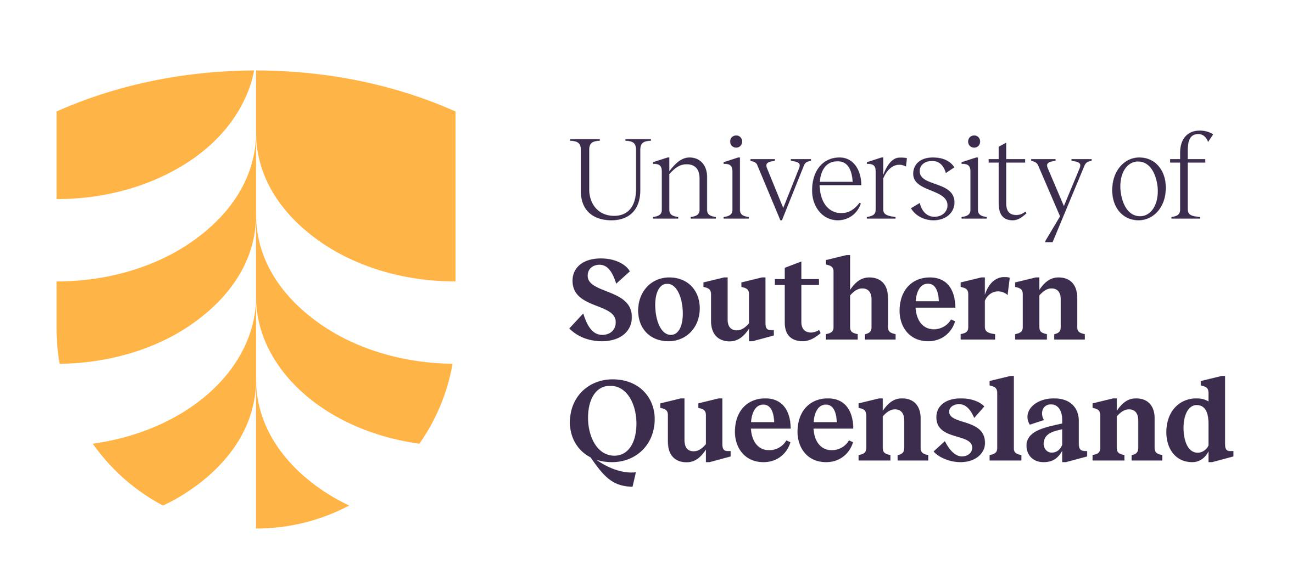
Want to create or adapt books like this? Learn more about how Pressbooks supports open publishing practices.
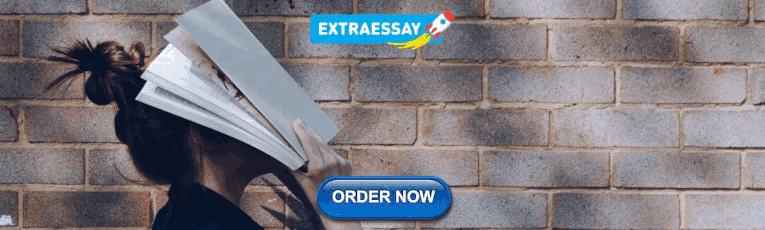
14 Quantitative analysis: Descriptive statistics
Numeric data collected in a research project can be analysed quantitatively using statistical tools in two different ways. Descriptive analysis refers to statistically describing, aggregating, and presenting the constructs of interest or associations between these constructs. Inferential analysis refers to the statistical testing of hypotheses (theory testing). In this chapter, we will examine statistical techniques used for descriptive analysis, and the next chapter will examine statistical techniques for inferential analysis. Much of today’s quantitative data analysis is conducted using software programs such as SPSS or SAS. Readers are advised to familiarise themselves with one of these programs for understanding the concepts described in this chapter.
Data preparation
In research projects, data may be collected from a variety of sources: postal surveys, interviews, pretest or posttest experimental data, observational data, and so forth. This data must be converted into a machine-readable, numeric format, such as in a spreadsheet or a text file, so that they can be analysed by computer programs like SPSS or SAS. Data preparation usually follows the following steps:
Data coding. Coding is the process of converting data into numeric format. A codebook should be created to guide the coding process. A codebook is a comprehensive document containing a detailed description of each variable in a research study, items or measures for that variable, the format of each item (numeric, text, etc.), the response scale for each item (i.e., whether it is measured on a nominal, ordinal, interval, or ratio scale, and whether this scale is a five-point, seven-point scale, etc.), and how to code each value into a numeric format. For instance, if we have a measurement item on a seven-point Likert scale with anchors ranging from ‘strongly disagree’ to ‘strongly agree’, we may code that item as 1 for strongly disagree, 4 for neutral, and 7 for strongly agree, with the intermediate anchors in between. Nominal data such as industry type can be coded in numeric form using a coding scheme such as: 1 for manufacturing, 2 for retailing, 3 for financial, 4 for healthcare, and so forth (of course, nominal data cannot be analysed statistically). Ratio scale data such as age, income, or test scores can be coded as entered by the respondent. Sometimes, data may need to be aggregated into a different form than the format used for data collection. For instance, if a survey measuring a construct such as ‘benefits of computers’ provided respondents with a checklist of benefits that they could select from, and respondents were encouraged to choose as many of those benefits as they wanted, then the total number of checked items could be used as an aggregate measure of benefits. Note that many other forms of data—such as interview transcripts—cannot be converted into a numeric format for statistical analysis. Codebooks are especially important for large complex studies involving many variables and measurement items, where the coding process is conducted by different people, to help the coding team code data in a consistent manner, and also to help others understand and interpret the coded data.
Data entry. Coded data can be entered into a spreadsheet, database, text file, or directly into a statistical program like SPSS. Most statistical programs provide a data editor for entering data. However, these programs store data in their own native format—e.g., SPSS stores data as .sav files—which makes it difficult to share that data with other statistical programs. Hence, it is often better to enter data into a spreadsheet or database where it can be reorganised as needed, shared across programs, and subsets of data can be extracted for analysis. Smaller data sets with less than 65,000 observations and 256 items can be stored in a spreadsheet created using a program such as Microsoft Excel, while larger datasets with millions of observations will require a database. Each observation can be entered as one row in the spreadsheet, and each measurement item can be represented as one column. Data should be checked for accuracy during and after entry via occasional spot checks on a set of items or observations. Furthermore, while entering data, the coder should watch out for obvious evidence of bad data, such as the respondent selecting the ‘strongly agree’ response to all items irrespective of content, including reverse-coded items. If so, such data can be entered but should be excluded from subsequent analysis.

Data transformation. Sometimes, it is necessary to transform data values before they can be meaningfully interpreted. For instance, reverse coded items—where items convey the opposite meaning of that of their underlying construct—should be reversed (e.g., in a 1-7 interval scale, 8 minus the observed value will reverse the value) before they can be compared or combined with items that are not reverse coded. Other kinds of transformations may include creating scale measures by adding individual scale items, creating a weighted index from a set of observed measures, and collapsing multiple values into fewer categories (e.g., collapsing incomes into income ranges).
Univariate analysis
Univariate analysis—or analysis of a single variable—refers to a set of statistical techniques that can describe the general properties of one variable. Univariate statistics include: frequency distribution, central tendency, and dispersion. The frequency distribution of a variable is a summary of the frequency—or percentages—of individual values or ranges of values for that variable. For instance, we can measure how many times a sample of respondents attend religious services—as a gauge of their ‘religiosity’—using a categorical scale: never, once per year, several times per year, about once a month, several times per month, several times per week, and an optional category for ‘did not answer’. If we count the number or percentage of observations within each category—except ‘did not answer’ which is really a missing value rather than a category—and display it in the form of a table, as shown in Figure 14.1, what we have is a frequency distribution. This distribution can also be depicted in the form of a bar chart, as shown on the right panel of Figure 14.1, with the horizontal axis representing each category of that variable and the vertical axis representing the frequency or percentage of observations within each category.
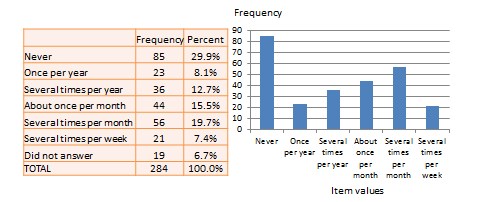
With very large samples, where observations are independent and random, the frequency distribution tends to follow a plot that looks like a bell-shaped curve—a smoothed bar chart of the frequency distribution—similar to that shown in Figure 14.2. Here most observations are clustered toward the centre of the range of values, with fewer and fewer observations clustered toward the extreme ends of the range. Such a curve is called a normal distribution .

