What are your chances of acceptance?
Calculate for all schools, your chance of acceptance.
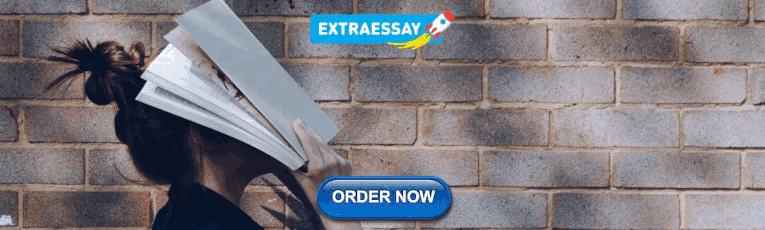
Your chancing factors
Extracurriculars.
Guide to SAT Math Problem Solving and Data Analysis + Practice Questions
Do you know how to improve your profile for college applications.
See how your profile ranks among thousands of other students using CollegeVine. Calculate your chances at your dream schools and learn what areas you need to improve right now — it only takes 3 minutes and it's 100% free.
Show me what areas I need to improve
What’s Covered:
Overview of sat math problem solving and data analysis, how will the sat impact my college chances.
- Strategies for Problem Solving and Data Analysis Questions
- Practice Questions for Problem Solving and Data Analysis
Final Tips and Strategies
Problem Solving and Data Analysis questions appear on the Calculator section of the SAT Math test and involve applying mathematical knowledge to real-world contexts. These problems can be tough, so if you want to improve your math score, here are some strategies and practice problems to help you out.
The SAT Math section contributes to half of the total SAT score. This section is scored out of 800 and includes three main categories, which each have a subscore out of 15.
Here is the breakdown of each category:
- Heart of Algebra: 33%
- Problem Solving and Data Analysis: 29%
- Passport to Advanced Math: 28%
Additional Topics in Math covers the remaining 10% and consists of a variety of different mathematical topics.
The Problem Solving and Data Analysis section tests students’ ability to solve real-world problems using mathematical understanding and skills. This includes quantitative reasoning, interpreting and synthesizing data, and creating representations. These questions never appear on the SAT No Calculator section, so you’ll always be allowed a calculator for them.
Problem Solving and Data Analysis questions ask students to:
- Use ratios, rates, proportional relationships, and scale drawings to solve single- and multistep problems.
- Solve single- and multi-step problems involving percentages.
- Solve single- and multi-step problems involving measurement quantities, units, and unit conversion.
- Given a scatterplot, use linear, quadratic, or exponential models to describe how the variables are related.
- Use the relationship between two variables to investigate key features of the graph.
- Compare linear growth with exponential growth.
- Use two-way tables to summarize categorical data and relative frequencies, and calculate conditional probability.
- Make inferences about population parameters based on sample data.
- Use statistics to investigate measures of center of data and analyze shape, center, and spread.
- Evaluate reports to make inferences, justify conclusions, and determine appropriateness of data collection methods.
Many selective colleges use a metric called the Academic Index (AI) to assess an application’s strength. The AI is calculated based on GPA and SAT/ACT scores, so you should make sure your scores are competitive to increase your chances of admission. Some colleges even automatically reject applicants with AIs that are too low.
To see how your SAT score compares, use CollegeVine’s free Admissions Chances Calculator . This tool will let you know the impact of your SAT score on your chances and will even offer advice to improve other aspects of your profile.
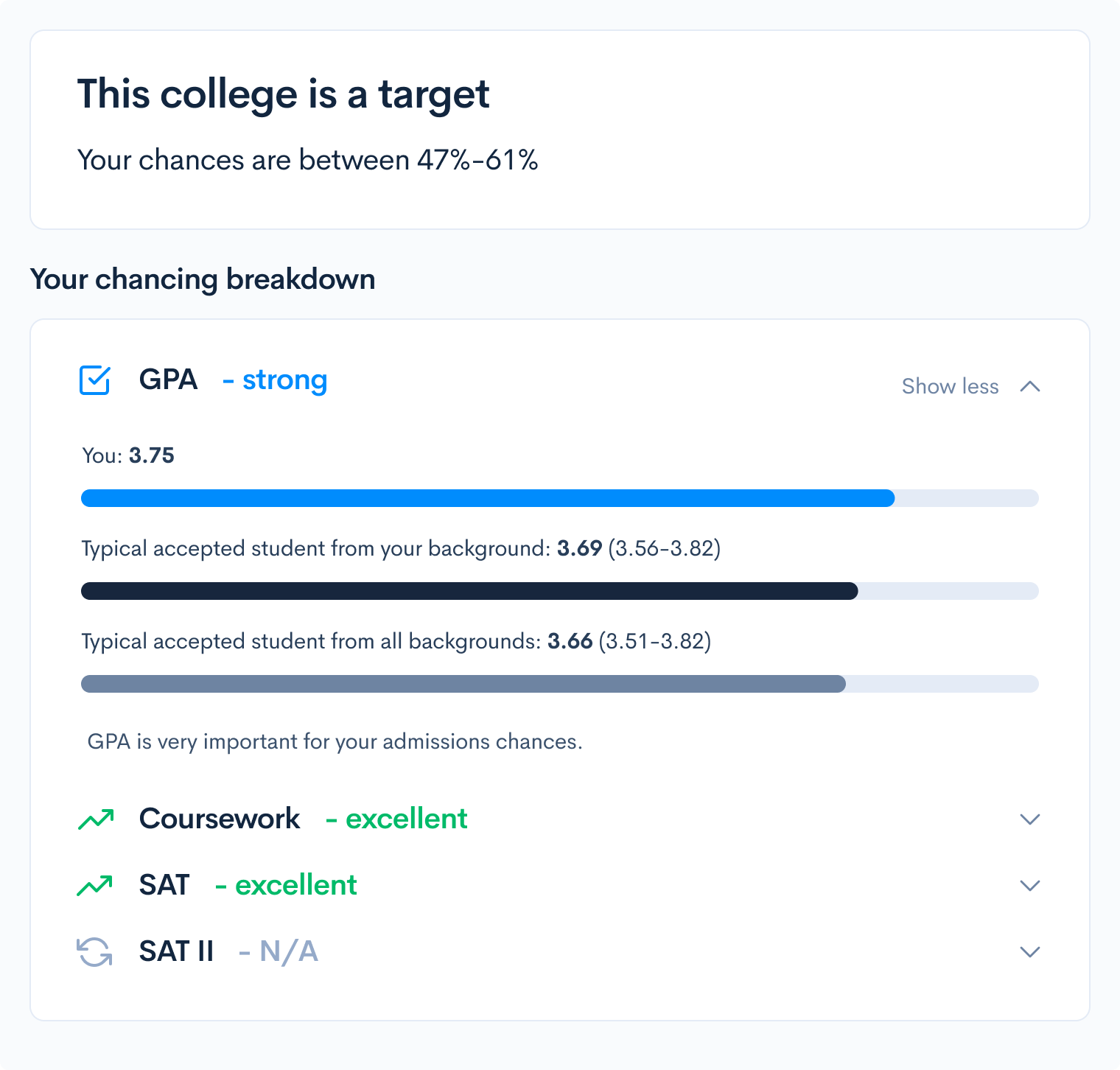
Strategies to Solve Problem Solving and Data Analysis Problems
Problem Solving and Data Analysis problems often involve graphs or data tables, so it’s important to pay attention to titles and labels to make sure you don’t misinterpret the information.
As you read the question, underline or circle any important numeric information. Also, pay close attention to what exactly the question is asking for.
Because Problem Solving and Data Analysis problems vary, there is no concrete algorithm to approach them. These questions are typically more conceptual than calculation-based, so though a calculator is allowed, you probably won’t need it aside from simple arithmetic. Therefore, the key to these problems is reading carefully and knowing concepts like proportions, median, mean, percent increase, etc.
10 Difficult Problem Solving and Data Analysis Questions
Here are some sample difficult Problem Solving and Data Analysis questions and explanations of how to solve them. Remember, these questions only appear on the Calculator section of the exam, so you will have access to a calculator for all of them.
1. Measures of Central Tendency (Mean/Median/Mode)
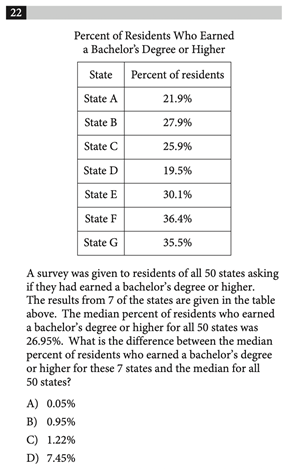
Correct Answer: B
This problem involves computing the median. If you have a graphing calculator, this could be done via lists, but since there are only 7 data values, it might be faster to just write this one out. The median is the measure of the middle of the data set, so start by ordering the values from smallest to largest. This results in the following list: 19.5%, 21.9%, 25.9%, 27.9%, 30.1%, 35.5%, 36.4%. From here, we can clearly see that the middle value is 27.9%, so that is our median.
However, we’re not done here. We now have to compute the difference between the median we just calculated and the median for all 50 states, 26.95%. Subtracting these two values yields 0.95%, which corresponds to answer choice B.
2. Percent Increase
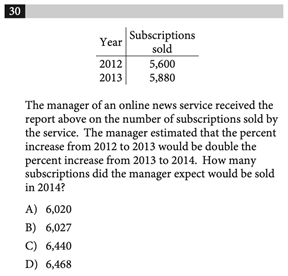
Percent increase can be a tricky concept if you don’t remember this rule of thumb: “new minus old over old.” In this case, for the percent increase from 2012 to 2013, we take the “new” value, 5,880, and subtract the “old” value, 5,600. This is 280, which we then divide by the “old” to get .05, which is 5%.
Since the percent increase from 2012 to 2013 was 5%, and this is double the predicted increase from 2013 to 2014, we know that the percent increase from 2013 to 2014 will be half of 5%, or 2.5%.
Then, to calculate the number of subscriptions sold in 2014, we multiply the value in 2013, 5,880, by 2.5%. This yields 147, which means that in 2014, 147 additional subscriptions were sold. So, the total amount of subscriptions sold in 2014 is 5,880 + 147 = 6,027.
3. Analyzing Graphical Data
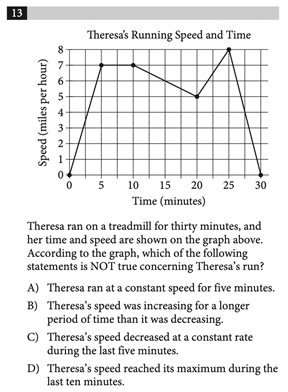
Since we are presented with a graph, let’s make note of what this graph is showing us. On the y-axis we have speed, and on the x-axis we have time. So, this graph is showing us how Theresa’s speed varies with time.
When the graph is flat, the speed is unchanging and is therefore constant. When the graph has a positive slope, the speed is increasing, and when the graph has a negative slope, the speed is decreasing. The rates at which it increases and decreases will be constant since the graph is composed of straight lines (and a line has a constant slope, which means it changes at a constant rate).
For questions asking which statement is not true, it’s crucial to take the time to read through each answer choice. First, choice A states that Theresa ran at a constant speed for five minutes. We can see that this is true, since from 5 to 10 minutes, the graph is flat. Next, choice C says that the speed decreased at a constant rate during the last five minutes. This is also true because from 25 to 30 minutes, the graph is a line with negative slope, which indicates decreasing speed. Finally, choice D claims the maximum speed occurs during the last 10 minutes. We can see that the maximum speed (the highest point on the graph) occurs at 25 minutes, which is within the last 10 minutes, so choice D is also true.
By process of elimination, choice B should be correct, but let’s verify. Choice B states that Theresa’s speed was increasing for a longer time than it was decreasing. Speed was increasing from 0 to 5 and 20 to 25 minutes, for a total of 10 minutes. Speed was decreasing from 10 to 20 and 25 to 30 minutes, for a total of 15 minutes. So, the speed decreased for a longer time than it increased, and choice B is false, making it the correct answer.
4. Inference
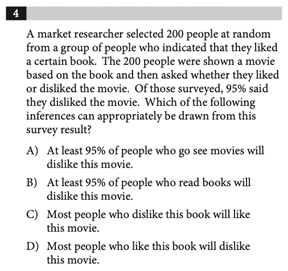
Correct Answer: D
For questions involving surveys, always remember that generalizations can only be made to the specific population studied. For example, if a study is given to a select group of 5th grade math students, when analyzing the results, you can only generalize to 5th grade math students, not all math students or all 5th graders.
In this case, the group surveyed was people who liked the book. From these people, 95% disliked the movie. So, from this survey, most people who like the book will then dislike the movie, which corresponds to choice D.
Choices A and C are incorrect since they generalize to people who see movies and people who dislike the book, which doesn’t apply to the population studied. Choice B is incorrect since it falsely generalizes to all people who read books.
5. Proportions
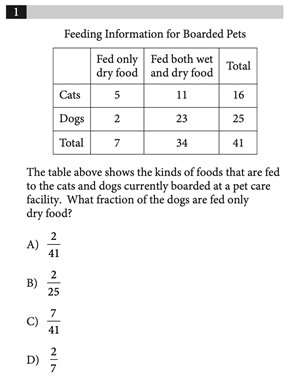
Proportion problems are usually fairly quick, but easy to mess up on if not read carefully. For proportions, the denominator is the total number of things in the group we’re looking at, and the numerator is the specific characteristic we want.
This question asks for the fraction of the dogs that are fed only dry food. So, the group we’re looking at is “dogs” and the characteristic we want is that they “are fed only dry food.”
From the table, the total number of dogs is 25. This means the denominator will be 25. Next, we must find the number of dogs which are also only fed dry food, which is 2, according to the table. So, our numerator is 2, and the answer is 2/25.
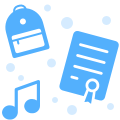
Discover your chances at hundreds of schools
Our free chancing engine takes into account your history, background, test scores, and extracurricular activities to show you your real chances of admission—and how to improve them.
6. Scale Factors
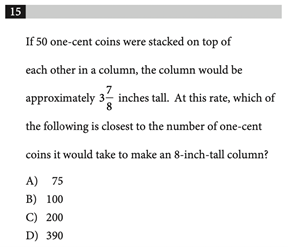
For this question, let’s start with what we know: the column is 8 inches tall. We know that 50 coins creates a \(3\frac{7}{8}\) inch column, which is approximately 4 inches. Since this question asks for an approximation, we know that 8 is slightly more than double \(3\frac{7}{8}\), so we’ll need slightly more than \(50\:\cdot\: 2\) pennies to create an 8-inch column. Answer B is the closest number to our approximate value.
If you wanted to be precise, you could set up an equation:
\(8 in\:\cdot\:\frac{50 coins}{3\frac{7}{8} in}\)
Because 50 coins corresponds to a column which is \(3\frac{7}{8}\) inches tall, we set those two values up in a fraction. We decide which value goes in the numerator and which in the denominator based on the units: since we started with 8 inches, we need the \(3\frac{7}{8}\) inches to be in the denominator so that the inches cancel. Then, we are left with the unit in the numerator, which is coins. The question asks for the number of coins, so this is exactly what we want.
At this point, you would use your calculator to solve the expression, and get about 103 coins. This value is closest to answer choice B.
