Lesson 1: The Language and TerminologyIntroductionMost people think of statistics as the study of the numerical features of a subject/population. It means the same to statisticians, but also emphasizes the methods of collecting data, summarizing and presenting data, and drawing inferences from data. We all see on TV how political pundits justify opposing points of view by presenting statistics from respectable sources. How could something be a science when it justifies two opposing points of view? The answer is that statistics has a scientific basis but it can be misrepresented in use. Example . During the saga of President Clinton's impeachment, we observed the following: - One pundit says that, according to statistics, the majority of Americans think that character matters .
- The other pundit says, also according to statistics, that the majority of Americans think the president is doing a good job .
The implication here is that one of them was "wrong." But the science of statistics says that both were correct. Data was collected and analyzed, and it was found that the majority of Americans think that character matters and that the majority of Americans think the president is doing a good job . It does not matter to the science of statistics which one of the statistically established facts you or I want to believe. Another point about the nature of statistics as a science is that it is not a deterministic science . It does not have laws like force is equal to mass times acceleration . Statements in statistics come with a probability (i.e., quantified chance) of being correct . When a weatherman says that it will rain today he means that there is, say, a ninety five percent chance that it will rain today . Roughly, this means that if he makes the same prediction one hundred times he will be correct 95 times, and it will not rain the other 5 days. The problem is that sometimes a weatherman will hide the information that there is a 95 percent chance only . Such information hiding is sometimes done for simplicity. Before I conclude this introduction, let me tell you an interesting anecdote about the development of this subject. When the proposal to establish the Indian Statistical Institute in Calcutta was considered by the government of India in the early part of the last century, some critics said, then why not an institute in astrology? At the inception of statistics as a science there was a lot of skepticism about its scientific validity. Those days are gone, and statistics is not likened to astrology any more! Statistics is a well-founded and precise science. It is a nondeterministic science in nature; it makes precise probabilistic statements only. In this course we will be talking about two branches of statistics. The first one is called descriptive statistics and deals with methods of processing, summarizing, and presenting data. The other part deals with the scientific methods of drawing inferences and forecasting from the data, and is called inferential or inductive statistics . In the rest of this lesson and the next we deal with descriptive statistics, which includes the presentation of data in the form of tables, graphs, and computations of various averages of data. 1.1 Basic Definitions and ConceptsIn statistics we use a small representative "sample" to study a big "population." The reason for this is the cost or even the impossibility of studying the whole population. Population and SampleDefinitions . A complete collection of data on the group under study is called the population or the universe . A member of the population is called a sampling unit . Therefore, the population consists of all its sampling units. A S ample is a collection of sampling units selected from the population. Most often, we will work with numerical characteristics (like height, weight, and salary) of a group. So usually the population is a large collection of numbers and the sample is a small subset of the population . Example . Suppose we are studying the daily rainfall in Lawrence. Since daily rainfall could be from 0 inches to anything above 0, the population here is all nonnegative numbers (i.e., the interval [0, ∞ ) ). A sample from this population would be the observed amount of daily rainfall in Lawrence on some number of days. A sample of size 11 would be the observed daily rainfall in Lawrence on 11 days. Many definitions of variables are available in standard textbooks. For our purpose the following definition will suffice. Definition . A variable is a rule or a formula or a mechanism that associates a value with each member of the population. So, given a member w, a variable X assigns a value X (w) to w. For us X (w) will be a characteristic (like height, weight, time, salary) of the population. Example. Suppose we are studying the KU student population. The population is the whole collection of KU students. A KU student is a sample unit. If GPA is the "characteristic" that we are studying, then X = the GPA of a student is a variable. So, given a student, X has a value. For example: X(Donald Smith) = 3.25, | X(Sam Donaldson) = 3.11, | X(Karen Currie) = 3.89, | X(King Who) = 2.13 | On the other hand, if GENDER is the "characteristic" that we are studying, then Y = gender of a student is a variable. So, given a student, Y has a value. For example: Y(Donald Smith) = Male, | Y(Sam Donaldson) = Male, | Y(Karen Currie) = Female , | Y(King Who) = Male | If HEIGHT is the characteristic that we are studying, then Z = height of students is a variable. To give another example, if credit hours completed is the characteristic studied, T = the number of course credit hours completed so far by a student is a variable. Similarly, given any other characteristic like weight, annual income, annual expenditure, you can construct a variable for this population. A variable that takes numerical values is called a quantitative variable . So, the variables X, Z, and T above are quantitative variables, while Y is not. A variable that takes non-numerical values is called a qualitative variable . So, the variable Y above is a qualitative variable. We will mostly be concerned with quantitative variables . We discuss two types of quantitative variables: continuous and discrete variables. A quantitative variable that can assume any numerical value over an interval is called a continuous variable . Since Z above can (hypothetically) assume any value between 0 to 100 inches, Z is a continuous variable. T assumes only integer values and is therefore not a continuous variable. A different way to understand a discrete variable is that the possible values of the variable can be written down (or can be counted) in a (finite or infinite) list. We say that the values of a discrete variable are countable. A quantitative variable is called a discrete variable if its possible values consist of breaks between successive values. If a variable assumes only a finite number of values, then it is also called a finite variable. Otherwise the variable is called an infinite variable. A finite variable is definitely a discrete variable. The variable T above is a discrete variable. Examples of Continuous and Discrete Variables- The examples of continuous variables are weight, length, volume, area, and time.