Lastly, the mode is the most frequently occurring value in a distribution of values. In the previous example, the most frequently occurring value is 15, which is the mode of the above set of test scores. Note that any value that is estimated from a sample, such as mean, median, mode, or any of the later estimates are called a statistic .

Bivariate analysis
Bivariate analysis examines how two variables are related to one another. The most common bivariate statistic is the bivariate correlation —often, simply called ‘correlation’—which is a number between -1 and +1 denoting the strength of the relationship between two variables. Say that we wish to study how age is related to self-esteem in a sample of 20 respondents—i.e., as age increases, does self-esteem increase, decrease, or remain unchanged?. If self-esteem increases, then we have a positive correlation between the two variables, if self-esteem decreases, then we have a negative correlation, and if it remains the same, we have a zero correlation. To calculate the value of this correlation, consider the hypothetical dataset shown in Table 14.1.
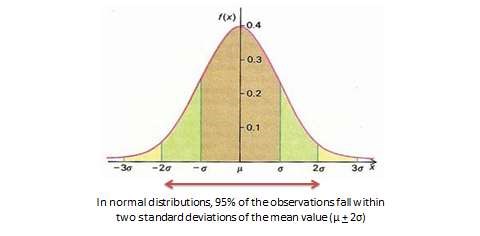
After computing bivariate correlation, researchers are often interested in knowing whether the correlation is significant (i.e., a real one) or caused by mere chance. Answering such a question would require testing the following hypothesis:
![Rendered by QuickLaTeX.com \[H_0:\quad r = 0 \]](https://usq.pressbooks.pub/app/uploads/quicklatex/quicklatex.com-74bb8e9e674477ba33a9eb751bfd254d_l3.png)
Social Science Research: Principles, Methods and Practices (Revised edition) Copyright © 2019 by Anol Bhattacherjee is licensed under a Creative Commons Attribution-NonCommercial-ShareAlike 4.0 International License , except where otherwise noted.
Share This Book
The Art of Sophisticated Quantitative Description in Higher Education Research
- Reference work entry
- First Online: 23 February 2022
- Cite this reference work entry
- Daniel Klasik 3 &
- William Zahran 3
Part of the book series: Higher Education: Handbook of Theory and Research ((HATR,volume 37))
1521 Accesses
3 Citations
While the emphasis on causal research in education has become increasingly important in recent years, thoughtful, descriptive analysis remains necessary for providing the conceptual grounding for experimental and quasi-experimental research and understanding our world. Sophisticated quantitative description is an approach to research that does not attempt to determine a causal impact. Instead, its purpose is to critically analyze and present data using purposeful methods to build a theory-driven story about a phenomenon that future research can investigate further. Sophisticated description can offer new ways to look at problems of research and practice, provide context and explanation for causal findings, or open new avenues of research. This chapter defines sophisticated quantitative description and provides an overview of its uses in higher education research. It outlines the numerous goals of sophisticated descriptive research and offers potential methods and approaches for conducting sophisticated description. Exemplars and discussion of published sophisticated descriptive research from the higher education literature are included throughout. The chapter concludes with an application of sophisticated description for analyzing college application behavior in the United States using social network analysis.
Nicholas Hillman was the Associate Editor for this chapter.
This is a preview of subscription content, log in via an institution to check access.
Access this chapter
Subscribe and save.
- Get 10 units per month
- Download Article/Chapter or eBook
- 1 Unit = 1 Article or 1 Chapter
- Cancel anytime
- Available as PDF
- Read on any device
- Instant download
- Own it forever
- Available as EPUB and PDF
- Durable hardcover edition
- Dispatched in 3 to 5 business days
- Free shipping worldwide - see info
Tax calculation will be finalised at checkout
Purchases are for personal use only
Institutional subscriptions
Similar content being viewed by others
A primer on the validity typology and threats to validity in education research
Structural Equation Modeling Approaches in Educational Research and Practice
Alvero, A. J., Giebel, S., Gebre-Medhin, B., Antonio, A.L., Stevens, M.L., & Domingue, B.W. (2021). Essay content is strongly related to household income and SAT scores: Evidence from 60,000 undergraduate applications . Science Advances, 7(42), eabi903.
Google Scholar
Arellano, L. (2020). Capitalizing baccalaureate degree attainment: Identifying student and institution level characteristics that ensure success for Latinxs. The Journal of Higher Education, 91 (4), 588–619.
Article Google Scholar
Attewell, P., Lavin, D., Domina, T., & Levey, T. (2006). New evidence on college remediation. The Journal of Higher Education, 77 (5), 886–924.
Avery, C., & Kane, T. (2004). Student perceptions of college opportunities: The Boston COACH program. In C. Hoxby (Ed.), College choices: The economics of where to go, when to go, and how to pay for it (pp. 355–391). University of Chicago Press.
Chapter Google Scholar
Avery, C., & Turner, S. (2012). Student Loans: Do College Students Borrow Too Much Or Not Enough?. Journal of Economic Perspectives, 26 (1), 165–92.
Baker, R. (2018). Understanding college students’ major choices using social network analysis. Research in Higher Education, 59 (2), 198–225.
Baker, R., Klasik, D., & Reardon, S. F. (2018). Race and stratification in college enrollment over time. AERA Open, 4 (1), 1–28.
Barringer, S. N., Leahey, E., & Salazar, K. (2020). What catalyzes research universities to commit to interdisciplinary research? Research in Higher Education, 61 (6), 679–705.
Belasco, A. S. (2013). Creating college opportunity: School counselors and their influence on postsecondary enrollment. Research in Higher Education, 54 (7), 781–804.
Bettinger, E. P., & Baker, R. B. (2014). The effects of student coaching: An evaluation of a randomized experiment in student advising. Educational Evaluation and Policy Analysis, 36 (1), 3–19.
Biancani, S., & McFarland, D. A. (2013). Social networks research in higher education. In M. Paulsen (Ed.), Higher education: Handbook of theory and research, volume 28 (pp. 151–215). Springer.
Bielby, R. M., House, E., Flaster, A., & DesJardins, S. L. (2013). Instrumental variables: Conceptual issues and an application considering high school course taking. In M. Paulsen (Ed.), Higher education: Handbook of theory and research, volume 28 (pp. 263–321). Springer.
Black, D., & Smith, J. (2004). How robust is the evidence on the effects of college quality? Evidence from matching. Journal of Econometrics, 121 , 99.
Blinder, A. S. (1973). Wage discrimination: Reduced form and structural estimates. The Journal of Human Resources, 8 (4), 436.
Bowen, W. G., Chingos, M. M., & McPherson, M. S. (2009). Crossing the finish line: Completing College at America’s public universities . Princeton University Press.
Book Google Scholar
Bowman, N. A., Miller, A., Woosley, S., Maxwell, N. P., & Kolze, M. J. (2019). Understanding the link between noncognitive attributes and college retention. Research in Higher Education, 60 (2), 135–152.
Connelly, R., Playford, C. J., Gayle, V., & Dibben, C. (2016). The role of administrative data in the big data revolution in social science research. Social Science Research, 59 , 1–12.
Dale, S., & Krueger, A. B. (2014). Estimating the return to college selectivity over the career using administrative earnings data. Journal of Human Resources, 49 (2), 323-358.
Deming, D. J., Goldin, C., & Katz, L. F. (2012). The for-profit postsecondary school sector: Nimble critters or agile predators? Journal of Economic Perspectives, 26 (1), 139–164.
Dillon, E. W., & Smith, J. A. (2020). The consequences of academic match between students and colleges. Journal of Human Resources, 55 (3), 767-808.
Douglas, D., & Salzman, H. (2020). Math counts: Major and gender differences in college mathematics coursework. The Journal of Higher Education, 91 (1), 84–112.
Dynarski, S., Libassi, C. J., Michelmore, K., & Owen, S. (2021). Closing the gap: The effect of reducing complexity and uncertainty in college pricing on the choices of low-income students. American Economic Review, 111 (6), 1721–1756.
Flores, S. M., Park, T. J., & Baker, D. J. (2017). The racial college completion gap: Evidence from Texas. The Journal of Higher Education, 88 (6), 894–921.
Furquim, F., Corral, D., & Hillman, N. (2020). A primer for interpreting and designing difference-in-differences studies in higher education research. In L. W. Perna (Ed.), Higher education: Handbook of theory and research, Volume 35 (pp. 1–58). Springer.
Greene, J. A., Oswald, C. A., & Pomerantz, J. (2015). Predictors of retention and achievement in a massive open online course. American Educational Research Journal, 52 (5), 925–955.
Gurantz, O., Howell, J., Hurwitz, M., Larson, C., Pender, M., & White, B. (2020). A national-level informational experiment to promote enrollment in selective colleges. Journal of Policy Analysis and Management, 42 (2), 453–479.
