry videos. In Proceedings of the IEEE/CVF Conference on Computer Vision and Pattern Recognition , pp. 8112–8121 (2019).
Wu, Y., Wen, Q., Chen, Q. Optimizing video prediction via video frame interpolation. In Proceedings of the IEEE/CVF Conference on Computer Vision and Pattern Recognition , pp. 17814–17823 (2022).
Huang, Z., Zhang, T., Heng, W., Shi, B., Zhou, S. Real-time intermediate flow estimation for video frame interpolation. In Proceedings of the European Conference on Computer Vision (ECCV) (2022).
Schindelin, J. et al. Fiji: an open-source platform for biological-image analysis. Nat. Methods 9 , 676–682 (2012).
Article CAS PubMed Google Scholar
Object Research Systems (ORS) Inc., M. Dragonfly 3.1 (Computer Software). Available online: http://www.theobjects.com/dragonfly (2016).
Zheng, H. & Zhu, Y. Perspectives on in situ electron microscopy. Ultramicroscopy 180 , 188–196 (2017).
Arganda-Carreras, I. et al. Trainable weka segmentation: a machine learning tool for microscopy pixel classification. Bioinformatics 33 , 2424–2426 (2017).
Ridler, T. et al. Picture thresholding using an iterative selection method. IEEE Trans. Syst. Man Cybern. 8 , 630–632 (1978).
Sara, U., Akter, M. & Uddin, M. S. Image quality assessment through fsim, ssim, mse and psnr—a comparative study. J. Comput. Commun. 7 , 8–18 (2019).
Gabbett, C. Electrical, mechanical & morphological characterisation of nanosheet networks. PhD thesis, Trinity College Dublin (2021).
Cooper, S. J., Bertei, A., Shearing, P. R., Kilner, J. & Brandon, N. P. Taufactor: An open-source application for calculating tortuosity factors from tomographic data. SoftwareX 5 , 203–210 (2016).
Tjaden, B., Brett, D. J. & Shearing, P. R. Tortuosity in electrochemical devices: a review of calculation approaches. Int. Mater. Rev. 63 , 47–67 (2018).
TauFactor. https://github.com/tldr-group/taufactor (2023).
Tadel, F. et al. Meg/eeg group analysis with brainstorm. Front. Neurosci. 13 , https://doi.org/10.3389/fnins.2019.00076 (2019).
Brainstorm. http://neuroimage.usc.edu/brainstorm (2023).
Shattuck, D. W. & Leahy, R. M. Brainsuite: an automated cortical surface identification tool. Med. Image Anal. 6 , 129–142 (2002).
Thompson, P. M. et al. Dynamics of gray matter loss in alzheimer’s disease. J. Neurosci. 23 , 994–1005 (2003).
Article CAS PubMed PubMed Central Google Scholar
Nakazawa, T. et al. Multiple-region grey matter atrophy as a predictor for the development of dementia in a community: the hisayama study. J. Neurol. Neurosurg. Psychiatry 93 , 263–271 (2022).
Withers, P. J. et al. X-ray computed tomography. Nat. Rev. Methods Primers 1 , 18 (2021).
Yu, L. et al. Radiation dose reduction in computed tomography: techniques and future perspective. Imaging Med. 1 , 65 (2009).
Article PubMed PubMed Central Google Scholar
Clark, K. et al. The Cancer Imaging Archive (TCIA): maintaining and operating a public information repository. J. Digit. Imaging 26 , 1045–1057 (2013).
Rutherford, M. et al. A DICOM dataset for evaluation of medical image de-identification (Pseudo-PHI-DICOM-Data) [Data set]. The Cancer Imaging Archive https://doi.org/10.7937/s17z-r072 (2021).
Verdun, F. et al. Image quality in ct: From physical measurements to model observers. Phys. Med. 31 , 823–843 (2015).
Lavery, L., Harris, W., Bale, H. & Merkle, A. Recent advancements in 3d x-ray microscopes for additive manufacturing. Microsc. Microanal. 22 , 1762–1763 (2016).
Lim, C., Yan, B., Yin, L. & Zhu, L. Geometric characteristics of three dimensional reconstructed anode electrodes of lithium ion batteries. Energies 7 , 2558–2572 (2014).
Parihar, A.S., Varshney, D., Pandya, K., Aggarwal, A. A comprehensive survey on video frame interpolation techniques. Vis. Comput. 38 , 295–319 (2022).
Bao, W. et al. Depth-aware video frame interpolation. In Proceedings of the IEEE/CVF Conference on Computer Vision and Pattern Recognition , pp. 3703–3712 (2019).
Jiang, H. et al. Super slomo: High quality estimation of multiple intermediate frames for video interpolation. In Proceedings of the IEEE Conference on Computer Vision and Pattern Recognition, pp. 9000–9008 (2018).
Park, J., Lee, C., Kim, C.-S. Asymmetric bilateral motion estimation for video frame interpolation. In Proceedings of the IEEE/CVF International Conference on Computer Vision , pp. 14539–14548 (2021).
Bradski, G. The OpenCV Library. Dr. Dobb’s Journal of Software Tools (2000).
Practical-RIFE. https://github.com/hzwer/Practical-RIFE (2023).
Xue, T., Chen, B., Wu, J., Wei, D. & Freeman, W. T. Video enhancement with task-oriented flow. Int. J. Comput. Vis. 127 , 1106–1125 (2019).
Real-Time Intermediate Flow Estimation for Video Frame Interpolation. https://github.com/megvii-research/ECCV2022-RIFE (2023).
Gambini, L. et al. Video Frame Interpolation Neural Network for 3D Tomography Across Different Length Scales. RIFE-3D-tom https://doi.org/10.5281/zenodo.13228384 (2024).
Download references
Acknowledgements
L.G. is supported by Science Foundation Ireland (AMBER Center grant 12/RC/2278-P2). L.J. is supported by the SFI-Royal Society Fellowship (grant URF/RI/191637). J.N.C. and C.G. are supported by the European Union through the Horizon Europe project 2D-PRINTABLE (GA-101135196). L.D. is supported by the SFI-funded Center for Doctoral Training in Advanced Characterization of Materials (award number 18/EPSRC-CDT/3581). The authors thank David Caldwell, John Anthony Lee, Sojo Joseph, and Irene Hernandez Giron for useful discussions. The authors also acknowledge Elizabeth Bock, Peter Donhauser, Francois Tadel, and Sylvain Baillet for sharing the MRI dataset collected at the MEG Unit Lab, McConnell Brain Imaging Center, Montreal Neurological Institute, McGill University, Canada. All calculations have been performed on the Boyle cluster maintained by the Trinity Center for High-Performance Computing. The hardware was funded through grants from the European Research Council and Science Foundation Ireland. The calculations were accelerated on Nvidia Quadro RTX 8000 GPUs, provided by Nvidia under the Nvidia Academic Hardware Grant Program, which we kindly acknowledge. Microscopy characterization and analysis have been performed at the CRANN Advanced Microscopy Laboratory (AML www.tcd.ie/crann/aml/ ).
Author information
Authors and affiliations.
CRANN Institute and AMBER Centre, Trinity College Dublin, Dublin 2, Ireland
Laura Gambini, Cian Gabbett, Luke Doolan, Lewys Jones, Jonathan N. Coleman & Stefano Sanvito
School of Physics, Trinity College Dublin, Dublin 2, Ireland
Advanced Microscopy Laboratory, Trinity College Dublin, Dublin 2, Ireland
Lewys Jones
Mater Misericordiae University Hospital, Dublin 7, Ireland
Paddy Gilligan
You can also search for this author in PubMed Google Scholar
Contributions
S.S. and L.G. conceived the project and designed the AI workflow. L.G. performed all the numerical analysis and image processing. C.G. and L.D. provided support for the image binarisation procedure and the calculation of porosity, tortuosity, and intrinsic diffusivity. C.G., L.D., L.J., and J.N.C., designed and performed the FIB-SEM experiments and provided the relative data. P.G. provided support with the medical data analysis. S.S., J.N.C., and L.J. provided supervision. The manuscript was written by L.G. and S.S. with contributions from all the authors. Funding was secured by S.S.
Corresponding author
Correspondence to Laura Gambini .
Ethics declarations
Competing interests.
The authors declare no competing interests.
Peer review
Peer review information.
Nature Communications thanks Boxin Shi, Jessi van der Hoeven and the other anonymous reviewer(s) for their contribution to the peer review of this work. A peer review file is available.
Additional information
Publisher’s note Springer Nature remains neutral with regard to jurisdictional claims in published maps and institutional affiliations.
Supplementary information
Supplementary information, peer review file, reporting summary, source data, source data, rights and permissions.
Open Access This article is licensed under a Creative Commons Attribution-NonCommercial-NoDerivatives 4.0 International License, which permits any non-commercial use, sharing, distribution and reproduction in any medium or format, as long as you give appropriate credit to the original author(s) and the source, provide a link to the Creative Commons licence, and indicate if you modified the licensed material. You do not have permission under this licence to share adapted material derived from this article or parts of it. The images or other third party material in this article are included in the article’s Creative Commons licence, unless indicated otherwise in a credit line to the material. If material is not included in the article’s Creative Commons licence and your intended use is not permitted by statutory regulation or exceeds the permitted use, you will need to obtain permission directly from the copyright holder. To view a copy of this licence, visit http://creativecommons.org/licenses/by-nc-nd/4.0/ .
Reprints and permissions
About this article
Cite this article.
Gambini, L., Gabbett, C., Doolan, L. et al. Video frame interpolation neural network for 3D tomography across different length scales. Nat Commun 15 , 7962 (2024). https://doi.org/10.1038/s41467-024-52260-2
Download citation
Received : 11 September 2023
Accepted : 02 September 2024
Published : 11 September 2024
DOI : https://doi.org/10.1038/s41467-024-52260-2
Share this article
Anyone you share the following link with will be able to read this content:
Sorry, a shareable link is not currently available for this article.
Provided by the Springer Nature SharedIt content-sharing initiative
By submitting a comment you agree to abide by our Terms and Community Guidelines . If you find something abusive or that does not comply with our terms or guidelines please flag it as inappropriate.
Quick links
- Explore articles by subject
- Guide to authors
- Editorial policies
Sign up for the Nature Briefing newsletter — what matters in science, free to your inbox daily.

An editorially independent publication supported by the Simons Foundation.
Get the latest news delivered to your inbox.