- For this course, examples of discrete variables are always the number of something—number of typos, number of road accidents, number of phone calls.
Parameters and StatisticsDefinition 1. Given a set of data, any numerical value computed from the data using a formula or a rule is called a quantitative measure of the data. Definition 2. A quantitative measure of a population data is called a parameter . In other words, parameters belong to the whole population and are computed (if feasible) from the WHOLE population data. Examples: the average GPA of all KU students, the height of the tallest student in KU, the average income of the entire KU student population. One way to study a population is to know some of the parameters of the population. Unfortunately, computing such parameters could be expensive or even impossible. Essentially, parameters are unknown and the main game of statistics is to try to estimate parameters on the basis of small samples collected from the population . Definition 3. A quantitative measure of a sample data is called a statistic . So, any constant that we compute from a sample is a statistic. We use these statistics to estimate the parameters of the population. For example, the average height computed from a sample is a reasonable estimate for the (parameter) average height of the KU student population. Obviously, we do not expect the value of the statistic to be exactly equal to the parameter value. Hopefully, the error will be small or will exceed our tolerable limit very rarely (say once in a 100 trials) . Why do we need a statistic? Sometimes it will be impossible to know the actual value of a parameter. For example, let μ be the mean length of the life of light bulbs produced by a company. In this case, the company cannot test all the bulbs it produces to find a mean length. So, the best it can do is to test a few bulbs, compute the sample mean length (a statistic) of the life of these bulbs and use it as an estimate for the mean length (parameter μ ) of the life for all the bulbs it produces. Definition 4. The data that has not been processed or organized in any form is called raw data . When the data is arranged in an increasing or decreasing order, then it is called an array . The range of the data is the difference between the largest and the smallest value of the data. range = highest value - lowest value. 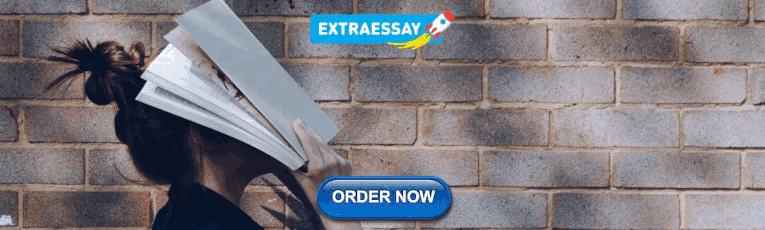 1.2 Frequency DistributionIn this section we talk about representation of data organized in tabular form. Such a representation is called a frequency distribution . We are mostly concerned with numerical data (i.e., quantititative data), but also consider some non-numerical data (i.e., qualitative data). Example. (from Khazanie, p. 18) The following is data on the blood group of 36 patients in a hospital: O | A | B | O | A | A | A | O | O | O | A | O | A | B | O | O | O | AB | B | A | A | O | O | A | A | O | AB | O | A | A | B | A | O | A | O | O | We have four types of blood groups, namely, O, A, B, AB. Each of these blood groups may be referred to as a " class ." The frequency of a class is defined as the number of data members that belong to that class. For example, the frequency of the class O is 16; the frequency of class A is 14. A table that lists the classes and the corresponding frequency is called the frequency distribution of this qualitative data. Following is the frequency distribution of this data: Blood Group | Frequency | O | 16 | A | 14 | B | 4 | AB | 2 | Total | 36 | Ungrouped DataFor the quantitative data, we consider two types of frequency table. When we are working with a large set of data we group that data into a few classes and construct a "frequency table," which we will discuss later. If the data set is small or if the number of values that appear in the data is small we need not group the data. Instead, we make a list of all the data members and give the corresponding frequency for each data member in a table. The number of times a data member (i.e., value) appears in the data is called the frequency of the data member. A list that presents the data members and the corresponding frequency in a tabular form is called a frequency table or frequency distribution . The relative frequency and percentage frequency of a data member x are defined as follows: relative frequency of x = | frequency of x | percentage frequency of x = | frequency of x | · 100. | The frequency table may also contain the relative and percentage frequency. Since we did not group the data into a few classes, we call