7. Line of Best Fit/Scatterplots
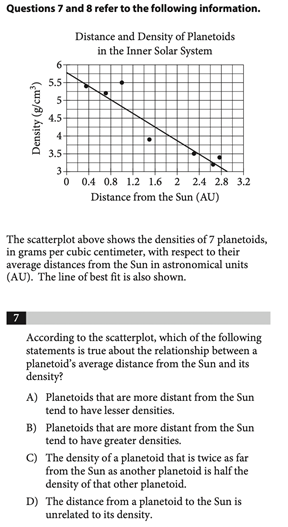
Correct Answer: A
Once again, since we have a graph, let’s take a moment to read the labels. The y-axis shows density and the x-axis shows distance from the sun.
We also see that the line of best fit is sloping downwards. As the distance increases, the density seems to decrease. So, choice A is correct in that larger distances correspond to lesser densities.
Though it wasn’t explicit in this question, an important thing to note about scatterplots is that these relationships show correlation, not causation. Choice C is incorrect because it falsely implies that changes in distance cause changes in density. Choice D is incorrect since though there is no cause and effect relationship, there is a correlation between these two variables.
8. Geometric Applications of Proportions
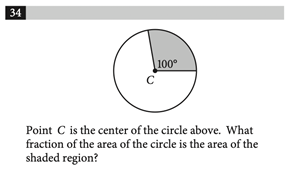
Correct Answer: 5/18, .277, .278
This problem could be confusing in that so little information is given. However, this problem requires that you recall that the proportion of degrees is equivalent to the proportion of area. So, for this problem, all you have to do is divide 100 by 360, which is the total number of degrees in a circle.
Then, the answer is 100/360, or 5/18. If you’re faced with a similar problem on the test, where there is little to no numeric information, try to work with the numbers you do have and find helpful relationships.
9. Unit Conversions
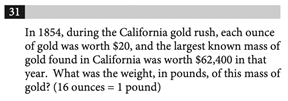
Correct Answer: 195
Unit conversions are fairly simple once you set up the expression correctly. Start with the information given.
For this problem, the price is $62,400, so we will start with this value. Next, we will multiply this value by fractions. Each fraction will consist of a numerator and denominator which are equivalent, so multiplying by these fractions is the same as multiplying by 1. Here is what the expression would look like:
\(\$62,400\:\cdot\:\frac{1 ounce}{\$20}\:\cdot\:\frac{1 pound}{16 ounces}\)
We decide which value to put in the denominator based on the units. In this case, the dollars and the ounces cancel, leaving us with pounds, which is what the question asked for. Solving this expression results in 195 pounds, the answer to the question.
10. Probability
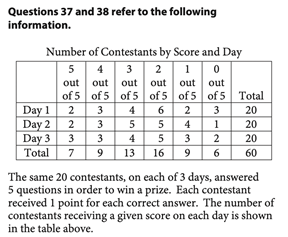
Correct Answer: 5/7, .714
Probability questions are similar to proportion questions in that the denominator should be the group we’re looking at and the numerator should be the characteristic we want.
In this case, it is given that we’re looking at contestants who received a score of 5 on one of the three days, and there is a total of 7 such contestants. The characteristic we want is that the contestant received a score of 5 on Day 2 or Day 3. The number of contestants who fit this description is 2 + 3 = 5, so the probability is 5/7.
For Problem Solving and Data Analysis problems, make sure that your answer addresses what the question asked for. Wrong answer choices on the SAT often reflect common student mistakes, so take the time to read Problem Solving and Data Analysis questions carefully.
When studying for the SAT Math section, try to do plenty of practice problems. The best way to get better at math is to do more math.
Here are some other articles that will help you prepare for the SAT Math section:
- 15 Hardest SAT Math Questions
- 30 SAT Math Formulas You Need to Know
- Guide to SAT Math Heart of Algebra + Practice Questions
- 5 Common SAT Math Mistakes to Avoid
- 5 Tips to Boost Your Math SAT Score
Related CollegeVine Blog Posts
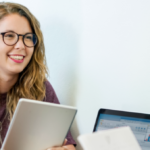
How to analyze a problem
May 7, 2023 Companies that harness the power of data have the upper hand when it comes to problem solving. Rather than defaulting to solving problems by developing lengthy—sometimes multiyear—road maps, they’re empowered to ask how innovative data techniques could resolve challenges in hours, days or weeks, write senior partner Kayvaun Rowshankish and coauthors. But when organizations have more data than ever at their disposal, which data should they leverage to analyze a problem? Before jumping in, it’s crucial to plan the analysis, decide which analytical tools to use, and ensure rigor. Check out these insights to uncover ways data can take your problem-solving techniques to the next level, and stay tuned for an upcoming post on the potential power of generative AI in problem-solving.
The data-driven enterprise of 2025
How data can help tech companies thrive amid economic uncertainty
How to unlock the full value of data? Manage it like a product
Data ethics: What it means and what it takes
Author Talks: Think digital
Five insights about harnessing data and AI from leaders at the frontier
Real-world data quality: What are the opportunities and challenges?
How a tech company went from moving data to using data: An interview with Ericsson’s Sonia Boije
Harnessing the power of external data
Problem Solving and Data Analysis
We have lots of free resources and videos to help you prepare for the SAT. These materials are for the redesigned SAT which is for you if you are taking the SAT in March 2016 and beyond.
Related Pages More Lessons for SAT Math More Resources for SAT
Problem Solving and Data Analysis includes questions that test your ability to
- create a representation of the problem.
- consider the units involved.
- pay attention to the meaning of quantities.
- know and use different properties of mathematical properties and representations.
- apply key principles of statistics.
- estimate the probability of a simple or compound event.
There are many ways that you can be tested and practicing different types of questions will help you to be prepared for the SAT.
The following video lessons will show you how to solve a variety of problem solving and data analysis questions in different situations.
Ratio, Proportion, Units and Percentages
There will be questions on ratios. A ratio represents the proportional relationship between quantities. Fractions can be used to represent ratios.
There will also be questions involving percentages. Percent is a type proportion that means “per 100”.
You will need to convert units when required by the question. One way to perform unit conversion is to write it out as a series of multiplication steps.
Question 1 Question 2 Question 3 Question 4 Question 5 Question 6 Question 7 Question 8
Charts, Graphs and Tables
The questions in Problem Solving and Data Analysis focus on linear, quadratic and exponential relationships which may be represented by charts, graphs or tables. A model is linear if the difference in quantity is constant. A model is exponential if the ratio in the quantity is constant.
A line of best fit is a straight line that best represents the data on a scatterplot. It is written in y = mx + c.
You need to know the formulas for simple and compound interest. Simple Interest: A = P(1 + rt) Compound Interest: A = P(1 + r/n) nt where A is the total amount, P is the principal, r is the interest rate, t is the time period and n is the number of times the interest is compounded per year.
Probability measures how likely an event is. The formula to calculate the probability of an event is: Probability = (number of favorable outcomes)/(total number of possible outcomes)
Question 1 Question 2 Question 3 Question 4 Question 5 Question 6 Question 7 Question 8 Question 9 Question 10 Question 11 Question 12 Question 13 Question 14 Question 15
Data and Statistics
You need to know that mean, median, and mode are measures of center for a data set, while range and standard deviation are measures of spread. You will not be asked to calculate the standard deviation of a set of data, but you do need to understand that a larger standard deviation means that the values are more spread out from the mean. You may be asked to compare the standard deviation of two data sets by approximating the spread from the mean.
You do not need to calculate the margins of error or confidence level, but you do need to know what these concepts are and how to interpret them in context. Take note that the questions in the SAT will always use 95% confidence levels. Some questions may give you the confidence level and ask you to find the value for which the interval applies. When the confidence level is kept the same, the size of the margin of error is affected by the standard deviation and the sample size. The larger the standard deviation, the larger the margin of error. The larger the sample size, the smaller the margin of error. The margin of error and confidence interval are estimates for the entire population and do not apply to values of individual objects in the populations.
The results of a sample can be generalized to the entire population only if the subjects in the sample are selected randomly. Conclusions about cause and effect can appropriately be drawn only if the subjects are randomly assigned to treatment.
Question 1 Question 2 Question 3 Question 4 Question 5 Question 6 Question 7 Question 8 Question 9
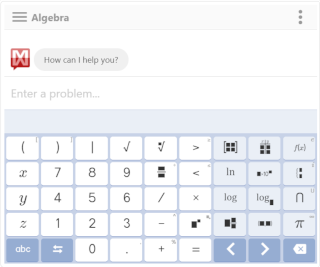
We welcome your feedback, comments and questions about this site or page. Please submit your feedback or enquiries via our Feedback page.
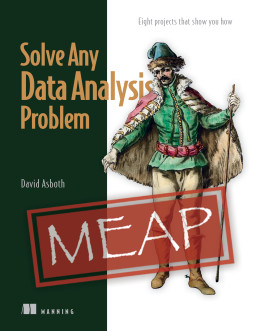
10 of 11 chapters available
Solve Any Data Analysis Problem you own this product $(document).ready(function() { $.ajax({ url: "/ajax/getWishListDetails" }).done(function (data) { if (!jQuery.isEmptyObject(data) && data['wishlistProductIds']) { $(".wishlist-container").each(function() { if (data.wishlistProductIds.indexOf($(this).find('.wishlist-toggle').data('product-id')) > -1) { $(this).addClass("on-wishlist"); } }); } }); $.ajax({ url: "/ajax/getProductOwnershipDetails?productId=3091" }).done(function (data) { if (!jQuery.isEmptyObject(data)) { if (data['ownership']) { $(".wishlist-container").hide(); $(".ownership-indicator").addClass('owned'); $(document.body).addClass("user-owns-product"); } } }); }); document.addEventListener("subscription-status-loaded", function(e){ var status = e && e.detail && e.detail['status']; if(status != "ACTIVE" && status != "PAUSED"){ return; } if(window.readingListsServerVars != null){ $(document).ready(function() { var $readingListToggle = $(".reading-list-toggle"); $(document.body).append(' '); $(document.body).append(' loading reading lists ... '); function adjustReadingListIcon(isInReadingList){ $readingListToggle.toggleClass("fa-plus", !isInReadingList); $readingListToggle.toggleClass("fa-check", isInReadingList); var tooltipMessage = isInReadingList ? "edit in reading lists" : "add to reading list"; $readingListToggle.attr("title", tooltipMessage); $readingListToggle.attr("data-original-title", tooltipMessage); } $.ajax({ url: "/readingList/isInReadingList", data: { productId: 3091 } }).done(function (data) { adjustReadingListIcon(data && data.hasProductInReadingList); }).catch(function(e){ console.log(e); adjustReadingListIcon(false); }); $readingListToggle.on("click", function(){ if(codePromise == null){ showToast() } loadCode().then(function(store){ store.requestReadingListSpecificationForProduct({ id: window.readingListsServerVars.externalId, manningId: window.readingListsServerVars.productId, title: window.readingListsServerVars.title }); ReadingLists.ReactDOM.render( ReadingLists.React.createElement(ReadingLists.ManningOnlineReadingListModal, { store: store, }), document.getElementById("reading-lists-modal") ); }).catch(function(e){ console.log("Error loading code reading list code"); }); }); var codePromise var readingListStore function loadCode(){ if(codePromise) { return codePromise } return codePromise = new Promise(function (resolve, reject){ $.getScript(window.readingListsServerVars.libraryLocation).done(function(){ hideToast() readingListStore = new ReadingLists.ReadingListStore( new ReadingLists.ReadingListProvider( new ReadingLists.ReadingListWebProvider( ReadingLists.SourceApp.marketplace, getDeploymentType() ) ) ); readingListStore.onReadingListChange(handleChange); readingListStore.onReadingListModalChange(handleChange); resolve(readingListStore); }).catch(function(){ hideToast(); console.log("Error downloading reading lists source"); $readingListToggle.css("display", "none"); reject(); }); }); } function handleChange(){ if(readingListStore != null) { adjustReadingListIcon(readingListStore.isInAtLeastOneReadingList({ id: window.readingListsServerVars.externalId, manningId: window.readingListsServerVars.productId })); } } var $readingListToast = $("#reading-list-toast"); function showToast(){ $readingListToast.css("display", "flex"); setTimeout(function(){ $readingListToast.addClass("shown"); }, 16); } function hideToast(){ $readingListToast.removeClass("shown"); setTimeout(function(){ $readingListToast.css("display", "none"); }, 150); } function getDeploymentType(){ switch(window.readingListsServerVars.deploymentType){ case "development": case "test": return ReadingLists.DeploymentType.dev; case "qa": return ReadingLists.DeploymentType.qa; case "production": return ReadingLists.DeploymentType.prod; case "docker": return ReadingLists.DeploymentType.docker; default: console.error("Unknown deployment environment, defaulting to production"); return ReadingLists.DeploymentType.prod; } } }); } });
- MEAP began November 2023
- Publication in Early 2025 ( estimated )
- ISBN 9781633437531
- 325 pages (estimated)
- printed in black & white
- eBook pdf, ePub, online
- print includes eBook
- subscription from $19.99 includes this product
pro $24.99 per month
- access to all Manning books, MEAPs, liveVideos, liveProjects, and audiobooks!
- choose one free eBook per month to keep
- exclusive 50% discount on all purchases
lite $19.99 per month
- access to all Manning books, including MEAPs!
5, 10 or 20 seats+ for your team - learn more
- High-value skills to tackle specific analytical problems
- Deconstructing problems for faster, practical solutions
- Data modeling, PDF data extraction, and categorical data manipulation
- Handling vague metrics, deciphering inherited projects, and defining customer records
about the book
About the reader, about the author, related titles.
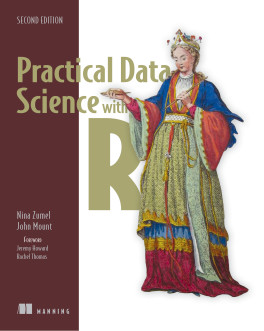
choose your plan
- choose another free product every time you renew
- choose twelve free products per year
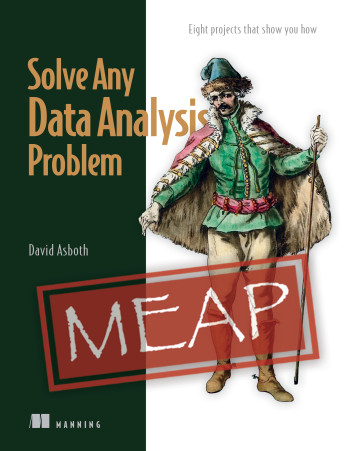
- five seats for your team
- Subscribe to our Newsletter
- Manning on LinkedIn
- Manning on Instagram
- Manning on Facebook
- Manning on Twitter
- Manning on YouTube
- Manning on Twitch
- Manning on Mastodon
how to play
- guess the geekle in 5-, 6-, 7- tries.
- each guess must be a valid 4-6 letter tech word. hit enter to submit.
- after each guess, the color of the tiles will change to show how close your guess was to the word.
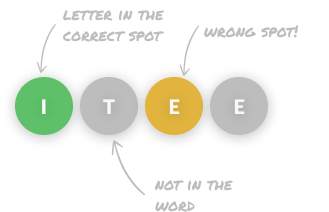
geekle is based on a wordle clone .
