
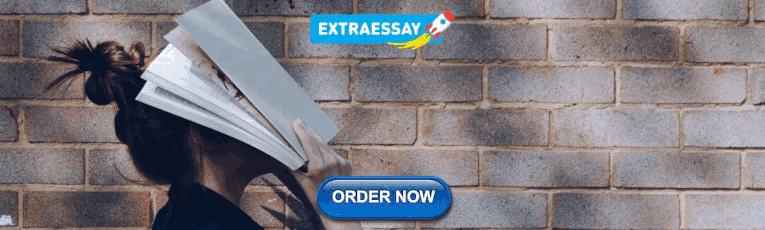
Research Topics & Ideas: Data Science
50 Topic Ideas To Kickstart Your Research Project
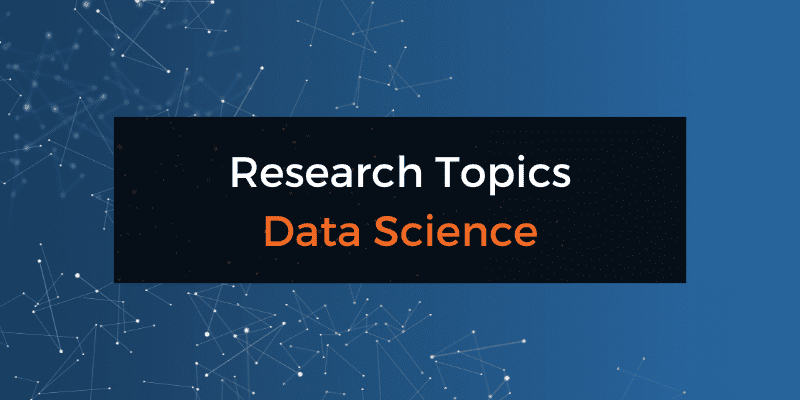
If you’re just starting out exploring data science-related topics for your dissertation, thesis or research project, you’ve come to the right place. In this post, we’ll help kickstart your research by providing a hearty list of data science and analytics-related research ideas , including examples from recent studies.
PS – This is just the start…
We know it’s exciting to run through a list of research topics, but please keep in mind that this list is just a starting point . These topic ideas provided here are intentionally broad and generic , so keep in mind that you will need to develop them further. Nevertheless, they should inspire some ideas for your project.
To develop a suitable research topic, you’ll need to identify a clear and convincing research gap , and a viable plan to fill that gap. If this sounds foreign to you, check out our free research topic webinar that explores how to find and refine a high-quality research topic, from scratch. Alternatively, consider our 1-on-1 coaching service .
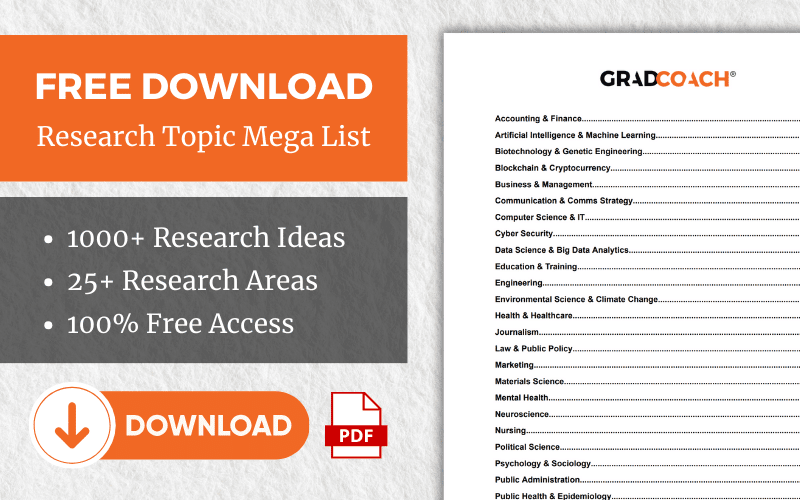
Data Science-Related Research Topics
- Developing machine learning models for real-time fraud detection in online transactions.
- The use of big data analytics in predicting and managing urban traffic flow.
- Investigating the effectiveness of data mining techniques in identifying early signs of mental health issues from social media usage.
- The application of predictive analytics in personalizing cancer treatment plans.
- Analyzing consumer behavior through big data to enhance retail marketing strategies.
- The role of data science in optimizing renewable energy generation from wind farms.
- Developing natural language processing algorithms for real-time news aggregation and summarization.
- The application of big data in monitoring and predicting epidemic outbreaks.
- Investigating the use of machine learning in automating credit scoring for microfinance.
- The role of data analytics in improving patient care in telemedicine.
- Developing AI-driven models for predictive maintenance in the manufacturing industry.
- The use of big data analytics in enhancing cybersecurity threat intelligence.
- Investigating the impact of sentiment analysis on brand reputation management.
- The application of data science in optimizing logistics and supply chain operations.
- Developing deep learning techniques for image recognition in medical diagnostics.
- The role of big data in analyzing climate change impacts on agricultural productivity.
- Investigating the use of data analytics in optimizing energy consumption in smart buildings.
- The application of machine learning in detecting plagiarism in academic works.
- Analyzing social media data for trends in political opinion and electoral predictions.
- The role of big data in enhancing sports performance analytics.
- Developing data-driven strategies for effective water resource management.
- The use of big data in improving customer experience in the banking sector.
- Investigating the application of data science in fraud detection in insurance claims.
- The role of predictive analytics in financial market risk assessment.
- Developing AI models for early detection of network vulnerabilities.
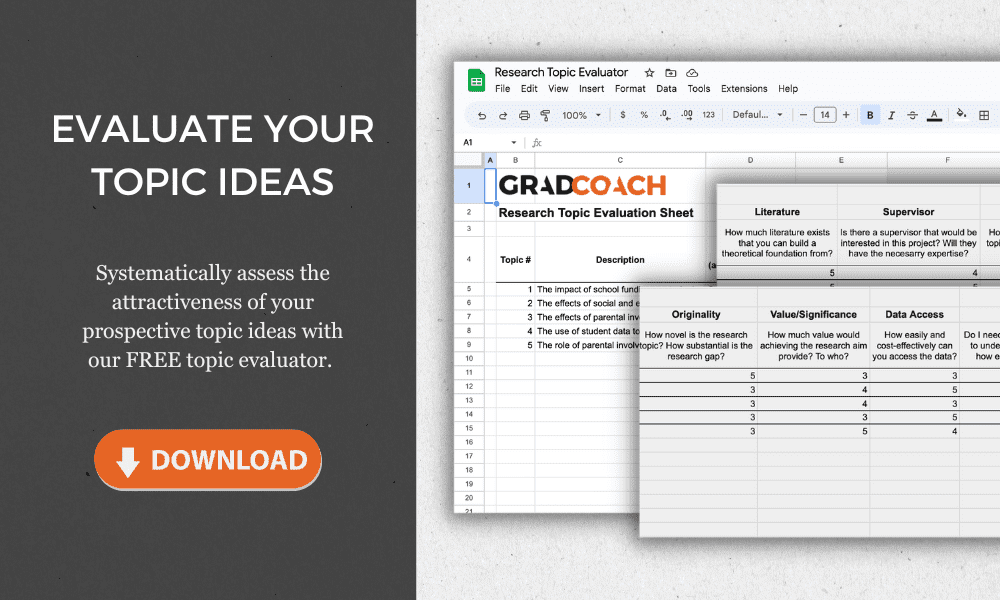
Data Science Research Ideas (Continued)
- The application of big data in public transportation systems for route optimization.
- Investigating the impact of big data analytics on e-commerce recommendation systems.
- The use of data mining techniques in understanding consumer preferences in the entertainment industry.
- Developing predictive models for real estate pricing and market trends.
- The role of big data in tracking and managing environmental pollution.
- Investigating the use of data analytics in improving airline operational efficiency.
- The application of machine learning in optimizing pharmaceutical drug discovery.
- Analyzing online customer reviews to inform product development in the tech industry.
- The role of data science in crime prediction and prevention strategies.
- Developing models for analyzing financial time series data for investment strategies.
- The use of big data in assessing the impact of educational policies on student performance.
- Investigating the effectiveness of data visualization techniques in business reporting.
- The application of data analytics in human resource management and talent acquisition.
- Developing algorithms for anomaly detection in network traffic data.
- The role of machine learning in enhancing personalized online learning experiences.
- Investigating the use of big data in urban planning and smart city development.
- The application of predictive analytics in weather forecasting and disaster management.
- Analyzing consumer data to drive innovations in the automotive industry.
- The role of data science in optimizing content delivery networks for streaming services.
- Developing machine learning models for automated text classification in legal documents.
- The use of big data in tracking global supply chain disruptions.
- Investigating the application of data analytics in personalized nutrition and fitness.
- The role of big data in enhancing the accuracy of geological surveying for natural resource exploration.
- Developing predictive models for customer churn in the telecommunications industry.
- The application of data science in optimizing advertisement placement and reach.
Recent Data Science-Related Studies
While the ideas we’ve presented above are a decent starting point for finding a research topic, they are fairly generic and non-specific. So, it helps to look at actual studies in the data science and analytics space to see how this all comes together in practice.
Below, we’ve included a selection of recent studies to help refine your thinking. These are actual studies, so they can provide some useful insight as to what a research topic looks like in practice.
- Data Science in Healthcare: COVID-19 and Beyond (Hulsen, 2022)
- Auto-ML Web-application for Automated Machine Learning Algorithm Training and evaluation (Mukherjee & Rao, 2022)
- Survey on Statistics and ML in Data Science and Effect in Businesses (Reddy et al., 2022)
- Visualization in Data Science VDS @ KDD 2022 (Plant et al., 2022)
- An Essay on How Data Science Can Strengthen Business (Santos, 2023)
- A Deep study of Data science related problems, application and machine learning algorithms utilized in Data science (Ranjani et al., 2022)
- You Teach WHAT in Your Data Science Course?!? (Posner & Kerby-Helm, 2022)
- Statistical Analysis for the Traffic Police Activity: Nashville, Tennessee, USA (Tufail & Gul, 2022)
- Data Management and Visual Information Processing in Financial Organization using Machine Learning (Balamurugan et al., 2022)
- A Proposal of an Interactive Web Application Tool QuickViz: To Automate Exploratory Data Analysis (Pitroda, 2022)
- Applications of Data Science in Respective Engineering Domains (Rasool & Chaudhary, 2022)
- Jupyter Notebooks for Introducing Data Science to Novice Users (Fruchart et al., 2022)
- Towards a Systematic Review of Data Science Programs: Themes, Courses, and Ethics (Nellore & Zimmer, 2022)
- Application of data science and bioinformatics in healthcare technologies (Veeranki & Varshney, 2022)
- TAPS Responsibility Matrix: A tool for responsible data science by design (Urovi et al., 2023)
- Data Detectives: A Data Science Program for Middle Grade Learners (Thompson & Irgens, 2022)
- MACHINE LEARNING FOR NON-MAJORS: A WHITE BOX APPROACH (Mike & Hazzan, 2022)
- COMPONENTS OF DATA SCIENCE AND ITS APPLICATIONS (Paul et al., 2022)
- Analysis on the Application of Data Science in Business Analytics (Wang, 2022)
As you can see, these research topics are a lot more focused than the generic topic ideas we presented earlier. So, for you to develop a high-quality research topic, you’ll need to get specific and laser-focused on a specific context with specific variables of interest. In the video below, we explore some other important things you’ll need to consider when crafting your research topic.
Get 1-On-1 Help
If you’re still unsure about how to find a quality research topic, check out our Research Topic Kickstarter service, which is the perfect starting point for developing a unique, well-justified research topic.
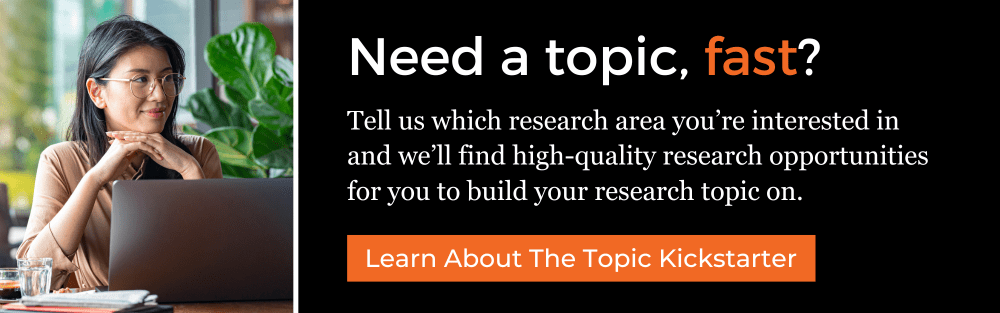
I have to submit dissertation. can I get any help
Submit a Comment Cancel reply
Your email address will not be published. Required fields are marked *
Save my name, email, and website in this browser for the next time I comment.
- Print Friendly
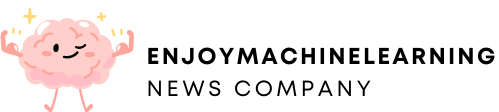
37 Research Topics In Data Science To Stay On Top Of
- February 22, 2024
As a data scientist, staying on top of the latest research in your field is essential.
The data science landscape changes rapidly, and new techniques and tools are constantly being developed.
To keep up with the competition, you need to be aware of the latest trends and topics in data science research.
In this article, we will provide an overview of 37 hot research topics in data science.
We will discuss each topic in detail, including its significance and potential applications.
These topics could be an idea for a thesis or simply topics you can research independently.
Stay tuned – this is one blog post you don’t want to miss!
37 Research Topics in Data Science
1.) predictive modeling.
Predictive modeling is a significant portion of data science and a topic you must be aware of.
Simply put, it is the process of using historical data to build models that can predict future outcomes.
Predictive modeling has many applications, from marketing and sales to financial forecasting and risk management.
As businesses increasingly rely on data to make decisions, predictive modeling is becoming more and more important.
While it can be complex, predictive modeling is a powerful tool that gives businesses a competitive advantage.
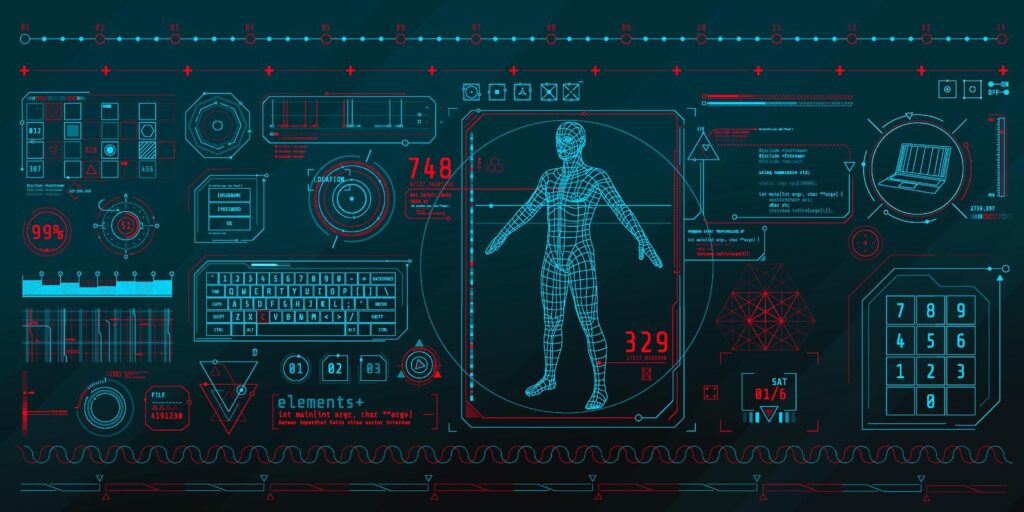
2.) Big Data Analytics
These days, it seems like everyone is talking about big data.
And with good reason – organizations of all sizes are sitting on mountains of data, and they’re increasingly turning to data scientists to help them make sense of it all.
But what exactly is big data? And what does it mean for data science?
Simply put, big data is a term used to describe datasets that are too large and complex for traditional data processing techniques.
Big data typically refers to datasets of a few terabytes or more.
But size isn’t the only defining characteristic – big data is also characterized by its high Velocity (the speed at which data is generated), Variety (the different types of data), and Volume (the amount of the information).
Given the enormity of big data, it’s not surprising that organizations are struggling to make sense of it all.
That’s where data science comes in.
Data scientists use various methods to wrangle big data, including distributed computing and other decentralized technologies.
With the help of data science, organizations are beginning to unlock the hidden value in their big data.
By harnessing the power of big data analytics, they can improve their decision-making, better understand their customers, and develop new products and services.
3.) Auto Machine Learning
Auto machine learning is a research topic in data science concerned with developing algorithms that can automatically learn from data without intervention.
This area of research is vital because it allows data scientists to automate the process of writing code for every dataset.
This allows us to focus on other tasks, such as model selection and validation.
Auto machine learning algorithms can learn from data in a hands-off way for the data scientist – while still providing incredible insights.
This makes them a valuable tool for data scientists who either don’t have the skills to do their own analysis or are struggling.
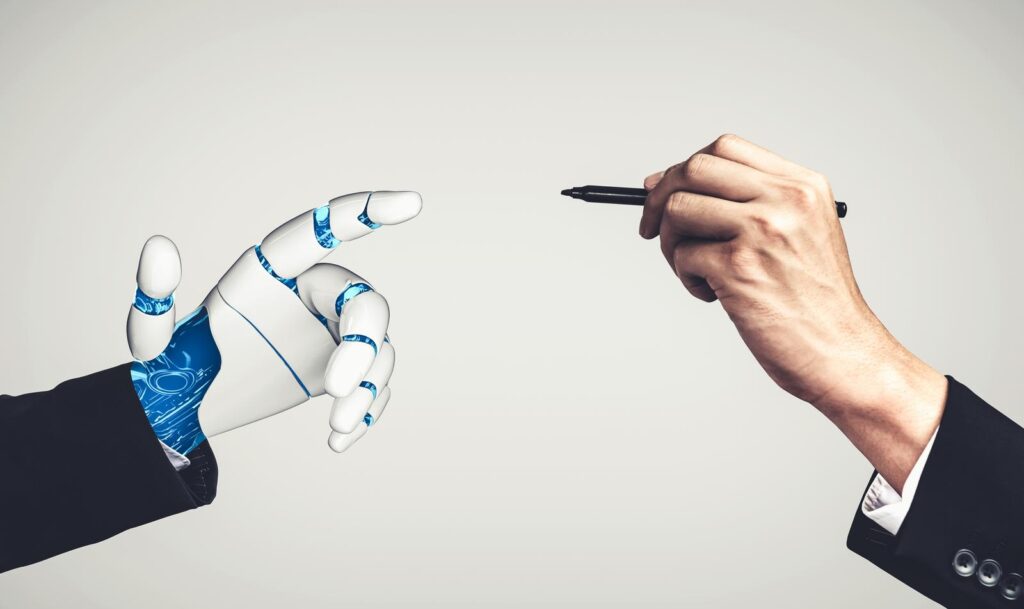
4.) Text Mining
Text mining is a research topic in data science that deals with text data extraction.
This area of research is important because it allows us to get as much information as possible from the vast amount of text data available today.
Text mining techniques can extract information from text data, such as keywords, sentiments, and relationships.
This information can be used for various purposes, such as model building and predictive analytics.
5.) Natural Language Processing
Natural language processing is a data science research topic that analyzes human language data.
This area of research is important because it allows us to understand and make sense of the vast amount of text data available today.
Natural language processing techniques can build predictive and interactive models from any language data.
Natural Language processing is pretty broad, and recent advances like GPT-3 have pushed this topic to the forefront.
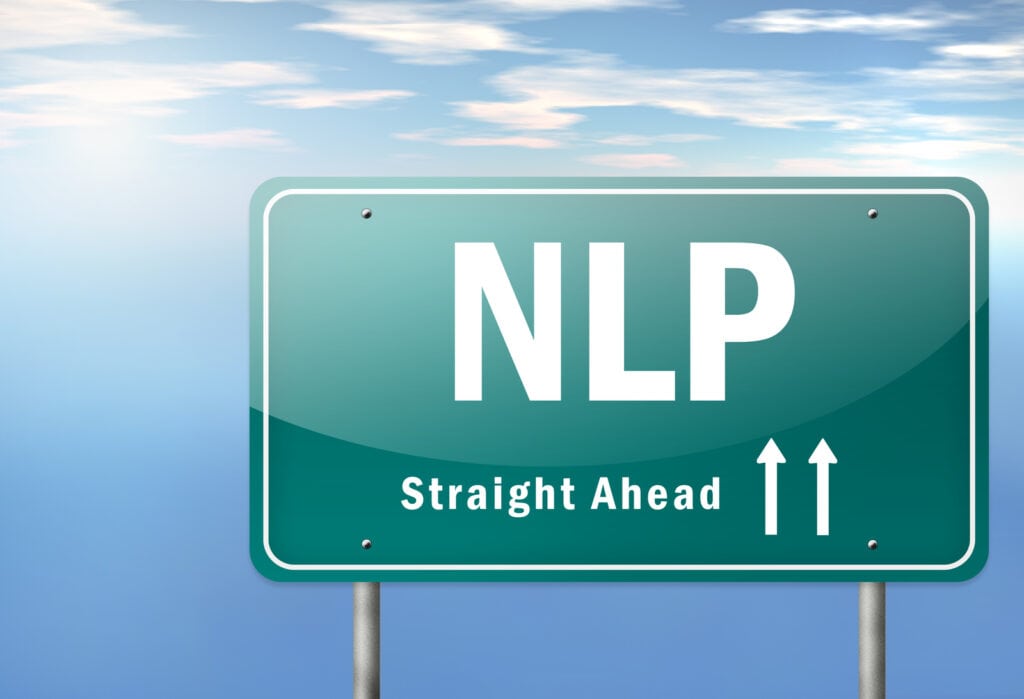
6.) Recommender Systems
Recommender systems are an exciting topic in data science because they allow us to make better products, services, and content recommendations.
Businesses can better understand their customers and their needs by using recommender systems.
This, in turn, allows them to develop better products and services that meet the needs of their customers.
Recommender systems are also used to recommend content to users.
This can be done on an individual level or at a group level.
Think about Netflix, for example, always knowing what you want to watch!
Recommender systems are a valuable tool for businesses and users alike.
7.) Deep Learning
Deep learning is a research topic in data science that deals with artificial neural networks.
These networks are composed of multiple layers, and each layer is formed from various nodes.
Deep learning networks can learn from data similarly to how humans learn, irrespective of the data distribution.
This makes them a valuable tool for data scientists looking to build models that can learn from data independently.
The deep learning network has become very popular in recent years because of its ability to achieve state-of-the-art results on various tasks.
There seems to be a new SOTA deep learning algorithm research paper on https://arxiv.org/ every single day!
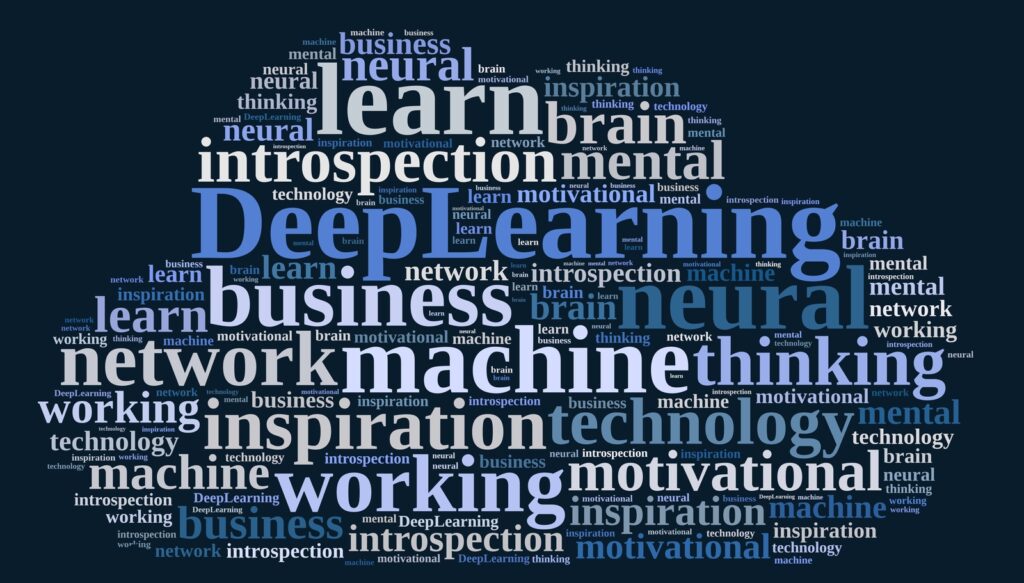
8.) Reinforcement Learning
Reinforcement learning is a research topic in data science that deals with algorithms that can learn on multiple levels from interactions with their environment.
This area of research is essential because it allows us to develop algorithms that can learn non-greedy approaches to decision-making, allowing businesses and companies to win in the long term compared to the short.
9.) Data Visualization
Data visualization is an excellent research topic in data science because it allows us to see our data in a way that is easy to understand.
Data visualization techniques can be used to create charts, graphs, and other visual representations of data.
This allows us to see the patterns and trends hidden in our data.
Data visualization is also used to communicate results to others.
This allows us to share our findings with others in a way that is easy to understand.
There are many ways to contribute to and learn about data visualization.
Some ways include attending conferences, reading papers, and contributing to open-source projects.
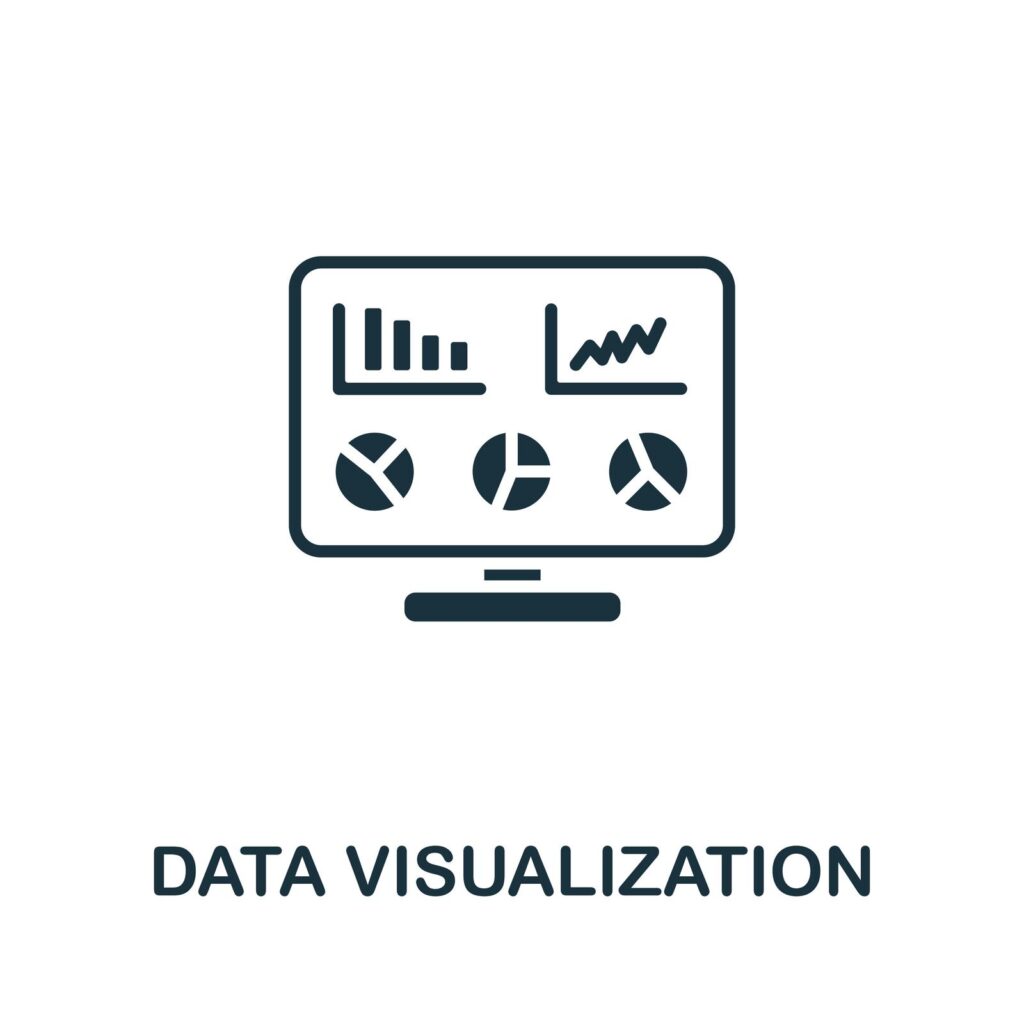
10.) Predictive Maintenance
Predictive maintenance is a hot topic in data science because it allows us to prevent failures before they happen.
This is done using data analytics to predict when a failure will occur.
This allows us to take corrective action before the failure actually happens.
While this sounds simple, avoiding false positives while keeping recall is challenging and an area wide open for advancement.
11.) Financial Analysis
Financial analysis is an older topic that has been around for a while but is still a great field where contributions can be felt.
Current researchers are focused on analyzing macroeconomic data to make better financial decisions.
This is done by analyzing the data to identify trends and patterns.
Financial analysts can use this information to make informed decisions about where to invest their money.
Financial analysis is also used to predict future economic trends.
This allows businesses and individuals to prepare for potential financial hardships and enable companies to be cash-heavy during good economic conditions.
Overall, financial analysis is a valuable tool for anyone looking to make better financial decisions.
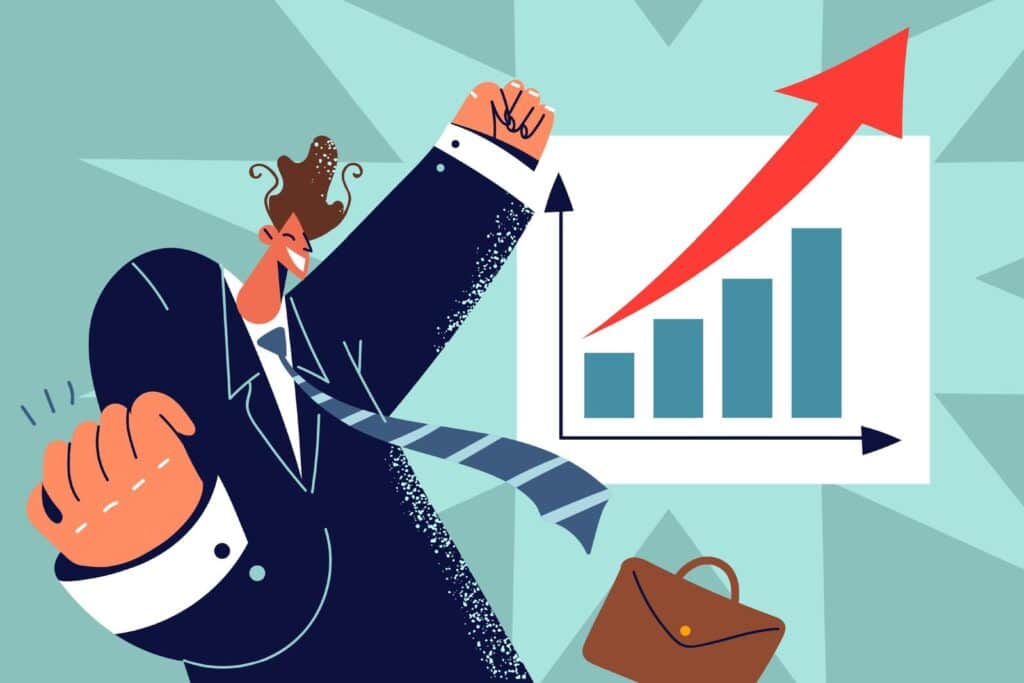
12.) Image Recognition
Image recognition is one of the hottest topics in data science because it allows us to identify objects in images.
This is done using artificial intelligence algorithms that can learn from data and understand what objects you’re looking for.
This allows us to build models that can accurately recognize objects in images and video.
This is a valuable tool for businesses and individuals who want to be able to identify objects in images.
Think about security, identification, routing, traffic, etc.
Image Recognition has gained a ton of momentum recently – for a good reason.
13.) Fraud Detection
Fraud detection is a great topic in data science because it allows us to identify fraudulent activity before it happens.
This is done by analyzing data to look for patterns and trends that may be associated with the fraud.
Once our machine learning model recognizes some of these patterns in real time, it immediately detects fraud.
This allows us to take corrective action before the fraud actually happens.
Fraud detection is a valuable tool for anyone who wants to protect themselves from potential fraudulent activity.
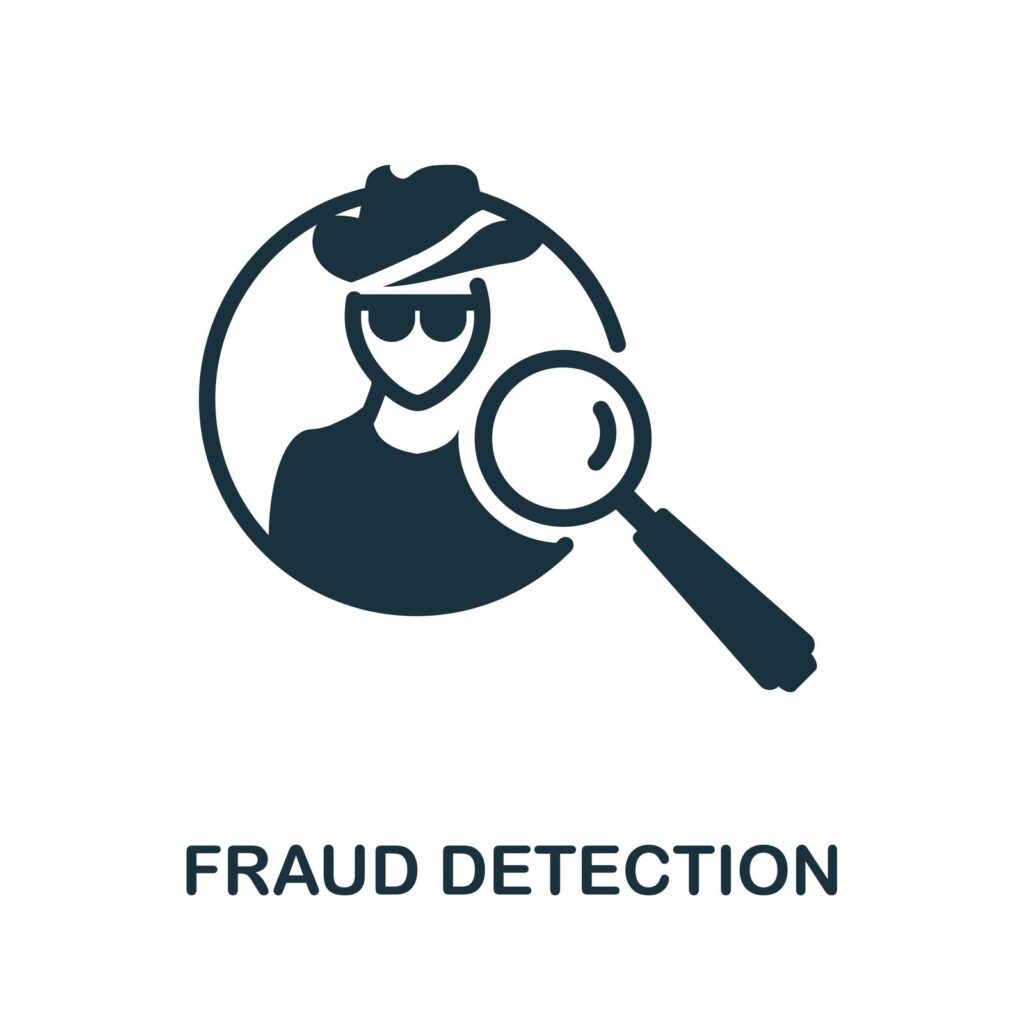
14.) Web Scraping
Web scraping is a controversial topic in data science because it allows us to collect data from the web, which is usually data you do not own.
This is done by extracting data from websites using scraping tools that are usually custom-programmed.
This allows us to collect data that would otherwise be inaccessible.
For obvious reasons, web scraping is a unique tool – giving you data your competitors would have no chance of getting.
I think there is an excellent opportunity to create new and innovative ways to make scraping accessible for everyone, not just those who understand Selenium and Beautiful Soup.
15.) Social Media Analysis
Social media analysis is not new; many people have already created exciting and innovative algorithms to study this.
However, it is still a great data science research topic because it allows us to understand how people interact on social media.
This is done by analyzing data from social media platforms to look for insights, bots, and recent societal trends.
Once we understand these practices, we can use this information to improve our marketing efforts.
For example, if we know that a particular demographic prefers a specific type of content, we can create more content that appeals to them.
Social media analysis is also used to understand how people interact with brands on social media.
This allows businesses to understand better what their customers want and need.
Overall, social media analysis is valuable for anyone who wants to improve their marketing efforts or understand how customers interact with brands.
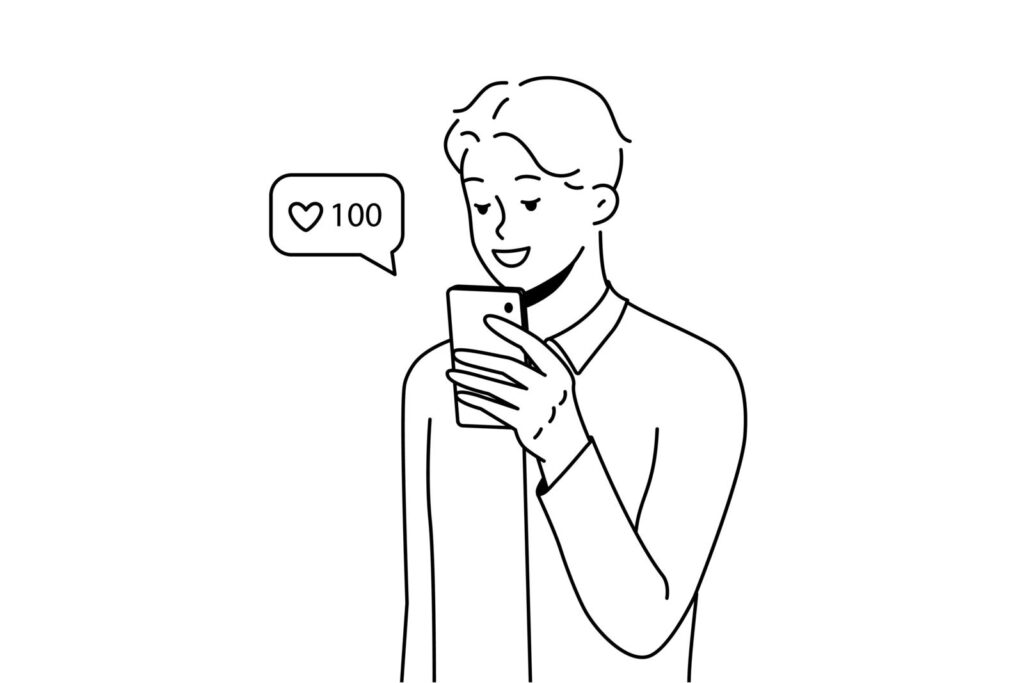
16.) GPU Computing
GPU computing is a fun new research topic in data science because it allows us to process data much faster than traditional CPUs .
Due to how GPUs are made, they’re incredibly proficient at intense matrix operations, outperforming traditional CPUs by very high margins.
While the computation is fast, the coding is still tricky.
There is an excellent research opportunity to bring these innovations to non-traditional modules, allowing data science to take advantage of GPU computing outside of deep learning.
17.) Quantum Computing
Quantum computing is a new research topic in data science and physics because it allows us to process data much faster than traditional computers.
It also opens the door to new types of data.
There are just some problems that can’t be solved utilizing outside of the classical computer.
For example, if you wanted to understand how a single atom moved around, a classical computer couldn’t handle this problem.
You’ll need to utilize a quantum computer to handle quantum mechanics problems.
This may be the “hottest” research topic on the planet right now, with some of the top researchers in computer science and physics worldwide working on it.
You could be too.
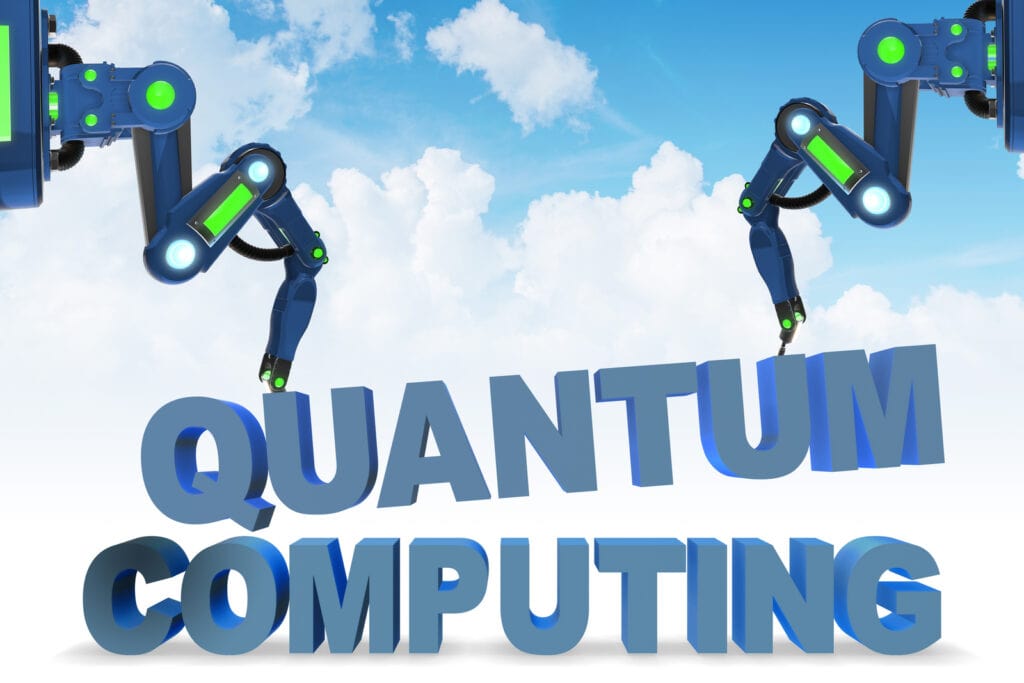
18.) Genomics
Genomics may be the only research topic that can compete with quantum computing regarding the “number of top researchers working on it.”
Genomics is a fantastic intersection of data science because it allows us to understand how genes work.
This is done by sequencing the DNA of different organisms to look for insights into our and other species.
Once we understand these patterns, we can use this information to improve our understanding of diseases and create new and innovative treatments for them.
Genomics is also used to study the evolution of different species.
Genomics is the future and a field begging for new and exciting research professionals to take it to the next step.
19.) Location-based services
Location-based services are an old and time-tested research topic in data science.
Since GPS and 4g cell phone reception became a thing, we’ve been trying to stay informed about how humans interact with their environment.
This is done by analyzing data from GPS tracking devices, cell phone towers, and Wi-Fi routers to look for insights into how humans interact.
Once we understand these practices, we can use this information to improve our geotargeting efforts, improve maps, find faster routes, and improve cohesion throughout a community.
Location-based services are used to understand the user, something every business could always use a little bit more of.
While a seemingly “stale” field, location-based services have seen a revival period with self-driving cars.
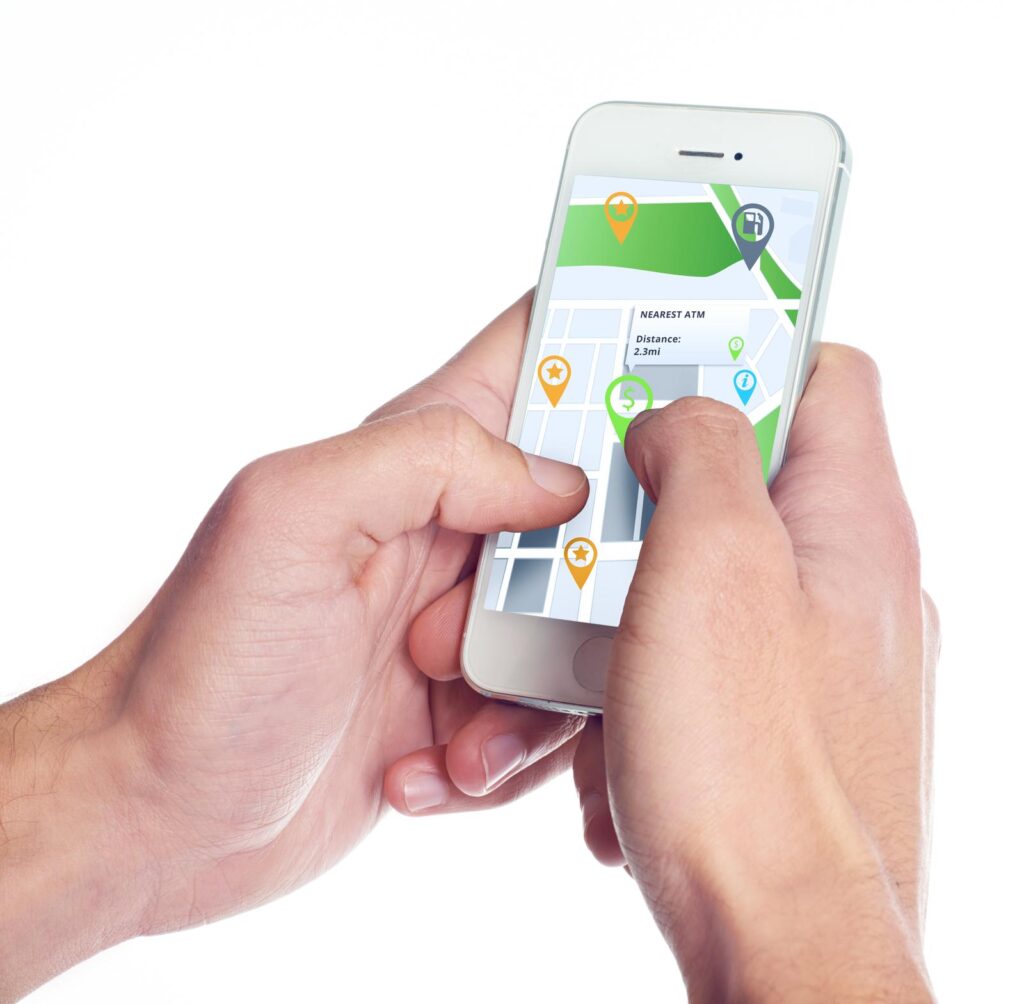
20.) Smart City Applications
Smart city applications are all the rage in data science research right now.
By harnessing the power of data, cities can become more efficient and sustainable.
But what exactly are smart city applications?
In short, they are systems that use data to improve city infrastructure and services.
This can include anything from traffic management and energy use to waste management and public safety.
Data is collected from various sources, including sensors, cameras, and social media.
It is then analyzed to identify tendencies and habits.
This information can make predictions about future needs and optimize city resources.
As more and more cities strive to become “smart,” the demand for data scientists with expertise in smart city applications is only growing.
21.) Internet Of Things (IoT)
The Internet of Things, or IoT, is exciting and new data science and sustainability research topic.
IoT is a network of physical objects embedded with sensors and connected to the internet.
These objects can include everything from alarm clocks to refrigerators; they’re all connected to the internet.
That means that they can share data with computers.
And that’s where data science comes in.
Data scientists are using IoT data to learn everything from how people use energy to how traffic flows through a city.
They’re also using IoT data to predict when an appliance will break down or when a road will be congested.
Really, the possibilities are endless.
With such a wide-open field, it’s easy to see why IoT is being researched by some of the top professionals in the world.
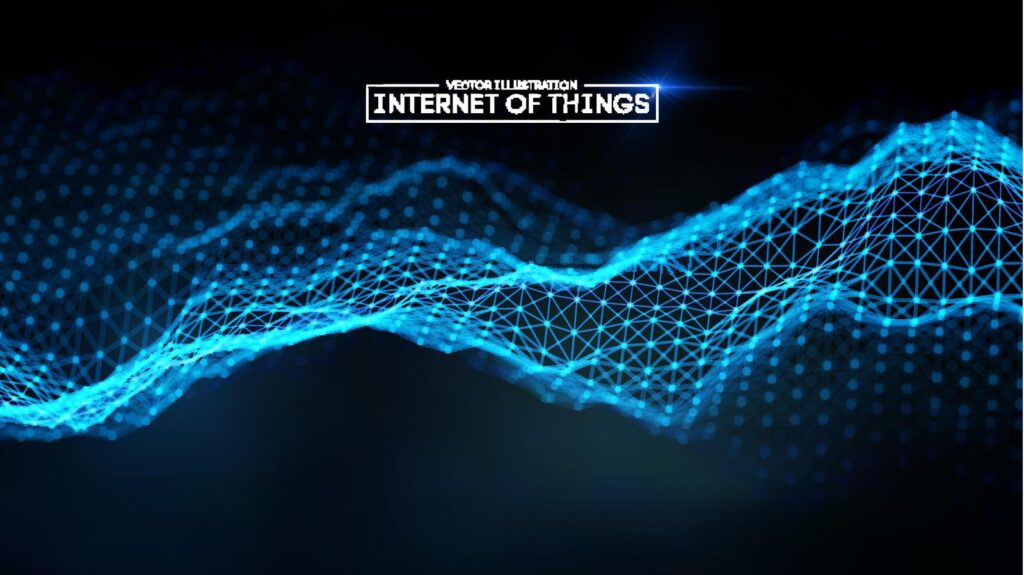
22.) Cybersecurity
Cybersecurity is a relatively new research topic in data science and in general, but it’s already garnering a lot of attention from businesses and organizations.
After all, with the increasing number of cyber attacks in recent years, it’s clear that we need to find better ways to protect our data.
While most of cybersecurity focuses on infrastructure, data scientists can leverage historical events to find potential exploits to protect their companies.
Sometimes, looking at a problem from a different angle helps, and that’s what data science brings to cybersecurity.
Also, data science can help to develop new security technologies and protocols.
As a result, cybersecurity is a crucial data science research area and one that will only become more important in the years to come.
23.) Blockchain
Blockchain is an incredible new research topic in data science for several reasons.
First, it is a distributed database technology that enables secure, transparent, and tamper-proof transactions.
Did someone say transmitting data?
This makes it an ideal platform for tracking data and transactions in various industries.
Second, blockchain is powered by cryptography, which not only makes it highly secure – but is a familiar foe for data scientists.
Finally, blockchain is still in its early stages of development, so there is much room for research and innovation.
As a result, blockchain is a great new research topic in data science that vows to revolutionize how we store, transmit and manage data.
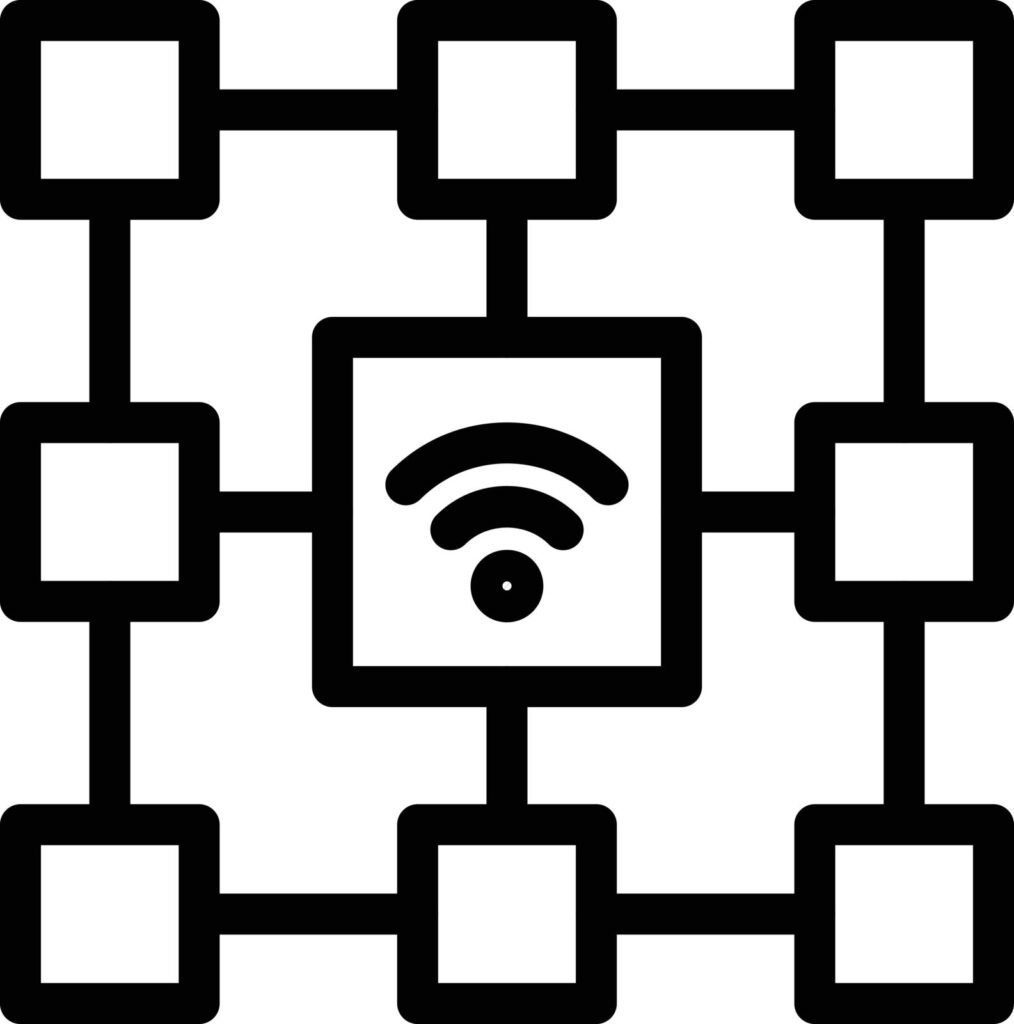
24.) Sustainability
Sustainability is a relatively new research topic in data science, but it is gaining traction quickly.
To keep up with this demand, The Wharton School of the University of Pennsylvania has started to offer an MBA in Sustainability .
This demand isn’t shocking, and some of the reasons include the following:
Sustainability is an important issue that is relevant to everyone.
Datasets on sustainability are constantly growing and changing, making it an exciting challenge for data scientists.
There hasn’t been a “set way” to approach sustainability from a data perspective, making it an excellent opportunity for interdisciplinary research.
As data science grows, sustainability will likely become an increasingly important research topic.
25.) Educational Data
Education has always been a great topic for research, and with the advent of big data, educational data has become an even richer source of information.
By studying educational data, researchers can gain insights into how students learn, what motivates them, and what barriers these students may face.
Besides, data science can be used to develop educational interventions tailored to individual students’ needs.
Imagine being the researcher that helps that high schooler pass mathematics; what an incredible feeling.
With the increasing availability of educational data, data science has enormous potential to improve the quality of education.
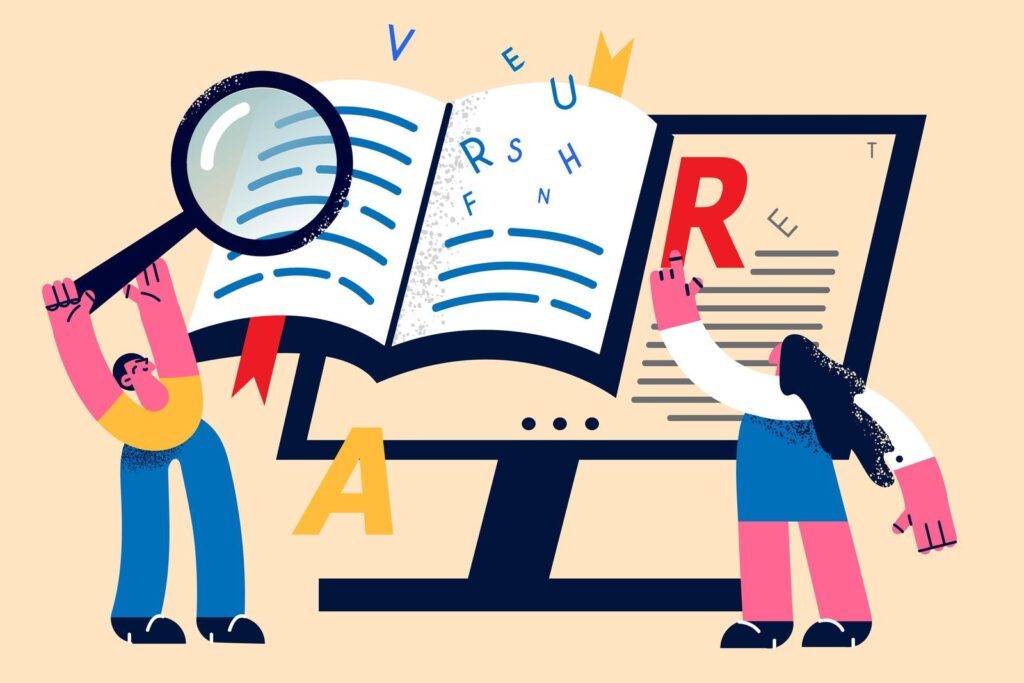
26.) Politics
As data science continues to evolve, so does the scope of its applications.
Originally used primarily for business intelligence and marketing, data science is now applied to various fields, including politics.
By analyzing large data sets, political scientists (data scientists with a cooler name) can gain valuable insights into voting patterns, campaign strategies, and more.
Further, data science can be used to forecast election results and understand the effects of political events on public opinion.
With the wealth of data available, there is no shortage of research opportunities in this field.
As data science evolves, so does our understanding of politics and its role in our world.
27.) Cloud Technologies
Cloud technologies are a great research topic.
It allows for the outsourcing and sharing of computer resources and applications all over the internet.
This lets organizations save money on hardware and maintenance costs while providing employees access to the latest and greatest software and applications.
I believe there is an argument that AWS could be the greatest and most technologically advanced business ever built (Yes, I know it’s only part of the company).
Besides, cloud technologies can help improve team members’ collaboration by allowing them to share files and work on projects together in real-time.
As more businesses adopt cloud technologies, data scientists must stay up-to-date on the latest trends in this area.
By researching cloud technologies, data scientists can help organizations to make the most of this new and exciting technology.
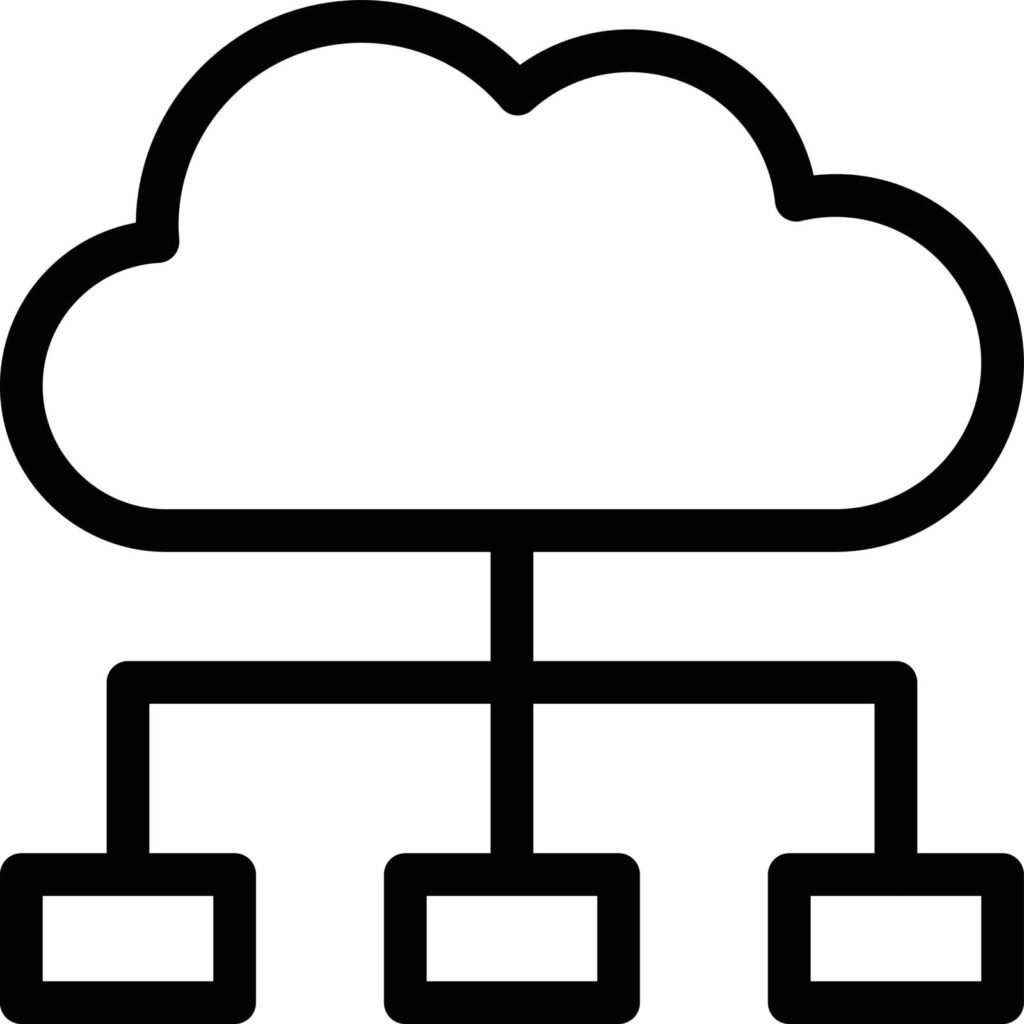
28.) Robotics
Robotics has recently become a household name, and it’s for a good reason.
First, robotics deals with controlling and planning physical systems, an inherently complex problem.
Second, robotics requires various sensors and actuators to interact with the world, making it an ideal application for machine learning techniques.
Finally, robotics is an interdisciplinary field that draws on various disciplines, such as computer science, mechanical engineering, and electrical engineering.
As a result, robotics is a rich source of research problems for data scientists.
29.) HealthCare
Healthcare is an industry that is ripe for data-driven innovation.
Hospitals, clinics, and health insurance companies generate a tremendous amount of data daily.
This data can be used to improve the quality of care and outcomes for patients.
This is perfect timing, as the healthcare industry is undergoing a significant shift towards value-based care, which means there is a greater need than ever for data-driven decision-making.
As a result, healthcare is an exciting new research topic for data scientists.
There are many different ways in which data can be used to improve healthcare, and there is a ton of room for newcomers to make discoveries.
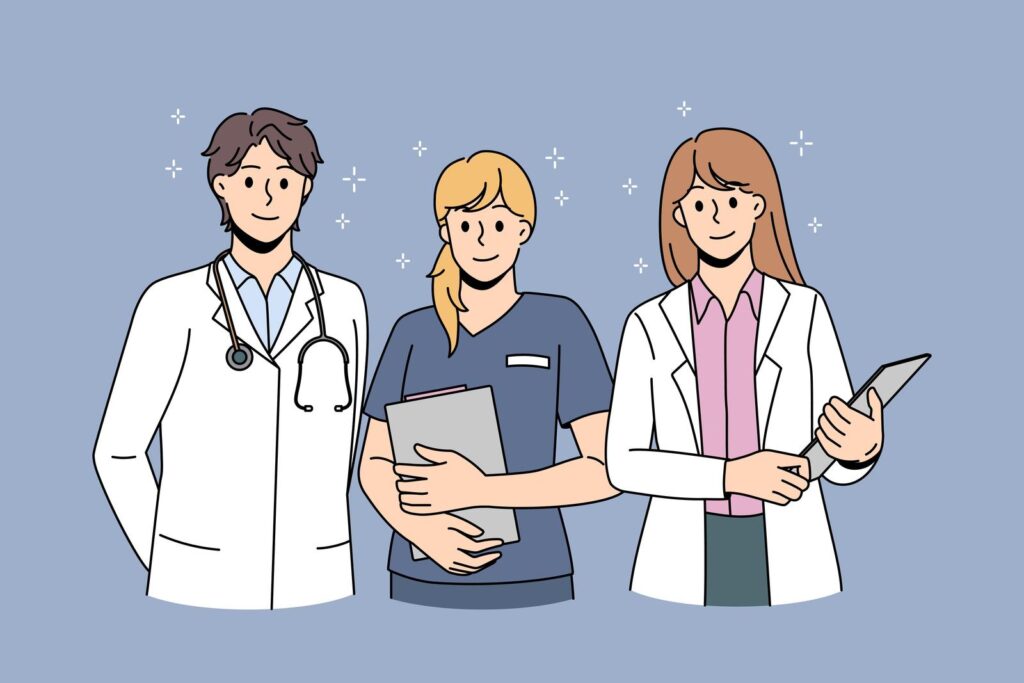
30.) Remote Work
There’s no doubt that remote work is on the rise.
In today’s global economy, more and more businesses are allowing their employees to work from home or anywhere else they can get a stable internet connection.
But what does this mean for data science? Well, for one thing, it opens up a whole new field of research.
For example, how does remote work impact employee productivity?
What are the best ways to manage and collaborate on data science projects when team members are spread across the globe?
And what are the cybersecurity risks associated with working remotely?
These are just a few of the questions that data scientists will be able to answer with further research.
So if you’re looking for a new topic to sink your teeth into, remote work in data science is a great option.
31.) Data-Driven Journalism
Data-driven journalism is an exciting new field of research that combines the best of both worlds: the rigor of data science with the creativity of journalism.
By applying data analytics to large datasets, journalists can uncover stories that would otherwise be hidden.
And telling these stories compellingly can help people better understand the world around them.
Data-driven journalism is still in its infancy, but it has already had a major impact on how news is reported.
In the future, it will only become more important as data becomes increasingly fluid among journalists.
It is an exciting new topic and research field for data scientists to explore.
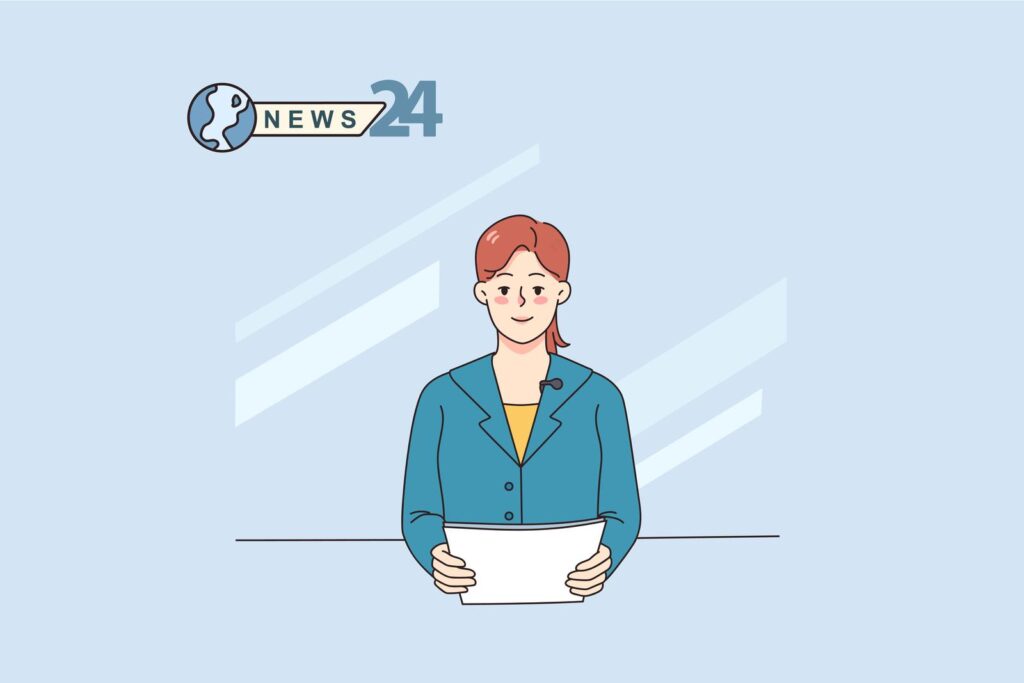
32.) Data Engineering
Data engineering is a staple in data science, focusing on efficiently managing data.
Data engineers are responsible for developing and maintaining the systems that collect, process, and store data.
In recent years, there has been an increasing demand for data engineers as the volume of data generated by businesses and organizations has grown exponentially.
Data engineers must be able to design and implement efficient data-processing pipelines and have the skills to optimize and troubleshoot existing systems.
If you are looking for a challenging research topic that would immediately impact you worldwide, then improving or innovating a new approach in data engineering would be a good start.
33.) Data Curation
Data curation has been a hot topic in the data science community for some time now.
Curating data involves organizing, managing, and preserving data so researchers can use it.
Data curation can help to ensure that data is accurate, reliable, and accessible.
It can also help to prevent research duplication and to facilitate the sharing of data between researchers.
Data curation is a vital part of data science. In recent years, there has been an increasing focus on data curation, as it has become clear that it is essential for ensuring data quality.
As a result, data curation is now a major research topic in data science.
There are numerous books and articles on the subject, and many universities offer courses on data curation.
Data curation is an integral part of data science and will only become more important in the future.
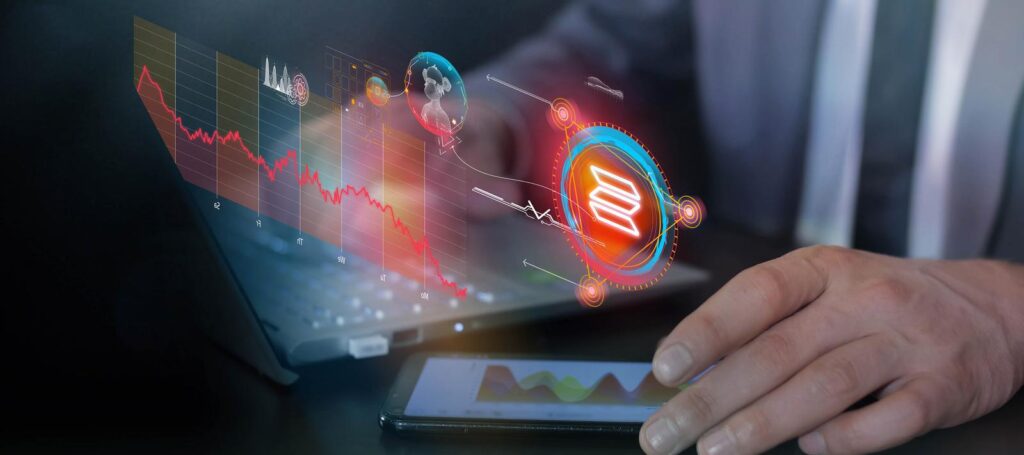
34.) Meta-Learning
Meta-learning is gaining a ton of steam in data science. It’s learning how to learn.
So, if you can learn how to learn, you can learn anything much faster.
Meta-learning is mainly used in deep learning, as applications outside of this are generally pretty hard.
In deep learning, many parameters need to be tuned for a good model, and there’s usually a lot of data.
You can save time and effort if you can automatically and quickly do this tuning.
In machine learning, meta-learning can improve models’ performance by sharing knowledge between different models.
For example, if you have a bunch of different models that all solve the same problem, then you can use meta-learning to share the knowledge between them to improve the cluster (groups) overall performance.
I don’t know how anyone looking for a research topic could stay away from this field; it’s what the Terminator warned us about!
35.) Data Warehousing
A data warehouse is a system used for data analysis and reporting.
It is a central data repository created by combining data from multiple sources.
Data warehouses are often used to store historical data, such as sales data, financial data, and customer data.
This data type can be used to create reports and perform statistical analysis.
Data warehouses also store data that the organization is not currently using.
This type of data can be used for future research projects.
Data warehousing is an incredible research topic in data science because it offers a variety of benefits.
Data warehouses help organizations to save time and money by reducing the need for manual data entry.
They also help to improve the accuracy of reports and provide a complete picture of the organization’s performance.
Data warehousing feels like one of the weakest parts of the Data Science Technology Stack; if you want a research topic that could have a monumental impact – data warehousing is an excellent place to look.
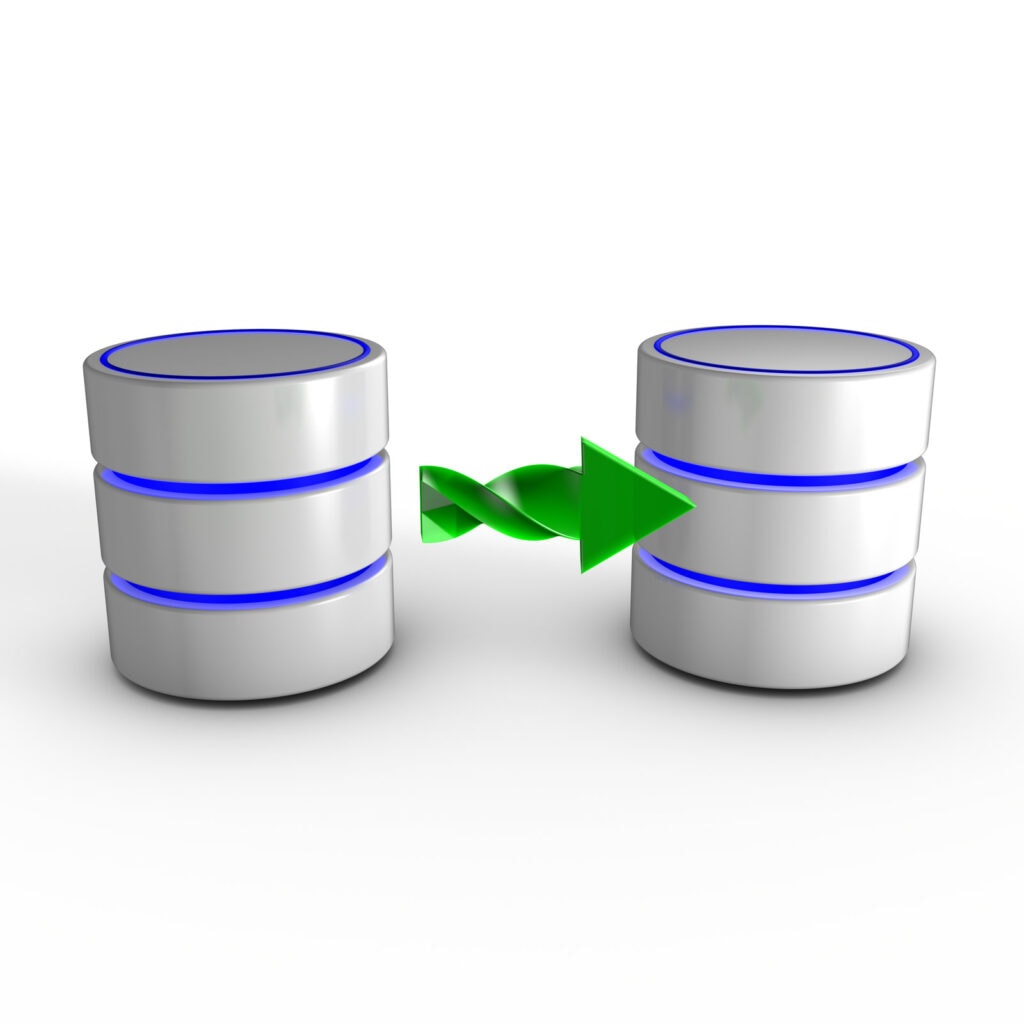
36.) Business Intelligence
Business intelligence aims to collect, process, and analyze data to help businesses make better decisions.
Business intelligence can improve marketing, sales, customer service, and operations.
It can also be used to identify new business opportunities and track competition.
BI is business and another tool in your company’s toolbox to continue dominating your area.
Data science is the perfect tool for business intelligence because it combines statistics, computer science, and machine learning.
Data scientists can use business intelligence to answer questions like, “What are our customers buying?” or “What are our competitors doing?” or “How can we increase sales?”
Business intelligence is a great way to improve your business’s bottom line and an excellent opportunity to dive deep into a well-respected research topic.
37.) Crowdsourcing
One of the newest areas of research in data science is crowdsourcing.
Crowdsourcing is a process of sourcing tasks or projects to a large group of people, typically via the internet.
This can be done for various purposes, such as gathering data, developing new algorithms, or even just for fun (think: online quizzes and surveys).
But what makes crowdsourcing so powerful is that it allows businesses and organizations to tap into a vast pool of talent and resources they wouldn’t otherwise have access to.
And with the rise of social media, it’s easier than ever to connect with potential crowdsource workers worldwide.
Imagine if you could effect that, finding innovative ways to improve how people work together.
That would have a huge effect.
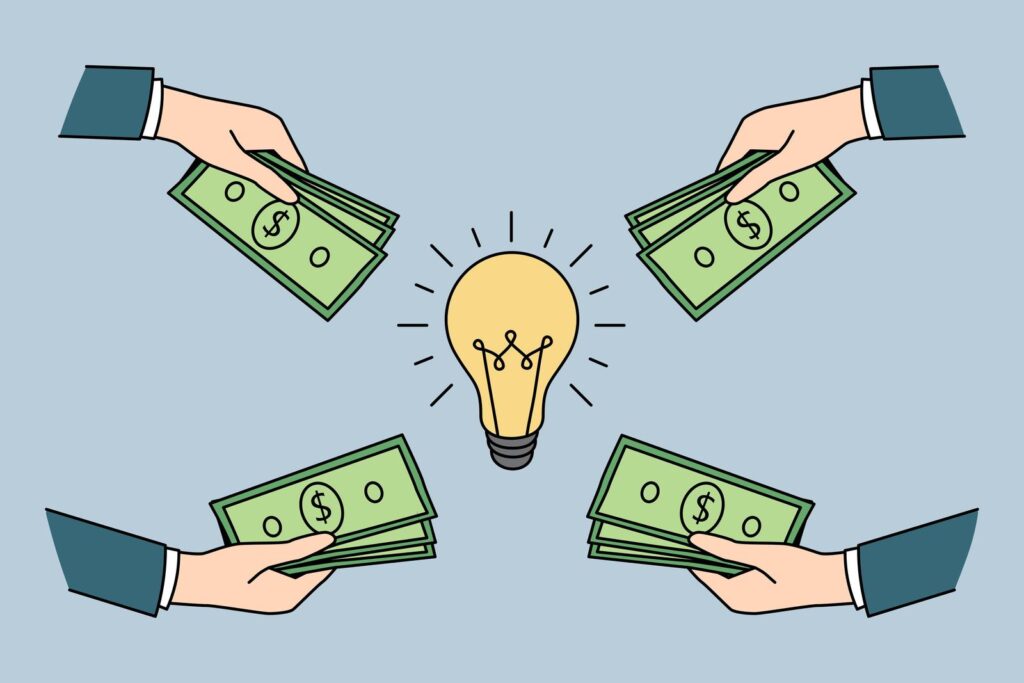
Final Thoughts, Are These Research Topics In Data Science For You?
Thirty-seven different research topics in data science are a lot to take in, but we hope you found a research topic that interests you.
If not, don’t worry – there are plenty of other great topics to explore.
The important thing is to get started with your research and find ways to apply what you learn to real-world problems.
We wish you the best of luck as you begin your data science journey!
Other Data Science Articles
We love talking about data science; here are a couple of our favorite articles:
- Why Are You Interested In Data Science?
- Recent Posts
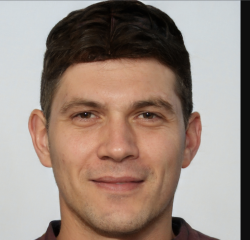
- Master Data Analysis with Excel [Unlock Insider Tips] - September 5, 2024
- What Does a Software Development Team Consist Of? [Secrets Revealed] - September 4, 2024
- How to Use Volvo VIDA Software Like a Pro [Expert Tips] - September 4, 2024