Type search term(s) and press enter
- Comment Comments
- Save Article Read Later Read Later
Novel Architecture Makes Neural Networks More Understandable
September 11, 2024
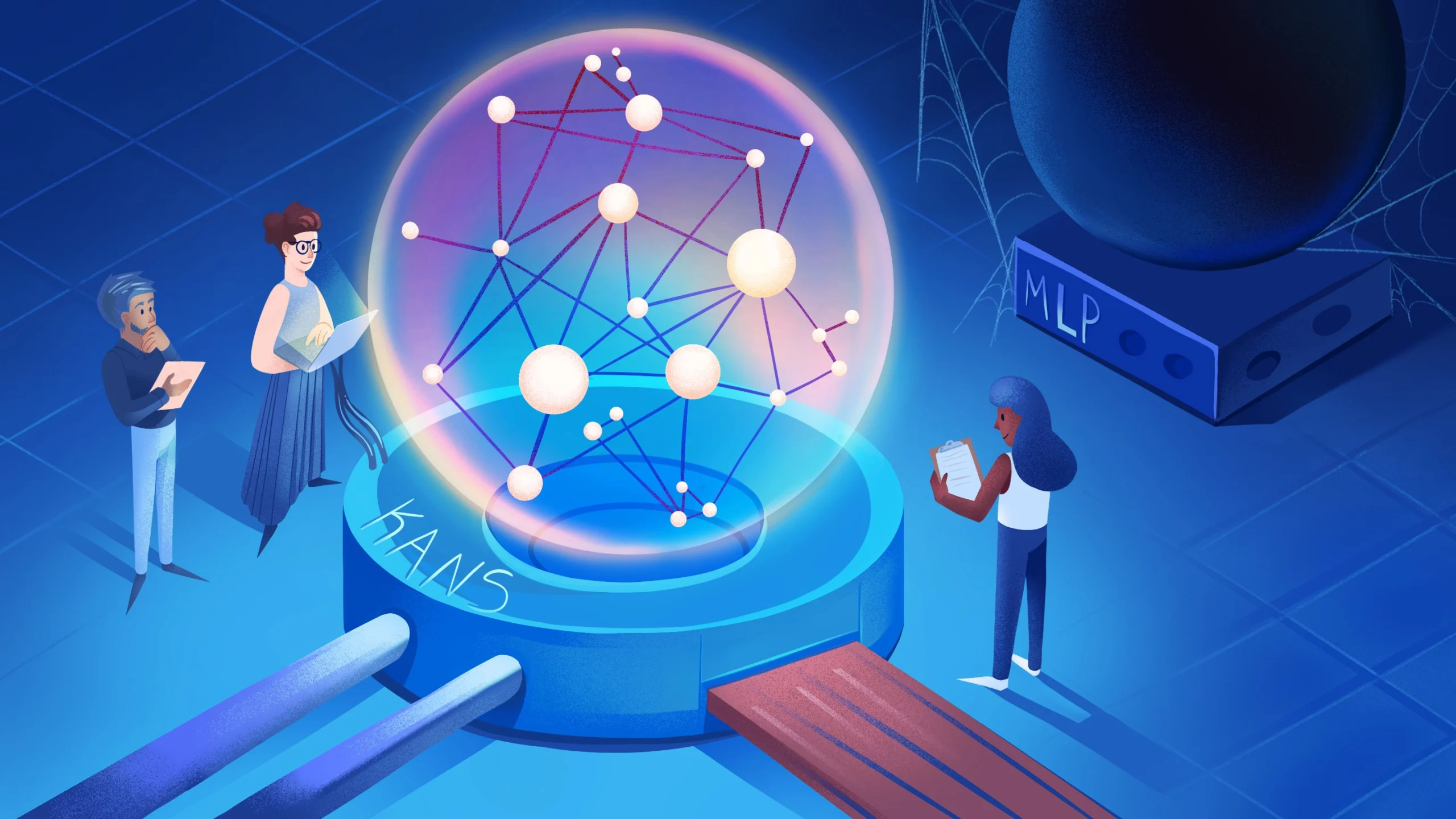
Nico Roper for Quanta Magazine
Introduction
“Neural networks are currently the most powerful tools in artificial intelligence,” said Sebastian Wetzel , a researcher at the Perimeter Institute for Theoretical Physics. “When we scale them up to larger data sets, nothing can compete.”
And yet, all this time, neural networks have had a disadvantage. The basic building block of many of today’s successful networks is known as a multilayer perceptron, or MLP. But despite a string of successes, humans just can’t understand how networks built on these MLPs arrive at their conclusions, or whether there may be some underlying principle that explains those results. The amazing feats that neural networks perform, like those of a magician, are kept secret, hidden behind what’s commonly called a black box.
AI researchers have long wondered if it’s possible for a different kind of network to deliver similarly reliable results in a more transparent way.
An April 2024 study introduced an alternative neural network design, called a Kolmogorov-Arnold network (KAN), that is more transparent yet can also do almost everything a regular neural network can for a certain class of problems. It’s based on a mathematical idea from the mid-20th century that has been rediscovered and reconfigured for deployment in the deep learning era.
Although this innovation is just a few months old, the new design has already attracted widespread interest within research and coding communities. “KANs are more interpretable and may be particularly useful for scientific applications where they can extract scientific rules from data,” said Alan Yuille , a computer scientist at Johns Hopkins University. “[They’re] an exciting, novel alternative to the ubiquitous MLPs.” And researchers are already learning to make the most of their newfound powers.
Fitting the Impossible
A typical neural network works like this: Layers of artificial neurons (or nodes) connect to each other using artificial synapses (or edges). Information passes through each layer, where it is processed and transmitted to the next layer, until it eventually becomes an output. The edges are weighted, so that those with greater weights have more influence than others. During a period known as training, these weights are continually tweaked to get the network’s output closer and closer to the right answer.
A common objective for neural networks is to find a mathematical function, or curve, that best connects certain data points. The closer the network can get to that function, the better its predictions and the more accurate its results. If your neural network models some physical process, the output function will ideally represent an equation describing the physics — the equivalent of a physical law.
For MLPs, there’s a mathematical theorem that tells you how close a network can get to the best possible function. One consequence of this theorem is that an MLP cannot perfectly represent that function.
But KANs, in the right circumstances, can.
KANs go about function fitting — connecting the dots of the network’s output — in a fundamentally different way than MLPs. Instead of relying on edges with numerical weights, KANs use functions. These edge functions are nonlinear, meaning they can represent more complicated curves. They’re also learnable, so they can be tweaked with far greater sensitivity than the simple numerical weights of MLPs.
Yet for the past 35 years, KANs were thought to be fundamentally impractical. A 1989 paper co-authored by Tomaso Poggio, a physicist turned computational neuroscientist at the Massachusetts Institute of Technology, explicitly stated that the mathematical idea at the heart of a KAN is “irrelevant in the context of networks for learning.”
One of Poggio’s concerns goes back to the mathematical concept at the heart of a KAN. In 1957, the mathematicians Andrey Kolmogorov and Vladimir Arnold showed — in separate though complementary papers — that if you have a single mathematical function that uses many variables, you can transform it into a combination of many functions that each have a single variable.
There’s an important catch, however. The single-variable functions the theorem spits out might not be “smooth,” meaning they can have sharp edges like the vertex of a V. That’s a problem for any network that tries to use the theorem to re-create the multivariable function. The simpler, single-variable pieces need to be smooth so that they can learn to bend the right way during training, in order to match the target values.
So KANs looked like a dim prospect — until a cold day this past January, when Ziming Liu , a physics graduate student at MIT, decided to revisit the subject. He and his adviser, the MIT physicist Max Tegmark , had been working on making neural networks more understandable for scientific applications — hoping to offer a peek inside the black box — but things weren’t panning out. In an act of desperation, Liu decided to look into the Kolmogorov-Arnold theorem. “Why not just try it and see how it works, even if people hadn’t given it much attention it in the past?” he asked.
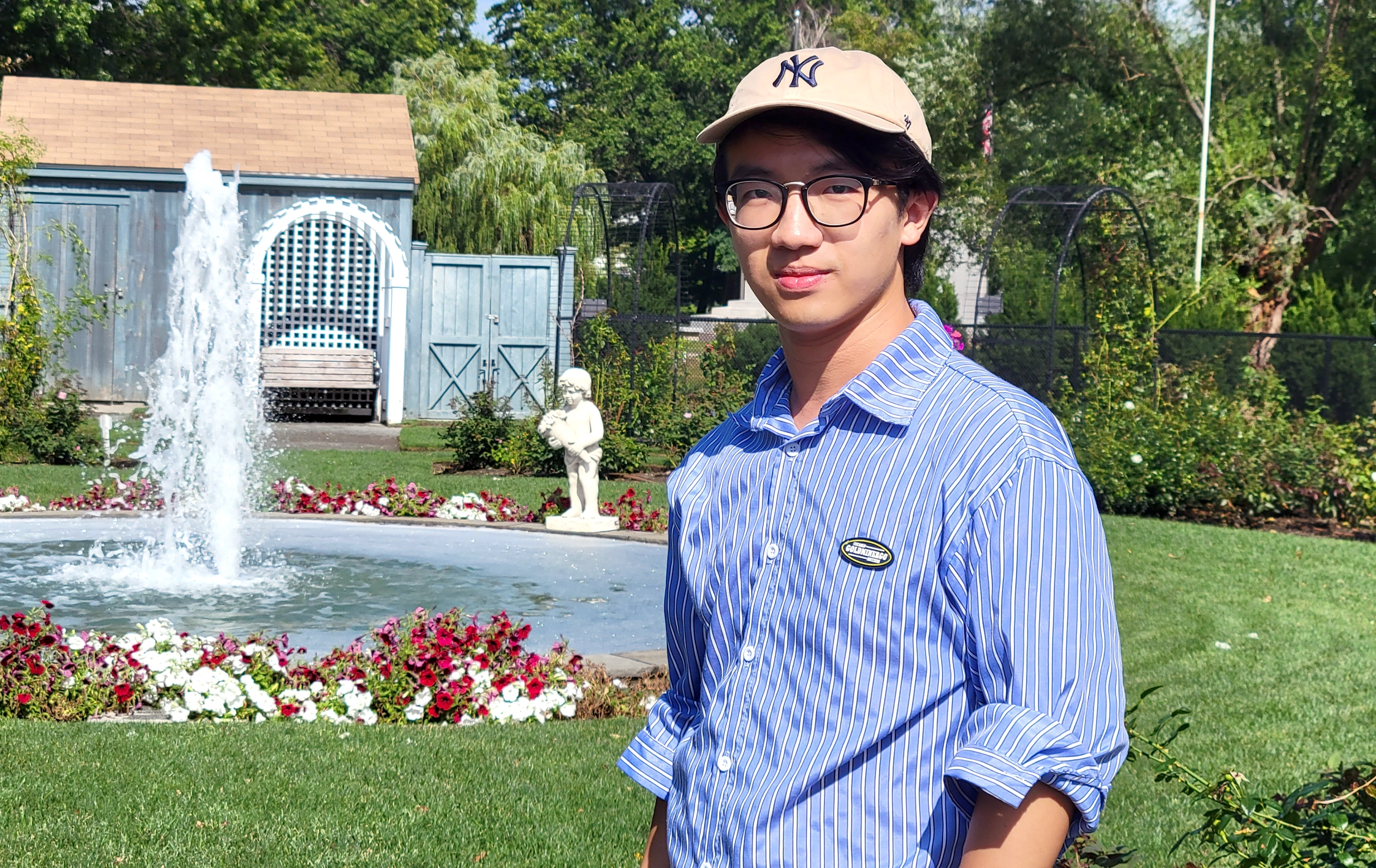
Ziming Liu used the Kolmogorov-Arnold theorem to build a new kind of neural network.