Hemelt, S. W., Stange, K. M., Furquim, F., Simon, A., & Sawyer, J. E. (2020). Why is math cheaper than English? Understanding cost differences in higher education. Journal of Labor Economics, 39 (2), 397-435.
Hillman, N. W. (2016). Geography of college opportunity: The case of education deserts. American Educational Research Journal, 53 (4), 987–1021.
Ho, A. D., & Reardon, S. F. (2012). Estimating achievement gaps from test scores reported in ordinal “proficiency” categories. Journal of Educational and Behavioral Statistics, 37 (4), 489–517.
Hoekstra, M. (2009). The effect of attending the flagship state university on earnings: A discontinuity-based approach. The Review of Economics and Statistics, 91 (4), 717.
Holzman, B., Klasik, D., & Baker, R. (2020). Gaps in the college application gauntlet. Research in Higher Education, 61 , 795–822.
Hossler, D., Braxton, J. M., & Coopersmith, G. (1989). Understanding student college choices. In J. C. Smart (Ed.), Higher education: Handbook of theory and research (Vol. 5, pp. 231–288). Agathon Press.
Hossler, D., & Gallagher, K. S. (1987). Studying student college choice: A three-phase model and the implications for policymakers. College and University, 62 (3), 207–221.
Hoxby, C. (2009). The changing selectivity of American colleges. Journal of Economic Perspectives, 23 (4), 95–118.
Hoxby, C., & Turner, S. (2013). Expanding college opportunities for high-achieving, low-income students (Stanford Institute for economic policy research discussion paper no. 12-014). Stanford Institute for Economic Policy Research.
Hoxby, C. M., & Avery, C. (2013). The missing “one-offs”: The hidden supply of high-achieving, low-income students. Brookings Papers on Economic Activity 2013(1), 1-65.
Hurwitz, M., Smith, J., Niu, S., & Howell, J. (2015). The Maine question: How is 4-year college enrollment affected by mandatory college entrance exams? Educational Evaluation and Policy Analysis, 31 (1), 138–159.
Imenda, S. (2014). Is there a conceptual difference between theoretical and conceptual frameworks? Journal of Social Sciences, 38 (2), 185–195.
Iyengar, S. S., & Lepper, M. R. (2000). When choice is demotivating: Can one desire too much of a good thing?. Journal of Personality and Social Psychology, 79 (6), 995–1006.
Klasik, D. (2012). The college application gauntlet: A systematic analysis of the steps to four-year college enrollment. Research in Higher Education, 53 (5), 506–549.
Klasik, D. (2013). The ACT of enrollment: The college enrollment effects of state-required college entrance exam testing. Educational Researcher, 42 (3), 151–160.
Klasik, D., Blagg, K., & Pekor, Z. (2018). Out of the education desert: How limited local college options are associated with inequity in postsecondary opportunities. Social Sciences, 7 (9), 165.
Klasik, D., & Hutt, E. L. (2019). Bobbing for bad apples: Accreditation, quantitative performance measures, and the identification of low-performing colleges. The Journal of Higher Education, 90 (3), 427–461.
Kurban, E. R., & Cabrera, A. F. (2020). Building readiness and intention towards STEM fields of study: Using HSLS:09 and SEM to examine this complex process among high school students. The Journal of Higher Education, 91 (4), 620–650.
Leighton, M., & Speer, J. D. (2020). Labor market returns to college major specificity. European Economic Review, 128 , 103489.
Loeb, S., Dynarski, S., McFarland, D., Morris, P., Reardon, S., & Reber, S. (2017). Descriptive analysis in education: A guide for researchers . National Center for Education Evaluation and Regional Assistance.
Long, B. T. (2004). How have college decisions changed over time? An application of the conditional logistic choice model. Journal of Econometrics, 121 (1–2), 271–296.
Long, M. C. (2008). College quality and early adult outcomes. Economics of Education Review, 27 , 588.
McCall, B. P., & Bielby, R. M. (2012). Regression discontinuity design: Recent developments and a guide to practice for researchers in higher education. In J. C. Smart & M. B. Paulsen (Eds.), Higher education: Handbook of theory and research (Vol. 27, pp. 249–290).
McDonough, P. M. (1997). Choosing colleges: How social class and schools structure opportunity . State University of New York Press.
McDonough, P. M. (2005). Counseling matters: Knowledge, assistance, and organizational commitment in college preparation. In W. G. Tierney, Z. B. Corwin, & J. E. Colyar (Eds.), Preparing for college: Nine elements of effective outreach . State University of New York Press.
Miller, G. N. (2020). I’ll know one when I see it: Using social network analysis to define comprehensive institutions through organizational identity. Research in Higher Education, 61 (1), 51–87.
Niu, S. X., & Tienda, M. (2007). Choosing colleges: Identifying and modeling choice sets. Social Science Research, 37 (2), 416–433.
Oaxaca, R. (1973). Male-female wage differentials in urban labor markets. International Economic Review, 14 (3), 693.
Padgett, J. F., & Ansell, C. K. (1993). Robust action and the rise of the Medici, 1400–1434. American Journal of Sociology, 98 (6), 1259–1319.
Perna, L. W. (2006). Studying college choice: A proposed conceptual model. In J. C. Smart (Ed.), Higher education: Handbook of theory and research (Vol. 21, pp. 99–157). Springer.
Roderick, M., Coca, V., & Nagaoka, J. (2011). Potholes on the road to college: High school effects in shaping urban students’ participation in college application, four-year college enrollment, and college match. Sociology of Education, 84 (3), 178–211.
Roderick, M., Nagaoka, J., Coca, V., & Moeller, E. (2008). From high school to the future: Potholes on the road to college . Consortium on Chicago School Research at the University of Chicago.
Rosenboom, V., & Blagg, K. (2018). Disconnected from higher education: How geography and internet speed limit access to higher education . The Urban Institute.
Salazar, K. G., Jaquette, O., & Han, C. (2021). Coming soon to a neighborhood near you? Off-campus recruiting by public research universities. American Educational Research Journal , Online First.
Scott-Clayton, J., & Rodriguez, O. (2015). Development, discouragement, or diversion? New evidence on the effects of college remediation policy. Education Finance and Policy, 10 (1), 4–45.
Skinner, B. T. (2019). Choosing college in the 2000s: An updated analysis using the conditional logistic choice model. Research in Higher Education, 60 (2), 153–183.
Smith, D. A., & White, D. R. (1992). Structure and dynamics of the global economy: Network analysis of international trade 1965–1980. Social Forces, 70 (4), 857–893.
Smith, J. (2018). The sequential college application process. Education Finance and Policy, 13 (4), 545–575.
Smith, J., Pender, M., & Howell, J. (2013). The full extent of student-college academic undermatch. Economics of Education Review, 32 , 247–261.
Turley, R. N. L. (2009). College proximity: Mapping access to opportunity. Sociology of Education, 82 (2), 126–146.
Wang, X. (2016). Course-taking patterns of community college students beginning in STEM: Using data mining techniques to reveal viable STEM transfer pathways. Research in Higher Education, 57 (5), 544–569.
Weiler, W. C. (1994). Transition from consideration of a college to the decision to apply. Research in Higher Education, 35 (6), 1994.
Wells, R. S., Kolek, E. A., Williams, E. A., & Saunders, D. B. (2015). “How we know what we know”: A systematic comparison of research methods employed in higher education journals, 1996–2000 v. 2006–2010. The Journal of Higher Education, 86 (2), 171–198.
Xie, Y., Fang, M., & Shauman, K. (2015). STEM education. Annual Review of Sociology, 41 (1), 331–357.
Xu, D., Jaggars, S. S., Fletcher, J., & Fink, J. E. (2018). Are community college transfer students “a good bet” for 4-year admissions? Comparing academic and labor-market outcomes between transfer and native 4-year college students. The Journal of Higher Education, 89 (4), 478–502.
Zemsky, R., & Oedel, P. (1983). The structure of college choice . College Entrance Examination Board.
Download references
Acknowledgments
The social network analysis of students’ college application choices described in this chapter was supported by a National Academy of Education/Spencer Foundation postdoctoral fellowship.
Author information
Authors and affiliations.
University of North Carolina at Chapel Hill, Chapel Hill, NC, USA
Daniel Klasik & William Zahran
You can also search for this author in PubMed Google Scholar
Corresponding author
Correspondence to Daniel Klasik .
Editor information
Editors and affiliations.
University of Pennsylvania, Philadelphia, PA, USA
Laura W. Perna
Rights and permissions
Reprints and permissions
Copyright information
© 2022 The Author(s), under exclusive license to Springer Nature Switzerland AG
About this entry
Cite this entry.