this the frequency distribution of the ungrouped data . Example 1.2.1 To estimate the mean time taken to complete a three-mile drive by a race car, the race car did several time trials, and the following sample of times taken (in seconds) to complete the laps was collected: 50 | 48 | 49 | 46 | 54 | 53 | 52 | 51 | 47 | 56 | 52 | 51 | 51 | 53 | 50 | 49 | 48 | 54 | 53 | 51 | 52 | 54 | 54 | 53 | 55 | 48 | 51 | 50 | 52 | 49 | 51 | 53 | 55 | 54 | 50 | | Note that there are 35 observations here. So we say that the size of the sample (or data) is 35. Also the values present are 46, 47, 48, 49, 50, 51, 52, 53, 54, 55, 56. Since there are only 11 distinct values present we can make a frequency table for the ungrouped data. The following is the frequency distribution of this ungrouped data: Time (in seconds) | Frequency | Relative Frequency | Percentage Frequency | 46 | 1 | 1/35 | 2.86 | 47 | 1 | 1/35 | 2.86 | 48 | 3 | 3/35 | 8.57 | 49 | 3 | 3/35 | 8.57 | 50 | 4 | 4/35 | 11.43 | 51 | 6 | 6/35 | 17.14 | 52 | 4 | 4/35 | 11.43 | 53 | 5 | 5/35 | 14.29 | 54 | 5 | 5/35 | 14.29 | 55 | 2 | 2/35 | 5.71 | 56 | 1 | 1/35 | 2.86 | Total | 35 | 1 | 100 | Grouped DataWhen we are working with a large set of data that has too many distinct class member (i.e., values) then we group the whole set of data into a few class intervals and give the corresponding "frequency" of the class. When the data is presented in this way, the data is called grouped data . The number of data members that fall in a class interval is called the class frequency and the relative and percentage frequencies are computed by the same formula as above. A list that gives various class intervals and the corresponding class frequencies in a tabular form is called a class frequency table or class frequency distribution of the data. The frequency distribution may also include the relative and percentage frequencies. Grouped Data and Loss of Information Sometimes it is convenient or necessary to group data into class intervals and construct a class frequency distribution. This is the case when there are too many distinct numbers present in the data—too many even to fit into a simple table on a page for presentation. In such situations, we group the data in a few class intervals. While class frequency distribution is very good for presentation and convenient for other reasons, we lose a lot of information in this process. There is no way we can recover the original data from the class frequency distribution. Given a set of data, a good question would be, How many class intervals should we have? The answer is that it should not be too few nor should it be too many. If we take too few (say one), then all the information will be lost. On the other hand, if we take too many, we will have the problem of having to work with ungrouped data. (In this course we will always tell you how many classes to take.) Although sometimes it may be necessary to take class intervals of varying width, in this course we only consider classes of equal class width. Steps to Construct Frequency Distribution- Range : Pick a suitable number L less than or equal to the smallest value present in the data. Pick a suitable number H greater than or equal to the highest value present in the data. The range R that we consider is R = H - L.
- Number of Classes : Decide on a suitable number of classes. (In this course we will tell you the number of classes.)
[L,L+w],[L+w,L+2w],[L+2w, L+3w], ...,[H-w,H] Since this definition creates an ambiguous situation in which a data value may fall into two classes, we need a convention to address this situation. - Frequency : Find the frequency for each of the classes. You can use an advanced calculator or some software (like Excel) to count frequencies.
A few more important definitions. The above intervals are called class intervals . The w above is called the class size or width . The lower end of the class is called lower limit and the upper end of the class is called upper limit . The class mark is the midpoint of the class, defined as follows: class mark = | lower limit of class+ upper limit of class | . | A class limit is also called a class boundary . I took a slightly different approach when I defined the classes, so that for us class limits and class boundaries are the same. Although all the approaches are essentially the same, many slightly different approaches are possible depending on the situation. Example 1.2.2 The following is the weight (in ounces), at birth, of a certain number of babies. 