| Name of variable | Symbol | Measurement |
---|
Dependent variable | Financial performance | Return on asset | ROA | |
| Return on equity | ROE | |
Independent variable | Credit risk | Nonperforming loans | NPLs | |
Capital adequacy ratio | CAR | |
| Bank-specific factors | Cost-efficiency ratio | CER | |
| Average lending rate | ALR | |
Liquidity ratio | LR | |
Control variables | Bank size | BZ | Log (total assets) |
Inflation | Inflation | Annual inflation rate declared by word bank |
Age | Age | Age of commercial banks |
Results for autocorrelation for South Asia countries
Wooldridge test for autocorrelation in panel data in developing countries |
---|
Model ROA | Model ROE |
---|
= 111.092 | = 7.447 |
Prob > = 0.0000 | Prob > = 0.0138 |
Descriptive statistics
Variables | Mean | Maximum | Minimum | Std. dev. | Observations |
---|
ROA | 0.986 | 10.408 | −6.234 | 1.905 | 190 |
ROE | 7.964 | 100.158 | −268.759 | 39.175 | 190 |
NPL | 7.206 | 64.058 | 0.271 | 9.659 | 190 |
CAR | 13.885 | 39.130 | 1.050 | 4.183 | 190 |
CER | 27.920 | 68.696 | 13.050 | 9.808 | 190 |
ALR | 8.723 | 14.701 | 5.542 | 1.615 | 190 |
LR | 68.016 | 107.179 | 25.027 | 14.177 | 190 |
YES | 3.899 | 5.008 | 2.318 | 0.589 | 190 |
YES | 49.131 | 111 | 5 | 34.171 | 190 |
YES | 9.449 | 20.92 | 2.540 | 3.891 | 190 |
Correlation figures
Variable | ROA | ROE | NPL | CAR | CER | LR | ALR | SIZE | AGE | INF |
---|
ROA | 1 | | | | | | | | | |
ROE | 0.757 | 1 | | | | | | | | |
NPL | −0.225 | −0.378 | 1 | | | | | | | |
CAR | 0.184 | 0.156 | −0.284 | 1 | | | | | | |
CER | −0.170 | −0.195 | 0.058 | 0.407 | 1 | | | | | |
LR | 0.026 | 0.010 | −0.305 | −0.121 | −0.402 | 1 | | | | |
ALR | 0.140 | 0.020 | 0.143 | 0.091 | 0.133 | −0.237 | 1 | | | |
YES | 0.298 | 0.305 | −0.213 | −0.025 | −0.335 | 0.457 | −0.441 | 1 | | |
YES | −0.007 | 0.019 | −0.182 | −0.063 | −0.310 | 0.213 | −0.197 | 0.099 | 1 | |
YES | −0.013 | −0.171 | 0.163 | −0.019 | 0.024 | 0.152 | 0.520 | −0.162 | −0.135 | 1 |
ROA model (pooled regression and fixed effect GMM result)
Pooled regression | Generalized method of moments |
---|
Variable | Coefficient | Std. Error | -Statistic | Prob. | Coefficient | Std. Error | -Statistic | Prob. |
---|
C | −5.449 | 1.754 | −3.105 | 0.002* | −7.098 | 1.959 | −3.621 | 0.000 |
NPL | −0.034 | 0.014 | −2.434 | 0.015** | −0.032 | 0.015 | −2.088 | 0.038** |
CAR | 0.080 | 0.033 | 2.417 | 0.016** | 0.085 | 0.036 | 2.329 | 0.021** |
CER | −0.043 | 0.015 | −2.824 | 0.005* | −0.048 | 0.016 | −2.972 | 0.003* |
ALR | 0.409 | 0.099 | 4.131 | 0.000* | 0.492 | 0.112 | 4.392 | 0.000* |
LR | −0.025 | 0.011 | −2.296 | 0.022** | −0.027 | 0.013 | −2.125 | 0.035** |
YES | 1.371 | 0.254 | 5.383 | 0.000* | 1.649 | 0.292 | 5.645 | 0.000* |
YES | −0.002 | 0.003 | −0.588 | 0.557 | −0.000 | 0.004 | −0.026 | 0.978 |
YES | −0.031 | 0.039 | −0.805 | 0.421 | −0.032 | 0.061 | −0.525 | 0.599 |
| 0.282 | | | | 0.372 | | | |
Adjusted | 0.250 | | | | 0.358 | | | |
S.E. of regression | 1.650 | | | | 1.672 | | | |
Durbin–Watson stat | 1.834 | | | | 1.980 | | | |
Hause test ( ) | | | | | 50.960 | | | |
-value( ) | | | | | 0.000 | | | |