Klasik, D., Zahran, W. (2022). The Art of Sophisticated Quantitative Description in Higher Education Research. In: Perna, L.W. (eds) Higher Education: Handbook of Theory and Research. Higher Education: Handbook of Theory and Research, vol 37. Springer, Cham. https://doi.org/10.1007/978-3-030-76660-3_12
Download citation
DOI : https://doi.org/10.1007/978-3-030-76660-3_12
Published : 23 February 2022
Publisher Name : Springer, Cham
Print ISBN : 978-3-030-76659-7
Online ISBN : 978-3-030-76660-3
eBook Packages : Education Reference Module Humanities and Social Sciences Reference Module Education
Share this entry
Anyone you share the following link with will be able to read this content:
Sorry, a shareable link is not currently available for this article.
Provided by the Springer Nature SharedIt content-sharing initiative
- Publish with us
Policies and ethics
- Find a journal
- Track your research
- Search Menu
Sign in through your institution
- Browse content in Arts and Humanities
- Browse content in Archaeology
- Anglo-Saxon and Medieval Archaeology
- Archaeological Methodology and Techniques
- Archaeology by Region
- Archaeology of Religion
- Archaeology of Trade and Exchange
- Biblical Archaeology
- Contemporary and Public Archaeology
- Environmental Archaeology
- Historical Archaeology
- History and Theory of Archaeology
- Industrial Archaeology
- Landscape Archaeology
- Mortuary Archaeology
- Prehistoric Archaeology
- Underwater Archaeology
- Zooarchaeology
- Browse content in Architecture
- Architectural Structure and Design
- History of Architecture
- Residential and Domestic Buildings
- Theory of Architecture
- Browse content in Art
- Art Subjects and Themes
- History of Art
- Industrial and Commercial Art
- Theory of Art
- Biographical Studies
- Byzantine Studies
- Browse content in Classical Studies
- Classical History
- Classical Philosophy
- Classical Mythology
- Classical Numismatics
- Classical Literature
- Classical Reception
- Classical Art and Architecture
- Classical Oratory and Rhetoric
- Greek and Roman Papyrology
- Greek and Roman Epigraphy
- Greek and Roman Law
- Greek and Roman Archaeology
- Late Antiquity
- Religion in the Ancient World
- Social History
- Digital Humanities
- Browse content in History
- Colonialism and Imperialism
- Diplomatic History
- Environmental History
- Genealogy, Heraldry, Names, and Honours
- Genocide and Ethnic Cleansing
- Historical Geography
- History by Period
- History of Emotions
- History of Agriculture
- History of Education
- History of Gender and Sexuality
- Industrial History
- Intellectual History
- International History
- Labour History
- Legal and Constitutional History
- Local and Family History
- Maritime History
- Military History
- National Liberation and Post-Colonialism
- Oral History
- Political History
- Public History
- Regional and National History
- Revolutions and Rebellions
- Slavery and Abolition of Slavery
- Social and Cultural History
- Theory, Methods, and Historiography
- Urban History
- World History
- Browse content in Language Teaching and Learning
- Language Learning (Specific Skills)
- Language Teaching Theory and Methods
- Browse content in Linguistics
- Applied Linguistics
- Cognitive Linguistics
- Computational Linguistics
- Forensic Linguistics
- Grammar, Syntax and Morphology
- Historical and Diachronic Linguistics
- History of English
- Language Evolution
- Language Reference
- Language Acquisition
- Language Variation
- Language Families
- Lexicography
- Linguistic Anthropology
- Linguistic Theories
- Linguistic Typology
- Phonetics and Phonology
- Psycholinguistics
- Sociolinguistics
- Translation and Interpretation
- Writing Systems
- Browse content in Literature
- Bibliography
- Children's Literature Studies
- Literary Studies (Romanticism)
- Literary Studies (American)
- Literary Studies (Asian)
- Literary Studies (European)
- Literary Studies (Eco-criticism)
- Literary Studies (Modernism)
- Literary Studies - World
- Literary Studies (1500 to 1800)
- Literary Studies (19th Century)
- Literary Studies (20th Century onwards)
- Literary Studies (African American Literature)
- Literary Studies (British and Irish)
- Literary Studies (Early and Medieval)
- Literary Studies (Fiction, Novelists, and Prose Writers)
- Literary Studies (Gender Studies)
- Literary Studies (Graphic Novels)
- Literary Studies (History of the Book)
- Literary Studies (Plays and Playwrights)
- Literary Studies (Poetry and Poets)
- Literary Studies (Postcolonial Literature)
- Literary Studies (Queer Studies)
- Literary Studies (Science Fiction)
- Literary Studies (Travel Literature)
- Literary Studies (War Literature)
- Literary Studies (Women's Writing)
- Literary Theory and Cultural Studies
- Mythology and Folklore
- Shakespeare Studies and Criticism
- Browse content in Media Studies
- Browse content in Music
- Applied Music
- Dance and Music
- Ethics in Music
- Ethnomusicology
- Gender and Sexuality in Music
- Medicine and Music
- Music Cultures
- Music and Media
- Music and Religion
- Music and Culture
- Music Education and Pedagogy
- Music Theory and Analysis
- Musical Scores, Lyrics, and Libretti
- Musical Structures, Styles, and Techniques
- Musicology and Music History
- Performance Practice and Studies
- Race and Ethnicity in Music
- Sound Studies
- Browse content in Performing Arts
- Browse content in Philosophy
- Aesthetics and Philosophy of Art
- Epistemology
- Feminist Philosophy
- History of Western Philosophy
- Metaphysics
- Moral Philosophy
- Non-Western Philosophy
- Philosophy of Language
- Philosophy of Mind
- Philosophy of Perception
- Philosophy of Science
- Philosophy of Action
- Philosophy of Law
- Philosophy of Religion
- Philosophy of Mathematics and Logic
- Practical Ethics
- Social and Political Philosophy
- Browse content in Religion
- Biblical Studies
- Christianity
- East Asian Religions
- History of Religion
- Judaism and Jewish Studies
- Qumran Studies
- Religion and Education
- Religion and Health
- Religion and Politics
- Religion and Science
- Religion and Law
- Religion and Art, Literature, and Music
- Religious Studies
- Browse content in Society and Culture
- Cookery, Food, and Drink
- Cultural Studies
- Customs and Traditions
- Ethical Issues and Debates
- Hobbies, Games, Arts and Crafts
- Natural world, Country Life, and Pets
- Popular Beliefs and Controversial Knowledge
- Sports and Outdoor Recreation
- Technology and Society
- Travel and Holiday
- Visual Culture
- Browse content in Law
- Arbitration
- Browse content in Company and Commercial Law
- Commercial Law
- Company Law
- Browse content in Comparative Law
- Systems of Law
- Competition Law
- Browse content in Constitutional and Administrative Law
- Government Powers
- Judicial Review
- Local Government Law
- Military and Defence Law
- Parliamentary and Legislative Practice
- Construction Law
- Contract Law
- Browse content in Criminal Law
- Criminal Procedure
- Criminal Evidence Law
- Sentencing and Punishment
- Employment and Labour Law
- Environment and Energy Law
- Browse content in Financial Law
- Banking Law
- Insolvency Law
- History of Law
- Human Rights and Immigration
- Intellectual Property Law
- Browse content in International Law
- Private International Law and Conflict of Laws
- Public International Law
- IT and Communications Law
- Jurisprudence and Philosophy of Law
- Law and Politics
- Law and Society
- Browse content in Legal System and Practice
- Courts and Procedure
- Legal Skills and Practice
- Legal System - Costs and Funding
- Primary Sources of Law
- Regulation of Legal Profession
- Medical and Healthcare Law
- Browse content in Policing
- Criminal Investigation and Detection
- Police and Security Services
- Police Procedure and Law
- Police Regional Planning
- Browse content in Property Law
- Personal Property Law
- Restitution
- Study and Revision
- Terrorism and National Security Law
- Browse content in Trusts Law
- Wills and Probate or Succession
- Browse content in Medicine and Health
- Browse content in Allied Health Professions
- Arts Therapies
- Clinical Science
- Dietetics and Nutrition
- Occupational Therapy
- Operating Department Practice
- Physiotherapy
- Radiography
- Speech and Language Therapy
- Browse content in Anaesthetics
- General Anaesthesia
- Clinical Neuroscience
- Browse content in Clinical Medicine
- Acute Medicine
- Cardiovascular Medicine
- Clinical Genetics
- Clinical Pharmacology and Therapeutics
- Dermatology
- Endocrinology and Diabetes
- Gastroenterology
- Genito-urinary Medicine
- Geriatric Medicine
- Infectious Diseases
- Medical Toxicology
- Medical Oncology
- Pain Medicine
- Palliative Medicine
- Rehabilitation Medicine
- Respiratory Medicine and Pulmonology
- Rheumatology
- Sleep Medicine
- Sports and Exercise Medicine
- Community Medical Services
- Critical Care
- Emergency Medicine
- Forensic Medicine
- Haematology
- History of Medicine
- Browse content in Medical Skills
- Clinical Skills
- Communication Skills
- Nursing Skills
- Surgical Skills
- Browse content in Medical Dentistry
- Oral and Maxillofacial Surgery