74 | 105 | 124 | 110 | 119 | 137 | 96 | 110 | 120 | 115 | 140 | 65 | 135 | 123 | 129 | 72 | 121 | 117 | 96 | 107 | 80 | 91 | 74 | 123 | 124 | 124 | 134 | 78 | 138 | 106 | 130 | 97 | 145 | 93 | 133 | 128 | 96 | 126 | 124 | 125 | 127 | 62 | 127 | 92 | 95 | 118 | 126 | 94 | 127 | 121 | 117 | 124 | 93 | 135 | 156 | 143 | 125 | 120 | 147 | 138 | 72 | 119 | 89 | 81 | 113 | 91 | 133 | 127 | 138 | 122 | 110 | 113 | 100 | 115 | 110 | 135 | 141 | 97 | 127 | 120 | 110 | 107 | 111 | 126 | 132 | 120 | 108 | 148 | 143 | 103 | 92 | 124 | 150 | 86 | 121 | 98 | 74 | 85 | 99 | We will construct a class frequency table of this data by dividing the whole range of data into class intervals. Solution: Note that the lowest value is 62 and the highest value is 156. We take L = 60, H = 160, so R = H-W = 100. We made such a choice of L and H, precisely so that R = 100 is a "nice" number. Now we decide to have 5 class intervals and so w = R/5 = 20. According to what I said above, our classes should be : [60, 80], [80,100], [100,120], [120,140], [140, 160]. But if we do so then there is a risk that some data members (like 80, 100, 120, 140) will fall in two classes. One way to avoid this is to add .5 to all the class boundaries. So, our classes are [60.5, 80.5], [80.5, 100.5], [100.5, 120.5], [120.5, 140.5], [140.5, 160.5]. So the frequency distribution is as follows: Classes | Frequency | Relative Frequency | Percentage Frequency | 60.5 - 80.5 | 9 | 9/99 | 9.09 | 80.5 - 100.5 | 20 | 20/99 | 20.20 | 100.5 - 120.5 | 25 | 25/99 | 25.26 | 120.5 - 140.5 | 37 | 37/99 | 37.38 | 140.5 - 160.5 | 8 | 8/99 | 8.08 | Total | 99 | 1 | 100 | 1.3 Pictorial Representation of DataAnother way to represent data is to use pictures and graphs. We see such pictorial representation in newspapers and other sources every day. Pictorial representation is particularly important when you have to represent data to people with limited technical background, like newspaper readers or a governmental or congressional body. The Pie ChartThe pie chart is a commonly used pictorial representation of data. When you do your tax return every year, you find a few pie charts in the instruction book for form 1040. These charts show what proportion/percentage of each tax dollar goes for particular expenses. I reproduced the following pie charts from the 1040 instruction book of 1999. The HistogramAmong pictorial representations, the most useful in this course is the histogram. The histogram of data is the graphical representation of the frequency distribution of the data, where we plot the variable on the horizontal axis and above each class interval, we erect a bar of the height equal to the frequency of the class . Such a histogram is called a frequency histogram . If, instead, we erect bars of height equal to the relative frequency, then the graph is called a relative frequency histogram . Similarly, we can construct a percentage frequency histogram . Remark. Take a look at the Stem and Leaf Diagram discussed in any textbook. Example 1.3.1. Following is the frequency table of data on height (in inches) of some babies at birth. Sketch the histogram of the following data: Height | Frequency | 16-17 | 3 | 17-18 | 8 | 18-19 | 34 | 19-20 | 60 | 20-21 | 72 | 21-22 | 18 | The Cumulative Frequency DistributionsFor a given value x of a variable, the cumulative frequency of the data, for x, is the number of data members that are less than or equal to x. Definition . Given a frequency distribution of some data, for a class boundary x, the cumulative frequency is the sum of all the class frequenies less or equal to x. The cumulative frequency distribution is a table that gives the cumulative frequencies against some x values (for us the class boundaries). We also define cumulative relative frequency and cumulative percentage frequency as follows: cumulative relative frequency of x = | cumulative frequency of x | cumulative percentage frequency of x= | cumulative frequency | ×100 | Example 1.3.2 Once again we consider the data on birth weight of babies in Example 1.2 that we discussed in the last section. A cumulative frequency distribution can be constructed from the frequency distribution. Solution: We have seen the frequency distribution before. The following is the cumulative distributions: Weight | Cumulative Frequency | Relative-Cumulative Frequency | Cumulative Percentage Frequency | 60.5 | 0 | 0 | 0 | 80.5 | 9 | 9/99 | 9.09 | 100.5 | 29 | 29/100 | 29.29 | 120.5 | 54 | 54/99 | 54.55 | 140.5 | 91 | 91/99 | 91.92 | 160.5 | 99 | 1 | 100 | Use of CalculatorsBecause we will be using calculators (TI-83) extensively