Research Areas
Main navigation.
The world is being transformed by data and data-driven analysis is rapidly becoming an integral part of science and society. Stanford Data Science is a collaborative effort across many departments in all seven schools. We strive to unite existing data science research initiatives and create interdisciplinary collaborations, connecting the data science and related methodologists with disciplines that are being transformed by data science and computation.
Our work supports research in a variety of fields where incredible advances are being made through the facilitation of meaningful collaborations between domain researchers, with deep expertise in societal and fundamental research challenges, and methods researchers that are developing next-generation computational tools and techniques, including:
Data Science for Wildland Fire Research
In recent years, wildfire has gone from an infrequent and distant news item to a centerstage isssue spanning many consecutive weeks for urban and suburban communities. Frequent wildfires are changing everyday lives for California in numerous ways -- from public safety power shutoffs to hazardous air quality -- that seemed inconceivable as recently as 2015. Moreover, elevated wildfire risk in the western United States (and similar climates globally) is here to stay into the foreseeable future. There is a plethora of problems that need solutions in the wildland fire arena; many of them are well suited to a data-driven approach.
Seminar Series
Data Science for Physics
Astrophysicists and particle physicists at Stanford and at the SLAC National Accelerator Laboratory are deeply engaged in studying the Universe at both the largest and smallest scales, with state-of-the-art instrumentation at telescopes and accelerator facilities
Data Science for Economics
Many of the most pressing questions in empirical economics concern causal questions, such as the impact, both short and long run, of educational choices on labor market outcomes, and of economic policies on distributions of outcomes. This makes them conceptually quite different from the predictive type of questions that many of the recently developed methods in machine learning are primarily designed for.
Data Science for Education
Educational data spans K-12 school and district records, digital archives of instructional materials and gradebooks, as well as student responses on course surveys. Data science of actual classroom interaction is also of increasing interest and reality.
Data Science for Human Health
It is clear that data science will be a driving force in transitioning the world’s healthcare systems from reactive “sick-based” care to proactive, preventive care.
Data Science for Humanity
Our modern era is characterized by massive amounts of data documenting the behaviors of individuals, groups, organizations, cultures, and indeed entire societies. This wealth of data on modern humanity is accompanied by massive digitization of historical data, both textual and numeric, in the form of historic newspapers, literary and linguistic corpora, economic data, censuses, and other government data, gathered and preserved over centuries, and newly digitized, acquired, and provisioned by libraries, scholars, and commercial entities.
Data Science for Linguistics
The impact of data science on linguistics has been profound. All areas of the field depend on having a rich picture of the true range of variation, within dialects, across dialects, and among different languages. The subfield of corpus linguistics is arguably as old as the field itself and, with the advent of computers, gave rise to many core techniques in data science.
Data Science for Nature and Sustainability
Many key sustainability issues translate into decision and optimization problems and could greatly benefit from data-driven decision making tools. In fact, the impact of modern information technology has been highly uneven, mainly benefiting large firms in profitable sectors, with little or no benefit in terms of the environment. Our vision is that data-driven methods can — and should — play a key role in increasing the efficiency and effectiveness of the way we manage and allocate our natural resources.
Ethics and Data Science
With the emergence of new techniques of machine learning, and the possibility of using algorithms to perform tasks previously done by human beings, as well as to generate new knowledge, we again face a set of new ethical questions.
The Science of Data Science
The practice of data analysis has changed enormously. Data science needs to find new inferential paradigms that allow data exploration prior to the formulation of hypotheses.
- How It Works
- PhD thesis writing
- Master thesis writing
- Bachelor thesis writing
- Dissertation writing service
- Dissertation abstract writing
- Thesis proposal writing
- Thesis editing service
- Thesis proofreading service
- Thesis formatting service
- Coursework writing service
- Research paper writing service
- Architecture thesis writing
- Computer science thesis writing
- Engineering thesis writing
- History thesis writing
- MBA thesis writing
- Nursing dissertation writing
- Psychology dissertation writing
- Sociology thesis writing
- Statistics dissertation writing
- Buy dissertation online
- Write my dissertation
- Cheap thesis
- Cheap dissertation
- Custom dissertation
- Dissertation help
- Pay for thesis
- Pay for dissertation
- Senior thesis
- Write my thesis
214 Best Big Data Research Topics for Your Thesis Paper
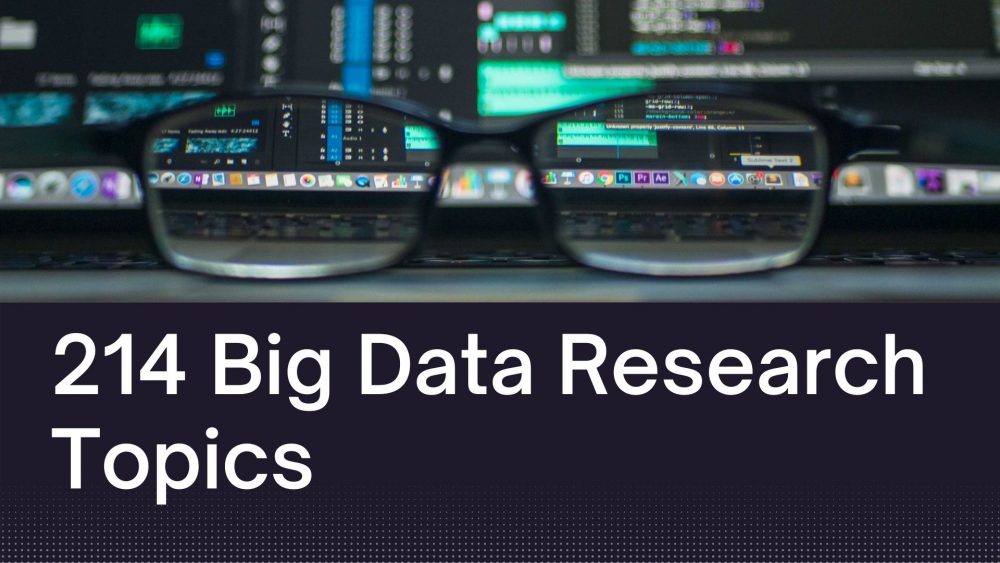
Finding an ideal big data research topic can take you a long time. Big data, IoT, and robotics have evolved. The future generations will be immersed in major technologies that will make work easier. Work that was done by 10 people will now be done by one person or a machine. This is amazing because, in as much as there will be job loss, more jobs will be created. It is a win-win for everyone.
Big data is a major topic that is being embraced globally. Data science and analytics are helping institutions, governments, and the private sector. We will share with you the best big data research topics.
On top of that, we can offer you the best writing tips to ensure you prosper well in your academics. As students in the university, you need to do proper research to get top grades. Hence, you can consult us if in need of research paper writing services.
Big Data Analytics Research Topics for your Research Project
Are you looking for an ideal big data analytics research topic? Once you choose a topic, consult your professor to evaluate whether it is a great topic. This will help you to get good grades.
- Which are the best tools and software for big data processing?
- Evaluate the security issues that face big data.
- An analysis of large-scale data for social networks globally.
- The influence of big data storage systems.
- The best platforms for big data computing.
- The relation between business intelligence and big data analytics.
- The importance of semantics and visualization of big data.
- Analysis of big data technologies for businesses.
- The common methods used for machine learning in big data.
- The difference between self-turning and symmetrical spectral clustering.
- The importance of information-based clustering.
- Evaluate the hierarchical clustering and density-based clustering application.
- How is data mining used to analyze transaction data?
- The major importance of dependency modeling.
- The influence of probabilistic classification in data mining.
Interesting Big Data Analytics Topics
Who said big data had to be boring? Here are some interesting big data analytics topics that you can try. They are based on how some phenomena are done to make the world a better place.
- Discuss the privacy issues in big data.
- Evaluate the storage systems of scalable in big data.
- The best big data processing software and tools.
- Data mining tools and techniques are popularly used.
- Evaluate the scalable architectures for parallel data processing.
- The major natural language processing methods.
- Which are the best big data tools and deployment platforms?
- The best algorithms for data visualization.
- Analyze the anomaly detection in cloud servers
- The scrutiny normally done for the recruitment of big data job profiles.
- The malicious user detection in big data collection.
- Learning long-term dependencies via the Fourier recurrent units.
- Nomadic computing for big data analytics.
- The elementary estimators for graphical models.
- The memory-efficient kernel approximation.
Big Data Latest Research Topics
Do you know the latest research topics at the moment? These 15 topics will help you to dive into interesting research. You may even build on research done by other scholars.
- Evaluate the data mining process.
- The influence of the various dimension reduction methods and techniques.
- The best data classification methods.
- The simple linear regression modeling methods.
- Evaluate the logistic regression modeling.
- What are the commonly used theorems?
- The influence of cluster analysis methods in big data.
- The importance of smoothing methods analysis in big data.
- How is fraud detection done through AI?
- Analyze the use of GIS and spatial data.
- How important is artificial intelligence in the modern world?
- What is agile data science?
- Analyze the behavioral analytics process.
- Semantic analytics distribution.
- How is domain knowledge important in data analysis?
Big Data Debate Topics
If you want to prosper in the field of big data, you need to try even hard topics. These big data debate topics are interesting and will help you to get a better understanding.
- The difference between big data analytics and traditional data analytics methods.
- Why do you think the organization should think beyond the Hadoop hype?
- Does the size of the data matter more than how recent the data is?
- Is it true that bigger data are not always better?
- The debate of privacy and personalization in maintaining ethics in big data.
- The relation between data science and privacy.
- Do you think data science is a rebranding of statistics?
- Who delivers better results between data scientists and domain experts?
- According to your view, is data science dead?
- Do you think analytics teams need to be centralized or decentralized?
- The best methods to resource an analytics team.
- The best business case for investing in analytics.
- The societal implications of the use of predictive analytics within Education.
- Is there a need for greater control to prevent experimentation on social media users without their consent?
- How is the government using big data; for the improvement of public statistics or to control the population?
University Dissertation Topics on Big Data
Are you doing your Masters or Ph.D. and wondering the best dissertation topic or thesis to do? Why not try any of these? They are interesting and based on various phenomena. While doing the research ensure you relate the phenomenon with the current modern society.
- The machine learning algorithms are used for fall recognition.
- The divergence and convergence of the internet of things.
- The reliable data movements using bandwidth provision strategies.
- How is big data analytics using artificial neural networks in cloud gaming?
- How is Twitter accounts classification done using network-based features?
- How is online anomaly detection done in the cloud collaborative environment?
- Evaluate the public transportation insights provided by big data.
- Evaluate the paradigm for cancer patients using the nursing EHR to predict the outcome.
- Discuss the current data lossless compression in the smart grid.
- How does online advertising traffic prediction helps in boosting businesses?
- How is the hyperspectral classification done using the multiple kernel learning paradigm?
- The analysis of large data sets downloaded from websites.
- How does social media data help advertising companies globally?
- Which are the systems recognizing and enforcing ownership of data records?
- The alternate possibilities emerging for edge computing.
The Best Big Data Analysis Research Topics and Essays
There are a lot of issues that are associated with big data. Here are some of the research topics that you can use in your essays. These topics are ideal whether in high school or college.
- The various errors and uncertainty in making data decisions.
- The application of big data on tourism.
- The automation innovation with big data or related technology
- The business models of big data ecosystems.
- Privacy awareness in the era of big data and machine learning.
- The data privacy for big automotive data.
- How is traffic managed in defined data center networks?
- Big data analytics for fault detection.
- The need for machine learning with big data.
- The innovative big data processing used in health care institutions.
- The money normalization and extraction from texts.
- How is text categorization done in AI?
- The opportunistic development of data-driven interactive applications.
- The use of data science and big data towards personalized medicine.
- The programming and optimization of big data applications.
The Latest Big Data Research Topics for your Research Proposal
Doing a research proposal can be hard at first unless you choose an ideal topic. If you are just diving into the big data field, you can use any of these topics to get a deeper understanding.
- The data-centric network of things.
- Big data management using artificial intelligence supply chain.
- The big data analytics for maintenance.
- The high confidence network predictions for big biological data.
- The performance optimization techniques and tools for data-intensive computation platforms.
- The predictive modeling in the legal context.
- Analysis of large data sets in life sciences.
- How to understand the mobility and transport modal disparities sing emerging data sources?
- How do you think data analytics can support asset management decisions?
- An analysis of travel patterns for cellular network data.
- The data-driven strategic planning for citywide building retrofitting.
- How is money normalization done in data analytics?
- Major techniques used in data mining.
- The big data adaptation and analytics of cloud computing.
- The predictive data maintenance for fault diagnosis.
Interesting Research Topics on A/B Testing In Big Data
A/B testing topics are different from the normal big data topics. However, you use an almost similar methodology to find the reasons behind the issues. These topics are interesting and will help you to get a deeper understanding.
- How is ultra-targeted marketing done?
- The transition of A/B testing from digital to offline.
- How can big data and A/B testing be done to win an election?
- Evaluate the use of A/B testing on big data
- Evaluate A/B testing as a randomized control experiment.
- How does A/B testing work?
- The mistakes to avoid while conducting the A/B testing.
- The most ideal time to use A/B testing.
- The best way to interpret results for an A/B test.
- The major principles of A/B tests.
- Evaluate the cluster randomization in big data
- The best way to analyze A/B test results and the statistical significance.
- How is A/B testing used in boosting businesses?
- The importance of data analysis in conversion research
- The importance of A/B testing in data science.
Amazing Research Topics on Big Data and Local Governments
Governments are now using big data to make the lives of the citizens better. This is in the government and the various institutions. They are based on real-life experiences and making the world better.
- Assess the benefits and barriers of big data in the public sector.
- The best approach to smart city data ecosystems.
- The big analytics used for policymaking.
- Evaluate the smart technology and emergence algorithm bureaucracy.
- Evaluate the use of citizen scoring in public services.
- An analysis of the government administrative data globally.
- The public values are found in the era of big data.
- Public engagement on local government data use.
- Data analytics use in policymaking.
- How are algorithms used in public sector decision-making?
- The democratic governance in the big data era.
- The best business model innovation to be used in sustainable organizations.
- How does the government use the collected data from various sources?
- The role of big data for smart cities.
- How does big data play a role in policymaking?
Easy Research Topics on Big Data
Who said big data topics had to be hard? Here are some of the easiest research topics. They are based on data management, research, and data retention. Pick one and try it!
- Who uses big data analytics?
- Evaluate structure machine learning.
- Explain the whole deep learning process.
- Which are the best ways to manage platforms for enterprise analytics?
- Which are the new technologies used in data management?
- What is the importance of data retention?
- The best way to work with images is when doing research.
- The best way to promote research outreach is through data management.
- The best way to source and manage external data.
- Does machine learning improve the quality of data?
- Describe the security technologies that can be used in data protection.
- Evaluate token-based authentication and its importance.
- How can poor data security lead to the loss of information?
- How to determine secure data.
- What is the importance of centralized key management?
Unique IoT and Big Data Research Topics
Internet of Things has evolved and many devices are now using it. There are smart devices, smart cities, smart locks, and much more. Things can now be controlled by the touch of a button.
- Evaluate the 5G networks and IoT.
- Analyze the use of Artificial intelligence in the modern world.
- How do ultra-power IoT technologies work?
- Evaluate the adaptive systems and models at runtime.
- How have smart cities and smart environments improved the living space?
- The importance of the IoT-based supply chains.
- How does smart agriculture influence water management?
- The internet applications naming and identifiers.
- How does the smart grid influence energy management?
- Which are the best design principles for IoT application development?
- The best human-device interactions for the Internet of Things.
- The relation between urban dynamics and crowdsourcing services.
- The best wireless sensor network for IoT security.
- The best intrusion detection in IoT.
- The importance of big data on the Internet of Things.
Big Data Database Research Topics You Should Try
Big data is broad and interesting. These big data database research topics will put you in a better place in your research. You also get to evaluate the roles of various phenomena.
- The best cloud computing platforms for big data analytics.
- The parallel programming techniques for big data processing.
- The importance of big data models and algorithms in research.
- Evaluate the role of big data analytics for smart healthcare.
- How is big data analytics used in business intelligence?
- The best machine learning methods for big data.
- Evaluate the Hadoop programming in big data analytics.
- What is privacy-preserving to big data analytics?
- The best tools for massive big data processing
- IoT deployment in Governments and Internet service providers.
- How will IoT be used for future internet architectures?
- How does big data close the gap between research and implementation?
- What are the cross-layer attacks in IoT?
- The influence of big data and smart city planning in society.
- Why do you think user access control is important?
Big Data Scala Research Topics
Scala is a programming language that is used in data management. It is closely related to other data programming languages. Here are some of the best scala questions that you can research.
- Which are the most used languages in big data?
- How is scala used in big data research?
- Is scala better than Java in big data?
- How is scala a concise programming language?
- How does the scala language stream process in real-time?
- Which are the various libraries for data science and data analysis?
- How does scala allow imperative programming in data collection?
- Evaluate how scala includes a useful REPL for interaction.
- Evaluate scala’s IDE support.
- The data catalog reference model.
- Evaluate the basics of data management and its influence on research.
- Discuss the behavioral analytics process.
- What can you term as the experience economy?
- The difference between agile data science and scala language.
- Explain the graph analytics process.
Independent Research Topics for Big Data
These independent research topics for big data are based on the various technologies and how they are related. Big data will greatly be important for modern society.
- The biggest investment is in big data analysis.
- How are multi-cloud and hybrid settings deep roots?
- Why do you think machine learning will be in focus for a long while?
- Discuss in-memory computing.
- What is the difference between edge computing and in-memory computing?
- The relation between the Internet of things and big data.
- How will digital transformation make the world a better place?
- How does data analysis help in social network optimization?
- How will complex big data be essential for future enterprises?
- Compare the various big data frameworks.
- The best way to gather and monitor traffic information using the CCTV images
- Evaluate the hierarchical structure of groups and clusters in the decision tree.
- Which are the 3D mapping techniques for live streaming data.
- How does machine learning help to improve data analysis?
- Evaluate DataStream management in task allocation.
- How is big data provisioned through edge computing?
- The model-based clustering of texts.
- The best ways to manage big data.
- The use of machine learning in big data.
Is Your Big Data Thesis Giving You Problems?
These are some of the best topics that you can use to prosper in your studies. Not only are they easy to research but also reflect on real-time issues. Whether in University or college, you need to put enough effort into your studies to prosper. However, if you have time constraints, we can provide professional writing help. Are you looking for online expert writers? Look no further, we will provide quality work at a cheap price.
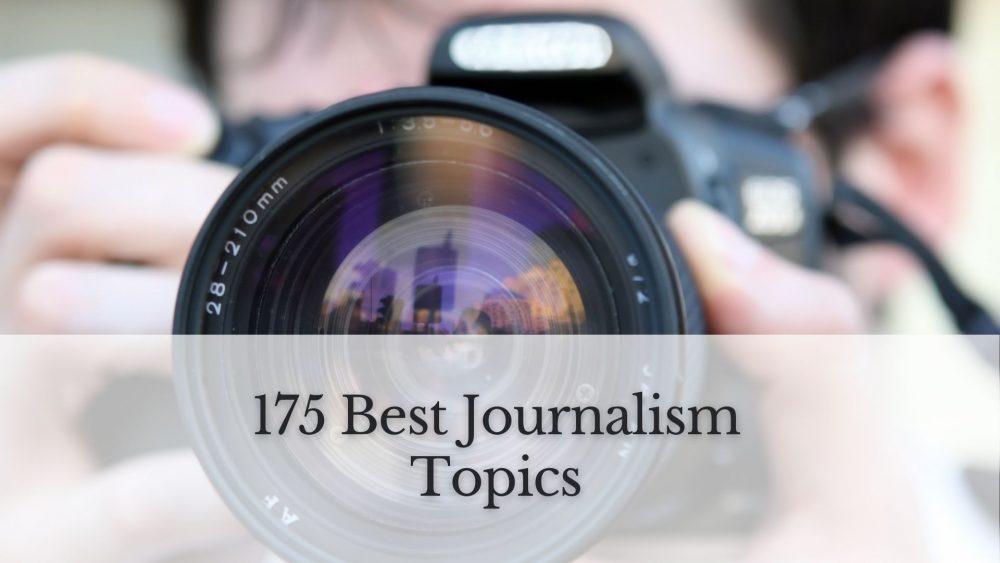
Leave a Reply Cancel reply
Your email address will not be published. Required fields are marked *
Comment * Error message
Name * Error message
Email * Error message
Save my name, email, and website in this browser for the next time I comment.
As Putin continues killing civilians, bombing kindergartens, and threatening WWIII, Ukraine fights for the world's peaceful future.
Ukraine Live Updates
Ten Research Challenge Areas in Data Science
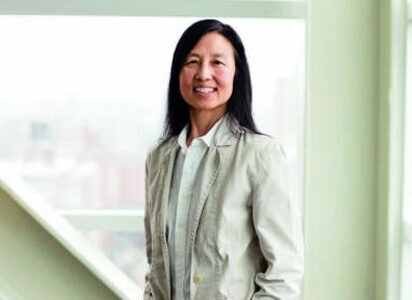
Although data science builds on knowledge from computer science, mathematics, statistics, and other disciplines, data science is a unique field with many mysteries to unlock: challenging scientific questions and pressing questions of societal importance.
Is data science a discipline?
Data science is a field of study: one can get a degree in data science, get a job as a data scientist, and get funded to do data science research. But is data science a discipline, or will it evolve to be one, distinct from other disciplines? Here are a few meta-questions about data science as a discipline.
- What is/are the driving deep question(s) of data science? Each scientific discipline (usually) has one or more “deep” questions that drive its research agenda: What is the origin of the universe (astrophysics)? What is the origin of life (biology)? What is computable (computer science)? Does data science inherit its deep questions from all its constituency disciplines or does it have its own unique ones?
- What is the role of the domain in the field of data science? People (including this author) (Wing, J.M., Janeia, V.P., Kloefkorn, T., & Erickson, L.C. (2018)) have argued that data science is unique in that it is not just about methods, but about the use of those methods in the context of a domain—the domain of the data being collected and analyzed; the domain for which a question to be answered comes from collecting and analyzing the data. Is the inclusion of a domain inherent in defining the field of data science? If so, is the way it is included unique to data science?
- What makes data science data science? Is there a problem unique to data science that one can convincingly argue would not be addressed or asked by any of its constituent disciplines, e.g., computer science and statistics?
Ten research areas
While answering the above meta-questions is still under lively debate, including within the pages of this journal, we can ask an easier question, one that also underlies any field of study: What are the research challenge areas that drive the study of data science? Here is a list of ten. They are not in any priority order, and some of them are related to each other. They are phrased as challenge areas, not challenge questions. They are not necessarily the “top ten” but they are a good ten to start the community discussing what a broad research agenda for data science might look like. 1
- Scientific understanding of learning, especially deep learning algorithms. As much as we admire the astonishing successes of deep learning, we still lack a scientific understanding of why deep learning works so well. We do not understand the mathematical properties of deep learning models. We do not know how to explain why a deep learning model produces one result and not another. We do not understand how robust or fragile they are to perturbations to input data distributions. We do not understand how to verify that deep learning will perform the intended task well on new input data. Deep learning is an example of where experimentation in a field is far ahead of any kind of theoretical understanding.
- Causal reasoning. Machine learning is a powerful tool to find patterns and examine correlations, particularly in large data sets. While the adoption of machine learning has opened many fruitful areas of research in economics, social science, and medicine, these fields require methods that move beyond correlational analyses and can tackle causal questions. A rich and growing area of current study is revisiting causal inference in the presence of large amounts of data. Economists are already revisiting causal reasoning by devising new methods at the intersection of economics and machine learning that make causal inference estimation more efficient and flexible (Athey, 2016), (Taddy, 2019). Data scientists are just beginning to explore multiple causal inference, not just to overcome some of the strong assumptions of univariate causal inference, but because most real-world observations are due to multiple factors that interact with each other (Wang & Blei, 2018).
- Precious data. Data can be precious for one of three reasons: the dataset is expensive to collect; the dataset contains a rare event (low signal-to-noise ratio ); or the dataset is artisanal—small and task-specific. A good example of expensive data comes from large, one-of, expensive scientific instruments, e.g., the Large Synoptic Survey Telescope, the Large Hadron Collider, the IceCube Neutrino Detector at the South Pole. A good example of rare event data is data from sensors on physical infrastructure, such as bridges and tunnels; sensors produce a lot of raw data, but the disastrous event they are used to predict is (thankfully) rare. Rare data can also be expensive to collect. A good example of artisanal data is the tens of millions of court judgments that China has released online to the public since 2014 (Liebman, Roberts, Stern, & Wang, 2017) or the 2+ million US government declassified documents collected by Columbia’s History Lab (Connelly, Madigan, Jervis, Spirling, & Hicks, 2019). For each of these different kinds of precious data, we need new data science methods and algorithms, taking into consideration the domain and intended uses of the data.
- Multiple, heterogeneous data sources. For some problems, we can collect lots of data from different data sources to improve our models. For example, to predict the effectiveness of a specific cancer treatment for a human, we might build a model based on 2-D cell lines from mice, more expensive 3-D cell lines from mice, and the costly DNA sequence of the cancer cells extracted from the human. State-of-the-art data science methods cannot as yet handle combining multiple, heterogeneous sources of data to build a single, accurate model. Since many of these data sources might be precious data, this challenge is related to the third challenge. Focused research in combining multiple sources of data will provide extraordinary impact.
- Inferring from noisy and/or incomplete data. The real world is messy and we often do not have complete information about every data point. Yet, data scientists want to build models from such data to do prediction and inference. A great example of a novel formulation of this problem is the planned use of differential privacy for Census 2020 data (Garfinkel, 2019), where noise is deliberately added to a query result, to maintain the privacy of individuals participating in the census. Handling “deliberate” noise is particularly important for researchers working with small geographic areas such as census blocks, since the added noise can make the data uninformative at those levels of aggregation. How then can social scientists, who for decades have been drawing inferences from census data, make inferences on this “noisy” data and how do they combine their past inferences with these new ones? Machine learning’s ability to better separate noise from signal can improve the efficiency and accuracy of those inferences.
- Trustworthy AI. We have seen rapid deployment of systems using artificial intelligence (AI) and machine learning in critical domains such as autonomous vehicles, criminal justice, healthcare, hiring, housing, human resource management, law enforcement, and public safety, where decisions taken by AI agents directly impact human lives. Consequently, there is an increasing concern if these decisions can be trusted to be correct, reliable, robust, safe, secure, and fair, especially under adversarial attacks. One approach to building trust is through providing explanations of the outcomes of a machine learned model. If we can interpret the outcome in a meaningful way, then the end user can better trust the model. Another approach is through formal methods, where one strives to prove once and for all a model satisfies a certain property. New trust properties yield new tradeoffs for machine learned models, e.g., privacy versus accuracy; robustness versus efficiency. There are actually multiple audiences for trustworthy models: the model developer, the model user, and the model customer. Ultimately, for widespread adoption of the technology, it is the public who must trust these automated decision systems.
- Computing systems for data-intensive applications. Traditional designs of computing systems have focused on computational speed and power: the more cycles, the faster the application can run. Today, the primary focus of applications, especially in the sciences (e.g., astronomy, biology, climate science, materials science), is data. Also, novel special-purpose processors, e.g., GPUs, FPGAs, TPUs, are now commonly found in large data centers. Even with all these data and all this fast and flexible computational power, it can still take weeks to build accurate predictive models; however, applications, whether from science or industry, want real-time predictions. Also, data-hungry and compute-hungry algorithms, e.g., deep learning, are energy hogs (Strubell, Ganesh, & McCallum, 2019). We should consider not only space and time, but also energy consumption, in our performance metrics. In short, we need to rethink computer systems design from first principles, with data (not compute) the focus. New computing systems designs need to consider: heterogeneous processing; efficient layout of massive amounts of data for fast access; the target domain, application, or even task; and energy efficiency.
- Automating front-end stages of the data life cycle. While the excitement in data science is due largely to the successes of machine learning, and more specifically deep learning, before we get to use machine learning methods, we need to prepare the data for analysis. The early stages in the data life cycle (Wing, 2019) are still labor intensive and tedious. Data scientists, drawing on both computational and statistical methods, need to devise automated methods that address data cleaning and data wrangling, without losing other desired properties, e.g., accuracy, precision, and robustness, of the end model.One example of emerging work in this area is the Data Analysis Baseline Library (Mueller, 2019), which provides a framework to simplify and automate data cleaning, visualization, model building, and model interpretation. The Snorkel project addresses the tedious task of data labeling (Ratner et al., 2018).
- Privacy. Today, the more data we have, the better the model we can build. One way to get more data is to share data, e.g., multiple parties pool their individual datasets to build collectively a better model than any one party can build. However, in many cases, due to regulation or privacy concerns, we need to preserve the confidentiality of each party’s dataset. An example of this scenario is in building a model to predict whether someone has a disease or not. If multiple hospitals could share their patient records, we could build a better predictive model; but due to Health Insurance Portability and Accountability Act (HIPAA) privacy regulations, hospitals cannot share these records. We are only now exploring practical and scalable ways, using cryptographic and statistical methods, for multiple parties to share data and/or share models to preserve the privacy of each party’s dataset. Industry and government are exploring and exploiting methods and concepts, such as secure multi-party computation, homomorphic encryption, zero-knowledge proofs, and differential privacy, as part of a point solution to a point problem.
- Ethics. Data science raises new ethical issues. They can be framed along three axes: (1) the ethics of data: how data are generated, recorded, and shared; (2) the ethics of algorithms: how artificial intelligence, machine learning, and robots interpret data; and (3) the ethics of practices: devising responsible innovation and professional codes to guide this emerging science (Floridi & Taddeo, 2016) and for defining Institutional Review Board (IRB) criteria and processes specific for data (Wing, Janeia, Kloefkorn, & Erickson 2018). Example ethical questions include how to detect and eliminate racial, gender, socio-economic, or other biases in machine learning models.
Closing remarks
As many universities and colleges are creating new data science schools, institutes, centers, etc. (Wing, Janeia, Kloefkorn, & Erickson 2018), it is worth reflecting on data science as a field. Will data science as an area of research and education evolve into being its own discipline or be a field that cuts across all other disciplines? One could argue that computer science, mathematics, and statistics share this commonality: they are each their own discipline, but they each can be applied to (almost) every other discipline. What will data science be in 10 or 50 years?
Acknowledgements
I would like to thank Cliff Stein, Gerad Torats-Espinosa, Max Topaz, and Richard Witten for their feedback on earlier renditions of this article. Many thanks to all Columbia Data Science faculty who have helped me formulate and discuss these ten (and other) challenges during our Fall 2019 retreat.
Athey, S. (2016). “Susan Athey on how economists can use machine learning to improve policy,” Retrieved from https://siepr.stanford.edu/news/susan-athey-how-economists-can-use-machine-learning-improve-policy
Berger, J., He, X., Madigan, C., Murphy, S., Yu, B., & Wellner, J. (2019), Statistics at a Crossroad: Who is for the Challenge? NSF workshop report. Retrieved from https://hub.ki/groups/statscrossroad
Connelly, M., Madigan, D., Jervis, R., Spirling, A., & Hicks, R. (2019). The History Lab. Retrieved from http://history-lab.org/
Floridi , L. & Taddeo , M. (2016). What is Data Ethics? Philosophical Transactions of the Royal Society A , vol. 374, issue 2083, December 2016.
Garfinkel, S. (2019). Deploying Differential Privacy for the 2020 Census of Population and Housing. Privacy Enhancing Technologies Symposium, Stockholm, Sweden. Retrieved from http://simson.net/ref/2019/2019-07-16%20Deploying%20Differential%20Privacy%20for%20the%202020%20Census.pdf
Liebman, B.L., Roberts, M., Stern, R.E., & Wang, A. (2017). Mass Digitization of Chinese Court Decisions: How to Use Text as Data in the Field of Chinese Law. UC San Diego School of Global Policy and Strategy, 21 st Century China Center Research Paper No. 2017-01; Columbia Public Law Research Paper No. 14-551. Retrieved from https://scholarship.law.columbia.edu/faculty_scholarship/2039
Mueller, A. (2019). Data Analysis Baseline Library. Retrieved from https://libraries.io/github/amueller/dabl
Ratner, A., Bach, S., Ehrenberg, H., Fries, J., Wu, S, & Ré, C. (2018). Snorkel: Rapid Training Data Creation with Weak Supervision . Proceedings of the 44 th International Conference on Very Large Data Bases.
Strubell E., Ganesh, A., & McCallum, A. (2019),”Energy and Policy Considerations for Deep Learning in NLP. Proceedings of the 57th Annual Meeting of the Association for Computational Linguistics (ACL).
Taddy, M. (2019). Business Data Science: Combining Machine Learning and Economics to Optimize, Automate, and Accelerate Business Decisions , Mc-Graw Hill.
Wang, Y. & Blei, D.M. (2018). The Blessings of Multiple Causes, Retrieved from https://arxiv.org/abs/1805.06826
Wing, J.M. (2019), The Data Life Cycle, Harvard Data Science Review , vol. 1, no. 1.
Wing, J.M., Janeia, V.P., Kloefkorn, T., & Erickson, L.C. (2018). Data Science Leadership Summit, Workshop Report, National Science Foundation. Retrieved from https://dl.acm.org/citation.cfm?id=3293458
J.M. Wing, “ Ten Research Challenge Areas in Data Science ,” Voices, Data Science Institute, Columbia University, January 2, 2020. arXiv:2002.05658 .
Jeannette M. Wing is Avanessians Director of the Data Science Institute and professor of computer science at Columbia University.
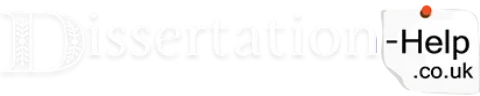
Click here to place an order for topic brief service to get instant approval from your professor.
99 Best Data Science Dissertation Topics
Table of Contents
What is a Data Science Dissertation?
A Data Science Dissertation is a research project where students explore the vast field of data science. This involves analyzing large sets of data, creating models, and finding patterns to solve problems or make decisions. In a data science dissertation, you might work on topics like machine learning, big data analytics, or predictive modeling. The goal is to contribute new insights or methods to the field of data science.
Why are Data Science Dissertation Topics Important?
Data science is one of the most in-demand fields today. Companies rely on data to make informed decisions, predict trends, and understand their customers better. By choosing a data science topic, you can explore real-world problems and provide solutions that can be applied in various industries like healthcare, finance, or technology. Your dissertation could help advance the field, making your research valuable and relevant.
Writing Tips for Data Science Dissertation
- Select a Relevant Topic: Pick a topic that is current and has a practical application. This will make your research more meaningful and impactful.
- Use Quality Data: Ensure you have access to high-quality and reliable data. Good data is crucial for accurate analysis and valid conclusions.
- Explain Your Methods Clearly: Data science can be complex, so clearly explain your methods and why you chose them. This helps others understand and replicate your work.
- Visualize Your Results: Use charts, graphs, and other visual tools to present your findings. This makes your dissertation easier to understand and more engaging.
List of Data Science Dissertation Topics
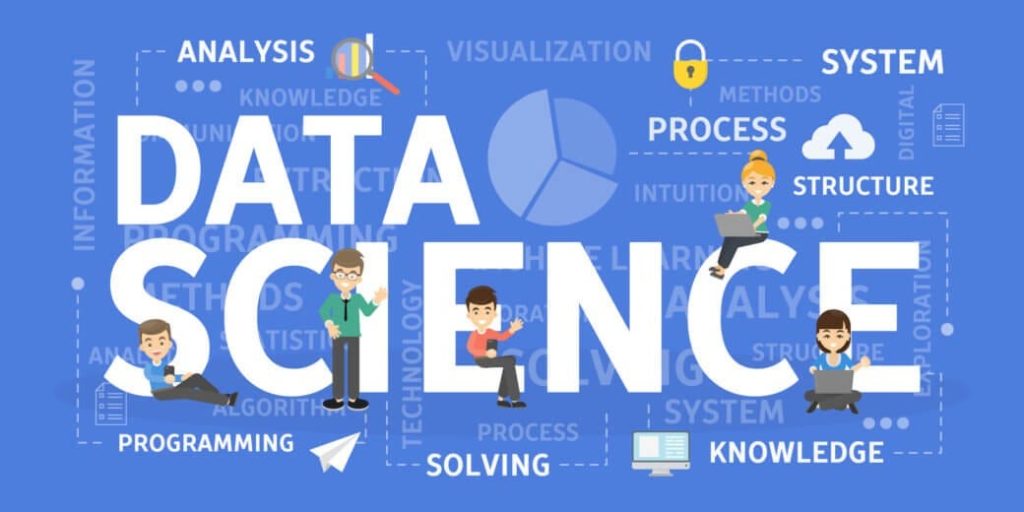
Machine Learning and Artificial Intelligence
- Enhancing Fraud Detection Systems using Deep Learning Algorithms
- Personalized Recommendation Systems: A Comparative Analysis of Machine Learning Approaches
- Predictive Modeling for Disease Diagnosis and Treatment
Big Data Analytics
- Optimizing Supply Chain Management through Big Data Analytics
- Sentiment Analysis on Social Media Data: Understanding Customer Perception
- Big Data-driven Strategies for Urban Planning and Development
Natural Language Processing (NLP)
- Automated Text Summarization Techniques: A Comparative Study
- Language Translation Models: Challenges and Opportunities
- Sentiment Analysis in Political Discourse: Uncovering Public Opinion
Data Mining and Knowledge Discovery
- Association Rule Mining for Market Basket Analysis
- Clustering Techniques for Customer Segmentation in E-commerce
- Predictive Analytics in Stock Market Forecasting
Health Informatics
- Predictive Modeling for Early Disease Detection
- Wearable Devices and Remote Patient Monitoring: A Data-driven Approach
- Data Privacy and Security in Healthcare Data Sharing Platforms
Business Intelligence and Analytics
- Data-driven Decision Making in Marketing Campaigns
- Customer Lifetime Value Prediction: A Machine Learning Approach
- Performance Analytics for Business Process Optimization
IoT and Sensor Data Analytics
- Smart Cities: Leveraging IoT Data for Urban Sustainability
- Predictive Maintenance in Industrial IoT: Anomaly Detection Techniques
- Environmental Monitoring using Sensor Networks: Challenges and Opportunities
Image and Video Analysis
- Object Detection and Recognition in Surveillance Videos
- Medical Image Analysis: Applications in Diagnosis and Treatment
- Deep Learning Approaches for Facial Recognition Systems
Social Network Analysis
- Influence Detection in Social Networks: A Graph-based Approach.
- Community Detection and Analysis in Online Social Platforms
- Fake News Detection using Social Network Analysis Techniques
Time Series Analysis
- Forecasting Demand in Retail: Time Series Models for Sales Prediction
- Financial Market Volatility Prediction using Time Series Analysis
- Energy Consumption Forecasting: A Comparative Study of Forecasting Models
Spatial Data Analysis
- Geographic Information Systems (GIS) for Urban Planning
- Spatial-Temporal Analysis of Crime Patterns: A Case Study
- Environmental Impact Assessment using Spatial Data Analysis Techniques
Bioinformatics
- Genomic Data Analysis: Towards Precision Medicine
- Protein Structure Prediction using Machine Learning Algorithms
- Computational Drug Discovery: Opportunities and Challenges
Data Privacy and Ethics
- Privacy-preserving Data Mining Techniques: Balancing Utility and Privacy
- Ethical Considerations in AI-driven Decision-Making Systems
- GDPR Compliance in Data-driven Businesses: Challenges and Solutions
Deep Learning Applications
- Deep Reinforcement Learning for Autonomous Vehicles
- Generative Adversarial Networks (GANs) for Synthetic Data Generation
- Deep Learning Models for Natural Language Understanding
Blockchain and Data Science
- Blockchain-enabled Data Sharing Platforms: Opportunities and Challenges
- Decentralized Data Marketplaces: A Paradigm Shift in Data Economy
- Security and Privacy in Blockchain-based Data Analytics
- Computer Science Research Topics (Approved Titles)
- Which topics are best for thesis in Computer Science?
- Information Systems Dissertation Topics Ideas
Writing a data science dissertation is an exciting opportunity to dive deep into a topic that interests you. Whether you’re exploring machine learning algorithms , data mining techniques, or the ethical implications of data usage, your research can make a significant impact. Choose a topic that aligns with your interests and has real-world relevance and remember to explain your methods and results clearly.
1. What are some common data science dissertation topics?
Common topics include machine learning applications, big data analytics, data visualization techniques, and the impact of AI on data processing.
2. How do I choose a data science dissertation topic?
Choose a topic that you find interesting, has enough data available, and is relevant to current trends in the field of data science.
3. What tools do I need for a data science dissertation?
You may need tools like Python, R, SQL, and data visualization software like Tableau or Power BI.
4. How long should my data science dissertation be?
The length varies, but most data science dissertations are around 80 to 120 pages. Check your institution’s guidelines for specific requirements.
Data Science Dissertation Topics Brief Service
Are you struggling to find the perfect Data Science Dissertation Topic tailored to your interests and expertise? Our customized topics brief service is designed to provide personalized guidance and support in selecting a dissertation topic that aligns with your academic goals. Fill the form below to get started on your journey towards academic excellence in data science.
Paid Topic Mini Proposal (500 Words)
You will get the topics first and then the mini proposal which includes:
- An explanation why we choose this topic.
- 2-3 research questions.
- Key literature resources identification.
- Suitable methodology including raw sample size and data collection method
- View a Sample of Service
Note: After submitting your order please must check your email [inbox/spam] folders for order confirmation and login details. If the email goes in spam please mark not as spam to avoid any communication gap between us.
Get An Expert Dissertation Writing Help To Achieve Good Grades
By placing an order with us, you can get;
- Writer consultation before payment to ensure your work is in safe hands.
- Free topic if you don't have one
- Draft submissions to check the quality of the work as per supervisor's feedback
- Free revisions
- Complete privacy
- Plagiarism Free work
- Guaranteed 2:1 (With help of your supervisor's feedback)
- 2 Instalments plan
- Special discounts
Other Posts
- 39 Information Systems Dissertation Topics Ideas February 20, 2022 -->
- 57 Best Forensic Science Dissertation Topics in 2023 March 17, 2020 -->
- 59 Anthropology Dissertation Topics Ideas & Examples March 17, 2020 -->
- 99 Internet Dissertation Topics Ideas and Examples February 7, 2020 -->
- Artificial Intelligence Dissertation Topics – AI February 20, 2024 -->
- Best 59 Networking Dissertation Topics Ideas & Examples February 26, 2020 -->
- Computer Science Research Topics (Approved Titles) February 4, 2020 -->
- IT Dissertation Topics and Ideas for Students February 26, 2020 -->
- Which topics are best for thesis in Computer Science? March 11, 2020 -->
WhatsApp and Get 35% off promo code now!
- Advertise with Us
.png)
- Cryptocurrencies
Top 10 Must-Read Data Science Research Papers in 2022
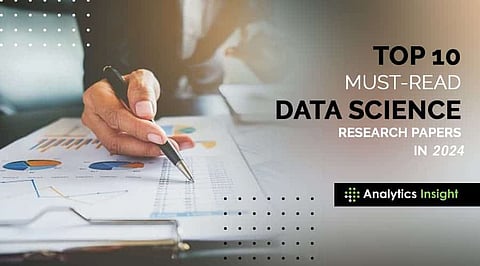
Data Science plays a vital role in many sectors such as small businesses, software companies, and the list goes on. Data Science understands customer preferences, demographics, automation, risk management, and many other valuable insights. Data Science can analyze and aggregate industry data. It has a frequency and real-time nature of data collection.
There are many data science enthusiasts out there who are totally into Data Science. The sad part is that they couldn't follow up with the latest research papers of Data Science. Here, Analytics Insight brings you the latest Data Science Research Papers. These research papers consist of different data science topics including the present fast passed technologies such as AI, ML, Coding, and many others. Data Science plays a very major role in applying AI, ML, and Coding. With the help of data science, we can improve our applications in various sectors. Here are the Data Science Research Papers in 2024
10DATA SCIENTISTS THAT TECH ENTHUSIASTS CAN FOLLOW ON LINKEDIN
ARE YOU A JOB SEEKER? KNOW THE IMPACT OF AI AND DATA SCIENCE
TOP 10 PYTHON + DATA SCIENCE COURSES YOU SHOULD TAKE UP IN 2022
The Research Papers Includes
Documentation matters: human-centered ai system to assist data science code documentation in computational notebooks.
The research paper is written by April Yi Wang, Dakuo Wang, Jaimie Drozda, Michael Muller, Soya Park, Justin D. Weisz, Xuye Lui, Lingfei Wu, Casey Dugan.
This research paper is all about AMC transactions on Computer-Human Interaction. This is a combination of code and documentation. In this research paper, the researchers have Themisto an automated documentation generation system. This explores how human-centered AI systems can support data scientists in Machine Learning code documentation.
Assessing the effects of fuel energy consumption, foreign direct investment and GDP on CO2 emission: New data science evidence from Europe & Central Asia
The research paper is written by- Muhammad Mohsin, SobiaNaseem, Muddassar Sarfraz Tamoor, Azam
This research paper deals with how bad the effects of fuel consumption are and how data science is playing a vital role in extracting such huge information.
Impact on Stock Market across Covid-19 Outbreak
The research paper is written by-CharmiGotecha
This paper analyses the impacts of a pandemic from 2019-2022 and how it has affected the world with the help of data science tools. It also talks about how data science played a major role in recovering the world from covid losses.
Exploring the political pulse of a country using data science tools
The research paper is written by Miguel G. Folgado, Veronica Sanz
This paper deals with how data science tools/techniques are used to analyses complex human communication. This study paper is an example of how Twitter data and different types of data science tools for political analysis.
Situating Data Science
The research paper is written by-Michelle HodaWilkerson, Joseph L. Polman
This research paper gives detailed information about regulating procurement understanding the ends and means of public procurement regulation.
VeridicalFlow: a Python package for building trustworthy data science pipelines with PCS
The research paper is written by- James Duncan, RushKapoor, Abhineet Agarwal, Chandan Singh, Bin Yu
This research paper is more of a journal of open-source software than a study paper. It deals with the open-source software that is the programs available in the systems that are related to data science.
From AI ethics principles to data science practice: a reflection and a gap analysis based on recent frameworks and practical experience
The research paper is written by-IlinaGeorgieva, ClaudioLazo, Tjerk Timan, Anne Fleur van Veenstra
This study paper deals with the field of AI ethics, its frameworks, evaluation, and much more. This paper contributes ethical AI by mapping AI ethical principles onto the lifestyle of artificial intelligence -based digital services or products to investigate their applicability for the practice of data science.
Building an Effective Data Science Practice
The research paper is written by Vineet Raina, Srinath Krishnamurthy
This paper is a complete guide for an effective data science practice. It gives an idea about how the data science team can be helpful and how productive they can be.
Detection of Road Traffic Anomalies Based on Computational Data Science
The research paper is written by Jamal Raiyn
This research paper gives an idea about autonomous vehicles will have control over every function and how data science will be part of taking full control over all the functions. Also, to manage large amounts of data collected from traffic in various formats, a Computational Data Science approach is proposed by the researchers.
Data Science Data Governance [AI Ethics]
The research paper is written by Joshua A. Kroll
This paper analyses and gives brief yet complete information about the best practices opted by organizations to manage their data which encompass the full range of responsibilities borne by the use of data in automated decision making, including data security, privacy, avoidance of undue discrimination, accountability, and transparency.
Join our WhatsApp Channel to get the latest news, exclusives and videos on WhatsApp
_____________
Disclaimer: Analytics Insight does not provide financial advice or guidance. Also note that the cryptocurrencies mentioned/listed on the website could potentially be scams, i.e. designed to induce you to invest financial resources that may be lost forever and not be recoverable once investments are made. You are responsible for conducting your own research (DYOR) before making any investments. Read more here.
Related Stories