*Indicates significance at 1% level, ** Indicates significance at 5% level, *** Indicates significance at the 10% level Pooled regression | Generalized method of moments |
---|
Variable | Coefficient | Std. Error | -Statistic | Prob. | Coefficient | Std. Error | -Statistic | Prob. |
---|
C | −16.229 | 39.885 | −0.407 | 0.685 | −26.740 | 44.139 | −0.606 | 0.546 |
NPL | −1.418 | 0.327 | −4.337 | 0.000* | −1.379 | 0.354 | −3.893 | 0.000* |
CAR | 1.261 | 0.713 | 1.769 | 0.079*** | 1.315 | 0.774 | 1.699 | 0.091*** |
CER | −1.035 | 0.350 | −2.953 | 0.004* | −1.032 | 0.375 | −2.754 | 0.007* |
LR | −0.464 | 0.232 | −2.000 | 0.047** | −0.463 | 0.262 | −1.768 | 0.079*** |
ALR | 3.981 | 2.037 | 1.954 | 0.052*** | 4.331 | 2.238 | 1.936 | 0.055*** |
YES | 16.309 | 6.355 | 2.566 | 0.011** | 18.481 | 7.001 | 2.640 | 0.009* |
YES | −0.113 | 0.105 | −1.082 | 0.281 | −0.097 | 0.118 | −0.824 | 0.411 |
YES | −1.623 | 0.734 | −2.211 | 0.028** | −1.867 | 0.857 | −2.178 | 0.031** |
| 0.234 | | | | 0.265 | | | |
Adjusted | 0.231 | | | | 0.249 | | | |
S.E. of regression | 30.442 | | | | 29.378 | | | |
Durbin–Watson stat | 1.340 | | | | 1.511 | | | |
Hause test ( ) | | | | | 18.183 | | | |
-value ( ) | | | | | 0.000 | | | |
*Indicates significance at 1% level, ** Indicates significance at 5% level, *** Indicates significance at the 10% level South Asian countries (ROA) |
---|
Variable | Fixed | Random | Prob |
---|
NPLs | −0.066068 | −0.034634 | 0.0046 |
ALR | 0.222311 | 0.215984 | 0.9208 |
CAR | 0.001613 | 0.068108 | 0.0002 |
CER | −0.027827 | −0.030749 | 0.8033 |
LR | 0.014278 | −0.001034 | 0.0021 |
SG | 0.011874 | 0.011150 | 0.7116 |
SIZE | −4.446678 | 0.339918 | 0.0000 |
INFLATION | −0.055072 | −0.053349 | 0.8938 |
AGE | 0.273576 | −0.002688 | 0.0000 |
South Asian countries (ROE) |
---|
Variable | Fixed | Random | Prob. |
---|
NPLs | −1.381440 | −0.918537 | 0.9468 |
ALR | −1.535941 | 0.727034 | 0.1002 |
CAR | 0.008182 | −0.096010 | 0.6395 |
CER | −0.328610 | −0.232542 | 0.5979 |
LR | −0.012691 | −0.067185 | 0.0082 |
SG | 0.029615 | 0.088314 | 0.5599 |
SIZE | −12.082609 | 0.382527 | 0.1095 |
INFL | −0.390149 | 0.087808 | 0.8251 |
AGE | −0.687654 | −0.007677 | 0.2538 |
Extra tables and figures in the Google drop box and available at: https://www.dropbox.com/sh/dro0gkowf3t542r/AAC3QQ5lKQTpLdke7UNxRUEea?dl=0
Accornero , M. , Cascarino , G. , Felici , R. , Parlapiano , F. and Sorrentino , A.M. ( 2018 ), “ Credit risk in banks' exposures to non-financial firms ”, European Financial Management , Vol. 24 No. 5 , pp. 775 - 791 .
Adebayo , M. , Adeyanju , D. and Olabode , S. ( 2011 ), “ Liquidity management and commercial banks' profitability in Nigeria ”, Research Journal of Finance and Accounting , Vol. 2 No. 8 .
Akhtar , M.F. , Ali , K. and Sadaqat , S. ( 2011 ), “ Factors influencing the profitability of Islamic banks of Pakistan ”, International Research Journal of Finance and Economics , Vol. 66 , pp. 125 - 132 .
Aspal , P.K. , Dhawan , S. and Nazneen , A. ( 2019 ), “ Significance of bank specific and macroeconomic determinants on performance of Indian private sector banks ”, International Journal of Economics and Financial Issues , Vol. 9 No. 2 , p. 168 .