in this course, let me explain how you enter data in the TI-83. Use of Calculators (TI-83): | Enter Your Data: | It is not easy to construct a frequency table of a data set unless you are systematic. Traditionally, we used "tally marks" to count the frequency. Now you can use some software programs (e.g., Excel). Let me show you a method, using a calculator (TI-83). Exercise 1.2.1 To estimate the mean time taken to complete a three-mile drive by a race car, the race car did several time trials, and the following sample of times taken (in seconds) to complete the laps was collected: The following is the frequency distribution of this ungrouped data: Construct a histogram. Exercise 1.2.2. The following is the weight (in ounces), at birth, of 96 babies born in Lawrence Memorial Hospital in May 2000. 94 | 105 | 124 | 110 | 119 | 137 | 96 | 110 | 120 | 115 | 119 | 104 | 135 | 123 | 129 | 72 | 121 | 117 | 96 | 107 | 80 | 80 | 96 | 123 | 124 | 124 | 134 | 78 | 138 | 106 | 130 | 97 | 134 | 111 | 133 | 128 | 96 | 126 | 124 | 125 | 127 | 62 | 127 | 96 | 116 | 118 | 126 | 94 | 127 | 121 | 117 | 124 | 93 | 135 | 112 | 120 | 125 | 120 | 147 | 138 | 72 | 119 | 89 | 81 | 113 | 100 | 109 | 127 | 138 | 122 | 110 | 113 | 100 | 115 | 110 | 135 | 120 | 97 | 127 | 120 | 110 | 107 | 111 | 126 | 132 | 120 | 108 | 148 | 133 | 103 | 92 | 124 | 150 | 86 | 121 | 98 | | | | Construct a class frequency table of this data by dividing the the whole range of data into class intervals: [60.5-70.5], [70.5-80.5], [80.5-90.5], [90.5-100.5], [100.5-110.5], [110.5-120.5], [120.5-130.5], [130.5-140.5], [140.5-150.5] Exercise 1.2.3 . The following are the length (in inches), at birth, of 96 babies born in Lawrence Memorial Hospital in May 2000. 18 | 18.5 | 19 | 18.5 | 19 | 21 | 18 | 19 | 20 | 20.5 | 19 | 19 | 21.5 | 19.5 | 20 | 17 | 20 | 20 | 19 | 20.5 | 18 | 18.5 | 20 | 19.5 | 20.75 | 20 | 21 | 18 | 20.5 | 20 | 21 | 19 | 20.5 | 19 | 20 | 19.5 | 17.75 | 20 | 19.5 | 20 | 20.5 | 17 | 21 | 18.5 | 20 | 20 | 20 | 18.5 | 19.5 | 19 | 18 | 20.5 | 18 | 20 | 19 | 19 | 19.5 | 20 | 20.75 | 21 | 17.75 | 19 | 18 | 19 | 20 | 18.5 | 20 | 19 | 21 | 19 | 19.5 | 20 | 20 | 19 | 19.5 | 20 | 19.5 | 18.5 | 20.5 | 19.5 | 20.25 | 20 | 19.5 | 19.5 | 20 | 20 | 20 | 21 | 20 | 19 | 18.5 | 20.5 | 21.5 | 18 | 19.5 | 18 | | | | | Construct a frequency table for this data by dividing the whole range into class intervals: [16-17], [17-18], [18-19], [19-20], [20-21], [21-22]. Note: If a data member falls on the boundary, count it in the right/upper class-interval. Solution Exercise 1.2.4 . The following data represents the number of typos in a sample of 30 books published by some publisher. 156 | 159 | 162 | 160 | 156 | 162 | 159 | 160 | 156 | 156 | 160 | 162 | 156 | 159 | 162 | 156 | 162 | 158 | 160 | 158 | 159 | 162 | 158 | 158 | 162 | 160 | 159 | 162 | 162 | 160 | Construct a frequency table (by sorting in your calculator). Also construct a histogram. Solution Exercise 1.2.5 . Following is data on the hourly wages (paid only in whole dollars) in an industry. 9 | 11 | 8 | 9 | 10 | 11 | 7 | 10 | 12 | 13 | 7 | 11 | 8 | 11 | 14 | 9 | 10 | 9 | 11 | 7 | 13 | 13 | 14 | 12 | 9 | 8 | 12 | 14 | 15 | 9 | 9 | 7 | 12 | 7 | 12 | 7 | 7 | 11 | 13 | 9 | 11 | 9 | 9 | 9 | 10 | 14 | 11 | 12 | 14 | 7 | Exercise 1.2.6 . Following is data on the hourly wages (paid only in whole dollars) of 99 employees in an industry. 7 | 11 | 7 | 11 | 10 | 9 | 10 | 10 | 12 | 13 | 7 | 8 | 11 | 11 | 14 | 9 | 7 | 9 | 11 | 7 | 9 | 13 | 12 | 14 | 7 | 8 | 7 | 14 | 15 | 9 | 9 | 7 | 11 | 9 | 12 | 9 | 12 | 11 | 14 | 9 | 12 | 13 | 7 | 9 | 10 | 14 | 11 | 12 | 13 | 7 | 15 | 15 | 16 | 16 | 15 | 16 | 11 | 7 | 18 | 19 | 15 | 16 | 15 | 15 | 16 | 16 | 17 | 16 | 16 | 13 | 15 | 15 | 16 | 15 | 16 | 15 | 15 | 17 | 16 | 12 | 16 | 15 | 15 | 16 | 15 | 15 | 19 | 8 | 16 | 17 | 16 | 16 | 15 | 16 | 16 | 16 | 13 | 12 | 8 | | Construct a frequency table (by sorting in your calculator). After you have completed all the exercises for this lesson, you should begin your homework assignment. Click on the homework link at the top of this lesson. To access your homework you will be asked for your KUID and password. If you do not have a KUID use your Student Code that was provided by Independent Study in the welcome letter you received after you enrolled. Your password is your last name with only the first letter capitalized. back to top Pardon Our InterruptionAs you were browsing something about your browser made us think you were a bot. There are a few reasons this might happen: - You've disabled JavaScript in your web browser.
