
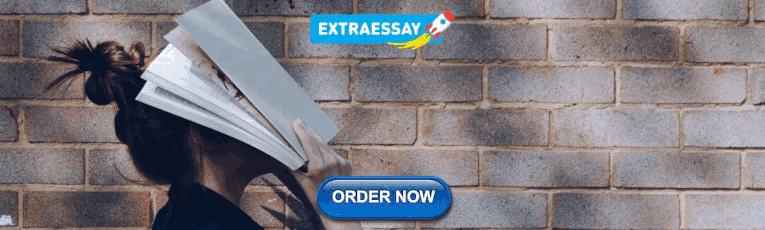
Identifying Empirical Research Articles
Identifying empirical articles.
- Searching for Empirical Research Articles
What is Empirical Research?
An empirical research article reports the results of a study that uses data derived from actual observation or experimentation. Empirical research articles are examples of primary research. To learn more about the differences between primary and secondary research, see our related guide:
- Primary and Secondary Sources
By the end of this guide, you will be able to:
- Identify common elements of an empirical article
- Use a variety of search strategies to search for empirical articles within the library collection
Look for the IMRaD layout in the article to help identify empirical research. Sometimes the sections will be labeled differently, but the content will be similar.
- I ntroduction: why the article was written, research question or questions, hypothesis, literature review
- M ethods: the overall research design and implementation, description of sample, instruments used, how the authors measured their experiment
- R esults: output of the author's measurements, usually includes statistics of the author's findings
- D iscussion: the author's interpretation and conclusions about the results, limitations of study, suggestions for further research
Parts of an Empirical Research Article
Parts of an empirical article.
The screenshots below identify the basic IMRaD structure of an empirical research article.
Introduction
The introduction contains a literature review and the study's research hypothesis.

The method section outlines the research design, participants, and measures used.

Results
The results section contains statistical data (charts, graphs, tables, etc.) and research participant quotes.

The discussion section includes impacts, limitations, future considerations, and research.

Learn the IMRaD Layout: How to Identify an Empirical Article
This short video overviews the IMRaD method for identifying empirical research.
- Next: Searching for Empirical Research Articles >>
- Last Updated: Nov 16, 2023 8:24 AM
CityU Home - CityU Catalog

- USC Libraries
- Research Guides
- Identify Empirical Articles
*Education: Identify Empirical Articles
- Google Scholar tips and instructions
- Newspaper Articles
- Video Tutorials
- EDUC 508 Library Session
- Statistics and Data
- Tests & Measurements
- Citation Managers
- APA Style This link opens in a new window
- Scan & Deliver and Interlibrary Loan
- Educational Leadership
- Global Executive Ed.D.
- Marriage & Family Therapy
- Organizational Change & Leadership
- Literacy Education
- Accreditation
- Journal Ranking Metrics
- Publishing Your Research
- Education and STEM Databases
How to Recognize Empirical Journal Articles
Definition of an empirical study: An empirical research article reports the results of a study that uses data derived from actual observation or experimentation. Empirical research articles are examples of primary research.
Parts of a standard empirical research article: (articles will not necessary use the exact terms listed below.)
- Abstract ... A paragraph length description of what the study includes.
- Introduction ...Includes a statement of the hypotheses for the research and a review of other research on the topic.
- Who are participants
- Design of the study
- What the participants did
- What measures were used
- Results ...Describes the outcomes of the measures of the study.
- Discussion ...Contains the interpretations and implications of the study.
- References ...Contains citation information on the material cited in the report. (also called bibliography or works cited)
Characteristics of an Empirical Article:
- Empirical articles will include charts, graphs, or statistical analysis.
- Empirical research articles are usually substantial, maybe from 8-30 pages long.
- There is always a bibliography found at the end of the article.
Type of publications that publish empirical studies:
- Empirical research articles are published in scholarly or academic journals
- These journals are also called “peer-reviewed,” or “refereed” publications.
Examples of such publications include:
- American Educational Research Journal
- Computers & Education
- Journal of Educational Psychology
Databases that contain empirical research: (selected list only)
- List of other useful databases by subject area
This page is adapted from Eric Karkhoff's Sociology Research Guide: Identify Empirical Articles page (Cal State Fullerton Pollak Library).
Sample Empirical Articles
Roschelle, J., Feng, M., Murphy, R. F., & Mason, C. A. (2016). Online Mathematics Homework Increases Student Achievement. AERA Open . ( L INK TO ARTICLE )
Lester, J., Yamanaka, A., & Struthers, B. (2016). Gender microaggressions and learning environments: The role of physical space in teaching pedagogy and communication. Community College Journal of Research and Practice , 40(11), 909-926. ( LINK TO ARTICLE )
- << Previous: Newspaper Articles
- Next: Workshops and Webinars >>
- Last Updated: Aug 15, 2024 2:42 PM
- URL: https://libguides.usc.edu/education
Penn State University Libraries
Empirical research in the social sciences and education.
- What is Empirical Research and How to Read It
- Finding Empirical Research in Library Databases
- Designing Empirical Research
- Ethics, Cultural Responsiveness, and Anti-Racism in Research
- Citing, Writing, and Presenting Your Work
Contact the Librarian at your campus for more help!

Introduction: What is Empirical Research?
Empirical research is based on observed and measured phenomena and derives knowledge from actual experience rather than from theory or belief.
How do you know if a study is empirical? Read the subheadings within the article, book, or report and look for a description of the research "methodology." Ask yourself: Could I recreate this study and test these results?
Key characteristics to look for:
- Specific research questions to be answered
- Definition of the population, behavior, or phenomena being studied
- Description of the process used to study this population or phenomena, including selection criteria, controls, and testing instruments (such as surveys)
Another hint: some scholarly journals use a specific layout, called the "IMRaD" format, to communicate empirical research findings. Such articles typically have 4 components:
- Introduction: sometimes called "literature review" -- what is currently known about the topic -- usually includes a theoretical framework and/or discussion of previous studies
- Methodology: sometimes called "research design" -- how to recreate the study -- usually describes the population, research process, and analytical tools used in the present study
- Results: sometimes called "findings" -- what was learned through the study -- usually appears as statistical data or as substantial quotations from research participants
- Discussion: sometimes called "conclusion" or "implications" -- why the study is important -- usually describes how the research results influence professional practices or future studies
Reading and Evaluating Scholarly Materials
Reading research can be a challenge. However, the tutorials and videos below can help. They explain what scholarly articles look like, how to read them, and how to evaluate them:
- CRAAP Checklist A frequently-used checklist that helps you examine the currency, relevance, authority, accuracy, and purpose of an information source.
- IF I APPLY A newer model of evaluating sources which encourages you to think about your own biases as a reader, as well as concerns about the item you are reading.
- Credo Video: How to Read Scholarly Materials (4 min.)
- Credo Tutorial: How to Read Scholarly Materials
- Credo Tutorial: Evaluating Information
- Credo Video: Evaluating Statistics (4 min.)
- Credo Tutorial: Evaluating for Diverse Points of View
- Next: Finding Empirical Research in Library Databases >>
- Last Updated: Aug 13, 2024 3:16 PM
- URL: https://guides.libraries.psu.edu/emp

Free APA Journals ™ Articles
Recently published articles from subdisciplines of psychology covered by more than 90 APA Journals™ publications.
For additional free resources (such as article summaries, podcasts, and more), please visit the Highlights in Psychological Research page.
- Basic / Experimental Psychology
- Clinical Psychology
- Core of Psychology
- Developmental Psychology
- Educational Psychology, School Psychology, and Training
- Forensic Psychology
- Health Psychology & Medicine
- Industrial / Organizational Psychology & Management
- Neuroscience & Cognition
- Social Psychology & Social Processes
- Moving While Black: Intergroup Attitudes Influence Judgments of Speed (PDF, 71KB) Journal of Experimental Psychology: General February 2016 by Andreana C. Kenrick, Stacey Sinclair, Jennifer Richeson, Sara C. Verosky, and Janetta Lun
- Recognition Without Awareness: Encoding and Retrieval Factors (PDF, 116KB) Journal of Experimental Psychology: Learning, Memory, and Cognition September 2015 by Fergus I. M. Craik, Nathan S. Rose, and Nigel Gopie
- The Tip-of-the-Tongue Heuristic: How Tip-of-the-Tongue States Confer Perceptibility on Inaccessible Words (PDF, 91KB) Journal of Experimental Psychology: Learning, Memory, and Cognition September 2015 by Anne M. Cleary and Alexander B. Claxton
- Cognitive Processes in the Breakfast Task: Planning and Monitoring (PDF, 146KB) Canadian Journal of Experimental Psychology / Revue canadienne de psychologie expérimentale September 2015 by Nathan S. Rose, Lin Luo, Ellen Bialystok, Alexandra Hering, Karen Lau, and Fergus I. M. Craik
- Searching for Explanations: How the Internet Inflates Estimates of Internal Knowledge (PDF, 138KB) Journal of Experimental Psychology: General June 2015 by Matthew Fisher, Mariel K. Goddu, and Frank C. Keil
- Client Perceptions of Corrective Experiences in Cognitive Behavioral Therapy and Motivational Interviewing for Generalized Anxiety Disorder: An Exploratory Pilot Study (PDF, 62KB) Journal of Psychotherapy Integration March 2017 by Jasmine Khattra, Lynne Angus, Henny Westra, Christianne Macaulay, Kathrin Moertl, and Michael Constantino
- Attention-Deficit/Hyperactivity Disorder Developmental Trajectories Related to Parental Expressed Emotion (PDF, 160KB) Journal of Abnormal Psychology February 2016 by Erica D. Musser, Sarah L. Karalunas, Nathan Dieckmann, Tara S. Peris, and Joel T. Nigg
- The Integrated Scientist-Practitioner: A New Model for Combining Research and Clinical Practice in Fee-For-Service Settings (PDF, 58KB) Professional Psychology: Research and Practice December 2015 by Jenna T. LeJeune and Jason B. Luoma
- Psychotherapists as Gatekeepers: An Evidence-Based Case Study Highlighting the Role and Process of Letter Writing for Transgender Clients (PDF, 76KB) Psychotherapy September 2015 by Stephanie L. Budge
- Perspectives of Family and Veterans on Family Programs to Support Reintegration of Returning Veterans With Posttraumatic Stress Disorder (PDF, 70KB) Psychological Services August 2015 by Ellen P. Fischer, Michelle D. Sherman, Jean C. McSweeney, Jeffrey M. Pyne, Richard R. Owen, and Lisa B. Dixon
- "So What Are You?": Inappropriate Interview Questions for Psychology Doctoral and Internship Applicants (PDF, 79KB) Training and Education in Professional Psychology May 2015 by Mike C. Parent, Dana A. Weiser, and Andrea McCourt
- Cultural Competence as a Core Emphasis of Psychoanalytic Psychotherapy (PDF, 81KB) Psychoanalytic Psychology April 2015 by Pratyusha Tummala-Narra
- The Role of Gratitude in Spiritual Well-Being in Asymptomatic Heart Failure Patients (PDF, 123KB) Spirituality in Clinical Practice March 2015 by Paul J. Mills, Laura Redwine, Kathleen Wilson, Meredith A. Pung, Kelly Chinh, Barry H. Greenberg, Ottar Lunde, Alan Maisel, Ajit Raisinghani, Alex Wood, and Deepak Chopra
- Nepali Bhutanese Refugees Reap Support Through Community Gardening (PDF, 104KB) International Perspectives in Psychology: Research, Practice, Consultation January 2017 by Monica M. Gerber, Jennifer L. Callahan, Danielle N. Moyer, Melissa L. Connally, Pamela M. Holtz, and Beth M. Janis
- Does Monitoring Goal Progress Promote Goal Attainment? A Meta-Analysis of the Experimental Evidence (PDF, 384KB) Psychological Bulletin February 2016 by Benjamin Harkin, Thomas L. Webb, Betty P. I. Chang, Andrew Prestwich, Mark Conner, Ian Kellar, Yael Benn, and Paschal Sheeran
- Youth Violence: What We Know and What We Need to Know (PDF, 388KB) American Psychologist January 2016 by Brad J. Bushman, Katherine Newman, Sandra L. Calvert, Geraldine Downey, Mark Dredze, Michael Gottfredson, Nina G. Jablonski, Ann S. Masten, Calvin Morrill, Daniel B. Neill, Daniel Romer, and Daniel W. Webster
- Supervenience and Psychiatry: Are Mental Disorders Brain Disorders? (PDF, 113KB) Journal of Theoretical and Philosophical Psychology November 2015 by Charles M. Olbert and Gary J. Gala
- Constructing Psychological Objects: The Rhetoric of Constructs (PDF, 108KB) Journal of Theoretical and Philosophical Psychology November 2015 by Kathleen L. Slaney and Donald A. Garcia
- Expanding Opportunities for Diversity in Positive Psychology: An Examination of Gender, Race, and Ethnicity (PDF, 119KB) Canadian Psychology / Psychologie canadienne August 2015 by Meghana A. Rao and Stewart I. Donaldson
- Racial Microaggression Experiences and Coping Strategies of Black Women in Corporate Leadership (PDF, 132KB) Qualitative Psychology August 2015 by Aisha M. B. Holder, Margo A. Jackson, and Joseph G. Ponterotto
- An Appraisal Theory of Empathy and Other Vicarious Emotional Experiences (PDF, 151KB) Psychological Review July 2015 by Joshua D. Wondra and Phoebe C. Ellsworth
- An Attachment Theoretical Framework for Personality Disorders (PDF, 100KB) Canadian Psychology / Psychologie canadienne May 2015 by Kenneth N. Levy, Benjamin N. Johnson, Tracy L. Clouthier, J. Wesley Scala, and Christina M. Temes
- Emerging Approaches to the Conceptualization and Treatment of Personality Disorder (PDF, 111KB) Canadian Psychology / Psychologie canadienne May 2015 by John F. Clarkin, Kevin B. Meehan, and Mark F. Lenzenweger
- A Complementary Processes Account of the Development of Childhood Amnesia and a Personal Past (PDF, 585KB) Psychological Review April 2015 by Patricia J. Bauer
- Terminal Decline in Well-Being: The Role of Social Orientation (PDF, 238KB) Psychology and Aging March 2016 by Denis Gerstorf, Christiane A. Hoppmann, Corinna E. Löckenhoff, Frank J. Infurna, Jürgen Schupp, Gert G. Wagner, and Nilam Ram
- Student Threat Assessment as a Standard School Safety Practice: Results From a Statewide Implementation Study (PDF, 97KB) School Psychology Quarterly June 2018 by Dewey Cornell, Jennifer L. Maeng, Anna Grace Burnette, Yuane Jia, Francis Huang, Timothy Konold, Pooja Datta, Marisa Malone, and Patrick Meyer
- Can a Learner-Centered Syllabus Change Students’ Perceptions of Student–Professor Rapport and Master Teacher Behaviors? (PDF, 90KB) Scholarship of Teaching and Learning in Psychology September 2016 by Aaron S. Richmond, Jeanne M. Slattery, Nathanael Mitchell, Robin K. Morgan, and Jared Becknell
- Adolescents' Homework Performance in Mathematics and Science: Personal Factors and Teaching Practices (PDF, 170KB) Journal of Educational Psychology November 2015 by Rubén Fernández-Alonso, Javier Suárez-Álvarez, and José Muñiz
- Teacher-Ready Research Review: Clickers (PDF, 55KB) Scholarship of Teaching and Learning in Psychology September 2015 by R. Eric Landrum
- Enhancing Attention and Memory During Video-Recorded Lectures (PDF, 83KB) Scholarship of Teaching and Learning in Psychology March 2015 by Daniel L. Schacter and Karl K. Szpunar
- The Alleged "Ferguson Effect" and Police Willingness to Engage in Community Partnership (PDF, 70KB) Law and Human Behavior February 2016 by Scott E. Wolfe and Justin Nix
- Randomized Controlled Trial of an Internet Cognitive Behavioral Skills-Based Program for Auditory Hallucinations in Persons With Psychosis (PDF, 92KB) Psychiatric Rehabilitation Journal September 2017 by Jennifer D. Gottlieb, Vasudha Gidugu, Mihoko Maru, Miriam C. Tepper, Matthew J. Davis, Jennifer Greenwold, Ruth A. Barron, Brian P. Chiko, and Kim T. Mueser
- Preventing Unemployment and Disability Benefit Receipt Among People With Mental Illness: Evidence Review and Policy Significance (PDF, 134KB) Psychiatric Rehabilitation Journal June 2017 by Bonnie O'Day, Rebecca Kleinman, Benjamin Fischer, Eric Morris, and Crystal Blyler
- Sending Your Grandparents to University Increases Cognitive Reserve: The Tasmanian Healthy Brain Project (PDF, 88KB) Neuropsychology July 2016 by Megan E. Lenehan, Mathew J. Summers, Nichole L. Saunders, Jeffery J. Summers, David D. Ward, Karen Ritchie, and James C. Vickers
- The Foundational Principles as Psychological Lodestars: Theoretical Inspiration and Empirical Direction in Rehabilitation Psychology (PDF, 68KB) Rehabilitation Psychology February 2016 by Dana S. Dunn, Dawn M. Ehde, and Stephen T. Wegener
- Feeling Older and Risk of Hospitalization: Evidence From Three Longitudinal Cohorts (PDF, 55KB) Health Psychology Online First Publication — February 11, 2016 by Yannick Stephan, Angelina R. Sutin, and Antonio Terracciano
- Anger Intensification With Combat-Related PTSD and Depression Comorbidity (PDF, 81KB) Psychological Trauma: Theory, Research, Practice, and Policy January 2016 by Oscar I. Gonzalez, Raymond W. Novaco, Mark A. Reger, and Gregory A. Gahm
- Special Issue on eHealth and mHealth: Challenges and Future Directions for Assessment, Treatment, and Dissemination (PDF, 32KB) Health Psychology December 2015 by Belinda Borrelli and Lee M. Ritterband
- Posttraumatic Growth Among Combat Veterans: A Proposed Developmental Pathway (PDF, 110KB) Psychological Trauma: Theory, Research, Practice, and Policy July 2015 by Sylvia Marotta-Walters, Jaehwa Choi, and Megan Doughty Shaine
- Racial and Sexual Minority Women's Receipt of Medical Assistance to Become Pregnant (PDF, 111KB) Health Psychology June 2015 by Bernadette V. Blanchfield and Charlotte J. Patterson
- An Examination of Generational Stereotypes as a Path Towards Reverse Ageism (PDF, 205KB) The Psychologist-Manager Journal August 2017 By Michelle Raymer, Marissa Reed, Melissa Spiegel, and Radostina K. Purvanova
- Sexual Harassment: Have We Made Any Progress? (PDF, 121KB) Journal of Occupational Health Psychology July 2017 By James Campbell Quick and M. Ann McFadyen
- Multidimensional Suicide Inventory-28 (MSI-28) Within a Sample of Military Basic Trainees: An Examination of Psychometric Properties (PDF, 79KB) Military Psychology November 2015 By Serena Bezdjian, Danielle Burchett, Kristin G. Schneider, Monty T. Baker, and Howard N. Garb
- Cross-Cultural Competence: The Role of Emotion Regulation Ability and Optimism (PDF, 100KB) Military Psychology September 2015 By Bianca C. Trejo, Erin M. Richard, Marinus van Driel, and Daniel P. McDonald
- The Effects of Stress on Prospective Memory: A Systematic Review (PDF, 149KB) Psychology & Neuroscience September 2017 by Martina Piefke and Katharina Glienke
- Don't Aim Too High for Your Kids: Parental Overaspiration Undermines Students' Learning in Mathematics (PDF, 164KB) Journal of Personality and Social Psychology November 2016 by Kou Murayama, Reinhard Pekrun, Masayuki Suzuki, Herbert W. Marsh, and Stephanie Lichtenfeld
- Sex Differences in Sports Interest and Motivation: An Evolutionary Perspective (PDF, 155KB) Evolutionary Behavioral Sciences April 2016 by Robert O. Deaner, Shea M. Balish, and Michael P. Lombardo
- Asian Indian International Students' Trajectories of Depression, Acculturation, and Enculturation (PDF, 210KB) Asian American Journal of Psychology March 2016 By Dhara T. Meghani and Elizabeth A. Harvey
- Cynical Beliefs About Human Nature and Income: Longitudinal and Cross-Cultural Analyses (PDF, 163KB) January 2016 Journal of Personality and Social Psychology by Olga Stavrova and Daniel Ehlebracht
- Annual Review of Asian American Psychology, 2014 (PDF, 384KB) Asian American Journal of Psychology December 2015 By Su Yeong Kim, Yishan Shen, Yang Hou, Kelsey E. Tilton, Linda Juang, and Yijie Wang
- Resilience in the Study of Minority Stress and Health of Sexual and Gender Minorities (PDF, 40KB) Psychology of Sexual Orientation and Gender Diversity September 2015 by Ilan H. Meyer
- Self-Reported Psychopathy and Its Association With Criminal Cognition and Antisocial Behavior in a Sample of University Undergraduates (PDF, 91KB) Canadian Journal of Behavioural Science / Revue canadienne des sciences du comportement July 2015 by Samantha J. Riopka, Richard B. A. Coupland, and Mark E. Olver
Journals Publishing Resource Center
Find resources for writing, reviewing, and editing articles for publishing with APA Journals™.
Visit the resource center
Journals information
- Frequently Asked Questions
- Journal statistics and operations data
- Special Issues
- Email alerts
- Copyright and permissions
Contact APA Publications

- Ask a Librarian
Research: Overview & Approaches
- Getting Started with Undergraduate Research
- Planning & Getting Started
- Building Your Knowledge Base
- Locating Sources
- Reading Scholarly Articles
- Creating a Literature Review
- Productivity & Organizing Research
- Scholarly and Professional Relationships
Introduction to Empirical Research
Databases for finding empirical research, guided search, google scholar, examples of empirical research, sources and further reading.
- Interpretive Research
- Action-Based Research
- Creative & Experimental Approaches
Your Librarian

- Introductory Video This video covers what empirical research is, what kinds of questions and methods empirical researchers use, and some tips for finding empirical research articles in your discipline.

- Guided Search: Finding Empirical Research Articles This is a hands-on tutorial that will allow you to use your own search terms to find resources.

- Study on radiation transfer in human skin for cosmetics
- Long-Term Mobile Phone Use and the Risk of Vestibular Schwannoma: A Danish Nationwide Cohort Study
- Emissions Impacts and Benefits of Plug-In Hybrid Electric Vehicles and Vehicle-to-Grid Services
- Review of design considerations and technological challenges for successful development and deployment of plug-in hybrid electric vehicles
- Endocrine disrupters and human health: could oestrogenic chemicals in body care cosmetics adversely affect breast cancer incidence in women?
- << Previous: Scholarly and Professional Relationships
- Next: Interpretive Research >>
- Last Updated: Aug 13, 2024 12:18 PM
- URL: https://guides.lib.purdue.edu/research_approaches

- University of La Verne
- Subject Guides
Identify Empirical Research Articles
- Interactive Tutorial
- Literature Matrix
- Guide to the Successful Thesis and Dissertation: A Handbook for Students and Faculty
- Practical Guide to the Qualitative Dissertation
- Guide to Writing Empirical Papers, Theses, and Dissertations
What is a Literature Review--YouTube
Literature Review Guides
- How to write a literature review
- The Literature Review:a few steps on conducting it Permission granted from Writing at the University of Toronto
- Six steps for writing a literature review This blog, written by Tanya Golash-Bozal PhD an Associate Professor of Sociology at the University of California at Merced, offers a very nice and simple advice on how to write a literature review from the point of view of an experience professional.
- The Writing Center, University of North Carolina at Chapel Hill Permission granted to use this guide.
- Writing Center University of North Carolina
- Literature Reviews Otago Polytechnic in New Zealand produced this guide and in my opinion, it is one of the best. NOTE: Except where otherwise noted, content on this site is licensed under a Creative Commons Attribution-NonCommercial-ShareAlike 4.0 International License. However, all images and Otago Polytechnic videos are copyrighted.
What Are Empirical Articles?
As a student at the University of La Verne, faculty may instruct you to read and analyze empirical articles when writing a research paper, a senior or master's project, or a doctoral dissertation. How can you recognize an empirical article in an academic discipline? An empirical research article is an article which reports research based on actual observations or experiments. The research may use quantitative research methods, which generate numerical data and seek to establish causal relationships between two or more variables.(1) Empirical research articles may use qualitative research methods, which objectively and critically analyze behaviors, beliefs, feelings, or values with few or no numerical data available for analysis.(2)
How can I determine if I have found an empirical article?
When looking at an article or the abstract of an article, here are some guidelines to use to decide if an article is an empirical article.
- Is the article published in an academic, scholarly, or professional journal? Popular magazines such as Business Week or Newsweek do not publish empirical research articles; academic journals such as Business Communication Quarterly or Journal of Psychology may publish empirical articles. Some professional journals, such as JAMA: Journal of the American Medical Association publish empirical research. Other professional journals, such as Coach & Athletic Director publish articles of professional interest, but they do not publish research articles.
- Does the abstract of the article mention a study, an observation, an analysis or a number of participants or subjects? Was data collected, a survey or questionnaire administered, an assessment or measurement used, an interview conducted? All of these terms indicate possible methodologies used in empirical research.
- Introduction -The introduction provides a very brief summary of the research.
- Methodology -The method section describes how the research was conducted, including who the participants were, the design of the study, what the participants did, and what measures were used.
- Results -The results section describes the outcomes of the measures of the study.
- Discussion -The discussion section contains the interpretations and implications of the study.
- Conclusion -
- References -A reference section contains information about the articles and books cited in the report and should be substantial.
- How long is the article? An empirical article is usually substantial; it is normally seven or more pages long.
When in doubt if an article is an empirical research article, share the article citation and abstract with your professor or a librarian so that we can help you become better at recognizing the differences between empirical research and other types of scholarly articles.
How can I search for empirical research articles using the electronic databases available through Wilson Library?
- A quick and somewhat superficial way to look for empirical research is to type your search terms into the database's search boxes, then type STUDY OR STUDIES in the final search box to look for studies on your topic area. Be certain to use the ability to limit your search to scholarly/professional journals if that is available on the database. Evaluate the results of your search using the guidelines above to determine if any of the articles are empirical research articles.
- In EbscoHost databases, such as Education Source , on the Advanced Search page you should see a PUBLICATION TYPE field; highlight the appropriate entry. Empirical research may not be the term used; look for a term that may be a synonym for empirical research. ERIC uses REPORTS-RESEARCH. Also find the field for INTENDED AUDIENCE and highlight RESEARCHER. PsycArticles and Psycinfo include a field for METHODOLOGY where you can highlight EMPIRICAL STUDY. National Criminal Justice Reference Service Abstracts has a field for DOCUMENT TYPE; highlight STUDIES/RESEARCH REPORTS. Then evaluate the articles you find using the guidelines above to determine if an article is empirical.
- In ProQuest databases, such as ProQuest Psychology Journals , on the Advanced Search page look under MORE SEARCH OPTIONS and click on the pull down menu for DOCUMENT TYPE and highlight an appropriate type, such as REPORT or EVIDENCE BASED. Also look for the SOURCE TYPE field and highlight SCHOLARLY JOURNALS. Evaluate the search results using the guidelines to determine if an article is empirical.
- Pub Med Central , Sage Premier , Science Direct , Wiley Interscience , and Wiley Interscience Humanities and Social Sciences consist of scholarly and professional journals which publish primarily empirical articles. After conducting a subject search in these databases, evaluate the items you find by using the guidelines above for deciding if an article is empirical.
- "Quantitative research" A Dictionary of Nursing. Oxford University Press, 2008. Oxford Reference Online. Oxford University Press. University of La Verne. 25 August 2009
- "Qualitative analysis" A Dictionary of Public Health. Ed. John M. Last, Oxford University Press, 2007. Oxford Reference Online . Oxford University Press. University of La Verne. 25 August 2009
Empirical Articles:Tips on Database Searching
- Identifying Empirical Articles
- Next: Interactive Tutorial >>
- Last Updated: Jul 29, 2024 7:29 PM
- URL: https://laverne.libguides.com/empirical-articles

Identify Empirical Research Articles
- What is empirical research?
- Finding empirical research in library databases
- Research design
- Need additional help?
Getting started
According to the APA , empirical research is defined as the following: "Study based on facts, systematic observation, or experiment, rather than theory or general philosophical principle." Empirical research articles are generally located in scholarly, peer-reviewed journals and often follow a specific layout known as IMRaD: 1) Introduction - This provides a theoretical framework and might discuss previous studies related to the topic at hand. 2) Methodology - This describes the analytical tools used, research process, and the populations included. 3) Results - Sometimes this is referred to as findings, and it typically includes statistical data. 4) Discussion - This can also be known as the conclusion to the study, this usually describes what was learned and how the results can impact future practices.
In addition to IMRaD, it's important to see a conclusion and references that can back up the author's claims.
Characteristics to look for
In addition to the IMRaD format mentioned above, empirical research articles contain several key characteristics for identification purposes:
- The length of empirical research is often substantial, usually eight to thirty pages long.
- You should see data of some kind, this includes graphs, charts, or some kind of statistical analysis.
- There is always a bibliography found at the end of the article.
Publications
Empirical research articles can be found in scholarly or academic journals. These types of journals are often referred to as "peer-reviewed" publications; this means qualified members of an academic discipline review and evaluate an academic paper's suitability in order to be published.
The CRAAP Checklist should be utilized to help you examine the currency, relevancy, authority, accuracy, and purpose of an information resource. This checklist was developed by California State University's Meriam Library .
This page has been adapted from the Sociology Research Guide: Identify Empirical Articles at Cal State Fullerton Pollak Library.
- << Previous: Home
- Next: Finding empirical research in library databases >>
- Last Updated: Feb 22, 2024 10:12 AM
- URL: https://paloaltou.libguides.com/empiricalresearch
Empirical Research
- Living reference work entry
- First Online: 22 May 2017
- Cite this living reference work entry
- Emeka Thaddues Njoku 2
544 Accesses
1 Citations
The term “empirical” entails gathered data based on experience, observations, or experimentation. In empirical research, knowledge is developed from factual experience as opposed to theoretical assumption and usually involved the use of data sources like datasets or fieldwork, but can also be based on observations within a laboratory setting. Testing hypothesis or answering definite questions is a primary feature of empirical research. Empirical research, in other words, involves the process of employing working hypothesis that are tested through experimentation or observation. Hence, empirical research is a method of uncovering empirical evidence.
Through the process of gathering valid empirical data, scientists from a variety of fields, ranging from the social to the natural sciences, have to carefully design their methods. This helps to ensure quality and accuracy of data collection and treatment. However, any error in empirical data collection process could inevitably render such...
This is a preview of subscription content, log in via an institution to check access.
Access this chapter
Institutional subscriptions
Bibliography
Bhattacherjee, A. (2012). Social science research: Principles, methods, and practices. Textbooks Collection . Book 3.
Google Scholar
Comte, A., & Bridges, J. H. (Tr.) (1865). A general view of positivism . Trubner and Co. (reissued by Cambridge University Press , 2009).
Dilworth, C. B. (1982). Empirical research in the literature class. English Journal, 71 (3), 95–97.
Article Google Scholar
Heisenberg, W. (1971). Positivism, metaphysics and religion. In R. N. Nanshen (Ed.), Werner Heisenberg – Physics and beyond – Encounters and conversations , World Perspectives. 42. Translator: Arnold J. Pomerans. New York: Harper and Row.
Hossain, F. M. A. (2014). A critical analysis of empiricism. Open Journal of Philosophy, 2014 (4), 225–230.
Kant, I. (1783). Prolegomena to any future metaphysic (trans: Bennett, J.). Early Modern Texts. www.earlymoderntexts.com
Koch, S. (1992). Psychology’s Bridgman vs. Bridgman’s Bridgman: An essay in reconstruction. Theory and Psychology, 2 (3), 261–290.
Matin, A. (1968). An outline of philosophy . Dhaka: Mullick Brothers.
Mcleod, S. (2008). Psychology as science. http://www.simplypsychology.org/science-psychology.html
Popper, K. (1963). Conjectures and refutations: The growth of scientific knowledge . London: Routledge.
Simmel, G. (1908). The problem areas of sociology in Kurt H. Wolf: The sociology of Georg Simmel . London: The Free Press.
Weber, M. (1991). The nature of social action. In W. G. Runciman (Ed.), Weber: Selections in translation . Cambridge: Cambridge University Press.
Download references
Author information
Authors and affiliations.
Department of Political Science, University of Ibadan, Ibadan, Oyo, 200284, Nigeria
Emeka Thaddues Njoku
You can also search for this author in PubMed Google Scholar
Corresponding author
Correspondence to Emeka Thaddues Njoku .
Editor information
Editors and affiliations.
Rhinebeck, New York, USA
David A. Leeming
Rights and permissions
Reprints and permissions
Copyright information
© 2017 Springer-Verlag GmbH Germany
About this entry
Cite this entry.
Njoku, E.T. (2017). Empirical Research. In: Leeming, D. (eds) Encyclopedia of Psychology and Religion. Springer, Berlin, Heidelberg. https://doi.org/10.1007/978-3-642-27771-9_200051-1
Download citation
DOI : https://doi.org/10.1007/978-3-642-27771-9_200051-1
Received : 01 April 2017
Accepted : 08 May 2017
Published : 22 May 2017
Publisher Name : Springer, Berlin, Heidelberg
Print ISBN : 978-3-642-27771-9
Online ISBN : 978-3-642-27771-9
eBook Packages : Springer Reference Behavioral Science and Psychology Reference Module Humanities and Social Sciences Reference Module Business, Economics and Social Sciences
- Publish with us
Policies and ethics
- Find a journal
- Track your research

An official website of the United States government
The .gov means it’s official. Federal government websites often end in .gov or .mil. Before sharing sensitive information, make sure you’re on a federal government site.
The site is secure. The https:// ensures that you are connecting to the official website and that any information you provide is encrypted and transmitted securely.
- Publications
- Account settings
Preview improvements coming to the PMC website in October 2024. Learn More or Try it out now .
- Advanced Search
- Journal List
- HHS Author Manuscripts