Belas , J. , Smrcka , L. , Gavurova , B. and Dvorsky , J. ( 2018 ), “ The impact of social and economic factors in the credit risk management of SME ”, Technological and Economic Development of Economy , Vol. 24 No. 3 , pp. 1215 - 1230 .
Berger , A.N. and DeYoung , R. ( 1997 ), “ Problem loans and cost efficiency in commercial banks ”, Journal of Banking and Finance , Vol. 21 , pp. 849 - 870 .
Blum , J. and Hellwig , M. ( 1995 ), “ The macroeconomic implications of capital adequacy requirements for banks ”, European Economic Review , pp. 739 - 749 .
Brooks , C. ( 2014 ), Introductory Econometrics for Finance , Cambridge University Press .
Chimkono , E.E. , Muturi , W. and Njeru , A. ( 2016 ), “ Effect of non-performing loans and other factors on performance of commercial banks in Malawi ”, International Journal of Economics, Commerce and Management , Vol. IV , pp. 1 - 15 .
Ekinci , R. and Poyraz , G. ( 2019 ), “ The effect of credit risk on financial performance of deposit banks in Turkey ”, Procedia Computer Science , Vol. 158 , pp. 979 - 987 .
Francis , B.B. , Hasan , I. , Song , L. and Yeung , B. ( 2015 ), “ What determines bank-specific variations in bank stock returns? Global evidence ”, Journal of Financial Intermediation , Vol. 24 No. 3 , pp. 312 - 324 .
Ghenimi , A. , Chaibi , H. and Omri , M.A.B. ( 2017 ), “ The effects of liquidity risk and credit risk on bank stability: evidence from the MENA region ”, Borsa Istanbul Review , Vol. 17 No. 4 , pp. 238 - 248 .
Ghosh , A. ( 2015 ), “ Banking-industry specific and regional economic determinants of non-performing loans: evidence from US states ”, Journal of Financial Stability , Vol. 20 , pp. 93 - 104 .
Hamza , S.M. ( 2017 ), “ Impact of credit risk management on banks performance: a case study in Pakistan banks ”, European Journal of Business and Management , Vol. 9 No. 1 , pp. 57 - 64 .
Hasanov , F. , Bayramli , N. and Al-Musehel , N. ( 2018 ), “ Bank-specific and macroeconomic determinants of bank profitability: evidence from an oil-dependent economy ”, International Journal of Financial Studies , Vol. 6 No. 3 , p. 78 .
Hassan , M.K. , Khan , A. and Paltrinieri , A. ( 2019 ), “ Liquidity risk, credit risk and stability in Islamic and conventional banks ”, Research in International Business and Finance , Vol. 48 , pp. 17 - 31 .
Khan , M.A. , Siddique , A. and Sarwar , Z. ( 2020 ), “ Determinants of non-performing loans in the banking sector in developing state ”, Asian Journal of Accounting Research .
Kusi , B.A. , Agbloyor , E.K. , Ansah-Adu , K. and Gyeke-Dako , A. ( 2017 ), “ Bank credit risk and credit information sharing in Africa: does credit information sharing institutions and context matter? ”, Research in International Business and Finance , Vol. 42 , pp. 1123 - 1136 .
Lassoued , M. ( 2018 ), “ Comparative study on credit risk in Islamic banking institutions: the case of Malaysia ”, The Quarterly Review of Economics and Finance , Vol. 70 , pp. 267 - 278 .
Louzis , D.P. , Vouldis , A.T. and Metaxas , V.L. ( 2012 ), “ Macroeconomic and bank-specific determinants of non-performing loans in Greece: a comparative study of mortgage, business and consumer loan portfolios ”, Journal of Banking and Finance , Vol. 36 No. 4 , pp. 1012 - 1027 .
Masood , O. and Ashraf , M. ( 2012 ), “ Bank-specific and macroeconomic profitability determinants of Islamic banks: the case of different countries ”, Qualitative Research in Financial Markets , Vol. 4 Nos 2/3 , pp. 255 - 268 .
Ofori-Abebrese , G. , Pickson , R.B. and Opare , E. ( 2016 ), “ The effect of bank specific factors on loan performance of HFC bank in Ghana ”, International Journal of Economics and Finance , Vol. 8 No. 7 , p. 185 .
Oluwafemi , A.S. , Adebisi , A.N.S. , Simeon , O. and Olawale , O. ( 2013 ), “ Risk management and financial performance of banks in Nigeria ”, Journal of Business and Management , Vol. 14 No. 6 , pp. 52 - 56 .
Rachman , R.A. , Kadarusman , Y.B. , Anggriono , K. and Setiadi , R. ( 2018 ), “ bank-specific factors affecting non-performing loans in developing countries: case study of Indonesia ”, The Journal of Asian Finance, Economics and Business (JAFEB) , Vol. 5 No. 2 , pp. 35 - 42 .