Wenting Gong
Tegmark was familiar with Poggio’s paper and thought the effort would lead to another dead end. But Liu was undeterred, and Tegmark soon came around. They recognized that even if the single-value functions generated by the theorem were not smooth, the network could still approximate them with smooth functions. They further understood that most of the functions we come across in science are smooth, which would make perfect (rather than approximate) representations potentially attainable. Liu didn’t want to abandon the idea without first giving it a try, knowing that software and hardware had advanced dramatically since Poggio’s paper came out 35 years ago. Many things are possible in 2024, computationally speaking, that were not even conceivable in 1989.
Liu worked on the idea for about a week, during which he developed some prototype KAN systems, all with two layers — the simplest possible networks, and the type researchers had focused on over the decades. Two-layer KANs seemed like the obvious choice because the Kolmogorov-Arnold theorem essentially provides a blueprint for such a structure. The theorem specifically breaks down the multivariable function into distinct sets of inner functions and outer functions. (These stand in for the activation functions along the edges that substitute for the weights in MLPs.) That arrangement lends itself naturally to a KAN structure with an inner and outer layer of neurons — a common arrangement for simple neural networks.
But to Liu’s dismay, none of his prototypes performed well on the science-related chores he had in mind. Tegmark then made a key suggestion: Why not try a KAN with more than two layers, which might be able to handle more sophisticated tasks?
That outside-the-box idea was the breakthrough they needed. Liu’s fledgling networks started showing promise, so the pair soon reached out to colleagues at MIT, the California Institute of Technology and Northeastern University. They wanted mathematicians on their team, plus experts in the areas they planned to have their KAN analyze.
In their April paper , the group showed that KANs with three layers were indeed possible, providing an example of a three-layer KAN that could exactly represent a function (whereas a two-layer KAN could not). And they didn’t stop there. The group has since experimented with up to six layers, and with each one, the network is able to align with a more complicated output function. “We found that we could stack as many layers as we want, essentially,” said Yixuan Wang , one of the co-authors.
Proven Improvements
The authors also turned their networks loose on two real-world problems. The first relates to a branch of mathematics called knot theory . In 2021, a team from DeepMind announced they’d built an MLP that could predict a certain topological property for a given knot after being fed enough of the knot’s other properties. Three years later, the new KAN duplicated that feat. Then it went further and showed how the predicted property was related to all the others — something, Liu said, that “MLPs can’t do at all.”
The second problem involves a phenomenon in condensed matter physics called Anderson localization. The goal was to predict the boundary at which a particular phase transition will occur, and then to determine the mathematical formula that describes that process. No MLP has ever been able to do this. Their KAN did.
But the biggest advantage that KANs hold over other forms of neural networks, and the principal motivation behind their recent development, Tegmark said, lies in their interpretability. In both of those examples, the KAN didn’t just spit out an answer; it provided an explanation. “What does it mean for something to be interpretable?” he asked. “If you give me some data, I will give you a formula you can write down on a T-shirt.”
The ability of KANs to do this, limited though it’s been so far, suggests that these networks could theoretically teach us something new about the world, said Brice Ménard , a physicist at Johns Hopkins who studies machine learning. “If the problem is actually described by a simple equation, the KAN network is pretty good at finding it,” he said. But he cautioned that the domain in which KANs work best is likely to be restricted to problems — such as those found in physics — where the equations tend to have very few variables.
Liu and Tegmark agree, but don’t see it as a drawback. “Almost all of the famous scientific formulas” — such as E = mc 2 — “can be written in terms of functions of one or two variables,” Tegmark said. “The vast majority of calculations we do depend on one or two variables. KANs exploit that fact and look for solutions of that form.”
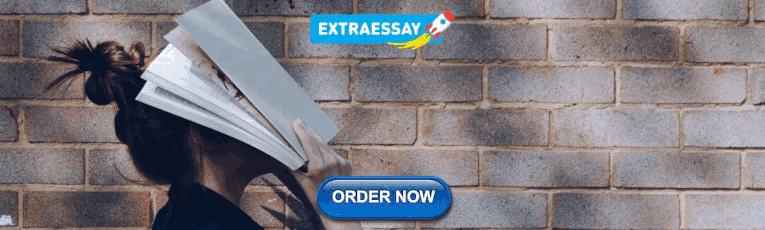
The Ultimate Equations
Liu and Tegmark’s KAN paper quickly caused a stir, garnering 75 citations within about three months. Soon other groups were working on their own KANs. A paper by Yizheng Wang of Tsinghua University and others that appeared online in June showed that their Kolmogorov-Arnold-informed neural network (KINN) “significantly outperforms” MLPs for solving partial differential equations (PDEs). That’s no small matter, Wang said: “PDEs are everywhere in science.”
A July paper by researchers at the National University of Singapore was more mixed. They concluded that KANs outperformed MLPs in tasks related to interpretability, but found that MLPs did better with computer vision and audio processing. The two networks were roughly equal at natural language processing and other machine learning tasks. For Liu, those results were not surprising, given that the original KAN group’s focus has always been on “science-related tasks,” where interpretability is the top priority.
Meanwhile, Liu is striving to make KANs more practical and easier to use. In August, he and his collaborators posted a new paper called “KAN 2.0,” which he described as “more like a user manual than a conventional paper.” This version is more user-friendly, Liu said, offering a tool for multiplication, among other features, that was lacking in the original model.
This type of network, he and his co-authors maintain, represents more than just a means to an end. KANs foster what the group calls “curiosity-driven science,” which complements the “application-driven science” that has long dominated machine learning. When observing the motion of celestial bodies, for example, application-driven researchers focus on predicting their future states, whereas curiosity-driven researchers hope to uncover the physics behind the motion. Through KANs, Liu hopes, researchers could get more out of neural networks than just help on an otherwise daunting computational problem. They might focus instead on simply gaining understanding for its own sake.
Get highlights of the most important news delivered to your email inbox
Also in Computer Science
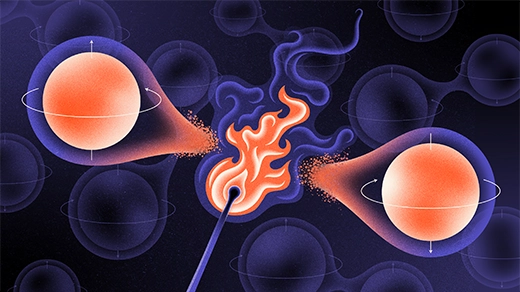
Computer Scientists Prove That Heat Destroys Quantum Entanglement
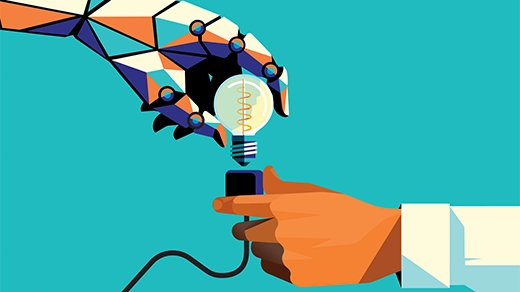
Are Robots About to Level Up?
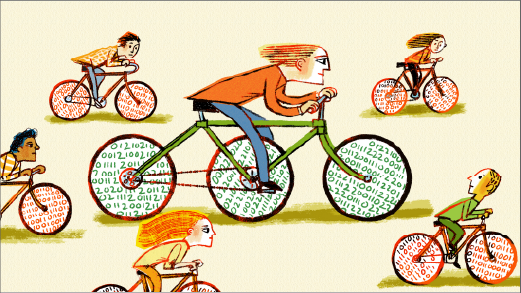
How Base 3 Computing Beats Binary
Comment on this article.
Quanta Magazine moderates comments to facilitate an informed, substantive, civil conversation. Abusive, profane, self-promotional, misleading, incoherent or off-topic comments will be rejected. Moderators are staffed during regular business hours (New York time) and can only accept comments written in English.
