
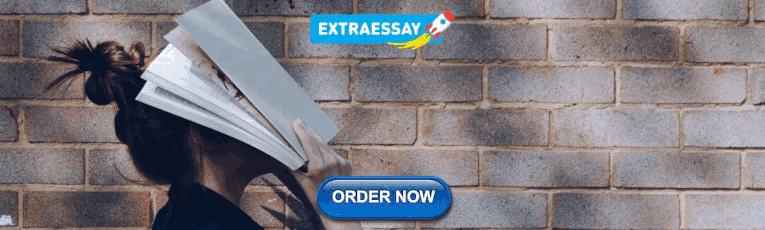
Causal Hypothesis
Ai generator.
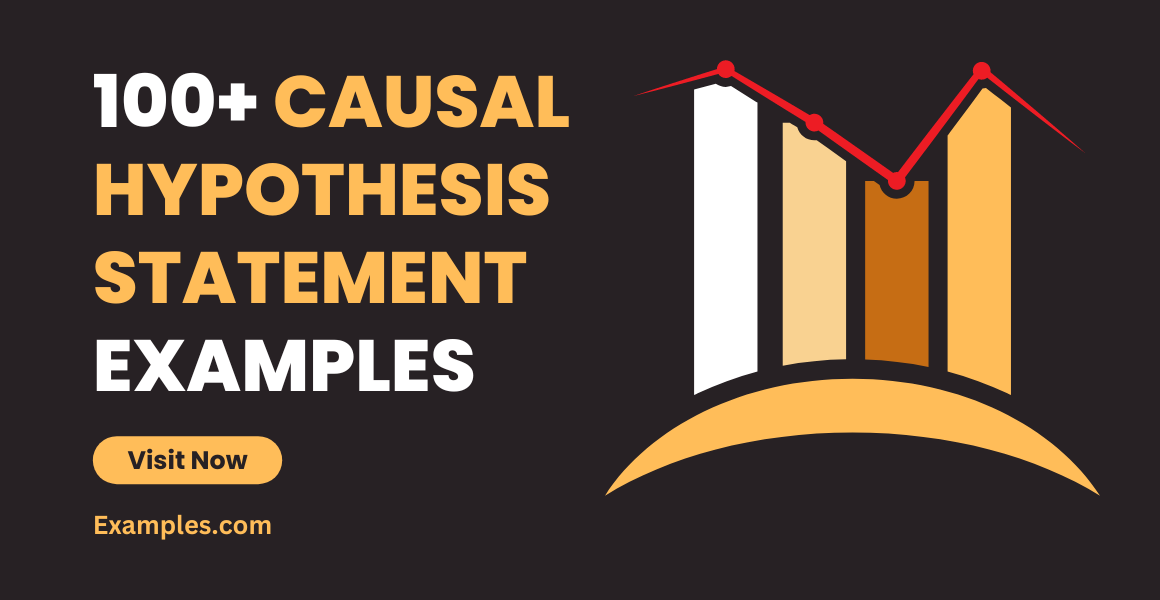
In scientific research, understanding causality is key to unraveling the intricacies of various phenomena. A causal hypothesis is a statement that predicts a cause-and-effect relationship between variables in a study. It serves as a guide to study design, data collection, and interpretation of results. This thesis statement segment aims to provide you with clear examples of causal hypotheses across diverse fields, along with a step-by-step guide and useful tips for formulating your own. Let’s delve into the essential components of constructing a compelling causal hypothesis.
What is Causal Hypothesis?
A causal hypothesis is a predictive statement that suggests a potential cause-and-effect relationship between two or more variables. It posits that a change in one variable (the independent or cause variable) will result in a change in another variable (the dependent or effect variable). The primary goal of a causal hypothesis is to determine whether one event or factor directly influences another. This type of Simple hypothesis is commonly tested through experiments where one variable can be manipulated to observe the effect on another variable.
What is an example of a Causal Hypothesis Statement?
Example 1: If a person increases their physical activity (cause), then their overall health will improve (effect).
Explanation: Here, the independent variable is the “increase in physical activity,” while the dependent variable is the “improvement in overall health.” The hypothesis suggests that by manipulating the level of physical activity (e.g., by exercising more), there will be a direct effect on the individual’s health.
Other examples can range from the impact of a change in diet on weight loss, the influence of class size on student performance, or the effect of a new training method on employee productivity. The key element in all causal hypotheses is the proposed direct relationship between cause and effect.
100 Causal Hypothesis Statement Examples
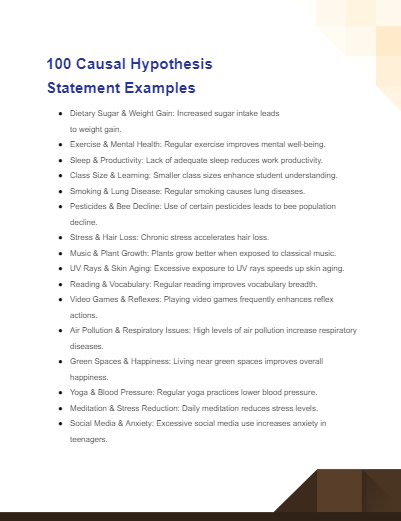
Size: 185 KB
Causal hypotheses predict cause-and-effect relationships, aiming to understand the influence one variable has on another. Rooted in experimental setups, they’re essential for deriving actionable insights in many fields. Delve into these 100 illustrative examples to understand the essence of causal relationships.
- Dietary Sugar & Weight Gain: Increased sugar intake leads to weight gain.
- Exercise & Mental Health: Regular exercise improves mental well-being.
- Sleep & Productivity: Lack of adequate sleep reduces work productivity.
- Class Size & Learning: Smaller class sizes enhance student understanding.
- Smoking & Lung Disease: Regular smoking causes lung diseases.
- Pesticides & Bee Decline: Use of certain pesticides leads to bee population decline.
- Stress & Hair Loss: Chronic stress accelerates hair loss.
- Music & Plant Growth: Plants grow better when exposed to classical music.
- UV Rays & Skin Aging: Excessive exposure to UV rays speeds up skin aging.
- Reading & Vocabulary: Regular reading improves vocabulary breadth.
- Video Games & Reflexes: Playing video games frequently enhances reflex actions.
- Air Pollution & Respiratory Issues: High levels of air pollution increase respiratory diseases.
- Green Spaces & Happiness: Living near green spaces improves overall happiness.
- Yoga & Blood Pressure: Regular yoga practices lower blood pressure.
- Meditation & Stress Reduction: Daily meditation reduces stress levels.
- Social Media & Anxiety: Excessive social media use increases anxiety in teenagers.
- Alcohol & Liver Damage: Regular heavy drinking leads to liver damage.
- Training & Job Efficiency: Intensive training improves job performance.
- Seat Belts & Accident Survival: Using seat belts increases chances of surviving car accidents.
- Soft Drinks & Bone Density: High consumption of soft drinks decreases bone density.
- Homework & Academic Performance: Regular homework completion improves academic scores.
- Organic Food & Health Benefits: Consuming organic food improves overall health.
- Fiber Intake & Digestion: Increased dietary fiber enhances digestion.
- Therapy & Depression Recovery: Regular therapy sessions improve depression recovery rates.
- Financial Education & Savings: Financial literacy education increases personal saving rates.
- Brushing & Dental Health: Brushing teeth twice a day reduces dental issues.
- Carbon Emission & Global Warming: Higher carbon emissions accelerate global warming.
- Afforestation & Climate Stability: Planting trees stabilizes local climates.
- Ad Exposure & Sales: Increased product advertisement boosts sales.
- Parental Involvement & Academic Success: Higher parental involvement enhances student academic performance.
- Hydration & Skin Health: Regular water intake improves skin elasticity and health.
- Caffeine & Alertness: Consuming caffeine increases alertness levels.
- Antibiotics & Bacterial Resistance: Overuse of antibiotics leads to increased antibiotic-resistant bacteria.
- Pet Ownership & Loneliness: Having pets reduces feelings of loneliness.
- Fish Oil & Cognitive Function: Regular consumption of fish oil improves cognitive functions.
- Noise Pollution & Sleep Quality: High levels of noise pollution degrade sleep quality.
- Exercise & Bone Density: Weight-bearing exercises increase bone density.
- Vaccination & Disease Prevention: Proper vaccination reduces the incidence of related diseases.
- Laughter & Immune System: Regular laughter boosts the immune system.
- Gardening & Stress Reduction: Engaging in gardening activities reduces stress levels.
- Travel & Cultural Awareness: Frequent travel increases cultural awareness and tolerance.
- High Heels & Back Pain: Prolonged wearing of high heels leads to increased back pain.
- Junk Food & Heart Disease: Excessive junk food consumption increases the risk of heart diseases.
- Mindfulness & Anxiety Reduction: Practicing mindfulness lowers anxiety levels.
- Online Learning & Flexibility: Online education offers greater flexibility to learners.
- Urbanization & Wildlife Displacement: Rapid urbanization leads to displacement of local wildlife.
- Vitamin C & Cold Recovery: High doses of vitamin C speed up cold recovery.
- Team Building Activities & Work Cohesion: Regular team-building activities improve workplace cohesion.
- Multitasking & Productivity: Multitasking reduces individual task efficiency.
- Protein Intake & Muscle Growth: Increased protein consumption boosts muscle growth in individuals engaged in strength training.
- Mentoring & Career Progression: Having a mentor accelerates career progression.
- Fast Food & Obesity Rates: High consumption of fast food leads to increased obesity rates.
- Deforestation & Biodiversity Loss: Accelerated deforestation results in significant biodiversity loss.
- Language Learning & Cognitive Flexibility: Learning a second language enhances cognitive flexibility.
- Red Wine & Heart Health: Moderate red wine consumption may benefit heart health.
- Public Speaking Practice & Confidence: Regular public speaking practice boosts confidence.
- Fasting & Metabolism: Intermittent fasting can rev up metabolism.
- Plastic Usage & Ocean Pollution: Excessive use of plastics leads to increased ocean pollution.
- Peer Tutoring & Academic Retention: Peer tutoring improves academic retention rates.
- Mobile Usage & Sleep Patterns: Excessive mobile phone use before bed disrupts sleep patterns.
- Green Spaces & Mental Well-being: Living near green spaces enhances mental well-being.
- Organic Foods & Health Outcomes: Consuming organic foods leads to better health outcomes.
- Art Exposure & Creativity: Regular exposure to art boosts creativity.
- Gaming & Hand-Eye Coordination: Engaging in video games improves hand-eye coordination.
- Prenatal Music & Baby’s Development: Exposing babies to music in the womb enhances their auditory development.
- Dark Chocolate & Mood Enhancement: Consuming dark chocolate can elevate mood.
- Urban Farms & Community Engagement: Establishing urban farms promotes community engagement.
- Reading Fiction & Empathy Levels: Reading fiction regularly increases empathy.
- Aerobic Exercise & Memory: Engaging in aerobic exercises sharpens memory.
- Meditation & Blood Pressure: Regular meditation can reduce blood pressure.
- Classical Music & Plant Growth: Plants exposed to classical music show improved growth.
- Pollution & Respiratory Diseases: Higher pollution levels increase respiratory diseases’ incidence.
- Parental Involvement & Child’s Academic Success: Direct parental involvement in schooling enhances children’s academic success.
- Sugar Intake & Tooth Decay: High sugar intake is directly proportional to tooth decay.
- Physical Books & Reading Comprehension: Reading physical books improves comprehension better than digital mediums.
- Daily Journaling & Self-awareness: Maintaining a daily journal enhances self-awareness.
- Robotics Learning & Problem-solving Skills: Engaging in robotics learning fosters problem-solving skills in students.
- Forest Bathing & Stress Relief: Immersion in forest environments (forest bathing) reduces stress levels.
- Reusable Bags & Environmental Impact: Using reusable bags reduces environmental pollution.
- Affirmations & Self-esteem: Regularly reciting positive affirmations enhances self-esteem.
- Local Produce Consumption & Community Economy: Buying and consuming local produce boosts the local economy.
- Sunlight Exposure & Vitamin D Levels: Regular sunlight exposure enhances Vitamin D levels in the body.
- Group Study & Learning Enhancement: Group studies can enhance learning compared to individual studies.
- Active Commuting & Fitness Levels: Commuting by walking or cycling improves overall fitness.
- Foreign Film Watching & Cultural Understanding: Watching foreign films increases understanding and appreciation of different cultures.
- Craft Activities & Fine Motor Skills: Engaging in craft activities enhances fine motor skills.
- Listening to Podcasts & Knowledge Expansion: Regularly listening to educational podcasts broadens one’s knowledge base.
- Outdoor Play & Child’s Physical Development: Encouraging outdoor play accelerates physical development in children.
- Thrift Shopping & Sustainable Living: Choosing thrift shopping promotes sustainable consumption habits.
- Nature Retreats & Burnout Recovery: Taking nature retreats aids in burnout recovery.
- Virtual Reality Training & Skill Acquisition: Using virtual reality for training accelerates skill acquisition in medical students.
- Pet Ownership & Loneliness Reduction: Owning a pet significantly reduces feelings of loneliness among elderly individuals.
- Intermittent Fasting & Metabolism Boost: Practicing intermittent fasting can lead to an increase in metabolic rate.
- Bilingual Education & Cognitive Flexibility: Being educated in a bilingual environment improves cognitive flexibility in children.
- Urbanization & Loss of Biodiversity: Rapid urbanization contributes to a loss of biodiversity in the surrounding environment.
- Recycled Materials & Carbon Footprint Reduction: Utilizing recycled materials in production processes reduces a company’s overall carbon footprint.
- Artificial Sweeteners & Appetite Increase: Consuming artificial sweeteners might lead to an increase in appetite.
- Green Roofs & Urban Temperature Regulation: Implementing green roofs in urban buildings contributes to moderating city temperatures.
- Remote Work & Employee Productivity: Adopting a remote work model can boost employee productivity and job satisfaction.
- Sensory Play & Child Development: Incorporating sensory play in early childhood education supports holistic child development.
Causal Hypothesis Statement Examples in Research
Research hypothesis often delves into understanding the cause-and-effect relationships between different variables. These causal hypotheses attempt to predict a specific effect if a particular cause is present, making them vital for experimental designs.
- Artificial Intelligence & Job Market: Implementation of artificial intelligence in industries causes a decline in manual jobs.
- Online Learning Platforms & Traditional Classroom Efficiency: The introduction of online learning platforms reduces the efficacy of traditional classroom teaching methods.
- Nano-technology & Medical Treatment Efficacy: Using nano-technology in drug delivery enhances the effectiveness of medical treatments.
- Genetic Editing & Lifespan: Advancements in genetic editing techniques directly influence the lifespan of organisms.
- Quantum Computing & Data Security: The rise of quantum computing threatens the security of traditional encryption methods.
- Space Tourism & Aerospace Advancements: The demand for space tourism propels advancements in aerospace engineering.
- E-commerce & Retail Business Model: The surge in e-commerce platforms leads to a decline in the traditional retail business model.
- VR in Real Estate & Buyer Decisions: Using virtual reality in real estate presentations influences buyer decisions more than traditional methods.
- Biofuels & Greenhouse Gas Emissions: Increasing biofuel production directly reduces greenhouse gas emissions.
- Crowdfunding & Entrepreneurial Success: The availability of crowdfunding platforms boosts the success rate of start-up enterprises.
Causal Hypothesis Statement Examples in Epidemiology
Epidemiology is a study of how and why certain diseases occur in particular populations. Causal hypotheses in this field aim to uncover relationships between health interventions, behaviors, and health outcomes.
- Vaccine Introduction & Disease Eradication: The introduction of new vaccines directly leads to the reduction or eradication of specific diseases.
- Urbanization & Rise in Respiratory Diseases: Increased urbanization causes a surge in respiratory diseases due to pollution.
- Processed Foods & Obesity Epidemic: The consumption of processed foods is directly linked to the rising obesity epidemic.
- Sanitation Measures & Cholera Outbreaks: Implementing proper sanitation measures reduces the incidence of cholera outbreaks.
- Tobacco Consumption & Lung Cancer: Prolonged tobacco consumption is the primary cause of lung cancer among adults.
- Antibiotic Misuse & Antibiotic-Resistant Strains: Misuse of antibiotics leads to the evolution of antibiotic-resistant bacterial strains.
- Alcohol Consumption & Liver Diseases: Excessive and regular alcohol consumption is a leading cause of liver diseases.
- Vitamin D & Rickets in Children: A deficiency in vitamin D is the primary cause of rickets in children.
- Airborne Pollutants & Asthma Attacks: Exposure to airborne pollutants directly triggers asthma attacks in susceptible individuals.
- Sedentary Lifestyle & Cardiovascular Diseases: Leading a sedentary lifestyle is a significant risk factor for cardiovascular diseases.
Causal Hypothesis Statement Examples in Psychology
In psychology, causal hypotheses explore how certain behaviors, conditions, or interventions might influence mental and emotional outcomes. These hypotheses help in deciphering the intricate web of human behavior and cognition.
- Childhood Trauma & Personality Disorders: Experiencing trauma during childhood increases the risk of developing personality disorders in adulthood.
- Positive Reinforcement & Skill Acquisition: The use of positive reinforcement accelerates skill acquisition in children.
- Sleep Deprivation & Cognitive Performance: Lack of adequate sleep impairs cognitive performance in adults.
- Social Isolation & Depression: Prolonged social isolation is a significant cause of depression among teenagers.
- Mindfulness Meditation & Stress Reduction: Regular practice of mindfulness meditation reduces symptoms of stress and anxiety.
- Peer Pressure & Adolescent Risk Taking: Peer pressure significantly increases risk-taking behaviors among adolescents.
- Parenting Styles & Child’s Self-esteem: Authoritarian parenting styles negatively impact a child’s self-esteem.
- Multitasking & Attention Span: Engaging in multitasking frequently leads to a reduced attention span.
- Childhood Bullying & Adult PTSD: Individuals bullied during childhood have a higher likelihood of developing PTSD as adults.
- Digital Screen Time & Child Development: Excessive digital screen time impairs cognitive and social development in children.
Causal Inference Hypothesis Statement Examples
Causal inference is about deducing the cause-effect relationship between two variables after considering potential confounders. These hypotheses aim to find direct relationships even when other influencing factors are present.
- Dietary Habits & Chronic Illnesses: Even when considering genetic factors, unhealthy dietary habits increase the chances of chronic illnesses.
- Exercise & Mental Well-being: When accounting for daily stressors, regular exercise improves mental well-being.
- Job Satisfaction & Employee Turnover: Even when considering market conditions, job satisfaction inversely relates to employee turnover.
- Financial Literacy & Savings Behavior: When considering income levels, financial literacy is directly linked to better savings behavior.
- Online Reviews & Product Sales: Even accounting for advertising spends, positive online reviews boost product sales.
- Prenatal Care & Child Health Outcomes: When considering genetic factors, adequate prenatal care ensures better health outcomes for children.
- Teacher Qualifications & Student Performance: Accounting for socio-economic factors, teacher qualifications directly influence student performance.
- Community Engagement & Crime Rates: When considering economic conditions, higher community engagement leads to lower crime rates.
- Eco-friendly Practices & Brand Loyalty: Accounting for product quality, eco-friendly business practices boost brand loyalty.
- Mental Health Support & Workplace Productivity: Even when considering workload, providing mental health support enhances workplace productivity.
What are the Characteristics of Causal Hypothesis
Causal hypotheses are foundational in many research disciplines, as they predict a cause-and-effect relationship between variables. Their unique characteristics include:
- Cause-and-Effect Relationship: The core of a causal hypothesis is to establish a direct relationship, indicating that one variable (the cause) will bring about a change in another variable (the effect).
- Testability: They are formulated in a manner that allows them to be empirically tested using appropriate experimental or observational methods.
- Specificity: Causal hypotheses should be specific, delineating clear cause and effect variables.
- Directionality: They typically demonstrate a clear direction in which the cause leads to the effect.
- Operational Definitions: They often use operational definitions, which specify the procedures used to measure or manipulate variables.
- Temporal Precedence: The cause (independent variable) always precedes the effect (dependent variable) in time.
What is a causal hypothesis in research?
In research, a causal hypothesis is a statement about the expected relationship between variables, or explanation of an occurrence, that is clear, specific, testable, and falsifiable. It suggests a relationship in which a change in one variable is the direct cause of a change in another variable. For instance, “A higher intake of Vitamin C reduces the risk of common cold.” Here, Vitamin C intake is the independent variable, and the risk of common cold is the dependent variable.
What is the difference between causal and descriptive hypothesis?
- Causal Hypothesis: Predicts a cause-and-effect relationship between two or more variables.
- Descriptive Hypothesis: Describes an occurrence, detailing the characteristics or form of a particular phenomenon.
- Causal: Consuming too much sugar can lead to diabetes.
- Descriptive: 60% of adults in the city exercise at least thrice a week.
- Causal: To establish a causal connection between variables.
- Descriptive: To give an accurate portrayal of the situation or fact.
- Causal: Often involves experiments.
- Descriptive: Often involves surveys or observational studies.
How do you write a Causal Hypothesis? – A Step by Step Guide
- Identify Your Variables: Pinpoint the cause (independent variable) and the effect (dependent variable). For instance, in studying the relationship between smoking and lung health, smoking is the independent variable while lung health is the dependent variable.
- State the Relationship: Clearly define how one variable affects another. Does an increase in the independent variable lead to an increase or decrease in the dependent variable?
- Be Specific: Avoid vague terms. Instead of saying “improved health,” specify the type of improvement like “reduced risk of cardiovascular diseases.”
- Use Operational Definitions: Clearly define any terms or variables in your hypothesis. For instance, define what you mean by “regular exercise” or “high sugar intake.”
- Ensure It’s Testable: Your hypothesis should be structured so that it can be disproven or supported by data.
- Review Existing Literature: Check previous research to ensure that your hypothesis hasn’t already been tested, and to ensure it’s plausible based on existing knowledge.
- Draft Your Hypothesis: Combine all the above steps to write a clear, concise hypothesis. For instance: “Regular exercise (defined as 150 minutes of moderate exercise per week) decreases the risk of cardiovascular diseases.”
Tips for Writing Causal Hypothesis
- Simplicity is Key: The clearer and more concise your hypothesis, the easier it will be to test.
- Avoid Absolutes: Using words like “all” or “always” can be problematic. Few things are universally true.
- Seek Feedback: Before finalizing your hypothesis, get feedback from peers or mentors.
- Stay Objective: Base your hypothesis on existing literature and knowledge, not on personal beliefs or biases.
- Revise as Needed: As you delve deeper into your research, you may find the need to refine your hypothesis for clarity or specificity.
- Falsifiability: Always ensure your hypothesis can be proven wrong. If it can’t be disproven, it can’t be validated either.
- Avoid Circular Reasoning: Ensure that your hypothesis doesn’t assume what it’s trying to prove. For example, “People who are happy have a positive outlook on life” is a circular statement.
- Specify Direction: In causal hypotheses, indicating the direction of the relationship can be beneficial, such as “increases,” “decreases,” or “leads to.”
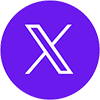
Text prompt
- Instructive
- Professional
10 Examples of Public speaking
20 Examples of Gas lighting
Educational resources and simple solutions for your research journey
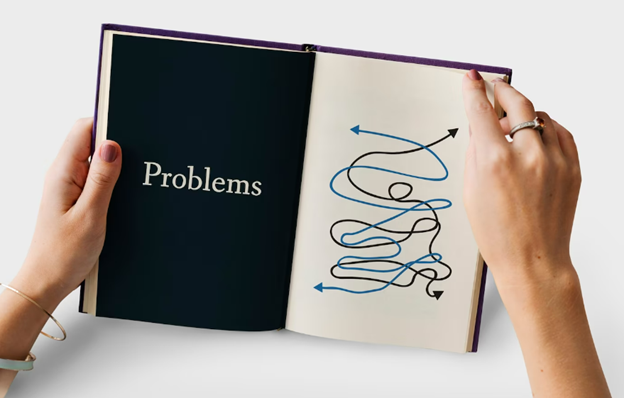
What is a Research Hypothesis: How to Write it, Types, and Examples
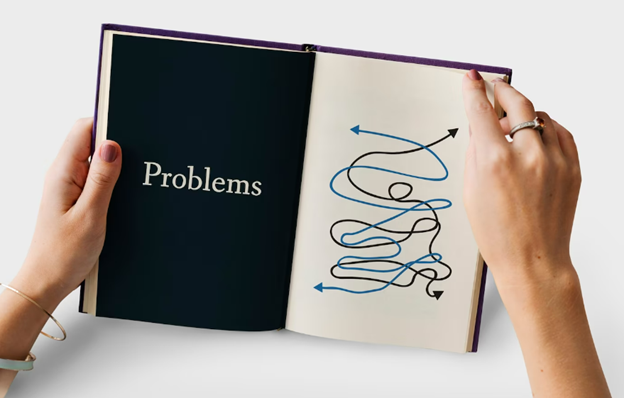
Any research begins with a research question and a research hypothesis . A research question alone may not suffice to design the experiment(s) needed to answer it. A hypothesis is central to the scientific method. But what is a hypothesis ? A hypothesis is a testable statement that proposes a possible explanation to a phenomenon, and it may include a prediction. Next, you may ask what is a research hypothesis ? Simply put, a research hypothesis is a prediction or educated guess about the relationship between the variables that you want to investigate.
It is important to be thorough when developing your research hypothesis. Shortcomings in the framing of a hypothesis can affect the study design and the results. A better understanding of the research hypothesis definition and characteristics of a good hypothesis will make it easier for you to develop your own hypothesis for your research. Let’s dive in to know more about the types of research hypothesis , how to write a research hypothesis , and some research hypothesis examples .
Table of Contents
What is a hypothesis ?
A hypothesis is based on the existing body of knowledge in a study area. Framed before the data are collected, a hypothesis states the tentative relationship between independent and dependent variables, along with a prediction of the outcome.
What is a research hypothesis ?
Young researchers starting out their journey are usually brimming with questions like “ What is a hypothesis ?” “ What is a research hypothesis ?” “How can I write a good research hypothesis ?”
A research hypothesis is a statement that proposes a possible explanation for an observable phenomenon or pattern. It guides the direction of a study and predicts the outcome of the investigation. A research hypothesis is testable, i.e., it can be supported or disproven through experimentation or observation.