- Paediatric Dentistry
- Restorative Dentistry and Orthodontics
- Surgical Dentistry
- Medical Ethics
- Medical Statistics and Methodology
- Browse content in Neurology
- Clinical Neurophysiology
- Neuropathology
- Nursing Studies
- Browse content in Obstetrics and Gynaecology
- Gynaecology
- Occupational Medicine
- Ophthalmology
- Otolaryngology (ENT)
- Browse content in Paediatrics
- Neonatology
- Browse content in Pathology
- Chemical Pathology
- Clinical Cytogenetics and Molecular Genetics
- Histopathology
- Medical Microbiology and Virology
- Patient Education and Information
- Browse content in Pharmacology
- Psychopharmacology
- Browse content in Popular Health
- Caring for Others
- Complementary and Alternative Medicine
- Self-help and Personal Development
- Browse content in Preclinical Medicine
- Cell Biology
- Molecular Biology and Genetics
- Reproduction, Growth and Development
- Primary Care
- Professional Development in Medicine
- Browse content in Psychiatry
- Addiction Medicine
- Child and Adolescent Psychiatry
- Forensic Psychiatry
- Learning Disabilities
- Old Age Psychiatry
- Psychotherapy
- Browse content in Public Health and Epidemiology
- Epidemiology
- Public Health
- Browse content in Radiology
- Clinical Radiology
- Interventional Radiology
- Nuclear Medicine
- Radiation Oncology
- Reproductive Medicine
- Browse content in Surgery
- Cardiothoracic Surgery
- Gastro-intestinal and Colorectal Surgery
- General Surgery
- Neurosurgery
- Paediatric Surgery
- Peri-operative Care
- Plastic and Reconstructive Surgery
- Surgical Oncology
- Transplant Surgery
- Trauma and Orthopaedic Surgery
- Vascular Surgery
- Browse content in Science and Mathematics
- Browse content in Biological Sciences
- Aquatic Biology
- Biochemistry
- Bioinformatics and Computational Biology
- Developmental Biology
- Ecology and Conservation
- Evolutionary Biology
- Genetics and Genomics
- Microbiology
- Molecular and Cell Biology
- Natural History
- Plant Sciences and Forestry
- Research Methods in Life Sciences
- Structural Biology
- Systems Biology
- Zoology and Animal Sciences
- Browse content in Chemistry
- Analytical Chemistry
- Computational Chemistry
- Crystallography
- Environmental Chemistry
- Industrial Chemistry
- Inorganic Chemistry
- Materials Chemistry
- Medicinal Chemistry
- Mineralogy and Gems
- Organic Chemistry
- Physical Chemistry
- Polymer Chemistry
- Study and Communication Skills in Chemistry
- Theoretical Chemistry
- Browse content in Computer Science
- Artificial Intelligence
- Computer Architecture and Logic Design
- Game Studies
- Human-Computer Interaction
- Mathematical Theory of Computation
- Programming Languages
- Software Engineering
- Systems Analysis and Design
- Virtual Reality
- Browse content in Computing
- Business Applications
- Computer Security
- Computer Games
- Computer Networking and Communications
- Digital Lifestyle
- Graphical and Digital Media Applications
- Operating Systems
- Browse content in Earth Sciences and Geography
- Atmospheric Sciences
- Environmental Geography
- Geology and the Lithosphere
- Maps and Map-making
- Meteorology and Climatology
- Oceanography and Hydrology
- Palaeontology
- Physical Geography and Topography
- Regional Geography
- Soil Science
- Urban Geography
- Browse content in Engineering and Technology
- Agriculture and Farming
- Biological Engineering
- Civil Engineering, Surveying, and Building
- Electronics and Communications Engineering
- Energy Technology
- Engineering (General)
- Environmental Science, Engineering, and Technology
- History of Engineering and Technology
- Mechanical Engineering and Materials
- Technology of Industrial Chemistry
- Transport Technology and Trades
- Browse content in Environmental Science
- Applied Ecology (Environmental Science)
- Conservation of the Environment (Environmental Science)
- Environmental Sustainability
- Environmentalist Thought and Ideology (Environmental Science)
- Management of Land and Natural Resources (Environmental Science)
- Natural Disasters (Environmental Science)
- Nuclear Issues (Environmental Science)
- Pollution and Threats to the Environment (Environmental Science)
- Social Impact of Environmental Issues (Environmental Science)
- History of Science and Technology
- Browse content in Materials Science
- Ceramics and Glasses
- Composite Materials
- Metals, Alloying, and Corrosion
- Nanotechnology
- Browse content in Mathematics
- Applied Mathematics
- Biomathematics and Statistics
- History of Mathematics
- Mathematical Education
- Mathematical Finance
- Mathematical Analysis
- Numerical and Computational Mathematics
- Probability and Statistics
- Pure Mathematics
- Browse content in Neuroscience
- Cognition and Behavioural Neuroscience
- Development of the Nervous System
- Disorders of the Nervous System
- History of Neuroscience
- Invertebrate Neurobiology
- Molecular and Cellular Systems
- Neuroendocrinology and Autonomic Nervous System
- Neuroscientific Techniques
- Sensory and Motor Systems
- Browse content in Physics
- Astronomy and Astrophysics
- Atomic, Molecular, and Optical Physics
- Biological and Medical Physics
- Classical Mechanics
- Computational Physics
- Condensed Matter Physics
- Electromagnetism, Optics, and Acoustics
- History of Physics
- Mathematical and Statistical Physics
- Measurement Science
- Nuclear Physics
- Particles and Fields
- Plasma Physics
- Quantum Physics
- Relativity and Gravitation
- Semiconductor and Mesoscopic Physics
- Browse content in Psychology
- Affective Sciences
- Clinical Psychology
- Cognitive Psychology
- Cognitive Neuroscience
- Criminal and Forensic Psychology
- Developmental Psychology
- Educational Psychology
- Evolutionary Psychology
- Health Psychology
- History and Systems in Psychology
- Music Psychology
- Neuropsychology
- Organizational Psychology
- Psychological Assessment and Testing
- Psychology of Human-Technology Interaction
- Psychology Professional Development and Training
- Research Methods in Psychology
- Social Psychology
- Browse content in Social Sciences
- Browse content in Anthropology
- Anthropology of Religion
- Human Evolution
- Medical Anthropology
- Physical Anthropology
- Regional Anthropology
- Social and Cultural Anthropology
- Theory and Practice of Anthropology
- Browse content in Business and Management
- Business Ethics
- Business Strategy
- Business History
- Business and Technology
- Business and Government
- Business and the Environment
- Comparative Management
- Corporate Governance
- Corporate Social Responsibility
- Entrepreneurship
- Health Management
- Human Resource Management
- Industrial and Employment Relations
- Industry Studies
- Information and Communication Technologies
- International Business
- Knowledge Management
- Management and Management Techniques
- Operations Management
- Organizational Theory and Behaviour
- Pensions and Pension Management
- Public and Nonprofit Management
- Social Issues in Business and Management
- Strategic Management
- Supply Chain Management
- Browse content in Criminology and Criminal Justice
- Criminal Justice
- Criminology
- Forms of Crime
- International and Comparative Criminology
- Youth Violence and Juvenile Justice
- Development Studies
- Browse content in Economics
- Agricultural, Environmental, and Natural Resource Economics
- Asian Economics
- Behavioural Finance
- Behavioural Economics and Neuroeconomics
- Econometrics and Mathematical Economics
- Economic History
- Economic Systems
- Economic Methodology
- Economic Development and Growth
- Financial Markets
- Financial Institutions and Services
- General Economics and Teaching
- Health, Education, and Welfare
- History of Economic Thought
- International Economics
- Labour and Demographic Economics
- Law and Economics
- Macroeconomics and Monetary Economics
- Microeconomics
- Public Economics
- Urban, Rural, and Regional Economics
- Welfare Economics
- Browse content in Education
- Adult Education and Continuous Learning
- Care and Counselling of Students
- Early Childhood and Elementary Education
- Educational Equipment and Technology
- Educational Strategies and Policy
- Higher and Further Education
- Organization and Management of Education
- Philosophy and Theory of Education
- Schools Studies
- Secondary Education
- Teaching of a Specific Subject
- Teaching of Specific Groups and Special Educational Needs
- Teaching Skills and Techniques
- Browse content in Environment
- Applied Ecology (Social Science)
- Climate Change
- Conservation of the Environment (Social Science)
- Environmentalist Thought and Ideology (Social Science)
- Management of Land and Natural Resources (Social Science)
- Natural Disasters (Environment)
- Pollution and Threats to the Environment (Social Science)
- Social Impact of Environmental Issues (Social Science)
- Sustainability
- Browse content in Human Geography
- Cultural Geography
- Economic Geography
- Political Geography
- Browse content in Interdisciplinary Studies
- Communication Studies
- Museums, Libraries, and Information Sciences
- Browse content in Politics
- African Politics
