| | , and , 2010, vol. 47, issue 3, 391-415 We investigate the multiple effects of writing a business plan prior to start‐up on new venture performance. We argue that the impact of business plans depends on the purpose for and circumstances in which they are being used. We offer an empirical methodology which can account for these multiple effects while disentangling real impact effects from selection effects. We apply this to English data where we find that business plans promote employment growth. This is found to be due to the impact of the plan and not selection effects. 2010 (24) (external link) This item may be available elsewhere in EconPapers: for items with the same title. BibTeX RIS (EndNote, ProCite, RefMan) HTML/Text This journal article can be ordered from for this article Journal of Management Studies is currently edited by , and in Journal of Management Studies from Bibliographic data for series maintained by Wiley-Blackwell Digital Licensing ( ) and Christopher F. Baum ( ). | | - Business Management
- Business Administration
- Business Plans
Multiple Effects of Business Plans on New Ventures- This person is not on ResearchGate, or hasn't claimed this research yet.
 Discover the world's research - 25+ million members
- 160+ million publication pages
- 2.3+ billion citations
No full-text available To read the full-text of this research, you can request a copy directly from the authors.  - Eric Pardede
- Rosli Mohd Saad
- Abdullah Abdul Ghani
 - Mike Wright
- Shiri Breznitz
- Vangelis Souitaris
- J TECHNOL TRANSFER
     - STRATEGIC MANAGE J
- Richard B. Robinson
   - Thomas M. Box
- Larry R. Watts
       - Catherine Armington
- SMALL BUS ECON
- Jianwen Liao
 - Garry D. Bruton
- Yuri Rubanik
- OXFORD B ECON STAT
- Andrew Burke
- Felix R. FitzRoy
 - Christopher L Gilbert
- Recruit researchers
- Join for free
- Login Email Tip: Most researchers use their institutional email address as their ResearchGate login Password Forgot password? Keep me logged in Log in or Continue with Google Welcome back! Please log in. Email · Hint Tip: Most researchers use their institutional email address as their ResearchGate login Password Forgot password? Keep me logged in Log in or Continue with Google No account? Sign up
Multiple Effects of Business Plans on New Ventures- Andrew Burke , Stuart A. Fraser , F. Greene
- Published 5 March 2009
- Business, Economics
- Entrepreneurship & Management eJournal
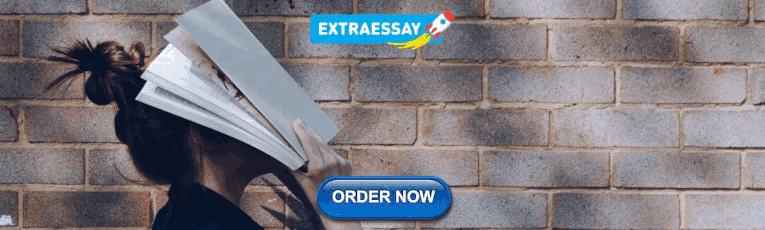 8 CitationsAssessing the effectiveness of guided preparation for new venture creation and performance: theory and practice, modeling and documentation of business plan by optimization of the administration's function, process and behaviour, evaluating the effect of a pilot drought policy measure on farmer practices and behaviour, an emerging ecosystem for student start-ups, effectuation : a study of concept ’ s use, perancangan perniagaan dan prestasi perniagaan: kajian ke atas pks islam di malaysia, from business model to business plan, 93 references, institutional forces and the written business plan. Does business planning facilitate the development of new venturesWhat makes a new business start-up successful, fast-growing businesses, the effects of pre-venture plan timing and perceived environmental uncertainty on the persistence of emerging firms, milestones for successful venture planning, understanding the small business sector, strategic planning and financial performance: a meta‐analytic review*, are all the potential entrepreneurs so good, the relationship between written business plans and the failure of small businesses in the u.s., related papers. Showing 1 through 3 of 0 Related Papers 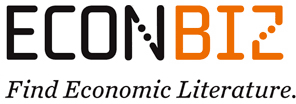 - The multiple effects of busine...
- More details
 The multiple effects of business planning on new venture performance Year of publication: | | Authors: | ; ; | Published in: | . - Oxford : Blackwell, ISSN 0022-2380, ZDB-ID 242497-6. - Vol. 47.2010, 3, p. 391-415 | Subject: | | | | | | | | | | Extent: | |
---|
Type of publication: | Article |
---|
Type of publication (narrower categories): | Aufsatz in Zeitschrift ; Article in journal |
---|
Language: | English |
---|
Source: | | - EndNote - Citavi, Endnote, RefWorks, ...
- Zotero, Mendeley, RefWorks, ...
When do novel business models lead to high performance? : a configurational approach to value drivers, competitive strategy, and firm environment Leppänen, Petteri, (2023) Multiple Effects of Business Plans on New Ventures Burke, Andrew E., (2009) How they started : how 30 good ideas became great businesses Lester, David, (2007) The Multiple Effects of Business Planning on New Venture Performance Burke, Andrew, (2010) Sources of bias in the recall of self-generated data: The role of anchoring Fraser, Stuart, (2007) The effects of experience on entrepreneurial optimism and uncertainty Fraser, Stuart, (2006) The Multiple Effects of Business Planning on New Venture PerformanceAbstract: we investigate the multiple effects of writing a business plan prior to start-up on new venture performance. we argue that the impact of business plans depends on the purpose for and circumstances in which they are being used. we offer an empirical methodology which can account for these multiple effects while disentangling real impact effects from selection effects. we apply this to english data where we find that business plans promote employment growth. this is found to be due to the impact of the plan and … show more. Search citation statements Paper Sections Citation Types Year Published Publication Types Relationship Cited by 109 publication sReferences 73 publication s, the impact of habitual entrepreneurial experience on new firm closure outcomes. In this paper, we argue that it is difficult for habitual entrepreneurs to use their experiential knowledge to develop a more viable new firm than novice entrepreneurs. Hindered by the difficulty of disentangling how actions lead to outcomes in low predictive environments such as new firm settings; hampered by the novelty and uncertainty of new firm closure; and misguided by subjective beliefs about their ability, we contend that habitual entrepreneurs close their new firm just as quickly as novice entrepreneurs and are just as likely to go bankrupt. Using large-scale panel data that track new firm closure amongst 7400 new German firms, we find that the new firms run by habitual entrepreneurs close just as quickly as those run by novice entrepreneurs. We also find that habituals are just as likely as novices to see their new business go bankrupt. Are More Frequent Releases Always Better? Dynamics of Pivoting, Scaling, and the Minimum Viable ProductUsing the system dynamics methodology, we model the minimum viable product (MVP) Gender and the use of external business advice: a Swedish studyIf you would like to write for this, or any other Emerald publication, then please use our Emerald for Authors service information about how to choose which publication to write for and submission guidelines are available for all. Please visit www.emeraldinsight.com/authors for more information. About Emerald www.emeraldinsight.comEmerald is a global publisher linking research and practice to the benefit of society. The company manages a portfolio of more than 290 journals and over 2,350 books and book series volumes, as well as providing an extensive range of online products and additional customer resources and services.Emerald is both COUNTER 4 and TRANSFER compliant. The organization is a partner of the Committee on Publication Ethics (COPE) and also works with Portico and the LOCKSS initiative for digital archive preservation. *Related content and download information correct at time of download. scite is a Brooklyn-based organization that helps researchers better discover and understand research articles through Smart Citations–citations that display the context of the citation and describe whether the article provides supporting or contrasting evidence. scite is used by students and researchers from around the world and is funded in part by the National Science Foundation and the National Institute on Drug Abuse of the National Institutes of Health. Contact Info[email protected] 10624 S. Eastern Ave., Ste. A-614 Henderson, NV 89052, USA Blog Terms and Conditions API Terms Privacy Policy Contact Cookie Preferences Do Not Sell or Share My Personal Information Copyright © 2024 scite LLC. All rights reserved. Made with 💙 for researchers Part of the Research Solutions Family. - Search Menu
- Sign in through your institution
- Browse content in A - General Economics and Teaching
- Browse content in A1 - General Economics
- A11 - Role of Economics; Role of Economists; Market for Economists
- A13 - Relation of Economics to Social Values
- A14 - Sociology of Economics
- Browse content in C - Mathematical and Quantitative Methods
- Browse content in C0 - General
- C02 - Mathematical Methods
- Browse content in C1 - Econometric and Statistical Methods and Methodology: General
- C10 - General
- C11 - Bayesian Analysis: General
- C12 - Hypothesis Testing: General
- C13 - Estimation: General
- C14 - Semiparametric and Nonparametric Methods: General
- C15 - Statistical Simulation Methods: General
- C18 - Methodological Issues: General
- Browse content in C2 - Single Equation Models; Single Variables
- C21 - Cross-Sectional Models; Spatial Models; Treatment Effect Models; Quantile Regressions
- C22 - Time-Series Models; Dynamic Quantile Regressions; Dynamic Treatment Effect Models; Diffusion Processes
- C23 - Panel Data Models; Spatio-temporal Models
- Browse content in C3 - Multiple or Simultaneous Equation Models; Multiple Variables
- C32 - Time-Series Models; Dynamic Quantile Regressions; Dynamic Treatment Effect Models; Diffusion Processes; State Space Models
- C38 - Classification Methods; Cluster Analysis; Principal Components; Factor Models
- Browse content in C4 - Econometric and Statistical Methods: Special Topics
- C45 - Neural Networks and Related Topics
- Browse content in C5 - Econometric Modeling
- C50 - General
- C51 - Model Construction and Estimation
- C52 - Model Evaluation, Validation, and Selection
- C53 - Forecasting and Prediction Methods; Simulation Methods
- C55 - Large Data Sets: Modeling and Analysis
- C58 - Financial Econometrics
- Browse content in C6 - Mathematical Methods; Programming Models; Mathematical and Simulation Modeling
- C61 - Optimization Techniques; Programming Models; Dynamic Analysis
- C62 - Existence and Stability Conditions of Equilibrium
- C65 - Miscellaneous Mathematical Tools
- Browse content in C7 - Game Theory and Bargaining Theory
- C70 - General
- C72 - Noncooperative Games
- C73 - Stochastic and Dynamic Games; Evolutionary Games; Repeated Games
- C78 - Bargaining Theory; Matching Theory
- Browse content in C8 - Data Collection and Data Estimation Methodology; Computer Programs
- C81 - Methodology for Collecting, Estimating, and Organizing Microeconomic Data; Data Access
- Browse content in C9 - Design of Experiments
- C91 - Laboratory, Individual Behavior
- C92 - Laboratory, Group Behavior
- C93 - Field Experiments
- Browse content in D - Microeconomics
- Browse content in D0 - General
- D03 - Behavioral Microeconomics: Underlying Principles
- Browse content in D1 - Household Behavior and Family Economics
- D10 - General
- D11 - Consumer Economics: Theory
- D12 - Consumer Economics: Empirical Analysis
- D14 - Household Saving; Personal Finance
- D15 - Intertemporal Household Choice: Life Cycle Models and Saving
- D18 - Consumer Protection
- Browse content in D2 - Production and Organizations
- D20 - General
- D21 - Firm Behavior: Theory
- D22 - Firm Behavior: Empirical Analysis
- D23 - Organizational Behavior; Transaction Costs; Property Rights
- D24 - Production; Cost; Capital; Capital, Total Factor, and Multifactor Productivity; Capacity
- D25 - Intertemporal Firm Choice: Investment, Capacity, and Financing
- Browse content in D3 - Distribution
- D30 - General
- D31 - Personal Income, Wealth, and Their Distributions
- Browse content in D4 - Market Structure, Pricing, and Design
- D40 - General
- D43 - Oligopoly and Other Forms of Market Imperfection
- D44 - Auctions
- D47 - Market Design
- D49 - Other
- Browse content in D5 - General Equilibrium and Disequilibrium
- D50 - General
- D51 - Exchange and Production Economies
- D52 - Incomplete Markets
- D53 - Financial Markets
- Browse content in D6 - Welfare Economics
- D60 - General
- D61 - Allocative Efficiency; Cost-Benefit Analysis
- D62 - Externalities
- Browse content in D7 - Analysis of Collective Decision-Making
- D70 - General
- D71 - Social Choice; Clubs; Committees; Associations
- D72 - Political Processes: Rent-seeking, Lobbying, Elections, Legislatures, and Voting Behavior
- D73 - Bureaucracy; Administrative Processes in Public Organizations; Corruption
- D74 - Conflict; Conflict Resolution; Alliances; Revolutions
- D78 - Positive Analysis of Policy Formulation and Implementation
- Browse content in D8 - Information, Knowledge, and Uncertainty
- D80 - General
- D81 - Criteria for Decision-Making under Risk and Uncertainty
- D82 - Asymmetric and Private Information; Mechanism Design
- D83 - Search; Learning; Information and Knowledge; Communication; Belief; Unawareness
- D84 - Expectations; Speculations
- D85 - Network Formation and Analysis: Theory
- D86 - Economics of Contract: Theory
- D87 - Neuroeconomics
- Browse content in D9 - Micro-Based Behavioral Economics
- D90 - General
- D91 - Role and Effects of Psychological, Emotional, Social, and Cognitive Factors on Decision Making
- D92 - Intertemporal Firm Choice, Investment, Capacity, and Financing
- Browse content in E - Macroeconomics and Monetary Economics
- Browse content in E0 - General
- E00 - General
- E03 - Behavioral Macroeconomics
- Browse content in E1 - General Aggregative Models
- E17 - Forecasting and Simulation: Models and Applications
- Browse content in E2 - Consumption, Saving, Production, Investment, Labor Markets, and Informal Economy
- E20 - General
- E21 - Consumption; Saving; Wealth
- E22 - Investment; Capital; Intangible Capital; Capacity
- E23 - Production
- E24 - Employment; Unemployment; Wages; Intergenerational Income Distribution; Aggregate Human Capital; Aggregate Labor Productivity
- Browse content in E3 - Prices, Business Fluctuations, and Cycles
- E30 - General
- E31 - Price Level; Inflation; Deflation
- E32 - Business Fluctuations; Cycles
- E37 - Forecasting and Simulation: Models and Applications
- Browse content in E4 - Money and Interest Rates
- E40 - General
- E41 - Demand for Money
- E42 - Monetary Systems; Standards; Regimes; Government and the Monetary System; Payment Systems
- E43 - Interest Rates: Determination, Term Structure, and Effects
- E44 - Financial Markets and the Macroeconomy
- E47 - Forecasting and Simulation: Models and Applications
- Browse content in E5 - Monetary Policy, Central Banking, and the Supply of Money and Credit
- E50 - General
- E51 - Money Supply; Credit; Money Multipliers
- E52 - Monetary Policy
- E58 - Central Banks and Their Policies
- Browse content in E6 - Macroeconomic Policy, Macroeconomic Aspects of Public Finance, and General Outlook
- E60 - General
- E61 - Policy Objectives; Policy Designs and Consistency; Policy Coordination
- E62 - Fiscal Policy
- E63 - Comparative or Joint Analysis of Fiscal and Monetary Policy; Stabilization; Treasury Policy
- E64 - Incomes Policy; Price Policy
- E65 - Studies of Particular Policy Episodes
- E66 - General Outlook and Conditions
- Browse content in E7 - Macro-Based Behavioral Economics
- E71 - Role and Effects of Psychological, Emotional, Social, and Cognitive Factors on the Macro Economy
- Browse content in F - International Economics
- Browse content in F0 - General
- F02 - International Economic Order and Integration
- Browse content in F1 - Trade
- F14 - Empirical Studies of Trade
- Browse content in F2 - International Factor Movements and International Business
- F21 - International Investment; Long-Term Capital Movements
- F22 - International Migration
- F23 - Multinational Firms; International Business
- Browse content in F3 - International Finance
- F30 - General
- F31 - Foreign Exchange
- F32 - Current Account Adjustment; Short-Term Capital Movements
- F33 - International Monetary Arrangements and Institutions
- F34 - International Lending and Debt Problems
- F36 - Financial Aspects of Economic Integration
- F37 - International Finance Forecasting and Simulation: Models and Applications
- F38 - International Financial Policy: Financial Transactions Tax; Capital Controls
- Browse content in F4 - Macroeconomic Aspects of International Trade and Finance
- F40 - General
- F41 - Open Economy Macroeconomics
- F42 - International Policy Coordination and Transmission
- F43 - Economic Growth of Open Economies
- F44 - International Business Cycles
- F47 - Forecasting and Simulation: Models and Applications
- Browse content in F5 - International Relations, National Security, and International Political Economy
- F51 - International Conflicts; Negotiations; Sanctions
- Browse content in F6 - Economic Impacts of Globalization
- F63 - Economic Development
- F65 - Finance
- Browse content in G - Financial Economics
- Browse content in G0 - General
- G00 - General
- G01 - Financial Crises
- G02 - Behavioral Finance: Underlying Principles
- Browse content in G1 - General Financial Markets
- G10 - General
- G11 - Portfolio Choice; Investment Decisions
- G12 - Asset Pricing; Trading volume; Bond Interest Rates
- G13 - Contingent Pricing; Futures Pricing
- G14 - Information and Market Efficiency; Event Studies; Insider Trading
- G15 - International Financial Markets
- G17 - Financial Forecasting and Simulation
- G18 - Government Policy and Regulation
- G19 - Other
- Browse content in G2 - Financial Institutions and Services
- G20 - General
- G21 - Banks; Depository Institutions; Micro Finance Institutions; Mortgages
- G22 - Insurance; Insurance Companies; Actuarial Studies
- G23 - Non-bank Financial Institutions; Financial Instruments; Institutional Investors
- G24 - Investment Banking; Venture Capital; Brokerage; Ratings and Ratings Agencies
- G28 - Government Policy and Regulation
- G29 - Other
- Browse content in G3 - Corporate Finance and Governance
- G30 - General
- G31 - Capital Budgeting; Fixed Investment and Inventory Studies; Capacity
- G32 - Financing Policy; Financial Risk and Risk Management; Capital and Ownership Structure; Value of Firms; Goodwill
- G33 - Bankruptcy; Liquidation
- G34 - Mergers; Acquisitions; Restructuring; Corporate Governance
- G35 - Payout Policy
- G38 - Government Policy and Regulation
- G39 - Other
- Browse content in G4 - Behavioral Finance
- G40 - General
- G41 - Role and Effects of Psychological, Emotional, Social, and Cognitive Factors on Decision Making in Financial Markets
- Browse content in G5 - Household Finance
- G50 - General
- G51 - Household Saving, Borrowing, Debt, and Wealth
- G52 - Insurance
- G53 - Financial Literacy
- Browse content in H - Public Economics
- H0 - General
- Browse content in H1 - Structure and Scope of Government
- H11 - Structure, Scope, and Performance of Government
- H19 - Other
- Browse content in H2 - Taxation, Subsidies, and Revenue
- H22 - Incidence
- H24 - Personal Income and Other Nonbusiness Taxes and Subsidies; includes inheritance and gift taxes
- H25 - Business Taxes and Subsidies
- H26 - Tax Evasion and Avoidance
- Browse content in H3 - Fiscal Policies and Behavior of Economic Agents
- H31 - Household
- Browse content in H4 - Publicly Provided Goods
- H40 - General
- H41 - Public Goods
- Browse content in H5 - National Government Expenditures and Related Policies
- H50 - General
- H52 - Government Expenditures and Education
- H53 - Government Expenditures and Welfare Programs
- H54 - Infrastructures; Other Public Investment and Capital Stock
- H55 - Social Security and Public Pensions
- H56 - National Security and War
- H57 - Procurement
- Browse content in H6 - National Budget, Deficit, and Debt
- H63 - Debt; Debt Management; Sovereign Debt
- Browse content in H7 - State and Local Government; Intergovernmental Relations
- H70 - General
- H72 - State and Local Budget and Expenditures
- H74 - State and Local Borrowing
- H75 - State and Local Government: Health; Education; Welfare; Public Pensions
- Browse content in H8 - Miscellaneous Issues
- H81 - Governmental Loans; Loan Guarantees; Credits; Grants; Bailouts
- Browse content in I - Health, Education, and Welfare