Writing Empirical Articles: Transparency, Reproducibility, Clarity, and Memorability
Morton Ann Gernsbacher
Department of Psychology, University of Wisconsin–Madison
https://orcid.org/0000-0003-0397-3329
This article provides recommendations for writing empirical journal articles that enable transparency, reproducibility, clarity, and memorability. Recommendations for transparency include preregistering methods, hypotheses, and analyses; submitting registered reports; distinguishing confirmation from exploration; and showing your warts. Recommendations for reproducibility include documenting methods and results fully and cohesively, by taking advantage of open-−science tools, and citing sources responsibly. Recommendations for clarity include writing short paragraphs, composed of short sentences; writing comprehensive abstracts; and seeking feedback from a naive audience. Recommendations for memorability include writing narratively; embracing the hourglass shape of empirical articles; beginning articles with a hook; and synthesizing, rather than Mad Libbing, previous literature.
They began three and a half centuries ago ( Wells, 1998 ). Since then, they’ve been written and read; cited, abstracted, and extracted; paywalled and unpaywalled; pre-printed and reprinted. They arose as correspondences between pairs of scientists ( Kronick, 1984 ), then morphed into publicly disseminated conference presentations ( Schaffner, 1994 ). By the 20th century, they’ d grown into the format we use today ( Mack, 2015 ). They are empirical journal articles, and their raison d’être was and continues to be communicating science.
Many of us baby boomers honed our empirical-article writing skills by following Bem’s (1987) how-to guide. We applied Bem’s recommendations to our own articles, and we assigned his chapter to our students and postdocs. The 2004 reprint of Bem’s chapter retains a high recommendation from the American Psychological Association (2010) in its “Guide for New Authors”; it appears in scores of graduate and undergraduate course syllabi ( Gernsbacher, 2017a ); and its advice is offered by numerous universities’ writing centers (e.g., Harvard College, 2008 ; Purdue Online Writing Lab, 2012 ; University of Connecticut, n.d. ; University of Minnesota, n.d. ; University of Washington, 2010 ).
However, psychological scientists have recently confronted their questionable research practices ( John, Loewenstein, & Prelec, 2012 ), many of which arise during the writing (or revising) process ( Sacco, Bruton, & Brown, 2018 ). Questionable research practices include
- failing to report all the studies conducted, conditions manipulated, participants tested, data collected, or other “researcher degrees of freedom” ( Simmons, Nelson, & Simonsohn, 2011 , p. 1359);
- fishing through statistical analyses to report only those meeting a certain level of statistical significance, which is a practice known as p -hacking ( Simonsohn, Nelson, & Simmons, 2014 );
- reporting an unpredicted result as though it had been hypothesized all along, which is a practice known as hypothesizing after the results are known (often referred to as HARKing; Kerr, 1998 ); and
- promising that the reported results bear implications beyond the populations sampled or materials and tasks administered ( Simons, Shoda, & Lindsay, 2017 ).
Unfortunately, some of these questionable reporting practices seem to be sanctioned in Bem’s how-to guide ( Devlin, 2017 ; Vazire, 2014 ). For example, Bem’s chapter seems to encourage authors to p -hack their data. Authors are advised to
examine [your data] from every angle. Analyze the sexes separately. Make up new composite indexes. If a datum suggests a new hypothesis, try to find additional evidence for it elsewhere in the data. If you see dim traces of interesting patterns, try to reorganize the data to bring them into bolder relief. If there are participants you don’t like, or trials, observers, or interviewers who gave you anomalous results, drop them (temporarily). Go on a fishing expedition for something — anything — interesting ( Bem, 1987 , p. 172; Bem, 2004 , pp. 186–187).
Bem’s chapter has also been interpreted as encouraging authors to hypothesize after the results are known ( Wagenmakers, Wetzels, Borsboom, & van der Maas, 2011 ). After acknowledging “there are two possible articles you can write: (a) the article you planned to write when you designed your study or (b) the article that makes the most sense now that you have seen the results,” Bem noted the two potential articles “are rarely the same” and directed authors to write the latter article by “recentering your article around the new findings and subordinating or even ignoring your original hypotheses” ( Bem, 1987 , pp. 171—173; Bem, 2004 , pp. 186–187).
This article provides recommendations for writing empirical journal articles that communicate research processes and products transparently with enough detail to allow replication and reproducibility. 1 Like Bem’s chapter, this article also provides recommendations for writing empirical articles that are clear and memorable.
Open materials for this article, which are available at https://osf.io/q3pna/ , include a list of publicly available course syllabi that mention Bem’s (1987 , 2004) “Writing the Empirical Journal Article” chapter and a tally of word and sentence counts, along with citation counts, for Clark and Clark (1939 , 1940 , 1947) , Harlow (1958) , Miller (1956) , and Tolman (1948) .
Recommendations for Transparency
Researchers write empirical journal articles to report and record why they conducted their studies, how they conducted their studies, and what they observed in their studies. The value of these archival records depends on how transparently researchers write their reports. Writing transparently, means, as the vernacular connotes, writing frankly.
Preregister your study
The best way to write transparent empirical articles is through preregistration ( Chambers et al., 2013 ). Preregistering a study involves specifying the study’s motivation, hypothesis, method, materials, sample, and analysis plan—basically everything but the results and discussion of those results—before the study is conducted.
Preregistration is a “time-stamped research plan that you can point to after conducting a study to prove to yourself and others that you really are testing a predicted relationship” (Mellor, as cited in Graf, 2017 , para.3). Indeed, most of our common statistical tests rest on the assumption that we have preregistered, or at the least previously specified, our predictions ( Wagenmakers, Wetzels, Borsboom, van der Maas, & Kievit, 2012 ).
For more than 20 years, medical journals have required preregistration for researchers conducting clinical trials ( Maxwell, Kelley, & Rausch, 2008 ). More recently, sites such as Open Science Framework and AsPredicted.org allow all types of researchers to document their preregistration, and preregistration is considered a best practice by psychologists of many stripes: cognitive ( de Groot, 2014 ), clinical ( Tackett et al., 2017 ), comparative ( Stevens, 2017 ), developmental ( Donnellan, Lucas, Fraley, & Roisman, 2013 ), social ( van 't Veerab & Giner-Sorolla, 2016 ), personality ( Asendorpf et al., 2013 ), relationship ( Campbell, Loving, & Lebelc, 2014 ), neuroscience ( Button et al., 2013 ), and neuroimaging ( Poldrack et al., 2017 ).
The benefits of preregistration are plentiful, both to our sciences and to ourselves. As Mellor noted (cited in Graf, 2017 , para. 8), “Every step that goes into a preregistration: writing the hypotheses, defining the variables, and creating statistical tests, are steps that we all have to take at some point. Making them before data collection can improve the researcher’s study design.” Misconceptions about preregistration are also plentiful. For instance, some researchers mistakenly believe that if a study is preregistered, unpredicted analyses cannot be reported; they can, but they need to be identified as exploratory (see, e.g., Neuroskeptic, 2013 ). Other researchers worry that purely exploratory research cannot be preregistered; it can, but it needs to be identified as exploratory (see, e.g., McIntosh, 2017 ). Preregistration manifests transparency and is, therefore, one of the most important steps in conducting and reporting research transparently.
Submit a registered report
A further step in writing transparent articles is to submit a registered report. Registered reports are journal articles for which both the authors’ preregistrations and their subsequent manuscripts undergo peer review. (Pre-registration outside of submission as a registered- report journal article does not require peer review, only documentation.)
Registered reports epitomize how most of us were trained to do research. For our dissertations and masters’ theses, even our senior theses, we submitted our work to review at two stages: after we designed the study (e.g., at our dissertation proposal meeting) and after we collected and analyzed the data and interpreted our results (e.g., at our final defense). The same two-stage review occurs with registered-report journal articles ( Nosek & Lakens, 2014 ). More and more journals are providing authors with the option to publish registered reports (for a list, see Center for Open Science, n.d. ). The beauty of registered reports is that, as with our dissertations, our success depends not on the shimmer of our results but on the soundness of our ideas and the competence of our execution.
Distinguish confirmation from exploration
Writing transparently means distinguishing confirmation from exploration. To be sure, exploration is a valid and important mode of scientific inquiry. The exploratory analyses Bem wrote about (“examine [your data] from every angle”) are vital for discovery—and should not be discouraged. However, it is also vital to distinguish exploratory from confirmatory analyses.
For example, clarify whether “additional exploratory analysis was conducted” ( Brockhaus, 1980 , p. 517), “data were derived from an exploratory questionnaire” ( Scogin & Bienias, 1988 , p. 335), or “results . . . should be interpreted cautiously because of their exploratory nature” ( Martin & Stelmaczonek, 1988 , p. 387). Entire research projects may be exploratory ( McIntosh, 2017 ), but they must be identified as such (e.g., “Prediction of Improvement in Group Therapy: An Exploratory Study,” Yalom, Houts, Zimerberg, & Rand, 1967 ; and “Personality and Probabilistic Thinking: An Exploratory Study,” Wright & Phillips, 1979 ).
Show your warts
Scientific reporting demands showing your work ( Vazire, 2017 ); transparent scientific reporting demands showing your warts. If participants were excluded, explain why and how many: for example, “Two of these subjects were excluded because of their inability to comply with the imagery instructions at least 75% of the time” ( Sadalla, Burroughs, & Staplin, 1980 , p. 521).
Similarly, if data were lost, explain why and how many: for example, “Ratings for two subjects were lost to equipment error” ( Vrana, Spence, & Lang, 1988 , p. 488) or “Because of experimenter error, processing times were not available for 11 subjects” ( McDaniel & Einstein, 1986 , p. 56).
If one or more pilot studies were conducted, state that. If experiments were conducted in an order different from the reported order, state that. If participants participated in more than one study, state that. If measures were recalculated, stimuli were refashioned, procedures were reconfigured, variables were dropped, items were modified—if anything transgressed the pre- specified plan and approach—state that.
Writing transparently also requires acknowledging when results are unpredicted: for example, “An unexpected result of Experiment 1 was the lack of an age . . . effect . . . due to different presentation rates” ( Kliegl, Smith, & Bakes, 1989 , p. 251) or “Unexpectedly, the female preponderance in depressive symptoms is strongly demonstrated in every age group in this high school sample” ( Allgood-Merten, Lewinsohn, & Hops, 1990 , p. 59). Concede when hypotheses lack support: for example, “we were unable to demonstrate that free care benefited people with a high income” ( Brook et al., 1983 , p. 1431) or “we cannot reject the null hypothesis with any confidence” ( Tannenbaum & Smith, 1964 , p. 407).
Consider placing a Statement of Transparency in either your manuscript or your supplementary materials: for example, “Statement of Transparency: The data used in the present study were initially collected as part of a larger exploratory study” ( Werner & Milyavskaya, 2017 , p. 3) or “As described in the Statement of Transparency in our online supplemental materials, we also collected additional variables and conducted further analyses that we treat as exploratory” ( Gehlbach et al., 2016 , p. 344).
Consider ending your manuscript with a Constraints on Generality statement ( Simons et al., 2017 ), which “defines the scope of the conclusions that are justified by your data” and “clarifies which aspects of your sample of participants, materials, and procedures should be preserved in a direct replication” (p. 1125; see Simons et al., 2017 , for examples).
Recommendations for Reproducibility
The soul of science is that its results are reproducible. Reproducible results are repeatable, reliable, and replicable. But reproducing a result, or simply trying to reproduce it, requires knowing in detail how that previous result was obtained. Therefore, writing for reproducibility means providing enough detail so readers will know how each result was obtained.
Document your research fully
Many researchers appreciate that empirical studies need to be reported accurately and completely—in fact, fully enough to allow other researchers to reproduce them— but they encounter a barrier: Many journals enforce word limits; some even limit the number of tables and figures that can accompany each article or the number of sources that can be cited. Journals’ limits can stymie authors’ efforts to write for reproducibility.
After using the maximum number of words allowed for methods and results, turn to open-science tools. Repositories, such as Open Science Framework (osf.io), Pub-Med Central (ncbi.nlm.nih.gov/pmc/), and Mendeley Data (mendeley.com/datasets), allow researchers to make their materials and data publicly available, which is a best practice quickly becoming mandatory ( Lindsay, 2017 ; Munafò et al., 2017 ; Nosek et al., 2015 ). These repositories also allow researchers to make detailed documentation of their methods and results publicly available.
For example, I recently analyzed 5 million books, 25 million abstracts, and 150 million journal articles to examine scholars’ use of person-first (e.g., person with a disability ) versus identity-first (e.g., disabled person ) language ( Gernsbacher, 2017b ). Because the journal that published my article limited me to 2,000 words, eight citations, and zero tables or figures, I created and posted on Open Science Framework an accompanying technical report ( Gernsbacher, 2016 ), which served as my open notebook. For the current article, I also created a technical report ( Gernsbacher, 2017a ) to document the course syllabi that assign Bem’s chapter (mentioned earlier) and the word counts that illustrate classic articles’ concision (mentioned later).
By taking advantage of open-science repositories, authors can document
- why they qualify for the 21-Word Solution, which is a statement authors can place in their Method section to verify they have “report[ed] how [they] determined [their] sample size, all data exclusions (if any), all manipulations, and all measures in the study” ( Simmons, Nelson, & Simonsohn, 2012 , p. 4);
- how they fulfilled the Preferred Reporting Items for Systematic Reviews and Meta-Analyses check-list ( PRISMA, 2015 ); and
- that they have met other methodological or statistical criteria (e.g., they have provided their data, materials, and code; Lindsay, 2017 ).
An accompanying technical report can serve as a publicly accessible lab notebook, which also comes in handy for selfish reasons ( Markowetz, 2015 ; McKiernan et al., 2016 ). A tidy, publicly accessible lab notebook can be, like tidy computer documentation, “a love letter you write to your future self” ( Conway, 2005 , p. 143).
Document your research cohesively
Documentation should also be cohesive. For instance, rather than posting a slew of separate supplementary files, consider combining all the supporting text, summary data, and supplementary tables and figures into one composite file. More helpfully, annotate the composite file with a table of contents or a set of in-file bookmarks.
A well-indexed composite file can reduce the frustration readers (and reviewers) incur when required to open multiple supplementary files (often generically named Supp. Fig.1, Supp. Fig. 2, etc.). Posting a well-indexed composite file on an open-science platform can also ensure that valuable information is available outside of journals’ paywalls, with guaranteed access beyond the life of an individual researcher’s or journal’s Web site (e.g., Open Science Framework guarantees their repository for 50 years).
Cite sources responsibly
As Simkin and Roychowdhury (2003) advised in the title of their study demonstrating high rates of erroneous citations, “read before you cite.” Avoid “drive by citations” ( Perrin, 2009 ), which reference a study so generically as to appear pro forma. Ensure that a specific connection exists between your claim and the source you cite to support that claim. Is the citation the original statement of the idea, a comprehensive review, an example of a similar study, or a counterclaim? If so, make that connection clear, rather than simply grabbing and citing the first article that pops up in a Google Scholar search.
Interrogate a reference before citing it, rather than citing it simply because other articles do. For example, I tallied hundreds of articles that mistakenly cited Rizzolatti et al. (1996) as providing empirical evidence for mirror neurons in humans—despite neither Rizzolatti et al.’s data nor their text supporting that claim ( Gallese, Gernsbacher, Heyes, Hickok, & Iacoboni, 2011 ).
Try to include a linked DOI (digital object identifier) for every reference you cite. Clicking on a linked DOI takes your readers directly to the original source, without having to search for it by its title, authors, journal, or the like. 2 Moreover, a DOI, like an ISBN, provides a permanent link to a published work; therefore, DOIs obviate link rot and guarantee greater longevity than standard URLs, even journal publishers’ URLs.
Recommendations for Clarity
Empirical articles are becoming more difficult to read, as an analysis of nearly three-quarter million articles in more than 100 high-impact journals recently demonstrated ( Plavén-Sigray, Matheson, Schiffler, & Thompson, 2017 ). Sentences in empirical articles have grown longer, and vocabulary has grown more abstruse. Therefore, the primary recommendation for achieving clarity in empirical articles is simple: Write concisely using plain language ( Box 1 provides additional suggestions and resources for clear writing).
Additional Recommendations for Clear Writing
Use precise terms.
Concision requires precision. Rather than writing that a dependent variable is related to, influenced by, or affected by the independent variable, state the exact relation between the two variables or the precise effect one variable has on another. Did manipulating the independent variable increase, decrease, improve, worsen, augment, diminish, negate, strengthen, weaken, delay, or accelerate the dependent variable?
Most important, use precise terms in your title. Follow the example of Parker, Garry, Engle, Harper, and Clfasefi (2008) , who titled their article “Psychotropic Placebos Reduce the Misinformation Effect by Increasing Monitoring at Test” rather than “The Effects of Psychotropic Placebos on Memory.”
Omit Needless Words
Numerous wordy expressions can be replaced by one word. For example, replace due to the fact that, for the reason that, or owing to the fact that with because; replace for the purpose of with for; have the capability of with can; in the event that with if; during the course of with during; fewer in number with fewer; in order to with to; and whether or not with whether . And replace the well-worn and wordy expression that appears in numerous acknowledgements, we wish to thank , with simply we thank .
Build Parallel Structures
Parallel structure aids comprehension ( Fraizer, Taft, Roeper, Clifton, & Ehrlich, 1984 ), whereas disjointed structure (e.g., Time flies like an arrow; fruit flies like a banana ) impedes comprehension ( Gernsbacher, 1997 ). Simons (2012) demonstrated how to build parallel structure with the example sentence Active reconstruction of a past experience differs from passively hearing a story about it. That sentence lacks parallel structure because the first half uses a noun phrase ( Active reconstruction ), whereas the second half uses a gerundive nominal ( passively hearing ). But the sentence can easily be made parallel: Actively reconstructing a past experience differs from passively hearing a story about it.
Listen to Your Writing
Try reading aloud what you have written (or use text-to-speech software). Listening to your writing is a great way to catch errors and get a feel for whether your writing is too stilted (and your sentences are too long).
Read About Writing
Read about how to write clearly in Pinker’s (2015) book, Zinsser’s (2016) book, Wagenmakers’s (2009) article, Simons’s (2012) guide, and Gernsbacher’s (2013) graduate-level open-access course. Try testing the clarity of your writing with online readability indices (e.g., https://readable.io/text or https://wordcounttools.com )
Write short sentences
Every writing guide, from Strunk and White’s (1959) venerable Elements of Style to the prestigious journal Nature ’s (2014) guide, admonishes writers to use shorter, rather than longer, sentences. Shorter sentences are not only easier to understand, but also better at conveying complex information ( Flesch, 1948 ).
The trick to writing short sentences is to restrict each sentence to one and only one idea. Resist the temptation to embed multiple clauses or parentheticals, which challenge comprehension. Instead, break long, rambling sentences into crisp, more concise ones. For example, write the previous three short sentences rather than the following long sentence: The trick to writing short sentences is to restrict each sentence to one and only one idea by breaking long, rambling sentences into crisp, more concise ones while resisting the temptation to embed multiple clauses or parentheticals, which challenge comprehension.
How short is short enough? The Oxford Guide to Plain English ( Cutts, 2013 ) recommends averaging no more than 15 to 20 words per sentence. Such short, crisp sentences have been the mainstay of many great psychological scientists, including Kenneth and Mamie Clark. Their 1939, 1940, and 1947 articles reporting young Black children’s racial identification and self-esteem have garnered more than 2,500 citations. These articles figured persuasively in Brown v. Board of Education (1954) . And these articles’ sentences averaged 16 words.
Write short paragraphs
Combine short sentences into short paragraphs. Aim for around five sentences per paragraph. Harlow’s “The Nature of Love” (1958) , Tolman’s “Cognitive Maps in Rats and Men” (1948) , and Miller’s “The Magical Number Seven, Plus or Minus Two” (1956) , which have been cited more than 2,000, 5,000, and 25,000 times, respectively, average five sentences per paragraph.
The prototypical five-sentence paragraph comprises a topic sentence, three supporting sentences, and a conclusion sentence. For example, a paragraph in Parker, Garry, Engle, Harper, and Clifasefi’s (2008 , p. 410) article begins with the following topic sentence: “One of the puzzles of human behaviour is how taking a substance that does nothing can cause something.” The paragraph continues with three (in this case, conjoined) supporting sentences: “Phoney painkillers can lessen our pain or make it worse; phoney alcohol can lead us to do things we might otherwise resist, and phoney feedback can even cause us to shed body fat.” The paragraph then concludes with the sentence “Perhaps Kirsch (2004, p. 341) said it best: ‘Placebos are amazing.’”
Write comprehensive abstracts
Compiling a technical report and placing it on an open-source platform can circumvent a journal’s word limit for a manuscript. However, a journal’s word limit for an abstract is more difficult to circumvent. That limit is firm, and an abstract can often be the sole content that is read, particularly if the rest of the article lies behind a paywall. Therefore, authors need to make the most of their 150 or 250 words so that an abstract can inform on its own ( Mensh & Kording, 2017 ).
A clear abstract states the study’s primary hypothesis; its major methodology, including its sample size and sampled population; its main findings, along with their summary statistics; and its key implications. A clear abstract is explicit, concrete, and comprehensive, which was advice offered by Bem (1987 , 2004) .
Seek naive feedback
One of the best ways to ensure that a message is clear is to assess its clarity according to a naive audience ( Traxler & Gernsbacher, 1995 ). Indeed, the more naive the audience, the more informative the feedback ( Traxler & Gernsbacher, 1992 , 1993 ).
Unfortunately, some researchers seek feedback on their manuscripts from only their coauthors or fellow lab members. But coauthors and fellow lab members are hardly naive. Better feedback can be obtained from readers who are unfamiliar with the research—and unfamiliar with even the research area. If those readers say the writing is unclear (or a figure or table is confusing), it is, by definition, unclear (or confusing); it is best to revise for clarity.
Recommendations for Memorability
Most researchers want their articles not only to be read but also to be remembered. The goal in writing a memorable article is not necessarily to pen a flashy article; rather, the goal is to compose an article that enables readers to remember what they have read days or months later, as well as paragraphs or pages later ( Gernsbacher, 1990 ).
Write narratively
The primary tool for increasing memorability is writing narratively ( Bruner, 1991 ). An empirical article should tell a story, not in the sense of a tall tale but in the spirit of a coherent and logical narrative.
Even authors who bristle at the notion of scholarly articles as stories must surely recognize that empirical articles resemble Aristotelian narratives: Introduction sections begin with a premise (the previous literature) that leads to an inciting incident (however, ...) and conclude with a therefore (the methods used to combat the inciting incident).
Thus, Introduction sections and Method sections are empirical articles’ Act One, their setups. Results sections are empirical articles’ Act Two, their confrontations. And Discussion sections are empirical articles’ Act Three, their resolutions.
Writing Act One (introduction and methods) prior to collecting data, as we would do if submitting a registered report, helps us adhere to Feynman’s (1974) warning not to fool ourselves (e.g., not to misremember what we did vs. did not predict and, consequently, which analyses are vs. are not confirmatory).
Writing all sections narratively, as setup, confrontation, and then resolution, should increase their short- and long-term memorability. Similarly, writing methods and results as sequences of events should increase their memorability.
For methods, Bem (1987 , 2004) recommended leading readers through the procedure as if they were research participants, which is an excellent idea. For results, readers can be led through the analytic pipeline in the sequence in which it occurred.
Embrace the hourglass
Bem advised that an article should be written “in the shape of an hourglass. It begins with broad general statements, progressively narrows down to the specifics of your study, and then broadens out again to more general considerations” ( Bem, 1987 , p. 175; Bem, 2004 , p. 189). That advice should also not be jettisoned ( Devlin, 2017 ).
Call it the hourglass or call it the “broad- narrow-broad” structure ( Mensh & Kording, 2017 , p. 4), the notion is that well-written empirical articles begin broadly (theories and questions), narrow to specifics (methods and results), and end broadly (implications). Authors who embrace the hourglass shape aid their readers, particularly readers who skim ( Weinstein, 2016 ).
Begin with a hook
Journal editors advise that articles “should offer a clear, direct, and compelling story that first hooks the reader” (Rains, 2012, p. 497). For example, Oyserman et al. (2017) began their article with the following hook, which led directly to a statement articulating what their article was about (illustrated here in italics):
Will you be going to that networking lunch? Will you be tempted by a donut at 4 pm? Will you be doing homework at 9 pm? If, like many people, your responses are based on your gut sense of who you are—shy or outgoing, a treat lover or a dieter, studious or a procrastinator—you made three assumptions about identity: that motivation and behavior are identity based, that identities are chronically on the mind, and that identities are stable. (p. 139)
As another example, Newman et al. (2014) began their article with the following hook:
In its classic piece, “Clinton Deploys Vowels to Bosnia,” the satirical newspaper The Onion quoted Trszg Grzdnjkln, 44. “I have six children and none of them has a name that is understandable to me or to anyone else. Mr. Clinton, please send my poor, wretched family just one ‘E.’ Please.” The Onion was onto something when it suggested that people with hard to pronounce names suffer while their more pronounceable counter parts benefit. (p. 1, italics added)
As a third example, Jakimik and Glenberg (1990) began their article with the following hook:
You’re zipping through an article in your favorite journal when your reading stops with a thud. The author has just laid out two alternative hypotheses and then referred to one of them as “the former approach.” But now you are confused about which was first, which was second. You curse the author and your own lack of concentration, reread the setup rehearsing the order of the two hypotheses, and finally figure out which alternative the author was referring to. We have experienced this problem, too, and we do not think that it is simply a matter of lack of concentration. The subject of this article is the reason for difficulty with referring devices such as “the former approach.” (p. 582, italics added)
Synthesize previous literature (rather than Mad Lib it)
In the game of Mad Libs, one player generates a list of words from specified categories, for instance, a proper name, an activity, and a number. Then, the other player fills a template sentence with that list of generated terms.
In a similar way, some authors review the literature by Mad Libbing terms into sentence templates, for example, “_____ [author’s name] investigated _____ [research topic] with _____ [number] of participants and found a statistically significant effect of _____ [variable] on _____ [variable].”
A more memorable, albeit more difficult, way to review the literature is to synthesize it, as Aronson (1969) illustrated in his synthesis of previous studies on cognitive dissonance:
The research [on cognitive dissonance] has been as diverse as it has been plentiful; its range extends from maze running in rats (Lawrence and Festinger, 1962) to the development of values in children (Aronson and Carlsmith, 1963); from the hunger of college sophomores (Brehm et al., 1964) to the proselytizing behavior of religious zealots (Festinger et al., 1956). The proliferation of research testing and extending dissonance theory results from the generality and simplicity of the theory. (p. 1)
Notice that Aronson wrote a coherent narrative in which phenomena, not researchers, are the topics. That is what is meant by synthesizing, not Mad Libbing, previous literature.
Even technical literature can be synthesized rather than Mad Libbed, as Guillem et al. (2011) demonstrated:
Cortical acetylcholine (ACh) release from the basal forebrain is essential for proper sensory processing and cognition (1–3) and tunes neuronal and synaptic activity in the underlying cortical networks (4,5). Loss of cholinergic function during aging and Alzheimer’s disease results in cognitive decline, notably a loss of memory and the ability to sustain attention (6,7). Interfering with the cholinergic system strongly affects cognition (3,8–13). Rapid changes in prefrontal cortical ACh levels at the scale of seconds are correlated with attending and detecting cues (14,15). Various types of nicotinic ACh receptor (nAChR) subunits are expressed in the prefrontal cortex (PFC) (16–18) ... However, the causal relation between nAChR β2 subunits (henceforth β2-nAChRs) expressed in the medial PFC (mPFC) and attention performance has not yet been demonstrated. (p. 888)
Guillem et al. began with a premise (“Cortical acetyl- choline (ACh) release from the basal forebrain is essential”), which they then supported with the literature. They further developed their premise (“Loss of cholinergic function during aging and Alzheimer’s disease results in cognitive decline,” “Interfering with the cholinergic system strongly affects cognition,” and “Rapid changes in prefrontal cortical ACh levels ... are correlated with attending and detecting cues”), and they concluded with their “However.” They synthesized the literature to tell a story.
Writing clearly and memorably need not be orthogonal to writing transparently and enabling reproducibility. For example, in their seminal article on false memories for words presented in lists, Roediger and McDermott (1995)
- documented their experimental procedure fully enough to allow replication, including most recently a preregistered replication ( Zwaan et al., 2017 );
- provided their research materials openly (in an appendix);
- told their story in short paragraphs (average length of 5.1 sentences) and short sentences (average length of 18 words);
- embraced an hourglass shape (e.g., their discussion began by relating their study to prior work, continued by contrasting experiments that measured false recall vs. false recognition, extended to discussing phenomenological experience, and broadened to articulating implications); and
- transparently acknowledged parallel efforts by another research team (“While working on this article, we learned that Don Read was conducting similar research, which is described briefly in Lindsay & Read, 1994,” p. 804).
A well-written empirical article that enables reproducibility and transparency can also be clear and memorable.
Barring extraordinary disruption, empirical journal articles are likely to survive at least a couple more decades. Authors will continue to write empirical articles to communicate why they did their studies, how they did their studies, what they observed, and what those observations mean. And readers will continue to read empirical articles to receive this communication. The most successful articles will continue to embody Grice’s (1975) maxims for communication: They will be informative, truthful, relevant, clear, and memorable.
1 Some researchers distinguish between replication, which they define as corroborating previous results by collecting new data, and reproduction, which they define as corroborating previous results by analyzing previous data ( Peng, 2011 ). Other researchers consider the two terms to be synonymous ( Shuttleworth, 2009 ), or they propose that the two terms should be used synonymously ( Goodman, Fanelli, & Ioannidis, 2016 ).
2 To make a linked, or clickable, DOI, simply add the preface https://doi.org to the alphanumeric string.
Author Contributions
Declaration of Conflicting Interests
The author(s) declared no conflicts of interest with respect to the authorship or the publication of this article.
Data and materials are available via Open Science Framework and can be accessed at https://osf.io/uxych . The Open Practices Disclosure for this article can be found at http://journals.sagepub.com/doi/suppl/10.1177/2515245918754485 . This article has received badges for Open Data and Open Materials. More information about the Open Practices badges can be found at http://www.psychologicalscience.org/publications/badges .
- Allgood-Merten B, Lewinsohn PM, Hops H. Sex differences and adolescent depression. Journal of Abnormal Psychology. 1990; 99 :55–63. doi: 10.1037/0021-843X.99.1.55. [ PubMed ] [ CrossRef ] [ Google Scholar ]
- American Psychological Association. Preparing manuscripts for publication in psychology journals: A guide for new authors. 2010 https://www.apa.org/pubs/authors/new-author-guide.pdf .
- Aronson E. The theory of cognitive dissonance: A current perspective. In: Berkowitz L, editor. Advances in experimental social psychology. Vol. 4. New York, NY: Academic Press; 1969. pp. 1–34. [ CrossRef ] [ Google Scholar ]
- Asendorpf JB, Conner M, De Fruyt F, De Houwer J, Denissen JJA, Fiedler K, Wicherts JM. Recommendations for increasing replicability in psychology. European Journal of Personality. 2013; 27 :108–119. doi: 10.1002/per.1919. [ CrossRef ] [ Google Scholar ]
- Bem DJ. Writing the empirical journal article. In: Zanna MP, Darley JM, editors. The compleat academic: A practical guide for the beginning social scientist. Hillsdale, NJ: Erlbaum; 1987. pp. 171–201. [ Google Scholar ]
- Bem DJ. Writing the empirical journal article. In: Darley JM, Zanna MP, Roediger HL III, editors. The compleat academic: A practical guide for the beginning social scientist. 2. Washington, DC: American Psychological Association; 2004. pp. 185–219. [ Google Scholar ]
- Brockhaus RH. Risk taking propensity of entrepreneurs. Academy of Management Journal. 1980; 23 :509–520. doi: 10.5465/ambpp.1976.4975934. [ CrossRef ] [ Google Scholar ]
- Brook RH, Ware JE, Jr, Rogers WH, Keeler EB, Davies AR, Donald CA, Newhouse JP. Does free care improve adults’ health? Results from a randomized controlled trial. The New England Journal of Medicine. 1983; 308 :1426–1434. doi: 10.1056/NEJM198312083092305. [ PubMed ] [ CrossRef ] [ Google Scholar ]
- Brown v. Board of Educ., 347 U.S. 483 (1954).
- Bruner J. The narrative construction of reality. Critical Inquiry. 1991; 18 :1–21. doi: 10.1086/448619. [ CrossRef ] [ Google Scholar ]
- Button KS, Ioannidis JPA, Mokrysz C, Nosek BA, Flint J, Robinson ESJ, Munafò MR. Power failure: Why small sample size undermines the reliability of neuroscience. Nature Reviews Neuroscience. 2013; 14 :365–376. doi: 10.1038/nrn3475. [ PubMed ] [ CrossRef ] [ Google Scholar ]
- Campbell L, Loving TJ, Lebelc EB. Enhancing transparency of the research process to increase accuracy of findings: A guide for relationship researchers. Personal Relationships. 2014; 21 :531–545. doi: 10.1111/pere.12053. [ CrossRef ] [ Google Scholar ]
- Center for OpenScience. Registered Reports: Peer review before results are known to align scientific values and practices. n.d https://cos.io/rr/
- Chambers C, Munafo M more than 80 signatories. Trust in science would be improved by study pre-registration. The Guardian. 2013 Jun 5; https://www.theguardian.com/science/blog/2013/jun/05/trust-in-science-study-pre-registration .
- Clark KB, Clark MK. The development of consciousness of self and the emergence of racial identification in Negro preschool children. Journal of Social Psychology. 1939; 10 :591–599. doi: 10.1080/00224545.1939.9713394. . [ CrossRef ] [ Google Scholar ]
- Clark KB, Clark MK. Skin color as a factor in racial identification of Negro preschool children. Journal of Social Psychology. 1940; 11 :159–169. doi: 10.1080/00224545.1940.9918741. [ CrossRef ] [ Google Scholar ]
- Clark KB, Clark MK. Racial identification and preference in Negro children. In: Newcomb TM, Hartley EL, editors. Readings in social psychology. New York, NY: Henry Holt; 1947. pp. 169–178. [ Google Scholar ]
- Conway D. Perl best practices: Standards and styles for developing maintainable code. Sebastopol, CA: O’Reilly Media; 2005. https://books.google.com/books?isbn=0596555024 . [ Google Scholar ]
- Cutts M. Oxford guide to plain English. 4. Oxford, England: Oxford University Press; 2013. [ Google Scholar ]
- de Groot AD. The meaning of “significance” for different types of research [translated and annotated by Eric-Jan Wagenmakers, Denny Borsboom, Josine Verhagen, Rogier Kievit, Marjan Bakker, Angelique Cramer, Dora Matzke, Don Mellenbergh, and Han L. J. van der Maas] Acta Psychlogica. 2014; 148 :188–194. doi: 10.1016/j.actpsy.2014.02.001. [ PubMed ] [ CrossRef ] [ Google Scholar ]
- Devlin AS. The research experience: Planning, conducting, and reporting research. Thousand Oaks, CA: Sage; 2017. [ Google Scholar ]
- Donnellan MB, Lucas RE, Fraley RC, Roisman GI. Methodological reform 101 for developmental researchers. Developmental Psychologist. 2013 Summer;:34–40. http://www.apadivisions.org/division-7/publications/newsletters/developmental/2013/07/issue.pdf .
- Feynman RP. Cargo cult science. 1974 http://calteches.library.caltech.edu/51/2/CargoCult.htm .
- Flesch R. A new readability yardstick. Journal of Applied Psychology. 1948; 32 :221–233. doi: 10.1037/h0057532. [ PubMed ] [ CrossRef ] [ Google Scholar ]
- Fraizer L, Taft L, Roeper T, Clifton C, Ehrlich K. Parallel structure: A source of facilitation in sentence comprehension. Memory & Cognition. 1984; 12 :421–430. doi: 10.3758/BF03198303. [ PubMed ] [ CrossRef ] [ Google Scholar ]
- Gallese V, Gernsbacher MA, Heyes C, Hickok G, Iacboni M. Mirror neuron forum. Perspectives on Psychological Science. 2011; 6 :369–407. doi: 10.1177/1745691611413392. [ PMC free article ] [ PubMed ] [ CrossRef ] [ Google Scholar ]
- Gehlbach H, Brinkworth ME, King AM, Hsu LM, McIntyre J, Rogers T. Creating birds of similar feathers: Leveraging similarity to improve teacher-student relationships and academic achievement. Journal of Educational Psychology. 2016; 108 :342–352. doi: 10.1037/edu0000042. [ CrossRef ] [ Google Scholar ]
- Gernsbacher MA. Language comprehension as structure building. Hillsdale, NJ: Erlbaum; 1990. [ Google Scholar ]
- Gernsbacher MA. Coherence cues mapping during comprehension. In: Costermans J, Fayol M, editors. Processing interclausal relationships: Studies in the production and comprehension of text. Hillsdale, NJ: Erlbaum; 1997. pp. 3–21. [ Google Scholar ]
- Gernsbacher MA. Improving scholarly communication: An online course. 2013 https://osf.io/z9dh7 .
- Gernsbacher MA. Analyses of person-first language in scholarly writing: Tech report. 2016 https://osf.io/j7w4b . [ PMC free article ] [ PubMed ]
- Gernsbacher MA. Recommendations for writing empirical journal articles: Technical report. 2017a https://osf.io/q3pna .
- Gernsbacher MA. The use of person-first language in scholarly writing may accentuate stigma. Journal of Child Psychology and Psychiatry. 2017b; 58 :859–861. doi: 10.1111/jcpp.12706. [ PMC free article ] [ PubMed ] [ CrossRef ] [ Google Scholar ]
- Goodman SN, Fanelli D, Ioannidis JPA. What does research reproducibility mean? Science Translational Medicine. 2016; 8 doi: 10.1126/scitranslmed.aaf5027. Article 341ps12. [ PubMed ] [ CrossRef ] [ Google Scholar ]
- Graf C. 8 answers about Registered Reports, research preregistration, and why both are important. Wiley Exchange. 2017 Dec 6; https://hub.wiley.com/community/exchanges/discover/blog/2017/12/05/8-answers-about-registered-reports-research-preregistration-and-why-both-are-important .
- Grice HP. Logic and conversation. In: Cole P, Morgan J, editors. Syntax and semantics: Vol. 3. Speech acts. New York, NY: Academic Press; 1975. pp. 43–58. [ Google Scholar ]
- Guillem K, Bloem B, Poorthuis RB, Loos M, Smit AB, Maskos U, Mansvelder HD. Nicotinic ace-tylcholine receptor β2 subunits in the medial pre-frontal cortex control attention. Science. 2011; 12 :888–891. doi: 10.1126/science.1207079. [ PubMed ] [ CrossRef ] [ Google Scholar ]
- Harlow HF. The nature of love. American Psychologist. 1958; 13 :673–685. doi: 10.1037/h0047884. . [ PubMed ] [ CrossRef ] [ Google Scholar ]
- Harvard College. A brief guide to writing the psychology paper. 2008 https://writingproject.fas.harvard.edu/files/hwp/files/bg_psychology.pdf .
- Jakimik J, Glenberg A. Verbal learning meets psycholinguistics:Modality effects in the comprehension of anaphora. Journal of Memory and Language. 1990; 29 :582–590. doi: 10.1016/0749-596X(90)90053-3. [ CrossRef ] [ Google Scholar ]
- John LK, Loewenstein G, Prelec D. Measuring the prevalence of questionable research practices with incentives for truth telling. Psychological Science. 2012; 23 :524–532. doi: 10.1177/0956797611430953. [ PubMed ] [ CrossRef ] [ Google Scholar ]
- Kerr NL. HARKing: Hypothesizing After the Results are Known. Personality and Social Psychology Review. 1998; 2 :196–217. doi: 10.1207/s15327957pspr0203_4. [ PubMed ] [ CrossRef ] [ Google Scholar ]
- Kliegl R, Smith J, Bakes PB. Testing-the-limits and the study of adult age differences in cognitive plasticity of a mnemonic skill. Developmental Psychology. 1989; 25 :247–256. doi: 10.1037/0012-1649.25.2.247. [ CrossRef ] [ Google Scholar ]
- Kronick DA. Literature of the life sciences: The historical background. Bulletin of the New York Academy of Medicine. 1984; 60 :857–875. . https://www.ncbi.nlm.nih.gov/pmc/articles/PMC1911798/ [ PMC free article ] [ PubMed ] [ Google Scholar ]
- Lindsay DS. Sharing data and materials in Psychological Science. Psychological Science. 2017; 28 :699–702. doi: 10.1177/0956797617704015. [ PubMed ] [ CrossRef ] [ Google Scholar ]
- Mack C. 350 years of scientific journals. Journal of Micro/Nanolithography, MEMS, and MOEMS. 2015; 14 :010101:1–010101:3. doi: 10.1117/1.JMM.14.1.010101. [ CrossRef ] [ Google Scholar ]
- Markowetz F. Five selfish reasons to work reproducibly. Genome Biology. 2015; 16 doi: 10.1186/s13059-015-0850-7. Article 274. [ PMC free article ] [ PubMed ] [ CrossRef ] [ Google Scholar ]
- Martin J, Stelmaczonek K. Participants’ identification and recall of important events in counseling. Journal of Counseling Psychology. 1988; 35 :385–390. doi: 10.1037/0022-0167.35.4.385. [ CrossRef ] [ Google Scholar ]
- Maxwell SE, Kelley K, Rausch JR. Sample size planning for statistical power and accuracy in parameter estimation. Annual Review of Psychology. 2008; 59 :537–563. doi: 10.1146/annurev.psych.59.103006.093735. [ PubMed ] [ CrossRef ] [ Google Scholar ]
- McDaniel MA, Einstein GO. Bizarre imagery as an effective memory aid: The importance of distinctiveness. Journal of Experimental Psychology: Learning, Memory, and Cognition. 1986; 12 :54–65. doi: 10.1037/0278-7393.12.1.54. . [ PubMed ] [ CrossRef ] [ Google Scholar ]
- McIntosh RD. Exploratory reports: A new article type for Cortex. Cortex. 2017; 96 :A1–A4. doi: 10.1016/j.cortex.2017.07.014. [ PubMed ] [ CrossRef ] [ Google Scholar ]
- McKiernan EC, Bourne PE, Brown CT, Buck S, Kenall A, Lin J, Yarkoni T. How open science helps researchers succeed. eLife. 2016; 5 doi: 10.7554/eLife.16800. Article e16800. [ PMC free article ] [ PubMed ] [ CrossRef ] [ Google Scholar ]
- Mensh B, Kording K. Ten simple rules for structuring papers. PLOS Computational Biology. 2017; 13 (9) doi: 10.1371/journal.pcbi.1005619. Article e1005619. [ PMC free article ] [ PubMed ] [ CrossRef ] [ Google Scholar ]
- Miller GA. The magical number seven, plus or minus two: Some limits on our capacity for processing information. Psychological Review. 1956; 63 :81–97. doi: 10.1037/h0043158. [ PubMed ] [ CrossRef ] [ Google Scholar ]
- Munafò MR, Nosek BA, Bishop DVM, Button KS, Chambers CD, Percie du Sert N, Ioannidis JPA. A manifesto for reproducible science. Nature Human Behavior. 2017; 1 (1) doi: 10.1038/s41562-016-0021. Article 0021. [ PMC free article ] [ PubMed ] [ CrossRef ] [ Google Scholar ]
- Nature. Effective writing. Scitable. 2014 https://www.nature.com/scitable/topicpage/effective-writing-13815989 .
- Neuroskeptic. For preregistration in fundamental research. Discover. 2013 Apr 25; http://blogs.discovermagazine.com/neuroskeptic/2013/04/25/for-preregistration-in-fundamental-research .
- Newman EJ, Sanson M, Miller EK, Quigley-McBride A, Foster JL, Bernstein DM, Garry M. People with easier to pronounce names promote truthiness of claims. PLOS ONE. 2014; 9 (2) doi: 10.1371/journal.pone.0088671. Article e88671. [ PMC free article ] [ PubMed ] [ CrossRef ] [ Google Scholar ]
- Nosek BA, Alter G, Banks GC, Borsboom D, Bowman SD, Breckler SJ, Yarkoni T. Promoting an open research culture. Science. 2015; 348 :1422–1425. doi: 10.1126/science.aab2374. [ PMC free article ] [ PubMed ] [ CrossRef ] [ Google Scholar ]
- Nosek BA, Lakens D. Registered Reports: A method to increase the credibility of published results. Social Psychology. 2014; 45 :137–141. doi: 10.1027/1864-9335/a000192. [ CrossRef ] [ Google Scholar ]
- Oyserman D, Lewis NA, Jr, Yan VX, Fisher O, O’Donnell SC, Horowitz E. An identity-based motivation framework for self-regulation. Psychological Inquiry. 2017; 28 :139–147. doi: 10.1080/1047840X.2017.1337406. [ CrossRef ] [ Google Scholar ]
- Parker S, Garry M, Engle RW, Harper DN, Clfasefi SL. Psychotropic placebos reduce the misinformation effect by increasing monitoring at test. Memory. 2008; 16 :410–419. doi: 10.1080/09658210801956922. [ PubMed ] [ CrossRef ] [ Google Scholar ]
- Peng RD. Reproducible research in computational science. Simply Statistics. 2011 Dec 2; https://simplystatistics.org/2011/12/02/reproducible-research-in-computational-science/ [ PMC free article ] [ PubMed ]
- Perrin A. Drive by citations. Scatterplot. 2009 Apr 30; https://scatter.wordpress.com/2009/04/30/drive-by-citat ions/
- Pinker S. The sense of style: The thinking person’s guide to writing in the 21st century. New York, NY: Penguin Books; 2015. [ Google Scholar ]
- Plavén-Sigray P, Matheson GJ, Schiffler BC, Thompson WH. The readability of scientific texts is decreasing over time. eLife. 2017; 6 doi: 10.7554/eLife.27725. Article e27725. [ PMC free article ] [ PubMed ] [ CrossRef ] [ Google Scholar ]
- Poldrack RA, Baker CI, Durnez J, Gorgolewski KJ, Matthews PM, Munafò MR, Yarkoni T. Scanning the horizon: Towards transparent and reproducible neuroimaging research. Nature Reviews Neuroscience. 2017; 18 :115–126. doi: 10.1038/nrn.2016.167. [ PMC free article ] [ PubMed ] [ CrossRef ] [ Google Scholar ]
- PRISMA. PRISMA checklist. 2015 http://prisma-statement.org/prismastatement/Checklist.aspx .
- Purdue Online Writing Lab. References and sources for more information. Writing in psychology: Experimental report writing. 2012 https://owl.english.purdue.edu/owl/resource/670/06 .
- Ragins BR. Editor’s comments: Reflections on the craft of clear writing. Academy of Management Review. 2012; 37 :493–501. doi: 10.5465/amr.2012.0165. [ CrossRef ] [ Google Scholar ]
- Rizzolatti G, Fadiga L, Matelli M, Bettinardi V, Paulesu E, Perani D, Fazio F. Localization of grasp representations in humans by PET: 1. Observation versus execution. Experimental Brain Research. 1996; 111 :246–252. doi: 10.1007/BF00227301. . [ PubMed ] [ CrossRef ] [ Google Scholar ]
- Roediger HL, McDermott KB. Creating false memories: Remembering words not presented in lists. Journal of Experimental Psychology: Learning, Memory, and Cognition. 1995; 21 :803–814. doi: 10.1037/0278-7393.21.4.803. . [ CrossRef ] [ Google Scholar ]
- Sacco DF, Bruton SV, Brown M. In defense of the questionable: Defining the basis of research scientists’ engagement in questionable research practices. Journal of Empirical Research on Human Research Ethics. 2018; 13 :101–110. doi: 10.1177/1556264617743834. [ PubMed ] [ CrossRef ] [ Google Scholar ]
- Sadalla EK, Burroughs WJ, Staplin LJ. Reference points in spatial cognition. Journal of Experimental Psychology: Human Learning and Memory. 1980; 6 :516–528. doi: 10.1037/0278-7393.6.5.516. [ PubMed ] [ CrossRef ] [ Google Scholar ]
- Schaffner AC. The future of scientific journals: Lessons from the past. Information Technology and Libraries. 1994; 13 :239–247. https://eric.ed.gov/?id=EJ496591 . [ Google Scholar ]
- Scogin F, Bienias JL. A three-year follow-up of older adult participants in a memory-skills training program. Psychology and Aging. 1988; 3 :334–337. doi: 10.1037/0882-7974.3.4.334. [ PubMed ] [ CrossRef ] [ Google Scholar ]
- Shuttleworth M. Reproducibility. Explorable. 2009 Jun 14; https://explorable.com/reproducibility .
- Simkin MV, Roychowdhury VP. Read before you cite! Complex Systems. 2003; 14 :269–274. http://www.complex-systems.com/abstracts/v14_i03_a05/ [ Google Scholar ]
- Simmons J, Nelson L, Simonsohn U. A 21 word solution. Dialogue: The Official Newsletter of the Society for Personality and Social Psychology. 2012; 26 (2):4–7. http://spsp.org/sites/default/files/dialogue_26%282%29.pdf . [ Google Scholar ]
- Simmons JP, Nelson LD, Simonsohn U. False-positive psychology: Undisclosed flexibility in data collection and analysis allows presenting anything as significant. Psychological Science. 2011; 22 :1359–1366. doi: 10.1177/0956797611417632. [ PubMed ] [ CrossRef ] [ Google Scholar ]
- Simons D. Musings on writing. 2012 http://www.dansimons.com/resources/Simons_on_writing.pdf .
- Simons DJ, Shoda Y, Lindsay DS. Constraints on Generality (COG): A proposed addition to all empirical papers. Perspectives on Psychological Science. 2017; 12 :1123–1128. doi: 10.1177/1745691617708630. [ PubMed ] [ CrossRef ] [ Google Scholar ]
- Simonsohn U, Nelson LD, Simmons JP. P- curve: A key to the file-drawer. Journal of Experimental Psychology: General. 2014; 143 :534–547. doi: 10.1037/a0033242. . [ PubMed ] [ CrossRef ] [ Google Scholar ]
- Stevens JR. Replicability and reproducibility in comparative psychology. Frontiers in Psychology. 2017; 8 doi: 10.3389/fpsyg.2017.00862. Article 862. [ PMC free article ] [ PubMed ] [ CrossRef ] [ Google Scholar ]
- Strunk W, Jr, White EB. The elements of style. New York, NY: Macmillan; 1959. [ Google Scholar ]
- Tackett JL, Lilienfeld SO, Patrick CJ, Johnson SL, Krueger RF, Miller JD, Shrout PE. It’s time to broaden the replicability conversation: Thoughts for and from clinical psychological science. Perspectives on Psychological Science. 2017; 12 :742–756. doi: 10.1177/1745691617690042. [ PubMed ] [ CrossRef ] [ Google Scholar ]
- Tannenbaum AS, Smith CG. Effects of member influence in an organization: Phenomenology versus organization structure. Journal of Abnormal and Social Psychology. 1964; 69 :401–410. doi: 10.1037/h0041238. [ PubMed ] [ CrossRef ] [ Google Scholar ]
- Tolman EC. Cognitive maps in rats and men. Psychological Review. 1948; 55 :189–208. doi: 10.1037/h0061626. . [ PubMed ] [ CrossRef ] [ Google Scholar ]
- Traxler MJ, Gernsbacher MA. Improving written communication through minimal feedback. Language and Cognitive Processes. 1992; 7 :1–22. doi: 10.1080/01690969208409378. [ PMC free article ] [ PubMed ] [ CrossRef ] [ Google Scholar ]
- Traxler MJ, Gernsbacher MA. Improving written communication through perspective-taking. Language and Cognitive Processes. 1993; 8 :311–334. doi: 10.1080/01690969308406958. [ PMC free article ] [ PubMed ] [ CrossRef ] [ Google Scholar ]
- Traxler MJ, Gernsbacher MA. Improving coherence in written communication. In: Gernsbacher MA, Givón T, editors. Coherence in spontaneous text. Philadelphia, PA: John Benjamins; 1995. pp. 216–237. [ Google Scholar ]
- University of Connecticut. Writing in psychology. n.d https://writingcenter.uconn.edu/writing-in-psychology-4 .
- University of Minnesota. Writing a research report in American Psychological Association (APA) style. n.d http://open.lib.umn.edu/psychologyresearchmethods/chapter/11-2-writing-a-research-report-in-american-psychological-association-apa-style/
- University of Washington. Writing an empirical paper in APA style. 2010 https://depts.washington.edu/psych/files/writing_center/APApaper.pdf .
- van 't Veerab AE, Giner-Sorolla R. Pre-registration in social psychology—a discussion and suggested template. Journal of Experimental Social Psychology. 2016; 67 :2–12. doi: 10.1016/j.jesp.2016.03.004. [ CrossRef ] [ Google Scholar ]
- Vazire S. Life after Bem. sometimes i’m wrong. 2014 Mar; http://sometimesimwrong.typepad.com/wrong/2014/03/life-after-bem.html .
- Vazire S. Safer science: Making psychological science more transparent & replicable. Paper presented at the annual meeting of the Western Psychological Association; Sacramento, CA. 2017. https://osf.io/q7mv2 . [ Google Scholar ]
- Vrana SR, Spence EL, Lang PJ. The startle probe response: A new measure of emotion? Journal of Abnormal Psychology. 1988; 97 :487–491. doi: 10.1037/0021-843X.97.4.487. [ PubMed ] [ CrossRef ] [ Google Scholar ]
- Wagenmakers E-J. Teaching graduate students how to write clearly. 2009 http://www.ejwagenmakers.com/2009/TeachingTipsWriting.pdf .
- Wagenmakers E-J, Wetzels R, Borsboom D, van der Maas HLJ. Why psychologists must change the way they analyze their data: The case of psi: Comment on Bem (2011) Journal of Personality and Social Psychology. 2011; 100 :426–432. doi: 10.1037/a0022790. [ PubMed ] [ CrossRef ] [ Google Scholar ]
- Wagenmakers E-J, Wetzels R, Borsboom D, van der Maas HLJ, Kievit RA. An agenda for purely confirmatory research. Perspectives on Psychological Science. 2012; 7 :632–638. doi: 10.1177/1745691612463078. [ PubMed ] [ CrossRef ] [ Google Scholar ]
- Weinstein Y. Want to read a lot of journal articles, but don’t have enough time? Use this simple cheat sheet [Tweet] 2016 Feb 3; [@doctorwhy] https://twitter.com/doctorwhy/status/694986529903677440 .
- Wells A. Exploring the development of the independent, electronic, scholarly journal. Master’s thesis. 1998 https://web.archive.org/web/20121113062753/http://panizzi.shef.ac.uk/elecdiss/edl0001/ch0200.html .
- Werner KM, Milyavskaya M. We may not know what we want, but do we know what we need? Examining the ability to forecast need satisfaction in goal pursuit. Social Psychological & Personality Science. 2017 doi: 10.1177/1948550617720274. [ CrossRef ]
- Wright GN, Phillips LD. Personality and probabilistic thinking: An exploratory study. British Journal of Psychology. 1979; 70 :295–303. doi: 10.1111/j.2044-8295.1979.tb01686.x. [ CrossRef ] [ Google Scholar ]
- Yalom ID, Houts PS, Zimerberg SM, Rand KH. Prediction of improvement in group therapy: An exploratory study. Archives of General Psychiatry. 1967; 17 :159–168. doi: 10.1001/archpsyc.1967.01730260031005. [ PubMed ] [ CrossRef ] [ Google Scholar ]
- Zinsser W. On writing well: The classic guide to writing nonfiction. New York, NY: Harper Perennial; 2016. [ Google Scholar ]
- Zwaan RA, Pecher D, Paolacci G, Bouwmeester S, Verkoeijen P, Dijkstra K, Zeelenberg R. Participant nonnaiveté and the reproducibility of cognitive psychology. Psychonomic Bulletin & Review. 2017 doi: 10.3758/s13423-017-1348-y. [ PubMed ] [ CrossRef ]