Top 10 Data Science Project Ideas in 2024
Join over 2 million students who advanced their careers with 365 Data Science. Learn from instructors who have worked at Meta, Spotify, Google, IKEA, Netflix, and Coca-Cola and master Python, SQL, Excel, machine learning, data analysis, AI fundamentals, and more.
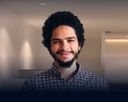
Data science is a practical field. You need various hands-on skills to stand out and advance your career. One of the best ways to obtain them is by building end-to-end data science projects that solve complex problems using real-world datasets.
Not sure where to start?
In this article, we provide 10 case studies from finance, healthcare, marketing, manufacturing, and other industries. You can use them as inspiration and adapt them to the domain of your interest.
All projects involve real business cases. Each one starts with a brief description of the problem, followed by an outline of the methodology, then the expected output, and finally, a recommended dataset and a relevant research paper. Most of the datasets are available on Kaggle or can be web scraped.
If you wish to start a project without the trouble of selecting and locating resources, we've prepared a series of engaging and relevant projects on our platform. These projects offer valuable hands-on practice to test your skills.
You can also include them in your portfolio to demonstrate to potential employers your experience in tackling everyday job challenges. For more information, check out the projects page on our website.
Below, we present 10 data science project ideas with step-by-step solutions. But first, we’ll explain what the data science life cycle is and how to execute an end-to-end project. Continue reading to learn to how to recognize and use your resources to turn information into a data science project.
Top 10 Data Science Project Ideas: Table of Contents
The data science life cycle, hospital treatment pricing prediction, youtube comments analysis, illegal fishing classification.
- Bank Customer Segmentation
Dogecoin Cryptocurrency Prices Predictor with LSTM
Book recommendation system, gender detection and age prediction using deep learning, speech emotion recognition for customer satisfaction, traveling agency customer service chatbots, detection of metallic surface defects.
- Data Science Project Ideas: Next Steps\
End-to-end projects involve real-world problems which you solve using the 6 stages of the data science life cycle:
- Business understanding
- Data understanding
- Data preparation
Here’s how to execute a data science project from end to end in more detail.
First, you define the business questions, requirements, and performance measurement. After that, you collect data to answer these questions. Then come the cleaning and preparation processes to get the data ready for exploration and analysis. These are the understanding stages.
But we’re not done yet.
Next comes the data preparation process. It involves the preprocessing and engineering of the features to prepare for the modeling step. Once that’s done, you can train the models on the prepared data. Depending on the task you are working on, you can do one of two things:
- Deploy the model on a live server and integrate it into a mobile or web application; then, monitor it and iterate again if needed, or
- Build dashboards based on the insights extracted from the data and the modeling step.
That wraps up the data science life cycle. Before you start working, you need some ideas for a data science project.
For starters, select a domain you are interested in. You can choose one that fits your educational background or previous work experience. This will give you a head start as you will know the field.
After that, you need to explore the common problems in this domain and how data science can solve them. Finally, choose a case study and formulate the business questions. Only then can you apply the life cycle we discussed above.
Now, let’s get started with a few project ideas.
The increasing cost of healthcare services is a major concern, especially for patients in the US. However, if planned properly, it can be reduced significantly.
The purpose of this project is to predict hospital charges before admitting a patient. Data science projects like this one are a great addition to your portfolio, especially if you want to pursue a career in healthcare .
Project Description
This will allow people to compare the costs at different medical institutions and plan their finances accordingly in case of elective admissions. It will also enable insurance companies to predict how much a patient with a particular medical condition might claim after a hospitalization.
You can solve this project using predictive analysis . This type of advanced analytics allows us to make predictions about future outcomes based on historical data. Typically, it involves statistical modeling, data mining, and machine learning techniques. In this case, we estimate hospital treatment costs based on the patient’s clinical data at admission.
Methodology
- Collect the hospital package pricing dataset
- Explore and understand the data
- Clean the data
- Perform engineering and preprocessing to prepare for the modeling step
- Select the suitable predictive model and train it with the data
- Deploy the model on a live server and integrate it into a web application to predict the pricing in real time
- Monitor the model in production and iterate
Expected Output
There are two expected outputs from this project:
- Analytical dashboard with insights extracted from the data that can be delivered to hospital and insurance companies
- Deployed predictive model into production on a live server that can be integrated into a web or mobile application and predict treatment costs in real time
Suggest Dataset:
- Package Pricing at Mission Hospital
Research Paper:
- Predicting the Inpatient Hospital Cost Using Machine Learning
This following example is form the marketing and finance domain .
Sentiment analysis or opinion mining refers to the analysis of the attitudes, feedback, and emotions users express on social media and other online platforms. It involves the detection of patterns in natural language that allude to people’s attitudes toward certain products or topics.
YouTube is the second most popular website in the world. Its comments section is a great source of user opinions on various topics. There are many examples of how you can approach such a data science project.
Let’s explore one of them.
You can analyze YouTube comments with natural language processing techniques. Begin by scraping text data using the library YouTube-Comment-Scraper-Python. It fetches comments utilizing browser automation.
Then, apply natural processing and text processing techniques to extract features, analyze them, and find the answers to the business questions you posed. You can build a dashboard to present the insights.
- Define the business questions you want to answer
- Build a web scrapper to collect data
- Clean the scraped data
- Text preprocessing to extract features
- Exploratory data analysis to extract insights from the data
- Build dashboards to present the insights interactively
Dashboards with insights from the scraped data.
Suggested Data
- Most Liked Comments on YouTube
- Analysis and Classification of User Comments on YouTube Videos
- Sentiment Analysis on YouTube Comments: A Brief Study
Marine life has a significant impact on our planet, providing food, oxygen, and biodiversity. Unfortunately, 90% of the large fish are gone primarily as a result of overfishing . In addition, many major fisheries notice increases in illegal fishing, undermining the efforts to conserve and manage fish stocks.
Detecting fishing activities in the ocean is a crucial step in achieving sustainability. It’s also an excellent big data project to add to your portfolio.
Identifying whether a vessel is fishing illegally and where this activity is likely to occur is a major step in ending illegal, unreported, and unregulated (IUU) fishing. However, monitoring the oceans is costly, time-consuming, and logistically difficult.
To overcome these challenges, we must improve the ability to detect and predict illegal fishing. This can be done using classification machine learning models to recognize and trace illegal fishing activity by collecting and processing GPS data from ships, as well as other pieces of information. The classification algorithm can distinguish these ships by type, fishing gear, and fishing behaviors.
- Collect the fishing watch dataset
- Perform data exploration to understand it better
- Perform engineering to extract features from the data
- Train classification models to categorize the fishing activity
- Deploy the trained model on a live server and integrate it into a web application
- Finish by monitoring the model in production and iterating
Deployed model running in a live server and used within a web service or mobile application to predict illegal fishing in real time.
Suggested Dataset
- Global Fishing Watch datasets
Research Papers
- Fishing Activity Detection from AIS Data Using Autoencoders
- Predicting Illegal Fishing on the Patagonia Shelf from Oceanographic Seascapes
The competition in the banking sector is increasing. To improve their services and retain and attract clients, banking and non-bank institutions need to modernize their marketing and customer strategies through personalization.
There are various data science models that could aid these efforts. Here, we focus on customer segmentation analysis .
Customer or market segmentation helps develop more effective investment and personalization strategies with the available information about clients. This is the process of grouping customers based on common characteristics, such as demographics or behaviors. This substantially improves targeting.
In this project, we segment Indian bank customers using data from more than one million transactions. We extract valuable information from these clusters and build dashboards with the insights. The final outputs can be used to improve products and marketing strategies.
- Define the questions you would like to answer with the data
- Collect the customer dataset
- Perform exploratory data analysis to have a better understanding of the data
- Perform feature preprocessing
- Train clustering models to segment the data into a selected number of groups
- Conduct cluster analysis to extract insights
- Build dashboards with the insights
Dashboards with marketing insights extracted from the segmented customers.
- A Customer Segmentation Approach in Commercial Banks
Dogecoin became one of the most popularity cryptocurrencies in recent years. Its price peaked in 2021, and it’s been slowly decreasing in 2022. That’s the case with most cryptocurrencies in the current economic situation.
However, the constant fluctuations make it hard for a human being to predict with accuracy the future prices. As such, automated algorithms are commonly used in finance .
This is an extremely valuable data science project for your resume if you want to pursue a career in this domain. If that’s your goal, you also need to learn how to use Python for Finance .
In this section, we discuss a time series forecasting project, commonly encountered in the financial sector .
A time series is a sequence of data points distributed over a time span. With forecasting, we can recognize patterns and predict future incidents based on historical trends. This type of data analytics projects can be conducted using several models, including ARIMA (autoregressive integrated moving average), regression algorithms, and long short-term memory (LSTM).
- Collect the historical price data of the Dogecoin cryptocurrency
- Manipulate and clean the data
- Explore the data to have a better understanding
- Train a deep learning model to predict the future change in prices
- Deploy the model on a live server to predict the changes in real time
Deployed model into production integrated into a cryptocurrency trading web or mobile application. You can also build a dashboard based on the data insights to help understand the dynamics of Dogecoin.
- Dogecoin Historical Price Data
Project Overview
Flawed products can result in substantial financial losses, so defect detection is crucial in manufacturing. Although human detection systems are still the traditional method employed, computer vision techniques are more effective.
In this example, we build a system to detect defects in metallic objects or surfaces during different phases of the production processes.
The types of defects can be aesthetic, such as stains, or potentially damaging the product’s functionality, such as notches, scratches, burns, lack of rectification, bumps, burrs, flatness, lack of thread, countersunk, rust, or cracks.
Since the appearance of metallic surfaces changes substantially with different lighting, defects are hard to detect even using computer vision. For this reason, lighting is a crucial component in solving such types of data science problems. Otherwise, the methodology of this project is standard.
- Collect the metal surface defects dataset
- Data cleaning and exploration
- Feature extraction
- Train models for defects detection and classification
- Deploy the model into production on an embedded system
A deployed model on an embedded system that can detect and classify metallic surface defects in different conditions and environments.
- Metal Surface Defects Dataset
- Online Metallic Surface Defect Detection Using Deep Learning
Data Science Project Ideas: Next Steps
Having diverse and complex data science projects in your portfolio is a great way to demonstrate your skills to future employers. You can choose one from the list above or use it as inspiration and come up with your own idea.
But first, make sure you have the necessary skills to solve these problems. If you want to start with something simpler, try the 365 Data Science Career Track . That way, you can build your foundational knowledge and gradually progress to more advanced topics. In the meantime, the instructors will guide you through the completion of real-life data science projects. Sign up and start your learning journey with a selection of free courses.
World-Class
Data Science
Learn with instructors from:
Youssef Hosni
Computer Vision Researcher / Data Scientist
Youssef is a computer vision researcher working towards his Ph.D. His research focuses on developing real-time computer vision algorithms for healthcare applications. He also worked as a data scientist, using customers' data to gain a better understanding of their behavior. Youssef is passionate about data and believes in AI's power to improve people's lives. He hopes to transfer his passion to others and guide them into this wide field through his writings.
We Think you'll also like
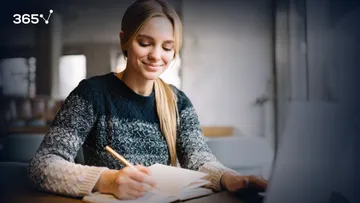
Career Advice
Top 5 Motivational Tips for Studying Data Science in 2024
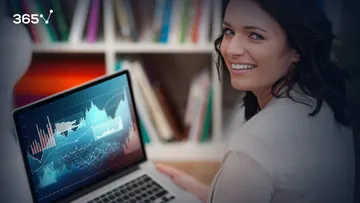
Job Interview Tips
Top 18 Probability and Statistics Interview Questions for Data Scientists
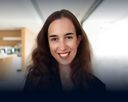
How to Transition Your Career into Data Science in 2024: A Step-by-Step Guide
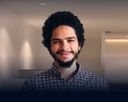
Best Free Data Science Resources for Beginners (2024)
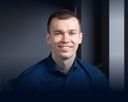