- Browse content in I1 - Health
- I11 - Analysis of Health Care Markets
- I12 - Health Behavior
- I13 - Health Insurance, Public and Private
- I14 - Health and Inequality
- I18 - Government Policy; Regulation; Public Health
- Browse content in I2 - Education and Research Institutions
- I22 - Educational Finance; Financial Aid
- I23 - Higher Education; Research Institutions
- I28 - Government Policy
- Browse content in I3 - Welfare, Well-Being, and Poverty
- I30 - General
- I38 - Government Policy; Provision and Effects of Welfare Programs
- Browse content in J - Labor and Demographic Economics
- Browse content in J0 - General
- J00 - General
- Browse content in J1 - Demographic Economics
- J11 - Demographic Trends, Macroeconomic Effects, and Forecasts
- J12 - Marriage; Marital Dissolution; Family Structure; Domestic Abuse
- J13 - Fertility; Family Planning; Child Care; Children; Youth
- J15 - Economics of Minorities, Races, Indigenous Peoples, and Immigrants; Non-labor Discrimination
- J16 - Economics of Gender; Non-labor Discrimination
- J18 - Public Policy
- Browse content in J2 - Demand and Supply of Labor
- J20 - General
- J21 - Labor Force and Employment, Size, and Structure
- J22 - Time Allocation and Labor Supply
- J23 - Labor Demand
- J24 - Human Capital; Skills; Occupational Choice; Labor Productivity
- J26 - Retirement; Retirement Policies
- J28 - Safety; Job Satisfaction; Related Public Policy
- Browse content in J3 - Wages, Compensation, and Labor Costs
- J30 - General
- J31 - Wage Level and Structure; Wage Differentials
- J32 - Nonwage Labor Costs and Benefits; Retirement Plans; Private Pensions
- J33 - Compensation Packages; Payment Methods
- J38 - Public Policy
- Browse content in J4 - Particular Labor Markets
- J41 - Labor Contracts
- J44 - Professional Labor Markets; Occupational Licensing
- J45 - Public Sector Labor Markets
- J46 - Informal Labor Markets
- J49 - Other
- Browse content in J5 - Labor-Management Relations, Trade Unions, and Collective Bargaining
- J51 - Trade Unions: Objectives, Structure, and Effects
- J52 - Dispute Resolution: Strikes, Arbitration, and Mediation; Collective Bargaining
- Browse content in J6 - Mobility, Unemployment, Vacancies, and Immigrant Workers
- J61 - Geographic Labor Mobility; Immigrant Workers
- J62 - Job, Occupational, and Intergenerational Mobility
- J63 - Turnover; Vacancies; Layoffs
- J64 - Unemployment: Models, Duration, Incidence, and Job Search
- J65 - Unemployment Insurance; Severance Pay; Plant Closings
- J68 - Public Policy
- Browse content in J7 - Labor Discrimination
- J71 - Discrimination
- Browse content in J8 - Labor Standards: National and International
- J88 - Public Policy
- Browse content in K - Law and Economics
- Browse content in K1 - Basic Areas of Law
- K12 - Contract Law
- Browse content in K2 - Regulation and Business Law
- K22 - Business and Securities Law
- K23 - Regulated Industries and Administrative Law
- Browse content in K3 - Other Substantive Areas of Law
- K31 - Labor Law
- K32 - Environmental, Health, and Safety Law
- K34 - Tax Law
- K35 - Personal Bankruptcy Law
- Browse content in K4 - Legal Procedure, the Legal System, and Illegal Behavior
- K42 - Illegal Behavior and the Enforcement of Law
- Browse content in L - Industrial Organization
- Browse content in L1 - Market Structure, Firm Strategy, and Market Performance
- L10 - General
- L11 - Production, Pricing, and Market Structure; Size Distribution of Firms
- L13 - Oligopoly and Other Imperfect Markets
- L14 - Transactional Relationships; Contracts and Reputation; Networks
- L15 - Information and Product Quality; Standardization and Compatibility
- Browse content in L2 - Firm Objectives, Organization, and Behavior
- L21 - Business Objectives of the Firm
- L22 - Firm Organization and Market Structure
- L23 - Organization of Production
- L24 - Contracting Out; Joint Ventures; Technology Licensing
- L25 - Firm Performance: Size, Diversification, and Scope
- L26 - Entrepreneurship
- L29 - Other
- Browse content in L3 - Nonprofit Organizations and Public Enterprise
- L33 - Comparison of Public and Private Enterprises and Nonprofit Institutions; Privatization; Contracting Out
- Browse content in L4 - Antitrust Issues and Policies
- L43 - Legal Monopolies and Regulation or Deregulation
- L44 - Antitrust Policy and Public Enterprises, Nonprofit Institutions, and Professional Organizations
- Browse content in L5 - Regulation and Industrial Policy
- L51 - Economics of Regulation
- Browse content in L6 - Industry Studies: Manufacturing
- L66 - Food; Beverages; Cosmetics; Tobacco; Wine and Spirits
- Browse content in L8 - Industry Studies: Services
- L81 - Retail and Wholesale Trade; e-Commerce
- L85 - Real Estate Services
- L86 - Information and Internet Services; Computer Software
- Browse content in L9 - Industry Studies: Transportation and Utilities
- L92 - Railroads and Other Surface Transportation
- L94 - Electric Utilities
- Browse content in M - Business Administration and Business Economics; Marketing; Accounting; Personnel Economics
- Browse content in M0 - General
- M00 - General
- Browse content in M1 - Business Administration
- M12 - Personnel Management; Executives; Executive Compensation
- M13 - New Firms; Startups
- M14 - Corporate Culture; Social Responsibility
- M16 - International Business Administration
- Browse content in M2 - Business Economics
- M20 - General
- M21 - Business Economics
- Browse content in M3 - Marketing and Advertising
- M30 - General
- M31 - Marketing
- M37 - Advertising
- Browse content in M4 - Accounting and Auditing
- M40 - General
- M41 - Accounting
- M42 - Auditing
- M48 - Government Policy and Regulation
- Browse content in M5 - Personnel Economics
- M51 - Firm Employment Decisions; Promotions
- M52 - Compensation and Compensation Methods and Their Effects
- M54 - Labor Management
- Browse content in N - Economic History
- Browse content in N1 - Macroeconomics and Monetary Economics; Industrial Structure; Growth; Fluctuations
- N10 - General, International, or Comparative
- N12 - U.S.; Canada: 1913-
- Browse content in N2 - Financial Markets and Institutions
- N20 - General, International, or Comparative
- N21 - U.S.; Canada: Pre-1913
- N22 - U.S.; Canada: 1913-
- N23 - Europe: Pre-1913
- N24 - Europe: 1913-
- N25 - Asia including Middle East
- N27 - Africa; Oceania
- Browse content in N3 - Labor and Consumers, Demography, Education, Health, Welfare, Income, Wealth, Religion, and Philanthropy
- N32 - U.S.; Canada: 1913-
- Browse content in N4 - Government, War, Law, International Relations, and Regulation
- N43 - Europe: Pre-1913
- Browse content in N7 - Transport, Trade, Energy, Technology, and Other Services
- N71 - U.S.; Canada: Pre-1913
- Browse content in N8 - Micro-Business History
- N80 - General, International, or Comparative
- N82 - U.S.; Canada: 1913-
- Browse content in O - Economic Development, Innovation, Technological Change, and Growth
- Browse content in O1 - Economic Development
- O11 - Macroeconomic Analyses of Economic Development
- O12 - Microeconomic Analyses of Economic Development
- O13 - Agriculture; Natural Resources; Energy; Environment; Other Primary Products
- O16 - Financial Markets; Saving and Capital Investment; Corporate Finance and Governance
- O17 - Formal and Informal Sectors; Shadow Economy; Institutional Arrangements
- Browse content in O2 - Development Planning and Policy
- O23 - Fiscal and Monetary Policy in Development
- Browse content in O3 - Innovation; Research and Development; Technological Change; Intellectual Property Rights
- O30 - General
- O31 - Innovation and Invention: Processes and Incentives
- O32 - Management of Technological Innovation and R&D
- O33 - Technological Change: Choices and Consequences; Diffusion Processes
- O34 - Intellectual Property and Intellectual Capital
- O35 - Social Innovation
- O38 - Government Policy
- Browse content in O4 - Economic Growth and Aggregate Productivity
- O40 - General
- O43 - Institutions and Growth
- Browse content in O5 - Economywide Country Studies
- O53 - Asia including Middle East
- Browse content in P - Economic Systems
- Browse content in P1 - Capitalist Systems
- P16 - Political Economy
- P18 - Energy: Environment
- Browse content in P2 - Socialist Systems and Transitional Economies
- P26 - Political Economy; Property Rights
- Browse content in P3 - Socialist Institutions and Their Transitions
- P31 - Socialist Enterprises and Their Transitions
- P34 - Financial Economics
- P39 - Other
- Browse content in P4 - Other Economic Systems
- P43 - Public Economics; Financial Economics
- P48 - Political Economy; Legal Institutions; Property Rights; Natural Resources; Energy; Environment; Regional Studies
- Browse content in Q - Agricultural and Natural Resource Economics; Environmental and Ecological Economics
- Browse content in Q0 - General
- Q02 - Commodity Markets
- Browse content in Q3 - Nonrenewable Resources and Conservation
- Q31 - Demand and Supply; Prices
- Q32 - Exhaustible Resources and Economic Development
- Browse content in Q4 - Energy
- Q40 - General
- Q41 - Demand and Supply; Prices
- Q42 - Alternative Energy Sources
- Q43 - Energy and the Macroeconomy
- Browse content in Q5 - Environmental Economics
- Q50 - General
- Q51 - Valuation of Environmental Effects
- Q53 - Air Pollution; Water Pollution; Noise; Hazardous Waste; Solid Waste; Recycling
- Q54 - Climate; Natural Disasters; Global Warming
- Q56 - Environment and Development; Environment and Trade; Sustainability; Environmental Accounts and Accounting; Environmental Equity; Population Growth
- Browse content in R - Urban, Rural, Regional, Real Estate, and Transportation Economics
- Browse content in R0 - General
- R00 - General
- Browse content in R1 - General Regional Economics
- R10 - General
- R11 - Regional Economic Activity: Growth, Development, Environmental Issues, and Changes
- R12 - Size and Spatial Distributions of Regional Economic Activity
- Browse content in R2 - Household Analysis
- R20 - General
- R21 - Housing Demand
- R23 - Regional Migration; Regional Labor Markets; Population; Neighborhood Characteristics
- Browse content in R3 - Real Estate Markets, Spatial Production Analysis, and Firm Location
- R30 - General
- R31 - Housing Supply and Markets
- R32 - Other Spatial Production and Pricing Analysis
- R33 - Nonagricultural and Nonresidential Real Estate Markets
- R38 - Government Policy
- Browse content in R4 - Transportation Economics
- R41 - Transportation: Demand, Supply, and Congestion; Travel Time; Safety and Accidents; Transportation Noise
- Browse content in R5 - Regional Government Analysis
- R51 - Finance in Urban and Rural Economies
- Browse content in Z - Other Special Topics
- Browse content in Z1 - Cultural Economics; Economic Sociology; Economic Anthropology
- Z11 - Economics of the Arts and Literature
- Z13 - Economic Sociology; Economic Anthropology; Social and Economic Stratification
- Advance articles
- Editor's Choice
- Author Guidelines
- Submission Site
- Open Access
- About The Review of Financial Studies
- Editorial Board
- Advertising and Corporate Services
- Journals Career Network
- Self-Archiving Policy
- Dispatch Dates
- Terms and Conditions
- Journals on Oxford Academic
- Books on Oxford Academic
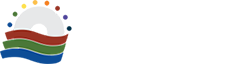 Article Contents1. institutional setting, 2. empirical strategy, 3. outcome variables, 5. discussion, 6. conclusions. The Effects of Business Accelerators on Venture Performance: Evidence from Start-Up Chile- Article contents
- Figures & tables
- Supplementary Data
Juanita Gonzalez-Uribe, Michael Leatherbee, The Effects of Business Accelerators on Venture Performance: Evidence from Start-Up Chile, The Review of Financial Studies , Volume 31, Issue 4, April 2018, Pages 1566–1603, https://doi.org/10.1093/rfs/hhx103 - Permissions Icon Permissions
Do business accelerators affect new venture performance? We investigate this question in the context of Start-Up Chile, an ecosystem accelerator. We focus on two treatment conditions typically found in business accelerators: basic services of funding and coworking space, and additional entrepreneurship schooling. Using a regression discontinuity design, we show that schooling bundled with basic services can significantly increase new venture performance. In contrast, we find no evidence that basic services affect performance on their own. Our results are most relevant for ecosystem accelerators that attract young and early-stage businesses and suggest that entrepreneurial capital matters in new ventures. Received September 1, 2015; editorial decision July 30, 2017 by Editor Francesca Cornelli. Business accelerators are an increasingly important institutional form of entrepreneurial ecosystems. Since the first investor-led accelerator debuted in 2005 (i.e., Y Combinator), thousands of business accelerators have sprung up worldwide. 1 These fixed-term, cohort-based programs offer start-ups a combination of cash, shared office space, and entrepreneurship schooling. Accelerators distinguish themselves from other early-stage financiers by their strong emphasis on entrepreneurship schooling, which is believed to provide “entrepreneurial capital” to participants who are otherwise lacking it. Although evidence about “managerial capital” constraints (e.g., Bruhn, Karlan, and Schoar 2010 ; Bloom and van Reenen 2010 ) seems to justify this emphasis, little rigorous evidence exists on the effect of business accelerators on new venture performance and on the importance of entrepreneurial capital in new firms. 2 This lack of evidence is particularly pressing given the importance of new ventures for economic development ( Davis, Haltiwanger, and Schuh 1996 ; Haltiwanger, Jarmin, and Miranda 2013 ) and the relevant public and private resources being spent to foster entrepreneurial activity. 3 This paper provides the first quasi-experimental evidence of the effect of business accelerator programs on new venture performance. It advances prior work on accelerators that, until now, has focused on these programs’ conceptual definitions and has faced challenges in distinguishing the programs’ effects from venture selection ( Cohen and Hochberg 2014 ). This paper also provides first-time evidence on the importance of entrepreneurial capital in new ventures. 4 In contrast, prior related work has concentrated on the role of managerial capital in established firms and nontransformational ventures (cf. McKenzie and Woodruff 2014 ). Business accelerators are an ideal context for studying the role of entrepreneurial capital in new ventures. Entrepreneurial capital refers to the set of skills and resources needed to start and grow nascent businesses. This type of capital can include know-how about seizing opportunities and growing a business ( Bingham, Eisenhardt, and Furr 2007 ); cultivating a good reputation to attract employees, investors, and customers ( Rao 1994 ; Zott and Huy 2007 ); and accessing valuable social networks ( Granovetter 1973 ). Business accelerators’ emphasis on entrepreneurship schooling has led practitioners to dub these institutions “the new business schools for entrepreneurs” ( Golomb 2015 ). 5 The exact form of this schooling varies across programs, but generally includes formal instruction (e.g., workshops and seminars on a wide range of entrepreneurship topics), guidance (e.g., access to mentors), networking opportunities, and start-up progress monitoring or accountability ( Cohen and Hochberg 2014 ). Similar to how business schools can increase managerial capital, entrepreneurship schools in accelerators can increase entrepreneurial capital by conferring certification (cf. Spence 1973 ; Arrow 1973 ) and increasing the productivity of founders (cf. Becker 1975 ). Start-ups can benefit from certification because of the large information asymmetries about the performance potential of these firms. They can also benefit from productivity increases, which are otherwise hampered by market frictions such as informational constraints. For example, some founders may simply be unaware of the importance of building business networks and not look for networking opportunities outside the accelerator. 6 Our setting is Start-Up Chile, an ecosystem accelerator aimed at high-growth, early-stage ventures. In contrast to investor-led accelerators (e.g., Y Combinator and TechStars), which typically aim to make a return on their investment, ecosystem accelerators (e.g., Village Capital and Parallel 18) aim to stimulate start-up activity in their focal region ( Clarysse, Wright, and Van Hove 2015 ). Similar to other ecosystem accelerators worldwide, Start-Up Chile offers participants an equity-free cash infusion, shared coworking office space, and the possibility of being selected into an exclusive subprogram, which we refer to as the entrepreneurship school. In addition to the potential certification from acceptance into the entrepreneurship school, participants are provided services typical of business accelerators: guidance and accountability, via monthly meetings with program staff, program peers, and industry experts; opportunities for networking (including representing the program at high-profile events); and advertisement on the Start-Up Chile Web page. Using a fuzzy regression discontinuity design (RDD), which exploits the fact that the program accepts a fixed number of participants every round based on an application score, we provide estimates of local average treatment effects of basic accelerator services (i.e., cash and coworking space) on start-up performance. Furthermore, exploiting a unique feature of Start-Up Chile—that only 20% of participants are selected into the entrepreneurship school, based on a business plan “pitch” competition and an informal qualification score cutoff—we are able to provide estimates of local causal effects of entrepreneurship schooling bundled with basic services, and distinguish this from the effect of basic services alone. We estimate that the combination of participation in the entrepreneurship school and access to the basic services of cash and coworking space leads to significantly higher venture fundraising and scale within the first 4.75 years of entry to the accelerator for the subpopulation “randomized in” by the pitch competition. Our more conservative results indicate that entrepreneurship schooling increases the probability of securing additional financing by 21.0%, which corresponds to a 0.29-standard-deviation increase over the sample mean. We further estimate that entrepreneurship schooling results in an increase of three times the amount of capital raised, helping firms increase their fundraising performance, resulting in an unconditional average increase of 37,000 USD to 112,000 USD, which is a 0.30-standard-deviation increase over the mean. Schooling also appears to increase venture scale: we estimate it results in a twofold increase in employees, helping firms go from an unconditional average of 0.9 employees to 1.8, a 0.34-standard-deviation increase over the sample mean. By contrast, we find no evidence that basic accelerator services of cash and coworking space have a treatment effect on fundraising, scale, or survival—at least not for the subpopulation of start-ups randomized in by the selection rule. An important challenge of working with start-up performance data is the collection of outcome measures for all accelerator applicant start-ups. Similar to prior research, we hand-collected Web-based performance measures for all applicants ( Kerr, Lerner, and Schoar 2014 ; Goldfarb, Kirsch, and Miller 2007 ; Hallen, Bingham, and Cohen 2016 ). In addition, we collected complementary outcome data using two surveys. Results based on both types of outcome metrics point to the same conclusion: the combination of basic services and entrepreneurship schooling in ecosystem accelerators is more effective than providing basic services only. This conclusion is consistent with recent work showing that the impact of consulting services for nontransformational ventures is much larger than simply improving access to capital (cf. Bruhn, Karlan, and Schoar 2010 ). It is also consistent with the view that entrepreneurial capital—similar to managerial capital—is a type of capital that is missing among certain populations (cf. Bruhn, Karlan, and Schoar 2010 ). A second empirical challenge of studying business accelerators is distinguishing between treatment and selection effects. The setting of Start-Up Chile provides us with the opportunity to advance in