Sociology Research Guide: Identifying & Finding Empirical Articles
- Evaluating Your Sources
- Types of Publications
- Identifying & Finding Empirical Articles
- Known Item Searching
- EndNote Web
How to Recognize Empirical Journal Articles
Definition of an empirical study: An empirical research article reports the results of a study that uses data derived from actual observation or experimentation. Empirical research articles are examples of primary research.
Parts of a standard empirical research article: (articles will not necessary use the exact terms listed below.)
- Abstract ... A paragraph length description of what the study includes.
- Introduction ...Includes a statement of the hypotheses for the research and a review of other research on the topic.
- Method ...A description of how the research was conducted, such as: ◊ Who are participants ◊ Design of the study ◊ What the participants did ◊ What measures were used
- Results ...Describes the outcomes of the measures of the study.
- Discussion...C ontains the interpretations and implications of the study.
- References...C ontains citation information on the material cited in the report. (also called bibliography or works cited)
Characteristics of an Empirical Article:
- Empirical articles will include charts, graphs, or statistical analysis.
- Empirical research articles are usually substantial, maybe from 8-30 pages long.
- There is always a bibliography found at the end of the article.
Type of publications that publish empirical studies:
- Empirical research articles are published in scholarly or academic journals These journals are also called “peer-reviewed,” or “refereed” publications.
- Examples of such publications include: ◊ American Journal of Sociology ◊ Sociological Quarterly ◊ Sociological Methods and Research
Databases that contain empirical research: (selected list only)
- Academic Search Premier add these words to your search terms: method* or research or research design or survey* or data or result*
- PsycINFO limit your searches by Form/Content Type to Empirical Study
- ERIC limit to Pub. Type to Reports - Research/Technical Change one dropdown box to Record. This finds REPORTS--RESEARCH.
- Sociological Abstracts 1963 - present.
- Sports Discus Change search limit to Level = Advanced for original scientific research
Empirical Articles - Sample Research Tips
Empirical articles detail original research/studies that have been done.
Some of the major components of empirical articles include the following: Abstract , Introduction , Method , Results , Discussion , References
Locating Empirical Articles in APA PsycINFO
Use the "Methodology" limiter to select: EMPIRICAL STUDY
Locating Empirical Articles in ERIC
Use the "Publication Type" limiter to select: Reports - Research
Locating Empirical Articles in Other Databases
Use terms such as: study , research , empirical , methods , methodology , research design , survey , data , results
Please remember to use OR between your combination of terms. For example, you may enter the following in one search box:
study OR research OR empirical
research OR methods OR data OR results
- << Previous: Articles
- Next: Known Item Searching >>
- Last Updated: Aug 13, 2024 11:02 AM
- URL: https://libraryguides.fullerton.edu/sociology
This site is maintained by Pollak Library .
To report problems or comments with this site, please contact [email protected] . © California State University, Fullerton. All Rights Reserved.
Web Accessibility
CSUF is committed to ensuring equal accessibility to our users. Let us know about any accessibility problems you encounter using this website. We'll do our best to improve things and get you the information you need.
- Adobe Reader
- Microsoft Viewers
- Report An ATI Issue
- Accessible @ CSUF

Empirical Research
- Search for Empirical Articles
- Related Guides
Ask a Librarian:
La Plata : 301-934-7676
Leonardtown : 240-725-5360
Prince Frederick : 443-550-6060
What is an Empirical Article?
An empirical research article reports research based on actual observation or experiment. The research may use quantitative or qualitative research methods.
Quantitative Research uses numerical data to try to establish causal relationships between variables (“Based on 100 interactions, A causes B.”)
Qualitative Research objectively and critically analyzes behaviors, beliefs, feelings, or other values (“People suffering from Illness A tend to be more cautious.”)
How to Identify an Empirical Article
What type of source is your article published in?
Popular Magazines ( Time , People , Psychology Today , WebMD , etc.): usually NOT empirical
Journals (Academic, Scholarly, Peer-reviewed, Professional): sometimes YES
An abstract is a brief summary or overview of the article. Abstracts for empirical research articles:
May describe a study, observation, or analysis
May mention participants or subjects, data, surveys, questionnaires, assessments, interviews, or other measurements
Empirical articles (and scholarly articles in general) are usually at least 5 pages (often up to 20 pages long).
Article Sections
Empirical articles may include headings or subheadings for sections such as:
Introduction
Literature Review
Methodology or Methods
Data & Analysis
Empirical research articles often include some sort of (quantitative and/or qualitative) data. This may be included in the article as charts, tables, graphs, or appendices.
Note: If you are not sure if an article is an empirical research article, share the article citation and abstract with your professor. This can help you to become better at recognizing the differences between empirical research and other types of scholarly articles, and also ensures your article is acceptable for the assignment.
- Empirical Research Printable handout with tips for searching for and identifying empirical research.
Empirical Research Articles [VIDEO]
- Empirical Articles [PDF] Slides from the Empirical Research Articles video.
- Next: Search for Empirical Articles >>
- Last Updated: Aug 21, 2023 12:50 PM
- URL: https://libguides.csmd.edu/empirical_research

- Introduction & Help
- DSM-5 & Reference Books
- Books & E-Books
- Find Empirical Research Articles
- Search Tips
- Streaming Video
- APA Style This link opens in a new window
Finding Empirical Research Articles
- Introduction
- Methods or Methodology
- Results or Findings
The method for finding empirical research articles varies depending upon the database* being used.
1. The PsycARTICLES and PsycInfo databases (both from the APA) includes a Methodology filter that can be used to identify empirical studies. Look for the filter on the Advanced Search screen. To see a list and description of all of the of methodology filter options in PsycARTICLES and PsycInfo visit the APA Databases Methodology Field Values page .