Characteristics of a good hypothesis
Here are the characteristics of a good hypothesis :
- Clearly formulated and free of language errors and ambiguity
- Concise and not unnecessarily verbose
- Has clearly defined variables
- Testable and stated in a way that allows for it to be disproven
- Can be tested using a research design that is feasible, ethical, and practical
- Specific and relevant to the research problem
- Rooted in a thorough literature search
- Can generate new knowledge or understanding.
How to create an effective research hypothesis
A study begins with the formulation of a research question. A researcher then performs background research. This background information forms the basis for building a good research hypothesis . The researcher then performs experiments, collects, and analyzes the data, interprets the findings, and ultimately, determines if the findings support or negate the original hypothesis.
Let’s look at each step for creating an effective, testable, and good research hypothesis :
- Identify a research problem or question: Start by identifying a specific research problem.
- Review the literature: Conduct an in-depth review of the existing literature related to the research problem to grasp the current knowledge and gaps in the field.
- Formulate a clear and testable hypothesis : Based on the research question, use existing knowledge to form a clear and testable hypothesis . The hypothesis should state a predicted relationship between two or more variables that can be measured and manipulated. Improve the original draft till it is clear and meaningful.
- State the null hypothesis: The null hypothesis is a statement that there is no relationship between the variables you are studying.
- Define the population and sample: Clearly define the population you are studying and the sample you will be using for your research.
- Select appropriate methods for testing the hypothesis: Select appropriate research methods, such as experiments, surveys, or observational studies, which will allow you to test your research hypothesis .
Remember that creating a research hypothesis is an iterative process, i.e., you might have to revise it based on the data you collect. You may need to test and reject several hypotheses before answering the research problem.
How to write a research hypothesis
When you start writing a research hypothesis , you use an “if–then” statement format, which states the predicted relationship between two or more variables. Clearly identify the independent variables (the variables being changed) and the dependent variables (the variables being measured), as well as the population you are studying. Review and revise your hypothesis as needed.
An example of a research hypothesis in this format is as follows:
“ If [athletes] follow [cold water showers daily], then their [endurance] increases.”
Population: athletes
Independent variable: daily cold water showers
Dependent variable: endurance
You may have understood the characteristics of a good hypothesis . But note that a research hypothesis is not always confirmed; a researcher should be prepared to accept or reject the hypothesis based on the study findings.
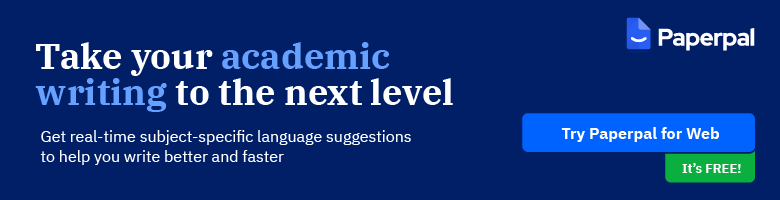
Research hypothesis checklist
Following from above, here is a 10-point checklist for a good research hypothesis :
- Testable: A research hypothesis should be able to be tested via experimentation or observation.
- Specific: A research hypothesis should clearly state the relationship between the variables being studied.
- Based on prior research: A research hypothesis should be based on existing knowledge and previous research in the field.
- Falsifiable: A research hypothesis should be able to be disproven through testing.
- Clear and concise: A research hypothesis should be stated in a clear and concise manner.
- Logical: A research hypothesis should be logical and consistent with current understanding of the subject.
- Relevant: A research hypothesis should be relevant to the research question and objectives.
- Feasible: A research hypothesis should be feasible to test within the scope of the study.
- Reflects the population: A research hypothesis should consider the population or sample being studied.
- Uncomplicated: A good research hypothesis is written in a way that is easy for the target audience to understand.
By following this research hypothesis checklist , you will be able to create a research hypothesis that is strong, well-constructed, and more likely to yield meaningful results.
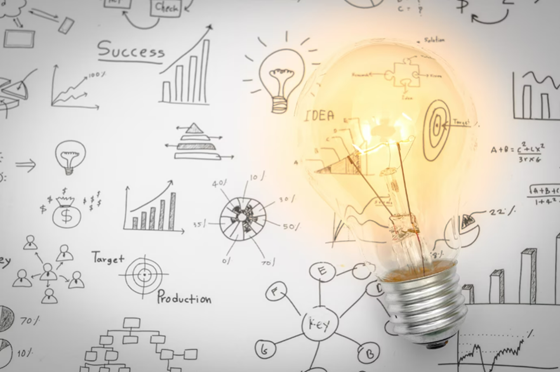
Types of research hypothesis
Different types of research hypothesis are used in scientific research:
1. Null hypothesis:
A null hypothesis states that there is no change in the dependent variable due to changes to the independent variable. This means that the results are due to chance and are not significant. A null hypothesis is denoted as H0 and is stated as the opposite of what the alternative hypothesis states.
Example: “ The newly identified virus is not zoonotic .”
2. Alternative hypothesis:
This states that there is a significant difference or relationship between the variables being studied. It is denoted as H1 or Ha and is usually accepted or rejected in favor of the null hypothesis.
Example: “ The newly identified virus is zoonotic .”
3. Directional hypothesis :
This specifies the direction of the relationship or difference between variables; therefore, it tends to use terms like increase, decrease, positive, negative, more, or less.
Example: “ The inclusion of intervention X decreases infant mortality compared to the original treatment .”
4. Non-directional hypothesis:
While it does not predict the exact direction or nature of the relationship between the two variables, a non-directional hypothesis states the existence of a relationship or difference between variables but not the direction, nature, or magnitude of the relationship. A non-directional hypothesis may be used when there is no underlying theory or when findings contradict previous research.
Example, “ Cats and dogs differ in the amount of affection they express .”
5. Simple hypothesis :
A simple hypothesis only predicts the relationship between one independent and another independent variable.
Example: “ Applying sunscreen every day slows skin aging .”
6 . Complex hypothesis :
A complex hypothesis states the relationship or difference between two or more independent and dependent variables.
Example: “ Applying sunscreen every day slows skin aging, reduces sun burn, and reduces the chances of skin cancer .” (Here, the three dependent variables are slowing skin aging, reducing sun burn, and reducing the chances of skin cancer.)
7. Associative hypothesis:
An associative hypothesis states that a change in one variable results in the change of the other variable. The associative hypothesis defines interdependency between variables.
Example: “ There is a positive association between physical activity levels and overall health .”
8 . Causal hypothesis:
A causal hypothesis proposes a cause-and-effect interaction between variables.
Example: “ Long-term alcohol use causes liver damage .”
Note that some of the types of research hypothesis mentioned above might overlap. The types of hypothesis chosen will depend on the research question and the objective of the study.
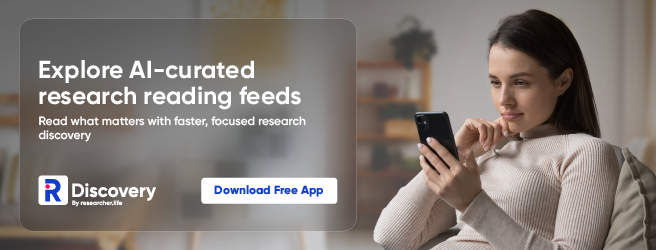
Research hypothesis examples
Here are some good research hypothesis examples :
“The use of a specific type of therapy will lead to a reduction in symptoms of depression in individuals with a history of major depressive disorder.”
“Providing educational interventions on healthy eating habits will result in weight loss in overweight individuals.”
“Plants that are exposed to certain types of music will grow taller than those that are not exposed to music.”
“The use of the plant growth regulator X will lead to an increase in the number of flowers produced by plants.”
Characteristics that make a research hypothesis weak are unclear variables, unoriginality, being too general or too vague, and being untestable. A weak hypothesis leads to weak research and improper methods.
Some bad research hypothesis examples (and the reasons why they are “bad”) are as follows:
“This study will show that treatment X is better than any other treatment . ” (This statement is not testable, too broad, and does not consider other treatments that may be effective.)
“This study will prove that this type of therapy is effective for all mental disorders . ” (This statement is too broad and not testable as mental disorders are complex and different disorders may respond differently to different types of therapy.)
“Plants can communicate with each other through telepathy . ” (This statement is not testable and lacks a scientific basis.)
Importance of testable hypothesis
If a research hypothesis is not testable, the results will not prove or disprove anything meaningful. The conclusions will be vague at best. A testable hypothesis helps a researcher focus on the study outcome and understand the implication of the question and the different variables involved. A testable hypothesis helps a researcher make precise predictions based on prior research.
To be considered testable, there must be a way to prove that the hypothesis is true or false; further, the results of the hypothesis must be reproducible.
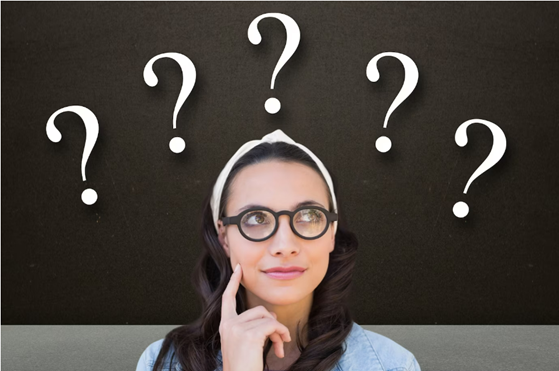
Frequently Asked Questions (FAQs) on research hypothesis
1. What is the difference between research question and research hypothesis ?
A research question defines the problem and helps outline the study objective(s). It is an open-ended statement that is exploratory or probing in nature. Therefore, it does not make predictions or assumptions. It helps a researcher identify what information to collect. A research hypothesis , however, is a specific, testable prediction about the relationship between variables. Accordingly, it guides the study design and data analysis approach.
2. When to reject null hypothesis ?
A null hypothesis should be rejected when the evidence from a statistical test shows that it is unlikely to be true. This happens when the test statistic (e.g., p -value) is less than the defined significance level (e.g., 0.05). Rejecting the null hypothesis does not necessarily mean that the alternative hypothesis is true; it simply means that the evidence found is not compatible with the null hypothesis.
3. How can I be sure my hypothesis is testable?
A testable hypothesis should be specific and measurable, and it should state a clear relationship between variables that can be tested with data. To ensure that your hypothesis is testable, consider the following:
- Clearly define the key variables in your hypothesis. You should be able to measure and manipulate these variables in a way that allows you to test the hypothesis.
- The hypothesis should predict a specific outcome or relationship between variables that can be measured or quantified.
- You should be able to collect the necessary data within the constraints of your study.
- It should be possible for other researchers to replicate your study, using the same methods and variables.
- Your hypothesis should be testable by using appropriate statistical analysis techniques, so you can draw conclusions, and make inferences about the population from the sample data.
- The hypothesis should be able to be disproven or rejected through the collection of data.
4. How do I revise my research hypothesis if my data does not support it?
If your data does not support your research hypothesis , you will need to revise it or develop a new one. You should examine your data carefully and identify any patterns or anomalies, re-examine your research question, and/or revisit your theory to look for any alternative explanations for your results. Based on your review of the data, literature, and theories, modify your research hypothesis to better align it with the results you obtained. Use your revised hypothesis to guide your research design and data collection. It is important to remain objective throughout the process.
5. I am performing exploratory research. Do I need to formulate a research hypothesis?
As opposed to “confirmatory” research, where a researcher has some idea about the relationship between the variables under investigation, exploratory research (or hypothesis-generating research) looks into a completely new topic about which limited information is available. Therefore, the researcher will not have any prior hypotheses. In such cases, a researcher will need to develop a post-hoc hypothesis. A post-hoc research hypothesis is generated after these results are known.
6. How is a research hypothesis different from a research question?
A research question is an inquiry about a specific topic or phenomenon, typically expressed as a question. It seeks to explore and understand a particular aspect of the research subject. In contrast, a research hypothesis is a specific statement or prediction that suggests an expected relationship between variables. It is formulated based on existing knowledge or theories and guides the research design and data analysis.
7. Can a research hypothesis change during the research process?
Yes, research hypotheses can change during the research process. As researchers collect and analyze data, new insights and information may emerge that require modification or refinement of the initial hypotheses. This can be due to unexpected findings, limitations in the original hypotheses, or the need to explore additional dimensions of the research topic. Flexibility is crucial in research, allowing for adaptation and adjustment of hypotheses to align with the evolving understanding of the subject matter.
8. How many hypotheses should be included in a research study?
The number of research hypotheses in a research study varies depending on the nature and scope of the research. It is not necessary to have multiple hypotheses in every study. Some studies may have only one primary hypothesis, while others may have several related hypotheses. The number of hypotheses should be determined based on the research objectives, research questions, and the complexity of the research topic. It is important to ensure that the hypotheses are focused, testable, and directly related to the research aims.
9. Can research hypotheses be used in qualitative research?
Yes, research hypotheses can be used in qualitative research, although they are more commonly associated with quantitative research. In qualitative research, hypotheses may be formulated as tentative or exploratory statements that guide the investigation. Instead of testing hypotheses through statistical analysis, qualitative researchers may use the hypotheses to guide data collection and analysis, seeking to uncover patterns, themes, or relationships within the qualitative data. The emphasis in qualitative research is often on generating insights and understanding rather than confirming or rejecting specific research hypotheses through statistical testing.
Editage All Access is a subscription-based platform that unifies the best AI tools and services designed to speed up, simplify, and streamline every step of a researcher’s journey. The Editage All Access Pack is a one-of-a-kind subscription that unlocks full access to an AI writing assistant, literature recommender, journal finder, scientific illustration tool, and exclusive discounts on professional publication services from Editage.
Based on 22+ years of experience in academia, Editage All Access empowers researchers to put their best research forward and move closer to success. Explore our top AI Tools pack, AI Tools + Publication Services pack, or Build Your Own Plan. Find everything a researcher needs to succeed, all in one place – Get All Access now starting at just $14 a month !
Related Posts

What are the Best Research Funding Sources

Inductive vs. Deductive Research Approach

An official website of the United States government
The .gov means it’s official. Federal government websites often end in .gov or .mil. Before sharing sensitive information, make sure you’re on a federal government site.
The site is secure. The https:// ensures that you are connecting to the official website and that any information you provide is encrypted and transmitted securely.
- Publications
- Account settings
Preview improvements coming to the PMC website in October 2024. Learn More or Try it out now .
- Advanced Search
- Journal List
- J Korean Med Sci
- v.37(16); 2022 Apr 25