overcoming this challenge. Under the assumptions of no precise sorting of start-ups in the vicinity of the capacity cutoff for the basic services, or in the vicinity of the informal pitch competition qualification cutoff for the entrepreneurship school, our results estimate the local treatment effects of basic services and schooling around each respective cutoff. We present evidence in support of these identification assumptions. We also test and provide suggestive evidence against potential methodological concerns such as influential observations, survey- and Web-reporting biases, and demotivation effects on pitch competition losers. Although we cannot fully rule out these concerns (because we econometricians only have partial information), the preponderance of evidence suggests that the regression discontinuity design (RDD) estimates are valid. In terms of the external validity of our findings, the program-level similarity between Start-Up Chile and other ecosystem accelerators suggests that the results are representative of these types of programs at large. Moreover, a cross-program comparison of average applicants with a sample of ecosystem accelerators worldwide sharpens the external validity of our predictions; the findings are particularly valid for ecosystem accelerators that attract young entrepreneurs and early-stage start-ups. We contribute to several bodies of research. First, a growing body of literature focuses on the effects of early-stage financiers on new ventures, but has mostly explored venture capital and angel investors (e.g., Hellmann and Puri 2000 , 2002 ; Kerr, Lerner, and Schoar 2014 ; Lerner et al. 2015 ). Our paper complements this stream of work by focusing on an increasingly important early-stage financier: business accelerators. Second, our results complement the emerging work on business accelerators, which we roughly classify into three literature streams. The first stream focuses on conceptual descriptions of the accelerator model ( Bernthal 2015 ; Cohen 2013 ; Cohen and Hochberg 2014 ; Kim and Wagman 2014 ; Radojevich-Kelley and Hoffman 2012 ). A second stream investigates the potential effects of accelerators on regional development ( Fehder and Hochberg 2014 ). The third stream explores these programs’ potential effects on new venture outcomes and founders ( Hallen, Bingham, and Cohen 2016 ; Yu 2016 ; Smith and Hannigan 2015 ; Leatherbee and Eesley 2014 ). Our work is most closely related to this third stream, which generally faces identification challenges in rigorously distinguishing the value-added role of business accelerator services. Our contribution is the identification of the performance-enhancing effect of entrepreneurship schooling combined with basic services relative to basic services alone. Thus, we help distinguish, for the first time, which program services can affect new venture performance. Prior work finds suggestive evidence of how the bundle of services provided by accelerators can affect performance, but does not distinguish the role of any specific service. In particular, Hallen, Bingham, and Cohen (2016) argue that ventures can indirectly learn from the experience of others affiliated with the accelerator. Leatherbee and Eesley (2014) argue that founders can improve their entrepreneurial opportunity discovery behaviors through their interaction with more active peers. Yu (2016) and Smith and Hannigan (2015) argue that accelerators can help to speed up success or failure by resolving the uncertainty about the inherent potential of the start-up more quickly. Third, our paper builds on the literature about firms’ management practices and business-training programs. Consistent with the importance of managerial capital, empirical studies show a strong association between managerial practices and company performance ( Acemoglu et al. 2007 ; Bloom and van Reenen 2010 ). However, the evidence pertaining to new ventures (and thus to the effect of entrepreneurial rather than managerial capital) is mixed ( McKenzie and Woodruff 2014 ) and mostly relates to nontransformational ventures. We contribute to this literature by distinguishing entrepreneurial capital from managerial capital. Whereas prior literature has defined and studied managerial capital as an input factor for established firms ( Bertrand and Schoar 2003 ; de Mel, McKenzie, and Woodruff 2008 ; McKenzie and Woodruff 2008 ; Bloom, Sadun, and van Reenen 2016 ), we focus on the input factors useful for emerging transformational ventures ( Schoar 2010 ) before the firm is established, when entrepreneurs are searching for the business opportunity. 1.1 Research settingWe focus on the case of Start-Up Chile, an ecosystem accelerator launched in August 2010 and sponsored by the Chilean government. Its main aim is the attraction of early-stage, high-potential entrepreneurs from across the globe, and the transformation of the domestic entrepreneurship ecosystem. 7 As of August 2015, approximately 1,000 start-ups had participated in the program, and nearly 6,000 had applied. Like other business accelerators worldwide, Start-Up Chile is a fixed-term, cohort-based program that offers participants shared office space and equity-free seed capital (roughly US |${\$}$| 40,000 delivered in two installments: 50% at the beginning and the remaining 50% three months later, conditional on survival). In addition, it offers entrepreneurship schooling to a select few participants. On average, each cohort consists of 100 competitively selected participants, who, similar to other ecosystem accelerators worldwide, relocate to the programs’ headquarters for six months. 8 As explained in more detail below ( Section 1.3 ), the selection process is based on the relative quality of the submitted application, as evaluated by external judges. At the end of their term, participating start-ups “graduate” through a “demo day” competition (i.e., a formal presentation of the companies to external investors). Like traditional business accelerators, Start-Up Chile also offers entrepreneurship schooling. The unique feature in our setting, however, is that these sought-after schooling services are only available to a few participants. On average, 20 participants in every cohort are competitively selected to take part in the entrepreneurship school. As explained in more detail in Section 1.4 , the selection procedure for the entrepreneurship school consists of a competition, dubbed “pitch-day,” where start-ups pitch their businesses and are evaluated by judges. The schooled participants are the poster children of the program and, similar to other accelerators, their names are advertised on the program’s Web page and in specialized news releases. In addition to potential certification from acceptance into the entrepreneurship school, participants receive two key additional services, which are similar to the schooling services typically offered at business accelerators: guidance and accountability, as well as networking opportunities. 9 The guidance and accountability are imparted via 30-minute monthly meetings with program staff, program peers, and industry experts (no one is compensated or holds an equity stake), where milestones are set and entrepreneurs are held socially accountable for their self-defined strategic goals. Industry experts are generally Chileans connected to the Start-Up Chile network and are assigned to schooled participants according to industry. The networking opportunities arise because participants represent the program at high-profile public events and host (by holding one-on-one meetings) high-profile Start-Up Chile guests, such as Steve Wozniak and Paul Ahlstrom. Start-Up Chile provided us with all the application data, including application scores and final selection decisions into the program and the entrepreneurship school, for seven cohorts. Our sample consists of 3,258 applicants (616 participants and 2,642 nonparticipants). Participants for generation 1 (7) arrived in Santiago, Chile, in June 2011 (June 2013) and graduated in January 2012 (January 2014). In addition, Start-Up Chile granted us access to confidential records of the pitch-day competitions, including pitch-day scores and final selection decisions. Because the entrepreneurship school was launched in generation 4, these additional data are only available for generations 4–7 and amount to 276 pitch-day competitors (59 schooled participants; 217 nonschooled competitors). Based on the program’s records, we constructed six covariates to use as controls in our empirical strategy: the age of the entrepreneur ( Age ), indicator variables for domestic and female applicants ( Chilean, Female ), the natural logarithm of the number of employees ( Employees before ), and indicator variables for capital raised before application to the program ( Capital raised before ) and for start-ups that already had a working prototype or had one in development at application ( Prototype ). Table 1 provides summary statistics of our sample. On average, applicant founders are 30 years old; 21% of them are Chilean; and 14% are female. Applicant start-ups have between two and three employees, on average; 26% have previously raised capital; and 49% are working to develop a prototype or have already developed one. Main variables Variable . | Obs. . | Mean . | SD . | Min. . | Max. . |
---|
| | | | | | Age | 1,582 | 30.33 | 6.76 | 19.00 | 84.00 | Chilean | 3,258 | 0.21 | 0.41 | 0.00 | 1.00 | Female | 1,906 | 0.14 | 0.34 | 0.00 | 1.00 | Employees before | 2,248 | 2.46 | 1.46 | 1.00 | 10.00 | Capital raised before | 2,779 | 0.26 | 0.44 | 0.00 | 1.00 | Prototype | 3,258 | 0.49 | 0.50 | 0.00 | 1.00 | | | | | | | Rank | 3,258 | 260.91 | 164.33 | 1.00 | 656.00 | Pitch-day score | 276 | 3.14 | 0.70 | 0.00 | 4.50 | Acceleration | 3,258 | 0.19 | 0.39 | 0.00 | 1.00 | School | 3,258 | 0.02 | 0.14 | 0.00 | 1.00 | | | | | | | Web capital indicator | 3,258 | 0.026 | 0.159 | 0.00 | 1.00 | Web capital raised | 3,258 | 0.491 | 2.336 | 0.00 | 16.93 | Web employees | 3,258 | 0.534 | 1.939 | 0.00 | 11.00 | Web traction | 3,258 | 0.063 | 0.284 | 0.00 | 4.78 | Web survival | 3,258 | 0.212 | 0.409 | 0.00 | 1.00 | | | | | | | Survey A. capital indicator | 319 | 0.658 | 0.475 | 0.00 | 1.00 | Survey A. capital raised | 318 | 6.973 | 5.246 | 0.00 | 14.51 | Survey A. valuation | 318 | 7.664 | 6.512 | 0.00 | 16.52 | Survey A. employees | 319 | 0.542 | 0.799 | 0.00 | 3.43 | Survey A. traction | 319 | 3.673 | 4.610 | 0.00 | 13.12 | Survey A. survival | 319 | 0.618 | 0.487 | 0.00 | 1.00 | | | | | | | Survey P. capital indicator | 145 | 0.579 | 0.495 | 0.00 | 1.00 | Survey P. capital raised | 145 | 7.118 | 6.262 | 0.00 | 18.60 | Survey P. valuation | 145 | 4.673 | 6.957 | 0.00 | 19.56 | Survey P. employees | 145 | 1.333 | 1.255 | 0.00 | 4.81 | Survey P. traction | 145 | 6.823 | 6.142 | 0.00 | 16.81 | Survey P. survival | 145 | 0.641 | 0.481 | 0.00 | 1.00 |
Variable . | Obs. . | Mean . | SD . | Min. . | Max. . |
---|
| | | | | | Age | 1,582 | 30.33 | 6.76 | 19.00 | 84.00 | Chilean | 3,258 | 0.21 | 0.41 | 0.00 | 1.00 | Female | 1,906 | 0.14 | 0.34 | 0.00 | 1.00 | Employees before | 2,248 | 2.46 | 1.46 | 1.00 | 10.00 | Capital raised before | 2,779 | 0.26 | 0.44 | 0.00 | 1.00 | Prototype | 3,258 | 0.49 | 0.50 | 0.00 | 1.00 | | | | | | | Rank | 3,258 | 260.91 | 164.33 | 1.00 | 656.00 | Pitch-day score | 276 | 3.14 | 0.70 | 0.00 | 4.50 | Acceleration | 3,258 | 0.19 | 0.39 | 0.00 | 1.00 | School | 3,258 | 0.02 | 0.14 | 0.00 | 1.00 | | | | | | | Web capital indicator | 3,258 | 0.026 | 0.159 | 0.00 | 1.00 | Web capital raised | 3,258 | 0.491 | 2.336 | 0.00 | 16.93 | Web employees | 3,258 | 0.534 | 1.939 | 0.00 | 11.00 | Web traction | 3,258 | 0.063 | 0.284 | 0.00 | 4.78 | Web survival | 3,258 | 0.212 | 0.409 | 0.00 | 1.00 | | | | | | | Survey A. capital indicator | 319 | 0.658 | 0.475 | 0.00 | 1.00 | Survey A. capital raised | 318 | 6.973 | 5.246 | 0.00 | 14.51 | Survey A. valuation | 318 | 7.664 | 6.512 | 0.00 | 16.52 | Survey A. employees | 319 | 0.542 | 0.799 | 0.00 | 3.43 | Survey A. traction | 319 | 3.673 | 4.610 | 0.00 | 13.12 | Survey A. survival | 319 | 0.618 | 0.487 | 0.00 | 1.00 | | | | | | | Survey P. capital indicator | 145 | 0.579 | 0.495 | 0.00 | 1.00 | Survey P. capital raised | 145 | 7.118 | 6.262 | 0.00 | 18.60 | Survey P. valuation | 145 | 4.673 | 6.957 | 0.00 | 19.56 | Survey P. employees | 145 | 1.333 | 1.255 | 0.00 | 4.81 | Survey P. traction | 145 | 6.823 | 6.142 | 0.00 | 16.81 | Survey P. survival | 145 | 0.641 | 0.481 | 0.00 | 1.00 |
The table presents summary statistics of the main variables used in the analysis. The first and second sections include variables extracted from the applications and the Start-Up Chile records. The third section includes Web-based outcome variables, which were collected during first quarter of 2014 (mid-2015) from Facebook and LinkedIn (CB Insights). The last two sections include survey-based outcome variables. The first survey was distributed to all applicants during October 2014, and the second survey was distributed to all participants during the first quarter of 2016. For variable definitions, see Sections 1.3 and 3 . Our sample is comparable to prior research on early-stage ventures, particularly in terms of the number of employees (e.g., Haltiwanger, Jarmin, and Miranda 2013 ) and industry representation (e.g., Puri and Zarutskie 2012 ) (see Appendix 2 for further details). Our sample is also comparable to the ecosystem business-accelerator genre. Using information from the Entrepreneurship Database (ED) program at Emory University, 10 which has records of multiple ecosystem accelerators worldwide, we report in Appendix Tables 2.1 and 2.2 comparisons between the preapplication start-ups (founders) in our sample and those of the ED database (reported under the heading “ED”). The tables show that, relative to average applicants in other ecosystem accelerators worldwide, the average Start-Up Chile applicant is younger, less likely to be female, has a younger and more underdeveloped business, and is less likely to have raised capital prior to potential participation. 1.3 Accelerator selection processSelection into the basic Start-Up Chile program is a two-part process. First, entrepreneurs submit their applications through an online platform operated by YouNoodle—a private company based in California that runs application processes for accelerator programs worldwide. YouNoodle sends the applications to a network of entrepreneurship experts, who judge and evaluate applications based on three criteria: the quality of the founding team, the merits of the project, and its potential impact on Chile’s entrepreneurial community. For every generation that applies to Start-Up Chile, YouNoodle averages the judges’ scores and ranks start-ups from best to worst. No ties are permitted: if companies tie, they are ranked randomly. Importantly, applicants do not know who their judges are, nor do they know their position in the rankings; thus it is impossible for applicants to manipulate the ranking process. Three to five expert judges are assigned randomly to each application. YouNoodle’s network consists of approximately 200 entrepreneurship experts: roughly 40% from Silicon Valley, 25% from Latin America, 20% from EMEA, and 10% from the rest of the United States. Each expert evaluates approximately 10 start-ups per generation, the identity of the other judges evaluating the same start-ups is unknown, and no single judge sees all applications. Thus, judges are unlikely to be able to precisely manipulate the rankings (e.g., to help an applicant friend qualify). A committee at the Chilean Economic Development Agency (CORFO), which funds Start-Up Chile, handles the second part of the selection process, making the final decision based on YouNoodle’s ranking. A capacity threshold is prespecified for each cohort (normally 100), 11 and the top-ranking companies—those ranking higher than the threshold—are typically selected. 12 The threshold corresponds to the predetermined size of the cohort, and the government determines the threshold as a function of its budget before the application process begins. Perfect compliance with the selection rule does not occur; not all applicants that meet the 100-company threshold ultimately participate, and not all of the accepted participants are ranked higher than the threshold. Two reasons explain the less-than-perfect compliance: (1) earlier stage start-ups (as opposed to established businesses) receive preference, especially in sectors that are not traditional to the Chilean economy, and (2) some selected applicants ultimately reject the offer. In the latter case, other candidates, usually ranking lower, are selected. 1.4 Entrepreneurship school selection processTwo months into the program, participants can apply to the program’s entrepreneurship school. The entrepreneurship school is not available to all participants, because monitoring requirements are too burdensome for the staff, and providing the preferential access to external speakers and staff’s contacts to all participants is infeasible. On average, 80% of the accelerator participants chose to compete for a spot in the school, and roughly 20% of competitors are selected. The selection procedure for the entrepreneurship school is also a two-part process, starting with a competition dubbed “pitch-day.” On pitch-day, competing start-ups formally present, or “pitch,” their business to a group of local judges (who are independent from the accelerator application-process judges), that is comprised of both external (i.e., staff at other private accelerators in Chile, e.g., Telefonica’s Wayra) and internal (i.e., staff at Start-Up Chile) members. Participants are allotted five minutes for their pitch, and, overall, the competition generally lasts for two hours. A guideline for the pitch is provided. Judges score competitors on five criteria: (1) the problem their business is trying to solve, (2) the proposed solution, (3) the business model, (4) the size of the market, and (5) fundraising needs. Judges keep records of the scores they assign to competitors by criteria. The Start-Up Chile staff handles the second part of the selection process, making the final decision based on the average pitch-day scores—a weighted average of the scores per criterion across judges, where the weights (by criteria) are determined ex ante by Start-up Chile. 13 The potential for precise manipulation of pitch-day scores is small. Competitors do not know the identity of judges until minutes prior to the competition. Moreover, the external judges have no clear incentive for manipulation because they have no “skin in the game.” In addition, although some judges might want to help a participating friend, they cannot precisely manipulate the average score: judges independently score each start-up across the five criteria and no one judge oversees all the scores. No formal restriction exists on the number of participants allowed in the entrepreneurship school, and 15 participants win a spot in every cohort on average. However, an informal selection rule is implicit in the data—competitors scoring above 3.6 are 51.9% more likely to be chosen (conditional on the pitch-day score; see Column 1 in Table 4 ). Conversations with the staff indicate that there is an informal rule in the reviewing process: competitors below the normative “quality bar” of a 3.6 pitch-day score are generally desk rejected. Discontinuity probability of schooling at the pitch-day score of 3.6 . | (1) . | (2) . | (3) . | (4) . | (5) . |
---|
. | p|$=$|1 . | p|$=$|1 & controls . | p|$=$|1& controls & h|$=$|1.5 . | p|$=$|1 & h|$=$|1 . | p|$=$|2 & controls . |
---|
|$\mathit{Above}$| | 0.519 | 0.509 | 0.420 | 0.440 | 0.341 | | (0.072) | (0.073) | (0.085) | (0.094) | (0.097) | Observations | 276 | 276 | 265 | 248 | 276 | R-squared | 0.398 | 0.435 | 0.440 | 0.385 | 0.455 |
. | (1) . | (2) . | (3) . | (4) . | (5) . |
---|
. | p|$=$|1 . | p|$=$|1 & controls . | p|$=$|1& controls & h|$=$|1.5 . | p|$=$|1 & h|$=$|1 . | p|$=$|2 & controls . |
---|
|$\mathit{Above}$| | 0.519 | 0.509 | 0.420 | 0.440 | 0.341 | | (0.072) | (0.073) | (0.085) | (0.094) | (0.097) | Observations | 276 | 276 | 265 | 248 | 276 | R-squared | 0.398 | 0.435 | 0.440 | 0.385 | 0.455 |