A Guide to Data Science Research Projects

Ria Cheruvu
Towards Data Science
Starting a data science research project can be challenging, whether you’re a novice or a seasoned engineer — you want your project to be meaningful , accessible , and valuable to the data science community and your portfolio. In this post, I’ll introduce two frameworks you can use as a guide for your data science research projects. Please note this guide isn’t mean to be exhaustive — it’s based on my experiences working with data science and machine learning that I think can be helpful for beginner data scientists.
Research Process — Ideation to Implementation
- Identify the research problem you want to explore or solve.
- Ask yourself the following questions:
- Do I want to focus on engineering work or pure research?
For example, if you are building a new machine learning application, engineering might involve building a framework and user interface for the algorithm and using deployment infrastructures to speed up data access.
Pure research can involve trying to manipulate the properties of the model in innovative ways (e.g., create a new loss function) or to create an entirely new model, such as the type of research you see in papers from NeurIPS and similar conferences.
It is possible to also do a blend of engineering and pure research — you need to think about the approach that best fits the research goal.
- Is the goal of my research to outperform an existing baseline (e.g., get the highest score for the ImageNet dataset), or to explore a research field using an innovative methodology where a solid baseline may not have been already established? Both types of research problems are valuable for data science.
3. Perform a literature review. Look at existing work in the field and analyze the strengths and weaknesses of relevant methodologies. Identify gaps in the literature you are interested in exploring.
4. Design your proposed solution and implement a baseline/prototype (see the development process below).
5. Iteratively develop the prototype, based on your answers from Step 2.
Development Process — Prerequisites
The goal of the following steps is to guide you to pick the optimal data storage option, machine learning model, and development and deployment processes for your use case. After outlining these aspects, I recommend the development process can then be started.
- What is the type of the data and how will it be stored?
- If the variable you are attempting to predict is numerical, you could define your problem statement as a normal regression application. Few of the popular ML models for approaching this are linear regression, decision trees, support vector machines, and neural networks or deep learning.
- If the data is ordered by time, you could consider time series methods — there are traditional algorithms for this in addition to deep learning methods.
- If you are working with a large amount of data, depending on the data format, you could consider exploring big data storage and processing solutions, such as Apache Spark, Cassandra, Redis, etc. Some of the big data storage solutions easily and natively support machine learning tools/algorithms.
2. Where will the model be hosted and how will it be deployed? Can the deliverable be structured as a pipeline or system, and if so, what do the inputs and outputs if the system look like?
As part of this step, you’ll need to ask the question: “Are there any constraints on the types of models that I can use?” For example, will the model be used for a streaming data application (data is fed to the model consistently over certain time periods, such as 1 hour) or a typical prediction on a large dataset? Will your users access the model via command line or a web page?
You could consider using tools like Luigi and Apache AirFlow to help with the pipelining and workflow management of your project.
3. What are the critical and good-to-have characteristics of the models I am looking for and what ML algorithms fit that description?
To answer this, you’ll need to circle back to the goal of your project. Here are some example questions you might ask:
- Is your goal to predict and improve a certain factor or event based on data?
- Do you think understanding the features your algorithm is using to make a prediction is important (e.g., to understand how to improve that certain factor/event)?
This might determine your model choice. For example, with decision trees, you can identify which features your algorithm is considering most important for the prediction and the decision path, but these models may not provide great accuracy for your application. However, neural networks could provide great accuracy, but are more challenging to interpret.
There are solutions that allow you to get both interpretability and good accuracy. For example, neural networks and decision trees can be combined through Neural-Backed Decision Trees , although these approaches may have certain trade-offs (e.g., requiring pre-trained weights). You’ll need to investigate the pros and cons of the approaches you’re considering to evaluate how they fit into your problem statement.
4. How will you debug and evaluate your models? What are the metrics you will consider and any visualizations?
- The metrics you choose can be dependent on existing approaches in the literature, which you may have identified during the research process. For example, you may choose to include Matthews Correlation Coefficient or balanced accuracy, in addition to the original accuracy metric, when working with imbalanced data.
- As you consider what tools and processes you will implement for your pipeline, remember to document any important changes in hyperparameters you may make to your models or use a tool such as Weights and Biases , for debug purposes.
- Visualizations are an important part of the development process — they can be used for debugging your model (e.g., learning curves), interpretability (e.g., salience maps), and for data analysis and final reporting (e.g., word clouds). Before starting your development, try to think about the types of visualizations that can add to the implementation, quality, and value of your project.
Each step within these two frameworks requires some thought, and trial and error. I’ll be covering some helpful tips on these steps in future blog posts, so if you found this post helpful, stay tuned for more!
Originally published at http://demystifymachinelearning.wordpress.com on April 5, 2021.
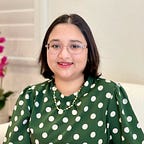
Written by Ria Cheruvu
AI SW Architect and Evangelist at Intel, master’s in data science. Opinions are my own.
Text to speech