Raphael , G. ( 2013 ), “ Bank-specific, industry-specific and macroeconomic determinants of bank efficiency in Tanzania: a two stage analysis ”, European Journal of Business and Management , Vol. 5 No. 2 , pp. 142 - 154 .
Sekaran , U. ( 2006 ), Research Methods for Business: A Skill Building Approach , John Wiley & Sons .
Siddique , A. , Masood , O. , Javaria , K. and Huy , D.T.N. ( 2020 ), “ A comparative study of performance of commercial banks in ASIAN developing and developed countries ”, Insights Into Regional Development .
Corresponding author
Related articles, all feedback is valuable.
Please share your general feedback
Report an issue or find answers to frequently asked questions
Contact Customer Support
About Stanford GSB
- The Leadership
- Dean’s Updates
- School News & History
- Commencement
- Business, Government & Society
- Centers & Institutes
- Center for Entrepreneurial Studies
- Center for Social Innovation
- Stanford Seed
About the Experience
- Learning at Stanford GSB
- Experiential Learning
- Guest Speakers
- Entrepreneurship
- Social Innovation
- Communication
- Life at Stanford GSB
- Collaborative Environment
- Activities & Organizations
- Student Services
- Housing Options
- International Students
Full-Time Degree Programs
- Why Stanford MBA
- Academic Experience
- Financial Aid
- Why Stanford MSx
- Research Fellows Program
- See All Programs
Non-Degree & Certificate Programs
- Executive Education
- Stanford Executive Program
- Programs for Organizations
- The Difference
- Online Programs
- Stanford LEAD
- Seed Transformation Program
- Aspire Program
- Seed Spark Program
- Faculty Profiles
- Academic Areas
- Awards & Honors
- Conferences
Faculty Research
- Publications
- Working Papers
- Case Studies
Research Hub
- Research Labs & Initiatives
- Business Library
- Data, Analytics & Research Computing
- Behavioral Lab
Research Labs
- Cities, Housing & Society Lab
- Golub Capital Social Impact Lab
Research Initiatives
- Corporate Governance Research Initiative
- Corporations and Society Initiative
- Policy and Innovation Initiative
- Rapid Decarbonization Initiative
- Stanford Latino Entrepreneurship Initiative
- Value Chain Innovation Initiative
- Venture Capital Initiative
- Career & Success
- Climate & Sustainability
- Corporate Governance
- Culture & Society
- Finance & Investing
- Government & Politics
- Leadership & Management
- Markets and Trade
- Operations & Logistics
- Opportunity & Access
- Technology & AI
- Opinion & Analysis
- Email Newsletter
Welcome, Alumni
- Communities
- Digital Communities & Tools
- Regional Chapters
- Women’s Programs
- Identity Chapters
- Find Your Reunion
- Career Resources
- Job Search Resources
- Career & Life Transitions
- Programs & Webinars
- Career Video Library
- Alumni Education
- Research Resources
- Volunteering
- Alumni News
- Class Notes
- Alumni Voices
- Contact Alumni Relations
- Upcoming Events
Admission Events & Information Sessions
- MBA Program
- MSx Program
- PhD Program
- Alumni Events
- All Other Events
- Operations, Information & Technology
- Organizational Behavior
- Political Economy
- Classical Liberalism
- The Eddie Lunch
- Accounting Summer Camp
- California Econometrics Conference
- California Quantitative Marketing PhD Conference
- California School Conference
- China India Insights Conference
- Homo economicus, Evolving
- Political Economics (2023–24)
- Scaling Geologic Storage of CO2 (2023–24)
- A Resilient Pacific: Building Connections, Envisioning Solutions
- Adaptation and Innovation
- Changing Climate
- Civil Society
- Climate Impact Summit
- Climate Science
- Corporate Carbon Disclosures
- Earth’s Seafloor
- Environmental Justice
- Operations and Information Technology
- Organizations
- Sustainability Reporting and Control
- Taking the Pulse of the Planet
- Urban Infrastructure
- Watershed Restoration
- Junior Faculty Workshop on Financial Regulation and Banking
- Ken Singleton Celebration
- Marketing Camp
- Quantitative Marketing PhD Alumni Conference
- Presentations
- Theory and Inference in Accounting Research
- Stanford Closer Look Series
- Quick Guides
- Core Concepts
- Journal Articles
- Glossary of Terms
- Faculty & Staff
- Subscribe to Corporate Governance Emails
- Researchers & Students
- Research Approach
- Charitable Giving
- Financial Health
- Government Services
- Workers & Careers
- Short Course
- Adaptive & Iterative Experimentation
- Incentive Design
- Social Sciences & Behavioral Nudges
- Bandit Experiment Application
- Conferences & Events
- Get Involved
- Reading Materials
- Teaching & Curriculum
- Energy Entrepreneurship
- Faculty & Affiliates
- SOLE Report
- Responsible Supply Chains
- Current Study Usage
- Pre-Registration Information
- Participate in a Study
Interest Rate Risk in Banking
We develop a theoretical and empirical framework to estimate bank franchise value. In contrast to regulatory guidance and some existing models, we show that sticky deposits combined with low deposit rate betas do not imply a negative duration for franchise value. Operating costs could in principle generate negative duration, but they are more than offset by fixed interest rate spreads that arise largely from banks’ lending activity. As a result, bank franchise value declines as interest rates rise, and this decline exacerbates, rather than offsets, losses on banks’ security holdings. We also show that in the cross section, banks with the least responsive deposit rate tend to invest the most in long-term securities, suggesting that they are motivated to hedge cash flows rather than market value. Finally, despite significant losses to both asset and franchise values stemming from recent rate hikes, our analysis suggests that most U.S. banks still retain sufficient franchise value to remain solvent as ongoing concerns.
