News: Teamscope has become Studypages Data! 🎉
News: Teamscope joins StudyPages 🎉
Data collection in the fight against COVID-19
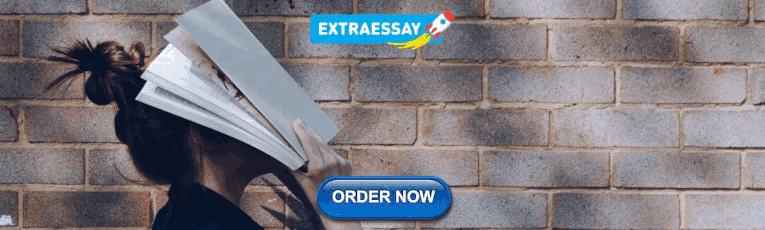
Data Sharing
6 repositories to share your research data.

Dear Diary, I have been struggling with an eating disorder for the past few years. I am afraid to eat and afraid I will gain weight. The fear is unjustified as I was never overweight. I have weighed the same since I was 12 years old, and I am currently nearing my 25th birthday. Yet, when I see my reflection, I see somebody who is much larger than reality. I told my therapist that I thought I was fat. She said it was 'body dysmorphia'. She explained this as a mental health condition where a person is apprehensive about their appearance and suggested I visit a nutritionist. She also told me that this condition was associated with other anxiety disorders and eating disorders. I did not understand what she was saying as I was in denial; I had a problem, to begin with. I wanted a solution without having to address my issues. Upon visiting my nutritionist, he conducted an in-body scan and told me my body weight was dangerously low. I disagreed with him. I felt he was speaking about a different person than the person I saw in the mirror. I felt like the elephant in the room- both literally and figuratively. He then made the simple but revolutionary suggestion to keep a food diary to track what I was eating. This was a clever way for my nutritionist and me to be on the same page. By recording all my meals, drinks, and snacks, I was able to see what I was eating versus what I was supposed to be eating. Keeping a meal diary was a powerful and non-invasive way for my nutritionist to walk in my shoes for a specific time and understand my eating (and thinking) habits. No other methodology would have allowed my nutritionist to capture so much contextual and behavioural information on my eating patterns other than a daily detailed food diary. However, by using a paper and pen, I often forgot (or intentionally did not enter my food entries) as I felt guilty reading what I had eaten or that I had eaten at all. I also did not have the visual flexibility to express myself through using photos, videos, voice recordings, and screen recordings. The usage of multiple media sources would have allowed my nutritionist to observe my behaviour in real-time and gain a holistic view of my physical and emotional needs. I confessed to my therapist my deliberate dishonesty in completing the physical food diary and why I had been reluctant to participate in the exercise. My therapist then suggested to my nutritionist and me to transition to a mobile diary study. Whilst I used a physical diary (paper and pen), a mobile diary study app would have helped my nutritionist and me reach a common ground (and to be on the same page) sooner rather than later. As a millennial, I wanted to feel like journaling was as easy as Tweeting or posting a picture on Instagram. But at the same time, I wanted to know that the information I provided in a digital diary would be as safe and private as it would have been as my handwritten diary locked in my bedroom cabinet. Further, a digital food diary study platform with push notifications would have served as a constant reminder to log in my food entries as I constantly check my phone. It would have also made the task of writing a food diary less momentous by transforming my journaling into micro-journaling by allowing me to enter one bite at a time rather than the whole day's worth of meals at once. Mainly, the digital food diary could help collect the evidence that I was not the elephant in the room, but rather that the elephant in the room was my denied eating disorder. Sincerely, The elephant in the room
Why share research data?
Sharing information stimulates science. When researchers choose to make their data publicly available, they are allowing their work to contribute far beyond their original findings.
The benefits of data sharing are immense. When researchers make their data public, they increase transparency and trust in their work, they enable others to reproduce and validate their findings, and ultimately, contribute to the pace of scientific discovery by allowing others to reuse and build on top of their data.
"If I have seen further it is by standing on the shoulders of Giants." Isaac Newton, 1675.
While the benefits of data sharing and open science are categorical, sadly 86% of medical research data is never reused . In a 2014 survey conducted by Wiley with over 2000 researchers across different fields, found that 21% of surveyed researchers did not know where to share their data and 16% how to do so.
In a series of articles on Data Sharing we seek to break down this process for you and cover everything you need to know on how to share your research outputs.
In this first article, we will introduce essential concepts of public data and share six powerful platforms to upload and share datasets.
What is a Research Data Repository?
The best way to publish and share research data is with a research data repository. A repository is an online database that allows research data to be preserved across time and helps others find it.
Apart from archiving research data, a repository will assign a DOI to each uploaded object and provide a web page that tells what it is, how to cite it and how many times other researchers have cited or downloaded that object.
What is a DOI?
When a researcher uploads a document to an online data repository, a digital object identifier (DOI) will be assigned. A DOI is a globally unique and persistent string (e.g. 10.6084/m9.figshare.7509368.v1) that identifies your work permanently.
A data repository can assign a DOI to any document, such as spreadsheets, images or presentation, and at different levels of hierarchy, like collection images or a specific chapter in a book.
The DOI contains metadata that provides users with relevant information about an object, such as the title, author, keywords, year of publication and the URL where that document is stored.
The International DOI Foundation (IDF) developed and introduced the DOI in 2000. Registration Agencies, a federation of independent organizations, register DOIs and provide the necessary infrastructure that allows researchers to declare and maintain metadata.
Key benefits of the DOI system:
- A more straightforward way to track research outputs
- Gives certainty to scientific work
- DOI's versioning system tracks changes to work overtime
- Can be assigned to any document
- Enables proper indexation and citation of research outputs
Once a document has a DOI, others can easily cite it. A handy tool to convert DOI's into a citation is DOI Citation Formatter .
Six repositories to share research data
Now that we have covered the role of a DOI and a data repository, below is a list of 6 data repositories for publishing and sharing research data.
1. figshare
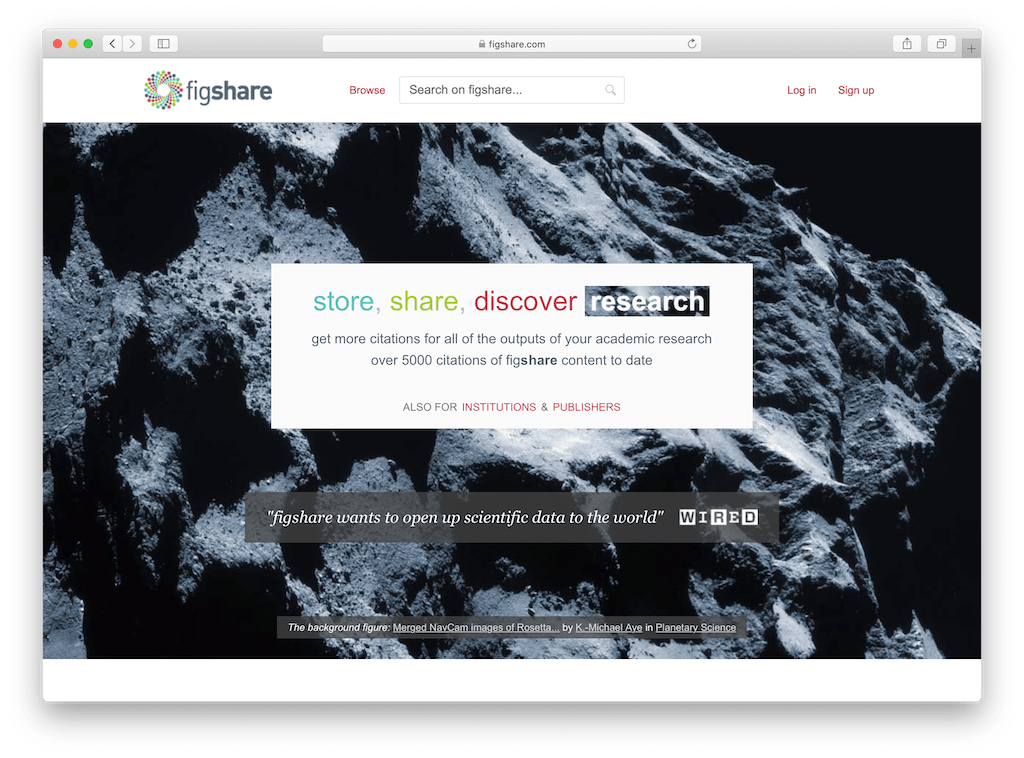
Figshare is an open access data repository where researchers can preserve their research outputs, such as datasets, images, and videos and make them discoverable.
Figshare allows researchers to upload any file format and assigns a digital object identifier (DOI) for citations.
Mark Hahnel launched Figshare in January 2011. Hahnel first developed the platform as a personal tool for organizing and publishing the outputs of his PhD in stem cell biology. More than 50 institutions now use this solution.
Figshare releases' The State of Open Data' every year to assess the changing academic landscape around open research.
Free accounts on Figshare can upload files of up to 5gb and get 20gb of free storage.
2. Mendeley Data
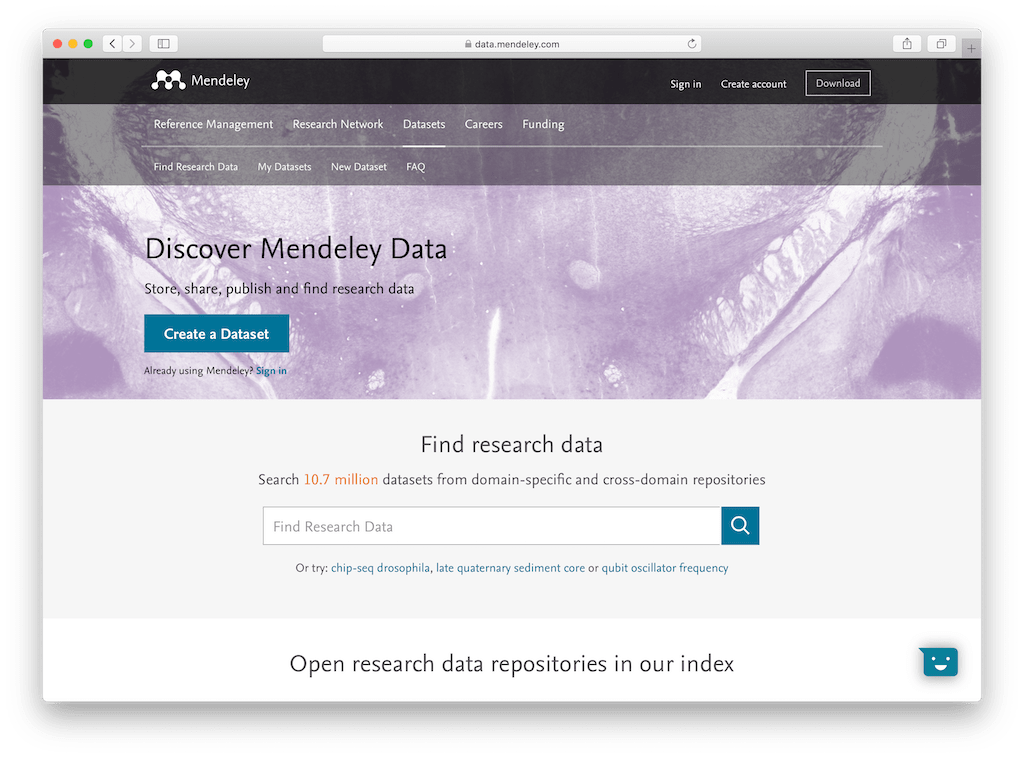
Mendeley Data is an open research data repository, where researchers can store and share their data. Datasets can be shared privately between individuals, as well as publicly with the world.
Mendeley's mission is to facilitate data sharing. In their own words, "when research data is made publicly available, science benefits:
- the findings can be verified and reproduced- the data can be reused in new ways
- discovery of relevant research is facilitated
- funders get more value from their funding investment."
Datasets uploaded to Mendeley Data go into a moderation process where they are reviewed. This ensures the content constitutes research data, is scientific, and does not contain a previously published research article.
Researchers can upload and store their work free of cost on Mendeley Data.
If appropriately used in the 21st century, data could save us from lots of failed interventions and enable us to provide evidence-based solutions towards tackling malaria globally. This is also part of what makes the ALMA scorecard generated by the African Leaders Malaria Alliance an essential tool for tracking malaria intervention globally. If we are able to know the financial resources deployed to fight malaria in an endemic country and equate it to the coverage and impact, it would be easier to strengthen accountability for malaria control and also track progress in malaria elimination across the continent of Africa and beyond.
Odinaka Kingsley Obeta
West African Lead, ALMA Youth Advisory Council/Zero Malaria Champion
There is a smarter way to do research.
Build fully customizable data capture forms, collect data wherever you are and analyze it with a few clicks — without any training required.
3. Dryad Digital Repository

Dryad is a curated general-purpose repository that makes data discoverable, freely reusable, and citable.
Most types of files can be submitted (e.g., text, spreadsheets, video, photographs, software code) including compressed archives of multiple files.
Since a guiding principle of Dryad is to make its contents freely available for research and educational use, there are no access costs for individual users or institutions. Instead, Dryad supports its operation by charging a $120US fee each time data is published.
4. Harvard Dataverse
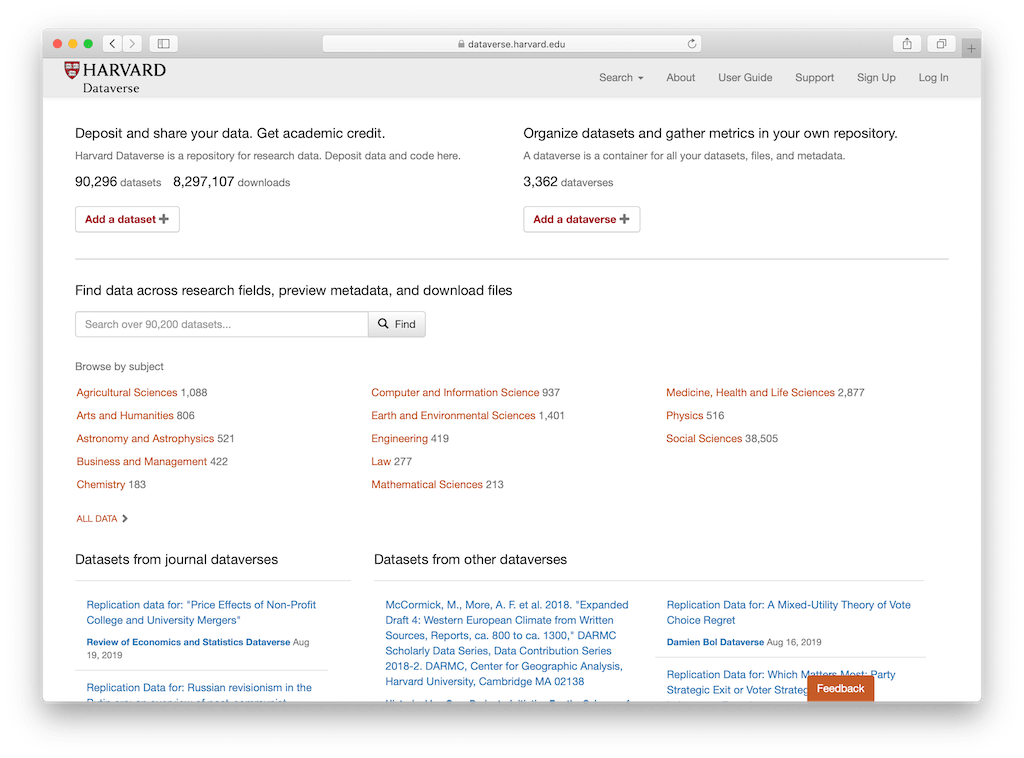
Harvard Dataverse is an online data repository where scientists can preserve, share, cite and explore research data.
The Harvard Dataverse repository is powered by the open-source web application Dataverse, developed by Insitute of Quantitative Social Science at Harvard.
Researchers, journals and institutions may choose to install the Dataverse web application on their own server or use Harvard's installation. Harvard Dataverse is open to all scientific data from all disciplines.
Harvard Dataverse is free and has a limit of 2.5 GB per file and 10 GB per dataset.
5. Open Science Framework

OSF is a free, open-source research management and collaboration tool designed to help researchers document their project's lifecycle and archive materials. It is built and maintained by the nonprofit Center for Open Science.
Each user, project, component, and file is given a unique, persistent uniform resource locator (URL) to enable sharing and promote attribution. Projects can also be assigned digital object identifiers (DOIs) if they are made publicly available.
OSF is a free service.

Zenodo is a general-purpose open-access repository developed under the European OpenAIRE program and operated by CERN.
Zenodo was first born as the OpenAire orphan records repository, with the mission to provide open science compliance to researchers without an institutional repository, irrespective of their subject area, funder or nation.
Zenodo encourages users to early on in their research lifecycle to upload their research outputs by allowing them to be private. Once an associated paper is published, datasets are automatically made open.
Zenodo has no restriction on the file type that researchers may upload and accepts dataset of up to 50 GB.
Research data can save lives, help develop solutions and maximise our knowledge. Promoting collaboration and cooperation among a global research community is the first step to reduce the burden of wasted research.
Although the waste of research data is an alarming issue with billions of euros lost every year, the future is optimistic. The pressure to reduce the burden of wasted research is pushing journals, funders and academic institutions to make data sharing a strict requirement.
We hope with this series of articles on data sharing that we can light up the path for many researchers who are weighing the benefits of making their data open to the world.
The six research data repositories shared in this article are a practical way for researchers to preserve datasets across time and maximize the value of their work.
Cover image by Copernicus Sentinel data (2019), processed by ESA, CC BY-SA 3.0 IG .
References:
“Harvard Dataverse,” Harvard Dataverse, https://library.harvard.edu/services-tools/harvard-dataverse
“Recommended Data Repositories.” Nature, https://go.nature.com/2zdLYTz
“DOI Marketing Brochure,” International DOI Foundation, http://bit.ly/2KU4HsK
“Managing and sharing data: best practice for researchers.” UK Data Archive, http://bit.ly/2KJHE53
Wikipedia contributors, “Figshare,” Wikipedia, The Free Encyclopedia, https://en.wikipedia.org/w/index.php?title=Figshare&oldid=896290279 (accessed August 20, 2019).
Walport, M., & Brest, P. (2011). Sharing research data to improve public health. The Lancet, 377(9765), 537–539. https://doi.org/10.1016/s0140-6736(10)62234-9
Foster, E. D., & Deardorff, A. (2017). Open Science Framework (OSF). Journal of the Medical Library Association : JMLA , 105 (2), 203–206. doi:10.5195/jmla.2017.88
Wikipedia contributors, "Zenodo," Wikipedia, The Free Encyclopedia, https://en.wikipedia.org/w/index.php?title=Zenodo&oldid=907771739 (accessed August 20, 2019).
Wikipedia contributors, "Dryad (repository)," Wikipedia, The Free Encyclopedia, https://en.wikipedia.org/w/index.php?title=Dryad_(repository)&oldid=879494242 (accessed August 20, 2019).
“How and Why Researchers Share Data (and Why They don't),” The Wiley Network, Liz Ferguson , http://bit.ly/31TzVHs
“Frequently Asked Questions,” Mendeley Data, https://data.mendeley.com/faq
Dear Digital Diary, I realized that there is an unquestionable comfort in being misunderstood. For to be understood, one must peel off all the emotional layers and be exposed. This requires both vulnerability and strength. I guess by using a physical diary (a paper and a pen), I never felt like what I was saying was analyzed or judged. But I also never thought I was understood. Paper does not talk back.Using a daily digital diary has required emotional strength. It has required the need to trust and the need to provide information to be helped and understood. Using a daily diary has needed less time and effort than a physical diary as I am prompted to interact through mobile notifications. I also no longer relay information from memory, but rather the medical or personal insights I enter are real-time behaviours and experiences. The interaction is more organic. I also must confess this technology has allowed me to see patterns in my behaviour that I would have otherwise never noticed. I trust that the data I enter is safe as it is password protected. I also trust that I am safe because my doctor and nutritionist can view my records in real-time. Also, with the data entered being more objective and diverse through pictures and voice recordings, my treatment plan has been better suited to my needs. Sincerely, No more elephants in this room
Diego Menchaca
Diego is the founder and CEO of Teamscope. He started Teamscope from a scribble on a table. It instantly became his passion project and a vehicle into the unknown. Diego is originally from Chile and lives in Nijmegen, the Netherlands.
More articles on
How to successfully share research data.
Harvard Dataverse
Harvard Dataverse is an online data repository where you can share, preserve, cite, explore, and analyze research data. It is open to all researchers, both inside and out of the Harvard community.
Harvard Dataverse provides access to a rich array of datasets to support your research. It offers advanced searching and text mining in over 2,000 dataverses, 75,000 datasets, and 350,000+ files, representing institutions, groups, and individuals at Harvard and beyond.
Explore Harvard Dataverse
The Harvard Dataverse repository runs on the open-source web application Dataverse , developed at the Institute for Quantitative Social Science . Dataverse helps make your data available to others, and allows you to replicate others' work more easily. Researchers, journals, data authors, publishers, data distributors, and affiliated institutions all receive academic credit and web visibility.
Why Create a Personal Dataverse?
- Easy set up
- Display your data on your personal website
- Brand it uniquely as your research program
- Makes your data more discoverable to the research community
- Satisfies data management plans
Terms to know
- A Dataverse repository is the software installation, which then hosts multiple virtual archives called dataverses .
- Each dataverse contains datasets, and each dataset contains descriptive metadata and data files (including documentation and code that accompany the data).
- As an organizing method, dataverses may also contain other dataverses.
Related Services and Tools
Research data services, qualitative research support.
What is a research repository, and why do you need one?
Last updated
31 January 2024
Reviewed by
Miroslav Damyanov
Without one organized source of truth, research can be left in silos, making it incomplete, redundant, and useless when it comes to gaining actionable insights.
A research repository can act as one cohesive place where teams can collate research in meaningful ways. This helps streamline the research process and ensures the insights gathered make a real difference.
- What is a research repository?
A research repository acts as a centralized database where information is gathered, stored, analyzed, and archived in one organized space.
In this single source of truth, raw data, documents, reports, observations, and insights can be viewed, managed, and analyzed. This allows teams to organize raw data into themes, gather actionable insights , and share those insights with key stakeholders.
Ultimately, the research repository can make the research you gain much more valuable to the wider organization.
- Why do you need a research repository?
Information gathered through the research process can be disparate, challenging to organize, and difficult to obtain actionable insights from.
Some of the most common challenges researchers face include the following:
Information being collected in silos
No single source of truth
Research being conducted multiple times unnecessarily
No seamless way to share research with the wider team
Reports get lost and go unread
Without a way to store information effectively, it can become disparate and inconclusive, lacking utility. This can lead to research being completed by different teams without new insights being gathered.
A research repository can streamline the information gathered to address those key issues, improve processes, and boost efficiency. Among other things, an effective research repository can:
Optimize processes: it can ensure the process of storing, searching, and sharing information is streamlined and optimized across teams.
Minimize redundant research: when all information is stored in one accessible place for all relevant team members, the chances of research being repeated are significantly reduced.
Boost insights: having one source of truth boosts the chances of being able to properly analyze all the research that has been conducted and draw actionable insights from it.
Provide comprehensive data: there’s less risk of gaps in the data when it can be easily viewed and understood. The overall research is also likely to be more comprehensive.
Increase collaboration: given that information can be more easily shared and understood, there’s a higher likelihood of better collaboration and positive actions across the business.
- What to include in a research repository
Including the right things in your research repository from the start can help ensure that it provides maximum benefit for your team.
Here are some of the things that should be included in a research repository:
An overall structure
There are many ways to organize the data you collect. To organize it in a way that’s valuable for your organization, you’ll need an overall structure that aligns with your goals.
You might wish to organize projects by research type, project, department, or when the research was completed. This will help you better understand the research you’re looking at and find it quickly.
Including information about the research—such as authors, titles, keywords, a description, and dates—can make searching through raw data much faster and make the organization process more efficient.
All key data and information
It’s essential to include all of the key data you’ve gathered in the repository, including supplementary materials. This prevents information gaps, and stakeholders can easily stay informed. You’ll need to include the following information, if relevant:
Research and journey maps
Tools and templates (such as discussion guides, email invitations, consent forms, and participant tracking)
Raw data and artifacts (such as videos, CSV files, and transcripts)
Research findings and insights in various formats (including reports, desks, maps, images, and tables)
Version control
It’s important to use a system that has version control. This ensures the changes (including updates and edits) made by various team members can be viewed and reversed if needed.
- What makes a good research repository?
The following key elements make up a good research repository that’s useful for your team:
Access: all key stakeholders should be able to access the repository to ensure there’s an effective flow of information.
Actionable insights: a well-organized research repository should help you get from raw data to actionable insights faster.
Effective searchability : searching through large amounts of research can be very time-consuming. To save time, maximize search and discoverability by clearly labeling and indexing information.
Accuracy: the research in the repository must be accurately completed and organized so that it can be acted on with confidence.
Security: when dealing with data, it’s also important to consider security regulations. For example, any personally identifiable information (PII) must be protected. Depending on the information you gather, you may need password protection, encryption, and access control so that only those who need to read the information can access it.
- How to create a research repository
Getting started with a research repository doesn’t have to be convoluted or complicated. Taking time at the beginning to set up the repository in an organized way can help keep processes simple further down the line.
The following six steps should simplify the process:
1. Define your goals
Before diving in, consider your organization’s goals. All research should align with these business goals, and they can help inform the repository.
As an example, your goal may be to deeply understand your customers and provide a better customer experience . Setting out this goal will help you decide what information should be collated into your research repository and how it should be organized for maximum benefit.
2. Choose a platform
When choosing a platform, consider the following:
Will it offer a single source of truth?
Is it simple to use
Is it relevant to your project?
Does it align with your business’s goals?
3. Choose an organizational method
To ensure you’ll be able to easily search for the documents, studies, and data you need, choose an organizational method that will speed up this process.
Choosing whether to organize your data by project, date, research type, or customer segment will make a big difference later on.
4. Upload all materials
Once you have chosen the platform and organization method, it’s time to upload all the research materials you have gathered. This also means including supplementary materials and any other information that will provide a clear picture of your customers.
Keep in mind that the repository is a single source of truth. All materials that relate to the project at hand should be included.
5. Tag or label materials
Adding metadata to your materials will help ensure you can easily search for the information you need. While this process can take time (and can be tempting to skip), it will pay off in the long run.
The right labeling will help all team members access the materials they need. It will also prevent redundant research, which wastes valuable time and money.
6. Share insights
For research to be impactful, you’ll need to gather actionable insights. It’s simpler to spot trends, see themes, and recognize patterns when using a repository. These insights can be shared with key stakeholders for data-driven decision-making and positive action within the organization.
- Different types of research repositories
There are many different types of research repositories used across organizations. Here are some of them:
Data repositories: these are used to store large datasets to help organizations deeply understand their customers and other information.
Project repositories: data and information related to a specific project may be stored in a project-specific repository. This can help users understand what is and isn’t related to a project.
Government repositories: research funded by governments or public resources may be stored in government repositories. This data is often publicly available to promote transparent information sharing.
Thesis repositories: academic repositories can store information relevant to theses. This allows the information to be made available to the general public.
Institutional repositories: some organizations and institutions, such as universities, hospitals, and other companies, have repositories to store all relevant information related to the organization.
- Build your research repository in Dovetail
With Dovetail, building an insights hub is simple. It functions as a single source of truth where research can be gathered, stored, and analyzed in a streamlined way.
1. Get started with Dovetail
Dovetail is a scalable platform that helps your team easily share the insights you gather for positive actions across the business.
2. Assign a project lead
It’s helpful to have a clear project lead to create the repository. This makes it clear who is responsible and avoids duplication.
3. Create a project
To keep track of data, simply create a project. This is where you’ll upload all the necessary information.
You can create projects based on customer segments, specific products, research methods , or when the research was conducted. The project breakdown will relate back to your overall goals and mission.
4. Upload data and information
Now, you’ll need to upload all of the necessary materials. These might include data from customer interviews , sales calls, product feedback , usability testing , and more. You can also upload supplementary information.
5. Create a taxonomy
Create a taxonomy to organize the data effectively by ensuring that each piece of information will be tagged and organized.
When creating a taxonomy, consider your goals and how they relate to your customers. Ensure those tags are relevant and helpful.
6. Tag key themes
Once the taxonomy is created, tag each piece of information to ensure you can easily filter data, group themes, and spot trends and patterns.
With Dovetail, automatic clustering helps quickly sort through large amounts of information to uncover themes and highlight patterns. Sentiment analysis can also help you track positive and negative themes over time.
7. Share insights
With Dovetail, it’s simple to organize data by themes to uncover patterns and share impactful insights. You can share these insights with the wider team and key stakeholders, who can use them to make customer-informed decisions across the organization.
8. Use Dovetail as a source of truth
Use your Dovetail repository as a source of truth for new and historic data to keep data and information in one streamlined and efficient place. This will help you better understand your customers and, ultimately, deliver a better experience for them.
Should you be using a customer insights hub?
Do you want to discover previous research faster?
Do you share your research findings with others?
Do you analyze research data?
Start for free today, add your research, and get to key insights faster
Editor’s picks
Last updated: 18 April 2023
Last updated: 27 February 2023
Last updated: 6 February 2023
Last updated: 5 February 2023
Last updated: 16 April 2023
Last updated: 9 March 2023
Last updated: 30 April 2024
Last updated: 12 December 2023
Last updated: 11 March 2024
Last updated: 4 July 2024
Last updated: 6 March 2024
Last updated: 5 March 2024
Last updated: 13 May 2024
Latest articles
Related topics, .css-je19u9{-webkit-align-items:flex-end;-webkit-box-align:flex-end;-ms-flex-align:flex-end;align-items:flex-end;display:-webkit-box;display:-webkit-flex;display:-ms-flexbox;display:flex;-webkit-flex-direction:row;-ms-flex-direction:row;flex-direction:row;-webkit-box-flex-wrap:wrap;-webkit-flex-wrap:wrap;-ms-flex-wrap:wrap;flex-wrap:wrap;-webkit-box-pack:center;-ms-flex-pack:center;-webkit-justify-content:center;justify-content:center;row-gap:0;text-align:center;max-width:671px;}@media (max-width: 1079px){.css-je19u9{max-width:400px;}.css-je19u9>span{white-space:pre;}}@media (max-width: 799px){.css-je19u9{max-width:400px;}.css-je19u9>span{white-space:pre;}} decide what to .css-1kiodld{max-height:56px;display:-webkit-box;display:-webkit-flex;display:-ms-flexbox;display:flex;-webkit-align-items:center;-webkit-box-align:center;-ms-flex-align:center;align-items:center;}@media (max-width: 1079px){.css-1kiodld{display:none;}} build next, decide what to build next, log in or sign up.
Get started for free
- Utility Menu