Top 100 Data Science Project Ideas For Final Year
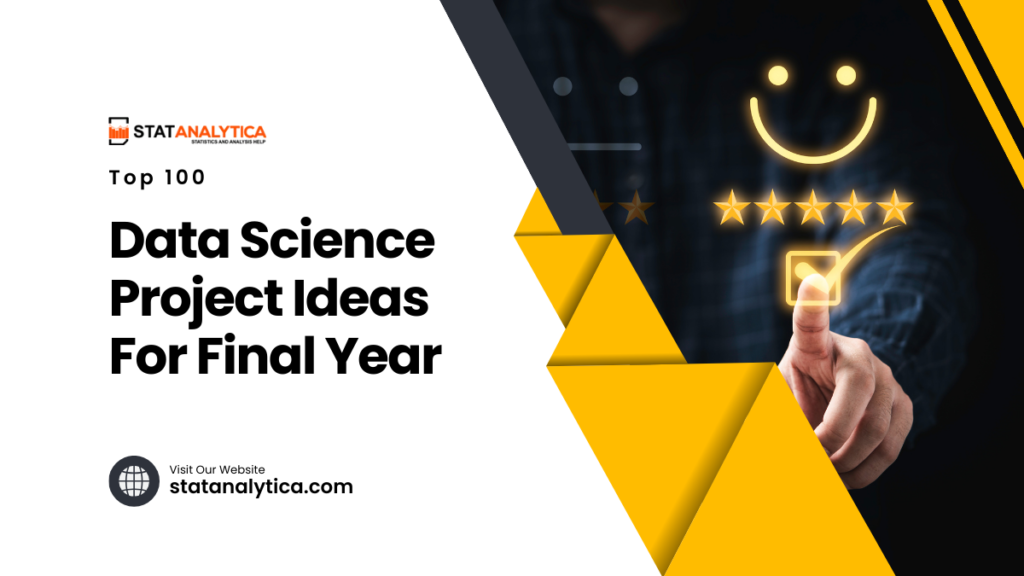
Are you a final year student diving into the world of data science, seeking inspiration for your final project? Look no further! In this blog, we’ll explore a variety of engaging and practical data science project ideas for final year that are perfect for showcasing your skills and creativity. Whether you’re interested in analyzing data trends, building machine learning models, or delving into natural language processing, we’ve got you covered. Let’s dive in!
What is Data Science?
Table of Contents
Data science is a multidisciplinary field that combines various techniques, algorithms, and tools to extract insights and knowledge from structured and unstructured data. At its core, data science involves the use of statistical analysis, machine learning, data mining, and data visualization to uncover patterns, trends, and correlations within datasets.
In simpler terms, data science is about turning raw data into actionable insights. It involves collecting, cleaning, and organizing data, analyzing it to identify meaningful patterns or relationships, and using those insights to make informed decisions or predictions.
Data science encompasses a wide range of applications across industries and domains, including but not limited to:
- Business: Analyzing customer behavior, optimizing marketing strategies, and improving operational efficiency.
- Healthcare: Predicting patient outcomes, diagnosing diseases, and personalized medicine.
- Finance: Fraud detection, risk management, and algorithmic trading.
- Technology: Natural language processing, image recognition, and recommendation systems.
- Environmental Science: Climate modeling, predicting natural disasters, and analyzing environmental data.
In summary, data science is a powerful discipline that leverages data-driven approaches to solve complex problems, drive innovation, and generate value in various fields and industries.
It plays a crucial role in today’s data-driven world, enabling organizations to make better decisions, improve processes, and create new opportunities for growth and development.
How to Select Data Science Project Ideas For Final Year?
Selecting the right data science project idea for your final year is crucial as it can shape your learning experience, showcase your skills to potential employers, and contribute to solving real-world problems. Here’s a step-by-step guide on how to select data science project ideas for your final year:
- Understand Your Interests and Strengths
Reflect on your interests within the field of data science. Are you passionate about healthcare, finance, social media, or environmental issues? Consider your strengths as well.
Are you proficient in programming languages like Python or R? Do you have experience with statistical analysis, machine learning, or data visualization? Identifying your interests and strengths will help narrow down project ideas that align with your skills and passions.
- Consider the Impact
Think about the impact you want your project to have. Do you aim to address a specific problem or challenge in society, industry, or academia?
Consider the potential beneficiaries of your project and how it can contribute to positive change. Projects with a clear and measurable impact are often more compelling and rewarding.
- Assess Data Availability
Check the availability of relevant datasets for your project idea. Are there publicly available datasets that you can use for analysis? Can you collect data through web scraping, APIs, or surveys?
Ensure that the data you plan to work with is reliable, relevant, and adequately sized to support your analysis and modeling efforts.
- Define Clear Objectives
Clearly define the objectives of your project. What do you aim to accomplish? Are you exploring trends, building predictive models, or developing new algorithms?
Establishing clear objectives will guide your project’s scope, methodology, and evaluation criteria.
- Explore Project Feasibility
Evaluate the feasibility of your project idea given the resources and time constraints of your final year.
Consider factors such as data availability, computational requirements, and the complexity of the techniques you plan to use. Choose a project idea that is challenging yet achievable within your timeframe and resources.
- Seek Inspiration and Guidance
Look for inspiration from existing data science projects, research papers, and industry case studies. Attend workshops, conferences, or webinars related to data science to stay updated on emerging trends and technologies.
Seek guidance from your professors, mentors, or industry professionals who can provide valuable insights and feedback on your project ideas.
- Brainstorm and Refine
Brainstorm multiple project ideas and refine them based on feedback, feasibility, and alignment with your interests and goals.
Consider interdisciplinary approaches that combine data science with other fields such as healthcare, finance, or environmental science. Iterate on your ideas until you find one that excites you and meets the criteria outlined above.
- Plan for Iterative Development
Recognize that data science projects often involve iterative development and refinement.
Plan to iterate on your project as you gather new insights, experiment with different techniques, and incorporate feedback from stakeholders. Embrace the iterative process as an opportunity for continuous learning and improvement.
By following these steps, you can select a data science project idea for your final year that is engaging, impactful, and aligned with your interests and aspirations. Remember to stay curious, persistent, and open to exploring new ideas throughout your project journey.
Exploratory Data Analysis Projects
- Analysis of demographic trends using census data
- Social media sentiment analysis
- Customer segmentation for marketing strategies
- Stock market trend analysis
- Crime rates and patterns in urban areas
Machine Learning Projects
- Healthcare outcome prediction
- Fraud detection in financial transactions
- E-commerce recommendation systems
- Housing price prediction
- Sentiment analysis for product reviews
Natural Language Processing (NLP) Projects
- Text summarization for news articles
- Topic modeling for large text datasets
- Named Entity Recognition (NER) for extracting entities from text
- Social media comment sentiment analysis
- Language translation tools for multilingual communication
Big Data Projects
- IoT data analysis
- Real-time analytics for streaming data
- Recommendation systems using big data platforms
- Social network data analysis
- Predictive maintenance for industrial equipment
Data Visualization Projects
- Interactive COVID-19 dashboard
- Geographic information system (GIS) for spatial data analysis
- Network visualization for social media connections
- Time-series analysis for financial data
- Climate change data visualization
Healthcare Projects
- Disease outbreak prediction
- Patient readmission rate prediction
- Drug effectiveness analysis
- Medical image classification
- Electronic health record analysis
Finance Projects
- Stock price prediction
- Credit risk assessment
- Portfolio optimization
- Fraud detection in banking transactions
- Financial market trend analysis
Marketing Projects
- Customer churn prediction
- Market segmentation analysis
- Brand sentiment analysis
- Ad campaign optimization
- Social media influencer identification
E-commerce Projects
- Product recommendation systems
- Customer lifetime value prediction
- Market basket analysis
- Price elasticity modeling
- User behavior analysis
Education Projects
- Student performance prediction
- Dropout rate analysis
- Personalized learning recommendation systems
- Educational resource allocation optimization
- Student sentiment analysis
Environmental Projects
- Air quality prediction
- Climate change impact analysis
- Wildlife conservation modeling
- Water quality monitoring
- Renewable energy forecasting
Social Media Projects
- Trend detection
- Fake news detection
- Influencer identification
- Social network analysis
- Hashtag sentiment analysis
Retail Projects
- Inventory management optimization
- Demand forecasting
- Customer segmentation for targeted marketing
- Price optimization
Telecommunications Projects
- Network performance optimization
- Fraud detection
- Call volume forecasting
- Subscriber segmentation analysis
Supply Chain Projects
- Inventory optimization
- Supplier risk assessment
- Route optimization
- Supply chain network analysis
Automotive Projects
- Predictive maintenance for vehicles
- Traffic congestion prediction
- Vehicle defect detection
- Autonomous vehicle behavior analysis
- Fleet management optimization
Energy Projects
- Predictive maintenance for equipment
- Energy consumption forecasting
- Renewable energy optimization
- Grid stability analysis
- Demand response optimization
Agriculture Projects
- Crop yield prediction
- Pest detection
- Soil quality analysis
- Irrigation optimization
- Farm management systems
Human Resources Projects
- Employee churn prediction
- Performance appraisal analysis
- Diversity and inclusion analysis
- Recruitment optimization
- Employee sentiment analysis
Travel and Hospitality Projects
- Demand forecasting for hotel bookings
- Customer sentiment analysis for reviews
- Pricing strategy optimization
- Personalized travel recommendations
- Destination popularity prediction
Embarking on data science projects in their final year presents students with an excellent opportunity to apply their skills, gain practical experience, and make a tangible impact.
Whether it’s exploring demographic trends, building predictive models, or visualizing complex datasets, these projects offer a platform for innovation and learning.
By undertaking these data science project ideas for final year, final year students can hone their data science skills and prepare themselves for a successful career in this rapidly evolving field.
Related Posts
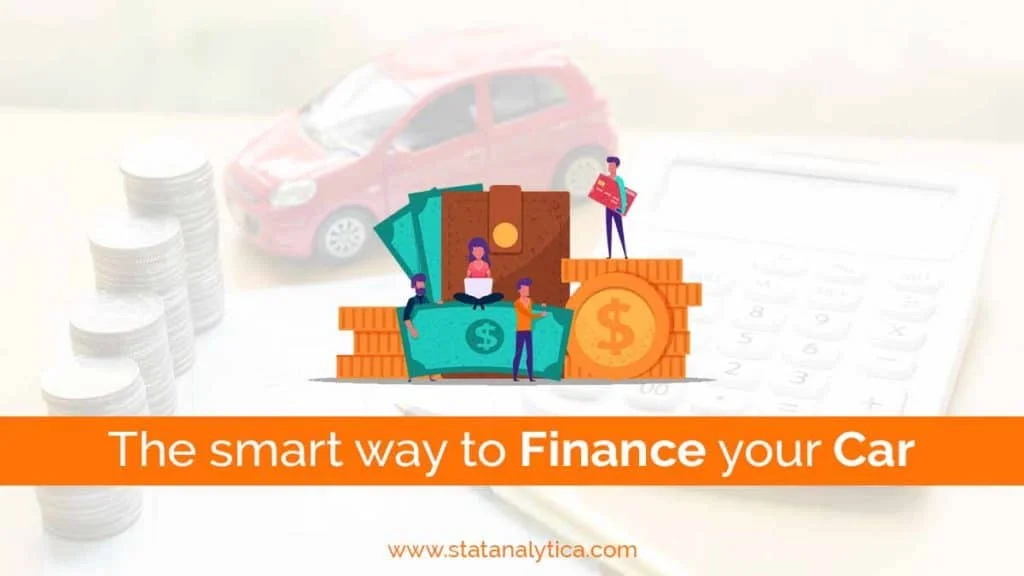
Step by Step Guide on The Best Way to Finance Car
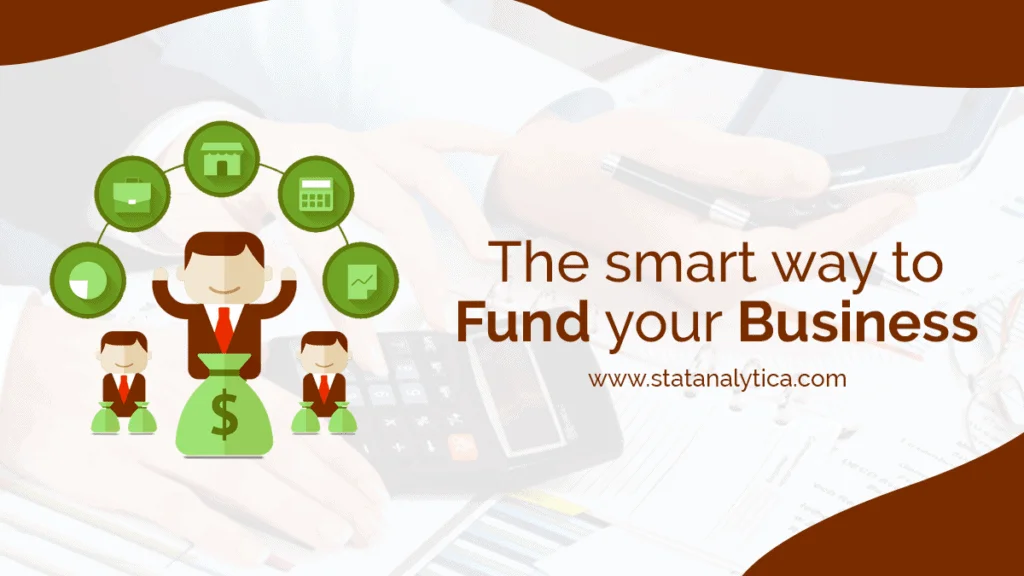
The Best Way on How to Get Fund For Business to Grow it Efficiently
Mon - Sat 9:00am - 12:00am
- Get a quote
List of Best Research and Thesis Topic Ideas for Data Science in 2022
In an era driven by digital and technological transformation, businesses actively seek skilled and talented data science potentials capable of leveraging data insights to enhance business productivity and achieve organizational objectives. In keeping with an increasing demand for data science professionals, universities offer various data science and big data courses to prepare students for the tech industry. Research projects are a crucial part of these programs and a well- executed data science project can make your CV appear more robust and compelling. A broad range of data science topics exist that offer exciting possibilities for research but choosing data science research topics can be a real challenge for students . After all, a good research project relies first and foremost on data analytics research topics that draw upon both mono-disciplinary and multi-disciplinary research to explore endless possibilities for real –world applications.
As one of the top-most masters and PhD online dissertation writing services , we are geared to assist students in the entire research process right from the initial conception to the final execution to ensure that you have a truly fulfilling and enriching research experience. These resources are also helpful for those students who are taking online classes .
By taking advantage of our best digital marketing research topics in data science you can be assured of producing an innovative research project that will impress your research professors and make a huge difference in attracting the right employers.
Get an Immediate Response
Discuss your requirments with our writers
Get 3 Customize Research Topic within 24 Hours
Undergraduate Masters PhD Others
Data science thesis topics
We have compiled a list of data science research topics for students studying data science that can be utilized in data science projects in 2022. our team of professional data experts have brought together master or MBA thesis topics in data science that cater to core areas driving the field of data science and big data that will relieve all your research anxieties and provide a solid grounding for an interesting research projects . The article will feature data science thesis ideas that can be immensely beneficial for students as they cover a broad research agenda for future data science . These ideas have been drawn from the 8 v’s of big data namely Volume, Value, Veracity, Visualization, Variety, Velocity, Viscosity, and Virility that provide interesting and challenging research areas for prospective researches in their masters or PhD thesis . Overall, the general big data research topics can be divided into distinct categories to facilitate the research topic selection process.
- Security and privacy issues
- Cloud Computing Platforms for Big Data Adoption and Analytics
- Real-time data analytics for processing of image , video and text
- Modeling uncertainty
How “The Research Guardian” Can Help You A lot!
Our top thesis writing experts are available 24/7 to assist you the right university projects. Whether its critical literature reviews to complete your PhD. or Master Levels thesis.
DATA SCIENCE PHD RESEARCH TOPICS
The article will also guide students engaged in doctoral research by introducing them to an outstanding list of data science thesis topics that can lead to major real-time applications of big data analytics in your research projects.
- Intelligent traffic control ; Gathering and monitoring traffic information using CCTV images.
- Asymmetric protected storage methodology over multi-cloud service providers in Big data.
- Leveraging disseminated data over big data analytics environment.
- Internet of Things.
- Large-scale data system and anomaly detection.
What makes us a unique research service for your research needs?
We offer all –round and superb research services that have a distinguished track record in helping students secure their desired grades in research projects in big data analytics and hence pave the way for a promising career ahead. These are the features that set us apart in the market for research services that effectively deal with all significant issues in your research for.
- Plagiarism –free ; We strictly adhere to a non-plagiarism policy in all our research work to provide you with well-written, original content with low similarity index to maximize chances of acceptance of your research submissions.
- Publication; We don’t just suggest PhD data science research topics but our PhD consultancy services take your research to the next level by ensuring its publication in well-reputed journals. A PhD thesis is indispensable for a PhD degree and with our premier best PhD thesis services that tackle all aspects of research writing and cater to essential requirements of journals , we will bring you closer to your dream of being a PhD in the field of data analytics.
- Research ethics: Solid research ethics lie at the core of our services where we actively seek to protect the privacy and confidentiality of the technical and personal information of our valued customers.
- Research experience: We take pride in our world –class team of computing industry professionals equipped with the expertise and experience to assist in choosing data science research topics and subsequent phases in research including findings solutions, code development and final manuscript writing.
- Business ethics: We are driven by a business philosophy that‘s wholly committed to achieving total customer satisfaction by providing constant online and offline support and timely submissions so that you can keep track of the progress of your research.
Now, we’ll proceed to cover specific research problems encompassing both data analytics research topics and big data thesis topics that have applications across multiple domains.
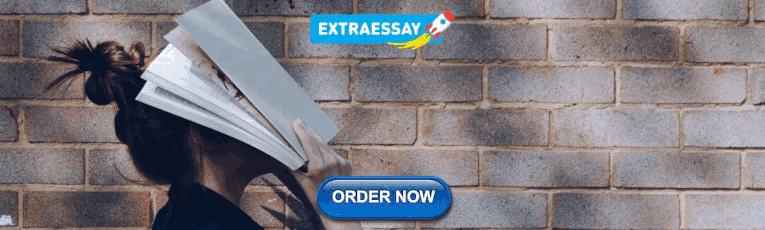
Get Help from Expert Thesis Writers!
TheresearchGuardian.com providing expert thesis assistance for university students at any sort of level. Our thesis writing service has been serving students since 2011.
Multi-modal Transfer Learning for Cross-Modal Information Retrieval
Aim and objectives.
The research aims to examine and explore the use of CMR approach in bringing about a flexible retrieval experience by combining data across different modalities to ensure abundant multimedia data.
- Develop methods to enable learning across different modalities in shared cross modal spaces comprising texts and images as well as consider the limitations of existing cross –modal retrieval algorithms.
- Investigate the presence and effects of bias in cross modal transfer learning and suggesting strategies for bias detection and mitigation.
- Develop a tool with query expansion and relevance feedback capabilities to facilitate search and retrieval of multi-modal data.
- Investigate the methods of multi modal learning and elaborate on the importance of multi-modal deep learning to provide a comprehensive learning experience.
The Role of Machine Learning in Facilitating the Implication of the Scientific Computing and Software Engineering
- Evaluate how machine learning leads to improvements in computational APA reference generator tools and thus aids in the implementation of scientific computing
- Evaluating the effectiveness of machine learning in solving complex problems and improving the efficiency of scientific computing and software engineering processes.
- Assessing the potential benefits and challenges of using machine learning in these fields, including factors such as cost, accuracy, and scalability.
- Examining the ethical and social implications of using machine learning in scientific computing and software engineering, such as issues related to bias, transparency, and accountability.
Trustworthy AI
The research aims to explore the crucial role of data science in advancing scientific goals and solving problems as well as the implications involved in use of AI systems especially with respect to ethical concerns.
- Investigate the value of digital infrastructures available through open data in aiding sharing and inter linking of data for enhanced global collaborative research efforts
- Provide explanations of the outcomes of a machine learning model for a meaningful interpretation to build trust among users about the reliability and authenticity of data
- Investigate how formal models can be used to verify and establish the efficacy of the results derived from probabilistic model.
- Review the concept of Trustworthy computing as a relevant framework for addressing the ethical concerns associated with AI systems.
The Implementation of Data Science and their impact on the management environment and sustainability
The aim of the research is to demonstrate how data science and analytics can be leveraged in achieving sustainable development.
- To examine the implementation of data science using data-driven decision-making tools
- To evaluate the impact of modern information technology on management environment and sustainability.
- To examine the use of data science in achieving more effective and efficient environment management
- Explore how data science and analytics can be used to achieve sustainability goals across three dimensions of economic, social and environmental.
Big data analytics in healthcare systems
The aim of the research is to examine the application of creating smart healthcare systems and how it can lead to more efficient, accessible and cost –effective health care.
- Identify the potential Areas or opportunities in big data to transform the healthcare system such as for diagnosis, treatment planning, or drug development.
- Assessing the potential benefits and challenges of using AI and deep learning in healthcare, including factors such as cost, efficiency, and accessibility
- Evaluating the effectiveness of AI and deep learning in improving patient outcomes, such as reducing morbidity and mortality rates, improving accuracy and speed of diagnoses, or reducing medical errors
- Examining the ethical and social implications of using AI and deep learning in healthcare, such as issues related to bias, privacy, and autonomy.
Large-Scale Data-Driven Financial Risk Assessment
The research aims to explore the possibility offered by big data in a consistent and real time assessment of financial risks.
- Investigate how the use of big data can help to identify and forecast risks that can harm a business.
- Categories the types of financial risks faced by companies.
- Describe the importance of financial risk management for companies in business terms.
- Train a machine learning model to classify transactions as fraudulent or genuine.
Scalable Architectures for Parallel Data Processing
Big data has exposed us to an ever –growing volume of data which cannot be handled through traditional data management and analysis systems. This has given rise to the use of scalable system architectures to efficiently process big data and exploit its true value. The research aims to analyses the current state of practice in scalable architectures and identify common patterns and techniques to design scalable architectures for parallel data processing.
- To design and implement a prototype scalable architecture for parallel data processing
- To evaluate the performance and scalability of the prototype architecture using benchmarks and real-world datasets
- To compare the prototype architecture with existing solutions and identify its strengths and weaknesses
- To evaluate the trade-offs and limitations of different scalable architectures for parallel data processing
- To provide recommendations for the use of the prototype architecture in different scenarios, such as batch processing, stream processing, and interactive querying
Robotic manipulation modelling
The aim of this research is to develop and validate a model-based control approach for robotic manipulation of small, precise objects.
- Develop a mathematical model of the robotic system that captures the dynamics of the manipulator and the grasped object.
- Design a control algorithm that uses the developed model to achieve stable and accurate grasping of the object.
- Test the proposed approach in simulation and validate the results through experiments with a physical robotic system.
- Evaluate the performance of the proposed approach in terms of stability, accuracy, and robustness to uncertainties and perturbations.
- Identify potential applications and areas for future work in the field of robotic manipulation for precision tasks.
Big data analytics and its impacts on marketing strategy
The aim of this research is to investigate the impact of big data analytics on marketing strategy and to identify best practices for leveraging this technology to inform decision-making.
- Review the literature on big data analytics and marketing strategy to identify key trends and challenges
- Conduct a case study analysis of companies that have successfully integrated big data analytics into their marketing strategies
- Identify the key factors that contribute to the effectiveness of big data analytics in marketing decision-making
- Develop a framework for integrating big data analytics into marketing strategy.
- Investigate the ethical implications of big data analytics in marketing and suggest best practices for responsible use of this technology.
Looking For Customize Thesis Topics?
Take a review of different varieties of thesis topics and samples from our website TheResearchGuardian.com on multiple subjects for every educational level.
Platforms for large scale data computing: big data analysis and acceptance
To investigate the performance and scalability of different large-scale data computing platforms.
- To compare the features and capabilities of different platforms and determine which is most suitable for a given use case.
- To identify best practices for using these platforms, including considerations for data management, security, and cost.
- To explore the potential for integrating these platforms with other technologies and tools for data analysis and visualization.
- To develop case studies or practical examples of how these platforms have been used to solve real-world data analysis challenges.
Distributed data clustering
Distributed data clustering can be a useful approach for analyzing and understanding complex datasets, as it allows for the identification of patterns and relationships that may not be immediately apparent.
To develop and evaluate new algorithms for distributed data clustering that is efficient and scalable.
- To compare the performance and accuracy of different distributed data clustering algorithms on a variety of datasets.
- To investigate the impact of different parameters and settings on the performance of distributed data clustering algorithms.
- To explore the potential for integrating distributed data clustering with other machine learning and data analysis techniques.
- To apply distributed data clustering to real-world problems and evaluate its effectiveness.
Analyzing and predicting urbanization patterns using GIS and data mining techniques".
The aim of this project is to use GIS and data mining techniques to analyze and predict urbanization patterns in a specific region.
- To collect and process relevant data on urbanization patterns, including population density, land use, and infrastructure development, using GIS tools.
- To apply data mining techniques, such as clustering and regression analysis, to identify trends and patterns in the data.
- To use the results of the data analysis to develop a predictive model for urbanization patterns in the region.
- To present the results of the analysis and the predictive model in a clear and visually appealing way, using GIS maps and other visualization techniques.
Use of big data and IOT in the media industry
Big data and the Internet of Things (IoT) are emerging technologies that are transforming the way that information is collected, analyzed, and disseminated in the media sector. The aim of the research is to understand how big data and IoT re used to dictate information flow in the media industry
- Identifying the key ways in which big data and IoT are being used in the media sector, such as for content creation, audience engagement, or advertising.
- Analyzing the benefits and challenges of using big data and IoT in the media industry, including factors such as cost, efficiency, and effectiveness.
- Examining the ethical and social implications of using big data and IoT in the media sector, including issues such as privacy, security, and bias.
- Determining the potential impact of big data and IoT on the media landscape and the role of traditional media in an increasingly digital world.
Exigency computer systems for meteorology and disaster prevention
The research aims to explore the role of exigency computer systems to detect weather and other hazards for disaster prevention and response
- Identifying the key components and features of exigency computer systems for meteorology and disaster prevention, such as data sources, analytics tools, and communication channels.
- Evaluating the effectiveness of exigency computer systems in providing accurate and timely information about weather and other hazards.
- Assessing the impact of exigency computer systems on the ability of decision makers to prepare for and respond to disasters.
- Examining the challenges and limitations of using exigency computer systems, such as the need for reliable data sources, the complexity of the systems, or the potential for human error.
Network security and cryptography
Overall, the goal of research is to improve our understanding of how to protect communication and information in the digital age, and to develop practical solutions for addressing the complex and evolving security challenges faced by individuals, organizations, and societies.
- Developing new algorithms and protocols for securing communication over networks, such as for data confidentiality, data integrity, and authentication
- Investigating the security of existing cryptographic primitives, such as encryption and hashing algorithms, and identifying vulnerabilities that could be exploited by attackers.
- Evaluating the effectiveness of different network security technologies and protocols, such as firewalls, intrusion detection systems, and virtual private networks (VPNs), in protecting against different types of attacks.
- Exploring the use of cryptography in emerging areas, such as cloud computing, the Internet of Things (IoT), and blockchain, and identifying the unique security challenges and opportunities presented by these domains.
- Investigating the trade-offs between security and other factors, such as performance, usability, and cost, and developing strategies for balancing these conflicting priorities.
Meet Our Professionals Ranging From Renowned Universities
Related topics.
- Sports Management Research Topics
- Special Education Research Topics
- Software Engineering Research Topics
- Primary Education Research Topics
- Microbiology Research Topics
- Luxury Brand Research Topics
- Cyber Security Research Topics
- Commercial Law Research Topics
- Change Management Research Topics
- Artificial intelligence Research Topics
20 Data Science Topics and Areas
It is no doubt that data science topics and areas are some of the hottest business points today.
We collected some basic and advanced topics in data science to give you ideas on where to master your skills.
In today’s landscape, businesses are investing in corporate data science training to enhance their employees’ data science capabilities.
Data science topics also are hot subjects you can use as directions to prepare yourself for data science job interview questions.
1. The core of data mining process
This is an example of a wide data science topic.
What is it?
Data mining is an iterative process that involves discovering patterns in large data sets. It includes methods and techniques such as machine learning, statistics, database systems and etc.
The two main data mining objectives are to find out patterns and establish trends and relationship in a dataset in order to solve problems.
The general stages of the data mining process are: problem definition, data exploration, data preparation, modeling, evaluation, and deployment.
Core terms related to data mining are classification, predictions, association rules, data reduction, data exploration, supervised and unsupervised learning, datasets organization, sampling from datasets, building a model and etc.
2. Data visualization
Data visualization is the presentation of data in a graphical format.
It enables decision-makers of all levels to see data and analytics presented visually, so they can identify valuable patterns or trends.
Data visualization is another broad subject that covers the understanding and use of basic types of graphs (such as line graphs, bar graphs, scatter plots , histograms, box and whisker plots , heatmaps.
You cannot go without these graphs. In addition, here you need to learn about multidimensional variables with adding variables and using colors, size, shapes, animations.
Manipulation also plays a role here. You should be able to rascal, zoom, filter, aggregate data.
Using some specialized visualizations such as map charts and tree maps is a hot skill too.
3. Dimension reduction methods and techniques
Dimension Reduction process involves converting a data set with vast dimensions into a dataset with lesser dimensions ensuring that it provides similar information in short.
In other words, dimensionality reduction consists of series of techniques and methods in machine learning and statistics to decrease the number of random variables.
There are so many methods and techniques to perform dimension reduction.
The most popular of them are Missing Values, Low Variance, Decision Trees, Random Forest, High Correlation, Factor Analysis, Principal Component Analysis, Backward Feature Elimination.
4. Classification
Classification is a core data mining technique for assigning categories to a set of data.
The purpose is to support gathering accurate analysis and predictions from the data.
Classification is one of the key methods for making the analysis of a large amount of datasets effective.
Classification is one of the hottest data science topics too. A data scientist should know how to use classification algorithms to solve different business problems.
This includes knowing how to define a classification problem, explore data with univariate and bivariate visualization, extract and prepare data, build classification models, evaluate models, and etc. Linear and non-linear classifiers are some of the key terms here.
5. Simple and multiple linear regression
Linear regression models are among the basic statistical models for studying relationships between an independent variable X and Y dependent variable.
It is a mathematical modeling which allows you to make predictions and prognosis for the value of Y depending on the different values of X.
There are two main types of linear regression: simple linear regression models and multiple linear regression models.
Key points here are terms such as correlation coefficient, regression line, residual plot, linear regression equation and etc. For the beginning, see some simple linear regression examples .
6. K-nearest neighbor (k-NN)
N-nearest-neighbor is a data classification algorithm that evaluates the likelihood a data point to be a member of one group. It depends on how near the data point is to that group.
As one of the key non-parametric method used for regression and classification, k-NN can be classified as one of the best data science topics ever.
Determining neighbors, using classification rules, choosing k are a few of the skills a data scientist should have. K-nearest neighbor is also one of the key text mining and anomaly detection algorithms .
7. Naive Bayes
Naive Bayes is a collection of classification algorithms which are based on the so-called Bayes Theorem .
Widely used in Machine Learning, Naive Bayes has some crucial applications such as spam detection and document classification.
There are different Naive Bayes variations. The most popular of them are the Multinomial Naive Bayes, Bernoulli Naive Bayes, and Binarized Multinomial Naive Bayes.
8. Classification and regression trees (CART)
When it comes to algorithms for predictive modeling machine learning, decision trees algorithms have a vital role.
The decision tree is one of the most popular predictive modeling approaches used in data mining, statistics and machine learning that builds classification or regression models in the shape of a tree (that’s why they are also known as regression and classification trees).
They work for both categorical data and continuous data.
Some terms and topics you should master in this field involve CART decision tree methodology, classification trees, regression trees, interactive dihotomiser, C4.5, C5.5, decision stump, conditional decision tree, M5, and etc.
9. Logistic regression
Logistic regression is one of the oldest data science topics and areas and as the linear regression, it studies the relationship between dependable and independent variable.
However, we use logistic regression analysis where the dependent variable is dichotomous (binary).
You will face terms such as sigmoid function, S-shaped curve, multiple logistic regression with categorical explanatory variables, multiple binary logistic regression with a combination of categorical and continuous predictors and etc.
10. Neural Networks
Neural Networks act as a total hit in the machine learning nowadays. Neural networks (also known as artificial neural networks) are systems of hardware and/or software that mimic the human brain neurons operation.
The above were some of the basic data science topics. Here is a list of more interesting and advanced topics:
11. Discriminant analysis
12. Association rules
13. Cluster analysis
14. Time series
15. Regression-based forecasting
16. Smoothing methods
17. Time stamps and financial modeling
18. Fraud detection
19. Data engineering – Hadoop, MapReduce, Pregel.
20. GIS and spatial data
For continuous learning, explore online data science courses for mastering these topics.
What are your favorite data science topics? Share your thoughts in the comment field above.
About The Author