- Asian Politics
- Chinese Politics
- Comparative Politics
- Conflict Politics
- Elections and Electoral Studies
- Environmental Politics
- Ethnic Politics
- European Union
- Foreign Policy
- Gender and Politics
- Human Rights and Politics
- Indian Politics
- International Relations
- International Organization (Politics)
- Irish Politics
- Latin American Politics
- Middle Eastern Politics
- Political Behaviour
- Political Economy
- Political Institutions
- Political Methodology
- Political Communication
- Political Philosophy
- Political Sociology
- Political Theory
- Politics and Law
- Politics of Development
- Public Policy
- Public Administration
- Qualitative Political Methodology
- Quantitative Political Methodology
- Regional Political Studies
- Russian Politics
- Security Studies
- State and Local Government
- UK Politics
- US Politics
- Browse content in Regional and Area Studies
- African Studies
- Asian Studies
- East Asian Studies
- Japanese Studies
- Latin American Studies
- Middle Eastern Studies
- Native American Studies
- Scottish Studies
- Browse content in Research and Information
- Research Methods
- Browse content in Social Work
- Addictions and Substance Misuse
- Adoption and Fostering
- Care of the Elderly
- Child and Adolescent Social Work
- Couple and Family Social Work
- Direct Practice and Clinical Social Work
- Emergency Services
- Human Behaviour and the Social Environment
- International and Global Issues in Social Work
- Mental and Behavioural Health
- Social Justice and Human Rights
- Social Policy and Advocacy
- Social Work and Crime and Justice
- Social Work Macro Practice
- Social Work Practice Settings
- Social Work Research and Evidence-based Practice
- Welfare and Benefit Systems
- Browse content in Sociology
- Childhood Studies
- Community Development
- Comparative and Historical Sociology
- Disability Studies
- Economic Sociology
- Gender and Sexuality
- Gerontology and Ageing
- Health, Illness, and Medicine
- Marriage and the Family
- Migration Studies
- Occupations, Professions, and Work
- Organizations
- Population and Demography
- Race and Ethnicity
- Social Theory
- Social Movements and Social Change
- Social Research and Statistics
- Social Stratification, Inequality, and Mobility
- Sociology of Religion
- Sociology of Education
- Sport and Leisure
- Urban and Rural Studies
- Browse content in Warfare and Defence
- Defence Strategy, Planning, and Research
- Land Forces and Warfare
- Military Administration
- Military Life and Institutions
- Naval Forces and Warfare
- Other Warfare and Defence Issues
- Peace Studies and Conflict Resolution
- Weapons and Equipment

- < Previous chapter
- Next chapter >
4 Descriptive Analysis
- Published: March 2018
- Cite Icon Cite
- Permissions Icon Permissions
Descriptive statistics allow researchers to use numbers to begin to tell the stories that exist in their data. This chapter presents an overview of the basic statistical tools researchers can use to summarize, display, and interpret data. The chapter presents guidelines for interpreting data and examples of typical sorts of data that music education researchers may gather. Statistical analyses suitable for identifying how data are distributed, determining typical values of a distribution, and describing how individuals differ on measured variables of interest are described. Approaches for graphing data that are appropriate for variables of different measurement scales are also described.
Personal account
- Sign in with email/username & password
- Get email alerts
- Save searches
- Purchase content
- Activate your purchase/trial code
- Add your ORCID iD
Institutional access
Sign in with a library card.
- Sign in with username/password
- Recommend to your librarian
- Institutional account management
- Get help with access
Access to content on Oxford Academic is often provided through institutional subscriptions and purchases. If you are a member of an institution with an active account, you may be able to access content in one of the following ways:
IP based access
Typically, access is provided across an institutional network to a range of IP addresses. This authentication occurs automatically, and it is not possible to sign out of an IP authenticated account.
Choose this option to get remote access when outside your institution. Shibboleth/Open Athens technology is used to provide single sign-on between your institution’s website and Oxford Academic.
- Click Sign in through your institution.
- Select your institution from the list provided, which will take you to your institution's website to sign in.
- When on the institution site, please use the credentials provided by your institution. Do not use an Oxford Academic personal account.
- Following successful sign in, you will be returned to Oxford Academic.
If your institution is not listed or you cannot sign in to your institution’s website, please contact your librarian or administrator.
Enter your library card number to sign in. If you cannot sign in, please contact your librarian.
Society Members
Society member access to a journal is achieved in one of the following ways:
Sign in through society site
Many societies offer single sign-on between the society website and Oxford Academic. If you see ‘Sign in through society site’ in the sign in pane within a journal:
- Click Sign in through society site.
- When on the society site, please use the credentials provided by that society. Do not use an Oxford Academic personal account.
If you do not have a society account or have forgotten your username or password, please contact your society.
Sign in using a personal account
Some societies use Oxford Academic personal accounts to provide access to their members. See below.
A personal account can be used to get email alerts, save searches, purchase content, and activate subscriptions.
Some societies use Oxford Academic personal accounts to provide access to their members.
Viewing your signed in accounts
Click the account icon in the top right to:
- View your signed in personal account and access account management features.
- View the institutional accounts that are providing access.
Signed in but can't access content
Oxford Academic is home to a wide variety of products. The institutional subscription may not cover the content that you are trying to access. If you believe you should have access to that content, please contact your librarian.
For librarians and administrators, your personal account also provides access to institutional account management. Here you will find options to view and activate subscriptions, manage institutional settings and access options, access usage statistics, and more.
Our books are available by subscription or purchase to libraries and institutions.
Month: | Total Views: |
---|---|
October 2022 | 11 |
November 2022 | 13 |
December 2022 | 4 |
January 2023 | 16 |
February 2023 | 6 |
March 2023 | 2 |
April 2023 | 3 |
May 2023 | 2 |
June 2023 | 2 |
July 2023 | 3 |
August 2023 | 8 |
September 2023 | 14 |
October 2023 | 1 |
November 2023 | 5 |
December 2023 | 6 |
January 2024 | 6 |
February 2024 | 2 |
March 2024 | 3 |
April 2024 | 14 |
May 2024 | 11 |
June 2024 | 2 |
July 2024 | 8 |
- About Oxford Academic
- Publish journals with us
- University press partners
- What we publish
- New features
- Open access
- Rights and permissions
- Accessibility
- Advertising
- Media enquiries
- Oxford University Press
- Oxford Languages
- University of Oxford
Oxford University Press is a department of the University of Oxford. It furthers the University's objective of excellence in research, scholarship, and education by publishing worldwide
- Copyright © 2024 Oxford University Press
- Cookie settings
- Cookie policy
- Privacy policy
- Legal notice
This Feature Is Available To Subscribers Only
Sign In or Create an Account
This PDF is available to Subscribers Only
For full access to this pdf, sign in to an existing account, or purchase an annual subscription.

An official website of the United States government
The .gov means it’s official. Federal government websites often end in .gov or .mil. Before sharing sensitive information, make sure you’re on a federal government site.
The site is secure. The https:// ensures that you are connecting to the official website and that any information you provide is encrypted and transmitted securely.
- Publications
- Account settings
Preview improvements coming to the PMC website in October 2024. Learn More or Try it out now .
- Advanced Search
- Journal List
- Indian Dermatol Online J
- v.10(1); Jan-Feb 2019
Types of Variables, Descriptive Statistics, and Sample Size
Feroze kaliyadan.
Department of Dermatology, King Faisal University, Al Hofuf, Saudi Arabia
Vinay Kulkarni
1 Department of Dermatology, Prayas Amrita Clinic, Pune, Maharashtra, India
This short “snippet” covers three important aspects related to statistics – the concept of variables , the importance, and practical aspects related to descriptive statistics and issues related to sampling – types of sampling and sample size estimation.