- See the Current DEI Report
- Supporting Data
- Research & Insights
- Share Your Thoughts
- Search Fund Primer
- Affiliated Faculty
- Faculty Advisors
- Louis W. Foster Resource Center
- Defining Social Innovation
- Impact Compass
- Global Health Innovation Insights
- Faculty Affiliates
- Student Awards & Certificates
- Changemakers
- Dean Jonathan Levin
- Dean Garth Saloner
- Dean Robert Joss
- Dean Michael Spence
- Dean Robert Jaedicke
- Dean Rene McPherson
- Dean Arjay Miller
- Dean Ernest Arbuckle
- Dean Jacob Hugh Jackson
- Dean Willard Hotchkiss
- Faculty in Memoriam
- Stanford GSB Firsts
- Annual Alumni Dinner
- Class of 2024 Candidates
- Certificate & Award Recipients
- Dean’s Remarks
- Keynote Address
- Teaching Approach
- Analysis and Measurement of Impact
- The Corporate Entrepreneur: Startup in a Grown-Up Enterprise
- Data-Driven Impact
- Designing Experiments for Impact
- Digital Marketing
- The Founder’s Right Hand
- Marketing for Measurable Change
- Product Management
- Public Policy Lab: Financial Challenges Facing US Cities
- Public Policy Lab: Homelessness in California
- Lab Features
- Curricular Integration
- View From The Top
- Formation of New Ventures
- Managing Growing Enterprises
- Startup Garage
- Explore Beyond the Classroom
- Stanford Venture Studio
- Summer Program
- Workshops & Events
- The Five Lenses of Entrepreneurship
- Leadership Labs
- Executive Challenge
- Arbuckle Leadership Fellows Program
- Selection Process
- Training Schedule
- Time Commitment
- Learning Expectations
- Post-Training Opportunities
- Who Should Apply
- Introductory T-Groups
- Leadership for Society Program
- Certificate
- 2024 Awardees
- 2023 Awardees
- 2022 Awardees
- 2021 Awardees
- 2020 Awardees
- 2019 Awardees
- 2018 Awardees
- Social Management Immersion Fund
- Stanford Impact Founder Fellowships
- Stanford Impact Leader Prizes
- Social Entrepreneurship
- Stanford GSB Impact Fund
- Economic Development
- Energy & Environment
- Stanford GSB Residences
- Environmental Leadership
- Stanford GSB Artwork
- A Closer Look
- California & the Bay Area
- Voices of Stanford GSB
- Business & Beneficial Technology
- Business & Sustainability
- Business & Free Markets
- Business, Government, and Society Forum
- Second Year
- Global Experiences
- JD/MBA Joint Degree
- MA Education/MBA Joint Degree
- MD/MBA Dual Degree
- MPP/MBA Joint Degree
- MS Computer Science/MBA Joint Degree
- MS Electrical Engineering/MBA Joint Degree
- MS Environment and Resources (E-IPER)/MBA Joint Degree
- Academic Calendar
- Clubs & Activities
- LGBTQ+ Students
- Military Veterans
- Minorities & People of Color
- Partners & Families
- Students with Disabilities
- Student Support
- Residential Life
- Student Voices
- MBA Alumni Voices
- A Week in the Life
- Career Support
- Employment Outcomes
- Cost of Attendance
- Knight-Hennessy Scholars Program
- Yellow Ribbon Program
- BOLD Fellows Fund
- Application Process
- Loan Forgiveness
- Contact the Financial Aid Office
- Evaluation Criteria
- GMAT & GRE
- English Language Proficiency
- Personal Information, Activities & Awards
- Professional Experience
- Letters of Recommendation
- Optional Short Answer Questions
- Application Fee
- Reapplication
- Deferred Enrollment
- Joint & Dual Degrees
- Entering Class Profile
- Event Schedule
- Ambassadors
- New & Noteworthy
- Ask a Question
- See Why Stanford MSx
- Is MSx Right for You?