Open source research data repository software

Researchers

Institutions

Participate in a vibrant and growing community that is helping to drive the norms for sharing, preserving, citing, exploring, and analyzing research data. Contribute code extensions, documentation, testing, and/or standards. Integrate research analysis, visualization and exploration tools, or other research and data archival systems with the Dataverse Project. Want to contribute?
Dataverse Repositories - A World View
A2bd36de0711ca11586e77791213138c.
View more Metrics
Dataverse Software 6.3 Release
Dataverse 6.3 is now available. Many thanks to all of the community members who contributed code, suggestions, bug reports, and other assistance across the project.
Release Overview
This release contains a number of updates and new features including:
- New Contributor Guide . The UX Working Group released a new Dataverse Contributor Guide .
- Search Performance Improvements...
Announcing Dataverse 6.2
Dataverse 6.2 is now available! Thank you to the community members who contributed code, suggestions, bug reports, and other assistance across the project.
- Search and Facet by License. Licenses have been added to the search facets in the search side panel to filter datasets by license (e.g. CC0). Licenses can also be used to filter the Search API results.
- When Returning Datasets to Authors, Reviewers Can Add a Note to the Author.
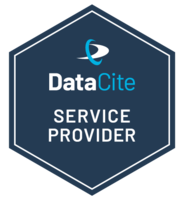
Thank you for visiting nature.com. You are using a browser version with limited support for CSS. To obtain the best experience, we recommend you use a more up to date browser (or turn off compatibility mode in Internet Explorer). In the meantime, to ensure continued support, we are displaying the site without styles and JavaScript.
- View all journals
- Explore content
- About the journal
- Publish with us
- Sign up for alerts
- Open access
- Published: 29 August 2023
re3data – Indexing the Global Research Data Repository Landscape Since 2012
- Heinz Pampel ORCID: orcid.org/0000-0003-3334-2771 1 , 2 ,
- Nina Leonie Weisweiler ORCID: orcid.org/0000-0001-6967-9443 2 ,
- Dorothea Strecker ORCID: orcid.org/0000-0002-9754-3807 1 ,
- Michael Witt 3 ,
- Paul Vierkant 4 ,
- Kirsten Elger 5 ,
- Roland Bertelmann 2 ,
- Matthew Buys 4 ,
- Lea Maria Ferguson 2 ,
- Maxi Kindling 6 ,
- Rachael Kotarski ORCID: orcid.org/0000-0001-6843-7960 7 &
- Vivien Petras 1
Scientific Data volume 10 , Article number: 571 ( 2023 ) Cite this article
3541 Accesses
2 Citations
25 Altmetric
Metrics details
For more than ten years, re3data, a global registry of research data repositories (RDRs), has been helping scientists, funding agencies, libraries, and data centers with finding, identifying, and referencing RDRs. As the world’s largest directory of RDRs, re3data currently describes over 3,000 RDRs on the basis of a comprehensive metadata schema. The service allows searching for RDRs of any type and from all disciplines, and users can filter results based on a wide range of characteristics. The re3data RDR descriptions are available as Open Data accessible through an API and are utilized by numerous Open Science services. re3data is engaged in various initiatives and projects concerning data management and is mentioned in the policies of many scientific institutions, funding organizations, and publishers. This article reflects on the ten-year experience of running re3data and discusses ten key issues related to the management of an Open Science service that caters to RDRs worldwide.
Similar content being viewed by others
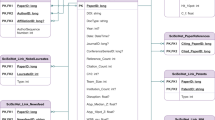
SciSciNet: A large-scale open data lake for the science of science research
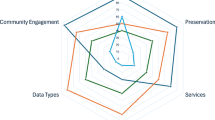
Biomedical Data Repository Concepts and Management Principles
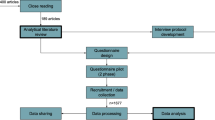
A dataset describing data discovery and reuse practices in research
Introduction.
In the 2010s, making research data publicly accessible gained importance: Terms such as e-science 1 and cyberscience 2 were shaping discourses about scientific work in the digital age. Various discussions within the scientific community 3 , 4 , 5 , 6 , 7 , 8 resulted in an increased awareness of the value of permanent access to research data. Policy recommendations of the Organization for Economic Co-operation and Development (OECD) 9 or the European Commission 10 reflected this shift.
The need for professional data management was increasingly emphasized with the publication of the now widely recognized FAIR Data Principles 11 . Researchers, academic institutions, and funders started to address this issue in policies 12 , initiatives and networks 13 , 14 , 15 , and infrastructures 16 , 17 , 18 , 19 . For example, the National Science Foundation (NSF) in the United States published a Data Sharing Policy in 2011, in which the funding agency required beneficiaries to provide information about data handling in a Data Management Plan 20 . In Germany, the German Research Foundation (DFG) published a similar statement regarding access to research data in the 2010s 21 , 22 .
The handling of research data was also discussed in library and computing center communities: In 2009, the German Initiative for Networked Information (DINI), a network of information infrastructure providers, published a position paper on the need for research data management (RDM) at higher education institutions 23 . Through the discussions within DINI, the need for a registry of RDRs became evident. At the time, the Directory of Open Access Repositories (OpenDOAR) 24 had already established itself as a directory of subject and institutional Open Access repositories. However, there was no comparable directory for RDRs, and it remained unclear how many repositories dedicated to research data existed.
In 2011, a consortium of research institutions in Germany submitted a proposal to the German Research Foundation (DFG), asking for funding to develop ‘re3data – Registry of Research Data Repositories’ 25 . Members of the consortium were the Karlsruhe Institute of Technology (KIT), the Humboldt-Universität zu Berlin, and the Helmholtz Open Science Office at the GFZ German Research Centre for Geosciences. The DFG approved the proposal in the same year. The project aimed to develop a service that would help researchers identify suitable RDRs to store their research data. re3data went online in 2012, and already listed 400 RDRs one year later 26 .
While working on the registry, the project team in Germany became aware of a similar initiative in the USA. With support from the Institute of Museum and Library Services, Purdue and Pennsylvania State University libraries developed Databib, a ‘curated, global, online catalog of research data repositories’ 27 . Databib went online in the same year 28 . At the time, RDRs were indexed and curated by library staff at re3data partner institutions, whereas Databib had established an international editorial board to curate RDR descriptions 27 . Databib and re3data signed a Memorandum of Understanding in 2012, and, following excellent cooperation, the two services merged in 2014 29 . The merger brought together successful ideas from each service: The metadata schemas were combined, resulting in version 2.2 of the re3data metadata schema 30 , and the sets of RDR descriptions were merged. The international editorial board of Databib was expanded to include re3data editors. Development of the IT infrastructure of re3data continued, combining the expertise both services had built. For operating the service, a management duo was installed, comprising a member each from institutions representing re3data and Databib.
The two services have always been closely corresponding with DataCite, an international not-for-profit organization that aims to ensure that research outputs and resources are openly available and connected so that their reuse can advance knowledge across and between disciplines, now and in the future 31 . In this process, the main objective was to cover the interests of the global community of operators more comprehensively. In 2015, the DataCite Executive Board and the General Assembly decided to enter into an agreement with re3data, making re3data a DataCite partner service 29 . In 2017, re3data won the Oberly Award for Bibliography in the Agricultural or Natural Sciences from the American Libraries Association 32 .
Today, re3data is the largest directory of RDRs worldwide, indexing over 3,000 RDRs as of March 2023. re3data is widely used by academic institutions, funding organizations, publishers, journals, and various other stakeholders, such as the European Open Science Cloud (EOSC) and the National Research Data Infrastructure in Germany (NFDI). re3data metadata is also used to monitor and study the landscape of RDRs, and it is reused by numerous tools and services. Third-party-funded projects support the continuous development of the service. Currently, the DFG is funding the development of the service within the project re3data COREF 33 , 34 . In addition, the project partners DataCite and KIT bring the re3data perspective into EOSC projects such as FAIRsFAIR (completed) 35 and FAIR-IMPACT 29 .
This article outlines the decade-long experience of managing a widely used registry that supports a diverse and global community of stakeholders. The article is clustered around ten key issues that have emerged over time. For each of the ten issues, we first present a brief definition from the perspective of re3data. We then describe our approach to addressing the issue, and finally, we offer a reflection on our work.
The section outlines ten key issues that have emerged in the last ten years of operating re3data.
For re3data, Open Science means providing unrestricted access to the re3data metadata and schema, transparency of the indexing process, as well as open communication with the community of global RDRs.
At all times, re3data has been committed to Open Science by striving to be transparent and by sharing metadata. The openness of re3data pertains not only to the handling of its metadata and the associated infrastructure, but also to collaborative engagements with the community of research data stewards and other stakeholders in the field of research data management.
An example of this is the development of the re3data metadata schema: The initial version of the schema integrated a request for comments that allowed stakeholders to offer suggestions and improvements 26 . This participatory approach, accompanied by a public relations campaign, has yielded positive outcomes. Numerous experts engaged in the request for comments and contributed their perspective and expertise. Based on the positive feedback, we subsequently integrated a participatory phase in further updates of the metadata schema 30 , 36 .
In addition to this general commitment to openness, re3data has made its metadata available under the Creative Commons deed CC0. Due to adopting this highly permissive license, re3data metadata is strongly utilized by other parties, thereby enabling the development of new and innovative services and tools. Moreover, adaptable Jupyter Notebooks 37 have been published to facilitate the use of the re3data metadata. Additionally, workshops 38 have been arranged to support individuals in working with the notebooks and re3data data in general.
As a registry of RDRs, re3data also promotes Open Science by helping researchers find suitable repositories for publishing their data. For researchers who are looking for a repository that supports Open Science practices, re3data offers concise information on repository openness via its icon system. A recent analysis showed that most repositories indexed in re3data are considered ‘open’ 39 .
Lessons learned
The extensive reuse of re3data metadata increases its overall value, and participatory phases allow for incorporating different perspectives and experiences.
Quality assurance
For re3data, quality assurance encompasses all processes to ensure a service that meets the needs of a global community, as well as verifiably high-quality information.
High-quality RDR descriptions are at the core of re3data. Therefore, continuous efforts ensure that re3data metadata describes appropriately and correctly. Figure 1 shows the editorial process in re3data. Anyone, for example RDR operators, can submit repositories to be indexed in re3data by providing the repository name, URL, and some other core properties via a web form 40 . The re3data editorial board analyzes if the suggested RDR conforms with the re3data registration policy 40 . The policy requires that the RDR is operated by a legal entity, such as a library or university, and that the terms of use are clearly communicated. Additionally, the RDR must have a focus on storing and providing access to research data. If an RDR meets these requirements, it is indexed based on the re3data metadata schema. A member of the editorial board creates an initial RDR description, which is then reviewed by another editor. This approach has proven effective in resolving any inconsistencies in interpreting RDR characteristics. An indexing manual explains how the schema is to be applied and helps to ensure consistency between RDR descriptions. Once this review is complete, the RDR description is made publicly visible.
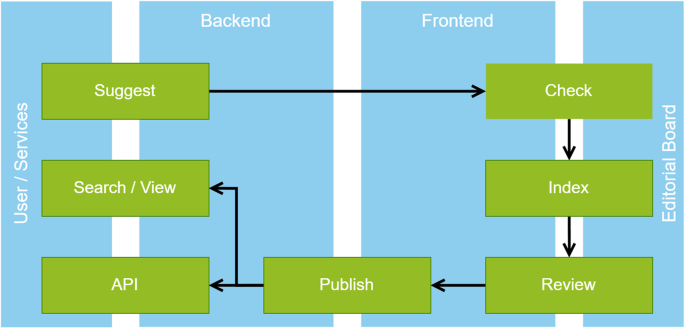
Schematic overview of the editorial process in re3data.
re3data applies a number of measures to ensure the long-term quality and consistency of RDR descriptions, including automated quality checks. For example, it is periodically checked whether the URLs of the RDR still resolve – if not, the entry of a RDR is reexamined. Figure 2 shows a screenshot of a re3data RDR description.
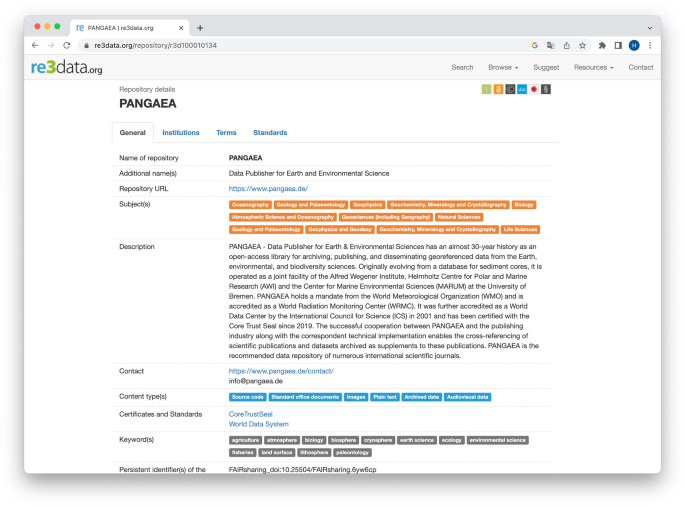
Screenshot of the re3data description of the research data repository PANGAEA 97 .
The re3data metadata schema on which RDR descriptions are based is reviewed and updated regularly to ensure that users’ changing information needs are met. Operators of an RDR, as well as any other person, can suggest changes to RDR descriptions by submitting a change request. A link for filing a change request can be found at the bottom of each RDR description in re3data. Once a change request has been submitted, a member of the editorial board will review the proposed changes and verify them against information on the RDR website. If the change request is deemed valid, the RDR description will be adapted accordingly.
As part of the project re3data COREF, quality assurance practices at RDRs were systematically investigated. The aim was to understand how RDRs ensure high-quality data, and to better reflect these measures in the metadata schema. The results of the study 41 , which were based on a survey among RDR operators, show that approaches to quality assurance are diverse and depend on the mission and scope of the RDR. However, RDRs are key actors in enabling quality assurance. Furthermore, there is a path dependence of data review on the review process of textual publications. In addition to the study, a workshop 42 , 43 was held with CoreTrustSeal that focused on quality assurance measures RDRs have implemented. CoreTrustSeal is a RDR certification organization launched in 2017 that defines requirements for base-level certification for RDRs 44 .
Combining manual and automated verification was shown to be most effective in ensuring that RDR descriptions remain consistent while meeting users’ diverse information needs.
Community engagement
For re3data, community engagement encompasses all activities that ensure interaction with the global RDR community in a participatory process.
Collaboration has always been a central principle for re3data. This is reflected in the fact that research communities, RDR providers, and other relevant stakeholders contribute significantly to the completeness and accuracy of the re3data metadata as well as its further technical and conceptual development. Examples include the participatory phase during the revision of the metadata schema, the involvement of important stakeholders in the development of the re3data Conceptual Model for User Stories 45 , 46 , or the activities that investigate data quality assurance at RDRs.
re3data engages in collaborations in various forms with diverse stakeholders, for example:
In collaboration with the Canadian Data Repositories Inventory Project and later with the Digital Research Alliance of Canada, both initiatives aiming at describing the Canadian landscape of RDRs comprehensively, descriptions of Canadian RDRs in re3data were improved, and additional RDRs were indexed 47 , 48 .
A collaboration initiative was initiated in Germany with the Helmholtz Metadata Collaboration (HMC). In this initiative, the descriptions of research data infrastructures within the Helmholtz Association are being reviewed and enhanced 49 .
re3data also engages in international networks, particularly within the Research Data Alliance (RDA). Activities focus on several RDA working and interest groups 50 , 51 , 52 that touch on topics relevant to RDR registries.
Combining strategies of engagement connects the service to its stakeholders and creates opportunities for collaboration and innovation.
Interoperability
For re3data, interoperability means facilitating interactions and metadata exchange with the global RDR community by relying on established standards.
Interoperability is a necessary condition to integrate a service into a global network of diverse stakeholders. International standards must be implemented to achieve this, for example with the re3data API 53 . The API can be used to query various parameters of an RDR as expressed in the metadata schema. The API enables the machine readability and integration of re3data metadata into other services. The re3data API is based on the RESTful API concept and is well-documented. Applying the HATEOAS principles 54 enables the decoupling of clients and servers, and thus allows for independent development of server functionality. This results in a robust interface that promotes interoperability and reduces barriers to future use. Also, re3data supports OpenSearch, a standard that enables interaction with search results in a format suitable for syndication and aggregation.
Interoperability also guides the development of the metadata schema: Established vocabularies and standards are used to describe RDRs wherever possible. Examples of standards used in the metadata schema include:
ISO 639-3 for language information, for example a RDR name
ISO 8601 for the use of date information on a RDR
DFG Classification of Subject Areas for subject information on a RDR
In addition, re3data pursues interoperability by jointly working on a mapping between the DFG Classification of Subject Areas used by re3data and the OECD Fields of Science classification used by DataCite 55 .
re3data records whether an RDR has obtained formal certification, for example by World Data System (WDS) or CoreTrustSeal. The certification status, along with other properties, is visualized by the re3data icon system that makes the core properties of RDRs easily accessible visually. The icon system provides information about the openness of the RDR and its data collection, the use of PID systems, as well as the certification status. The icon system can also be integrated into RDR websites via badges 56 . Figure 3 shows an example of a re3data badge.
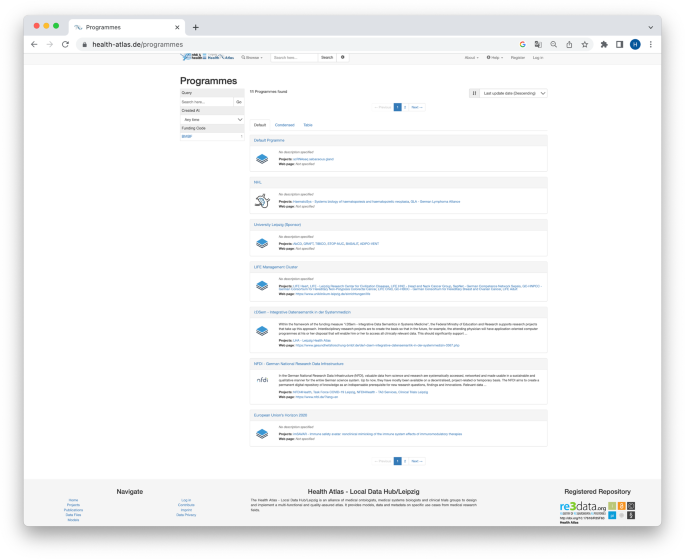
The re3data badge integrated in the research data repository Health Atlas.
re3data captures information that might be relevant to metadata aggregator services, including API URLs, as well as the metadata standard(s) used. In offering this information in a standardized form, re3data fosters the development of services that span multiple collections, such as data portals. For example, as part of the FAIRsFAIR project work, re3data metadata has been integrated into DataCite Commons 57 to embed repository information in the DataCite PID Graph. This step not only improves the discoverability of repositories that support research data management in accordance with the FAIR principles but also serves as a basis for the development of new services such as the FAIR assessment tool F-UJI 35 , 58 .
The adherence to established standards facilitates the reuse of re3data metadata and increases the integration of the service into the broader Open Science landscape.
Developement
For re3data, continuous development ensures that the service is able to respond dynamically to evolving requirements of the global RDR community.
Maintaining a registry for an international community poses a significant challenge, particularly the continued provision of reliable technical operations and a governance structure capable of responding adequately to user demands. re3data has found suitable solutions to these challenges, which have enabled the service to be in operation for more than ten years. The long-standing collaboration with DataCite has contributed to this success. Participation in third-party-funded projects has facilitated the collaborative development of core service elements together with partners. Participation in committees such as those surrounding EOSC and RDA, as well as active engagement with the RDR community, have motivated discussions about changing requirements and led to the continuous evolution of the registry.
Responsibilities for specific tasks are divided among several entities, such as a working group responsible for guiding future directions of the service and the editorial board responsible for maintaining re3data metadata. In addition, there are teams responsible for technology as well as for outreach and communication. The working group includes experts from DataCite and other stakeholders, who discuss current requirements, prioritize developments, and ensure coordination with RDR operators worldwide. In addition to these entities, coordination with third-party-funded projects involving re3data is ongoing.
Continuous and agile development addresses the users’ constantly evolving needs. Operating a registry that meets those needs in the long term requires flexibility.
Sustainability
For re3data, sustainability means ensuring a long-term and reliable service to the global RDR community.
Maintaining the sustainable operation of a service like re3data beyond an initial project phase is a challenge. For re3data, the consortium model has proven effective, as the service is supported by a wide range of scientific institutions. This model, which is embedded in the governance of re3data, allows the operation of the service to be sustained through self-funding while also enabling important developments to be undertaken within the scope of third-party projects. Thanks to funding received from the DFG (re3data COREF project) and the European Union’s Horizon 2020 program (FAIRsFAIR project), significant investments have been made in the IT infrastructure and overall advancement of the service in recent years.
A strategy based on diverse revenue streams contributes to securing funding for the service long-term.
For re3data, being mentioned in policies comes with a responsibility for operating a reliable service and maintaining high-quality metadata for the global RDR community.
During the development of the re3data service, the partners engaged in dialogues with various stakeholders that were interested in using the registry to refer to RDRs in their policies. They might do this, for example, to recommend or mandate the use of RDRs in general for publishing research data, or the use of a specific RDR. Today, re3data is mentioned in the policies of several funding agencies, scientific institutions, and journals. These actors use re3data to identify RDRs operated by specific academic institutions that were developed using funding from a funding organization, or that store data that are the basis of a journal article. Examples of policies and policy guidance documents that refer to re3data:
Academic institutions:
Brandon University, Canada 59
Technische Universität Berlin, Germany 60
University of Edinburgh, United Kingdom 61
University of Eastern Finland 62
Western Norway University of Applied Sciences 63
Bill & Melinda Gates Foundation, USA 64
European Commission 65 and ERC, EU 66
National Science Foundation (NSF), USA 67
NIH, USA 68
Journals and Publishers:
Taylor & Francis, United Kingdom 69
Springer Nature, United Kingdom 70
Sage, United Kingdom 71
Wiley, Germany 72
Regular searches are conducted to track mentions of re3data in policies. On the re3data website, a list of policies referring to re3data is maintained and regularly updated 73 .
As a result of being mentioned in policies so frequently, re3data receives inquiries from researchers for information on listed RDRs almost daily. These inquiries are usually forwarded to the RDR directly.
Policies represent firm support for research data management by academic institutions, funders, and journals and publishers. By facilitating the search for and referencing of RDRs in policies, re3data further promotes Open Science practices.
For re3data, data reuse is one of the main objectives, ensuring that third parties can rely on re3data metadata to build services that support the global RDR community.
Because re3data metadata are published as open data, third parties are free to integrate it into their systems. Several service operators have already taken advantage of this opportunity. In general, there are three types of services that work with re3data data:
Services for finding and describing RDRs: These services usually work with a subset of re3data metadata. Sometimes, the data is manually curated, and then integrated into external services based on specific parameters. Examples include:
DARIAH-EU has developed its Data Deposit Recommendation Service based on a subset of re3data metadata, which helps humanities researchers find suitable RDRs 74 , 75 .
The American Geophysical Union (AGU) has utilized re3data metadata to create a dedicated gateway for RDRs in the geosciences with its Repository Finder tool 76 , 77 , which was later incorporated into the DataCite Commons web search interface.
Services for monitoring the landscape of RDRs: These services analyze re3data metadata using specific parameters and visualize the results. Examples include:
OpenAIRE has integrated re3data metadata into its Open Science Observatory to provide information on RDRs that are part of OpenAIRE 78 .
The European Commission operates the Open Science Monitor, a dashboard that analyzes re3data metadata. The following metrics are displayed: number of RDRs by subject, number of RDRs by access type, and number of RDRs by country 79 , 80 .
Services for assessing RDRs: These services use re3data metadata and other data sources to evaluate RDRs more comprehensively. Examples include:
The F-UJI Automated FAIR Data Assessment Tool is a web-based service that assesses the degree to which individual datasets conform to the FAIR Data principles. The tool utilizes re3data metadata to evaluate characteristics of the RDR that store the datasets 81 .
Charité Metrics Dashboard, a dashboard on responsible research practices from the Berlin Institute of Health at Charité in Berlin, Germany, builds on F-UJI data and combines this information with additional re3data metadata 82 .
These examples underscore the value Open Science tools like re3data generate by making their data openly available without restrictions. As a result of the permissive licensing, re3data metadata can be used for new and innovative applications, establishing re3data as a vital data provider for the global Open Science community.
Permissive licensing and extensive collaboration have turned re3data into a key data provider in the Open Science ecosystem.
Metadata for research
For re3data, providing RDR descriptions also means offering metadata that enables analyses of the global RDR community.
In research disciplines studying data infrastructures, for example library and information science or science and technology studies, re3data is regularly used for information on the state of research infrastructures. As re3data has been mapping the landscape of data infrastructures for ten years, it has evolved into a tool that is used for monitoring Open Science activities, research data management, and other topics. Studies reusing re3data metadata include analyses of the overall RDR landscape, the landscape of RDRs in a specific domain, or the RDR landscape of a region or country. Some examples of studies reusing re3data metadata for research are:
Overall studies: Boyd 83 examined the extent to which RDR exhibit properties of infrastructures. Khan & Ahangar 84 and Hansson & Dahlgren 85 focused on the openness of RDRs from a global perspective.
Regional studies: Bauer et al . 86 examined Austrian RDRs, Cho 87 Asian RDRs, Milzow et al . 88 Swiss RDRs, and Schöpfel 89 French RDRs.
Domain studies: Gómez et al . 90 and Li & Liu 91 investigated the landscape of RDRs in humanities and social science. Prashar & Chander 92 focused on computer science.
Members of the re3data team have also published studies reusing re3data metadata, including studies of the global state of RDR 93 , openness 39 , and quality assurance of RDRs 41 .
In response to the demand for information on the RDR landscape, the re3data graphical user interface provides various visualizations of the current state of RDRs. For example, re3data metadata can be browsed visually by subject category and on a map. In addition, the metrics page of re3data shows how RDRs are distributed across central properties of the metadata schema 94 .
The start page of re3data includes a recommendation for how to cite the service if it was used as a source in papers:
re3data - Registry of Research Data Repositories. https://doi.org/10.17616/R3D last accessed: [date].
In citing the service, the use of re3data as a data source in research and the service in general becomes more visible.
The increasing number of studies reusing re3data metadata shows a real demand for reliable information on the global RDR landscape.
Communications
For re3data, communication means engaging in dialogue with relevant stakeholders in the global RDR community.
Broad-based public relations are very important for a service catering to a global community. In recent years, re3data has pursued a communication strategy that includes the following elements:
Conference presentations: It has been proven effective to represent the service at conferences, paving new ways to engage with the community.
Mailing lists: The re3data team regularly informs members of a variety of mailing lists about news from the service.
Social media: re3data communicates current developments via Mastodon ( https://openbiblio.social/@re3data ) and Twitter ( https://twitter.com/re3data ).
Help desk: Communication via the help desk is essential for the re3data service. The help desk team answers questions about RDR descriptions, as well as general questions about data management. The number of general inquiries, e.g., for finding a suitable RDR, has increased over the years.
Blog: The project re3data COREF operates a blog that informs about developments in the project 95 . Some blog posts are also published in the DataCite Blog 96 .
Establishing broad-based communication channels enables the service to reach and engage with relevant stakeholders in a variety of formats.
Over the past ten years, re3data has evolved into a reliable and valuable Open Science service. The service offers high-quality RDR descriptions from all disciplines and regions. re3data is managed cooperatively; new features are developed in third-party projects.
Four basic principles guide the development of re3data: openness, community engagement, high-quality metadata, and ongoing consideration of users’ needs. These principles ensure that the activities of the service align with the values and interests of its stakeholders. In the context of these principles, ten key issues for the operation of the service have emerged over the last ten years.
In the past two years, following in-depth conversations with diverse parties, a new conceptual model for re3data was developed 45 . This process contributed to a better understanding of the needs of RDR operators and other stakeholders. The conceptual model will guide developments of re3data, embedding the service further in the evolving ecosystem of Open Science services with the intention to support researchers, scientific institutions, funding organizations, publishers, and journals in implementing the FAIR principles and realizing an interconnected global research data ecosystem.
This article describes the history and current status of the global registry re3data. Based on operational experience, it reflects on some of the basic principles that have shaped the service since its inception.
Having been launched more than ten years ago, re3data is now the most comprehensive registry of RDRs. The service currently describes more than 3,000 RDRs and caters to a diverse user base including RDR operators, researchers, funding agencies, and publishers. Ten key issues that are relevant for operating an Open Science service like re3data are identified, discussed, and reflected: openness, quality assurance, community engagement, interoperability, development, sustainability, policies, data reuse, metadata for research, and communications. For each of the key issues, we provide a definition, explain the approach applied by the re3data service, and describe what the re3data team learned from working on each issue.
Among other aspects, the paper outlines the design, governance, and objectives of re3data, providing important background information on a service that has evolved into a central data source on the global RDR landscape.
Data availability
The re3data RDR descriptions are openly available via https://re3data.org under a CC0 deed.
Code availability
The source code of the directory is not publicly released. The re3data subject ontology and several Jupyter notebooks with examples for using the re3data API can be found at: https://github.com/re3data .
National Science Foundation Cyberinfrastructure Council. Cyberinfrastructure Vision for 21st Century Discovery 2007 . https://www.nsf.gov/pubs/2007/nsf0728/nsf0728.pdf (2023).
National Science Foundation. Revolutionizing Science and Engineering through Cyberinfrastructure: Report of the National Science Foundation Blue-Ribbon Advisory Panel on Cyberinfrastructure 2003 . https://www.nsf.gov/cise/sci/reports/atkins.pdf (2023).
How to encourage the right behaviour. Nature 416 , 1–1 (2002).
Let Data Speak to Data. Nature 438 , 531–531 (2005).
The Royal Society. Science as an Open Enterprise https://royalsociety.org/~/media/Royal_Society_Content/policy/projects/sape/2012-06-20-SAOE.pdf (2023).
Data for the masses. Nature 457 , 129–129 (2009).
Data’s shameful neglect. Nature 461 , 145–145 (2009).
Science Staff. Challenges and opportunities. Science 331 , 692–693 (2011).
Article Google Scholar
OECD. OECD Principles and Guidelines for Access to Research Data from Public Funding (2007).
European Commission. Commission Recommendation of 17 July 2012 on Access to and Preservation of Scientific Information (2012).
Wilkinson, M. D. et al . The FAIR guiding principles for scientific data management and stewardship. Scientific Data 3 , 160018 (2016).
Pampel, H. & Bertelmann, R. in Handbuch Forschungsdatenmanagement (2011) . https://opus4.kobv.de/opus4-fhpotsdam/frontdoor/index/index/docId/195 (2023).
European Commission. European Cloud Initiative - Building a Competitive Data and Knowledge Economy in Europe (2016).
Michener,W. et al . DataONE: Data observation network for earth preserving data and enabling innovation in the biological and environmental sciences. D-Lib Magazine 17 (2011).
Parsons, M. A. The Research Data Alliance: Implementing the technology, practice and connections of a data infrastructure. Bul. Am. Soc. Info. Sci. Tech. 39 , 33–36 (2013).
Borgman, C. L. Big Data, Little Data, No Data: Scholarship in the Networked World (The MIT Press, 2016).
Manghi, P., Manola, N., Horstmann, W. & Peters, D. An Infrastructure for Managing EC Funded Research Output - The OpenAIRE Project 2010 . https://publications.goettingen-research-online.de/handle/2/57068 (2023).
Blanke, T., Bryant, M., Hedges, M., Aschenbrenner, A. & Priddy, M. Preparing DARIAH in 2011 IEEE Seventh International Conference on eScience , 158–165 (IEEE, 2011).
Hey, T. & Trefethen, A. in Scientific Collaboration on the Internet (eds Olson, G. M., Zimmerman, A. & Bos, N.) 14–31 (The MIT Press, 2008).
National Science Board. Digital Research Data Sharing and Management 2011 . https://www.nsf.gov/nsb/publications/2011/nsb1124.pdf (2023).
Deutsche Forschungsgemeinschaft. Empfehlungen Zur Gesicherten Aufbewahrung Und Bereitstellung Digitaler Forschungsprimar daten 2009 . https://www.dfg.de/download/pdf/foerderung/programme/lis/ua_inf_empfehlungen_200901.pdf (2023).
Allianz der deutschen Wissenschaftsorganisationen. Grundsatze Zum Umgang Mit Forschungsdaten https://doi.org/10.2312/ALLIANZOA.019 (2010).
DINI Working Group Electronic Publishing. Positionspapier Forschungsdaten. https://doi.org/10.18452/1489 (2009).
OpenDOAR. https://beta.jisc.ac.uk/opendoar (2023).
Deutsche Forschungsgemeinschaft. Re3data.Org - Registry of Research Data Repositories. Community Building, Net working and Research Data Management Services GEPRIS . https://gepris.dfg.de/gepris/projekt/209240528?context=projekt&task=showDetail&id=209240528& (2023).
Pampel, H. et al . Making research data repositories visible: The re3data.org registry. PLoS ONE 8 (ed Suleman, H.) e78080 (2013).
Witt, M. Databib: Cataloging the World’s Data Repositories 2013 . https://ir.inflibnet.ac.in:8443/ir/handle/1944/1778 (2023).
Witt, M. & Giarlo, M. Databib: IMLS LG-46-11-0091-11 Final Report (White Paper) 2012 . https://docs.lib.purdue.edu/libreports/2 (2023).
Buys, M. Strategic Collaboration 2022 . https://datacite.org/assets/re3data%20and%20DataCite_openHours.pdf (2023).
Vierkant, P. et al . Metadata Schema for the Description of Research Data Repositories: version 2.2 . https://doi.org/10.2312/RE3.006 (2014).
Brase, J. DataCite - A Global Registration Agency for Research Data in 2009 Fourth International Conference on Cooperation and Promotion of Information Resources in Science and Technology , 257–261 (IEEE, 2009).
Witt, M. DataCite’s Re3data Wins Oberly Award from the American Libraries Association https://doi.org/10.5438/0001-0HN* .
Deutsche Forschungsgemeinschaft. Re3data – Offene Und Nutzerorientierte Referenz Fur Forschungsdatenrepositorien (Re3data COREF) GEPRIS . https://gepris.dfg.de/gepris/projekt/422587133?context=projekt&task=showDetail&id=422587133& (2023).
re3data COREF. Re3data COREF Project https://coref.project.re3data.org/project (2023).
FAIRsFAIR. Repository Discovery in DataCite Commons https://www.fairsfair.eu/repository-discovery-datacite-commons (2023).
Strecker, D. et al . Metadata Schema for the Description of Research Data Repositories: version 3.1 . https://doi.org/10.48440/RE3.010 (2021).
re3data. Examples for using the re3data API GitHub . https://github.com/re3data/using_the_re3data_API (2023).
Schabinger, R., Strecker, D., Wang, Y. & Weisweiler, N. L. Introducing Re3data – the Registry of Research Data Repositories . https://doi.org/10.5281/ZENODO.5592123 (2021).
re3data COREF. How Open Are Repositories in Re3data? https://coref.project.re3data.org/blog/how-open-are-repositories-in-re3data (2023).
re3data. Suggest https://www.re3data.org/suggest (2023).
Kindling, M. & Strecker, D. Data quality assurance at research data repositories. Data Science Journal 21 , 18 (2022).
Kindling, M. et al . Report on re3data COREF/CoreTrustSeal workshop on quality management at research data repositories. Informationspraxis 8 (2022).
Kindling, M., Strecker, D. & Wang, Y. Data Quality Assurance at Research Data Repositories: Survey Data (Zenodo, 2022).
L’Hours, H., Kleemola, M. & De Leeuw, L. CoreTrustSeal: From academic collaboration to sustainable services. IASSIST Quarterly 43 , 1–17 (2019).
Vierkant, P. et al . Re3data Conceptual Model for User Stories . https://doi.org/10.48440/RE3.012 (2021).
Weisweiler, N. L. et al . Re3data Stakeholder Survey and Workshop Report . https://doi.org/10.48440/RE3.013 (2021).
Webster, P. Integrating Discovery and Access to Canadian Data Sources. Contributing to Academic Library Data Services by Sharing Data Source Knowledge Nation Wide in. In collab. with Haigh, S. (2017). https://library.ifla.org/id/eprint/2514/ (2023).
Dearborn, D. et al . Summary Report: Canadian Research Data Repositories and the Re3data Repository Registry in collab. with Labrador, A. & Purcell, F. (2023).
Helmholtz Open Science Office. Community Building for Research Data Repositories in Helmholtz https://os.helmholtz.de/en/open-science-in-helmholtz/networking/community-building-research-data-repositories/ (2023).
Research Data Alliance. Libraries for Research Data IG https://www.rd-alliance.org/groups/libraries-research-data.html (2023).
Research Data Alliance. Data Repository Attributes WG https://www.rd-alliance.org/groups/data-repository-attributes-wg (2023).
Research Data Alliance. Data GranularityWG https://www.rd-alliance.org/groups/data-granularity-wg (2023).
re3data. API https://www.re3data.org/api/doc (2023).
HATEOAS https://en.wikipedia.org/w/index.php?title=HATEOAS&oldid=1141349344 (2023).
Ninkov, A. B. et al . Mapping Metadata - Improving Dataset Discipline Classification . https://doi.org/10.5281/ZENODO.6948238 (2022).
Pampel, H. Re3data.Org Reaches a Milestone and Begins Offering Badges https://doi.org/10.5438/KTR7-ZJJH .
DataCite. DataCite Commons https://commons.datacite.org/repositories (2023).
Wimalaratne, S. et al . D4.7 Tools for Finding and Selecting Certified Repositories for Researchers and Other Stakeholders. https://doi.org/10.5281/ZENODO.6090418 (2022).
Brandon University. Research Data Management Strategy https://www.brandonu.ca/research/files/Research-Data-Strategy.pdf (2023).
Technical University Berlin. Research Data Policy of TU Berlin https://www.tu.berlin/en/working-at-tu-berlin/important-documents/guidelinesdirectives/research-data-policy (2023).
The University of Edinburgh. Research Data Management Policy https://www.ed.ac.uk/information-services/about/policies-and-regulations/research-data-policy (2023).
University of Eastern Finland. Data management at the end of research https://www.uef.fi/en/datasupport/data-management-at-the-end-of-research (n. d.).
Western Norway University of Applied Sciences. Research Data https://www.hvl.no/en/library/research-and-publish/publishing/research-data/ (2023).
Gates Open Access Policy. Data Sharing Requirements https://openaccess.gatesfoundation.org/how-to-comply/data-sharing-requirements/ (2023).
European Commission. Horizon Europe (HORIZON) - Programme Guide 2022 . https://ec.europa.eu/info/funding-tenders/opportunities/docs/2021-2027/horizon/guidance/programme-guide_horizon_en.pdf (2023).
European Research Council. Open Research Data and Data Management Plans - Information for ERC Grantee 2022 . https://erc.europa.eu/sites/default/files/document/file/ERC_info_document-Open_Research_Data_and_Data_Management_Plans.pdf (2023).
National Science Foundation. Dear Colleague Letter: Effective Practices for Making Research Data Discoverable and Citable (Data Sharing) https://www.nsf.gov/pubs/2022/nsf22055/nsf22055.jsp (2023).
National Institutes of Health. Repositories for Sharing Scientific Data https://sharing.nih.gov/data-management-and-sharing-policy/sharing-scientific-data/repositories-for-sharing-scientific-data (2023).
Taylor and Francis. Understanding and Using Data Repositories https://authorservices.taylorandfrancis.com/data-sharing/share-your-data/repositories/ (2023).
Scientific Data. Data Repository Guidance https://www.nature.com/sdata/policies/repositories (2023).
SAGE. Research Data Sharing FAQs https://us.sagepub.com/en-us/nam/research-data-sharing-faqs (2023).
Wiley. Data Sharing Policy https://authorservices.wiley.com/author-resources/Journal-Authors/open-access/data-sharing-citation/data-sharing-policy.html (2023).
re3data. Publications https://www.re3data.org/publications (2023).
Buddenbohm, S., de Jong, M., Minel, J.-L. & Moranville, Y. Find research data repositories for the humanities - the data deposit recommendation service. Int. J. Digit. Hum. 1 , 343–362 (2021).
DARIAH. DDRS https://ddrs-dev.dariah.eu/ddrs/ (2023).
Witt, M. et al . in Digital Libraries: Supporting Open Science (eds Manghi, P., Candela, L. & Silvello, G.) 86–96 (Springer, 2019).
DataCite. DataCite Repository Selector https://repositoryfinder.datacite.org/ (2023).
OpenAIRE. Open Science Observatory https://osobservatory.openaire.eu/home (2023).
The Lisbon Council. Open Science Monitor Methodological Note 2019 . https://research-and-innovation.ec.europa.eu/system/files/2020-01/open_science_monitor_methodological_note_april_2019.pdf (2023).
European Commission. Facts and Figures for Open Research Data https://research-and-innovation.ec.europa.eu/strategy/strategy-2020-2024/our-digital-future/open-science/open-science-monitor/facts-and-figures-open-research-data_en (2023).
Devaraju, A. & Huber, R. F-UJI - An Automated FAIR Data Assessment Tool Zenodo . https://doi.org/10.5281/ZENODO.4063720 (2023).
Berlin Institute of Health. ChariteMetrics Dashboard https://quest-dashboard.charite.de/#tabMethods (2023).
Boyd, C. Understanding research data repositories as infrastructures. P. J. Asso. for Info. Science & Tech. 58 , 25–35 (2021).
Khan, N. A. & Ahangar, H. Emerging Trends in Open Research Data in 2017 9th International Conference on Information and Knowledge Technology , 141–146 (2017).
Hansson, K. & Dahlgren, A. Open research data repositories: Practices, norms, and metadata for sharing images. J. Asso. for Info. Science & Tech. 73 , 303–316 (2022).
Bauer, B. & Ferus, A. Osterreichische Repositorien in OpenDOAR und re3data.org: Entwicklung und Status von Infrastrukturen fur Green Open Access und Forschungsdaten. Mitteilungen der VOB 71 , 70–86 (2018).
Cho, J. Study of Asian RDR based on re3data. EL 37 , 302–313 (2019).
Milzow, K., von Arx, M., Sommer, C., Cahenzli, J. & Perini, L. Open Research Data: SNSF Monitoring Report 2017-2018. https://doi.org/10.5281/ZENODO.3618123 (2020).
Schopfel, J. in Schopfel, J. & Rebouillat, V. Research Data Sharing and Valorization: Developments, Tendencies, Models (Wiley, 2022).
Gomez, N.-D., Mendez, E. & Hernandez-Perez, T. Data and metadata research in the social sciences and humanities: An approach from data repositories in these disciplines. EPI 25 , 545 (2016).
Li, Z. & Liu, W. Characteristics Analysis of Research Data Repositories in Humanities and Social Science - Based on Re3data.Org in 4th International Symposium on Social Science (Atlantis Press, 2018).
Prashar, P. & Chander, H. Research Data Management through Research Data Repositories in the Field of Computer Sciences https://ir.inflibnet.ac.in:8443/ir/bitstream/1944/2400/1/43.pdf (2023).
Kindling, M. et al . The landscape of research data repositories in 2015: A re3data analysis. D-Lib Magazine 23 (2017).
re3data. Statistics https://www.re3data.org/metrics (2023).
re3data COREF. Re3data COREF Blog https://coref.project.re3data.org/ (2023).
Witt, M., Weisweiler, N. L. & Ulrich, R. Happy 10th Anniversary, Re3data! DataCite . https://doi.org/10.5438/MQW0-YT07 .
Felden, J. et al . PANGAEA - Data Publisher for Earth & Environmental Science. Scientific Data 10 , 347 (2023).
Article ADS PubMed PubMed Central Google Scholar
Download references
Acknowledgements
This work has been supported by the German Research Foundation (DFG) under the projects re3data.org - Registry of Research Data Repositories. Community Building, Networking and Research Data Management Services (Grant ID: 209240528) and re3data – Offene und nutzerorientierte Referenz für Forschungsdatenrepositorien (re3data COREF) (Grant ID: 422587133). The article processing charge was funded by the Deutsche Forschungsgemeinschaft (DFG, German Research Foundation) – 491192747 and the Open Access Publication Fund of Humboldt-Universität zu Berlin.
Open Access funding enabled and organized by Projekt DEAL.
Author information
Authors and affiliations.
Humboldt-Universität zu Berlin, Berlin School of Library and Information Science, Berlin, Germany
Heinz Pampel, Dorothea Strecker & Vivien Petras
Helmholtz Association, Helmholtz Open Science Office, Potsdam, Germany
Heinz Pampel, Nina Leonie Weisweiler, Roland Bertelmann & Lea Maria Ferguson
University of Purdue, Distributed Data Curation Center, West Lafayette, IN, USA
Michael Witt
DataCite - International Data Citation Initiative e. V, Hannover, Germany
Paul Vierkant & Matthew Buys
GFZ German Research Centre for Geosciences, Library and Information Services, Potsdam, Germany
Kirsten Elger
Freie Universität Berlin, Open-Access-Büro Berlin, Berlin, Germany
Maxi Kindling
University of Bath, Library, Bath, UK
Rachael Kotarski
You can also search for this author in PubMed Google Scholar
Contributions
H.P., N.L.W., D.S. and M.W. wrote the first draft. P.V., E.K., R.B., M.B., L.M.F., M.K., R.K. and V.P. provided critical feedback and helped shape the manuscript. H.P., N.L.W., D.S., M.W., P.V., E.K., R.B., M.B., L.M.F., M.K., R.K. and V.P. contributed to the final writing and revision of the manuscript.
Corresponding authors
Correspondence to Heinz Pampel or Nina Leonie Weisweiler .
Ethics declarations
Competing interests.
The authors declare no competing interests.
Additional information
Publisher’s note Springer Nature remains neutral with regard to jurisdictional claims in published maps and institutional affiliations.
Rights and permissions
Open Access This article is licensed under a Creative Commons Attribution 4.0 International License, which permits use, sharing, adaptation, distribution and reproduction in any medium or format, as long as you give appropriate credit to the original author(s) and the source, provide a link to the Creative Commons licence, and indicate if changes were made. The images or other third party material in this article are included in the article’s Creative Commons licence, unless indicated otherwise in a credit line to the material. If material is not included in the article’s Creative Commons licence and your intended use is not permitted by statutory regulation or exceeds the permitted use, you will need to obtain permission directly from the copyright holder. To view a copy of this licence, visit http://creativecommons.org/licenses/by/4.0/ .
Reprints and permissions
About this article
Cite this article.
Pampel, H., Weisweiler, N.L., Strecker, D. et al. re3data – Indexing the Global Research Data Repository Landscape Since 2012. Sci Data 10 , 571 (2023). https://doi.org/10.1038/s41597-023-02462-y
Download citation
Received : 13 July 2023
Accepted : 09 August 2023
Published : 29 August 2023
DOI : https://doi.org/10.1038/s41597-023-02462-y
Share this article
Anyone you share the following link with will be able to read this content:
Sorry, a shareable link is not currently available for this article.
Provided by the Springer Nature SharedIt content-sharing initiative
This article is cited by
Garbage in, garbage out: mitigating risks and maximizing benefits of ai in research.
- Brooks Hanson
- Shelley Stall
Nature (2023)
Quick links
- Explore articles by subject
- Guide to authors
- Editorial policies
Sign up for the Nature Briefing newsletter — what matters in science, free to your inbox daily.