What is a variable?[ 1 , 2 ] To put it in very simple terms, a variable is an entity whose value varies. A variable is an essential component of any statistical data. It is a feature of a member of a given sample or population, which is unique, and can differ in quantity or quantity from another member of the same sample or population. Variables either are the primary quantities of interest or act as practical substitutes for the same. The importance of variables is that they help in operationalization of concepts for data collection. For example, if you want to do an experiment based on the severity of urticaria, one option would be to measure the severity using a scale to grade severity of itching. This becomes an operational variable. For a variable to be “good,” it needs to have some properties such as good reliability and validity, low bias, feasibility/practicality, low cost, objectivity, clarity, and acceptance. Variables can be classified into various ways as discussed below.
Quantitative vs qualitative
A variable can collect either qualitative or quantitative data. A variable differing in quantity is called a quantitative variable (e.g., weight of a group of patients), whereas a variable differing in quality is called a qualitative variable (e.g., the Fitzpatrick skin type)
A simple test which can be used to differentiate between qualitative and quantitative variables is the subtraction test. If you can subtract the value of one variable from the other to get a meaningful result, then you are dealing with a quantitative variable (this of course will not apply to rating scales/ranks).
Quantitative variables can be either discrete or continuous
Discrete variables are variables in which no values may be assumed between the two given values (e.g., number of lesions in each patient in a sample of patients with urticaria).
Continuous variables, on the other hand, can take any value in between the two given values (e.g., duration for which the weals last in the same sample of patients with urticaria). One way of differentiating between continuous and discrete variables is to use the “mid-way” test. If, for every pair of values of a variable, a value exactly mid-way between them is meaningful, the variable is continuous. For example, two values for the time taken for a weal to subside can be 10 and 13 min. The mid-way value would be 11.5 min which makes sense. However, for a number of weals, suppose you have a pair of values – 5 and 8 – the midway value would be 6.5 weals, which does not make sense.
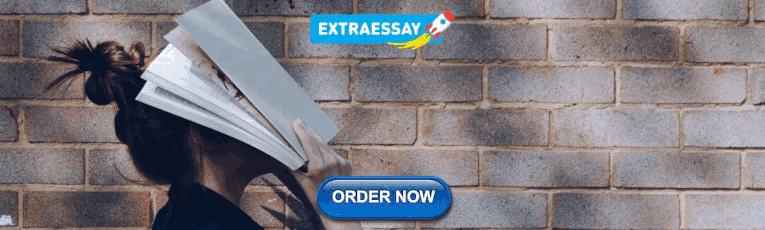
Under the umbrella of qualitative variables, you can have nominal/categorical variables and ordinal variables
Nominal/categorical variables are, as the name suggests, variables which can be slotted into different categories (e.g., gender or type of psoriasis).
Ordinal variables or ranked variables are similar to categorical, but can be put into an order (e.g., a scale for severity of itching).
Dependent and independent variables
In the context of an experimental study, the dependent variable (also called outcome variable) is directly linked to the primary outcome of the study. For example, in a clinical trial on psoriasis, the PASI (psoriasis area severity index) would possibly be one dependent variable. The independent variable (sometime also called explanatory variable) is something which is not affected by the experiment itself but which can be manipulated to affect the dependent variable. Other terms sometimes used synonymously include blocking variable, covariate, or predictor variable. Confounding variables are extra variables, which can have an effect on the experiment. They are linked with dependent and independent variables and can cause spurious association. For example, in a clinical trial for a topical treatment in psoriasis, the concomitant use of moisturizers might be a confounding variable. A control variable is a variable that must be kept constant during the course of an experiment.
Descriptive Statistics
Statistics can be broadly divided into descriptive statistics and inferential statistics.[ 3 , 4 ] Descriptive statistics give a summary about the sample being studied without drawing any inferences based on probability theory. Even if the primary aim of a study involves inferential statistics, descriptive statistics are still used to give a general summary. When we describe the population using tools such as frequency distribution tables, percentages, and other measures of central tendency like the mean, for example, we are talking about descriptive statistics. When we use a specific statistical test (e.g., Mann–Whitney U-test) to compare the mean scores and express it in terms of statistical significance, we are talking about inferential statistics. Descriptive statistics can help in summarizing data in the form of simple quantitative measures such as percentages or means or in the form of visual summaries such as histograms and box plots.
Descriptive statistics can be used to describe a single variable (univariate analysis) or more than one variable (bivariate/multivariate analysis). In the case of more than one variable, descriptive statistics can help summarize relationships between variables using tools such as scatter plots.
Descriptive statistics can be broadly put under two categories:
- Sorting/grouping and illustration/visual displays
- Summary statistics.
Sorting and grouping
Sorting and grouping is most commonly done using frequency distribution tables. For continuous variables, it is generally better to use groups in the frequency table. Ideally, group sizes should be equal (except in extreme ends where open groups are used; e.g., age “greater than” or “less than”).
Another form of presenting frequency distributions is the “stem and leaf” diagram, which is considered to be a more accurate form of description.
Suppose the weight in kilograms of a group of 10 patients is as follows:
56, 34, 48, 43, 87, 78, 54, 62, 61, 59
The “stem” records the value of the “ten's” place (or higher) and the “leaf” records the value in the “one's” place [ Table 1 ].
Stem and leaf plot
0 | - |
1 | - |
2 | - |
3 | 4 |
4 | 3 8 |
5 | 4 6 9 |
6 | 1 2 |
7 | 8 |
8 | 7 |
9 | - |
Illustration/visual display of data
The most common tools used for visual display include frequency diagrams, bar charts (for noncontinuous variables) and histograms (for continuous variables). Composite bar charts can be used to compare variables. For example, the frequency distribution in a sample population of males and females can be illustrated as given in Figure 1 .

Composite bar chart
A pie chart helps show how a total quantity is divided among its constituent variables. Scatter diagrams can be used to illustrate the relationship between two variables. For example, global scores given for improvement in a condition like acne by the patient and the doctor [ Figure 2 ].

Scatter diagram
Summary statistics
The main tools used for summary statistics are broadly grouped into measures of central tendency (such as mean, median, and mode) and measures of dispersion or variation (such as range, standard deviation, and variance).
Imagine that the data below represent the weights of a sample of 15 pediatric patients arranged in ascending order:
30, 35, 37, 38, 38, 38, 42, 42, 44, 46, 47, 48, 51, 53, 86
Just having the raw data does not mean much to us, so we try to express it in terms of some values, which give a summary of the data.
The mean is basically the sum of all the values divided by the total number. In this case, we get a value of 45.
The problem is that some extreme values (outliers), like “'86,” in this case can skew the value of the mean. In this case, we consider other values like the median, which is the point that divides the distribution into two equal halves. It is also referred to as the 50 th percentile (50% of the values are above it and 50% are below it). In our previous example, since we have already arranged the values in ascending order we find that the point which divides it into two equal halves is the 8 th value – 42. In case of a total number of values being even, we choose the two middle points and take an average to reach the median.
The mode is the most common data point. In our example, this would be 38. The mode as in our case may not necessarily be in the center of the distribution.
The median is the best measure of central tendency from among the mean, median, and mode. In a “symmetric” distribution, all three are the same, whereas in skewed data the median and mean are not the same; lie more toward the skew, with the mean lying further to the skew compared with the median. For example, in Figure 3 , a right skewed distribution is seen (direction of skew is based on the tail); data values' distribution is longer on the right-hand (positive) side than on the left-hand side. The mean is typically greater than the median in such cases.

Location of mode, median, and mean
Measures of dispersion
The range gives the spread between the lowest and highest values. In our previous example, this will be 86-30 = 56.
A more valuable measure is the interquartile range. A quartile is one of the values which break the distribution into four equal parts. The 25 th percentile is the data point which divides the group between the first one-fourth and the last three-fourth of the data. The first one-fourth will form the first quartile. The 75 th percentile is the data point which divides the distribution into a first three-fourth and last one-fourth (the last one-fourth being the fourth quartile). The range between the 25 th percentile and 75 th percentile is called the interquartile range.
Variance is also a measure of dispersion. The larger the variance, the further the individual units are from the mean. Let us consider the same example we used for calculating the mean. The mean was 45.
For the first value (30), the deviation from the mean will be 15; for the last value (86), the deviation will be 41. Similarly we can calculate the deviations for all values in a sample. Adding these deviations and averaging will give a clue to the total dispersion, but the problem is that since the deviations are a mix of negative and positive values, the final total becomes zero. To calculate the variance, this problem is overcome by adding squares of the deviations. So variance would be the sum of squares of the variation divided by the total number in the population (for a sample we use “n − 1”). To get a more realistic value of the average dispersion, we take the square root of the variance, which is called the “standard deviation.”
The box plot
The box plot is a composite representation that portrays the mean, median, range, and the outliers [ Figure 4 ].