A Practical Guide to Writing Quantitative and Qualitative Research Questions and Hypotheses in Scholarly Articles
Edward barroga.
1 Department of General Education, Graduate School of Nursing Science, St. Luke’s International University, Tokyo, Japan.
Glafera Janet Matanguihan
2 Department of Biological Sciences, Messiah University, Mechanicsburg, PA, USA.
The development of research questions and the subsequent hypotheses are prerequisites to defining the main research purpose and specific objectives of a study. Consequently, these objectives determine the study design and research outcome. The development of research questions is a process based on knowledge of current trends, cutting-edge studies, and technological advances in the research field. Excellent research questions are focused and require a comprehensive literature search and in-depth understanding of the problem being investigated. Initially, research questions may be written as descriptive questions which could be developed into inferential questions. These questions must be specific and concise to provide a clear foundation for developing hypotheses. Hypotheses are more formal predictions about the research outcomes. These specify the possible results that may or may not be expected regarding the relationship between groups. Thus, research questions and hypotheses clarify the main purpose and specific objectives of the study, which in turn dictate the design of the study, its direction, and outcome. Studies developed from good research questions and hypotheses will have trustworthy outcomes with wide-ranging social and health implications.
INTRODUCTION
Scientific research is usually initiated by posing evidenced-based research questions which are then explicitly restated as hypotheses. 1 , 2 The hypotheses provide directions to guide the study, solutions, explanations, and expected results. 3 , 4 Both research questions and hypotheses are essentially formulated based on conventional theories and real-world processes, which allow the inception of novel studies and the ethical testing of ideas. 5 , 6
It is crucial to have knowledge of both quantitative and qualitative research 2 as both types of research involve writing research questions and hypotheses. 7 However, these crucial elements of research are sometimes overlooked; if not overlooked, then framed without the forethought and meticulous attention it needs. Planning and careful consideration are needed when developing quantitative or qualitative research, particularly when conceptualizing research questions and hypotheses. 4
There is a continuing need to support researchers in the creation of innovative research questions and hypotheses, as well as for journal articles that carefully review these elements. 1 When research questions and hypotheses are not carefully thought of, unethical studies and poor outcomes usually ensue. Carefully formulated research questions and hypotheses define well-founded objectives, which in turn determine the appropriate design, course, and outcome of the study. This article then aims to discuss in detail the various aspects of crafting research questions and hypotheses, with the goal of guiding researchers as they develop their own. Examples from the authors and peer-reviewed scientific articles in the healthcare field are provided to illustrate key points.
DEFINITIONS AND RELATIONSHIP OF RESEARCH QUESTIONS AND HYPOTHESES
A research question is what a study aims to answer after data analysis and interpretation. The answer is written in length in the discussion section of the paper. Thus, the research question gives a preview of the different parts and variables of the study meant to address the problem posed in the research question. 1 An excellent research question clarifies the research writing while facilitating understanding of the research topic, objective, scope, and limitations of the study. 5
On the other hand, a research hypothesis is an educated statement of an expected outcome. This statement is based on background research and current knowledge. 8 , 9 The research hypothesis makes a specific prediction about a new phenomenon 10 or a formal statement on the expected relationship between an independent variable and a dependent variable. 3 , 11 It provides a tentative answer to the research question to be tested or explored. 4
Hypotheses employ reasoning to predict a theory-based outcome. 10 These can also be developed from theories by focusing on components of theories that have not yet been observed. 10 The validity of hypotheses is often based on the testability of the prediction made in a reproducible experiment. 8
Conversely, hypotheses can also be rephrased as research questions. Several hypotheses based on existing theories and knowledge may be needed to answer a research question. Developing ethical research questions and hypotheses creates a research design that has logical relationships among variables. These relationships serve as a solid foundation for the conduct of the study. 4 , 11 Haphazardly constructed research questions can result in poorly formulated hypotheses and improper study designs, leading to unreliable results. Thus, the formulations of relevant research questions and verifiable hypotheses are crucial when beginning research. 12
CHARACTERISTICS OF GOOD RESEARCH QUESTIONS AND HYPOTHESES
Excellent research questions are specific and focused. These integrate collective data and observations to confirm or refute the subsequent hypotheses. Well-constructed hypotheses are based on previous reports and verify the research context. These are realistic, in-depth, sufficiently complex, and reproducible. More importantly, these hypotheses can be addressed and tested. 13
There are several characteristics of well-developed hypotheses. Good hypotheses are 1) empirically testable 7 , 10 , 11 , 13 ; 2) backed by preliminary evidence 9 ; 3) testable by ethical research 7 , 9 ; 4) based on original ideas 9 ; 5) have evidenced-based logical reasoning 10 ; and 6) can be predicted. 11 Good hypotheses can infer ethical and positive implications, indicating the presence of a relationship or effect relevant to the research theme. 7 , 11 These are initially developed from a general theory and branch into specific hypotheses by deductive reasoning. In the absence of a theory to base the hypotheses, inductive reasoning based on specific observations or findings form more general hypotheses. 10
TYPES OF RESEARCH QUESTIONS AND HYPOTHESES
Research questions and hypotheses are developed according to the type of research, which can be broadly classified into quantitative and qualitative research. We provide a summary of the types of research questions and hypotheses under quantitative and qualitative research categories in Table 1 .
Quantitative research questions | Quantitative research hypotheses |
---|---|
Descriptive research questions | Simple hypothesis |
Comparative research questions | Complex hypothesis |
Relationship research questions | Directional hypothesis |
Non-directional hypothesis | |
Associative hypothesis | |
Causal hypothesis | |
Null hypothesis | |
Alternative hypothesis | |
Working hypothesis | |
Statistical hypothesis | |
Logical hypothesis | |
Hypothesis-testing | |
Qualitative research questions | Qualitative research hypotheses |
Contextual research questions | Hypothesis-generating |
Descriptive research questions | |
Evaluation research questions | |
Explanatory research questions | |
Exploratory research questions | |
Generative research questions | |
Ideological research questions | |
Ethnographic research questions | |
Phenomenological research questions | |
Grounded theory questions | |
Qualitative case study questions |
Research questions in quantitative research
In quantitative research, research questions inquire about the relationships among variables being investigated and are usually framed at the start of the study. These are precise and typically linked to the subject population, dependent and independent variables, and research design. 1 Research questions may also attempt to describe the behavior of a population in relation to one or more variables, or describe the characteristics of variables to be measured ( descriptive research questions ). 1 , 5 , 14 These questions may also aim to discover differences between groups within the context of an outcome variable ( comparative research questions ), 1 , 5 , 14 or elucidate trends and interactions among variables ( relationship research questions ). 1 , 5 We provide examples of descriptive, comparative, and relationship research questions in quantitative research in Table 2 .
Quantitative research questions | |
---|---|
Descriptive research question | |
- Measures responses of subjects to variables | |
- Presents variables to measure, analyze, or assess | |
What is the proportion of resident doctors in the hospital who have mastered ultrasonography (response of subjects to a variable) as a diagnostic technique in their clinical training? | |
Comparative research question | |
- Clarifies difference between one group with outcome variable and another group without outcome variable | |
Is there a difference in the reduction of lung metastasis in osteosarcoma patients who received the vitamin D adjunctive therapy (group with outcome variable) compared with osteosarcoma patients who did not receive the vitamin D adjunctive therapy (group without outcome variable)? | |
- Compares the effects of variables | |
How does the vitamin D analogue 22-Oxacalcitriol (variable 1) mimic the antiproliferative activity of 1,25-Dihydroxyvitamin D (variable 2) in osteosarcoma cells? | |
Relationship research question | |
- Defines trends, association, relationships, or interactions between dependent variable and independent variable | |
Is there a relationship between the number of medical student suicide (dependent variable) and the level of medical student stress (independent variable) in Japan during the first wave of the COVID-19 pandemic? |
Hypotheses in quantitative research
In quantitative research, hypotheses predict the expected relationships among variables. 15 Relationships among variables that can be predicted include 1) between a single dependent variable and a single independent variable ( simple hypothesis ) or 2) between two or more independent and dependent variables ( complex hypothesis ). 4 , 11 Hypotheses may also specify the expected direction to be followed and imply an intellectual commitment to a particular outcome ( directional hypothesis ) 4 . On the other hand, hypotheses may not predict the exact direction and are used in the absence of a theory, or when findings contradict previous studies ( non-directional hypothesis ). 4 In addition, hypotheses can 1) define interdependency between variables ( associative hypothesis ), 4 2) propose an effect on the dependent variable from manipulation of the independent variable ( causal hypothesis ), 4 3) state a negative relationship between two variables ( null hypothesis ), 4 , 11 , 15 4) replace the working hypothesis if rejected ( alternative hypothesis ), 15 explain the relationship of phenomena to possibly generate a theory ( working hypothesis ), 11 5) involve quantifiable variables that can be tested statistically ( statistical hypothesis ), 11 6) or express a relationship whose interlinks can be verified logically ( logical hypothesis ). 11 We provide examples of simple, complex, directional, non-directional, associative, causal, null, alternative, working, statistical, and logical hypotheses in quantitative research, as well as the definition of quantitative hypothesis-testing research in Table 3 .
Quantitative research hypotheses | |
---|---|
Simple hypothesis | |
- Predicts relationship between single dependent variable and single independent variable | |
If the dose of the new medication (single independent variable) is high, blood pressure (single dependent variable) is lowered. | |
Complex hypothesis | |
- Foretells relationship between two or more independent and dependent variables | |
The higher the use of anticancer drugs, radiation therapy, and adjunctive agents (3 independent variables), the higher would be the survival rate (1 dependent variable). | |
Directional hypothesis | |
- Identifies study direction based on theory towards particular outcome to clarify relationship between variables | |
Privately funded research projects will have a larger international scope (study direction) than publicly funded research projects. | |
Non-directional hypothesis | |
- Nature of relationship between two variables or exact study direction is not identified | |
- Does not involve a theory | |
Women and men are different in terms of helpfulness. (Exact study direction is not identified) | |
Associative hypothesis | |
- Describes variable interdependency | |
- Change in one variable causes change in another variable | |
A larger number of people vaccinated against COVID-19 in the region (change in independent variable) will reduce the region’s incidence of COVID-19 infection (change in dependent variable). | |
Causal hypothesis | |
- An effect on dependent variable is predicted from manipulation of independent variable | |
A change into a high-fiber diet (independent variable) will reduce the blood sugar level (dependent variable) of the patient. | |
Null hypothesis | |
- A negative statement indicating no relationship or difference between 2 variables | |
There is no significant difference in the severity of pulmonary metastases between the new drug (variable 1) and the current drug (variable 2). | |
Alternative hypothesis | |
- Following a null hypothesis, an alternative hypothesis predicts a relationship between 2 study variables | |
The new drug (variable 1) is better on average in reducing the level of pain from pulmonary metastasis than the current drug (variable 2). | |
Working hypothesis | |
- A hypothesis that is initially accepted for further research to produce a feasible theory | |
Dairy cows fed with concentrates of different formulations will produce different amounts of milk. | |
Statistical hypothesis | |
- Assumption about the value of population parameter or relationship among several population characteristics | |
- Validity tested by a statistical experiment or analysis | |
The mean recovery rate from COVID-19 infection (value of population parameter) is not significantly different between population 1 and population 2. | |
There is a positive correlation between the level of stress at the workplace and the number of suicides (population characteristics) among working people in Japan. | |
Logical hypothesis | |
- Offers or proposes an explanation with limited or no extensive evidence | |
If healthcare workers provide more educational programs about contraception methods, the number of adolescent pregnancies will be less. | |
Hypothesis-testing (Quantitative hypothesis-testing research) | |
- Quantitative research uses deductive reasoning. | |
- This involves the formation of a hypothesis, collection of data in the investigation of the problem, analysis and use of the data from the investigation, and drawing of conclusions to validate or nullify the hypotheses. |
Research questions in qualitative research
Unlike research questions in quantitative research, research questions in qualitative research are usually continuously reviewed and reformulated. The central question and associated subquestions are stated more than the hypotheses. 15 The central question broadly explores a complex set of factors surrounding the central phenomenon, aiming to present the varied perspectives of participants. 15
There are varied goals for which qualitative research questions are developed. These questions can function in several ways, such as to 1) identify and describe existing conditions ( contextual research question s); 2) describe a phenomenon ( descriptive research questions ); 3) assess the effectiveness of existing methods, protocols, theories, or procedures ( evaluation research questions ); 4) examine a phenomenon or analyze the reasons or relationships between subjects or phenomena ( explanatory research questions ); or 5) focus on unknown aspects of a particular topic ( exploratory research questions ). 5 In addition, some qualitative research questions provide new ideas for the development of theories and actions ( generative research questions ) or advance specific ideologies of a position ( ideological research questions ). 1 Other qualitative research questions may build on a body of existing literature and become working guidelines ( ethnographic research questions ). Research questions may also be broadly stated without specific reference to the existing literature or a typology of questions ( phenomenological research questions ), may be directed towards generating a theory of some process ( grounded theory questions ), or may address a description of the case and the emerging themes ( qualitative case study questions ). 15 We provide examples of contextual, descriptive, evaluation, explanatory, exploratory, generative, ideological, ethnographic, phenomenological, grounded theory, and qualitative case study research questions in qualitative research in Table 4 , and the definition of qualitative hypothesis-generating research in Table 5 .
Qualitative research questions | |
---|---|
Contextual research question | |
- Ask the nature of what already exists | |
- Individuals or groups function to further clarify and understand the natural context of real-world problems | |
What are the experiences of nurses working night shifts in healthcare during the COVID-19 pandemic? (natural context of real-world problems) | |
Descriptive research question | |
- Aims to describe a phenomenon | |
What are the different forms of disrespect and abuse (phenomenon) experienced by Tanzanian women when giving birth in healthcare facilities? | |
Evaluation research question | |
- Examines the effectiveness of existing practice or accepted frameworks | |
How effective are decision aids (effectiveness of existing practice) in helping decide whether to give birth at home or in a healthcare facility? | |
Explanatory research question | |
- Clarifies a previously studied phenomenon and explains why it occurs | |
Why is there an increase in teenage pregnancy (phenomenon) in Tanzania? | |
Exploratory research question | |
- Explores areas that have not been fully investigated to have a deeper understanding of the research problem | |
What factors affect the mental health of medical students (areas that have not yet been fully investigated) during the COVID-19 pandemic? | |
Generative research question | |
- Develops an in-depth understanding of people’s behavior by asking ‘how would’ or ‘what if’ to identify problems and find solutions | |
How would the extensive research experience of the behavior of new staff impact the success of the novel drug initiative? | |
Ideological research question | |
- Aims to advance specific ideas or ideologies of a position | |
Are Japanese nurses who volunteer in remote African hospitals able to promote humanized care of patients (specific ideas or ideologies) in the areas of safe patient environment, respect of patient privacy, and provision of accurate information related to health and care? | |
Ethnographic research question | |
- Clarifies peoples’ nature, activities, their interactions, and the outcomes of their actions in specific settings | |
What are the demographic characteristics, rehabilitative treatments, community interactions, and disease outcomes (nature, activities, their interactions, and the outcomes) of people in China who are suffering from pneumoconiosis? | |
Phenomenological research question | |
- Knows more about the phenomena that have impacted an individual | |
What are the lived experiences of parents who have been living with and caring for children with a diagnosis of autism? (phenomena that have impacted an individual) | |
Grounded theory question | |
- Focuses on social processes asking about what happens and how people interact, or uncovering social relationships and behaviors of groups | |
What are the problems that pregnant adolescents face in terms of social and cultural norms (social processes), and how can these be addressed? | |
Qualitative case study question | |
- Assesses a phenomenon using different sources of data to answer “why” and “how” questions | |
- Considers how the phenomenon is influenced by its contextual situation. | |
How does quitting work and assuming the role of a full-time mother (phenomenon assessed) change the lives of women in Japan? |
Qualitative research hypotheses | |
---|---|
Hypothesis-generating (Qualitative hypothesis-generating research) | |
- Qualitative research uses inductive reasoning. | |
- This involves data collection from study participants or the literature regarding a phenomenon of interest, using the collected data to develop a formal hypothesis, and using the formal hypothesis as a framework for testing the hypothesis. | |
- Qualitative exploratory studies explore areas deeper, clarifying subjective experience and allowing formulation of a formal hypothesis potentially testable in a future quantitative approach. |
Qualitative studies usually pose at least one central research question and several subquestions starting with How or What . These research questions use exploratory verbs such as explore or describe . These also focus on one central phenomenon of interest, and may mention the participants and research site. 15
Hypotheses in qualitative research
Hypotheses in qualitative research are stated in the form of a clear statement concerning the problem to be investigated. Unlike in quantitative research where hypotheses are usually developed to be tested, qualitative research can lead to both hypothesis-testing and hypothesis-generating outcomes. 2 When studies require both quantitative and qualitative research questions, this suggests an integrative process between both research methods wherein a single mixed-methods research question can be developed. 1
FRAMEWORKS FOR DEVELOPING RESEARCH QUESTIONS AND HYPOTHESES
Research questions followed by hypotheses should be developed before the start of the study. 1 , 12 , 14 It is crucial to develop feasible research questions on a topic that is interesting to both the researcher and the scientific community. This can be achieved by a meticulous review of previous and current studies to establish a novel topic. Specific areas are subsequently focused on to generate ethical research questions. The relevance of the research questions is evaluated in terms of clarity of the resulting data, specificity of the methodology, objectivity of the outcome, depth of the research, and impact of the study. 1 , 5 These aspects constitute the FINER criteria (i.e., Feasible, Interesting, Novel, Ethical, and Relevant). 1 Clarity and effectiveness are achieved if research questions meet the FINER criteria. In addition to the FINER criteria, Ratan et al. described focus, complexity, novelty, feasibility, and measurability for evaluating the effectiveness of research questions. 14
The PICOT and PEO frameworks are also used when developing research questions. 1 The following elements are addressed in these frameworks, PICOT: P-population/patients/problem, I-intervention or indicator being studied, C-comparison group, O-outcome of interest, and T-timeframe of the study; PEO: P-population being studied, E-exposure to preexisting conditions, and O-outcome of interest. 1 Research questions are also considered good if these meet the “FINERMAPS” framework: Feasible, Interesting, Novel, Ethical, Relevant, Manageable, Appropriate, Potential value/publishable, and Systematic. 14
As we indicated earlier, research questions and hypotheses that are not carefully formulated result in unethical studies or poor outcomes. To illustrate this, we provide some examples of ambiguous research question and hypotheses that result in unclear and weak research objectives in quantitative research ( Table 6 ) 16 and qualitative research ( Table 7 ) 17 , and how to transform these ambiguous research question(s) and hypothesis(es) into clear and good statements.
Variables | Unclear and weak statement (Statement 1) | Clear and good statement (Statement 2) | Points to avoid |
---|---|---|---|
Research question | Which is more effective between smoke moxibustion and smokeless moxibustion? | “Moreover, regarding smoke moxibustion versus smokeless moxibustion, it remains unclear which is more effective, safe, and acceptable to pregnant women, and whether there is any difference in the amount of heat generated.” | 1) Vague and unfocused questions |
2) Closed questions simply answerable by yes or no | |||
3) Questions requiring a simple choice | |||
Hypothesis | The smoke moxibustion group will have higher cephalic presentation. | “Hypothesis 1. The smoke moxibustion stick group (SM group) and smokeless moxibustion stick group (-SLM group) will have higher rates of cephalic presentation after treatment than the control group. | 1) Unverifiable hypotheses |
Hypothesis 2. The SM group and SLM group will have higher rates of cephalic presentation at birth than the control group. | 2) Incompletely stated groups of comparison | ||
Hypothesis 3. There will be no significant differences in the well-being of the mother and child among the three groups in terms of the following outcomes: premature birth, premature rupture of membranes (PROM) at < 37 weeks, Apgar score < 7 at 5 min, umbilical cord blood pH < 7.1, admission to neonatal intensive care unit (NICU), and intrauterine fetal death.” | 3) Insufficiently described variables or outcomes | ||
Research objective | To determine which is more effective between smoke moxibustion and smokeless moxibustion. | “The specific aims of this pilot study were (a) to compare the effects of smoke moxibustion and smokeless moxibustion treatments with the control group as a possible supplement to ECV for converting breech presentation to cephalic presentation and increasing adherence to the newly obtained cephalic position, and (b) to assess the effects of these treatments on the well-being of the mother and child.” | 1) Poor understanding of the research question and hypotheses |
2) Insufficient description of population, variables, or study outcomes |
a These statements were composed for comparison and illustrative purposes only.
b These statements are direct quotes from Higashihara and Horiuchi. 16
Variables | Unclear and weak statement (Statement 1) | Clear and good statement (Statement 2) | Points to avoid |
---|---|---|---|
Research question | Does disrespect and abuse (D&A) occur in childbirth in Tanzania? | How does disrespect and abuse (D&A) occur and what are the types of physical and psychological abuses observed in midwives’ actual care during facility-based childbirth in urban Tanzania? | 1) Ambiguous or oversimplistic questions |
2) Questions unverifiable by data collection and analysis | |||
Hypothesis | Disrespect and abuse (D&A) occur in childbirth in Tanzania. | Hypothesis 1: Several types of physical and psychological abuse by midwives in actual care occur during facility-based childbirth in urban Tanzania. | 1) Statements simply expressing facts |
Hypothesis 2: Weak nursing and midwifery management contribute to the D&A of women during facility-based childbirth in urban Tanzania. | 2) Insufficiently described concepts or variables | ||
Research objective | To describe disrespect and abuse (D&A) in childbirth in Tanzania. | “This study aimed to describe from actual observations the respectful and disrespectful care received by women from midwives during their labor period in two hospitals in urban Tanzania.” | 1) Statements unrelated to the research question and hypotheses |
2) Unattainable or unexplorable objectives |
a This statement is a direct quote from Shimoda et al. 17
The other statements were composed for comparison and illustrative purposes only.
CONSTRUCTING RESEARCH QUESTIONS AND HYPOTHESES
To construct effective research questions and hypotheses, it is very important to 1) clarify the background and 2) identify the research problem at the outset of the research, within a specific timeframe. 9 Then, 3) review or conduct preliminary research to collect all available knowledge about the possible research questions by studying theories and previous studies. 18 Afterwards, 4) construct research questions to investigate the research problem. Identify variables to be accessed from the research questions 4 and make operational definitions of constructs from the research problem and questions. Thereafter, 5) construct specific deductive or inductive predictions in the form of hypotheses. 4 Finally, 6) state the study aims . This general flow for constructing effective research questions and hypotheses prior to conducting research is shown in Fig. 1 .

Research questions are used more frequently in qualitative research than objectives or hypotheses. 3 These questions seek to discover, understand, explore or describe experiences by asking “What” or “How.” The questions are open-ended to elicit a description rather than to relate variables or compare groups. The questions are continually reviewed, reformulated, and changed during the qualitative study. 3 Research questions are also used more frequently in survey projects than hypotheses in experiments in quantitative research to compare variables and their relationships.
Hypotheses are constructed based on the variables identified and as an if-then statement, following the template, ‘If a specific action is taken, then a certain outcome is expected.’ At this stage, some ideas regarding expectations from the research to be conducted must be drawn. 18 Then, the variables to be manipulated (independent) and influenced (dependent) are defined. 4 Thereafter, the hypothesis is stated and refined, and reproducible data tailored to the hypothesis are identified, collected, and analyzed. 4 The hypotheses must be testable and specific, 18 and should describe the variables and their relationships, the specific group being studied, and the predicted research outcome. 18 Hypotheses construction involves a testable proposition to be deduced from theory, and independent and dependent variables to be separated and measured separately. 3 Therefore, good hypotheses must be based on good research questions constructed at the start of a study or trial. 12
In summary, research questions are constructed after establishing the background of the study. Hypotheses are then developed based on the research questions. Thus, it is crucial to have excellent research questions to generate superior hypotheses. In turn, these would determine the research objectives and the design of the study, and ultimately, the outcome of the research. 12 Algorithms for building research questions and hypotheses are shown in Fig. 2 for quantitative research and in Fig. 3 for qualitative research.

EXAMPLES OF RESEARCH QUESTIONS FROM PUBLISHED ARTICLES
- EXAMPLE 1. Descriptive research question (quantitative research)
- - Presents research variables to be assessed (distinct phenotypes and subphenotypes)
- “BACKGROUND: Since COVID-19 was identified, its clinical and biological heterogeneity has been recognized. Identifying COVID-19 phenotypes might help guide basic, clinical, and translational research efforts.
- RESEARCH QUESTION: Does the clinical spectrum of patients with COVID-19 contain distinct phenotypes and subphenotypes? ” 19
- EXAMPLE 2. Relationship research question (quantitative research)
- - Shows interactions between dependent variable (static postural control) and independent variable (peripheral visual field loss)
- “Background: Integration of visual, vestibular, and proprioceptive sensations contributes to postural control. People with peripheral visual field loss have serious postural instability. However, the directional specificity of postural stability and sensory reweighting caused by gradual peripheral visual field loss remain unclear.
- Research question: What are the effects of peripheral visual field loss on static postural control ?” 20
- EXAMPLE 3. Comparative research question (quantitative research)
- - Clarifies the difference among groups with an outcome variable (patients enrolled in COMPERA with moderate PH or severe PH in COPD) and another group without the outcome variable (patients with idiopathic pulmonary arterial hypertension (IPAH))
- “BACKGROUND: Pulmonary hypertension (PH) in COPD is a poorly investigated clinical condition.
- RESEARCH QUESTION: Which factors determine the outcome of PH in COPD?
- STUDY DESIGN AND METHODS: We analyzed the characteristics and outcome of patients enrolled in the Comparative, Prospective Registry of Newly Initiated Therapies for Pulmonary Hypertension (COMPERA) with moderate or severe PH in COPD as defined during the 6th PH World Symposium who received medical therapy for PH and compared them with patients with idiopathic pulmonary arterial hypertension (IPAH) .” 21
- EXAMPLE 4. Exploratory research question (qualitative research)
- - Explores areas that have not been fully investigated (perspectives of families and children who receive care in clinic-based child obesity treatment) to have a deeper understanding of the research problem
- “Problem: Interventions for children with obesity lead to only modest improvements in BMI and long-term outcomes, and data are limited on the perspectives of families of children with obesity in clinic-based treatment. This scoping review seeks to answer the question: What is known about the perspectives of families and children who receive care in clinic-based child obesity treatment? This review aims to explore the scope of perspectives reported by families of children with obesity who have received individualized outpatient clinic-based obesity treatment.” 22
- EXAMPLE 5. Relationship research question (quantitative research)
- - Defines interactions between dependent variable (use of ankle strategies) and independent variable (changes in muscle tone)
- “Background: To maintain an upright standing posture against external disturbances, the human body mainly employs two types of postural control strategies: “ankle strategy” and “hip strategy.” While it has been reported that the magnitude of the disturbance alters the use of postural control strategies, it has not been elucidated how the level of muscle tone, one of the crucial parameters of bodily function, determines the use of each strategy. We have previously confirmed using forward dynamics simulations of human musculoskeletal models that an increased muscle tone promotes the use of ankle strategies. The objective of the present study was to experimentally evaluate a hypothesis: an increased muscle tone promotes the use of ankle strategies. Research question: Do changes in the muscle tone affect the use of ankle strategies ?” 23
EXAMPLES OF HYPOTHESES IN PUBLISHED ARTICLES
- EXAMPLE 1. Working hypothesis (quantitative research)
- - A hypothesis that is initially accepted for further research to produce a feasible theory
- “As fever may have benefit in shortening the duration of viral illness, it is plausible to hypothesize that the antipyretic efficacy of ibuprofen may be hindering the benefits of a fever response when taken during the early stages of COVID-19 illness .” 24
- “In conclusion, it is plausible to hypothesize that the antipyretic efficacy of ibuprofen may be hindering the benefits of a fever response . The difference in perceived safety of these agents in COVID-19 illness could be related to the more potent efficacy to reduce fever with ibuprofen compared to acetaminophen. Compelling data on the benefit of fever warrant further research and review to determine when to treat or withhold ibuprofen for early stage fever for COVID-19 and other related viral illnesses .” 24
- EXAMPLE 2. Exploratory hypothesis (qualitative research)
- - Explores particular areas deeper to clarify subjective experience and develop a formal hypothesis potentially testable in a future quantitative approach
- “We hypothesized that when thinking about a past experience of help-seeking, a self distancing prompt would cause increased help-seeking intentions and more favorable help-seeking outcome expectations .” 25
- “Conclusion
- Although a priori hypotheses were not supported, further research is warranted as results indicate the potential for using self-distancing approaches to increasing help-seeking among some people with depressive symptomatology.” 25
- EXAMPLE 3. Hypothesis-generating research to establish a framework for hypothesis testing (qualitative research)
- “We hypothesize that compassionate care is beneficial for patients (better outcomes), healthcare systems and payers (lower costs), and healthcare providers (lower burnout). ” 26
- Compassionomics is the branch of knowledge and scientific study of the effects of compassionate healthcare. Our main hypotheses are that compassionate healthcare is beneficial for (1) patients, by improving clinical outcomes, (2) healthcare systems and payers, by supporting financial sustainability, and (3) HCPs, by lowering burnout and promoting resilience and well-being. The purpose of this paper is to establish a scientific framework for testing the hypotheses above . If these hypotheses are confirmed through rigorous research, compassionomics will belong in the science of evidence-based medicine, with major implications for all healthcare domains.” 26
- EXAMPLE 4. Statistical hypothesis (quantitative research)
- - An assumption is made about the relationship among several population characteristics ( gender differences in sociodemographic and clinical characteristics of adults with ADHD ). Validity is tested by statistical experiment or analysis ( chi-square test, Students t-test, and logistic regression analysis)
- “Our research investigated gender differences in sociodemographic and clinical characteristics of adults with ADHD in a Japanese clinical sample. Due to unique Japanese cultural ideals and expectations of women's behavior that are in opposition to ADHD symptoms, we hypothesized that women with ADHD experience more difficulties and present more dysfunctions than men . We tested the following hypotheses: first, women with ADHD have more comorbidities than men with ADHD; second, women with ADHD experience more social hardships than men, such as having less full-time employment and being more likely to be divorced.” 27
- “Statistical Analysis
- ( text omitted ) Between-gender comparisons were made using the chi-squared test for categorical variables and Students t-test for continuous variables…( text omitted ). A logistic regression analysis was performed for employment status, marital status, and comorbidity to evaluate the independent effects of gender on these dependent variables.” 27
EXAMPLES OF HYPOTHESIS AS WRITTEN IN PUBLISHED ARTICLES IN RELATION TO OTHER PARTS
- EXAMPLE 1. Background, hypotheses, and aims are provided
- “Pregnant women need skilled care during pregnancy and childbirth, but that skilled care is often delayed in some countries …( text omitted ). The focused antenatal care (FANC) model of WHO recommends that nurses provide information or counseling to all pregnant women …( text omitted ). Job aids are visual support materials that provide the right kind of information using graphics and words in a simple and yet effective manner. When nurses are not highly trained or have many work details to attend to, these job aids can serve as a content reminder for the nurses and can be used for educating their patients (Jennings, Yebadokpo, Affo, & Agbogbe, 2010) ( text omitted ). Importantly, additional evidence is needed to confirm how job aids can further improve the quality of ANC counseling by health workers in maternal care …( text omitted )” 28
- “ This has led us to hypothesize that the quality of ANC counseling would be better if supported by job aids. Consequently, a better quality of ANC counseling is expected to produce higher levels of awareness concerning the danger signs of pregnancy and a more favorable impression of the caring behavior of nurses .” 28
- “This study aimed to examine the differences in the responses of pregnant women to a job aid-supported intervention during ANC visit in terms of 1) their understanding of the danger signs of pregnancy and 2) their impression of the caring behaviors of nurses to pregnant women in rural Tanzania.” 28
- EXAMPLE 2. Background, hypotheses, and aims are provided
- “We conducted a two-arm randomized controlled trial (RCT) to evaluate and compare changes in salivary cortisol and oxytocin levels of first-time pregnant women between experimental and control groups. The women in the experimental group touched and held an infant for 30 min (experimental intervention protocol), whereas those in the control group watched a DVD movie of an infant (control intervention protocol). The primary outcome was salivary cortisol level and the secondary outcome was salivary oxytocin level.” 29
- “ We hypothesize that at 30 min after touching and holding an infant, the salivary cortisol level will significantly decrease and the salivary oxytocin level will increase in the experimental group compared with the control group .” 29
- EXAMPLE 3. Background, aim, and hypothesis are provided
- “In countries where the maternal mortality ratio remains high, antenatal education to increase Birth Preparedness and Complication Readiness (BPCR) is considered one of the top priorities [1]. BPCR includes birth plans during the antenatal period, such as the birthplace, birth attendant, transportation, health facility for complications, expenses, and birth materials, as well as family coordination to achieve such birth plans. In Tanzania, although increasing, only about half of all pregnant women attend an antenatal clinic more than four times [4]. Moreover, the information provided during antenatal care (ANC) is insufficient. In the resource-poor settings, antenatal group education is a potential approach because of the limited time for individual counseling at antenatal clinics.” 30
- “This study aimed to evaluate an antenatal group education program among pregnant women and their families with respect to birth-preparedness and maternal and infant outcomes in rural villages of Tanzania.” 30
- “ The study hypothesis was if Tanzanian pregnant women and their families received a family-oriented antenatal group education, they would (1) have a higher level of BPCR, (2) attend antenatal clinic four or more times, (3) give birth in a health facility, (4) have less complications of women at birth, and (5) have less complications and deaths of infants than those who did not receive the education .” 30
Research questions and hypotheses are crucial components to any type of research, whether quantitative or qualitative. These questions should be developed at the very beginning of the study. Excellent research questions lead to superior hypotheses, which, like a compass, set the direction of research, and can often determine the successful conduct of the study. Many research studies have floundered because the development of research questions and subsequent hypotheses was not given the thought and meticulous attention needed. The development of research questions and hypotheses is an iterative process based on extensive knowledge of the literature and insightful grasp of the knowledge gap. Focused, concise, and specific research questions provide a strong foundation for constructing hypotheses which serve as formal predictions about the research outcomes. Research questions and hypotheses are crucial elements of research that should not be overlooked. They should be carefully thought of and constructed when planning research. This avoids unethical studies and poor outcomes by defining well-founded objectives that determine the design, course, and outcome of the study.
Disclosure: The authors have no potential conflicts of interest to disclose.
Author Contributions:
- Conceptualization: Barroga E, Matanguihan GJ.
- Methodology: Barroga E, Matanguihan GJ.
- Writing - original draft: Barroga E, Matanguihan GJ.
- Writing - review & editing: Barroga E, Matanguihan GJ.
What Are the Elements of a Good Hypothesis?
Hero Images/Getty Images
- Scientific Method
- Chemical Laws
- Periodic Table
- Projects & Experiments
- Biochemistry
- Physical Chemistry
- Medical Chemistry
- Chemistry In Everyday Life
- Famous Chemists
- Activities for Kids
- Abbreviations & Acronyms
- Weather & Climate
- Ph.D., Biomedical Sciences, University of Tennessee at Knoxville
- B.A., Physics and Mathematics, Hastings College
A hypothesis is an educated guess or prediction of what will happen. In science, a hypothesis proposes a relationship between factors called variables. A good hypothesis relates an independent variable and a dependent variable. The effect on the dependent variable depends on or is determined by what happens when you change the independent variable . While you could consider any prediction of an outcome to be a type of hypothesis, a good hypothesis is one you can test using the scientific method. In other words, you want to propose a hypothesis to use as the basis for an experiment.
Cause and Effect or 'If, Then' Relationships
A good experimental hypothesis can be written as an if, then statement to establish cause and effect on the variables. If you make a change to the independent variable, then the dependent variable will respond. Here's an example of a hypothesis:
If you increase the duration of light, (then) corn plants will grow more each day.
The hypothesis establishes two variables, length of light exposure, and the rate of plant growth. An experiment could be designed to test whether the rate of growth depends on the duration of light. The duration of light is the independent variable, which you can control in an experiment . The rate of plant growth is the dependent variable, which you can measure and record as data in an experiment.
Key Points of Hypothesis
When you have an idea for a hypothesis, it may help to write it out in several different ways. Review your choices and select a hypothesis that accurately describes what you are testing.
- Does the hypothesis relate an independent and dependent variable? Can you identify the variables?
- Can you test the hypothesis? In other words, could you design an experiment that would allow you to establish or disprove a relationship between the variables?
- Would your experiment be safe and ethical?
- Is there a simpler or more precise way to state the hypothesis? If so, rewrite it.
What If the Hypothesis Is Incorrect?
It's not wrong or bad if the hypothesis is not supported or is incorrect. Actually, this outcome may tell you more about a relationship between the variables than if the hypothesis is supported. You may intentionally write your hypothesis as a null hypothesis or no-difference hypothesis to establish a relationship between the variables.
For example, the hypothesis:
The rate of corn plant growth does not depend on the duration of light.
This can be tested by exposing corn plants to different length "days" and measuring the rate of plant growth. A statistical test can be applied to measure how well the data support the hypothesis. If the hypothesis is not supported, then you have evidence of a relationship between the variables. It's easier to establish cause and effect by testing whether "no effect" is found. Alternatively, if the null hypothesis is supported, then you have shown the variables are not related. Either way, your experiment is a success.
Need more examples of how to write a hypothesis ? Here you go:
- If you turn out all the lights, you will fall asleep faster. (Think: How would you test it?)
- If you drop different objects, they will fall at the same rate.
- If you eat only fast food, then you will gain weight.
- If you use cruise control, then your car will get better gas mileage.
- If you apply a top coat, then your manicure will last longer.
- If you turn the lights on and off rapidly, then the bulb will burn out faster.
- What Is a Testable Hypothesis?
- What Are Examples of a Hypothesis?
- What Is a Hypothesis? (Science)
- Scientific Hypothesis Examples
- Six Steps of the Scientific Method
- Scientific Method Flow Chart
- Null Hypothesis Examples
- Understanding Simple vs Controlled Experiments
- Scientific Method Vocabulary Terms
- What Is a Controlled Experiment?
- What Is an Experimental Constant?
- Scientific Variable
- What Is the Difference Between a Control Variable and Control Group?
- DRY MIX Experiment Variables Acronym
- Random Error vs. Systematic Error
- The Role of a Controlled Variable in an Experiment