How to choose a suitable data repository for your research data
Governments, funders, and institutions worldwide are increasingly introducing open data policies and mandates to encourage researchers to share their research data openly. Depositing your data in a publicly accessible research data repository that assigns a persistent identifier (PI or PID) ensures that your dataset remains available to humans and machines in the future. National institutes, funders, and journals often maintain a list of endorsed repositories for your use. You may need to set out your intention to deposit your research data in a repository as part of a data management plan (DMP). Still, choosing the best repository from such lists can often be daunting. Here, we offer some preliminary guidance on selecting the most suitable repository for your research data.
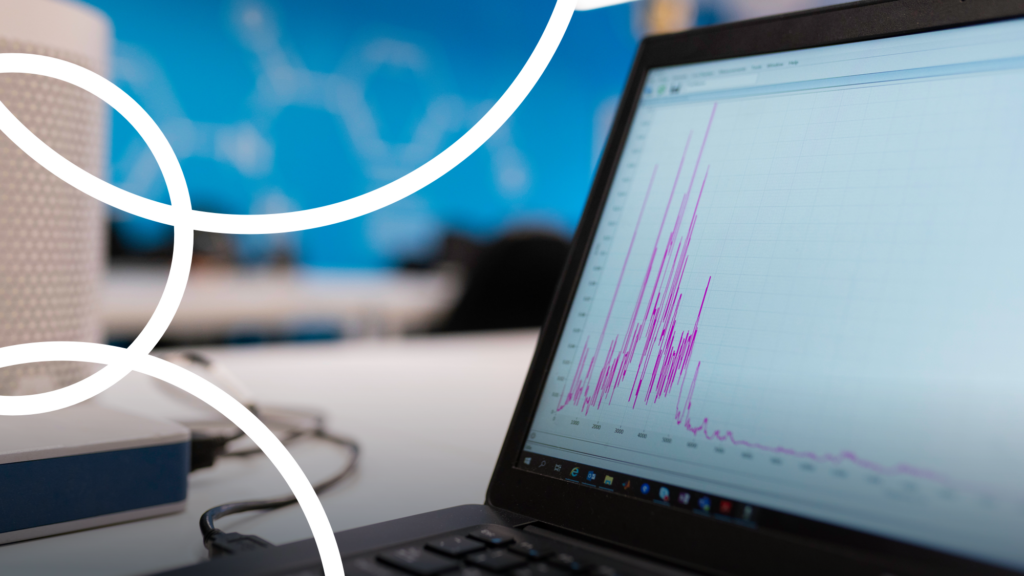
Where to share your data?
You know you want to make your data openly available, but where should you host it? Some researchers opt to host their data solely on a laboratory website or as part of a publication’s supplementary. However, sharing data (or any other research outputs) in this ways hinders others from finding and reusing it. That’s where data repositories come in.
What is a data repository?
According to the Registry of Research Data Repositories (re3data.org ) — a global registry of research data repositories — a repository is an online storage infrastructure for researchers to store data, code, and other research outputs for scholarly publication. Research data means information objects generated by scholarly projects for example, through experiments, measurements, surveys, or interviews. Depositing your data in a publicly accessible, recognized repository ensures that your dataset continues to be available to both humans and machines in a usable form.
An open access data repository openly stores data, including scientific data from research projects in a way that allows immediate user access to anyone. There are no limitations to the repository access. As such, repositories make data findable, accessible, and usable in the long term, by using sustainable file formats and providing persistent identifiers and informative descriptive data (metadata).
Choosing a data repository
Nowadays, it is widely considered best practice to deposit your data in a publicly available repository, where it is assigned a persistent identifier (PI or PID) and can be accessed by anyone, anywhere. Where you deposit your data will depend on any applicable legal and ethical factors, who funded the work, and where you hope to publish. However, there are a few simple questions you can ask yourself to make selecting an appropriate repository easier.
Question #1: Does your data contain personal or sensitive information that cannot be anonymized?
If you answered ‘yes’ to this question, consider a controlled access repository.
There may be cases where openly sharing data is not feasible due to ethical or confidentiality considerations. Depending on what the Institutional Review Board approving your study said about data sharing, and what your participants consented to, it may still be possible to make your data accessible to authenticated users via a controlled-access repository or a generalist repository that allows you to limit access to your data.
Some of the repositories that allow you to limit access to your data include:
- Figshare – You can generate a ‘private sharing link’ for free. You can send this link via email address, and the recipient can access the data without logging in or having a Figshare account.
- Zenodo – Funded by CERN, OpenAIRE, and Horizon 2020, Zenodo lets users deposit restricted files and share access with others if they meet certain requirements.
- OSF – You can make your project private or public and alternate between the two settings.
If you answered ‘no’ to this question, move on to question #2.
Question #2: Is there a discipline-specific repository for your dataset?
If you answered ‘yes’ to this question, consider a discipline-specific repository.
Research data differs significantly across disciplines. Discipline-specific repositories offer specialist domain knowledge and curation expertise for particular data types. Plus, using a discipline-specific repository can also make your data more visible to others in your research community. We recommend speaking to your institutional librarian, funder, or colleagues for guidance on choosing a repository relevant to your discipline.
If you answered ‘no’ to this question, move on to question #3.
Question #3: Does your institutional repository accept data?
If you answered ‘yes’ to this question, consider your institutional repository.
Many institutions offer support providing repository infrastructure to their researchers for managing and depositing data. Institutional repositories that accept datasets provide stewardship, helping to ensure that your dataset is preserved and accessible.
If you answered ‘no’ to this question, consider a generalist data repository.
General data repositories accept datasets regardless of discipline or institution. These repositories support a wide variety of file types and are particularly useful where a discipline-specific repository does not exist.
Some examples of generalist data repositories include:
- 4TU.ResearchData
- ANDS contributing repositories
- Dryad Digital Repository
- Harvard Dataverse
- Mendeley Data
- Open Science Framework
- Science Data Bank
- Code Ocean
Common questions about data repositories
What is a digital object identifier (doi).
When a researcher uploads a document to an online data repository, a digital object identifier (DOI) will be assigned. A DOI is a globally unique and persistent string that identifies your work permanently. A data repository can assign a DOI to any document. The DOI contains metadata that provides users with relevant information about an object, such as the title, author, keywords, year of publication, and the URL where that document is stored.
How do I find a ‘FAIR aligned’ repository?
The repository finder tool, developed by DataCite allows you to search for certified repositories that support the FAIR data principles. The FAIR data principles aim to make research data more F inable, A ccessible, I nteroperable, and R eusable (FAIR). Both FAIRsharing and Re3Data provide information on an array of criteria to help you identify the repositories most suited to your needs.
Should I use a discipline specific repository?
If your funder does not have a preferred repository of choice, you may wish to use a discipline-specific repository which is frequently used in your field of research. This type of repository will make it easy for your research community to find your data. There are many repositories of this type,including, GEO or GenBank for genetic data, or the UK Data Service for Social Sciences and Humanities data.
What is versioning?
Some repositories accommodate changes to deposited datasets through versioning. Selecting a repository that features versioning gives you the flexibility to add new data, restructure, and improve your dataset. Each version of your dataset is uniquely identifiable and maintained – meaning others can find, access, reuse, and cite whichever version of the dataset they require. What about my software and code? Software and code are important research outputs. In addition to using a version control system such as GitHub, you should deposit your source code in a data repository where it will be assigned a unique identifier. Using such a repository will ensure your code is openly and permanently available.
How do I share de-identified research data?
Repositories vary widely so it’s essential you choose the repository best suited to your research whether it be a subject specific, general, funder, or institutional repository. If you would like to share de-identified data then one option is the NICHD DASH . This repository allows researchers to store and access de-identified data from NICHD funded research for the purposes of secondary research use.
Can I share research data with restricted access?
Restricted data deposit is possible. If you need to preserve study participant anonymity in clinical datasets, then there are repositories suitable for datasets requiring restricted data access. We suggest contacting repositories directly to determine those with data access controls best suited to the specific requirements of your study.
Do I have to pay to deposit data to a repository?
Always check whether your repository requires a data publication fee. Not all repositories require data publication charges, and if your chosen repository does require a fee, you could still be entitled to sponsorship by a publisher or funder. Zenodo and Figshare both allow registered users to deposit data free of charge. However, Dryad charges a data publication fee.
What about my software and code?
Software and code are important research outputs. In addition to using a version control system such as GitHub, you should deposit your source code in a data repository where it will be assigned a unique identifier. Using such a repository will ensure your code is openly and permanently available.
Choosing a repository for your research data might seem difficult at first, but sharing your data openly is vital to increasing the reproducibility of research. In turn, you can expect greater visibility for your work and a wider potential impact.
Discover everything you need to know about making your research data open and FAIR.
Other blog posts
When did peer review start: the origins and evolution of peer review through time.
Peer review is not just quality control, it is the backbone of modern scientific and academic publis...
How to respond to peer reviewers comments: top tips on addressing reviewer feedback
The peer review process is a fundamental component of scholarly publishing, ensuring the quality and...
How to write a peer review report: tips and tricks for constructive reviews
Peer review is an integral part of scholarly communication and academic publishing. A key player in ...
Research Data Management for Purdue
The Purdue University Research Repository (PURR) provides an online, collaborative working space and data-sharing platform to support Purdue researchers and their collaborators.
Full spectrum support from planning to publication
Meet funders’ data requirements with our helpful resources and expert advice.
Collaborate
Your own file sharing space and update feed make team collaboration easy.
Publish your data, get a DOI and track your impact. Leave the archiving to us.
Our services
Expert help with Data Management Planning
PURR offers several layers of assistance with data management planning including step-by-step guidance, boilerplate text for your proposal , sample plans, and individual consultations.
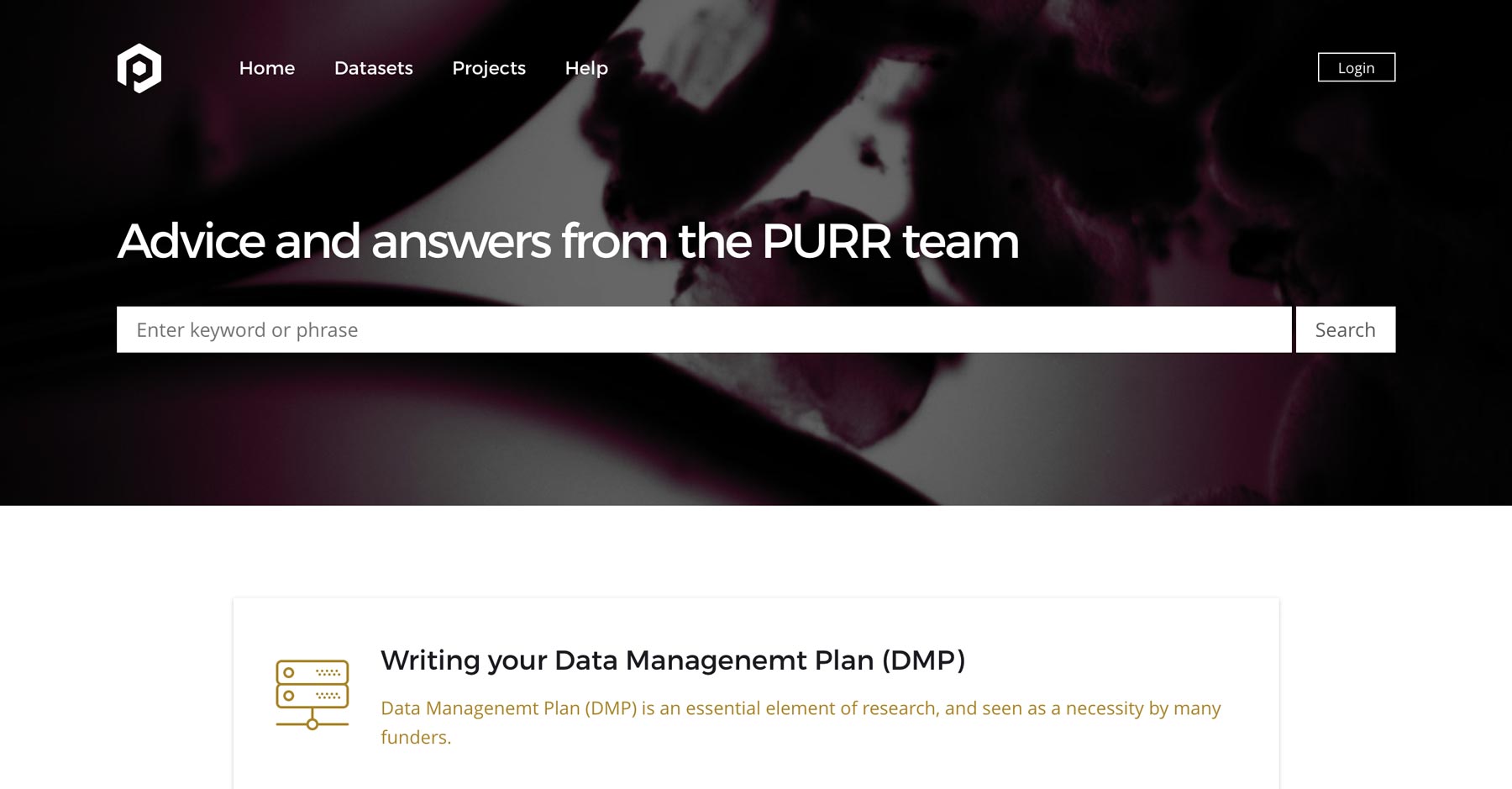
Storage for all of your projects
PURR measures storage by the project not the user, so you always have room for something new. All projects start with 100GB of storage . Is your project grant funded? Let us know and we’ll bump that up to 1 TB at no cost to you.
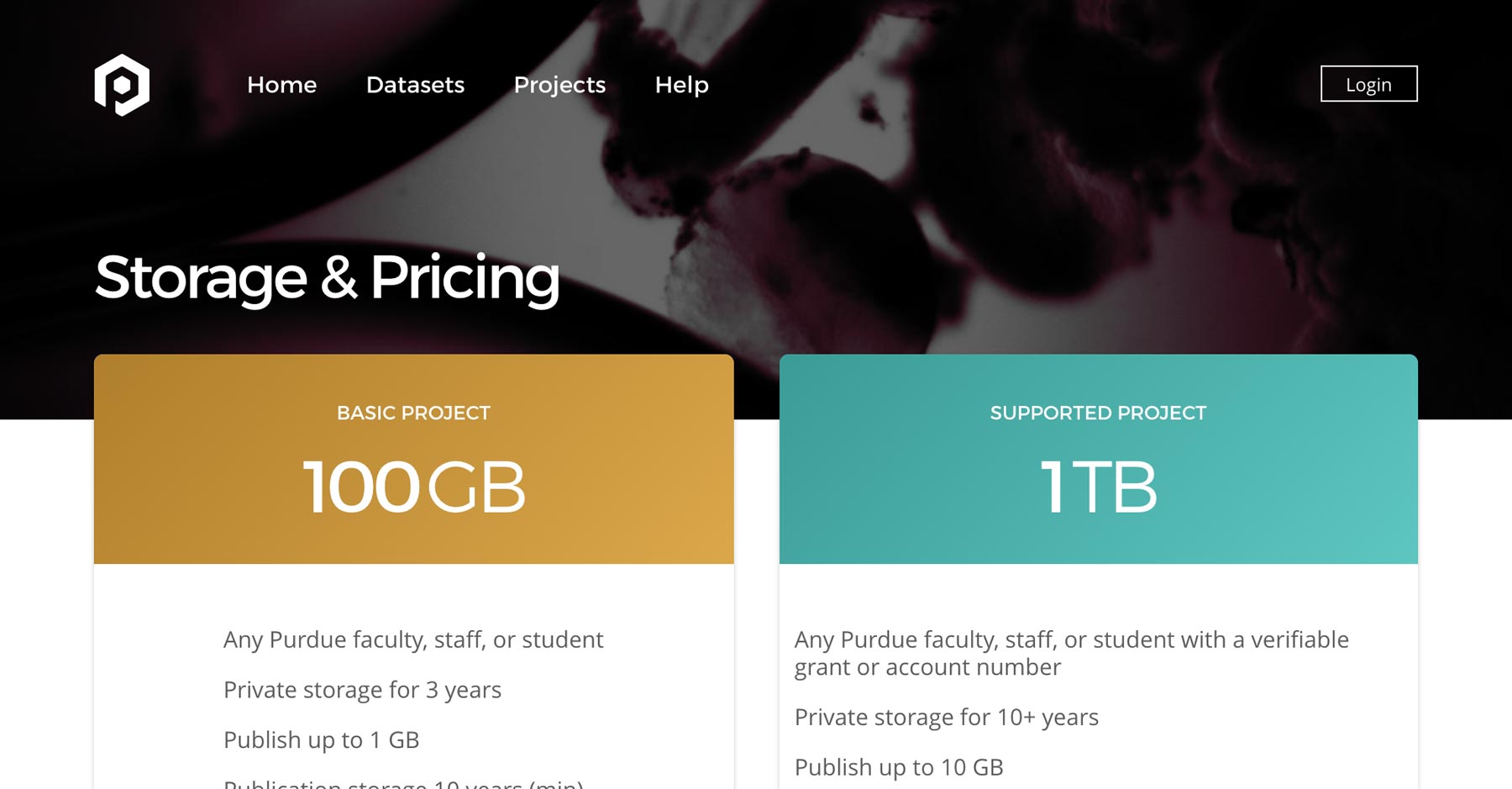
Get credit for your data
Make it easy for researchers to find and cite your data by publishing with PURR. Each dataset gets a Digital Object Identifier (DOI) that makes it easier for people to cite your data and give you credit. PURR even helps keep track of when your dataset gets cited.
Measuring Impact
PURR’s most re-used dataset has been cited 99 times since it was published in 2015, including more than 50 articles in 25 journals with an average Journal Impact Factor of 3.185.
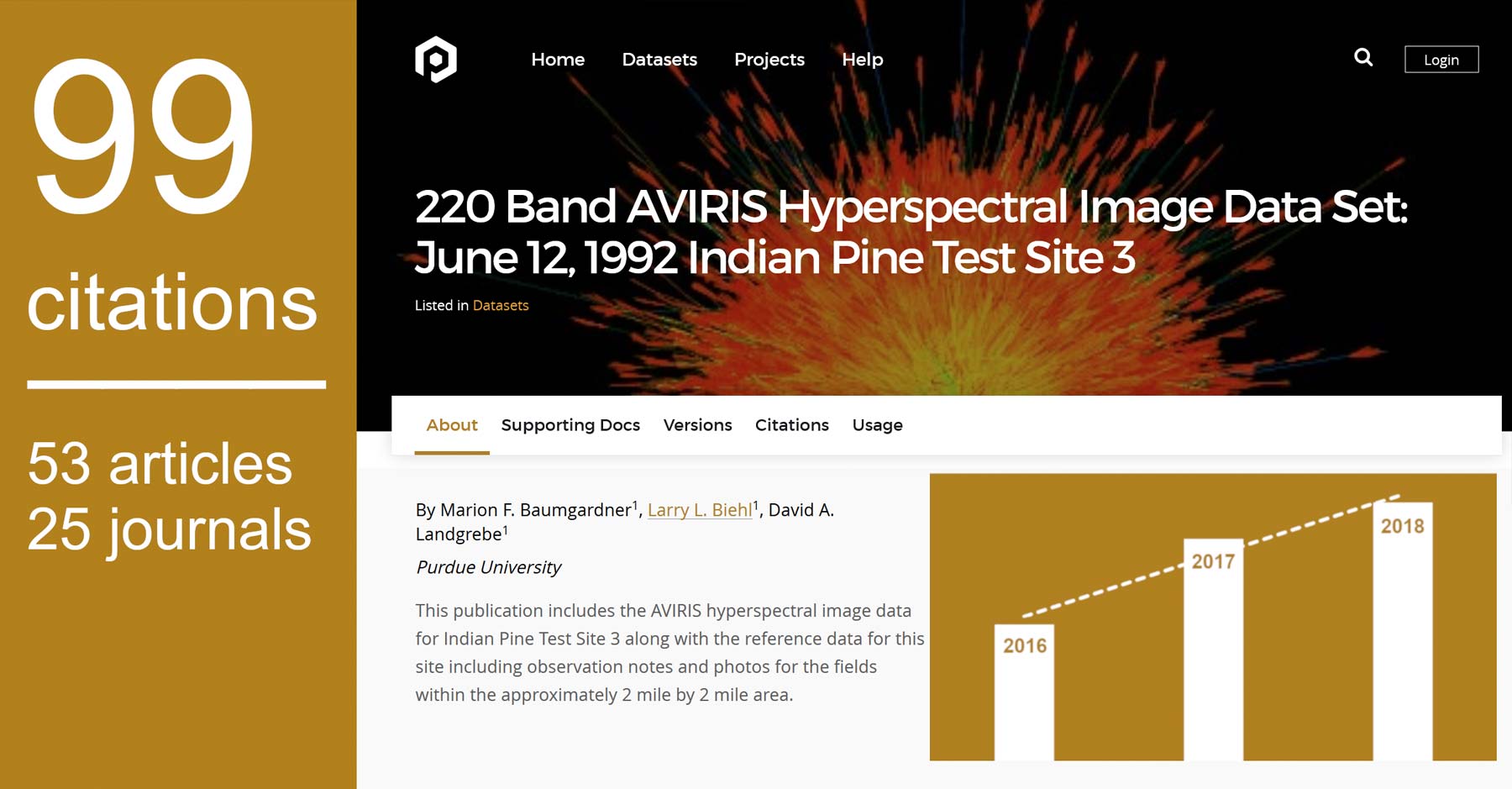
Computer Science
Discover new and interesting datasets
Explore the open data behind Purdue's world-class research. PURR publishes and archives digital datasets from researchers across campus and welcomes all kinds of open data from images and videos to spreadsheets and source code.
A Hybrid Physics-Guided Deep Learning Modeling Framework for Predicting Surface Soil Moisture
Xuan Xi, Qianlai Zhuang, Xinyu Liu
Tahta Limanı Archaeological Survey: Processed Ceramics, 2015 and 2017
Günder Varinlioglu, Noah Kaye, Stanislav Pejša, Nicholas Kregotis Rauh
Source Data for Organic Optoelectronic Synapse
Ke Chen, Hang Hu, Inho Song, Habtom B Gobeze, Won-June Lee, Ashkan Abtahi, Kirk S. Schanze, Jianguo Mei
Row Selection in Remote Sensing for Maize and Sorghum
Mitchell R Tuinstra, Seth A Tolley
General Aviation Flight Phase Identification using Automatic Dependent Surveillance-Broadcast...
Qilei Zhang, John H Mott
Explore more datasets
Purr at a glance, have a question, featured dataset.
James A McCann, Purdue Michael Jones-Correa , Cornell
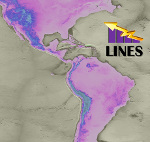
An original national survey of the foreign-born Latino population in the US who were interviewed during and/or immediately after the 2012 national elections in the US.
Start Your Research Project
Create a Data Management Plan Learn about the detailed requirements for your data management plan (DMP). Funding agency requirements are very specific and our DMP resources can help you to clear up any confusion. Get Started ›
Upload Research Data to Your Project Create a project to upload and share your data with collaborators using our step-by-step form to guide you through the process. Invite collaborators from other institutions to join your project. Create a Project ›
Publish your Dataset Package, describe, and publish your dataset with a Datacite DOI. Publishing will ensure your dataset is citable, reusable, and archived for the long-term. See Published Datasets ›
.png)
How to build a research repository: a step-by-step guide to getting started
Research repositories have the potential to be incredibly powerful assets for any research-driven organisation. But when it comes to building one, it can be difficult to know where to start. In this post, we provide some practical tips to define a clear vision and strategy for your repository.
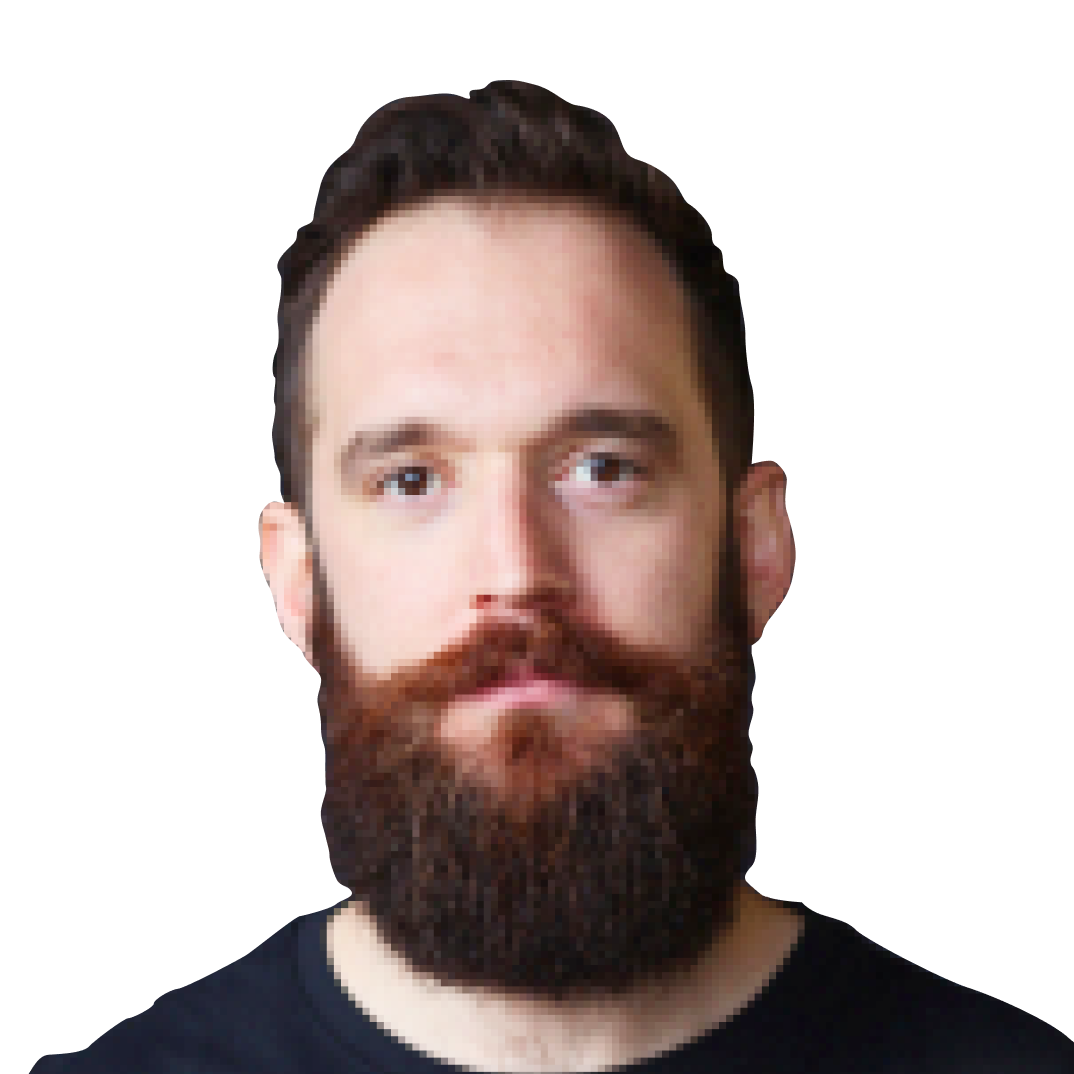
Done right, research repositories have the potential to be incredibly powerful assets for any research-driven organisation. But when it comes to building one, it can be difficult to know where to start.
As a result, we see tons of teams jumping in without clearly defining upfront what they actually hope to achieve with the repository, and ending up disappointed when it doesn't deliver the results.
Aside from being frustrating and demoralising for everyone involved, building an unused repository is a waste of money, time, and opportunity.
So how can you avoid this?
In this post, we provide some practical tips to define a clear vision and strategy for your repository in order to help you maximise your chances of success.
🚀 This post is also available as a free, interactive Miro template that you can use to work through each exercise outlined below - available for download here .
Defining the end goal for your repository
To start, you need to define your vision.
Only by setting a clear vision, can you start to map out the road towards realising it.
Your vision provides something you can hold yourself accountable to - acting as a north star. As you move forward with the development and roll out of your repository, this will help guide you through important decisions like what tool to use, and who to engage with along the way.
The reality is that building a research repository should be approached like any other product - aiming for progress, over perfection with each iteration of the solution.
Starting with a very simple question like "what do we hope to accomplish with our research repository within the first 12 months?" is a great starting point.
You need to be clear on the problems that you’re looking to solve - and the desired outcomes from building your repository - before deciding on the best approach.
Building a repository is an investment, so it’s important to consider not just what you want to achieve in the next few weeks or months, but also in the longer term to ensure your repository is scalable.
Whatever the ultimate goal (or goals), capturing the answer to this question will help you to focus on outcomes over output .
🔎 How to do this in practice…
1. complete some upfront discovery.
In a previous post we discussed how to conduct some upfront discovery to help with understanding today’s biggest challenges when it comes to accessing and leveraging research insights.
⏰ You should aim to complete your upfront discovery within a couple of hours, spending 20-30 mins interviewing each stakeholder (we recommend talking with at least 5 people, both researchers and non-researchers).
2. Prioritise the problems you want to solve
Start by spending some time reviewing the current challenges your team and organisation are facing when it comes to leveraging research and insights.
You can run a simple affinity mapping exercise to highlight the common themes from your discovery and prioritise the top 1-3 problems that you’d like to solve using your repository.
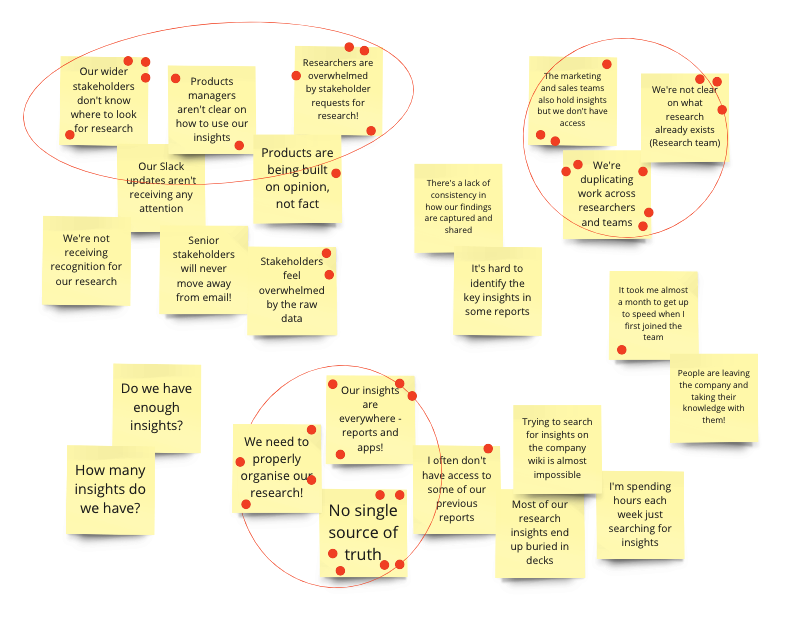
💡 Example challenges might include:
Struggling to understand what research has already been conducted to-date, leading to teams repeating previous research
Looking for better ways to capture and analyse raw data e.g. user interviews
Spending lots of time packaging up research findings for wider stakeholders
Drowning in research reports and artefacts, and in need of a better way to access and leverage existing insights
Lacking engagement in research from key decision makers across the organisation
⏰ You should aim to confirm what you want to focus on solving with your repository within 45-60 mins (based on a group of up to 6 people).
3. Consider what future success looks like
Next you want to take some time to think about what success looks like one year from now, casting your mind to the future and capturing what you’d like to achieve with your repository in this time.
A helpful exercise is to imagine the headline quotes for an internal company-wide newsletter talking about the impact that your new research repository has had across the business.
The ‘ Jobs to be done ’ framework provides a helpful way to format the outputs for this activity, helping you to empathise with what the end users of your repository might expect to experience by way of outcomes.