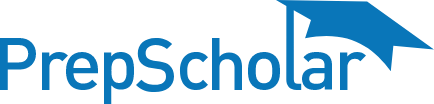
Choose Your Test
- Search Blogs By Category
- College Admissions
- AP and IB Exams
- GPA and Coursework
Problem Solving and Data Analysis: Key SAT Math Concepts
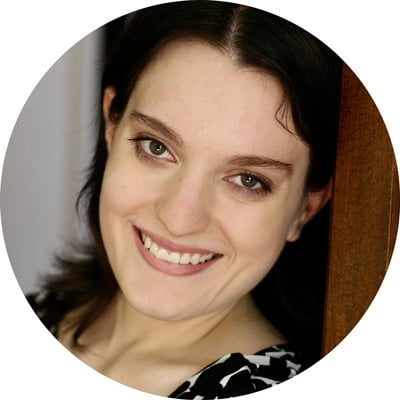
SAT Math is divided intro three domains:
- Heart of Algebra
- Problem Solving and Data Analysis
- Passport to Advanced Math
It's a good idea to get really familiar with what's going to be on the test, where it was derived, and what the SAT is really testing.
This post will focus on one domain— Problem Solving and Data Analysis . This is an opportunity to get cozy with these concepts, and with the overall tapes of information that test-makers are looking for. Problem Solving and Data Analysis problems are all about applying your math knowledge to practical situations and looking at actual statistics instead of abstract, theoretical scenarios.
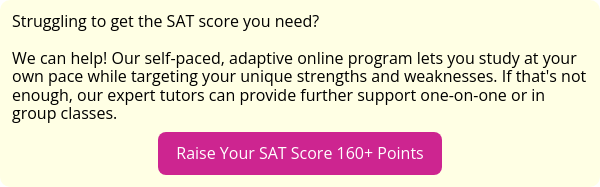
Basic Information
There are 17 questions in this domain (out of 58 total math questions). They aren't labeled or otherwise indicated on the test— you're never told which type of question you're working on.
There are no Problem Solving and Data Analysis problems on the no-calculator section. You will always be permitted to use your approved calculator for questions from this domain —though you may not always need it.
You will receive a subscore on a scale of 1-15 on this domain.
There will be both multiple choice and grid-in questions.
You'll be dealing with both single-step and multistep problems; sometimes, it's just a matter of reading the data and parroting it back, while other problems require a bit more manipulation of the numbers.
General Concepts
The test-makers want to know that you understand math thoroughly enough to use your skills in real-world settings .
Quantitative reasoning is also crucial; you should be able to work with numbers and draw conclusions about what they imply.
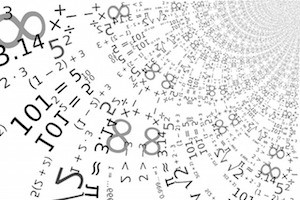
You’ll be working with a lot of numbers.
Real-World Applications
You should be able to build a representation of a problem. If a scenario is described to you, you should be able to model it mathematically by describing it with expressions and equations.
You should know to consider the units involved. If there happens to be a shift of units (from feet to miles, or something like that), you should account for that as you calculate.
You should keep track of the practical meaning of quantities . You're going to be representing real values with variables: don't forget what those variables represent. Also, be sure you understand how a change in one of these variables or quantities affects what's happening in the equation. For example, in a line ($y=mx+b$) with a positive slope (or value of $m$), increasing $x$ will also result in an increase in $y$.
Data and Statistics
There are a lot of graphs, charts, and tables that could be covered on the test. You should be capable of analyzing one-variable data in bar graphs, histograms, line graphs, and box-and-whisker plots—as well as two-variable data in scatterplots and two-way tables. In other words, you should be fluent in reading these various representations of data.
You should be able to describe overall patterns. You'll have to identify positive and negative trends. You should be able to distinguish between linear and exponential growth.
Specific Skills
There are a number of skills that you'll want to be handy with on the day of the exam. In this section you'll find a discussion of these skills, including what they are and what they look like in action.
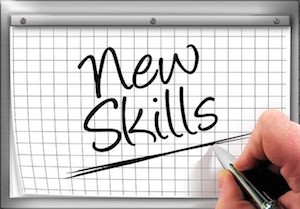
In fact, let’s hope these skills aren’t all that new!
Statistical Analysis
The measures of center are arithmetic mean (average) and median. If they can't be calculated from what's given, you may still need to draw some conclusions about them. Even if you can't find the actual number, there may be a question about what possible values are, or how the values compare to another set of data. Outliers typically affect the mean, but not the median.
The measure of spread to know is standard deviation. You've got to have the basic theory, but you won't need to calculate the exact value. You should be able to look at two sets of data and see which set is more spread out—that is, which has the greater standard deviation.
Insofar as the precision of estimates is concerned, everything depends on the variability of the data and the sample size; smaller variability combined with a larger sample size makes for estimates that are more precise in terms of the actual population.
Another tidbit of interest you should know is that randomization combats bias .
You should, in context, be able to work with margins of error, which are affected by sample size and standard deviation.
Confidence intervals should also be taken into account. The SAT always works with confidence intervals of 95%. This doesn’t mean that 95% of the population is necessarily described by the figure that’s been derived, just that we can be 95% certain that the descriptive figure that’s been reached is accurate.
When analyzing the relationship between two variables, remember, correlation is not causation. If subjects for a sample are selected randomly, we can generalize to the entire population reasonably well. If subjects are randomly assigned to test groups, we can reasonably speculate about cause and effect. Otherwise, though, we’re out of luck.
You should also be able to evaluate reports to make inferences, justify conclusions, and determine the appropriateness of data collection methods .
Take a look at this problem:
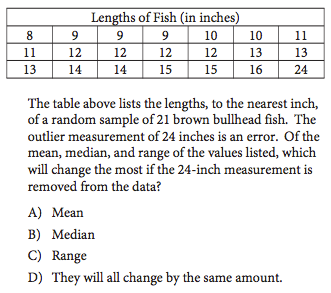
The correct answer here is (C). We know that removing one very high number from the set is not going to affect the median—the middle three values are all 12, so we know the median will still be 12, even if the middle of the data is shifted over one. The mean will shift somewhat if we don’t include the 24-inch measurement, but there are 20 other data points that anchor the mean at a relatively consistent value if any one value is removed. The range, however, will change from $24-8=16$ to $16-8=8$. The range gets cut in half if we remove the 24-inch measurement! That’s the measure that will change the most.
Mathematical Models
The domain of the SAT that we’re examining gives special attention to mathematical models. You must, therefore, be able to create and use a model.
When two variables are presented in a graph, table, or other chart , you should be handy with analyzing and drawing conclusions with regards to the relationship between these variables.
Relationships between variables can be modeled by functions, but remember the function is only a model! It may give scientifically accurate predictions, or it may just describe a general trend. You may be asked whether a model is good, acceptable, or entirely inappropriate.
Let’s look at this problem:
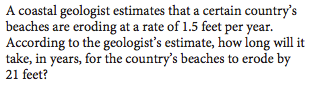
The geologist in the question provides a model regarding the country’s beach erosion. According to that model, beaches erode at a rate of 1.5 feet per year. 21 feet of erosion would therefore take 14 years, as $14(1.5)=21$.
The functions you’re asked to work with may be linear, quadratic, and/or exponential. Linear and exponential are discussed in more detail below.
An important example of linear growth is simple interest , where you earn interest on your principal, each period, but not on any interest that has been added since that first deposit. This is modeled by the function: $A = P(1+rt)$. $P$ is the principal, $r$ is the interest rate, and $t$ is the amount of time interest has been accruing.
Exponential
An important example of exponential growth is compound interest , where you earn interest on the interest you’ve previously earned. This is modeled by the function: $A = P (1+r/n)^{nt}$, where $P$ is the principal, $r$ is the interest rate (typically annual), $n$ is the number of times the interest compounds per period (typically a year), and $t$ is the amount of time that has passed since the principal began accruing interest.
Be careful! The stated rate of change may not be the same as the rate of change over time. This is typical of compound interest:
- You might take a loan at 9%, but if it compounds monthly, you’re really taking a loan at $(1+.09/12)^12 – 1 = 9.38%$ at the end of the year.
- On the other hand, you might make a deposit that accrues interest at a rate of 5%, but it compounds quarterly, so you’re really getting $(1+.05/4)^4 – 1 = 5.095%$ at the end of the year.
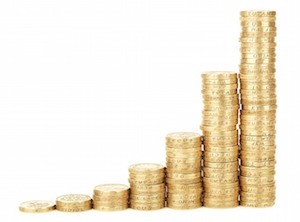
Math and money are very closely linked.
Ratios, Proportions, Units, and Percentages
You’ll need to be familiar with direct proportionality/variation : $y = kx$, where $k$ is a unitless constant of proportion. This relationship may also be expressed as $x_1/y_1 = x_2/y_2$.
You’ll need to know how percent increase and percent decrease work. Be careful about how you approach these problems; they can get a little tricky. Remember, for instance, that if you have a 20%-off coupon for an item that’s on a 20%-off sale, you won’t save 40%. You’ll save 36%, as you’ll pay 80% of 80% of the original price: $x(.8)(.8) = (.64)x = x - (.36)x$.
Take a look at this pair of problems:
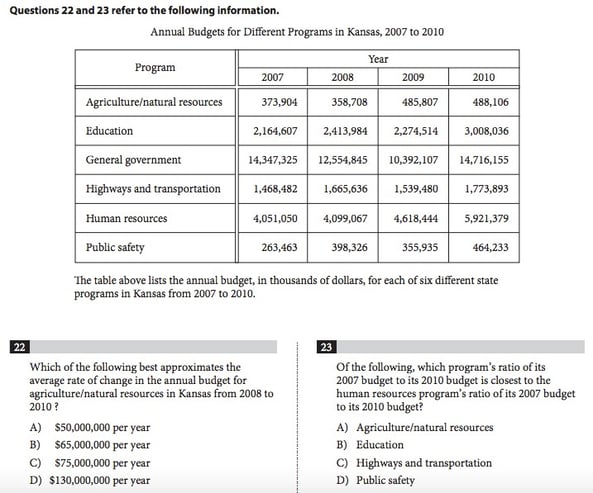
This is the sort of situation where you’re asked to deal with quantities in very practical terms. These aren’t just numbers floating in and out of an abstract function ; no, these numbers represent that annual budget, in thousands of dollars, for each of six different state programs in Kansas from 2007 to 2010.
The first problem is asking for the approximate average rate of change in the annual budget for agriculture/natural resources in Kansas from 2008 to 2010. From 2008 to 2009, the budget grew by 127,099 thousands of dollars, or $127,099,000. From 2009 to 2010, the budget grew by 2,299 thousands of dollars, or $2,299,000. Thus, we add those two figures together, divide by two, and find that the average growth was $64,699,000, or, when rounding, (B).
For the next problem, we are comparing the 2007-budget-to-2010-budget ratios across the various programs. We will first need to find those ratios:
Agriculture/natural resources — $373,904/488,106=.766$
Education — $2,164,607/3,008,036=.7196$
Highways and transportation — $1,468,482/1,773,893=.8278$
Public safety — $263,463/464,233=.5675$
Out of these, the closest to human resources ($4,051,050/5,921,379=.6841$), is (B).
- Probability
Two events are independent if one happening has nothing to do with another , like the sun shining and you eating a sandwich for lunch. The sun may shine, and you may eat a sandwich for lunch, but one does not cause or prevent the other.
Two events are mutually exclusive if they cannot both occur , like me wearing a hat and me not wearing a hat. I can’t do both.
For independent, non-mutually exclusive events: P(A and B) = P(A)*P(B), whereas P(A or B) = P(A) + P(B) - P(A and B).
For mutually exclusive events: P(A or B) = P(A) + P(B).
There are other formulas for more complicated scenarios, but these will get you pretty far — they’re all you should need on the SAT.
Let’s take a look at this problem:
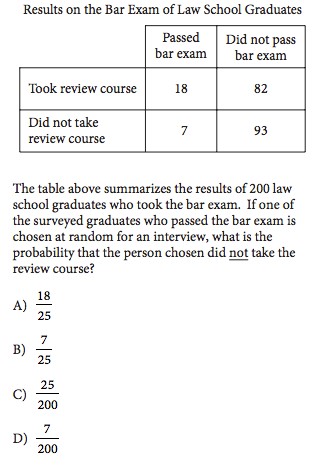
Twenty-five people passed the bar exam; of these, seven did not take the review course. So, the probability that the interviewed person in question did not take the bar exam is 7/25, or (B).
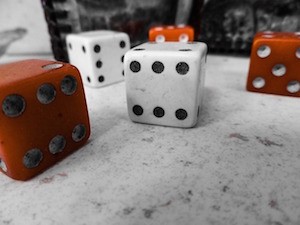
Math and gambling are closely linked, too. It’s all about that probability.
This domain of the test is calculation-heavy, although there are some theoretical questions.
These questions compose almost a third of the test . They cover, roughly:
- Statistical analysis
- Proportions
- Real-world data
Your answers will be scored to yield one of three subscores for the Math section.
What’s Next?
That was a fair amount of information. Digest it a little; then, a great next stop would be perusing our overall guide to SAT Math , including directions to a number of other great posts .
Now, because Problem Solving and Data Analysis problems are so information-heavy , you may wish to practice with some SAT word problems to get used to that much verbal data being thrown your way.
As you’re trying these practice problems, you’ll want to know how to use them to your best advantage .
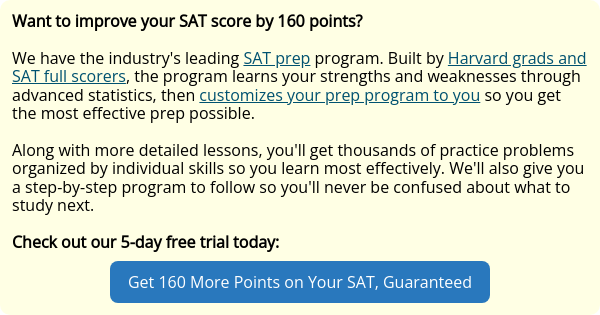
Trending Now
How to Get Into Harvard and the Ivy League
How to Get a Perfect 4.0 GPA
How to Write an Amazing College Essay
What Exactly Are Colleges Looking For?
ACT vs. SAT: Which Test Should You Take?
When should you take the SAT or ACT?
Get Your Free
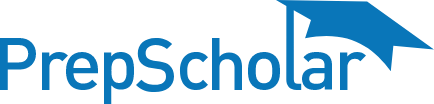
Find Your Target SAT Score
Free Complete Official SAT Practice Tests
How to Get a Perfect SAT Score, by an Expert Full Scorer
Score 800 on SAT Math
Score 800 on SAT Reading and Writing
How to Improve Your Low SAT Score
Score 600 on SAT Math
Score 600 on SAT Reading and Writing
Find Your Target ACT Score
Complete Official Free ACT Practice Tests
How to Get a Perfect ACT Score, by a 36 Full Scorer
Get a 36 on ACT English
Get a 36 on ACT Math
Get a 36 on ACT Reading
Get a 36 on ACT Science
How to Improve Your Low ACT Score
Get a 24 on ACT English
Get a 24 on ACT Math
Get a 24 on ACT Reading
Get a 24 on ACT Science
Stay Informed
Get the latest articles and test prep tips!