- You're a power user moving through this website with super-human speed.
- You've disabled cookies in your web browser.
- A third-party browser plugin, such as Ghostery or NoScript, is preventing JavaScript from running. Additional information is available in this support article .
To regain access, please make sure that cookies and JavaScript are enabled before reloading the page. 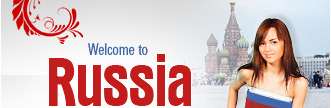 - Visit Our Blog about Russia to know more about Russian sights, history
- Check out our Russian cities and regions guides
- Follow us on Twitter and Facebook to better understand Russia
- Info about getting Russian visa , the main airports , how to rent an apartment
- Our Expert answers your questions about Russia, some tips about sending flowers
 Russian regions- Altay republic
- Irkutsk oblast
- Kemerovo oblast
- Khakassia republic
- Krasnoyarsk krai
- Novosibirsk oblast
- Omsk oblast
- Tomsk oblast
- Tuva republic
- Map of Russia
- All cities and regions
- Blog about Russia
- News from Russia
- How to get a visa
- Flights to Russia
- Russian hotels
- Renting apartments
- Russian currency
- FIFA World Cup 2018
- Submit an article
- Flowers to Russia
- Ask our Expert
Omsk Oblast, RussiaThe capital city of Omsk oblast: Omsk . Omsk Oblast - OverviewOmsk Oblast is a federal subject of Russia located in the south-eastern part of Siberia, in the Siberian Federal District. Omsk is the capital city of the region. The population of Omsk Oblast is about 1,879,500 (2022), the area - 141,140 sq. km. Omsk oblast flagOmsk oblast coat of arms. 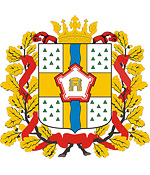 Omsk oblast map, RussiaOmsk oblast latest news and posts from our blog:. 10 November, 2019 / Tomsk - the view from above . 3 July, 2016 / Omsk - the view from above . 20 October, 2012 / The bear at the gate . 2 August, 2012 / Omsk city from bird's eye view . 14 December, 2011 / Time-lapse video of Omsk city . More posts.. History of Omsk OblastAncient people began to settle in the area of the middle reaches of the Irtysh River about 45,000 years ago. This region became the place of numerous migrations of different peoples, of interpenetration of forest and steppe cultures. In the Middle Ages, the territory of the present Omsk region was part of the Western Turkic Khanate and the Siberian Khanate. As a result, an ethnic group of the Siberian Tatars was formed. This region was also inhabited by Kazakhs and other peoples. The history of the development of the Irtysh by Russians is connected first of all with the legendary Yermak. Although even before him, in the 15th century, Russian merchants from the Urals visited the Siberian Khanate. In the early 18th century, major reforms carried out by Peter the Great required large expenses. The first Russian emperor turned his attention to the east. He sent a detachment of Cossacks under the command of the lieutenant-colonel I.D.Bukhgolts from the town of Tobolsk up the Irtysh River in search of gold deposits. More Historical Facts… The expedition failed because of resistance from the nomads Dzhungars. Russians were forced to take a step back. In 1716, they founded a fortress at the mouth of the Om River - future Omsk. Russian peasants began to settle in the land around the fortress. To the south of Omsk, a line of outposts was constructed for protection from the nomads. In 1782, the fortress became a town. Omsk district was formed on the basis of the southern part of Tarsky district and, in 1785, the town of Omsk was given a coat of arms. Omsk became an important center for the study of Siberia and Central Asia. This region like other parts of Siberia was used as a place for political exile. In the 19th century, the people exiled to Siberia were the Decembrists, Petrashevts, Narodniki, representatives of other revolutionary parties and organizations, participants of the Polish national movement. These people had a major cultural impact on the local population. The great Russian writer F.M.Dostoyevsky was one of the prisoners of the Omsk jail. In the late 19th and early 20th century, Siberia experienced significant changes. Large-scale migration of peasants led to the rapid growth of the local economy, especially agriculture. Due to its favorable economic and geographical location, at the intersection of the Trans-Siberian Railway and the Irtysh River, Omsk rapidly turned into a large transport, trade and industrial center of Western