Writing a Strong Hypothesis Statement
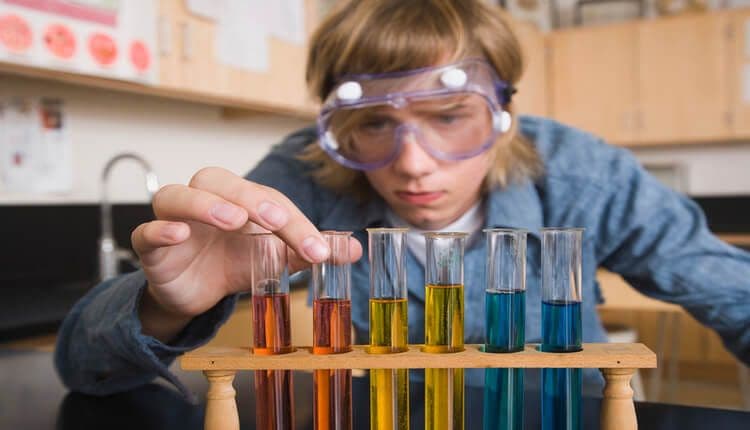
All good theses begins with a good thesis question. However, all great theses begins with a great hypothesis statement. One of the most important steps for writing a thesis is to create a strong hypothesis statement.
What is a hypothesis statement?
A hypothesis statement must be testable. If it cannot be tested, then there is no research to be done.
Simply put, a hypothesis statement posits the relationship between two or more variables. It is a prediction of what you think will happen in a research study. A hypothesis statement must be testable. If it cannot be tested, then there is no research to be done. If your thesis question is whether wildfires have effects on the weather, “wildfires create tornadoes” would be your hypothesis. However, a hypothesis needs to have several key elements in order to meet the criteria for a good hypothesis.
In this article, we will learn about what distinguishes a weak hypothesis from a strong one. We will also learn how to phrase your thesis question and frame your variables so that you are able to write a strong hypothesis statement and great thesis.
What is a hypothesis?
A hypothesis statement posits, or considers, a relationship between two variables.
As we mentioned above, a hypothesis statement posits or considers a relationship between two variables. In our hypothesis statement example above, the two variables are wildfires and tornadoes, and our assumed relationship between the two is a causal one (wildfires cause tornadoes). It is clear from our example above what we will be investigating: the relationship between wildfires and tornadoes.
A strong hypothesis statement should be:
- A prediction of the relationship between two or more variables
A hypothesis is not just a blind guess. It should build upon existing theories and knowledge . Tornadoes are often observed near wildfires once the fires reach a certain size. In addition, tornadoes are not a normal weather event in many areas; they have been spotted together with wildfires. This existing knowledge has informed the formulation of our hypothesis.
Depending on the thesis question, your research paper might have multiple hypothesis statements. What is important is that your hypothesis statement or statements are testable through data analysis, observation, experiments, or other methodologies.
Formulating your hypothesis
One of the best ways to form a hypothesis is to think about “if...then” statements.
Now that we know what a hypothesis statement is, let’s walk through how to formulate a strong one. First, you will need a thesis question. Your thesis question should be narrow in scope, answerable, and focused. Once you have your thesis question, it is time to start thinking about your hypothesis statement. You will need to clearly identify the variables involved before you can begin thinking about their relationship.
One of the best ways to form a hypothesis is to think about “if...then” statements . This can also help you easily identify the variables you are working with and refine your hypothesis statement. Let’s take a few examples.
If teenagers are given comprehensive sex education, there will be fewer teen pregnancies .
In this example, the independent variable is whether or not teenagers receive comprehensive sex education (the cause), and the dependent variable is the number of teen pregnancies (the effect).
If a cat is fed a vegan diet, it will die .
Here, our independent variable is the diet of the cat (the cause), and the dependent variable is the cat’s health (the thing impacted by the cause).
If children drink 8oz of milk per day, they will grow taller than children who do not drink any milk .
What are the variables in this hypothesis? If you identified drinking milk as the independent variable and growth as the dependent variable, you are correct. This is because we are guessing that drinking milk causes increased growth in the height of children.
Refining your hypothesis
Do not be afraid to refine your hypothesis throughout the process of formulation.
Do not be afraid to refine your hypothesis throughout the process of formulation. A strong hypothesis statement is clear, testable, and involves a prediction. While “testable” means verifiable or falsifiable, it also means that you are able to perform the necessary experiments without violating any ethical standards. Perhaps once you think about the ethics of possibly harming some cats by testing a vegan diet on them you might abandon the idea of that experiment altogether. However, if you think it is really important to research the relationship between a cat’s diet and a cat’s health, perhaps you could refine your hypothesis to something like this:
If 50% of a cat’s meals are vegan, the cat will not be able to meet its nutritional needs .
Another feature of a strong hypothesis statement is that it can easily be tested with the resources that you have readily available. While it might not be feasible to measure the growth of a cohort of children throughout their whole lives, you may be able to do so for a year. Then, you can adjust your hypothesis to something like this:
I f children aged 8 drink 8oz of milk per day for one year, they will grow taller during that year than children who do not drink any milk .
As you work to narrow down and refine your hypothesis to reflect a realistic potential research scope, don’t be afraid to talk to your supervisor about any concerns or questions you might have about what is truly possible to research.
What makes a hypothesis weak?
We noted above that a strong hypothesis statement is clear, is a prediction of a relationship between two or more variables, and is testable. We also clarified that statements, which are too general or specific are not strong hypotheses. We have looked at some examples of hypotheses that meet the criteria for a strong hypothesis, but before we go any further, let’s look at weak or bad hypothesis statement examples so that you can really see the difference.
Bad hypothesis 1: Diabetes is caused by witchcraft .
While this is fun to think about, it cannot be tested or proven one way or the other with clear evidence, data analysis, or experiments. This bad hypothesis fails to meet the testability requirement.
Bad hypothesis 2: If I change the amount of food I eat, my energy levels will change .
This is quite vague. Am I increasing or decreasing my food intake? What do I expect exactly will happen to my energy levels and why? How am I defining energy level? This bad hypothesis statement fails the clarity requirement.
Bad hypothesis 3: Japanese food is disgusting because Japanese people don’t like tourists .
This hypothesis is unclear about the posited relationship between variables. Are we positing the relationship between the deliciousness of Japanese food and the desire for tourists to visit? or the relationship between the deliciousness of Japanese food and the amount that Japanese people like tourists? There is also the problematic subjectivity of the assessment that Japanese food is “disgusting.” The problems are numerous.
The null hypothesis and the alternative hypothesis
The null hypothesis, quite simply, posits that there is no relationship between the variables.
What is the null hypothesis?
The hypothesis posits a relationship between two or more variables. The null hypothesis, quite simply, posits that there is no relationship between the variables. It is often indicated as H 0 , which is read as “h-oh” or “h-null.” The alternative hypothesis is the opposite of the null hypothesis as it posits that there is some relationship between the variables. The alternative hypothesis is written as H a or H 1 .
Let’s take our previous hypothesis statement examples discussed at the start and look at their corresponding null hypothesis.
H a : If teenagers are given comprehensive sex education, there will be fewer teen pregnancies .
H 0 : If teenagers are given comprehensive sex education, there will be no change in the number of teen pregnancies .
The null hypothesis assumes that comprehensive sex education will not affect how many teenagers get pregnant. It should be carefully noted that the null hypothesis is not always the opposite of the alternative hypothesis. For example:
If teenagers are given comprehensive sex education, there will be more teen pregnancies .
These are opposing statements that assume an opposite relationship between the variables: comprehensive sex education increases or decreases the number of teen pregnancies. In fact, these are both alternative hypotheses. This is because they both still assume that there is a relationship between the variables . In other words, both hypothesis statements assume that there is some kind of relationship between sex education and teen pregnancy rates. The alternative hypothesis is also the researcher’s actual predicted outcome, which is why calling it “alternative” can be confusing! However, you can think of it this way: our default assumption is the null hypothesis, and so any possible relationship is an alternative to the default.
Step-by-step sample hypothesis statements
Now that we’ve covered what makes a hypothesis statement strong, how to go about formulating a hypothesis statement, refining your hypothesis statement, and the null hypothesis, let’s put it all together with some examples. The table below shows a breakdown of how we can take a thesis question, identify the variables, create a null hypothesis, and finally create a strong alternative hypothesis.
|
|
|
|
Does the quality of sex education in public schools impact teen pregnancy rates? | Comprehensive sex education in public schools will lower teen pregnancy rates | The quality of sex education in public schools has no effect on teen pregnancy rates | |
Do wildfires that burn for more than 2 weeks have an impact on local weather systems? | Wildfires that burn for more than two weeks cause tornadoes because the heat they give off impacts wind patterns | Wildfires have no impact on local weather systems | |
Will a cat remain in good health on a vegan diet? | A cat’s health will suffer if it is only fed a vegan diet because cats are obligate carnivores | A cat’s diet has no impact on its health | |
Does walking for 30 minutes a day impact human health? | Walking for 30 minutes a day will improve cardiovascular health and brain function in humans | Walking for 30 minutes a day will neither improve or harm human health |
Once you have formulated a solid thesis question and written a strong hypothesis statement, you are ready to begin your thesis in earnest. Check out our site for more tips on writing a great thesis and information on thesis proofreading and editing services.
Editor’s pick
Get free updates.
Subscribe to our newsletter for regular insights from the research and publishing industry!
Review Checklist
Start with a clear thesis question
Think about “if-then” statements to identify your variables and the relationship between them
Create a null hypothesis
Formulate an alternative hypothesis using the variables you have identified
Make sure your hypothesis clearly posits a relationship between variables
Make sure your hypothesis is testable considering your available time and resources
What makes a hypothesis strong? +
A hypothesis is strong when it is testable, clear, and identifies a potential relationship between two or more variables.
What makes a hypothesis weak? +
A hypothesis is weak when it is too specific or too general, or does not identify a clear relationship between two or more variables.
What is the null hypothesis? +
The null hypothesis posits that the variables you have identified have no relationship.
Have a language expert improve your writing
Run a free plagiarism check in 10 minutes, automatically generate references for free.
- Knowledge Base
- Methodology
- How to Write a Strong Hypothesis | Guide & Examples
How to Write a Strong Hypothesis | Guide & Examples
Published on 6 May 2022 by Shona McCombes .
A hypothesis is a statement that can be tested by scientific research. If you want to test a relationship between two or more variables, you need to write hypotheses before you start your experiment or data collection.
Table of contents
What is a hypothesis, developing a hypothesis (with example), hypothesis examples, frequently asked questions about writing hypotheses.
A hypothesis states your predictions about what your research will find. It is a tentative answer to your research question that has not yet been tested. For some research projects, you might have to write several hypotheses that address different aspects of your research question.
A hypothesis is not just a guess – it should be based on existing theories and knowledge. It also has to be testable, which means you can support or refute it through scientific research methods (such as experiments, observations, and statistical analysis of data).
Variables in hypotheses
Hypotheses propose a relationship between two or more variables . An independent variable is something the researcher changes or controls. A dependent variable is something the researcher observes and measures.
In this example, the independent variable is exposure to the sun – the assumed cause . The dependent variable is the level of happiness – the assumed effect .
Prevent plagiarism, run a free check.
Step 1: ask a question.
Writing a hypothesis begins with a research question that you want to answer. The question should be focused, specific, and researchable within the constraints of your project.
Step 2: Do some preliminary research
Your initial answer to the question should be based on what is already known about the topic. Look for theories and previous studies to help you form educated assumptions about what your research will find.
At this stage, you might construct a conceptual framework to identify which variables you will study and what you think the relationships are between them. Sometimes, you’ll have to operationalise more complex constructs.
Step 3: Formulate your hypothesis
Now you should have some idea of what you expect to find. Write your initial answer to the question in a clear, concise sentence.
Step 4: Refine your hypothesis
You need to make sure your hypothesis is specific and testable. There are various ways of phrasing a hypothesis, but all the terms you use should have clear definitions, and the hypothesis should contain:
- The relevant variables
- The specific group being studied
- The predicted outcome of the experiment or analysis
Step 5: Phrase your hypothesis in three ways
To identify the variables, you can write a simple prediction in if … then form. The first part of the sentence states the independent variable and the second part states the dependent variable.
In academic research, hypotheses are more commonly phrased in terms of correlations or effects, where you directly state the predicted relationship between variables.
If you are comparing two groups, the hypothesis can state what difference you expect to find between them.
Step 6. Write a null hypothesis
If your research involves statistical hypothesis testing , you will also have to write a null hypothesis. The null hypothesis is the default position that there is no association between the variables. The null hypothesis is written as H 0 , while the alternative hypothesis is H 1 or H a .
Research question | Hypothesis | Null hypothesis |
---|---|---|
What are the health benefits of eating an apple a day? | Increasing apple consumption in over-60s will result in decreasing frequency of doctor’s visits. | Increasing apple consumption in over-60s will have no effect on frequency of doctor’s visits. |
Which airlines have the most delays? | Low-cost airlines are more likely to have delays than premium airlines. | Low-cost and premium airlines are equally likely to have delays. |
Can flexible work arrangements improve job satisfaction? | Employees who have flexible working hours will report greater job satisfaction than employees who work fixed hours. | There is no relationship between working hour flexibility and job satisfaction. |
How effective is secondary school sex education at reducing teen pregnancies? | Teenagers who received sex education lessons throughout secondary school will have lower rates of unplanned pregnancy than teenagers who did not receive any sex education. | Secondary school sex education has no effect on teen pregnancy rates. |
What effect does daily use of social media have on the attention span of under-16s? | There is a negative correlation between time spent on social media and attention span in under-16s. | There is no relationship between social media use and attention span in under-16s. |
Hypothesis testing is a formal procedure for investigating our ideas about the world using statistics. It is used by scientists to test specific predictions, called hypotheses , by calculating how likely it is that a pattern or relationship between variables could have arisen by chance.
A hypothesis is not just a guess. It should be based on existing theories and knowledge. It also has to be testable, which means you can support or refute it through scientific research methods (such as experiments, observations, and statistical analysis of data).
A research hypothesis is your proposed answer to your research question. The research hypothesis usually includes an explanation (‘ x affects y because …’).
A statistical hypothesis, on the other hand, is a mathematical statement about a population parameter. Statistical hypotheses always come in pairs: the null and alternative hypotheses. In a well-designed study , the statistical hypotheses correspond logically to the research hypothesis.
Cite this Scribbr article
If you want to cite this source, you can copy and paste the citation or click the ‘Cite this Scribbr article’ button to automatically add the citation to our free Reference Generator.
McCombes, S. (2022, May 06). How to Write a Strong Hypothesis | Guide & Examples. Scribbr. Retrieved 26 August 2024, from https://www.scribbr.co.uk/research-methods/hypothesis-writing/
Is this article helpful?
Shona McCombes
Other students also liked, operationalisation | a guide with examples, pros & cons, what is a conceptual framework | tips & examples, a quick guide to experimental design | 5 steps & examples.
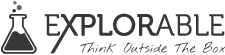
- Foundations
- Write Paper
Search form
- Experiments
- Anthropology
- Self-Esteem
- Social Anxiety