2. When using databases that do not provide a methodology filter—including ProQuest Psychology Journals and Academic Search Complete—experiment with using keywords to retrieve articles on your topic that contain empirical research. For example:
- empirical research
- empirical study
- quantitative study
- qualitative study
- longitudinal study
- observation
- questionnaire
- methodology
- participants
Qualitative research can be challenging to find as these methodologies are not always well-indexed in the databases. Here are some suggested keywords for retrieving articles that include qualitative research.
- qualitative
- ethnograph*
- observation*
- "case study”
- "focus group"
- "phenomenological research"
- "conversation analysis"
*Recommended databases are listed on the Databases: Find Journal Articles page of this guide.
- << Previous: Databases: Find Journal Articles
- Next: Search Tips >>
- Last Updated: Jun 18, 2024 9:09 AM
- URL: https://libguides.bentley.edu/psychology
Thank you for visiting nature.com. You are using a browser version with limited support for CSS. To obtain the best experience, we recommend you use a more up to date browser (or turn off compatibility mode in Internet Explorer). In the meantime, to ensure continued support, we are displaying the site without styles and JavaScript.
- View all journals
- Explore content
- About the journal
- Publish with us
- Sign up for alerts
- Review Article
- Published: 01 June 2023
Data, measurement and empirical methods in the science of science
- Lu Liu 1 , 2 , 3 , 4 ,
- Benjamin F. Jones ORCID: orcid.org/0000-0001-9697-9388 1 , 2 , 3 , 5 , 6 ,
- Brian Uzzi ORCID: orcid.org/0000-0001-6855-2854 1 , 2 , 3 &
- Dashun Wang ORCID: orcid.org/0000-0002-7054-2206 1 , 2 , 3 , 7
Nature Human Behaviour volume 7 , pages 1046–1058 ( 2023 ) Cite this article
20k Accesses
18 Citations
116 Altmetric
Metrics details
- Scientific community
The advent of large-scale datasets that trace the workings of science has encouraged researchers from many different disciplinary backgrounds to turn scientific methods into science itself, cultivating a rapidly expanding ‘science of science’. This Review considers this growing, multidisciplinary literature through the lens of data, measurement and empirical methods. We discuss the purposes, strengths and limitations of major empirical approaches, seeking to increase understanding of the field’s diverse methodologies and expand researchers’ toolkits. Overall, new empirical developments provide enormous capacity to test traditional beliefs and conceptual frameworks about science, discover factors associated with scientific productivity, predict scientific outcomes and design policies that facilitate scientific progress.
Similar content being viewed by others
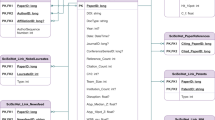
SciSciNet: A large-scale open data lake for the science of science research
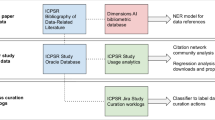
A dataset for measuring the impact of research data and their curation
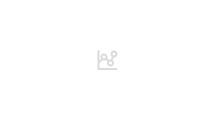
Envisioning a “science diplomacy 2.0”: on data, global challenges, and multi-layered networks
Scientific advances are a key input to rising standards of living, health and the capacity of society to confront grand challenges, from climate change to the COVID-19 pandemic 1 , 2 , 3 . A deeper understanding of how science works and where innovation occurs can help us to more effectively design science policy and science institutions, better inform scientists’ own research choices, and create and capture enormous value for science and humanity. Building on these key premises, recent years have witnessed substantial development in the ‘science of science’ 4 , 5 , 6 , 7 , 8 , 9 , which uses large-scale datasets and diverse computational toolkits to unearth fundamental patterns behind scientific production and use.
The idea of turning scientific methods into science itself is long-standing. Since the mid-20th century, researchers from different disciplines have asked central questions about the nature of scientific progress and the practice, organization and impact of scientific research. Building on these rich historical roots, the field of the science of science draws upon many disciplines, ranging from information science to the social, physical and biological sciences to computer science, engineering and design. The science of science closely relates to several strands and communities of research, including metascience, scientometrics, the economics of science, research on research, science and technology studies, the sociology of science, metaknowledge and quantitative science studies 5 . There are noticeable differences between some of these communities, mostly around their historical origins and the initial disciplinary composition of researchers forming these communities. For example, metascience has its origins in the clinical sciences and psychology, and focuses on rigour, transparency, reproducibility and other open science-related practices and topics. The scientometrics community, born in library and information sciences, places a particular emphasis on developing robust and responsible measures and indicators for science. Science and technology studies engage the history of science and technology, the philosophy of science, and the interplay between science, technology and society. The science of science, which has its origins in physics, computer science and sociology, takes a data-driven approach and emphasizes questions on how science works. Each of these communities has made fundamental contributions to understanding science. While they differ in their origins, these differences pale in comparison to the overarching, common interest in understanding the practice of science and its societal impact.
Three major developments have encouraged rapid advances in the science of science. The first is in data 9 : modern databases include millions of research articles, grant proposals, patents and more. This windfall of data traces scientific activity in remarkable detail and at scale. The second development is in measurement: scholars have used data to develop many new measures of scientific activities and examine theories that have long been viewed as important but difficult to quantify. The third development is in empirical methods: thanks to parallel advances in data science, network science, artificial intelligence and econometrics, researchers can study relationships, make predictions and assess science policy in powerful new ways. Together, new data, measurements and methods have revealed fundamental new insights about the inner workings of science and scientific progress itself.
With multiple approaches, however, comes a key challenge. As researchers adhere to norms respected within their disciplines, their methods vary, with results often published in venues with non-overlapping readership, fragmenting research along disciplinary boundaries. This fragmentation challenges researchers’ ability to appreciate and understand the value of work outside of their own discipline, much less to build directly on it for further investigations.
Recognizing these challenges and the rapidly developing nature of the field, this paper reviews the empirical approaches that are prevalent in this literature. We aim to provide readers with an up-to-date understanding of the available datasets, measurement constructs and empirical methodologies, as well as the value and limitations of each. Owing to space constraints, this Review does not cover the full technical details of each method, referring readers to related guides to learn more. Instead, we will emphasize why a researcher might favour one method over another, depending on the research question.
Beyond a positive understanding of science, a key goal of the science of science is to inform science policy. While this Review mainly focuses on empirical approaches, with its core audience being researchers in the field, the studies reviewed are also germane to key policy questions. For example, what is the appropriate scale of scientific investment, in what directions and through what institutions 10 , 11 ? Are public investments in science aligned with public interests 12 ? What conditions produce novel or high-impact science 13 , 14 , 15 , 16 , 17 , 18 , 19 , 20 ? How do the reward systems of science influence the rate and direction of progress 13 , 21 , 22 , 23 , 24 , and what governs scientific reproducibility 25 , 26 , 27 ? How do contributions evolve over a scientific career 28 , 29 , 30 , 31 , 32 , and how may diversity among scientists advance scientific progress 33 , 34 , 35 , among other questions relevant to science policy 36 , 37 .
Overall, this review aims to facilitate entry to science of science research, expand researcher toolkits and illustrate how diverse research approaches contribute to our collective understanding of science. Section 2 reviews datasets and data linkages. Section 3 reviews major measurement constructs in the science of science. Section 4 considers a range of empirical methods, focusing on one study to illustrate each method and briefly summarizing related examples and applications. Section 5 concludes with an outlook for the science of science.
Historically, data on scientific activities were difficult to collect and were available in limited quantities. Gathering data could involve manually tallying statistics from publications 38 , 39 , interviewing scientists 16 , 40 , or assembling historical anecdotes and biographies 13 , 41 . Analyses were typically limited to a specific domain or group of scientists. Today, massive datasets on scientific production and use are at researchers’ fingertips 42 , 43 , 44 . Armed with big data and advanced algorithms, researchers can now probe questions previously not amenable to quantification and with enormous increases in scope and scale, as detailed below.
Publication datasets cover papers from nearly all scientific disciplines, enabling analyses of both general and domain-specific patterns. Commonly used datasets include the Web of Science (WoS), PubMed, CrossRef, ORCID, OpenCitations, Dimensions and OpenAlex. Datasets incorporating papers’ text (CORE) 45 , 46 , 47 , data entities (DataCite) 48 , 49 and peer review reports (Publons) 33 , 50 , 51 have also become available. These datasets further enable novel measurement, for example, representations of a paper’s content 52 , 53 , novelty 15 , 54 and interdisciplinarity 55 .
Notably, databases today capture more diverse aspects of science beyond publications, offering a richer and more encompassing view of research contexts and of researchers themselves (Fig. 1 ). For example, some datasets trace research funding to the specific publications these investments support 56 , 57 , allowing high-scale studies of the impact of funding on productivity and the return on public investment. Datasets incorporating job placements 58 , 59 , curriculum vitae 21 , 59 and scientific prizes 23 offer rich quantitative evidence on the social structure of science. Combining publication profiles with mentorship genealogies 60 , 61 , dissertations 34 and course syllabi 62 , 63 provides insights on mentoring and cultivating talent.
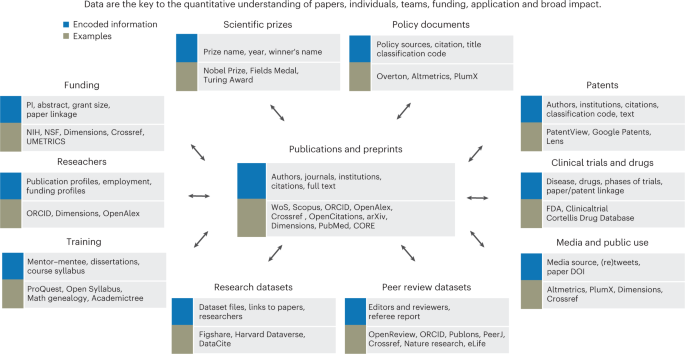
This figure presents commonly used data types in science of science research, information contained in each data type and examples of data sources. Datasets in the science of science research have not only grown in scale but have also expanded beyond publications to integrate upstream funding investments and downstream applications that extend beyond science itself.
Finally, today’s scope of data extends beyond science to broader aspects of society. Altmetrics 64 captures news media and social media mentions of scientific articles. Other databases incorporate marketplace uses of science, including through patents 10 , pharmaceutical clinical trials and drug approvals 65 , 66 . Policy documents 67 , 68 help us to understand the role of science in the halls of government 69 and policy making 12 , 68 .
While datasets of the modern scientific enterprise have grown exponentially, they are not without limitations. As is often the case for data-driven research, drawing conclusions from specific data sources requires scrutiny and care. Datasets are typically based on published work, which may favour easy-to-publish topics over important ones (the streetlight effect) 70 , 71 . The publication of negative results is also rare (the file drawer problem) 72 , 73 . Meanwhile, English language publications account for over 90% of articles in major data sources, with limited coverage of non-English journals 74 . Publication datasets may also reflect biases in data collection across research institutions or demographic groups. Despite the open science movement, many datasets require paid subscriptions, which can create inequality in data access. Creating more open datasets for the science of science, such as OpenAlex, may not only improve the robustness and replicability of empirical claims but also increase entry to the field.
As today’s datasets become larger in scale and continue to integrate new dimensions, they offer opportunities to unveil the inner workings and external impacts of science in new ways. They can enable researchers to reach beyond previous limitations while conducting original studies of new and long-standing questions about the sciences.
Measurement
Here we discuss prominent measurement approaches in the science of science, including their purposes and limitations.
Modern publication databases typically include data on which articles and authors cite other papers and scientists. These citation linkages have been used to engage core conceptual ideas in scientific research. Here we consider two common measures based on citation information: citation counts and knowledge flows.
First, citation counts are commonly used indicators of impact. The term ‘indicator’ implies that it only approximates the concept of interest. A citation count is defined as how many times a document is cited by subsequent documents and can proxy for the importance of research papers 75 , 76 as well as patented inventions 77 , 78 , 79 . Rather than treating each citation equally, measures may further weight the importance of each citation, for example by using the citation network structure to produce centrality 80 , PageRank 81 , 82 or Eigenfactor indicators 83 , 84 .
Citation-based indicators have also faced criticism 84 , 85 . Citation indicators necessarily oversimplify the construct of impact, often ignoring heterogeneity in the meaning and use of a particular reference, the variations in citation practices across fields and institutional contexts, and the potential for reputation and power structures in science to influence citation behaviour 86 , 87 . Researchers have started to understand more nuanced citation behaviours ranging from negative citations 86 to citation context 47 , 88 , 89 . Understanding what a citation actually measures matters in interpreting and applying many research findings in the science of science. Evaluations relying on citation-based indicators rather than expert judgements raise questions regarding misuse 90 , 91 , 92 . Given the importance of developing indicators that can reliably quantify and evaluate science, the scientometrics community has been working to provide guidance for responsible citation practices and assessment 85 .
Second, scientists use citations to trace knowledge flows. Each citation in a paper is a link to specific previous work from which we can proxy how new discoveries draw upon existing ideas 76 , 93 and how knowledge flows between fields of science 94 , 95 , research institutions 96 , regions and nations 97 , 98 , 99 , and individuals 81 . Combinations of citation linkages can also approximate novelty 15 , disruptiveness 17 , 100 and interdisciplinarity 55 , 95 , 101 , 102 . A rapidly expanding body of work further examines citations to scientific articles from other domains (for example, patents, clinical drug trials and policy documents) to understand the applied value of science 10 , 12 , 65 , 66 , 103 , 104 , 105 .
Individuals
Analysing individual careers allows researchers to answer questions such as: How do we quantify individual scientific productivity? What is a typical career lifecycle? How are resources and credits allocated across individuals and careers? A scholar’s career can be examined through the papers they publish 30 , 31 , 106 , 107 , 108 , with attention to career progression and mobility, publication counts and citation impact, as well as grant funding 24 , 109 , 110 and prizes 111 , 112 , 113 ,
Studies of individual impact focus on output, typically approximated by the number of papers a researcher publishes and citation indicators. A popular measure for individual impact is the h -index 114 , which takes both volume and per-paper impact into consideration. Specifically, a scientist is assigned the largest value h such that they have h papers that were each cited at least h times. Later studies build on the idea of the h -index and propose variants to address limitations 115 , these variants ranging from emphasizing highly cited papers in a career 116 , to field differences 117 and normalizations 118 , to the relative contribution of an individual in collaborative works 119 .
To study dynamics in output over the lifecycle, individuals can be studied according to age, career age or the sequence of publications. A long-standing literature has investigated the relationship between age and the likelihood of outstanding achievement 28 , 106 , 111 , 120 , 121 . Recent studies further decouple the relationship between age, publication volume and per-paper citation, and measure the likelihood of producing highly cited papers in the sequence of works one produces 30 , 31 .
As simple as it sounds, representing careers using publication records is difficult. Collecting the full publication list of a researcher is the foundation to study individuals yet remains a key challenge, requiring name disambiguation techniques to match specific works to specific researchers. Although algorithms are increasingly capable at identifying millions of career profiles 122 , they vary in accuracy and robustness. ORCID can help to alleviate the problem by offering researchers the opportunity to create, maintain and update individual profiles themselves, and it goes beyond publications to collect broader outputs and activities 123 . A second challenge is survivorship bias. Empirical studies tend to focus on careers that are long enough to afford statistical analyses, which limits the applicability of the findings to scientific careers as a whole. A third challenge is the breadth of scientists’ activities, where focusing on publications ignores other important contributions such as mentorship and teaching, service (for example, refereeing papers, reviewing grant proposals and editing journals) or leadership within their organizations. Although researchers have begun exploring these dimensions by linking individual publication profiles with genealogical databases 61 , 124 , dissertations 34 , grants 109 , curriculum vitae 21 and acknowledgements 125 , scientific careers beyond publication records remain under-studied 126 , 127 . Lastly, citation-based indicators only serve as an approximation of individual performance with similar limitations as discussed above. The scientific community has called for more appropriate practices 85 , 128 , ranging from incorporating expert assessment of research contributions to broadening the measures of impact beyond publications.
Over many decades, science has exhibited a substantial and steady shift away from solo authorship towards coauthorship, especially among highly cited works 18 , 129 , 130 . In light of this shift, a research field, the science of team science 131 , 132 , has emerged to study the mechanisms that facilitate or hinder the effectiveness of teams. Team size can be proxied by the number of coauthors on a paper, which has been shown to predict distinctive types of advance: whereas larger teams tend to develop ideas, smaller teams tend to disrupt current ways of thinking 17 . Team characteristics can be inferred from coauthors’ backgrounds 133 , 134 , 135 , allowing quantification of a team’s diversity in terms of field, age, gender or ethnicity. Collaboration networks based on coauthorship 130 , 136 , 137 , 138 , 139 offer nuanced network-based indicators to understand individual and institutional collaborations.
However, there are limitations to using coauthorship alone to study teams 132 . First, coauthorship can obscure individual roles 140 , 141 , 142 , which has prompted institutional responses to help to allocate credit, including authorship order and individual contribution statements 56 , 143 . Second, coauthorship does not reflect the complex dynamics and interactions between team members that are often instrumental for team success 53 , 144 . Third, collaborative contributions can extend beyond coauthorship in publications to include members of a research laboratory 145 or co-principal investigators (co-PIs) on a grant 146 . Initiatives such as CRediT may help to address some of these issues by recording detailed roles for each contributor 147 .
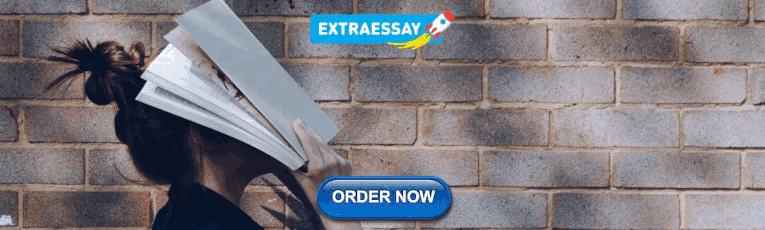
Institutions
Research institutions, such as departments, universities, national laboratories and firms, encompass wider groups of researchers and their corresponding outputs. Institutional membership can be inferred from affiliations listed on publications or patents 148 , 149 , and the output of an institution can be aggregated over all its affiliated researchers 150 . Institutional research information systems (CRIS) contain more comprehensive research outputs and activities from employees.
Some research questions consider the institution as a whole, investigating the returns to research and development investment 104 , inequality of resource allocation 22 and the flow of scientists 21 , 148 , 149 . Other questions focus on institutional structures as sources of research productivity by looking into the role of peer effects 125 , 151 , 152 , 153 , how institutional policies impact research outcomes 154 , 155 and whether interdisciplinary efforts foster innovation 55 . Institution-oriented measurement faces similar limitations as with analyses of individuals and teams, including name disambiguation for a given institution and the limited capacity of formal publication records to characterize the full range of relevant institutional outcomes. It is also unclear how to allocate credit among multiple institutions associated with a paper. Moreover, relevant institutional employees extend beyond publishing researchers: interns, technicians and administrators all contribute to research endeavours 130 .
In sum, measurements allow researchers to quantify scientific production and use across numerous dimensions, but they also raise questions of construct validity: Does the proposed metric really reflect what we want to measure? Testing the construct’s validity is important, as is understanding a construct’s limits. Where possible, using alternative measurement approaches, or qualitative methods such as interviews and surveys, can improve measurement accuracy and the robustness of findings.
Empirical methods
In this section, we review two broad categories of empirical approaches (Table 1 ), each with distinctive goals: (1) to discover, estimate and predict empirical regularities; and (2) to identify causal mechanisms. For each method, we give a concrete example to help to explain how the method works, summarize related work for interested readers, and discuss contributions and limitations.
Descriptive and predictive approaches
Empirical regularities and generalizable facts.
The discovery of empirical regularities in science has had a key role in driving conceptual developments and the directions of future research. By observing empirical patterns at scale, researchers unveil central facts that shape science and present core features that theories of scientific progress and practice must explain. For example, consider citation distributions. de Solla Price first proposed that citation distributions are fat-tailed 39 , indicating that a few papers have extremely high citations while most papers have relatively few or even no citations at all. de Solla Price proposed that citation distribution was a power law, while researchers have since refined this view to show that the distribution appears log-normal, a nearly universal regularity across time and fields 156 , 157 . The fat-tailed nature of citation distributions and its universality across the sciences has in turn sparked substantial theoretical work that seeks to explain this key empirical regularity 20 , 156 , 158 , 159 .
Empirical regularities are often surprising and can contest previous beliefs of how science works. For example, it has been shown that the age distribution of great achievements peaks in middle age across a wide range of fields 107 , 121 , 160 , rejecting the common belief that young scientists typically drive breakthroughs in science. A closer look at the individual careers also indicates that productivity patterns vary widely across individuals 29 . Further, a scholar’s highest-impact papers come at a remarkably constant rate across the sequence of their work 30 , 31 .
The discovery of empirical regularities has had important roles in shaping beliefs about the nature of science 10 , 45 , 161 , 162 , sources of breakthrough ideas 15 , 163 , 164 , 165 , scientific careers 21 , 29 , 126 , 127 , the network structure of ideas and scientists 23 , 98 , 136 , 137 , 138 , 139 , 166 , gender inequality 57 , 108 , 126 , 135 , 143 , 167 , 168 , and many other areas of interest to scientists and science institutions 22 , 47 , 86 , 97 , 102 , 105 , 134 , 169 , 170 , 171 . At the same time, care must be taken to ensure that findings are not merely artefacts due to data selection or inherent bias. To differentiate meaningful patterns from spurious ones, it is important to stress test the findings through different selection criteria or across non-overlapping data sources.
Regression analysis
When investigating correlations among variables, a classic method is regression, which estimates how one set of variables explains variation in an outcome of interest. Regression can be used to test explicit hypotheses or predict outcomes. For example, researchers have investigated whether a paper’s novelty predicts its citation impact 172 . Adding additional control variables to the regression, one can further examine the robustness of the focal relationship.
Although regression analysis is useful for hypothesis testing, it bears substantial limitations. If the question one wishes to ask concerns a ‘causal’ rather than a correlational relationship, regression is poorly suited to the task as it is impossible to control for all the confounding factors. Failing to account for such ‘omitted variables’ can bias the regression coefficient estimates and lead to spurious interpretations. Further, regression models often have low goodness of fit (small R 2 ), indicating that the variables considered explain little of the outcome variation. As regressions typically focus on a specific relationship in simple functional forms, regressions tend to emphasize interpretability rather than overall predictability. The advent of predictive approaches powered by large-scale datasets and novel computational techniques offers new opportunities for modelling complex relationships with stronger predictive power.
Mechanistic models
Mechanistic modelling is an important approach to explaining empirical regularities, drawing from methods primarily used in physics. Such models predict macro-level regularities of a system by modelling micro-level interactions among basic elements with interpretable and modifiable formulars. While theoretical by nature, mechanistic models in the science of science are often empirically grounded, and this approach has developed together with the advent of large-scale, high-resolution data.
Simplicity is the core value of a mechanistic model. Consider for example, why citations follow a fat-tailed distribution. de Solla Price modelled the citing behaviour as a cumulative advantage process on a growing citation network 159 and found that if the probability a paper is cited grows linearly with its existing citations, the resulting distribution would follow a power law, broadly aligned with empirical observations. The model is intentionally simplified, ignoring myriad factors. Yet the simple cumulative advantage process is by itself sufficient in explaining a power law distribution of citations. In this way, mechanistic models can help to reveal key mechanisms that can explain observed patterns.
Moreover, mechanistic models can be refined as empirical evidence evolves. For example, later investigations showed that citation distributions are better characterized as log-normal 156 , 173 , prompting researchers to introduce a fitness parameter to encapsulate the inherent differences in papers’ ability to attract citations 174 , 175 . Further, older papers are less likely to be cited than expected 176 , 177 , 178 , motivating more recent models 20 to introduce an additional aging effect 179 . By combining the cumulative advantage, fitness and aging effects, one can already achieve substantial predictive power not just for the overall properties of the system but also the citation dynamics of individual papers 20 .
In addition to citations, mechanistic models have been developed to understand the formation of collaborations 136 , 180 , 181 , 182 , 183 , knowledge discovery and diffusion 184 , 185 , topic selection 186 , 187 , career dynamics 30 , 31 , 188 , 189 , the growth of scientific fields 190 and the dynamics of failure in science and other domains 178 .
At the same time, some observers have argued that mechanistic models are too simplistic to capture the essence of complex real-world problems 191 . While it has been a cornerstone for the natural sciences, representing social phenomena in a limited set of mathematical equations may miss complexities and heterogeneities that make social phenomena interesting in the first place. Such concerns are not unique to the science of science, as they represent a broader theme in computational social sciences 192 , 193 , ranging from social networks 194 , 195 to human mobility 196 , 197 to epidemics 198 , 199 . Other observers have questioned the practical utility of mechanistic models and whether they can be used to guide decisions and devise actionable policies. Nevertheless, despite these limitations, several complex phenomena in the science of science are well captured by simple mechanistic models, showing a high degree of regularity beneath complex interacting systems and providing powerful insights about the nature of science. Mixing such modelling with other methods could be particularly fruitful in future investigations.
Machine learning
The science of science seeks in part to forecast promising directions for scientific research 7 , 44 . In recent years, machine learning methods have substantially advanced predictive capabilities 200 , 201 and are playing increasingly important parts in the science of science. In contrast to the previous methods, machine learning does not emphasize hypotheses or theories. Rather, it leverages complex relationships in data and optimizes goodness of fit to make predictions and categorizations.
Traditional machine learning models include supervised, semi-supervised and unsupervised learning. The model choice depends on data availability and the research question, ranging from supervised models for citation prediction 202 , 203 to unsupervised models for community detection 204 . Take for example mappings of scientific knowledge 94 , 205 , 206 . The unsupervised method applies network clustering algorithms to map the structures of science. Related visualization tools make sense of clusters from the underlying network, allowing observers to see the organization, interactions and evolution of scientific knowledge. More recently, supervised learning, and deep neural networks in particular, have witnessed especially rapid developments 207 . Neural networks can generate high-dimensional representations of unstructured data such as images and texts, which encode complex properties difficult for human experts to perceive.
Take text analysis as an example. A recent study 52 utilizes 3.3 million paper abstracts in materials science to predict the thermoelectric properties of materials. The intuition is that the words currently used to describe a material may predict its hitherto undiscovered properties (Fig. 2 ). Compared with a random material, the materials predicted by the model are eight times more likely to be reported as thermoelectric in the next 5 years, suggesting that machine learning has the potential to substantially speed up knowledge discovery, especially as data continue to grow in scale and scope. Indeed, predicting the direction of new discoveries represents one of the most promising avenues for machine learning models, with neural networks being applied widely to biology 208 , physics 209 , 210 , mathematics 211 , chemistry 212 , medicine 213 and clinical applications 214 . Neural networks also offer a quantitative framework to probe the characteristics of creative products ranging from scientific papers 53 , journals 215 , organizations 148 , to paintings and movies 32 . Neural networks can also help to predict the reproducibility of papers from a variety of disciplines at scale 53 , 216 .
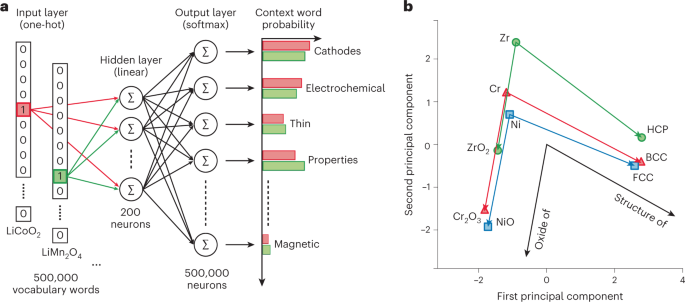
This figure illustrates the word2vec skip-gram methods 52 , where the goal is to predict useful properties of materials using previous scientific literature. a , The architecture and training process of the word2vec skip-gram model, where the 3-layer, fully connected neural network learns the 200-dimensional representation (hidden layer) from the sparse vector for each word and its context in the literature (input layer). b , The top two principal components of the word embedding. Materials with similar features are close in the 2D space, allowing prediction of a material’s properties. Different targeted words are shown in different colours. Reproduced with permission from ref. 52 , Springer Nature Ltd.
While machine learning can offer high predictive accuracy, successful applications to the science of science face challenges, particularly regarding interpretability. Researchers may value transparent and interpretable findings for how a given feature influences an outcome, rather than a black-box model. The lack of interpretability also raises concerns about bias and fairness. In predicting reproducible patterns from data, machine learning models inevitably include and reproduce biases embedded in these data, often in non-transparent ways. The fairness of machine learning 217 is heavily debated in applications ranging from the criminal justice system to hiring processes. Effective and responsible use of machine learning in the science of science therefore requires thoughtful partnership between humans and machines 53 to build a reliable system accessible to scrutiny and modification.
Causal approaches
The preceding methods can reveal core facts about the workings of science and develop predictive capacity. Yet, they fail to capture causal relationships, which are particularly useful in assessing policy interventions. For example, how can we test whether a science policy boosts or hinders the performance of individuals, teams or institutions? The overarching idea of causal approaches is to construct some counterfactual world where two groups are identical to each other except that one group experiences a treatment that the other group does not.
Towards causation
Before engaging in causal approaches, it is useful to first consider the interpretative challenges of observational data. As observational data emerge from mechanisms that are not fully known or measured, an observed correlation may be driven by underlying forces that were not accounted for in the analysis. This challenge makes causal inference fundamentally difficult in observational data. An awareness of this issue is the first step in confronting it. It further motivates intermediate empirical approaches, including the use of matching strategies and fixed effects, that can help to confront (although not fully eliminate) the inference challenge. We first consider these approaches before turning to more fully causal methods.
Matching. Matching utilizes rich information to construct a control group that is similar to the treatment group on as many observable characteristics as possible before the treatment group is exposed to the treatment. Inferences can then be made by comparing the treatment and the matched control groups. Exact matching applies to categorical values, such as country, gender, discipline or affiliation 35 , 218 . Coarsened exact matching considers percentile bins of continuous variables and matches observations in the same bin 133 . Propensity score matching estimates the probability of receiving the ‘treatment’ on the basis of the controlled variables and uses the estimates to match treatment and control groups, which reduces the matching task from comparing the values of multiple covariates to comparing a single value 24 , 219 . Dynamic matching is useful for longitudinally matching variables that change over time 220 , 221 .
Fixed effects. Fixed effects are a powerful and now standard tool in controlling for confounders. A key requirement for using fixed effects is that there are multiple observations on the same subject or entity (person, field, institution and so on) 222 , 223 , 224 . The fixed effect works as a dummy variable that accounts for the role of any fixed characteristic of that entity. Consider the finding where gender-diverse teams produce higher-impact papers than same-gender teams do 225 . A confounder may be that individuals who tend to write high-impact papers may also be more likely to work in gender-diverse teams. By including individual fixed effects, one accounts for any fixed characteristics of individuals (such as IQ, cultural background or previous education) that might drive the relationship of interest.
In sum, matching and fixed effects methods reduce potential sources of bias in interpreting relationships between variables. Yet, confounders may persist in these studies. For instance, fixed effects do not control for unobserved factors that change with time within the given entity (for example, access to funding or new skills). Identifying casual effects convincingly will then typically require distinct research methods that we turn to next.
Quasi-experiments
Researchers in economics and other fields have developed a range of quasi-experimental methods to construct treatment and control groups. The key idea here is exploiting randomness from external events that differentially expose subjects to a particular treatment. Here we review three quasi-experimental methods: difference-in-differences, instrumental variables and regression discontinuity (Fig. 3 ).