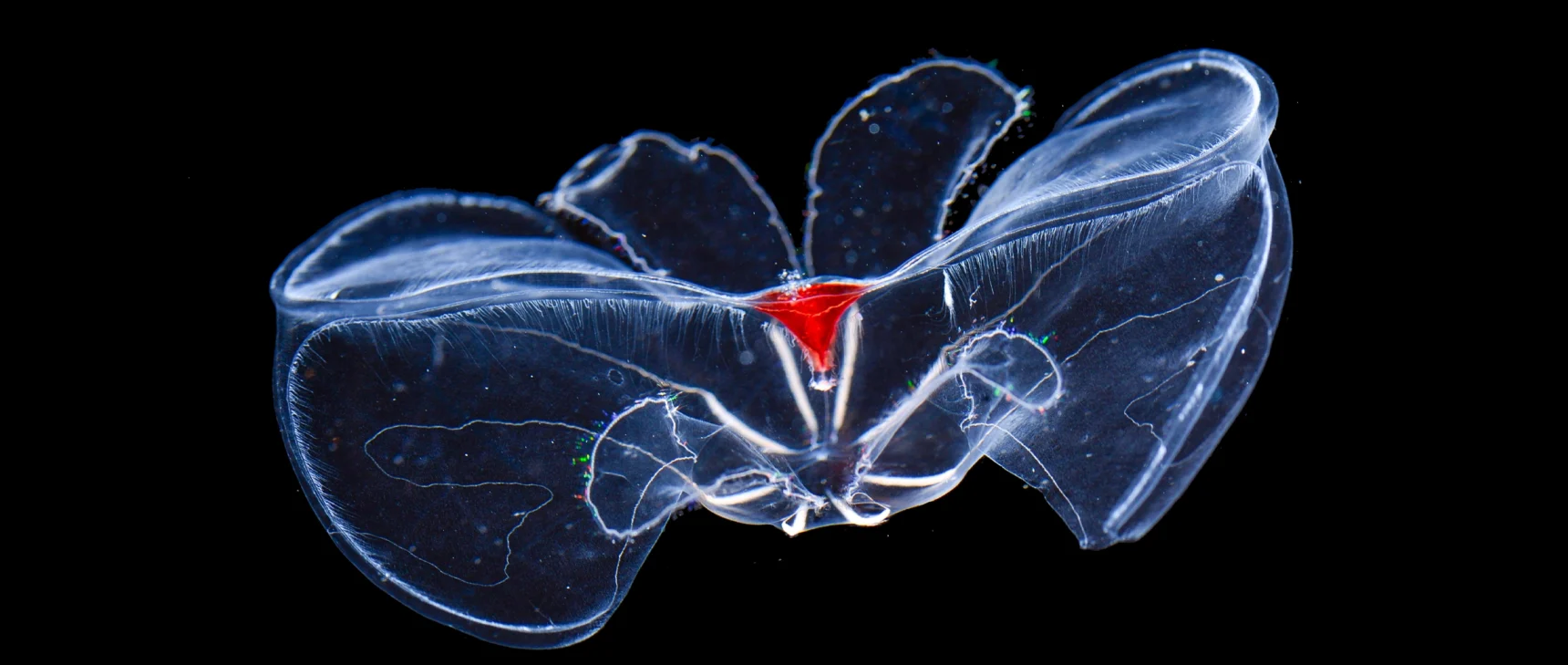
Next article
Use your social network.
Forgot your password ?
We’ll email you instructions to reset your password
Enter your new password
- Corpus ID: 272599965
All-optical Fourier neural network using partially coherent light
- Jianwei Qin , Yanbing Liu , +3 authors Fangwei Ye
- Published 12 September 2024
- Physics, Engineering
Figures from this paper
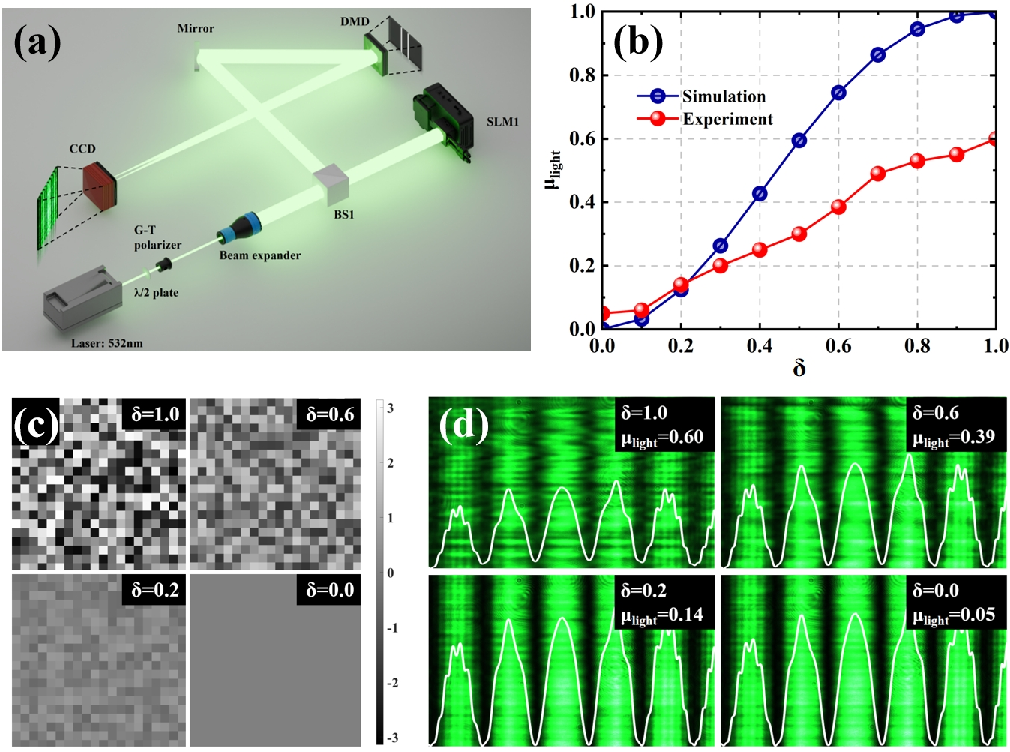
33 References
Partial coherence enhances parallelized photonic computing, multimodal deep learning using on-chip diffractive optics with in situ training capability, fundamentals and recent developments of free-space optical neural networks, memory-less scattering imaging with ultrafast convolutional optical neural networks, towards constructing a doe-based practical optical neural system for ship recognition in remote sensing images, role of spatial coherence in diffractive optical neural networks, super-resolution orbital angular momentum holography, universal linear intensity transformations using spatially incoherent diffractive processors, zero-power optical convolutional neural network using incoherent light, image sensing with multilayer nonlinear optical neural networks, related papers.
Showing 1 through 3 of 0 Related Papers
Artificial neural networks and their application to sequence recognition
Downloadable content.
- Bengio, Yoshua
- This thesis studies the introduction of a priori structure into the design of learning systems based on artificial neural networks applied to sequence recognition, in particular to phoneme recognition in continuous speech. Because we are interested in sequence analysis, algorithms for training recurrent networks are studied and an original algorithm for constrained recurrent networks is proposed and test results are reported. We also discuss the integration of connectionist models with other analysis tools that have been shown to be useful for sequences, such as dynamic programming and hidden Markov models. We introduce an original algorithm to perform global optimization of a neural network/hidden Markov model hybrid, and show how to perform such a global optimization on all the parameters of the system. Finally, we consider some alternatives to sigmoid networks: Radial Basis Functions, and a method for searching for better learning rules using a priori knowledge and optimization algorithms.
- Computer Science.
- Artificial Intelligence.
- McGill University
- https://escholarship.mcgill.ca/concern/theses/qv33rx48q
- All items in eScholarship@McGill are protected by copyright with all rights reserved unless otherwise indicated.
- School of Computer Science
- Doctor of Philosophy
- Theses & Dissertations
Thumbnail | Title | Date Uploaded | Visibility | Actions |
---|---|---|---|---|
2019-09-28 | Public |
Information
- Author Services
Initiatives
You are accessing a machine-readable page. In order to be human-readable, please install an RSS reader.
All articles published by MDPI are made immediately available worldwide under an open access license. No special permission is required to reuse all or part of the article published by MDPI, including figures and tables. For articles published under an open access Creative Common CC BY license, any part of the article may be reused without permission provided that the original article is clearly cited. For more information, please refer to https://www.mdpi.com/openaccess .
Feature papers represent the most advanced research with significant potential for high impact in the field. A Feature Paper should be a substantial original Article that involves several techniques or approaches, provides an outlook for future research directions and describes possible research applications.
Feature papers are submitted upon individual invitation or recommendation by the scientific editors and must receive positive feedback from the reviewers.
Editor’s Choice articles are based on recommendations by the scientific editors of MDPI journals from around the world. Editors select a small number of articles recently published in the journal that they believe will be particularly interesting to readers, or important in the respective research area. The aim is to provide a snapshot of some of the most exciting work published in the various research areas of the journal.
Original Submission Date Received: .
- Active Journals
- Find a Journal
- Proceedings Series
- For Authors
- For Reviewers
- For Editors
- For Librarians
- For Publishers
- For Societies
- For Conference Organizers
- Open Access Policy
- Institutional Open Access Program
- Special Issues Guidelines
- Editorial Process
- Research and Publication Ethics
- Article Processing Charges
- Testimonials
- Preprints.org
- SciProfiles
- Encyclopedia
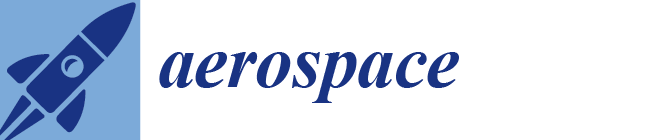
Article Menu

- Subscribe SciFeed
- Recommended Articles
- Google Scholar
- on Google Scholar
- Table of Contents
Find support for a specific problem in the support section of our website.
Please let us know what you think of our products and services.
Visit our dedicated information section to learn more about MDPI.
JSmol Viewer
A neural network with physical mechanism for predicting airport aviation noise.
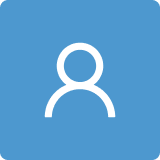
1. Introduction
2. methodology, 2.1. ecac model.
- The propagation of aviation noise remains isotropic.
- The aircraft engine power, climb or descent angle, flap settings, and other flight states remain unchanged in each segmented track, i.e., the flight state is updated once per track segment.
- The ground is a constant single medium with negligible noise absorption.
2.2. Neural Networks
2.2.1. input features.
- Flight trajectory
- Slant distance d and azimuth angle ω . Aircraft position relative to a ground observer.
- Ground-speed v and heading of the aircraft θ .
- Arrival or departure. Indication of the operation type of the flight
- Airport temperature, humidity, and atmospheric pressure at the nearest 1 min time
- Wind speed and direction at the nearest 2 min time
- Engine thrust and aircraft weight constructed using ECAC model
2.2.2. Neural Network
2.3. implementation.