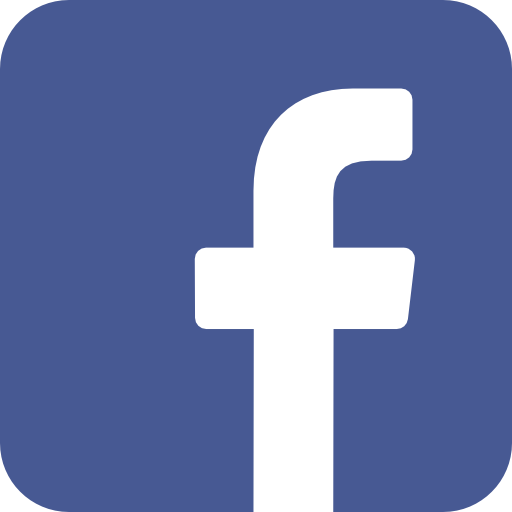
Vero is a firsthand expert at standardized testing and the college application process. Though neither parent had graduated high school, and test prep was out of the question, she scored in the 99th percentile on both the SAT and ACT, taking each test only once. She attended Dartmouth, graduating as salutatorian of 2013. She later worked as a professional tutor. She has a great passion for the arts, especially theater.
Ask a Question Below
Have any questions about this article or other topics? Ask below and we'll reply!
- Business Essentials
- Leadership & Management
- Credential of Leadership, Impact, and Management in Business (CLIMB)
- Entrepreneurship & Innovation
- Digital Transformation
- Finance & Accounting
- Business in Society
- For Organizations
- Support Portal
- Media Coverage
- Founding Donors
- Leadership Team
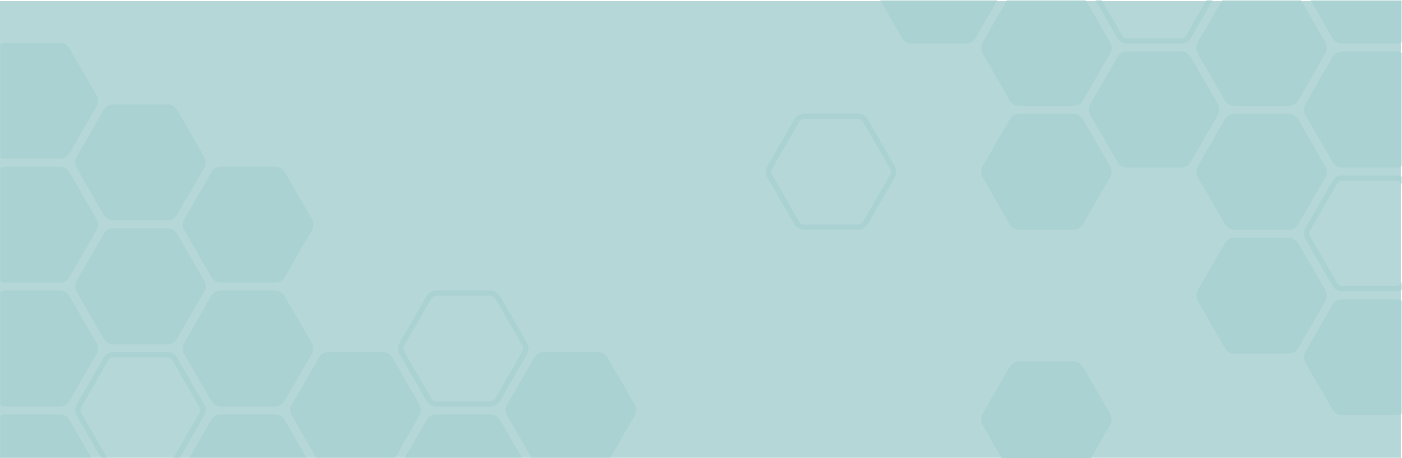
- Harvard Business School →
- HBS Online →
- Business Insights →
Business Insights
Harvard Business School Online's Business Insights Blog provides the career insights you need to achieve your goals and gain confidence in your business skills.
- Career Development
- Communication
- Decision-Making
- Earning Your MBA
- Negotiation
- News & Events
- Productivity
- Staff Spotlight
- Student Profiles
- Work-Life Balance
- AI Essentials for Business
- Alternative Investments
- Business Analytics
- Business Strategy
- Business and Climate Change
- Creating Brand Value
- Design Thinking and Innovation
- Digital Marketing Strategy
- Disruptive Strategy
- Economics for Managers
- Entrepreneurship Essentials
- Financial Accounting
- Global Business
- Launching Tech Ventures
- Leadership Principles
- Leadership, Ethics, and Corporate Accountability
- Leading Change and Organizational Renewal
- Leading with Finance
- Management Essentials
- Negotiation Mastery
- Organizational Leadership
- Power and Influence for Positive Impact
- Strategy Execution
- Sustainable Business Strategy
- Sustainable Investing
- Winning with Digital Platforms
4 Ways to Improve Your Analytical Skills
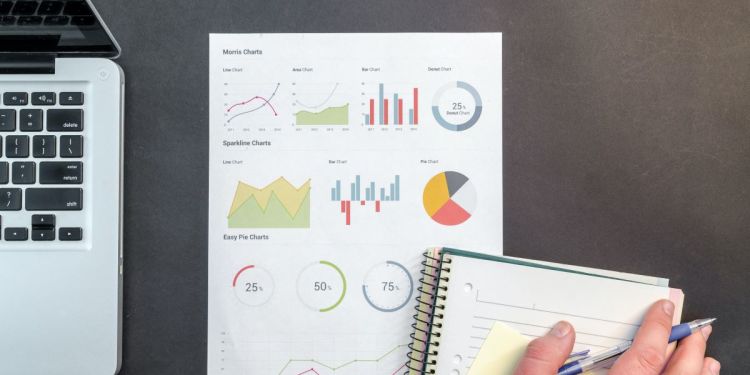
- 07 Jan 2021
Data is ubiquitous. It’s collected at every purchase made, flight taken, ad clicked, and social media post liked—which means it’s never been more crucial to understand how to analyze it.
“Never before has so much data about so many different things been collected and stored every second of every day,” says Harvard Business School Professor Jan Hammond in the online course Business Analytics .
The volume of data you encounter can be overwhelming and raise several questions: Can I trust the data’s source? Is it structured in a way that makes sense? What story does it tell, and what actions does it prompt?
Data literacy and analytical skills can enable you to answer these questions and not only make sense of raw data, but use it to drive impactful change at your organization.
Here’s a look at what it means to be data literate and four ways to improve your analytical skills.
Access your free e-book today.
What Is Data Literacy?
Data literacy is the ability to analyze, interpret, and question data. A dataset is made up of numerous data points that, when viewed together, tell a story.
Before conducting an analysis, it’s important to ensure your data’s quality and structure is in accordance with your organization’s needs.
“In order to transform data into actionable information, you first need to evaluate its quality,” says Professor Dustin Tingley in the Harvard Online course Data Science Principles . “But evaluating the quality of your data is just the first step. You’ll also need to structure your data. Without structure, it’s nearly impossible to extract any information.”
When you’re able to look at quality data, structure it, and analyze it, trends emerge. The next step is to reflect on your analysis and take action.
Tingley shares several questions to ask yourself once you’ve analyzed your dataset: “Did all the steps I took make sense? If so, how should I respond to my analysis? If not, what should I go back and improve?”
For example, you may track users who click a button to download an e-book from your website.
After ensuring your data’s quality and structuring it in a way that makes sense, you begin your analysis and find that a user’s age is positively correlated with their likelihood to click. What story does this trend tell? What does it say about your users, product offering, and business strategy?
To answer these questions, you need strong analytical skills, which you can develop in several ways.
Related: Business Analytics: What It Is & Why It’s Important
How to Improve Your Analytical Skills
Analysis is an important skill to have in any industry because it enables you to support decisions with data, learn more about your customers, and predict future trends.
Key analytical skills for business include:
- Visualizing data
- Determining the relationship between two or more variables
- Forming and testing hypotheses
- Performing regressions using statistical programs, such as Microsoft Excel
- Deriving actionable conclusions from data analysis
If you want to provide meaningful conclusions and data-based recommendations to your team, here are four ways to bolster your analytical skills.
Related: How to Learn Business Analytics Without A Business Background
1. Consider Opposing Viewpoints
While engaging with opposing viewpoints can help you expand your perspective, combat bias, and show your fellow employees their opinions are valued, it can also be a useful way to practice analytical skills.
When analyzing data, it’s crucial to consider all possible interpretations and avoid getting stuck in one way of thinking.
For instance, revisit the example of tracking users who click a button on your site to download an e-book. The data shows that the user’s age is positively correlated with their likelihood to click the button; as age increases, downloads increase, too. At first glance, you may interpret this trend to mean that a user chooses to download the e-book because of their age.
This conclusion, however, doesn’t take into consideration the vast number of variables that change with age. For instance, perhaps the real reason your older users are more likely to download the e-book is their higher level of responsibility at work, higher average income, or higher likelihood of being parents.
This example illustrates the need to consider multiple interpretations of data, and specifically shows the difference between correlation (the trending of two or more variables in the same direction) and causation (when a trend in one variable causes a trend to occur in one or more other variables).
“Data science is built on a foundation of critical thinking,” Tingley says in Data Science Principles . “From the first step of determining the quality of a data source to determining the accuracy of an algorithm, critical thinking is at the heart of every decision data scientists—and those who work with them—make.”
To practice this skill, challenge yourself to question your assumptions and ask others for their opinions. The more you actively engage with different viewpoints, the less likely you are to get stuck in a one-track mindset when analyzing data.
2. Play Games or Brain Teasers
If you’re looking to sharpen your skills on a daily basis, there are many simple, enjoyable ways to do so.
Games, puzzles, and stories that require visualizing relationships between variables, examining situations from multiple angles, and drawing conclusions from known data points can help you build the skills necessary to analyze data.
Some fun ways to practice analytical thinking include:
- Crossword puzzles
- Mystery novels
- Logic puzzles
- Strategic board games or card games
These options can supplement your analytics coursework and on-the-job experience. Some of them also allow you to spend time with friends or family. Try engaging with one each day to hone your analytical mindset.
Related: 3 Examples of Business Analytics in Action
3. Take an Online Analytics Course
Whether you want to learn the basics, brush up on your skills, or expand your knowledge, taking an analytics course is an effective way to improve. A course can enable you to focus on the content you want to learn, engage with the material presented by a professional in the field, and network and interact with others in the data analytics space.
For a beginner, courses like Harvard Online's Data Science Principles can provide a foundation in the language of data. A more advanced course, like Harvard Online's Data Science for Business , may be a fit if you’re looking to explore specific facets of analytics, such as forecasting and machine learning. If you’re interested in hands-on applications of analytical formulas, a course like HBS Online's Business Analytics could be right for you. The key is to understand what skills you hope to gain, then find a course that best fits your needs.
If you’re balancing a full-time job with your analytics education, an online format may be a good choice . It offers the flexibility to engage with course content whenever and wherever is most convenient for you.
An online course may also present the opportunity to network and build relationships with other professionals devoted to strengthening their analytical skills. A community of like-minded learners can prove to be an invaluable resource as you learn and advance your career.
Related: Is An Online Business Analytics Course Worth It?
4. Engage With Data
Once you have a solid understanding of data science concepts and formulas, the next step is to practice. Like any skill, analytical skills improve the more you use them.
Mock datasets—which you can find online or create yourself—present a low-risk option for putting your skills to the test. Import the data into Microsoft Excel, then explore: make mistakes, try that formula you’re unsure of, and ask big questions of your dataset. By testing out different analyses, you can gain confidence in your knowledge.
Once you’re comfortable, engage with your organization’s data. Because these datasets have inherent meaning to your business's financial health, growth, and strategic direction, analyzing them can produce evidence and insights that support your decisions and drive change at your organization.

Investing in Your Data Literacy
As data continues to be one of businesses’ most valuable resources, taking the time and effort to build and bolster your analytical skill set is vital.
“Much more data are going to be available; we’re only seeing the beginning now,” Hammond says in a previous article . “If you don’t use the data, you’re going to fall behind. People that have those capabilities—as well as an understanding of business contexts—are going to be the ones that will add the most value and have the greatest impact.”
Are you interested in furthering your data literacy? Download our Beginner’s Guide to Data & Analytics to learn how you can leverage the power of data for professional and organizational success.

About the Author
- Understanding Classroom Layout and Design
- Differentiated Instruction: A Comprehensive Overview
- Physical Science Lesson Plans for 6-8 Learners
- Active Learning: A Comprehensive Overview
- Classroom Management
- Behavior management techniques
- Classroom rules
- Classroom routines
- Classroom organization
- Assessment Strategies
- Summative assessment techniques
- Formative assessment techniques
- Portfolio assessment
- Performance-based assessment
- Teaching Strategies
- Active learning
- Inquiry-based learning
- Differentiated instruction
- Project-based learning
- Learning Theories
- Behaviorism
- Social Learning Theory
- Cognitivism
- Constructivism
- Critical Thinking Skills
- Analysis skills
- Creative thinking skills
- Problem-solving skills
- Evaluation skills
- Metacognition
- Metacognitive strategies
- Self-reflection and metacognition
- Goal setting and metacognition
- Teaching Methods and Techniques
- Direct instruction methods
- Indirect instruction methods
- Lesson Planning Strategies
- Lesson sequencing strategies
- Unit planning strategies
- Differentiated Instruction Strategies
- Differentiated instruction for English language learners
- Differentiated instruction for gifted students
- Standards and Benchmarks
- State science standards and benchmarks
- National science standards and benchmarks
- Curriculum Design
- Course design and alignment
- Backward design principles
- Curriculum mapping
- Instructional Materials
- Textbooks and digital resources
- Instructional software and apps
- Engaging Activities and Games
- Hands-on activities and experiments
- Cooperative learning games
- Learning Environment Design
- Classroom technology integration
- Classroom layout and design
- Instructional Strategies
- Collaborative learning strategies
- Problem-based learning strategies
- 9-12 Science Lesson Plans
- Life science lesson plans for 9-12 learners
- Earth science lesson plans for 9-12 learners
- Physical science lesson plans for 9-12 learners
- K-5 Science Lesson Plans
- Earth science lesson plans for K-5 learners
- Life science lesson plans for K-5 learners
- Physical science lesson plans for K-5 learners
- 6-8 Science Lesson Plans
- Earth science lesson plans for 6-8 learners
- Life science lesson plans for 6-8 learners
- Physical science lesson plans for 6-8 learners
- Science Learning
- Analysis Skills: Understanding Critical Thinking and Science Learning
This article provides an overview of the analysis skills necessary for critical thinking and science learning. It offers tips and strategies to help readers develop their analysis skills.

Having strong analysis skills is essential for success in any field, whether it's science, business, or any other field. Critical thinking and science learning are two key components of having these skills. In order to become an expert in any field, one must be able to analyze information and make informed decisions. This article will explore the importance of analysis skills and how to develop them through critical thinking and science learning.