💡 Example headlines might include:
“When starting a new research project, people are clear on the research that’s already been conducted, so that we’re not repeating previous research” Research Manager
“During a study, we’re able to quickly identify and share the key insights from our user interviews to help increase confidence around what our customers are currently struggling with” Researcher
“Our designers are able to leverage key insights when designing the solution for a new user journey or product feature, helping us to derisk our most critical design decisions” Product Design Director
“Our product roadmap is driven by customer insights, and building new features based on opinion is now a thing of the past” Head of Product
“We’ve been able to use the key research findings from our research team to help us better articulate the benefits of our product and increase the number of new deals” Sales Lead
“Our research is being referenced regularly by C-level leadership at our quarterly townhall meetings, which has helped to raise the profile of our team and the research we’re conducting” Head of Research
Ask yourself what these headlines might read and add these to the front page of a newspaper image.
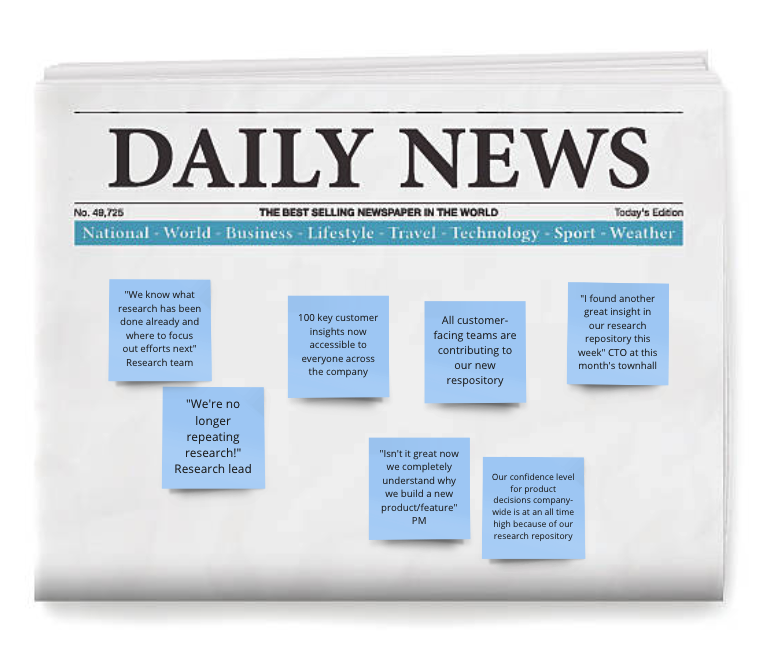
You then want to discuss each of these headlines across the group and fold these into a concise vision statement for your research repository - something memorable and inspirational that you can work towards achieving.
💡Example vision statements:
‘Our research repository makes it easy for anyone at our company to access the key learnings from our research, so that key decisions across the organisation are driven by insight’
‘Our research repository acts as a single source of truth for all of our research findings, so that we’re able to query all of our existing insights from one central place’
‘Our research repository helps researchers to analyse and synthesise the data captured from user interviews, so that we’re able to accelerate the discovery of actionable insights’
‘Our research repository is used to drive collaborative research across researchers and teams, helping to eliminate data silos, foster innovation and advance knowledge across disciplines’
‘Our research repository empowers people to make a meaningful impact with their research by providing a platform that enables the translation of research findings into remarkable products for our customers’
⏰ You should aim to agree the vision for your repository within 45-60 mins (based on a group of up to 6 people).
Creating a plan to realise your vision
Having a vision alone isn't going to make your repository a success. You also need to establish a set of short-term objectives, which you can use to plan a series of activities to help you make progress towards this.
Focus your thinking around the more immediate future, and what you want to achieve within the first 3 months of building your repository.
Alongside the short-term objectives you’re going to work towards, it’s also important to consider how you’ll measure your progress, so that you can understand what’s working well, and what might require further attention.
Agreeing a set of success metrics is key to holding yourself accountable to making a positive impact with each new iteration. This also helps you to demonstrate progress to others from as early on in the process as possible.
1. Establish 1-3 short term objectives
Take your vision statement and consider the first 1-3 results that you want to achieve within the first 3 months of working towards this.
These objectives need to be realistic and achievable given the 3 month timeframe, so that you’re able to build some momentum and set yourself up for success from the very start of the process.
💡Example objectives:
Improve how insights are defined and captured by the research team
Revisit our existing research to identify what data we want to add to our new research repository
Improve how our research findings are organised, considering how our repository might be utilised by researchers and wider teams
Initial group of champions bought-in and actively using our research repository
Improve the level of engagement with our research from wider teams and stakeholders
Capture your 3 month objectives underneath your vision, leaving space to consider the activities that you need to complete in order to realise each of these.
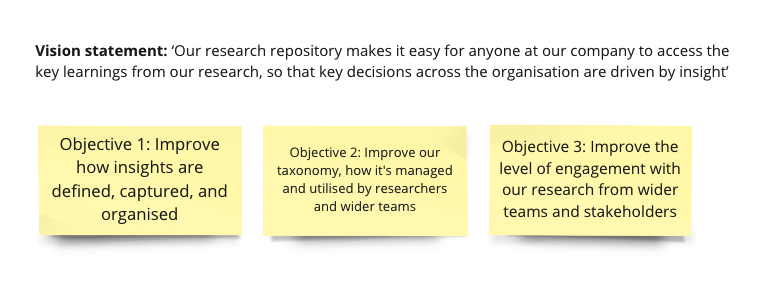
2. Identify how to achieve each objective
Each activity that you commit to should be something that an individual or small group of people can comfortably achieve within the first 3 months of building your repository.
Come up with some ideas for each objective and then prioritise completing the activities that will result in the biggest impact, with the least effort first.
💡Example activities:
Agree a definition for strategic and tactical insights to help with identifying the previous data that we want to add to our new research repository
Revisit the past 6 months of research and capture the data we want to add to our repository as an initial body of knowledge
Create the first draft taxonomy for our research repository, testing this with a small group of wider stakeholders
Launch the repository with an initial body of knowledge to a group of wider repository champions
Start distributing a regular round up of key insights stored in the repository
You can add your activities to a simple kanban board , ordering your ‘To do’ column with the most impactful tasks up top, and using this to track your progress and make visible who’s working on which tasks throughout the initial build of your repository.
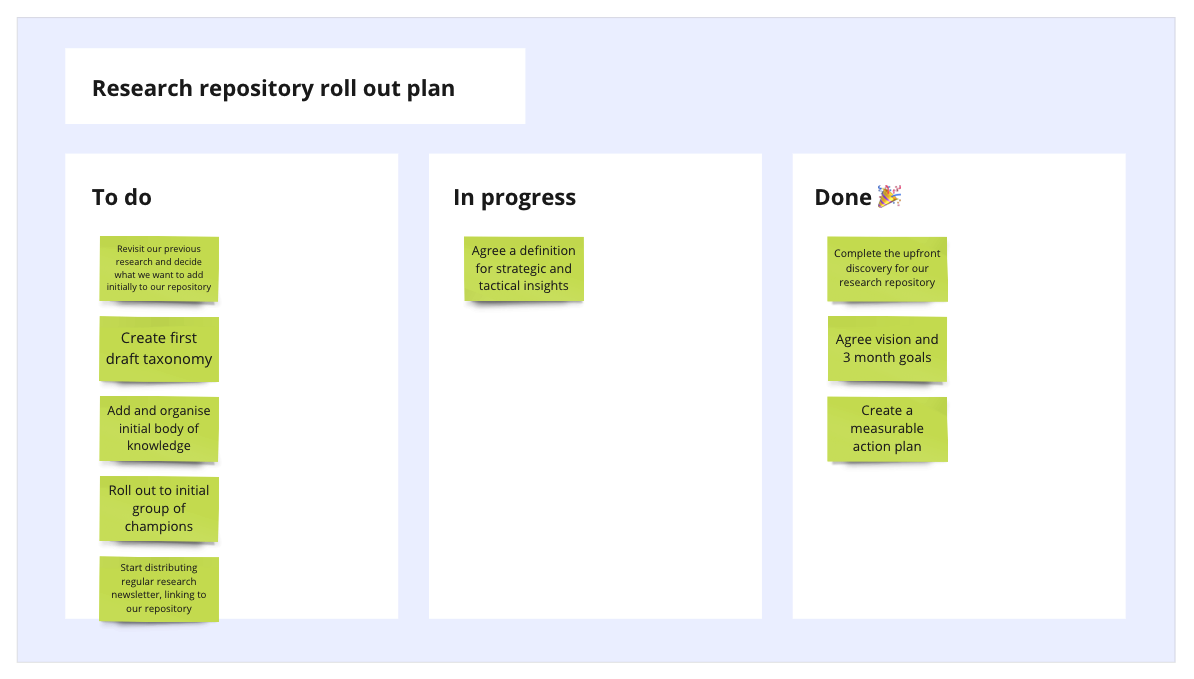
This is something you can come back to a revisit as you move throughout the wider roll out of your repository - adding any new activities into the board and moving these through to ‘Done’ as they’re completed.
⚠️ At this stage it’s also important to call out any risks or dependencies that could derail your progress towards completing each activity, such as capacity, or requiring support from other individuals or teams.
3. Agree how you’ll measure success
Lastly, you’ll need a way to measure success as you work on the activities you’ve associated with each of your short term objectives.
We recommend choosing 1-3 metrics that you can measure and track as you move forward with everything, considering ways to capture and review the data for each of these.
⚠️ Instead of thinking of these metrics as targets, we recommend using them to measure your progress - helping you to identify any activities that aren’t going so well and might require further attention.
💡Example success metrics:
Usage metrics - Number of insights captured, Active users of the repository, Number of searches performed, Number of insights viewed and shared
User feedback - Usability feedback for your repository, User satisfaction ( CSAT ), NPS aka how likely someone is to recommend using your repository
Research impact - Number of stakeholder requests for research, Time spent responding to requests, Level of confidence, Repeatable value of research, Amount of duplicated research, Time spent onboarding new joiners
Wider impact - Mentions of your research (and repository) internally, Links to your research findings from other initiatives e.g. discovery projects, product roadmaps, Customers praising solutions that were fuelled by your research
Think about how often you want to capture and communicate this information to the rest of the team, to help motivate everyone to keep making progress.
By establishing key metrics, you can track your progress and determine whether your repository is achieving its intended goals.
⏰ You should aim to create a measurable action plan for your repository within 60-90 mins (based on a group of up to 6 people).
🚀 Why not use our free, downloadable Miro template to start putting all of this into action today - available for download here .
To summarise
As with the development of any product, the cost of investing time upfront to ensure you’re building the right thing for your end users, is far lower than the cost of building the wrong thing - repositories are no different!
A well-executed research repository can be an extremely valuable asset for your organisation, but building one requires consideration and planning - and defining a clear vision and strategy upfront will help to maximise your chances of success.
It’s important to not feel pressured to nail every objective that you set in the first few weeks or months. Like any product, the further you progress, the more your strategy will evolve and shift. The most important thing is getting started with the right foundations in place, and starting to drive some real impact.
We hope this practical guide will help you to get started on building an effective research repository for your organisation. Thanks and happy researching!

Work with our team of experts
At Dualo we help teams to define a clear vision and strategy for their research repository as part of the ‘Discover, plan and set goals’ module facilitated by our Dualo Academy team. If you’re interested in learning more about how we work with teams, book a short call with us to discuss how we can support you with the development of your research repository and knowledge management process.
Nick Russell
I'm one of the Co-Founders of Dualo, passionate about research, design, product, and AI. Always open to chatting with others about these topics.
Insights to your inbox
Join our growing community and be the first to see fresh content.
Repo Ops ideas worth stealing
Interviews with leaders
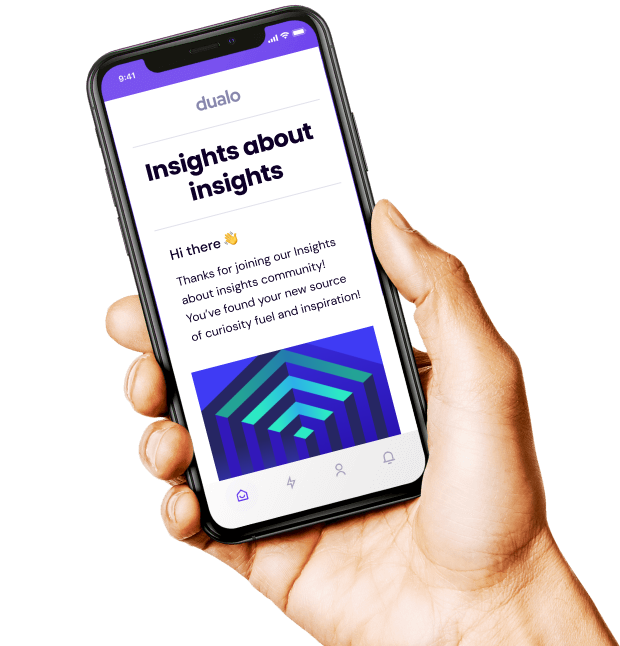
Related Articles
.jpeg)
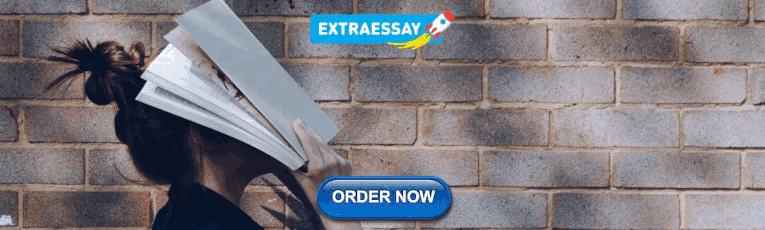
How to measure and communicate research impact and ROI

Repositories are not just for researchers
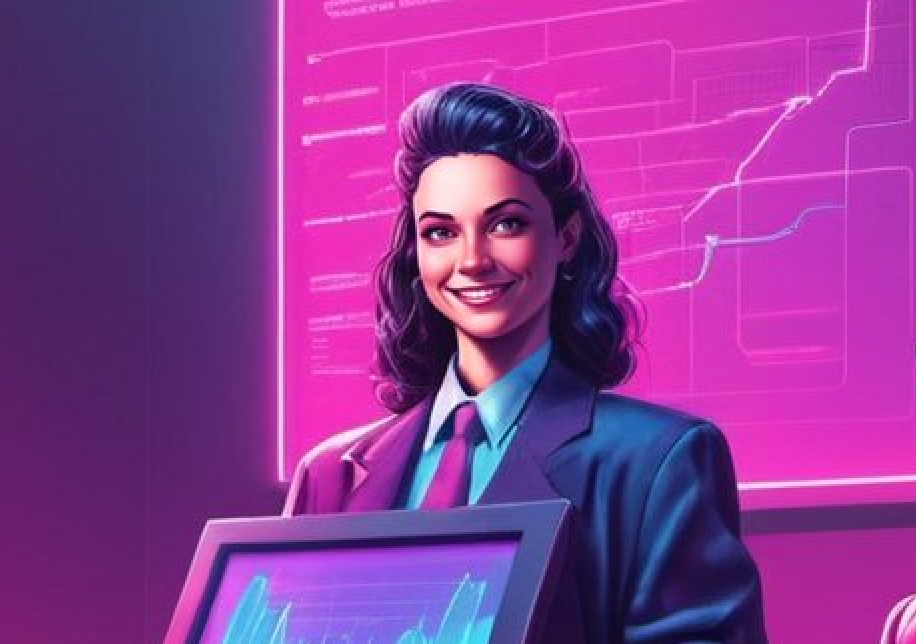
How top 1% researchers build UXR case studies
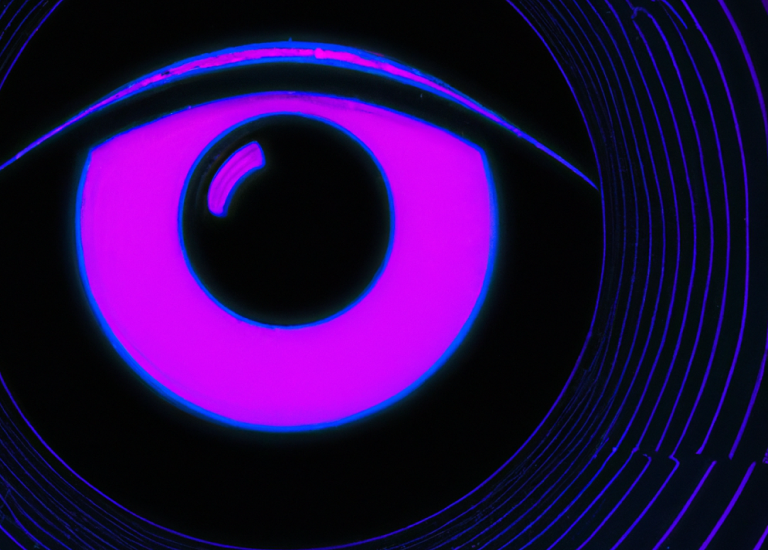
Navigating generative AI in UX Research: a deep dive into data privacy
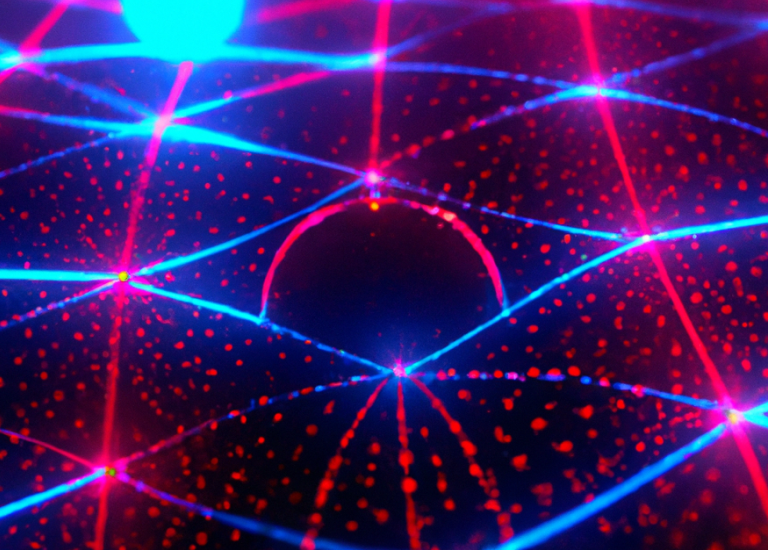
Welcoming a new age of knowledge management for user research
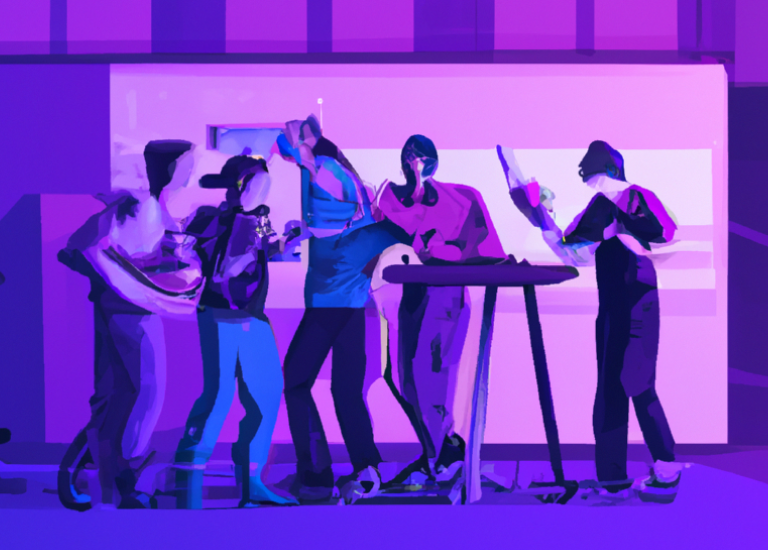
Building a research repository? Avoid these common pitfalls

Redirect Notice

Selecting a Data Repository
Learn how to evaluate and select appropriate data repositories.
As outlined in NIH's Supplemental Policy Information: Selecting a Repository for Data Resulting from NIH-Supported Research , using a quality data repository generally improves the FAIRness (Findable, Accessible, Interoperable, and Re-usable) of the data. For that reason, NIH strongly encourages the use of established repositories to the extent possible for preserving and sharing scientific data.
While NIH supports many data repositories, there are also many biomedical data repositories and generalist repositories supported by other organizations, both public and private. Researchers may wish to consult experts in their own institutions (e.g., librarians, data managers) for assistance in selecting an appropriate data repository.
NIH encourages researchers to select data repositories that exemplify the desired characteristics below, including when a data repository is supported or provided by a cloud-computing or high-performance computing platform. These desired characteristics aim to ensure that data are managed and shared in ways that are consistent with FAIR data principles.
- For data generated from research subject to such policies or funded under such opportunities, researchers should use the designated data repository(ies).
- Primary consideration should be given to data repositories that are discipline or data-type specific to support effective data discovery and reuse. For a list of NIH-supported repositories, visit Repositories for Sharing Scientific Data .
- Small datasets (up to 2 GB in size) may be included as supplementary material to accompany articles submitted to PubMed Central ( instructions ).
- Data repositories, including generalist repositories or institutional repositories, that make data available to the larger research community, institutions, or the broader public.
- Large datasets may benefit from cloud-based data repositories for data access, preservation, and sharing.
See Repositories for Sharing Scientific Data for a listing of NIH-supported data repositories.
Desirable Characteristics for All Data Repositories
When choosing a repository to manage and share data resulting from Federally funded research, here are some desirable characteristics to look for:
- Unique Persistent Identifiers: Assigns datasets a citable, unique persistent identifier, such as a digital object identifier (DOI) or accession number, to support data discovery, reporting, and research assessment. The identifier points to a persistent landing page that remains accessible even if the dataset is de-accessioned or no longer available.
- Long-Term Sustainability: Has a plan for long-term management of data, including maintaining integrity, authenticity, and availability of datasets; building on a stable technical infrastructure and funding plans; and having contingency plans to ensure data are available and maintained during and after unforeseen events.
- Metadata: Ensures datasets are accompanied by metadata to enable discovery, reuse, and citation of datasets, using schema that are appropriate to, and ideally widely used across, the community(ies) the repository serves. Domain-specific repositories would generally have more detailed metadata than generalist repositories.
- Curation and Quality Assurance: Provides, or has a mechanism for others to provide, expert curation and quality assurance to improve the accuracy and integrity of datasets and metadata.
- Free and Easy Access: Provides broad, equitable, and maximally open access to datasets and their metadata free of charge in a timely manner after submission, consistent with legal and ethical limits required to maintain privacy and confidentiality, Tribal sovereignty, and protection of other sensitive data.
- Broad and Measured Reuse: Makes datasets and their metadata available with broadest possible terms of reuse; and provides the ability to measure attribution, citation, and reuse of data (i.e., through assignment of adequate metadata and unique PIDs).
- Clear Use Guidance: Provides accompanying documentation describing terms of dataset access and use (e.g., particular licenses, need for approval by a data use committee).
- Security and Integrity: Has documented measures in place to meet generally accepted criteria for preventing unauthorized access to, modification of, or release of data, with levels of security that are appropriate to the sensitivity of data.
- Confidentiality: Has documented capabilities for ensuring that administrative, technical, and physical safeguards are employed to comply with applicable confidentiality, risk management, and continuous monitoring requirements for sensitive data.
- Common Format: Allows datasets and metadata downloaded, accessed, or exported from the repository to be in widely used, preferably non-proprietary, formats consistent with those used in the community(ies) the repository serves.
- Provenance: Has mechanisms in place to record the origin, chain of custody, and any modifications to submitted datasets and metadata.
- Retention Policy: Provides documentation on policies for data retention within the repository.
Additional Considerations for Human Data
When working with human participant data, including de-identified human data, here are some additional characteristics to look for:
- Fidelity to Consent: Uses documented procedures to restrict dataset access and use to those that are consistent with participant consent and changes in consent.
- Restricted Use Compliant: Uses documented procedures to communicate and enforce data use restrictions, such as preventing reidentification or redistribution to unauthorized users.
- Privacy: Implements and provides documentation of measures (for example, tiered access, credentialing of data users, security safeguards against potential breaches) to protect human subjects’ data from inappropriate access.
- Plan for Breach: Has security measures that include a response plan for detected data breaches.
- Download Control: Controls and audits access to and download of datasets (if download is permitted).
- Violations: Has procedures for addressing violations of terms-of-use by users and data mismanagement by the repository.
- Request Review: Makes use of an established and transparent process for reviewing data access requests.
Repositories for Scientific Data
See Repositories for Sharing Scientific Data for a listing of NIH-affiliated data repositories.
Related Resources
Repositories for Sharing Scientific Data
Click through the PLOS taxonomy to find articles in your field.
For more information about PLOS Subject Areas, click here .
Recommended Repositories
All data, software and code underlying reported findings should be deposited in appropriate public repositories, unless already provided as part of the article. Repositories may be either subject-specific repositories that accept specific types of structured data and/or software, or cross-disciplinary generalist repositories that accept multiple data and/or software types.
If field-specific standards for data or software deposition exist, PLOS requires authors to comply with these standards. Authors should select repositories appropriate to their field of study (for example, ArrayExpress or GEO for microarray data; GenBank, EMBL, or DDBJ for gene sequences). PLOS has identified a set of established repositories, listed below, that are recognized and trusted within their respective communities. PLOS does not dictate repository selection for the data availability policy.
For further information on environmental and biomedical science repositories and field standards, we suggest utilizing FAIRsharing . Additionally, the Registry of Research Data Repositories ( Re3Data ) is a full scale resource of registered data repositories across subject areas. Both FAIRsharing and Re3Data provide information on an array of criteria to help researchers identify the repositories most suitable for their needs (e.g., licensing, certificates and standards, policy, etc.).
If no specialized community-endorsed public repository exists, institutional repositories that use open licenses permitting free and unrestricted use or public domain, and that adhere to best practices pertaining to responsible sharing, sustainable digital preservation, proper citation, and openness are also suitable for deposition.
If authors use repositories with stated licensing policies, the policies should not be more restrictive than the Creative Commons Attribution (CC BY) license .
Cross-disciplinary repositories
- Dryad Digital Repository
- Harvard Dataverse Network
- Network Data Exchange (NDEx)
- Open Science Framework
- Swedish National Data Service
Repositories by type
Biochemistry
* |
*Data entered in the STRENDA DB submission form are automatically checked for compliance and receive a fact sheet PDF with warnings for any missing information.
Biomedical Sciences
Marine Sciences
- SEA scieNtific Open data Edition (SEANOE)
Model Organisms
Neuroscience
- Functional Connectomes Project International Neuroimaging Data-Sharing Initiative (FCP/INDI)
- German Neuroinformatics Node/G-Node (GIN)
- NeuroMorpho.org
Physical Sciences
Social Sciences
- Inter-university Consortium for Political and Social Research (ICPSR)
- Qualitative Data Repository
- UK Data Service
Structural Databases
Taxonomic & Species Diversity
Unstructured and/or Large Data
PLOS would like to thank the Open Access Nature Publishing Group journal, Scientific Data , for their own list of recommended repositories .
Repository Criteria
The list of repositories above is not exhaustive and PLOS encourages the use of any repository that meet the following criteria:
Dataset submissions should be open to all researchers whose research fits the scientific scope of the repository. PLOS’ list does not include repositories that place geographical or affiliation restrictions on submission of datasets.
Repositories must assign a stable persistent identifier (PID) for each dataset at publication, such as a digital object identifier (DOI) or an accession number.
- Repositories must provide the option for data to be available under CC0 or CC BY licenses (or equivalents that are no less restrictive). Specifically, there must be no restrictions on derivative works or commercial use.
- Repositories should make datasets available to any interested readers at no cost, and with no registration requirements that unnecessarily restrict access to data. PLOS will not recommend repositories that charge readers access fees or subscription fees.