Silvia Valcheva
Silvia Valcheva is a digital marketer with over a decade of experience creating content for the tech industry. She has a strong passion for writing about emerging software and technologies such as big data, AI (Artificial Intelligence), IoT (Internet of Things), process automation, etc.
Leave a Reply Cancel Reply
This site uses Akismet to reduce spam. Learn how your comment data is processed .
- Subscription
21 Data Science Projects for Beginners (with Source Code)
Looking to start a career in data science but lack experience? This is a common challenge. Many aspiring data scientists find themselves in a tricky situation: employers want experienced candidates, but how do you gain experience without a job? The answer lies in building a strong portfolio of data science projects .
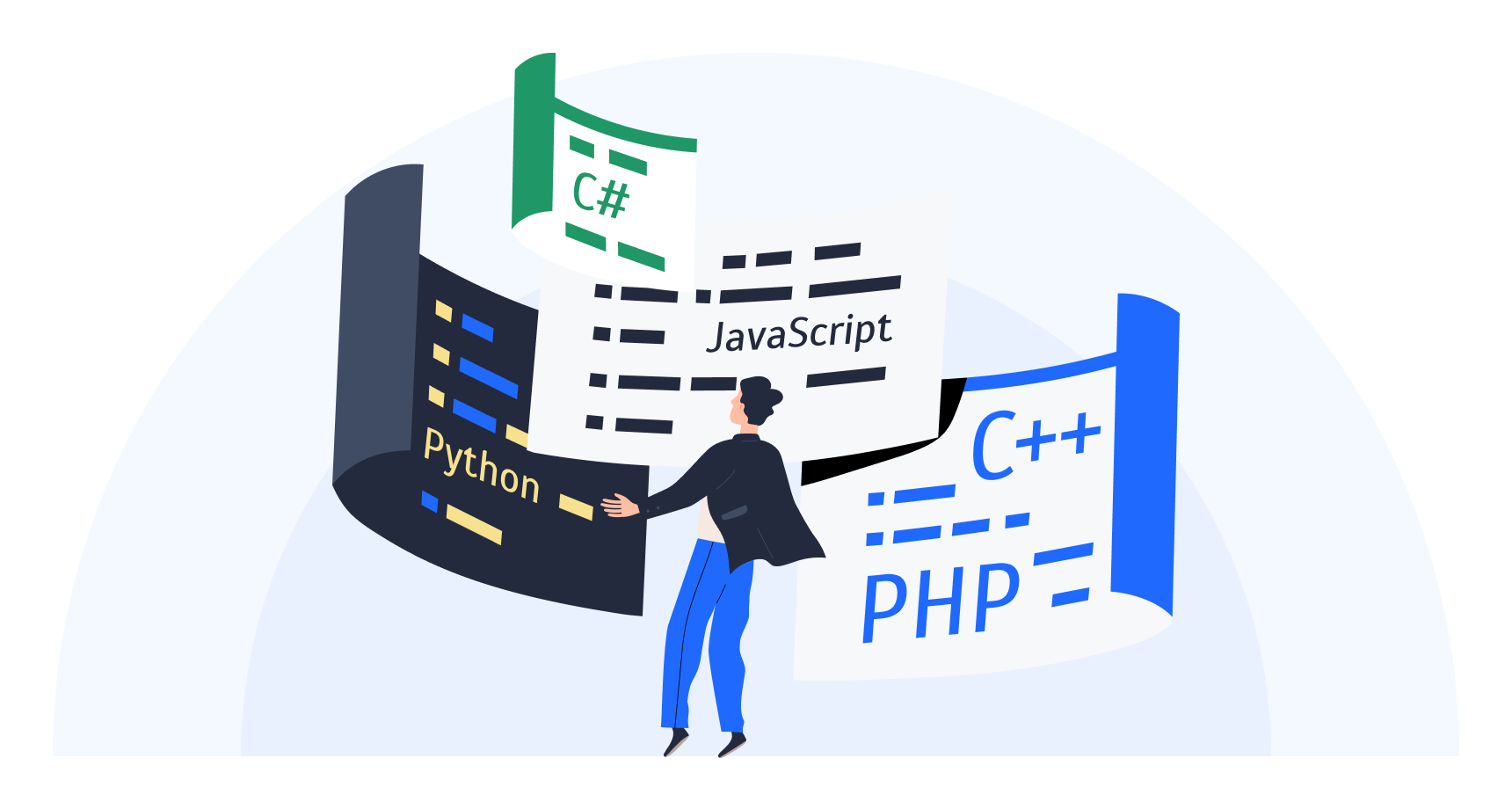
A well-crafted portfolio of data science projects is more than just a collection of your work. It's a powerful tool that:
- Shows your ability to solve real-world problems
- Highlights your technical skills
- Proves you're ready for professional challenges
- Makes up for a lack of formal work experience
By creating various data science projects for your portfolio, you can effectively demonstrate your capabilities to potential employers, even if you don't have any experience . This approach helps bridge the gap between your theoretical knowledge and practical skills.
Why start a data science project?
Simply put, starting a data science project will improve your data science skills and help you start building a solid portfolio of projects. Let's explore how to begin and what tools you'll need.
Steps to start a data science project
- Define your problem : Clearly state what you want to solve .
- Gather and clean your data : Prepare it for analysis.
- Explore your data : Look for patterns and relationships .
Hands-on experience is key to becoming a data scientist. Projects help you:
- Apply what you've learned
- Develop practical skills
- Show your abilities to potential employers
Common tools for building data science projects
To get started, you might want to install:
- Programming languages : Python or R
- Data analysis tools : Jupyter Notebook and SQL
- Version control : Git
- Machine learning and deep learning libraries : Scikit-learn and TensorFlow , respectively, for more advanced data science projects
These tools will help you manage data, analyze it, and keep track of your work.
Overcoming common challenges
New data scientists often struggle with complex datasets and unfamiliar tools. Here's how to address these issues:
- Start small : Begin with simple projects and gradually increase complexity.
- Use online resources : Dataquest offers free guided projects to help you learn.
- Join a community : Online forums and local meetups can provide support and feedback.
Setting up your data science project environment
To make your setup easier :
- Use Anaconda : It includes many necessary tools, like Jupyter Notebook.
- Implement version control: Use Git to track your progress .
Skills to focus on
According to KDnuggets , employers highly value proficiency in SQL, database management, and Python libraries like TensorFlow and Scikit-learn. Including projects that showcase these skills can significantly boost your appeal in the job market.
In this post, we'll explore 21 diverse data science project ideas. These projects are designed to help you build a compelling portfolio, whether you're just starting out or looking to enhance your existing skills. By working on these projects, you'll be better prepared for a successful career in data science.
Choosing the right data science projects for your portfolio
Building a strong data science portfolio is key to showcasing your skills to potential employers. But how do you choose the right projects? Let's break it down.
Balancing personal interests, skills, and market demands
When selecting projects, aim for a mix that :
- Aligns with your interests
- Matches your current skill level
- Highlights in-demand skills
- Projects you're passionate about keep you motivated.
- Those that challenge you help you grow.
- Focusing on sought-after skills makes your portfolio relevant to employers.
For example, if machine learning and data visualization are hot in the job market, including projects that showcase these skills can give you an edge.
A step-by-step approach to selecting data science projects
- Assess your skills : What are you good at? Where can you improve?
- Identify gaps : Look for in-demand skills that interest you but aren't yet in your portfolio.
- Plan your projects : Choose 3-5 substantial projects that cover different stages of the data science workflow. Include everything from data cleaning to applying machine learning models .
- Get feedback and iterate : Regularly ask for input on your projects and make improvements.
Common data science project pitfalls and how to avoid them
Many beginners underestimate the importance of early project stages like data cleaning and exploration. To overcome data science project challeges :
- Spend enough time on data preparation
- Focus on exploratory data analysis to uncover patterns before jumping into modeling
By following these strategies, you'll build a portfolio of data science projects that shows off your range of skills. Each one is an opportunity to sharpen your abilities and demonstrate your potential as a data scientist.
Real learner, real results
Take it from Aleksey Korshuk , who leveraged Dataquest's project-based curriculum to gain practical data science skills and build an impressive portfolio of projects:
The general knowledge that Dataquest provides is easily implemented into your projects and used in practice.
Through hands-on projects, Aleksey gained real-world experience solving complex problems and applying his knowledge effectively. He encourages other learners to stay persistent and make time for consistent learning:
I suggest that everyone set a goal, find friends in communities who share your interests, and work together on cool projects. Don't give up halfway!
Aleksey's journey showcases the power of a project-based approach for anyone looking to build their data skills. By building practical projects and collaborating with others, you can develop in-demand skills and accomplish your goals, just like Aleksey did with Dataquest.
21 Data Science Project Ideas
Excited to dive into a data science project? We've put together a collection of 21 varied projects that are perfect for beginners and apply to real-world scenarios. From analyzing app market data to exploring financial trends, these projects are organized by difficulty level, making it easy for you to choose a project that matches your current skill level while also offering more challenging options to tackle as you progress.
Beginner Data Science Projects
- Profitable App Profiles for the App Store and Google Play Markets
- Exploring Hacker News Posts
- Exploring eBay Car Sales Data
- Finding Heavy Traffic Indicators on I-94
- Storytelling Data Visualization on Exchange Rates
- Clean and Analyze Employee Exit Surveys
- Star Wars Survey
Intermediate Data Science Projects
- Exploring Financial Data using Nasdaq Data Link API
- Popular Data Science Questions
- Investigating Fandango Movie Ratings
- Finding the Best Markets to Advertise In
- Mobile App for Lottery Addiction
- Building a Spam Filter with Naive Bayes
- Winning Jeopardy
Advanced Data Science Projects
- Predicting Heart Disease
- Credit Card Customer Segmentation
- Predicting Insurance Costs
- Classifying Heart Disease
- Predicting Employee Productivity Using Tree Models
- Optimizing Model Prediction
- Predicting Listing Gains in the Indian IPO Market Using TensorFlow
In the following sections, you'll find detailed instructions for each project. We'll cover the tools you'll use and the skills you'll develop. This structured approach will guide you through key data science techniques across various applications.
1. Profitable App Profiles for the App Store and Google Play Markets
Difficulty Level: Beginner
In this beginner-level data science project, you'll step into the role of a data scientist for a company that builds ad-supported mobile apps. Using Python and Jupyter Notebook, you'll analyze real datasets from the Apple App Store and Google Play Store to identify app profiles that attract the most users and generate the highest revenue. By applying data cleaning techniques, conducting exploratory data analysis, and making data-driven recommendations, you'll develop practical skills essential for entry-level data science positions.
Tools and Technologies
- Jupyter Notebook
Prerequisites
To successfully complete this project, you should be comfortable with Python fundamentals such as:
- Variables, data types, lists, and dictionaries
- Writing functions with arguments, return statements, and control flow
- Using conditional logic and loops for data manipulation
- Working with Jupyter Notebook to write, run, and document code
Step-by-Step Instructions
- Open and explore the App Store and Google Play datasets
- Clean the datasets by removing non-English apps and duplicate entries
- Analyze app genres and categories using frequency tables
- Identify app profiles that attract the most users
- Develop data-driven recommendations for the company's next app development project
Expected Outcomes
Upon completing this project, you'll have gained valuable skills and experience, including:
- Cleaning and preparing real-world datasets for analysis using Python
- Conducting exploratory data analysis to identify trends in app markets
- Applying frequency analysis to derive insights from data
- Translating data findings into actionable business recommendations
Relevant Links and Resources
- Example Solution Code
2. Exploring Hacker News Posts
In this beginner-level data science project, you'll analyze a dataset of submissions to Hacker News, a popular technology-focused news aggregator. Using Python and Jupyter Notebook, you'll explore patterns in post creation times, compare engagement levels between different post types, and identify the best times to post for maximum comments. This project will strengthen your skills in data manipulation, analysis, and interpretation, providing valuable experience for aspiring data scientists.
To successfully complete this project, you should be comfortable with Python concepts for data science such as:
- String manipulation and basic text processing
- Working with dates and times using the datetime module
- Using loops to iterate through data collections
- Basic data analysis techniques like calculating averages and sorting
- Creating and manipulating lists and dictionaries
- Load and explore the Hacker News dataset, focusing on post titles and creation times
- Separate and analyze 'Ask HN' and 'Show HN' posts
- Calculate and compare the average number of comments for different post types
- Determine the relationship between post creation time and comment activity
- Identify the optimal times to post for maximum engagement
- Manipulating strings and datetime objects in Python for data analysis
- Calculating and interpreting averages to compare dataset subgroups
- Identifying time-based patterns in user engagement data
- Translating data insights into practical posting strategies
- Original Hacker News Posts dataset on Kaggle
3. Exploring eBay Car Sales Data
In this beginner-level data science project, you'll analyze a dataset of used car listings from eBay Kleinanzeigen, a classifieds section of the German eBay website. Using Python and pandas, you'll clean the data, explore the included listings, and uncover insights about used car prices, popular brands, and the relationships between various car attributes. This project will strengthen your data cleaning and exploratory data analysis skills, providing valuable experience in working with real-world, messy datasets.
To successfully complete this project, you should be comfortable with pandas fundamentals and have experience with:
- Loading and inspecting data using pandas
- Cleaning column names and handling missing data
- Using pandas to filter, sort, and aggregate data
- Creating basic visualizations with pandas
- Handling data type conversions in pandas
- Load the dataset and perform initial data exploration
- Clean column names and convert data types as necessary
- Analyze the distribution of car prices and registration years
- Explore relationships between brand, price, and vehicle type
- Investigate the impact of car age on pricing
- Cleaning and preparing a real-world dataset using pandas
- Performing exploratory data analysis on a large dataset
- Creating data visualizations to communicate findings effectively
- Deriving actionable insights from used car market data
- Original eBay Kleinanzeigen Dataset on Kaggle
4. Finding Heavy Traffic Indicators on I-94
In this beginner-level data science project, you'll analyze a dataset of westbound traffic on the I-94 Interstate highway between Minneapolis and St. Paul, Minnesota. Using Python and popular data visualization libraries, you'll explore traffic volume patterns to identify indicators of heavy traffic. You'll investigate how factors such as time of day, day of the week, weather conditions, and holidays impact traffic volume. This project will enhance your skills in exploratory data analysis and data visualization, providing valuable experience in deriving actionable insights from real-world time series data.
To successfully complete this project, you should be comfortable with data visualization in Python techniques and have experience with:
- Data manipulation and analysis using pandas
- Creating various plot types (line, bar, scatter) with Matplotlib
- Enhancing visualizations using seaborn
- Interpreting time series data and identifying patterns
- Basic statistical concepts like correlation and distribution
- Load and perform initial exploration of the I-94 traffic dataset
- Visualize traffic volume patterns over time using line plots
- Analyze traffic volume distribution by day of the week and time of day
- Investigate the relationship between weather conditions and traffic volume
- Identify and visualize other factors correlated with heavy traffic
- Creating and interpreting complex data visualizations using Matplotlib and seaborn
- Analyzing time series data to uncover temporal patterns and trends
- Using visual exploration techniques to identify correlations in multivariate data
- Communicating data insights effectively through clear, informative plots
- Original Metro Interstate Traffic Volume Data Set
5. Storytelling Data Visualization on Exchange Rates
In this beginner-level data science project, you'll create a storytelling data visualization about Euro exchange rates against the US Dollar. Using Python and Matplotlib, you'll analyze historical exchange rate data from 1999 to 2021, identifying key trends and events that have shaped the Euro-Dollar relationship. You'll apply data visualization principles to clean data, develop a narrative around exchange rate fluctuations, and create an engaging and informative visual story. This project will strengthen your ability to communicate complex financial data insights effectively through visual storytelling.
To successfully complete this project, you should be familiar with storytelling through data visualization techniques and have experience with:
- Creating and customizing plots with Matplotlib
- Applying design principles to enhance data visualizations
- Working with time series data in Python
- Basic understanding of exchange rates and economic indicators
- Load and explore the Euro-Dollar exchange rate dataset
- Clean the data and calculate rolling averages to smooth out fluctuations
- Identify significant trends and events in the exchange rate history
- Develop a narrative that explains key patterns in the data
- Create a polished line plot that tells your exchange rate story
- Crafting a compelling narrative around complex financial data
- Designing clear, informative visualizations that support your story
- Using Matplotlib to create publication-quality line plots with annotations
- Applying color theory and typography to enhance visual communication
- ECB Euro reference exchange rate: US dollar
6. Clean and Analyze Employee Exit Surveys
In this beginner-level data science project, you'll analyze employee exit surveys from the Department of Education, Training and Employment (DETE) and the Technical and Further Education (TAFE) institute in Queensland, Australia. Using Python and pandas, you'll clean messy data, combine datasets, and uncover insights into resignation patterns. You'll investigate factors such as years of service, age groups, and job dissatisfaction to understand why employees leave. This project offers hands-on experience in data cleaning and exploratory analysis, essential skills for aspiring data analysts.
To successfully complete this project, you should be familiar with data cleaning techniques in Python and have experience with:
- Basic pandas operations for data manipulation
- Handling missing data and data type conversions
- Merging and concatenating DataFrames
- Using string methods in pandas for text data cleaning
- Basic data analysis and aggregation techniques
- Load and explore the DETE and TAFE exit survey datasets
- Clean column names and handle missing values in both datasets
- Standardize and combine the "resignation reasons" columns
- Merge the DETE and TAFE datasets for unified analysis
- Analyze resignation reasons and their correlation with employee characteristics
- Applying data cleaning techniques to prepare messy, real-world datasets
- Combining data from multiple sources using pandas merge and concatenate functions
- Creating new categories from existing data to facilitate analysis
- Conducting exploratory data analysis to uncover trends in employee resignations
- DETE Exit Survey Dataset
7. Star Wars Survey
In this beginner-level data science project, you'll analyze survey data about the Star Wars film franchise. Using Python and pandas, you'll clean and explore data collected by FiveThirtyEight to uncover insights about fans' favorite characters, film rankings, and how opinions vary across different demographic groups. You'll practice essential data cleaning techniques like handling missing values and converting data types, while also conducting basic statistical analysis to reveal trends in Star Wars fandom.
To successfully complete this project, you should be familiar with combining, analyzing, and visualizing data while having experience with:
- Converting data types in pandas DataFrames
- Filtering and sorting data
- Basic data aggregation and analysis techniques
- Load the Star Wars survey data and explore its structure
- Analyze the rankings of Star Wars films among respondents
- Explore viewership and character popularity across different demographics
- Investigate the relationship between fan characteristics and their opinions
- Applying data cleaning techniques to prepare survey data for analysis
- Using pandas to explore and manipulate structured data
- Performing basic statistical analysis on categorical and numerical data
- Interpreting survey results to draw meaningful conclusions about fan preferences
- Original Star Wars Survey Data on GitHub
8. Exploring Financial Data using Nasdaq Data Link API
Difficulty Level: Intermediate
In this beginner-friendly data science project, you'll analyze real-world economic data to uncover market trends. Using Python, you'll interact with the Nasdaq Data Link API to retrieve financial datasets, including stock prices and economic indicators. You'll apply data wrangling techniques to clean and structure the data, then use pandas and Matplotlib to analyze and visualize trends in stock performance and economic metrics. This project provides hands-on experience in working with financial APIs and analyzing market data, skills that are highly valuable in data-driven finance roles.
- requests (for API calls)
To successfully complete this project, you should be familiar with working with APIs and web scraping in Python , and have experience with:
- Making HTTP requests and handling responses using the requests library
- Parsing JSON data in Python
- Data manipulation and analysis using pandas DataFrames
- Creating line plots and other basic visualizations with Matplotlib
- Basic understanding of financial terms and concepts
- Set up authentication for the Nasdaq Data Link API
- Retrieve historical stock price data for a chosen company
- Clean and structure the API response data using pandas
- Analyze stock price trends and calculate key statistics
- Fetch and analyze additional economic indicators
- Create visualizations to illustrate relationships between different financial metrics
- Interacting with financial APIs to retrieve real-time and historical market data
- Cleaning and structuring JSON data for analysis using pandas
- Calculating financial metrics such as returns and moving averages
- Creating informative visualizations of stock performance and economic trends
- Nasdaq Data Link API Documentation
9. Popular Data Science Questions
In this beginner-friendly data science project, you'll analyze data from Data Science Stack Exchange to uncover trends in the data science field. You'll identify the most frequently asked questions, popular technologies, and emerging topics. Using SQL and Python, you'll query a database to extract post data, then use pandas to clean and analyze it. You'll visualize trends over time and across different subject areas, gaining insights into the evolving landscape of data science. This project offers hands-on experience in combining SQL, data analysis, and visualization skills to derive actionable insights from a real-world dataset.
To successfully complete this project, you should be familiar with querying databases with SQL and Python and have experience with:
- Writing SQL queries to extract data from relational databases
- Data cleaning and manipulation using pandas DataFrames
- Basic data analysis techniques like grouping and aggregation
- Creating line plots and bar charts with Matplotlib
- Interpreting trends and patterns in data
- Connect to the Data Science Stack Exchange database and explore its structure
- Write SQL queries to extract data on questions, tags, and view counts
- Use pandas to clean the extracted data and prepare it for analysis
- Analyze the distribution of questions across different tags and topics
- Investigate trends in question popularity and topic relevance over time
- Visualize key findings using Matplotlib to illustrate data science trends
- Extracting specific data from a relational database using SQL queries
- Cleaning and preprocessing text data for analysis using pandas
- Identifying trends and patterns in data science topics over time
- Creating meaningful visualizations to communicate insights about the data science field
- Data Science Stack Exchange Data Explorer
10. Investigating Fandango Movie Ratings
In this beginner-friendly data science project, you'll investigate potential bias in Fandango's movie rating system. Following up on a 2015 analysis that found evidence of inflated ratings, you'll compare 2015 and 2016 movie ratings data to determine if Fandango's system has changed. Using Python, you'll perform statistical analysis to compare rating distributions, calculate summary statistics, and visualize changes in rating patterns. This project will strengthen your skills in data manipulation, statistical analysis, and data visualization while addressing a real-world question of rating integrity.
To successfully complete this project, you should be familiar with fundamental statistics concepts and have experience with:
- Data manipulation using pandas (e.g., loading data, filtering, sorting)
- Calculating and interpreting summary statistics in Python
- Creating and customizing plots with matplotlib
- Comparing distributions using statistical methods
- Interpreting results in the context of the research question
- Load the 2015 and 2016 Fandango movie ratings datasets using pandas
- Clean the data and isolate the samples needed for analysis
- Compare the distribution shapes of 2015 and 2016 ratings using kernel density plots
- Calculate and compare summary statistics for both years
- Analyze the frequency of each rating class (e.g., 4.5 stars, 5 stars) for both years
- Determine if there's evidence of a change in Fandango's rating system
- Conducting a comparative analysis of rating distributions using Python
- Applying statistical techniques to investigate potential bias in ratings
- Creating informative visualizations to illustrate changes in rating patterns
- Drawing and communicating data-driven conclusions about rating system integrity
- Original FiveThirtyEight Article on Fandango Ratings
11. Finding the Best Markets to Advertise In
In this beginner-friendly data science project, you'll analyze survey data from freeCodeCamp to determine the best markets for an e-learning company to advertise its programming courses. Using Python and pandas, you'll explore the demographics of new coders, their locations, and their willingness to pay for courses. You'll clean the data, handle outliers, and use frequency analysis to identify countries with the most potential customers. By the end, you'll provide data-driven recommendations on where the company should focus its advertising efforts to maximize its return on investment.
To successfully complete this project, you should have a solid grasp on how to summarize distributions using measures of central tendency, interpret variance using z-scores , and have experience with:
- Filtering and sorting DataFrames
- Handling missing data and outliers
- Calculating summary statistics (mean, median, mode)
- Creating and manipulating new columns based on existing data
- Load the freeCodeCamp 2017 New Coder Survey data
- Identify and handle missing values in the dataset
- Analyze the distribution of participants across different countries
- Calculate the average amount students are willing to pay for courses by country
- Identify and handle outliers in the monthly spending data
- Determine the top countries based on number of potential customers and their spending power
- Cleaning and preprocessing survey data for analysis using pandas
- Applying frequency analysis to identify key markets
- Handling outliers to ensure accurate calculations of spending potential
- Combining multiple factors to make data-driven business recommendations
- freeCodeCamp 2017 New Coder Survey Results
12. Mobile App for Lottery Addiction
In this beginner-friendly data science project, you'll develop the core logic for a mobile app aimed at helping lottery addicts better understand their chances of winning. Using Python, you'll create functions to calculate probabilities for the 6/49 lottery game, including the chances of winning the big prize, any prize, and the expected return on buying a ticket. You'll also compare lottery odds to real-life situations to provide context. This project will strengthen your skills in probability theory, Python programming, and applying mathematical concepts to real-world problems.
To successfully complete this project, you should be familiar with probability fundamentals and have experience with:
- Writing functions in Python with multiple parameters
- Implementing combinatorics calculations (factorials, combinations)
- Working with control structures (if statements, for loops)
- Performing mathematical operations in Python
- Basic set theory and probability concepts
- Implement the factorial and combinations functions for probability calculations
- Create a function to calculate the probability of winning the big prize in a 6/49 lottery
- Develop a function to calculate the probability of winning any prize
- Design a function to compare lottery odds with real-life event probabilities
- Implement a function to calculate the expected return on buying a lottery ticket
- Implementing complex probability calculations using Python functions
- Translating mathematical concepts into practical programming solutions
- Creating user-friendly outputs to effectively communicate probability concepts
- Applying programming skills to address a real-world social issue
13. Building a Spam Filter with Naive Bayes
In this beginner-friendly data science project, you'll build a spam filter using the multinomial Naive Bayes algorithm. Working with the SMS Spam Collection dataset, you'll implement the algorithm from scratch to classify messages as spam or ham (non-spam). You'll calculate word frequencies, prior probabilities, and conditional probabilities to make predictions. This project will deepen your understanding of probabilistic machine learning algorithms, text classification, and the practical application of Bayesian methods in natural language processing.
To successfully complete this project, you should be familiar with conditional probability and have experience with:
- Python programming, including working with dictionaries and lists
- Understand probability concepts like conditional probability and Bayes' theorem
- Text processing techniques (tokenization, lowercasing)
- Pandas for data manipulation
- Understanding of the Naive Bayes algorithm and its assumptions
- Load and explore the SMS Spam Collection dataset
- Preprocess the text data by tokenizing and cleaning the messages
- Calculate the prior probabilities for spam and ham messages
- Compute word frequencies and conditional probabilities
- Implement the Naive Bayes algorithm to classify messages
- Test the model and evaluate its accuracy on unseen data
- Implementing the multinomial Naive Bayes algorithm from scratch
- Applying Bayesian probability calculations in a real-world context
- Preprocessing text data for machine learning applications
- Evaluating a text classification model's performance
- SMS Spam Collection Dataset
14. Winning Jeopardy
In this beginner-friendly data science project, you'll analyze a dataset of Jeopardy questions to uncover patterns that could give you an edge in the game. Using Python and pandas, you'll explore over 200,000 Jeopardy questions and answers, focusing on identifying terms that appear more often in high-value questions. You'll apply text processing techniques, use the chi-squared test to validate your findings, and develop strategies for maximizing your chances of winning. This project will strengthen your data manipulation skills and introduce you to practical applications of natural language processing and statistical testing.
To successfully complete this project, you should be familiar with intermediate statistics concepts like significance and hypothesis testing with experience in:
- String operations and basic regular expressions in Python
- Implementing the chi-squared test for statistical analysis
- Working with CSV files and handling data type conversions
- Basic natural language processing concepts (e.g., tokenization)
- Load the Jeopardy dataset and perform initial data exploration
- Clean and preprocess the data, including normalizing text and converting dollar values
- Implement a function to find the number of times a term appears in questions
- Create a function to compare the frequency of terms in low-value vs. high-value questions
- Apply the chi-squared test to determine if certain terms are statistically significant
- Analyze the results to develop strategies for Jeopardy success
- Processing and analyzing large text datasets using pandas
- Applying statistical tests to validate hypotheses in data analysis
- Implementing custom functions for text analysis and frequency comparisons
- Deriving actionable insights from complex datasets to inform game strategy
- J! Archive - Fan-created archive of Jeopardy! games and players
15. Predicting Heart Disease
Difficulty Level: Advanced
In this challenging but guided data science project, you'll build a K-Nearest Neighbors (KNN) classifier to predict the risk of heart disease. Using a dataset from the UCI Machine Learning Repository, you'll work with patient features such as age, sex, chest pain type, and cholesterol levels to classify patients as having a high or low risk of heart disease. You'll explore the impact of different features on the prediction, optimize the model's performance, and interpret the results to identify key risk factors. This project will strengthen your skills in data preprocessing, exploratory data analysis, and implementing classification algorithms for healthcare applications.
- scikit-learn
To successfully complete this project, you should be familiar with supervised machine learning in Python and have experience with:
- Implementing machine learning workflows with scikit-learn
- Understanding and interpreting classification metrics (accuracy, precision, recall)
- Feature scaling and preprocessing techniques
- Basic data visualization with Matplotlib
- Load and explore the heart disease dataset from the UCI Machine Learning Repository
- Preprocess the data, including handling missing values and scaling features
- Split the data into training and testing sets
- Implement a KNN classifier and evaluate its initial performance
- Optimize the model by tuning the number of neighbors (k)
- Analyze feature importance and their impact on heart disease prediction
- Interpret the results and summarize key findings for healthcare professionals
- Implementing and optimizing a KNN classifier for medical diagnosis
- Evaluating model performance using various metrics in a healthcare context
- Analyzing feature importance in predicting heart disease risk
- Translating machine learning results into actionable healthcare insights
- UCI Machine Learning Repository: Heart Disease Dataset
16. Credit Card Customer Segmentation
In this challenging but guided data science project, you'll perform customer segmentation for a credit card company using unsupervised learning techniques. You'll analyze customer attributes such as credit limit, purchases, cash advances, and payment behaviors to identify distinct groups of credit card users. Using the K-means clustering algorithm, you'll segment customers based on their spending habits and credit usage patterns. This project will strengthen your skills in data preprocessing, exploratory data analysis, and applying machine learning for deriving actionable business insights in the financial sector.
To successfully complete this project, you should be familiar with unsupervised machine learning in Python and have experience with:
- Implementing K-means clustering with scikit-learn
- Feature scaling and dimensionality reduction techniques
- Creating scatter plots and pair plots with Matplotlib and seaborn
- Interpreting clustering results in a business context
- Load and explore the credit card customer dataset
- Perform exploratory data analysis to understand relationships between customer attributes
- Apply principal component analysis (PCA) for dimensionality reduction
- Implement K-means clustering on the transformed data
- Visualize the clusters using scatter plots of the principal components
- Analyze cluster characteristics to develop customer profiles
- Propose targeted strategies for each customer segment
- Applying K-means clustering to segment customers in the financial sector
- Using PCA for dimensionality reduction in high-dimensional datasets
- Interpreting clustering results to derive meaningful customer profiles
- Translating data-driven insights into actionable marketing strategies
- Credit Card Dataset for Clustering on Kaggle
17. Predicting Insurance Costs
In this challenging but guided data science project, you'll predict patient medical insurance costs using linear regression. Working with a dataset containing features such as age, BMI, number of children, smoking status, and region, you'll develop a model to estimate insurance charges. You'll explore the relationships between these factors and insurance costs, handle categorical variables, and interpret the model's coefficients to understand the impact of each feature. This project will strengthen your skills in regression analysis, feature engineering, and deriving actionable insights in the healthcare insurance domain.
To successfully complete this project, you should be familiar with linear regression modeling in Python and have experience with:
- Implementing linear regression models with scikit-learn
- Handling categorical variables (e.g., one-hot encoding)
- Evaluating regression models using metrics like R-squared and RMSE
- Creating scatter plots and correlation heatmaps with seaborn
- Load and explore the insurance cost dataset
- Perform data preprocessing, including handling categorical variables
- Conduct exploratory data analysis to visualize relationships between features and insurance costs
- Create training/testing sets to build and train a linear regression model using scikit-learn
- Make predictions on the test set and evaluate the model's performance
- Visualize the actual vs. predicted values and residuals
- Implementing end-to-end linear regression analysis for cost prediction
- Handling categorical variables in regression models
- Interpreting regression coefficients to derive business insights
- Evaluating model performance and understanding its limitations in healthcare cost prediction
- Medical Cost Personal Datasets on Kaggle
18. Classifying Heart Disease
In this challenging but guided data science project, you'll work with the Cleveland Clinic Foundation heart disease dataset to develop a logistic regression model for predicting heart disease. You'll analyze features such as age, sex, chest pain type, blood pressure, and cholesterol levels to classify patients as having or not having heart disease. Through this project, you'll gain hands-on experience in data preprocessing, model building, and interpretation of results in a medical context, strengthening your skills in classification techniques and feature analysis.
To successfully complete this project, you should be familiar with logistic regression modeling in Python and have experience with:
- Implementing logistic regression models with scikit-learn
- Evaluating classification models using metrics like accuracy, precision, and recall
- Interpreting model coefficients and odds ratios
- Creating confusion matrices and ROC curves with seaborn and Matplotlib
- Load and explore the Cleveland Clinic Foundation heart disease dataset
- Perform data preprocessing, including handling missing values and encoding categorical variables
- Conduct exploratory data analysis to visualize relationships between features and heart disease presence
- Create training/testing sets to build and train a logistic regression model using scikit-learn
- Visualize the ROC curve and calculate the AUC score
- Summarize findings and discuss the model's potential use in medical diagnosis
- Implementing end-to-end logistic regression analysis for medical diagnosis
- Interpreting odds ratios to understand risk factors for heart disease
- Evaluating classification model performance using various metrics
- Communicating the potential and limitations of machine learning in healthcare
19. Predicting Employee Productivity Using Tree Models
In this challenging but guided data science project, you'll analyze employee productivity in a garment factory using tree-based models. You'll work with a dataset containing factors such as team, targeted productivity, style changes, and working hours to predict actual productivity. By implementing both decision trees and random forests, you'll compare their performance and interpret the results to provide actionable insights for improving workforce efficiency. This project will strengthen your skills in tree-based modeling, feature importance analysis, and applying machine learning to solve real-world business problems in manufacturing.
To successfully complete this project, you should be familiar with decision trees and random forest modeling and have experience with:
- Implementing decision trees and random forests with scikit-learn
- Evaluating regression models using metrics like MSE and R-squared
- Interpreting feature importance in tree-based models
- Creating visualizations of tree structures and feature importance with Matplotlib
- Load and explore the employee productivity dataset
- Perform data preprocessing, including handling categorical variables and scaling numerical features
- Create training/testing sets to build and train a decision tree regressor using scikit-learn
- Visualize the decision tree structure and interpret the rules
- Implement a random forest regressor and compare its performance to the decision tree
- Analyze feature importance to identify key factors affecting productivity
- Fine-tune the random forest model using grid search
- Summarize findings and provide recommendations for improving employee productivity
- Implementing and comparing decision trees and random forests for regression tasks
- Interpreting tree structures to understand decision-making processes in productivity prediction
- Analyzing feature importance to identify key drivers of employee productivity
- Applying hyperparameter tuning techniques to optimize model performance
- UCI Machine Learning Repository: Garment Employee Productivity Dataset
20. Optimizing Model Prediction
In this challenging but guided data science project, you'll work on predicting the extent of damage caused by forest fires using the UCI Machine Learning Repository's Forest Fires dataset. You'll analyze features such as temperature, relative humidity, wind speed, and various fire weather indices to estimate the burned area. Using Python and scikit-learn, you'll apply advanced regression techniques, including feature engineering, cross-validation, and regularization, to build and optimize linear regression models. This project will strengthen your skills in model selection, hyperparameter tuning, and interpreting complex model results in an environmental context.
To successfully complete this project, you should be familiar with optimizing machine learning models and have experience with:
- Implementing and evaluating linear regression models using scikit-learn
- Applying cross-validation techniques to assess model performance
- Understanding and implementing regularization methods (Ridge, Lasso)
- Performing hyperparameter tuning using grid search
- Interpreting model coefficients and performance metrics
- Load and explore the Forest Fires dataset, understanding the features and target variable
- Preprocess the data, handling any missing values and encoding categorical variables
- Perform feature engineering, creating interaction terms and polynomial features
- Implement a baseline linear regression model and evaluate its performance
- Apply k-fold cross-validation to get a more robust estimate of model performance
- Implement Ridge and Lasso regression models to address overfitting
- Use grid search with cross-validation to optimize regularization hyperparameters
- Compare the performance of different models using appropriate metrics (e.g., RMSE, R-squared)
- Interpret the final model, identifying the most important features for predicting fire damage
- Visualize the results and discuss the model's limitations and potential improvements
- Implementing advanced regression techniques to optimize model performance
- Applying cross-validation and regularization to prevent overfitting
- Conducting hyperparameter tuning to find the best model configuration
- Interpreting complex model results in the context of environmental science
- UCI Machine Learning Repository: Forest Fires Dataset
21. Predicting Listing Gains in the Indian IPO Market Using TensorFlow
In this challenging but guided data science project, you'll develop a deep learning model using TensorFlow to predict listing gains in the Indian Initial Public Offering (IPO) market. You'll analyze historical IPO data, including features such as issue price, issue size, subscription rates, and market conditions, to forecast the percentage increase in share price on the day of listing. By implementing a neural network classifier, you'll categorize IPOs into different ranges of listing gains. This project will strengthen your skills in deep learning, financial data analysis, and using TensorFlow for real-world predictive modeling tasks in the finance sector.
To successfully complete this project, you should be familiar with deep learning in TensorFlow and have experience with:
- Building and training neural networks using TensorFlow and Keras
- Preprocessing financial data for machine learning tasks
- Implementing classification models and interpreting their results
- Evaluating model performance using metrics like accuracy and confusion matrices
- Basic understanding of IPOs and stock market dynamics
- Load and explore the Indian IPO dataset using pandas
- Preprocess the data, including handling missing values and encoding categorical variables
- Engineer features relevant to IPO performance prediction
- Split the data into training/testing sets then design a neural network architecture using Keras
- Compile and train the model on the training data
- Evaluate the model's performance on the test set
- Fine-tune the model by adjusting hyperparameters and network architecture
- Analyze feature importance using the trained model
- Visualize the results and interpret the model's predictions in the context of IPO investing
- Implementing deep learning models for financial market prediction using TensorFlow
- Preprocessing and engineering features for IPO performance analysis
- Evaluating and interpreting classification results in the context of IPO investments
- Applying deep learning techniques to solve real-world financial forecasting problems
- Securities and Exchange Board of India (SEBI) IPO Statistics
How to Prepare for a Data Science Job
Landing a data science job requires strategic preparation. Here's what you need to know to stand out in this competitive field:
- Research job postings to understand employer expectations
- Develop relevant skills through structured learning
- Build a portfolio of hands-on projects
- Prepare for interviews and optimize your resume
- Commit to continuous learning
Research Job Postings
Start by understanding what employers are looking for. Check out data science job listings on these platforms:
Steps to Get Job-Ready
Focus on these key areas:
- Skill Development: Enhance your programming, data analysis, and machine learning skills. Consider a structured program like Dataquest's Data Scientist in Python path .
- Hands-On Projects: Apply your skills to real projects. This builds your portfolio of data science projects and demonstrates your abilities to potential employers.
- Put Your Portfolio Online: Showcase your projects online. GitHub is an excellent platform for hosting and sharing your work.
Pick Your Top 3 Data Science Projects
Your projects are concrete evidence of your skills. In applications and interviews, highlight your top 3 data science projects that demonstrate:
- Critical thinking
- Technical proficiency
- Problem-solving abilities
We have a ton of great tips on how to create a project portfolio for data science job applications .
Resume and Interview Preparation
Your resume should clearly outline your project experiences and skills . When getting ready for data science interviews , be prepared to discuss your projects in great detail. Practice explaining your work concisely and clearly.
Job Preparation Advice
Preparing for a data science job can be daunting. If you're feeling overwhelmed:
- Remember that everyone starts somewhere
- Connect with mentors for guidance
- Join the Dataquest community for support and feedback on your data science projects
Continuous Learning
Data science is an evolving field. To stay relevant:
- Keep up with industry trends
- Stay curious and open to new technologies
- Look for ways to apply your skills to real-world problems
Preparing for a data science job involves understanding employer expectations, building relevant skills, creating a strong portfolio, refining your resume, preparing for interviews, addressing challenges, and committing to ongoing learning. With dedication and the right approach, you can position yourself for success in this dynamic field.
Data science projects are key to developing your skills and advancing your data science career. Here's why they matter:
- They provide hands-on experience with real-world problems
- They help you build a portfolio to showcase your abilities
- They boost your confidence in handling complex data challenges
In this post, we've explored 21 beginner-friendly data science project ideas ranging from easier to harder. These projects go beyond just technical skills. They're designed to give you practical experience in solving real-world data problems – a crucial asset for any data science professional.
We encourage you to start with any of these beginner data science projects that interests you. Each one is structured to help you apply your skills to realistic scenarios, preparing you for professional data challenges. While some of these projects use SQL, you'll want to check out our post on 10 Exciting SQL Project Ideas for Beginners for dedicated SQL project ideas to add to your data science portfolio of projects.
Hands-on projects are valuable whether you're new to the field or looking to advance your career. Start building your project portfolio today by selecting from the diverse range of ideas we've shared. It's an important step towards achieving your data science career goals.
More learning resources
Applying to business analyst jobs, part 1: the application, how to fill in job application forms and when to apply.
Learn data skills 10x faster
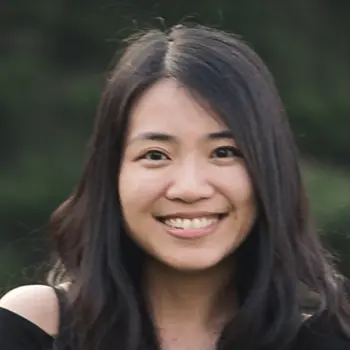
Join 1M+ learners
Enroll for free
- Data Analyst (Python)
- Gen AI (Python)
- Business Analyst (Power BI)
- Business Analyst (Tableau)
- Machine Learning
- Data Analyst (R)
8 Key Data Science Trends For 2024 & 2025
You may also like:
- Important Computer Science Trends
- Top Cryptocurrency Trends
- Important Technology Trends
Here are the 8 fastest-growing data science trends for 2024 and beyond.
We'll also outline how these trends will impact both data scientists’ work and everyday life.
Whether you’re actively involved in the data science community, or just concerned about your data privacy, these are the top trends to know.
1. Generative AI use continues to grow
Generative AI is impacting nearly every industry, from advertising to computer science .
Notably, it looks like generative AI usage is poised for even more growth in 2024 and 2025.
Google found that 64% of developers feel a "sense of urgency" to use generative AI.
And another survey of business leaders found that 85% of them plan to use AI to replace low-level tasks by the end of 2024.
However, there's skepticism within the data science field whether generative AI is living up to the hype.
One survey by MIT found that 52% of tech CEOs were interested in using generative AI in their organizations.
However, only 13% had an actual plan for doing so.
And others in tech are concerned that over-reliance on AI could cause issues in the future.
In fact, Sourcegraph discovered that 76% of developers are excited by the potential that AI brings to the table.
However, there were also significant concerns about AI leading to increased tech debt, sprawl, and code to manage .
2. Explosion in deepfake video and audio
Deepfakes use artificial intelligence to manipulate or create content to represent someone else.
Often this is an image or video of one person modified to someone else’s likeness.
But it can be audio too.
An AI company deepfaked popular podcaster Joe Rogan’s voice so effectively it instantly went viral on social media.
And, thanks to advancements in generative AI, the tech has only improved since.
There’s huge scope for this technology to be used maliciously.
Another voice deep fake was used to scam a UK-based energy company out of €220,000 .
The CEO believed he was on the phone with a colleague and was told to urgently transfer the money to the bank account of a Hungarian supplier.
In fact, the call had been spoofed with deep fake technology to mimic the man’s voice and “melody”.
In fact, there's growing search interest in a practice known as "voice phishing". Which is essentially the "official" term for the practice.
As well as hoaxes and financial fraud, deepfakes can also be weaponized to discredit business figures and politicians.
Governments are starting to protect against this with legislation and social media regulation.
And with technology that can identify deepfake videos.
But the battle with deepfakes has only just begun.
3. More applications created with Python
Python is the go-to programming language for data analysis.
Why is this?
Because Python has a huge number of free data science libraries such as Pandas and machine learning libraries like Scikit-learn .
It can even be used to develop blockchain applications.
Add to this a friendly learning curve for beginners, and you have a recipe for success.
According to Stack Overflow, Python is now the 4th most popular language in general .
(Only behind mainstays like JavaScript, HTML and SQL).
And the popularity growth trend shows it’s has the potential to be #2 or even #1 within the next few years.
4. Increased demand for End-to-end AI solutions
Enterprise AI company Dataiku is now worth $4.6 billion ( according to TechCrunch ) after Google bought a stake in the company.
The AI startup helps enterprise customers clean their large data sets and build machine learning models.
This way, companies like General Electric and Unilever can gain valuable, deep-learning insights from their massive amounts of data.
And automate important data management tasks.
Previously, businesses would have to seek expertise in all the different parts of the process and piece it together themselves.
But Dataiku handles the entire data science cycle from start to finish with a single product.
And because of this, they stand out.
Businesses want end-to-end data science solutions. And startups that provide this will eat the market.
5. Companies hire more data analysts
Demand for data analysts has shot through the roof over the last few years.
And, thanks largely to data coming in from the Internet of Things (IoT) and advances in cloud computing, global data storage is set to grow from 45 zettabytes to 175 zettabytes by 2025 .
So the need for experts to parse and analyze all of this data is set to rise.
Why are so many data analysts required?
After all, there are plenty of data analytics programs out there that can sort through it all.
And "digital transformation" has supposedly replaced many human-led business tasks.
Sure, machines can help analyze data.
But big data is often extremely messy and lacking in proper structure.
Which is why humans are needed to manually tidy training data before it is ingested by machine learning algorithms.
It’s also increasingly common for data people to be involved on the output end too.
AI-produced results are not always reliable or accurate, so machine learning companies often use humans to clean up the final data.
And write up an analysis of what they find in a way that non-tech stakeholders can understand it.
The data science and machine learning methods of the 2020s will be less artificial and automated than initially expected.
Augmented intelligence and human-in-the-loop artificial intelligence will likely become a big trend in data science.
6. Data scientists joining Kaggle
Kaggle has grown quickly to become the world's largest data science community.
And with over 15 million users across 194 countries, it’s not slowing down.
Many budding data scientists now start with Kaggle to begin their machine learning journey.
And post the progress of their machine learning projects in real-time.
Users can even share data sets and enter competitions to solve data science challenges with neural networks.
Or work with other data scientists to build models in Kaggle’s web-based data science workbench.
Academic papers have actually been published based on Kaggle competition findings too.
Successful projects from Kaggle’s hundreds of competitions will likely continue to push boundaries in the field of data science.
7. Increased interest in consumer data protection
Consumer awareness about data privacy rose in the wake of the Cambridge Analytica scandal .
In fact, CIGI-Ipsos found that more than half of all consumers became more interested in data privacy in the year following the revelations.
Platforms like Facebook and Google, which previously harvested and shared user data freely, have since faced legal backlash and public scrutiny.
This broader data privacy trend means that large data sets will soon be walled off and harder to come by.
Businesses and data scientists will need to navigate legislation such as the California Consumer Privacy Act which came into effect at the start of 2020.
And this could become a bane for data science when it comes to the future acquisition and use of consumer data.
8. AI devs combating adversarial machine learning
Adversarial machine learning is where an attacker inputs data into a machine learning model with the aim of causing mistakes.
Essentially, it is an optical illusion designed for a machine.
Anti-surveillance clothing takes this approach to the masses.
They’re specifically designed to confuse face detection algorithms with bold shapes and patterns.
According to a Northeastern University study , this clothing can help prevent individuals' automated tracking via surveillance cameras.
Data scientists will need to defend against adversarial inputs like this. And provide trick examples for models to train on so as not to be fooled.
Adversarial training measures for models like this will become essential in the next decade.
Wrapping Up
Those are the 7 biggest data science trends over the next 3-4 years.
Data science, like any science, is changing by the day. From data governance to deepfake technology, the data science industry is set for some major shakeups.
Hopefully keeping tabs on these trends will help you stay one step ahead.
Find Thousands of Trending Topics With Our Platform
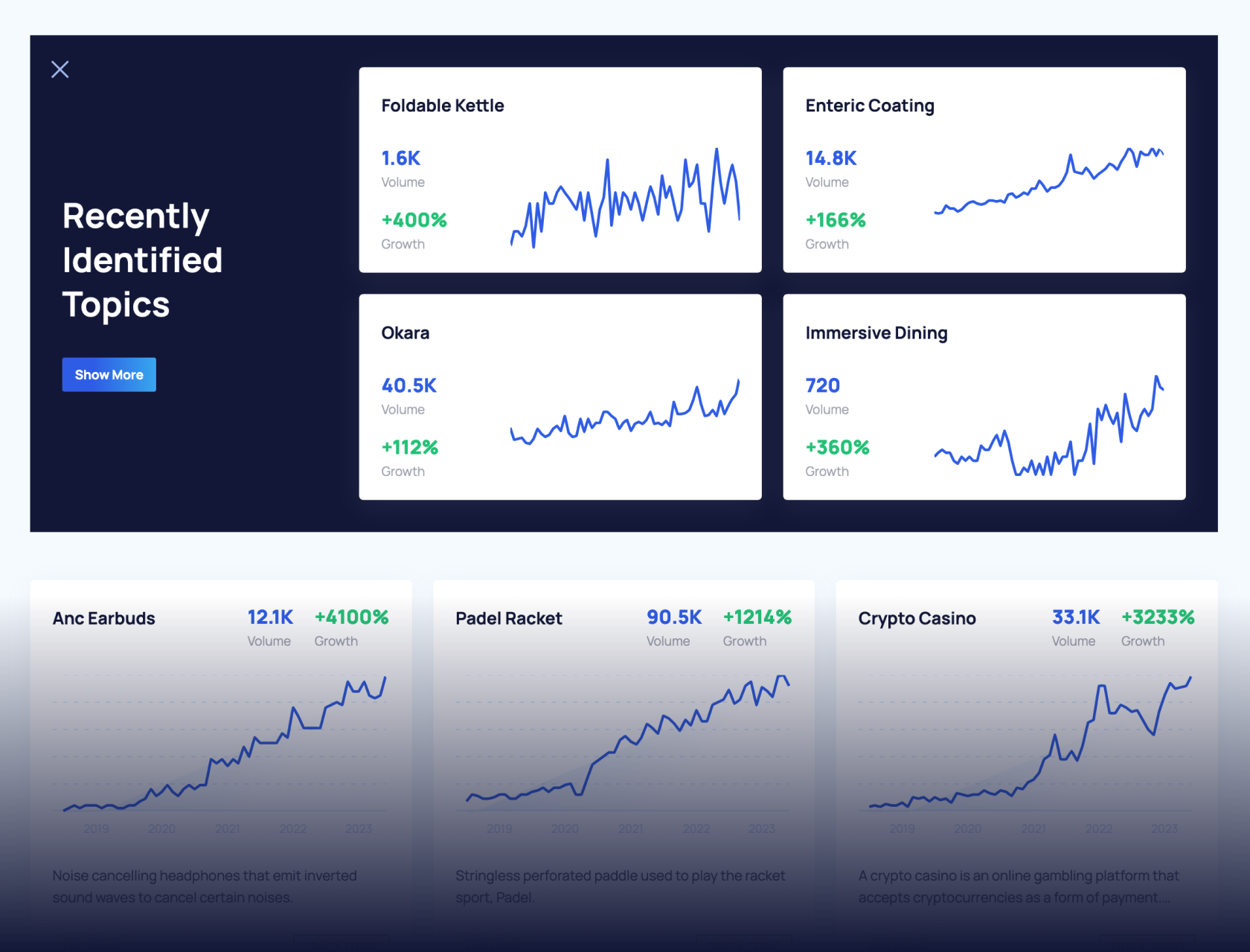
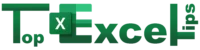
31+ Best Data Science Project Ideas For Beginners To Advance
August 31, 2024
Emmy Williamson
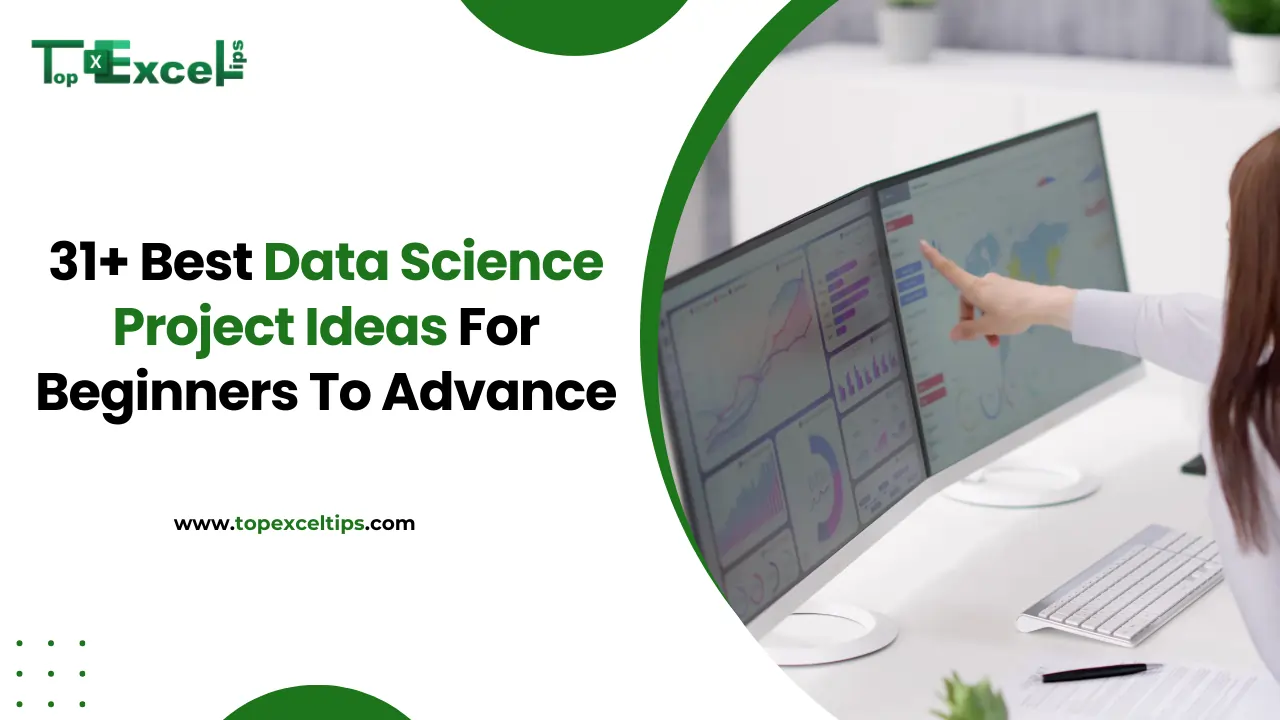
Getting hands-on experience is really important in data science. Learning about data is one thing, but using that knowledge in real projects is where you truly understand. Whether you’re just starting or want to improve your skills, working on Data Science Project Ideas is a great way to advance.
In this guide, you’ll find over 31+ project ideas for data science, suitable for all skill levels—from beginners to advanced. These projects will help you practice and understand different parts of data science, like analyzing data and building machine learning models. You’ll get to work with real data, solve real problems, and make a portfolio to show off your skills.
From simple tasks to more complex challenges, this guide has project ideas that match your experience level and will help you grow. Explore these ideas and turn your knowledge into practical skills.
Table of Contents
Survey Results: Difficulties in Selecting the Right Project Idea
We recently ran a poll with around 178 participants, and the findings revealed a similar difficulty for many of them. The majority of respondents said they needed help deciding on a project concept.
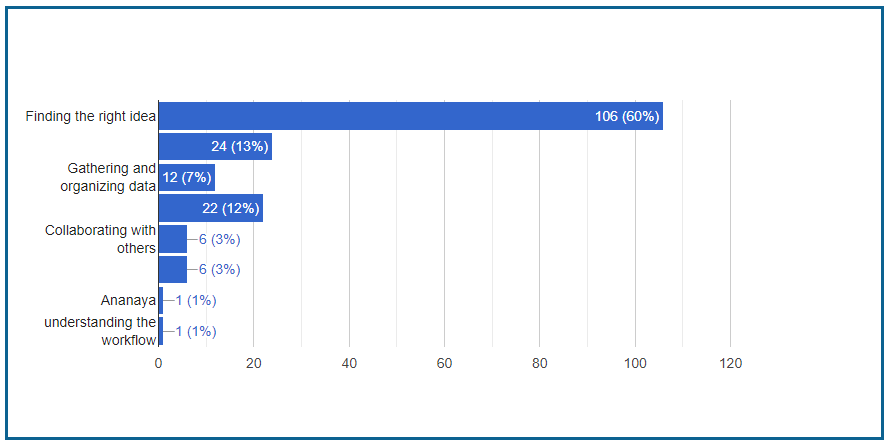
What is Data Science?
Data science is about using data to make smart decisions. It involves collecting information, cleaning it up, analyzing it, and figuring out what it means. By combining skills from statistics, math, and computer science, data science helps turn raw data into useful insights.
Data scientists use tools and techniques to find patterns and trends in large sets of data. This helps businesses understand their information and use it effectively.
Why Data Science Matters in Tech?
- Better Decisions : Data science helps companies make better choices by providing clear insights from data. This allows businesses to see trends, predict what might happen, and create effective plans.
- Improving Products and Efficiency : In tech, data science helps develop new products and make existing ones better. It also makes processes more efficient, like improving recommendation systems or user experiences.
- Staying Ahead : Companies that employ data science can stay ahead of the competition. Understanding consumer behavior and industry trends allows organizations to react swiftly and stay ahead.
- Personalized Experiences : Data science helps businesses offer customized services. By looking at customer preferences, companies can make recommendations and marketing more relevant to each person.
- Solving Problems : Data science helps solve complex problems by finding hidden patterns in data. Whether it’s predicting problems, spotting fraud, or understanding customers, data science provides solutions.
31+ Data Science Project Ideas: For Beginner to Advance Level
Here are the best Data Science Project Ideas For Beginner to Advance Level
Beginner Projects
- Description : Make a model to guess which Titanic passengers survived based on old data. You’ll clean the data, choose important features, and use simple classification methods.
- Core Skills : Classification, data cleaning, feature selection.
- Technologies : Python, scikit-learn, pandas, Jupyter Notebook.
- Description : Build a system that recommends movies based on user ratings. This project involves using basic recommendation techniques to suggest movies to users.
- Core Skills : Recommendation systems, data analysis.
- Technologies : Python, pandas, scikit-learn, Surprise library.
- Description : Use the Iris dataset to sort different types of iris flowers by their measurements. This involves exploring the data, creating visuals, and using classification models like k-nearest Neighbors (k-NN).
- Core Skills : Data exploration, classification, data visualization.
- Technologies : Python, scikit-learn, matplotlib, seaborn.
- Description : This project examines stock price data to find patterns and trends. It involves analyzing time series data and creating visualizations.
- Core Skills : Time series analysis data visualization.
- Technologies : Python, pandas, matplotlib, numpy.
- Description : Create charts to show weather data, such as temperature and rainfall. This project focuses on cleaning data and visualizing trends.
- Core Skills : Data visualization and basic statistics.
- Technologies : Python, pandas, matplotlib, seaborn.
- Description : Predict which customers might leave a service using historical data. You’ll use classification methods to understand and predict customer behavior.
- Core Skills : Classification, data cleaning, model evaluation.
- Description : Analyze tweets to see if they are positive, negative, or neutral. This project uses natural language processing (NLP) to understand tweet sentiments.
- Core Skills : NLP, sentiment analysis.
- Technologies : Python, NLTK, TextBlob, pandas.
- Description : Analyze sales data to find trends and factors affecting sales. This involves aggregating data, visualizing it, and performing basic statistical analysis.
- Core Skills : Data aggregation, statistical analysis, data visualization.
- Description : Create a model to predict housing prices based on features like size and location. This project involves using regression analysis and evaluating the model.
- Core Skills : Regression analysis, model evaluation.
- Description : Predict house prices using data about house features. This project includes cleaning data, selecting features, and applying regression methods.
- Core Skills : Regression analysis, data cleaning, feature selection.
Also Read: |
Intermediate Projects
- Description : Use K-Means clustering to group customers based on their buying habits. This project involves clustering data and scaling features.
- Core Skills : Clustering, feature scaling, customer segmentation.
- Technologies : Python, scikit-learn, pandas, matplotlib.
- Description : Forecast future stock prices using the ARIMA model. This project involves analyzing time series data and making predictions.
- Core Skills : Time series forecasting, ARIMA modeling.
- Technologies : Python, statsmodels, pandas, numpy.
- Description : Collect data from websites by scraping. This project covers extracting and cleaning data from web pages.
- Core Skills : Web scraping, data extraction.
- Technologies : Python, BeautifulSoup, requests, pandas.
- Description : Create an interactive dashboard to show key metrics and trends. This involves using tools to make dynamic visualizations.
- Core Skills : Data visualization dashboard creation.
- Technologies : Tableau, Plotly, Python, pandas.
- Description : Predict what customers will buy based on their past behavior. This project uses predictive modeling and feature engineering.
- Core Skills : Predictive modeling, feature engineering.
- Description : Classify text documents using natural language processing techniques. This involves processing text, extracting features, and using classification algorithms.
- Core Skills : Text classification, NLP, feature extraction.
- Technologies : Python, NLTK, scikit-learn, pandas.
- Description : Use logistic regression to predict if customers will stop using a service. This includes data preprocessing and model evaluation.
- Core Skills : Logistic regression, model evaluation.
- Description : Analyze movie reviews to see if they are positive or negative. This project uses advanced NLP techniques for sentiment analysis.
- Core Skills : Sentiment analysis, NLP.
- Description : Detect fraudulent transactions using machine learning models. This project involves identifying anomalies in financial data.
- Core Skills : Anomaly detection, fraud detection.
- Technologies : Python, scikit-learn, pandas, numpy.
- Description : Build a model to forecast future sales based on past data. This project involves using machine learning methods and analyzing time series data.
- Core Skills : Predictive modeling, time series analysis.
- Technologies : Python, scikit-learn, pandas, statsmodels.
Advanced Projects
- Description : Use Convolutional Neural Networks (CNNs) to classify images. This involves deep learning and image processing techniques.
- Core Skills : Deep learning, image classification, CNNs.
- Technologies : Python, TensorFlow, Keras, OpenCV.
- Description : Create text that makes sense based on given input using advanced NLP models. This project involves training language models.
- Core Skills : Natural language generation, deep learning.
- Technologies : Python, TensorFlow, Keras, GPT models.
- Description : Predict when equipment will fail using data from IoT sensors. This project involves analyzing sensor data and applying predictive modeling.
- Core Skills : Predictive maintenance, IoT data analysis.
- Technologies : Python, scikit-learn, pandas, IoT platforms.
- Description : This project uses Long-Short-Term Memory (LSTM) networks to forecast time series data. It involves advanced forecasting techniques and deep learning.
- Core Skills : Time series forecasting, LSTM networks.
- Technologies : Python, TensorFlow, Keras, pandas.
- Description : Develop a chatbot that can have conversations with users using NLP and machine learning. This involves creating and training conversational AI systems.
- Core Skills : Conversational AI, chatbot development, NLP.
- Technologies : Python, TensorFlow, Keras, Rasa, Dialogflow.
- Description : Build an advanced recommendation system using matrix factorization methods. This involves collaborative filtering and advanced recommendation algorithms.
- Core Skills : Recommendation algorithms, matrix factorization.
- Technologies : Python, scikit-learn, Surprise library, pandas.
- Description : Analyze social media data to measure how influential different users and topics are. This project involves using analytics tools to assess influence.
- Core Skills : Social media analysis, influence measurement.
- Technologies : Python, social media APIs , pandas, network analysis tools.
- Description : Generate captions for images using deep learning models. This involves combining image processing with natural language generation techniques.
- Core Skills : Image captioning, deep learning.
- Description : Build a dashboard that shows sentiment analysis of live social media or news feeds. This project involves real-time data processing and visualization.
- Core Skills : Real-time data analysis, sentiment analysis, dashboard development.
- Technologies : Python, Flask, Plotly, NLTK.
- Description : Detect unusual patterns in network traffic data that might indicate security threats. This project uses anomaly detection techniques on network data.
- Core Skills : Anomaly detection, network security analysis.
- Technologies : Python, scikit-learn, pandas, network analysis tools.
- Description : Create a system that suggests personalized learning resources based on a user’s skills and interests. This project involves using recommendation algorithms and educational data.
- Core Skills : Personalized recommendations data analysis.
- Technologies : Python, scikit-learn, pandas, educational data platforms.
How to Start a Data Science Project
Here are the steps to follow for starting a Data Science Project
1. Define the Problem
- Understand What You Want : Figure out what problem you are trying to solve. What do you want to learn or achieve with your project?
- Define specific goals: Establish what success means to you and what you aim to accomplish.
2. Gather Data
- Find Where to Get Data : Look for sources where you can get the data you need. This could be public datasets, company databases, or data you collect yourself.
- Collect the Data : Make sure the data you gather is relevant and enough for your project.
3. Prepare the Data
- Clean Up the Data : Fix any issues with the data, such as missing information or mistakes. Clean data is important for good results.
- Format the Data : Adjust the data so it’s ready for analysis. This might mean normalizing numbers, scaling data, or turning categories into numerical values.
4. Explore the Data
- Look at the Data : Examine the data to understand its patterns and structure. Use charts and summaries to get insights.
- Find Key Features : Identify which parts of the data are most important for solving your problem.
5. Choose a Model
- Pick a Model : Based on your problem (like classification, prediction, or grouping), choose a suitable model or algorithm.
- Train the Model : Using your data, train the model how to make predictions or judgments.
6. Evaluate the Model
- Test the Model : Check how well your model performs with a separate set of data.
- Measure Performance : Look at how well the model did using metrics like accuracy or precision.
7. Fine-Tune and Improve
- Adjust Settings : Change the model’s settings to make it work better.
- Validate : Test the model on different data to make sure it works well in various situations.
8. Interpret Results
- Understand What You Found : See if the results meet your goals and answer your questions.
- Explain the Findings : Describe what the results mean for your project.
9. Communicate Findings
- Create Visuals : Make charts and graphs to show your results clearly.
- Write a Report : Summarize what you did, what you found, and what it means in an easy-to-understand report.
10. Deploy the Solution
- Use the Model : If applicable, put the model into use where others can benefit from it.
- Monitor : Keep track of how the model performs over time to make sure it stays useful.
11. Improve and Update
- Get Feedback : Ask for feedback on your work and make changes if needed.
- Update the Model : Make improvements based on new data or changes in requirements.
Following these steps will help you start and complete a data science project, from identifying the problem to using and refining your solution.
Overcoming Challenges in Data Science Projects: Simple Steps to Get Back on Track
1. review the problem.
- Check Your Goals : Make sure you know exactly what you want to achieve. Are your project goals still clear?
- Break It Down : Divide the problem into smaller steps if it feels too complicated.
2. Check Your Data
- Fix Data Issues : Make sure your data is clean and correct. Problems with the data can cause issues.
- Try More Data : Use other datasets or add more data if needed to improve your results.
3. Ask for Help
- Get Advice : Talk to colleagues, mentors, or online communities for suggestions.
- Describe Your Problem : Explain what’s wrong to get better help.
4. Recheck Your Methods
- Verify Your Approach : Make sure you’re using the right techniques and models.
- Try Different Methods : Experiment with other approaches if your current one isn’t working.
5. Fix Your Code
- Find Errors : Look through your code for mistakes.
- Use Debugging Tools : Use tools or add print statements to find out where things are going wrong.
6. Take a Break
- Step Away : Sometimes, taking a short break can help you see things more clearly.
- Come Back with Fresh Eyes : Returning after a break can help you solve problems better.
7. Check Documentation
- Read Instructions : Look at the documentation for the tools or libraries you’re using.
- Find Examples : Look for examples or tutorials that show how to solve similar problems.
8. Experiment and Improve
- Try New Things : Be open to trying different strategies or models.
- Refine Your Work : Make improvements based on what you learn.
9. Keep Notes
- Record Your Work : Write down what you’ve tried and what’s worked or not worked.
- Review Your Notes : Check your notes to see if there’s anything you’ve missed.
10. Stay Persistent
- Keep Going : It’s normal to face challenges. Keep working through them.
- Learn from Mistakes : Use setbacks as chances to learn and improve.
These steps help you get past roadblocks and keep moving forward with your data science project.
Final Words
Working on data science project ideas can be both fun and rewarding, whether you’re new to the field or have some experience. The 31+ data science project ideas we’ve discussed are great for all skill levels, helping you gain practical experience and build a good portfolio.
Each of these project ideas offers a chance to learn and improve. Don’t hesitate to try different methods, ask for help if needed, and make adjustments along the way. Every project will help you get better and prepare you for real-world challenges.
What level of experience is needed for these projects?
The 31+ data science project ideas cover different levels of difficulty. Beginners can start with simpler projects, while those with more experience can try more complex ones. Pick projects that match your skill level.
How do I pick the right data science project?
Choose a project that interests you and fits your skill level. Think about the type of data and the problem you want to solve. Start with easier projects to build your confidence before moving on to harder ones.
What tools and technologies will I need?
Common tools for data science include Python, R, SQL, machine learning libraries (like scikit-learn or TensorFlow), and data visualization tools (such as Tableau or Matplotlib). The tools you need will depend on your project.
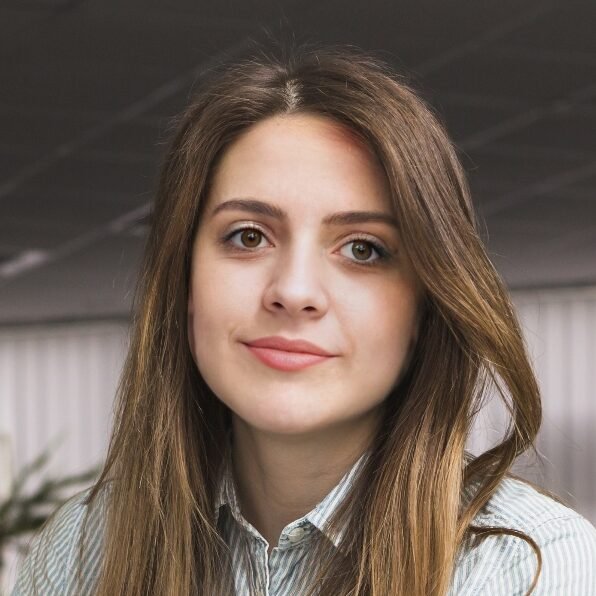
About the author
Hi, I’m Emmy Williamson! With over 20 years in IT, I’ve enjoyed sharing project ideas and research on my blog to make learning fun and easy.
So, my blogging story started when I met my friend Angelina Robinson. We hit it off and decided to team up. Now, in our 50s, we've made TopExcelTips.com to share what we know with the world. My thing? Making tricky topics simple and exciting.
Come join me on this journey of discovery and learning. Let's see what cool stuff we can find!
Leave a Comment Cancel reply
Save my name, email, and website in this browser for the next time I comment.
Top Excel Tips
Top Excel Tips teaches you Excel. We have lessons, project ideas, and helpful stuff. Our goal is to make you great at using Excel.
Copyright © Top Excel Tips | All Rights Reserved
Numbers, Facts and Trends Shaping Your World
Read our research on:
Full Topic List
Regions & Countries
Publications
- Our Methods
- Short Reads
- Tools & Resources
Read Our Research On:
Data Science
Measuring news consumption in a digital era.
As news outlets morph and multiply, both surveys and passive data collection tools face challenges.
What is machine learning, and how does it work?
How does a computer ‘see’ gender, sign up for our methods newsletter.
The latest on survey methods, data science and more, delivered quarterly.
Rising share of lawmakers – but few Republicans – are using the term Latinx on social media
One-quarter of United States lawmakers mentioned the term on Facebook or Twitter during the 116th Congress.
Sharp decline in remittances expected in 2020 amid COVID-19 lockdowns in top sending nations
Remittances – money sent by migrants to their home countries – are projected to fall by a record 20% this year.
Our latest Methods 101 video explains the basics of machine learning and how it allows our researchers to analyze data on a large scale.
For Global Legislators on Twitter, an Engaged Minority Creates Outsize Share of Content
Although most national officials use the platform, their posts receive only a small number of likes and retweets.
Nigerians living near a major Belt and Road project grew more positive toward China after it was completed
Our analysis assesses the relationship between Nigerians’ distance to a major Chinese investment in their country and their views toward China.
Tweets by members of Congress tell the story of an escalating COVID-19 crisis
More than half of all tweets sent by members of the U.S. Congress between March 11 and 21 were related to the coronavirus outbreak.
Q&A: Why we studied American sermons and how we did it
Dennis Quinn, computational social scientist, explains how our analysis of sermons came together and the challenges that arise when religion meets big data.
The Digital Pulpit: A Nationwide Analysis of Online Sermons
This Pew Research Center analysis harnesses computational techniques to identify, collect and analyze the sermons that U.S. churches livestream or share on their websites each week.
10 facts about Americans and YouTube
Using public opinion surveys and large-scale data analysis, we have studied the content on YouTube and how the U.S. public engages with it.
REFINE YOUR SELECTION
Research teams.
901 E St. NW, Suite 300 Washington, DC 20004 USA (+1) 202-419-4300 | Main (+1) 202-857-8562 | Fax (+1) 202-419-4372 | Media Inquiries
Research Topics
- Email Newsletters
ABOUT PEW RESEARCH CENTER Pew Research Center is a nonpartisan fact tank that informs the public about the issues, attitudes and trends shaping the world. It conducts public opinion polling, demographic research, media content analysis and other empirical social science research. Pew Research Center does not take policy positions. It is a subsidiary of The Pew Charitable Trusts .
© 2024 Pew Research Center
Training in open research, including AI, ethics, data visualisation, and more – register now
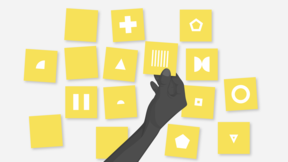
Whether you're a seasoned researcher or just starting out, our webinars provide valuable opportunities to reevaluate your working methods, acquire new insights, and enhance existing knowledge on open science and research. Our webinars remain free and accessible to all, regardless of prior knowledge. While the primary audience includes researchers, university staff, and students, anyone with an interest in these subjects is encouraged to join. Each training season introduces entirely new topics, along with updates to our recurring webinars based on your valuable feedback.
Find all training sessions here
This autumn we have a whole host of new webinars:
- ABC of Open Access Publishing, Oct 23, 2024
- Export Control, Sanctions, and Research Security, Oct 30, 2024
- Artificial Intelligence, Medical Decision Making and Research Ethics, Oct 31, 2024
- Visualise Your Research Data, Nov 6, 2024 (Note: date to be updated!)
- Research Ethics and Legal Questions in Web-Based Research, Nov 7, 2024
- Exploring Machine Learning in Research, Nov 11, 2024
- MAGICS: Methods for Digitising and Virtualising Human Behaviour, Nov 14, 2024
- Hands-on Data Protection (two-day workshop), Dec 3 & 5, 2024
Many of our webinars will be recorded and published on the Aalto Research Services YouTube channel . There you can also find previous recordings. Our lectures include interactive segments or a Q&A session at the end, which won't be recorded to respect your privacy. We encourage you to actively participate, pose questions to our experts, and contribute to the discussion. While some sessions may feature specific information regarding Aalto researchers, the fundamental principles presented are universally applicable.
Join the conversation – we're excited to see you in the autumn training sessions!
Questions about the training and data management can be sent to: [email protected] .
RDM and open science support at Aalto University:
Research data management (rdm) and open science.
Properly managed research data creates competitive edge and is an important part of a high-quality research process. Here you will find links to support, services and instructions for research data management.
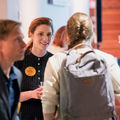
Training in Research Data Management and Open Science
We offer free and open to all training in research data management and open science.
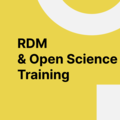
Data Agents
Data Agents are researchers who work to improve data management in their department, school, or unit.
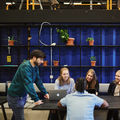
Open science and research
The principle of openness is the key principle of science and research. At Aalto University, the most visible forms of open science are open access publications, open research data and metadata, and combining openness and commercialisation.