- Experiments >
- Cause and Effect
Establishing Cause and Effect
Cause and effect is one of the most commonly misunderstood concepts in science and is often misused by lawyers, the media, politicians and even scientists themselves, in an attempt to add legitimacy to research.
This article is a part of the guide:
- Experimental Research
- Pretest-Posttest
- Third Variable
- Research Bias
- Independent Variable
Browse Full Outline
- 1 Experimental Research
- 2.1 Independent Variable
- 2.2 Dependent Variable
- 2.3 Controlled Variables
- 2.4 Third Variable
- 3.1 Control Group
- 3.2 Research Bias
- 3.3.1 Placebo Effect
- 3.3.2 Double Blind Method
- 4.1 Randomized Controlled Trials
- 4.2 Pretest-Posttest
- 4.3 Solomon Four Group
- 4.4 Between Subjects
- 4.5 Within Subject
- 4.6 Repeated Measures
- 4.7 Counterbalanced Measures
- 4.8 Matched Subjects
The basic principle of causality is determining whether the results and trends seen in an experiment are actually caused by the manipulation or whether some other factor may underlie the process.
Unfortunately, the media and politicians often jump upon scientific results and proclaim that it conveniently fits their beliefs and policies. Some scientists, fixated upon 'proving' that their view of the world is correct, leak their results to the press before allowing the peer review process to check and validate their work.
Some examples of this are rife in alternative therapy, when a group of scientists announces that they have found the next healthy superfood or that a certain treatment cured swine flu. Many of these claims deviate from the scientific process and pay little heed to cause and effect, diluting the claims of genuine researchers in the field.
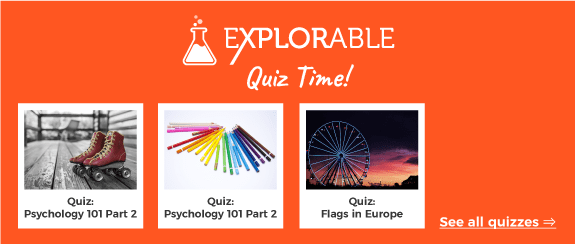
What is Cause and Effect? - The Temporal Issue
The key principle of establishing cause and effect is proving that the effects seen in the experiment happened after the cause.
This seems to be an extremely obvious statement, but that is not always the case. Natural phenomena are complicated and intertwined, often overlapping and making it difficult to establish a natural order. Think about it this way: in an experiment to study the effects of depression upon alcohol consumption, researchers find that people who suffer from higher levels of depression drink more, and announce that this correlation shows that depression drives people to drink.
However, is this necessarily the case? Depression could be the cause that makes people drink more but it is equally possible that heavy consumption of alcohol, a depressant, makes people more depressed. This type of classic 'chicken and egg' argument makes establishing causality one of the most difficult aspects of scientific research . It is also one of the most important factors, because it can misdirect scientists. It also leaves the research open to manipulation by interest groups, who will take the results and proclaim them as a truth.
With the above example, an alcoholic drink manufacturer could use the second interpretation to claim that alcohol is not a factor in depression and that the responsibility is upon society to ensure that people do not become depressed. An anti-alcohol group, on the other hand, could claim that alcohol is harmful and use the results to lobby for harsher drinking laws. The same research leads to two different interpretations and, the answer given to the media can depend upon who funds the work.
Unfortunately, most of the general public are not scientists and cannot be expected to filter every single news item that they read for quality or delve into which group funded research. Even respected and trusted newspapers, journals and internet resources can fall into the causality trap, so marketing groups can influence perceptions.
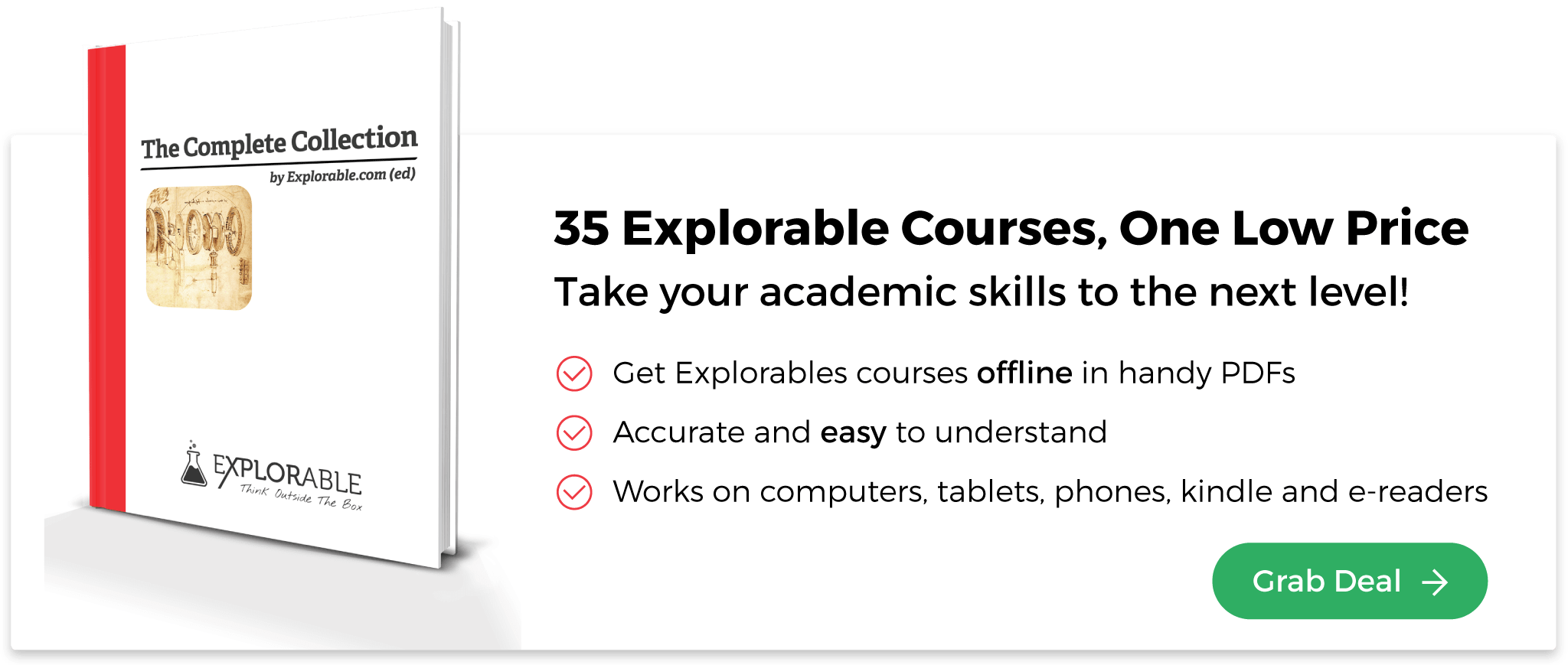
What is Cause and Effect? - The Danger of Alternative Explanations
The other problem with causality is that a researcher cannot always guarantee that their particular manipulation of a variable was the sole reason for the perceived trends and correlation.
In a complex experiment, it is often difficult to isolate and neutralize the influence of confounding variables . This makes it exceptionally difficult for the researcher to state that their treatment is the sole cause, so any research program must contain measures to establish the cause and effect relationship.
In the physical sciences, such as physics and chemistry, it is fairly easy to establish causality, because a good experimental design can neutralize any potentially confounding variables. Sociology, at the other extreme, is exceptionally prone to causality issues, because individual humans and social groups vary so wildly and are subjected to a wide range of external pressures and influences.
For results to have any meaning, a researcher must make causality the first priority, simply because it can have such a devastating effect upon validity. Most experiments with some validity issues can be salvaged, and produce some usable data. An experiment with no established cause and effect, on the other hand, will be practically useless and a waste of resources.
How to Establish Cause and Effect
The first thing to remember with causality, especially in the non-physical sciences, is that it is impossible to establish complete causality.
However, the magical figure of 100% proof of causality is what every researcher must strive for, to ensure that a group of their peers will accept the results. The only way to do this is through a strong and well-considered experimental design, often containing pilot studies to establish cause and effect before plowing on with a complex and expensive study.
The temporal factor is usually the easiest aspect to neutralize, simply because most experiments involve administering a treatment and then observing the effects, giving a linear temporal relationship. In experiments that use historical data, as with the drinking/depression example, this can be a little more complex. Most researchers performing such a program will supplement it with a series of individual case studies, and interviewing a selection of the participants , in depth, will allow the researchers to find the order of events.
For example, interviewing a sample of the depressed heavy drinkers will establish whether they felt that they were depressed before they started drinking or if the depression came later. The process of establishing cause and effect is a matter of ensuring that the potential influence of 'missing variables' is minimized.
One notable example, by the researchers Balnaves and Caputi, looked at the academic performance of university students and attempted to find a correlation with age. Indeed, they found that older, more mature students performed significantly better. However, as they pointed out, you cannot simply say that age causes the effect of making people into better students. Such a simplistic assumption is called a spurious relationship, the process of 'leaping to conclusions.'
In fact, there is a whole host of reasons why a mature student performs better: they have more life experience and confidence, and many feel that it is their last chance to succeed; my graduation year included a 75-year-old man, and nobody studied harder! Mature students may well have made a great financial sacrifice, so they are a little more determined to succeed. Establishing cause and effect is extremely difficult in this case, so the researchers interpreted the results very carefully.
Another example is the idea that because people who eat a lot of extra virgin olive oil live for longer, olive oil makes people live longer. While there is some truth behind this, you have to remember that most regular olive oil eaters also eat a Mediterranean diet, have active lifestyles, and generally less stress. These also have a strong influence, so any such research program should include studies into the effect of these - this is why a research program is not always a single experiment but often a series of experiments.
History Threats and Their Influence Upon Cause and Effect
One of the biggest threats to internal validity through incorrect application of cause and effect is the 'history' threat.
This is where another event actually caused the effect noticed, rather than your treatment or manipulation. Most researchers perform a pre-test upon a group, administer the treatment and then measure the post-test results ( pretest-posttest-design ). If the results are better, it is easy to assume that the treatment caused the result, but this is not necessarily the case.
For example, take the case of an educational researcher wishing to measure the effect of a new teaching method upon the mathematical aptitude of students. They pre-test, teach the new program for a few months and then posttest. Results improve, and they proclaim that their program works.
However, the research was ruined by a historical threat: during the course of the research, a major television network released a new educational series called 'Maths made Easy,' which most of the students watched. This influenced the results and compromised the validity of the experiment.
Fortunately, the solution to this problem is easy: if the researcher uses a two group pretest-posttest design with a control group , the control group will be equally influenced by the historical event, so the researcher can still establish a good baseline. There are a number of other 'single group' threats, but establishing a good control driven study largely eliminates these threats to causality.
Social Threats and Their Influence Upon Cause and Effect
Social threats are a big problem for social researchers simply because they are one of the most difficult of the threats to minimize. These types of threats arise from issues within the participant groups or the researchers themselves. In an educational setting, with two groups of children, one treated and one not, there are a number of potential issues.
- Diffusion or Imitation of Treatment: With this threat, information travels between groups and smoothes out any differences in the results. In a school, for example, students mix outside classes and may swap information or coach the control group about some of the great new study techniques that they have learned. It is practically impossible and extremely unfair to expect students not to mix, so this particular threat is always an issue.
- Compensatory Rivalry: Quite simply, this is where the control group becomes extremely jealous of the treatment group. They might think that the research is unfair, because their fellow students are earning better grades. As a result, they try much harder to show that they are equally as clever, reducing the difference between the two groups.
- Demoralization and Resentment: This jealousy may have the opposite effect and manifest as a built up resentment that the other group is receiving favorable treatment. The control group , quite simply, gives up and does not bother trying and their grades plummet. This makes the educational program appear to be much more successful than it really is.
- Compensatory Equalization of Treatment: This type of social threat arises from the attitude of the researchers or external contributors. If, for example, teachers and parents perceive that there is some unfairness in the system, they might try to compensate, by giving extra tuition or access to better teaching resources. This can easily cause compensatory rivalry, too, if a teacher spurs on the control group to try harder and outdo the others.
These social effects are extremely difficult to minimize without creating other threats to internal validity .
For example, using different schools is one idea, but this can lead to other internal validity issues, especially because the participant groups cannot be randomized. In reality, this is why most social research programs incorporate a variety of different methods and include more than one experiment, to establish the potential level of these threats and incorporate them into the interpretation of the data.
Cause and Effect - The Danger of Multiple Group Threats
Multiple group threats are a danger to causality caused by differences between two or more groups of participants. The main example of this is selection bias , or assignment bias, where the two groups are assigned unevenly, perhaps leaving one group with a larger proportion of high achievers. This will skew the results and mask the effects of the entire experiment.
While there are other types of multiple group threat, they are all subtypes of selection bias and involve the two groups receiving different treatment. If the groups are selected from different socio-economic backgrounds, or one has a much better teacher, this can skew the results. Without going into too much detail, the only way to reduce the influence of multiple group threats is through randomization , matched pairs designs or another assignment type.
As can be seen, establishing cause and effect is one of the most important factors in designing a robust research experiment. One of the best ways to learn about causality is through experience and analysis - every time you see some innovative research or findings in the media, think about what the results are trying to tell you and whether the researchers are justified in drawing their conclusions .
This does not have to be restricted to 'hard' science, because political researchers are the worst habitual offenders. Archaeology, economics and market research are other areas where cause and effect is important, so should provide some excellent examples of how to establish cause and effect.
- Psychology 101
- Flags and Countries
- Capitals and Countries
Martyn Shuttleworth (Sep 20, 2009). Establishing Cause and Effect. Retrieved Aug 28, 2024 from Explorable.com: https://explorable.com/cause-and-effect
You Are Allowed To Copy The Text
The text in this article is licensed under the Creative Commons-License Attribution 4.0 International (CC BY 4.0) .
This means you're free to copy, share and adapt any parts (or all) of the text in the article, as long as you give appropriate credit and provide a link/reference to this page.
That is it. You don't need our permission to copy the article; just include a link/reference back to this page. You can use it freely (with some kind of link), and we're also okay with people reprinting in publications like books, blogs, newsletters, course-material, papers, wikipedia and presentations (with clear attribution).
Want to stay up to date? Follow us!
Get all these articles in 1 guide.
Want the full version to study at home, take to school or just scribble on?
Whether you are an academic novice, or you simply want to brush up your skills, this book will take your academic writing skills to the next level.
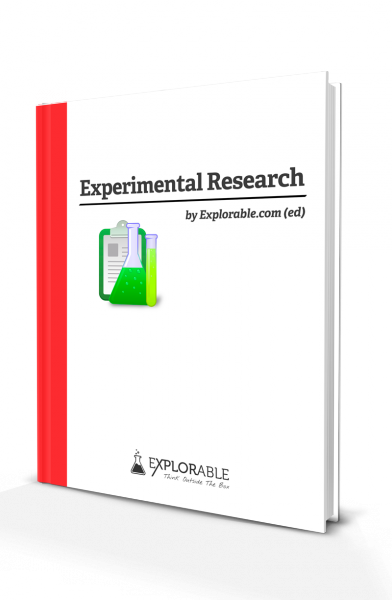
Download electronic versions: - Epub for mobiles and tablets - For Kindle here - For iBooks here - PDF version here
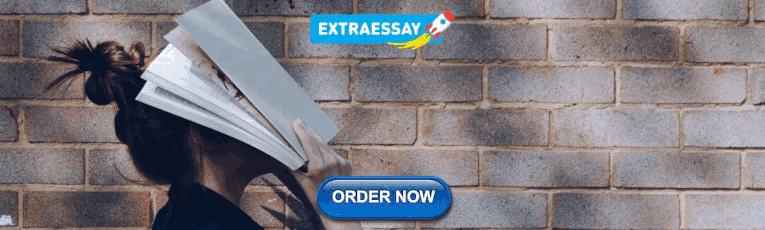
Save this course for later
Don't have time for it all now? No problem, save it as a course and come back to it later.
Footer bottom
- Privacy Policy
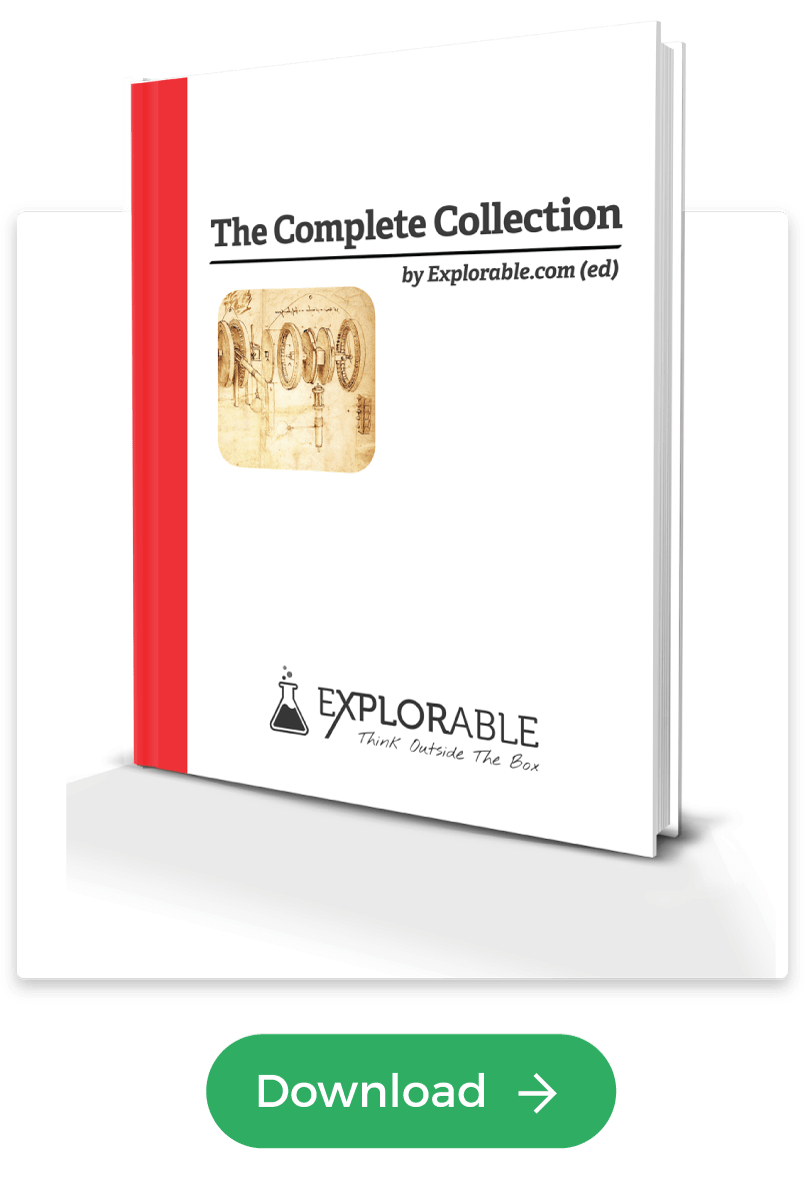
- Subscribe to our RSS Feed
- Like us on Facebook
- Follow us on Twitter
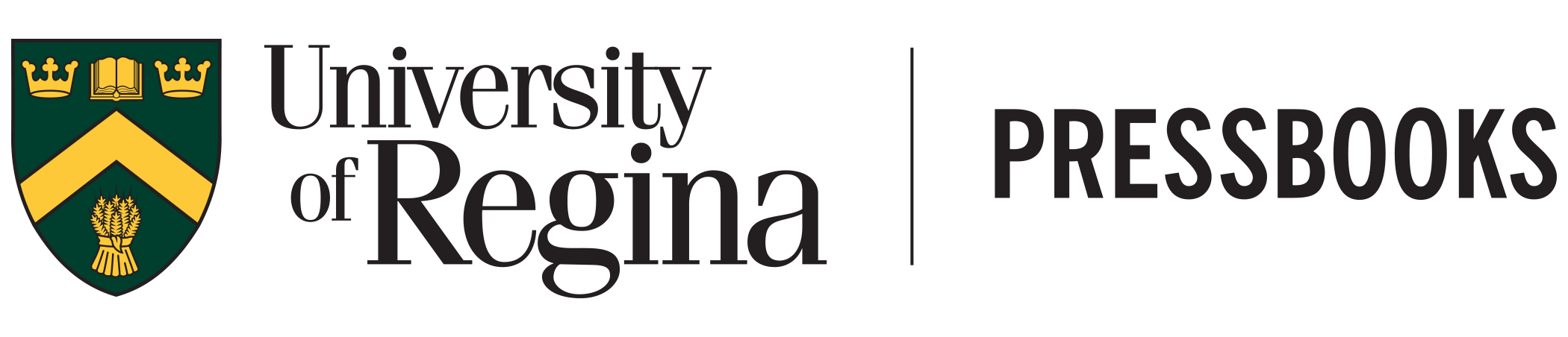
Want to create or adapt books like this? Learn more about how Pressbooks supports open publishing practices.
13 4.2 Causality
Learning objectives.
- Define and provide an example of idiographic and nomothetic causal explanations
- Describe the role of causality in quantitative research as compared to qualitative research
- Identify, define, and describe each of the main criteria for nomothetic causal explanations
- Describe the difference between and provide examples of independent, dependent, and control variables
- Define hypothesis, be able to state a clear hypothesis, and discuss the respective roles of quantitative and qualitative research when it comes to hypotheses
Most social scientific studies attempt to provide some kind of causal explanation. In other words, it is about cause and effect. A study on an intervention to prevent child abuse is trying to draw a connection between the intervention and changes in child abuse. Causality refers to the idea that one event, behavior, or belief will result in the occurrence of another, subsequent event, behavior, or belief. It seems simple, but you may be surprised to learn there is more than one way to explain how one thing causes another. How can that be? How could there be many ways to understand causality?
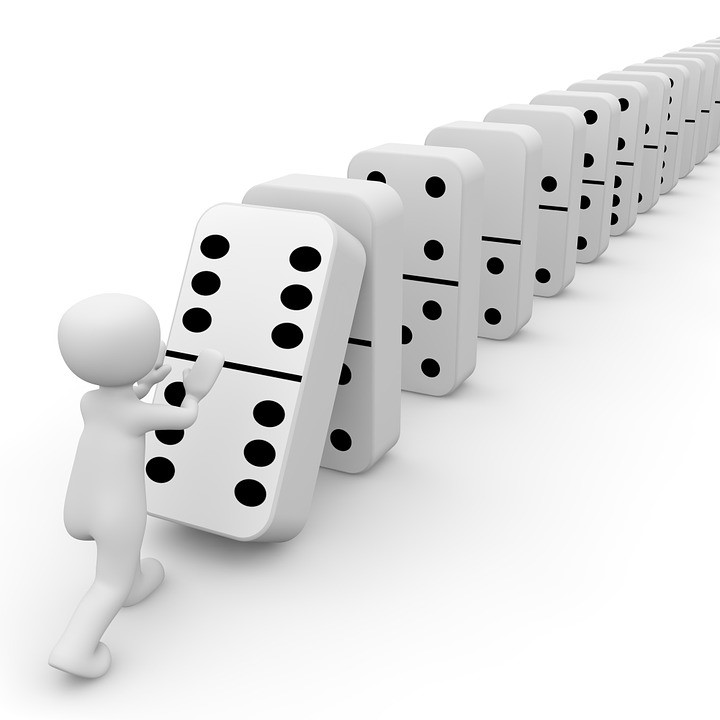
Think back to our chapter on paradigms, which were analytic lenses comprised of assumptions about the world. You’ll remember the positivist paradigm as the one that believes in objectivity and social constructionist paradigm as the one that believes in subjectivity. Both paradigms are correct, though incomplete, viewpoints on the social world and social science.
A researcher operating in the social constructionist paradigm would view truth as subjective. In causality, that means that in order to try to understand what caused what, we would need to report what people tell us. Well, that seems pretty straightforward, right? Well, what if two different people saw the same event from the exact same viewpoint and came up with two totally different explanations about what caused what? A social constructionist might say that both people are correct. There is not one singular truth that is true for everyone, but many truths created and shared by people.
When social constructionists engage in science, they are trying to establish one type of causality—idiographic causality. The word idiographic comes from the root word “idio” which means peculiar to one, personal, and distinct. An idiographic causal explanation means that you will attempt to explain or describe your phenomenon exhaustively, based on the subjective understandings of your participants. Idiographic causal explanations are intended to explain one particular context or phenomenon. These explanations are bound with the narratives people create about their lives and experience, and are embedded in a cultural, historical, and environmental context. Idiographic causal explanations are so powerful because they convey a deep understanding of a phenomenon and its context. From a social constructionist perspective, the truth is messy. Idiographic research involves finding patterns and themes in the causal themes established by your research participants.
If that doesn’t sound like what you normally think of as “science,” you’re not alone. Although the ideas behind idiographic research are quite old in philosophy, they were only applied to the sciences at the start of the last century. If we think of famous Western scientists like Newton or Darwin, they never saw truth as subjective. They operated with the understanding there were objectively true laws of science that were applicable in all situations. In their time, another paradigm–the positivist paradigm–was dominant and continues its dominance today. When positivists try to establish causality, they are like Newton and Darwin, trying to come up with a broad, sweeping explanation that is universally true for all people. This is the hallmark of a nomothetic causal explanation . The word nomothetic is derived from the root word “nomo” which means related to a law or legislative, and “thetic” which means something that establishes. Put the root words together and it means something that is establishing a law, or in our case, a universal explanation.
Nomothetic causal explanations are incredibly powerful. They allow scientists to make predictions about what will happen in the future, with a certain margin of error. Moreover, they allow scientists to generalize —that is, make claims about a large population based on a smaller sample of people or items. Generalizing is important. We clearly do not have time to ask everyone their opinion on a topic, nor do we have the ability to look at every interaction in the social world. We need a type of causal explanation that helps us predict and estimate truth in all situations.
If these still seem like obscure philosophy terms, let’s consider an example. Imagine you are working for a community-based non-profit agency serving people with disabilities. You are putting together a report to help lobby the state government for additional funding for community support programs, and you need to support your argument for additional funding at your agency. If you looked at nomothetic research, you might learn how previous studies have shown that, in general, community-based programs like yours are linked with better health and employment outcomes for people with disabilities. Nomothetic research seeks to explain that community-based programs are better for everyone with disabilities. If you looked at idiographic research, you would get stories and experiences of people in community-based programs. These individual stories are full of detail about the lived experience of being in a community-based program. Using idiographic research, you can understand what it’s like to be a person with a disability and then communicate that to the state government. For example, a person might say “I feel at home when I’m at this agency because they treat me like a family member” or “this is the agency that helped me get my first paycheck.”
Neither kind of causal explanation is better than the other. A decision to conduct idiographic research means that you will attempt to explain or describe your phenomenon exhaustively, attending to cultural context and subjective interpretations. A decision to conduct nomothetic research, on the other hand, means that you will try to explain what is true for everyone and predict what will be true in the future. In short, idiographic explanations have greater depth, and nomothetic explanations have greater breadth. More importantly, social workers understand the value of both approaches to understanding the social world. A social worker helping a client with substance abuse issues seeks idiographic knowledge when they ask about that client’s life story, investigate their unique physical environment, or probe how they understand their addiction. At the same time, a social worker also uses nomothetic knowledge to guide their interventions. Nomothetic research may help guide them to minimize risk factors and maximize protective factors or use an evidence-based therapy, relying on knowledge about what in general helps people with substance abuse issues.
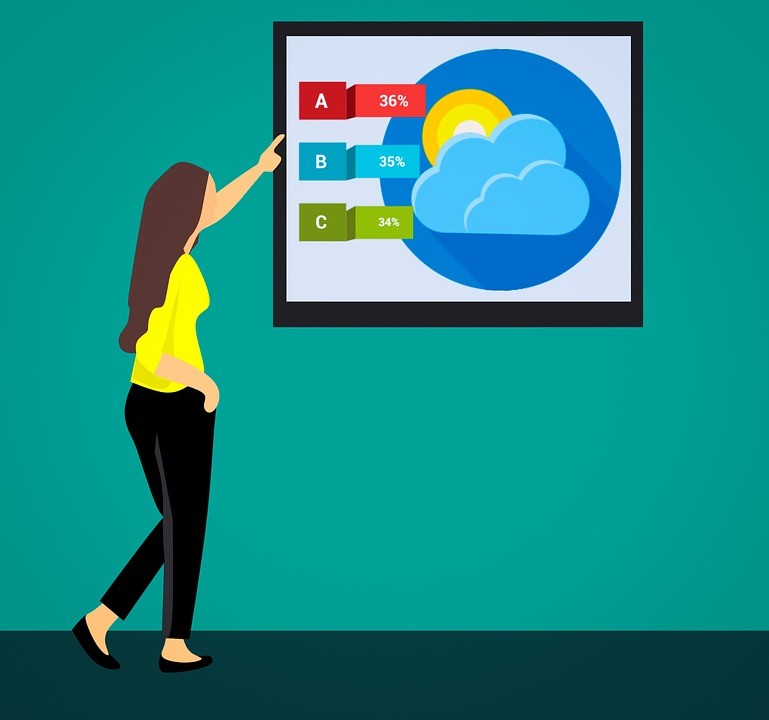
Nomothetic causal explanations
If you are trying to generalize about causality, or create a nomothetic causal explanation, then the rest of these statements are likely to be true: you will use quantitative methods, reason deductively, and engage in explanatory research. How can we make that prediction? Let’s take it part by part.
Because nomothetic causal explanations try to generalize, they must be able to reduce phenomena to a universal language, mathematics. Mathematics allows us to precisely measure, in universal terms, phenomena in the social world. Because explanatory researchers want a clean “x causes y” explanation, they need to use the universal language of mathematics to achieve their goal. That’s why nomothetic causal explanations use quantitative methods. It’s helpful to note that not all quantitative studies are explanatory. For example, a descriptive study could reveal the number of people without homes in your county, though it won’t tell you why they are homeless. But nearly all explanatory studies are quantitative.
What we’ve been talking about here is an association between variables. When one variable precedes or predicts another, we have what researchers call independent and dependent variables. Two variables can be associated without having a causal relationship. However, when certain conditions are met (which we describe later in this chapter), the independent variable is considered as a “ cause ” of the dependent variable. For our example on spanking and aggressive behavior, spanking would be the independent variable and aggressive behavior addiction would be the dependent variable. In causal explanations, the independent variable is the cause, and the dependent variable is the effect. Dependent variables depend on independent variables. If all of that gets confusing, just remember this graphical depiction:
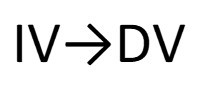
The strength of the association between the independent variable and dependent variable is another important factor to take into consideration when attempting to make causal claims when your research approach is nomothetic. In this context, strength refers to statistical significance . When the association between two variables is shown to be statistically significant, we can have greater confidence that the data from our sample reflect a true association between those variables in the target population. Statistical significance is usually represented in statistics as the p- value . Generally a p -value of .05 or less indicates the association between the two variables is statistically significant.
A hypothesis is a statement describing a researcher’s expectation regarding the research findings. Hypotheses in quantitative research are nomothetic causal explanations that the researcher expects to demonstrate. Hypotheses are written to describe the expected association between the independent and dependent variables. Your prediction should be taken from a theory or model of the social world. For example, you may hypothesize that treating clinical clients with warmth and positive regard is likely to help them achieve their therapeutic goals. That hypothesis would be using the humanistic theories of Carl Rogers. Using previous theories to generate hypotheses is an example of deductive research. If Rogers’ theory of unconditional positive regard is accurate, your hypothesis should be true.
Let’s consider a couple of examples. In research on sexual harassment (Uggen & Blackstone, 2004), one might hypothesize, based on feminist theories of sexual harassment, that more females than males will experience specific sexually harassing behaviors. What is the causal explanation being predicted here? Which is the independent and which is the dependent variable? In this case, we hypothesized that a person’s gender (independent variable) would predict their likelihood to experience sexual harassment (dependent variable).
Sometimes researchers will hypothesize that an association will take a specific direction. As a result, an increase or decrease in one area might be said to cause an increase or decrease in another. For example, you might choose to study the association between age and support for legalization of marijuana. Perhaps you’ve taken a sociology class and, based on the theories you’ve read, you hypothesize that age is negatively related to support for marijuana legalization. In fact, there are empirical data that support this hypothesis. Gallup has conducted research on this very question since the 1960s (Carroll, 2005). What have you just hypothesized? You have hypothesized that as people get older, the likelihood of their supporting marijuana legalization decreases. Thus, as age (your independent variable) moves in one direction (up), support for marijuana legalization (your dependent variable) moves in another direction (down). So, positive associations involve two variables going in the same direction and negative associations involve two variables going in opposite directions. If writing hypotheses feels tricky, it is sometimes helpful to draw them out and depict each of the two hypotheses we have just discussed.
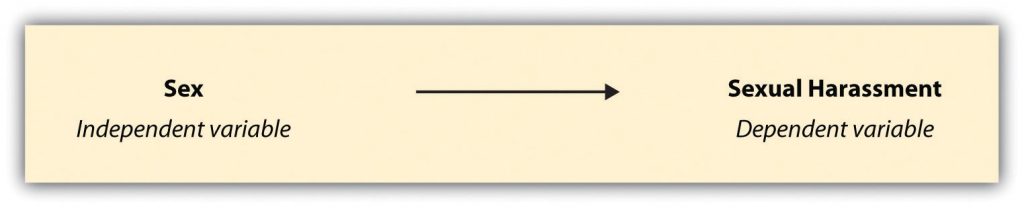
It’s important to note that once a study starts, it is unethical to change your hypothesis to match the data that you found. For example, what happens if you conduct a study to test the hypothesis from Figure 4.3 on support for marijuana legalization, but you find no association between age and support for legalization? It means that your hypothesis was wrong, but that’s still valuable information. It would challenge what the existing literature says on your topic, demonstrating that more research needs to be done to figure out the factors that impact support for marijuana legalization. Don’t be embarrassed by negative results, and definitely don’t change your hypothesis to make it appear correct all along!
Establishing causality in nomothetic research
Let’s say you conduct your study and you find evidence that supports your hypothesis, as age increases, support for marijuana legalization decreases. Success! Causal explanation complete, right? Not quite. You’ve only established one of the criteria for causality. The main criteria for causality have to do with covariation, plausibility, temporality, and spuriousness. In our example from Figure 4.3, we have established only one criteria—covariation. When variables covary , they vary together. Both age and support for marijuana legalization vary in our study. Our sample contains people of varying ages and varying levels of support for marijuana legalization and they vary together in a patterned way–when age increases, support for legalization decreases.
Just because there might be some correlation between two variables does not mean that a causal explanation between the two is really plausible. Plausibility means that in order to make the claim that one event, behavior, or belief causes another, the claim has to make sense. It makes sense that people from previous generations would have different attitudes towards marijuana than younger generations. People who grew up in the time of Reefer Madness or the hippies may hold different views than those raised in an era of legalized medicinal and recreational use of marijuana.
Once we’ve established that there is a plausible association between the two variables, we also need to establish that the cause happened before the effect, the criterion of temporality . A person’s age is a quality that appears long before any opinions on drug policy, so temporally the cause comes before the effect. It wouldn’t make any sense to say that support for marijuana legalization makes a person’s age increase. Even if you could predict someone’s age based on their support for marijuana legalization, you couldn’t say someone’s age was caused by their support for legalization.
Finally, scientists must establish nonspuriousness. A spurious association is one in which an association between two variables appears to be causal but can in fact be explained by some third variable. For example, we could point to the fact that older cohorts are less likely to have used marijuana. Maybe it is actually use of marijuana that leads people to be more open to legalization, not their age. This is often referred to as the third variable problem, where a seemingly true causal explanation is actually caused by a third variable not in the hypothesis. In this example, the association between age and support for legalization could be more about having tried marijuana than the age of the person.
Quantitative researchers are sensitive to the effects of potentially spurious associations. They are an important form of critique of scientific work. As a result, they will often measure these third variables in their study, so they can control for their effects. These are called control variables , and they refer to variables whose effects are controlled for mathematically in the data analysis process. Control variables can be a bit confusing, but think about it as an argument between you, the researcher, and a critic.
Researcher: “The older a person is, the less likely they are to support marijuana legalization.” Critic: “Actually, it’s more about whether a person has used marijuana before. That is what truly determines whether someone supports marijuana legalization.” Researcher: “Well, I measured previous marijuana use in my study and mathematically controlled for its effects in my analysis. The association between age and support for marijuana legalization is still statistically significant and is the most important association here.”
Let’s consider a few additional, real-world examples of spuriousness. Did you know, for example, that high rates of ice cream sales have been shown to cause drowning? Of course, that’s not really true, but there is a positive association between the two. In this case, the third variable that causes both high ice cream sales and increased deaths by drowning is time of year, as the summer season sees increases in both (Babbie, 2010). Here’s another good one: it is true that as the salaries of Presbyterian ministers in Massachusetts rise, so too does the price of rum in Havana, Cuba. Well, duh, you might be saying to yourself. Everyone knows how much ministers in Massachusetts love their rum, right? Not so fast. Both salaries and rum prices have increased, true, but so has the price of just about everything else (Huff & Geis, 1993).
Finally, research shows that the more firefighters present at a fire, the more damage is done at the scene. What this statement leaves out, of course, is that as the size of a fire increases so too does the amount of damage caused as does the number of firefighters called on to help (Frankfort-Nachmias & Leon-Guerrero, 2011). In each of these examples, it is the presence of a third variable that explains the apparent association between the two original variables.
In sum, the following criteria must be met for a correlation to be considered causal:
- The two variables must vary together.
- The association must be plausible.
- The cause must precede the effect in time.
- The association must be nonspurious (not due to a third variable).
Once these criteria are met, there is a nomothetic causal explanation, one that is objectively true. However, this is difficult for researchers to achieve. You will almost never hear researchers say that they have proven their hypotheses. A statement that bold implies that a association has been shown to exist with absolute certainty and that there is no chance that there are conditions under which the hypothesis would not be true. Instead, researchers tend to say that their hypotheses have been supported (or not). This more cautious way of discussing findings allows for the possibility that new evidence or new ways of examining an association will be discovered. Researchers may also discuss a null hypothesis. The null hypothesis is one that predicts no association between the variables being studied. If a researcher fails to accept the null hypothesis, she is saying that the variables in question are likely to be related to one another.
Idiographic causal explanations
If you not trying to generalize, but instead are trying to establish an idiographic causal explanation, then you are likely going to use qualitative methods, reason inductively, and engage in exploratory or descriptive research. We can understand these assumptions by walking through them, one by one.
Researchers seeking idiographic causal explanation are not trying to generalize, so they have no need to reduce phenomena to mathematics. In fact, using the language of mathematics to reduce the social world down is a bad thing, as it robs the causality of its meaning and context. Idiographic causal explanations are bound within people’s stories and interpretations. Usually, these are expressed through words. Not all qualitative studies analyze words, as some can use interpretations of visual or performance art, but the vast majority of social science studies do.
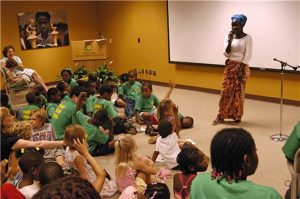
But wait, we predicted that an idiographic causal explanation would use descriptive or exploratory research. How can we build causality if we are just describing or exploring a topic? Wouldn’t we need to do explanatory research to build any kind of causal explanation? To clarify, explanatory research attempts to establish nomothetic causal explanations—an independent variable is demonstrated to cause changes a dependent variable. Exploratory and descriptive qualitative research are actually descriptions of the causal explanations established by the participants in your study. Instead of saying “x causes y,” your participants will describe their experiences with “x,” which they will tell you was caused by and influenced a variety of other factors, depending on time, environment, and subjective experience. As stated before, idiographic causal explanations are messy. The job of a social science researcher is to accurately identify patterns in what participants describe.
Let’s consider an example. What would you say if you were asked why you decided to become a social worker? If we interviewed many social workers about their decisions to become social workers, we might begin to notice patterns. We might find out that many social workers begin their careers based on a variety of factors, such as: personal experience with a disability or social injustice, positive experiences with social workers, or a desire to help others. No one factor is the “most important factor,” like with nomothetic causal explanations. Instead, a complex web of factors, contingent on context, emerge in the dataset when you interpret what people have said.
Finding patterns in data, as you’ll remember from Chapter 2, is what inductive reasoning is all about. A qualitative researcher collects data, usually words, and notices patterns. Those patterns inform the theories we use in social work. In many ways, the idiographic causal explanations created in qualitative research are like the social theories we reviewed in Chapter 2 and other theories you use in your practice and theory courses. Theories are explanations about how different concepts are associated with each other how that network of associations works in the real world. While you can think of theories like Systems Theory as Theory (with a capital “T”), inductive causality is like theory with a small “t.” It may apply only to the participants, environment, and moment in time in which the data were gathered. Nevertheless, it contributes important information to the body of knowledge on the topic studied.
Unlike nomothetic causal explanations, there are no formal criteria (e.g., covariation) for establishing causality in idiographic causal explanations. In fact, some criteria like temporality and nonspuriousness may be violated. For example, if an adolescent client says, “It’s hard for me to tell whether my depression began before my drinking, but both got worse when I was expelled from my first high school,” they are recognizing that oftentimes it’s not so simple that one thing causes another. Sometimes, there is a reciprocal association where one variable (depression) impacts another (alcohol abuse), which then feeds back into the first variable (depression) and also into other variables (school). Other criteria, such as covariation and plausibility still make sense, as the associations you highlight as part of your idiographic causal explanation should still be plausibly true and it elements should vary together.
Similarly, idiographic causal explanations differ in terms of hypotheses. If you recall from the last section, hypotheses in nomothetic causal explanations are testable predictions based on previous theory. In idiographic research, instead of predicting that “x will decrease y,” researchers will use previous literature to figure out what concepts might be important to participants and how they believe participants might respond during the study. Based on an analysis of the literature a researcher may formulate a few tentative hypotheses about what they expect to find in their qualitative study. Unlike nomothetic hypotheses, these are likely to change during the research process. As the researcher learns more from their participants, they might introduce new concepts that participants talk about. Because the participants are the experts in idiographic causal explanation, a researcher should be open to emerging topics and shift their research questions and hypotheses accordingly.
Complementary approaches to causality
Over time, as more qualitative studies are done and patterns emerge across different studies and locations, more sophisticated theories emerge that explain phenomena across multiple contexts. In this way, qualitative researchers use idiographic causal explanations for theory building or the creation of new theories based on inductive reasoning. Quantitative researchers, on the other hand, use nomothetic causal explanations for theory testing , wherein a hypothesis is created from existing theory (big T or small t) and tested mathematically (i.e., deductive reasoning). Once a theory is developed from qualitative data, a quantitative researcher can seek to test that theory. In this way, qualitatively-derived theory can inspire a hypothesis for a quantitative research project.
Two different baskets
Idiographic and nomothetic causal explanations form the “two baskets” of research design elements pictured in Figure 4.4 below. Later on, they will also determine the sampling approach, measures, and data analysis in your study.
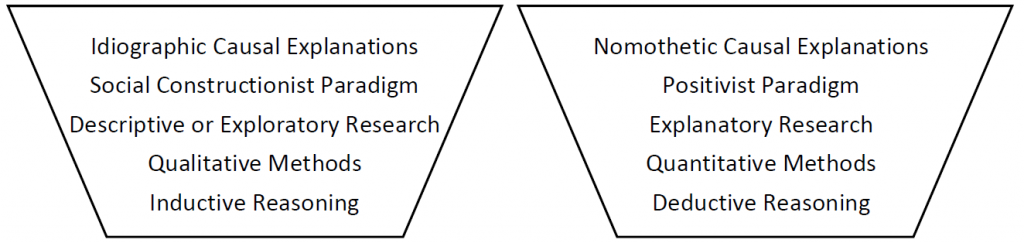
In most cases, mixing components from one basket with the other would not make sense. If you are using quantitative methods with an idiographic question, you wouldn’t get the deep understanding you need to answer an idiographic question. Knowing, for example, that someone scores 20/35 on a numerical index of depression symptoms does not tell you what depression means to that person. Similarly, qualitative methods are not often used to deductive reasoning because qualitative methods usually seek to understand a participant’s perspective, rather than test what existing theory says about a concept.
However, these are not hard-and-fast rules. There are plenty of qualitative studies that attempt to test a theory. There are fewer social constructionist studies with quantitative methods, though studies will sometimes include quantitative information about participants. Researchers in the critical paradigm can fit into either bucket, depending on their research question, as they focus on the liberation of people from oppressive internal (subjective) or external (objective) forces.
We will explore later on in this chapter how researchers can use both buckets simultaneously in mixed methods research. For now, it’s important that you understand the logic that connects the ideas in each bucket. Not only is this fundamental to how knowledge is created and tested in social work, it speaks to the very assumptions and foundations upon which all theories of the social world are built!
Key Takeaways
- Idiographic research focuses on subjectivity, context, and meaning.
- Nomothetic research focuses on objectivity, prediction, and generalizing.
- In qualitative studies, the goal is generally to understand the multitude of causes that account for the specific instance the researcher is investigating.
- In quantitative studies, the goal may be to understand the more general causes of some phenomenon rather than the idiosyncrasies of one particular instance.
- For nomothetic causal explanations, an association must be plausible and nonspurious, and the cause must precede the effect in time.
- In a nomothetic causal explanations, the independent variable causes changes in a dependent variable.
- Hypotheses are statements, drawn from theory, which describe a researcher’s expectation about an association between two or more variables.
- Qualitative research may create theories that can be tested quantitatively.
- The choice of idiographic or nomothetic causal explanation requires a consideration of methods, paradigm, and reasoning.
- Depending on whether you seek a nomothetic or idiographic causal explanation, you are likely to employ specific research design components.
- Causality-the idea that one event, behavior, or belief will result in the occurrence of another, subsequent event, behavior, or belief
- Control variables- potential “third variables” effects are controlled for mathematically in the data analysis process to highlight the relationship between the independent and dependent variable
- Covariation- the degree to which two variables vary together
- Dependent variable- a variable that depends on changes in the independent variable
- Generalize- to make claims about a larger population based on an examination of a smaller sample
- Hypothesis- a statement describing a researcher’s expectation regarding what she anticipates finding
- Idiographic research- attempts to explain or describe your phenomenon exhaustively, based on the subjective understandings of your participants
- Independent variable- causes a change in the dependent variable
- Nomothetic research- provides a more general, sweeping explanation that is universally true for all people
- Plausibility- in order to make the claim that one event, behavior, or belief causes another, the claim has to make sense
- Spurious relationship- an association between two variables appears to be causal but can in fact be explained by some third variable
- Statistical significance- confidence researchers have in a mathematical relationship