Additionally, it will discuss the benefits of seeking help from Profs online stata tutors to further hone these skills. Critical thinking is the process of making rational judgments about a situation or problem. It involves gathering facts, weighing evidence, and forming conclusions based on what you learn. Science learning refers to the process of acquiring knowledge about scientific theories, experiments, and discoveries. Both of these skills are necessary for gaining a thorough understanding of any topic. This article will explain why it is important to develop both analysis skills and critical thinking.
It will also provide tips on how to use critical thinking and science learning to enhance your analysis skills. Finally, it will discuss the importance of using both skills together in order to achieve better results. Analysis skills are essential for critical thinking and science learning. They involve the ability to think logically, break down problems into smaller parts, identify patterns and relationships, and use data to draw conclusions. Analytical thinking is the process of examining information, breaking it down into smaller components, and understanding how the components are related.
Problem-solving skills involve using a systematic approach to identify solutions to complex problems. Data analysis involves gathering, organizing, and analyzing data in order to draw meaningful conclusions. Having strong analysis skills is important for making informed decisions, solving complex problems, and understanding complex topics. It can help you identify potential consequences of actions, develop alternative solutions, and think critically about different perspectives.
It can also be beneficial when learning new topics or researching different areas of study. There are several strategies that you can use to improve your analysis skills. To improve analytical thinking, try breaking down complex problems into smaller parts and using data to identify patterns or relationships. For problem-solving skills, try brainstorming potential solutions and using a systematic approach to evaluate the options.
To improve data analysis skills, practice organizing data into meaningful categories and interpreting the data to draw meaningful conclusions. Analysis skills can be applied in various contexts. In business, data analysis can help identify trends and make informed decisions. Analytical thinking can be used to solve complex problems or develop new products or services.
In science, problem-solving skills can be used to explore new ideas or research topics. There are a variety of resources available to help you further develop your analysis skills. Books such as The Art of Analyzing Data by John DeKok provide an introduction to data analysis and how to use data for decision-making. Online courses such as Analytical Thinking & Problem Solving by edX provide an in-depth exploration of how to develop problem-solving skills.
Analytical thinking
Data analysis requires the ability to collect, organize, and interpret data in order to draw meaningful conclusions. Having strong analysis skills is important for a variety of reasons. They can help us make better decisions by allowing us to evaluate different options and make informed choices. They can also help us understand complex topics by breaking them down into more manageable pieces.
Finally, they can help us solve complex problems by allowing us to identify patterns, draw connections between different elements, and develop creative solutions. To develop analysis skills, it is important to practice analytical thinking, problem-solving, and data analysis. To improve analytical thinking, it is helpful to break down problems into smaller parts and look for patterns or connections between different elements. To improve problem-solving skills, it is helpful to brainstorm potential solutions and use a systematic approach when evaluating them.
To improve data analysis skills, it is important to collect relevant data, organize it in a meaningful way, and interpret it accurately. Analysis skills can be applied in a variety of contexts. In business, for example, data analysis can be used to identify trends or insights about customer behavior . Analytical thinking can be used to evaluate complex problems and develop creative solutions.
Problem-solving skills can be used to develop new products or services or find ways to improve existing ones. In science learning, analysis skills are essential for understanding complex topics and developing hypotheses that can be tested through experimentation. There are many resources available for those interested in further developing their analysis skills. Books such as The Art of Analytical Thinking , Data Analysis for Beginners , and The Power of Problem-Solving provide useful tips and strategies for improving analytical thinking, problem-solving, and data analysis.
Examples of Analysis Skills
Analytical thinking:, problem-solving skills:, why are analysis skills important, what are analysis skills.
It involves breaking down a problem into its component parts, examining each part in detail, and then using evidence-based logic to solve the problem. Problem-solving is another type of analysis skill that involves identifying the root cause of a problem and developing an effective strategy to address it. Data analysis is another type of analysis skill that involves collecting, analyzing, and interpreting data in order to draw meaningful conclusions. Analysis skills are important because they enable a person to think critically and make informed decisions. These skills are also essential for science learning, as they enable a person to analyze data, identify patterns, and draw conclusions.
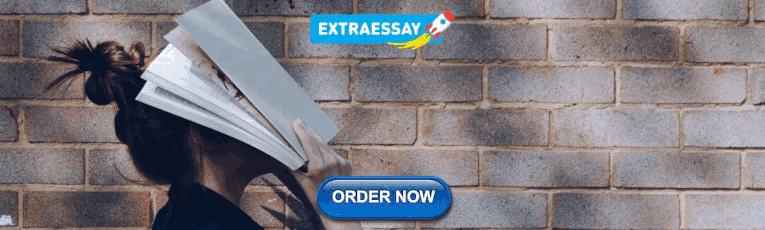
How to Develop Analysis Skills
Using data:, systematic approach:, resources for developing analysis skills.
Some books that may be of particular interest include “Analytical Thinking: A Guide to Critical Thinking and Problem Solving” by Paul C. Nuttal, “The Analytic Thinker: Mastering the Skills of Reasoning, Analysis, and Critique” by Michael Starbird, and “The Art of Thinking Clearly: Better Thinking, Better Decisions” by Rolf Dobelli. Online courses can also be a great resource for developing analysis skills. Coursera, edX, and Udemy offer a wide range of courses on analytical thinking, problem-solving, and critical thinking.
These courses can provide an in-depth look at the fundamentals of analytical thinking and how it can be applied in different contexts. Websites can also be a great source of information on analytical thinking and problem-solving. There are many sites dedicated to the subject, such as the Cognitive Science Lab at Stanford University, which offers resources on cognitive psychology, artificial intelligence, and more. The website Thinking Critically by Peter Facione offers a wealth of information on critical thinking, including tips and strategies for developing analysis skills.
This allows for more informed decision-making and analysis. Additionally, analyzing data can help identify patterns and draw conclusions from the information. When looking for patterns, it is important to consider the context in which the data was gathered and how it relates to the overall problem. To improve problem-solving skills, brainstorming can be an effective technique. Brainstorming involves coming up with multiple ideas, without judging them, and then assessing their viability.
Books can be an excellent resource for developing analytical thinking skills. Popular titles include Thinking, Fast and Slow by Daniel Kahneman, The Art of Thinking Clearly by Rolf Dobelli, Thinking Critically by John Chaffee, and The Power of Thinking Differently by Edward de Bono. Online courses are also available to help readers better understand analytical thinking. Popular options include Coursera's Introduction to Logic and Critical Thinking , edX's Analytical Thinking: A Strategic Skill for Leaders , and Udemy's Data Analysis & Visualization in R: Analytical Thinking . Websites can also provide helpful resources for developing analytical thinking skills.
Sites like Khan Academy, which offers free tutorials on topics like logic and problem solving, as well as websites like Brilliant.org, which offers free online courses on a variety of topics, can be great resources for honing analytical skills. Finally, there are many other materials that readers can use to further develop their analytical thinking skills. These can include podcasts, videos, articles, and more. All of these resources can provide insight into different aspects of analytical thinking and help readers hone their skills. Analysis skills are essential for critical thinking and science learning. This article has provided an overview of what analysis skills are, why they are important, and tips and strategies for developing these skills.

Shahid Lakha
Shahid Lakha is a seasoned educational consultant with a rich history in the independent education sector and EdTech. With a solid background in Physics, Shahid has cultivated a career that spans tutoring, consulting, and entrepreneurship. As an Educational Consultant at Spires Online Tutoring since October 2016, he has been instrumental in fostering educational excellence in the online tutoring space. Shahid is also the founder and director of Specialist Science Tutors, a tutoring agency based in West London, where he has successfully managed various facets of the business, including marketing, web design, and client relationships. His dedication to education is further evidenced by his role as a self-employed tutor, where he has been teaching Maths, Physics, and Engineering to students up to university level since September 2011. Shahid holds a Master of Science in Photon Science from the University of Manchester and a Bachelor of Science in Physics from the University of Bath.
New Articles

- Indirect Instruction Methods: A Comprehensive Overview
This guide provides an in-depth overview of indirect instruction methods, including their applications and benefits.

- Backward Design Principles: Understanding Curriculum Design
This article explains the concept of backward design principles and how they are used in curriculum design.

- Exploring Self-Reflection and Metacognition
This article explores the meaning of self-reflection and metacognition, and how they are related to learning.

- Integrating Technology into the Classroom
This article provides an overview of classroom technology integration and how it can improve learning experiences in the classroom.
Leave Reply
Your email address will not be published. Required fields are marked *
I agree that spam comments wont´t be published
- Behavior Management Techniques
- Behaviorism: A Comprehensive Overview
- Social Learning Theory Explained
- Summative Assessment Techniques: An Overview
- Formative Assessment Techniques
- Inquiry-Based Learning: An Introduction to Teaching Strategies
- Understanding Cognitivism: A Learning Theory
- Creative Thinking Skills
- Constructivism: Exploring the Theory of Learning
- Problem-solving Skills: A Comprehensive Overview
- Classroom Rules - A Comprehensive Overview
- Exploring Portfolio Assessment: An Introduction
- Evaluation Skills: A Comprehensive Overview
- Classroom Routines: A Comprehensive Overview
Effective Classroom Organization Strategies for Science Teaching
- Project-Based Learning: An In-Depth Look
- Performance-Based Assessment: A Comprehensive Overview
- Understanding Direct Instruction Methods
- State Science Standards and Benchmarks
- Course Design and Alignment
- The Advantages of Textbooks and Digital Resources
- Engaging Hands-on Activities and Experiments
An Overview of Metacognitive Strategies
- Engaging Cooperative Learning Games
- Lesson Sequencing Strategies: A Comprehensive Overview
- Instructional Software and Apps: A Comprehensive Overview
- Understanding Curriculum Mapping
- Collaborative Learning Strategies
- Understanding National Science Standards and Benchmarks
- Exploring Problem-Based Learning Strategies
- Unit Planning Strategies
- Exploring Goal Setting and Metacognition
- Life Science Lesson Plans for 9-12 Learners
- Earth Science Lesson Plans for K-5 Learners
- Differentiated Instruction for English Language Learners
- Life Science Lesson Plans for K-5 Learners
- Earth Science Lesson Plans for 6-8 Learners
- Earth Science Lesson Plans for 9-12 Learners
- Life Science Lesson Plans for 6-8 Learners
Physical Science Lesson Plans for 9-12 Learners
- Physical Science Lesson Plans for K-5 Learners
- Differentiated Instruction Strategies for Gifted Students
Recent Posts

Which cookies do you want to accept?
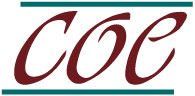
- Integrated Approach
- Testimonials
- Business Process Reengineering
- Career Development
- Competency Development
Data Analytics
- Innovation Strategy
- Innovation Readiness Survey (IRS)
- Innovative Leader Survey (ILS)
- Exploring Innovation
- Lean Six Sigma Methodology
- Lean Six Sigma and Data Analytics
- Strategic Workforce Planning
- iiP – Investing in People
- Innovation and Change
- Innovation and Productivity
- Lean Six Sigma
- Lean Innovation Training (LIT)
- Articles and Case Studies
- Data Analytics for OD
- Lean Six Sigma Nuggets
- Leadership Competency Cards
- Lean Innovation Tool Kit
- Bridging Cards
- Data Science für Einsteiger
- Praxisbuch Lean Six Sigma
- Data Analytics with R
- Sign up for Newsletter
Introducing Data Analytics and Data Science into Your Organisation with Carefully Crafted Solutions.
“I only believe in statistics that I doctored myself.” Winston Churchill
Data analytics, or data analysis , is the process of screening, cleaning, transforming, and modeling data with the objective of discovering useful information, suggesting conclusions, and supporting problem solving as well as decision making. There are multiple approaches, including a variety of techniques and tools used for data analytics. Data analytics finds applications in many different environments. As such, it usually covers two steps, graphical analysis and statistical analysis. The selection of tools for a given data analytics task depends on the overall objective, the source and types of data given.
Above all, Data Analytics, as part of Data Science, marks the foundation of all disciplines that are part of Artificial Intelligence (AI).
Objectives of Data Analytics
The objective of the data analytics task can be to screen or inspect the data in order to find out whether the data fulfils certain requirements. These requirements can be a certain distribution, a certain homogeneity of the dataset (no outliers) or just the behaviour of the data under certain stratification conditions (using demographics).
More often than not, another objective would be the analysis of data, in particular survey data , to determine the reliability of the survey instrument used to the collect data. Cronbach’s Alpha is often applied to perform this task. Cronbach’s Alpha determines whether survey items (questions/statements) that belong to the same factor are really behaving in a similar way, i.e. showing the same characteristic as other items in that factor. Testing reliability of a survey instrument is a prerequisite for further analysis using the dataset in question.
Data Preparation Before Data Analysis
Often enough, data is not ready for analysis. This can be due to a data collection format that is not in sync with subsequent analysis tools. This can also be due to a distribution that makes it harder to analyse the data. Hence, reorganising , standardising or transforming (to normal distribution) the dataset might be necessary.
Data Analytics with Descriptive Statistics
Descriptive Statistics includes a set of tools that is used to quantitatively describe a set of data. It usually indicates central tendency, variability, minimum, maximum as well as distribution and deviation from this distribution ( kurtosis , skewness ). Descriptive statistics might also highlight potential outliers for further inspection and action.
Data Analytics with Predictive Statistics
In contrast to descriptive statistics characterising a certain given set of data, inferential statistics uses a subset of the population, a sample, to draw conclusions regarding the population. The inherent risk depends on the required confidence level, confidence interval and the sample size at hand as well as the variation in the data set. Hence, the test result indicates this risk.
Data Analytics with Factor Analysis
Factor Analysis helps determine clusters in datasets, i.e. it finds empirical variables that show a similar variability. These variables may therefore construct the same factor. A factor is a dependent, unobserved variable that includes multiple observed variables in the same cluster. Under certain circumstances, this can lead to a reduction of observed variables and hence the increase of sample size in the remaining unobserved variables (factors). So, both outcomes improve the power of subsequent statistical analysis of the data.
Factor analysis can use different approaches to pursue a multitude of objectives. Exploratory factor analysis (EFA) is used to identify complex interrelationships among items and determine clusters/factors whilst there is no predetermination of factors. Confirmatory factor analysis (CFA) is used to test the hypothesis that the items are associated with specific factors. In this case, factors are predetermined before the analysis.
Data Analytics For Problem Solving
Data analytics can be helpful in problem solving by establishing the significance of the relationship between problems (Y) and potential root causes (X). As a result, a large variety of tools is available. The selection of tools for a given data analytics task depends on the overall objective, the source and types of data. Discrete data, such as counts or attributes require different tools than continuous data, such as measurements. Whilst continuous data are transformable into discrete data for decision making, this process is irreversible.
Depending on the data in X and Y, regression analysis or hypothesis testing will be used to answer the question whether there is a relationship between problem and alleged root cause. These tools do not take away the decision, they rather tell the risk for a certain conclusion. The decision is still to be made by the process owner ( example ).
Analytics was never intended to replace intuition, but to supplement it instead. Stuart Farrand, Data Scientist at Pivotal Insight
Applications for data analytics are evident in all private and public organisations without limits. For example, already some decades ago, companies like Motorola and General Electric discovered the power of data analytics and made this the core of their Six Sigma movement. As a result, these organisations made sure, that problem solving is based on data and applied data analytics wherever appropriate. Nowadays, data analytics or data science is vital part of problem solving and most Lean Six Sigma projects. So, Six Sigma Black Belts are usually well-versed in this kind of data analysis and make good candidates for a Data Scientist career track.