- Repositories must have a long-term data management plan (including funding) to ensure that datasets are maintained for the foreseeable future.
- Repositories should demonstrate acceptance and usage within the relevant research community, for example, via use of the repository for data deposition for multiple published articles.
- Repositories should have an entry in FAIRsharing.org to allow it to be linked to the PLOS entry .
Please note, the list of recommended repositories is not actively maintained. Please use the resources at the top of the page and the criteria above to help select an appropriate repository.
Integrations
What's new?
In-Product Prompts
Participant Management
Interview Studies
Prototype Testing
Card Sorting
Tree Testing
Live Website Testing
Automated Reports
Templates Gallery
Choose from our library of pre-built mazes to copy, customize, and share with your own users
Browse all templates
Financial Services
Tech & Software
Product Designers
Product Managers
User Researchers
By use case
Concept & Idea Validation
Wireframe & Usability Test
Content & Copy Testing
Feedback & Satisfaction
Content Hub
Educational resources for product, research and design teams
Explore all resources
Question Bank
Research Maturity Model
Guides & Reports
Help Center
Future of User Research Report
The Optimal Path Podcast
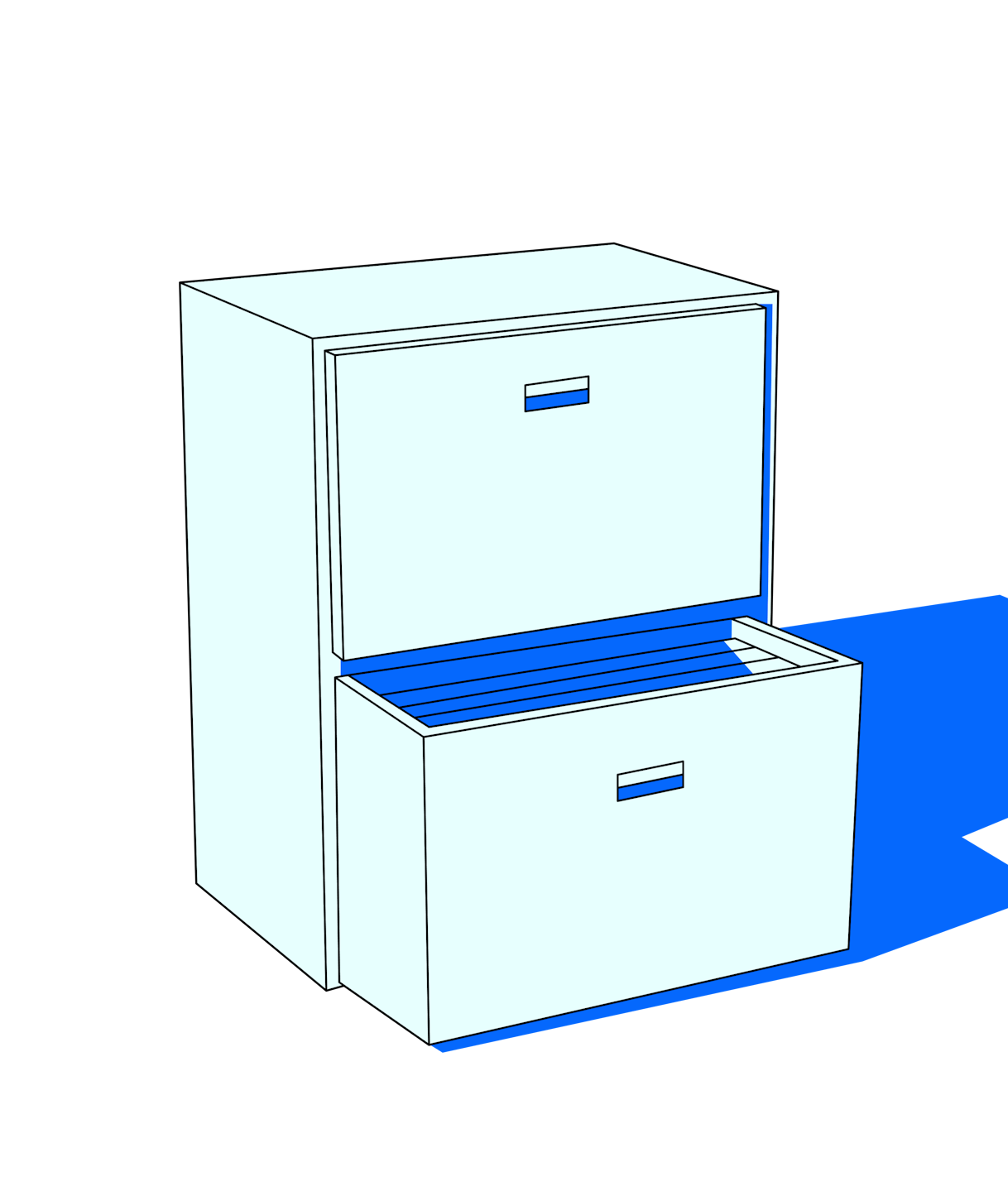
User Research
Jan 23, 2024
How to build a UX research repository (that people actually use)
Extend the shelf life of your research and set your team up for long-term success with a robust research repository. Here’s how to build yours from scratch.
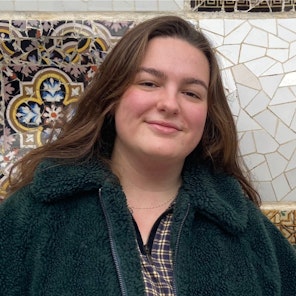
Ella Webber
Every UX research report was once a mountain of raw, unstructured data. User research repositories help collate that data, disseminate insights, democratize research, and spread the value of user research throughout your organization.
However, building (and maintaining) an accessible user research repository is no simple task. Getting people to use it is a whole other ball game.
In this guide, we’ll break down the specifics of user research repositories, some best practices and the benefits of building your own research library, plus how to get started, and our favorite examples of robust research repositories.
Fill your research repository with critical user insights
Drive business success and make informed decisions with Maze to extract valuable insights from user research
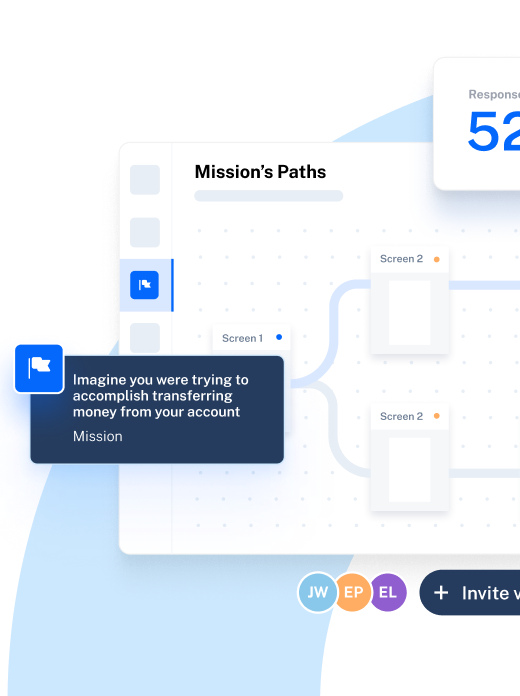
What is a research repository in UX research?
A user research repository is a centralized database which includes all your user research data, UX research reports , and artifacts. Different teams—like design, product, sales, and marketing—can find insights from past projects to contextualize present scenarios and make informed decisions.
Storing all your research data in a single place ensures every team has access to user insights and can use them to make research-driven decisions. Typically maintained by a research operations team, a well-structured research repository is an important step toward breaking down silos and democratizing user research for the entire organization.
If you’re looking to improve research maturity across your organization and start scaling UX research , building a watertight user research repository is your first step.
What’s included in a research repository?
Building a UX research repository can be challenging. Between compiling all the data, creating a collaborative space, and making it easily accessible to the teams who need it, you might be struggling to identify a start point.
Here’s a checklist of all the essentials to streamline the setup:
✅ Mission and vision ✅ Research roadmap ✅ Key methodologies ✅ Tools and templates ✅ Research findings ✅ Raw data and artifacts
Mission and vision
Whether you have a dedicated user research team or involve multiple departments in the UX research process , you need a clear mission and vision statement to create a shared purpose and foster collaboration. Not only should you include your wider UX research strategy and vision, but a ‘North Star’ for your repository, too.
For example, the mission statement for your repository could be, “Streamline our UX design process and promote informed decision-making with a centralized hub of user feedback and insights.”
Research roadmap
A clear UX roadmap makes it easy to prioritize your research efforts and seamlessly organize your repository. It analyzes your objectives and outlines all upcoming projects in a given timeline. You can use this roadmap to catalog your previous research campaigns and plan ahead .
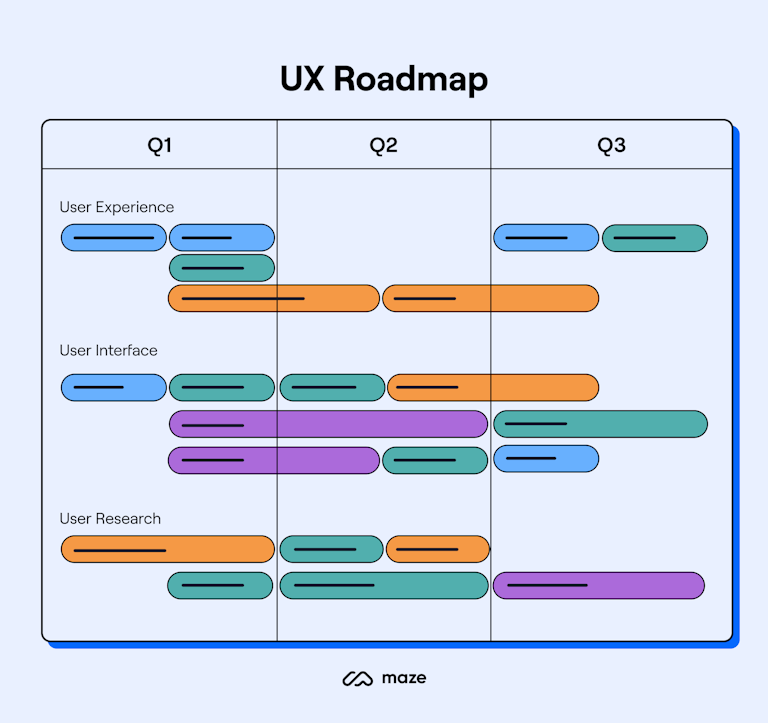
Key methodologies
You should also list all the research methods you follow to create repeatable success. You can save SOPs for different methodologies to minimize the scope of error and set your team members up for success. Mia Mishek , UX Research Operations Program Manager at Pax8 , explains:
“Every repository should include common documents related to the research at hand, such as a brief, moderation guide/test script, and readout. Having all the documents easily accessible allows others to cross-reference while consuming research and use past research as a jumping-off point for further research.”
Tools and templates
Create a list of collaboration and product management tools for different steps in the product research process , such as usability testing , interviews, note-taking, data analysis, and more. Outline these and don’t forget to give quick access links to all your UX research tools .
Outlining instructions and key templates for specific research methods or analysis techniques can be useful. Consider including any tried-and-tested question repositories or best practices.
Research findings
Your repository should include a set of findings from every study. While you can add the final reports for all projects, it’s also a good practice to add quick takeaways and tags to make your collection easily searchable.
If you’ve conducted different types of analysis, it’s worth linking these here, too. Whether that’s a photo of your thematic analysis workshop, a walkthrough video of your results, or a link to digital affinity diagram.
Raw data and artifacts
Alongside research reports, you can store all the raw data from each study, like user interview recordings and transcriptions. Your team members can revisit this data to plan upcoming projects effectively or connect the dots between past and present insights.
Depending on how you store this, you may want to consider keeping piles of raw data in a ‘view only’ or locked area of the repository, to avoid risk of accidental tampering or deletion.
What are the benefits of a research repository?
User research is an ongoing process. The trickiest part for most teams when pursuing continuous research is breaking down silos and establishing a democratized approach to prevent wasteful overlap, unnecessary effort, and a lack of knowledge-sharing.
A good research repository fosters a culture of collaboration and supports user-centric design through collectively prioritizing and understanding your users.
Here are a few core benefits of building a user research repository:
Quickly access user research data
An easily searchable UX research repository makes it easy to filter through a mountain of data and find specific insights without pouring hours into it. Mia emphasizes the importance of making the information easily accessible:
“You should be able to go into the repository, understand what research has been done on X topic, and get the information you’re after. If you need someone else to walk you through the repository, or if there’s missing information, then it’s not doing its job.”
By creating a self-serve database, you can make all the data accessible to everyone and save time spent on reviewing prior research to feed existing efforts.
Inspire ideas and prioritize future research
A research repository can also help in identifying knowledge gaps in your existing research and highlight topics worth further exploration. Analyzing your past data can spark ideas for innovative features and guide your research efforts.
Different teams can utilize a research repository to help guide the product roadmap on areas that still need to be explored in the app, or areas that need to be revisited.
Mia Mishek , UX Research Operations Program Leader at Pax8
Build a shared knowledge library
One crucial advantage of a repository is that it helps democratize user research. Not only does it highlight the value of research and showcase the efforts of your product and research teams, but by centralizing research findings, you’re making it easier for everyone to make data-informed, user-centric decisions.
A research repository also provides versatility and other use cases to your research insights—from product managers to sales leaders, all stakeholders can access user insights for making research-driven decisions across the organization. Whether that’s informing a sales pitch, product roadmap, or business strategy; there’s endless applications for UX research.
This practice of knowledge-sharing and democratizing user insights is a big step in building a truly user-centered approach to product development.
Contextualize new data with past evidence
Your repository records all the raw data from past projects, making it easier to compare and contrast new findings with previous user research. This data also allows researchers to develop more nuanced reports by connecting the dots between present and past data.
Mia explains how these repositories cut down on the redundant effort of trying to dig up old research data on any topic: “A repository benefits UX researchers and designers because it’s not uncommon to ask what research was done on XYZ area before conducting more research. No one wants to do reductive work, so without a repository, it’s easy to forget past research on similar topics.”
What’s more, research libraries avoid the same research being repeated; instead allowing as many people as possible to benefit from the research, while minimizing the resources and time used.
4 Best research repository tools and templates
You don’t need a specialized tool to create a user research repository. A well-organized, shared Google Drive or Notion teamspace with detailed documentation can be just as effective. However, if you can, a dedicated tool is going to make your life a lot easier.
Here are four research repository tools to consider for storing existing and new research insights on, and working cross-functionally with multiple teams.
1. Confluence
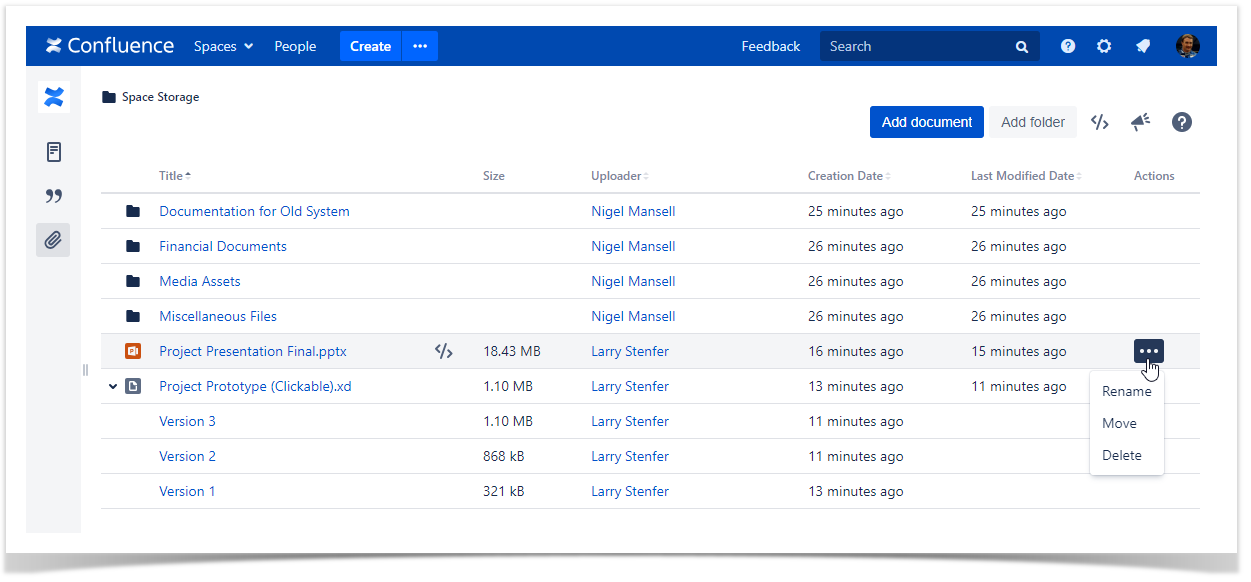
Confluence is a team workspace tool by Atlassian that streamlines remote work. You can use this platform to create research docs from scratch, share them with your team, and save them for future reference. Plus, the tool lets you design wikis for each research study to organize everything—raw data, findings, and reports—in a structured manner.
You also get a centralized space to store data and docs from extra accounts, so multiple people can contribute to and access your repository.
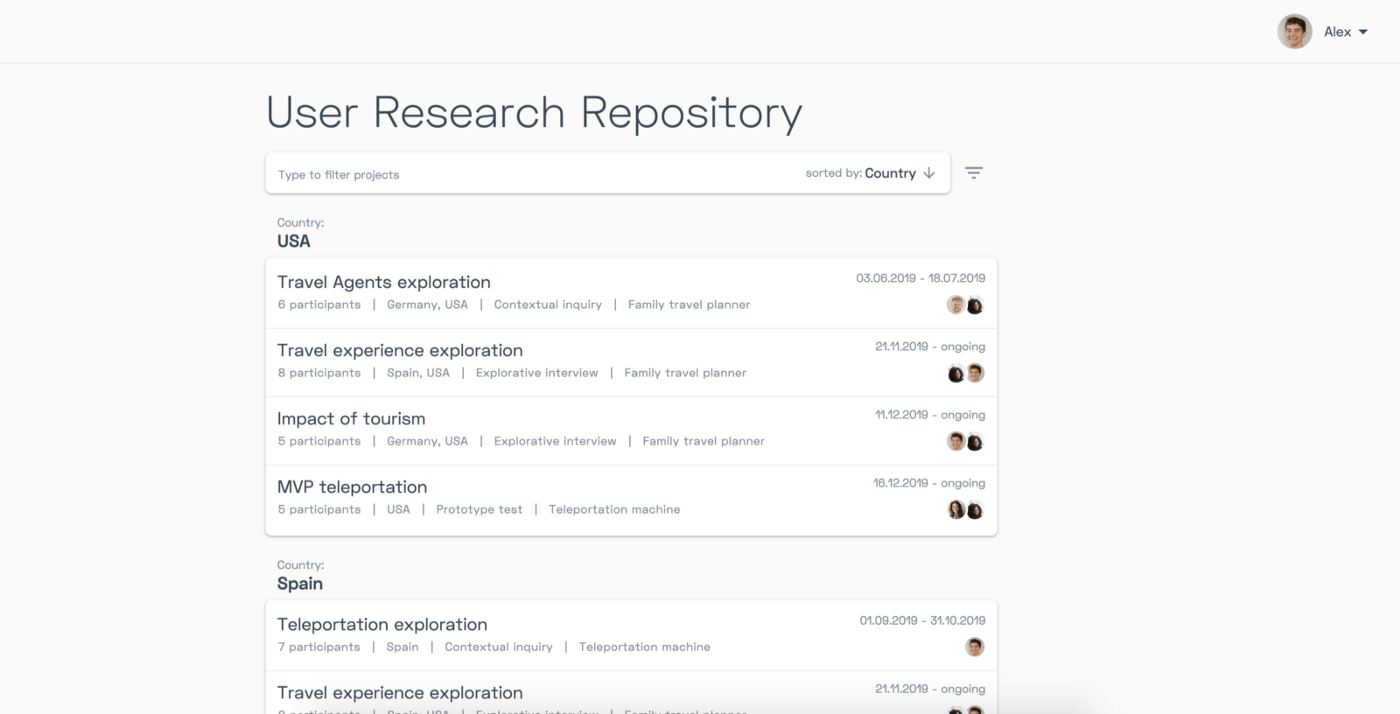
Condens is a centralized UX research and analysis platform for storing, structuring, and analyzing user research data–and sharing those insights across your organization. You can collaborate on data analysis, create pattern recognition, and create artifacts for comprehensive outcomes.
With a detailed research repository guide to help you on your way, it's a great tool for teams of any size. Plus, you can also embed live Maze reports, alongside other UX research and analysis tools.
3. Dovetail
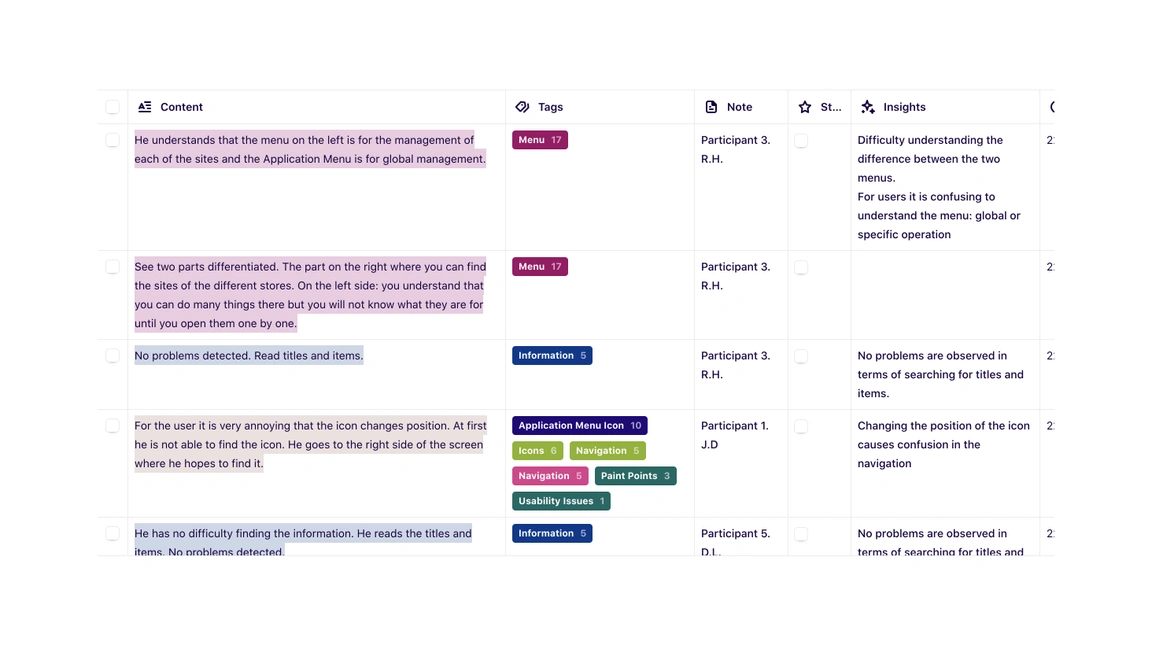
Dovetail is a user research platform for collecting, analyzing, and storing research projects. You can save and retrieve all documents from a single database, while tags, labels, and descriptions also simplify the task of cataloging past data.
The platform gives you a strong search function to quickly find any file or data from the entire hub. You can also use multiple templates to migrate data from different platforms to Dovetail.
4. Airtable
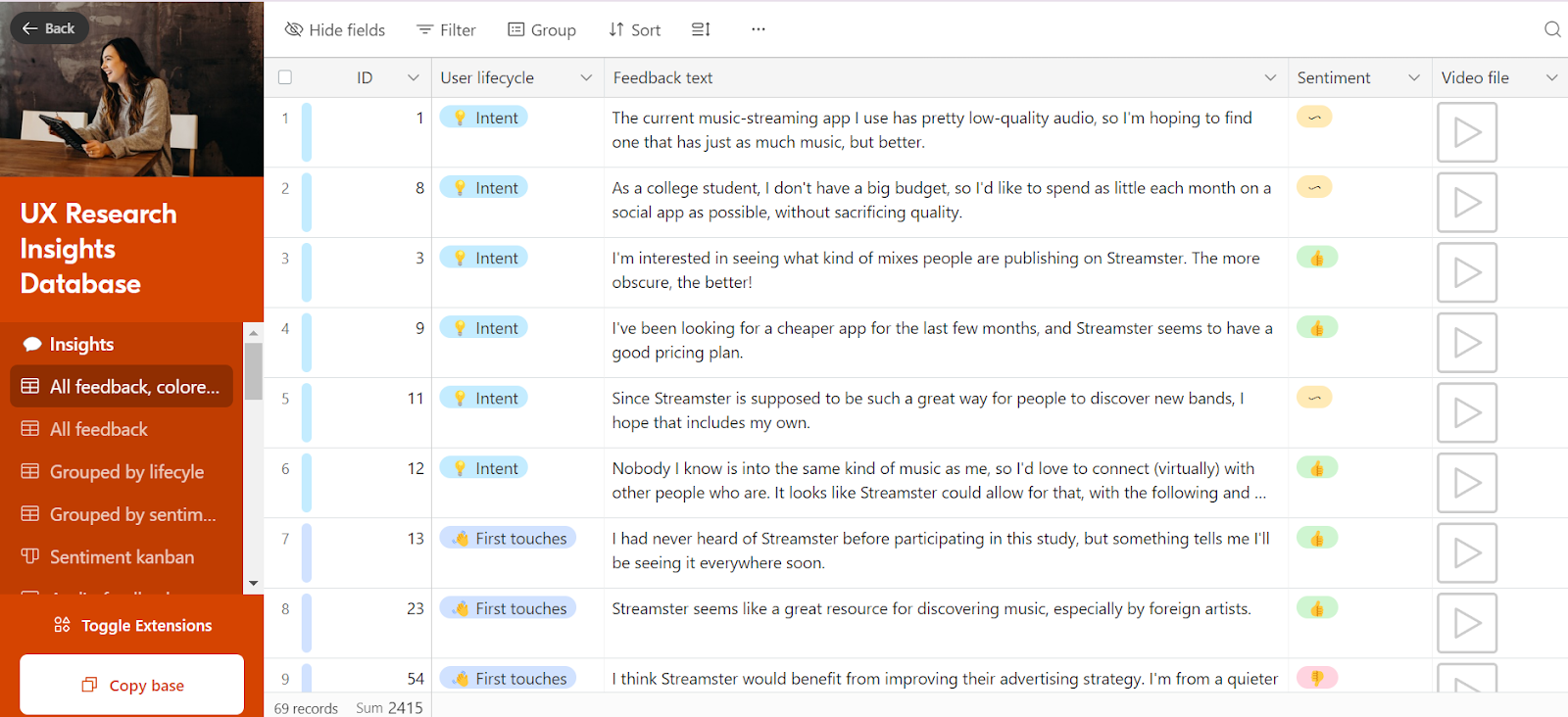
Airtable is a low-code tool for building apps that enables you to create a custom database for your UX research projects. It’s ideal for product teams looking to set up the entire repository from scratch because you need to configure everything independently.
You get a high degree of flexibility to integrate different data sources, design a customized interface, and access data in dynamic views. What’s more, you can build an interactive relational database to request resources from others and stay on top of the status of existing work.
Here’s a research repository database to get started.
Creating a UX research repository: 5 Best practices
Designing a bespoke repository to organize your research requires careful planning, a thorough setup workflow, and continuous maintenance. But once it’s ready, you’ll wonder how your product team survived without it. To get you started, here’s our five best practices to implement this process effectively and kickstart your repository.
1. Define clear objectives for your repository
Start by outlining what you want to achieve with a shared research library. You might want to standardize research methodologies across the board or build alignment between multiple teams to create more consistent outputs.
This goal-setting exercise gives all team members a purpose to pursue in upcoming projects. When they know what success looks like, they can strategically plan research questions and choose analysis methods.
Knowing your objectives will also help shortlist the best research and usability testing tools . You can invest in a good platform by evaluating a few core capabilities needed to achieve your goals (more on that shortly).
2. Create a structure and define taxonomy
You can structure your UX repository as a database with multiple fields. For example, here are a few fields to easily categorize responses when documenting user experience research:
- Key insights
- User quotes
- Criticality
- Sources of knowledge
- Possible solutions that were considered
Besides creating a structure to document a research study, you also need a well-defined taxonomy to help people find information. Defining your research taxonomy will help you categorize information effectively and design consistent naming conventions.
For example, you can create a set of predefined categories for every research study like:
- Focus country: USA, Australia, Canada, France
- Collected feedback: Feature request, feature enhancement, bugs
- Methodology: Usability testing, user interview, survey
- User journey stage: Before activation, power user, after renewal
💡 Less jargon, more alignment
Involve multiple stakeholders when defining the terminology for your library, and check it aligns with any internal Style Guides or glossaries. This ensures alignment from the outset, and makes it easy for everyone to filter results and find what they need.
3. Distribute knowledge through atomic research
Atomic research is an approach to UX research that prioritizes user research data organization. It proposes that you conduct research so that every piece of the project becomes easily reusable and accessible to all stakeholders.
According to the atomic research approach , you need to consider four components to organize your repository:
- Experiments (We did this): Explain the research methodology and the steps you followed in conducting the study
- Facts (We saw this): Document the main findings evident from the data gathered in the study
- Insights (Which made us think): Capture the key insights extracted from analyzing the research data
- Opportunities (So we did that): List the decisions and action items resulting from the research analysis
Using atomic research, you can create nuggets to organize information in your repository.
Nuggets are the smallest unit of information containing one specific insight, like a user quote, data point, or observation. The different types of nuggets to categorize your research data include observations , evidence , and tags . By breaking down a vast study into smaller nuggets, you can make your repository informative at a glance. You can use your defined taxonomy to label these nuggets.
4. Identify the creators and consumers in your team
Before outlining your repository’s structure, you need to define workflows for creating, reviewing, and maintaining the library. Spend some time defining who will:
- Own the setup process and create the overall guidelines
- Access past documents and add contributions consistently
- Maintain the documents for easy accessibility
- Only need to access customer insights
Assigning these roles makes it easy to estimate your team's bandwidth for building and maintaining such a massive library. You can also manage permissions in your repository platform to give everyone access to relevant materials and protect confidential resources.
Mia explains why this is important to make your repository more meaningful for end-users:
“You need to keep in mind the JTBD (jobs to be done) framework when building a repository. What do the folks accessing your repository need to do? Who are those people? You need to build your repository with the purpose of those distinct users.”
5. Shortlist and finalize tools based on your goals
When evaluating different research repository tools, consider your requirements and compare different platforms against the essential features you need for this repository. If you’re creating one for the first time, it’s okay to create an experimental setup to understand the impact.
Here are a few key factors to consider when shortlisting research repository tools:
- Ease of setup and use: Choose a platform with a gentle learning curve, especially if you have a big team with multiple members. A quick setup and user-friendly interface can maximize adoption and make your repository more accessible.
- Collaboration capabilities: A good repository lets you interact with different team members through comments, chat boxes, or tags. You can also manage permissions and set up different roles to share relevant research with specific stakeholders and team members .
- Tagging and searchability: Your repository is only as good as its ability to show precise search results for any keyword. Consider the ease of labeling new information and test the search function to check the accuracy of the results.
- Export and integrations: You’ll need to export some data or streamline your entire research ops setup by integrating different tools. So, evaluate each tool’s integration capabilities and the options to export information.
Plus, your ideal tool might be a combination of tools. For example, Steven Zhang , former Senior Software Engineer at Airtable, used a combination of Gong and Airtable when first building a UX research repository . It’s about considering your needs and finding what works for your team.
Democratize user research in your organization
A UX research repository gives you easy access to insights from past projects, and enables you to map new insights to old findings for a more nuanced understanding of your users.
More importantly, building a single source of truth for your entire organization means everyone on your team can access research data to inform their projects.
Different teams can use this data to make strategic design decisions, iterate product messaging, or deliver meaningful customer support.
Sound good? That’s what we thought—build your repository today to evangelize and democratize UX research in your organization.
Need a seamless solution to collect meaningful research insights?
Maze helps you collect and analyze research to find purposeful data for your product roadmap
Frequently asked questions about UX research repository
How do I create a user research repository?
You can create a user research repository with these best practices:
- Define clear objectives for your repository
- Create a structure and define taxonomy
- Distribute knowledge through atomic research
- Identify the creators and consumers in your team
- Shortlist and finalize tools based on your goals
What makes a good research repository?
A good research repository tells the team's mission and vision for using research. It's also easily searchable with relevant tags and labels to categorize documents, and includes tools, templates, and other resources for better adoption.
What’s the purpose of a research repository?
A research repository aims to make your UX research accessible to everyone. It democratizes research operations and fosters knowledge-sharing, giving everyone on your team access to critical insights and firsthand user feedback.