ECAC-based single-event noise prediction algorithms |
Input: 1. Standardized trajectory , includes monitoring time, longitude, latitude, altitude, ground speed, flight number and heading 2. Meteorological feature datasets includes temperature, atmospheric pressure, relative humidity, wind speed and direction 3. ANP Database Process: 1. Determine the coordinates of ground monitoring observation points 2. Match( ) // Match NPD curves 3. // Update to correct the NPD curve 4. for i 1 to do ; // Calculate engine thrust ; // Calculate aircraft weight ; // Calculate Sound Exposure Level using ECAC end for Output: ECAC model-calculated single-event noise sound exposure levels |
Implementation steps of DNN algorithm |
Input: 1. Standardized trajectory , includes monitoring time, longitude, latitude, altitude, ground speed, flight number and heading 2. Meteorological feature datasets includes temperature, atmospheric pressure, relative humidity, wind speed and direction 3. Number of hidden layers 4. Number of neurons in each hidden layer Loss function construction: The loss function of the DNN is to calculate the mean square error between the output value of the model and the true value Output: DNN model-calculated single-event noise sound exposure levels |
Implementation steps of HPNN algorithm |
Input: 1. Standardized trajectory , includes monitoring time, longitude, latitude, altitude, ground speed, flight number and heading 2. Meteorological feature datasets includes temperature, atmospheric pressure, relative humidity, wind speed and direction 3. Number of hidden layers 4. Number of neurons in each hidden layer 5. ECAC model-calculated single-event noise sound exposure levels Loss function construction: The loss function of HPNN is the same as that of DNN, which calculates the mean square error between the model output value and the true value . Output: HPNN model-calculated single-event noise sound exposure levels |
Implementation steps of PGNN algorithm |
Input: 1. Standardized trajectory , includes monitoring time, longitude, latitude, altitude, ground speed, flight number and heading 2. Meteorological feature datasets includes temperature, atmospheric pressure, relative humidity, wind speed and direction 3. Number of hidden layers 4. Number of neurons in each hidden layer 5. ECAC model-calculated single-event noise sound exposure levels 6. Physical constraint weight Loss function construction: Add physical condition constraints to the loss function of the original HPNN model to guide the model to optimize in the direction of physical consistency and obtain the loss function . Output: PGNN model-calculated single-event noise sound exposure levels |
The training process of DNN, HPNN, and PGNN |
1. Back propagation optimization is performed with the goal of minimizing the loss function construction. The Adam optimizer is used to update the model weight parameters and continuously adjust the parameters to optimize the model. By using a K-fold cross-validation method (specifically set to k = 5), we iteratively train the model using the training set data. In each round of cross-validation, the model is trained on a training subset and evaluated on a validation subset. 2. If the error metrics obtained after cross-validation meet the expected performance criteria, this indicates that the model training is complete and meets the predefined accuracy requirements. Conversely, if the error does not meet expectations, we will repeat the training and optimization steps of the model until we obtain results that satisfy the desired training model performance. |
3. Validation
3.1. data description, 3.2. evaluation metrics, 3.3. model training and experimental design.
- Physically driven ECAC model;
- DNN neural network model, which uses trajectory and meteorological features as inputs and monitored noise information as labels;
- HPNN model, which adds ECAC physical output characteristics as input to the DNN model;
- PGNN model, which incorporates physical laws into a modified loss function based on the HPNN framework to guide the entire training process.
3.4. Analysis
4. conclusions, author contributions, data availability statement, acknowledgments, conflicts of interest.
- Jackson, D.C.; Rindfleisch, T.C.; Alonso, J.J. A system for measurement and analysis of aircraft noise impacts. Eng. Proc. 2021 , 13 , 6. [ Google Scholar ] [ CrossRef ]
- Pretto, M.; Giannattasio, P.; De Gennaro, M.; Zanon, A.; Kühnelt, H. Web data for computing real-world noise from civil aviation. Transp. Res. Part D Transp. Environ. 2019 , 69 , 224–249. [ Google Scholar ] [ CrossRef ]
- Pretto, M.; Giannattasio, P.; De Gennaro, M.; Zanon, A.; Kuehnelt, H. Forecasts of future scenarios for airport noise based on collection and processing of web data. Eur. Transp. Res. Rev. 2020 , 12 , 4. [ Google Scholar ] [ CrossRef ]
- Feng, H.; Zhou, Y.; Zeng, W.; Ding, C. Review on metrics and prediction methods of civil aviation noise. Int. J. Aeronaut. Space Sci. 2023 , 24 , 1199–1213. [ Google Scholar ] [ CrossRef ]
- Gao, Z.; Mavris, D.N. Statistics and machine learning in aviation environmental impact analysis: A survey of recent progress. Aerospace 2022 , 9 , 750. [ Google Scholar ] [ CrossRef ]
- Pretto, M.; Giannattasio, P.; De Gennaro, M. Mixed analysis-synthesis approach for estimating airport noise from civil air traffic. Transp. Res. Part D Transp. Environ. 2022 , 106 , 103248. [ Google Scholar ] [ CrossRef ]
- Bertsch, L.; Sanders, L.; Thomas, R.H.; LeGriffon, I.; June, J.C.; Clark, I.A.; Lorteau, M. Comparative assessment of aircraft system noise simulation tools. J. Aircr. 2021 , 58 , 867–884. [ Google Scholar ] [ CrossRef ]
- Revoredo, T.; Mora-Camino, F.; Slama, J. A two-step approach for the prediction of dynamic aircraft noise impact. Aerosp. Sci. Technol. 2016 , 59 , 122–131. [ Google Scholar ] [ CrossRef ]
- Lukić, M.; Gavran, D.; Fric, S.; Ilić, V.; Vranjevac, S.; Trpčevski, F. Prediction of aircraft noise using machine learning. In Proceedings of the 27th International Congress on Sound and Vibration, Online, 11–16 July 2021. [ Google Scholar ]
- Barron, A.R. Universal approximation bounds for superpositions of a sigmoidal function. IEEE Trans. Inf. Theory 1993 , 39 , 930–945. [ Google Scholar ] [ CrossRef ]
- Feng, H.; Zhou, Y.; Ding, C.; Zeng, W.; Guo, W. Aircraft Noise Prediction Based on Machine Learning Model. Trans. Nanjing Univ. Aeronaut. Astronaut. 2023 , 40 , 54–61. [ Google Scholar ]
- Vabalas, A.; Gowen, E.; Poliakoff, E.; Casson, A.J. Machine learning algorithm validation with a limited sample size. PLoS ONE 2019 , 14 , e0224365. [ Google Scholar ] [ CrossRef ] [ PubMed ]
- Jia, X.; Willard, J.; Karpatne, A.; Read, J.S.; Zwart, J.A.; Steinbach, M.; Kumar, V. Physics-guided machine learning for scientific discovery: An application in simulating lake temperature profiles. ACM/IMS Trans. Data Sci. 2021 , 2 , 1–26. [ Google Scholar ] [ CrossRef ]
- Zhou, H.; Qiu, Y.; Feng, Y.; Liu, J. Power prediction of wind turbine in the wake using hybrid physical process and machine learning models. Renew. Energy 2022 , 198 , 568–586. [ Google Scholar ] [ CrossRef ]
- June, J.C.; Thomas, R.H.; Guo, Y. System Noise Prediction Uncertainty Quantification for a Hybrid Wing–Body Transport Concept. AIAA J. 2020 , 58 , 1157–1170. [ Google Scholar ] [ CrossRef ]
- Gabrielian, A.B.; Puranik, T.G.; Bendarkar, M.V.; Kirby, M.; Mavris, D.; Monteiro, D. Noise model validation using real world operations data. In Proceedings of the 2021 AIAA Aviation and Aeronautics Forum and Exposition, Online, 2–6 August 2021; p. 2136. [ Google Scholar ]
- Simons, D.G.; Besnea, I.; Mohammadloo, T.H.; Melkert, J.A.; Snellen, M. Comparative assessment of measured and modelled aircraft noise around Amsterdam Airport Schiphol. Transp. Res. Part D Transp. Environ. 2022 , 105 , 103216. [ Google Scholar ] [ CrossRef ]
- Thomas, R.H.; Burley, C.L.; Guo, Y. Progress of aircraft system noise assessment with uncertainty quantification for the environmentally responsible aviation project. In Proceedings of the 22nd AIAA/CEAS Aeroacoustics Conference, Lyon, France, 30 May 2016; p. 3040. [ Google Scholar ]
- von Rueden, L.; Mayer, S.; Sifa, R.; Bauckhage, C.; Garcke, J. Combining machine learning and simulation to a hybrid modelling approach: Current and future directions. In Proceedings of the Advances in Intelligent Data Analysis XVIII: 18th International Symposium on Intelligent Data Analysis, IDA 2020, Konstanz, Germany, 27–29 April 2020; Proceedings 18. pp. 548–560. [ Google Scholar ]
- Jurj, S.L.; Grundt, D.; Werner, T.; Borchers, P.; Rothemann, K.; Möhlmann, E. Increasing the safety of adaptive cruise control using physics-guided reinforcement learning. Energies 2021 , 14 , 7572. [ Google Scholar ] [ CrossRef ]
- Monaco, S.; Apiletti, D.; Malnati, G. Theory-guided deep learning algorithms: An experimental evaluation. Electronics 2022 , 11 , 2850. [ Google Scholar ] [ CrossRef ]
- Murphy, C.P.; Kerekes, J.P. Physics-guided neural network for predicting chemical signatures. Appl. Opt. 2021 , 60 , 3176–3181. [ Google Scholar ] [ CrossRef ]
- Changdar, S.; Bhaumik, B.; De, S. Physics-based smart model for prediction of viscosity of nanofluids containing nanoparticles using deep learning. J. Comput. Des. Eng. 2021 , 8 , 600–614. [ Google Scholar ] [ CrossRef ]
- Karpatne, A.; Atluri, G.; Faghmous, J.H.; Steinbach, M.; Banerjee, A.; Ganguly, A.; Shekhar, S.; Samatova, N.; Kumar, V. Theory-guided data science: A new paradigm for scientific discovery from data. IEEE Trans. Knowl. Data Eng. 2017 , 29 , 2318–2331. [ Google Scholar ] [ CrossRef ]
- Uzun, M.; Demirezen, M.U.; Inalhan, G. Physics guided deep learning for data-driven aircraft fuel consumption modeling. Aerospace 2021 , 8 , 44. [ Google Scholar ] [ CrossRef ]
- Bode, M.; Gauding, M.; Lian, Z.; Denker, D.; Davidovic, M.; Kleinheinz, K.; Jitsev, J.; Pitsch, H. Using physics-informed enhanced super-resolution generative adversarial networks for subfilter modeling in turbulent reactive flows. Proc. Combust. Inst. 2021 , 38 , 2617–2625. [ Google Scholar ] [ CrossRef ]
- Reichstein, M.; Camps-Valls, G.; Stevens, B.; Jung, M.; Denzler, J.; Carvalhais, N.; Prabhat, F. Deep learning and process understanding for data-driven Earth system science. Nature 2019 , 566 , 195–204. [ Google Scholar ] [ CrossRef ]
- Cang, R.; Li, H.; Yao, H.; Jiao, Y.; Ren, Y. Improving direct physical properties prediction of heterogeneous materials from imaging data via convolutional neural network and a morphology-aware generative model. Comput. Mater. Sci. 2018 , 150 , 212–221. [ Google Scholar ] [ CrossRef ]
- Sadowski, P.; Fooshee, D.; Subrahmanya, N.; Baldi, P. Synergies between quantum mechanics and machine learning in reaction prediction. J. Chem. Inf. Model. 2016 , 56 , 2125–2128. [ Google Scholar ] [ CrossRef ]
- Yazdani, A.; Lu, L.; Raissi, M.; Karniadakis, G.E. Systems biology informed deep learning for inferring parameters and hidden dynamics. PLoS Comput. Biol. 2020 , 16 , e1007575. [ Google Scholar ] [ CrossRef ]
- Bernardo, J.E.; Kirby, M.; Mavris, D. Development of a rapid fleet-level noise computation model. J. Aircr. 2015 , 52 , 721–733. [ Google Scholar ] [ CrossRef ]
- Kim, S.; Lim, D.; Lee, K. Reduced-order modeling applied to the aviation environmental design tool for rapid noise prediction. J. Aerosp. Eng. 2018 , 31 , 04018056. [ Google Scholar ] [ CrossRef ]
- Ecac, E. CEAC DOC. 29: Report on standard method of computing noise contours around civil airports, volume 2: Technical guide. In Proceedings of the European Civil Aviation Conference (ECAC), Neuilly-sur-Seine, France, 3 September 2016. [ Google Scholar ]
- Zaporozhets, O.; Levchenko, L. Accuracy of noise-power-distance definition on results of single aircraft noise event calculation. Aerospace 2021 , 8 , 121. [ Google Scholar ] [ CrossRef ]
- International Civil Aviation Organization. Recommended Method for Computing Noise Contours Around Airports , 2nd ed.; International Civil Aviation Organization: Montreal, QC, Canada, 2018. [ Google Scholar ]
- Torija, A.J.; Self, R.H. Aircraft classification for efficient modelling of environmental noise impact of aviation. J. Air Transp. Manag. 2018 , 67 , 157–168. [ Google Scholar ] [ CrossRef ]
- Synodinos, A.P.; Self, R.H.; Torija, A.J. Framework for predicting noise–power–distance curves for novel aircraft designs. J. Aircr. 2018 , 55 , 781–791. [ Google Scholar ] [ CrossRef ]
- Boeker, E.R.; Dinges, E.; He, B.; Fleming, G.; Roof, C.J.; Gerbi, P.J.; Rapoza, A.S.; Hermann, J. Integrated Noise Model (INM) Version 7.0 Technical Manual ; Federal Aviation Administration; Office of Environment and Energy: Fairfax County, VA, USA, 2008.