To sum it up, we offer multiple training solutions as public and in-house courses. Please, check out our upcoming events .
Internet of Things for Starters
Managers need data analytics, automating a mess yields an automated mess, data analysis – plot the data, plot the data, plot the data, can we predict when our staff is leaving, leading digital-ready workforce, analytical storytelling, great, we have improved … or not, do you understand your survey results, making sense of the wilcoxon test, making sense of chi-squared test – finding differences in proportions, making sense of test for equal variances, make use of your survey data – kano them, making sense of the two-proportions test, making sense of linear regression.
Architect of High-Performing Organisations
+65 61000 263
Our Locations
Copyright © 2024 by COE Pte Ltd. All Rights Reserved.
Guide to Industrial Analytics
Solving Data Science Problems for Manufacturing and the Internet of Things
- © 2021
- Richard Hill ORCID: https://orcid.org/0000-0003-0105-7730 0 ,
- Stuart Berry 1
Department of Computer Science, University of Huddersfield, Huddersfield, UK
You can also search for this author in PubMed Google Scholar
Department of Computing and Mathematics, University of Derby, Derby, UK
- Describes data science techniques for solving problems in manufacturing and the Industrial Internet of Things
- Presents case study examples using commonly available software to solve real-world problems
- Empowers a practical understanding of essential modeling and analytics skills for system-oriented problem solving
Part of the book series: Texts in Computer Science (TCS)
9408 Accesses
2 Citations
1 Altmetric
This is a preview of subscription content, log in via an institution to check access.
Access this book
Subscribe and save.
- Get 10 units per month
- Download Article/Chapter or eBook
- 1 Unit = 1 Article or 1 Chapter
- Cancel anytime
- Available as EPUB and PDF
- Read on any device
- Instant download
- Own it forever
- Compact, lightweight edition
- Dispatched in 3 to 5 business days
- Free shipping worldwide - see info
- Durable hardcover edition
Tax calculation will be finalised at checkout
Other ways to access
Licence this eBook for your library
Institutional subscriptions
About this book
This textbook describes the hands-on application of data science techniques to solve problems in manufacturing and the Industrial Internet of Things (IIoT). Monitoring and managing operational performance is a crucial activity for industrial and business organisations. The emergence of low-cost, accessible computing and storage, through Industrial Digital Technologies (IDT) and Industry 4.0, has generated considerable interest in innovative approaches to doing more with data.
Data science, predictive analytics, machine learning, artificial intelligence and general approaches to modelling, simulating and visualising industrial systems have often been considered topics only for research labs and academic departments.
This textbook debunks the mystique around applied data science and shows readers, using tutorial-style explanations and real-life case studies, how practitioners can develop their own understanding of performance to achieve tangible business improvements. All exercises can be completed with commonly available tools, many of which are free to install and use.
Readers will learn how to use tools to investigate, diagnose, propose and implement analytics solutions that will provide explainable results to deliver digital transformation.
Similar content being viewed by others
An industrial big data pipeline for data-driven analytics maintenance applications in large-scale smart manufacturing facilities.
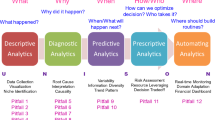
Pitfalls and protocols of data science in manufacturing practice
Experiences from the Internet-of-Production: Using “Data-Models-in-the-Middle” to Fight Complexity and Facilitate Reuse
- Optimization
- Batch Manufacture
- Industrial Internet of Things (IIoT)
- Internet of Things (IoT)
- Industry 4.0
- Machine Learning (ML)
- Manufacturing Systems
- Production Control
- Forecasting/Prediction
- Manufacturing Resource Planning (MRP II)
- Capacity Planning
Table of contents (10 chapters)
Front matter, introductory concepts, an introduction to industrial analytics.
- Richard Hill, Stuart Berry
Data, Analysis and Statistics
Measuring operations, data for production planning and control, simulating industrial processes, from process to system simulation, constructing machine learning models for prediction, application, case study: confectionery production, minimum information set for effective control, business adoption of analytics, back matter, authors and affiliations.
Richard Hill
Stuart Berry
About the authors
Dr. Richard Hill is Professor of Intelligent Systems, Head of the Department of Computer Science, and the Director of the Centre for Industrial Analytics at the University of Huddersfield, UK. His other publications include the Springer titles Guide to Vulnerability Analysis for Computer Networks and Systems , Guide to Security in SDN and NFV , Guide to Security Assurance for Cloud Computing , Big-Data Analytics and Cloud Computing , Guide to Cloud Computing , and Cloud Computing for Enterprise Architectures .
Dr. Stuart Berry is Emeritus Fellow in the Department of Computing and Mathematics at the University of Derby, UK. His other publications include the Springer title Guide to Computational Modelling for Decision Processes .
Bibliographic Information
Book Title : Guide to Industrial Analytics
Book Subtitle : Solving Data Science Problems for Manufacturing and the Internet of Things
Authors : Richard Hill, Stuart Berry
Series Title : Texts in Computer Science
DOI : https://doi.org/10.1007/978-3-030-79104-9
Publisher : Springer Cham
eBook Packages : Computer Science , Computer Science (R0)
Copyright Information : Springer Nature Switzerland AG 2021
Hardcover ISBN : 978-3-030-79103-2 Published: 28 September 2021
Softcover ISBN : 978-3-030-79106-3 Published: 29 September 2022
eBook ISBN : 978-3-030-79104-9 Published: 27 September 2021
Series ISSN : 1868-0941
Series E-ISSN : 1868-095X
Edition Number : 1
Number of Pages : XXV, 275
Number of Illustrations : 64 b/w illustrations, 108 illustrations in colour
Topics : Data Mining and Knowledge Discovery , Big Data , Manufacturing, Machines, Tools, Processes , Machine Learning , Computer Communication Networks , Simulation and Modeling
- Publish with us
Policies and ethics
- Find a journal
- Track your research
Data Analysis for Social Science
Elena Llaudet
30% off with code PUP30
Before you purchase audiobooks and ebooks
Please note that audiobooks and ebooks purchased from this site must be accessed on the Princeton University Press app. After you make your purchase, you will receive an email with instructions on how to download the app. Learn more about audio and ebooks .
Support your local independent bookstore.
- United States
- United Kingdom
Data Analysis for Social Science: A Friendly and Practical Introduction
- Elena Llaudet and Kosuke Imai
An ideal textbook for complete beginners—teaches from scratch R, statistics, and the fundamentals of quantitative social science
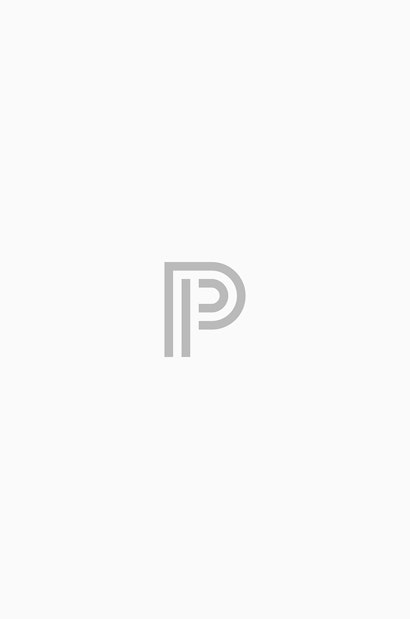
- Request Exam Copy
- Download Cover
Data Analysis for Social Science provides a friendly introduction to the statistical concepts and programming skills needed to conduct and evaluate social scientific studies. Assuming no prior knowledge of statistics and coding and only minimal knowledge of math, the book teaches the fundamentals of survey research, predictive models, and causal inference while analyzing data from published studies with the statistical program R. It teaches not only how to perform the data analyses but also how to interpret the results and identify the analyses’ strengths and limitations.
- Progresses by teaching how to solve one kind of problem after another, bringing in methods as needed. It teaches, in this order, how to (1) estimate causal effects with randomized experiments, (2) visualize and summarize data, (3) infer population characteristics, (4) predict outcomes, (5) estimate causal effects with observational data, and (6) generalize from sample to population.
- Flips the script of traditional statistics textbooks. It starts by estimating causal effects with randomized experiments and postpones any discussion of probability and statistical inference until the final chapters. This unconventional order engages students by demonstrating from the very beginning how data analysis can be used to answer interesting questions, while reserving more abstract, complex concepts for later chapters.
- Provides a step-by-step guide to analyzing real-world data using the powerful, open-source statistical program R, which is free for everyone to use. The datasets are provided on the book’s website so that readers can learn how to analyze data by following along with the exercises in the book on their own computer.
- Assumes no prior knowledge of statistics or coding.
- Specifically designed to accommodate students with a variety of math backgrounds. It includes supplemental materials for students with minimal knowledge of math and clearly identifies sections with more advanced material so that readers can skip them if they so choose.
- Provides cheatsheets of statistical concepts and R code.
- Comes with instructor materials (upon request), including sample syllabi, lecture slides, and additional replication-style exercises with solutions and with the real-world datasets analyzed.
Looking for a more advanced introduction? Consider Quantitative Social Science by Kosuke Imai. In addition to covering the material in Data Analysis for Social Science , it teaches diffs-in-diffs models, heterogeneous effects, text analysis, and regression discontinuity designs, among other things.
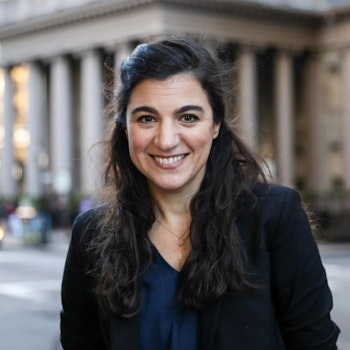
- 1.1 Book Overview
- 1.2 Chapter Summaries
- 1.3 How to Use This Book
- 1.4.1 Learning to Code
- 1.5 Getting Ready
- 1.6.1 Doing Calculations in R
- 1.6.2 Creating Objects in R
- 1.6.3 Using Functions in R
- 1.7.1 Setting the Working Directory
- 1.7.2 Loading the Dataset
- 1.7.3 Understanding the Data
- 1.7.4 Identifying the Types of Variables Included
- 1.7.5 Identifying the Number of Observations
- 1.8.1 Accessing Variables inside Dataframes
- 1.8.2 Means
- 1.9 Summary
- 1.10.1 Concepts and Notation
- 1.10.2 R Symbols and Operators
- 1.10.3 R Functions
- 2.1 Project STAR
- 2.2.1 Treatment Variables
- 2.2.2 Outcome Variables
- 2.3 Individual Causal Effects
- 2.4.1 Randomized Experiments and the Difference-in-Means Estimator
- 2.5.1 Relational Operators in R
- 2.5.2 Creating New Variables
- 2.5.3 Subsetting Variables
- 2.6 Summary
- 2.7.1 Concepts and Notation
- 2.7.2 R Symbols and Operators
- 2.7.3 R Functions
- 3.1 The EU Referendum in the UK
- 3.2.1 Random Sampling
- 3.2.2 Potential Challenges
- 3.3.1 Predicting the Referendum Outcome
- 3.3.2 Frequency Tables
- 3.3.3 Tables of Proportions
- 3.4.1 Handling Missing Data
- 3.4.2 Two-Way Frequency Tables
- 3.4.3 Two-Way Tables of Proportions
- 3.4.4 Histograms
- 3.4.5 Density Histograms
- 3.4.6 Descriptive Statistics
- 3.5.1 Scatter Plots
- 3.5.2 Correlation
- 3.6 Summary
- 3.7.1 Concepts and Notation
- 3.7.2 R Symbols and Operators
- 3.7.3 R Functions
- 4.1 GDP and Night-Time Light Emissions
- 4.2 Predictors, Observed vs. Predicted Outcomes, andPrediction Errors
- 4.3.1 The Linear Regression Model
- 4.3.2 The Intercept Coefficient
- 4.3.3 The Slope Coefficient
- 4.3.4 The Least Squares Method
- 4.4.1 Relationship between GDP and Prior GDP
- 4.4.2 With Natural Logarithm Transformations
- 4.5 Predicting GDP Growth Using Night-Time LightEmissions
- 4.6.1 How Well Do the Three Predictive Modelsin This Chapter Fit the Data?
- 4.7 Summary
- 4.8 Appendix: Interpretation of the Slope in the Log-Log Linear Model
- 4.9.1 Concepts and Notation
- 4.9.2 R Functions
- 5.1 Russian State-Controlled TV Coverage of 2014Ukrainian Affairs
- 5.2.1 Confounding Variables
- 5.2.2 Why Are Confounders a Problem?
- 5.2.3 Confounders in Randomized Experiments
- 5.3.1 Using the Simple Linear Model to Computethe Difference-in-Means Estimator
- 5.3.2 Controlling for Confounders Using aMultiple Linear Regression Model
- 5.4.1 Using the Simple Linear Model to Computethe Difference-in-Means Estimator
- 5.4.2 Controlling for Confounders Using aMultiple Linear Regression Model
- 5.5.1 Randomized Experiments vs.Observational Studies
- 5.5.2 The Role of Randomization
- 5.5.3 How Good Are the Two Causal Analysesin This Chapter?
- 5.5.4 How Good Was the Causal Analysis inChapter 2?
- 5.5.5 The Coefficient of Determination, R 2
- 5.6 Summary
- 5.7.1 Concepts and Notation
- 5.7.2 R Functions
- 6.1 What Is Probability?