About Us Subscribe to our Newsletter

The University of Arizona Research Data Repository (ReDATA) is the institution's official repository for publicly archiving and sharing research materials (e.g., data, code, images, videos, etc.) created by University of Arizona researchers. ReDATA helps the UArizona community:
- Comply with funder and journal data sharing policies
- Comply with university data retention policies for primary data
- Archive data associated with published articles, theses/dissertations, and completed research projects
In support of the FAIR (findable, accessible, interoperable, reusable) data principles, all submissions are assigned a Digital Object Identifier (DOI) for citation purposes and undergo a curatorial review by a ReDATA team member prior to publication.
How to include ReDATA in grant applications
How to prepare and deposit materials
Tutorials, General Information, FAQs
Guidance for submitting data associated with journal publications
About the ReDATA team
You can contact the ReDATA team by scheduling a consultation or you may email us directly at [email protected]
Follow us on LinkedIn , Instagram , or Mastodon
Was this page useful?

- NIH Grants & Funding
- Blog Policies
NIH Extramural Nexus

Unlocking the Potential of Data Reuse: Award Challenge for Researchers

Biomedical research has generated millions of datasets and we are issuing a $500,000 challenge to get the most out of them. The 2024 DataWorks! Prize, a partnership between the NIH Office of Data Science Strategy and the Federation of American Societies for Experimental Biology (FASEB) , invites you to conduct a secondary research analysis project that generates new scientific findings from existing datasets. Data reuse plays a critical role in advancing biomedical research by making it possible to test new hypotheses without duplicating data collection efforts, and this challenge aims to highlight innovative and impactful secondary analysis projects.
The future of biological and biomedical research hinges on researcher’s ability to share and reuse data. The DataWorks! Prize is an opportunity for the research community to complete a secondary analysis project and receive recognition and rewards for these innovative and impactful research endeavors. This is the third iteration of the DataWorks! Prize, a challenge focused on recognizing and rewarding the impact of data sharing and reuse on human health.
To participate, research teams must submit a proposal for a secondary analysis research project that incorporates data from one or more of the Generalist Repository Ecosystem Initiative (GREI) repositories ; other repositories including domain-specific repositories can be used as well (. If selected to advance, teams will receive up to a $25,000 award to work on the completion of the proposed project. This year, the DataWorks! Prize will award up to $500,000 across ten awardees, including a Grand Prize winner ($100,000) and up to two Distinguished Achievement Awards ($75,000 each).
Beyond monetary awards, the DataWorks! Prize offers the research community a chance to learn from peers and apply those lessons to their research practices. The innovative approaches and tools from prize winners will be highlighted in a symposium, providing a platform that supports community learning–where researchers can share their methods, lessons learned, and best practices, thereby fostering a culture of continuous improvement and collaboration within the scientific community.
The DataWorks! Prize is part of the NIH Office of Data Science Strategy’s ongoing support for data stewardship and management, in alignment with the NIH Data Management and Sharing Policy . This policy promotes the management and sharing of scientific data from NIH-funded or conducted research, establishing requirements for Data Management and Sharing Plans and emphasizing good data management practices. It aims to maximize the appropriate sharing of scientific data, with justified limitations or exceptions.
The DataWorks! Prize will be open for submissions on August 14, 2024. Participants must complete the first round of submissions by October 23, 2024. Visit Challenge.gov for more information and to apply.
RELATED NEWS
Before submitting your comment, please review our blog comment policies.
Your email address will not be published. Required fields are marked *
Promoting Data Sharing: The Moral Obligations of Public Funding Agencies
- Original Research/Scholarship
- Open access
- Published: 06 August 2024
- Volume 30 , article number 35 , ( 2024 )
Cite this article
You have full access to this open access article
- Christian Wendelborn ORCID: orcid.org/0000-0002-8012-1835 1 nAff2 ,
- Michael Anger ORCID: orcid.org/0000-0002-9328-510X 1 &
- Christoph Schickhardt ORCID: orcid.org/0000-0003-2038-1456 1
262 Accesses
Explore all metrics
Sharing research data has great potential to benefit science and society. However, data sharing is still not common practice. Since public research funding agencies have a particular impact on research and researchers, the question arises: Are public funding agencies morally obligated to promote data sharing? We argue from a research ethics perspective that public funding agencies have several pro tanto obligations requiring them to promote data sharing. However, there are also pro tanto obligations that speak against promoting data sharing in general as well as with regard to particular instruments of such promotion. We examine and weigh these obligations and conclude that all things considered funders ought to promote the sharing of data. Even the instrument of mandatory data sharing policies can be justified under certain conditions.
Similar content being viewed by others
Preparedness for Research Data Sharing: A Study of University Researchers in Three European Countries
Data Decisions and Ethics: The Case of Stakeholder-Engaged Research
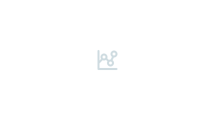
Openness in Big Data and Data Repositories
Avoid common mistakes on your manuscript.
Introduction
The potential benefits of sharing research data for science and society have been widely acknowledged and emphasised. Some disciplines or sub-disciplines have a longstanding tradition and well established practices of data sharing, for instance, astrophysics, climate research and biomedical genomic research. However, despite various efforts to promote and encourage data sharing, for instance by scientific journals, it is still not common practice in most fields of the sciences. As public funding agencies have considerable influence on both the scientific communities as well as the individual researchers, the question arises whether they are morally obligated to promote data sharing. In order to answer this question, we examine the following more specific three questions from the perspective of research ethics:
Do public funders have general pro tanto moral obligations that require them to promote data sharing?
Do public funders have general pro tanto moral obligations that speak against promoting data sharing?
What pro tanto moral obligations have to be considered in the particular case of using mandatory data sharing policies, i.e., policies that require researchers to share data?
Answering these questions is a desideratum of (bio)ethical research on issues of data sharing. Although it is stated that individual researchers have a scientific responsibility (Bauchner et al., 2016 ; Fischer & Zigmond, 2010 ) and even a moral obligation to share data (Schickhardt et al., 2016 ), the moral responsibilities and obligations of public funding agencies in matters of data sharing have not been discussed systematically and explicitly from the perspective of research ethics. While it is common to postulate that funders “should” encourage data sharing or that it is their “responsibility” to do so, we want to carry out an in-depth ethical analysis of funders’ moral obligations. In doing so, we also contribute to an analysis of what funders are generally morally obligated to – another question that has thus far been rather neglected in research ethics and discussed primarily in terms of priority-setting and with regard to the general obligation to benefit society (Pierson & Millum, 2018 ; Pratt & Hyder, 2017 , 2019 ). Thus, we will provide a broader analysis of general moral obligations of funders and evaluate what they imply with regard to promoting data sharing in particular.
We proceed as follows: After some preliminary remarks in Sect. " Preliminary Remarks ", we provide a brief review of empirical data on the current status quo of data sharing in Sect. " The Current State of Data Sharing and of Promoting Data Sharing ". In Sect. " The Moral Obligations of Funders and the Promotion of Data Sharing ", we set out that funders have three general moral pro tanto obligations that require them to promote data sharing. In Sect. " Further Relevant Moral Obligations ", we examine two pro tanto obligations that both speak in favour of and against promoting data sharing. We conclude Sect. " Further Relevant Moral Obligations " by weighing all pro tanto obligations. In Sect. " Mandatory Data Sharing Policies and Academic Freedom ", we ethically assess the specific instrument of promoting data sharing by way of mandatory policies with regards to academic freedom. We conclude and summarise our arguments in Sect. " Summary and Conclusion ".
Preliminary Remarks
In the following, we use the term “research data” and “data” as referring to digital data that is collected and/or generated during a research project. We use the term “data sharing” as referring to the act of making data available for other researchers – either for the purpose of transparency of studies and replication of published research results or for the purpose of other researchers using the data for their own research questions and projects (secondary research use). Footnote 1 Data sharing is increasingly supposed to meet the requirements of the FAIR principles, i.e., data should be findable, accessible, interoperable and re-usable (Wilkinson et al., 2016 ). Data can be shared in various ways, for example via open access or restricted or controlled access, and by using Data Use and Access Committees or data sharing licenses. Footnote 2 Restricted or controlled access comes, for instance, with additional data protection requirements when personal data are involved. Data sharing activities (and data sharing policies by funders) must comply with the applicable local laws and regulations. In EU countries, the possibilities for international sharing of non-anonymous data are dependent on the EU GDPR, making personal data sharing difficult between EU countries and the US, for example. As to legal challenges to international data sharing raised by local laws, there are possible legal approaches (contracts) and technical solutions such as code-to-data approaches, when the data remains at the location of the data producer or the repository and is only analysed there on behalf of the other researcher. Footnote 3
We define public funding agencies, following the European Commission Joint Research Centre (2017), as organisational entities that distribute public funding for research on behalf of either regional, national, or transnational governments. The definition covers both i) funding agencies operating at arm’s length from the public administration and enjoying relative autonomy from the government and ii) ministries and offices within the government that fund research projects. The definition comprises of centralised and non-discipline specific agencies such as the German Research Foundation (Deutsche Forschungsgemeinschaft), de-centralised and discipline specific agencies such as the National Institutes of Health in the US or the UK Research Councils, as well as international funding agencies and bodies such as the European Commission. When we speak of research funding, we refer to funders who grant funds to individual researchers or groups of researchers (collaborative projects or research consortia). Against the background of the existing organisation of the (academic) science system with its systematic competition between researchers and the importance of scientific publications, we assume that funded researchers use the funding to seek and publish new findings and that they do so in a somehow exclusive way that does not involve the immediate disclosure of all data and results. The tendencies of competition, exclusive use of data and the pursuit of (more or less) exclusive first scientific publications of previously unknown research results are the reasons why funders' policies on sharing research data and overcoming data secrecy are important, at least at some point in the project and research cycle. Traditionally, research projects funded in this way tend to be hypothesis driven. However, as research methods, the nature of projects and the associated research funding evolve rapidly and potentially change in the era of Big Data and AI, the boundaries are blurring, and some things may change. There might be more scientific community-led research projects that are designed to be less exclusive and competitive, with community participation, immediate disclosure, and data sharing at the forefront from the start. A historical example is the Human Genome Project. Funding of such community-led research projects is not the focus of our paper, but community-led research is worth mentioning and discussing in further research.
As public funders are public (or even state) institutions and spend public money that they receive from the government, their moral obligations are related to their public and therefore political character. Our analysis of the moral obligations assumes a liberal-democratic and rights-based normative-ethical framework. To put it simply, public institutions are normatively conceived as "by the people, for the people and of the people", and citizens, including researchers, have fundamental liberal rights vis-à-vis the state and public institutions, especially negative rights that protect them from state interference. These moral rights, which play an important role in our analysis, include academic freedom and the rights to privacy and informational self-determination.
We confine our analysis in this article only to the promotion of data sharing within academic science and exclude the question of the promotion of data sharing from publicly funded academic science with private for-profit companies.
We do not limit our argument to funders that focus on a particular scientific discipline (for instance, biomedical funders), as we believe that the pro tanto obligations we will attribute to funders do not depend on the specific characteristics of particular scientific disciplines. However, we think that when applying our framework in practice, context factors that depend on the features of a certain discipline or a specific research project need to be taken into account.
Some of the following arguments for the moral pro tanto obligations of public funders can be translated mutatis mutandis to private funders, but not all of them can. Particularly those arguments that refer to the special status of public funders as public institutions that spend public money and have particular responsibilities towards the public and the rights of citizens cannot be applied to private funders. The obligations of private funders call for a separate analysis in a separate paper.
This paper presents an ethical analysis of the moral obligations of funders and is not concerned with legal rights and obligations that pertain to funders in a particular national state or legal area such as the European Union. We assume that the moral obligations presented below do not conflict with the legal requirements of (public) funders in any legal context. However, our claims that funders have a moral obligation to promote data sharing and that they should also implement mandatory data sharing policies under certain circumstances have implications for the revision of (templates for) future legally binding funding contracts between funders and funded researchers. In this respect our ethical analysis has legal implications.
We take a pro tanto obligation as an obligation that has some weight in determining what an actor morally ought to do all things considered (Dabbagh, 2018 ). Suppose I promise my friend to visit her tonight; however, my daughter is sick, and I ought to stay with her. I have then two pro tanto obligations that prescribe conflicting actions. To find out what I am obligated to do all things considered , I must find out which of the two obligations weighs heavier. Footnote 4 Therefore, when we examine pro tanto obligations that require the promotion of data sharing, these obligations must be weighed against other pro tanto obligations that speak against such promotion.
The Current State of Data Sharing and of Promoting Data Sharing
As to the current state of data sharing, there are differences across scientific disciplines (Tedersoo et al., 2021a , 2021b ). Some disciplines, such as astrophysics, climate research or genomic research, have a long history of data sharing. For instance, genomics research paved the way with the important and pioneering Fort Lauderdale (Fort Lauderdale Agreement, 2003 ) and Bermuda principles (First International Strategy Meeting on Human Genome Sequencing, 1996 ) on data sharing (Kaye et al., 2009 ) within the revolutionary and community driven Human Genome Project and has created a genomic commons, i.e., openly available data bases for genetic and genomic driven biomedical research (Contreras & Knoppers, 2018 ; National Cancer Institute; National Library of Medicine). With the exception of some more advanced scientific disciplines or sub-disciplines, the sharing of research data for purposes of transparency and secondary use still remains the exception rather than the norm in most fields and disciplines of the sciences (Danchev et al., 2021 ; Gabelica et al., 2022 ; Naudet et al., 2021 ; Ohmann et al., 2021 ; Thelwall et al., 2020 ; Watson, 2022 ; Strcic et al., 2022 ; Gorman, 2020 ; Towse et al., 2021 ). While there is an increased awareness of the benefits and importance of data sharing in all of the sciences and although various initiatives of funders and journals promote data sharing, for instance through data sharing policies, data sharing is still not common practice. Several studies report rather low rates of compliance with data sharing expectations or requirements of funders and journals (Couture et al., 2018 ; Federer et al., 2018 ; Gabelica et al., 2022 ; Naudet et al., 2018 , 2021 ; Danchev et al., 2021 ). Studies also report a gap between high in-principle support for data sharing, and low in-practice intention (Tan et al., 2021 ).
It is frequently emphasised that funders should improve and intensify their current efforts to promote data sharing. Some see the need to create incentives, for example by including a record of past data sharing as an additional criterion for the reviews of grant applications (Perrier et al., 2020 ; Terry et al., 2018 ). Since the majority of funders’ data sharing policies do not strictly require the sharing of data (Ohmann et al., 2021 ), some authors call for stronger policies with strict requirements for data sharing (Couture et al., 2018 ; Naudet et al., 2021 ; Ohmann et al., 2021 ; Sim et al., 2020 ; Stewart et al., 2022 ; Tedersoo et al., 2021a , 2021b ) Footnote 5 and contest the lack of monitoring and enforcing compliance (Couture et al., 2018 ; Kozlov, 2022 ). However, as a series of interviews shows, funders struggle to implement data sharing requirements, incentives, monitoring, and sanctions for non-compliance for various reasons (Anger et al., 2022 , 2024 ).
In consideration of the foregoing and from the perspective of research ethics, the question arises whether public funders are morally obligated to promote data sharing. To answer this question, in the next section we set out a description and analysis of funders' general moral obligations and their relevance for data sharing.
The Moral Obligations of Funders and the Promotion of Data Sharing
We will argue that funding agencies have several general moral pro tanto obligations requiring them to promote data sharing: The obligation to benefit society, the obligation to promote scientific progress as such and the obligation to promote scientific integrity. Our methodological approach consists of first introducing and explaining the individual moral obligations in order to then briefly justify them with reference to plausible and, for the most part, generally shared fundamental considerations, values or norms.
The Obligation to Benefit Society
Publicly funded research should benefit society, or, as it is sometimes put, it should have social value. Footnote 6 As a requirement for public funders, this means funders should base their decisions on considerations of social value. Barsdorf and Millum ( 2017 ) argue that funders ought to consider the social value in particular in their priority-setting, i.e., when setting goals and priorities for the research they fund. We extend the obligation to promote social value to all decisions and actions of public funding agencies. Footnote 7 Benefitting society or social value is sometimes conceptualised in terms of well-being. The concept of well-being is notoriously controversial in philosophy (as it relates to the complicated and controversial topic of the “good life”). In research ethics, the benefits at stake in the social value obligation are sometimes framed more pragmatically, for example when Resnik ( 2018b ) (following Kitcher 2001 ) states that benefits are “practical applications in technology, industry, medicine, engineering, criminal justice, the military, and public policy”, and that these applications “can also produce economic growth and prosperity”. We limit our conception of social value (benefit) to a more basic understanding (which does not include potentially problematic or controversial elements such as military and economic growth): We understand it in terms of the basic goods of health and wealth (housing, food, employment, income, etc.), infrastructure development (for communications, travel, etc.), and environmental protection (as natural resources).
What are the justifying reasons for this obligation? First of all, it must be pointed out that the obligation can be understood in different ways, depending on whether the population to be benefited is the local or the global population. Barsdorf and Millum ( 2017 ), for instance, argue that for health research the social value obligation of funders is towards the global and not the local (national) population of the funders’ country. In the literature, this question (local vs. global) is controversial. In general, the controversial positions on this question also depend on the justification one is willing to accept for the obligation. For instance, if one justifies the obligation as owed to the citizens as tax payers who finance the state and the public funder via taxes, then it is rather obvious to understand social value as benefit for the national tax paying population. In contrast, if one considers the social value obligation of funders as owed to all humans all over the world, it suggests itself to understand the social value broadly in terms of global benefit for all humans. Such a global understanding of the social value obligation could be justified with considerations of beneficence towards every human being or with a universalistic-egalitarian account of human rights. Global understandings of the obligation are likely to give priority to poor populations of the global South. We deem a combination of a local and a global understanding as being the most plausible one: funders have a primary obligation to foster social value on the national level, and an additional (weaker) social value obligation on a global level. But even this combined view raises questions and cannot be elaborated here. Most importantly for the purpose of our paper, we believe that the question concerning the understanding of the social value obligation(s) of funders (towards national vs global population or both) is not relevant for our question about the promotion of data sharing by funders. At first glance it might seem that a local reading of the social value obligation suggests that funders should promote sharing of research data only among local/national researchers. However, the contrary is much more plausible, at least for the academic sciences. Most fields of modern academic scientific research are international endeavours and advancements are achieved through multiple and interacting contributions from scientists from different countries. In most disciplines, there is no such thing as a „national current state of scientific progress “. As for sharing research data from the academic and publicly financed sciences with private for-profit companies, it might be plausible to assume that sharing data only with national companies is more likely to benefit the national population than sharing data with for-profit companies from abroad. However, this assumption can also be challenged, for example, in light of the rapid and effective development of vaccines during the covid pandemic. Most importantly, the sharing of research data from the publicly funded academic sciences with private for-profit companies is a very specific topic that we do not address in this paper. Footnote 8 As far as sharing of research data between academic researchers is concerned, it is plausible to assume: The more data are shared on a national and international level, and the more science advances – which in almost all scientific disciplines occurs as an international advancement -, the more likely national populations will benefit.
A last and more specific reason for funders’ obligation to foster social benefit is the following, which applies only to research involving humans or animals: If funders fund research that exposes animals and humans to risks and burdens, the funding can only be justified if the potential benefits for society are maximised (National Commission for the Protection of Human Subjects of Biomedical & Behavioral Research, 1978 ; World Medical Association, 2013 ). Footnote 9
The concept of social value refers to (classical and much debated) questions of distributive justice: Of all persons concerned, who should benefit how much ? Following Barsdorf and Millum, we think the obligation to benefit society, i.e., the social value obligation, should be understood according to a prioritarian account of social value. On a prioritarian account, benefits should be distributed such that the distribution (expectedly) maximises “weighted well-being” (or in our terms “weighted social benefit”), i.e. the well-being of the worse off gets some priority in the distribution of benefits.
Let's put this in the following proposition and call it the social value obligation for public funders:
Funders have a pro tanto obligation to align their decisions and actions in such a way that the research they fund maximises weighted social benefit. Footnote 10
Now, what is the relevance of the social value obligation for matters of promoting data sharing? We develop our answer step by step:
First step . Data sharing has the potential to optimise research in terms of i) progressiveness, ii) cost and iii) quality (Fischer & Zigmond, 2010 ; Sardanelli et al., 2018 ). Ad i) The sharing of research data accelerates research, enables more cooperation and collaboration between researchers and disciplines, allows for the integration and pooling of data from disparate sources into large data sets, and bears the potential for innovative research, meta-analyses and new lines of inquiry that can lead to better diagnoses and treatments. Ad ii) It reduces costs and is efficient as reusing the data increases the value of the initial investment. Ad iii) It allows research findings to be verified or reproduced based on the original data and thus increases the quality of research and potentially reduces “research waste” (i.e., research with questionably quality).
Second step . Given this efficiency-, quality- and progress-enhancing potential of data sharing, it is rational to assume that the following holds true: A world in which funded researchers share their data is better in terms of social value than a world in which funded researchers do not share their data. Notice that this holds true only under the following conditions: a) Funders must set research funding priorities according to the social value obligation. It is plausible to assume that only the sharing of data from research projects that were selected according to the right priorities (expectedly) maximises weighted social benefit. b) The funding of secondary use and decisions on data access for secondary use must be aligned to the social value obligation as well. Footnote 11
Third step . From the claim that a world in which funded researchers share their data is better in terms of social value it does not directly follow that funding agencies are obligated to promote a world in which researchers share their data, for two reasons:
If there are alternative actions than promoting data sharing that lead to a larger increase in weighed social benefit and that cannot (for cost or other reasons) be taken together with promoting data sharing, then these alternative actions should be taken. For instance, perhaps an initiative to promote translational biomedical research increases weighed social benefit more than the promotion of data sharing and the funder's budget can only finance one of the two initiatives.
Realising a world in which researchers share data comes with costs, for instance for warranting long-term storage and data availability or for incentivising data sharing. Hence, it may be that the means to realise a data sharing-world are so costly that they cancel out the benefits data sharing brings, so that realising this world does not maximise weighted social benefit and ought not to be done.
However, we think that both possibilities are very unlikely. Ad 1. We deem it highly unlikely that there are alternatives that are incompatible with promoting data sharing and more efficient in terms of social value. Ad 2. We think that the means to realise a world in which researchers share their data are not so costly that they cancel out the benefits. For instance, incentivising data sharing or making data sharing mandatory are means that can be expected to promote data sharing without being too costly. Footnote 12
Therefore, we conclude: To fulfil the social value obligation, funders pro tanto ought to promote data sharing. Footnote 13
This conclusion leaves open which specific means of promotion funders are required to take. Since there are many ways of promoting data sharing, some of which are cheaper, some of which are more effective, the social value obligation – in principle – requires a specific means of promotion. For example, incentivising data sharing (for instance, through data sharing prizes or other forms of recognition) might be cheaper but less effective, whereas mandatory policies in combination with monitoring and sanctioning might be more expensive but lead to a greater extent of data sharing. It is an empirical question which of these different means (or combination of means) maximises weighted social benefit (for each situation of each individual funder). We cannot answer this question here. For now, we confine ourselves to the conclusion that the social value-obligation pro tanto requires funders to promote data sharing and leave it open which specific means of promotion they ought to apply. Footnote 14
The Obligation to Promote Scientific Progress
In addition to the social value obligation, public funding agencies have a pro tanto obligation to promote scientific progress. Since scientific progress is likely to increase the social value of scientific research, one reason for funders’ obligation to promote scientific progress is the already discussed social value obligation. However, beyond social value there are also other reasons for the obligation to promote scientific progress and these reasons ground an independent obligation to promote scientific progress. In the following, we focus on these reasons that justify the obligation to foster scientific progress independently from social value.
In democratic countries, public funders have an obligation to promote scientific progress, i.e., the growth of (significant) scientific knowledge and understanding, Footnote 15 because it is their mandate to support a science system that is geared towards producing scientific knowledge (independently of considerations of social benefits). In most democratic countries this mandate is institutionalised on a constitutional level. In this sense, funders owe this obligation to the (democratic) public and the citizens.
There is a set of further reasons that justify the obligation of funders to support the scientific system and foster scientific progress with considerations of the value of scientific knowledge and progress. The value of science and scientific progress touches on complex questions about whether knowledge is valuable in itself and/or (only) insofar as it is somehow conducive to realising other values or ends. We do not want to take a position here on the hotly contested question about whether scientific knowledge (or progress) are intrinsically valuable (end in itself). Footnote 16 We just want to point to the aspects of knowledge that make knowledge instrumentally valuable apart from its instrumental value for the benefits of society. i) Scientific knowledge can be instrumentally valuable when it satisfies “human curiosity” (Kitcher, 2001 ) and the desire for a practically disinterested understanding of the natural world. ii) Scientific knowledge is a precondition and a contributory factor for the ability and freedom of “pursuing our own good in our own way” (Mill, 2008 ) and making reflective decisions about the goals of our own lives. By expanding our understanding of the world and our place in it, scientific progress can contribute to the exercise of this elementary freedom and can thus be seen as valuable for a self-governed and autonomous life (Kitcher, 2001 ; Wilholt, 2012 ). iii) scientific knowledge and progress is valuable for a functioning democracy insofar as (growth of) knowledge is a requirement for processes of informed deliberation, opinion-forming and decision-making (Brown & Guston, 2009 ). Now, this set of three reasons (i-iii) could be understood as reflecting not only the values and interests of the citizens (or tax payers) of the funder’s country, but also the values and interests of all people all over the world. Although it is plausible to some extent that the three reasons also reflect values or interests of people around the world, we do not think that this can establish a relationship in terms of strong moral rights and obligations between the global population and the local funder. Due to the rather loose relationship between persons in each country of the world on the one hand and the local state and funder on the other hand, only rather weak reasons for funders to promote scientific progress could result from the global understanding of the three reasons.
So far, we have argued that the obligation of funders to promote scientific progress is primarily owed to the public and the citizens (and rather weakly to the global population). But of course the question arises whether funders owe the promotion of scientific progress also to scientists or the scientific community. We think that this is the case. Scientists have the professional obligation to strive for scientific knowledge and progress. To fulfil this professional obligation, they depend on the scientific system in which funders play an important role. Scientists need a functional system that is designed to enable and promote scientific progress. Therefore, it is plausible that funders owe the obligation to promote scientific progress to the scientists as well.
We take the scientific progress obligation as follows:
Funders have a pro tanto obligation to align their decisions and actions such that the research they fund maximises scientific progress.
What relevance does this obligation have when discussing funders’ role in promoting data sharing? First and in general terms, this obligation to maximise scientific progress does not necessarily require funders to exercise intensive control and strong intervention in science. Keeping funders largely out of the methodological and content-related decisions of researchers is plausibly conducive to a functioning and progress-making scientific system. However, specific measures or interventions on the part of funders (for instance through policies) might have the potential to promote scientific progress. The promotion of data sharing plausibly is such an intervention: As we argued in Sect. " The Obligation to Benefit Society ", a scientific system in which researchers share their data can be expected to be a more efficient, effective, and innovative scientific system, and this means that it is also a better system in terms of scientific progress than a system in which researchers do not share data. Funders can contribute to realising such a system through various means (such as, for instance, data sharing policies) and thus promoting scientific progress.
However, as it is the case of the social value obligation as well, it does not follow directly that funders are obligated to promote data sharing. This depends on whether there are other means than promoting data sharing which are more conducive to scientific progress (and which cannot be taken together with the promotion of data sharing). Again (as with the social value obligation), this is an empirical question that we cannot answer here. Nonetheless, we think it is plausible to assume that promoting data sharing is an effective and efficient means to promoting scientific progress and that it is rather unlikely there are other more efficient and effective actions or means, which, at the same time, are incompatible (for cost or other reasons) with the promotion of data sharing. Footnote 17
Accordingly, to fulfil their moral obligation to use the resources at their disposal to maximise scientific progress requires them to promote data sharing.
The Obligation to Promote the Epistemic Integrity of Research
Public funding agencies have an obligation to promote the integrity of the research they fund—a view which is widely held (Bouter, 2016 , 2018 , 2020 ; Mejlgaard et al., 2020 ; Titus & Bosch, 2010 ), but not systematically developed and justified. To give a more detailed account of this obligation, we start with clarifying the concept of research integrity.
Research integrity relates to a set of professional norms and obligations that morally regulate and prescribe how researchers ought to conduct research. These norms and obligations can be differentiated between epistemic and socio-moral norms and obligations . Footnote 18 Epistemic norms or obligations are grounded in the goals or nature of science (Resnik, 1998 ), i.e., (roughly) the goals to obtain knowledge and understanding through reliable methods of inquiry. These obligations prohibit misconduct that is problematic from the point of view of epistemic rationality . Epistemic obligations are, for instance, the obligation not to fabricate, falsify, or misrepresent data. Epistemic obligations form what one might call epistemic research integrity . We take epistemic research integrity to be mainly about avoiding practices that lead to deception, inaccuracy, and imprecision in research and (the presentation) of research results. We thus follow Winter and Kosolosky ( 2013 ), who explicate the notion of epistemic research integrity by drawing on the property of deceptiveness and “define the epistemic integrity of a practice as a function of the degree to which the statements resulting from this practice are deceptive.”
Socio-moral obligations result from the fact that research can negatively affect the rights and interests of individuals or groups outside science. Such non-epistemic obligations take into account general responsibilities and potential effects of science for society and humanity and comprises, for example, obligations to obtain consent and to minimise risks for participants and third parties. These socio-moral obligations constitute what one might call socio-moral research integrity .
In the following, we focus only on epistemic research integrity and investigate whether funders’ obligation to promote epistemic research integrity implies that they ought to promote data sharing. We briefly address the relationship between data sharing and socio-moral research integrity in Sect. " Further Relevant Moral Obligations ".
The promotion of epistemic research integrity is required by the two above mentioned obligations of funders to promote social value and scientific progress since epistemic integrity arguably furthers social value and scientific progress or is even a prerequisite for them. Now, the goal of this section is to show that there are reasons independent from social value and scientific progress that ground or justify an obligation of funders to maximize epistemic research integrity. There are two reasons for this as an independent obligation in its own right:
Funders should promote the epistemic integrity of research for two reasons. 1. As public funders are either governmental institutions or at least spend public money, they should ensure that the activities they finance abide by professional norms and standards. Funders are not supposed to spend public money on activities where “anything goes” but rather fund activities and work that are lege artis . This is owed to the citizens and taxpayers and required by the recognition of the value of a rules-based scientific system. 2. Funders must guarantee a fair and rule-based research environment and competition. This is primarily owed to the scientists, among other things, to protect the honest and bona fide researchers against unfair and dishonest competitors.
In the following, we take the obligation of funders to promote epistemic research integrity as follows:
Funders have a pro tanto obligation to align their decisions and actions such that they maximise the epistemic integrity of research.
What does the obligation to promote epistemic research integrity imply for the question of whether funders ought to promote data sharing? To answer this question, we must investigate whether data sharing is required by epistemic research integrity.
To begin, we must differentiate between two different perspectives on epistemic research integrity. One perspective can be labelled as normative - philosophical and takes research integrity as a set of philosophically justified norms. The other perspective can be labelled as the community consensus perspective and takes research integrity as a set of norms that are agreed on and prescribed by the scientific community and that are codified in statements and codes of conduct by scientific societies and associations. These two perspectives usually do not display great discrepancies in terms of concrete norms of research integrity, but in principle they are not necessarily congruent. For reasons of space, we cannot give a systematic answer to the question of which of the two perspectives takes normative priority when they have conflicting norms and prescriptions. However, in the following we first examine the relationship between epistemic integrity and data sharing from a philosophical perspective and then describe how this relationship is treated in relevant codes of conduct and guidelines on research integrity. We will show that the two perspectives converge to some extent, and where they do not clearly converge, we will explain what this means for funders. We will do this in turn for data sharing for transparency (A.) and data sharing for secondary use (B.). Footnote 19
A. Epistemic Integrity and Data Sharing for Transparency
1. Philosophical perspective : Philosophers of science consider practices that enable “each scientist to scrutinize the work of others in his field, to verify and replicate results [and that make] it more likely that flaws will be uncovered” (Haack, 2007 ) to be prescribed by an important epistemic norm. The pertinent norm here is what David Resnik calls the “principle of openness” (Resnik, 1998 ) or what Susan Haack calls the epistemic norm of “evidence-sharing” (Haack, 2007 ). According to this understanding, practices of evidence-sharing enable collective efforts of communicating, reviewing, critiquing, and reproducing the evidence claimed by researchers as supporting their scientific claims and research results, i.e., evidence “which includes the methodology applied, the data acquired, and the process of methodology implementation, data analysis and outcome interpretation” (Munafò et al., 2017 ). Footnote 20 The sharing of evidence is a necessary condition for science as rational communication and argumentation and a requirement for efforts of reviewing and assessing scientific claims. Evidence-sharing can thus be understood as part of an organized skepticism Footnote 21 that increases the credibility of scientific claims and characterises (the ideal of) modern science as a specific social and cooperative enterprise. Following Winter and Koslovsky (2013), the principle of openness and the norm of evidence-sharing can be understood as prescribing practices that prevent and guard against deceptiveness.
One of these practices is arguably data transparency, i.e., transparency with respect to data on which an already published scientific paper is based. We want to explicate at least two reasons for why data transparency is an important norm of evidence-sharing and openness.
Data sharing as a prerequisite for replication . It is widely agreed that replication studies have epistemic value and are an essential and important part of scientific practice at least in a substantial part of the quantitative empirical sciences. Even those who caution against the crisis narrative in connection with failed replications or even doubt the epistemic value of replications for all disciplines (Leonelli, 2018 ) agree with this proposition. However, a precondition and minimal requirement for conducting replication studies is that the original studies can be (computationally or analytically) reproduced , that is, the published findings can be reproduced when the reported analyses are repeated upon the raw data (Hardwicke et al., 2021 ; Nuijten et al., 2018 ; Peels & Bouter, 2021 ). If a result cannot be reproduced, there is no need to even attempt a replication – since something with the analysis or the data must have gone wrong. Therefore, if we agree that efforts to replicate should be enabled and encouraged (due to its important epistemic value for research), then we must also recognise the importance of data transparency.
Data sharing as means for preventing and detecting breaches of epistemic integrity. Although the empirical evidence about the prevalence of scientific misconduct and questionable research practices (QRP) should be handled with care, studies suggest that it is non-negligible. For instance, a survey among researchers in The Netherlands found that “over the last three years one in two researchers engaged frequently in at least one QRP, while one in twelve reported having falsified or fabricated their research at least once” – with the highest prevalence estimate for fabrication and falsification in the life and medical sciences (Gopalakrishna et al., 2022 ). Similarly worrisome results with regard to different forms of questionable research practices or misconduct are reported in (Boutron & Ravaud, 2018 ; John et al., 2012 ; Kaiser et al., 2021 ). Footnote 22 Additionally, we think that it is not entirely unreasonable to assume that the widespread lack of transparency (particularly the much-reported difficulties of obtaining data even after personal requests) is at least somewhat indicative of a non-negligible prevalence of scientific misconduct and questionable research (data) practices. Footnote 23
The possibility of keeping data opaque enables misconduct or at least makes it more difficult to detect it. As data transparency makes it easier to detect (at least some forms of) fraud and questionable research practices and can function as a deterrent (Fischer & Zigmond, 2010 ; Gopalakrishna et al., 2022 ; Hedrick, 1988 ; Winter & Kosolosky, 2013 ), we argue that data sharing for transparency can help prevent and detect unethical scientific practices.
Since data transparency is a prerequisite for reproducibility and a means for preventing and detecting misconduct and questionable research practices, we conclude that there are good (normative-philosophical) arguments for taking data sharing for transparency as an important requirement of epistemic research integrity.
2. The community consensus perspective: The scientific community also sees data sharing as an important part of epistemic integrity (All European Academies ALLEA, 2017 ; Deutsche Forschungsgemeinschaft (DFG), 2019 ; Kretser et al., 2019 ; National Academies Press (US), 2017 ; Netherlands Code of Conduct for Research Integrity, 2018 ; Resnik & Shamoo, 2011 ; World Conference on Research Integrity, 2010 ). However, most of these guidelines and codes of conduct do not explicitly differentiate between epistemic and socio-moral integrity of research and many do not clearly differentiate between the purposes of data sharing (i.e., the purposes of transparency and secondary use). Therefore, we must deduce from the context what the respective statements refer to. We cannot do this in a systematic way here. But our impression is that many documents emphasise the values of transparency and honesty and explicitly or implicitly refer to these values when they state the importance of data sharing for research integrity. It thus seems there is a (international and trans-disciplinary) consensus that data sharing for purposes of transparency is a part of epistemic integrity. For example, the Netherland Code of Conduct explicitly connects data availability with the value of transparency, and the German DFG also explicitly refers to data sharing for the purpose of confirmability (“Nachvollziehbarkeit”).
Hence, both perspectives—the normative-philosophical and the community consensus perspectives—support the proposition that data sharing for transparency is an important component of epistemic research integrity.
B. Epistemic Integrity and Data Sharing for Secondary Use
Philosophical perspective : While data sharing for transparency clearly falls within the scope of epistemic research integrity, the same cannot be said about data sharing for secondary use. Since we follow Winter and Kosolosky ( 2013 ) and “define the epistemic integrity of a practice as a function of the degree to which the statements resulting from this practice are deceptive”, we believe that data sharing for secondary use is not part of epistemic research integrity. Although one might argue that secondary use of data has the potential to correct for misleading or deceptive statements from original studies, we think that the main importance of sharing data for secondary use is that it promotes scientific progress and social value. Data sharing for secondary use is of rather secondary importance when it comes to correcting misleading scientific statements or results. It does not seem to be a strict requirement of epistemic integrity but more of a supererogatory practice. Therefore, from a philosophical perspective, the promotion of data sharing for secondary use is not required by the obligation to promote epistemic research integrity
Community consensus perspective : Only a few guidelines and codes of conduct explicitly state that data sharing for secondary use is a requirement of research integrity (for instance, DFG, 2019 ). Many do not mention data sharing for secondary use explicitly, and some do not even seem to consider it implicitly. Thus, there does not appear to be a clear and unambiguous international consensus on the relationship between data sharing for secondary use and epistemic integrity. And since most of these documents do not differentiate explicitly between epistemic and socio-moral integrity, it is not clear whether data sharing for secondary use is considered as important from an epistemic perspective or from a non-epistemic, socio-moral perspective. Footnote 24