- Temporality- whatever cause you identify must happen before the effect
- Theory building- the creation of new theories based on inductive reasoning
- Theory testing- when a hypothesis is created from existing theory and tested mathematically
Image attributions
Mikado by 3dman_eu CC-0
Weather TV Forecast by mohamed_hassan CC-0
Figures 4.2 and 4.3 were copied from Blackstone, A. (2012) Principles of sociological inquiry: Qualitative and quantitative methods. Saylor Foundation. Retrieved from: https://saylordotorg.github.io/text_principles-of-sociological-inquiry-qualitative-and-quantitative-methods/ Shared under CC-BY-NC-SA 3.0 License
Beatrice Birra Storytelling at African Art Museum by Anthony Cross public domain
Foundations of Social Work Research Copyright © 2020 by Rebecca L. Mauldin is licensed under a Creative Commons Attribution-NonCommercial-ShareAlike 4.0 International License , except where otherwise noted.
Share This Book
What is causal research design?
Last updated
14 May 2023
Reviewed by
Short on time? Get an AI generated summary of this article instead
Examining these relationships gives researchers valuable insights into the mechanisms that drive the phenomena they are investigating.
Organizations primarily use causal research design to identify, determine, and explore the impact of changes within an organization and the market. You can use a causal research design to evaluate the effects of certain changes on existing procedures, norms, and more.
This article explores causal research design, including its elements, advantages, and disadvantages.
Analyze your causal research
Dovetail streamlines causal research analysis to help you uncover and share actionable insights
- Components of causal research
You can demonstrate the existence of cause-and-effect relationships between two factors or variables using specific causal information, allowing you to produce more meaningful results and research implications.
These are the key inputs for causal research:
The timeline of events
Ideally, the cause must occur before the effect. You should review the timeline of two or more separate events to determine the independent variables (cause) from the dependent variables (effect) before developing a hypothesis.
If the cause occurs before the effect, you can link cause and effect and develop a hypothesis .
For instance, an organization may notice a sales increase. Determining the cause would help them reproduce these results.
Upon review, the business realizes that the sales boost occurred right after an advertising campaign. The business can leverage this time-based data to determine whether the advertising campaign is the independent variable that caused a change in sales.
Evaluation of confounding variables
In most cases, you need to pinpoint the variables that comprise a cause-and-effect relationship when using a causal research design. This uncovers a more accurate conclusion.
Co-variations between a cause and effect must be accurate, and a third factor shouldn’t relate to cause and effect.
Observing changes
Variation links between two variables must be clear. A quantitative change in effect must happen solely due to a quantitative change in the cause.
You can test whether the independent variable changes the dependent variable to evaluate the validity of a cause-and-effect relationship. A steady change between the two variables must occur to back up your hypothesis of a genuine causal effect.
- Why is causal research useful?
Causal research allows market researchers to predict hypothetical occurrences and outcomes while enhancing existing strategies. Organizations can use this concept to develop beneficial plans.
Causal research is also useful as market researchers can immediately deduce the effect of the variables on each other under real-world conditions.
Once researchers complete their first experiment, they can use their findings. Applying them to alternative scenarios or repeating the experiment to confirm its validity can produce further insights.
Businesses widely use causal research to identify and comprehend the effect of strategic changes on their profits.
- How does causal research compare and differ from other research types?
Other research types that identify relationships between variables include exploratory and descriptive research .
Here’s how they compare and differ from causal research designs:
Exploratory research
An exploratory research design evaluates situations where a problem or opportunity's boundaries are unclear. You can use this research type to test various hypotheses and assumptions to establish facts and understand a situation more clearly.
You can also use exploratory research design to navigate a topic and discover the relevant variables. This research type allows flexibility and adaptability as the experiment progresses, particularly since no area is off-limits.
It’s worth noting that exploratory research is unstructured and typically involves collecting qualitative data . This provides the freedom to tweak and amend the research approach according to your ongoing thoughts and assessments.
Unfortunately, this exposes the findings to the risk of bias and may limit the extent to which a researcher can explore a topic.
This table compares the key characteristics of causal and exploratory research:
|
|
|
Main research statement | Research hypotheses | Research question |
Amount of uncertainty characterizing decision situation | Clearly defined | Highly ambiguous |
Research approach | Highly structured | Unstructured |
When you conduct it | Later stages of decision-making | Early stages of decision-making |
Descriptive research
This research design involves capturing and describing the traits of a population, situation, or phenomenon. Descriptive research focuses more on the " what " of the research subject and less on the " why ."
Since descriptive research typically happens in a real-world setting, variables can cross-contaminate others. This increases the challenge of isolating cause-and-effect relationships.
You may require further research if you need more causal links.
This table compares the key characteristics of causal and descriptive research.
|
|
|
Main research statement | Research hypotheses | Research question |
Amount of uncertainty characterizing decision situation | Clearly defined | Partially defined |
Research approach | Highly structured | Structured |
When you conduct it | Later stages of decision-making | Later stages of decision-making |
Causal research examines a research question’s variables and how they interact. It’s easier to pinpoint cause and effect since the experiment often happens in a controlled setting.
Researchers can conduct causal research at any stage, but they typically use it once they know more about the topic.
In contrast, causal research tends to be more structured and can be combined with exploratory and descriptive research to help you attain your research goals.
- How can you use causal research effectively?
Here are common ways that market researchers leverage causal research effectively:
Market and advertising research
Do you want to know if your new marketing campaign is affecting your organization positively? You can use causal research to determine the variables causing negative or positive impacts on your campaign.
Improving customer experiences and loyalty levels
Consumers generally enjoy purchasing from brands aligned with their values. They’re more likely to purchase from such brands and positively represent them to others.
You can use causal research to identify the variables contributing to increased or reduced customer acquisition and retention rates.
Could the cause of increased customer retention rates be streamlined checkout?
Perhaps you introduced a new solution geared towards directly solving their immediate problem.
Whatever the reason, causal research can help you identify the cause-and-effect relationship. You can use this to enhance your customer experiences and loyalty levels.
Improving problematic employee turnover rates
Is your organization experiencing skyrocketing attrition rates?
You can leverage the features and benefits of causal research to narrow down the possible explanations or variables with significant effects on employees quitting.
This way, you can prioritize interventions, focusing on the highest priority causal influences, and begin to tackle high employee turnover rates.
- Advantages of causal research
The main benefits of causal research include the following:
Effectively test new ideas
If causal research can pinpoint the precise outcome through combinations of different variables, researchers can test ideas in the same manner to form viable proof of concepts.
Achieve more objective results
Market researchers typically use random sampling techniques to choose experiment participants or subjects in causal research. This reduces the possibility of exterior, sample, or demography-based influences, generating more objective results.
Improved business processes
Causal research helps businesses understand which variables positively impact target variables, such as customer loyalty or sales revenues. This helps them improve their processes, ROI, and customer and employee experiences.
Guarantee reliable and accurate results
Upon identifying the correct variables, researchers can replicate cause and effect effortlessly. This creates reliable data and results to draw insights from.
Internal organization improvements
Businesses that conduct causal research can make informed decisions about improving their internal operations and enhancing employee experiences.
- Disadvantages of causal research
Like any other research method, casual research has its set of drawbacks that include:
Extra research to ensure validity
Researchers can't simply rely on the outcomes of causal research since it isn't always accurate. There may be a need to conduct other research types alongside it to ensure accurate output.
Coincidence
Coincidence tends to be the most significant error in causal research. Researchers often misinterpret a coincidental link between a cause and effect as a direct causal link.
Administration challenges
Causal research can be challenging to administer since it's impossible to control the impact of extraneous variables .
Giving away your competitive advantage
If you intend to publish your research, it exposes your information to the competition.
Competitors may use your research outcomes to identify your plans and strategies to enter the market before you.
- Causal research examples
Multiple fields can use causal research, so it serves different purposes, such as.
Customer loyalty research
Organizations and employees can use causal research to determine the best customer attraction and retention approaches.
They monitor interactions between customers and employees to identify cause-and-effect patterns. That could be a product demonstration technique resulting in higher or lower sales from the same customers.
Example: Business X introduces a new individual marketing strategy for a small customer group and notices a measurable increase in monthly subscriptions.
Upon getting identical results from different groups, the business concludes that the individual marketing strategy resulted in the intended causal relationship.
Advertising research
Businesses can also use causal research to implement and assess advertising campaigns.
Example: Business X notices a 7% increase in sales revenue a few months after a business introduces a new advertisement in a certain region. The business can run the same ad in random regions to compare sales data over the same period.
This will help the company determine whether the ad caused the sales increase. If sales increase in these randomly selected regions, the business could conclude that advertising campaigns and sales share a cause-and-effect relationship.
Educational research
Academics, teachers, and learners can use causal research to explore the impact of politics on learners and pinpoint learner behavior trends.
Example: College X notices that more IT students drop out of their program in their second year, which is 8% higher than any other year.
The college administration can interview a random group of IT students to identify factors leading to this situation, including personal factors and influences.
With the help of in-depth statistical analysis, the institution's researchers can uncover the main factors causing dropout. They can create immediate solutions to address the problem.
Is a causal variable dependent or independent?
When two variables have a cause-and-effect relationship, the cause is often called the independent variable. As such, the effect variable is dependent, i.e., it depends on the independent causal variable. An independent variable is only causal under experimental conditions.
What are the three criteria for causality?
The three conditions for causality are:
Temporality/temporal precedence: The cause must precede the effect.
Rationality: One event predicts the other with an explanation, and the effect must vary in proportion to changes in the cause.
Control for extraneous variables: The covariables must not result from other variables.
Is causal research experimental?
Causal research is mostly explanatory. Causal studies focus on analyzing a situation to explore and explain the patterns of relationships between variables.
Further, experiments are the primary data collection methods in studies with causal research design. However, as a research design, causal research isn't entirely experimental.
What is the difference between experimental and causal research design?
One of the main differences between causal and experimental research is that in causal research, the research subjects are already in groups since the event has already happened.
On the other hand, researchers randomly choose subjects in experimental research before manipulating the variables.
Should you be using a customer insights hub?
Do you want to discover previous research faster?
Do you share your research findings with others?
Do you analyze research data?
Start for free today, add your research, and get to key insights faster
Editor’s picks
Last updated: 18 April 2023
Last updated: 27 February 2023
Last updated: 22 August 2024
Last updated: 5 February 2023
Last updated: 16 August 2024
Last updated: 9 March 2023
Last updated: 30 April 2024
Last updated: 12 December 2023
Last updated: 11 March 2024
Last updated: 4 July 2024
Last updated: 6 March 2024
Last updated: 5 March 2024
Last updated: 13 May 2024
Latest articles
Related topics, .css-je19u9{-webkit-align-items:flex-end;-webkit-box-align:flex-end;-ms-flex-align:flex-end;align-items:flex-end;display:-webkit-box;display:-webkit-flex;display:-ms-flexbox;display:flex;-webkit-flex-direction:row;-ms-flex-direction:row;flex-direction:row;-webkit-box-flex-wrap:wrap;-webkit-flex-wrap:wrap;-ms-flex-wrap:wrap;flex-wrap:wrap;-webkit-box-pack:center;-ms-flex-pack:center;-webkit-justify-content:center;justify-content:center;row-gap:0;text-align:center;max-width:671px;}@media (max-width: 1079px){.css-je19u9{max-width:400px;}.css-je19u9>span{white-space:pre;}}@media (max-width: 799px){.css-je19u9{max-width:400px;}.css-je19u9>span{white-space:pre;}} decide what to .css-1kiodld{max-height:56px;display:-webkit-box;display:-webkit-flex;display:-ms-flexbox;display:flex;-webkit-align-items:center;-webkit-box-align:center;-ms-flex-align:center;align-items:center;}@media (max-width: 1079px){.css-1kiodld{display:none;}} build next, decide what to build next, log in or sign up.
Get started for free
Have a language expert improve your writing
Run a free plagiarism check in 10 minutes, generate accurate citations for free.
- Knowledge Base
Methodology
- Correlation vs. Causation | Difference, Designs & Examples
Correlation vs. Causation | Difference, Designs & Examples
Published on July 12, 2021 by Pritha Bhandari . Revised on June 22, 2023.
Correlation means there is a statistical association between variables. Causation means that a change in one variable causes a change in another variable.
In research, you might have come across the phrase “correlation doesn’t imply causation.” Correlation and causation are two related ideas, but understanding their differences will help you critically evaluate sources and interpret scientific research.
Table of contents
What’s the difference, why doesn’t correlation mean causation, correlational research, third variable problem, regression to the mean, spurious correlations, directionality problem, causal research, other interesting articles, frequently asked questions about correlation and causation.
Correlation describes an association between types of variables : when one variable changes, so does the other. A correlation is a statistical indicator of the relationship between variables. These variables change together: they covary. But this covariation isn’t necessarily due to a direct or indirect causal link.
Causation means that changes in one variable brings about changes in the other; there is a cause-and-effect relationship between variables. The two variables are correlated with each other and there is also a causal link between them.
Prevent plagiarism. Run a free check.
There are two main reasons why correlation isn’t causation. These problems are important to identify for drawing sound scientific conclusions from research.
The third variable problem means that a confounding variable affects both variables to make them seem causally related when they are not. For example, ice cream sales and violent crime rates are closely correlated, but they are not causally linked with each other. Instead, hot temperatures, a third variable, affects both variables separately. Failing to account for third variables can lead research biases to creep into your work.
The directionality problem occurs when two variables correlate and might actually have a causal relationship, but it’s impossible to conclude which variable causes changes in the other. For example, vitamin D levels are correlated with depression, but it’s not clear whether low vitamin D causes depression, or whether depression causes reduced vitamin D intake.
You’ll need to use an appropriate research design to distinguish between correlational and causal relationships:
- Correlational research designs can only demonstrate correlational links between variables.
- Experimental designs can test causation.
In a correlational research design, you collect data on your variables without manipulating them.
Correlational research is usually high in external validity , so you can generalize your findings to real life settings. But these studies are low in internal validity , which makes it difficult to causally connect changes in one variable to changes in the other.
These research designs are commonly used when it’s unethical, too costly, or too difficult to perform controlled experiments. They are also used to study relationships that aren’t expected to be causal.
Without controlled experiments, it’s hard to say whether it was the variable you’re interested in that caused changes in another variable. Extraneous variables are any third variable or omitted variable other than your variables of interest that could affect your results.
Limited control in correlational research means that extraneous or confounding variables serve as alternative explanations for the results. Confounding variables can make it seem as though a correlational relationship is causal when it isn’t.
When two variables are correlated, all you can say is that changes in one variable occur alongside changes in the other.
Receive feedback on language, structure, and formatting
Professional editors proofread and edit your paper by focusing on:
- Academic style
- Vague sentences
- Style consistency
See an example
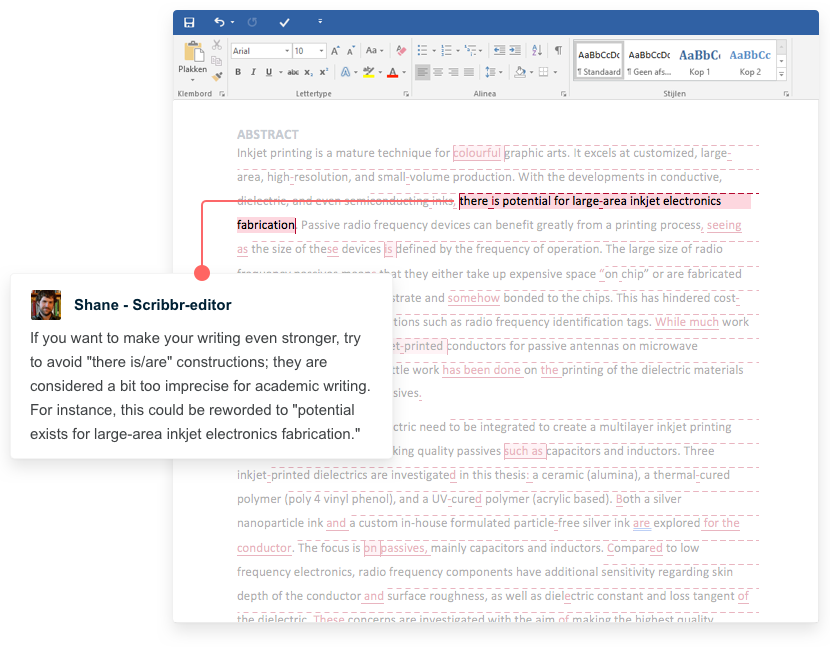
Regression to the mean is observed when variables that are extremely higher or extremely lower than average on the first measurement move closer to the average on the second measurement. Particularly in research that intentionally focuses on the most extreme cases or events, RTM should always be considered as a possible cause of an observed change.
Players or teams featured on the cover of SI have earned their place by performing exceptionally well. But athletic success is a mix of skill and luck, and even the best players don’t always win.
Chances are that good luck will not continue indefinitely, and neither can exceptional success.
A spurious correlation is when two variables appear to be related through hidden third variables or simply by coincidence.
The Theory of the Stork draws a simple causal link between the variables to argue that storks physically deliver babies. This satirical study shows why you can’t conclude causation from correlational research alone.
When you analyze correlations in a large dataset with many variables, the chances of finding at least one statistically significant result are high. In this case, you’re more likely to make a type I error . This means erroneously concluding there is a true correlation between variables in the population based on skewed sample data.
To demonstrate causation, you need to show a directional relationship with no alternative explanations. This relationship can be unidirectional, with one variable impacting the other, or bidirectional, where both variables impact each other.
A correlational design won’t be able to distinguish between any of these possibilities, but an experimental design can test each possible direction, one at a time.
- Physical activity may affect self esteem
- Self esteem may affect physical activity
- Physical activity and self esteem may both affect each other
In correlational research, the directionality of a relationship is unclear because there is limited researcher control. You might risk concluding reverse causality, the wrong direction of the relationship.
Causal links between variables can only be truly demonstrated with controlled experiments . Experiments test formal predictions, called hypotheses , to establish causality in one direction at a time.
Experiments are high in internal validity , so cause-and-effect relationships can be demonstrated with reasonable confidence.
You can establish directionality in one direction because you manipulate an independent variable before measuring the change in a dependent variable.
In a controlled experiment, you can also eliminate the influence of third variables by using random assignment and control groups.
Random assignment helps distribute participant characteristics evenly between groups so that they’re similar and comparable. A control group lets you compare the experimental manipulation to a similar treatment or no treatment (or a placebo, to control for the placebo effect ).
If you want to know more about statistics , methodology , or research bias , make sure to check out some of our other articles with explanations and examples.
- Chi square test of independence
- Statistical power
- Descriptive statistics
- Degrees of freedom
- Pearson correlation
- Null hypothesis
- Double-blind study
- Case-control study
- Research ethics
- Data collection
- Hypothesis testing
- Structured interviews
Research bias
- Hawthorne effect
- Unconscious bias
- Recall bias
- Halo effect
- Self-serving bias
- Information bias
A correlation reflects the strength and/or direction of the association between two or more variables.
- A positive correlation means that both variables change in the same direction.
- A negative correlation means that the variables change in opposite directions.
- A zero correlation means there’s no relationship between the variables.
Correlation describes an association between variables : when one variable changes, so does the other. A correlation is a statistical indicator of the relationship between variables.
Causation means that changes in one variable brings about changes in the other (i.e., there is a cause-and-effect relationship between variables). The two variables are correlated with each other, and there’s also a causal link between them.
While causation and correlation can exist simultaneously, correlation does not imply causation. In other words, correlation is simply a relationship where A relates to B—but A doesn’t necessarily cause B to happen (or vice versa). Mistaking correlation for causation is a common error and can lead to false cause fallacy .
The third variable and directionality problems are two main reasons why correlation isn’t causation .
The third variable problem means that a confounding variable affects both variables to make them seem causally related when they are not.
The directionality problem is when two variables correlate and might actually have a causal relationship, but it’s impossible to conclude which variable causes changes in the other.
Controlled experiments establish causality, whereas correlational studies only show associations between variables.
- In an experimental design , you manipulate an independent variable and measure its effect on a dependent variable. Other variables are controlled so they can’t impact the results.
- In a correlational design , you measure variables without manipulating any of them. You can test whether your variables change together, but you can’t be sure that one variable caused a change in another.
In general, correlational research is high in external validity while experimental research is high in internal validity .
Cite this Scribbr article
If you want to cite this source, you can copy and paste the citation or click the “Cite this Scribbr article” button to automatically add the citation to our free Citation Generator.
Bhandari, P. (2023, June 22). Correlation vs. Causation | Difference, Designs & Examples. Scribbr. Retrieved August 26, 2024, from https://www.scribbr.com/methodology/correlation-vs-causation/
Is this article helpful?
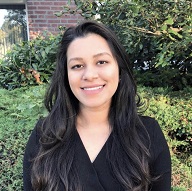
Pritha Bhandari
Other students also liked, correlational research | when & how to use, guide to experimental design | overview, steps, & examples, confounding variables | definition, examples & controls, what is your plagiarism score.
Root out friction in every digital experience, super-charge conversion rates, and optimize digital self-service
Uncover insights from any interaction, deliver AI-powered agent coaching, and reduce cost to serve
Increase revenue and loyalty with real-time insights and recommendations delivered to teams on the ground
Know how your people feel and empower managers to improve employee engagement, productivity, and retention
Take action in the moments that matter most along the employee journey and drive bottom line growth
Whatever they’re are saying, wherever they’re saying it, know exactly what’s going on with your people
Get faster, richer insights with qual and quant tools that make powerful market research available to everyone
Run concept tests, pricing studies, prototyping + more with fast, powerful studies designed by UX research experts
Track your brand performance 24/7 and act quickly to respond to opportunities and challenges in your market
Explore the platform powering Experience Management
- Free Account
- Product Demos
- For Digital
- For Customer Care
- For Human Resources
- For Researchers
- Financial Services
- All Industries
Popular Use Cases
- Customer Experience
- Employee Experience
- Net Promoter Score
- Voice of Customer
- Customer Success Hub
- Product Documentation
- Training & Certification
- XM Institute
- Popular Resources
- Customer Stories
- Artificial Intelligence
Market Research
- Partnerships
- Marketplace
The annual gathering of the experience leaders at the world’s iconic brands building breakthrough business results, live in Salt Lake City.
- English/AU & NZ
- Español/Europa
- Español/América Latina
- Português Brasileiro
- REQUEST DEMO
- Experience Management
- Causal Research
Try Qualtrics for free
Causal research: definition, examples and how to use it.
16 min read Causal research enables market researchers to predict hypothetical occurrences & outcomes while improving existing strategies. Discover how this research can decrease employee retention & increase customer success for your business.
What is causal research?
Causal research, also known as explanatory research or causal-comparative research, identifies the extent and nature of cause-and-effect relationships between two or more variables.
It’s often used by companies to determine the impact of changes in products, features, or services process on critical company metrics. Some examples:
- How does rebranding of a product influence intent to purchase?
- How would expansion to a new market segment affect projected sales?
- What would be the impact of a price increase or decrease on customer loyalty?
To maintain the accuracy of causal research, ‘confounding variables’ or influences — e.g. those that could distort the results — are controlled. This is done either by keeping them constant in the creation of data, or by using statistical methods. These variables are identified before the start of the research experiment.
As well as the above, research teams will outline several other variables and principles in causal research:
- Independent variables
The variables that may cause direct changes in another variable. For example, the effect of truancy on a student’s grade point average. The independent variable is therefore class attendance.
- Control variables
These are the components that remain unchanged during the experiment so researchers can better understand what conditions create a cause-and-effect relationship.
This describes the cause-and-effect relationship. When researchers find causation (or the cause), they’ve conducted all the processes necessary to prove it exists.
- Correlation
Any relationship between two variables in the experiment. It’s important to note that correlation doesn’t automatically mean causation. Researchers will typically establish correlation before proving cause-and-effect.
- Experimental design
Researchers use experimental design to define the parameters of the experiment — e.g. categorizing participants into different groups.
- Dependent variables
These are measurable variables that may change or are influenced by the independent variable. For example, in an experiment about whether or not terrain influences running speed, your dependent variable is the terrain.
Why is causal research useful?
It’s useful because it enables market researchers to predict hypothetical occurrences and outcomes while improving existing strategies. This allows businesses to create plans that benefit the company. It’s also a great research method because researchers can immediately see how variables affect each other and under what circumstances.
Also, once the first experiment has been completed, researchers can use the learnings from the analysis to repeat the experiment or apply the findings to other scenarios. Because of this, it’s widely used to help understand the impact of changes in internal or commercial strategy to the business bottom line.
Some examples include:
- Understanding how overall training levels are improved by introducing new courses
- Examining which variations in wording make potential customers more interested in buying a product
- Testing a market’s response to a brand-new line of products and/or services
So, how does causal research compare and differ from other research types?
Well, there are a few research types that are used to find answers to some of the examples above:
1. Exploratory research
As its name suggests, exploratory research involves assessing a situation (or situations) where the problem isn’t clear. Through this approach, researchers can test different avenues and ideas to establish facts and gain a better understanding.
Researchers can also use it to first navigate a topic and identify which variables are important. Because no area is off-limits, the research is flexible and adapts to the investigations as it progresses.
Finally, this approach is unstructured and often involves gathering qualitative data, giving the researcher freedom to progress the research according to their thoughts and assessment. However, this may make results susceptible to researcher bias and may limit the extent to which a topic is explored.
2. Descriptive research
Descriptive research is all about describing the characteristics of the population, phenomenon or scenario studied. It focuses more on the “what” of the research subject than the “why”.
For example, a clothing brand wants to understand the fashion purchasing trends amongst buyers in California — so they conduct a demographic survey of the region, gather population data and then run descriptive research. The study will help them to uncover purchasing patterns amongst fashion buyers in California, but not necessarily why those patterns exist.
As the research happens in a natural setting, variables can cross-contaminate other variables, making it harder to isolate cause and effect relationships. Therefore, further research will be required if more causal information is needed.
Get started on your market research journey with Strategic Research
How is causal research different from the other two methods above?
Well, causal research looks at what variables are involved in a problem and ‘why’ they act a certain way. As the experiment takes place in a controlled setting (thanks to controlled variables) it’s easier to identify cause-and-effect amongst variables.
Furthermore, researchers can carry out causal research at any stage in the process, though it’s usually carried out in the later stages once more is known about a particular topic or situation.
Finally, compared to the other two methods, causal research is more structured, and researchers can combine it with exploratory and descriptive research to assist with research goals.
Summary of three research types
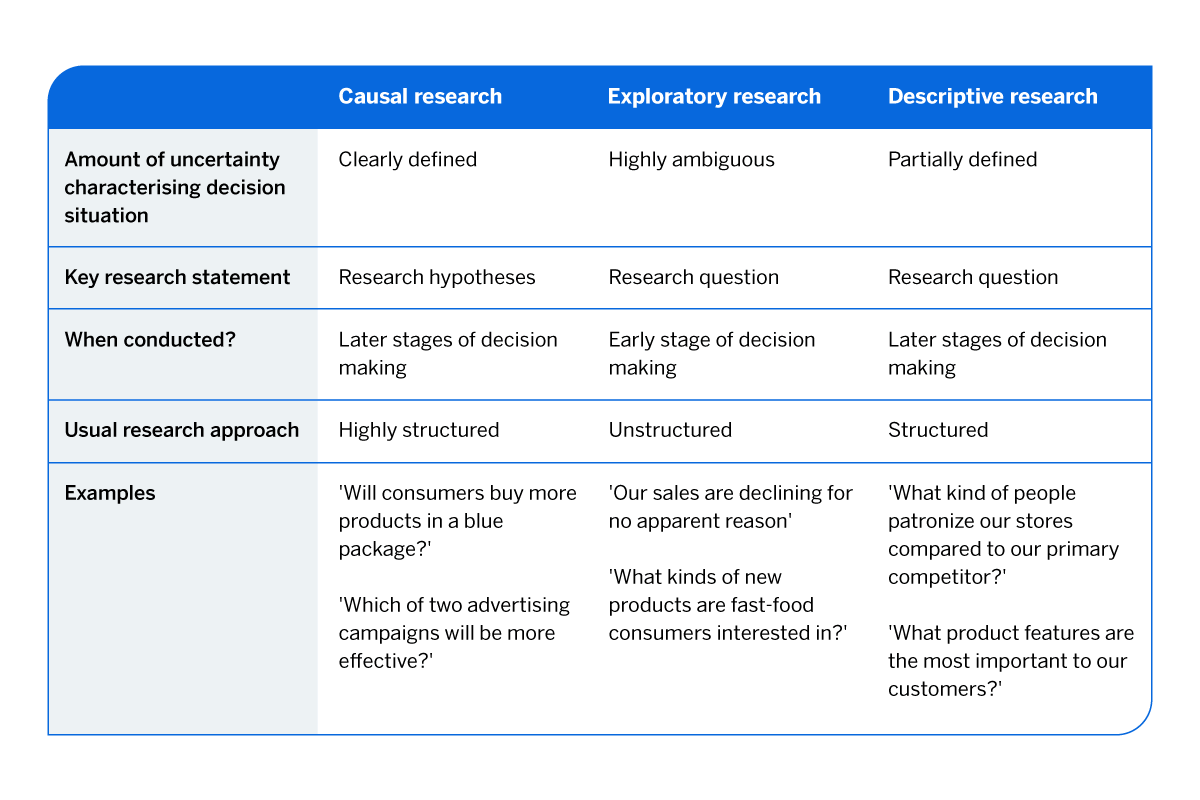
What are the advantages of causal research?
- Improve experiences
By understanding which variables have positive impacts on target variables (like sales revenue or customer loyalty), businesses can improve their processes, return on investment, and the experiences they offer customers and employees.
- Help companies improve internally
By conducting causal research, management can make informed decisions about improving their employee experience and internal operations. For example, understanding which variables led to an increase in staff turnover.
- Repeat experiments to enhance reliability and accuracy of results
When variables are identified, researchers can replicate cause-and-effect with ease, providing them with reliable data and results to draw insights from.
- Test out new theories or ideas
If causal research is able to pinpoint the exact outcome of mixing together different variables, research teams have the ability to test out ideas in the same way to create viable proof of concepts.
- Fix issues quickly
Once an undesirable effect’s cause is identified, researchers and management can take action to reduce the impact of it or remove it entirely, resulting in better outcomes.
What are the disadvantages of causal research?
- Provides information to competitors
If you plan to publish your research, it provides information about your plans to your competitors. For example, they might use your research outcomes to identify what you are up to and enter the market before you.
- Difficult to administer
Causal research is often difficult to administer because it’s not possible to control the effects of extraneous variables.
- Time and money constraints
Budgetary and time constraints can make this type of research expensive to conduct and repeat. Also, if an initial attempt doesn’t provide a cause and effect relationship, the ROI is wasted and could impact the appetite for future repeat experiments.
- Requires additional research to ensure validity
You can’t rely on just the outcomes of causal research as it’s inaccurate. It’s best to conduct other types of research alongside it to confirm its output.
- Trouble establishing cause and effect
Researchers might identify that two variables are connected, but struggle to determine which is the cause and which variable is the effect.
- Risk of contamination
There’s always the risk that people outside your market or area of study could affect the results of your research. For example, if you’re conducting a retail store study, shoppers outside your ‘test parameters’ shop at your store and skew the results.
How can you use causal research effectively?
To better highlight how you can use causal research across functions or markets, here are a few examples:
Market and advertising research
A company might want to know if their new advertising campaign or marketing campaign is having a positive impact. So, their research team can carry out a causal research project to see which variables cause a positive or negative effect on the campaign.
For example, a cold-weather apparel company in a winter ski-resort town may see an increase in sales generated after a targeted campaign to skiers. To see if one caused the other, the research team could set up a duplicate experiment to see if the same campaign would generate sales from non-skiers. If the results reduce or change, then it’s likely that the campaign had a direct effect on skiers to encourage them to purchase products.
Improving customer experiences and loyalty levels
Customers enjoy shopping with brands that align with their own values, and they’re more likely to buy and present the brand positively to other potential shoppers as a result. So, it’s in your best interest to deliver great experiences and retain your customers.
For example, the Harvard Business Review found that an increase in customer retention rates by 5% increased profits by 25% to 95%. But let’s say you want to increase your own, how can you identify which variables contribute to it?Using causal research, you can test hypotheses about which processes, strategies or changes influence customer retention. For example, is it the streamlined checkout? What about the personalized product suggestions? Or maybe it was a new solution that solved their problem? Causal research will help you find out.
Improving problematic employee turnover rates
If your company has a high attrition rate, causal research can help you narrow down the variables or reasons which have the greatest impact on people leaving. This allows you to prioritize your efforts on tackling the issues in the right order, for the best positive outcomes.
For example, through causal research, you might find that employee dissatisfaction due to a lack of communication and transparency from upper management leads to poor morale, which in turn influences employee retention.
To rectify the problem, you could implement a routine feedback loop or session that enables your people to talk to your company’s C-level executives so that they feel heard and understood.
How to conduct causal research first steps to getting started are:
1. Define the purpose of your research
What questions do you have? What do you expect to come out of your research? Think about which variables you need to test out the theory.
2. Pick a random sampling if participants are needed
Using a technology solution to support your sampling, like a database, can help you define who you want your target audience to be, and how random or representative they should be.
3. Set up the controlled experiment
Once you’ve defined which variables you’d like to measure to see if they interact, think about how best to set up the experiment. This could be in-person or in-house via interviews, or it could be done remotely using online surveys.
4. Carry out the experiment
Make sure to keep all irrelevant variables the same, and only change the causal variable (the one that causes the effect) to gather the correct data. Depending on your method, you could be collecting qualitative or quantitative data, so make sure you note your findings across each regularly.
5. Analyze your findings
Either manually or using technology, analyze your data to see if any trends, patterns or correlations emerge. By looking at the data, you’ll be able to see what changes you might need to do next time, or if there are questions that require further research.
6. Verify your findings
Your first attempt gives you the baseline figures to compare the new results to. You can then run another experiment to verify your findings.
7. Do follow-up or supplemental research
You can supplement your original findings by carrying out research that goes deeper into causes or explores the topic in more detail. One of the best ways to do this is to use a survey. See ‘Use surveys to help your experiment’.
Identifying causal relationships between variables
To verify if a causal relationship exists, you have to satisfy the following criteria:
- Nonspurious association
A clear correlation exists between one cause and the effect. In other words, no ‘third’ that relates to both (cause and effect) should exist.
- Temporal sequence
The cause occurs before the effect. For example, increased ad spend on product marketing would contribute to higher product sales.
- Concomitant variation
The variation between the two variables is systematic. For example, if a company doesn’t change its IT policies and technology stack, then changes in employee productivity were not caused by IT policies or technology.
How surveys help your causal research experiments?
There are some surveys that are perfect for assisting researchers with understanding cause and effect. These include:
- Employee Satisfaction Survey – An introductory employee satisfaction survey that provides you with an overview of your current employee experience.
- Manager Feedback Survey – An introductory manager feedback survey geared toward improving your skills as a leader with valuable feedback from your team.
- Net Promoter Score (NPS) Survey – Measure customer loyalty and understand how your customers feel about your product or service using one of the world’s best-recognized metrics.
- Employee Engagement Survey – An entry-level employee engagement survey that provides you with an overview of your current employee experience.
- Customer Satisfaction Survey – Evaluate how satisfied your customers are with your company, including the products and services you provide and how they are treated when they buy from you.
- Employee Exit Interview Survey – Understand why your employees are leaving and how they’ll speak about your company once they’re gone.
- Product Research Survey – Evaluate your consumers’ reaction to a new product or product feature across every stage of the product development journey.
- Brand Awareness Survey – Track the level of brand awareness in your target market, including current and potential future customers.
- Online Purchase Feedback Survey – Find out how well your online shopping experience performs against customer needs and expectations.
That covers the fundamentals of causal research and should give you a foundation for ongoing studies to assess opportunities, problems, and risks across your market, product, customer, and employee segments.
If you want to transform your research, empower your teams and get insights on tap to get ahead of the competition, maybe it’s time to leverage Qualtrics CoreXM.
Qualtrics CoreXM provides a single platform for data collection and analysis across every part of your business — from customer feedback to product concept testing. What’s more, you can integrate it with your existing tools and services thanks to a flexible API.
Qualtrics CoreXM offers you as much or as little power and complexity as you need, so whether you’re running simple surveys or more advanced forms of research, it can deliver every time.
Get started on your market research journey with CoreXM
Related resources
Market intelligence 10 min read, marketing insights 11 min read, ethnographic research 11 min read, qualitative vs quantitative research 13 min read, qualitative research questions 11 min read, qualitative research design 12 min read, primary vs secondary research 14 min read, request demo.
Ready to learn more about Qualtrics?
- Skip to secondary menu
- Skip to main content
- Skip to primary sidebar
Statistics By Jim
Making statistics intuitive
Correlation vs Causation: Understanding the Differences
By Jim Frost Leave a Comment
Correlation vs causation in statistics is a critical distinction. And you’ve undoubtedly heard that correlation doesn’t imply causation. Why is that the case, what are the differences between them, and why do they matter? Those are the topics of this post!
Correlation vs Causation Definitions
Let’s first compare the definitions of correlation vs causation.
What is Correlation?
Correlation means that as one variable changes, another tends to change in a specific direction. In other words, two variables move together. Positive and negative correlations exist.
- Positive correlation: X increases and Y tends to increase.
- Negative correlation: X increases and Y tends to decrease.
For example, as people’s heights grow, their weight tends to increase, creating the positive correlation below. Or, as school absences increase, grades tend to decrease. That’s a negative correlation.