- Published: 4.9.2024
- Updated: 4.9.2024
Read more news

Everyday choices: Kristjana Adalgeirsdóttir, what does an architect do in a war zone?

Broaden your perspective with the latest research at the Marsio exhibitions

‘Running a business isn’t so bad after all’

“Enhancing Equity, Diversity and Inclusion in the workplace” - MOOC course opens in October 2024
- Data Science
- Data Analysis
- Data Visualization
- Machine Learning
- Deep Learning
- Computer Vision
- Artificial Intelligence
- AI ML DS Interview Series
- AI ML DS Projects series
- Data Engineering
- Web Scrapping
Choosing the Right Tools and Technologies for Data Science Projects
In the ever-evolving field of data science, selecting the right tools and technologies is crucial to the success of any project. With numerous options available—from programming languages and data processing frameworks to visualization tools and machine learning libraries—making informed decisions can greatly impact your project’s outcomes.
Table of Content
Programming Languages
Data processing frameworks, data visualization tools, machine learning libraries.
This article provides a comprehensive guide to the essential tools and technologies for data science projects, helping you choose the right ones based on your project’s needs.
Python Programming Language
Python is widely recognized as the most popular programming language in data science. Its simplicity, versatility, and extensive ecosystem make it a preferred choice for many data scientists.
Key Libraries:
- NumPy: Facilitates numerical operations and array handling.
- Pandas: Essential for data manipulation and analysis.
- Scikit-learn: Used for machine learning and data mining.
- Matplotlib and Seaborn: Popular for data visualization.
- TensorFlow and PyTorch: Key frameworks for deep learning.
- Data cleaning and preparation
- Machine learning and statistical modeling
- Data visualization and exploratory data analysis
- Extensive libraries and frameworks
- Strong community support and documentation
- Integration with other tools and technologies
Limitations:
- Slower execution speed compared to compiled languages
- May require optimization for large-scale data processing
R Programming Languages
R is a language specifically designed for statistical computing and data visualization. It is widely used in academia and research due to its powerful statistical analysis capabilities.
Key Packages:
- ggplot2: For advanced data visualization.
- dplyr and tidyr: For data manipulation and transformation.
- caret: For machine learning.
- shiny: For building interactive web applications.
- Statistical analysis
- Data visualization and reporting
- Research and academic applications
- Specialized for statistical analysis and data visualization
- Rich ecosystem of packages for various statistical methods
- Excellent for exploratory data analysis and reporting
- Steeper learning curve for non-statisticians
- Less suited for production-level applications compared to Python
Apache Hadoop
Hadoop is an open-source framework designed for distributed storage and processing of large data sets using a cluster of commodity hardware. It handles massive amounts of data efficiently.
Components:
- HDFS (Hadoop Distributed File System): For distributed data storage.
- MapReduce: For distributed data processing.
- YARN (Yet Another Resource Negotiator): For resource management and job scheduling.
- Big data processing and storage
- Data warehousing
- Large-scale data analytics
- Scalability to handle large data volumes
- Fault tolerance and high availability
- Open-source with a large ecosystem
- Complexity in setup and management
- Slower processing speed compared to newer technologies
Apache Spark
Spark is a unified analytics engine for large-scale data processing, known for its speed and ease of use. It can handle both batch and real-time data processing tasks.
- Spark Core: Provides the basic functionalities for distributed task dispatching, scheduling, and monitoring.
- Spark SQL: Allows querying data via SQL and integrating with various data sources.
- Spark Streaming: Enables real-time data stream processing.
- MLlib: A machine learning library that provides scalable algorithms.
- GraphX: For graph processing.
- Real-time data processing
- Advanced analytics and machine learning
- ETL (Extract, Transform, Load) tasks
- High performance with in-memory processing
- Supports batch and real-time processing
- Rich set of libraries for machine learning and graph processing
- Requires memory management for large datasets
- Can be complex to set up and optimize
Tableau is a leading data visualization tool known for its ease of use and powerful interactive dashboards. It allows users to create a wide range of visualizations and share them easily.
- Drag-and-drop interface for creating visualizations
- Integration with multiple data sources
- Interactive dashboards and real-time data updates
- Business intelligence and reporting
- Interactive data visualization
- Data exploration and analysis
- User-friendly interface
- Strong community and support
- Versatile visualization options
- Can be expensive for enterprise versions
- Limited customization compared to programming-based tools
Power BI is a business analytics tool from Microsoft that provides interactive visualizations and business intelligence capabilities. It integrates seamlessly with other Microsoft products.
- Integration with various data sources, including Microsoft products
- Interactive dashboards and reports
- Advanced analytics with built-in AI features
- Business analytics and reporting
- Interactive dashboards
- Data-driven decision-making
- Integration with Microsoft ecosystem
- Cost-effective compared to some other tools
- May require familiarity with Microsoft products
- Less flexibility in customization compared to some other tools
TensorFlow is an open-source machine learning framework developed by Google. It is widely used for building and deploying machine learning models, particularly deep learning models.
- Support for various machine learning and deep learning algorithms
- Scalability for large datasets and distributed computing
- TensorFlow Serving for model deployment
- Deep learning and neural network models
- Large-scale machine learning projects
- Production-grade model deployment
- Comprehensive set of tools and libraries
- Strong support for deep learning and neural networks
- Scalability and production readiness
- Steeper learning curve for beginners
PyTorch is an open-source deep learning framework developed by Facebook. It is known for its flexibility and ease of use, particularly in research and development.
- Dynamic computation graph for flexible model building
- Integration with Python for ease of use
- Strong support for GPU acceleration
- Deep learning research and prototyping
- Dynamic neural network architectures
- Production and research applications
- Intuitive and flexible API
- Strong support for research and experimentation
- Easy to debug and experiment with
- Less mature ecosystem compared to TensorFlow
- Can be less optimized for production environments
Choosing the right tools and technologies for your data science project involves evaluating your specific needs, including data structure, processing requirements, visualization needs, and machine learning goals. Python and R are excellent choices for programming, with Python being more versatile and R specializing in statistical analysis. For data processing, Hadoop and Spark offer powerful capabilities, with Spark providing superior performance for both batch and real-time processing. Visualization tools like Tableau and Power BI offer user-friendly options for creating interactive dashboards and reports, while machine learning libraries such as TensorFlow and PyTorch cater to various deep learning and machine learning needs. By carefully considering these options, you can select the tools that best align with your project’s objectives and ensure its success.
Please Login to comment...
Similar reads.
- AI-ML-DS Blogs
- Data Science Blogs
- Top Android Apps for 2024
- Top Cell Phone Signal Boosters in 2024
- Best Travel Apps (Paid & Free) in 2024
- The Best Smart Home Devices for 2024
- 15 Most Important Aptitude Topics For Placements [2024]
Improve your Coding Skills with Practice
What kind of Experience do you want to share?