Therefore, from a community consensus perspective there is no clear consensus that data sharing for secondary use is a requirement of (epistemic) research integrity. From this perspective then, the obligation of funders to promote epistemic research integrity does not require the promotion of data sharing for secondary use. However, if there are specific disciplinary or national communities that explicitly take data sharing for secondary use as part of research integrity, those funders for whom this consensus is pertinent might have a reason to promote this kind of data sharing with reference to the obligation to promote research integrity. This holds true even though from a philosophical perspective data sharing for secondary use is not a part of epistemic research integrity: If the pertinent community takes data sharing for secondary use as part of (epistemic) integrity, funders might take this as a reason to promote it. Footnote 25
Therefore, and to conclude this whole Sect. " The Obligation to Promote the Epistemic Integrity of Research " about research integrity: Since funders have the obligation to promote epistemic research integrity, and since data sharing for transparency is an important part of epistemic research integrity, funders pro tanto ought to promote data sharing for transparency. From a philosophical perspective, epistemic research integrity does not require data sharing for secondary use, and from a community consensus perspective it is clearly considered as part of epistemic integrity only in a few cases of specific scientific communities. Therefore, a universal obligation for funders to promote data sharing for secondary use cannot be derived from considerations of epistemic research integrity.
Further Relevant Moral Obligations
In this section, we present two further obligations that partially speak in favour of funders promoting data sharing and partially against it. After presenting these various obligations in the following, we will close the section by weighing all pertinent obligations of funders and come to an all things considered judgement.
Funders have a pro tanto obligation to respect the rights of individuals and to not harm human or non-human beings, which includes the obligation to not induce, cause or increase risks of harm and of rights violations. This includes the obligation to respect privacy and informational autonomy of data subjects and not to induce, cause or increase informational risks or harms This obligation is part of the obligation to promote the socio-moral integrity of funded research and it speaks both in favour and against the promotion of data sharing:
As data sharing reduces the need for ever-new data collection, data sharing also reduces the amount and frequency of research procedures in interventional and non-interventional studies that carry risks for participants (Fischer & Zigmond, 2010 ). Hence, in this regard the obligation to respect the rights of persons and to not harm anybody speaks in favour of funders’ promoting data sharing.
The sharing of research data and its ensuing secondary use increases informational risks for data subjects. Prima facie , this speaks against the promotion of data sharing. However, if subjects are informed about these risks and give consent to the usage of their data despite these risks, this increase of informational risks does not represent an infringement of the obligation not to harm. Volenti non fit inuria . Thus, the risks do not speak against funders promoting data sharing if consent is obtained in funded research. Of course, this argument raises the question of a model that offers research subjects appropriate information and opportunities to consent or reject consent and, at the same time, allows for data sharing without causing unreasonable practical burdens or hurdles (Manson, 2019 ; Mikkelsen et al., 2019 ; Ploug & Holm, 2016 ). We deem that broad consent, if combined with a normative and technical governance framework and data protection measures, is an appropriate information and consent model. In order to meet their obligation to respect the rights of data subjects, funders should thus recommend that broad consent be embedded in appropriate normative and technical governance frameworks. Irrespective of the question of informed consent, informational risks exist due to data misuse and data breaches. Erlich & Narayanan, 2014 ; Hayden, 2013 ; Homer et al., 2008 ; Levy et al., 2007 have shown how different techniques could be used for breaching (particularly genetic) privacy. These risks pro tanto speak against the promotion of the sharing of personal data.
The pooling of data from different sources and the use of big data methods enables predictions about sensitive information regarding persons or groups other than the original data subjects (Mühlhoff, 2021 ). Some authors warn that this increases risks of stigmatisation and discrimination of marginalised groups (Favaretto et al., 2019 ; Reed-Berendt et al., 2022 ; Xafis et al., 2019 ). Promoting and accelerating data sharing and secondary use expand the opportunities for pooling and big data and thus might increase these risks. Thus, in this regard the obligation to minimise risks of harm speaks against the promotion of data sharing.
Funders also have a pro tanto obligation to increase public trust in science and research funding. This obligation partly speaks in favour and partially speaks against the promotion of data sharing. On the one hand, as data sharing promotes transparency and accountability, it can increase and consolidate public trust and confidence in science and research funding. Hence, in this respect funders ought to promote data sharing in order to promote public trust. On the other hand, since promoting data sharing increases risks for privacy and creates challenges for informational self-determination, concerns about these risks and challenges might reduce trust in the research system (Platt et al., 2018 ; Ploug, 2020 ). Hence, in this respect funders ought not to promote data sharing in order to promote or maintain public trust.
However, the extent to which the two obligations (not to harm and respect rights and to foster public trust) speak against the promotion of data sharing can be significantly reduced. In fact, funding agencies can and should do various things to minimise or prevent the pertaining risks:
They should fund technological as well as ethical, legal, and social research (ELSA-research) on practical solutions for data security and privacy protection with a particular view on problems and risks resulting from big data and machine learning.
Funders should promote research on data augmentation and synthetic data as potential approaches to handle limitations to data sharing due to risks for data subjects.
They should finance and promote data infrastructures and archives or repositories that can guarantee data privacy and security and require funded researchers use these trusted repositories.
Funders should fund the development and implementation of data access committees that take into account the aforementioned risks resulting from secondary use.
Funders should support data stewardship infrastructures that convey “a fiduciary (or trust) relation” that also takes into consideration the rights of patients and participants (Rosenbaum, 2010 ).
Funders should develop principles and provide best practices that support and enable researchers to provide appropriate forms of consent with regard to data sharing. They should create a framework for protecting the privacy of research participants that provides guidance on how participant information and (broad) consent forms are to be designed.
Funders should provide standards and best practice for contracts between data producers, repositories, and data re-users with special attention to data protection and security. Footnote 26
In all of the aforementioned measures, the participation and inclusion of patient representatives should be promoted and enabled. Footnote 27
Funders should require researchers to reflect upon and identify potential risks (early in the process) by creating a data management plan, elaborating how they address and intent to deal with or avoid these risks.
If the pertaining risks are addressed and thus comparatively small, the trust obligation and the not to harm obligation rather speak in favour of the promotion of data sharing or at least have no significant weight against data sharing. Even if they keep having some limited weight against data sharing, they are outweighed by the obligations in favour of promoting data sharing, i.e., the obligations of social value and scientific progress. Footnote 28 Of course, the more funders encourage and press researchers to share persona-related (non-anonymous) data, the more they are responsible for the impact of their policies on data subjects and the more they have to support researchers in protecting data subjects’ informational rights and privacy and this increases the financial and administrative costs and burdens for funders. However, we do not think that this outweighs the benefits in terms of social value and scientific progress.
The main conclusion of Sects. " The Moral Obligations of Funders and the Promotion of Data Sharing " and " Further Relevant Moral Obligations " is thus: Although there are two pro tanto obligations that speak against the promotion of data sharing by public funders, the pro tanto obligations in favour of the promotion weigh heavier (provided that the mentioned risk reducing measures are implemented). Public funders thus have an all things considered obligation to promote the sharing of data of funded researchers. Footnote 29
Mandatory Data Sharing Policies and Academic Freedom
Up to this point, we have not directly commented which means funders ought to use to promote data sharing. As we said, it is an empirical question what follows from the pro tanto obligations of funders to promote data sharing in terms of specific means to promote it.
However, in the following we want to examine a question with regard to a specific means of promoting data sharing: Mandatory Data Sharing Policies . Funders are increasingly advised to adopt policies that require data sharing (Sim et al., 2020 ). The NIH as a major funder has been setting standards in implementing such policies for years now and has recently implemented a new mandatory data sharing policy (Kozlov, 2022 ; National Institutes of Health, 2022 ). We find it plausible that such policies are comparatively effective and efficient. Mandatory data sharing policies can be designed at least with two different objectives: 1. They can require only the sharing of data that is the evidential basis for an already published paper and only for purposes of transparency and confirmability. 2. Or they can additionally require the sharing of data (either publication-related or all data that are generated during a research project) for purposes of secondary use.
As mandatory data sharing policies of public funders restrict the individual freedom of funded researchers (at least if these are dependent on third-party funding), the question arises whether such policies conflict with academic freedom . Do data sharing requirements implemented by public funders infringe on the academic freedom of individual researchers? Footnote 30
To answer this question, we have to clarify what academic freedom is and what it protects. From a philosophical perspective, Footnote 31 academic freedom is first and foremost the negative right of individual researchers against external intervention in their scientific work and decision-making. Academic freedom mainly concerns the freedom to choose research questions, theories, and methodologies as well as publication venues independently from outside intervention, in particular state intervention . This negative right of freedom from intervention of researchers thus corresponds to the negative duty of the state not to intervene.
As public funders are (semi-)governmental institutions whose funding comes predominantly from government budgets and on whose boards government representatives participate in decision-making, the following holds: Public funders have the negative obligation to respect the negative right to academic freedom of researchers.
The question now is whether mandatory data sharing policies violate the negative right of researchers to academic freedom? To answer this question, we must determine in more detail the scope of protection of academic freedom. From our perspective, the scope of protection of academic freedom includes only actions of researchers that are not violations of crucial and basic norms of epistemic research integrity. Such crucial and basic norms determine fundamental requirements of science and research as a specific kind of rational practice and communication. For instance, researchers that engage in data fabrication or falsification fail to meet such fundamental requirements. They thus violate crucial and basic norms of research integrity and engage in behaviour that is not protected by academic freedom.
Hence, we must answer the following questions:
Is the omission (or refusal) to share data that are the evidential basis of published research results for purposes of transparency and reproducibility a violation of fundamental requirements of scientific work and communication?
Is the omission (or refusal) to share data (either publication-related or all data that are generated during a research project) for purposes of secondary use a failure to meet such fundamental requirements?
Ad 1 : We believe that not sharing data that underlies research results (a published paper) for purposes of transparency is a violation of the fundamental requirements of scientific work and communication. This is clearly the case from the philosophical perspective on research integrity. Although not sharing data that underlies a published paper for transparency seems to be a less severe scientific misconduct as data fabrication or falsification, it clearly runs counter to one of the basic requirements of scientific communication and (collective) truth-seeking: To make one’s own scientific work transparent and reproducible. There is no reasonable justification for why researchers should be generally free to avoid that their published (!) work can be reviewed in all its parts. Footnote 32 We believe the philosophical perspective is backed by the perspective of the consensus of the scientific community. The community recognises data sharing for transparency as a key requirement of epistemic research integrity. Almost all codes of conduct and guidelines on research integrity emphasise the close relation between honesty, reproducibility, and data transparency (see Sect. " The Obligation to Promote the Epistemic Integrity of Research ". A). Therefore, research without sharing data for transparency is not protected by academic freedom. Thus, mandatory policies that require data sharing for transparency do not infringe on the right to academic freedom of individual researchers.
Ad 2 : In Sect. " The Obligation to Promote the Epistemic Integrity of Research ", we have already noted that neither in the community consensus nor in the normative-philosophical perspective data sharing for secondary use is a requirement of epistemic integrity. This means that the freedom to share or not to share data for secondary use is within the scope of protection of academic freedom. However, data sharing requirements for secondary use of public funders are not necessarily an infringement of academic freedom. First, it depends on how much researchers must rely on third-party funding in their research. If they have access to basic financial resources of their institutions and are not dependent on applying for additional public funding, then such requirements are not a restriction of their academic freedom. Second, data sharing requirements of public funders that enjoy relative autonomy from government and whose decisions are essentially made by scientists themselves do not represent state coercion but rather self-determination of the scientific community. However, academic freedom does not only protect individuals against state intervention but also against infringements through (parts of) the scientific community. Thus, data sharing requirements of public funders with state autonomy (and also those without state autonomy) do represent an infringement of academic freedom (at least for researchers that depend on their funding), though not in the classical sense of state infringements.
It must be noted, however, that this infringement of academic freedom is a fairly small one. The freedom to share or not to share data for secondary use does not belong to the core of academic freedom. The core arguably is the freedom to “follow a line of research where it leads” (Russell, 1993 ), i.e., the freedom to choose research questions, theories, and methodologies as well as publication venues independently from outside intervention. Nonetheless, it is an infringement, but we believe it can be mitigated by the following measures: Funders can a) offer the possibility for a justified exception from data sharing requirements (for instance, for reasons of data protection or dual use risks), b) allow for an embargo period in which the funded and data producing researcher has the exclusive privilege to use their data, c) consider discipline-specific standards for data management and sharing, and d) compensate for burden and costs financially (for instance, for fees of repositories for long-term storage or for data protection measures) and through investments in and supply of technical and administrative support (for instance, digital privacy and security safeguarding solutions and best practices). If funders implement measures like these, the infringement of academic freedom through mandatory data sharing policies becomes so small that it can be justified with reference to the other pro tanto obligations of funders, namely the obligations with respect to social value, scientific progress, and the minimisation of harm. Footnote 33
However, the justifiability of the infringement on academic freedom through mandatory data sharing policies is dependent on a further condition: Mandatory policies can only be justified, if there are no measures of promoting data sharing that are more effective and less invasive in terms of academic freedom.
A last word on the implications of the diagnosis that policies that require data sharing for secondary use infringe on the academic freedom of researchers: If public funders infringe on the academic freedom of researchers with reference to the benefits of data sharing, they have the responsibility to ensure that these benefits are realised. This requires two things of them: 1. Since the benefits of data sharing only materialise if reproduction and replication as well as secondary use are actually carried out, funders should fund appropriate projects. They should finance and reward reproduction and replication studies and set up a funding programme for secondary research. 2. Funders should fund research and monitoring on whether their own initiatives to promote data sharing are i) effective in terms of actual data sharing and ii) actually lead to the hoped-for benefits.
Summary and Conclusion
In this paper, we investigated the question whether public funders have a moral obligation to promote the sharing of research data generated in funded research projects. More specifically, we asked which of funders general moral obligations speak in favour of and which of these obligations speak against the promotion of data sharing. We draw the following conclusions: First, public funders have several general pro tanto obligations that (under certain conditions) require funders to promote data sharing. The main obligations are the social value-, scientific progress- and epistemic research integrity-obligation. Second, in the assessment of pro tanto obligations against promoting data sharing, we argued that – provided that funders take measures to minimise the risks for research subjects and third parties – the obligations in favour of promoting data sharing outweigh the obligations against. Therefore, we concluded with respect to our overall research question that public funders ought to promote data sharing all things considered .
With respect to our third specific research question whether mandatory data sharing policies are an ethically justifiable means of promoting data sharing, we argued: First, the scope of protection of academic freedom does not cover the omission or refusal to share data for purposes of transparency. Requirements to share data for the purpose of transparency therefore do not violate academic freedom. Second, the scope of protection does cover the omission or refusal to share data for secondary use, therefore requirements to share data for secondary use violate academic freedom to a small extent (at least for researchers that are dependent on public funding). However, such requirements and thus the violation of academic freedom can be justified with reference to the other pro tanto obligations that public funders have.
Sometimes research data can only be re-used when research methodologies that have been used to collect, generate and analyse the data (questionnaires, analytical codes, etc.) are shared as well (Goldacre et al., 2019 ). Thus, sharing these methodologies and other intermediary resources might equally be important as sharing the data themselves. However, due to some disanalogies between data and those resources (most saliently the fact that some of the latter can be seen as intellectual property), we confine our discussion here to research data.
The Creative Commons set of licenses are the most commonly used for sharing research data. These licenses are designed to be open, which means that data can be freely reused without requiring explicit permission as long as the terms of the license are adhered to. Such licences can be a good and efficient way of reducing costs and burdens for data sharing, while they may have limited applicability in cases of person-related data or for researchers who wish to retain control over the subsequent use of the data they produce.
If regulatory considerations limit the sharing of data generally or on an international level, the generation of synthetic data can be an alternative. However, (sharing of) synthetic data can only complement but not fully replace (the sharing of) non-synthetic data.
Pro tanto obligations are what David Ross ( 1930 ) called prima facie obligations. In line with the established terminology “ pro tanto and all things considered moral reasons” (Alvarez, 2016 ), we chose to deviate from Ross’ terminology, see also Hurtig ( 2007 ) and Dabbagh ( 2018 ).
Similar claims are made with regard to journal policies in Federer et al. ( 2018 ) and Gabelica et al. ( 2019 ).
For the debate about the social value requirement, see Barsdorf and Millum ( 2017 ), Pierson and Millum ( 2018 ), Resnik ( 2018a , 2018b ), Wendler and Rid ( 2017 ), Wertheimer ( 2015 ).
See also Bierer et al. ( 2018 ).
For further discussions on this topic see Winkler et al. ( 2023 ).
Notice that the references only state that research must have sufficient benefits for society in order to be justified if it exposes participants to risks. However, we find this implausible and believe that it has to maximise benefits. For it seems questionable to choose project A over the alternatively fundable projects B and C, if it can be expected that either project B or C have more social benefit than A.
Notice that this obligation does not require a short-sighted restriction to immediate benefits and “mere” application-oriented research but will plausibly take into account basic research that enables long-term fruitful and sustainable research by exploring fundamental causal mechanisms. Otherwise, maximisation would hardly be possible.
These conditions also secure that data sharing of funded projects does not facilitate the exploitation or extraction of resources from the underprivileged to the privileged or to private corporations and does not promote epistemically biased research. See Leonelli ( 2023 ) for examples of such detrimental effects of data sharing.
This issue of the cost–benefit balance of promoting data sharing is also pertinent for all other obligations we will discuss below. We will not mention it again though and assume for the rest of the paper that the benefits of promoting data sharing are greater than the costs.
Bierer et al. ( 2018 ) also argue that funders ought to promote data sharing in order to advance the social value of research. Notice that this obligation might be stronger or weaker for particular research fields or specific data. For instance, the social value of sharing particular health data in a pandemic or biomedical data in general is presumably bigger than the social value of the sharing of archaeological data about a particular Egyptian pharaoh.
We believe that on any other plausible account of social value, i.e., on any plausible distributive principle funders ought to promote data sharing and fund research that has social value. For instance, a utilitarian account of social value will give us the same conclusion.
On the notion of scientific progress and “significant” knowledge, see Bird ( 2007 ), Kitcher ( 2001 ), Niiniluoto ( 2019 ).
For the view that scientific knowledge has intrinsic value, see for instance Schwartz ( 2020 ).
What holds for the social value obligation also holds for the obligation to promote scientific progress: Depending on the particular research field and the particular data the obligation to promote data sharing in order to promote scientific progress is stronger or weaker. The sharing of particular (kinds of) data might bear more potential to promote scientific progress while the sharing of other (kinds of) data might bear less potential.
For different terminologies for both kinds of obligations, for instance internal vs. external norms, see Resnik ( 1996 ) and Reydon ( 2013 ). For an attempt to differentiate the justificatory grounds for the various kinds of obligations of scientists see Resnik ( 1998 ).
For a legal analysis of the relation between (semi-)governmental promotion of data sharing and good scientific practice in the context of German constitutional law see Fehling and Tormin ( 2021 ).
Strictly speaking, evidence is that which confirms or disconfirms a scientific claim, i.e., data. A methodology or an analysis is not evidence in this sense. However, we stick to the understanding of Munafò above because the sharing of evidence in his sense is required by the norm of evidence-sharing. At least we think that Haack has this in mind.
Robert Merton ( 1942 /1973) famously introduced this term in his description of “the normative structure” and the “ethos of science”, see also Ziman ( 2009 ).
Although Fanelli ( 2018 ) doubts that misconduct has a major impact on the scientific literature, she agrees that it is non-negligible.
For instance, Tsuyoshi Miyakawa ( 2020 ) reports the results of analyses on the manuscripts that he has handled as Editor-in-Chief of Molecular Brain as showing that “more than 97% of the 41 manuscripts did not present the raw data supporting their results when requested by an editor, suggesting a possibility that the raw data did not exist from the beginning, at least in some portions of these cases”.
The DFG Guideline ( 2019 ) is arguably a guideline exclusively for epistemic research integrity, and it is thus reasonable to assume that the explicit inclusion of data sharing for secondary use means that it is considered to be an epistemically required practice. However, the ALLEA code (ALLEA 2017 ), as some other codes, is not exclusively focused on epistemic integrity as it includes socio-moral obligations (for instance, to respect the needs and right of study participants). Its statement that data should be as open as possible, as closed as necessary can be understood as including data sharing for secondary use, but it remains open whether this is taken to be a requirement of epistemic integrity. It could be case that the justification for data sharing for secondary use is mainly seen in its benefit for society and scientific progress. If this is the reason why data sharing for secondary use is included in research integrity, then the research integrity obligation adds nothing to the social value and scientific progress obligation with respect to data sharing for secondary use – which we already discussed in Sect. " The Obligation to Benefit Society " and " The Obligation to Promote Scientific Progress ".
Only in cases in which there are strong philosophical or ethical reasons that speak against the community consensus, funders might not be allowed to follow this consensus. However, we believe this is not the case for the issue of data sharing for secondary use.
There have been intense and broad research and debates on ethical, legal, and social issues of privacy and data protection and other informational aspects of research subject protection in biomedical data intense research and data sharing for 10–15 years. Following the increasing activities of genomic data sharing, approaches and best practices have been developed to address challenges concerning data protection, privacy, and informational rights and autonomy. See for instance the GA4GH and its “Regulatory and Ethics Work Stream” ( https://www.ga4gh.org/how-we-work/workstreams/ ) that provides standard solutions for genetic data sharing and a framework for responsible sharing of genomic and health-related data ( https://www.ga4gh.org/genomic-data-toolkit/regulatory-ethics-toolkit/framework-for-responsible-sharing-of-genomic-and-health-related-data/ ) or the European Genome Archive (EGA) which also provides best practices for genetic data sharing.
We develop a systematic approach to funders' responsibilities for the protection and participation of data subjects from a legal and ethical perspective in Fehling et al. ( 2023 ).
Since the pertaining risks are mainly associated with data sharing for secondary use, and since data sharing for secondary use is not a requirement of research integrity, the weighing of obligations here must exclude the obligation to promote research integrity and focus only on scientific progress and social value.
Of course, we cannot exclude the possibility of very specific cases in certain areas of research where there are additional reasons against the promotion of data sharing which override the pro tanto obligation that speak in favour of promoting data sharing. For example, sharing huge amounts of high quality data used to develop machine learning programs in biomedicine with a Russian research institute closely linked to the Russian military complex might bear the risk of harmful consequences for society. Our all things considered claim should thus be understood as not applying to such special cases. For the possibility of such cases see footnote 11 and the reference to Leonelli ( 2023 ).
How differently the relation between academic freedom and data sharing requirements is perceived by German funders as compared to non-German funders is examined in more detail in Anger et al. ( 2024 ).
The following is a philosophical and not a legal analysis. For a legal analysis of the possibilities and limits of (semi-)governmental promotion of data sharing in the German context see Overkamp and Tormin ( 2022 ) and for the German and European context with a side glance at US constitutional law see Fehling and Tormin ( 2021 ).
Of course, there can be specific reasons in a particular case not to make data transparent for confirmation efforts (such as, for instance, privacy concerns). However, our point is that besides such special circumstances, there is no reason for why researchers ought to generally be free to refuse to make their data available for confirmation.
Of course, this depends on how strong these pro tanto obligations are with respect to particular (kinds of) data. As we explained in footnotes 13 and 17 the weight of these obligations depends on how much the sharing of particular data from a particular research field contributes to social value and scientific progress. We believe, however, that for the most part of the sciences the sharing of research data is so valuable in theses respects that an infringement of academic freedom can be justified.
All European Academies (ALLEA) (2017). The European Code of Conduct for Research Integrity. Retrieved 25 February 2022 https://allea.org/code-of-conduct/ .
Alvarez, M. (2016). Reasons for action: Justification, motivation, explanation. In E. N. Zalta (Ed.). The Stanford encyclopedia of philosophy (Winter 2017 edition). Retrieved June 14, 2022, from https://plato.stanford.edu/archives/win2017/entries/reasons-just-vs-expl/ .
Anger, M., Wendelborn, C., & Schickhardt, C. (2024). German funders’ data sharing policies—A qualitative interview study. PLoS ONE, 19 (2), e0296956. https://doi.org/10.1371/journal.pone.0296956
Article Google Scholar
Anger, M., Wendelborn, C., Winkler, E. C., & Schickhardt, C. (2022). Neither carrots nor sticks? Challenges surrounding data sharing from the perspective of research funding agencies—A qualitative expert interview study. PLoS ONE, 17 (9), e0273259. https://doi.org/10.1371/journal.pone.0273259
Barsdorf, N., & Millum, J. (2017). The social value of health research and the worst off. Bioethics, 31 (2), 105–115. https://doi.org/10.1111/bioe.12320
Bauchner, H., Golub, R. M., & Fontanarosa, P. B. (2016). Data sharing: An ethical and scientific imperative. JAMA, 315 (12), 1237–1239. https://doi.org/10.1001/jama.2016.2420
Begley, C. G., & Ioannidis, J. P. A. (2015). Reproducibility in science: Improving the standard for basic and preclinical research. Circulation Research, 116 (1), 116–126. https://doi.org/10.1161/CIRCRESAHA.114.303819
Bierer, B. E., Strauss, D. H., White, S. A., & Zarin, D. A. (2018). Universal funder responsibilities that advance social value. The American Journal of Bioethics AJOB, 18 (11), 30–32. https://doi.org/10.1080/15265161.2018.1523498
Bird, A. (2007). What is scientific progress? Noûs, 41 (1), 64–89. https://doi.org/10.1111/j.1468-0068.2007.00638.x
Bouter, L. (2016). What funding agencies and journals can do to prevent sloppy science. Retrieved June 14, 2022, from https://www.euroscientist.com/what-funding-agencies-and-journals-can-do-to-prevent-sloppy-science/ .
Bouter, L. (2020). What research institutions can do to foster research integrity. Science and Engineering Ethics, 26 (4), 2363–2369. https://doi.org/10.1007/s11948-020-00178-5
Bouter, L. M. (2018). Fostering responsible research practices is a shared responsibility of multiple stakeholders. Journal of Clinical Epidemiology, 96 , 143–146. https://doi.org/10.1016/j.jclinepi.2017.12.016
Boutron, I., & Ravaud, P. (2018). Misrepresentation and distortion of research in biomedical literature. Proceedings of the National Academy of Sciences of the United States of America, 115 (11), 2613–2619. https://doi.org/10.1073/pnas.1710755115
Brock, D. W. (2012). Priority to the worse off in health care resource prioritization. In R. Rhodes, M. Battin, & A. Silvers (Eds.), Medicine and social justice: Essays on the distribution of health care (pp. 155–164). Oxford University Press.
Chapter Google Scholar
Brown, M. B., & Guston, D. H. (2009). Science, democracy, and the right to research. Science and Engineering Ethics, 15 (3), 351–366. https://doi.org/10.1007/s11948-009-9135-4
Burton, P. R., Banner, N., Elliot, M. J., Knoppers, B. M., & Banks, J. (2017). Policies and strategies to facilitate secondary use of research data in the health sciences. International Journal of Epidemiology, 46 (6), 1729–1733. https://doi.org/10.1093/ije/dyx195
Chan, A.-W., Song, F., Vickers, A., Jefferson, T., Dickersin, K., Gøtzsche, P. C., Krumholz, H. M., Ghersi, D., & van der Worp, H. B. (2014). Increasing value and reducing waste: Addressing inaccessible research. The Lancet, 383 (9913), 257–266. https://doi.org/10.1016/S0140-6736(13)62296-5
Contreras, J., & Knoppers, B. M. (2018). The genomic commons. Annual Review of Genomics and Human Genetics, 19 , 429–453.
Couture, J. L., Blake, R. E., McDonald, G., & Ward, C. L. (2018). A funder-imposed data publication requirement seldom inspired data sharing. PLOS ONE , 13 (7). https://doi.org/10.1371/journal.pone.0199789 .
Dabbagh, H. (2018). The problem of explanation and reason-giving account of pro tanto duties in the Rossian ethical framework. Public Reason, 10 (1), 69–80.
Google Scholar
Danchev, Valentin, Min, Yan, Borghi, John, Baiocchi, Mike, & Ioannidis, John P. A. (2021). Evaluation of data sharing after implementation of the International Committee of Medical Journal Editors Data Sharing Statement Requirement. JAMA Network Open , 4 (1), e2033972. https://doi.org/10.1001/jamanetworkopen.2020.33972
Deutsche Forschungsgemeinschaft (DFG) (2019). Leitlinien zur Sicherung guter wissenschaftlicher Praxis: Kodex. Retrieved 25 February 2022 https://doi.org/10.5281/zenodo.3923602 .
Digital Science Report (2019). State of Open Data 2019. A selection of analyses and articles about open data, curated by Figshare. figshare. https://doi.org/10.6084/M9.FIGSHARE.10011788.V2 .
Eckert, Ester M., Di Cesare, Andrea, Fontaneto, Diego, Berendonk, Thomas U., Bürgmann, Helmut, Cytryn, Eddie et al. (2020). Every fifth published metagenome is not available to science. PLOS Biology 18 (4), e3000698. https://doi.org/10.1371/journal.pbio.3000698 .
Erlich, Y., & Narayanan, A. (2014). Routes for breaching and protecting genetic privacy. Nature Reviews Genetics, 15 , 409–421. https://doi.org/10.1038/nrg3723
Errington, T. M., Denis, A., Perfito, N., Iorns, E., & Nosek, B. A. (2021). Challenges for assessing replicability in preclinical cancer biology. eLife , 10 . https://doi.org/10.7554/eLife.67995 .
European Commission. Joint Research Centre. (2017). Analysis of national public research funding (PREF). In Handbook for data collection and indicators production . Publications Office. https://doi.org/10.2760/849945
Fanelli, D. (2018). Opinion: Is science really facing a reproducibility crisis, and do we need it to? Proceedings of the National Academy of Sciences of the United States of America, 115 (11), 2628–2631. https://doi.org/10.1073/pnas.1708272114
Favaretto, M., Clercq, E. de, & Elger, B. S. (2019). Big Data and discrimination: Perils, promises and solutions. A systematic review. Journal of Big Data , 6 (1). https://doi.org/10.1186/s40537-019-0177-4 .
Federer, L. M., Belter, C. W., Joubert, D. J., Livinski, A., Lu, Y.-L., Snyders, L. N., & Thompson, H. (2018). Data sharing in PLOS ONE: An analysis of data availability statements. PLOS ONE , 13 (5). https://doi.org/10.1371/journal.pone.0194768 .
Fehling, M., & Tormin, M. (2021). Das Teilen von Forschungsdaten zwischen Wissenschaftsfreiheit und guter wissenschaftlicher Praxis. Wissenschaftsrecht, 54 (3–4), 281. https://doi.org/10.1628/wissr-2021-0022
Fehling, M., Tormin, M., Wendelborn, C., & Schickhardt, C. (2023). Forschungsförderorganisationen in der Verantwortung zwischen Data Sharing und dem Schutz von Datensubjekten. Medizinrecht, 41 (11), 869–878. https://doi.org/10.1007/s00350-023-6599-1
First International Strategy Meeting on Human Genome Sequencing (1996): Bermuda principles. http://web.ornl.gov/sci/techresources/Human_Genome/research/bermuda.shtml#1 . Accessed 29 July 2023
Fischer, B. A., & Zigmond, M. J. (2010). The essential nature of sharing in science. Science and Engineering Ethics, 16 (4), 783–799. https://doi.org/10.1007/s11948-010-9239-x
Fort Lauderdale Agreement (2003). Sharing data from large-scale biological research projects: A system of tripartite responsibility. http://www.genome.gov/Pages/Research/WellcomeReport0303.pdf . Accessed 29 July 2023
Gabelica, M., Cavar, J., & Puljak, L. (2019). Authors of trials from high-ranking anesthesiology journals were not willing to share raw data. Journal of Clinical Epidemiology, 109 , 111–116. https://doi.org/10.1016/j.jclinepi.2019.01.012
Gabelica, M., Bojčić, R., & Puljak, L. (2022). Many researchers were not compliant with their published data sharing statement: A mixed-methods study. Journal of Clinical Epidemiology, 150 , 33–41. https://doi.org/10.1016/j.jclinepi.2022.05.019
Glasziou, P., Altman, D. G., Bossuyt, P., Boutron, I., Clarke, M., Julious, S., Michie, S., Moher, D., & Wager, E. (2014). Reducing waste from incomplete or unusable reports of biomedical research. The Lancet, 383 (9913), 267–276. https://doi.org/10.1016/S0140-6736(13)62228-X
Goldacre, B., Morton, C. E., & DeVito, N. J. (2019). Why researchers should share their analytic code. BMJ (Clinical Research ed.), 367 , l6365. https://doi.org/10.1136/bmj.l6365
Gopalakrishna, G., Riet, G. ter, Vink, G., Stoop, I., Wicherts, J. M., & Bouter, L. M. (2022). Prevalence of questionable research practices, research misconduct and their potential explanatory factors: A survey among academic researchers in the Netherlands. PLOS ONE , 17 (2). https://doi.org/10.1371/journal.pone.0263023 .
Gorman, D. M. (2020). Availability of research data in high-impact addiction journals with data sharing policies. Science and Engineering Ethics , 26 (3), S. 1625–1632. https://doi.org/10.1007/s11948-020-00203-7 .
Haack, S. (2007). The integrity of science: What it means, why it matters. Contrastes: Revista International de Filosofia 12 , S. 5–26. Online verfügbar unter https://papers.ssrn.com/sol3/papers.cfm?abstract_id=1105831 , zuletzt geprüft am 25. Februar 2022.
Hardwicke, T. E., Bohn, M., MacDonald, K., Hembacher, E., Nuijten, M. B., Peloquin, B. N., deMayo, B. E., Long, B., Yoon, E. J., & Frank, M. C. (2021). Analytic reproducibility in articles receiving open data badges at the Journal Psychological Science: An observational study. Royal Society Open Science , 8 (1). https://doi.org/10.1098/rsos.201494 .
Hardwicke, T. E., Mathur, M. B., MacDonald, K., Nilsonne, G., Banks, G. C., Kidwell, M. C., Hofelich Mohr, A., Clayton, E., Yoon, E. J., Henry Tessler, M., Lenne, R. L., Altman, S., Long, B., & Frank, M. C. (2018). Data availability, reusability, and analytic reproducibility: Evaluating the impact of a mandatory open data policy at the journal Cognition. Royal Society Open Science , 5 (8). https://doi.org/10.1098/rsos.180448 .
Hayden, E. C. (2013). Privacy protections: The genome hacker. Nature 497 (7448), S. 172–174. https://doi.org/10.1038/497172a .
Hedrick, T. E. (1988). Justifications for the sharing of social science data. Law and Human Behavior, 12 (2), 163–171. https://doi.org/10.1007/BF01073124
Herlitz, A. (2018). Health, priority to the worse off, and time. Medicine, Health Care, and Philosophy, 21 (4), 517–527. https://doi.org/10.1007/s11019-018-9825-2
Homer, N., Szelinger, S., Redman, M., Duggan, D., Tembe, W., & Muehling, J. et al. (2008). Resolving individuals contributing trace amounts of DNA to highly complex mixtures using high-density SNP genotyping microarrays. PLoS Genetics , 4 (8), e1000167. https://doi.org/10.1371/journal.pgen.1000167 .
Hurtig, K. (2007). On prima facie obligations and nonmonotonicity. Journal of Philosophical Logic, 36 (5), 599–604.
Iqbal, S. A., Wallach, J. D., Khoury, M. J., Schully, S. D., & Ioannidis, J. P. A. (2016). Reproducible research practices and transparency across the biomedical literature. PLOS Biology , 14 (1). https://doi.org/10.1371/journal.pbio.1002333 .
John, L. K., Loewenstein, G., & Prelec, D. (2012). Measuring the prevalence of questionable research practices with incentives for truth telling. Psychological Science, 23 (5), 524–532. https://doi.org/10.1177/0956797611430953
Kaiser, M., Drivdal, L., Hjellbrekke, J., Ingierd, H., & Rekdal, O. B. (2021). Questionable research practices and misconduct among Norwegian researchers. Science and Engineering Ethics , 28 (1). https://doi.org/10.1007/s11948-021-00351-4 .
Kaye, J., Heeney, C., Hawkins, N., de Vries, J., & Boddington, P. (2009). Data sharing in genomics—Re-shaping scientific practice. Nature Reviews Genetics, 10 (5), 331–335. https://doi.org/10.1038/nrg2573
Kitcher, P. (2001). Science, truth, and democracy . Oxford University Press. https://doi.org/10.1093/0195145836.001.0001
Kozlov, M. (2022). NIH issues a seismic mandate: Share data publicly. Nature . https://doi.org/10.1038/d41586-022-00402-1
Kretser, A., Murphy, D., Bertuzzi, S., Abraham, T., Allison, D. B., Boor, K. J., Dwyer, J., Grantham, A., Harris, L. J., Hollander, R., Jacobs-Young, C., Rovito, S., Vafiadis, D., Woteki, C., Wyndham, J., & Yada, R. (2019). Scientific Integrity principles and best practices: Recommendations from a scientific integrity consortium. Science and Engineering Ethics, 25 (2), 327–355. https://doi.org/10.1007/s11948-019-00094-3
Leonelli, S. (2018). Rethinking reproducibility as a criterion for research quality. In L. Fiorito (Ed.), Including a symposium on the work of Mary Morgan: Curiosity, imagination, and surprise (pp. 129–146). Emerald Publishing Limited.
Leonelli, S. (2023). Philosophy of open science . Cambridge University Press. https://doi.org/10.1017/9781009416368
Levy, S., Sutton, G., Ng, P. C., Feuk, L., Halpern, A. L., Walenz, B. P. et al. (2007): The diploid genome sequence of an individual human. PLoS Biology 5 (10), e254. https://doi.org/10.1371/journal.pbio.0050254 .
Manson, N. C. (2019). The biobank consent debate: Why ‘meta-consent’ is not the solution? Journal of Medical Ethics, 45 (5), 291–294. https://doi.org/10.1136/medethics-2018-105007
Mejlgaard, N., Bouter, L. M., Gaskell, G., Kavouras, P., Allum, N., Bendtsen, A.-K., Charitidis, C. A., Claesen, N., Dierickx, K., Domaradzka, A., Reyes Elizondo, A., Foeger, N., Hiney, M., Kaltenbrunner, W., Labib, K., Marušić, A., Sørensen, M. P., Ravn, T., Ščepanović, R. … Veltri, G. A. (2020). Research integrity: Nine ways to move from talk to walk. Nature , 586 (7829), 358–360. https://doi.org/10.1038/d41586-020-02847-8 .
Merton, R. (Ed.) (1942/1973). The sociology of science: Theoretical and empirical investigations . The University of Chicago Press.
Mikkelsen, R. B., Gjerris, M., Waldemar, G., & Sandøe, P. (2019). Broad consent for biobanks is best—provided it is also deep. BMC Medical Ethics, 20 (1), 71. https://doi.org/10.1186/s12910-019-0414-6
Mill, J. S. (2008). On liberty and other essays . Oxford University Press.
Miyakawa, T. (2020). No raw data, no science: Another possible source of the reproducibility crisis. Molecular Brain , 13 (1). https://doi.org/10.1186/s13041-020-0552-2 .
Mühlhoff, R. (2021). Predictive privacy: Towards an applied ethics of data analytics. Ethics and Information Technology, 23 (4), 675–690. https://doi.org/10.1007/s10676-021-09606-x
Munafò, M. R., Nosek, B. A., Bishop, D. V. M., Button, K. S., Chambers, C. D., Du Sert, N. P., Simonsohn, U., Wagenmakers, E.-J., Ware, J. J., & Ioannidis, J. P. A. (2017). A manifesto for reproducible science. Nature Human Behaviour , 1 . https://doi.org/10.1038/s41562-016-0021 .
National Academies Press (US) (2017). Fostering integrity in research . https://doi.org/10.17226/21896 .
National Cancer Institute (n.d.). Genomic data commons, accessed 27 July 2023, https://gdc.cancer.gov/
National Commission for the Protection of Human Subjects of Biomedical and Behavioral Research (1978). The Belmont report: Ethical principles and guidelines for the protection of human subjects of research. DHEW Pub , No (OS) 78–0014. US Govt Print Office.
National Institutes of Health (2022). NIH Data Sharing Policy 2023. Retrieved 23 June 2022 https://sharing.nih.gov/data-management-and-sharing-policy/about-data-management-sharing-policy/data-management-and-sharing-policy-overview .
National Library of Medicine (n.d.). ClinVar, accessed 27 July 2023, https://www.ncbi.nlm.nih.gov/clinvar/
Naudet, F., Sakarovitch, C., Janiaud, P., Cristea, I., Fanelli, D., Moher, D., & Ioannidis, J. P. A. (2018). Data sharing and reanalysis of randomized controlled trials in leading biomedical journals with a full data sharing policy: Survey of studies published in The BMJ and PLOS Medicine. BMJ , 360 . https://doi.org/10.1136/bmj.k400 .
Naudet, F., Siebert, M., Pellen, C., Gaba, J., Axfors, C., Cristea, I., Danchev, V., Mansmann, U., Ohmann, C., Wallach, J. D., Moher, D., & Ioannidis, J. P. A. (2021). Medical journal requirements for clinical trial data sharing: Ripe for improvement. PLOS Medicine , 18 (10). https://doi.org/10.1371/journal.pmed.1003844 .
Netherlands Code of Conduct for Research Integrity (2018).
Neylon, C. (2017). Compliance culture or culture change? The role of funders in improving data management and sharing practice amongst researchers. Research Ideas and Outcomes, 3 , e21705. https://doi.org/10.3897/rio.3.e21705
Niiniluoto, I. (2019). Scientific progress. In E. N. Zalta (Ed.). The Stanford encyclopedia of philosophy (Winter 2019 edition). Retrieved June 14, 2022, from https://plato.stanford.edu/archives/win2019/entries/scientific-progress/ .
Nuijten, M. B., Bakker, M., Maassen, E., & Wicherts, J. M. (2018). Verify original results through reanalysis before replicating. Behavioral and Brain Sciences , 41 . https://doi.org/10.1017/S0140525X18000791 .
Ohmann, C., Moher, D., Siebert, M., Motschall, E., & Naudet, F. (2021). Status, use and impact of sharing individual participant data from clinical trials: A scoping review. BMJ Open , 11 (8). https://doi.org/10.1136/bmjopen-2021-049228 .
Ottersen, T. (2013). Lifetime QALY prioritarianism in priority setting. Journal of Medical Ethics, 39 (3), 175–180. https://doi.org/10.1136/medethics-2012-100740
Overkamp, P., & Tormin, M. (2022). Staatliche Steuerungsmöglichkeiten zur Förderung des Teilens von Forschungsdaten. Ordnungen der Wissenschaft, 1 , 39–54.
Peels, R. (2019). Replicability and replication in the humanities. Research Integrity and Peer Review , 4 . https://doi.org/10.1186/s41073-018-0060-4 .
Peels, R., & Bouter, L. (2021). Replication and trustworthiness. Accountability in Research . https://doi.org/10.1080/08989621.2021.1963708
Perrier, L., Blondal, E., & MacDonald, H. (2020). The views, perspectives, and experiences of academic researchers with data sharing and reuse: A meta-synthesis. PLOS ONE , 15 (2). https://doi.org/10.1371/journal.pone.0229182 .
Persad, G. (2019). Justice and public health. In A. C. Mastroianni, J. P. Kahn, & N. E. Kass (Eds.), The Oxford handbook of public health ethics (pp. 32–46). Oxford University Press.
Pierson, L., & Millum, J. (2018). Health research priority setting: The duties of individual funders. The American Journal of Bioethics, 18 (11), 6–17. https://doi.org/10.1080/15265161.2018.1523490
Platt, J. E., Jacobson, P. D., & Kardia, S. L. R. (2018). Public trust in health information sharing: A measure of system trust. Health Services Research, 53 (2), 824–845. https://doi.org/10.1111/1475-6773.12654
Ploug, T. (2020). In defence of informed consent for health record research—Why arguments from ‘easy rescue’, ‘no harm’ and ‘consent bias’ fail. BMC Medical Ethics, 21 (1), 75. https://doi.org/10.1186/s12910-020-00519-w
Ploug, T., & Holm, S. (2016). Meta consent—A flexible solution to the problem of secondary use of health data. Bioethics, 30 (9), 721–732. https://doi.org/10.1111/bioe.12286
Powell, Kendall (2021). The broken promise that undermines human genome research. Nature 590 (7845), S. 198–201. https://doi.org/10.1038/d41586-021-00331-5 .
Pratt, B., & Hyder, A. A. (2017). Fair resource allocation to health research: Priority topics for bioethics scholarship. Bioethics, 31 (6), 454–466. https://doi.org/10.1111/bioe.12350
Pratt, B., & Hyder, A. A. (2019). Ethical responsibilities of health research funders to advance global health justice. Global Public Health, 14 (1), 80–90. https://doi.org/10.1080/17441692.2018.1471148
Rauh, S., Torgerson, T., Johnson, A. L., Pollard, J., Tritz, D., & Vassar, M. (2020). Reproducible and transparent research practices in published neurology research. Research Integrity and Peer Review , 5 . https://doi.org/10.1186/s41073-020-0091-5 .