This table shows the discontinuity in the probability of schooling around the pitch-day-score cutoff. Estimates are based on different specifications of the regression |$\mathit{school}=\tau +\mu \mathit{Above}+g(\mathit{Pitch}_{\it day}\mathit{Score}-3.6 )+Z'\phi +\varepsilon $| , where the outcome variable school is an indicator variable that equals 1 if the participant was selected into the entrepreneurship school, Above is an indicator variable that equals 1 if the participant scored 3.6 or higher during the pitch day, and |$g(\mathit{Pitch}_{\it day}\mathit{Score}-3.6)$| is a |$p$| th-degree polynomial of the normalized pitch-day score (i.e., pitch-day score minus the 3.6 cutoff). The type of specification is indicated at the top of each column including the degree of the polynomial used (p), and the inclusion of controls. Columns (3) and (4) include different bandwidth specifications (h) for pitch-day-score ranges of 2.1–5.0 (h |$=$| 1.5) and 2.6–4.5 (h |$=$| 1), respectively. The controls included are Capital raised before and generation fixed effects. To conserve space, the estimated coefficients for the constant and the polynomial terms are not presented in the table. Robust standard errors are presented in parentheses. * , ** , and *** indicate statistical significance at the 10%, 5%, and 1% level, respectively. This informal rule is evident in Table 2 , where we summarize the number of pitch-day competitors and schooled participants across generations—beginning with the fourth generation, during which the entrepreneurial school was introduced. For ease of exposition, we group participants across brackets of 0.5 pitch-day-score units. Panel A shows the distribution of average pitch-day scores, which concentrates around mid-range values. Column 2 shows 78% of average pitch-day scores are between 2.6 and 4.0, inclusive, whereas 15% (7%) of average pitch-day scores are lower (higher) than 2.6 (4.0). Panel B shows a stark jump in the probability of acceptance in to the entrepreneurship school between average pitch-day scores of 3.1–3.5 and 3.6–4.0, where the average acceptance rate of all generations increases from 8% to 54%. This jump represents a distinct and permanent shift in the relationship between schooling and the pitch-day score: it is present across all generations and thus not due to cross-sectional variation in scores across batches. 14 Number of applicants and acceptance rate of the entrepreneurship school . | . | |$A$| . | |$B$| . |
---|
. | . | Applicants’ school . | Acceptance rate school (%) . |
---|
Pitch-day . | All, share . | . | Generation . | . | Generation . |
---|
score bracket . | (%) . | All . | 4 . | 5 . | 6 . | 7 . | All . | 4 . | 5 . | 6 . | 7 . |
---|
0.0–0.9 | 1.4 | 4 | 0 | 4 | 0 | 0 | 0.0 | 0.0 | 0.0 | 0.0 | 0.0 | 1.0–1.5 | 0.4 | 1 | 0 | 1 | 0 | 0 | 0.0 | 0.0 | 0.0 | 0.0 | 0.0 | 1.6–2.0 | 4.7 | 13 | 3 | 6 | 1 | 3 | 0.0 | 0.0 | 0.0 | 0.0 | 0.0 | 2.1–2.5 | 9.1 | 25 | 3 | 12 | 6 | 4 | 0.0 | 0.0 | 0.0 | 0.0 | 0.0 | 2.6–3.0 | 30.1 | 83 | 10 | 35 | 16 | 22 | 9.6 | 0.0 | 8.6 | 0.0 | 22.7 | 3.1–3.5 | 27.9 | 77 | 25 | 11 | 33 | 8 | 7.8 | 0.0 | 36.4 | 0.0 | 25.0 | 3.6–4.0 | 19.6 | 54 | 16 | 8 | 22 | 8 | 53.7 | 50.0 | 75.0 | 40.9 | 75.0 | 4.1–4.5 | 6.9 | 19 | 5 | 3 | 11 | 0 | 84.2 | 100.0 | 66.7 | 81.8 | 0.0 | 4.6–5.0 | 0.0 | 0 | 0 | 0 | 0 | 0 | 0.0 | 0.0 | 0.0 | 0.0 | 0.0 |
. | . | |$A$| . | |$B$| . |
---|
. | . | Applicants’ school . | Acceptance rate school (%) . |
---|
Pitch-day . | All, share . | . | Generation . | . | Generation . |
---|
score bracket . | (%) . | All . | 4 . | 5 . | 6 . | 7 . | All . | 4 . | 5 . | 6 . | 7 . |
---|
0.0–0.9 | 1.4 | 4 | 0 | 4 | 0 | 0 | 0.0 | 0.0 | 0.0 | 0.0 | 0.0 | 1.0–1.5 | 0.4 | 1 | 0 | 1 | 0 | 0 | 0.0 | 0.0 | 0.0 | 0.0 | 0.0 | 1.6–2.0 | 4.7 | 13 | 3 | 6 | 1 | 3 | 0.0 | 0.0 | 0.0 | 0.0 | 0.0 | 2.1–2.5 | 9.1 | 25 | 3 | 12 | 6 | 4 | 0.0 | 0.0 | 0.0 | 0.0 | 0.0 | 2.6–3.0 | 30.1 | 83 | 10 | 35 | 16 | 22 | 9.6 | 0.0 | 8.6 | 0.0 | 22.7 | 3.1–3.5 | 27.9 | 77 | 25 | 11 | 33 | 8 | 7.8 | 0.0 | 36.4 | 0.0 | 25.0 | 3.6–4.0 | 19.6 | 54 | 16 | 8 | 22 | 8 | 53.7 | 50.0 | 75.0 | 40.9 | 75.0 | 4.1–4.5 | 6.9 | 19 | 5 | 3 | 11 | 0 | 84.2 | 100.0 | 66.7 | 81.8 | 0.0 | 4.6–5.0 | 0.0 | 0 | 0 | 0 | 0 | 0 | 0.0 | 0.0 | 0.0 | 0.0 | 0.0 |
The table presents the number of applicants (panel A) and the acceptance rate (panel B) of the entrepreneurship school across pitch-day-score brackets. 2.1 Exploiting the accelerator’s selection ruleWe use the capacity-threshold rule in the selection process of Start-Up Chile to estimate a local average treatment effect of basic accelerator services on new venture performance. This rule implies the probability of acceleration changes discontinuously at the capacity threshold as a function of the applicant’s ranking. Therefore, the difference in expected outcomes between start-ups on opposite sides of—but sufficiently near—the threshold can provide the basis for an unbiased local causal estimate. The main identification assumption is that ranks are not manipulated around the threshold. In this section, we begin by estimating the size of the discontinuity, and then present supportive evidence of the identification assumption. Finally, we describe the RDD empirical approach. The discontinuity in acceleration at the capacity threshold is visible in Figure 1 . We plot the fraction of participating applicants against the normalized rank (i.e., the ranking of the start-up minus the generation’s capacity threshold) calculated across bins of 10 ranks and plotted in dots. Because we plot acceleration against normalized ranking, higher-ranking companies are represented to the right of the capacity threshold, which corresponds to the 0 on the |$x$| -axis.  Fraction of accelerated applicants The figure shows the average fraction of accelerated applicants (dots) in bins of 10 applicants, as well as the fitted values and 90% confidence interval from the regression |${\it acceleration}_{s}=\delta +\gamma {\it higher}_{s}+f\left( {\it Rank}_{s}-{\it cutoff}^{g} \right)+X_{s}'\sigma +\varepsilon_{s}$| , where the outcome variable Acceleration is an indicator variable that equals 1 if the applicant participated in the accelerator, Higher , a variable that equals 1 if the applicant ranks above the ranking cutoff of the capacity threshold in its generation, and 0 otherwise, and |$f\left( {\it Rank}_{s}-{\it cutoff}^{g} \right)$| is a fourth-degree polynomial of the normalized rank. The vertical line represents the ranking cutoff normalized at 0 for the normalized rank. Only observations ranking below 301 are included in the plot. The relatively poor fit of the polynomial for companies ranking around 150 ( |$+$| 50 in the plot) is not mechanically driven by the change in capacity threshold in generation 2: the estimated participation rate for companies ranking in positions 150, 155, and 159 is lower than the observed probability of 0.6 across generations 3 to 8. In unreported analysis, we checked whether the participants ranking in these positions are observationally different (they are not) and whether a discontinuity exists here (it doesn’t). Alternative explanations for the poor fit include a statistical issue (i.e., we have information about only 7 generations, and in this sample, start-ups ranking around 150 happen to be of comparatively good quality) and checking thresholds by program officials (i.e., start-ups around 150 and 160 constitute the final checking threshold for judges, such that if some spots are still available, they are filled in with these). The estimated discontinuity is sizable, significant, and robust. Table 3 presents robust estimates of |$\gamma $| across different specifications of Equation ( 1 ), with varying polynomial degrees ( |$p)$| and rank bandwidths ( |$h$| ), including first-degree polynomials and 50 participants (ranks) around the threshold (Column 1), and additionally including generation fixed effects and controls (Column 2); as well as using the entire sample and including second-degree (Columns 3 and 4), third-degree (Columns 5 and 6), and fourth-degree polynomials (Columns 5 and 6) with and without controls. The coefficient of Column 7 (Column 8) implies that ranking higher than the capacity threshold increases the probability of acceleration by 16.6% (16.4%) relative to other start-ups in the same generation (and controlling for observable differences across start-ups). We plot the corresponding estimated probability of schooling of the estimates in Column 7 (and the 90% confidence interval) in Figure 1 ; the discontinuity is evident. Discontinuity probability of acceleration at the capacity threshold . | (1) . | (2) . | (3) . | (4) . | (5) . | (6) . | (7) . | (8) . |
---|
. | p|$=$|1 & h|$=$|50 . | p|$=$|1, controls & h|$=$|50 . | p|$=$|2 . | p|$=$|2 & controls . | p|$=$|3 . | p|$=$|3 & controls . | p|$=$|4 . | p|$=$|4 & controls . |
---|
Higher | 0.303 | 0.319 | 0.207 | 0.191 | 0.176 | 0.200 | 0.166 | 0.164 | | (0.071) | (0.083) | (0.035) | (0.044) | (0.042) | (0.048) | (0.041) | (0.049) | Obs. | 682 | 499 | 3,258 | 1,906 | 3,258 | 1,906 | 3,258 | 1,906 | R-squared | 0.070 | 0.128 | 0.397 | 0.447 | 0.398 | 0.447 | 0.399 | 0.451 |
. | (1) . | (2) . | (3) . | (4) . | (5) . | (6) . | (7) . | (8) . |
---|
. | p|$=$|1 & h|$=$|50 . | p|$=$|1, controls & h|$=$|50 . | p|$=$|2 . | p|$=$|2 & controls . | p|$=$|3 . | p|$=$|3 & controls . | p|$=$|4 . | p|$=$|4 & controls . |
---|
Higher | 0.303 | 0.319 | 0.207 | 0.191 | 0.176 | 0.200 | 0.166 | 0.164 | | (0.071) | (0.083) | (0.035) | (0.044) | (0.042) | (0.048) | (0.041) | (0.049) | Obs. | 682 | 499 | 3,258 | 1,906 | 3,258 | 1,906 | 3,258 | 1,906 | R-squared | 0.070 | 0.128 | 0.397 | 0.447 | 0.398 | 0.447 | 0.399 | 0.451 |
This table shows the discontinuity in the probability of acceleration around the capacity-threshold-ranking cutoff. Columns (1)–(8) report the coefficient of |$\mathit{Higher}$| ( |$\gamma )$| of the regression: |$\mathit{Acceleration}=\delta +\gamma \mathit{higher}+f\left( \mathit{Rank-Cutoff} \right)+X'\sigma +\varepsilon $| . The variable |$\mathit{Acceleration}$| equals 1 if the applicant participated in the accelerator; the variable |$\mathit{Higher}$| equals 1 if the applicant ranks higher than the capacity threshold in its generation, and 0 otherwise; and |$f\left( \mathit{Rank-Cutoff} \right)$| is a |$p$| th-degree polynomial of the modified rank (i.e., |$z=\mathit{Rank-Cutoff})$| . The type of specification is indicated at the top of each column, that is, inclusion of controls, the degree of the polynomial used (p), and the bandwidth (h), specified in terms of ranks included around the threshold. If no bandwidth is specified, then the full sample was used. The controls included are Chilean , Female , Capital raised before , Prototype , Young , and generation fixed effects. To conserve space, the estimated coefficients for the polynomial terms are not presented in the table. Robust standard errors are presented in parentheses. * , ** , and *** indicate statistical significance at the 10%, 5%, and 1% level, respectively. As mentioned in Section 1.3 , the manipulation of rankings is hard in this context. Results from two formal tests confirm this notion. First, Figure 2 shows that no discontinuity exists around the capacity threshold in the density of applicants. The McCrary (2008) test for the distribution of applicant scores results in a discontinuity estimate of |$-$| 0.026 with a standard error of 0.10. Second, Figure 3 demonstrates smoothness in observable covariates around the threshold; that is, companies ranking closely on either side of the capacity threshold are similar. We estimate Equation ( 1 ) using pre-determined covariates as dependent variables; Figure 3 plots such estimates against normalized rank. In contrast to the probability of acceleration, in no case can we reject the null hypothesis of no jump at the capacity threshold.  Density of judges’ application scores The figure presents a finely gridded histogram of the normalized application scores. For each applicant, the score of the capacity-threshold-ranking company (of its generation) is subtracted from the application score. Judges score applications from 1 to 10. Average scores range in practice from 1.28 to 8.9. The null hypothesis of no discontinuity in the distribution of the normalized application scores at the threshold cannot be rejected: the |$t$| -statistic from the McCrary test is |$-$| 0.267 (log difference in height is |$-$| 0.026 with standard error of 0.96). The McCrary test uses a local linear regression of the histogram separately on either side of the threshold to accommodate the discontinuity. For additional details, see McCrary (2008) .  Balanced sample around the application capacity threshold The figure shows evidence of a balanced sample near the capacity-threshold-ranking cutoff. Chilean ( Female ) is a dummy that equals 1 if the founder is Chilean (Female); Employees before is the number of workers the start-up reported at the time of application (censored at 10); Capital raised before is a dummy that equals 1 if the start-up fundraised before potential participation in the program; Prototype equals 1 if the start-up has a working prototype/or has one in development; and Young equals 1 if the start-up is less than a year old. All variables are as of the application date. The plots show averages grouped in bins of 10 applicants (dots), and the fitted values and 90% confidence interval from the regression |${\it Outcome}_{s}=\delta +\gamma {\it higher}_{s}+f({\it Rank}_{s}-{\it Cutoff}^{g} )+X_{s}'\mathrm{{\rm M}}+\varepsilon_{s}$| , with each of these variables as outcomes, Higher , a variable that equals 1 if the applicant ranks above the ranking cutoff of the capacity threshold in its generation, and 0 otherwise, and |$f({\it Rank}_{s}-{\it Cutoff}^{g} )$| is a fourth-degree polynomial of the normalized rank. The vertical line represents the ranking cutoff normalized at 0 for the normalized rank. 2.2 Exploiting the informal rule of the entrepreneurship school’s selection processWe use the informal rule in the selection process of the entrepreneurship school to estimate a local average treatment effect of the combination of schooling and basic services on new-venture performance. Our approach is similar to other papers in the literature exploiting de facto discontinuities in selection systems ( Kerr, Lerner, and Schoar 2014 ). Because the probability of schooling changes discontinuously at the 3.6 pitch-day score, the difference in expected outcomes between start-ups on opposite sides of—but sufficiently near—the threshold can thus provide the basis for an unbiased local causal estimate. The main identification concern is that competitors are manipulated around the 3.6 pitch-day score. As mentioned in Section 1.4 , precise manipulation is hard: competitors do not know the identity of judges beforehand, judges independently score each start-up, no judge oversees all the scores, and final pitch-day scores are a linear function of the judges’ scores. Nonetheless, such potential manipulation is of particular concern here because the pitch-day threshold does not correspond to a formal rule in the program (in contrast to the capacity threshold of basic services). In this section, we begin by estimating the size of the discontinuity. We then present evidence that is supportive of no manipulation. Finally, we describe the RDD empirical approach. The estimated discontinuity is sizable and significant. Column 1 (2) of Table 4 presents the estimate of |$\mu$| from a specification of Equation ( 3 ) using a first-degree polynomial, the entire sample, and excluding (including) controls. The coefficient of Column 1 (2) implies that scoring above the informal quality bar of 3.6 in the pitch-day score increases the probability of schooling by 51.9% (50.9%) relative to other competing accelerator participants in the same generation (and controlling for observable differences across start-ups). We plot the corresponding estimated probability of schooling of the estimates in Column 1 (and the 90% confidence interval) in Figure 4 ; the discontinuity is evident. Table 4 shows the discontinuity estimate is robust to different specifications of Equation ( 3 ), including restricting the sample to start-ups scoring 1.5 points around the cutoff (Column 3), restricting the sample to those scoring 1.0 point around the cutoff (Column 4), and using a second-degree polynomial (Column 5).  Fraction of schooled participants The figure shows the average fraction of schooled participants in bins of 0.2 pitch-day scores, and the fitted values and 90% confidence interval from the regression |${\it school}_{\it s}=\tau +\mu\,{\it Above}+{\it g}({{\it Pitch}_{\it day} \ {\it score}}_{\it s}-3.6)+\varepsilon_{\it s}$| , where the outcome variable School is an indicator variable that equals 1 if the participant was schooled; Above is an indicator variable that equals 1 if the participant scored above 3.6 on the pitch day; and |${\it g}({{\it Pitch}_{\it day} \ {\it score}}_{\it s}-3.6)$| is a first-degree polynomial of the pitch-day score. The vertical line represents the informal pitch-day-score cutoff of 3.6. We deploy two main tests for manipulation of start-ups around the 3.6 pitch-day score. First, in Figure 5 , we plot the density of competitors by average pitch-day score and show that no discontinuity exists around the 3.6 cutoff. More formally, we cannot reject the hypothesis of local continuity in the distribution of average pitch-day scores at the cutoff (the |$t$| -statistic from the McCrary test is |$-$| 0.191). This finding is as expected; given its informality, the quality bar threshold is unknown by participants and judges, which further limits the scope for manipulation. Second, we estimate Equation ( 3 ) using predetermined covariates as the dependent variable to verify that the start-ups above and below the cutoff are comparable ex ante. Figure 6 shows evidence of a balanced sample. The only significant difference in covariates regards the indicator variable Capital raised before —participants who scored above 3.6 on the pitch-day are significantly more likely to have secured external financing prior to joining the accelerator. Further inspection reveals, however, that such capital arises from nonspecialized financiers such as family and friends; no difference is evident when restricting the type of capital to Specialized capital raised before (which includes angel, accelerator, or VC fundraising) as shown in the figure. We include the variable Capital raised before as a control in our regressions, and verify that the results are unchanged by its inclusion.  Density of pitch-day scores The figure presents a finely gridded histogram of the pitch-day scores for all participants looking to qualify for the entrepreneurship school. Judges score applications from 0 to 5. In practice, average scores range from 0 to 4.45. The null hypothesis of no discontinuity in the distribution of the normalized application scores at the threshold cannot be rejected: the |$t$| -statistic from the McCrary test is |$-$| 0.191. The McCrary test uses a local linear regression of the histogram separately on either side of the threshold to accommodate the discontinuity. For additional details, see McCrary (2008) .  Balanced sample around the 3.6 pitch-day-score cutoff The figure shows evidence of a balanced sample near the pitch-day cutoff for pitch-day competitors. Chilean ( Female ) is a dummy that equals 1 if the founder is Chilean (Female), Employees before is the number of workers the start-up reported at the time of application (censored at 10), Capital raised before is a dummy that equals 1 if the start-up fundraised before potential participation in the program; Prototype equals 1 if the start-up has a working prototype/or has one in development; and Young equals 1 if the start-up is less than a year old. All variables are as of the application date. Plots show averages grouped in bins of 0.2 in pitch-day score. The plots also show the fitted values and 90% confidence interval of a modified version of the regression in Equation ( 2 ), |${\it Covariate}=\sigma +\omega\,{\it Above}+\check{g}({\it Pitch}_{\it day}\,{\it Score}-3.6 )+\epsilon $| , with each of these variables as outcomes, Above is an indicator variable that equals 1 if the participant scored above 3.6 on the pitch day, and |$\check{g}({\it Pitch}_{\it day}\,{\it Score}-3.6 )$| is a first-degree polynomial of the pitch-day score. The vertical line represents the informal pitch-day-score cutoff of 3.6. Collecting performance measures for all applicants to the accelerator is challenging. The vast majority of applicants are not registered in standard (local or foreign) business data sources. Moreover, the program did not collect performance data on nonparticipating applicants. Therefore, we use two strategies to address this challenge. First, similar to prior research ( Kerr, Lerner, and Schoar 2014 ; Goldfarb, Kirsch, and Miller 2007 ; Hallen, Bingham, and Cohen 2016 ), we hand-collected Web-based performance measures for all applicants. Second, we relied on two surveys: a post-application survey to all Applicants and a post-participation survey to all participants . All outcomes are measured within 4.75 years since potential entry to the accelerator. Greater details about this data-collection strategy and the definitions of each outcome variable can be found in Appendix 3. For our Web-based measures, we searched through the Facebook and LinkedIn (CB Insights) platforms during the first quarter of 2014 (mid-2015). Because participating generations arrived from 2011 through 2013, these metrics represent new venture performance outcomes between 0.75 and 2.75 years (2 and 4 years) since potential entry into the program. Our first survey was sent to all Applicants on October 2014 (between 1.3 and 3.3 years since potential entry). The response rate was 9%. 