a – c , This figure presents illustrations of ( a ) differences-in-differences, ( b ) instrumental variables and ( c ) regression discontinuity methods. The solid line in b represents causal links and the dashed line represents the relationships that are not allowed, if the IV method is to produce causal inference.
Difference-in-differences. Difference-in-difference regression (DiD) investigates the effect of an unexpected event, comparing the affected group (the treated group) with an unaffected group (the control group). The control group is intended to provide the counterfactual path—what would have happened were it not for the unexpected event. Ideally, the treated and control groups are on virtually identical paths before the treatment event, but DiD can also work if the groups are on parallel paths (Fig. 3a ). For example, one study 226 examines how the premature death of superstar scientists affects the productivity of their previous collaborators. The control group are collaborators of superstars who did not die in the time frame. The two groups do not show significant differences in publications before a death event, yet upon the death of a star scientist, the treated collaborators on average experience a 5–8% decline in their quality-adjusted publication rates compared with the control group. DiD has wide applicability in the science of science, having been used to analyse the causal effects of grant design 24 , access costs to previous research 155 , 227 , university technology transfer policies 154 , intellectual property 228 , citation practices 229 , evolution of fields 221 and the impacts of paper retractions 230 , 231 , 232 . The DiD literature has grown especially rapidly in the field of economics, with substantial recent refinements 233 , 234 .
Instrumental variables. Another quasi-experimental approach utilizes ‘instrumental variables’ (IV). The goal is to determine the causal influence of some feature X on some outcome Y by using a third, instrumental variable. This instrumental variable is a quasi-random event that induces variation in X and, except for its impact through X , has no other effect on the outcome Y (Fig. 3b ). For example, consider a study of astronomy that seeks to understand how telescope time affects career advancement 235 . Here, one cannot simply look at the correlation between telescope time and career outcomes because many confounds (such as talent or grit) may influence both telescope time and career opportunities. Now consider the weather as an instrumental variable. Cloudy weather will, at random, reduce an astronomer’s observational time. Yet, the weather on particular nights is unlikely to correlate with a scientist’s innate qualities. The weather can then provide an instrumental variable to reveal a causal relationship between telescope time and career outcomes. Instrumental variables have been used to study local peer effects in research 151 , the impact of gender composition in scientific committees 236 , patents on future innovation 237 and taxes on inventor mobility 238 .
Regression discontinuity. In regression discontinuity, policies with an arbitrary threshold for receiving some benefit can be used to construct treatment and control groups (Fig. 3c ). Take the funding paylines for grant proposals as an example. Proposals with scores increasingly close to the payline are increasingly similar in their both observable and unobservable characteristics, yet only those projects with scores above the payline receive the funding. For example, a study 110 examines the effect of winning an early-career grant on the probability of winning a later, mid-career grant. The probability has a discontinuous jump across the initial grant’s payline, providing the treatment and control groups needed to estimate the causal effect of receiving a grant. This example utilizes the ‘sharp’ regression discontinuity that assumes treatment status to be fully determined by the cut-off. If we assume treatment status is only partly determined by the cut-off, we can use ‘fuzzy’ regression discontinuity designs. Here the probability of receiving a grant is used to estimate the future outcome 11 , 110 , 239 , 240 , 241 .
Although quasi-experiments are powerful tools, they face their own limitations. First, these approaches identify causal effects within a specific context and often engage small numbers of observations. How representative the samples are for broader populations or contexts is typically left as an open question. Second, the validity of the causal design is typically not ironclad. Researchers usually conduct different robustness checks to verify whether observable confounders have significant differences between the treated and control groups, before treatment. However, unobservable features may still differ between treatment and control groups. The quality of instrumental variables and the specific claim that they have no effect on the outcome except through the variable of interest, is also difficult to assess. Ultimately, researchers must rely partly on judgement to tell whether appropriate conditions are met for causal inference.
This section emphasized popular econometric approaches to causal inference. Other empirical approaches, such as graphical causal modelling 242 , 243 , also represent an important stream of work on assessing causal relationships. Such approaches usually represent causation as a directed acyclic graph, with nodes as variables and arrows between them as suspected causal relationships. In the science of science, the directed acyclic graph approach has been applied to quantify the causal effect of journal impact factor 244 and gender or racial bias 245 on citations. Graphical causal modelling has also triggered discussions on strengths and weaknesses compared to the econometrics methods 246 , 247 .
Experiments
In contrast to quasi-experimental approaches, laboratory and field experiments conduct direct randomization in assigning treatment and control groups. These methods engage explicitly in the data generation process, manipulating interventions to observe counterfactuals. These experiments are crafted to study mechanisms of specific interest and, by designing the experiment and formally randomizing, can produce especially rigorous causal inference.
Laboratory experiments. Laboratory experiments build counterfactual worlds in well-controlled laboratory environments. Researchers randomly assign participants to the treatment or control group and then manipulate the laboratory conditions to observe different outcomes in the two groups. For example, consider laboratory experiments on team performance and gender composition 144 , 248 . The researchers randomly assign participants into groups to perform tasks such as solving puzzles or brainstorming. Teams with a higher proportion of women are found to perform better on average, offering evidence that gender diversity is causally linked to team performance. Laboratory experiments can allow researchers to test forces that are otherwise hard to observe, such as how competition influences creativity 249 . Laboratory experiments have also been used to evaluate how journal impact factors shape scientists’ perceptions of rewards 250 and gender bias in hiring 251 .
Laboratory experiments allow for precise control of settings and procedures to isolate causal effects of interest. However, participants may behave differently in synthetic environments than in real-world settings, raising questions about the generalizability and replicability of the results 252 , 253 , 254 . To assess causal effects in real-world settings, researcher use randomized controlled trials.
Randomized controlled trials. A randomized controlled trial (RCT), or field experiment, is a staple for causal inference across a wide range of disciplines. RCTs randomly assign participants into the treatment and control conditions 255 and can be used not only to assess mechanisms but also to test real-world interventions such as policy change. The science of science has witnessed growing use of RCTs. For instance, a field experiment 146 investigated whether lower search costs for collaborators increased collaboration in grant applications. The authors randomly allocated principal investigators to face-to-face sessions in a medical school, and then measured participants’ chance of writing a grant proposal together. RCTs have also offered rich causal insights on peer review 256 , 257 , 258 , 259 , 260 and gender bias in science 261 , 262 , 263 .
While powerful, RCTs are difficult to conduct in the science of science, mainly for two reasons. The first concerns potential risks in a policy intervention. For instance, while randomizing funding across individuals could generate crucial causal insights for funders, it may also inadvertently harm participants’ careers 264 . Second, key questions in the science of science often require a long-time horizon to trace outcomes, which makes RCTs costly. It also raises the difficulty of replicating findings. A relative advantage of the quasi-experimental methods discussed earlier is that one can identify causal effects over potentially long periods of time in the historical record. On the other hand, quasi-experiments must be found as opposed to designed, and they often are not available for many questions of interest. While the best approaches are context dependent, a growing community of researchers is building platforms to facilitate RCTs for the science of science, aiming to lower their costs and increase their scale. Performing RCTs in partnership with science institutions can also contribute to timely, policy-relevant research that may substantially improve science decision-making and investments.
Research in the science of science has been empowered by the growth of high-scale data, new measurement approaches and an expanding range of empirical methods. These tools provide enormous capacity to test conceptual frameworks about science, discover factors impacting scientific productivity, predict key scientific outcomes and design policies that better facilitate future scientific progress. A careful appreciation of empirical techniques can help researchers to choose effective tools for questions of interest and propel the field. A better and broader understanding of these methodologies may also build bridges across diverse research communities, facilitating communication and collaboration, and better leveraging the value of diverse perspectives. The science of science is about turning scientific methods on the nature of science itself. The fruits of this work, with time, can guide researchers and research institutions to greater progress in discovery and understanding across the landscape of scientific inquiry.
Bush, V . S cience–the Endless Frontier: A Report to the President on a Program for Postwar Scientific Research (National Science Foundation, 1990).
Mokyr, J. The Gifts of Athena (Princeton Univ. Press, 2011).
Jones, B. F. in Rebuilding the Post-Pandemic Economy (eds Kearney, M. S. & Ganz, A.) 272–310 (Aspen Institute Press, 2021).
Wang, D. & Barabási, A.-L. The Science of Science (Cambridge Univ. Press, 2021).
Fortunato, S. et al. Science of science. Science 359 , eaao0185 (2018).
Article PubMed PubMed Central Google Scholar
Azoulay, P. et al. Toward a more scientific science. Science 361 , 1194–1197 (2018).
Article PubMed Google Scholar
Clauset, A., Larremore, D. B. & Sinatra, R. Data-driven predictions in the science of science. Science 355 , 477–480 (2017).
Article CAS PubMed Google Scholar
Zeng, A. et al. The science of science: from the perspective of complex systems. Phys. Rep. 714 , 1–73 (2017).
Article Google Scholar
Lin, Z., Yin. Y., Liu, L. & Wang, D. SciSciNet: a large-scale open data lake for the science of science research. Sci. Data, https://doi.org/10.1038/s41597-023-02198-9 (2023).
Ahmadpoor, M. & Jones, B. F. The dual frontier: patented inventions and prior scientific advance. Science 357 , 583–587 (2017).
Azoulay, P., Graff Zivin, J. S., Li, D. & Sampat, B. N. Public R&D investments and private-sector patenting: evidence from NIH funding rules. Rev. Econ. Stud. 86 , 117–152 (2019).
Yin, Y., Dong, Y., Wang, K., Wang, D. & Jones, B. F. Public use and public funding of science. Nat. Hum. Behav. 6 , 1344–1350 (2022).
Merton, R. K. The Sociology of Science: Theoretical and Empirical Investigations (Univ. Chicago Press, 1973).
Kuhn, T. The Structure of Scientific Revolutions (Princeton Univ. Press, 2021).
Uzzi, B., Mukherjee, S., Stringer, M. & Jones, B. Atypical combinations and scientific impact. Science 342 , 468–472 (2013).
Zuckerman, H. Scientific Elite: Nobel Laureates in the United States (Transaction Publishers, 1977).
Wu, L., Wang, D. & Evans, J. A. Large teams develop and small teams disrupt science and technology. Nature 566 , 378–382 (2019).
Wuchty, S., Jones, B. F. & Uzzi, B. The increasing dominance of teams in production of knowledge. Science 316 , 1036–1039 (2007).
Foster, J. G., Rzhetsky, A. & Evans, J. A. Tradition and innovation in scientists’ research strategies. Am. Sociol. Rev. 80 , 875–908 (2015).
Wang, D., Song, C. & Barabási, A.-L. Quantifying long-term scientific impact. Science 342 , 127–132 (2013).
Clauset, A., Arbesman, S. & Larremore, D. B. Systematic inequality and hierarchy in faculty hiring networks. Sci. Adv. 1 , e1400005 (2015).
Ma, A., Mondragón, R. J. & Latora, V. Anatomy of funded research in science. Proc. Natl Acad. Sci. USA 112 , 14760–14765 (2015).
Article CAS PubMed PubMed Central Google Scholar
Ma, Y. & Uzzi, B. Scientific prize network predicts who pushes the boundaries of science. Proc. Natl Acad. Sci. USA 115 , 12608–12615 (2018).
Azoulay, P., Graff Zivin, J. S. & Manso, G. Incentives and creativity: evidence from the academic life sciences. RAND J. Econ. 42 , 527–554 (2011).
Schor, S. & Karten, I. Statistical evaluation of medical journal manuscripts. JAMA 195 , 1123–1128 (1966).
Platt, J. R. Strong inference: certain systematic methods of scientific thinking may produce much more rapid progress than others. Science 146 , 347–353 (1964).
Ioannidis, J. P. Why most published research findings are false. PLoS Med. 2 , e124 (2005).
Simonton, D. K. Career landmarks in science: individual differences and interdisciplinary contrasts. Dev. Psychol. 27 , 119 (1991).
Way, S. F., Morgan, A. C., Clauset, A. & Larremore, D. B. The misleading narrative of the canonical faculty productivity trajectory. Proc. Natl Acad. Sci. USA 114 , E9216–E9223 (2017).
Sinatra, R., Wang, D., Deville, P., Song, C. & Barabási, A.-L. Quantifying the evolution of individual scientific impact. Science 354 , aaf5239 (2016).
Liu, L. et al. Hot streaks in artistic, cultural, and scientific careers. Nature 559 , 396–399 (2018).
Liu, L., Dehmamy, N., Chown, J., Giles, C. L. & Wang, D. Understanding the onset of hot streaks across artistic, cultural, and scientific careers. Nat. Commun. 12 , 5392 (2021).
Squazzoni, F. et al. Peer review and gender bias: a study on 145 scholarly journals. Sci. Adv. 7 , eabd0299 (2021).
Hofstra, B. et al. The diversity–innovation paradox in science. Proc. Natl Acad. Sci. USA 117 , 9284–9291 (2020).
Huang, J., Gates, A. J., Sinatra, R. & Barabási, A.-L. Historical comparison of gender inequality in scientific careers across countries and disciplines. Proc. Natl Acad. Sci. USA 117 , 4609–4616 (2020).
Gläser, J. & Laudel, G. Governing science: how science policy shapes research content. Eur. J. Sociol. 57 , 117–168 (2016).
Stephan, P. E. How Economics Shapes Science (Harvard Univ. Press, 2012).
Garfield, E. & Sher, I. H. New factors in the evaluation of scientific literature through citation indexing. Am. Doc. 14 , 195–201 (1963).
Article CAS Google Scholar
de Solla Price, D. J. Networks of scientific papers. Science 149 , 510–515 (1965).
Etzkowitz, H., Kemelgor, C. & Uzzi, B. Athena Unbound: The Advancement of Women in Science and Technology (Cambridge Univ. Press, 2000).
Simonton, D. K. Scientific Genius: A Psychology of Science (Cambridge Univ. Press, 1988).
Khabsa, M. & Giles, C. L. The number of scholarly documents on the public web. PLoS ONE 9 , e93949 (2014).
Xia, F., Wang, W., Bekele, T. M. & Liu, H. Big scholarly data: a survey. IEEE Trans. Big Data 3 , 18–35 (2017).
Evans, J. A. & Foster, J. G. Metaknowledge. Science 331 , 721–725 (2011).
Milojević, S. Quantifying the cognitive extent of science. J. Informetr. 9 , 962–973 (2015).
Rzhetsky, A., Foster, J. G., Foster, I. T. & Evans, J. A. Choosing experiments to accelerate collective discovery. Proc. Natl Acad. Sci. USA 112 , 14569–14574 (2015).
Poncela-Casasnovas, J., Gerlach, M., Aguirre, N. & Amaral, L. A. Large-scale analysis of micro-level citation patterns reveals nuanced selection criteria. Nat. Hum. Behav. 3 , 568–575 (2019).
Hardwicke, T. E. et al. Data availability, reusability, and analytic reproducibility: evaluating the impact of a mandatory open data policy at the journal Cognition. R. Soc. Open Sci. 5 , 180448 (2018).
Nagaraj, A., Shears, E. & de Vaan, M. Improving data access democratizes and diversifies science. Proc. Natl Acad. Sci. USA 117 , 23490–23498 (2020).
Bravo, G., Grimaldo, F., López-Iñesta, E., Mehmani, B. & Squazzoni, F. The effect of publishing peer review reports on referee behavior in five scholarly journals. Nat. Commun. 10 , 322 (2019).
Tran, D. et al. An open review of open review: a critical analysis of the machine learning conference review process. Preprint at https://doi.org/10.48550/arXiv.2010.05137 (2020).
Tshitoyan, V. et al. Unsupervised word embeddings capture latent knowledge from materials science literature. Nature 571 , 95–98 (2019).
Yang, Y., Wu, Y. & Uzzi, B. Estimating the deep replicability of scientific findings using human and artificial intelligence. Proc. Natl Acad. Sci. USA 117 , 10762–10768 (2020).
Mukherjee, S., Uzzi, B., Jones, B. & Stringer, M. A new method for identifying recombinations of existing knowledge associated with high‐impact innovation. J. Prod. Innov. Manage. 33 , 224–236 (2016).
Leahey, E., Beckman, C. M. & Stanko, T. L. Prominent but less productive: the impact of interdisciplinarity on scientists’ research. Adm. Sci. Q. 62 , 105–139 (2017).
Sauermann, H. & Haeussler, C. Authorship and contribution disclosures. Sci. Adv. 3 , e1700404 (2017).
Oliveira, D. F. M., Ma, Y., Woodruff, T. K. & Uzzi, B. Comparison of National Institutes of Health grant amounts to first-time male and female principal investigators. JAMA 321 , 898–900 (2019).
Yang, Y., Chawla, N. V. & Uzzi, B. A network’s gender composition and communication pattern predict women’s leadership success. Proc. Natl Acad. Sci. USA 116 , 2033–2038 (2019).
Way, S. F., Larremore, D. B. & Clauset, A. Gender, productivity, and prestige in computer science faculty hiring networks. In Proc. 25th International Conference on World Wide Web 1169–1179. (ACM 2016)
Malmgren, R. D., Ottino, J. M. & Amaral, L. A. N. The role of mentorship in protege performance. Nature 465 , 622–626 (2010).
Ma, Y., Mukherjee, S. & Uzzi, B. Mentorship and protégé success in STEM fields. Proc. Natl Acad. Sci. USA 117 , 14077–14083 (2020).
Börner, K. et al. Skill discrepancies between research, education, and jobs reveal the critical need to supply soft skills for the data economy. Proc. Natl Acad. Sci. USA 115 , 12630–12637 (2018).
Biasi, B. & Ma, S. The Education-Innovation Gap (National Bureau of Economic Research Working papers, 2020).
Bornmann, L. Do altmetrics point to the broader impact of research? An overview of benefits and disadvantages of altmetrics. J. Informetr. 8 , 895–903 (2014).
Cleary, E. G., Beierlein, J. M., Khanuja, N. S., McNamee, L. M. & Ledley, F. D. Contribution of NIH funding to new drug approvals 2010–2016. Proc. Natl Acad. Sci. USA 115 , 2329–2334 (2018).
Spector, J. M., Harrison, R. S. & Fishman, M. C. Fundamental science behind today’s important medicines. Sci. Transl. Med. 10 , eaaq1787 (2018).
Haunschild, R. & Bornmann, L. How many scientific papers are mentioned in policy-related documents? An empirical investigation using Web of Science and Altmetric data. Scientometrics 110 , 1209–1216 (2017).
Yin, Y., Gao, J., Jones, B. F. & Wang, D. Coevolution of policy and science during the pandemic. Science 371 , 128–130 (2021).
Sugimoto, C. R., Work, S., Larivière, V. & Haustein, S. Scholarly use of social media and altmetrics: a review of the literature. J. Assoc. Inf. Sci. Technol. 68 , 2037–2062 (2017).
Dunham, I. Human genes: time to follow the roads less traveled? PLoS Biol. 16 , e3000034 (2018).
Kustatscher, G. et al. Understudied proteins: opportunities and challenges for functional proteomics. Nat. Methods 19 , 774–779 (2022).
Rosenthal, R. The file drawer problem and tolerance for null results. Psychol. Bull. 86 , 638 (1979).
Franco, A., Malhotra, N. & Simonovits, G. Publication bias in the social sciences: unlocking the file drawer. Science 345 , 1502–1505 (2014).
Vera-Baceta, M.-A., Thelwall, M. & Kousha, K. Web of Science and Scopus language coverage. Scientometrics 121 , 1803–1813 (2019).
Waltman, L. A review of the literature on citation impact indicators. J. Informetr. 10 , 365–391 (2016).
Garfield, E. & Merton, R. K. Citation Indexing: Its Theory and Application in Science, Technology, and Humanities (Wiley, 1979).
Kelly, B., Papanikolaou, D., Seru, A. & Taddy, M. Measuring Technological Innovation Over the Long Run Report No. 0898-2937 (National Bureau of Economic Research, 2018).
Kogan, L., Papanikolaou, D., Seru, A. & Stoffman, N. Technological innovation, resource allocation, and growth. Q. J. Econ. 132 , 665–712 (2017).
Hall, B. H., Jaffe, A. & Trajtenberg, M. Market value and patent citations. RAND J. Econ. 36 , 16–38 (2005).
Google Scholar
Yan, E. & Ding, Y. Applying centrality measures to impact analysis: a coauthorship network analysis. J. Am. Soc. Inf. Sci. Technol. 60 , 2107–2118 (2009).
Radicchi, F., Fortunato, S., Markines, B. & Vespignani, A. Diffusion of scientific credits and the ranking of scientists. Phys. Rev. E 80 , 056103 (2009).
Bollen, J., Rodriquez, M. A. & Van de Sompel, H. Journal status. Scientometrics 69 , 669–687 (2006).
Bergstrom, C. T., West, J. D. & Wiseman, M. A. The eigenfactor™ metrics. J. Neurosci. 28 , 11433–11434 (2008).
Cronin, B. & Sugimoto, C. R. Beyond Bibliometrics: Harnessing Multidimensional Indicators of Scholarly Impact (MIT Press, 2014).
Hicks, D., Wouters, P., Waltman, L., De Rijcke, S. & Rafols, I. Bibliometrics: the Leiden Manifesto for research metrics. Nature 520 , 429–431 (2015).
Catalini, C., Lacetera, N. & Oettl, A. The incidence and role of negative citations in science. Proc. Natl Acad. Sci. USA 112 , 13823–13826 (2015).
Alcacer, J. & Gittelman, M. Patent citations as a measure of knowledge flows: the influence of examiner citations. Rev. Econ. Stat. 88 , 774–779 (2006).
Ding, Y. et al. Content‐based citation analysis: the next generation of citation analysis. J. Assoc. Inf. Sci. Technol. 65 , 1820–1833 (2014).
Teufel, S., Siddharthan, A. & Tidhar, D. Automatic classification of citation function. In Proc. 2006 Conference on Empirical Methods in Natural Language Processing, 103–110 (Association for Computational Linguistics 2006)
Seeber, M., Cattaneo, M., Meoli, M. & Malighetti, P. Self-citations as strategic response to the use of metrics for career decisions. Res. Policy 48 , 478–491 (2019).
Pendlebury, D. A. The use and misuse of journal metrics and other citation indicators. Arch. Immunol. Ther. Exp. 57 , 1–11 (2009).
Biagioli, M. Watch out for cheats in citation game. Nature 535 , 201 (2016).
Jo, W. S., Liu, L. & Wang, D. See further upon the giants: quantifying intellectual lineage in science. Quant. Sci. Stud. 3 , 319–330 (2022).
Boyack, K. W., Klavans, R. & Börner, K. Mapping the backbone of science. Scientometrics 64 , 351–374 (2005).
Gates, A. J., Ke, Q., Varol, O. & Barabási, A.-L. Nature’s reach: narrow work has broad impact. Nature 575 , 32–34 (2019).
Börner, K., Penumarthy, S., Meiss, M. & Ke, W. Mapping the diffusion of scholarly knowledge among major US research institutions. Scientometrics 68 , 415–426 (2006).
King, D. A. The scientific impact of nations. Nature 430 , 311–316 (2004).
Pan, R. K., Kaski, K. & Fortunato, S. World citation and collaboration networks: uncovering the role of geography in science. Sci. Rep. 2 , 902 (2012).
Jaffe, A. B., Trajtenberg, M. & Henderson, R. Geographic localization of knowledge spillovers as evidenced by patent citations. Q. J. Econ. 108 , 577–598 (1993).
Funk, R. J. & Owen-Smith, J. A dynamic network measure of technological change. Manage. Sci. 63 , 791–817 (2017).
Yegros-Yegros, A., Rafols, I. & D’este, P. Does interdisciplinary research lead to higher citation impact? The different effect of proximal and distal interdisciplinarity. PLoS ONE 10 , e0135095 (2015).
Larivière, V., Haustein, S. & Börner, K. Long-distance interdisciplinarity leads to higher scientific impact. PLoS ONE 10 , e0122565 (2015).
Fleming, L., Greene, H., Li, G., Marx, M. & Yao, D. Government-funded research increasingly fuels innovation. Science 364 , 1139–1141 (2019).
Bowen, A. & Casadevall, A. Increasing disparities between resource inputs and outcomes, as measured by certain health deliverables, in biomedical research. Proc. Natl Acad. Sci. USA 112 , 11335–11340 (2015).
Li, D., Azoulay, P. & Sampat, B. N. The applied value of public investments in biomedical research. Science 356 , 78–81 (2017).
Lehman, H. C. Age and Achievement (Princeton Univ. Press, 2017).
Simonton, D. K. Creative productivity: a predictive and explanatory model of career trajectories and landmarks. Psychol. Rev. 104 , 66 (1997).
Duch, J. et al. The possible role of resource requirements and academic career-choice risk on gender differences in publication rate and impact. PLoS ONE 7 , e51332 (2012).
Wang, Y., Jones, B. F. & Wang, D. Early-career setback and future career impact. Nat. Commun. 10 , 4331 (2019).
Bol, T., de Vaan, M. & van de Rijt, A. The Matthew effect in science funding. Proc. Natl Acad. Sci. USA 115 , 4887–4890 (2018).
Jones, B. F. Age and great invention. Rev. Econ. Stat. 92 , 1–14 (2010).
Newman, M. Networks (Oxford Univ. Press, 2018).
Mazloumian, A., Eom, Y.-H., Helbing, D., Lozano, S. & Fortunato, S. How citation boosts promote scientific paradigm shifts and nobel prizes. PLoS ONE 6 , e18975 (2011).
Hirsch, J. E. An index to quantify an individual’s scientific research output. Proc. Natl Acad. Sci. USA 102 , 16569–16572 (2005).
Alonso, S., Cabrerizo, F. J., Herrera-Viedma, E. & Herrera, F. h-index: a review focused in its variants, computation and standardization for different scientific fields. J. Informetr. 3 , 273–289 (2009).
Egghe, L. An improvement of the h-index: the g-index. ISSI Newsl. 2 , 8–9 (2006).
Kaur, J., Radicchi, F. & Menczer, F. Universality of scholarly impact metrics. J. Informetr. 7 , 924–932 (2013).
Majeti, D. et al. Scholar plot: design and evaluation of an information interface for faculty research performance. Front. Res. Metr. Anal. 4 , 6 (2020).
Sidiropoulos, A., Katsaros, D. & Manolopoulos, Y. Generalized Hirsch h-index for disclosing latent facts in citation networks. Scientometrics 72 , 253–280 (2007).
Jones, B. F. & Weinberg, B. A. Age dynamics in scientific creativity. Proc. Natl Acad. Sci. USA 108 , 18910–18914 (2011).
Dennis, W. Age and productivity among scientists. Science 123 , 724–725 (1956).
Sanyal, D. K., Bhowmick, P. K. & Das, P. P. A review of author name disambiguation techniques for the PubMed bibliographic database. J. Inf. Sci. 47 , 227–254 (2021).
Haak, L. L., Fenner, M., Paglione, L., Pentz, E. & Ratner, H. ORCID: a system to uniquely identify researchers. Learn. Publ. 25 , 259–264 (2012).
Malmgren, R. D., Ottino, J. M. & Amaral, L. A. N. The role of mentorship in protégé performance. Nature 465 , 662–667 (2010).
Oettl, A. Reconceptualizing stars: scientist helpfulness and peer performance. Manage. Sci. 58 , 1122–1140 (2012).
Morgan, A. C. et al. The unequal impact of parenthood in academia. Sci. Adv. 7 , eabd1996 (2021).
Morgan, A. C. et al. Socioeconomic roots of academic faculty. Nat. Hum. Behav. 6 , 1625–1633 (2022).
San Francisco Declaration on Research Assessment (DORA) (American Society for Cell Biology, 2012).
Falk‐Krzesinski, H. J. et al. Advancing the science of team science. Clin. Transl. Sci. 3 , 263–266 (2010).
Cooke, N. J. et al. Enhancing the Effectiveness of Team Science (National Academies Press, 2015).
Börner, K. et al. A multi-level systems perspective for the science of team science. Sci. Transl. Med. 2 , 49cm24 (2010).
Leahey, E. From sole investigator to team scientist: trends in the practice and study of research collaboration. Annu. Rev. Sociol. 42 , 81–100 (2016).
AlShebli, B. K., Rahwan, T. & Woon, W. L. The preeminence of ethnic diversity in scientific collaboration. Nat. Commun. 9 , 5163 (2018).
Hsiehchen, D., Espinoza, M. & Hsieh, A. Multinational teams and diseconomies of scale in collaborative research. Sci. Adv. 1 , e1500211 (2015).
Koning, R., Samila, S. & Ferguson, J.-P. Who do we invent for? Patents by women focus more on women’s health, but few women get to invent. Science 372 , 1345–1348 (2021).
Barabâsi, A.-L. et al. Evolution of the social network of scientific collaborations. Physica A 311 , 590–614 (2002).
Newman, M. E. Scientific collaboration networks. I. Network construction and fundamental results. Phys. Rev. E 64 , 016131 (2001).
Newman, M. E. Scientific collaboration networks. II. Shortest paths, weighted networks, and centrality. Phys. Rev. E 64 , 016132 (2001).
Palla, G., Barabási, A.-L. & Vicsek, T. Quantifying social group evolution. Nature 446 , 664–667 (2007).
Ross, M. B. et al. Women are credited less in science than men. Nature 608 , 135–145 (2022).
Shen, H.-W. & Barabási, A.-L. Collective credit allocation in science. Proc. Natl Acad. Sci. USA 111 , 12325–12330 (2014).
Merton, R. K. Matthew effect in science. Science 159 , 56–63 (1968).
Ni, C., Smith, E., Yuan, H., Larivière, V. & Sugimoto, C. R. The gendered nature of authorship. Sci. Adv. 7 , eabe4639 (2021).
Woolley, A. W., Chabris, C. F., Pentland, A., Hashmi, N. & Malone, T. W. Evidence for a collective intelligence factor in the performance of human groups. Science 330 , 686–688 (2010).
Feldon, D. F. et al. Postdocs’ lab engagement predicts trajectories of PhD students’ skill development. Proc. Natl Acad. Sci. USA 116 , 20910–20916 (2019).
Boudreau, K. J. et al. A field experiment on search costs and the formation of scientific collaborations. Rev. Econ. Stat. 99 , 565–576 (2017).
Holcombe, A. O. Contributorship, not authorship: use CRediT to indicate who did what. Publications 7 , 48 (2019).
Murray, D. et al. Unsupervised embedding of trajectories captures the latent structure of mobility. Preprint at https://doi.org/10.48550/arXiv.2012.02785 (2020).
Deville, P. et al. Career on the move: geography, stratification, and scientific impact. Sci. Rep. 4 , 4770 (2014).
Edmunds, L. D. et al. Why do women choose or reject careers in academic medicine? A narrative review of empirical evidence. Lancet 388 , 2948–2958 (2016).
Waldinger, F. Peer effects in science: evidence from the dismissal of scientists in Nazi Germany. Rev. Econ. Stud. 79 , 838–861 (2012).
Agrawal, A., McHale, J. & Oettl, A. How stars matter: recruiting and peer effects in evolutionary biology. Res. Policy 46 , 853–867 (2017).
Fiore, S. M. Interdisciplinarity as teamwork: how the science of teams can inform team science. Small Group Res. 39 , 251–277 (2008).
Hvide, H. K. & Jones, B. F. University innovation and the professor’s privilege. Am. Econ. Rev. 108 , 1860–1898 (2018).
Murray, F., Aghion, P., Dewatripont, M., Kolev, J. & Stern, S. Of mice and academics: examining the effect of openness on innovation. Am. Econ. J. Econ. Policy 8 , 212–252 (2016).
Radicchi, F., Fortunato, S. & Castellano, C. Universality of citation distributions: toward an objective measure of scientific impact. Proc. Natl Acad. Sci. USA 105 , 17268–17272 (2008).
Waltman, L., van Eck, N. J. & van Raan, A. F. Universality of citation distributions revisited. J. Am. Soc. Inf. Sci. Technol. 63 , 72–77 (2012).
Barabási, A.-L. & Albert, R. Emergence of scaling in random networks. Science 286 , 509–512 (1999).
de Solla Price, D. A general theory of bibliometric and other cumulative advantage processes. J. Am. Soc. Inf. Sci. 27 , 292–306 (1976).
Cole, S. Age and scientific performance. Am. J. Sociol. 84 , 958–977 (1979).
Ke, Q., Ferrara, E., Radicchi, F. & Flammini, A. Defining and identifying sleeping beauties in science. Proc. Natl Acad. Sci. USA 112 , 7426–7431 (2015).
Bornmann, L., de Moya Anegón, F. & Leydesdorff, L. Do scientific advancements lean on the shoulders of giants? A bibliometric investigation of the Ortega hypothesis. PLoS ONE 5 , e13327 (2010).
Mukherjee, S., Romero, D. M., Jones, B. & Uzzi, B. The nearly universal link between the age of past knowledge and tomorrow’s breakthroughs in science and technology: the hotspot. Sci. Adv. 3 , e1601315 (2017).
Packalen, M. & Bhattacharya, J. NIH funding and the pursuit of edge science. Proc. Natl Acad. Sci. USA 117 , 12011–12016 (2020).
Zeng, A., Fan, Y., Di, Z., Wang, Y. & Havlin, S. Fresh teams are associated with original and multidisciplinary research. Nat. Hum. Behav. 5 , 1314–1322 (2021).
Newman, M. E. The structure of scientific collaboration networks. Proc. Natl Acad. Sci. USA 98 , 404–409 (2001).
Larivière, V., Ni, C., Gingras, Y., Cronin, B. & Sugimoto, C. R. Bibliometrics: global gender disparities in science. Nature 504 , 211–213 (2013).
West, J. D., Jacquet, J., King, M. M., Correll, S. J. & Bergstrom, C. T. The role of gender in scholarly authorship. PLoS ONE 8 , e66212 (2013).
Gao, J., Yin, Y., Myers, K. R., Lakhani, K. R. & Wang, D. Potentially long-lasting effects of the pandemic on scientists. Nat. Commun. 12 , 6188 (2021).
Jones, B. F., Wuchty, S. & Uzzi, B. Multi-university research teams: shifting impact, geography, and stratification in science. Science 322 , 1259–1262 (2008).
Chu, J. S. & Evans, J. A. Slowed canonical progress in large fields of science. Proc. Natl Acad. Sci. USA 118 , e2021636118 (2021).
Wang, J., Veugelers, R. & Stephan, P. Bias against novelty in science: a cautionary tale for users of bibliometric indicators. Res. Policy 46 , 1416–1436 (2017).
Stringer, M. J., Sales-Pardo, M. & Amaral, L. A. Statistical validation of a global model for the distribution of the ultimate number of citations accrued by papers published in a scientific journal. J. Assoc. Inf. Sci. Technol. 61 , 1377–1385 (2010).
Bianconi, G. & Barabási, A.-L. Bose-Einstein condensation in complex networks. Phys. Rev. Lett. 86 , 5632 (2001).
Bianconi, G. & Barabási, A.-L. Competition and multiscaling in evolving networks. Europhys. Lett. 54 , 436 (2001).
Yin, Y. & Wang, D. The time dimension of science: connecting the past to the future. J. Informetr. 11 , 608–621 (2017).
Pan, R. K., Petersen, A. M., Pammolli, F. & Fortunato, S. The memory of science: Inflation, myopia, and the knowledge network. J. Informetr. 12 , 656–678 (2018).
Yin, Y., Wang, Y., Evans, J. A. & Wang, D. Quantifying the dynamics of failure across science, startups and security. Nature 575 , 190–194 (2019).
Candia, C. & Uzzi, B. Quantifying the selective forgetting and integration of ideas in science and technology. Am. Psychol. 76 , 1067 (2021).
Milojević, S. Principles of scientific research team formation and evolution. Proc. Natl Acad. Sci. USA 111 , 3984–3989 (2014).
Guimera, R., Uzzi, B., Spiro, J. & Amaral, L. A. N. Team assembly mechanisms determine collaboration network structure and team performance. Science 308 , 697–702 (2005).
Newman, M. E. Coauthorship networks and patterns of scientific collaboration. Proc. Natl Acad. Sci. USA 101 , 5200–5205 (2004).
Newman, M. E. Clustering and preferential attachment in growing networks. Phys. Rev. E 64 , 025102 (2001).
Iacopini, I., Milojević, S. & Latora, V. Network dynamics of innovation processes. Phys. Rev. Lett. 120 , 048301 (2018).
Kuhn, T., Perc, M. & Helbing, D. Inheritance patterns in citation networks reveal scientific memes. Phys. Rev. 4 , 041036 (2014).
Jia, T., Wang, D. & Szymanski, B. K. Quantifying patterns of research-interest evolution. Nat. Hum. Behav. 1 , 0078 (2017).
Zeng, A. et al. Increasing trend of scientists to switch between topics. Nat. Commun. https://doi.org/10.1038/s41467-019-11401-8 (2019).
Siudem, G., Żogała-Siudem, B., Cena, A. & Gagolewski, M. Three dimensions of scientific impact. Proc. Natl Acad. Sci. USA 117 , 13896–13900 (2020).
Petersen, A. M. et al. Reputation and impact in academic careers. Proc. Natl Acad. Sci. USA 111 , 15316–15321 (2014).
Jin, C., Song, C., Bjelland, J., Canright, G. & Wang, D. Emergence of scaling in complex substitutive systems. Nat. Hum. Behav. 3 , 837–846 (2019).
Hofman, J. M. et al. Integrating explanation and prediction in computational social science. Nature 595 , 181–188 (2021).
Lazer, D. et al. Computational social science. Science 323 , 721–723 (2009).
Lazer, D. M. et al. Computational social science: obstacles and opportunities. Science 369 , 1060–1062 (2020).
Albert, R. & Barabási, A.-L. Statistical mechanics of complex networks. Rev. Mod. Phys. 74 , 47 (2002).
Newman, M. E. The structure and function of complex networks. SIAM Rev. 45 , 167–256 (2003).
Song, C., Qu, Z., Blumm, N. & Barabási, A.-L. Limits of predictability in human mobility. Science 327 , 1018–1021 (2010).
Alessandretti, L., Aslak, U. & Lehmann, S. The scales of human mobility. Nature 587 , 402–407 (2020).
Pastor-Satorras, R. & Vespignani, A. Epidemic spreading in scale-free networks. Phys. Rev. Lett. 86 , 3200 (2001).
Pastor-Satorras, R., Castellano, C., Van Mieghem, P. & Vespignani, A. Epidemic processes in complex networks. Rev. Mod. Phys. 87 , 925 (2015).
Goodfellow, I., Bengio, Y. & Courville, A. Deep Learning (MIT Press, 2016).
Bishop, C. M. Pattern Recognition and Machine Learning (Springer, 2006).
Dong, Y., Johnson, R. A. & Chawla, N. V. Will this paper increase your h-index? Scientific impact prediction. In Proc. 8th ACM International Conference on Web Search and Data Mining, 149–158 (ACM 2015)
Xiao, S. et al. On modeling and predicting individual paper citation count over time. In IJCAI, 2676–2682 (IJCAI, 2016)
Fortunato, S. Community detection in graphs. Phys. Rep. 486 , 75–174 (2010).
Chen, C. Science mapping: a systematic review of the literature. J. Data Inf. Sci. 2 , 1–40 (2017).
CAS Google Scholar
Van Eck, N. J. & Waltman, L. Citation-based clustering of publications using CitNetExplorer and VOSviewer. Scientometrics 111 , 1053–1070 (2017).
LeCun, Y., Bengio, Y. & Hinton, G. Deep learning. Nature 521 , 436–444 (2015).
Senior, A. W. et al. Improved protein structure prediction using potentials from deep learning. Nature 577 , 706–710 (2020).
Krenn, M. & Zeilinger, A. Predicting research trends with semantic and neural networks with an application in quantum physics. Proc. Natl Acad. Sci. USA 117 , 1910–1916 (2020).
Iten, R., Metger, T., Wilming, H., Del Rio, L. & Renner, R. Discovering physical concepts with neural networks. Phys. Rev. Lett. 124 , 010508 (2020).
Guimerà, R. et al. A Bayesian machine scientist to aid in the solution of challenging scientific problems. Sci. Adv. 6 , eaav6971 (2020).
Segler, M. H., Preuss, M. & Waller, M. P. Planning chemical syntheses with deep neural networks and symbolic AI. Nature 555 , 604–610 (2018).
Ryu, J. Y., Kim, H. U. & Lee, S. Y. Deep learning improves prediction of drug–drug and drug–food interactions. Proc. Natl Acad. Sci. USA 115 , E4304–E4311 (2018).
Kermany, D. S. et al. Identifying medical diagnoses and treatable diseases by image-based deep learning. Cell 172 , 1122–1131.e9 (2018).
Peng, H., Ke, Q., Budak, C., Romero, D. M. & Ahn, Y.-Y. Neural embeddings of scholarly periodicals reveal complex disciplinary organizations. Sci. Adv. 7 , eabb9004 (2021).
Youyou, W., Yang, Y. & Uzzi, B. A discipline-wide investigation of the replicability of psychology papers over the past two decades. Proc. Natl Acad. Sci. USA 120 , e2208863120 (2023).
Mehrabi, N., Morstatter, F., Saxena, N., Lerman, K. & Galstyan, A. A survey on bias and fairness in machine learning. ACM Computing Surveys (CSUR) 54 , 1–35 (2021).
Way, S. F., Morgan, A. C., Larremore, D. B. & Clauset, A. Productivity, prominence, and the effects of academic environment. Proc. Natl Acad. Sci. USA 116 , 10729–10733 (2019).
Li, W., Aste, T., Caccioli, F. & Livan, G. Early coauthorship with top scientists predicts success in academic careers. Nat. Commun. 10 , 5170 (2019).
Hendry, D. F., Pagan, A. R. & Sargan, J. D. Dynamic specification. Handb. Econ. 2 , 1023–1100 (1984).
Jin, C., Ma, Y. & Uzzi, B. Scientific prizes and the extraordinary growth of scientific topics. Nat. Commun. 12 , 5619 (2021).
Azoulay, P., Ganguli, I. & Zivin, J. G. The mobility of elite life scientists: professional and personal determinants. Res. Policy 46 , 573–590 (2017).
Slavova, K., Fosfuri, A. & De Castro, J. O. Learning by hiring: the effects of scientists’ inbound mobility on research performance in academia. Organ. Sci. 27 , 72–89 (2016).
Sarsons, H. Recognition for group work: gender differences in academia. Am. Econ. Rev. 107 , 141–145 (2017).
Campbell, L. G., Mehtani, S., Dozier, M. E. & Rinehart, J. Gender-heterogeneous working groups produce higher quality science. PLoS ONE 8 , e79147 (2013).
Azoulay, P., Graff Zivin, J. S. & Wang, J. Superstar extinction. Q. J. Econ. 125 , 549–589 (2010).
Furman, J. L. & Stern, S. Climbing atop the shoulders of giants: the impact of institutions on cumulative research. Am. Econ. Rev. 101 , 1933–1963 (2011).
Williams, H. L. Intellectual property rights and innovation: evidence from the human genome. J. Polit. Econ. 121 , 1–27 (2013).
Rubin, A. & Rubin, E. Systematic Bias in the Progress of Research. J. Polit. Econ. 129 , 2666–2719 (2021).
Lu, S. F., Jin, G. Z., Uzzi, B. & Jones, B. The retraction penalty: evidence from the Web of Science. Sci. Rep. 3 , 3146 (2013).
Jin, G. Z., Jones, B., Lu, S. F. & Uzzi, B. The reverse Matthew effect: consequences of retraction in scientific teams. Rev. Econ. Stat. 101 , 492–506 (2019).
Azoulay, P., Bonatti, A. & Krieger, J. L. The career effects of scandal: evidence from scientific retractions. Res. Policy 46 , 1552–1569 (2017).
Goodman-Bacon, A. Difference-in-differences with variation in treatment timing. J. Econ. 225 , 254–277 (2021).
Callaway, B. & Sant’Anna, P. H. Difference-in-differences with multiple time periods. J. Econ. 225 , 200–230 (2021).
Hill, R. Searching for Superstars: Research Risk and Talent Discovery in Astronomy Working Paper (Massachusetts Institute of Technology, 2019).
Bagues, M., Sylos-Labini, M. & Zinovyeva, N. Does the gender composition of scientific committees matter? Am. Econ. Rev. 107 , 1207–1238 (2017).
Sampat, B. & Williams, H. L. How do patents affect follow-on innovation? Evidence from the human genome. Am. Econ. Rev. 109 , 203–236 (2019).
Moretti, E. & Wilson, D. J. The effect of state taxes on the geographical location of top earners: evidence from star scientists. Am. Econ. Rev. 107 , 1858–1903 (2017).
Jacob, B. A. & Lefgren, L. The impact of research grant funding on scientific productivity. J. Public Econ. 95 , 1168–1177 (2011).
Li, D. Expertise versus bias in evaluation: evidence from the NIH. Am. Econ. J. Appl. Econ. 9 , 60–92 (2017).
Pearl, J. Causal diagrams for empirical research. Biometrika 82 , 669–688 (1995).
Pearl, J. & Mackenzie, D. The Book of Why: The New Science of Cause and Effect (Basic Books, 2018).
Traag, V. A. Inferring the causal effect of journals on citations. Quant. Sci. Stud. 2 , 496–504 (2021).
Traag, V. & Waltman, L. Causal foundations of bias, disparity and fairness. Preprint at https://doi.org/10.48550/arXiv.2207.13665 (2022).
Imbens, G. W. Potential outcome and directed acyclic graph approaches to causality: relevance for empirical practice in economics. J. Econ. Lit. 58 , 1129–1179 (2020).
Heckman, J. J. & Pinto, R. Causality and Econometrics (National Bureau of Economic Research, 2022).
Aggarwal, I., Woolley, A. W., Chabris, C. F. & Malone, T. W. The impact of cognitive style diversity on implicit learning in teams. Front. Psychol. 10 , 112 (2019).
Balietti, S., Goldstone, R. L. & Helbing, D. Peer review and competition in the Art Exhibition Game. Proc. Natl Acad. Sci. USA 113 , 8414–8419 (2016).
Paulus, F. M., Rademacher, L., Schäfer, T. A. J., Müller-Pinzler, L. & Krach, S. Journal impact factor shapes scientists’ reward signal in the prospect of publication. PLoS ONE 10 , e0142537 (2015).
Williams, W. M. & Ceci, S. J. National hiring experiments reveal 2:1 faculty preference for women on STEM tenure track. Proc. Natl Acad. Sci. USA 112 , 5360–5365 (2015).
Collaboration, O. S. Estimating the reproducibility of psychological science. Science 349 , aac4716 (2015).
Camerer, C. F. et al. Evaluating replicability of laboratory experiments in economics. Science 351 , 1433–1436 (2016).
Camerer, C. F. et al. Evaluating the replicability of social science experiments in Nature and Science between 2010 and 2015. Nat. Hum. Behav. 2 , 637–644 (2018).
Duflo, E. & Banerjee, A. Handbook of Field Experiments (Elsevier, 2017).
Tomkins, A., Zhang, M. & Heavlin, W. D. Reviewer bias in single versus double-blind peer review. Proc. Natl Acad. Sci. USA 114 , 12708–12713 (2017).
Blank, R. M. The effects of double-blind versus single-blind reviewing: experimental evidence from the American Economic Review. Am. Econ. Rev. 81 , 1041–1067 (1991).
Boudreau, K. J., Guinan, E. C., Lakhani, K. R. & Riedl, C. Looking across and looking beyond the knowledge frontier: intellectual distance, novelty, and resource allocation in science. Manage. Sci. 62 , 2765–2783 (2016).
Lane, J. et al. When Do Experts Listen to Other Experts? The Role of Negative Information in Expert Evaluations for Novel Projects Working Paper #21-007 (Harvard Business School, 2020).
Teplitskiy, M. et al. Do Experts Listen to Other Experts? Field Experimental Evidence from Scientific Peer Review (Harvard Business School, 2019).
Moss-Racusin, C. A., Dovidio, J. F., Brescoll, V. L., Graham, M. J. & Handelsman, J. Science faculty’s subtle gender biases favor male students. Proc. Natl Acad. Sci. USA 109 , 16474–16479 (2012).
Forscher, P. S., Cox, W. T., Brauer, M. & Devine, P. G. Little race or gender bias in an experiment of initial review of NIH R01 grant proposals. Nat. Hum. Behav. 3 , 257–264 (2019).
Dennehy, T. C. & Dasgupta, N. Female peer mentors early in college increase women’s positive academic experiences and retention in engineering. Proc. Natl Acad. Sci. USA 114 , 5964–5969 (2017).
Azoulay, P. Turn the scientific method on ourselves. Nature 484 , 31–32 (2012).
Download references
Acknowledgements
The authors thank all members of the Center for Science of Science and Innovation (CSSI) for invaluable comments. This work was supported by the Air Force Office of Scientific Research under award number FA9550-19-1-0354, National Science Foundation grant SBE 1829344, and the Alfred P. Sloan Foundation G-2019-12485.
Author information
Authors and affiliations.
Center for Science of Science and Innovation, Northwestern University, Evanston, IL, USA
Lu Liu, Benjamin F. Jones, Brian Uzzi & Dashun Wang
Northwestern Institute on Complex Systems, Northwestern University, Evanston, IL, USA
Kellogg School of Management, Northwestern University, Evanston, IL, USA
College of Information Sciences and Technology, Pennsylvania State University, University Park, PA, USA
National Bureau of Economic Research, Cambridge, MA, USA
Benjamin F. Jones
Brookings Institution, Washington, DC, USA
McCormick School of Engineering, Northwestern University, Evanston, IL, USA
- Dashun Wang
You can also search for this author in PubMed Google Scholar
Corresponding author
Correspondence to Dashun Wang .
Ethics declarations
Competing interests.
The authors declare no competing interests.
Peer review
Peer review information.
Nature Human Behaviour thanks Ludo Waltman, Erin Leahey and Sarah Bratt for their contribution to the peer review of this work.
Additional information
Publisher’s note Springer Nature remains neutral with regard to jurisdictional claims in published maps and institutional affiliations.
Rights and permissions
Springer Nature or its licensor (e.g. a society or other partner) holds exclusive rights to this article under a publishing agreement with the author(s) or other rightsholder(s); author self-archiving of the accepted manuscript version of this article is solely governed by the terms of such publishing agreement and applicable law.
Reprints and permissions
About this article
Cite this article.
Liu, L., Jones, B.F., Uzzi, B. et al. Data, measurement and empirical methods in the science of science. Nat Hum Behav 7 , 1046–1058 (2023). https://doi.org/10.1038/s41562-023-01562-4
Download citation
Received : 30 June 2022
Accepted : 17 February 2023
Published : 01 June 2023
Issue Date : July 2023
DOI : https://doi.org/10.1038/s41562-023-01562-4
Share this article
Anyone you share the following link with will be able to read this content:
Sorry, a shareable link is not currently available for this article.
Provided by the Springer Nature SharedIt content-sharing initiative
This article is cited by
Publication, funding, and experimental data in support of human reference atlas construction and usage.
- Yongxin Kong
- Katy Börner
Scientific Data (2024)
Rescaling the disruption index reveals the universality of disruption distributions in science
- Alex J. Yang
- Hongcun Gong
- Sanhong Deng
Scientometrics (2024)
Signs of Dysconscious Racism and Xenophobiaism in Knowledge Production and the Formation of Academic Researchers: A National Study
- Dina Zoe Belluigi
Journal of Academic Ethics (2024)
Scientific Data (2023)
Quick links
- Explore articles by subject
- Guide to authors
- Editorial policies
Sign up for the Nature Briefing newsletter — what matters in science, free to your inbox daily.