Reed-Berendt, R., Dove, E. S., & Pareek, M. (2022). The ethical implications of big data research in public health: “Big Data Ethics by Design” in the UK-REACH study. Ethics and Human Research, 44 (1), 2–17. https://doi.org/10.1002/eahr.500111
Resnik, D. (1996). Review: Ethics of scientific research by Shrader-Frechette, Kristin. Noûs , 30 (1), 133–143. https://doi.org/10.2307/2216307 .
Resnik, D. B. (1998). The ethics of science: An introduction. Philosophical issues in science. Routledge.
Resnik, D. B. (2018a). Difficulties with applying a strong social value requirement to clinical research. The Hastings Center Report, 48 (6), 35–37. https://doi.org/10.1002/hast.936
Resnik, D. B. (2018b). Examining the social benefits principle in research with human participants. Health Care Analysis, 26 (1), 66–80. https://doi.org/10.1007/s10728-016-0326-2
Resnik, D. B., & Shamoo, A. E. (2011). The singapore statement on research integrity. Accountability in Research, 18 (2), 71–75. https://doi.org/10.1080/08989621.2011.557296
Reydon, T. (2013). Wissenschaftsethik: Eine Einführung. UTB Philosophie, Naturwissenschaften , 4032. Ulmer.
Rosenbaum, S. (2010). Data governance and stewardship: Designing data stewardship entities and advancing data access. Health Services Research, 45 (5 Pt 2), 1442–1455. https://doi.org/10.1111/j.1475-6773.2010.01140.x
Ross, W. D. (1930). The right and the good . Clarendon.
Russell, C. (1993). Academic freedom. (1 st edition). Routledge.
Sardanelli, F., Alì, M., Hunink, M. G., Houssami, N., Sconfienza, L. M., & Di Leo, G. (2018). To share or not to share? Expected pros and cons of data sharing in radiological research. European Radiology, 28 (6), 2328–2335. https://doi.org/10.1007/s00330-017-5165-5
Schickhardt, C., Hosley, N., & Winkler, E. C. (2016). Researchers’ duty to share pre-publication data: From the prima facie duty to practice. In B. D. Mittelstadt & L. Floridi (Eds.), The ethics of biomedical big data (pp. 309–337). Springer.
Schwartz, J. S. J. (2020). The value of science in space exploration . Oxford University Press. https://doi.org/10.1093/oso/9780190069063.001.0001
Sen, A. (2002). Why health equity? Health Economics, 11 (8), 659–666. https://doi.org/10.1002/hec.762
Sim, I., Stebbins, M., Bierer, B. E., Butte, A. J., Drazen, J., Dzau, V., Hernandez, A. F., Krumholz, H. M., Lo, B., Munos, B., Perakslis, E., Rockhold, F., Ross, J. S., Terry, S. F., Yamamoto, K. R., Zarin, D. A., & Li, R. (2020). Time for NIH to lead on data sharing. Science, 367 (6484), 1308–1309. https://doi.org/10.1126/science.aba4456
Stewart, S. L. K., Pennington, C. R., da Silva, G. R., Ballou, N., Butler, J., Dienes, Z., Jay, C., Rossit, S., & Samara, A. (2022). Reforms to improve reproducibility and quality must be coordinated across the research ecosystem: The view from the UKRN local network leads. BMC Research Notes , 15 (1). https://doi.org/10.1186/s13104-022-05949-w .
Strcic, Josip, Civljak, Antonia, Glozinic, Terezija, Pacheco, Rafael Leite, Brkovic, Tonci, & Puljak, Livia (2022): Open data and data sharing in articles about COVID-19 published in preprint servers medRxiv and bioRxiv. Scientometrics 127 (5), S. 2791–2802. https://doi.org/10.1007/s11192-022-04346-1 .
Tan, Aidan Christopher, Askie, Lisa M., Hunter, Kylie Elizabeth, Barba, Angie, Simes, Robert John, & Seidler, Anna Lene (2021): Data sharing-trialists' plans at registration, attitudes, barriers and facilitators: A cohort study and cross-sectional survey. Research Synthesis Methods,1 2 (5), S. 641–657. https://doi.org/10.1002/jrsm.1500
Tedersoo, Leho, Küngas, Rainer, Oras, Ester, Köster, Kajar, Eenmaa, Helen, Leijen, Äli et al. (2021). Data sharing practices and data availability upon request differ across scientific disciplines. Scientific Data , 8 (1), Artikel 192. https://doi.org/10.1038/s41597-021-00981-0 .
Tedersoo, L., Küngas, R., Oras, E., Köster, K., Eenmaa, H., Leijen, Ä., Pedaste, M., Raju, M., Astapova, A., Lukner, H., Kogermann, K., & Sepp, T. (2021). Data sharing practices and data availability upon request differ across scientific disciplines. Scientific Data , 8 (1). https://doi.org/10.1038/s41597-021-00981-0 .
Terry, R. F., Littler, K., & Olliaro, P. L. (2018). Sharing health research data - the role of funders in improving the impact. F1000Research , 7 . https://doi.org/10.12688/f1000research.16523.2 .
Thelwall, M., Munafò, M., Mas-Bleda, A., Stuart, E., Makita, M., Weigert, V., Keene, C., Khan, N., Drax, K., & Kousha, K. (2020). Is useful research data usually shared? An investigation of genome-wide association study summary statistics. PLOS ONE , 15 (2). https://doi.org/10.1371/journal.pone.0229578 .
Titus, S., & Bosch, X. (2010). Tie funding to research integrity. Nature, 466 (7305), 436–437. https://doi.org/10.1038/466436a
Towse, John N., Ellis, David A., & Towse, Andrea S. (2021). Opening Pandora's Box: Peeking inside psychology's data sharing practices, and seven recommendations for change. Behavior Research Methods 53 (4), S. 1455–1468. https://doi.org/10.3758/s13428-020-01486-1 .
Watson, Clare (2022). Many researchers say they'll share data - but don't. Nature 606 (7916), S. 853. https://doi.org/10.1038/d41586-022-01692-1 .
Wendler, D., & Rid, A. (2017). In defense of a social value requirement for clinical research. Bioethics, 31 (2), 77–86. https://doi.org/10.1111/bioe.12325
Wertheimer, A. (2015). The social value requirement reconsidered. Bioethics, 29 (5), 301–308. https://doi.org/10.1111/bioe.12128
Wilholt, T. (2010). Scientific freedom: Its grounds and their limitations. Studies in History and Philosophy of Science Part A, 41 (2), 174–181. https://doi.org/10.1016/j.shpsa.2010.03.003
Wilholt, T. (2012). Die Freiheit der Forschung: Begründungen und Begrenzungen . Suhrkamp.
Wilkinson, M. D., Dumontier, M., Aalbersberg, I. J. J., Appleton, G., Axton, M., Baak, A., Blomberg, N., Boiten, J.-W., da Silva Santos, L. B., Bourne, P. E., Bouwman, J., Brookes, A. J., Clark, T., Crosas, M., Dillo, I., Dumon, O., Edmunds, S., Evelo, C. T., & Finkers, R. … Mons, B. (2016). The FAIR guiding principles for scientific data management and stewardship. Scientific Data , 3 . https://doi.org/10.1038/sdata.2016.18 .
Winkler, E. C., Jungkunz, M., Thorogood, A. et al. (2023). Patient data for commercial companies? An ethical framework for sharing patients’ data with for-profit companies for research . Journal of Medical Ethics. https://doi.org/10.1136/jme-2022-108781
de Winter, J., & Kosolosky, L. (2013). The epistemic integrity of scientific research. Science and Engineering Ethics, 19 (3), 757–774. https://doi.org/10.1007/s11948-012-9394-3
World Conference on Research Integrity (2010). Singapore Statement on Research Integrity. Retrieved 25 February 2022 https://wcrif.org/guidance/singapore-statement .
World Medical Association. (2013). World medical association declaration of Helsinki: Ethical principles for medical research involving human subjects. JAMA, 310 (20), 2191–2194. https://doi.org/10.1001/jama.2013.281053
Xafis, V., Schaefer, G. O., Labude, M. K., Brassington, I., Ballantyne, A., Lim, H. Y., Lipworth, W., Lysaght, T., Stewart, C., Sun, S., Laurie, G. T., & Tai, E. S. (2019). An ethics framework for big data in health and research. Asian Bioethics Review, 11 (3), 227–254. https://doi.org/10.1007/s41649-019-00099-x
Ziman, J. (2009). Real science. Cambridge University Press. https://doi.org/10.1017/CBO9780511541391
Download references
Acknowledgements
The authors would like to thank the following individuals and groups for their contributions to this project: our partners within the joint research project DATABLIC, Prof. Dr. Michael Fehling and Miriam Tormin (Bucerius Law School, Hamburg), Prof. Dr. Christiane Schwieren and Tamás Olah (University of Heidelberg); all members of the Section Translational Medical Ethics at the National Center for Tumour Diseases, Heidelberg, especially the head of section Prof. Dr. Dr. Eva Winkler; Maya Doering for assistence with literature review and formatting.
The work on this article has been funded by the German Ministry for Education and Research (Bundesministerium für Bildung und Forschung, funding reference no. 01GP1904A) as part of the joint research project DATABLIC. The funder had no role in research design, analysis, decision to publish, or preparation of the manuscript.
Author information
Christian Wendelborn
Present address: University of Konstanz, Konstanz, Germany
Authors and Affiliations
Section for Translational Medical Ethics, German Cancer Research Center (DKFZ), National Center for Tumor Diseases (NCT) Heidelberg, Heidelberg, Germany
Christian Wendelborn, Michael Anger & Christoph Schickhardt
You can also search for this author in PubMed Google Scholar
Contributions
Conceptualization: CW and CS. Methodology: CW and CS. Ethical analysis and investigation: CW and CS. Writing—original draft preparation: CW. Writing—review and editing: MA and CS. Supervision: CS. Project proposal and successful application: CS
Corresponding author
Correspondence to Christian Wendelborn .
Ethics declarations
Conflict of interest.
The authors declare that no competing interests exist.
Additional information
Publisher's note.
Springer Nature remains neutral with regard to jurisdictional claims in published maps and institutional affiliations.
Rights and permissions
Open Access This article is licensed under a Creative Commons Attribution 4.0 International License, which permits use, sharing, adaptation, distribution and reproduction in any medium or format, as long as you give appropriate credit to the original author(s) and the source, provide a link to the Creative Commons licence, and indicate if changes were made. The images or other third party material in this article are included in the article's Creative Commons licence, unless indicated otherwise in a credit line to the material. If material is not included in the article's Creative Commons licence and your intended use is not permitted by statutory regulation or exceeds the permitted use, you will need to obtain permission directly from the copyright holder. To view a copy of this licence, visit http://creativecommons.org/licenses/by/4.0/ .
Reprints and permissions
About this article
Wendelborn, C., Anger, M. & Schickhardt, C. Promoting Data Sharing: The Moral Obligations of Public Funding Agencies. Sci Eng Ethics 30 , 35 (2024). https://doi.org/10.1007/s11948-024-00491-3
Download citation
Received : 21 October 2022
Accepted : 08 June 2024
Published : 06 August 2024
DOI : https://doi.org/10.1007/s11948-024-00491-3
Share this article
Anyone you share the following link with will be able to read this content:
Sorry, a shareable link is not currently available for this article.
Provided by the Springer Nature SharedIt content-sharing initiative
- Data sharing
- Epistemic integrity
- Funding agencies
- Moral obligations
- Research integrity
- Scientific progress
- Scientific freedom
- Social value
- Find a journal
- Publish with us
- Track your research
The definitive guide to Project 2025
Media Matters presents a close look at Project 2025, from a secretive 180-day plan to a MAGA staffing database to extreme proposals that would turn back the clock on a whole host of issues
Written by Media Matters Staff
Research contributions from Madeline Peltz , John Knefel , Jacina Hollins-Borges , Jack Wheatley , Sophie Lawton , Justin Horowitz , Allison Fisher & Olivia Little
Published 08/08/24 10:36 AM EDT
Project 2025 is an extreme right-wing initiative organized by The Heritage Foundation to provide policy and personnel to the next Republican presidential administration. The effort involves more than 100 partner organizations, a database of potential MAGA staffers, a secret 180-day plan, and its nearly 900-page policy book — Mandate for Leadership: The Conservative Promise — represents a major threat to democracy. The below PDF is also available here .
The definitive guide to Pro... by Media Matters for America
New Media Matters research into Project 2025’s extremist goals
Proposals in the Mandate for Leadership would severely inhibit the federal government’s protections for reproductive rights, LGBTQ rights, labor and civil rights, and immigrants, as well as its climate change efforts. They would allow Trump to weaponize the justice system as his own personal retribution machine, gut the American system of checks and balances and purge the federal bureaucracy of experienced civil servants who haven’t pledged fealty to Trump. On their own platforms, Project 2025 partners frequently speak in even more draconian terms.
Here’s a sampling of the agenda that the Heritage Foundation and Project 2025 are pushing to implement on day one of a second Trump administration:
- Reimplementing “Schedule F,” a Trump-era executive order that removes civil service protection for career bureaucrats so they can be fired and replaced with far-right loyalists.
- Adopting an extreme anti-choice agenda that would restrict legal abortion drugs and emergency contraception, and that could also impact fertility-related health care like IVF and surrogacy services.
- Undermining checks and balances in the federal government and consolidating the president’s power to weaponize the Department of Justice and law enforcement against his political enemies.
- Institutionalizing the right-wing movement’s war on LGBTQ communities by promoting conversion therapy and stripping queer people of federal protections.
- Eviscerating labor rights, including union negotiating rights and protections for overtime pay.
- Allowing high-income earners to more easily cheat the IRS.
- Rejecting climate science in favor of Big Oil’s preferred policies by gutting federal agencies that protect the environment and dismantling regulations allowing polluting industries to extract even more oil and gas from federal lands with less protections.
- Potentially conducting mass deportations of millions of immigrants or those suspected to be immigrants, in part by revoking all Temporary Protected Status designations, which would put more than 863,000 people at risk.
- Overhauling the American education system by eliminating the Department of Education and making student loans more expensive.
- Purging the federal government of diversity, equity, and inclusion initiatives.
- Expelling transgender service members from the military and eliminating DEI initiatives from the military to “restore standards of lethality and excellence.”
Key Media Matters research on Project 2025
Media Matters has also recently published thorough research on Project 2025 and the MAGA media universe, including:
- An exhaustive analysis of Project 2025’s multi-pronged attacks on reproductive rights.
- A resource guide that outlines the specific policy and personnel priorities of Project 2025.
- A guide to the MAGA media universe.
- Deep-dive research on what leading MAGA figures expect from a second Trump term.
A full list of Media Matters content on Project 2025 can be found here .
More From Forbes
Capital one: the ongoing story of how one firm has been pioneering data, analytics, & ai innovation for over three decades.
- Share to Facebook
- Share to Twitter
- Share to Linkedin
Capital One
Pioneers are trailblazers. They operate as agents of transformation and change. They think differently. They innovate and introduce a new order. In the late 1980s, a pair of trailblazing strategy consultants came together with a new idea. Their idea? To use data to expand access to credit cards and do so more efficiently. This idea became the foundation for Capital One. In an industry historically dominated by century old banks, Capital One has been a trailblazer.
From start up to top ten bank, Capital One has revolutionized the credit card industry with data and technology. Capital One is approaching its 30th anniversary under the leadership of Founder, CEO, and Chairman, Rich Fairbank. Today, Capital One serves more than 100 million customers across a diverse set of businesses and has established one of the most widely recognized brands in banking.
I’ve had the good fortune to write about Capital One on multiple occasions over the years. In a 2019 Forbes article, From Analytics First to AI First at Capital One , co-authored with my industry colleague Tom Davenport, we wrote, “Capital One has long been known as a north star for financial services firms that aspire to be data-driven. Established in 1994 after spinning off of Signet Bank, the core idea behind the company’s formation was the “information-based strategy”—that important operational and financial decisions should be made on the basis of data and analytics”.
Data Leadership and the Role of Chief Data Officer (CDO) at Capital One
This past month, I had the privilege of interviewing Capital One’s current Chief Data Officer (CDO) and Executive Vice President, Amy Lenander, as part of a CDO panel that I have been organizing and moderating for the annual CDOIQ Symposium , which was launched 18 years ago at the Massachusetts Institute of Technology (MIT). Lenander was appointed CDO of Capital One in early 2023. She had previously led business functions for the company during a 20-year tenure, including a term as the CEO of Capital One U.K.
I asked Lenander about the evolution of the CDO role at Capital One. Lenander explained, “My role as Chief Data Officer is primarily about setting our company up to make the most of our data to power the future of Capital One.” She continued, “Part of the reason that the CDO role was created more than two decades ago was our recognition that establishing a rigorous, well-managed data ecosystem across the enterprise requires dedicated leadership from the top”.
Best High-Yield Savings Accounts Of 2024
Best 5% interest savings accounts of 2024.
Lenander understands the heritage of Capital One, noting, “In a company like Capital One, we need and expect data to enable associates across the company to discover insights, make decisions, and drive innovation and value for the business and our customers. This was true back in the early 2000s and remained true as we began our technology transformation and moved to the public cloud, and as the volume of data available continued to grow”.
Lenander appreciates that being data-driven is a way of life at Capital One and has been since its founding. Lenander comments, “What’s changed over time is the methods we use to find insights and how we use them to improve our business”. She continued, “Our data-driven culture means that there’s an incredibly strong pull from business leaders to continually learn about and use better and better techniques to drive more powerful insights. Along the way, every major division of our company has had leaders accountable for the data in their line of business, and data has remained central to our culture and to how we operate”.
Change has been a constant in the world of data over the past few decades. Lenander observes, “In a world where analytic techniques and even data management approaches are rapidly evolving, I look to build a team of talented people that are curious, great problem solvers, and continuous learners. In this environment, it’s also important to have a strong culture of experimentation and sharing lessons learned along the way”.
I asked Lenander about the importance of having a guiding data strategy at Capital One. Lenander explained, “We have an enterprise data strategy because one of the ways we can achieve our goals is by using a common set of data platforms across the company, and to achieve that, we need data owners across the company to work back from a common strategy”. She elaborated, “One of the benefits of that strategy is to make data available to use across the company, for example, so that we can use information about customers’ experiences with their bank account to inform how we might better service their credit card account”.
Lenander recognizes that what often differentiates one company from another is the ability to execute. Lenander comments, “This is one of those things where the goals and the strategy are relatively easy to say, but the execution is very difficult”. She continues, “Our data strategy is focused on ensuring we can make the most of our data as we continue to evolve our business”.
Ultimately, the value of data comes from the ability to serve the business. As a longtime business leader at Capital One, Lenander appreciates that, “Our data strategy is all about enabling the business. My prior experience leading businesses across Capital One has given me empathy and a deep understanding of where the value is in the business and where data can make the most difference”. She notes, “The goal of our data strategy is to make data well-managed and easy to find, understand, use and govern”.
The State of Data & AI at Capital One Today
In our 2019 Forbes article on AI at Capital One, we noted that Capital One was investing in how to apply AI in its business well before most of its peers. Lenander comments, “AI raises the stakes because AI is incredibly data-hungry, uses many more types of data, including unstructured data, and can be less explainable in the relationships that models are finding within data”. She understands that great AI requires great data, noting, “That means that there’s an even bigger need with AI to ensure data is of high quality”.
Lenander observes that, “Organizations that have plentiful, well-managed data have a huge advantage in their ability to leverage AI”. She continues, “Our investments in technology and data infrastructure and talent — along with our deep experience in machine learning — have set us up to be at the forefront of enterprises leveraging AI today”.
To continue to capitalize on momentum in data and AI, Capital One has established dedicated leadership roles to drive and evolve its enterprise strategies in these domains, which include the early 2023 appointment of Prem Natarajan as Executive Vice President, Chief Scientist, and Head of Enterprise AI for Capital One. Lenander and Natarajan serve as peers who partner closely on Capital One’s data journey. Natarajan leads Capital One’s AI initiatives, and both are part of a central Enterprise organization that has leaders accountable for core domains like AI and Data, as well as for core functions such as Engineering, Science, Design, and Product. The senior leaders of the Enterprise organization collectively drive Data, AI, and the innovation agenda for Capital One in close partnership with senior leaders across the company.
I asked Natarajan about his vision for AI at Capital One. Natarajan commented, “ From its inception, Capital One has had a reverence for insights derived from data. Culturally, we subscribe to data-driven methodologies for decision making”. He explains, “That has set us up very, very well for the current age of machine learning and AI”, adding, “When we think about future waves of AI and their impact, we take a cautiously optimistic view that is informed by our vision of an AI-powered enterprise in which all our associates and customers benefit from the real-time intelligent experiences and services that such an enterprise will deliver”.
Lenander adds her perspective, noting, “Data, machine learning and AI are central components of how we operate and serve our customers. Today we are embedding AI throughout our business with proprietary solutions built on our modern tech stack, and we have hundreds of use cases in production that use machine learning or AI to deliver value for our customers, associates, and the company”.
Developing Safeguards and Charting an AI Future
Natarajan is highly cognizant of the need for safeguards to ensure responsible AI use, recognizing that, as with any new and rapidly evolving technology, the potential benefits have to be balanced with a thoughtful, responsible approach that appropriately manages risk right from the start. This is especially the case due to the more powerful capabilities of modern Generative AI technologies. He comments, “We are anchoring on a test-and-learn approach to both identify the highest leverage areas for AI as well as the safest ways to deploy them while delivering benefits to our customers”.
Continuing on the topic of responsible AI, Natarajan states that across AI initiatives, Capital One is guided by a mission to build and deploy AI in a responsible, well-managed way that puts people first. Natarajan notes, “When developed responsibly, AI will continue to democratize access to a whole suite of insights, resources, and everyday conveniences across the entire social spectrum – in areas from finance to healthcare to education and more”. He continues, “Perhaps the most important safeguard is a cultural one, because it is such a strong determinant of practices and outcomes”.
Natarajan underscores the importance of thoughtful collaboration, commenting, “To maximize the benefits of AI, it is important to adopt an inclusive approach from the outset”. He adds, “A spirit of responsibility and thoughtfulness needs to pervade the entire development process, from research and experimentation to design, building, testing, and refining, through the whole development and production lifecycles”.
Capital One recognizes the need for extensive testing and implementation of human-centered guardrails before introducing AI systems into any customer or business setting. Natarajan comments, “For Capital One, this includes a Model Risk Management framework to evaluate, assess, validate, and govern models to effectively manage and mitigate risks associated with AI”. He notes that banks like Capital One have robust risk management infrastructure, oversight mechanisms, and governance capabilities that are required to manage risk and to deploy and scale AI appropriately.
Earlier this year, Capital One established the Center for AI and Responsible Financial Innovation with Columbia University and the Center for Responsible AI and Decision Making in Finance with the University of Southern California to advance state-of-the-art research in responsible AI and its application to finance. Capital One are also partners in multisector consortiums like the Partnership on AI, where Natarajan is on the Board, and with institutions like the National Sciences Foundation.
Natarajan comments, “We have a strong belief in the value of multi-sector partnerships between industry, academia, and government to ensure diverse perspectives and equities when developing, testing, and deploying AI”. He adds, “We are helping to advance research and strengthen our national capabilities in AI”.
Examples of Capital One initiatives that have been designed to measure the value of new data, analytics, and AI techniques, include:
1. A proprietary generative AI agent servicing tool which is helping agents access information to resolve customer questions more quickly and efficiently. For example, if a customer calls in about a lost or misplaced credit card, agents can get them a virtual card number immediately and have a new card delivered, so their ability to spend is uninterrupted and resolved more efficiently than ever. Lenander notes, “This tool has been used thousands of times by hundreds of agents, with over 95% of search results found highly relevant by our agents”.
2. An AI model that is used to customize the user experience across digital and mobile channels to help put the most relevant information in customers’ hands. Lenander comments, “The model is driving double digit improvement in relevance of personalization than the prior machine learning model and allows for rapid experimentation and iteration as we continue to enhance the customer experience”.
3. A proprietary fraud platform that leverages AI and machine learning to proactively surface and mitigate fraud in the time it takes a customer to swipe their card. Lenander notes, “We are continuing to experiment with new AI capabilities in fraud to stay at the leading edge of this space”.
Reflecting on the Capital One data and AI journey and how the company has evolved over the past three decades, Lenander concludes, “We have a long history of using data to drive our business strategies, and in a cloud and AI world, there is both massive opportunity and the potential for massive complexity”. She adds, “Cultivating a modern data ecosystem and being AI-ready is an ongoing journey, and we continually evolve our approach to data so that we can be best-prepared”.
Natarajan, reflecting on his mandate to advance the development and application of AI within Capital One, concludes, “Ultimately, AI is at its best when it empowers individuals and societies to achieve things that weren’t possible before – and we all need to come together to actively work towards that future”.
I look forward to seeing what comes next from Capital One as they further pioneer data, analytics, and AI leadership on the frontier of business innovation. What’s in your wallet?
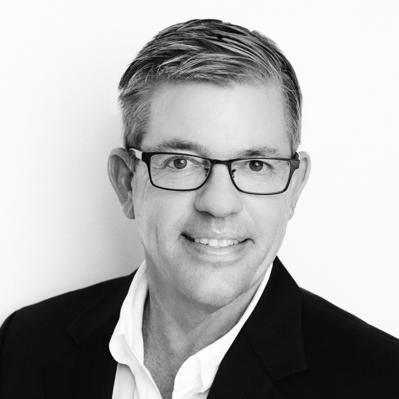
- Editorial Standards
- Reprints & Permissions
- Go back to Main Menu
- Client Log In
- MSCI Client Support Site
- Barra PortfolioManager
- MSCI ESG Manager
- MSCI ESG Direct
- Global Index Lens
- MSCI Real Assets Analytics Portal
- RiskManager 3
- CreditManager
- RiskManager 4
- Index Monitor
- MSCI Datscha
- MSCI Real Capital Analytics
- Total Plan/Caissa
- MSCI Fabric
- MSCI Carbon Markets
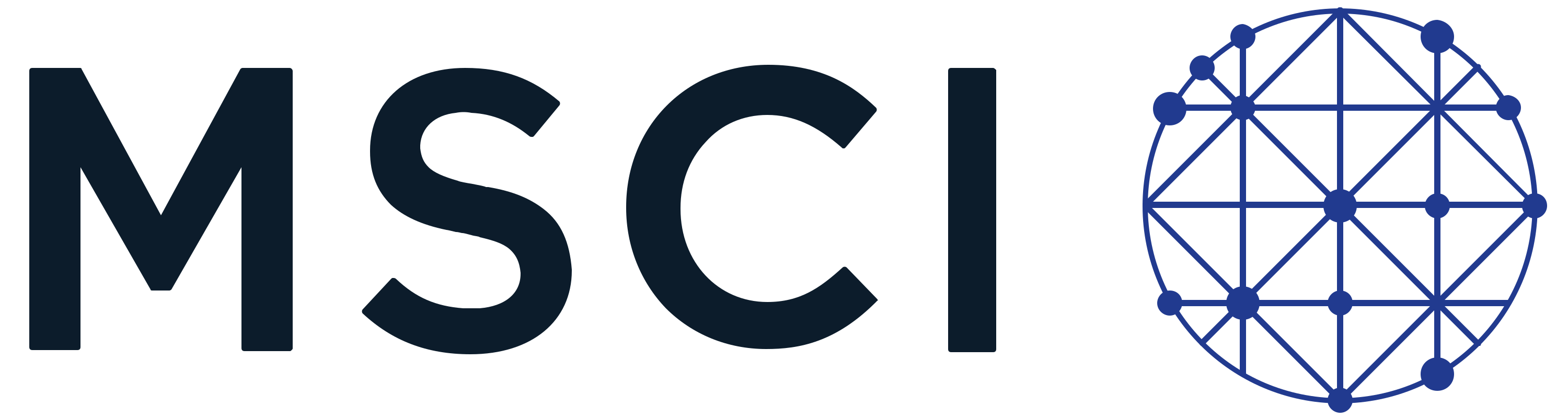
Navigation Menu
- Our Clients
Insights on MSCI One
Institutional client designed indexes (icdis), total portfolio footprinting, esg trends to watch, factor models, visualizing investment data.
- Our Solutions
- Go back to Our Solutions
- Analytics Overview
- Risk Management
- AI Portfolio Insights
- Multi-asset Class Factor Models
- Quantitative Investment Solutions
- Fixed Income Analytics
- Portfolio Management
- Crowding Solutions
- Regulatory Solutions
- Managed Solutions
- Climate Investing
- Climate Investing Overview
Implied Temperature Rise
Trends 2024.
- Biodiversity
- Carbon Markets
- GeoSpatial Asset Intelligence
- Portfolio Sustainability Insights
- Real Estate Climate Solutions
- Sustainable Investing
- Sustainable Investing Overview
ESG and Climate Funds in Focus
What is esg, role of capital in the net-zero revolution.
- Sustainability Reporting Services
- Factor Investing
- Factor Investing Overview
MSCI Japan Equity Factor Model
- Equity Factor Models
- Factor Indexes
- Indexes Overview
Index Education
Msci climate action corporate bond indexes.
- Client-Designed
- Direct Indexing
- Fixed Income
- Private Real Assets
Thematic Exposure Standard
- Go back to Indexes
- Resources Overview
MSCI Indexes Underlying Exchange Traded Products
- Communications
- Equity Factsheets
- Derivatives
- Methodology
- Performance
- Private Capital
- Private Capital Overview
Global Private Capital Performance Review
- Total Plan (formerly Caissa)
- Carbon Footprinting
- Private Capital Indexes
- Private Company Data Connect
- Real Assets
- Real Assets Overview
Real Estate Market Size
- Index Intel
- Portfolio Services
- Property Intel
- Private Real Assets Indexes
- Real Capital Analytics
- Research & Insights
- Go back to Research & Insights
- Research & Insights Overview
- Multi-Asset Class
- Real Estate
- Sustainability
- Events Overview
Capital for Climate Action Conference
- Data Explorer
- Developer Community
- Technology and Data
2022 Annual Report
- Go back to Who We Are
- Corporate Responsibility
- Corporate Responsibility Overview
- Enabling Sustainable Investing
- Environmental Sustainability
- Governance Practices
- Social Practices
- Sustainability Reports and Policies
- Diversity, Equity and Inclusion
Henry A. Fernandez
- Recognition
Main Search
Extended viewer.
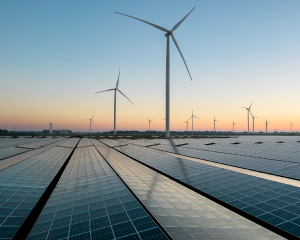
Related resources
- The First Core Carbon Principles-Qualifying Projects — the VCM Steps on the Gas
- Potential Impact of the Core Carbon Principles on the Global Carbon Credit Market
- Voluntary Carbon Market 1Q23 in Review – the State of Integrity
All blog articles
Renewable-Energy Carbon Credits Losing Steam
8 mins read August 07, 2024 | Tristan Loffler , Guy Turner , Jamie Saunders , Lucien Georgeson
Key findings
- Renewable-energy projects’ future appears uncertain due to integrity concerns, particularly around additionality. MSCI’s integrity assessments of 1,700+ projects show they are the lowest-rated project type, with 78% scoring less than three out of five (versus 30% across all other projects).
- In recognition of these concerns, on Aug. 6, the Integrity Council for the Voluntary Carbon Market (ICVCM) decided that renewable-energy projects would not receive its high integrity Core Carbon Principles (CCP) label.
- Despite the lower ratings of these projects, carbon credits can still play a role financing renewable-energy expansion. Efforts going forward should focus on projects that cannot operate without carbon-credit financing.
In support of society’s ever-growing need for energy, the global carbon-credit market has channeled finance to renewable projects for over a decade. Since 2010, over 750 million voluntary carbon credits have been issued by some 1,700 renewable-energy projects. This accounts for 30% of all credits issued, and 36% of credits retired by corporates. Some 40% of issued credits have come from wind projects, 30% from hydro, 15% from solar and 15% from other renewable types. [1]
Despite their historical prevalence, renewable-energy projects as a source of credits seem to be running out of steam. While global investment in renewable energy rose by 8% in 2023 to USD 623 billion, virtually none of this new funding was provided via carbon credits. [2]
Furthermore, in 2020, the two largest carbon-crediting standards, Gold Standard and Verra, imposed restrictions on the eligibility of new renewable-energy projects to generate carbon credits, citing falling costs of wind and solar power and prevalent government schemes to support their adoption. Verra now only permits projects from new renewable-energy projects in Least Developed Countries, and only if they are not large-scale hydro. Gold Standard only allows new projects in small-island and landlocked developing countries, and in nations where the penetration of the technology concerned is less than 5% of total grid-connected capacity, as well as offshore wind and waste-to-energy projects.
Finally, just this week (Aug. 6), the ICVCM announced that all renewable-energy credits would fail its integrity benchmark as they are “insufficiently rigorous” in their additionality. [3]
Assessing the integrity of renewable-energy carbon credits
Despite restrictions on new renewable-energy projects, there are still 350 million carbon credits available for purchase from currently registered projects, and more may be issued. Corporates looking to use these credits should certainly consider their integrity ahead of any purchase. [4]
MSCI Carbon Markets assessed the integrity of more than 1,700 existing renewable-energy projects, scoring each out of five. [5] While they all undoubtedly have climate benefits, they can carry material risks from a credit-integrity perspective.
On the positive side, renewable-energy projects score highly on their “quantification” risk (as the electricity generated by a renewable plant is generally easily and accurately measured) and “permanence” (given they prevent the release of carbon rather than storing it, unlike, say, forestry projects). Instead, their key integrity issue is their “additionality” (that the project in question would have proceeded without the carbon-credit revenue stream).
There are two typical ways to assess this additionality — through investment returns or “common practice.” [6] When considering the former, of the more than 1,700 registered projects we examined, carbon credits represented less than 4% of their total revenue, with hydro and solar closer to 3% (as shown in the exhibit below). With carbon credits representing such a low proportion of future revenue, it is less likely that they drove the decision to develop the renewable-energy plant, particularly for larger-scale hydro, wind or solar plants where upfront capital costs can run into hundreds of million dollars.
Percentage of project revenue coming from carbon credits
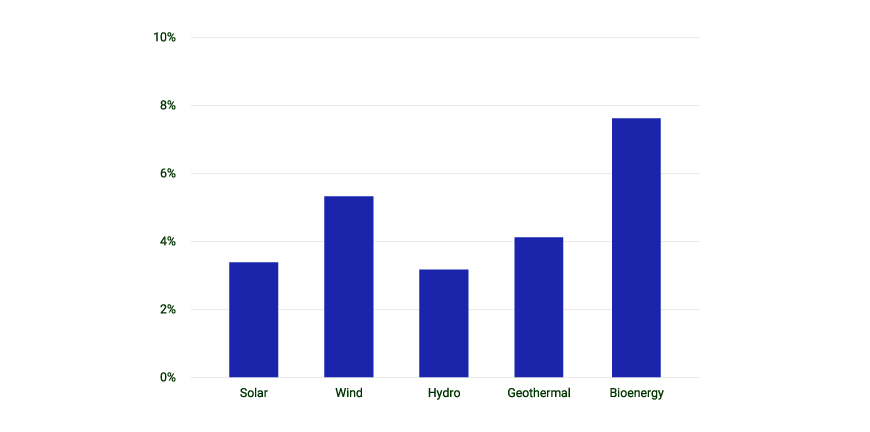
Investment returns don’t always tell the whole story, however. In some markets, there are other barriers to the adoption of renewable energy, such as lack of grid infrastructure, low creditworthiness of electricity purchasers, administrative hurdles or political risk. These aspects are captured in the common-practice assessment.
If a type of renewable energy is already prevalent in a market, then it suggests that the project would be feasible without the carbon-revenue stream. The typical threshold used is whether the technology represents 5% or more of the electricity mix. If this threshold is met it is assumed that common practice already exists, and if not, then there is a case for a carbon-credit project being additional.
The relevance of the common-practice test differs by market size. For larger markets, even 5% of the electricity mix requires significant technology deployment, to the point that it could be regarded as common practice, given the sheer size of the power sector.
This is illustrated by looking at the electricity mix of China, India and Turkey. Hydro power represented over 10% of the electricity mix in all three markets since 2000, meaning it wouldn’t be eligible on the common-practice basis. Wind would not, however, have passed the common-practice test in China until 2017, in Turkey until 2016 and still fails for India. Solar has never represented more than 5% of the electricity mix in any of these markets, but arguably is a widely investable technology type. Consequently, for these large developing economies, investment-return analysis is the more relevant test of additionality.
In smaller markets with less-developed infrastructure and support mechanisms for renewables, common practice is a more useful indicator of additionality. In these markets there are many reasons why renewables are challenging to develop, and carbon credits may play a stronger role in stimulating new investments.
Penetration of renewable-energy project subtypes by market (% electricity production)
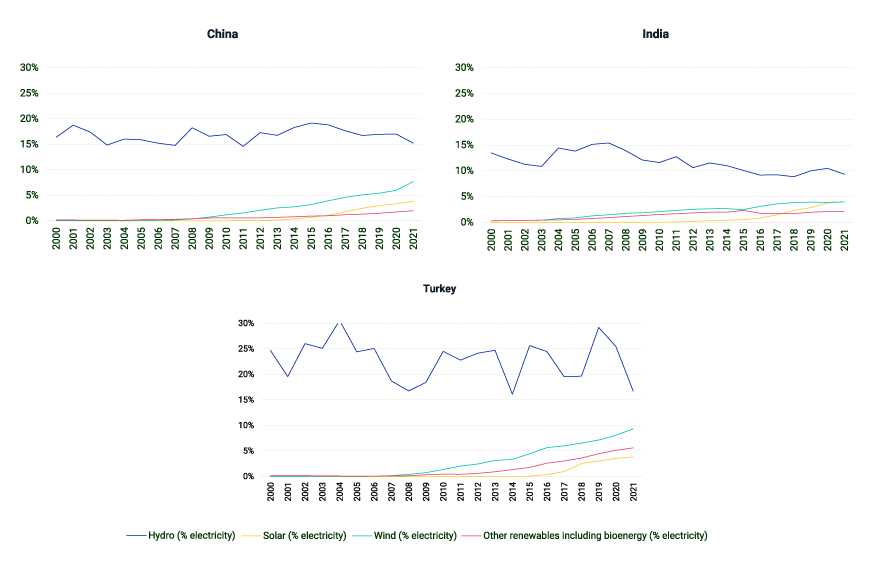
Buyer beware
Taking these criteria and others into account, our analysis shows that renewable-energy projects are typically the lowest rated for integrity in the carbon-credit market. While there is variation depending on project specifics, 78% of renewable-energy projects received an overall MSCI integrity score of less than three out of five, representing very high integrity risk, compared to 30% for other project types, as detailed in the final exhibit.
In the renewables sector, the highest integrity scores tend to correlate with technologies such as micro-scale and off-grid renewables projects and organic waste to energy, where the case for additionality from carbon-credit finance is strongest. These projects are often undertaken in less- and least-developed economies.
Number of voluntary carbon projects by overall integrity score
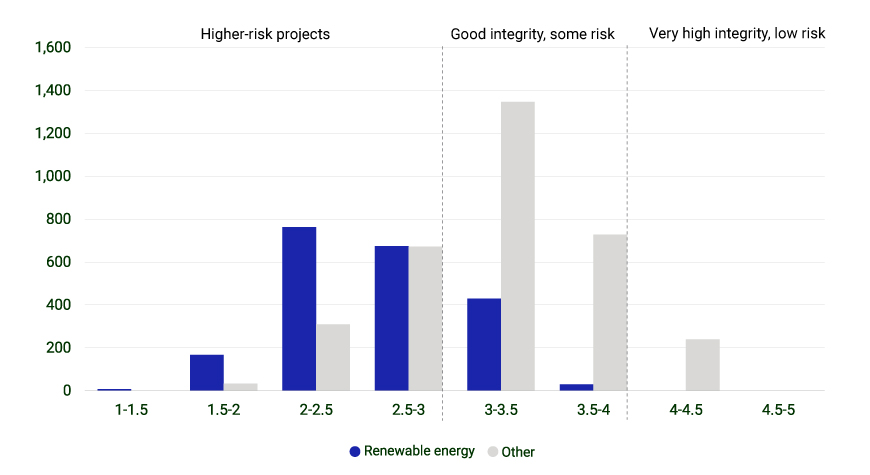
These integrity concerns, together with significant supply volume, are reflected in the price of credits. MSCI Carbon Markets data shows that renewable energy was consistently the lowest-priced type of carbon credit with average prices between just USD 1.80 and USD 2.80 per tonne of CO2 equivalent during the first half of 2024, compared to USD 10 or more for nature-restoration credits, and around USD 5 for clean-cooking credits. [7]
Silver linings
Displacing fossil-energy sources at scale will be expensive, despite the decline in the cost of clean energy. It remains to be seen, though, if the voluntary carbon market is the best-suited funding mechanism to support this growth in renewable-energy capacity.
Some think it is. The relatively new Global Carbon Council (GCC) registry contains nearly 1,000 renewable-energy projects in their pipeline, although it is unclear how many of these the GCC will eventually register. Given the ICVCM’s recent decision on renewable-energy projects, it seems unlikely many of these will pass the ICVCM’s eligibility tests.
Others are trying a new approach by using carbon credits to transform energy systems at a jurisdictional or systemic scale. The Energy Transition Accelerator (ETA) is one such example. First proposed in November 2022 by the U.S. government, it seeks to use high-integrity carbon crediting to mobilize between USD 70 and 200 billion in energy-transition finance in developing countries by 2035. Separately, the Coal to Clean Credit Initiative was launched in December 2023 by the Rockefeller Foundation in collaboration with ACEN Corporation, South Pole, the GEAPP and others. This initiative is exploring the use of carbon credits to finance the replacement of coal-fired power plants with renewable energy in emerging economies, with a first pilot project in the Philippines.
These initiatives could transform the degree of support that carbon markets provide to the energy sector, but their impact is likely to hinge on two factors. First, whether carbon credits will be directed toward the types of renewable-energy projects most at need of additional carbon-credit financing. [8] Second, whether demand for carbon credits can be secured in advance. Without this upfront commitment to buy credits, it becomes more challenging to justify the additionality of such capital-intensive projects.
The ICVCM’s recent decision to rule out all renewable-energy credits from CCP eligibility signals a commitment to establishing a significantly higher threshold for integrity going forward but is a somewhat blunt approach. Many renewable-energy projects score poorly on our integrity scale, but not all. The ICVCM’s strict line on all renewable projects comes at the expense of rejecting a smaller number of better-performing projects. The ICVCM has, however, said it is ready to review new, and more rigorous, renewable-energy methodologies, so it remains possible that some renewable-energy projects could still play a role in powering the carbon-credit market.
Tristan Loffler
Executive Director, MSCI Carbon Markets
Managing Director, MSCI Carbon Markets
Jamie Saunders
Lucien Georgeson
Vice President, MSCI Carbon Markets
Research and insights - signup bottom
Interested in our research.
Get the latest trends and insights straight to your inbox.
Select your topics and use cases to stay current with our award winning research, industry events, and latest products.
Email Sign-up
Sign up below to receive the latest updates and news from MSCI.
UtmAnalytics
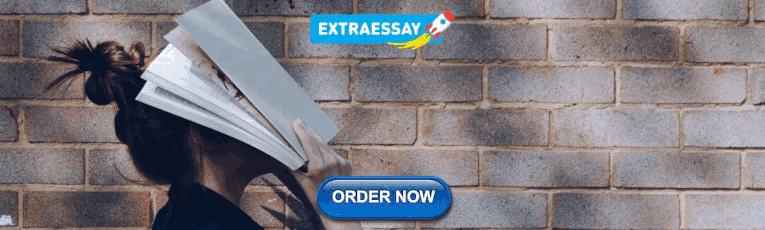
IMAGES
COMMENTS
1. figshare. Figshare is an open access data repository where researchers can preserve their research outputs, such as datasets, images, and videos and make them discoverable. Figshare allows researchers to upload any file format and assigns a digital object identifier (DOI) for citations. Mark Hahnel launched Figshare in January 2011.
Repositories for Sharing Scientific Data. In general, NIH does not endorse or require sharing data in any particular repository, although some initiatives and funding opportunities will have individual requirements. Overall, NIH encourages researchers to select the repository that is most appropriate for their data type and discipline.
Harvard Dataverse is an online data repository where you can share, preserve, cite, explore, and analyze research data. It is open to all researchers, both inside and out of the Harvard community. Harvard Dataverse provides access to a rich array of datasets to support your research. It offers advanced searching and text mining in over 2,000 ...
Helps researchers find data repositories where they can share data as they develop and implement their NIH Data Management and Sharing Plans ... OSF helps research teams work on projects privately or make the entire project publicly accessible for broad dissemination. As a workflow system, OSF enables connections to data, preprints, and data ...
ANDS Contributing Repositories - the data discovery service of the Australian Research Data Commons (ARDC). Dryad Digital Repository - an open source, community driven project that focuses on search, presentation, and discovery and delegates the responsibility for the data preservation function to the underlying repository with which it is ...
Project repositories: data and information related to a specific project may be stored in a project-specific repository. This can help users understand what is and isn't related to a project. Government repositories: research funded by governments or public resources may be stored in government repositories. This data is often publicly ...
Establish a research data management solution for your community. Federate with a growing list of Dataverse repositories worldwide for increased discoverability of your community's data. Participate in the drive to set norms for sharing, preserving, citing, exploring, and analyzing research data. Want to install a Dataverse repository?
Data Repositories. A key aspect of data sharing involves not only posting or publishing research articles on preprint servers or in scientific journals, but also making public the data, code, and materials that support the research. Data repositories are a centralized place to hold data, share data publicly, and organize data in a logical manner.
From Unsplash. Re3Data: Over 2,000 research data repositories, re3data has become the most comprehensive source of reference for research data infrastructures globally.; ELVIRA Biomedical Data Repository: High-dimensional datasets in the biomedical field. It focuses on journal-published data (Nature, Science, and others). Merck Molecular Health Activity Challenge: Datasets designed to foster ...
Abstract. For more than ten years, re3data, a global registry of research data repositories (RDRs), has been helping scientists, funding agencies, libraries, and data centers with finding ...
An open access data repository openly stores data, including scientific data from research projects in a way that allows immediate user access to anyone. There are no limitations to the repository access. As such, repositories make data findable, accessible, and usable in the long term, by using sustainable file formats and providing persistent ...
The Purdue University Research Repository (PURR) provides an online, collaborative working space and data-sharing platform to support Purdue researchers and their collaborators. ... Upload Research Data to Your Project Create a project to upload and share your data with collaborators using our step-by-step form to guide you through the process ...
Revisit the past 6 months of research and capture the data we want to add to our repository as an initial body of knowledgeCreate the first draft taxonomy for our research repository, testing this with a small group of wider stakeholdersLaunch the repository with an initial body of knowledge to a group of wider repository champions
Research Data Repositories Landscape. In 2009 the European Commission concluded that: "The landscape of data repositories across Europe is fairly heterogeneous, but there is a solid basis to develop a coherent strategy to overcome the fragmentation and enable research communities to better manage, use, share and preserve data" .The Commission characterizes the present landscape of ...
Selecting a Data Repository. For some programs and types of data, NIH and/or Institute, Center, Office (ICO) policy (ies) and funding opportunities identify particular data repositories (or sets of repositories) to be used to preserve and share data. For data generated from research subject to such policies or funded under such opportunities ...
Additionally, the Registry of Research Data Repositories is a full scale resource of registered data repositories across subject areas. Both FAIRsharing and Re3Data provide information on an array of criteria to help researchers identify the repositories most suitable for their needs (e.g., licensing, certificates and standards, policy, etc.).
Download Open Datasets on 1000s of Projects + Share Projects on One Platform. Explore Popular Topics Like Government, Sports, Medicine, Fintech, Food, More. Flexible Data Ingestion. Download Open Datasets on 1000s of Projects + Share Projects on One Platform. ... Learning Research Application Well-documented Well-maintained Clean data Original ...
A data repository is also known as a data library or data archive. This is a general term to refer to a data set isolated to be mined for data reporting and analysis. The data repository is a large database infrastructure — several databases — that collect, manage, and store data sets for data analysis, sharing and reporting.
A user research repository is a centralized database which includes all your user research data, UX research reports, and artifacts. Different teams—like design, product, sales, and marketing—can find insights from past projects to contextualize present scenarios and make informed decisions.
The following research data repositories are well established and/or allow users to control access to their data and are recommended for de-identified human subjects research. ... makes available human subjects data collected from hundreds of research projects across many scientific domains. De-identified human subjects data, harmonized to a ...
The University of Arizona Research Data Repository (ReDATA) is the institution's official repository for publicly archiving and sharing research materials (e.g., data, code, images, videos, etc.) created by University of Arizona researchers. ReDATA helps the UArizona community: Comply with funder and journal data sharing policies.
A research repository is a tool that professional user experience (UX) designers use to organize research across multiple professionals. A research repository handles two functions within an organization: growing the awareness of how user experience is important to leadership, product owners and organizations and supporting designers through ...
A research data repository — another common place to start when the driver of the knowledge management work is a ... The ResearchOps research repositories project aims to develop a set of ...
To participate, research teams must submit a proposal for a secondary analysis research project that incorporates data from one or more of the Generalist Repository Ecosystem Initiative (GREI) repositories; other repositories including domain-specific repositories can be used as well (. If selected to advance, teams will receive up to a $25,000 ...
In this blog, we will explore 10 GitHub repositories to help you master statistics. These repositories include code examples, books, Python libraries, guides, documentations, and visual learning materials. 1. Practical Statistics for Data Scientists . Repository: gedeck/practical-statistics-for-data-scientists
1. In the following, we use the term "research data" and "data" as referring to digital data that is collected and/or generated during a research project. We use the term "data sharing" as referring to the act of making data available for other researchers - either for the purpose of transparency of studies and replication of published research results or for the purpose of other ...
The effort involves more than 100 partner organizations, a database of potential MAGA staffers, a secret 180-day plan, ... New Media Matters research into Project 2025's extremist goals.
Reflecting on the Capital One data and AI journey and how the company has evolved over the past three decades, Lenander concludes, "We have a long history of using data to drive our business ...
large infrastructure projects—this is a task better suited for 1930s America, not 2030s America. So, that leaves me a bit pessimistic. That said, utilities and policymakers are starting to ... For research, models or other data related to one or more securities, markets or asset classes (including related services) that may be available to ...
Percentage of project revenue coming from carbon credits Based on a sample of 1,726 projects. Revenue data directly based on project data on estimated issuances, electricity generation and electricity-tariff prices. Source: Project documentation, MSCI Carbon Markets. Investment returns don't always tell the whole story, however.