15 During the first quarter of 2016, the accelerator conducted a second performance-outcome survey (between 2.75 and 4.75 years since entry), focusing only on Participants . The response rate was 72.4%. To distinguish between our three data sources, we identify the Web-based measures with the prefix “Web,” the applicant survey measures with “Survey A,” and the participant survey measures with “Survey P.” For each data source, we constructed five new venture performance proxies: Capital indicator as a binary variable for securing capital after potential participation in the accelerator; the natural logarithm of the value of Capital raised since inception, excluding the seed capital provided by the program to participants; the natural logarithm of the number of Employees ; market Traction as the natural logarithm of the sales (or Facebook likes, in the case of the Web-based measure) during the six preceding months; and a binary variable to indicate Survival . In addition, we were able to construct Valuation as a sixth performance proxy from our survey data sources, which corresponds to the natural logarithm of the pre-money valuation of the start-up. 16 We used logarithmic transformations of continuous outcome variables to mitigate the potential impact of outliers (see also Section 4.3 ). Table 1 presents the summary statistics of the five Web-based outcome measures. Within four years of potential entry into the program, the average applicant is 2.60% likely to secure specialized financing, raises 0.49 (log) dollars in capital, has 0.53 employees, has an average traction of 0.06 (log Facebook likes), and is 21.2% likely to survive. Table 1 also presents summary statistics of the survey-based performance metrics. Within 3.3 years of potential entry into the program, the average applicant-survey respondent is 65.80% likely to secure external funding, raises 6.97 (log) dollars in capital, has 0.54 (log) employees, an average traction of 3.67, and is 61.80% likely to survive. 17 Within 4.75 years of entry into the program, the average participant-survey respondent is 57.90% likely to secure funding, raises 7.12 (log) dollars in capital, has 1.33 (log) employees, an average traction of 6.82, and is 64.10% likely to survive. In Table 5 , we report correlations across our Web-based and survey-based proxies for new venture performance. All (except 2 out of 10) Web-based and survey-based performance metrics have a positive and statistically significant correlation, albeit a small one. This low correlation is likely due to differences across both data-collection systems in the timing of the collection, potential response biases, and variable definitions. Our Applicants survey lags (precedes) our Web-based metrics by 0.7 (0.7) years for Facebook and LinkedIn (CB Insights) data sources, whereas our participants survey lags our Web-based metrics by 2 (0.8) years for Facebook and LinkedIn (CB Insights) data sources. These lags can lead to important measurement differences: Haltiwanger, Jarmin, and Miranda (2013) and Hurst and Pugsley (2011) document large heterogeneity in young (less than 2 years) firm growth, and de Mel, McKenzie, and Woodruff (2014) document time heterogeneity across short- and long-term effects of business-training programs. Moreover, survey respondents may be systematically different from average program participants. For example, successful firms may be more (or less) likely to answer surveys. Indeed, survival rates in our survey-based samples appear particularly high relative to the average survival rates reported by Haltiwanger, Jarmin, and Miranda (2013) and Puri and Zarutskie (2012) from the universe of firms. Furthermore, survey- and Web-based metrics are defined differently. For example, survey respondents include capital from family in their reported fundraising, whereas CB Insights includes only specialized capital sources. Also, whereas survey respondents report the number of employees in their firms, Web-based measures report ranges (see Appendix 3 for additional details). Correlation Web-based and survey-based performance proxies . |
---|
. | Survey A. capital indicator . | Survey P. capital indicator . | Survey A. capital raised . | Survey P. capital raised . |
---|
Web capital indicator | 0.04 | 0.17 | | | | (0.53) | (0.05) | | | Web capital raised | | | 0.11 | 0.34 | | | | (0.05) | (0.00) | Observations | 319 | 145 | 319 | 145 | | | Survey A. employees | Survey P. employees | Survey A. traction | Survey P. traction | Web employees | 0.13 | 0.23 | | | | (0.02) | (0.01) | | | Web traction | | | 0.10 | 0.20 | | | | (0.07) | (0.00) | Observations | 319 | 145 | 319 | 145 | | | Survey A. survival | Survey P. survival | | | Web survival | 0.21 | –0.02 | | | | (0.00) | (0.80) | | | Observations | 319 | 145 | | |
. |
---|
. | Survey A. capital indicator . | Survey P. capital indicator . | Survey A. capital raised . | Survey P. capital raised . |
---|
Web capital indicator | 0.04 | 0.17 | | | | (0.53) | (0.05) | | | Web capital raised | | | 0.11 | 0.34 | | | | (0.05) | (0.00) | Observations | 319 | 145 | 319 | 145 | | | Survey A. employees | Survey P. employees | Survey A. traction | Survey P. traction | Web employees | 0.13 | 0.23 | | | | (0.02) | (0.01) | | | Web traction | | | 0.10 | 0.20 | | | | (0.07) | (0.00) | Observations | 319 | 145 | 319 | 145 | | | Survey A. survival | Survey P. survival | | | Web survival | 0.21 | –0.02 | | | | (0.00) | (0.80) | | | Observations | 319 | 145 | | |
The table presents correlations across Web-based and survey-based venture performance metrics. |$^{*}$| , |$^{**}$| , and |$^{***}$| indicate statistical significance at the 10%, 5%, and 1% level, respectively. 4.1 The effect of basic accelerator services on new venture performanceTable 6 summarizes the estimated effects of basic accelerator services on all outcome variables. Reported standard errors are heteroscedasticity robust. Columns 1 and 2 report ordinary least-squares (OLS) estimates comparing participants and nonparticipants, with and without controlling for covariates. Participants consistently outperform rejected applicants: results in Column 2 indicate participants are 5.6% more likely than nonparticipants to raise capital after the program. Venture performance and basic acceleration services . | (1) . | (2) . | (3) . | (4) . | (5) . | (6) . | (7) . | (8) . | (9) . | (10) . |
---|
Estimate . | OLS . | OLS & controls . | p|$=$|1 & h|$=$|50 . | p|$=$|1, controls & h|$=$|50 . | p|$=$|2 . | p|$=$|2 & controls . | p|$=$|3 . | p|$=$|3 & controls . | p|$=$|4 . | p|$=$|4 & controls . |
---|
Web capital indicator | 0.062 | 0.056 | –0.005 | –0.047 | 0.037 | 0.062 | 0.065 | 0.088 | 0.049 | 0.056 | | (0.011) | (0.012) | (0.089) | (0.119) | (0.071) | (0.110) | (0.098) | (0.116) | (0.103) | (0.142) | Web capital raised | 1.215 | 1.019 | 0.956 | 0.355 | 0.439 | 0.106 | 0.160 | 0.056 | 0.117 | 0.108 | | (0.159) | (0.168) | (1.244) | (1.501) | (0.999) | (1.454) | (1.443) | (1.558) | (1.497) | (1.876) | Web traction | 0.079 | 0.044 | –0.235 | –0.405 | 0.021 | 0.006 | –0.053 | –0.089 | –0.039 | –0.108 | | (0.018) | (0.020) | (0.184) | (0.229) | (0.125) | (0.190) | (0.206) | (0.217) | (0.203) | (0.244) | Web employees | 0.655 | 0.315 | –1.704 | –2.615 | –0.674 | –1.514 | –1.255 | –1.974 | –1.385 | –2.880 | | (0.112) | (0.131) | (1.301) | (1.709) | (0.911) | (1.462) | (1.275) | (1.506) | (1.375) | (2.050) | Web survival | 0.305 | 0.250 | –0.037 | –0.136 | 0.199 | 0.284 | 0.282 | 0.314 | 0.272 | 0.426 | | (0.021) | (0.023) | (0.233) | (0.265) | (0.165) | (0.218) | (0.225) | (0.223) | (0.238) | (0.284) | Survey A. capital indicator | 0.163 | 0.181 | –0.352 | –1.048 | –0.456 | –1.392 | –0.328 | –0.925 | –0.593 | –1.607 | | (0.054) | (0.067) | (0.342) | (0.818) | (0.701) | (1.764) | (0.571) | (1.010) | (0.762) | (2.118) | Survey A. capital raised | 2.477 | 2.829 | –4.067 | –11.296 | –5.207 | –14.214 | –4.273 | –10.467 | –6.460 | –16.954 | | (0.601) | (0.753) | (3.845) | (8.811) | (7.919) | (19.055) | (6.598) | (11.675) | (8.566) | (23.435) | Survey A. valuation | 1.068 | 2.058 | 1.091 | –2.250 | –0.682 | 0.055 | 1.110 | –0.776 | 1.672 | –6.340 | | (0.791) | (0.990) | (4.211) | (7.765) | (8.648) | (13.988) | (7.315) | (10.354) | (8.809) | (17.965) | Survey A. traction | 0.288 | 0.249 | –5.102 | –10.221 | –6.281 | –13.333 | –6.459 | –11.140 | –6.842 | –15.270 | | (0.569) | (0.696) | (3.208) | (8.211) | (6.998) | (16.935) | (5.837) | (10.847) | (7.350) | (20.882) | Survey A. employees | 0.092 | 0.161 | –0.575 | –2.123 | –1.274 | –3.610 | –0.967 | –2.541 | –1.319 | –3.454 | | (0.099) | (0.120) | (0.656) | (1.530) | (1.376) | (3.988) | (1.116) | (2.244) | (1.461) | (4.307) | Survey A. survival | 0.178 | 0.197 | –0.396 | –0.114 | 0.422 | 0.545 | 0.187 | 0.357 | 0.070 | 0.549 | | (0.055) | (0.069) | (0.348) | (0.679) | (0.640) | (0.954) | (0.540) | (0.674) | (0.654) | (1.077) |
. | (1) . | (2) . | (3) . | (4) . | (5) . | (6) . | (7) . | (8) . | (9) . | (10) . |
---|
Estimate . | OLS . | OLS & controls . | p|$=$|1 & h|$=$|50 . | p|$=$|1, controls & h|$=$|50 . | p|$=$|2 . | p|$=$|2 & controls . | p|$=$|3 . | p|$=$|3 & controls . | p|$=$|4 . | p|$=$|4 & controls . |
---|
Web capital indicator | 0.062 | 0.056 | –0.005 | –0.047 | 0.037 | 0.062 | 0.065 | 0.088 | 0.049 | 0.056 | | (0.011) | (0.012) | (0.089) | (0.119) | (0.071) | (0.110) | (0.098) | (0.116) | (0.103) | (0.142) | Web capital raised | 1.215 | 1.019 | 0.956 | 0.355 | 0.439 | 0.106 | 0.160 | 0.056 | 0.117 | 0.108 | | (0.159) | (0.168) | (1.244) | (1.501) | (0.999) | (1.454) | (1.443) | (1.558) | (1.497) | (1.876) | Web traction | 0.079 | 0.044 | –0.235 | –0.405 | 0.021 | 0.006 | –0.053 | –0.089 | –0.039 | –0.108 | | (0.018) | (0.020) | (0.184) | (0.229) | (0.125) | (0.190) | (0.206) | (0.217) | (0.203) | (0.244) | Web employees | 0.655 | 0.315 | –1.704 | –2.615 | –0.674 | –1.514 | –1.255 | –1.974 | –1.385 | –2.880 | | (0.112) | (0.131) | (1.301) | (1.709) | (0.911) | (1.462) | (1.275) | (1.506) | (1.375) | (2.050) | Web survival | 0.305 | 0.250 | –0.037 | –0.136 | 0.199 | 0.284 | 0.282 | 0.314 | 0.272 | 0.426 | | (0.021) | (0.023) | (0.233) | (0.265) | (0.165) | (0.218) | (0.225) | (0.223) | (0.238) | (0.284) | Survey A. capital indicator | 0.163 | 0.181 | –0.352 | –1.048 | –0.456 | –1.392 | –0.328 | –0.925 | –0.593 | –1.607 | | (0.054) | (0.067) | (0.342) | (0.818) | (0.701) | (1.764) | (0.571) | (1.010) | (0.762) | (2.118) | Survey A. capital raised | 2.477 | 2.829 | –4.067 | –11.296 | –5.207 | –14.214 | –4.273 | –10.467 | –6.460 | –16.954 | | (0.601) | (0.753) | (3.845) | (8.811) | (7.919) | (19.055) | (6.598) | (11.675) | (8.566) | (23.435) | Survey A. valuation | 1.068 | 2.058 | 1.091 | –2.250 | –0.682 | 0.055 | 1.110 | –0.776 | 1.672 | –6.340 | | (0.791) | (0.990) | (4.211) | (7.765) | (8.648) | (13.988) | (7.315) | (10.354) | (8.809) | (17.965) | Survey A. traction | 0.288 | 0.249 | –5.102 | –10.221 | –6.281 | –13.333 | –6.459 | –11.140 | –6.842 | –15.270 | | (0.569) | (0.696) | (3.208) | (8.211) | (6.998) | (16.935) | (5.837) | (10.847) | (7.350) | (20.882) | Survey A. employees | 0.092 | 0.161 | –0.575 | –2.123 | –1.274 | –3.610 | –0.967 | –2.541 | –1.319 | –3.454 | | (0.099) | (0.120) | (0.656) | (1.530) | (1.376) | (3.988) | (1.116) | (2.244) | (1.461) | (4.307) | Survey A. survival | 0.178 | 0.197 | –0.396 | –0.114 | 0.422 | 0.545 | 0.187 | 0.357 | 0.070 | 0.549 | | (0.055) | (0.069) | (0.348) | (0.679) | (0.640) | (0.954) | (0.540) | (0.674) | (0.654) | (1.077) |
This table reports the effects of basic acceleration services (cash and coworking space) on venture performance. Estimates are based on the regression |$\mathit{outcome}_{s}=\pi +\beta \mathit{acceleration}_{s}+\breve{f}\left( \mathit{Rank}_{s}-\mathit{Cutoff}^{g} \right)+X_{s}'\rho +\epsilon_{s}$| , where |$\mathit{Acceleration}$| is a variable that equals 1 if the applicant participated in the accelerator. The outcome variable is specified in the title columns of each row. The type of specification is indicated at the top of each column, that is, inclusion of controls, the degree of the polynomial used (p), and the bandwidth (h), specified in terms of ranks included around the threshold. If no bandwidth is specified, then the full sample was used. For the OLS estimate, the polynomials of the normalized ranking (i.e., |$\breve{f}\left( \mathit{Rank}_{s}-\mathit{Cutoff}^{g} \right))$| are excluded from the estimation. For the RDD estimate, |$\mathit{acceleration}$| is instrumented using |$\mathit{Higher}$| , a variable that equals 1 if the applicant ranks higher than the capacity threshold in its generation. To conserve space, the estimated coefficients for the constant and the polynomial terms in the second stage are not presented in the table. The controls included are Chilean , Female , Capital raised before , Prototype , and Young and generation fixed effects. Robust standard errors are presented in parentheses. |$^{*}$| , |$^{**}$| , and |$^{***}$| indicate statistical significance at the 10%, 5%, and 1% level, respectively. Columns 3–10 report estimates using the fuzzy RDD with different combinations of bandwidths ( |$h$| ) and polynomial degrees ( |$p)$| , following the same structure used in Table 3 . In contrast to OLS estimates, RDD specifications result in nonsignificant coefficients across all outcome variables, and generally smaller point estimates, which vary significantly across specifications in terms of both magnitude and sign. In an unreported analysis, we verify that results are the same if we allow polynomials to differ on either side of the threshold, excluding observations from generations 1 and 2 and participants in the entrepreneurship school. We also find no evidence of heterogeneity in the effect across several covariates such as gender, nationality, and age. Two interpretations are possible for the negative differences between the RDD and the OLS effects, because RDD estimates a local average treatment effect (cf. Lee and Lemieux 2010 ). Under the assumption of underlying heterogeneity in the treatment effect, these negative differences can reflect lower-than-average returns of basic services in the subpopulation that the selection rule randomizes into the program. Under the assumption that no such heterogeneity exists, the negative difference can also reflect the program’s ability to screen applicants. 4.2 The effect of entrepreneurship schooling on new venture performanceTable 7 reports the coefficients of each outcome variable regarding the estimated effect of participating in the entrepreneurship school. Column 1 (2) reports OLS estimates without (with) controls. Column 1 shows that schooled participants are 9.1% more likely to raise capital after the program. Column 2 shows fundraising ability prior to schooling does not explain the increase in fundraising performance—results continue to hold once we control for Capital raised before . Venture performance and the entrepreneurship school . | (1) . | (2) . | (3) . | (4) . | (5) . | (6) . | (7) . |
---|
Estimate . | OLS . | OLS & controls . | p|$=$|1 . | p|$=$|1 & controls . | p|$=$|1 & controls & h|$=$|1.5 . | p|$=$|1 & h|$=$|1 . | p|$=$|2 & controls . |
---|
Web capital indicator | 0.091 | 0.088 | 0.210 | 0.207 | 0.250 | 0.312 | 0.346 | | (0.052) | (0.052) | (0.118) | (0.115) | (0.161) | (0.189) | (0.253) | Web capital raised | 1.633 | 1.560 | 3.034 | 3.008 | 4.382 | 6.019 | 6.345 | | (0.745) | (0.683) | (1.576) | (1.504) | (2.175) | (2.667) | (3.374) | Web traction | 0.142 | 0.134 | 0.238 | 0.229 | 0.354 | 0.413 | 0.490 | | (0.077) | (0.063) | (0.128) | (0.115) | (0.159) | (0.202) | (0.243) | Web employees | 0.379 | 0.400 | 1.985 | 1.890 | 2.280 | 2.891 | 2.760 | | (0.384) | (0.349) | (1.086) | (1.124) | (1.374) | (1.360) | (2.045) | Web survival | 0.100 | 0.066 | 0.087 | 0.107 | 0.340 | 0.335 | 0.257 | | (0.071) | (0.068) | (0.183) | (0.180) | (0.255) | (0.278) | (0.363) | Survey P. capital indicator | 0.329 | 0.346 | 0.455 | 0.422 | 0.243 | 0.217 | 0.200 | | (0.080) | (0.080) | (0.199) | (0.210) | (0.316) | (0.296) | (0.403) | Survey P. capital raised | 4.246 | 4.501 | 6.253 | 5.739 | 3.551 | 3.613 | 3.130 | | (1.038) | (1.031) | (2.533) | (2.661) | (3.890) | (3.711) | (4.983) | Survey P. valuation | 2.411 | 2.218 | 5.520 | 4.984 | 8.794 | 7.972 | 13.752 | | (1.436) | (1.455) | (3.284) | (3.497) | (5.028) | (4.590) | (7.344) | Survey P. traction | 1.345 | 1.399 | 4.226 | 3.662 | 2.118 | –0.438 | 0.324 | | (1.197) | (1.223) | (2.733) | (2.868) | (4.183) | (3.848) | (5.202) | Survey P. employees | 0.548 | 0.580 | 0.871 | 0.693 | 0.897 | 0.779 | 1.009 | | (0.250) | (0.252) | (0.550) | (0.581) | (0.787) | (0.749) | (1.008) | Survey P. survival | 0.134 | 0.143 | –0.044 | –0.142 | –0.082 | –0.050 | –0.007 | | (0.088) | (0.090) | (0.219) | (0.232) | (0.316) | (0.303) | (0.414) |
. | (1) . | (2) . | (3) . | (4) . | (5) . | (6) . | (7) . |
---|
Estimate . | OLS . | OLS & controls . | p|$=$|1 . | p|$=$|1 & controls . | p|$=$|1 & controls & h|$=$|1.5 . | p|$=$|1 & h|$=$|1 . | p|$=$|2 & controls . |
---|
Web capital indicator | 0.091 | 0.088 | 0.210 | 0.207 | 0.250 | 0.312 | 0.346 | | (0.052) | (0.052) | (0.118) | (0.115) | (0.161) | (0.189) | (0.253) | Web capital raised | 1.633 | 1.560 | 3.034 | 3.008 | 4.382 | 6.019 | 6.345 | | (0.745) | (0.683) | (1.576) | (1.504) | (2.175) | (2.667) | (3.374) | Web traction | 0.142 | 0.134 | 0.238 | 0.229 | 0.354 | 0.413 | 0.490 | | (0.077) | (0.063) | (0.128) | (0.115) | (0.159) | (0.202) | (0.243) | Web employees | 0.379 | 0.400 | 1.985 | 1.890 | 2.280 | 2.891 | 2.760 | | (0.384) | (0.349) | (1.086) | (1.124) | (1.374) | (1.360) | (2.045) | Web survival | 0.100 | 0.066 | 0.087 | 0.107 | 0.340 | 0.335 | 0.257 | | (0.071) | (0.068) | (0.183) | (0.180) | (0.255) | (0.278) | (0.363) | Survey P. capital indicator | 0.329 | 0.346 | 0.455 | 0.422 | 0.243 | 0.217 | 0.200 | | (0.080) | (0.080) | (0.199) | (0.210) | (0.316) | (0.296) | (0.403) | Survey P. capital raised | 4.246 | 4.501 | 6.253 | 5.739 | 3.551 | 3.613 | 3.130 | | (1.038) | (1.031) | (2.533) | (2.661) | (3.890) | (3.711) | (4.983) | Survey P. valuation | 2.411 | 2.218 | 5.520 | 4.984 | 8.794 | 7.972 | 13.752 | | (1.436) | (1.455) | (3.284) | (3.497) | (5.028) | (4.590) | (7.344) | Survey P. traction | 1.345 | 1.399 | 4.226 | 3.662 | 2.118 | –0.438 | 0.324 | | (1.197) | (1.223) | (2.733) | (2.868) | (4.183) | (3.848) | (5.202) | Survey P. employees | 0.548 | 0.580 | 0.871 | 0.693 | 0.897 | 0.779 | 1.009 | | (0.250) | (0.252) | (0.550) | (0.581) | (0.787) | (0.749) | (1.008) | Survey P. survival | 0.134 | 0.143 | –0.044 | –0.142 | –0.082 | –0.050 | –0.007 | | (0.088) | (0.090) | (0.219) | (0.232) | (0.316) | (0.303) | (0.414) |