- 6.2 Axioms of Probability
- 6.3 Events, Random Variables, and ProbabilityDistributions
- 6.4.1 The Bernoulli Distribution
- 6.4.2 The Normal Distribution
- 6.4.3 The Standard Normal Distribution
- 6.4.4 Recap
- 6.5.1 The Law of Large Numbers
- 6.5.2 The Central Limit Theorem
- 6.5.3 Sampling Distribution of the Sample Mean
- 6.6 Summary
- 6.7 Appendix: For Loops
- 6.8.1 Concepts and Notation
- 6.8.2 R Symbols and Operators
- 6.8.3 R Functions
- 7.1 Estimators and Their Sampling Distributions
- 7.2.1 For the Sample Mean
- 7.2.2 For the Difference-in-Means Estimator
- 7.2.3 For Predicted Outcomes
- 7.3.1 With the Difference-in-Means Estimator
- 7.3.2 With Estimated Regression Coefficients
- 7.4 Statistical vs. Scientific Significance
- 7.5 Summary
- 7.6.1 Concepts and Notation
- 7.6.2 R Symbols and Operators
- 7.6.3 R Functions
- Index of Concepts
- Index of Mathematical Notation
- Index of R and RStudio
“This is the book that I plan to teach from next time I teach introductory statistics. As it is, I recommend it as a reference for students in more advanced classes such as Applied Regression and Causal Inference, if they want a clean refresher from first principles.”—Andrew Gelman, coauthor of Regression and Other Stories
“This is without doubt the best book to get started with data analysis in the social sciences. Readers learn best practices in research design, measurement, data analysis, and data visualization, all in an approachable and engaging way. My students—all of them complete novices—were easily able to conduct their own analyses after working through this book.”—Simon Weschle, Syracuse University
“I love this book. More importantly, my students love this book. Data Analysis for Social Science is the perfect introduction to causal inference, probability and statistics, and the open-source programming language R, for students without prior experience. With multiple exercises using R Markdown and a variety of datasets drawn from the research literature, Data Analysis for Social Science gives students a hands-on path to build their skills and confidence.”—Anna Harvey, New York University
“ Data Analysis for Social Science is a game changer! I have been teaching quantitative methods for fourteen years, and I never had such good results and engagement from my students until I adopted this book. The logic behind the content structure is much more intuitive than usual, focusing on understanding the applications of quantitative methods (particularly linear regressions) before introducing the theory. The book and the instructor resources it comes with are incredibly practical and well designed, with relevant datasets and examples. After all these years, it is really refreshing to find a book that has students in mind and stresses intuition over abstraction, without sacrificing complexity and rigor.”—Javier Sajuria, Queen Mary University of London
“ Data Analysis for Social Science helped me teach introductory research methods at the right level for the types of students in my class. This book provides detailed explanations, step-by-step examples, and repetition to ensure complete beginners are not overwhelmed and slowly build confidence. I also use it as an optional text for higher-level courses because it clearly explains concepts even PhD students are often confused about. Furthermore, the instructor resources that come with it are the best I’ve seen provided with a textbook and made adopting the book much easier.”—Mark Richardson, Georgetown University
“Data science from zero to sixty—gently, expertly, quickly.”—Gary King, Weatherhead University Professor, Harvard University
“This book will transform the way we teach data science in the social sciences. Assuming zero background knowledge, it takes readers step-by-step through the most important concepts of data analysis and coding without sacrificing rigor. With clear explanations, beautiful visuals, and engaging examples, Data Analysis for Social Science is the obvious choice for any student looking to build their data science tool kit.”—Molly Roberts, University of California, San Diego
“I highly recommend Data Analysis for Social Science ! It is exceptionally well-written and cleverly organized. I particularly love its problem-solving approach and how it is intertwined with R code. While most textbooks teach statistics without offering students a clear motivation, this one teaches statistics as a way to solve real problems with real datasets. For example, if you want to estimate average causal effects with randomized experiments, then you must learn to compute the mean of a subset of the data. Or, if you want to understand the precision of your estimates, then you need to learn probability (but not beforehand!). I am using this book in my undergraduate courses with great satisfaction, and my students appreciate its easily understandable explanations.”—Guillermo Solovey, University of Buenos Aires
“My favorite feature of Data Analysis for Social Science is that it puts causal inference first, before probability and statistical inference. I have found that this unconventional order is gentler and more engaging for complete beginners than the approach used in many other books. It also allows students with some prior knowledge of statistics to learn something new from the start.”—Max Goplerud, University of Pittsburgh
“ Data Analysis for Social Science is a great textbook for any undergraduate research methods course. I especially like that it teaches point estimates and uncertainty separately. In the past, when I taught these concepts together, I found students were overwhelmed. Breaking them up makes the statistics easier to understand. It’s a genius idea! I truly can’t recommend this book enough!”—Christopher Ojeda, University of California, Merced
“I have been teaching statistics for twenty-five years and I have never seen a book this well done. Data Analysis for Social Science is such a perfect combination of what students need to know. The authors’ descriptions of the basic logic of causality, along with the many practical examples and visuals, are amazing features. Also, I have been resisting teaching intro students R because I am very watchful of overloading their bandwidth and I worry about killing their spirit with buggy code; I want them to love data analysis as much as I do! This book made me a convert. I am going to spend the time to learn R so that I can assign this book.”—Vanessa Baird, University of Colorado, Boulder
“I have used Data Analysis for Social Science to teach required undergraduate courses with great success. Students liked the clear explanations and relevant real-world examples, and they even found coding in R fun! By the end, they walked away excited about how these skills opened up new career opportunities and helped them understand the research discussed in other classes.”—Alicia Cooperman, George Washington University
“Looking to get started with data science, but scared it’d be too complicated? This book has you covered. Data Analysis for Social Science truly delivers what the title claims: friendly and practical. The focus is on experimental data and causal inference much more than on multiple regression analysis, reflecting recent developments in the social sciences. I don’t think I’ve seen a more accessible introduction to R and RStudio—cheat sheets included!”—Didier Ruedin, University of Neuchâtel
“Following the step-by-step guidance provided in this book, I built my skills in R rather than another expensive proprietary software, allowing me to share my growing knowledge with my working-class, first-generation students. I am confident I can continue to independently develop these skills in ways that support both my teaching and research.”—Jamie D. Gravell, California State University, Stanislaus
“At last, we have a truly modern introduction to social science statistics. The authors do not shy away from topics like causal inference, and they gently and seamlessly integrate instructions on how to use R. This textbook is a generous gift to both students and teachers.”—Valerio Baćak, School of Criminal Justice, Rutgers University, Newark
“A very sensible and intuitive introduction to data science. Llaudet and Imai do an excellent job of explaining the why of data analysis along with the how. I would recommend this book to anyone looking for a nice primer on data science coupled with a good set of tools using the R software.”—Craig Depken, University of North Carolina, Charlotte
“ Data Analysis for Social Science is outstanding—by far the most effective introductory textbook on the topic I have come across in more than a decade of teaching this material. With the help of this textbook, students with no statistics or coding background end up thinking about analyses and handling data like pros. Every time I teach from this book, I am amazed by how easy it makes my job as an instructor.”—P. J. Lamberson, University of California, Los Angeles
Stay connected for new books and special offers. Subscribe to receive a welcome discount for your next order.
Buy a gift card for the book lover in your life and let them choose their next great read.
- ebook & Audiobook Cart
5 Steps on How to Approach a New Data Science Problem
Many companies struggle to reorganize their decision making around data and implement a coherent data strategy. The problem certainly isn’t lack of data but inability to transform it into actionable insights. Here's how to do it right.
A QUICK SUMMARY – FOR THE BUSY ONES
TABLE OF CONTENTS
Introduction
Data has become the new gold. 85 percent of companies are trying to be data-driven, according to last year’s survey by NewVantage Partners , and the global data science platform market is expected to reach $128.21 billion by 2022, up from $19.75 billion in 2016.
Clearly, data science is not just another buzzword with limited real-world use cases. Yet, many companies struggle to reorganize their decision making around data and implement a coherent data strategy. The problem certainly isn’t lack of data.
In the past few years alone, 90 percent of all of the world’s data has been created, and our current daily data output has reached 2.5 quintillion bytes, which is such a mind-bogglingly large number that it’s difficult to fully appreciate the break-neck pace at which we generate new data.
The real problem is the inability of companies to transform the data they have at their disposal into actionable insights that can be used to make better business decisions, stop threats, and mitigate risks.
In fact, there’s often too much data available to make a clear decision, which is why it’s crucial for companies to know how to approach a new data science problem and understand what types of questions data science can answer.
What types of questions can data science answer?
“Data science and statistics are not magic. They won’t magically fix all of a company’s problems. However, they are useful tools to help companies make more accurate decisions and automate repetitive work and choices that teams need to make,” writes Seattle Data Guy , a data-driven consulting agency.
The questions that can be answered with the help of data science fall under following categories:
- Identifying themes in large data sets : Which server in my server farm needs maintenance the most?
- Identifying anomalies in large data sets : Is this combination of purchases different from what this customer has ordered in the past?
- Predicting the likelihood of something happening : How likely is this user to click on my video?
- Showing how things are connected to one another : What is the topic of this online article?
- Categorizing individual data points : Is this an image of a cat or a mouse?
Of course, this is by no means a complete list of all questions that data science can answer. Even if it were, data science is evolving at such a rapid pace that it would most likely be completely outdated within a year or two from its publication.
Now that we’ve established the types of questions that can be reasonably expected to be answered with the help of data science, it’s time to lay down the steps most data scientists would take when approaching a new data science problem.
Step 1: Define the problem
First, it’s necessary to accurately define the data problem that is to be solved. The problem should be clear, concise, and measurable . Many companies are too vague when defining data problems, which makes it difficult or even impossible for data scientists to translate them into machine code.
Here are some basic characteristics of a well-defined data problem:
- The solution to the problem is likely to have enough positive impact to justify the effort.
- Enough data is available in a usable format.
- Stakeholders are interested in applying data science to solve the problem.
Step 2: Decide on an approach
There are many data science algorithms that can be applied to data, and they can be roughly grouped into the following families:
- Two-class classification : useful for any question that has just two possible answers.
- Multi-class classification : answers a question that has multiple possible answers.
- Anomaly detection : identifies data points that are not normal.
- Regression : gives a real-valued answer and is useful when looking for a number instead of a class or category.
- Multi-class classification as regression : useful for questions that occur as rankings or comparisons.
- Two-class classification as regression : useful for binary classification problems that can also be reformulated as regression.
- Clustering : answer questions about how data is organized by seeking to separate out a data set into intuitive chunks.
- Dimensionality reduction : reduces the number of random variables under consideration by obtaining a set of principal variables.
- Reinforcement learning algorithms : focus on taking action in an environment so as to maximize some notion of cumulative reward.
Step 3: Collect data
With the problem clearly defined and a suitable approach selected, it’s time to collect data. All collected data should be organized in a log along with collection dates and other helpful metadata.
It’s important to understand that collected data is seldom ready for analysis right away. Most data scientists spend much of their time on data cleaning , which includes removing missing values, identifying duplicate records, and correcting incorrect values.
Step 4: Analyze data
The next step after data collection and cleanup is data analysis. At this stage, there’s a certain chance that the selected data science approach won’t work. This is to be expected and accounted for. Generally, it’s recommended to start with trying all the basic machine learning approaches as they have fewer parameters to alter.
There are many excellent open source data science libraries that can be used to analyze data. Most data science tools are written in Python, Java, or C++.
<blockquote><p>“Tempting as these cool toys are, for most applications the smart initial choice will be to pick a much simpler model, for example using scikit-learn and modeling techniques like simple logistic regression,” – advises Francine Bennett, the CEO and co-founder of Mastodon C.</p></blockquote>
Step 5: Interpret results
After data analysis, it’s finally time to interpret the results. The most important thing to consider is whether the original problem has been solved. You might discover that your model is working but producing subpar results. One way how to deal with this is to add more data and keep retraining the model until satisfied with it.
Most companies today are drowning in data. The global leaders are already using the data they generate to gain competitive advantage, and others are realizing that they must do the same or perish. While transforming an organization to become data-driven is no easy task, the reward is more than worth the effort.
The 5 steps on how to approach a new data science problem we’ve described in this article are meant to illustrate the general problem-solving mindset companies must adopt to successfully face the challenges of our current data-centric era.
Frequently Asked Questions
Our promise
Every year, Brainhub helps 750,000+ founders, leaders and software engineers make smart tech decisions. We earn that trust by openly sharing our insights based on practical software engineering experience.
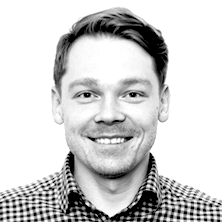
A serial entrepreneur, passionate R&D engineer, with 15 years of experience in the tech industry. Shares his expert knowledge about tech, startups, business development, and market analysis.
Popular this month
previous article in this collection
It's the first one.
next article in this collection
It's the last one.

FOR EMPLOYERS
Top 10 real-world data science case studies.
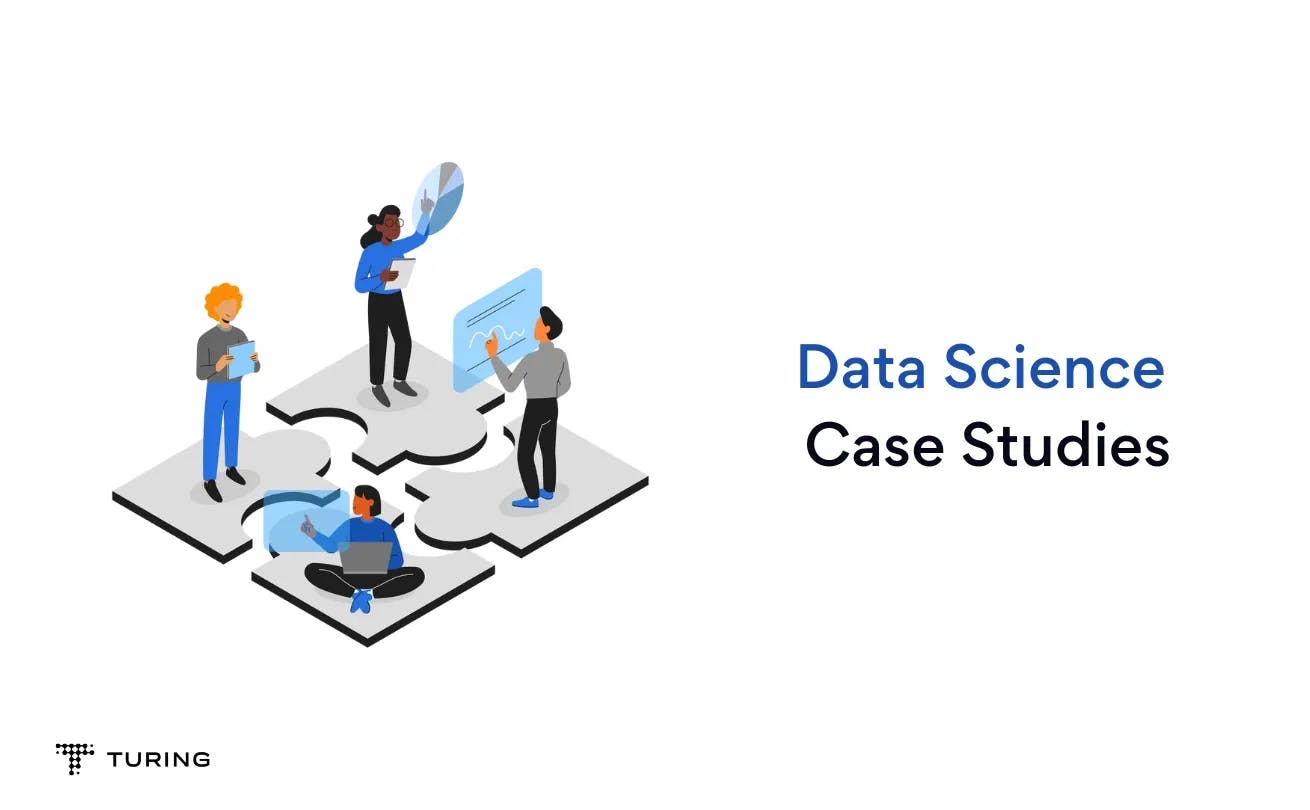
Aditya Sharma
Aditya is a content writer with 5+ years of experience writing for various industries including Marketing, SaaS, B2B, IT, and Edtech among others. You can find him watching anime or playing games when he’s not writing.
Frequently Asked Questions
Real-world data science case studies differ significantly from academic examples. While academic exercises often feature clean, well-structured data and simplified scenarios, real-world projects tackle messy, diverse data sources with practical constraints and genuine business objectives. These case studies reflect the complexities data scientists face when translating data into actionable insights in the corporate world.
Real-world data science projects come with common challenges. Data quality issues, including missing or inaccurate data, can hinder analysis. Domain expertise gaps may result in misinterpretation of results. Resource constraints might limit project scope or access to necessary tools and talent. Ethical considerations, like privacy and bias, demand careful handling.