- Lee, C.B.E.; Downs, R.; Gorshkov, S.; Hansen, A.; Koopmann, J.; Thrasher, T. Aviation Environmental Design Tool (AEDT) Version 3e Technical Manual ; DOT-VNTSC-FAA-22-04; Federal Aviation Administration; Office of Environment and Energy: Fairfax County, VA, USA, 2022.
- Berton, J.J. Aircraft Noise and Performance Data for a Notional Supersonic Business Jet. In Proceedings of the 28th AIAA/CEAS Aeroacoustics 2022 Conference, Southampton, UK, 14–17 June 2022; p. 3078. [ Google Scholar ]
- Thomas, J.L.; Hansman, R.J. Modeling, assessment, and flight demonstration of delayed deceleration approaches for community noise reduction. In Proceedings of the 2020 AIAA Aviation and Aeronautics Forum and Exposition, Online, 15–19 June 2020; p. 2874. [ Google Scholar ]
- Rodríguez-Díaz, A.; Adenso-Díaz, B.; González-Torre, P.L. A review of the impact of noise restrictions at airports. Transp. Res. Part D Transp. Environ. 2017 , 50 , 144–153. [ Google Scholar ] [ CrossRef ]
- Berton, J.J. Simultaneous use of ground reflection and lateral attenuation noise models. J. Aircr. 2022 , 59 , 536–543. [ Google Scholar ] [ CrossRef ]
- Tenney, A.S.; Glauser, M.N.; Lewalle, J. A deep learning approach to jet noise prediction. In Proceedings of the 2018 AIAA Aerospace Sciences Meeting, Kissimmee, FL, USA, 8–12 January 2018; p. 1736. [ Google Scholar ]
- Zellmann, C.; Schäffer, B.; Wunderli, J.-M.; Isermann, U.; Paschereit, C. Aircraft noise emission model accounting for aircraft flight parameters. J. Aircr. 2018 , 55 , 682–695. [ Google Scholar ] [ CrossRef ]
- Vela, A.E.; Oleyaei-Motlagh, Y. Ground level aviation noise prediction: A sequence to sequence modeling approach using LSTM recurrent neural networks. In Proceedings of the 2020 AIAA/IEEE 39th Digital Avionics Systems Conference (DASC), Online, 11–15 October 2020; pp. 1–8. [ Google Scholar ]
- Campos, L.M. On physical aeroacoustics with some implications for low-noise aircraft design and airport operations. Aerospace 2015 , 2 , 17–90. [ Google Scholar ] [ CrossRef ]
- Isermann, U.; Bertsch, L. Aircraft noise immission modeling. CEAS Aeronaut. J. 2019 , 10 , 287–311. [ Google Scholar ] [ CrossRef ]
- Gagliardi, P.; Teti, L.; Licitra, G. A statistical evaluation on flight operational characteristics affecting aircraft noise during take-off. Appl. Acoust. 2018 , 134 , 8–15. [ Google Scholar ] [ CrossRef ]
- Torija, A.J.; Self, R.H.; Flindell, I.H. Airport noise modelling for strategic environmental impact assessment of aviation. Appl. Acoust. 2018 , 132 , 49–57. [ Google Scholar ] [ CrossRef ]
- Chen, T.; Chen, H. Universal approximation to nonlinear operators by neural networks with arbitrary activation functions and its application to dynamical systems. IEEE Trans. Neural Netw. 1995 , 6 , 911–917. [ Google Scholar ] [ CrossRef ]
- Rumelhart, D.E.; Hinton, G.E.; Williams, R.J. Learning representations by back-propagating errors. Nature 1986 , 323 , 533–536. [ Google Scholar ] [ CrossRef ]
Click here to enlarge figure
Stage Length | Great Circle Distance (NM) | Weight (lb) |
---|---|---|
1 | 0–500 | 429,900 |
2 | 500–1000 | 442,400 |
3 | 1000–1500 | 456,100 |
4 | 1500–2500 | 483,100 |
5 | 2500–3500 | 516,400 |
6 | 3500–4500 | 551,700 |
7 | 4500–5500 | 589,400 |
8 | 5500–6500 | 629,500 |
9 | 6500+ | 656,000 |
Attribute Name | Attribute Meaning |
---|---|
Monitoring time | The point in time at which aircraft trajectory information is collected |
Longitude | The longitude of the aircraft in a spherical space coordinate system |
Latitude | The latitude of the aircraft in the spatial spherical coordinate system |
Altitude | The barometric altitude of the aircraft |
Ground speed | The projected ground speed of the aircraft |
Flight number | Exclusive number of the flight |
Heading | The direction of travel of the aircraft |
Model. | Hidden Layers | Neurons | η |
---|---|---|---|
DNN | 3 | [64, 64, 32] | — |
HPNN | 3 | [128, 64, 32] | — |
PGNN | 3 | [128, 64, 32] | 0.31 |
Model | MAE | MAPE | RMSE |
---|---|---|---|
ECAC | 2.24 | 2.39% | 3.11 |
DNN | 1.26 | 1.54% | 1.57 |
HPNN | 1.03 | 1.23% | 1.33 |
PGNN | 0.98 | 1.17% | 1.27 |
The statements, opinions and data contained in all publications are solely those of the individual author(s) and contributor(s) and not of MDPI and/or the editor(s). MDPI and/or the editor(s) disclaim responsibility for any injury to people or property resulting from any ideas, methods, instructions or products referred to in the content. |
Share and Cite
Zhu, D.; Peng, J.; Ding, C. A Neural Network with Physical Mechanism for Predicting Airport Aviation Noise. Aerospace 2024 , 11 , 747. https://doi.org/10.3390/aerospace11090747
Zhu D, Peng J, Ding C. A Neural Network with Physical Mechanism for Predicting Airport Aviation Noise. Aerospace . 2024; 11(9):747. https://doi.org/10.3390/aerospace11090747
Zhu, Dan, Jiayu Peng, and Cong Ding. 2024. "A Neural Network with Physical Mechanism for Predicting Airport Aviation Noise" Aerospace 11, no. 9: 747. https://doi.org/10.3390/aerospace11090747
Article Metrics
Article access statistics, further information, mdpi initiatives, follow mdpi.
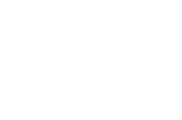
Subscribe to receive issue release notifications and newsletters from MDPI journals
Help | Advanced Search
Computer Science > Computer Vision and Pattern Recognition
Title: master's thesis : deep learning for visual recognition.
Abstract: The goal of our research is to develop methods advancing automatic visual recognition. In order to predict the unique or multiple labels associated to an image, we study different kind of Deep Neural Networks architectures and methods for supervised features learning. We first draw up a state-of-the-art review of the Convolutional Neural Networks aiming to understand the history behind this family of statistical models, the limit of modern architectures and the novel techniques currently used to train deep CNNs. The originality of our work lies in our approach focusing on tasks with a low amount of data. We introduce different models and techniques to achieve the best accuracy on several kind of datasets, such as a medium dataset of food recipes (100k images) for building a web API, or a small dataset of satellite images (6,000) for the DSG online challenge that we've won. We also draw up the state-of-the-art in Weakly Supervised Learning, introducing different kind of CNNs able to localize regions of interest. Our last contribution is a framework, build on top of Torch7, for training and testing deep models on any visual recognition tasks and on datasets of any scale.
Subjects: | Computer Vision and Pattern Recognition (cs.CV) |
Cite as: | [cs.CV] |
(or [cs.CV] for this version) | |
Focus to learn more arXiv-issued DOI via DataCite |
Submission history
Access paper:.
- Other Formats
References & Citations
- Google Scholar
- Semantic Scholar
DBLP - CS Bibliography
Bibtex formatted citation.