An official website of the United States government
The .gov means it’s official. Federal government websites often end in .gov or .mil. Before sharing sensitive information, make sure you’re on a federal government site.
The site is secure. The https:// ensures that you are connecting to the official website and that any information you provide is encrypted and transmitted securely.
- Publications
- Account settings
- My Bibliography
- Collections
- Citation manager
Save citation to file
Email citation, add to collections.
- Create a new collection
- Add to an existing collection
Add to My Bibliography
Your saved search, create a file for external citation management software, your rss feed.
- Search in PubMed
- Search in NLM Catalog
- Add to Search
The evolution of computational research in a data-centric world
Affiliations.
- 1 Titus Department of Clinical Pharmacy, Alfred E. Mann School of Pharmacy and Pharmaceutical Sciences, University of Southern California, Los Angeles, CA 90089, USA. Electronic address: [email protected].
- 2 Titus Department of Clinical Pharmacy, Alfred E. Mann School of Pharmacy and Pharmaceutical Sciences, University of Southern California, Los Angeles, CA 90089, USA.
- 3 Department of Pharmacology and Pharmaceutical Sciences, Alfred E. Mann School of Pharmacy and Pharmaceutical Sciences, University of Southern California, Los Angeles, CA 90089, USA.
- 4 Department of Molecular, Cell and Developmental Biology, University of California, Los Angeles, Los Angeles, CA 90095, USA.
- 5 Department of Genetics, The Alexander Silberman Institute of Life Sciences, The Hebrew University of Jerusalem, Jerusalem 9190401, Israel.
- 6 Genomics Research Department, King Fahad Medical City, Riyadh, Saudi Arabia; Department of Pathology & Laboratory Medicine, Emory University Hospital, Atlanta, GA, USA.
- 7 The Liver Transplant Unit, King Faisal Specialist Hospital and Research Center, Riyadh, Saudi Arabia; The Division of Gastroenterology and Hepatology, Johns Hopkins University, Baltimore, MD 21205, USA.
- 8 Department of Epidemiology & Biostatistics, Institute for Human Genetics, University of California, San Francisco, 513 Parnassus Avenue S965F, San Francisco, CA 94143, USA.
- 9 GV20 Oncotherapy, One Broadway, 14th Floor, Kendall Square, Cambridge, MA 02142, USA.
- 10 Biostatistics and Oncology at the Johns Hopkins Bloomberg School of Public Health and Johns Hopkins Data Science Lab, John Hopkins University, 615 N. Wolfe Street, Baltimore, MD 21205, USA.
- 11 EMBL European Bioinformatics Institute, Wellcome Genome Campus, Hinxton CB10 1SD, UK.
- 12 Department of Psychiatry and Human Genetics, Center for Neurobehavioral Genetics, University of California, Los Angeles, Los Angeles, CA, USA.
- 13 Bakar Computational Health Sciences Institute, University of California, San Francisco, 490 Illinois Street, San Francisco, CA 94158, USA.
- 14 Department of Computational Biomedicine, Cedars-Sinai Medical Center, 700 N. San Vicente Boulevard, Pacific Design Center Suite G540, West Hollywood, CA 90068, USA.
- 15 Department of Quantitative and Computational Biology, USC Dornsife College of Letters, Arts and Sciences, University of Southern California, Los Angeles, CA 90007, USA.
- 16 Department of Quantitative and Computational Biology, USC Dornsife College of Letters, Arts and Sciences, University of Southern California, Los Angeles, CA 90007, USA. Electronic address: [email protected].
- PMID: 39178828
- DOI: 10.1016/j.cell.2024.07.045
Computational data-centric research techniques play a prevalent and multi-disciplinary role in life science research. In the past, scientists in wet labs generated the data, and computational researchers focused on creating tools for the analysis of those data. Computational researchers are now becoming more independent and taking leadership roles within biomedical projects, leveraging the increased availability of public data. We are now able to generate vast amounts of data, and the challenge has shifted from data generation to data analysis. Here we discuss the pitfalls, challenges, and opportunities facing the field of data-centric research in biology. We discuss the evolving perception of computational data-driven research and its rise as an independent domain in biomedical research while also addressing the significant collaborative opportunities that arise from integrating computational research with experimental and translational biology. Additionally, we discuss the future of data-centric research and its applications across various areas of the biomedical field.
Copyright © 2024 Elsevier Inc. All rights reserved.
PubMed Disclaimer
Conflict of interest statement
Declaration of interests The authors declare no competing interests.
Publication types
- Search in MeSH
Related information
Linkout - more resources, full text sources.
- Elsevier Science
Miscellaneous
- NCI CPTAC Assay Portal
- Citation Manager
NCBI Literature Resources
MeSH PMC Bookshelf Disclaimer
The PubMed wordmark and PubMed logo are registered trademarks of the U.S. Department of Health and Human Services (HHS). Unauthorized use of these marks is strictly prohibited.
Using AgentM to watch for new research papers of interest
I’m getting enough of the pieces of AgentM in place that I’m able to get it to do useful things. I wrote a small program (ok AgentM wrote part of it) that fetches the last days worth of research papers from arxiv.org , filters them to the papers related to topics I care about, and then projects those filtered papers to a uniform markdown format for easy scanning:

It uses gpt-4o-mini so it’s cost effective to run and it took 6 or 7 minutes in total to process 553 papers. Here’s the meat of the code:
I did another pass other the 81 papers it selected as being on topic and had the model select the top 10 papers for the day using another projection:
Automating Knowledge Discovery from Scientific Literature via LLMs: A Dual-Agent Approach with Progressive Ontology Prompting
Read more This paper introduces a novel framework combining large language models (LLMs) with a dual-agent system to enhance knowledge extraction from scientific literature, achieving significant improvements in annotation accuracy.
why The integration of LLMs with a dual-agent system for knowledge extraction is a significant advancement, potentially transforming how scientific literature is analyzed and utilized.
Urban Mobility Assessment Using LLMs
Read more This work proposes an AI-based approach for synthesizing travel surveys using LLMs, addressing privacy concerns and demonstrating effectiveness across various U.S. metropolitan areas.
why The application of LLMs in urban mobility assessment offers a novel solution to privacy issues in travel surveys, with implications for urban planning and policy-making.
Towards Human-Level Understanding of Complex Process Engineering Schematics: A Pedagogical, Introspective Multi-Agent Framework for Open-Domain Question Answering
Read more The paper presents a multi-agent framework for interpreting process diagrams, enhancing data privacy and explainability while achieving superior performance in open-domain question answering tasks.
why This research enhances the understanding of complex engineering schematics, which is crucial for industries relying on process engineering, improving both privacy and explainability.
Classification of Safety Events at Nuclear Sites using Large Language Models
Read more This research develops an LLM-based classifier to categorize safety records at nuclear power stations, aiming to improve the efficiency and accuracy of safety classification processes.
why Improving safety classification at nuclear sites is critical for operational safety and regulatory compliance, making this application of LLMs highly impactful.
Non-instructional Fine-tuning: Enabling Instruction-Following Capabilities in Pre-trained Language Models without Instruction-Following Data
Read more The paper explores a novel approach to fine-tuning LLMs for instruction-following capabilities using non-instructional data, potentially broadening the scope of LLM applications.
why This approach could significantly expand the versatility of LLMs, allowing them to perform tasks without explicit instruction-following data, which is a major step forward in AI development.
Zero-Shot Visual Reasoning by Vision-Language Models: Benchmarking and Analysis
Read more This study benchmarks vision-language models’ zero-shot visual reasoning capabilities, revealing insights into their performance and limitations in complex reasoning tasks.
why Understanding the zero-shot capabilities of vision-language models is crucial for their application in areas requiring complex visual reasoning, such as autonomous vehicles and robotics.
Toward Large Language Models as a Therapeutic Tool: Comparing Prompting Techniques to Improve GPT-Delivered Problem-Solving Therapy
Read more The research assesses the impact of prompt engineering on LLMs delivering psychotherapy, highlighting the potential of AI in addressing mental health needs.
why The potential use of LLMs in psychotherapy could revolutionize mental health care, making therapy more accessible and personalized.
HoneyComb: A Flexible LLM-Based Agent System for Materials Science
Read more This paper introduces HoneyComb, an LLM-based agent system tailored for materials science, significantly improving task performance and accuracy.
why The application of LLMs in materials science could accelerate research and development in this field, leading to faster innovation and discovery.
Sequence to Sequence Reward Modeling: Improving RLHF by Language Feedback
Read more The study proposes a novel reward modeling method that enhances reinforcement learning from human feedback (RLHF) by utilizing language feedback, improving alignment with human preferences.
why Enhancing RLHF with language feedback could improve the alignment of AI systems with human values and preferences, which is essential for ethical AI development.
The creative psychometric item generator: a framework for item generation and validation using large language models
Read more This research develops a framework for generating valid creativity assessments using LLMs, demonstrating their potential in automating creativity testing processes.
why Automating creativity assessments with LLMs could transform educational and psychological testing, making it more efficient and accessible.
This is pretty awesome! I am tempted to write the underlying library in python.
I setup a placeholder GitHub project and would happily add you as a contributor.

That would be awesome!
My git user is icdev2dev. Thanks !

This is what I was thinking about:
I was just chatting with a long time Microsoft colleague (we created the Microsoft Bot Framework together) and he’s excited to create a .NET version of AgentM.
We were discussing that achieving absolute parity across languages isn’t super critical because you really want to lean into the strengths and paradigms of each language. What feels natural to a JavaScript developer isn’t going to feel as natural to a Python developer and it’s definitely not going to feel natural to a .net developer.
The important part is to maintain the spirit of AgentM across languages. To that end I’ll leave it up to you and others to determine what that means for Python.
That’s right.
But as I am thinking more about it, I believe that we shou;d also build in failure tolerance into the framework.
For example, if I have shown a set of sentences (amongst many such sets) to the LLM for the purpose of doing some labeling on sentences,I don’t want to lose that showing if the (distributed) job suddenly fails in between. It should be able to restart for the remaining jobs, complete those and then return the function to the caller.
The semantics of the threads allows for this distibuted jobs to fail and be restarted, I think.
I believe that that might be also what openAI might be pursuing as well (long lived jobs).
Yeah that’s a good suggestion…
@icdev2dev I just finished getting AgentM to convert all of its JS code to Python and it actually didn’t do too bad of a job. It’s easily 80% of the way there.

It got paths to some of the components wrong because it assumed everything was relative and in the same folder but given that it could only see one file at a time I think it did a good job. I told it which libraries I wanted it to use and it followed all of that guidance:

I’ll check the generated code into the python repo shortly.
Related Topics
Topic | Replies | Views | Activity | |
---|---|---|---|---|
Community | 7 | 133 | September 3, 2024 | |
Community , , | 3 | 407 | July 3, 2024 | |
Community | 1 | 3424 | May 7, 2024 | |
Community | 1 | 977 | May 3, 2023 | |
Community | 4 | 613 | January 3, 2024 |
- News & Media
- Chemical Biology
- Computational Biology
- Ecosystem Science
- Cancer Biology
- Exposure Science & Pathogen Biology
- Metabolic Inflammatory Diseases
- Advanced Metabolomics
- Mass Spectrometry-Based Measurement Technologies
- Spatial and Single-Cell Proteomics
- Structural Biology
- Biofuels & Bioproducts
- Human Microbiome
- Soil Microbiome
- Synthetic Biology
- Computational Chemistry
- Chemical Separations
- Chemical Physics
- Atmospheric Aerosols
- Human-Earth System Interactions
- Modeling Earth Systems
- Coastal Science
- Plant Science
- Subsurface Science
- Terrestrial Aquatics
- Materials in Extreme Environments
- Precision Materials by Design
- Science of Interfaces
- Friction Stir Welding & Processing
- Dark Matter
- Flavor Physics
- Fusion Energy Science
- Neutrino Physics
- Quantum Information Sciences
- Emergency Response
- AGM Program
- Tools and Capabilities
- Grid Architecture
- Grid Cybersecurity
- Grid Energy Storage
- Earth System Modeling
- Energy System Modeling
- Transmission
- Distribution
- Appliance and Equipment Standards
- Building Energy Codes
- Advanced Building Controls
- Advanced Lighting
- Building-Grid Integration
- Building and Grid Modeling
- Commercial Buildings
- Federal Performance Optimization
- Resilience and Security
- Grid Resilience and Decarbonization
- Building America Solution Center
- Energy Efficient Technology Integration
- Home Energy Score
- Electrochemical Energy Storage
- Flexible Loads and Generation
- Grid Integration, Controls, and Architecture
- Regulation, Policy, and Valuation
- Science Supporting Energy Storage
- Chemical Energy Storage
- Waste Processing
- Radiation Measurement
- Environmental Remediation
- Subsurface Energy Systems
- Carbon Capture
- Carbon Storage
- Carbon Utilization
- Advanced Hydrocarbon Conversion
- Fuel Cycle Research
- Advanced Reactors
- Reactor Operations
- Reactor Licensing
- Solar Energy
- Wind Resource Characterization
- Wildlife and Wind
- Community Values and Ocean Co-Use
- Wind Systems Integration
- Wind Data Management
- Distributed Wind
- Energy Equity & Health
- Environmental Monitoring for Marine Energy
- Marine Biofouling and Corrosion
- Marine Energy Resource Characterization
- Testing for Marine Energy
- The Blue Economy
- Environmental Performance of Hydropower
- Hydropower Cybersecurity and Digitalization
- Hydropower and the Electric Grid
- Materials Science for Hydropower
- Pumped Storage Hydropower
- Water + Hydropower Planning
- Grid Integration of Renewable Energy
- Geothermal Energy
- Algal Biofuels
- Aviation Biofuels
- Waste-to-Energy and Products
- Hydrogen & Fuel Cells
- Emission Control
- Energy-Efficient Mobility Systems
- Lightweight Materials
- Vehicle Electrification
- Vehicle Grid Integration
- Contraband Detection
- Pathogen Science & Detection
- Explosives Detection
- Threat-Agnostic Biodefense
- Discovery and Insight
- Proactive Defense
- Trusted Systems
- Nuclear Material Science
- Radiological & Nuclear Detection
- Nuclear Forensics
- Ultra-Sensitive Nuclear Measurements
- Nuclear Explosion Monitoring
- Global Nuclear & Radiological Security
- Disaster Recovery
- Global Collaborations
- Legislative and Regulatory Analysis
- Technical Training
- Additive Manufacturing
- Deployed Technologies
- Rapid Prototyping
- Systems Engineering
- 5G Security
- RF Signal Detection & Exploitation
- Climate Security
- Internet of Things
- Maritime Security
- Millimeter Wave
- Artificial Intelligence
- Graph and Data Analytics
- Software Engineering
- Computational Mathematics & Statistics
- High-Performance Computing
- Adaptive Autonomous Systems
- Visual Analytics
- Lab Objectives
- Publications & Reports
- Featured Research
- Diversity, Equity, Inclusion & Accessibility
- Lab Leadership
- Lab Fellows
- Staff Accomplishments
- Undergraduate Students
- Graduate Students
- Post-graduate Students
- University Faculty
- University Partnerships
- K-12 Educators and Students
- STEM Workforce Development
- STEM Outreach
- Meet the Team
- Internships
- Regional Impact
- Philanthropy
- Volunteering
- Available Technologies
- Industry Partnerships
- Licensing & Technology Transfer
- Entrepreneurial Leave
- Visual Intellectual Property Search (VIPS)
- Atmospheric Radiation Measurement User Facility
- Electricity Infrastructure Operations Center
- Energy Sciences Center
- Environmental Molecular Sciences Laboratory
- Grid Storage Launchpad
- Institute for Integrated Catalysis
- Interdiction Technology and Integration Laboratory
- PNNL Portland Research Center
- PNNL Seattle Research Center
- PNNL-Sequim (Marine and Coastal Research)
- Radiochemical Processing Laboratory
- Shallow Underground Laboratory
Long-Duration Energy Storage Can’t Wait
PNNL robotics, advanced instrumentation, and flow battery expertise to accelerate battery advances in new Energy Storage Research Alliance hub

Wei Wang is the Deputy Director of the Energy Storage Research Alliance (ESRA), which brings together world-class researchers from four national laboratories and 12 universities to enable next-generation battery and energy storage discovery.
(Photograph by Andrea Starr | Pacific Northwest National Laboratory)
The public wish list for battery makers is pretty straightforward. People want batteries that work for days without needing to be recharged, don’t leak or catch fire, and provide reliable energy storage for many years.
Our currently available energy storage technology meets those needs for several categories of batteries. But as a nation, the United States has an urgent unmet need for safe and reliable long-duration energy storage on a massive scale. Fulfilling that need will require new kinds of batteries capable of routinely providing energy to our electric grid and hauling heavy freight long distances.
The Energy Storage Research Alliance (ESRA) , a new Department of Energy (DOE) Energy Innovation hub, will meet those needs by accelerating the discovery of new battery materials and chemistries that use Earth-abundant components and green manufacturing processes.
The ESRA hub, one of new two energy storage-focused hubs created by DOE, includes leadership from three national laboratories: Pacific Northwest National Laboratory (PNNL) , Lawrence Berkeley National Laboratory (Berkeley Lab), and Argonne National Laboratory, which serves as the hub’s headquarters. In addition, 12 universities will participate in ESRA research.
“The ESRA will provide a platform for us to deepen our fundamental research in cost-efficient battery materials and affordable technologies,” said PNNL’s Wei Wang , ESRA deputy director and director of PNNL’s Energy Storage Materials Initiative .
Now is the time
The DOE investment of up to $62.5 million over 5 years enables the ESRA hub to put into place the scientists, tools, and emerging technologies to rapidly identify the most promising science-based approaches to large-scale energy storage.
“In the last decade, our scientific understanding of how to store and release energy in chemical bonds has advanced dramatically,” said Wang. “Now is the time to accelerate that fundamental understanding of the materials, chemistries, and properties that show promise in long-duration energy storage. Working with our partners, PNNL will leverage its investments in redox flow battery technology, high-throughput robotics, nuclear magnetic resonance spectroscopy, and the scientific acumen of our people.”
Long-duration grid energy storage expertise
As our electric grid decarbonizes and comes to depend more and more on these intermittent energy sources, safe, dependable long-term energy storage becomes essential. PNNL battery experts have established scientific and technical prowess, and many patented advances, in one of the most promising ways to store intermittent energy: redox flow battery science.
Wang, a global leader in flow battery technology, and his PNNL colleagues are developing an accelerated approach to discovery of even more efficient and longer-lasting flow battery materials for grid applications. In 2023, his research team provided the first lab-scale demonstration of a flow battery working stably and reliably for more than a year.
Through ESRA, Wang and his colleagues plan to explore a vastly increased number of new battery materials and chemistries, coupled with artificial intelligence, to learn faster and eliminate dead ends and blind alleys in their search.
“The ESRA hub builds upon PNNL’s past projects and capabilities for fundamental science in energy storage, which have grown and matured with DOE Office of Science support,” said Karl Mueller , director of program development for Physical and Computational Sciences at PNNL.
A molecular digital twin
To speed their effort, the scientists will deploy two tireless colleagues that are always available for more experiments. Dubbed Albert and Beverly, these two custom-built units are part robot, part workstation, part intelligent database. These lab dynamos have already sped the PNNL team’s pace of new battery materials discovery. Now, PNNL scientists will take them to a new level, collaborating with Argonne’s artificial intelligence technologies. PNNL will also partner with scientists at Berkeley Lab who have a similar experimental system to look at solid-state batteries. The efforts will complement each other in the new ESRA hub, said Wang. Together, the team will be able to further accelerate material discovery and move to predictive material design through machine learning insights.
“We can use machine learning to correlate structures to their properties,” said Wang. “If the machine learning algorithm learns enough from those data, then the next time we modify a new structure or come up with a new structure, the algorithm would be able to predict with high fidelity whether that new structure would have properties of interest.”
In this way, the scientific team can quickly move on from unpromising materials and focus on more productive ideas and prototypes. This combination of the robotic workstation and the machine learning algorithms make a sort of science-based molecular digital twin. The concept of a digital twin is well known in manufacturing, where digital prototypes guide real-world industrial design and manufacturing. Here, the team will extend that concept to their scientific discovery work.
“We know that chemical synthesis and experimental testing are the most time- and labor-intensive steps,” said Wang. “The molecular digital twin will help us be more efficient with time and resources.”
In addition to the digital twin, PNNL has signature characterization tools that will be housed in PNNL’s Grid Storage Launchpad, a new facility dedicated for energy storage research that opened last month.
Advanced nuclear magnetic resonance spectroscopy
Once a promising energy storage prototype is made, the research team will evaluate its ability to efficiently store energy, maintain its ability to charge and discharge, and be long-lasting. Researchers at PNNL have developed a unique facility, housed in PNNL’s Energy Sciences Center , to “watch” experimental energy storage systems in action.
Dynamic nuclear polarization solid-state nuclear magnetic resonance allows scientists to obtain signals from a wider range of materials in a dynamic environment close to surfaces that are important for the movement of mass or charge in a battery. This capability, along with specialized sample chambers developed at PNNL, allows scientists to track the movement of ions—the energy carriers—as they move within a liquid. In addition, the scientists will watch liquids interacting with both positive and negative electrodes. These interfaces are where many battery systems run into problems. Understanding the dynamic there is a huge endeavor.
“One of the biggest challenges in understanding complex chemistries found in energy storage systems is being able to track movement of the energy carriers and how they interact with the other elements of the system,” said Vijay Murugesan , a PNNL materials science expert and scientific thrust lead of the new ESRA hub. “We have developed the scientific and technical capabilities to track these energy storage molecules in real time, using advanced nuclear magnetic resonance spectroscopy.”
Wang added, “Achieving ESRA goals requires a team science approach, and we are committed to moving forward not only to achieve scientific goals, but also to train the next generation of energy storage research scientists and engineers with diverse backgrounds. Our partnerships with 12 research universities will help us accomplish that goal.”
The Energy Storage Research Alliance (ESRA), a DOE Energy Innovation hub led by Argonne National Laboratory, brings together world-class researchers from four national laboratories and 12 universities to enable next-generation battery and energy storage discovery. ESRA will enable transformative discoveries in materials chemistry, gain a fundamental understanding of electrochemical phenomena, lay the scientific foundations for breakthroughs in energy storage technologies, and train a next-generation battery workforce to ensure U.S. scientific and economic leadership.
Pacific Northwest National Laboratory draws on its distinguishing strengths in chemistry , Earth sciences , biology and data science to advance scientific knowledge and address challenges in sustainable energy and national security . Founded in 1965, PNNL is operated by Battelle for the Department of Energy’s Office of Science, which is the single largest supporter of basic research in the physical sciences in the United States. DOE’s Office of Science is working to address some of the most pressing challenges of our time. For more information, visit https://www.energy.gov/science/ . For more information on PNNL, visit PNNL's News Center . Follow us on Twitter , Facebook , LinkedIn and Instagram .
Published: September 3, 2024
Research topics
Data engineer/ Scientist
Organisational context and job purpose
The INEOS Energy Trading (IET) team forms part of the INEOS Energy group which combines the company’s fully integrated oil and gas exploration and production operations with its clean energy research and development activities.
IET is responsible for trading the gas production from INEOS’ upstream assets in the North Sea and also provides access to market for its internal businesses in countries across Europe with a significant overall energy demand (gas, financial power, carbon).
The primary role of the IET team is to ensure that energy trading and portfolio benefits are optimised for both the upstream and downstream parts of the INEOS business. This role is integral to further strengthening the analytical and trading capabilities of IET with a view to increasing trading activity in existing INEOS energy markets (gas, oil, carbon & LNG). This role will also be central to the aim of IET to maintain the position as a centre of excellence on all energy markets for the INEOS group.
Reporting directly into the Energy Trading Analytics manager, the successful candidate will take a prime role within the Analytics team delivering support to the trading team, senior management, as well the wider INEOS business.
Responsibilities And Accountabilities
- Help to drive forward AI and machine learning capabilities for Ineos Energy Trading.
- You will be a crucial part of the analytical team, enabling the use of market pricing and fundamental data to help the trading desk analysis for UK and Continental gas, prompt and curve, Carbon, LNG and Oil.
- Helping to build and maintain a comprehensive database (both historical and real-time) and leverage this data by designing and maintain industry-leading forecasting solutions with a focus on energy commodity price dynamics.
- Supporting the development of energy price forecasting models including AI based trading strategies.
- Analysing and challenging modelling methodologies and suggesting improvements.
- Working with third party vendors to supply data. Conduct exploratory and investigative analysis on new data sources.
- Building and maintaining a dashboard for analytical and trading team.
- Supporting ad-hoc analysis for trading desk and wider INEOS business.
Required Background
- Bachelor’s or master’s in mathematics, finance, statistics, engineering, computer science or related field
- Strong analytical skills, programming skills (Python preferred), ability to build/validate models and apply data science & machine learning to operational topics
- Experience in the energy sector would be beneficial but not essential (Ideally gas, power, carbon)
- Experience of getting the best results from messy data sets
Technical Skills
- Strong Python coding skills
- Attention to detail / thirst for real answers from data (how, why, what, when)
- Build / maintain ETL processes to feed IET’s data warehouse from various source APIs
- Experience with traditional statistical and ML-based methods for time-series modelling
- Experience in working multiple data types, including time series data
- Experience with widely used data science toolkits of Python (NumPy, pandas, scikit-learn, TensorFlow, tidyverse, mlr)
- Data visualization and dashboarding skills (e.g. Power BI, R Shiny)
- Experience of working on projects within the cloud (e.g. AWS)
- Experience of data storage platforms (SQL, NoSQL, Map-Reduce frameworks, etc.
- Experience presenting data visually (Plotly, D3, Tableau)
- Apply machine learning models / optimization in creative ways to heterogeneous data sets
Bahavioural Skills
- Analytical thinker and a commercial mind set
- Strong interpersonal skills and the ability to communicate with both business and technical minded colleagues at all levels
- Versatile worker who enjoys working within a team environment
- High energy levels and a hands-on approach
Closing date for applications: 9th September 2024
We use cookies to make sure you get the most from our website. Need to know more?
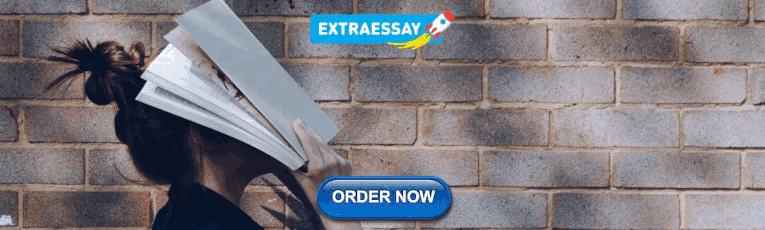
COMMENTS
Data Science-Related Research Topics. Developing machine learning models for real-time fraud detection in online transactions. The use of big data analytics in predicting and managing urban traffic flow. Investigating the effectiveness of data mining techniques in identifying early signs of mental health issues from social media usage.
As a result, cybersecurity is a crucial data science research area and one that will only become more important in the years to come. 23.) Blockchain. Blockchain is an incredible new research topic in data science for several reasons. First, it is a distributed database technology that enables secure, transparent, and tamper-proof transactions.
A data science research paper should start with a clear goal, stating what the study aims to investigate or achieve. This objective guides the entire paper, helping readers understand the purpose and direction of the research. 2. Detailed Methodology. Explaining how the research was conducted is crucial.
As we explore the depths of machine learning, natural language processing, big data analytics, and ethical considerations, we pave the way for innovation, shape the future of technology, and make a positive impact on the world. Discover exciting 99+ data science research topics and methodologies in this in-depth blog.
In this article, we have listed 10 such research and thesis topic ideas to take up as data science projects in 2022. Handling practical video analytics in a distributed cloud: With increased dependency on the internet, sharing videos has become a mode of data and information exchange. The role of the implementation of the Internet of Things ...
1. Introduction. Statistics and data science are more popular than ever in this era of data explosion and technological advances. Decades ago, John Tukey (Brillinger, 2014) said, "The best thing about being a statistician is that you get to play in everyone's backyard."More recently, Xiao-Li Meng (2009) said, "We no longer simply enjoy the privilege of playing in or cleaning up everyone ...
Abstract. To drive progress in the field of data science, we propose 10 challenge areas for the research community to pursue. Since data science is broad, with methods drawing from computer science, statistics, and other disciplines, and with applications appearing in all sectors, these challenge areas speak to the breadth of issues spanning ...
E ven though Big data is in the mainstream of operations as of 2020, there are still potential issues or challenges the researchers can address. Some of these issues overlap with the data science field. In this article, the top 20 interesting latest research problems in the combination of big data and data science are covered based on my personal experience (with due respect to the ...
Research Areas | Data Science. The world is being transformed by data and data-driven analysis is rapidly becoming an integral part of science and society. Stanford Data Science is a collaborative effort across many departments in all seven schools. We strive to unite existing data science research initiatives and create interdisciplinary ...
These 15 topics will help you to dive into interesting research. You may even build on research done by other scholars. Evaluate the data mining process. The influence of the various dimension reduction methods and techniques. The best data classification methods. The simple linear regression modeling methods.
J.M. Wing, " Ten Research Challenge Areas in Data Science," Voices, Data Science Institute, Columbia University, January 2, 2020. arXiv:2002.05658. Jeannette M. Wing is Avanessians Director of the Data Science Institute and professor of computer science at Columbia University. December 30, 2019.
A Data Science Dissertation is a research project where students explore the vast field of data science. This involves analyzing large sets of data, creating models, and finding patterns to solve problems or make decisions. In a data science dissertation, you might work on topics like machine learning, big data analytics, or predictive modeling.
These research papers consist of different data science topics including the present fast passed technologies such as AI, ML, Coding, and many others. Data Science plays a very major role in applying AI, ML, and Coding. With the help of data science, we can improve our applications in various sectors. Here are the Data Science Research Papers ...
The Data Science Life Cycle. End-to-end projects involve real-world problems which you solve using the 6 stages of the data science life cycle: Business understanding. Data understanding. Data preparation. Modeling. Validation. Deployment. Here's how to execute a data science project from end to end in more detail.
Apr 5, 2021. 49. Starting a data science research project can be challenging, whether you're a novice or a seasoned engineer — you want your project to be meaningful, accessible, and valuable to the data science community and your portfolio. In this post, I'll introduce two frameworks you can use as a guide for your data science research ...
Finance: Fraud detection, risk management, and algorithmic trading. Technology: Natural language processing, image recognition, and recommendation systems. Environmental Science: Climate modeling, predicting natural disasters, and analyzing environmental data. In summary, data science is a powerful discipline that leverages data-driven ...
These ideas have been drawn from the 8 v's of big data namely Volume, Value, Veracity, Visualization, Variety, Velocity, Viscosity, and Virility that provide interesting and challenging research areas for prospective researches in their masters or PhD thesis . Overall, the general big data research topics can be divided into distinct ...
There are so many methods and techniques to perform dimension reduction. The most popular of them are Missing Values, Low Variance, Decision Trees, Random Forest, High Correlation, Factor Analysis, Principal Component Analysis, Backward Feature Elimination. 4. Classification.
Step-by-Step Instructions. Connect to the Data Science Stack Exchange database and explore its structure. Write SQL queries to extract data on questions, tags, and view counts. Use pandas to clean the extracted data and prepare it for analysis. Analyze the distribution of questions across different tags and topics.
Ten Research Challenge Areas in Data Science. To drive progress in the field of data science, we propose 10 challenge areas for the research community to pursue. Since data science is broad, with methods drawing from computer science, statistics, and other disciplines, and with applications appearing in all sectors, these challenge areas speak ...
Data Science Projects involve using data to solve real-world problems and find new solutions. They are great for beginners who want to add work to their resume, especially if you're a final-year student.Data Science is a hot career in 2024, and by building data science projects you can start to gain industry insights.. Think about predicting movie ratings or analyzing trends in social media ...
Here are the 8 fastest-growing data science trends for 2024 and beyond. We'll also outline how these trends will impact both data scientists' work and everyday life. Whether you're actively involved in the data science community, or just concerned about your data privacy, these are the top trends to know. 1. Generative AI use continues to grow.
31+ Data Science Project Ideas: For Beginner to Advance Level . Here are the best Data Science Project Ideas For Beginner to Advance Level . Beginner Projects. Titanic Survival Prediction. Description: Make a model to guess which Titanic passengers survived based on old data. You'll clean the data, choose important features, and use simple ...
The Digital Pulpit: A Nationwide Analysis of Online Sermons. This Pew Research Center analysis harnesses computational techniques to identify, collect and analyze the sermons that U.S. churches livestream or share on their websites each week. short readsDec 4, 2019.
Aalto University offers free training in topics broadly related to research data management (RDM) and open science twice a year. Lecturers include our Data Agents, legal counsels, and even a few guest experts. ... acquire new insights, and enhance existing knowledge on open science and research. Our webinars remain free and accessible to all ...
In the ever-evolving field of data science, selecting the right tools and technologies is crucial to the success of any project. With numerous options available—from programming languages and data processing frameworks to visualization tools and machine learning libraries—making informed decisions can greatly impact your project's outcomes.
Computational data-centric research techniques play a prevalent and multi-disciplinary role in life science research. In the past, scientists in wet labs generated the data, and computational researchers focused on creating tools for the analysis of those data. Computational researchers are now beco …
I'm getting enough of the pieces of AgentM in place that I'm able to get it to do useful things. I wrote a small program (ok AgentM wrote part of it) that fetches the last days worth of research papers from arxiv.org, filters them to the papers related to topics I care about, and then projects those filtered papers to a uniform markdown format for easy scanning: It uses gpt-4o-mini so it ...
Wang added, "Achieving ESRA goals requires a team science approach, and we are committed to moving forward not only to achieve scientific goals, but also to train the next generation of energy storage research scientists and engineers with diverse backgrounds. Our partnerships with 12 research universities will help us accomplish that goal."
Required Background Bachelor's or master's in mathematics, finance, statistics, engineering, computer science or related field Strong analytical skills, programming skills (Python preferred), ability to build/validate models and apply data science & machine learning to operational topics Experience in the energy sector would be beneficial ...