Lastly, as data and business needs evolve, data science projects must adapt and stay relevant, posing an ongoing challenge.
Real-world data science case studies play a crucial role in helping companies make informed decisions. By analyzing their own data, businesses gain valuable insights into customer behavior, market trends, and operational efficiencies.
These insights empower data-driven strategies, aiding in more effective resource allocation, product development, and marketing efforts. Ultimately, case studies bridge the gap between data science and business decision-making, enhancing a company's ability to thrive in a competitive landscape.
Key takeaways from these case studies for organizations include the importance of cultivating a data-driven culture that values evidence-based decision-making. Investing in robust data infrastructure is essential to support data initiatives. Collaborating closely between data scientists and domain experts ensures that insights align with business goals.
Finally, continuous monitoring and refinement of data solutions are critical for maintaining relevance and effectiveness in a dynamic business environment. Embracing these principles can lead to tangible benefits and sustainable success in real-world data science endeavors.
Data science is a powerful driver of innovation and problem-solving across diverse industries. By harnessing data, organizations can uncover hidden patterns, automate repetitive tasks, optimize operations, and make informed decisions.
In healthcare, for example, data-driven diagnostics and treatment plans improve patient outcomes. In finance, predictive analytics enhances risk management. In transportation, route optimization reduces costs and emissions. Data science empowers industries to innovate and solve complex challenges in ways that were previously unimaginable.
Hire remote developers
Tell us the skills you need and we'll find the best developer for you in days, not weeks.
M.S. in International Security
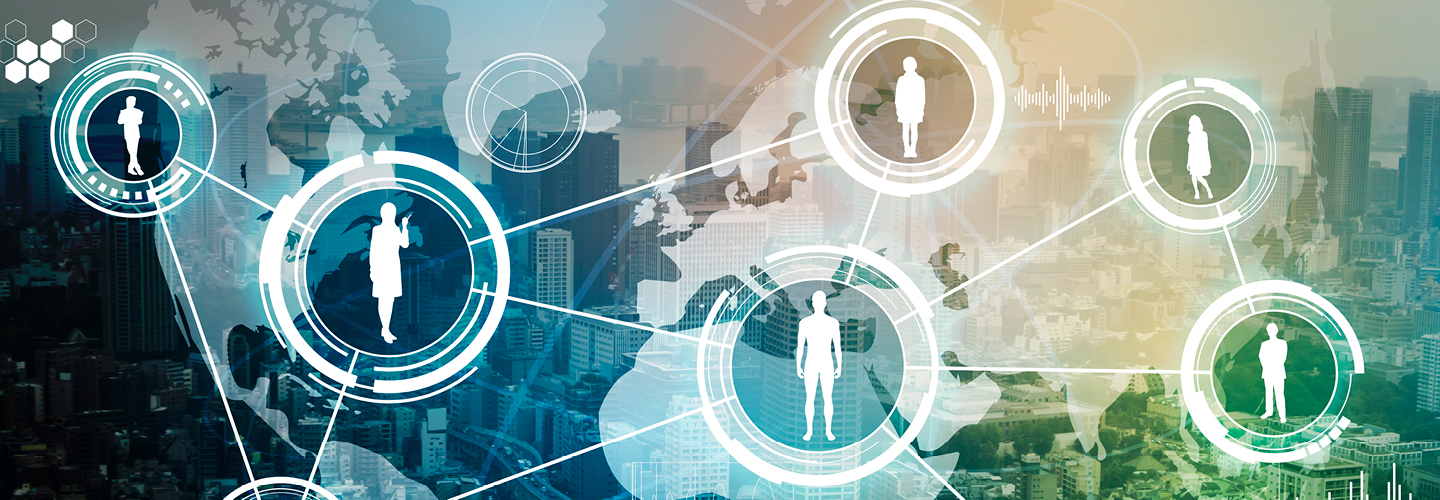
The single-year Master of Science in International Security (M.S. ISEC) offers students the opportunity to understand conceptual foundations and practical policy analysis of issues related to international security. The curriculum allows students to customize their program of study among a diverse set of subjects — including 21st-century great power rivalry; defense policy analysis and military strategy; crisis decision-making; comparative forms of statecraft; transnational terrorism; peacekeeping; regional security; geopolitics of energy; cross-cultural bargaining and negotiations; and cyber warfare. In addition to exposure to qualitative and quantitative research methods, students can acquire proficiency with tools such as data and visual analytics and modeling and simulation—in understanding ongoing international security challenges.
A 30-credit hour course load prepares graduates with the substantive knowledge and skills to distill, assess, and communicate the drivers and strategic consequences associated with emerging global security problems as experts and leaders in foreign and security policymaking, defense planning, the intelligence community, non-governmental organizations, and the private sector. The International Security program is part of the Nunn School’s mission of developing global policymakers for the 21st century.
The M.S. ISEC is also offered in a fully online format for working professionals. Learn more about the online program on the Georgia Tech Professional Education website .
Degree Requirements – 30 credit hours
INTA 6102 International Relations Theory | 3 |
INTA 6003 Empirical Research Methods | 3 |
INTA 6103 International Security | 3 |
International Security Courses | 12 |
Free Electives | 9 |
Program Benefits
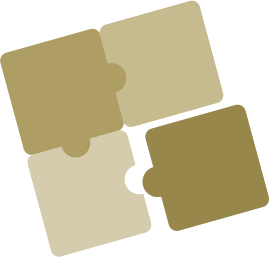
Problem Solving
The program provides students with the theoretical foundation and practical policy analytical skills to tackle issues directly related to contemporary International Security.
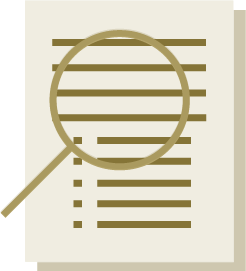
Scientific Analysis
Students develop a toolkit in visual analytics and modeling and simulation to understand ongoing international security challenges.
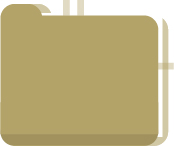
Career Development
Georgia Tech offers career counseling to help students discover their strengths and limitations, type of work environment they would excel in, and specific career interests.
Graduate Advising / Admissions
Chris McDermott Associate Director of Academic Programs - Advising
Habersham Building 781 Marietta St. NW Atlanta, GA 30332-0610 Email: [email protected] Twitter: @NunnSchoolGT
More About Advising
Relevant Links
Online M.S. ISEC
Admissions Instructions
Student Handbook
Admissions FAQ
Meet Our Alumni
Advertisement
Supported by
OpenAI Unveils New ChatGPT That Can Reason Through Math and Science
Driven by new technology called OpenAI o1, the chatbot can test various strategies and try to identify mistakes as it tackles complex tasks.
- Share full article

By Cade Metz
Reporting from San Francisco
Online chatbots like ChatGPT from OpenAI and Gemini from Google sometimes struggle with simple math problems . The computer code they generate is often buggy and incomplete. From time to time, they even make stuff up .
On Thursday, OpenAI unveiled a new version of ChatGPT that could alleviate these flaws. The company said the chatbot, underpinned by new artificial intelligence technology called OpenAI o1, could “reason” through tasks involving math, coding and science.
“With previous models like ChatGPT, you ask them a question and they immediately start responding,” said Jakub Pachocki, OpenAI’s chief scientist. “This model can take its time. It can think through the problem — in English — and try to break it down and look for angles in an effort to provide the best answer.”
In a demonstration for The New York Times, Dr. Pachocki and Szymon Sidor, an OpenAI technical fellow, showed the chatbot solving an acrostic, a kind of word puzzle that is significantly more complex than an ordinary crossword puzzle. The chatbot also answered a Ph.D.-level chemistry question and diagnosed an illness based on a detailed report about a patient’s symptoms and history.
The new technology is part of a wider effort to build A.I. that can reason through complex tasks. Companies like Google and Meta are building similar technologies, while Microsoft and its subsidiary GitHub are working to incorporate OpenAI’s new system into their products.
We are having trouble retrieving the article content.
Please enable JavaScript in your browser settings.
Thank you for your patience while we verify access. If you are in Reader mode please exit and log into your Times account, or subscribe for all of The Times.
Thank you for your patience while we verify access.
Already a subscriber? Log in .
Want all of The Times? Subscribe .
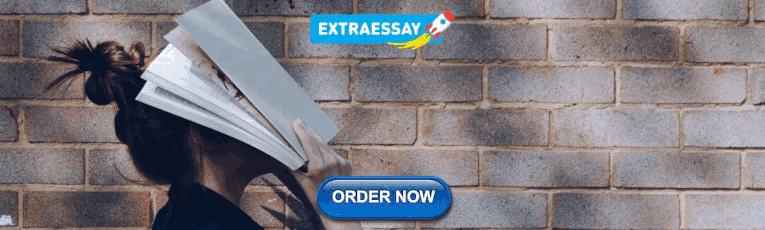
COMMENTS
Unit 7: Medium: Problem solving and data analysis. Our mission is to provide a free, world-class education to anyone, anywhere. Khan Academy is a 501 (c) (3) nonprofit organization. Donate or volunteer today!
The questions in Problem Solving and Data Analysis include both multiple-choice questions and student-produced response questions. he use of a calculator is allowed for all questions in this domain. Problem Solving and Data Analysis is one of the three SAT Math Test sub-scores, reported on a scale of 1 to 15.
Data to Insight: An Introduction to Data Analysis Chris Wild | Page 1 of 3 The place of data analysis in problem solving This model shows the process of abstracting and solving a statistical problem to help solve a larger real problem. A knowledge-based solution to the real problem requires better understanding of how some things work.
Break down problems into small steps. One of the essential strategies for problem-solving is to break down the problem into the smallest steps possible — atomic steps. Try to describe every single step. Don't write any code or start your search for the magic formula. Make notes in plain language.
Khan Academy
Here are some sample difficult Problem Solving and Data Analysis questions and explanations of how to solve them. Remember, these questions only appear on the Calculator section of the exam, so you will have access to a calculator for all of them. 1. Measures of Central Tendency (Mean/Median/Mode) Correct Answer: B.
Data Exploration: Exploratory data analysis is all about asking relevant questions and making sure all the possibilities are considered while solving a problem. Some of the mental models that could be adopted here are First-Principle Thinking, Second-Ordering Thinking, Bottom-up & Top-down Approaches, and Probabilistic Thinking.
Before jumping in, it's crucial to plan the analysis, decide which analytical tools to use, and ensure rigor. Check out these insights to uncover ways data can take your problem-solving techniques to the next level, and stay tuned for an upcoming post on the potential power of generative AI in problem-solving. The data-driven enterprise of 2025.
The questions in Problem Solving and Data Analysis focus on linear, quadratic and exponential relationships which may be represented by charts, graphs or tables. A model is linear if the difference in quantity is constant. A model is exponential if the ratio in the quantity is constant. A line of best fit is a straight line that best represents ...
Problem solving strategy 1: Simplify. Simplify to reduce complexity. Source: author. We start with the most obvious but often overlooked technique for problem solving; simplifying the initial problem into a less complex one. This can help to bring seemingly impossible problems within reach.
Complete eight data science projects that lock in important real world skills-along with a practical process you can use to learn any new technique quickly and efficiently. Solve Any Data Analysis Problem guides you through eight common scenarios you'll encounter as a data scientist or analyst. As you explore each project, you'll also master a proven process for quickly learning new skills ...
SAT Math is divided intro three domains: Heart of Algebra; Problem Solving and Data Analysis; Passport to Advanced Math; It's a good idea to get really familiar with what's going to be on the test, where it was derived, and what the SAT is really testing. This post will focus on one domain—Problem Solving and Data Analysis.This is an opportunity to get cozy with these concepts, and with the ...
4. Engage With Data. Once you have a solid understanding of data science concepts and formulas, the next step is to practice. Like any skill, analytical skills improve the more you use them. Mock datasets—which you can find online or create yourself—present a low-risk option for putting your skills to the test.
Analysis skills can be applied in various contexts. In business, data analysis can help identify trends and make informed decisions. Analytical thinking can be used to solve complex problems or develop new products or services. In science, problem-solving skills can be used to explore new ideas or research topics.
Data analytics, or data analysis, is the process of screening, cleaning, transforming, and modeling data with the objective of discovering useful information, suggesting conclusions, and supporting problem solving as well as decision making.There are multiple approaches, including a variety of techniques and tools used for data analytics. Data analytics finds applications in many different ...
Dr. Richard Hill is Professor of Intelligent Systems, Head of the Department of Computer Science, and the Director of the Centre for Industrial Analytics at the University of Huddersfield, UK.His other publications include the Springer titles Guide to Vulnerability Analysis for Computer Networks and Systems, Guide to Security in SDN and NFV, Guide to Security Assurance for Cloud Computing, Big ...
These problems will be because of various reasons — businesses, the environment, the stakeholders, and sometimes purely due to people's psychology. To solve those problems, data analysis is very important. Data crunching, business analysis and finding unique insights is a very essential part of management analysis and decision making.
Data Analysis for Social Science provides a friendly introduction to the statistical concepts and programming skills needed to conduct and evaluate social scientific studies. Assuming no prior knowledge of statistics and coding and only minimal knowledge of math, the book teaches the fundamentals of survey research, predictive models, and causal inference while analyzing data from published ...
Step 3: Collect data. With the problem clearly defined and a suitable approach selected, it's time to collect data. All collected data should be organized in a log along with collection dates and other helpful metadata. It's important to understand that collected data is seldom ready for analysis right away.
Data science is a powerful driver of innovation and problem-solving across diverse industries. By harnessing data, organizations can uncover hidden patterns, automate repetitive tasks, optimize operations, and make informed decisions. In healthcare, for example, data-driven diagnostics and treatment plans improve patient outcomes.
Analyzing the valid data through SPSS.26, among the 553 valid questionnaires, there are 235 males, accounting for 42.50%, and 318 females, accounting for 57.50%; in terms of the distribution of institutions, there are 205 people from the double first-class colleges (it refers to the universities and colleges supported by national strategy in ...
Laboratory experience is critical to foster college students' collaborative problem-solving (CPS) abilities, but whether students stay cognitively engaged in CPS tasks during online laboratory sessions remains unknown. This study applied multimodal data analysis to examine college students' (N = 36) cognitive engagement in CPS during their online experimentation experience. Groups of three ...
Nov 23, 2023. With the constant buzz around new tools and cutting-edge models, it's easy to lose sight of a basic truth: the real value in leveraging data lies in its ability to bring about tangible positive change. Whether it's around complex business decisions or our everyday routines, data-informed solutions are only as good as the ...
The single-year Master of Science in International Security (M.S. ISEC) offers students the opportunity to understand conceptual foundations and practical policy analysis of issues related to international security. The curriculum allows students to customize their program of study among a diverse set of subjects — including 21st-century ...
These enhanced reasoning capabilities may be particularly useful if you're tackling complex problems in science, coding, math, and similar fields. For example, o1 can be used by healthcare researchers to annotate cell sequencing data, by physicists to generate complicated mathematical formulas needed for quantum optics, and by developers in ...
News and Analysis OpenAI is in talks to raise about $6.5 billion as part of a deal that would value the company in the vicinity of $150 billion, a nearly $70 billion increase from its valuation ...