This table reports the effects of entrepreneurship schooling (bundled with the basic services) on venture performance. Estimates are based on the regression |$\mathit{Outcome}_{s}=\pi +\beta \mathit{school}_{s}+\breve{g}( {\mathit{Pitch}_{\mathit{day}}\mathit{score}}_{s}-3.6 )+Z_{s}'\varphi +\epsilon_{s}$| , where |$\mathit{school}_{s}$| is a variable that equals 1 if the participant was selected into the entrepreneurship school and |$g( \mathit{Pitch}_{\mathit{day}}\mathit{Score}-3.6)$| is a |$p$| th-degree polynomial of the normalized pitch-day score (i.e., pitch-day score minus the 3.6 cutoff). The outcome variable is specified in the title columns of each row, and the type of estimate is specified at the top of each column (i.e., the degree of the polynomial used (p), the bandwidth (h), and the inclusion of controls). Columns (5) and (6) include different bandwidth specifications (h) for pitch-day-score ranges of 2.1–5.0 (h |$=$| 1.5) and 2.6–4.5 (h |$=$| 1), respectively. For the OLS estimate, the polynomials are excluded from the estimation. For the RDD estimate, |$\mathit{School}_{s}$| is instrumented using |$\mathit{Above}$| , a variable that equals 1 if the participant scored 3.6 or higher on the pitch day. To conserve space, the estimated coefficients for the constant and the polynomial terms are not presented in the table. The controls included are Capital raised before and generation fixed effects. Robust standard errors are presented in parentheses. |$^{*}$| , |$^{**}$| , and |$^{***}$| indicate statistical significance at the 10%, 5%, and 1% level, respectively. Columns 3–7 report estimates using different specifications of the fuzzy RDD, with and without controlling for covariates, and using different combinations of bandwidths ( |$h)$| and polynomial degrees ( |$p)$| , following the same structure used in Table 4 . Evidence suggests positive and large causal effects of the entrepreneurship school. The first rows of Column (3) show that schooling increases the probability of fundraising by 21.0%, the amount of capital raised by a factor of three, market traction by 23.8%, the number of employees by a factor of two, and valuations by a factor of five. Scale effects partly explain the large magnitudes. For example, a twofold increase in employees roughly means that firms hire one more employee (from an unconditional average of 0.9 to 1.8). Similarly, a threefold increase in capital raised means firms increase fundraising from an unconditional average of 37,000 USD to 112,000 USD. Controlling for observable covariates (Column 4) only marginally affects the statistical significance, and, importantly, does not affect the magnitude of the estimated treatment effect. Table 8 shows that the economic magnitude of the entrepreneurship schooling effect is similar across different proxies of a given outcome. For example, Column 3 shows that entrepreneurship schooling increases the likelihood of fundraising by 0.29 and 0.39 standard deviations, respectively, according to the Web-based metric (Web Capital Indicator) and survey-based metric (Survey P. Capital Indicator). Similarly, the same column shows that, based on Web-based (survey-based) metrics, entrepreneurship schooling increases the amount of capital raised by 0.30 (0.43) standard deviations, market traction by 0.31 (0.30) standard deviations, and employees by 0.34 (0.28) standard deviations. Table 8 reports normalized coefficient estimates following the same structure used in Table 7 . Summary economic magnitude of entrepreneurship schooling effect . | (1) . | (2) . | (3) . | (4) . | (5) . | (6) . | (7) . |
---|
Estimate . | OLS . | OLS & controls . | p|$=$|1 . | p|$=$|1 & controls . | p|$=$|1 & controls & h|$=$|1.5 . | p|$=$|1 & h|$=$|1 . | p|$=$|2 & controls . |
---|
Web capital indicator | 0.126 | 0.122 | 0.290 | 0.286 | 0.350 | 0.433 | 0.478 | Web capital raised | 0.159 | 0.152 | 0.295 | 0.293 | 0.429 | 0.587 | 0.617 | Web traction | 0.184 | 0.174 | 0.307 | 0.296 | 0.457 | 0.531 | 0.633 | Web employees | 0.065 | 0.069 | 0.342 | 0.325 | 0.405 | 0.529 | 0.475 | Web survival | 0.083 | 0.055 | 0.072 | 0.088 | 0.286 | 0.288 | 0.212 | Survey P. capital indicator | 0.285 | 0.300 | 0.394 | 0.366 | 0.213 | 0.194 | 0.173 | Survey P. capital raised | 0.291 | 0.309 | 0.429 | 0.394 | 0.246 | 0.255 | 0.215 | Survey P. valuation | 0.149 | 0.137 | 0.341 | 0.308 | 0.552 | 0.512 | 0.849 | Survey P. traction | 0.094 | 0.098 | 0.295 | 0.256 | 0.151 | –0.032 | 0.023 | Survey P. employees | 0.105 | 0.116 | 0.284 | 0.222 | 0.168 | 0.395 | 0.126 | Survey P. survival | 0.119 | 0.127 | –0.039 | –0.127 | –0.075 | –0.046 | –0.007 |
. | (1) . | (2) . | (3) . | (4) . | (5) . | (6) . | (7) . |
---|
Estimate . | OLS . | OLS & controls . | p|$=$|1 . | p|$=$|1 & controls . | p|$=$|1 & controls & h|$=$|1.5 . | p|$=$|1 & h|$=$|1 . | p|$=$|2 & controls . |
---|
Web capital indicator | 0.126 | 0.122 | 0.290 | 0.286 | 0.350 | 0.433 | 0.478 | Web capital raised | 0.159 | 0.152 | 0.295 | 0.293 | 0.429 | 0.587 | 0.617 | Web traction | 0.184 | 0.174 | 0.307 | 0.296 | 0.457 | 0.531 | 0.633 | Web employees | 0.065 | 0.069 | 0.342 | 0.325 | 0.405 | 0.529 | 0.475 | Web survival | 0.083 | 0.055 | 0.072 | 0.088 | 0.286 | 0.288 | 0.212 | Survey P. capital indicator | 0.285 | 0.300 | 0.394 | 0.366 | 0.213 | 0.194 | 0.173 | Survey P. capital raised | 0.291 | 0.309 | 0.429 | 0.394 | 0.246 | 0.255 | 0.215 | Survey P. valuation | 0.149 | 0.137 | 0.341 | 0.308 | 0.552 | 0.512 | 0.849 | Survey P. traction | 0.094 | 0.098 | 0.295 | 0.256 | 0.151 | –0.032 | 0.023 | Survey P. employees | 0.105 | 0.116 | 0.284 | 0.222 | 0.168 | 0.395 | 0.126 | Survey P. survival | 0.119 | 0.127 | –0.039 | –0.127 | –0.075 | –0.046 | –0.007 |
This table reports the effects of entrepreneurship schooling (bundled with the basic services) on venture performance. Reported coefficients correspond to normalized betas of results in Table 7 , which can be interpreted as standard deviation changes in the outcome variables. Estimates are based on the regression |$\mathit{Outcome}_{s}=\pi +\beta \mathit{school}_{s}+\breve{g}( \mathit{Pitch}_{\mathit{day}}\mathit{score}_{s}-3.6 )+Z_{s}'\varphi +\epsilon_{s}$| , where |$\mathit{School}_{s}$| is a variable that equals 1 if the participant was selected into the entrepreneurship school and |$g( \mathit{Pitch}_{\mathit{day}}\mathit{Score}-3.6 )$| is a |$p$| th-degree polynomial of the normalized pitch-day score (i.e., pitch-day score minus the 3.6 cutoff). The outcome variable is specified in the title columns of each row, and the type of estimate is specified at the top of each column (i.e., the degree of the polynomial used (p), the bandwidth (h), and the inclusion of controls). Columns (5) and (6) include different bandwidth specifications (h) for pitch-day-score ranges of 2.1–5.0 (h |$=$| 1.5) and 2.6–4.5 (h |$=$| 1), respectively. For the OLS estimate, the polynomials are excluded from the estimation. For the RDD estimate, |$\mathit{School}_{s}$| is instrumented using |$\mathit{Above}$| , a variable that equals 1 if the participant scored 3.6 or higher on the pitch day. To conserve space, the estimated coefficients for the constant and the polynomial terms are not presented in the table. The controls included are Capital raised before and generation fixed effects. Robust standard errors are presented in parentheses. |$^{*}$| , |$^{**}$| , and |$^{***}$| indicate statistical significance at the 10%, 5%, and 1% level, respectively. The results in Columns 5–7 in Table 7 show that the findings are also qualitatively robust to using different bandwidths and polynomials in the RDD estimation (following the same structure as Table 4 ). They also continue to hold when we restrict the sample to the last two generations (for which data collection may be more accurate), and are stronger for companies in industries that require a Web presence, such as e-commerce, media, mobile, social media, and social networks. This finding is consistent with the notion that start-ups from the “new economy” grow faster than those from traditional industries, enabling us to observe performance differences earlier on. Finally, we find no evidence that the entrepreneurship school affects new venture survival (as measured by Web- or survey-based metrics), and the implied economic magnitude of the point estimates (Column 3, Table 8 ) is very small. Consistent with arguments by Maurer and Ebers (2006) , this finding may reflect the notion that start-ups can survive through the persistence of their founders, but fundraising and growth are more likely to rely on entrepreneurial capital. The positive difference between the OLS and RDD estimates ( Table 7 , Columns 2 and 3, respectively), suggests the RDD recovers the treatment effect for a subpopulation of start-ups with relatively high returns to entrepreneurship schooling. Such positive difference is a common result in the education literature, particularly in papers that exploit supply-side innovations for identification (such as selection processes based on qualification scores). In these papers, the instrumental variables estimates of the return to schooling typically exceed the corresponding OLS estimates, often by 20% or more ( Card 2001 ). The leading explanation proposed for this pattern is that supply-side innovations are most likely to affect the schooling choices of individuals who would otherwise have relatively low schooling because they face higher than average access costs. In that case, the “local average treatment effect” ( Imbens and Angrist 1994 ) reasoning suggests the instrumental variable estimator would yield estimated returns to schooling above the average marginal return to schooling in the population, and potentially above the corresponding OLS estimates. This explanation is also likely to hold in our setting. Start-ups that score close to the qualification threshold (“close calls”) are less likely to secure schooling elsewhere relative to start-ups that, during the pitch-day, are deemed to have more potential, partly because they have more entrepreneurial capital to begin with and are thus scored highly by judges (“high scorers”). Hence, whereas high scorers may only marginally benefit from schooling, close calls are likely to benefit substantially, as lack of entrepreneurial capital can explain their worse performance during the pitch-day. An alternative explanation for the positive difference, which is less likely to resonate in our setting, is based on the additional assumption that the treatment effect of schooling is homogeneous; that is, that high scorers and close calls benefit equally from schooling. Under this assumption, the positive difference between the RDD and the OLS estimates would suggest that the selection process for the entrepreneurship school picks lower-potential rather than higher-potential start-ups, and thus that the OLS is biased downward rather than upward. This alternative interpretation is unlikely to hold in our setting for two reasons. First, the assumption that treatment effects of entrepreneurship schooling are homogenous across start-ups is not realistic. Not only has the education literature shown evidence of heterogeneous effects from schooling (cf. Card 2001 ), prior work on accelerators has emphasized how the treatment effect of these programs is likely to be heterogeneous. In particular, accelerators appear to be associated with both the accelerated success of good start-ups and the accelerated failure of bad ones ( Yu 2016 ; Smith and Hannigan 2015 ). Second, while a criterion to select contestants based on their need for an “entrepreneurial capital subsidy” may be reasonable in certain situations, this criterion is not used in Start-Up Chile. Indeed, the Start-Up Chile selection process is based on a pitch competition , where judges are required to select the apparent highest performers, and no directives exist that guide staff to pick the weaker start-ups. 4.3 Additional robustness testsIn this section, we use complementary tests to provide suggestive evidence against six potential methodological concerns including identification issues, influential observations, potential demotivation effects on pitch competition losers, multiple types of survey- and Web-reporting biases, and potential schooling effects on reporting (rather than on real outcomes). One first concern is that the RDD estimate is a consequence of the selection process and not of schooling; that is, that the pitch-day score, not the entrepreneurship school, explains the superior performance of schooled ventures. Against this concern, the results in Figures 5 and 6 show little evidence of a discontinuity in the scores or in the covariates at the 3.6 threshold. Instead, we would expect to see a jump in sample characteristics if breaks in observable venture quality explained the results. Three additional tests provide further supportive evidence against this concern. First, Appendix Figure 4.1 shows that the dispersion of pitch-day scores across judges looks similar for ventures closely above and below the threshold. If a break in unobservable characteristics explained the RDD estimates, we would instead expect less dispersion of pitch-day scores (i.e., less disagreement among judges) immediately above the threshold. Second, Appendix Table 4.1 shows that pitch-day scores are generally uninformative about performance. In regressions projecting outcomes onto pitch-day scores, pitch-day scores are never significant once we control for |$Above$| . By contrast, if the selection process was driving the RDD estimates, we would expect the score to be informative about outcomes. The lack of pitch-day score predictability is not surprising; identifying high-quality start-ups is no easy task, even for expert investors who spend much more time and effort on due diligence than the judging time in the pitch competition (e.g., Kerr, Nanda, and Rhodes-Kropf 2014 ). Finally, Appendix Table 4.1 shows that |$Above$| only predicts performance among schooled entrepreneurs. If pitch-day scores fully explained the RDD estimates, |$Above$| should also predict the performance for nonschooled entrepreneurs. Together, these additional results suggest systematic quality differences between firms closely above and below the 3.6 pitch-day threshold are unlikely to drive RDD results. A second potential concern is that influential observations drive our results, as outliers typically explain aggregate performance in early-stage financiers. To test whether such potential outliers drive our results, we ran multiple “leave-one-out” regressions that exclude observations from the estimation one by one. We classify observations as influential if their removal changes the significance of our results; that is, if the |$p$| value of the estimate falls below 10%. Appendix Table 4.2 confirms that outliers are not the main explanation for the estimated effects and that no influential observations exist for four out of six of the main results in Table 7 . For the remaining two results (Web Capital Indicator and Web Employees), the removal of influential observations only slightly changes the significance (from 0.074 to 0.115, and 0.095 to 0.160), and does not dramatically change the point estimate. A third potential concern is that the failure to enter the entrepreneurship school may demotivate pitch-day competition losers, inducing a relative increase in the performance of schooled participants. Against this possibility, Table 7 shows that schooling has no effect on venture survival. This finding implies that if any demotivation was taking place, it would not be enough to fully discourage entrepreneurs from pushing their ventures further. 18 Appendix Table 4.3 presents further supportive evidence against this concern. We restrict the sample to losers and project venture outcomes based on pitch-day scores, |$Above$| , and the fraction of pitch-day winners that are in the same reference group as the start-up. Panel A (B) uses the ventures in the same industry (and location) of the start-up as a reference group. The table shows that the performance of losers does not correlate with the fraction of winners. If demotivation were the main explanation behind the findings, we would expect a negative relation between these two variables. If most similar start-ups are rejected, then the start-up is likely to blame the industry for the loss rather than its own individual performance. Finally, Appendix Figure 4.2 shows no decreasing pattern in performance across pitch-day scores below 3.6. If demotivation were the main explanation behind the effect, we would expect a dip in survival and performance to the left of the threshold. A fourth potential concern is that schooling increases the probability of Web and survey reporting, which could potentially bias the RDD estimates (e.g., Drexler, Fischer, and Schoar 2014 ). Against this possibility, the survey response rate is the same across schooled and nonschooled ventures (the estimated difference is 0.09 and the |$t$| -statistic is 1.18). In addition, although Start-Up Chile encourages the use of AngelList for communication purposes program-wide, the entrepreneurship school gives no additional nudge to open a profile on this site. Consistent with this notion, schooled and nonschooled participants were equally likely to have an AngelList profile in December 2013 (the estimated difference is 0.01; the |$t$| -statistic is 0.10). We refined this test using information from Google Insights, for which we timed Web activity (we downloaded information on Web activity per semester based on company name). We found that schooled and nonschooled participants have a similar Web presence immediately after their potential participation in the program (with a difference of 4.59 and a |$t$| -statistic of 0.80). A related concern is that schooled ventures may over-report performance in surveys, which would positively bias RDD estimates. For example, schooled ventures may want to exaggerate how well their firms benefited from the program. This concern is mitigated by results using Web sources, where entrepreneurs have both less control over what is reported and the potential for misreporting to be heavily punished. Although participants have the freedom to choose whether to open up profiles on social media platforms (e.g., Facebook), industry providers, such as CB Insights, sweep all Web sites collecting nonself-reported news about start-ups, which founders are less likely to be able to fully manipulate. Reputational considerations also likely mitigate rampant lying; misreporting fundraising can seriously affect start-ups’ chances of securing fundraising in the future. Relatedly, RDD estimates of survey-based metrics may also be positively biased if better applicants are more likely to answer surveys and judges’ scores reflect quality. This concern is mitigated by the little information content provided by pitch-day scores; whereas the best companies may be more likely to answer surveys, evidence suggests that judges do not appear to consistently assign them scores above 3.6 (see Appendix Table 4.1). Finally, RDD estimates may also be positively biased if schooling induces better record keeping, even if outcomes remain largely unchanged. We test for this potential source of bias by examining survey-reporting errors and their association with schooling (cf. Drexler, Fischer, and Schoar 2014 ; Berge, Bjorvatn, and Tungodden 2015 ; de Mel, McKenzie, and Woodruff 2014 ). We find that the propensity to err is not statistically different across schooled and nonschooled participants (estimated difference is 0.02 and |$t$| -statistic is |$-$| 0.99). 19 Our findings show evidence that entrepreneurship schooling, bundled with the basic services of cash and coworking space, appears to lead to significantly higher new venture performance within the first 4.75 years since entry to the accelerator. By contrast, basic services alone do not appear to have an effect on new venture performance. This is the first paper to provide this type of evidence for business accelerators, which have emerged as a new institutional form to support early stage start-ups. While nascent academic research has begun exploring this growing institutional form, very little rigorous evidence exists on the effect of business accelerators on new venture performance, and on distinguishing which of the services provided by these programs add value. The pattern of results suggests that entrepreneurial capital is a key factor for new venture performance. This pattern is also consistent with the well-established findings on how interventions that combine finance (especially grants) and business training are more effective in supporting subsistence businesses than finance alone (e.g., Bandiera et al. 2013 ). Moreover, the implied magnitude of the findings is also similar to prior papers on business training interventions. For example, Calderon, Cunha, and De Giorgi (2013) and de Mel, McKenzie, and Woodruff (2014) find a 20% and 41% increase in sales (within 12 and 8 months). These results are similar to our estimate of a 23.8% increase in Web-based venture traction (and are within the confidence interval of our survey-based results; see Column 3 in Table 7 ). Our estimates on employees are close to those of Glaub et al. (2012) , who estimate that treated firms have roughly twice as many workers as control firms after five to seven months of a three-day training intervention. This estimate is within the standard error bands of both our estimates on employment reported in Column 3 of Table 7 (198.5% and 87.1%, Web-based and survey-based, respectively). Finally, similar to our own noisy estimates for survival, most studies also find positive but insignificant impacts (cf. McKenzie and Woodruff 2014 ). In the rest of this section, we discuss three final points: the external validity of the results; the potential reasons why basic services alone have no apparent impact in our setting; and the potential mechanisms through which entrepreneurship schooling bundled with basic services can affect new venture performance. We also address the potential frictions preventing entrepreneurs from acquiring these services elsewhere. Although our setting does not allow us to pinpoint the exact mechanisms underlying entrepreneurship schooling, our discussion, grounded in the education literature, sheds light on future research avenues. 