- MSx Stories
- Leadership Development
- How You Will Learn
- Admission Events
- Personal Information
- GMAT, GRE & EA
- English Proficiency Tests
- Career Change
- Career Advancement
- Career Support and Resources
- Daycare, Schools & Camps
- U.S. Citizens and Permanent Residents
- Requirements
- Requirements: Behavioral
- Requirements: Quantitative
- Requirements: Macro
- Requirements: Micro
- Annual Evaluations
- Field Examination
- Research Activities
- Research Papers
- Dissertation
- Oral Examination
- Current Students
- Education & CV
- International Applicants
- Statement of Purpose
- Reapplicants
- Application Fee Waiver
- Deadline & Decisions
- Job Market Candidates
- Academic Placements
- Stay in Touch
- Faculty Mentors
- Current Fellows
- Standard Track
- Fellowship & Benefits
- Group Enrollment
- Program Formats
- Developing a Program
- Diversity & Inclusion
- Strategic Transformation
- Program Experience
- Contact Client Services
- Campus Experience
- Live Online Experience
- Silicon Valley & Bay Area
- Digital Credentials
- Faculty Spotlights
- Participant Spotlights
- Eligibility
- International Participants
- Stanford Ignite
- Frequently Asked Questions
- Founding Donors
- Program Contacts
- Location Information
- Participant Profile
- Network Membership
- Program Impact
- Collaborators
- Entrepreneur Profiles
- Company Spotlights
- Seed Transformation Network
- Responsibilities
- Current Coaches
- How to Apply
- Meet the Consultants
- Meet the Interns
- Intern Profiles
- Collaborate
- Research Library
- News & Insights
- Databases & Datasets
- Research Guides
- Consultations
- Research Workshops
- Career Research
- Research Data Services
- Course Reserves
- Course Research Guides
- Material Loan Periods
- Fines & Other Charges
- Document Delivery
- Interlibrary Loan
- Equipment Checkout
- Print & Scan
- MBA & MSx Students
- PhD Students
- Other Stanford Students
- Faculty Assistants
- Research Assistants
- Stanford GSB Alumni
- Telling Our Story
- Staff Directory
- Site Registration
- Alumni Directory
- Alumni Email
- Privacy Settings & My Profile
- Success Stories
- The Story of Circles
- Support Women’s Circles
- Stanford Women on Boards Initiative
- Alumnae Spotlights
- Insights & Research
- Industry & Professional
- Entrepreneurial Commitment Group
- Recent Alumni
- Half-Century Club
- Fall Reunions
- Spring Reunions
- MBA 25th Reunion
- Half-Century Club Reunion
- Faculty Lectures
- Ernest C. Arbuckle Award
- Alison Elliott Exceptional Achievement Award
- ENCORE Award
- Excellence in Leadership Award
- John W. Gardner Volunteer Leadership Award
- Robert K. Jaedicke Faculty Award
- Jack McDonald Military Service Appreciation Award
- Jerry I. Porras Latino Leadership Award
- Tapestry Award
- Student & Alumni Events
- Executive Recruiters
- Interviewing
- Land the Perfect Job with LinkedIn
- Negotiating
- Elevator Pitch
- Email Best Practices
- Resumes & Cover Letters
- Self-Assessment
- Whitney Birdwell Ball
- Margaret Brooks
- Bryn Panee Burkhart
- Margaret Chan
- Ricki Frankel
- Peter Gandolfo
- Cindy W. Greig
- Natalie Guillen
- Carly Janson
- Sloan Klein
- Sherri Appel Lassila
- Stuart Meyer
- Tanisha Parrish
- Virginia Roberson
- Philippe Taieb
- Michael Takagawa
- Terra Winston
- Johanna Wise
- Debbie Wolter
- Rebecca Zucker
- Complimentary Coaching
- Changing Careers
- Work-Life Integration
- Career Breaks
- Flexible Work
- Encore Careers
- Join a Board
- D&B Hoovers
- Data Axle (ReferenceUSA)
- EBSCO Business Source
- Global Newsstream
- Market Share Reporter
- ProQuest One Business
- RKMA Market Research Handbook Series
- Student Clubs
- Entrepreneurial Students
- Stanford GSB Trust
- Alumni Community
- How to Volunteer
- Springboard Sessions
- Consulting Projects
- 2020 – 2029
- 2010 – 2019
- 2000 – 2009
- 1990 – 1999
- 1980 – 1989
- 1970 – 1979
- 1960 – 1969
- 1950 – 1959
- 1940 – 1949
- Service Areas
- ACT History