- University of Memphis Libraries
- Research Guides
Empirical Research: Defining, Identifying, & Finding
Defining empirical research, what is empirical research, quantitative or qualitative.
- Introduction
- Database Tools
- Search Terms
- Image Descriptions
Calfee & Chambliss (2005) (UofM login required) describe empirical research as a "systematic approach for answering certain types of questions." Those questions are answered "[t]hrough the collection of evidence under carefully defined and replicable conditions" (p. 43).
The evidence collected during empirical research is often referred to as "data."
Characteristics of Empirical Research
Emerald Publishing's guide to conducting empirical research identifies a number of common elements to empirical research:
- A research question , which will determine research objectives.
- A particular and planned design for the research, which will depend on the question and which will find ways of answering it with appropriate use of resources.
- The gathering of primary data , which is then analysed.
- A particular methodology for collecting and analysing the data, such as an experiment or survey.
- The limitation of the data to a particular group, area or time scale, known as a sample [emphasis added]: for example, a specific number of employees of a particular company type, or all users of a library over a given time scale. The sample should be somehow representative of a wider population.
- The ability to recreate the study and test the results. This is known as reliability .
- The ability to generalize from the findings to a larger sample and to other situations.
If you see these elements in a research article, you can feel confident that you have found empirical research. Emerald's guide goes into more detail on each element.
Empirical research methodologies can be described as quantitative, qualitative, or a mix of both (usually called mixed-methods).
Ruane (2016) (UofM login required) gets at the basic differences in approach between quantitative and qualitative research:
- Quantitative research -- an approach to documenting reality that relies heavily on numbers both for the measurement of variables and for data analysis (p. 33).
- Qualitative research -- an approach to documenting reality that relies on words and images as the primary data source (p. 33).
Both quantitative and qualitative methods are empirical . If you can recognize that a research study is quantitative or qualitative study, then you have also recognized that it is empirical study.
Below are information on the characteristics of quantitative and qualitative research. This video from Scribbr also offers a good overall introduction to the two approaches to research methodology:
Characteristics of Quantitative Research
Researchers test hypotheses, or theories, based in assumptions about causality, i.e. we expect variable X to cause variable Y. Variables have to be controlled as much as possible to ensure validity. The results explain the relationship between the variables. Measures are based in pre-defined instruments.
Examples: experimental or quasi-experimental design, pretest & post-test, survey or questionnaire with closed-ended questions. Studies that identify factors that influence an outcomes, the utility of an intervention, or understanding predictors of outcomes.
Characteristics of Qualitative Research
Researchers explore “meaning individuals or groups ascribe to social or human problems (Creswell & Creswell, 2018, p3).” Questions and procedures emerge rather than being prescribed. Complexity, nuance, and individual meaning are valued. Research is both inductive and deductive. Data sources are multiple and varied, i.e. interviews, observations, documents, photographs, etc. The researcher is a key instrument and must be reflective of their background, culture, and experiences as influential of the research.
Examples: open question interviews and surveys, focus groups, case studies, grounded theory, ethnography, discourse analysis, narrative, phenomenology, participatory action research.
Calfee, R. C. & Chambliss, M. (2005). The design of empirical research. In J. Flood, D. Lapp, J. R. Squire, & J. Jensen (Eds.), Methods of research on teaching the English language arts: The methodology chapters from the handbook of research on teaching the English language arts (pp. 43-78). Routledge. http://ezproxy.memphis.edu/login?url=http://search.ebscohost.com/login.aspx?direct=true&db=nlebk&AN=125955&site=eds-live&scope=site .
Creswell, J. W., & Creswell, J. D. (2018). Research design: Qualitative, quantitative, and mixed methods approaches (5th ed.). Thousand Oaks: Sage.
How to... conduct empirical research . (n.d.). Emerald Publishing. https://www.emeraldgrouppublishing.com/how-to/research-methods/conduct-empirical-research .
Scribbr. (2019). Quantitative vs. qualitative: The differences explained [video]. YouTube. https://www.youtube.com/watch?v=a-XtVF7Bofg .
Ruane, J. M. (2016). Introducing social research methods : Essentials for getting the edge . Wiley-Blackwell. http://ezproxy.memphis.edu/login?url=http://search.ebscohost.com/login.aspx?direct=true&db=nlebk&AN=1107215&site=eds-live&scope=site .
- << Previous: Home
- Next: Identifying Empirical Research >>
- Last Updated: Apr 2, 2024 11:25 AM
- URL: https://libguides.memphis.edu/empirical-research
PSYC 200 Lab in Experimental Methods (Atlanta)
- Find Research Articles
Empirical vs. Review Articles
How to recognize empirical journal articles, scholarly vs. non-scholarly sources.
- Cite Sources
- Find Tests & Measures
- Find Research Methods Materials
- Post-Library Lab Activity on Finding Tests and Measures
- Find Books, E-books, and Films
Psychology Librarian

Know the difference between empirical and review articles.
Empirical article An empirical (research) article reports methods and findings of an original research study conducted by the authors of the article.
Literature Review article A review article or "literature review" discusses past research studies on a given topic.
Definition of an empirical study: An empirical research article reports the results of a study that uses data derived from actual observation or experimentation. Empirical research articles are examples of primary research.
Parts of a standard empirical research article: (articles will not necessary use the exact terms listed below.)
- Abstract ... A paragraph length description of what the study includes.
- Introduction ...Includes a statement of the hypotheses for the research and a review of other research on the topic.
- Who are participants
- Design of the study
- What the participants did
- What measures were used
- Results ...Describes the outcomes of the measures of the study.
- Discussion ...Contains the interpretations and implications of the study.
- References ...Contains citation information on the material cited in the report. (also called bibliography or works cited)
Characteristics of an Empirical Article:
- Empirical articles will include charts, graphs, or statistical analysis.
- Empirical research articles are usually substantial, maybe from 8-30 pages long.
- There is always a bibliography found at the end of the article.
Type of publications that publish empirical studies:
- Empirical research articles are published in scholarly or academic journals
- These journals are also called “peer-reviewed,” or “refereed” publications.
Examples of such publications include:
- Computers in Human Behavior
- Journal of Educational Psychology
Examples of databases that contain empirical research: (selected list only)
- Web of Science
This page is adapted from the Sociology Research Guide: Identify Empirical Articles page at Cal State Fullerton Pollak Library.
Know the difference between scholarly and non-scholarly articles.
"Scholarly" journal = "Peer-Reviewed" journal = "Refereed" journal
When researching your topic, you may come across many different types of sources and articles. When evaluating these sources, it is important to think about:
- Who is the author?
- Who is the audience or why was this written?
- Where was this published?
- Is this relevant to your research?
- When was this written? Has it been updated?
- Are there any citations? Who do they cite?
Written by , like academics, scientists, scholars, etc. | Written by , such as journalists, bloggers, etc. |
Written in the field. | Written . |
Written with language. | , or will define any specialized terms. |
Will include a of the sources that are cited. | , or may not have any citations. |
Published in | Published in a . |
Are often . | Are . |
Helpful Links and Guides
Here are helpful links and guides to check out for more information on scholarly sources:
- This database contains data on different types of serials and can be used to determine whether a periodical is peer-reviewed or not: Ulrich's Periodicals Directory
- The UC Berkeley Library published this useful guide on evaluating resources, including the differences between scholarly and popular sources, as well as how to find primary sources: UC Berkeley's Evaluating Resources LibGuide
- << Previous: Quick Poll
- Next: Cite Sources >>
- Last Updated: Feb 14, 2024 3:32 PM
- URL: https://guides.libraries.emory.edu/main/psyc200
- Skip to main content
- Skip to primary sidebar
- Skip to footer
- QuestionPro

- Solutions Industries Gaming Automotive Sports and events Education Government Travel & Hospitality Financial Services Healthcare Cannabis Technology Use Case AskWhy Communities Audience Contactless surveys Mobile LivePolls Member Experience GDPR Positive People Science 360 Feedback Surveys
- Resources Blog eBooks Survey Templates Case Studies Training Help center

Home Market Research
Empirical Research: Definition, Methods, Types and Examples
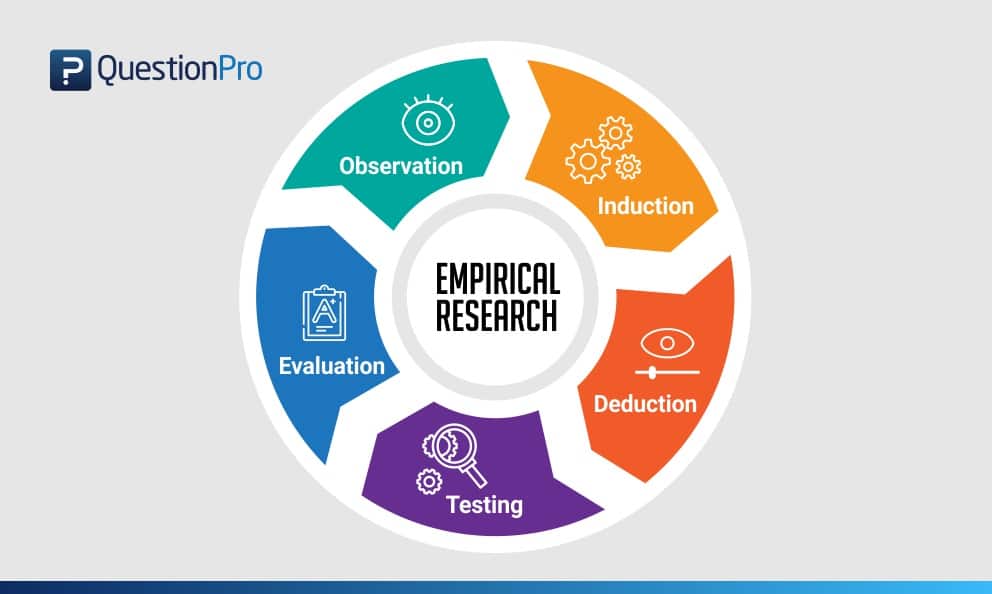
Content Index
Empirical research: Definition
Empirical research: origin, quantitative research methods, qualitative research methods, steps for conducting empirical research, empirical research methodology cycle, advantages of empirical research, disadvantages of empirical research, why is there a need for empirical research.
Empirical research is defined as any research where conclusions of the study is strictly drawn from concretely empirical evidence, and therefore “verifiable” evidence.
This empirical evidence can be gathered using quantitative market research and qualitative market research methods.
For example: A research is being conducted to find out if listening to happy music in the workplace while working may promote creativity? An experiment is conducted by using a music website survey on a set of audience who are exposed to happy music and another set who are not listening to music at all, and the subjects are then observed. The results derived from such a research will give empirical evidence if it does promote creativity or not.
LEARN ABOUT: Behavioral Research
You must have heard the quote” I will not believe it unless I see it”. This came from the ancient empiricists, a fundamental understanding that powered the emergence of medieval science during the renaissance period and laid the foundation of modern science, as we know it today. The word itself has its roots in greek. It is derived from the greek word empeirikos which means “experienced”.
In today’s world, the word empirical refers to collection of data using evidence that is collected through observation or experience or by using calibrated scientific instruments. All of the above origins have one thing in common which is dependence of observation and experiments to collect data and test them to come up with conclusions.
LEARN ABOUT: Causal Research
Types and methodologies of empirical research
Empirical research can be conducted and analysed using qualitative or quantitative methods.
- Quantitative research : Quantitative research methods are used to gather information through numerical data. It is used to quantify opinions, behaviors or other defined variables . These are predetermined and are in a more structured format. Some of the commonly used methods are survey, longitudinal studies, polls, etc
- Qualitative research: Qualitative research methods are used to gather non numerical data. It is used to find meanings, opinions, or the underlying reasons from its subjects. These methods are unstructured or semi structured. The sample size for such a research is usually small and it is a conversational type of method to provide more insight or in-depth information about the problem Some of the most popular forms of methods are focus groups, experiments, interviews, etc.
Data collected from these will need to be analysed. Empirical evidence can also be analysed either quantitatively and qualitatively. Using this, the researcher can answer empirical questions which have to be clearly defined and answerable with the findings he has got. The type of research design used will vary depending on the field in which it is going to be used. Many of them might choose to do a collective research involving quantitative and qualitative method to better answer questions which cannot be studied in a laboratory setting.
LEARN ABOUT: Qualitative Research Questions and Questionnaires
Quantitative research methods aid in analyzing the empirical evidence gathered. By using these a researcher can find out if his hypothesis is supported or not.
- Survey research: Survey research generally involves a large audience to collect a large amount of data. This is a quantitative method having a predetermined set of closed questions which are pretty easy to answer. Because of the simplicity of such a method, high responses are achieved. It is one of the most commonly used methods for all kinds of research in today’s world.
Previously, surveys were taken face to face only with maybe a recorder. However, with advancement in technology and for ease, new mediums such as emails , or social media have emerged.
For example: Depletion of energy resources is a growing concern and hence there is a need for awareness about renewable energy. According to recent studies, fossil fuels still account for around 80% of energy consumption in the United States. Even though there is a rise in the use of green energy every year, there are certain parameters because of which the general population is still not opting for green energy. In order to understand why, a survey can be conducted to gather opinions of the general population about green energy and the factors that influence their choice of switching to renewable energy. Such a survey can help institutions or governing bodies to promote appropriate awareness and incentive schemes to push the use of greener energy.
Learn more: Renewable Energy Survey Template Descriptive Research vs Correlational Research
- Experimental research: In experimental research , an experiment is set up and a hypothesis is tested by creating a situation in which one of the variable is manipulated. This is also used to check cause and effect. It is tested to see what happens to the independent variable if the other one is removed or altered. The process for such a method is usually proposing a hypothesis, experimenting on it, analyzing the findings and reporting the findings to understand if it supports the theory or not.
For example: A particular product company is trying to find what is the reason for them to not be able to capture the market. So the organisation makes changes in each one of the processes like manufacturing, marketing, sales and operations. Through the experiment they understand that sales training directly impacts the market coverage for their product. If the person is trained well, then the product will have better coverage.
- Correlational research: Correlational research is used to find relation between two set of variables . Regression analysis is generally used to predict outcomes of such a method. It can be positive, negative or neutral correlation.
LEARN ABOUT: Level of Analysis
For example: Higher educated individuals will get higher paying jobs. This means higher education enables the individual to high paying job and less education will lead to lower paying jobs.
- Longitudinal study: Longitudinal study is used to understand the traits or behavior of a subject under observation after repeatedly testing the subject over a period of time. Data collected from such a method can be qualitative or quantitative in nature.
For example: A research to find out benefits of exercise. The target is asked to exercise everyday for a particular period of time and the results show higher endurance, stamina, and muscle growth. This supports the fact that exercise benefits an individual body.
- Cross sectional: Cross sectional study is an observational type of method, in which a set of audience is observed at a given point in time. In this type, the set of people are chosen in a fashion which depicts similarity in all the variables except the one which is being researched. This type does not enable the researcher to establish a cause and effect relationship as it is not observed for a continuous time period. It is majorly used by healthcare sector or the retail industry.
For example: A medical study to find the prevalence of under-nutrition disorders in kids of a given population. This will involve looking at a wide range of parameters like age, ethnicity, location, incomes and social backgrounds. If a significant number of kids coming from poor families show under-nutrition disorders, the researcher can further investigate into it. Usually a cross sectional study is followed by a longitudinal study to find out the exact reason.
- Causal-Comparative research : This method is based on comparison. It is mainly used to find out cause-effect relationship between two variables or even multiple variables.
For example: A researcher measured the productivity of employees in a company which gave breaks to the employees during work and compared that to the employees of the company which did not give breaks at all.
LEARN ABOUT: Action Research
Some research questions need to be analysed qualitatively, as quantitative methods are not applicable there. In many cases, in-depth information is needed or a researcher may need to observe a target audience behavior, hence the results needed are in a descriptive analysis form. Qualitative research results will be descriptive rather than predictive. It enables the researcher to build or support theories for future potential quantitative research. In such a situation qualitative research methods are used to derive a conclusion to support the theory or hypothesis being studied.
LEARN ABOUT: Qualitative Interview
- Case study: Case study method is used to find more information through carefully analyzing existing cases. It is very often used for business research or to gather empirical evidence for investigation purpose. It is a method to investigate a problem within its real life context through existing cases. The researcher has to carefully analyse making sure the parameter and variables in the existing case are the same as to the case that is being investigated. Using the findings from the case study, conclusions can be drawn regarding the topic that is being studied.
For example: A report mentioning the solution provided by a company to its client. The challenges they faced during initiation and deployment, the findings of the case and solutions they offered for the problems. Such case studies are used by most companies as it forms an empirical evidence for the company to promote in order to get more business.
- Observational method: Observational method is a process to observe and gather data from its target. Since it is a qualitative method it is time consuming and very personal. It can be said that observational research method is a part of ethnographic research which is also used to gather empirical evidence. This is usually a qualitative form of research, however in some cases it can be quantitative as well depending on what is being studied.
For example: setting up a research to observe a particular animal in the rain-forests of amazon. Such a research usually take a lot of time as observation has to be done for a set amount of time to study patterns or behavior of the subject. Another example used widely nowadays is to observe people shopping in a mall to figure out buying behavior of consumers.
- One-on-one interview: Such a method is purely qualitative and one of the most widely used. The reason being it enables a researcher get precise meaningful data if the right questions are asked. It is a conversational method where in-depth data can be gathered depending on where the conversation leads.
For example: A one-on-one interview with the finance minister to gather data on financial policies of the country and its implications on the public.
- Focus groups: Focus groups are used when a researcher wants to find answers to why, what and how questions. A small group is generally chosen for such a method and it is not necessary to interact with the group in person. A moderator is generally needed in case the group is being addressed in person. This is widely used by product companies to collect data about their brands and the product.
For example: A mobile phone manufacturer wanting to have a feedback on the dimensions of one of their models which is yet to be launched. Such studies help the company meet the demand of the customer and position their model appropriately in the market.
- Text analysis: Text analysis method is a little new compared to the other types. Such a method is used to analyse social life by going through images or words used by the individual. In today’s world, with social media playing a major part of everyone’s life, such a method enables the research to follow the pattern that relates to his study.
For example: A lot of companies ask for feedback from the customer in detail mentioning how satisfied are they with their customer support team. Such data enables the researcher to take appropriate decisions to make their support team better.
Sometimes a combination of the methods is also needed for some questions that cannot be answered using only one type of method especially when a researcher needs to gain a complete understanding of complex subject matter.
We recently published a blog that talks about examples of qualitative data in education ; why don’t you check it out for more ideas?
Learn More: Data Collection Methods: Types & Examples
Since empirical research is based on observation and capturing experiences, it is important to plan the steps to conduct the experiment and how to analyse it. This will enable the researcher to resolve problems or obstacles which can occur during the experiment.
Step #1: Define the purpose of the research
This is the step where the researcher has to answer questions like what exactly do I want to find out? What is the problem statement? Are there any issues in terms of the availability of knowledge, data, time or resources. Will this research be more beneficial than what it will cost.
Before going ahead, a researcher has to clearly define his purpose for the research and set up a plan to carry out further tasks.
Step #2 : Supporting theories and relevant literature
The researcher needs to find out if there are theories which can be linked to his research problem . He has to figure out if any theory can help him support his findings. All kind of relevant literature will help the researcher to find if there are others who have researched this before, or what are the problems faced during this research. The researcher will also have to set up assumptions and also find out if there is any history regarding his research problem
Step #3: Creation of Hypothesis and measurement
Before beginning the actual research he needs to provide himself a working hypothesis or guess what will be the probable result. Researcher has to set up variables, decide the environment for the research and find out how can he relate between the variables.
Researcher will also need to define the units of measurements, tolerable degree for errors, and find out if the measurement chosen will be acceptable by others.
Step #4: Methodology, research design and data collection
In this step, the researcher has to define a strategy for conducting his research. He has to set up experiments to collect data which will enable him to propose the hypothesis. The researcher will decide whether he will need experimental or non experimental method for conducting the research. The type of research design will vary depending on the field in which the research is being conducted. Last but not the least, the researcher will have to find out parameters that will affect the validity of the research design. Data collection will need to be done by choosing appropriate samples depending on the research question. To carry out the research, he can use one of the many sampling techniques. Once data collection is complete, researcher will have empirical data which needs to be analysed.
LEARN ABOUT: Best Data Collection Tools
Step #5: Data Analysis and result
Data analysis can be done in two ways, qualitatively and quantitatively. Researcher will need to find out what qualitative method or quantitative method will be needed or will he need a combination of both. Depending on the unit of analysis of his data, he will know if his hypothesis is supported or rejected. Analyzing this data is the most important part to support his hypothesis.
Step #6: Conclusion
A report will need to be made with the findings of the research. The researcher can give the theories and literature that support his research. He can make suggestions or recommendations for further research on his topic.
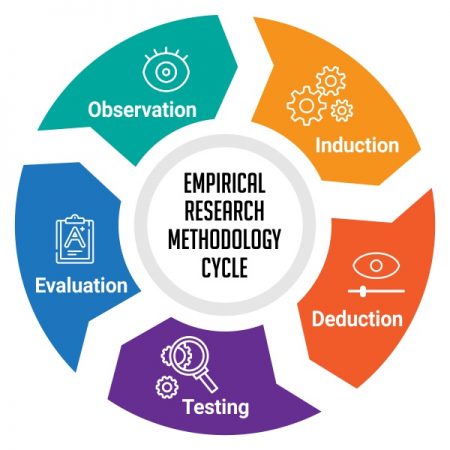
A.D. de Groot, a famous dutch psychologist and a chess expert conducted some of the most notable experiments using chess in the 1940’s. During his study, he came up with a cycle which is consistent and now widely used to conduct empirical research. It consists of 5 phases with each phase being as important as the next one. The empirical cycle captures the process of coming up with hypothesis about how certain subjects work or behave and then testing these hypothesis against empirical data in a systematic and rigorous approach. It can be said that it characterizes the deductive approach to science. Following is the empirical cycle.
- Observation: At this phase an idea is sparked for proposing a hypothesis. During this phase empirical data is gathered using observation. For example: a particular species of flower bloom in a different color only during a specific season.
- Induction: Inductive reasoning is then carried out to form a general conclusion from the data gathered through observation. For example: As stated above it is observed that the species of flower blooms in a different color during a specific season. A researcher may ask a question “does the temperature in the season cause the color change in the flower?” He can assume that is the case, however it is a mere conjecture and hence an experiment needs to be set up to support this hypothesis. So he tags a few set of flowers kept at a different temperature and observes if they still change the color?
- Deduction: This phase helps the researcher to deduce a conclusion out of his experiment. This has to be based on logic and rationality to come up with specific unbiased results.For example: In the experiment, if the tagged flowers in a different temperature environment do not change the color then it can be concluded that temperature plays a role in changing the color of the bloom.
- Testing: This phase involves the researcher to return to empirical methods to put his hypothesis to the test. The researcher now needs to make sense of his data and hence needs to use statistical analysis plans to determine the temperature and bloom color relationship. If the researcher finds out that most flowers bloom a different color when exposed to the certain temperature and the others do not when the temperature is different, he has found support to his hypothesis. Please note this not proof but just a support to his hypothesis.
- Evaluation: This phase is generally forgotten by most but is an important one to keep gaining knowledge. During this phase the researcher puts forth the data he has collected, the support argument and his conclusion. The researcher also states the limitations for the experiment and his hypothesis and suggests tips for others to pick it up and continue a more in-depth research for others in the future. LEARN MORE: Population vs Sample
LEARN MORE: Population vs Sample
There is a reason why empirical research is one of the most widely used method. There are a few advantages associated with it. Following are a few of them.
- It is used to authenticate traditional research through various experiments and observations.
- This research methodology makes the research being conducted more competent and authentic.
- It enables a researcher understand the dynamic changes that can happen and change his strategy accordingly.
- The level of control in such a research is high so the researcher can control multiple variables.
- It plays a vital role in increasing internal validity .
Even though empirical research makes the research more competent and authentic, it does have a few disadvantages. Following are a few of them.
- Such a research needs patience as it can be very time consuming. The researcher has to collect data from multiple sources and the parameters involved are quite a few, which will lead to a time consuming research.
- Most of the time, a researcher will need to conduct research at different locations or in different environments, this can lead to an expensive affair.
- There are a few rules in which experiments can be performed and hence permissions are needed. Many a times, it is very difficult to get certain permissions to carry out different methods of this research.
- Collection of data can be a problem sometimes, as it has to be collected from a variety of sources through different methods.
LEARN ABOUT: Social Communication Questionnaire
Empirical research is important in today’s world because most people believe in something only that they can see, hear or experience. It is used to validate multiple hypothesis and increase human knowledge and continue doing it to keep advancing in various fields.
For example: Pharmaceutical companies use empirical research to try out a specific drug on controlled groups or random groups to study the effect and cause. This way, they prove certain theories they had proposed for the specific drug. Such research is very important as sometimes it can lead to finding a cure for a disease that has existed for many years. It is useful in science and many other fields like history, social sciences, business, etc.
LEARN ABOUT: 12 Best Tools for Researchers
With the advancement in today’s world, empirical research has become critical and a norm in many fields to support their hypothesis and gain more knowledge. The methods mentioned above are very useful for carrying out such research. However, a number of new methods will keep coming up as the nature of new investigative questions keeps getting unique or changing.
Create a single source of real data with a built-for-insights platform. Store past data, add nuggets of insights, and import research data from various sources into a CRM for insights. Build on ever-growing research with a real-time dashboard in a unified research management platform to turn insights into knowledge.
LEARN MORE FREE TRIAL
MORE LIKE THIS
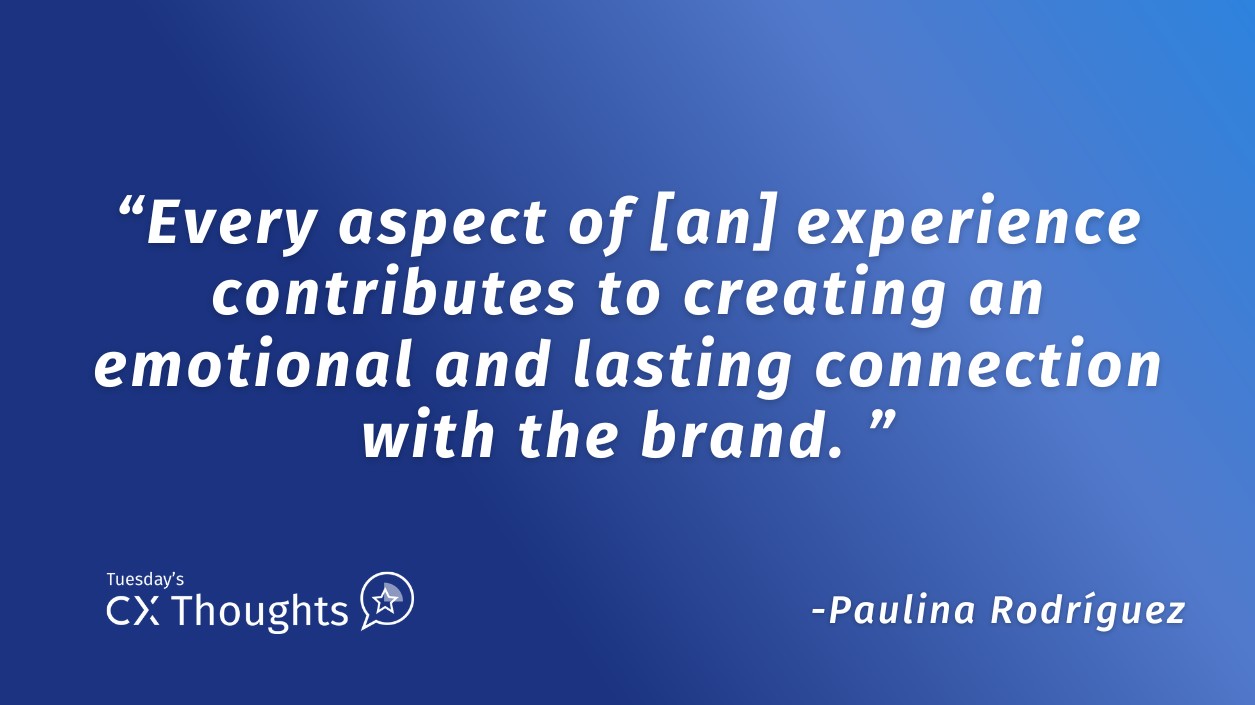
Customer Experience Lessons from 13,000 Feet — Tuesday CX Thoughts
Aug 20, 2024
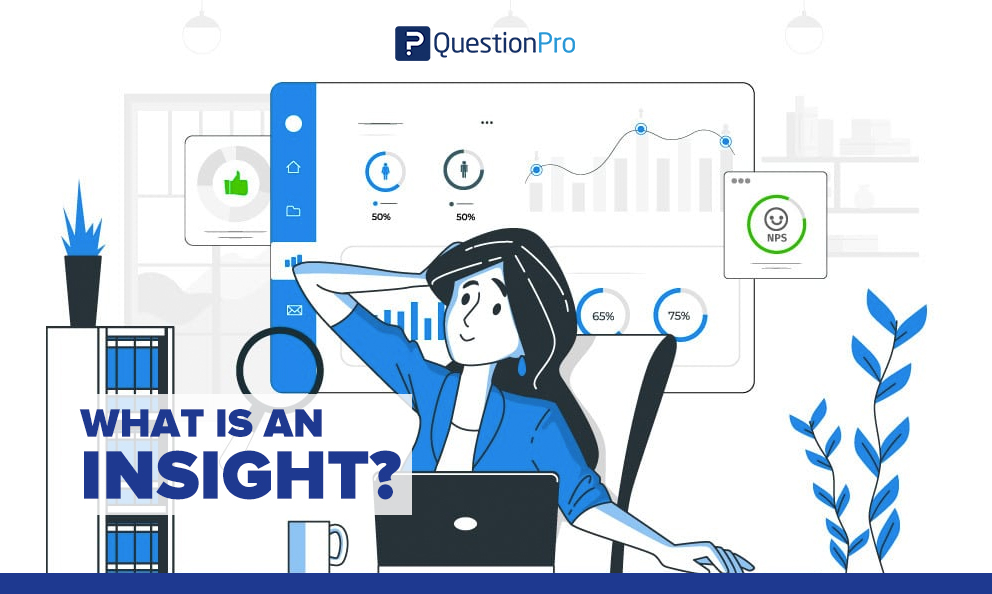
Insight: Definition & meaning, types and examples
Aug 19, 2024
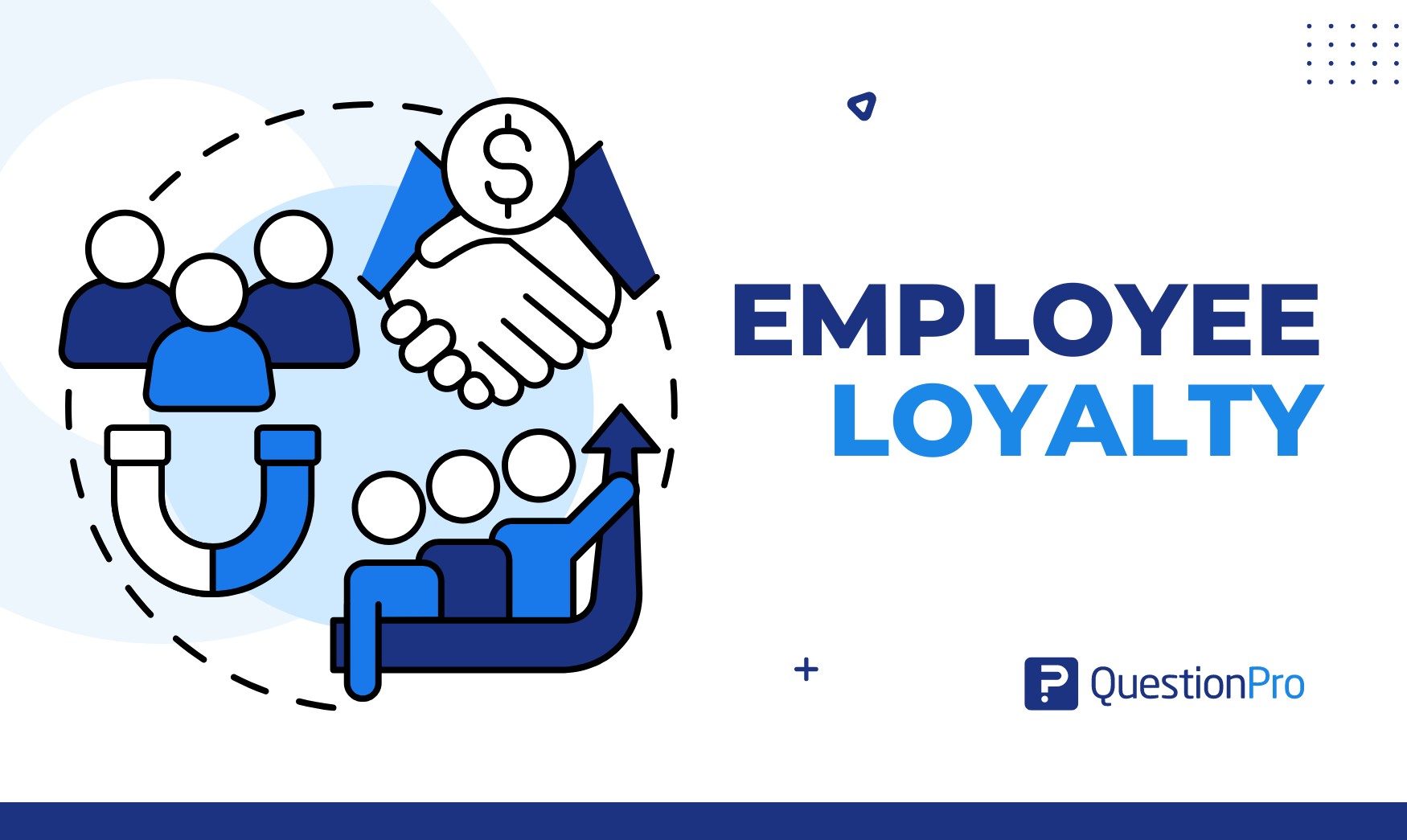
Employee Loyalty: Strategies for Long-Term Business Success
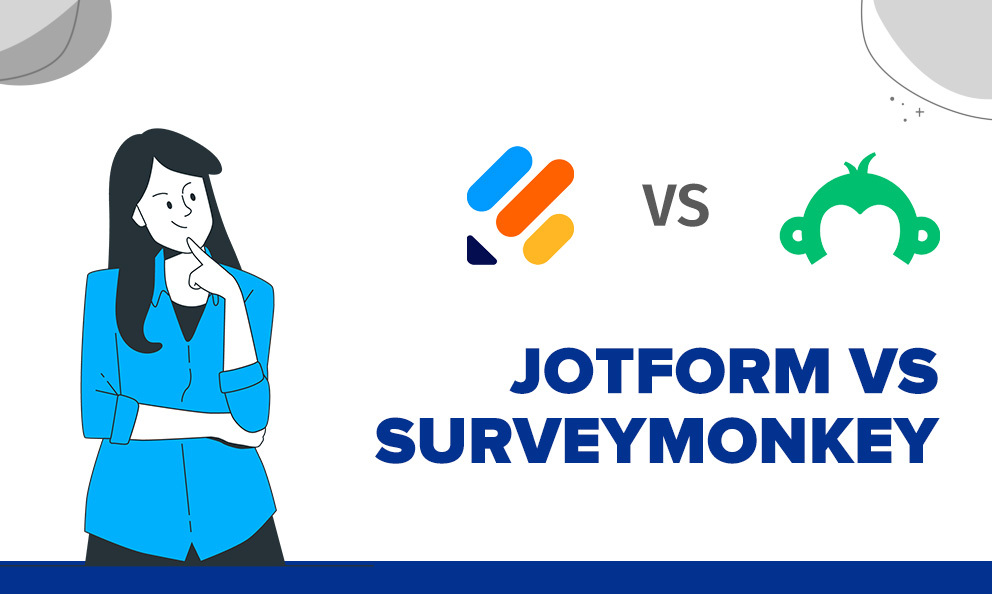
Jotform vs SurveyMonkey: Which Is Best in 2024
Aug 15, 2024
Other categories
- Academic Research
- Artificial Intelligence
- Assessments
- Brand Awareness
- Case Studies
- Communities
- Consumer Insights
- Customer effort score
- Customer Engagement
- Customer Experience
- Customer Loyalty
- Customer Research
- Customer Satisfaction
- Employee Benefits
- Employee Engagement
- Employee Retention
- Friday Five
- General Data Protection Regulation
- Insights Hub
- Life@QuestionPro
- Market Research
- Mobile diaries
- Mobile Surveys
- New Features
- Online Communities
- Question Types
- Questionnaire
- QuestionPro Products
- Release Notes
- Research Tools and Apps
- Revenue at Risk
- Survey Templates
- Training Tips
- Tuesday CX Thoughts (TCXT)
- Uncategorized
- What’s Coming Up
- Workforce Intelligence