Learn more about Understanding Correlation Coefficients .
What is Causation?
Causation indicates that changes in one variable trigger changes in another variable. For example, increasing the dosage of a medicine causes the severity of the symptoms to decrease.
Relationship between Correlation vs Causation
At first glance, those definitions certainly seem consistent, which is why they’re so frequently misunderstood. What is the relationship between correlation vs causation?
Correlation doesn’t imply causation, but causation suggests that correlation exists. The Venn diagram shows the relationship between the two.

Understanding why causation implies correlation is intuitive. If increasing medicine dosage decreases the symptoms, you’ll find a negative correlation between those variables. The causation creates the correlation.
Unfortunately, it’s less intuitive to understand how you can observe a correlation but not be sure about causation. Let’s dig into that issue.
Why Doesn’t Correlation Imply Causation?
Suppose you find a positive correlation between X and Y. How could it not be causal? After all, when X goes up, Y also goes up. It sounds like cause and effect , but it might not be. Statisticians refer to a non-causal association between variables as a spurious correlation.
They exist for multiple reasons. The fact that they exist all is why you can’t be sure that correlation indicates causation. Only a subset of correlations reflect a causal relationship. Hence the importance of understanding correlation vs causation.
Let’s cover three potential explanations for spurious correlations.
Related post : Understanding Spurious Correlations
Third Variable Problem
A third variable can create a spurious relationship between two variables. It depends on the pattern of correlations between the two variables you’re considering and a third variable.
Did you know a positive correlation exists between ice cream sales and shark attacks?
Now, ice cream sales do not cause an increase in shark attacks. So, what is going on?
It turns out that outside temperature positively correlates with ice cream sales and shark attack opportunities (because more people go to the beach). So, when temperature increases, both sales and attacks increase in unison, creating a spurious correlation between them. In this scenario, we call temperature a lurking variable or a confounder .
Direction of Causation
Sometimes two variables might have a causal relationship, but it’s unclear which variable is the cause and which is the effect.
Researchers find a correlation between the number of hours students spend on social media and their academic grades.
Scenario 1 : It could be that spending more time on social media distracts students from their studies, leading to lower grades.
Scenario 2 : Conversely, students struggling with their studies might turn to social media for escapism, meaning lower grades lead to increased social media usage.
In this situation, it’s ambiguous whether social media usage causes lower grades or if the reverse is true.
Pure Chance
Random chance in sample data can produce an apparent relationship between variables. If you collect enough random samples, randomness will occasionally create the appearance of a correlation where none actually exists. I wrote about this phenomenon in a post about data dredging. I show how entirely randomly generated data that should not have any correlations can produce them when you sift through enough data. See Data Dredging .
The graph below shows what appears to be a negative correlation, but I had computer software randomly generate many variables and then systematically dredge through the data and find correlations.