Bibliographic and Citation Tools
Code, data and media associated with this article, recommenders and search tools.
- Institution
arXivLabs: experimental projects with community collaborators
arXivLabs is a framework that allows collaborators to develop and share new arXiv features directly on our website.
Both individuals and organizations that work with arXivLabs have embraced and accepted our values of openness, community, excellence, and user data privacy. arXiv is committed to these values and only works with partners that adhere to them.
Have an idea for a project that will add value for arXiv's community? Learn more about arXivLabs .
Subscribe to the PwC Newsletter
Join the community, edit social preview.

Add a new code entry for this paper
Remove a code repository from this paper, mark the official implementation from paper authors, add a new evaluation result row.
TASK | DATASET | MODEL | METRIC NAME | METRIC VALUE | GLOBAL RANK | REMOVE |
---|
Remove a task
Add a method, remove a method, edit datasets, transformed physics-informed neural networks for the convection-diffusion equation.
12 Sep 2024 · Jiajing Guan , Howard Elman · Edit social preview
Singularly perturbed problems are known to have solutions with steep boundary layers that are hard to resolve numerically. Traditional numerical methods, such as Finite Difference Methods (FDMs), require a refined mesh to obtain stable and accurate solutions. As Physics-Informed Neural Networks (PINNs) have been shown to successfully approximate solutions to differential equations from various fields, it is natural to examine their performance on singularly perturbed problems. The convection-diffusion equation is a representative example of such a class of problems, and we consider the use of PINNs to produce numerical solutions of this equation. We study two ways to use PINNS: as a method for correcting oscillatory discrete solutions obtained using FDMs, and as a method for modifying reduced solutions of unperturbed problems. For both methods, we also examine the use of input transformation to enhance accuracy, and we explain the behavior of input transformations analytically, with the help of neural tangent kernels.
Code Edit Add Remove Mark official
Tasks edit add remove, datasets edit, results from the paper edit add remove, methods edit add remove.
Physics-Informed Neural Networks (PINNs)
What are physics-informed neural networks (pinns).
Physics-informed neural networks (PINNs) are neural networks that incorporate physical laws described by differential equations into their loss functions to guide the learning process toward solutions that are more consistent with the underlying physics. PINNs can be used to:
- Approximate solutions to partial differential equations (PDEs) and ordinary differential equations (ODEs).
- Solve inverse problems , such as estimating model parameters from limited data.
With Deep Learning Toolbox™, you can build and train PINNs, which enable rapid predictive analysis. You can integrate PINNs with MATLAB ® and Simulink ® for system-level simulation, control design, and design optimization.

Physics-informed neural networks (PINNs) include governing physical laws in the training of deep learning models to enable the prediction and modeling of complex phenomena while encouraging adherence to fundamental physical principles.
The Benefits of PINNs
PINNs are a class of physics-informed machine learning methods that seamlessly integrate physics knowledge with data. Often, PINNs get compared with purely data-driven methods and traditional numerical methods for solving problems involving PDEs and ODEs.
Unlike purely data-driven approaches, which learn mathematical relationships solely from input and output data, PINNs:
- Use prior physics knowledge.
- Make more accurate predictions outside of the training data set.
- Are more effective with limited or noisy training data.
Unlike traditional numerical methods for solving differential equations, such as finite element analysis for PDEs, PINNs:
- Are mesh-free.
- Can approximate high-dimensional PDE solutions.
- Can solve for missing model parameters such as unknown PDE or ODE coefficients.
- Can solve ill-posed problems where no boundary data exists.
- Can easily incorporate sparse or noisy measurements.
While PINNs offer potential benefits compared with purely data-driven methods and traditional numerical methods, PINNs do have some limitations and challenges, including:
- Limited convergence theory
- Lack of unified training strategies
- Computational cost of calculating high-order derivatives
- Difficulty learning high-frequency and multiscale components of PDE solutions
However, PINNs are a dynamic research area, with ongoing advancements expected to address and overcome these current challenges and limitations.
Choosing between PINNs, data-driven approaches, and traditional numerical methods depends on your application. The table below summarizes the benefits and limitations of each method.
Incorporate known physics | |||
Generalize well with limited or noisy training data | |||
Solve forward and inverse problems simultaneously | |||
Solve high-dimensional PDEs | |||
Enable fast “online” prediction | |||
Are mesh-free | |||
Have well-understood convergence theory | |||
Scale well to high-frequency and multiscale PDEs |
How PINNs Differ from Traditional Neural Networks
PINNs differ from traditional neural networks in their ability to incorporate a priori domain knowledge of the problem in the form of differential equations. This additional information enables PINNs to make more accurate predictions outside of the given measurement data. Furthermore, the additional physics knowledge regularizes the predicted solution in the presence of noisy measurement data, enabling PINNs to learn the true underlying signal rather than overfitting the noisy data.
For example, consider a scenario where the noisy measurements, \( θ_{meas} \), of a system of interest have been collected, and the objective is to predict future values of the system, \( θ_{pred} \), with a feedforward artificial neural network . The network is trained using the available measurements and will be used to predict unseen future values. Training a regression neural network typically involves minimizing the mean-squared error between the neural network’s predictions and the provided measurements.

Traditional neural networks adjust their parameters to minimize the error between the network’s prediction and observed measurements.
The neural network struggles to accurately predict values of the system outside of the training data.
A naïve neural network, trained using the trainnet function in Deep Learning Toolbox, overfits noisy measurements and performs poorly for t outside of available range. ( See MATLAB code. )
Acquiring more data could enhance predictions, yet this approach may be prohibitively expensive or impossible for many applications. However, often the domain expert possesses deeper knowledge about the underlying physical process that governs the system they are studying. Specifically, in this scenario, the measurements represent the angle of displacement from vertical of a payload swinging from a crane. This process can be simplistically represented by a damped pendulum, which can be approximately modeled for small angles by a linear, second-order differential equation:
\( θ^{''}(t)+2βθ^{′}(t)+ω^{2}_{0}θ(t)=0 \)
Rather than ignoring this knowledge, PINNs incorporate the differential equation as an additional, physics-informed term in the loss function. PINNs evaluate the residual of the differential equation at additional points in the domain, which provides more information to the PINN without the need for more measurements. While this toy example can be solved analytically , it illustrates the concepts behind PINNs.

PINNs, available in Deep Learning Toolbox, adjust their parameters to balance minimizing the error between the network’s prediction and observed measurements and the physics loss.
During training, PINNs find a balance between fitting the given measurements and the underlying physical process.
A PINN, created and trained using Deep Learning Toolbox, makes better predictions outside of the measurement data and is more robust to noise than the traditional neural network. ( See MATLAB code. )
By incorporating an extra physics loss term, PINNs can outperform traditional neural networks in making predictions in the presence of noisy measurements and in data regimes without measurements.
How PINNs Work
PINNs use optimization algorithms to iteratively update the parameters of a neural network until the value of a specified, physics-informed loss function is decreased to an acceptable level, pushing the network toward a solution of the differential equation.

In training PINNs for an ODE like the pendulum equation, an optimization algorithm adjusts the neural network’s parameters to reduce a loss function—comprising the differential equation residual from automatic differentiation (AD), boundary and initial conditions, and optionally other labeled data—to an acceptable level.
PINNs have loss functions, \( L \), which consist of several terms: the physics-informed loss term, \( L_{Physics} \), and optionally terms that evaluate the error between the values predicted by the network and any values prescribed by initial and/or boundary data, \( L_{Conds} \), and other additional measurements, \( L_{Data} \). The physics-informed loss term evaluates the residual of the differential equation at points in the domain using automatic differentiation (AD) or other numerical differentiation techniques. Because the physics-informed term does not compute the error between a prediction and a target value, this term can be considered an unsupervised loss term, meaning that the network can be trained with any points from the domain, even without measurements at these points.
First introduced in 2017, PINNs now have many variations, including:
- Bayesian PINNs (BPINNs), which use the Bayesian framework to allow for uncertainty quantification
- Variational PINNs (VPINNs), which incorporate the weak form of a PDE into the loss function
- First-order formulated PINNs (FO-PINNs), which can be faster and more accurate for solving higher-order PDEs than standard PINNs
In addition, PINNs can be used with different neural network architectures, such as graph neural networks (GNNs), Fourier neural operators (FNOs) , deep operator networks (DeepONets), and others, yielding so-called physics-informed versions of these architectures.
MATLAB and Deep Learning Toolbox comprehensively support the development of PINNs, from creating or importing diverse neural network architectures, to defining custom physics-informed loss functions with AD, to training using gradient-based optimization algorithms such as ADAM or L-BFGS, and finally to visualizing solutions with advanced MATLAB graphics.
PINN Applications
PINNs leverage the power of deep learning while improving compliance with physical laws, making them a versatile tool for applications where physics is fully or partially known, such as the case of a PDE or ODE with unknown coefficients. Applications of PINNs include:
- Heat transfer , specifically for modeling heat distribution and transfer processes. PINNs can embed the governing equations that model thermal processes in materials and systems, such as the heat equation, into the loss function. This approach ensures that the solutions adhere to these physical laws, leading to physically plausible predictions. In addition, PINNs can replace expensive numerical simulations to quickly approximate temperature distributions over parameterized geometries in design optimization applications. Furthermore, PINNs can be used in inverse problems to identify unknown material properties, such as thermal conductivity.
- Computational fluid dynamics (CFD) , specifically for approximating velocity, pressure, and temperature fields of fluids by incorporating the Navier-Stokes equations in the loss function. PINNs can be used in mesh-free forward simulations to accurately predict these quantities or in inverse problems where the goal is to infer unknown parameters or inputs, such as boundary conditions, source terms, or fluid properties, from observed data.
- Structural mechanics , for solving both forward and inverse problems by embedding the governing physical laws, such as equations of elasticity and structural dynamics, directly into the loss function. This integration enables PINNs to accurately predict structural responses such as deformations, stresses, and strains under various loads and conditions, as well as identify unknown material properties or external loads based on observed data. Particularly useful in scenarios where traditional analytical solutions are infeasible or data is scarce, PINNs reduce the dependency on extensive data sets by leveraging physical principles to guide the learning process. The flexibility of PINNs enables them to handle complex problems, including nonlinear material behavior and multiphysics modeling.
Once created and trained with Deep Learning Toolbox, PINNs can be seamlessly integrated with Optimization Toolbox™ for design optimization, connected to Simulink for system-level simulation, and leveraged across a wide range of other applications.