- Brandeis Library
- Research Guides
- Find Empirical Studies
- Find Articles & Databases
- Reference Works
- Find Data & Statistics
What is an Empirical Study?
Where to look for empirical studies, find empirical studies: apa psycinfo & apa psycarticles, find empirical studies: eric.
- Search Strategies
An empirical study reports the findings from a study that uses data derived from an actual experiment or observation. Key components of an empirical study:
- Abstract - Provides a brief overview of the research.
- Introduction - The introduction contextualizes the research by providing a review of previous research on the topic. It also is the section where the hypothesis is stated.
- Method - The methods area describes how the research was conducted, including the design of the study, who the participants were and what they did, and any measurements that were taken during the study.
- Results - The results section describes the outcome of the study.
- Discussion - The discussion section addresses the researchers' interpretations of their study and any future implications from their findings.
- References - A list of works that were cited in the study.
Try searching in the following databases for empirical studies in education:
- APA PsycArticles This link opens in a new window Covers general psychology and specialized, basic, applied, clinical and theoretical research in psychology. Contains all journal articles, letters to the editor and errata from each of 49 journals by the APA and 9 from allied organizations. Coverage 1988 to the present.
- APA PsycInfo (EBSCO) This link opens in a new window Citations and summaries of journal articles, book chapters, books, dissertations and technical reports in psychology. Includes information about the psychological aspects of related disciplines such as medicine, psychiatry, nursing, sociology, education, pharmacology, physiology, linguistics, anthropology, business and law. Coverage 1887 to present, includes 1,700+ international sources in over 35 languages.
- Education Research Complete (EBSCO) This link opens in a new window Scholarly journal articles, dissertations, professional development resources, and other materials on topics related to the field of education. Coverage includes early childhood through adult education and all education specialties.
- ERIC (ProQuest) This link opens in a new window Database sponsored by the U.S. Department of Education that provides access to scholarly journals, curriculum and teaching guides, research reports, and other materials related to the field of education.
There are a few strategies you can use to limit your search results to empirical studies within education and related disciplines.
Two of our databases, APA PsycINFO and APA PsycARTICLES offer the option to limit search results to a specific methodology, including empirical studies. The methodology search facet is located on the advanced search page for APA PsycINFO and APA PsycARTICLES near the bottom of the page:

ERIC (ProQuest) doesn't have an easy way to search for empirical studies. You can try adding "empirical" into your search terms or you can try limiting your search results to research reports by completing the following steps:
- Use the "Advanced Search" page
- Type in your search terms
- Scroll down the page to "Document Type" and select "143: Reports- Research"
- Click "Search"

Another strategy for finding empirical studies is to add different combinations of the following search terms:
- methodology (or method)
- action research
- participant observation OR participants
- qualitative or quantitative
- << Previous: Find Data & Statistics
- Next: Search Strategies >>
- Last Updated: Jul 28, 2024 10:16 PM
- URL: https://guides.library.brandeis.edu/education
Experimental (Empirical) Research Articles
- Library vs. Google
- Background Reading
- Keyword Searching
- Evaluating Sources
- Please Note: Searching PsycInfo
- Citing Sources
- Need more help?
How Can I Find Experimental (Empirical) Articles?
Many of the recommended databases in this research guide contain scholarly experimental articles (also known as empirical articles or research studies or primary research). Search in databases like:
- APA PsycInfo
- ScienceDirect
Because those databases are rich in scholarly experimental articles, any well-structured search that you enter will retrieve experimental/empirical articles. These searches, for example, will retrieve many experimental/empirical articles:
- caffeine AND "reaction time"
- aging AND ("cognitive function" OR "cognitive ability")
- "child development" AND play
Experimental (Empirical) Articles: How Will I Know One When I See One?
Scholarly experimental articles to conduct and publish an experiment, an author or team of authors designs an experiment, gathers data, then analyzes the data and discusses the results of the experiment. a published experiment or research study will therefore look very different from other types of articles (newspaper stories, magazine articles, essays, etc.) found in our library databases..
In fact, newspapers, magazines, and websites written by journalists report on psychology research all the time, summarizing published experiments in non-technical language for the general public. Although that kind of article can be interesting to read (and can even lead you to look up the original experiment published by the researchers themselves), to write a research paper about a psychology topic, you should, generally, use experimental articles written by researchers. The following guidelines will help you recognize an experimental article, written by the researchers themselves and published in a scholarly journal.
Structure of a Experimental Article Typically, an experimental article has the following sections:
- The author summarizes her article
- The author discusses the general background of her research topic; often, she will present a literature review, that is, summarize what other experts have written on this particular research topic
- The author describes the experiment she designed and conducted
- The author presents the data she gathered during her experiment
- The author offers ideas about the importance and implications of her research findings, and speculates on future directions that similar research might take
- The author gives a References list of sources she used in her paper
Look for articles structured in that way--they will be experimental/empirical articles.
Also, experimental/empirical articles are written in very formal, technical language (even the titles of the articles sound complicated!) and will usually contain numerical data presented in tables.
As noted above, when you search in a database like APA PsycInfo, it's really easy to find experimental/empirical articles, once you know what you're looking for. Just in case, though, here is a shortcut that might help:
First, do your keyword search, for example:

In the results screen, on the left-hand side, scroll down until you see "Methodology." You can use that menu to refine your search by limiting the articles to empirical studies only:

You can learn learn more about searching in APA PsycInfo, including advanced search limiters like methodology, age group, etc., from this APA guide .
- << Previous: Resources
- Next: Research Tips >>
- Last Updated: Aug 13, 2024 1:29 PM
- URL: https://libguides.umgc.edu/psychology
La Salle University
Connelly library, library main menu.
- Course Reserves
- Interlibrary Loan (ILL)
- Study Room Use & Reservations
- Technology & Printing
- Citation Guides
- Reserve Library Space
- Request Instruction
- Copyright Information
- Guides for Faculty
Special Collections
- University Archives
- Historical & Cultural Collections
- Rare Bibles & Prayer Books
- Historical Research Guides
- Information & Guidelines
- Staff Directory
- Meet with a Librarian
- Directions & Building Maps
Research Hub
- Research Tools
- Research Guides
Qualitative and Quantitative Research
What is "empirical research".
- empirical research
- Locating Articles in Cinahl and PsycInfo
- Locating Articles in PubMed
- Getting the Articles
Empirical research is based on observed and measured phenomena and derives knowledge from actual experience rather than from theory or belief.
How do you know if a study is empirical? Read the subheadings within the article, book, or report and look for a description of the research "methodology." Ask yourself: Could I recreate this study and test these results?
Key characteristics to look for:
- Specific research questions to be answered
- Definition of the population, behavior, or phenomena being studied
- Description of the process used to study this population or phenomena, including selection criteria, controls, and testing instruments (such as surveys)
Another hint: some scholarly journals use a specific layout, called the "IMRaD" format, to communicate empirical research findings. Such articles typically have 4 components:
- Introduction : sometimes called "literature review" -- what is currently known about the topic -- usually includes a theoretical framework and/or discussion of previous studies
- Methodology: sometimes called "research design" -- how to recreate the study -- usually describes the population, research process, and analytical tools
- Results : sometimes called "findings" -- what was learned through the study -- usually appears as statistical data or as substantial quotations from research participants
- Discussion : sometimes called "conclusion" or "implications" -- why the study is important -- usually describes how the research results influence professional practices or future studies
- << Previous: Home
- Next: Locating Articles in Cinahl and PsycInfo >>
Chat Assistance
Loading metrics
Open Access
Peer-reviewed
Preregistered Research Article
Preregistered Research Articles present research resulting from a Registered Report, where the study design and methods were peer reviewed before data collection.
See all article types »
An empirical appraisal of eLife’s assessment vocabulary
Roles Conceptualization, Data curation, Formal analysis, Funding acquisition, Investigation, Methodology, Project administration, Resources, Software, Supervision, Validation, Visualization, Writing – review & editing
* E-mail: [email protected]
Affiliation Melbourne School of Psychological Sciences, University of Melbourne, Melbourne, Australia

Roles Investigation, Writing – review & editing
Roles Conceptualization, Investigation, Supervision, Writing – review & editing
- Tom E. Hardwicke,
- Sarah R. Schiavone,
- Beth Clarke,
- Simine Vazire
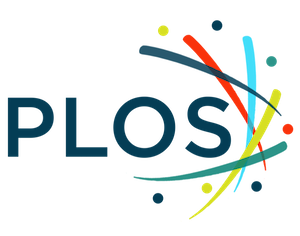
- Published: August 22, 2024
- https://doi.org/10.1371/journal.pbio.3002645
- Peer Review
- Reader Comments
Research articles published by the journal eLife are accompanied by short evaluation statements that use phrases from a prescribed vocabulary to evaluate research on 2 dimensions: importance and strength of support. Intuitively, the prescribed phrases appear to be highly synonymous (e.g., important/valuable, compelling/convincing) and the vocabulary’s ordinal structure may not be obvious to readers. We conducted an online repeated-measures experiment to gauge whether the phrases were interpreted as intended. We also tested an alternative vocabulary with (in our view) a less ambiguous structure. A total of 301 participants with a doctoral or graduate degree used a 0% to 100% scale to rate the importance and strength of support of hypothetical studies described using phrases from both vocabularies. For the eLife vocabulary, most participants’ implied ranking did not match the intended ranking on both the importance ( n = 59, 20% matched, 95% confidence interval [15% to 24%]) and strength of support dimensions ( n = 45, 15% matched [11% to 20%]). By contrast, for the alternative vocabulary, most participants’ implied ranking did match the intended ranking on both the importance ( n = 188, 62% matched [57% to 68%]) and strength of support dimensions ( n = 201, 67% matched [62% to 72%]). eLife’s vocabulary tended to produce less consistent between-person interpretations, though the alternative vocabulary still elicited some overlapping interpretations away from the middle of the scale. We speculate that explicit presentation of a vocabulary’s intended ordinal structure could improve interpretation. Overall, these findings suggest that more structured and less ambiguous language can improve communication of research evaluations.
Citation: Hardwicke TE, Schiavone SR, Clarke B, Vazire S (2024) An empirical appraisal of eLife’s assessment vocabulary. PLoS Biol 22(8): e3002645. https://doi.org/10.1371/journal.pbio.3002645
Academic Editor: Christopher D. Chambers, Cardiff University, UNITED KINGDOM OF GREAT BRITAIN AND NORTHERN IRELAND
Received: April 7, 2024; Accepted: July 9, 2024; Published: August 22, 2024 Note: As this is a Preregistered Research Article, the study design and methods were peer-reviewed before data collection. The time to acceptance includes the experimental time taken to perform the study. Learn more about Preregistered Research Articles .
Copyright: © 2024 Hardwicke et al. This is an open access article distributed under the terms of the Creative Commons Attribution License , which permits unrestricted use, distribution, and reproduction in any medium, provided the original author and source are credited.
Data Availability: All data, materials, and analysis scripts are publicly available on the Open Science Framework ( https://osf.io/mw2q4/files/osfstorage/ ). A reproducible version of the manuscript and associated computational environment is available in a Code Ocean container ( https://doi.org/10.24433/CO.4128032.v1 ).
Funding: This study was supported by funding awarded to to SV and TEH from the Melbourne School of Psychological Sciences, University of Melbourne. The funders did not play any role in the study design, data collection and analysis, decision to publish, or preparation of the manuscript.
Competing interests: SV is a member of the board of directors of The Public Library of Science (PLOS). This role has in no way influenced the outcome or development of this work or the peer-review process, nor does it alter our adherence to PLOS Biology policies on sharing data and materials. All other authors declare they have no conflicts of interest.
Introduction
Peer review is usually a black box—readers only know that a research paper eventually surpassed some ill-defined threshold for publication and rarely see the more nuanced evaluations of the reviewers and editor [ 1 ]. A minority of journals challenge this convention by making peer review reports publicly available [ 2 ]. One such journal, eLife, also accompanies articles with short evaluation statements (“eLife assessments”) representing the consensus opinions of editors and peer reviewers [ 3 ]. In 2022, eLife stated that these assessments would use phrases drawn from a common vocabulary ( Table 1 ) to convey their judgements on 2 evaluative dimensions: (1) “significance”; and (2) “strength of support” (for details see [ 4 ]). For example, a study may be described as having “landmark” significance and offering “exceptional” strength of support (for a complete example, see Box 1 ). The phrases are drawn from “widely used expressions” in prior eLife assessments and the stated goal is to “help convey the views of the editor and the reviewers in a clear and consistent manner” [ 4 ]. Here, we report a study which assessed whether the language used in eLife assessments is perceived clearly and consistently by potential readers. We also assessed alternative language that may improve communication.
- PPT PowerPoint slide
- PNG larger image
- TIFF original image
The significance dimension is represented by 5 phrases and the strength of support dimension is represented by 6 phrases. In a particular eLife assessment, readers only see 1 phrase from each of the evaluative dimensions. Phrases are accompanied by eLife definitions, but these are not shown in eLife assessments (though some words from the definitions may be used).
https://doi.org/10.1371/journal.pbio.3002645.t001
Our understanding (based on [ 4 ]) is that eLife intends the common vocabulary to represent different degrees of each evaluative dimension on an ordinal scale (e.g., “landmark” findings are more significant than “fundamental” findings and so forth); however, in our view the intended ordering is sometimes ambiguous or counterintuitive. For example, it does not seem obvious to us that an “important” study is necessarily more significant than a “valuable” study nor does a “compelling” study seem necessarily stronger than a “convincing” study. Additionally, several phrases like “solid” and “useful,” could be broadly interpreted, leading to a mismatch between intended meaning and perceived meaning. The phrases also do not cover the full continuum of measurement and are unbalanced in terms of positive and negative phrases. For example, the “significance” dimension has no negative phrases—the scale endpoints are “landmark” and “useful.” We also note that the definitions provided by eLife do not always map onto gradations of the same construct. For example, the eLife definitions of phrases on the significance dimension suggest that the difference between “useful,” “valuable,” and “important” is a matter of breadth/scope (whether the findings have implications beyond a specific subfield), whereas the difference between “fundamental” and “landmark” is a matter of degree. In short, we are concerned that several aspects of the eLife vocabulary may undermine communication of research evaluations to readers.
In Table 2 , we outline an alternative vocabulary that is intended to overcome these potential issues with the eLife vocabulary. Phrases in the alternative vocabulary explicitly state the relevant evaluative dimension (e.g., “support”) along with a modifying adjective that unambiguously represents degree (e.g., “very low”). The alternative vocabulary is intended to cover the full continuum of measurement and be balanced in terms of positive and negative phrases. We have also renamed “significance” to “importance” to avoid any confusion with statistical significance. We hope that these features will facilitate alignment of readers’ interpretations with the intended interpretations, improving the efficiency and accuracy of communication.
Box 1. A complete example of an eLife assessment. This particular example uses the phrase “important,” to convey the study’s significance, and the phrase “compelling,” to convey the study’s strength of support
“The overarching question of the manuscript is important and the findings inform the patterns and mechanisms of phage-mediated bacterial competition, with implications for microbial evolution and antimicrobial resistance. The strength of the evidence in the manuscript is compelling, with a huge amount of data and very interesting observations. The conclusions are well supported by the data. This manuscript provides a new co-evolutionary perspective on competition between lysogenic and phage-susceptible bacteria that will inform new studies and sharpen our understanding of phage-mediated bacterial co-evolution.” [ 5 ].
https://doi.org/10.1371/journal.pbio.3002645.t002
The utility of eLife assessments will depend (in part) on whether readers interpret the common vocabulary in the manner that eLife intends. Mismatches between eLife’s intentions and readers’ perceptions could lead to inefficient or inaccurate communication. In this study, we empirically evaluated how the eLife vocabulary ( Table 1 ) is interpreted and assessed whether an alternative vocabulary ( Table 2 ) elicited more desirable interpretations. Our goal was not to disparage eLife’s progressive efforts, but to make a constructive contribution towards a more transparent and informative peer review process. We hope that a vocabulary with good empirical performance will be more attractive and useful to other journals considering adopting eLife’s approach.
Our study is modelled on prior studies that report considerable individual differences in people’s interpretation of probabilistic phrases [ 6 – 12 ]. In a prototypical study of this kind, participants are shown a probabilistic statement like “It will probably rain tomorrow” and asked to indicate the likelihood of rain on a scale from 0% to 100%. Analogously, in our study participants read statements describing hypothetical scientific studies using phrases drawn from the eLife vocabulary or the alternative vocabulary and were asked to rate the study’s significance/importance or strength of support on a scale from 0 to 100. We used these responses to gauge the extent to which people’s interpretations of the vocabulary were consistent with each other and consistent with the intended rank order.
Research aims
Our overarching goal was to identify clear language for conveying evaluations of scientific papers. We hope that this will make it easier for other journals/platforms to follow in eLife’s footsteps and move towards more transparent and informative peer review.
With this overall goal in mind, we had 3 specific research aims:
- Aim One. To what extent do people share similar interpretations of phrases used to describe scientific research?
- Aim Two. To what extent do people’s (implied) ranking of phrases used to describe scientific research align with (a) each other; and (b) with the intended ranking?
- Aim Three. To what extent do different phrases used to describe scientific research elicit overlapping interpretations and do those interpretations imply broad coverage of the underlying measurement scale?
Our methods adhered to our preregistered plan ( https://doi.org/10.17605/OSF.IO/MKBTP ) with one minor deviation: our target sample size was 300, but we accidentally recruited an additional participant, so the actual sample size was 301.
This study was approved by a University of Melbourne ethics board (project ID: 26411).
We conducted an experiment with a repeated-measures design. Participants were shown short statements that described hypothetical scientific studies in terms of their significance/importance or strength of support using phrases drawn from the eLife vocabulary ( Table 1 ) and from the alternative vocabulary ( Table 2 ). The statements were organised into 4 blocks based on vocabulary and evaluative dimension; specifically, block one: eLife-significance (5 statements), block two: eLife-support (6 statements), block three: alternative-importance (5 statements), block four: alternative-support (5 statements). Each participant saw all 21 phrases and responded using a 0% to 100% slider scale to indicate their belief about each hypothetical study’s significance/importance or strength of support.
There were 21 statements that described hypothetical scientific studies using one of the 21 phrases included in the 2 vocabularies ( Table 1 ). Statements referred either to a study’s strength of support (e.g., Fig 1 ) or a study’s significance/importance (e.g., Supplementary Figure A in S1 Text ). For the alternative vocabulary, we used the term “importance” rather than “significance.” To ensure the statements were grammatically accurate, it was necessary to use slightly different phrasing when communicating significance with the eLife vocabulary (“This is an [phrase] study”) compared to communicating importance with the alternative vocabulary (“This study has [phrase] importance”; e.g., Supplementary Figure B in S1 Text ).
https://doi.org/10.1371/journal.pbio.3002645.g001
Additionally, there was 1 attention check statement (Supplementary Figure C in S1 Text ), a question asking participants to confirm their highest completed education level (options: Undergraduate degree (BA/BSc/other)/Graduate degree (MA/MSc/MPhil/other)/Doctorate degree (PhD/other)/Other), and a question asking participants the broad subject area of their highest completed education level (options: Arts and Humanities/Life Sciences and Biomedicine/Physical Sciences/Social Sciences/Other). The veridical materials are available at https://osf.io/jpgxe/ .
Sample source.
Participants were recruited from the online participant recruitment platform Prolific ( https://www.prolific.co/ ). As of 23rd August 2023, the platform had 123,064 members. Demographic information about Prolific members is provided in S8 Text .
Sample size.
As data collection unfolded, we intermittently checked how many participants had met the inclusion criteria, aiming to stop data collection when we had eligible data for our target sample size of 300 participants. Ultimately, 461 participants responded to the survey. Of these 461 participants, 156 participants failed the attention check and 12 participants took longer than 30 min to complete the study and were therefore excluded. No participants failed to respond to all 21 statements or completed the study too quickly (<5 min). We applied these exclusion criteria one-by-one which removed data from 160 participants and retained eligible data from 301 participants (we unintentionally recruited 1 additional participant).
Sample size justification.
The target sample size of 300 was based on our resource constraints and expectations about statistical power and precision (see S2 Text ).
Inclusion criteria.
Participants had to have a ≥95% approval rate for prior participation on the recruitment platform (Prolific). Additionally, Prolific prescreening questions were used to ensure that the study was only available to participants who reported that they speak fluent English, were aged between 18 and 70 years, and had completed a doctorate degree (PhD/other).
- Data collection and recruitment via the Prolific platform began on September 13, 2023 and was completed on September 14, 2023.
- After responding to the study advert ( https://osf.io/a25vq ), participants read an information sheet ( https://osf.io/39vay ) and provided consent ( https://osf.io/xdar7 ). During this process, they were told that the study seeks to understand “how people perceive words used to describe scientific studies so we can improve communication of research to the general public.”
- Participants completed the task remotely online via the Qualtrics platform. Before starting the main task, they read a set of instructions and responded to a practice statement ( S3 Text ).
- For the main task, statements were presented sequentially, and participants responded to them in their own time. The order of presentation was randomized, both between and within the 4 blocks of statements. After each statement, there was a 15-s filler task during which participants were asked to complete as many multiplication problems (e.g., 5 × 7 =?) as they could from a list of 10. The multiplication problems were randomly generated every time they appeared using the Qualtrics software. Only numbers between 1 and 15 were used to ensure that most of the problems were relatively straightforward to solve. A single “attention check” statement (Supplementary Figure C in S1 Text ) appeared after all 4 blocks had been completed.
- Participants were required to respond to each statement before they could continue to the next statement. The response slider could be readjusted as desired until the “next” button was pressed, after which participants could not return to or edit prior responses.
- After responding to all 21 statements and the attention check, participants were shown a debriefing document ( https://osf.io/a9gve ).
All analyses adhered to our preregistered plan ( https://doi.org/10.17605/OSF.IO/MKBTP ). Numbers in square brackets represent 95% confidence intervals computed with the Sison–Glaz method [ 13 ] for multinomial proportions or bootstrapped with the percentile method [ 14 ] for percentile estimates.
Participant characteristics
Participants stated that their highest completed education level was either a doctorate degree ( n = 287) or graduate degree ( n = 14). Participants reported that the subject areas that most closely represented their degrees were Life Sciences and Biomedicine ( n = 97), Social Sciences ( n = 77), Physical Sciences ( n = 57), Arts and Humanities ( n = 37), and various “other” disciplines ( n = 33).
Response distributions
The distribution of participants’ responses to each phrase is shown in Fig 2 (importance/significance dimension) and Fig 3 (strength of support dimension). These “ridgeline” plots [ 15 ] are kernel density distributions which represent the relative probability of observing different responses (akin to a smoothed histogram).
Tables 3 and 4 show the 25th, 50th (i.e., median), and 75th percentiles of responses for each phrase (as represented by the black vertical lines in Figs 2 and 3 ). The tables include 95% confidence intervals only for medians to make them easier to read; however, confidence intervals for all percentile estimates are available in Supplementary Table A in S5 Text and Supplementary Table B in in S5 Text .
Responses to each phrase on the importance/significance dimension as kernel density distributions with the 25th, 50th (i.e., median), and 75th quantiles represented by black vertical lines and the 25th–75th quantile region (i.e., interquartile range) highlighted in blue. The data underlying this figure can be found in https://osf.io/mw2q4/files/osfstorage .
https://doi.org/10.1371/journal.pbio.3002645.g002
Responses to each phrase on the strength of support dimension as kernel density distributions with the 25th, 50th (i.e., median), and 75th quantiles represented by black vertical lines and the 25th–75th quantile region (i.e., interquartile range) highlighted in blue. The data underlying this figure can be found in https://osf.io/mw2q4/files/osfstorage .
https://doi.org/10.1371/journal.pbio.3002645.g003
The data underlying this table can be found in https://osf.io/mw2q4/files/osfstorage .
https://doi.org/10.1371/journal.pbio.3002645.t003
https://doi.org/10.1371/journal.pbio.3002645.t004
Implied ranking of evaluative phrases
Do participants’ implied rankings match the intended rankings.
Although participants rated each statement separately on a continuous scale, these responses also imply an overall ranking of the phrases (in order of significance/importance or strength of support). Ideally, an evaluative vocabulary elicits implied rankings that are both consistent among participants and consistent with the intended ranking. Fig 4 shows the proportion of participants whose implied ranking matched the intended ranking (i.e., “correct ranking”) for the different evaluative dimensions and vocabularies.
On the significance/importance dimension, 59 (20% [15% to 24%]) participants’ implied rankings of the eLife vocabulary aligned with the intended ranking and 188 (62% [57% to 68%]) participants’ implied rankings of the alternative vocabulary aligned with the intended ranking. We performed an “exact” McNemar test and computed the McNemar odds ratio with Clopper–Pearson 95% confidence intervals adjusted with the “midp” method, as recommended by [ 16 ]. The McNemar test indicated that observing a difference between the vocabularies this large, or larger, is unlikely if the null hypothesis were true (odds ratio = 8.17, 95% CI [5.11, 13.69], p = 1.34e-26). The intended ranking was the most popular for both vocabularies; however, participants had 55 different implied rankings for the eLife vocabulary and 8 different implied rankings for the alternative vocabulary (for details, see Supplementary Tables A–D in S6 Text ). Note that these values should be compared with caution, as for the significance/importance dimension, the eLife vocabulary had more (6) phrases than the alternative vocabulary (which had 5 phrases and therefore fewer possible rankings).
On the strength of support dimension, 45 (15% [11% to 20%]) participants’ ratings of the eLife phrases were in accordance with the intended ranking relative to 201 (67% [62% to 72%]) participants who correctly ranked the alternative vocabulary. A McNemar test indicated that observing a difference between the vocabularies this large, or larger, is unlikely if the null hypothesis were true (odds ratio = 11.4, 95% CI [6.89, 20.01], p = 5.73e-35). The intended ranking was the most popular for both vocabularies; though for the eLife vocabulary, an unintended ranking that swapped the ordinal positions of “convincing” and “solid” came a close second, reflected in the ratings of 44 (15% [10% to 19%]) participants. Overall, there were 34 different implied rankings for the eLife vocabulary, relative to 10 implied rankings for the alternative vocabulary.
Error bars represent 95% confidence intervals using the Sison–Glaz method [ 13 ]. The data underlying this figure can be found in https://osf.io/mw2q4/files/osfstorage .
https://doi.org/10.1371/journal.pbio.3002645.g004
Quantifying ranking similarity.
Thus far, our analyses have emphasised the binary difference between readers’ implied rankings and eLife’s intended rankings. A complementary analysis quantifies the degree of similarity between rankings using Kendall’s tau distance ( K d )—a metric that describes the difference between 2 lists in terms of the number of adjacent pairwise swaps required to convert one list into the other [ 17 , 18 ]. The larger the distance, the larger the dissimilarity between the 2 lists. K d ranges from 0 (indicating a complete match) to n(n-1)/2 (where n is the size of one list). Because the eLife strength of support dimension has 6 phrases and all other dimensions have 5 phrases, we report the normalised K d which ranges from 0 (maximal similarity) to 1 (maximal dissimilarity). Further explanation of K d is provided in S7 Text .
Fig 5 illustrates the extent to which participants’ observed rankings deviated from the intended ranking in terms of normalised K d . This suggests that although deviations from the intended eLife ranking were common, they only tended to be on the order of 1 or 2 discordant rank pairs. By contrast, the alternative vocabulary rarely resulted in any deviations, and when it did, these were typically only in terms of one discordant rank pair.
Zero represents a perfect match (green bar), other values (grey bars) represent increasing dissimilarity from the intended ranking up to 1, which represents maximal dissimilarity. The data underlying this figure can be found in https://osf.io/mw2q4/files/osfstorage .
https://doi.org/10.1371/journal.pbio.3002645.g005
Locus of ranking deviations.
So far, we have examined how many participants adhered to the intended ranking ( Fig 4 ) and the extent to which their implied rankings deviated from the intended rankings ( Fig 5 ). However, these approaches do not optimally illustrate where the ranking deviations were concentrated (i.e., which phrases were typically being misranked). The heat maps in Fig 6 show the percentage of participants whose implied rankings matched or deviated from the intended ranking at the level of individual phrases. Ideally, a phrase’s observed rank will match its intended rank for 100% of participants. For example, the heat maps show that almost all participants (98%) correctly ranked “moderate importance” and “moderate support” in the alternative vocabulary. The heat maps also reveal phrases that were often misranked with each other, for example: “solid,” “convincing,” and “compelling” in the eLife vocabulary.
Darker colours and higher percentages indicate greater concordance between the implied rank and the intended rank of a particular phrases. The data underlying this figure can be found in https://osf.io/mw2q4/files/osfstorage .
https://doi.org/10.1371/journal.pbio.3002645.g006
Research articles published in eLife are accompanied by evaluation statements that use phrases from a prescribed vocabulary ( Table 1 ) to describe a study’s importance (e.g., “landmark”) and strength of support (e.g., “compelling”). If readers, reviewers, and editors interpret the prescribed vocabulary differently to the intended meaning, or inconsistently with each other, it could lead to miscommunication of research evaluations. In this study, we assessed the extent to which people’s interpretations of the eLife vocabulary are consistent with each other and consistent with the intended ordinal structure. We also examined whether an alternative vocabulary ( Table 2 ) improved consistency of interpretation.
Overall, the empirical data supported our initial intuitions: while some phrases in the eLife vocabulary were interpreted relatively consistently (e.g., “exceptional” and “landmark”), several phrases elicited broad interpretations that overlapped a great deal with other phrases’ interpretation (particularly the phrases “fundamental,” “important,” and “valuable” on the significance/importance dimension ( Fig 2 ) and “compelling,” “convincing,” and “useful” on the strength of support dimension ( Fig 3 )). This suggests these phrases are not ideal for discriminating between studies with different degrees of importance and strength of support. If the same phrases often mean different things to different people, there is a danger of miscommunication between the journal and its readers. Responses on the significance/importance dimension were largely confined to the upper half of the scale, which is unsurprising, given the absence of negative phrases. It is unclear if the exclusion of negative phrases was a deliberate choice on the part of eLife’s leadership (because articles with little importance would not be expected to make it through editorial triage) or an oversight. Most participants’ implied rankings of the phrases were misaligned with the ranking intended by eLife—20% of participants had aligned rankings on the significance/importance dimension and 15% had aligned rankings on the strength of support dimension ( Fig 4 ). The degree of mismatch was typically in the range of 1 or 2 discordant ranks ( Fig 5 ). Heat maps ( Fig 6 ) highlighted that phrases in the middle of scale (e.g., “solid,” “convincing”) were most likely to have discordant ranks.
By contrast, phrases in the alternative vocabulary tended to elicit more consistent interpretations across participants and interpretations that had less overlap with other phrases (Figs 2 and 3 and Tables 3 and 4 ). The alternative vocabulary was more likely to elicit implied rankings that matched the intended ranking—62% of participants had aligned rankings on the significance/importance dimension and 67% had aligned rankings on the strength of support dimension ( Fig 4 ). Mismatched rankings were usually misaligned by one rank ( Fig 5 ). Although the alternative vocabulary had superior performance to the eLife vocabulary, it was nevertheless imperfect. Specifically, interpretation of phrases away from the middle of the scale on both dimensions (e.g., “low importance” and “very low importance”) tended to have some moderate overlap (Figs 2 , 3 , and 6 ). We do not know what caused this overlap, but, as discussed in the next paragraph, one possibility is that it is overly optimistic to expect peoples’ intuitions to align when they judge phrases in isolation, without any knowledge of the underlying scale.
Rather than presenting evaluative phrases in isolation (as occurs for eLife readers and occurred for participants in our study), informing people of the underlying ordinal scale may help to improve communication of evaluative judgements. eLife could refer readers to an external explanation of the vocabulary; however, prior research on interpretation of probabilistic phrases suggests this may be insufficient as most people neglect to look up the information [ 6 , 19 ]. A more effective option might be to explicitly present the phrases in their intended ordinal structure [ 19 ]. For example, the full importance scale could be attached to each evaluation statement with the relevant phrase selected by reviewers/editors highlighted ( Fig 7A ). Additionally, phrases could be accompanied by mutually exclusive numerical ranges ( Fig 7B ); prior research suggests that this can improve consistency of interpretation for probabilistic phrases [ 19 ]. It is true that the limits of such ranges are arbitrary, and editors may be concerned that using numbers masks vague subjective evaluations in a veil of objectivity and precision. To some extent we share these concerns; however, the goal here is not to develop an “objective” measurement of research quality, but to have practical guidelines that improve accuracy of communication. Specifying a numerical range may help to calibrate the interpretations of evaluators and readers so that the uncertainty can be accurately conveyed. Future research could also explore the relationship between the number of items included in the vocabulary and the level of precision that reviewers/editors wish to communicate.
Explicit presentation of the intended ordinal structure (A–C), potentially with numerical ranges (B), could improve consistency of interpretation. Judgements by multiple reviewers could also be represented (by different arrows) without forcing consensus (C).
https://doi.org/10.1371/journal.pbio.3002645.g007
Our study has several important limitations. First, we did not address whether editor/reviewer opinions provide valid assessments of studies or whether the vocabularies provide valid measurements of those opinions. We also note that eLife assessments are formed via consensus, rather than representing the opinions of individuals, which raises questions about how social dynamics may affect the evaluation outcomes. It may be more informative to solicit and report individual assessments from each peer reviewer and editor, rather than force a consensus (e.g., see Fig 7C ). Although these are important issues, they are beyond the scope of this study, which is focused on clarity of communication.
Second, we are particularly interested in how the readership of eLife interpret the vocabularies, but because we do not have any demographic information about the readership, we do not know the extent to which our sample is similar to that population. We anticipated that the most relevant demographic characteristics were education status (because the content is technical), knowledge of subject area (because eLife publishes biomedical and life sciences), and language (because the content is in English). All of our participants reported speaking fluent English, the vast majority had doctoral degrees, and about one third had a degree in the Biomedical and Life Sciences. Relative to this sample, we expect the eLife readership probably consists of more professional scientists, but otherwise we think the sample is likely to be a good match to the target population. Also note that eLife explicitly states that eLife assessments are intended to be accessible to non-expert readers [ 4 ], therefore, our sample is still a relevant audience, even if it might contain fewer professional scientists than eLife’s readership.
Third, to maintain experimental control, we presented participants with very short statements that differed only in terms of the phrases we wished to evaluate. In practice however, these phrases will be embedded in a paragraph of text (e.g., Box 1 ) which may also contain “aspects” of the vocabulary definitions ( Table 1 ) “when appropriate” [ 4 ]. It is unclear if the inclusion of text from the intended phrase definitions will help to disambiguate the phrases and future research could explore this.
Fourth, participants were asked to respond to phrases with a point estimate; however, it is likely that a range of plausible values would more accurately reflect their interpretations [ 9 , 11 ]. Because asking participants to respond with a range (rather than a point estimate) creates technical and practical challenges in data collection and analysis, we opted to obtain point estimates only.
Overall, our study suggests that using more structured and less ambiguous language can improve communication of research evaluations. Relative to the eLife vocabulary, participants’ interpretations of our alternative vocabulary were more likely to align with each other, and with the intended interpretation. Nevertheless, some phrases in the alternatively vocabulary were not always interpreted as we intended, possibly because participants were not completely aware of the vocabulary’s underlying ordinal scale. Future research, in addition to finding optimal words to evaluate research, could attempt to improve interpretation by finding optimal ways to present them.
Supporting information
S1 text. example stimuli and attention check..
https://doi.org/10.1371/journal.pbio.3002645.s001
S2 Text. Sample size planning.
https://doi.org/10.1371/journal.pbio.3002645.s002
S3 Text. Task instructions.
https://doi.org/10.1371/journal.pbio.3002645.s003
S4 Text. Peer Community in Registered Reports Design Template.
https://doi.org/10.1371/journal.pbio.3002645.s004
S5 Text. Expanded versions of Table 3 and Table 4 percentile estimates with confidence intervals.
https://doi.org/10.1371/journal.pbio.3002645.s005
S6 Text. Frequency of implied rankings.
https://doi.org/10.1371/journal.pbio.3002645.s006
S7 Text. Further explanation of Kendall’s distance.
https://doi.org/10.1371/journal.pbio.3002645.s007
S8 Text. Demographics of Prolific members.
https://doi.org/10.1371/journal.pbio.3002645.s008
Acknowledgments
The Stage 1 version of this preregistered research article has been peer-reviewed and recommended by Peer Community in Registered Reports ( https://rr.peercommunityin.org/articles/rec?id=488 ).
Research transparency statement
The research question, methods, and analysis plan were peer reviewed and preregistered as a Stage One Registered Report via the Peer Community in Registered Reports platform ( https://doi.org/10.17605/OSF.IO/MKBTP ). There was only 1 minor deviation from the preregistered protocol (target sample size = 300; actual sample size = 301). A Peer Community in Registered Reports design table is available in S4 Text . All data, materials, and analysis scripts are publicly available on the Open Science Framework ( https://osf.io/mw2q4/files/osfstorage/ ). A reproducible version of the manuscript and associated computational environment is available in a Code Ocean container ( https://doi.org/10.24433/CO.4128032.v1 ).
- View Article
- Google Scholar
- PubMed/NCBI
- 4. eLife’s New Model: What is an eLife assessment? In: eLife [Internet]. eLife Sciences Publications Limited; 20 Oct 2022 [cited 2023 Jan 24]. Available from: https://elifesciences.org/inside-elife/db24dd46/elife-s-new-model-what-is-an-elife-assessment .
- 15. Wilke C. Fundamentals of data visualization: a primer on making informative and compelling figures. First edition. Sebastopol, CA: O’Reilly Media; 2019.
Information
- Author Services
Initiatives
You are accessing a machine-readable page. In order to be human-readable, please install an RSS reader.
All articles published by MDPI are made immediately available worldwide under an open access license. No special permission is required to reuse all or part of the article published by MDPI, including figures and tables. For articles published under an open access Creative Common CC BY license, any part of the article may be reused without permission provided that the original article is clearly cited. For more information, please refer to https://www.mdpi.com/openaccess .
Feature papers represent the most advanced research with significant potential for high impact in the field. A Feature Paper should be a substantial original Article that involves several techniques or approaches, provides an outlook for future research directions and describes possible research applications.
Feature papers are submitted upon individual invitation or recommendation by the scientific editors and must receive positive feedback from the reviewers.
Editor’s Choice articles are based on recommendations by the scientific editors of MDPI journals from around the world. Editors select a small number of articles recently published in the journal that they believe will be particularly interesting to readers, or important in the respective research area. The aim is to provide a snapshot of some of the most exciting work published in the various research areas of the journal.
Original Submission Date Received: .
- Active Journals
- Find a Journal
- Proceedings Series
- For Authors
- For Reviewers
- For Editors
- For Librarians
- For Publishers
- For Societies
- For Conference Organizers
- Open Access Policy
- Institutional Open Access Program
- Special Issues Guidelines
- Editorial Process
- Research and Publication Ethics
- Article Processing Charges
- Testimonials
- Preprints.org
- SciProfiles
- Encyclopedia
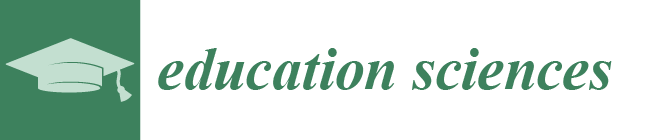
Article Menu