For these reasons, you might see a correlation in your data when there is no cause and effect. Or at least you might not be sure about it.
Why Establishing Correlation vs Causation is Important
Correlation only indicates that two variables move together, but it doesn’t tell us if one causes changes in the other. Relying solely on correlation can lead to misguided conclusions and ineffective or even harmful actions. Establishing causality ensures that we’re targeting the root cause of an issue rather than just an associated symptom.
For instance, a study published in the journal Language Sciences found a correlation between individuals who use taboo words (swear words) and higher levels of verbal intelligence. However, it’s essential to approach such findings with nuance. The correlation doesn’t suggest that swearing enhances intelligence. Instead, it might indicate that individuals with a richer vocabulary, encompassing both standard and taboo words, have a more extensive linguistic repertoire to express themselves. Misunderstanding this correlation could lead to the mistaken belief that increasing one’s use of swear words would boost intelligence, which is not what the study implies.
Alternatively, suppose you unknowingly find a spurious correlation between vitamins and improved health outcomes. Believing that the vitamins cause those improvements when it’s merely correlation leads to poor decision-making. After all, if the vitamins don’t cause health gains, then consuming more vitamins won’t produce better outcomes despite the correlation.
Scientists have found that people who regularly take vitamins have many pre-existing health habits and conditions that differ from non-vitamin consumers. Those differences are the likely causes for the improved health outcomes rather than the vitamins themselves. Read my posts about Observational Studies to see the long list of differences in the example.
These examples underscore the critical importance of distinguishing between correlation vs causation in decision-making.
How to Identify Causal vs. Correlational Relationships
Establishing correlation vs causation is often misunderstood. While correlation can provide hints about potential relationships between variables, it doesn’t prove that one variable causes another to change. That’s an entirely different matter. They might not be causally linked at all. Unfortunately, spurious correlations occur frequently, and there’s no statistical test for detecting them!
So, how do you distinguish between correlation vs causation?
To truly establish causality, researchers need specially designed experiments—randomized controlled trials (RCTs). RCTs randomly assign participants to either a treatment or control group. This random assignment helps ensure all groups start the same except for the treatment. If the outcomes differ at the end, analysts can attribute them to the treatment with high confidence. Learn more about Randomized Controlled Trials and Random Assignment in Experiments .
Conversely, Correlational Studies are suited for finding relationships quickly and inexpensively in preliminary studies but they are not suitable for establishing causality.
Sir Austin Bradford Hill proposed a set of nine criteria to help determine if a relationship is genuinely causal rather than merely correlational. These criteria are an exercise in critical thought. They prompt you to think about causation by highlighting vital properties to consider and how to apply your subject-area knowledge. The objective is to fulfill as many criteria as possible. While no single criterion is adequate, it’s usually impossible to meet all of them. For a deeper dive, read my post about Causation in Statistics: Hill’s Criteria .
Regardless of the method used, it’s crucial to approach the question of correlation vs causation with caution, ensuring that you base your conclusions on solid evidence, sound methodology, and critical thinking.
Austin Bradford Hill, “The Environment and Disease: Association or Causation?,” Proceedings of the Royal Society of Medicine , 58 (1965), 295-300.
Share this:

Reader Interactions
Comments and questions cancel reply.
- Election 2024
- Entertainment
- Newsletters
- Photography
- AP Buyline Personal Finance
- AP Buyline Shopping
- Press Releases
- Israel-Hamas War
- Russia-Ukraine War
- Global elections
- Asia Pacific
- Latin America
- Middle East
- Delegate Tracker
- AP & Elections
- U.S. Open Tennis
- College football
- Auto Racing
- Movie reviews
- Book reviews
- Financial Markets
- Business Highlights
- Financial wellness
- Artificial Intelligence
- Social Media
Takeaways from Fed Chair Powell’s speech at Jackson Hole
Federal Reserve Chairman Jerome Powell, left, speaks with Governor of the Bank of Canada Tiff Macklem outside of the Jackson Hole Economic Symposium at Jackson Lake Lodge in Grand Teton National Park near Moran, Wyo., on Aug. 23, 2024. (AP Photo/Amber Baesler)
Federal Reserve Chairman Jerome Powell, left, Governor of the Bank of Canada Tiff Macklem, center, and Governor of the Bank of England Andrew Bailey chat outside the Jackson Hole Economic Symposium at Jackson Lake Lodge in Grand Teton National Park near Moran, WY on Aug. 23, 2024. (AP Photo/Amber Baesler)
- Copy Link copied
WASHINGTON (AP) — Federal Reserve Chair Jerome Powell all but proclaimed victory in the fight against inflation and signaled that interest rate cuts are coming in a much-anticipated speech Friday in Jackson Hole, Wyoming.
Under Powell, the Fed raised its benchmark rate to the highest level in 23 years to subdue inflation that two years ago was running at the hottest pace in more than four decades. Inflation has come down steadily , and investors now expect the Fed to start cutting rates at its next meeting in September — an expectation that essentially got Powell’s endorsement Friday.
Powell declares victory over inflation
“My confidence has grown that inflation is on a sustainable path back to 2%,” Powell said in his keynote speech at the Fed’s annual economic conference in Jackson Hole.
He noted that inflation, according to the Fed’s preferred gauge, had fallen to 2.5% last from a peak of 7.1% two years ago. Measured by the better known consumer price index, inflation has dropped from a peak 9.1% in mid-2022 to 2.9% last month. Both are edging closer to the Fed’s 2% target.
Powell sounded confident that the Fed would achieve a so-called soft landing — containing inflation without causing a recession. “There is good reason to think that the economy will get back to 2% inflation while maintaining a strong labor market,’' he said.
Higher rates contributed to progress against inflation, as did the easing of supply chain bottlenecks and worker shortages that caused shipping delays and higher prices as the economy bounded back with unexpected strength from COVID-19 lockdowns.
Rate cuts are coming
Powell suggested Friday that rate cuts are all but inevitable. “The direction of travel is clear, and the timing and pace of rate cuts will depend on incoming data, the evolving outlook, and the balance of risks,” he said.
Last year, the Fed had predicted that it would trim rates three times this year. But the cuts kept getting pushed back as the progress against inflation faltered early in 2024 . Since then, the steady drop in inflation has resumed, giving the Fed more confidence that victory was in sight.
Fed misjudged inflation
Powell acknowledged that he and his Fed colleagues misjudged the inflationary threat when it emerged in early 2021. At the time, they expected the flareup of higher prices to be short-lived — the temporary consequence of pandemic-related supply chain disruptions. The pressure, they thought, would fade “fairly quickly without the need for a monetary policy response — in short, that the inflation would be transitory.’'
They weren’t alone in their optimism. “The good ship Transitory was a crowded one,’' Powell said, ”with most mainstream analysts and advanced-economy central bankers on board.’'
But the word “transitory″ came back to haunt the Fed as inflation proved more intractable than expected. It spread from goods that were subject to supply chain backlogs into services, where it is harder to dislodge without raising rates and risking severe economic pain in the form of layoffs and higher unemployment. The Fed proceeded to raise rates 11 times in 2022 and 2023.
Unpredictable economy leaves policymakers humble
Powell admitted that policymakers and economists have struggled to understand and respond to an economy that has been unpredictable since COVID-19 hit in early 2020. First, the pandemic shut down commerce and companies collectively slashed millions of jobs. Then the economy roared back with unexpected vigor, setting off inflationary pressures that been dormant since the early 1980s. When the Fed belated responded with aggressive rate hikes, economists predicted the hiring borrowing costs would cause a painful recession. But it didn’t.
“The limits of our knowledge — so clearly evident during the pandemic — demand humility and a questioning spirit focused on learnings lessons form the past and applying them flexibly to our current challenges,’' Powell said.
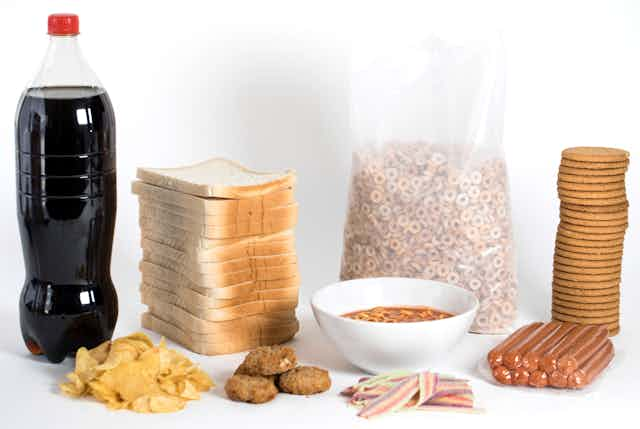
Blood sugar fluctuations after eating play an important role in anxiety and depression
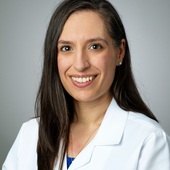
Family Medicine Resident and Nutrition Expert, Eastern Virginia Medical School
Disclosure statement
Mary Scourboutakos does not work for, consult, own shares in or receive funding from any company or organisation that would benefit from this article, and has disclosed no relevant affiliations beyond their academic appointment.
View all partners
The proverbial “sugar high” that follows the ingestion of a sweet treat is a familiar example of the potentially positive effects of food on mood.
On the flip side, feeling “hangry” – the phenomenon where hunger manifests in the form of anger or irritability – illustrates how what we eat, or don’t eat, can also provoke negative emotions.
The latest research suggests that blood sugar fluctuations are partly responsible for the connection between what we eat and how we feel. Through its effects on our hormones and our nervous system , blood sugar levels can be fuel for anxiety and depression .
Mental health is complex. There are countless social, psychological and biological factors that ultimately determine any one person’s experience. However, numerous randomized controlled trials have demonstrated that diet is one biological factor that can significantly influence risk for symptoms of depression and anxiety, especially in women.
As a family medicine resident with a Ph.D. in nutrition , I have witnessed the fact that antidepressant medications work for some patients but not others. Thus, in my view, mental health treatment strategies should target every risk factor, including nutrition.
The role of the glycemic index
Many of the randomized controlled trials that have proven the link between diet and mental health have tested the Mediterranean diet or a slightly modified version of it . The Mediterranean diet is typically characterized by lots of vegetables – especially dark green, leafy vegetables – fruit, olive oil, whole grains, legumes and nuts, with small amounts of fish, meat and dairy products. One of the many attributes of the Mediterranean diet that may be responsible for its effect on mood is its low glycemic index .
The glycemic index is a system that ranks foods and diets according to their potential to raise blood sugar. Thus, in keeping with the observation that blood sugar fluctuations affect mood, high glycemic index diets that produce drastic spikes in blood sugar have been associated with increased risk for depression and to some extent anxiety.
High glycemic index carbohydrates include white rice, white bread, crackers and baked goods. Therefore, diets high in these foods may increase risk for depression and anxiety. Meanwhile, low glycemic index carbs, such as parboiled rice and al dente pasta, that are more slowly absorbed and produce a smaller blood sugar spike are associated with decreased risk.
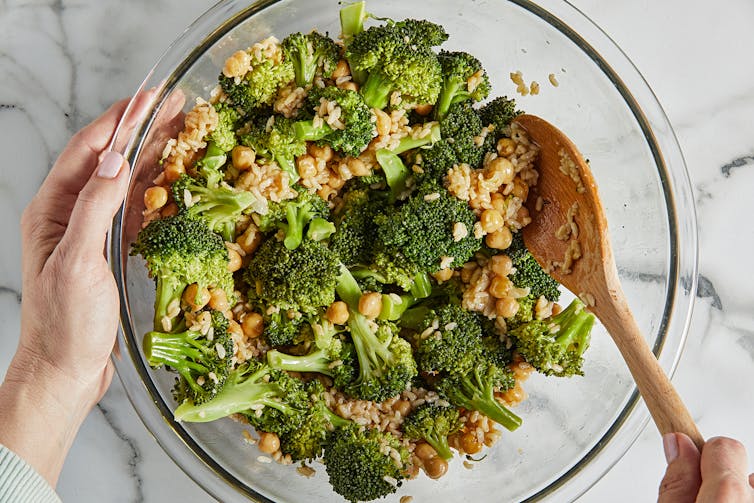
How diet affects mood
Many scientific mechanisms have been proposed to explain the connection between diet and mental health. One plausible explanation that links blood sugar fluctuations with mood is its effect on our hormones.
Every time we eat sugar or carbohydrates such as bread, rice, pasta, potatoes and crackers, the resulting rise in blood sugar triggers a cascade of hormones and signaling molecules. One example, dopamine – our brain’s pleasure signal – is the reason we can experience a “sugar high” following the consumption of dessert or baked goods. Dopamine is the body’s way of rewarding us for procuring the calories, or energy, that are necessary for survival.
Insulin is another hormone triggered by carbohydrates and sugar. Insulin’s job is to lower blood sugar levels by escorting the ingested sugar into our cells and tissues so that it can be used for energy. However, when we eat too much sugar, too many carbs, or high glycemic index carbs, the rapid increase in blood sugar prompts a drastic rise in insulin . This can result in blood sugar levels that dip below where they started.
This dip in blood sugar sparks the release of adrenaline and its cousin noradrenaline . Both of these hormones appropriately send glucose into the bloodstream to restore blood sugar to the appropriate level.
However, adrenaline influences more than just blood sugar levels. It also affects how we feel, and its release can manifest as anxiety, fear or aggression . Hence, diet affects mood through its effect on blood sugar levels, which trigger the hormones that dictate how we feel.
Interestingly, the rise in adrenaline that follows sugar and carbohydrate consumption doesn’t happen until four to five hours after eating . Thus, when eating sugar and carbs, dopamine makes us feel good in the short term; but in the long term, adrenaline can make us feel bad.
However, not everyone is equally affected. Identical meals can produce widely varying blood sugar responses in different people, depending on one’s sex , as well as genetics , sedentariness and the gut microbiome .
And it’s important to keep in mind that, as previously mentioned, mental health is complicated. So in certain circumstances, no amount of dietary optimization will overcome the social and psychological factors that may underpin one’s experience.
Nevertheless, a poor diet could certainly make a person’s experience worse and is thus relevant for anyone, especially women, hoping to optimize mental health. Research has shown that women, in particular, are more sensitive to the effects of the glycemic index and diet overall .
Unfortunately, simple solutions, such as swapping sugar for artificial sweeteners , are not an option. Research has shown that among all processed foods, artificial sweeteners and artificially sweetened beverages are most strongly associated with depression.
Optimizing mood with food
The most obvious way to stabilize blood sugar levels is to decrease sugar and carbohydrate intake . However, this is not the only way. Research has proven that simple changes can drastically mitigate volatile blood sugar fluctuations. Some strategies to stabilize blood sugar and optimize mood include:
Make low glycemic index carbohydrates such as parboiled rice, whole grain bread and al dente pasta dietary staples and be mindful of how many high glycemic index carbohydrates you consume. I give my patients this guide to increase their awareness of the glycemic index of various carbohydrates.
Eat carbohydrates earlier in the day such as breakfast or lunchtime, as opposed to later in the day, like dinner or, worse yet, as a nighttime snack. Our hormones follow a circadian rhythm, and carbs eaten earlier in the day produce a smaller blood sugar spike compared with carbs eaten later in the day.
Avoid eating carbohydrates on their own, such as snacking on a box of crackers or downing a bowl of rice. Always strive to combine carbohydrates with proteins such as beans, nuts, meat and fish, or with healthy fats such as olive oil and avocado. The combination of nutrients slows down the digestion of carbohydrates and thereby produces a smaller blood sugar spike.
Eat carbohydrates at the end of the meal, after eating vegetables and protein first. Just changing the order in which foods are eaten can drastically lower the blood sugar spike that comes after .
Eat a salad dressed with olive oil and vinegar prior to eating carbohydrates. The combination of vegetables, acid from the vinegar and fat from the olive oil, all work together to slow carbohydrate absorption and minimize the resultant blood sugar spike.
- Mental health
- Carbohydrates
- Blood sugar
- Mediterranean diet
- Glycemic index
- High blood sugar
- Antidepressant medications

Manager, Regional Training Hub

Head of Evidence to Action

Supply Chain - Assistant/Associate Professor (Tenure-Track)

OzGrav Postdoctoral Research Fellow

Casual Facilitator: GERRIC Student Programs - Arts, Design and Architecture
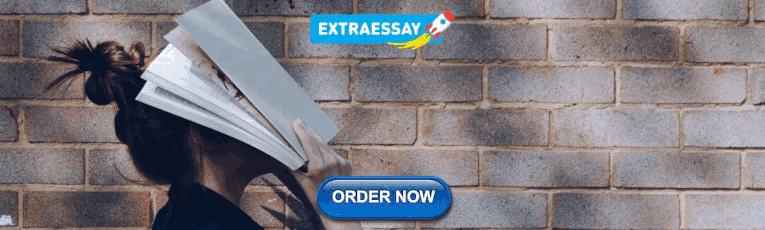
IMAGES
COMMENTS
5. Phrase your hypothesis in three ways. To identify the variables, you can write a simple prediction in if…then form. The first part of the sentence states the independent variable and the second part states the dependent variable. If a first-year student starts attending more lectures, then their exam scores will improve.
A causal hypothesis is a statement that predicts a cause-and-effect relationship between variables in a study. It serves as a guide to study design, data collection, and interpretation of results. This thesis statement segment aims to provide you with clear examples of causal hypotheses across diverse fields, along with a step-by-step guide and ...
A hypothesis is a statement that explains the predictions and reasoning of your research—an "educated guess" about how your scientific experiments will end. As a fundamental part of the scientific method, a good hypothesis is carefully written, but even the simplest ones can be difficult to put into words. ... Cause and effect.
They are used in experimental research designed to determine cause-and-effect relationships, such as randomized controlled trials in medical research or controlled experiments in psychology. ... This means you should be able to conduct experiments or make observations that can support or refute the hypothesis. Avoid vague or broad statements ...
A causal hypothesis proposes a cause-and-effect interaction between variables. Example: " Long-term alcohol use causes liver damage." Note that some of the types of research hypothesis mentioned above might overlap. The types of hypothesis chosen will depend on the research question and the objective of the study. Research hypothesis examples
A hypothesis states a presumed relationship between two variables in a way that can be tested with empirical data. It may take the form of a cause-effect statement, or an "if x,...then y" statement. The cause is called the independent variable; and the effect is called the dependent variable. Relationships can be of several forms: linear, or ...
Causal hypothesis - An effect on dependent variable is predicted from manipulation of independent variable: A change into a high-fiber diet (independent variable) will reduce the blood sugar level (dependent variable) of the patient. Null hypothesis - A negative statement indicating no relationship or difference between 2 variables
A hypothesis is an educated guess or prediction of what will happen. In science, a hypothesis proposes a relationship between factors called variables. ... then statement to establish cause and effect on the variables. If you make a change to the independent variable, then the dependent variable will respond. Here's an example of a hypothesis:
In our hypothesis statement example above, the two variables are wildfires and tornadoes, and our assumed relationship between the two is a causal one (wildfires cause tornadoes). It is clear from our example above what we will be investigating: the relationship between wildfires and tornadoes. A strong hypothesis statement should be: Clear
Step 5: Phrase your hypothesis in three ways. To identify the variables, you can write a simple prediction in if … then form. The first part of the sentence states the independent variable and the second part states the dependent variable. If a first-year student starts attending more lectures, then their exam scores will improve.
The key principle of establishing cause and effect is proving that the effects seen in the experiment happened after the cause. This seems to be an extremely obvious statement, but that is not always the case. Natural phenomena are complicated and intertwined, often overlapping and making it difficult to establish a natural order.
A hypothesis is a statement that predicts the relationship between a set of variables. ... Sam's second hypothesis is a causal hypothesis, because it signifies a cause-and-effect relationship ...
A hypothesis is a statement describing a researcher's expectation regarding the research findings. Hypotheses in quantitative research are nomothetic causal explanations that the researcher expects to demonstrate. ... For nomothetic causal explanations, an association must be plausible and nonspurious, and the cause must precede the effect in ...
Ideally, the cause must occur before the effect. You should review the timeline of two or more separate events to determine the independent variables (cause) from the dependent variables (effect) before developing a hypothesis. If the cause occurs before the effect, you can link cause and effect and develop a hypothesis.
Revised on June 22, 2023. Correlation means there is a statistical association between variables. Causation means that a change in one variable causes a change in another variable. In research, you might have come across the phrase "correlation doesn't imply causation.". Correlation and causation are two related ideas, but understanding ...
Generalizing causal statements depends on methods other than formal sampling theory, given that experiments with both random selection and random assignment are rare and surveys provide causal tests that are flawed when compared to experiments. ... failure to reject implies that the hypothesis of a general cause-effect relationship remains ...
Hill's Criteria of Causation. Determining whether a causal relationship exists requires far more in-depth subject area knowledge and contextual information than you can include in a hypothesis test. In 1965, Austin Hill, a medical statistician, tackled this question in a paper* that's become the standard.
Trouble establishing cause and effect; Researchers might identify that two variables are connected, but struggle to determine which is the cause and which variable is the effect. Risk of contamination; There's always the risk that people outside your market or area of study could affect the results of your research.
Study with Quizlet and memorize flashcards containing terms like Hypothesis, Dependent variable, Independent variable and more. ... a testable statement about the empirical relationship between cause and effect. Dependent variable. the variable that represents the effect in a casual explanation.
The cause is the initiating event or situation, and the effect is the result of the cause. The table below gives some examples of cause and effect. Cause. Effect. The lightning struck the tree ...
Researchers who instead decide to rely on observational data often attempt to deal with its weaknesses by cautiously avoiding causal language: They refer to "associations," "relationships," or tentative "links" between variables instead of clear cause-effect relationships, and they usually add a general disclaimer ("Of course, as ...
Study with Quizlet and memorize flashcards containing terms like QUESTION 1 The Ishikawa (fishbone) diagram is characterized by the following: A. It provides a quantitative relationship between an effect and multiple causes B. It creates a visual image of multiple potential causes and a single effect C. It always addresses four domains of causes: materials, management, measurement, and men ...
Correlation doesn't imply causation, but causation suggests that correlation exists. The Venn diagram shows the relationship between the two. Understanding why causation implies correlation is intuitive. If increasing medicine dosage decreases the symptoms, you'll find a negative correlation between those variables.
BBC Verify examined claims made by Harris, about her record and Trump's on the economy, abortion and immigration.
WASHINGTON (AP) — Federal Reserve Chair Jerome Powell all but proclaimed victory in the fight against inflation and signaled that interest rate cuts are coming in a much-anticipated speech Friday in Jackson Hole, Wyoming.. Under Powell, the Fed raised its benchmark rate to the highest level in 23 years to subdue inflation that two years ago was running at the hottest pace in more than four ...
High-carb diets, especially of ultraprocessed foods, create blood sugar spikes, while diets high in leafy, green vegetables and whole grains are absorbed more slowly and produce smaller fluctuations.
America's top central banker has unambiguously signaled that lower interest rates are finally on the horizon, marking a crucial milestone for the Federal Reserve's historic — and, so far ...
An early-season outbreak of epizootic hemorrhagic disease (EHD) in deer, which started in the northern region of Indiana this year, has been detected by the Indiana Department of Natural Resources (DNR). Humans are not at risk for contracting EHD. EHD is a viral disease that commonly affects white-tailed deer and is transmitted by biting midges, aka sand gnats or "no-see-ums." Cases ...
Rescuers are scrambling to evacuate flooded communities after heavy rains inundated parts of Bangladesh and northeast India, killing at least 36 people and causing rivers on both sides of the ...