Examples and How To
- Solve Partial Differential Equation with L-BFGS Method and Deep Learning - Example
- Solve Ordinary Differential Equation Using Neural Network - Example
- Solve Poisson Equation on Unit Disk Using Physics-Informed Neural Networks - Example
- Inverse Problems Using PINNs - Downloadable Code
- Physics-Informed Machine Learning: Cloud-Based Deep Learning and Acoustic Patterning for Organ Cell Growth Research - Article
Software Reference
- dlgradient : Compute gradients for custom training loops using automatic differentiation - Function
- dlnetwork : Deep learning neural network - Object
- Custom Training Loops - Documentation
- adamupdate : Update parameters using adaptive moment estimation (Adam) - Function
- lbfgsupdate : Update parameters using limited-memory BFGS (L-BFGS) - Function
See also: Deep Learning Toolbox , Partial Differential Equation Toolbox , finite element analysis , reduced order modeling , Hamiltonian neural network , dynamical system modeling using neural ODE , deep learning , convolutional neural networks (CNNs) , generative adversarial networks (GANs) , long short-term memory (LSTM) networks , recurrent neural networks (RNNs) , neural networks

Select a Web Site
Choose a web site to get translated content where available and see local events and offers. Based on your location, we recommend that you select: .
You can also select a web site from the following list
How to Get Best Site Performance
Select the China site (in Chinese or English) for best site performance. Other MathWorks country sites are not optimized for visits from your location.
- América Latina (Español)
- Canada (English)
- United States (English)
- Belgium (English)
- Denmark (English)
- Deutschland (Deutsch)
- España (Español)
- Finland (English)
- France (Français)
- Ireland (English)
- Italia (Italiano)
- Luxembourg (English)
- Netherlands (English)
- Norway (English)
- Österreich (Deutsch)
- Portugal (English)
- Sweden (English)
- United Kingdom (English)
Asia Pacific
- Australia (English)
- India (English)
- New Zealand (English)
- 简体中文 Chinese
- 日本 Japanese (日本語)
- 한국 Korean (한국어)
Contact your local office
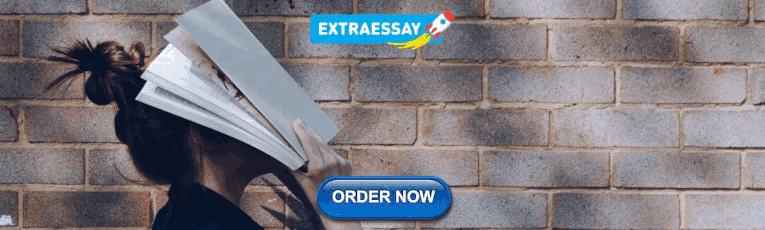
COMMENTS
This thesis is an overview of the progress made in traditional machine learning methods. It specifically discusses a major architecture, convolutional neural networks within deep learning, ... neural networks, including Alex Net, VGG Net, ZF Net, GoogLe Net, Microsoft ResNet and SENet. In chapter five we present the different frameworks that ...
In this thesis, I investigate two major directions for explaining deep neural networks. The first direction consists of feature-based post-hoc explanatory methods, that is, methods that aim to explain an already trained and fixed model (post-hoc), and that provide explanations in terms of input features, such as tokens for text and superpixels ...
Oulu University of Applied Sciences Information Technology, Internet Services. Author: Hung Dao Title of the bachelor's thesis: Image Classification Using Convolutional Neural Networks Supervisor: Jukka Jauhiainen Term and year of completion: Spring 2020 Number of pages: 31. The objective of this thesis was to study the application of deep ...
We believe that this framework can help in uence future developments in applications of neural networks, but we have not focused on that in this thesis. We have developed a mathematical framework for neural networks over nite dimen-sional inner product spaces with deterministic inputs and outputs.
Neural Networks Serge Aleshin-Guendel Computer Science Honors Thesis Boston College Advised by Professor Sergio Alvarez May 10, 2017. ... uses a speci c model called a neural network [2]. What follows in this thesis is an introduction to supervised learning, an introduction to neural networks, and my work on Convolutional Neural
Therefore, several directions for explaining neural models have recently been explored. In this thesis, I investigate two major directions for explaining deep neural networks. The rst direction consists of feature-based post-hoc explanatory methods, that is, methods that aim to explain an already trained and
The publications below describe work that is loosely related to this thesis but not described in the thesis: ImageNet Classification with Deep Convolutional Neural Networks Alex Krizhevsky, Ilya Sutskever, and Geoffrey Hinton. In Advances in Neural Information Pro-cessing Systems 26, (NIPS*26), 2012. (Krizhevsky et al., 2012)
Convolutional Neural Network Architectures Master Thesis of Martin Thoma Department of Computer Science Institute for Anthropomatics and FZI Research Center for Information Technology Reviewer: Prof. Dr.-Ing. R. Dillmann Second reviewer: Prof. Dr.-Ing. J. M. Zöllner Advisor: Dipl.-Inform. Michael Weber Research Period: 03. May 2017 ...
Through analyses of neural recordings, biological data, and simulations of neural network models, this thesis demonstrates the power and versatility of these new methods. By advancing RSA with topological techniques, this work provides a powerful new lens for understanding brains, computational models, and complex biological systems.
This thesis tackles the bottlenecks of modern methods directly, achieving state-of-the-art performance by efficiently designing efficient deep neural networks. These improvements don't only reduce computation or only improve accuracy; instead, our methods improve performance and reduce computational requirements, despite increasing search ...
Convolutional neural networks (CNNs or ConvNets) are a speci alized form of deep. neural networks for analyzing input data that contain some form of spatial structure. (Goodfellow et al. 2016 ...
In this thesis, we focus on two-layer neural networks with piecewise linear activations and show that they can be formulated as finite-dimensional convex programs with a regularization term that promotes sparsity, which is a variant of group Lasso. We first utilize semi-infinite programming theory to prove strong duality for finite-width neural ...
This thesis consists of four parts. Each part also studies one aspect of the theoretical landscape of learning: the representation power, generalization, extrapolation, and optimization. In Part I, we characterize the expressive power of graph neural networks for representing graphs, and build maximally powerful graph neural networks.
Abstract Graph Neural Networks Fernando Gama Alejandro Ribeiro The theme of this dissertation is machine learning on graph data. Graphs are generic models of
Summary. Neural networks have made tremendous advancements in a variety of machine learning tasks across different fields. Typically, neural networks have relied on heuristically optimizing a non-convex objective, raising doubts into their transparency, efficiency, and empirical performance. In this thesis, we show that a wide variety of neural ...
Neural network Five main methodologies are usually employed for video-frame interpolation, namely flow-based methods, convolutional neural networks (CNN), phase-based approaches, GANs, and hybrid ...
The aim of this thesis is to advance the state-of-the-art in supervised sequence labelling with recurrent networks in general, and long short-term memory in particular. Its two main contributions are (1) a new type of output layer that allows recurrent networks to be trained directly for sequence labelling tasks where the align-
neural networks can signi cantly improve spoken language understanding systems. iv. To my parents{Dave & Judy, and my brothers{Colin, John, & Richard. v. Acknowledgements This thesis comes from a close collaboration with my advisor, Andrew Ng. Andrew has been an amazing mentor in the process of planning and solving research problems,
Understanding how neural networks align with human cognitive processes is a crucial step toward developing more interpretable and reliable AI systems. Motivated by theories of human cognition, this study examines the relationship between \\emph{convexity} in neural network representations and \\emph{human-machine alignment} based on behavioral data. We identify a correlation between these two ...
A secondary contribution of this thesis is an algorithm, called BBP, that constructively induces simple neural networks. The motivation underlying this algorithm is similar to that for Trepan: to learn comprehensible models in problem domains in which neural networks have an especially appropriate inductive bias. The BBP algorithm, which is based
A typical neural network works like this: Layers of artificial neurons (or nodes) connect to each other using artificial synapses (or edges). Information passes through each layer, where it is processed and transmitted to the next layer, until it eventually becomes an output. The edges are weighted, so that those with greater weights have more ...
In this manuscript, optimized heterogeneous bi-directional recurrent neural network for early leaf disease detection and pesticides recommendation system (HBDRNN-ELD-PRS) is proposed. Initially, the input images are collected from plant dataset. To execute this, the collected input image is pre-processed using multimodal hierarchical graph ...
Optical neural networks present distinct advantages over traditional electrical counterparts, such as accelerated data processing and reduced energy consumption. While coherent light is conventionally employed in optical neural networks, our study proposes harnessing spatially incoherent light in all-optical Fourier neural networks. Contrary to numerical predictions of declining target ...
This thesis studies the introduction of a priori structure into the design of learning systems based on artificial neural networks applied to sequence recognition, in particular to phoneme recognition in continuous speech. Because we are interested in sequence analysis, algorithms for training recurrent networks are studied and an original ...
Airport noise prediction models are divided into physics-guided methods and data-driven methods. The prediction results of physics-guided methods are relatively stable, but their overall prediction accuracy is lower than that of data-driven methods. However, machine learning methods have a relatively high prediction accuracy, but their prediction stability is inferior to physics-guided methods ...
Master's Thesis : Deep Learning for Visual Recognition. The goal of our research is to develop methods advancing automatic visual recognition. In order to predict the unique or multiple labels associated to an image, we study different kind of Deep Neural Networks architectures and methods for supervised features learning. We first draw up a ...
Long Short-term Memory Recurrent Neural Networks for Classi cation of Acute Hypotensive Episodes by Alexander Scott Ja e Submitted to the Department of Electrical Engineering and Computer ... This thesis thus applies established neural network techniques to the established AHE prediction problem to produce novel results. 12. Chapter 2
The thesis also covers the practical applications of artificial neural network technology and how it is used in different fields of industry. Positive and negative properties of artificial neural networks and how they should be developed in the future will be observed. For this thesis a lot of research material from different research areas was ...
As Physics-Informed Neural Networks (PINNs) have been shown to successfully approximate solutions to differential equations from various fields, it is natural to examine their performance on singularly perturbed problems. The convection-diffusion equation is a representative example of such a class of problems, and we consider the use of PINNs ...
MATLAB and Deep Learning Toolbox comprehensively support the development of PINNs, from creating or importing diverse neural network architectures, to defining custom physics-informed loss functions with AD, to training using gradient-based optimization algorithms such as ADAM or L-BFGS, and finally to visualizing solutions with advanced MATLAB ...