5.1 External validityTwo issues can affect the external validity of results. The first is that our estimates correspond to local average treatment effects around qualification cutoffs, and the subpopulations of start-ups around those cutoffs may not necessarily be representative of average start-ups in the population. For example, these start-ups may be particularly sensitive to schooling services, which may explain the large magnitudes of our estimates. However, the local nature of the estimates does not necessarily decimate their external validity (cf. Card 2001 ). From a practical standpoint, our local estimates are particularly useful to policy makers. After all, policy interventions of entrepreneurship schooling are best suited to target subpopulations that are particularly lacking in entrepreneurial capital. The second potential issue is that Start-Up Chile is a unique program, and thus results from this setting may not generalize to other ecosystem accelerators. However, Start-Up Chile is actually very similar to the average ecosystem accelerator. For example, reallocation requirements for participants and the location of the program (typically an underdeveloped economic hub) are common traits. Indeed, we estimate that 58.6% of ecosystem accelerators worldwide require full founding team relocation, and an additional 31.0% have at least some partial relocation requirement. 20 Moreover, 37.9% of ecosystem accelerators are located in underdeveloped regions (17.9% Africa, 10.3% Latin America, 10.3% India); 37.9% are in the United States, but outside Silicon Valley; and the rest are in Europe, the United Kingdom, or Canada, but not typically in the capital cities of these countries. In addition, concerns regarding the ability of average ecosystem accelerators to replicate the visibility increase provided by Start-Up Chile do not appear first order. We estimate that news sponsors cover 76% of ecosystem accelerators (i.e., have at least one article in their press clips or the program futures in TechCrunch’s CrunchBase database). Also, the vast majority of accelerators publish the names of their participants on their Web pages (in fact, this practice is common across early-stage financial intermediaries), and finalize the program with a well-publicized demo day ( Cohen and Hochberg 2014 ). Finally, the guidance and accountability and networking opportunities provided by the entrepreneurship schooling at Start-Up Chile are prevalent among ecosystem accelerators, and among investor-led and corporate business accelerators more generally. In surveys on business accelerators, network development as well as guidance and accountability appear extensive, although quite heterogeneous across programs. Cohen and Hochberg (2014) argue that accelerator programs generally include seminars given by staff or guest speakers who often provide one-on-one guidance after the talk. Some programs also schedule meetings with several mentors; others may make introductions on an as-needed basis, or simply hand entrepreneurs a list of preselected mentors. A recent report by Nesta and the Department for Business, Energy and Industrial Strategy (2017) on accelerators in the United Kingdom documents a great deal of variation in the way that the entrepreneurship schooling services are imparted. In particular, of surveyed accelerators, 85% offer some form of mentoring; 45% offer seminars or workshops; and 12%, 11%, and 6% offer more specific help in the form of access to experts, legal/accountancy support, and technology support, respectively. Regardless of the similarities between Start-Up Chile and other ecosystem accelerators, we are, however, careful to emphasize the differences between average applicants to Start-Up Chile and other ecosystem accelerators worldwide, as mentioned in Section 1.3 . We argue that the external validity of our findings is likely confined to other ecosystem accelerators that focus on young founders and early-stage start-ups. 5.2 Why did basic services of cash and coworking space have no apparent impact on new venture performance?Other than the null hypothesis being true, one potential explanation is that start-ups near the capacity threshold are heterogeneous in their potential for success, and that basic services accelerate the inevitable outcomes (growth or failure) of participants relative to nonparticipants. In this case, the program might accelerate the success of high-potential business opportunities and expedite the demise of low-potential ones, with a resulting zero average treatment effect. For example, cash infusions can help founders discover fundamental flaws in their prototypes that justify the termination of the start-up or help them access new information that justifies the creation of a different start-up ( Yu 2016 ; Smith and Hannigan 2015 ; Leatherbee and Katila 2017 ). Other potential explanations are less consistent with the results. One first alternative is that we do not have enough power to reject the null. That is, that the effect is indeed positive, but we do not have a big enough sample to distinguish it from zero. Against the notion that this possibility is a first order explanation, Table 6 shows that estimated effects are often negative, sometimes close to zero, and switch signs across specifications. In addition, Appendix Table 4.4 summarizes back-of-the-envelope power calculations that suggest we have enough power in the majority of specifications. A second potential explanation is that the capital infusion is too small (not critical enough) to generate positive returns. While this explanation cannot be completely ruled out, it is not as likely to resonate in our setting for three main reasons. First, applicant start-ups are predominantly from the “new economy,” for which the necessary levels of physical capital stock to generate positive returns are generally low (e.g., Rajan and Zingales 2000 ). Second, start-ups in our sample are mostly in the business model discovery and validation phase, which is typically characterized by low levels of fixed costs. Third, the program estimates that relocation costs only represent 10% of the grant. In addition, the cash provided by Start-Up Chile is actually above the average stipend of |${\$}$| 22,890 provided in business accelerators, as reported in Cohen and Hochberg (2014) . A third potential explanation is that rejected applicants secured acceleration services elsewhere, thereby dampening the estimated effect of the basic services. The analysis of supplementary data does not support this alternative story. We collected information from Seed-DB 21 regarding nonparticipants’ acceptance into other programs, and found that only 2% of rejected applicants secured financing in other accelerators. This low probability is consistent with recent estimates of low acceptance rates in accelerators worldwide. According to FS6.com, a Web platform that runs 90% of applications to accelerators globally, less than 3.98% of applicants ever make it into an accelerator ( Butcher 2014 ). One last potential explanation is that the mechanisms to curb entrepreneurs’ opportunistic behavior 22 are not strong enough through the program’s basic services. Indeed, the value-adding monitoring and powerful allocation of control rights by financial intermediaries ( Hellmann 1998 ; Cornelli and Yosha 2003 ) are most pronounced in the entrepreneurship school. According to the program’s staff, however, even nonschooled participants are very motivated. Since the inception of the program, only one case of questionable use of funds has occurred, and corrective measures were taken. The lack of opportunistic behavior may be a due to reputational consequences acting as disciplinary devices (cf. Bernthal 2015 ). 5.3 How does entrepreneurship schooling affect performance?While our setting is not suited to distinguish the specific mechanisms by which entrepreneurship schooling can affect performance, our results provide an empirical foothold for future research to explore how specifically schooling matters. Based on the education literature, and consistent with interviews and surveys to participants, we distinguish two broad potential mechanisms (see Appendix 1): productivity increases ( Becker 1975 ) and certification ( Spence 1973 ; Arrow 1973 ). Productivity may increase via the instruction of entrepreneurship know-how from peers and staff (cf. Lerner and Malmendier 2013 ), access to valuable social networks ( Granovetter 1973 ; Ketchen, Ireland, and Snow 2007 ; Cai and Szeidl 2016 ), the structured accountability imposed by regular meetings (cf. Locke and Latham 2002 ; Cialdini and Goldstein 2004 ), and increases in the self-efficacy of founders ( Bandura 1982 ; Forbes 2005 ; Heckman and Kautz 2014 ). In the absence of business accelerators, start-ups may not realize these productivity increases because of market frictions, such as informational constraints. For example, accelerators may provide entrepreneurial know-how through experiential learning, which is a type of learning not typically found in traditional educational programs. Business school syllabi rarely provide such learning opportunities, as often professors lack the necessary hands-on business experience. Indeed, it is only until recently that universities have begun to replicate the business accelerator model in their educational programs. In addition, business accelerators may help overconfident entrepreneurs to recognize the value of accountability structures for performance, which they would not otherwise demand. Furthermore, some founders may simply be unaware of the importance of building business networks and not look for networking opportunities outside the accelerator. Certification may also be at play because business accelerators typically increase the exposure and legitimacy of ventures ( Zott and Huy 2007 ) via, for example, the promotion of start-ups on accelerators’ Web sites and during the demo days at the end of the programs ( Cohen and Hochberg 2014 ). Start-ups may need certification because of information asymmetries relative to the potential performance, which are typically prevalent in transformational ventures. Future research may extend exploration of the effects of entrepreneurship schooling to the founder-level of analysis. Understanding how accelerators influence the persistence of individuals on an entrepreneurial career path and how an entrepreneurial experience may influence an individual’s entrepreneurial capital for the creation of economic value regardless of her career path are two other important questions future research can seek to answer. Whether accelerators affect new venture performance is an important question with both theoretical and practical implications. However, until now little rigorous evidence has existed about whether accelerators are effective, and, if so, which services make them so. This paper provides the first quasi-experimental evidence of the effect of accelerator programs and the importance of entrepreneurial capital on new venture performance, shedding light on entrepreneurship schooling as a topic that is ripe for future research. We evaluated an ecosystem accelerator that provides participants with seed capital and coworking space. The accelerator also provides entrepreneurship-schooling services to a competitively select few. We find entrepreneurship schooling bundled with the basic services of cash and coworking space leads to significant increases in venture fundraising and scale. By contrast, we find no evidence that the basic accelerator services alone improve new venture performance. Regarding the policy design of ecosystem accelerator programs, if the objective is to accelerate start-ups, our results suggest that more resources should be allocated toward combining basic services with entrepreneurship schooling, rather than providing basic services on their own. This conclusion is particularly valid for programs that focus on young founders and early-stage start-ups. The findings are consistent with the view that entrepreneurial capital, similar to managerial capital, is a type of capital that is missing among certain populations (cf. Bruhn, Karlan, and Schoar 2010 ). Avenues for future research can include distinguishing the mechanisms through which entrepreneurship schooling and basic services in business accelerators affect entrepreneurial capital, including potential productivity increases and certification effects. We thank Start-Up Chile and YouNoodle for generous access to the data and numerous interviewed participants for their time. Special thanks go to Konrad Fernández, Horacio Melo, Nicolas Shea, Sebastian Vidal, and Rocío Fonseca for helpful discussions on the data, the program, and Chile’s entrepreneurial community. We also thank Pat Akey, Ulf Axelson, Taylor Begley, Shai Bernstein, Vicente Cunat, Slava Fos, Joan Farre-Mensa, Thomas Hellman, Stefan Lewellen, Ramana Nanda, Daniel Paravisini, Dina Pomeranz, Farzad Saidi, Merih Sevilir, Rui Silva, and Moqi Xu and the seminar participants at the Department of Finance at LSE, the NBER Entrepreneurship Meeting 2014, the Adam Smith Conference 2015, the UNC Junior Roundtable 2015, the 8th Private Equity Findings Symposium 2015, the CEPR ESSFM 2015, and the finance seminar at the University of Urbana Champaign. Su Wang provided excellent research assistance. Financial support from Abraaj Group, Chile’s National Commission for Scientific and Technological Research (CONICYT) Núcleo Milenio Research Center in Entrepreneurial Strategy Under Uncertainty (NS130028), Chile’s Production Development Corporation, and Aubrey Chernick Foundation is gratefully acknowledged. For the period of this research, Michael Leatherbee was part of Start-Up Chile’s Advisory Board. We thank the Editor (Francesca Cornelli) and two anonymous referees for detailed and insightful comments. Supplementary data can be found on The Review of Financial Studies web site. Acemoglu, D., Aghion, P. Lelarge, C. van Reenen, J. and Zilibotti F. 2007 . Technology, information, and the decentralization of the firm. Quarterly Journal of Economics 122 : 1759 – 99 . Google Scholar Applegate, L., Kerr, W. R. Lerner, J. Pomeranz, D. D. Herrero, G. and Scott. C. 2012 . Start-up Chile: April 2012. Case 812 – 158 . Harvard Business School , Cambridge, MA . Arrow, K. 1973 . Higher education as a filter. Journal of Public Economics 2 : 193 – 216 . Bandiera, O., Burgess, R. Das, N. Gulesci, S. Rasul, I. and Sulaiman. M. 2013 . Can basic entrepreneurship transform the economic lives of the poor? Working Paper , London School of Economics and Political Science . Google Preview Bandura, A. Self-efficacy mechanism in human agency . 1982 . American Psychologist 37 : 122 – 47 . Becker, G. S. 1975 . Human capital: A theoretical and empirical analysis, with special reference to education , Second Edition. New York : National Bureau of Economic Research, distributed by Columbia University Press . Berge, L. I. O., Bjorvatn, K. and Tungodden. B. 2015 . Human and financial capital for microenterprise development: Evidence from a field experiment in Tanzania. Management Science 61 : 707 – 22 . Bernthal, B. 2015 . Investment accelerators. Working Paper , Silicon Flatirons Center . Bertrand, M., and Schoar. A. 2003 . Managing with style: The effect of managers on firm policies. Quarterly Journal of Economics 118 : 1169 – 208 . Bingham C. B., Eisenhardt, K. M. and Furr. N. R. 2007 . What makes a process a capability? Heuristics, strategy, and effective capture of opportunities. Strategic Entrepreneurship Journal 1 : 27 – 47 . Bloom, N., Eifert, B. Mahajan, A. McKenzie, D. and Roberts. J. 2013 . Does management matter? Evidence from India. Quarterly Journal of Economics 128 : 1 – 51 . Bloom, N., Sadun, R. and van Reenen. J. 2016 . Management as a technology? Working Paper , Harvard Business School . Bloom, N., and van Reenen. J. 2010 . Why do management practices differ across firms and countries? Journal of Economic Perspectives 24 : 203 – 24 . Bone, J., Allen, O. and Haley. C. 2017 . Business incubator and accelerators: The national picture. BEIS Research Paper, Nesta . Bruhn, M., Karlan, D. and Schoar. A. 2010 . What capital is missing in developing countries? American Economic Review 100 : 629 – 33 . Bruhn, M., Karlan, D. and Schoar. A. 2016 . The impact of offering consulting services to small and medium enterprises: Evidence from a randomized trial in Mexico. Working Paper , World Bank . Brunet, S. M. Grof and Izquierdo. D. 2015 . Global Accelerator Report. http://gust.com/global-accelerator-report-2015/ . Butcher, M. 2014 . Who gets into accelerators? Persistent men with SaaS apps, says study. Tech Crunch , April 20. https://techcrunch.com/2014/04/20/who-gets-into-accelerators-persistent-men-with-saas-apps-says-study/ . Cai, J., and Szeidl. A. 2016 . Interfirm relationships and business performance. Research Paper , Department for International Development , China . Calderon, G., Cunha, J. M. and De Giorgi. G. 2013 . Business literacy and development: Evidence from a randomized controlled trial in rural Mexico. Working Paper , NBER . Card, D. 2001 . Estimating the return to schooling: Progress on some persistent econometric problems. Econometrica 69 : 1127 – 60 . Cialdini, R. B., and Goldstein. N. J. 2004 . Social influence: Compliance and conformity. Annual Review of Psychology 55 : 591 – 621 . Clarysse, B., Wright, M., and Van Hove, J. 2015 . A look inside accelerators: Building businesses. Research Paper , Nesta . Cohen, S. 2013 . What do accelerators do? Insights from incubators and angels. Innovations: Technology, Governance, Globalization 8 : 19 – 25 . Cohen, S. G., and Hochberg. Y. V. 2014 . Accelerating startups: The seed accelerator phenomenon. Working Paper . Cornelli, F., and Yosha. O. 2003 . Stage financing and the role of convertible securities. Review of Economic Studies 70 : 1 – 32 . Davis, S. J., Haltiwanger, J. C. and Schuh. S. 1996 . Job creation and destruction . Cambridge : MIT Press Books . de Mel, S., McKenzie, D. and Woodruff. C. 2008 . Returns to capital in microenterprises: Evidence from a field experiment. Quarterly Journal of Economics 123 : 1329 – 72 . de Mel, S., McKenzie, D. and Woodruff. C. 2014 . Business training and female enterprise start-up, growth, and dynamics: Experimental evidence from Sri Lanka. Journal of Development Economics 106 : 199 – 210 . Drexler, A., Fischer, G. and Schoar. A. 2014 . Keeping it simple: Financial literacy and rules of thumb. American Economic Journal: Applied Economics 6 : 1 – 31 . Fehder, D. C., and Hochberg. Y. V. 2014 . Accelerators and the regional supply of venture capital investment. Working Paper . Forbes, D. P. 2005 . The effects of strategic decision making on entrepreneurial self-efficacy. Entrepreneurship Theory and Practice 29 : 599 – 627 . Gelman, A., and Imbens. G. W. 2014 . Why high-order polynomials should not be used in regression discontinuity designs. Working Paper , NBER . Glaub, M., Frese, M. Fischer, S. and Hoppe. M. 2012 . A psychological personal initiative training enhances business success of African business owners. Mimeo, National University of Singapore Business School . Goldfarb, B., Kirsch, D. and Miller. D. A. 2007 . Was there too little entry during the Dot Com era? Journal of Financial Economics 86 : 100 – 144 . Golomb, V. M. 2015 . Accelerators are the new business school. Tech Crunch , July 11. http://techcrunch.com/2015/07/11/accelerators-are-the-new-business-school/ . Gonzalez-Uribe, J. 2014 . El caso de Start-Up Chile. Programa de atracción de talento para fomentar el emprendimiento. CAF, Development Bank of Latin America . Granovetter, M. S. 1973 . The strength of weak ties. American Journal of Sociology 78 : 1360 – 80 . Hallen, B. L., Bingham, C. B. and Cohen. S. L. G. 2016 . Do accelerators accelerate? A study of venture accelerators as a path to success. Working Paper . Haltiwanger, J. C., Jarmin, R. S. R.S. and Miranda. J. 2013 . Who creates jobs? Small versus large versus young. Review of Economics and Statistics 95 : 347 – 61 . Heckman, J. J., and Kautz. T. 2014 . Fostering and measuring skills: Interventions that improve character and cognition. In The Myth of Achievement Tests: The GED and the Role of Character in American Life . Eds. Heckman, J. J. Humphries, J. E. and Kautz, T. 341 – 430 . Chicago, IL : University of Chicago Press . Hellmann, T. 1998 . The allocation of control rights in venture capital contracts. RAND Journal of Economics 29 : 57 – 76 . Hellmann, T., and Puri. M. 2000 . The interaction between product market and financing strategy: The role of venture capital. Review of Financial Studies 13 : 959 – 84 . Hellmann, T., and Puri. M. 2002 . Venture capital and the professionalization of start-up firms: Empirical evidence. Journal of Finance 57 : 169 – 97 . Hurst, E., and Pugsley. B. W. 2011 . What do small businesses do? Brookings Papers on Economic Activity 43 : 73 – 142 . Imbens, G. W., and Angrist. J. D. 1994 . Identification and estimation of local average treatment effects. Econometrica 62 : 467 – 75 . Kerr, W. R., Lerner, J. and Schoar. A. 2014 . The consequences of entrepreneurial finance: Evidence from angel financings. Review of Financial Studies 27 : 20 – 55 . Kerr, W. R., Nanda, R. and Rhodes-Kropf. M. 2014 . Entrepreneurship as experimentation. Journal of Economic Perspectives 28 : 25 – 48 . Ketchen, D. J., Ireland, R. D. and Snow. C. C. 2007 . Strategic entrepreneurship, collaborative innovation and wealth creation. Strategic Entrepreneurship Journal 1 : 371 – 85 . Kim, J., and Wagman. L. 2014 . Portfolio size and information disclosure: An analysis of startup accelerators. Journal of Corporate Finance 29 : 520 – 34 . Klinger, B., and Schundeln. M. 2011 . Can entrepreneurial activity be taught? Quasi-experimental evidence from central America. World Development 39 : 1592 – 610 . Leatherbee, M. and Eesley, C.E. 2014 . Boulevard of broken behaviors: Socio-psychological mechanisms of entrepreneurship policies. Working Paper . Leatherbee, M. and Katila, R. 2017 . Stay the course or pivot? Antecedents of cognitive refinements of business models in young firms. Working Paper . Lee, D. S., and Lemieux. T. 2010 . Regression discontinuity designs in economics. Journal of Economic Literature 48 : 281 – 355 . Lerner, J., and Malmendier. U. 2013 . With a little help from my (random) friends: Success and failure in post-business school entrepreneurship. Review of Financial Studies 26 : 2411 – 52 . Lerner, J., Schoar, A., Sokolinski, S., and Wilson, K. 2015 . The globalization of angel investments. Working Paper , NBER . Locke, E. A. and Latham. G. P. 2002 . Building a practically useful theory of goal setting and task motivation: A 35-year odyssey. American Psychologist 57 : 705 – 17 . Maurer, I., and Ebers. M. 2006 . Dynamics of social capital and their performance implications: Lessons from biotechnology start-ups. Administrative Science Quarterly 51 : 262 – 92 . McCrary, J. 2008 . Manipulation of the running variable in the regression discontinuity design: A density test. Journal of Econometrics 142 : 698 – 714 . McKenzie, D. 2017 . Identifying and spurring high-growth entrepreneurship: Experimental evidence from a business plan competition. American Economic Review 107 : 2278 – 307 . McKenzie, D., and Woodruff. C. 2008 . Experimental evidence on returns to capital and access to finance in Mexico. World Bank Economic Review 22 : 457 – 82 . McKenzie, D., and Woodruff. C. 2014 . What are we learning from business training and entrepreneurship evaluations around the developing world?” . World Bank Research Observer 29 : 48 – 82 . Puri, M., and Zarutskie. R. 2012 . On the life cycle dynamics of venture capital and non-venture-capital financed firms. Journal of Finance 67 : 2247 – 93 . Radojevich-Kelley, N., and Hoffman. D. L. 2012 . Analysis of accelerator companies: An exploratory case study of their programs, processes, and early results. Small Business Institute Journal 8 : 54 – 70 . Rajan, R. G., and Zingales. L. 2000 . The governance of the new enterprise. Corporate governance: Theoretical and empirical perspectives . Cambridge, MA : Cambridge University Press . Rao, H. 1994 . The social construction of reputation: Certification contests, legitimation, and the survival of organizations in the American automobile industry: 1895-1912. Strategic Management Journal 15 : 29 – 44 . Roberts, M. R., and Whited. T. M. 2012 . Endogeneity in corporate finance. In Handbook of the economics of finance , Volume 2 . Eds. Constantinides, G. Harris, M. and Stulz, R. 493 – 572 Amsterdam : Elsevier . Schoar, A. 2010 . The divide between subsistence and transformational entrepreneurship. In Innovation policy and the economy , Volume 10 . Eds. Lerner J. and Stern, S. 57 – 81 . Washington, DC : National Bureau of Economic Research Innovation Policy and the Economy . Spence, M. 1973 . Job market signaling. Quarterly Journal of Economics 87 : 355 – 74 . Smith, S. W., and Hannigan. T. J. 2015 . Swinging for the fences: How do top accelerators impact the trajectories of new ventures? Working Paper . van der Klaauw, W. 2002 . Estimating the effect of financial aid offers on college enrollment: A regression–discontinuity approach. International Economic Review 43 : 1249 – 87 . Yu, S. 2016 . How do accelerators impact high-technology ventures? Working Paper . Zott, C., and Huy. Q. N. 2007 . How entrepreneurs use symbolic management to acquire resources. Administrative Science Quarterly 52 : 70 – 105 . 1 At least 4,397 institutions self-identify as an accelerator. See F6S available at https://www.f6s.com (last visited May 2016). 2 By new ventures, we mean start-ups that aim at becoming large, vibrant businesses (aka transformational ventures) ( Schoar 2010 ). 3 For example, see https://www.sba.gov/blogs/sba-launches-growth-accelerator-fund . 4 Our paper also complements the work by Klinger and Schundeln (2011) and McKenzie (2017) , who look at the impact of formal and structured business training programs offered by business plan competitions. 5 According to Natty Zola, the Managing Director of TechStars, business accelerators are “a proven way to quickly grow a start-up by learning from experts, finding great mentorship and connecting to a powerful network. They provide resources that reduce the cost of starting a company and the early capital a team needs to get their venture off the ground or to achieve key early milestones. They have become the new business school” ( Brunet, Grof, and Izquierdo 2015 ). We illustrate this association between business and entrepreneurship schools in Appendix Table 1.1. By drawing a parallel between the services offered by entrepreneurship schooling in a prototypical accelerator program and those offered by business schools, we unpack the performance-enhancing mechanisms that potentially underlie entrepreneurship schooling. 6 Bloom et al. (2013) find suggestive evidence that informational frictions help explain why modern managerial practices are not employed by participants (before the intervention). These firms are typically ex ante unaware of such practices or do not believe such practices will improve performance. 7 For additional details on Start-Up Chile, see Applegate et al. (2012) and Gonzalez-Uribe (2014) . 8 Relocation of founders is a common request for participation in business accelerators. See Section 5.1 for additional details. 9 Business accelerators typically offer guidance/accountability and networking opportunities as part of their entrepreneurial schooling services. There is, however, large variation in exactly how those services are imparted. See Section 5.1 for additional details. 10 See http://goizueta.emory.edu/faculty/socialenterprise/resources/database.html . 11 The only exception was generation 2, where the number of participants was set at 150. In unreported regressions, we exclude the first two generations of the program, and results are quantitatively unchanged. 12 Highly ranked companies are assigned low rank values; for example, the top company has a rank of 1. 13 We have detailed information on the pitch-day scores by criterion and by judges only for generation 6. For the rest of the generations, we only have access to the final (weighted average) pitch-day score. In generation 6, the weights used for each criterion were as follows: problem, 30%; solution, 20%; business model, 20%; market, 20%; and fundraising needs, 10%. 14 This jump is also evident in Figure 4 , where we plot the fraction of accepted participants in bins of 0.2 pitch-day-score units. 15 Because we received few responses from participants who competed for a spot in the entrepreneurship school (45), and even fewer from schooled participants (13), we only use this first survey to test basic accelerator services for Applicants . 16 For those applicants that have not secured external funding, this variable corresponds to their perceived valuation. 17 The average survival rate is lower than the fundraising rate because some of the companies that raised financing were not alive by the time of the survey, yet the founders answered the questionnaire. Results are robust to excluding these companies from the sample. 18 Because our sample is not comprised of subsistence entrepreneurs, common sense suggests that if entrepreneurs were not motivated, they would simply stop pursuing their ventures. 19 We record as errors all instances in which start-ups report (1) higher sales during the previous year than since they began operations, (2) higher sales in Chile than overall sales, (3) higher capital fundraising in Chile than overall, and (4) more employees in Chile than overall. 20 We use hand-collected descriptions of ecosystem accelerators surveyed by the Entrepreneurship Database Program at Goizueta Business School of Emory University on ecosystem accelerators worldwide to construct these estimates. 21 Seed-DB is an open-source accelerator database built on CrunchBase data ( http://www.seed-deb.com/ ). 22 The program takes no equity stake in participating start-ups. However, it engages in capital staging, and reputational consequences of opportunistic behavior are likely. Supplementary data Month: | Total Views: | September 2017 | 153 | October 2017 | 120 | November 2017 | 50 | December 2017 | 96 | January 2018 | 57 | February 2018 | 32 | March 2018 | 2,608 | April 2018 | 492 | May 2018 | 150 | June 2018 | 100 | July 2018 | 70 | August 2018 | 95 | September 2018 | 95 | October 2018 | 114 | November 2018 | 117 | December 2018 | 103 | January 2019 | 104 | February 2019 | 112 | March 2019 | 116 | April 2019 | 122 | May 2019 | 245 | June 2019 | 234 | July 2019 | 274 | August 2019 | 227 | September 2019 | 220 | October 2019 | 258 | November 2019 | 252 | December 2019 | 209 | January 2020 | 291 | February 2020 | 380 | March 2020 | 270 | April 2020 | 321 | May 2020 | 184 | June 2020 | 291 | July 2020 | 260 | August 2020 | 298 | September 2020 | 182 | October 2020 | 297 | November 2020 | 251 | December 2020 | 277 | January 2021 | 424 | February 2021 | 338 | March 2021 | 267 | April 2021 | 325 | May 2021 | 216 | June 2021 | 168 | July 2021 | 178 | August 2021 | 181 | September 2021 | 229 | October 2021 | 209 | November 2021 | 258 | December 2021 | 167 | January 2022 | 292 | February 2022 | 235 | March 2022 | 238 | April 2022 | 244 | May 2022 | 215 | June 2022 | 158 | July 2022 | 214 | August 2022 | 210 | September 2022 | 209 | October 2022 | 213 | November 2022 | 225 | December 2022 | 219 | January 2023 | 476 | February 2023 | 196 | March 2023 | 274 | April 2023 | 235 | May 2023 | 201 | June 2023 | 167 | July 2023 | 185 | August 2023 | 176 | September 2023 | 192 | October 2023 | 266 | November 2023 | 238 | December 2023 | 196 | January 2024 | 333 | February 2024 | 216 | March 2024 | 247 | April 2024 | 221 | May 2024 | 309 | June 2024 | 227 | July 2024 | 179 | August 2024 | 57 | Email alertsCiting articles via. - Recommend to your Library
Affiliations- Online ISSN 1465-7368
- Print ISSN 0893-9454
- Copyright © 2024 Society for Financial Studies
- About Oxford Academic
- Publish journals with us
- University press partners
- What we publish
- New features
- Open access
- Institutional account management
- Rights and permissions
- Get help with access
- Accessibility
- Advertising
- Media enquiries
- Oxford University Press
- Oxford Languages
- University of Oxford
Oxford University Press is a department of the University of Oxford. It furthers the University's objective of excellence in research, scholarship, and education by publishing worldwide - Copyright © 2024 Oxford University Press
- Cookie settings
- Cookie policy
- Privacy policy
- Legal notice
This Feature Is Available To Subscribers OnlySign In or Create an Account This PDF is available to Subscribers Only For full access to this pdf, sign in to an existing account, or purchase an annual subscription. 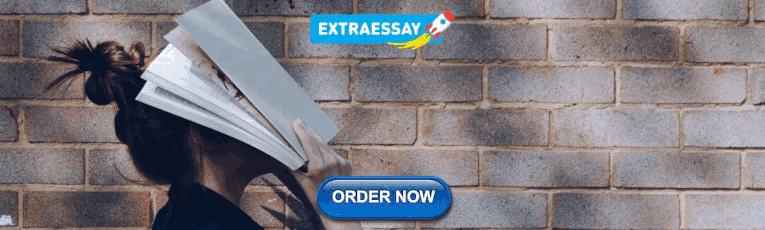 |
COMMENTS
The model has two critical features. First, it allows for the endogeneity of business plans in venture performance. This is important because if endogeneity is ignored, biased and inconsistent estimates of the impact of plans (due to the conflation of plan effects with unobservable ability/ motivation) are likely.
We offer an empirical methodology which can account for these multiple effects while disentangling real impact effects from selection effects. We apply this to English data where we find that business plans promote employment growth. This is found to be due to the impact of the plan and not selection effects.
The Effects of Pre-venture Plan Timing and Perceived Environmental Uncertainty on the Persistence of Emerging Firms. This paper explores the effects of when pre-venture planning occurs (early or late) in the sequence of activities accomplished during the process of new business emergence, and the moderating effects….
Downloadable! We investigate the multiple effects of writing a business plan prior to start‐up on new venture performance. We argue that the impact of business plans depends on the purpose for and circumstances in which they are being used. We offer an empirical methodology which can account for these multiple effects while disentangling real impact effects from selection effects.
T1 - The multiple effects of business planning on new venture performance. AU - Burke, Andrew. AU - Fraser, Stuart. AU - Greene, Francis J. PY - 2009/9/5. Y1 - 2009/9/5. N2 - We investigate the multiple effects of writing a business plan prior to start-up on new venture performance.
To disentangle these business planning impact effects are separated out from effects due to differences in venture/entrepreneur profiles. On the upside, we find that business planning results in the start-up of larger ventures (55% larger) and increases subsequent size (by 109%) but at the downside expense of an increased likelihood of first ...
The Multiple Effects of Business Planning on New Venture Performance. Andrew Burke, Stuart Fraser and Francis J. Greene. Journal of Management Studies, 2010, vol. 47, issue 3, 391-415 . Abstract: We investigate the multiple effects of writing a business plan prior to start‐up on new venture performance. We argue that the impact of business plans depends on the purpose for and circumstances ...
We investigate the impact of writing a business plan prior to start-up on new venture performance. Our analysis makes new contributions by examining multiple effects of business plans. This approach allows the impacts of business plans to be disentangled from selection effects due to differences in the profile and business context of ventures that are more or less likely to write a business plan.
Abstract. We investigate the multiple effects of writing a business plan prior to start-up on new venture performance. We argue that the impact of business plans depends on the purpose for and ...
We investigate the multiple effects of writing a business plan prior to start-up on new venture performance. We argue that the impact of business plans depends ... Andrew E. and Fraser, Stuart and Greene, Francis J., Multiple Effects of Business Plans on New Ventures (March 5, 2009). Journal of Management Studies, Forthcoming, Available at ...
and Latham, 1980), and suggest that business. planning should facilitate new venture develop. ment. Planning is an important precursor to action because it provides a framework within which sub sequent action takes place (Ansoff, 1991), thereby facilitating the achievement of goals (Locke and. Latham, 1980).
abstract We investigate the multiple effects of writing a business plan prior to start‐up on new venture performance. We argue that the impact of business plans depends on the purpose for and circumstances in which they are being used. We offer an empirical methodology which can account for these multiple effects while disentangling real impact effects from selection effects. We apply this ...
The net result is that this approach allows for more insightful estimates on the effect writing a business plan on new venture performance. We apply the model to UK data on 622 de novo entrepreneurs in relation to one performance measure, namely growth in the size of the venture as measured by employment size. The dataset allows us to
First, we theorize and investigate the effects of plan sequencing; arguing that a business plan written early on in new venture development increases the prospects of venture viability. Second, we examine plan duration effects, and argue that there is a curvilinear relationship between spending time on a plan and achieving venture viability ...
We investigate the multiple effects of writing a business plan prior to start-up on new venture performance. We argue that the impact of business plans depends on the purpose for and circumstances in which they are being used. We offer an empirical methodology which can account for these multiple effects while disentangling real impact effects from selection effects.
Entrepreneurs rely on different decision-making logics when starting new ventures, including causal and effectual reasoning. Extant research suggests that venture performance is positively associated with both causal business planning and effectual action-orientation, but studies have not yet tested the synergistic potential of these two logics.
The multiple effects of busine... More details; The multiple effects of business planning on new venture performance . Andrew Burke, Stuart Fraser and Francis J. Greene. Year of publication: 2010. Authors: Burke, Andrew; Fraser, Stuart; Greene, Francis J. Published in: Journal of ...
Supporting: 3, Contrasting: 1, Mentioning: 105 - We investigate the multiple effects of writing a business plan prior to start-up on new venture performance. We argue that the impact of business plans depends on the purpose for and circumstances in which they are being used. We offer an empirical methodology which can account for these multiple effects while disentangling real impact effects ...
Although evidence about "managerial capital" constraints (e.g., Bruhn, Karlan, and Schoar 2010; Bloom and van Reenen 2010) seems to justify this emphasis, little rigorous evidence exists on the effect of business accelerators on new venture performance and on the importance of entrepreneurial capital in new firms. 2 This lack of evidence is ...
We investigate the multiple effects of writing a business plan prior to start-up on new venture performance. We argue that the impact of business plans depends on the purpose for and circumstances in which they are being used. We offer an empirical methodology which can account for these multiple effects while disentangling real impact effects ...
The-Multiple-Effects-of-Business-Planning-onNew-Venture-Performance-1 - Free download as PDF File (.pdf), Text File (.txt) or read online for free. Multiple effects of business planning