- ACT Awards Celebration
- ACT Governance Structure
- Building Leadership for ACT
- Individual Leadership Positions
- Leadership Role Overview
- Purpose of the ACT Management Board
- Contact ACT
- Business & Nonprofit Communities
- Reunion Volunteers
- Ways to Give
- Fiscal Year Report
- Business School Fund Leadership Council
- Planned Giving Options
- Planned Giving Benefits
- Planned Gifts and Reunions
- Legacy Partners
- Giving News & Stories
- Giving Deadlines
- Development Staff
- Submit Class Notes
- Class Secretaries
- Board of Directors
- Health Care
- Sustainability
- Class Takeaways
- All Else Equal: Making Better Decisions
- If/Then: Business, Leadership, Society
- Grit & Growth
- Think Fast, Talk Smart
- Spring 2022
- Spring 2021
- Autumn 2020
- Summer 2020
- Winter 2020
- In the Media
- For Journalists
- DCI Fellows
- Other Auditors
- Academic Calendar & Deadlines
- Course Materials
- Entrepreneurial Resources
- Campus Drive Grove
- Campus Drive Lawn
- CEMEX Auditorium
- King Community Court
- Seawell Family Boardroom
- Stanford GSB Bowl
- Stanford Investors Common
- Town Square
- Vidalakis Courtyard
- Vidalakis Dining Hall
- Catering Services
- Policies & Guidelines
- Reservations
- Contact Faculty Recruiting
- Lecturer Positions
- Postdoctoral Positions
- Accommodations
- CMC-Managed Interviews
- Recruiter-Managed Interviews
- Virtual Interviews
- Campus & Virtual
- Search for Candidates
- Think Globally
- Recruiting Calendar
- Recruiting Policies
- Full-Time Employment
- Summer Employment
- Entrepreneurial Summer Program
- Global Management Immersion Experience
- Social-Purpose Summer Internships
- Process Overview
- Project Types
- Client Eligibility Criteria
- Client Screening
- ACT Leadership
- Social Innovation & Nonprofit Management Resources
- Develop Your Organization’s Talent
- Centers & Initiatives
- Student Fellowships
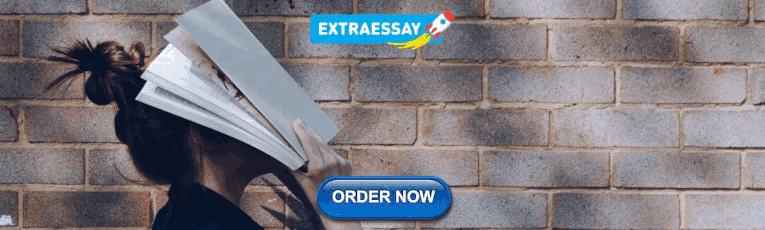
IMAGES
VIDEO
COMMENTS
The future of bank risk management. g Papers on RiskExecutive summaryBy 2025, risk functions in banks will likely need to be fundament. lly different than they are today. As hard as it may be to believe, the next ten years in risk management may be subject to more t. ansformation than the last decade. And unless banks start to act now and ...
This study presents a systematic literature review of regulation, profitability, and risk in the banking industry and explores the relationship between them. It proposes a policy initiative using a model that offers guidelines to establish the right mix among these variables. This is a systematic literature review study. Firstly, the necessary data are extracted using the relevant keywords ...
Credit risk-related research is vital for guiding new researchers and practitioners who want to improve their credit risk management practices. ... similar to banks and non-bank lenders, academics had to concentrate their interest on examining default predictions ... Data sets in papers published after 2014 (Period 6) mainly included the ...
These studies represent 43% of the reviewed sample, while the papers that use bank-specific and macroeconomic factors separately represent 38% and 14%, respectively. ... Understanding the impact of ownership structure on bank risk tolerance is significantly important to shape the banking sector operations and to help ... 6.1 Deepen the research ...
Cybersecurity risk assessment in banking is the process of identifying, anal yzing, and evaluating. the cyber threats and vulnerabilities that may affect the confidentiality, integrity, and ...
Abstract. The present study brings new insights to investigate the empirical estimation of banking risk behavior through advanced mechanisms. Consistent with the need to comply with the new age of finance, this study uniquely banks its case by employing nested tested modeling through a nexus of bank-specific parameters, governance mechanism, and industry dynamics.