Siberia, the largest city in Siberia. During the Second World War, about 100 industrial plants were evacuated from the European part of the USSR to Omsk. They became the basis of the local engineering industry. In 1949, the first refinery in Siberia was constructed in Omsk. In 1954-1956, during development of virgin lands, several large agricultural enterprises were built in the southern part of Omsk Oblast. In the 1970s, Omsk oblast became one of the most economically developed regions of Siberia. Pictures of Omsk Oblast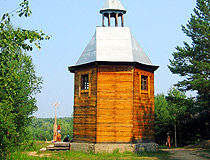 Wooden chapel in Omsk Oblast Author: Sedov Artem 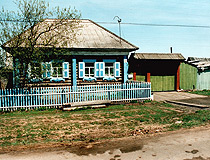 Country house in Omsk Oblast Author: Heinrich Jena 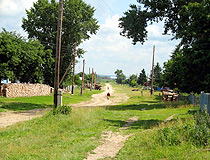 Provincial life in the Omsk region Author: Baranov Pavel Omsk Oblast - FeaturesOmsk Oblast is located in the south of the West Siberian Plain, in the middle reaches of the Irtysh River, with steppes in the south, which turn into forest steppes, forests and marshy tundra in the north. The territory of the region stretches for about 600 km from north to south and 300 km from west to east. In the south, Omsk Oblast borders with Kazakhstan. The largest cities and towns of Omsk Oblast are Omsk (1,126,000), Tara (28,500), Kalachinsk (21,900), Isylkul (21,700). The main river is the Irtysh with its tributaries (the Ishim, Om, Osha, and Tara). The Trans-Siberian Railway is an important traffic artery. There is an international airport in Omsk. The climate is continental and sharply continental. The average temperature in January is minus 19-20 degrees Celsius, in July - plus 17-18 degrees Celsius in the northern part and plus 19 degrees Celsius in the south. Omsk Oblast has such natural resources as oil, natural gas, brown coal, iron ore, various construction materials. Main manufacturing, construction and trade are carried out in Omsk. Industrial sector is represented by military, aerospace and agricultural engineering, petrochemical, light and food industries. Agriculture is represented by crops, dairy and beef cattle, pig and poultry farming. Cereals (wheat, rye, oats, barley), potatoes, vegetables, sunflower, and other crops are cultivated. Attractions of Omsk OblastA lot of sights can be found in Omsk. The most interesting places located outside the city are: - Achairsky Convent in the upper reaches of the Irtysh River, 50 km from Omsk;
- St. Nicholas Monastery in the village of Bolshekulache, 20 km from Omsk;
- Nature reserve “Bairovsky” created for the preservation and reproduction of rare and valuable species of birds and animals;
- Batakovo tract - a natural and archaeological park on the left bank of the Irtysh River, 150 km north of Omsk, in Bolsherechensky district;
- Znamenskiy museum of local lore dedicated to the history and nature of Omsk oblast, located in one of the oldest settlements of the region - in the village of Znamenskoye;
- Chudskaya mountain on the left bank of the Irtysh River, 3 km north of Znamenskoye;
- Lake Ulzhay - a relict water reservoir in the northwest of Kurumbelskaya steppe, in Cherlaksky district, 160 km from Omsk;
- Lake Ebeyty in the southwest of the region;
- Lake Platovskoye located to the north-east of the village of Platovo in Polstavskiy district;
- “Bird’s Haven” - a natural park located in Omsk;
- “Devil’s finger” - a rock on the right bank of the Irtysh, 2 km from the village of Serebryanoye, on the territory of Gorky district.
Omsk oblast of Russia photosNature of omsk oblast. 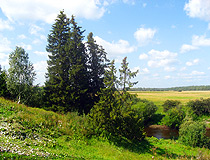 Omsk Oblast landscape Author: Vitali Ellert 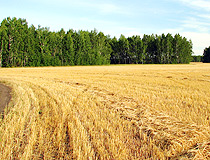 Omsk Oblast sceneryAuthor: Yury Ermakov 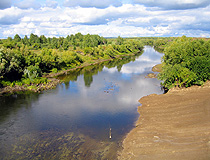 Small river in Omsk Oblast Author: Andrey Genze 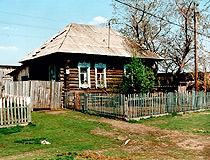 Wooden house in the Omsk region 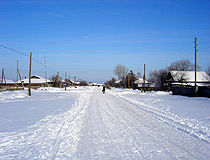 Winter in Omsk Oblast 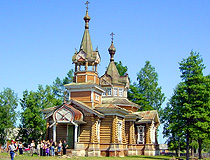 Wooden church in Omsk Oblast Rating: 2.9 /5 (140 votes cast) 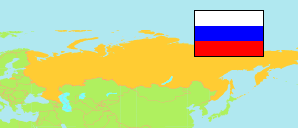 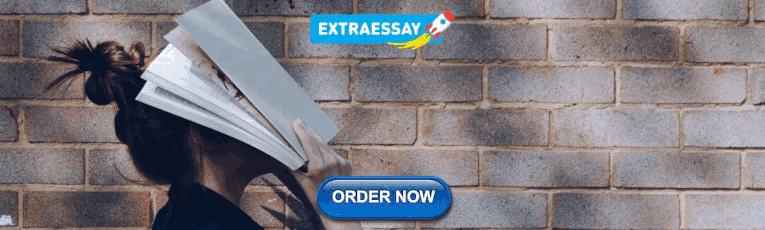 |
COMMENTS
Now, with expert-verified solutions from Elementary Statistics 14th Edition, you'll learn how to solve your toughest homework problems. Our resource for Elementary Statistics includes answers to chapter exercises, as well as detailed information to walk you through the process step by step. With Expert Solutions for thousands of practice ...