- Subscribe SciFeed
- Recommended Articles
- Google Scholar
- on Google Scholar
- Table of Contents
Find support for a specific problem in the support section of our website.
Please let us know what you think of our products and services.
Visit our dedicated information section to learn more about MDPI.
JSmol Viewer
How scientific is cognitive load theory research compared to the rest of educational psychology.
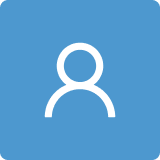
1. How Scientific Is Cognitive Load Theory Research Compared to the Rest of Educational Psychology?
1.1. overview, 1.2. causal conclusions and recommendations for practice, 1.3. the present review, 2.1. journal selection and search process, 2.2. coding and analysis, 3.1. research designs, 3.2. recommendations for practice, 4. discussion, 4.1. limitations and future directions, 4.2. conclusions, author contributions, conflicts of interest.
- Sweller, J. Cognitive load during problem solving: Effects on learning. Cogn. Sci. 1988 , 12 , 257–285. [ Google Scholar ] [ CrossRef ]
- Hassan, W.; Martella, A.M.; Robinson, D.H. Identifying the most cited articles and authors in educational psychology journals from 1988–2023. Educ. Psychol. Rev. 2024 . in press . [ Google Scholar ]
- Sweller, J.; Chandler, P. Evidence for cognitive load theory. Cogn. Instr. 1991 , 8 , 351–362. [ Google Scholar ] [ CrossRef ]
- Sweller, J.; van Merriënboer, J.J.G.; Paas, F. Cognitive architecture and instructional design: 20 years later. Educ. Psychol. Rev. 2019 , 31 , 261–292. [ Google Scholar ] [ CrossRef ]
- Schnotz, W.; Kürschner, C. A reconsideration of cognitive load theory. Educ. Psychol. Rev. 2007 , 19 , 469–508. [ Google Scholar ] [ CrossRef ]
- de Jong, T. Cognitive load theory, educational research, and instructional design: Some food for thought. Instr. Sci. 2010 , 38 , 105–134. [ Google Scholar ] [ CrossRef ]
- Brady, A.; Griffin, M.M.; Lewis, A.R.; Fong, C.J.; Robinson, D.H. How scientific is educational psychology research? The increasing trend of squeezing causality and recommendations from non-intervention studies. Educ. Psychol. Rev. 2023 , 35 , 37. [ Google Scholar ] [ CrossRef ]
- Robinson, D.H.; Wainer, H. It’s just an observation. Educ. Psychol. Rev. 2023 , 35 , 83. [ Google Scholar ] [ CrossRef ]
- Hsieh, P.; Acee, T.; Chung, W.-H.; Hsieh, Y.-P.; Kim, H.; Thomas, G.D.; You, J.-I.; Levin, J.R.; Robinson, D.H. Is educational intervention research on the decline? J. Educ. Psychol. 2005 , 97 , 523–529. [ Google Scholar ] [ CrossRef ]
- Robinson, D.H.; Levin, J.R.; Thomas, G.D.; Pituch, K.A.; Vaughn, S. The incidence of “causal” statements in teaching-and-learning research journals. Am. Educ. Res. J. 2007 , 44 , 400–413. [ Google Scholar ] [ CrossRef ]
- Reinhart, A.L.; Haring, S.H.; Levin, J.R.; Patall, E.A.; Robinson, D.H. Models of not-so good behavior: Yet another way to squeeze causality and recommendations for practice out of correlational data. J. Educ. Psychol. 2013 , 105 , 241–247. [ Google Scholar ] [ CrossRef ]
- Alexander, P.A. In praise of (reasoned and reasonable) speculation: A response to Robinson et al.’s moratorium on recommendations for practice. Educ. Psychol. Rev. 2013 , 25 , 303–308. [ Google Scholar ] [ CrossRef ]
- Harris, K.R. Disallowing recommendations for practice and policy: A proposal that is both too much and too little. Educ. Psychol. Rev. 2013 , 25 , 309–316. [ Google Scholar ] [ CrossRef ]
- Mayer, R.E. How to assess whether an instructional intervention has an effect on learning. Educ. Psychol. Rev. 2023 , 35 , 64. [ Google Scholar ] [ CrossRef ]
- Renkl, A. Why practice recommendations are important in use-inspired basic research and why too much caution is dysfunctional. Educ. Psychol. Rev. 2013 , 25 , 317–324. [ Google Scholar ] [ CrossRef ]
- Dumas, D.; Edelsbrunner, P. How to make recommendations for educational practice from correlational data using structural equation models. Educ. Psychol. Rev. 2023 , 35 , 48. [ Google Scholar ] [ CrossRef ]
- Grosz, M.P. Should researchers make causal inferences and recommendations for practice on the basis of nonexperimental studies? Educ. Psychol. Rev. 2023 , 35 , 57. [ Google Scholar ] [ CrossRef ]
- Grosz, M.P.; Rohrer, J.M.; Thoemmes, F. The taboo against explicit causal inference in nonexperimental psychology. Perspect. Psychol. Sci. 2020 , 15 , 1243–1255. [ Google Scholar ] [ CrossRef ]
- Zitzmann, S.; Machts, N.; Hübner, N.; Schauber, S.; Möller, J.; Lindner, C. The yet underestimated importance of communicating findings from educational trials to teachers, schools, school authorities, or policy makers (Comment on Brady et al. 2023)). Educ. Psychol. Rev. 2023 , 35 , 65. [ Google Scholar ] [ CrossRef ]
- Martella, A.M.; Martella, R.C.; Yatcilla, J.K.; Newson, A.; Shannon, E.N.; Voorhis, C. How rigorous is active learning research in STEM education? An examination of key internal validity controls in intervention studies. Educ. Psychol. Rev. 2023 , 35 , 107. [ Google Scholar ] [ CrossRef ]
- Shavelson, R.J.; Towne, L. Scientific Research in Education ; National Academy Press: Washington, DC, USA, 2002. [ Google Scholar ]
- Lawson, A.P.; Martella, A.M.; LaBonte, K.; Delgado, C.Y.; Zhao, F.; Gluck, J.A.; Munns, M.E.; Wells LeRoy, A.; Mayer, R.E. Confounded or controlled? A systematic review of media comparison studies involving immersive virtual reality for STEM education. Educ. Psychol. Rev. 2024 , 36 , 69. [ Google Scholar ] [ CrossRef ]
- Martella, R.C.; Nelson, J.R.; Morgan, R.L.; Marchand-Martella, N.E. Understanding and Interpreting Educational Research ; The Guilford Press: New York, NY, USA, 2013. [ Google Scholar ]
- Chen, Z.; Klahr, D. All other things being equal: Acquisition and transfer of the control of variables strategy. Child Dev. 1999 , 70 , 1098–1120. [ Google Scholar ] [ CrossRef ]
- Kaya, C. Internal validity: A must in research designs. Educ. Res. Rev. 2015 , 10 , 111–118. [ Google Scholar ] [ CrossRef ]
- Bichler, S.; Schwaighofer, M.; Stadler, M.; Bühner, M.; Greiff, S.; Fischer, F. How working memory capacity and shifting matter for learning with worked examples—A replication study. J. Educ. Psychol. 2020 , 112 , 1320–1337. [ Google Scholar ] [ CrossRef ]
- de Koning, B.B.; Rop, G.; Paas, F. Learning from split-attention materials: Effects of teaching physical and mental learning strategies. Contemp. Educ. Psychol. 2020 , 61 , 101873. [ Google Scholar ] [ CrossRef ]
- Merkt, M.; Lux, S.; Hoogerheide, V.; van Gog, T.; Schwan, S. A change of scenery: Does the setting of an instructional video affect learning? J. Educ. Psychol. 2020 , 112 , 1273–1283. [ Google Scholar ] [ CrossRef ]
- Miller-Cotto, D.; Byrnes, J.P. What’s the best way to characterize the relationship between working memory and achievement? An initial examination of competing theories. J. Educ. Psychol. 2020 , 112 , 1074–1084. [ Google Scholar ] [ CrossRef ]
- Schneider, S.; Nebel, S.; Beege, M.; Rey, G.D. The retrieval-enhancing effects of decorative pictures as memory cues in multimedia learning videos and subsequent performance tests. J. Educ. Psychol. 2020 , 112 , 1111–1127. [ Google Scholar ] [ CrossRef ]
- Zu, T.; Hutson, J.; Loschky, L.C.; Rebello, N.S. Using eye movements to measure intrinsic, extraneous, and germane load in a multimedia learning environment. J. Educ. Psychol. 2020 , 112 , 1338–1352. [ Google Scholar ] [ CrossRef ]
- Buchin, Z.L.; Mulligan, N.W. Retrieval-based learning and prior knowledge. J. Educ. Psychol. 2023 , 115 , 22–35. [ Google Scholar ] [ CrossRef ]
- Ehrhart, T.; Lindner, M.A. Computer-based multimedia testing: Effects of static and animated representational pictures and text modality. Contemp. Educ. Psychol. 2023 , 73 , 102151. [ Google Scholar ] [ CrossRef ]
- Hoch, E.; Sidi, Y.; Ackerman, R.; Hoogerheide, V.; Schiter, K. Comparing mental effort, difficulty, and confidence appraisals in problem-solving: A metacognitive perspective. Educ. Psychol. Rev. 2023 , 35 , 61. [ Google Scholar ] [ CrossRef ]
- Martin, A.J.; Ginns, P.; Nagy, R.P.; Collie, R.J.; Bostwick, K.C.P. Load reduction instruction in mathematics and English classrooms: A multilevel study of student and teacher reports. Contemp. Educ. Psychol. 2023 , 72 , 102147. [ Google Scholar ] [ CrossRef ]
- Park, B.; Korbach, A.; Ginns, P.; Brüken, R. How learners use their hands for learning: An eye-tracking study. Educ. Psychol. Rev. 2023 , 35 , 116. [ Google Scholar ] [ CrossRef ]
- Pengelley, J.; Whipp, P.R.; Rovis-Hermann, N. A testing load: Investigating test mode effects on test score, cognitive load and scratch paper use with secondary school students. Educ. Psychol. Rev. 2023 , 35 , 67. [ Google Scholar ] [ CrossRef ]
- Rau, M.A.; Beier, J.P. Exploring the effects of gesture-based collaboration on students’ benefit from a perceptual training. J. Educ. Psychol. 2023 , 115 , 267–289. [ Google Scholar ] [ CrossRef ]
- Sondermann, C.; Merkt, M. What is the effect of talking heads in educational videos with different types of narrated slides? Contemp. Educ. Psychol. 2023 , 74 , 102207. [ Google Scholar ] [ CrossRef ]
- Wang, F.; Cheng, M.; Mayer, R.E. Improving learning-by-teaching without audience interaction as a generative learning activity by minimizing the social presence of the audience. J. Educ. Psychol. 2023 , 115 , 783–797. [ Google Scholar ] [ CrossRef ]
- Yang, X.; Wang, F.; Mayer, R.E.; Hu, X.; Gu, C. Ocular foundations of the spatial contiguity principle: Designing multimedia materials for parafoveal vision. J. Educ. Psychol. 2023 , 115 , 1125–1140. [ Google Scholar ] [ CrossRef ]
- Sweller, J. The development of cognitive load theory: Replication crises and invorporation of other theories can lead to theory expansion. Educ. Psychol. Rev. 2023 , 35 , 95. [ Google Scholar ] [ CrossRef ]
- Zhang, L.; Kirschner, P.A.; Cobern, W.W.; Sweller, J. There is an evidence crisis in science educational policy. Educ. Psychol. Rev. 2022 , 34 , 1157–1176. [ Google Scholar ] [ CrossRef ]
- Sweller, J. Chapter two: Cognitive load theory. Psychol. Learn. Motiv. 2011 , 55 , 37–76. [ Google Scholar ] [ CrossRef ]
- Dempster, F.N. The spacing effect: A case study in the failure to apply the results of psychological research. Am. Psychol. 1988 , 43 , 627–634. [ Google Scholar ] [ CrossRef ]
Click here to enlarge figure
Authors | Journal | Method | Recommendations for Practice? |
---|---|---|---|
2020 | |||
Bichler et al. [ ] | Journal of Educational Psychology | Experimental | -- |
de Koning et al. [ ] | Contemporary Educational Psychology | Experimental | -- |
Merkt et al. [ ] | Journal of Educational Psychology | Experimental | -- |
Miller-Cotto & Byrnes [ ] | Journal of Educational Psychology | Observational/ Correlational | No |
Schneider et al. [ ] | Journal of Educational Psychology | Experimental | -- |
Zu et al. [ ] | Journal of Educational Psychology | Experimental | -- |
2023 | |||
Buchin & Mulligan [ ] | Journal of Educational Psychology | Experimental | -- |
Ehrhart & Lindner [ ] | Contemporary Educational Psychology | Experimental | -- |
Hoch et al. [ ] | Educational Psychology Review | Experimental | -- |
Martin et al. [ ] | Contemporary Educational Psychology | Observational/ Correlational | No |
Park el al. [ ] | Educational Psychology Review | Experimental | -- |
Pengelley et al. [ ] | Educational Psychology Review | Experimental | -- |
Rau & Beier [ ] | Journal of Educational Psychology | Intervention | -- |
Sondermann & Merket [ ] | Contemporary Educational Psychology | Experimental | -- |
Wang et al. [ ] | Journal of Educational Psychology | Experimental | -- |
Yang et al. [ ] | Journal of Educational Psychology | Experimental | -- |
The statements, opinions and data contained in all publications are solely those of the individual author(s) and contributor(s) and not of MDPI and/or the editor(s). MDPI and/or the editor(s) disclaim responsibility for any injury to people or property resulting from any ideas, methods, instructions or products referred to in the content. |
Share and Cite
Martella, A.M.; Lawson, A.P.; Robinson, D.H. How Scientific Is Cognitive Load Theory Research Compared to the Rest of Educational Psychology? Educ. Sci. 2024 , 14 , 920. https://doi.org/10.3390/educsci14080920
Martella AM, Lawson AP, Robinson DH. How Scientific Is Cognitive Load Theory Research Compared to the Rest of Educational Psychology? Education Sciences . 2024; 14(8):920. https://doi.org/10.3390/educsci14080920
Martella, Amedee Marchand, Alyssa P. Lawson, and Daniel H. Robinson. 2024. "How Scientific Is Cognitive Load Theory Research Compared to the Rest of Educational Psychology?" Education Sciences 14, no. 8: 920. https://doi.org/10.3390/educsci14080920
Article Metrics
Article access statistics, further information, mdpi initiatives, follow mdpi.
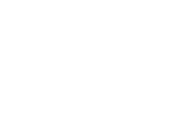
Subscribe to receive issue release notifications and newsletters from MDPI journals
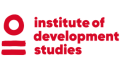
Determinants of nonperforming loans: empirical study in case of commercial banks in Ethiopia
Jimma university, ids item types, copyright holder, usage metrics.
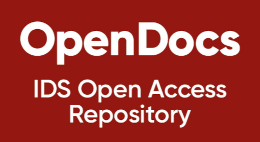

Advertisement
Understanding the Empirical Formula in Chemistry
- Share Content on Facebook
- Share Content on LinkedIn
- Share Content on Flipboard
- Share Content on Reddit
- Share Content via Email
In chemistry, there are a variety of methods that scientists use to identify a chemical compound, including a molecular formula, molar mass and molecular diagram. The simplest formula of these is known as the empirical formula .
In a textbook or other reference guide, empirical formulas tell us the chemical makeup of a certain compound, but they do not always tell us the exact number of atoms present in a molecule.
Clarity on a Microscopic Level
Empirical formula and molar mass, empirical formula vs. molecular formula, determining molecular formula using empirical formula.
The empirical formula was discovered by scientists in the mid-1800's, and for the first time, it allowed chemists to express what was going on with materials common and rare on a microscopic level.
These chemists would derive empirical formulas for specific chemical compounds in the lab by separating them into the individual elements that we can find on the periodic table and measuring the relative amounts present.
Example of a Chemical Compound
For instance, table salt (sodium chloride) can be divided into the pure elements present, which are sodium (NA) and chlorine (Cl) atoms. Individually these elements are highly unstable and tend to react with oxygen and water present in this atmosphere.
They're also poisonous if consumed on their own, but mixed together, they create a molecular compound which an essential part of our daily diet.
To arrive at the empirical formula for a compound like table salt , chemists would have to start a reaction separate it into its base atoms.
Assuming that 100 percent of the material had reacted, they could then weigh the molar mass of the sodium left over versus the molar mass of the chlorine to find the empirical makeup of the compound.
In the case of table salt , the mass of sodium to chloride is a mole ratio of 1:1, giving an empirical formula of NaCl, which also happens to be the correct chemical formula for the same compound.
Back in the 19th century, the methods used to split compounds into their atomic ingredients and find the resulting empirical formula were revolutionary in helping people understand what goes into creating chemicals and why they exhibit certain characteristics.
However, the empirical formula doesn't tell the full story about what's truly going on inside a molecule. More advanced chemical formulas had to be developed.
In labs today — as well as in the study of chemistry — you are much more likely to bump into the molecular formula for a compound rather than the empirical formula. The reason for this is that we now know that individual molecules tend to be more complex than the smallest whole number ratio of atoms within them.
Going by empirical formula alone, we would also end up with different compounds sharing the same formula, which isn't very useful when trying to categorize chemical properties. The total mass of a molecule could be much larger than its empirical formula would suggest.
Table salt is a very convenient compound to measure when it comes to empirical formula because it contains exactly one sodium and one chlorine atom per molecule. Not all compounds divide so perfectly, and knowing the simplest ratio of mass inside the molecule may not tell us exactly what is actually a single molecule.
What Molecular Formulas Tell Us About a Compound
Let's take two chemical compounds , acetylene and benzene, which share the same ratios and same empirical formula: CH, which is one hydrogen atom per one carbon atom. Compounds will share an empirical formula when the chemical formula of one is a multiple of the other. In this case the chemical formula for acetylene is C2H2 while benzene's is C6H6.
Although they have the same basic makeup, one molecule of benzyne has three times the number of atoms and three times the molar mass as an acetylene molecule. They are both classified as hydro-carbons but have different uses.
- Acetylene is used as a fuel for extremely high heat applications like welding.
- Benzene is considered too toxic when burned, and is instead used for industrial plastic production.
If chemists or industries were to assume that materials like acetylene and benzyne were actually the same compound based solely on their empirical formula, it could lead to flaws in the products they attempt to make, or even worse, it could lead to serious danger when a welder uses a highly toxic compound as fuel. Categorizing all these known chemicals correctly can literally save lives.
Chemists were first able to find the molecular formula of compounds using the empirical formula as a basis. The number of atoms which make up a molecular formula are always whole numbers which we multiply by the number ratio of the original empirical formula. The smallest number this factor can be is one.
To find the multiplication factor of a certain compound , scientists calculated the mole value, or average mass of an individual molecule. Going back to our examples of acetylene and benzene, laboratories were at some point able to calculate the mass values of those individual molecules, from which data they were able to obtain molecular formulas. Chemicals with entirely different atomic makeup may also share same factor.
Since we already know the molecular formula and the empirical formula for these compounds, we can work backwards in order to find the whole number by which our original formula is multiplied. The solutions look like this:
Please copy/paste the following text to properly cite this HowStuffWorks.com article:
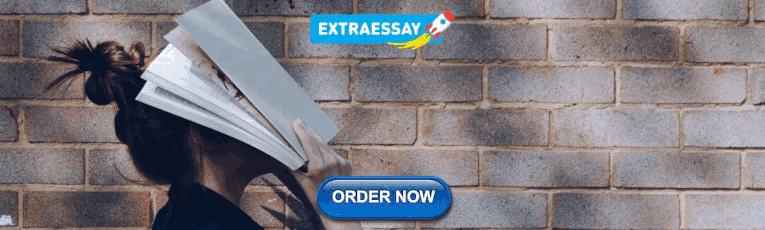
IMAGES
COMMENTS
Identifying Empirical Research Articles. Look for the IMRaD layout in the article to help identify empirical research.Sometimes the sections will be labeled differently, but the content will be similar. Introduction: why the article was written, research question or questions, hypothesis, literature review; Methods: the overall research design and implementation, description of sample ...
Empirical articles will include charts, graphs, or statistical analysis. Empirical research articles are usually substantial, maybe from 8-30 pages long. There is always a bibliography found at the end of the article. Type of publications that publish empirical studies: Empirical research articles are published in scholarly or academic journals.
Another hint: some scholarly journals use a specific layout, called the "IMRaD" format, to communicate empirical research findings. Such articles typically have 4 components: Introduction: sometimes called "literature review" -- what is currently known about the topic -- usually includes a theoretical framework and/or discussion of previous studies
Recently published articles from subdisciplines of psychology covered by more than 90 APA Journals™ publications. For additional free resources (such as article summaries, podcasts, and more), please visit the Highlights in Psychological Research page. Browse and read free articles from APA Journals across the field of psychology, selected by ...
Strategies for Empirical Research in Writing is a particularly accessible approach to both qualitative and quantitative empirical research methods, helping novices appreciate the value of empirical research in writing while easing their fears about the research process. This comprehensive book covers research methods ranging from traditional ...
An empirical research article is an article which reports research based on actual observations or experiments. The research may use quantitative research methods, which generate numerical data and seek to establish causal relationships between two or more variables. (1) Empirical research articles may use qualitative research methods, which ...
Once you know the characteristics of empirical research, the next question is how to find those characteristics when reading a scholarly, peer-reviewed journal article.Knowing the basic structure of an article will help you identify those characteristics quickly. The IMRaD Layout. Many scholarly, peer-reviewed journal articles, especially empirical articles, are structured according to the ...
According to the APA, empirical research is defined as the following: "Study based on facts, systematic observation, or experiment, rather than theory or general philosophical principle." Empirical research articles are generally located in scholarly, peer-reviewed journals and often follow a specific layout known as IMRaD:
Research problems and hypotheses are important means for attaining valuable knowledge. They are pointers or guides to such knowledge, or as formulated by Kerlinger ( 1986, p. 19): " … they direct investigation.". There are many kinds of problems and hypotheses, and they may play various roles in knowledge construction.
The term "empirical" entails gathered data based on experience, observations, or experimentation. In empirical research, knowledge is developed from factual experience as opposed to theoretical assumption and usually involved the use of data sources like datasets or fieldwork, but can also be based on observations within a laboratory setting.
We review empirical research on (social) psychology of morality to identify which issues and relations are well documented by existing data and which areas of inquiry are in need of further empirical evidence. An electronic literature search yielded a total of 1,278 relevant research articles published from 1940 through 2017.
This article provides recommendations for writing empirical journal articles that communicate research processes and products transparently with enough detail to allow replication and reproducibility. 1 Like Bem's chapter, this article also provides recommendations for writing empirical articles that are clear and memorable. Disclosures
Empirical research articles are usually substantial, maybe from 8-30 pages long. There is always a bibliography found at the end of the article. Type of publications that publish empirical studies: Empirical research articles are published in scholarly or academic journals
An empirical research article reports research based on actual observation or experiment. The research may use quantitative or qualitative research methods. Qualitative Research objectively and critically analyzes behaviors, beliefs, feelings, or other values ("People suffering from Illness A tend to be more cautious.")
The method for finding empirical research articles varies depending upon the database* being used. 1. The PsycARTICLES and PsycInfo databases (both from the APA) includes a Methodology filter that can be used to identify empirical studies. Look for the filter on the Advanced Search screen. To see a list and description of all of the of ...
The first is in data 9: modern databases include millions of research articles, grant proposals, patents and more. This windfall of data traces scientific activity in remarkable detail and at scale.
Empirical research methodologies can be described as quantitative, qualitative, or a mix of both (usually called mixed-methods). Ruane (2016) (UofM login required) gets at the basic differences in approach between quantitative and qualitative research: Quantitative research -- an approach to documenting reality that relies heavily on numbers both for the measurement of variables and for data ...
Characteristics of an Empirical Article: Empirical articles will include charts, graphs, or statistical analysis. Empirical research articles are usually substantial, maybe from 8-30 pages long. There is always a bibliography found at the end of the article. Type of publications that publish empirical studies: Empirical research articles are ...
Empirical research is defined as any research where conclusions of the study is strictly drawn from concretely empirical evidence, and therefore "verifiable" evidence. This empirical evidence can be gathered using quantitative market research and qualitative market research methods. For example: A research is being conducted to find out if ...
An empirical study reports the findings from a study that uses data derived from an actual experiment or observation. Key components of an empirical study: Abstract - Provides a brief overview of the research.; Introduction - The introduction contextualizes the research by providing a review of previous research on the topic.It also is the section where the hypothesis is stated.
Also, experimental/empirical articles are written in very formal, technical language (even the titles of the articles sound complicated!) and will usually contain numerical data presented in tables. Because experimental/empirical articles are written in technical language by researchers for other experts like themselves, the articles can be ...
An empirical article is a research article that reports the results of a study that uses data derived from actual observation or experimentation. There are several different sections of reports of empirical studies relating to the different steps of the scientific method.
Another hint: some scholarly journals use a specific layout, called the "IMRaD" format, to communicate empirical research findings. Such articles typically have 4 components: Introduction: sometimes called "literature review" -- what is currently known about the topic -- usually includes a theoretical framework and/or discussion of previous studies
In addition, all empirical literature had to have thematic relevance to the research question. Although scoping reviews can include grey literature, this review only included peer-reviewed articles to minimize bias and maintain credibility in this often divisive, emerging field of study (Munn et al., Citation 2022 ).
Research articles published by eLife are accompanied by statements that use prescribed phrases to evaluate importance and strength of support. This study uses an online repeated-measures experiment to gauge whether the eLife phrases were interpreted as intended, finding that most participants' implied ranking did not match the intended ranking.
Cognitive load theory (CLT) has driven numerous empirical studies for over 30 years and is a major theme in many of the most cited articles published between 1988 and 2023. However, CLT articles have not been compared to other educational psychology research in terms of the research designs used and the extent to which recommendations for practice are justified. As Brady and colleagues found ...
To understand the impacts of these increasingly popular bot moderators, we conduct an empirical study with data collected from 156 communities (subreddits) on Reddit. Based on a series of econometric analyses, we find that bots augment volunteer moderators by stimulating them to moderate a larger quantity of posts, and such effects are ...
As noted by Sharon (2007), loans have a vital contribution towards development of economy. However, its nonpayment also leads to incidence of huge loss on banks in particular and country in general. Hence, this study was conducted to examine both bank specific (loan to deposit ratio, capital adequacy ratio, return on asset and return on equity) and macroeconomic (lending rate, inflation and ...
In the case of table salt, the mass of sodium to chloride is a mole ratio of 1:1, giving an empirical formula of NaCl, which also happens to be the correct chemical formula for the same compound.. Back in the 19th century, the methods used to split compounds into their atomic ingredients and find the resulting empirical formula were revolutionary in helping people understand what goes into ...