Three main conclusions follow from the analysis of the World Bank's 2019 BRSS. First, reforms after the crisis led to an increase in capital requirements and regulatory capital holdings at financial institutions. These increases were accompanied by shifts toward asset categories with lower risk weights.
A thorough understanding of these latter would enable policymakers, regulators and bank managers to anticipate banks' failures and, academicians to advance their research. To facilitate further knowledge in this field, this paper reviews 69 studies published between 1987 and 2019 in 40 peer-reviewed journals.
In order to prevent and resolve systemic risk more effectively through deleveraging policy, this research takes China A-share listed commercial banks from 2011 to 2021 as samples, calculates the systemic risk spillover through the conditional value at risk model, re-estimates the leverage ratio with reference to the "Administration Measures for the Leverage Ratio of Commercial Banks (revised ...
Finally, the paper will outline the evolution of methodologies and theoretical underpinnings in credit risk management research and a landscape for possible future research directions. Banking prudence and efficiency to manage their risks in different business cycle and environment would help to alleviate crises and losses.
This paper, through a review of the available literature seeks to analyse and evaluate machine-learning techniques that have been researched in the context of banking risk management, and to identify areas or problems in risk management that have been inadequately explored and are potential areas for further research.
This paper has developed a banking risk index that incorporates the five main categories of banking risk with ten sub-variables measuring the risk components of each risk category. The study utilized data extracted from almost all the GCC banks (45 banks) over a 5-year period to develop the proposed risk index based on the Mahalanobis distance.
The paper also performs content analysis on top-cited papers of five risk categories to find major themes and theoretical, practical, and methodological contributions. These findings suggest scope for more research in risks other than credit and operating risks, as existing research is centred around modelling risk and predicting default rates ...
4.1. Random forest and relative value importance. We use the relative value importance (RVI) indicator from the random forests (RF) model introduced by Breiman (Citation 2001) to assess the contribution of the various studied factors to a bank's risk and profitability.RF is an ensemble learning method that combines several random algorithms, or decision trees, to arrive at the final output.
Abstract. Operational risk constitutes a large portion of a bank's risk exposure. Unlike other financial risks, operational risk is classified as a pure risk (only an opportunity of a loss), as it always leads to a financial loss for a bank. The failure to mitigate and manage operational risk effectively during past operational risk events ...
This research paper examines the profitability of commercial banks in Jordan and how it is affected by credit risk, liquidity risk, and higher capital requirements. The majority of financial institutions aim for profitability and profit maximization (Kargi, Citation 2011).
Aims & Scope. The Journal of Banking and Finance (JBF) publishes theoretical and empirical research papers spanning all the major research fields in finance and banking. The aim of the Journal of Banking and Finance is to provide an outlet for the increasing flow of scholarly research concerning financial institutions and the money and capital ...
An additional contribution of this paper is that it uses high quality bank level data from 115 countries around the world to compute some important indicators about the status of banking in these countries for the past 16 years in order to highlight the changing landscape of financial intermediation and the main functions of banks in the FinTech era.
Banks have three lines of defense for managing risk — and then regulators are the fourth line of defense. In the case of Silicon Valley Bank, all four failed. If banks want to manage risk better ...
Table 2 shows the descriptive statistics for all banks for the study period, 2000-2019. 5 The mean NPL ratio is 8.9, which is high compared to the world's average estimated at 5.03 during the same period (World Bank, 2019).Banks had an average loan growth of 13.95, and an inefficiency of 48.18, a relatively high capital adequacy ratio of 11.46 and an overall positive profitability during the ...
The present research paper provides empirical evidence on the interconnection between credit risk and bank-specific/internal factors on FP commercial banks. To analyze the data set, first, the study applies the descriptive analysis to identify the big picture of the data, then the correlation section and at the end, regression results are ...
RISK MANAGEMENT IN BANKING SECTOR. Assoc. Prof. Dr. V eclal GÜNDÜZ. Bahçeşehir Cyprus University. Banking and Finance. ORCID: 0000-0002-6002-582X. [email protected]. INTRODUCTION ...
Operating costs could in principle generate negative duration, but they are more than offset by fixed interest rate spreads that arise largely from banks' lending activity. As a result, bank franchise value declines as interest rates rise, and this decline exacerbates, rather than offsets, losses on banks' security holdings.
Such regression outcomes will enable the bank to link environmental risk factors to corporate default risk. A robustness check has also been conducted with the option of panel heteroscedasticity. The results do not change, and the crucial independent factors such as CO 2 emission, Z -score and rating remain statistically significant with ...