Elementary Statistics - 13th Edition - Solutions and Answers
Elementary Statistics, Chapter 1, Unit 1, Homework Problems. A newspaper posted this question on its website: "How often do you seek medical information online?" Of 1072 Internet users who chose to respond, 38% of them responded with "frequently." a) What term is used to describe this type of survey in which the people surveyed consist of those ...
Statistics and Probability
Understanding Elementary Statistics 13th Edition homework has never been easier than with Chegg Study. ... Unlike static PDF Elementary Statistics 13th Edition solution manuals or printed answer keys, our experts show you how to solve each problem step-by-step. No need to wait for office hours or assignments to be graded to find out where you ...
Class. Your Stat Class is the #1 FREE Statistics Course for Help Learning Elementary Statistics! Our Free Online Elementary Statistics Class Includes A Free Introductory Statistics Book, Lectures, Practice Problems, Tests, Videos, Help In Statistics And Tutoring. ENTER.
Textbook solutions for Elementary Statistics (13th Edition) 13th Edition Mario F. Triola and others in this series. View step-by-step homework solutions for your homework. Ask our subject experts for help answering any of your homework questions!
CH7.1. Problem. 1BSC. Step-by-step solution. Step 1 of 2. The correct answer is: Confidence level. The important feature of the poll was omitted is confidence level. That is (95%) Step 2 of 2.
Unlike static PDF Elementary Statistics 7th Edition solution manuals or printed answer keys, our experts show you how to solve each problem step-by-step. No need to wait for office hours or assignments to be graded to find out where you took a wrong turn. You can check your reasoning as you tackle a problem using our interactive solutions viewer.
Now, with expert-verified solutions from Elementary Statistics 11th Edition, you'll learn how to solve your toughest homework problems. Our resource for Elementary Statistics includes answers to chapter exercises, as well as detailed information to walk you through the process step by step. With Expert Solutions for thousands of practice ...
The central limit theorem is the most important result in elementary statistics. It will allow us to take any population - skewed, uniform, bi-modal, etc. and convert it to a problem with a normal distribution, provided the samples are random and the sample size is large enough. Once we have a normal distribution there are techniques we can ...
Elementary Statistics, 4th Edition
MATH 365, Elementary Statistics - Lesson One
Statistics document from Daytona State College, 3 pages, Elementary Statistics - Homework Assignments Homework Chapter 1 1. The population is/are the entire group of individuals or items being studied. 2. The sample is/are the subset of the population that is being studied. o Statistics are often used to genera
Access Elementary Statistics Plus MyLab Statistics with Pearson eText -- 18 Week Access Card Package 13th Edition Chapter 1.1 solutions now. Our solutions are written by Chegg experts so you can be assured of the highest quality!
Now, with expert-verified solutions from Elementary Statistics 12th Edition, you'll learn how to solve your toughest homework problems. Our resource for Elementary Statistics includes answers to chapter exercises, as well as detailed information to walk you through the process step by step. With Expert Solutions for thousands of practice ...
Omsk Oblast - Overview. Omsk Oblast is a federal subject of Russia located in the south-eastern part of Siberia, in the Siberian Federal District. Omsk is the capital city of the region. The population of Omsk Oblast is about 1,879,500 (2022), the area - 141,140 sq. km.
Main page; Contents; Current events; Random article; About Wikipedia; Contact us; Donate; Pages for logged out editors learn more
Omsk Oblast (Russian: О́мская о́бласть, romanized: Omskaya oblast') is a federal subject of Russia (an oblast), located in southwestern Siberia.The oblast has an area of 139,700 square kilometers (53,900 sq mi). Its population is 1,977,665 (2010 Census) [9] with the majority, 1.12 million, living in Omsk, the administrative center.One of the Omsk streets
Elementary Statistics, Chapter 2, Unit 1, Homework Problems
Contents: Cities and Settlements The population of all cities and urban settlements in Omsk Oblast according to census results and latest official estimates. The icon links to further information about a selected place including its population structure (gender).