The concept of skewness and kurtosis
Skewness is a measure of the symmetry of distribution. Basically if the distribution curve is symmetric, it looks the same on either side of the central point. When this is not the case, it is said to be skewed. Kurtosis is a representation of outliers. Distributions with high kurtosis tend to have “heavy tails” indicating a larger number of outliers, whereas distributions with low kurtosis have light tails, indicating lesser outliers. There are formulas to calculate both skewness and kurtosis [Figures [Figures5 5 – 8 ].

Positive skew

High kurtosis (positive kurtosis – also called leptokurtic)

Negative skew

Low kurtosis (negative kurtosis – also called “Platykurtic”)
Sample Size
In an ideal study, we should be able to include all units of a particular population under study, something that is referred to as a census.[ 5 , 6 ] This would remove the chances of sampling error (difference between the outcome characteristics in a random sample when compared with the true population values – something that is virtually unavoidable when you take a random sample). However, it is obvious that this would not be feasible in most situations. Hence, we have to study a subset of the population to reach to our conclusions. This representative subset is a sample and we need to have sufficient numbers in this sample to make meaningful and accurate conclusions and reduce the effect of sampling error.
We also need to know that broadly sampling can be divided into two types – probability sampling and nonprobability sampling. Examples of probability sampling include methods such as simple random sampling (each member in a population has an equal chance of being selected), stratified random sampling (in nonhomogeneous populations, the population is divided into subgroups – followed be random sampling in each subgroup), systematic (sampling is based on a systematic technique – e.g., every third person is selected for a survey), and cluster sampling (similar to stratified sampling except that the clusters here are preexisting clusters unlike stratified sampling where the researcher decides on the stratification criteria), whereas nonprobability sampling, where every unit in the population does not have an equal chance of inclusion into the sample, includes methods such as convenience sampling (e.g., sample selected based on ease of access) and purposive sampling (where only people who meet specific criteria are included in the sample).
An accurate calculation of sample size is an essential aspect of good study design. It is important to calculate the sample size much in advance, rather than have to go for post hoc analysis. A sample size that is too less may make the study underpowered, whereas a sample size which is more than necessary might lead to a wastage of resources.
We will first go through the sample size calculation for a hypothesis-based design (like a randomized control trial).
The important factors to consider for sample size calculation include study design, type of statistical test, level of significance, power and effect size, variance (standard deviation for quantitative data), and expected proportions in the case of qualitative data. This is based on previous data, either based on previous studies or based on the clinicians' experience. In case the study is something being conducted for the first time, a pilot study might be conducted which helps generate these data for further studies based on a larger sample size). It is also important to know whether the data follow a normal distribution or not.
Two essential aspects we must understand are the concept of Type I and Type II errors. In a study that compares two groups, a null hypothesis assumes that there is no significant difference between the two groups, and any observed difference being due to sampling or experimental error. When we reject a null hypothesis, when it is true, we label it as a Type I error (also denoted as “alpha,” correlating with significance levels). In a Type II error (also denoted as “beta”), we fail to reject a null hypothesis, when the alternate hypothesis is actually true. Type II errors are usually expressed as “1- β,” correlating with the power of the test. While there are no absolute rules, the minimal levels accepted are 0.05 for α (corresponding to a significance level of 5%) and 0.20 for β (corresponding to a minimum recommended power of “1 − 0.20,” or 80%).
Effect size and minimal clinically relevant difference
For a clinical trial, the investigator will have to decide in advance what clinically detectable change is significant (for numerical data, this is could be the anticipated outcome means in the two groups, whereas for categorical data, it could correlate with the proportions of successful outcomes in two groups.). While we will not go into details of the formula for sample size calculation, some important points are as follows:
In the context where effect size is involved, the sample size is inversely proportional to the square of the effect size. What this means in effect is that reducing the effect size will lead to an increase in the required sample size.
Reducing the level of significance (alpha) or increasing power (1-β) will lead to an increase in the calculated sample size.
An increase in variance of the outcome leads to an increase in the calculated sample size.
A note is that for estimation type of studies/surveys, sample size calculation needs to consider some other factors too. This includes an idea about total population size (this generally does not make a major difference when population size is above 20,000, so in situations where population size is not known we can assume a population of 20,000 or more). The other factor is the “margin of error” – the amount of deviation which the investigators find acceptable in terms of percentages. Regarding confidence levels, ideally, a 95% confidence level is the minimum recommended for surveys too. Finally, we need an idea of the expected/crude prevalence – either based on previous studies or based on estimates.
Sample size calculation also needs to add corrections for patient drop-outs/lost-to-follow-up patients and missing records. An important point is that in some studies dealing with rare diseases, it may be difficult to achieve desired sample size. In these cases, the investigators might have to rework outcomes or maybe pool data from multiple centers. Although post hoc power can be analyzed, a better approach suggested is to calculate 95% confidence intervals for the outcome and interpret the study results based on this.
Financial support and sponsorship
Conflicts of interest.
There are no conflicts of interest.
Have a language expert improve your writing
Run a free plagiarism check in 10 minutes, generate accurate citations for free.
- Knowledge Base
Methodology
- Descriptive Research | Definition, Types, Methods & Examples
Descriptive Research | Definition, Types, Methods & Examples
Published on May 15, 2019 by Shona McCombes . Revised on June 22, 2023.
Descriptive research aims to accurately and systematically describe a population, situation or phenomenon. It can answer what , where , when and how questions , but not why questions.
A descriptive research design can use a wide variety of research methods to investigate one or more variables . Unlike in experimental research , the researcher does not control or manipulate any of the variables, but only observes and measures them.
Table of contents
When to use a descriptive research design, descriptive research methods, other interesting articles.
Descriptive research is an appropriate choice when the research aim is to identify characteristics, frequencies, trends, and categories.
It is useful when not much is known yet about the topic or problem. Before you can research why something happens, you need to understand how, when and where it happens.
Descriptive research question examples
- How has the Amsterdam housing market changed over the past 20 years?
- Do customers of company X prefer product X or product Y?
- What are the main genetic, behavioural and morphological differences between European wildcats and domestic cats?
- What are the most popular online news sources among under-18s?
- How prevalent is disease A in population B?
Prevent plagiarism. Run a free check.
Descriptive research is usually defined as a type of quantitative research , though qualitative research can also be used for descriptive purposes. The research design should be carefully developed to ensure that the results are valid and reliable .
Survey research allows you to gather large volumes of data that can be analyzed for frequencies, averages and patterns. Common uses of surveys include:
- Describing the demographics of a country or region
- Gauging public opinion on political and social topics
- Evaluating satisfaction with a company’s products or an organization’s services
Observations
Observations allow you to gather data on behaviours and phenomena without having to rely on the honesty and accuracy of respondents. This method is often used by psychological, social and market researchers to understand how people act in real-life situations.
Observation of physical entities and phenomena is also an important part of research in the natural sciences. Before you can develop testable hypotheses , models or theories, it’s necessary to observe and systematically describe the subject under investigation.
Case studies
A case study can be used to describe the characteristics of a specific subject (such as a person, group, event or organization). Instead of gathering a large volume of data to identify patterns across time or location, case studies gather detailed data to identify the characteristics of a narrowly defined subject.
Rather than aiming to describe generalizable facts, case studies often focus on unusual or interesting cases that challenge assumptions, add complexity, or reveal something new about a research problem .
If you want to know more about statistics , methodology , or research bias , make sure to check out some of our other articles with explanations and examples.
- Normal distribution
- Degrees of freedom
- Null hypothesis
- Discourse analysis
- Control groups
- Mixed methods research
- Non-probability sampling
- Quantitative research
- Ecological validity
Research bias
- Rosenthal effect
- Implicit bias
- Cognitive bias
- Selection bias
- Negativity bias
- Status quo bias
Cite this Scribbr article
If you want to cite this source, you can copy and paste the citation or click the “Cite this Scribbr article” button to automatically add the citation to our free Citation Generator.
McCombes, S. (2023, June 22). Descriptive Research | Definition, Types, Methods & Examples. Scribbr. Retrieved August 11, 2024, from https://www.scribbr.com/methodology/descriptive-research/
Is this article helpful?
Shona McCombes
Other students also liked, what is quantitative research | definition, uses & methods, correlational research | when & how to use, descriptive statistics | definitions, types, examples, get unlimited documents corrected.
✔ Free APA citation check included ✔ Unlimited document corrections ✔ Specialized in correcting academic texts
Academia.edu no longer supports Internet Explorer.
To browse Academia.edu and the wider internet faster and more securely, please take a few seconds to upgrade your browser .
Enter the email address you signed up with and we'll email you a reset link.
- We're Hiring!
- Help Center
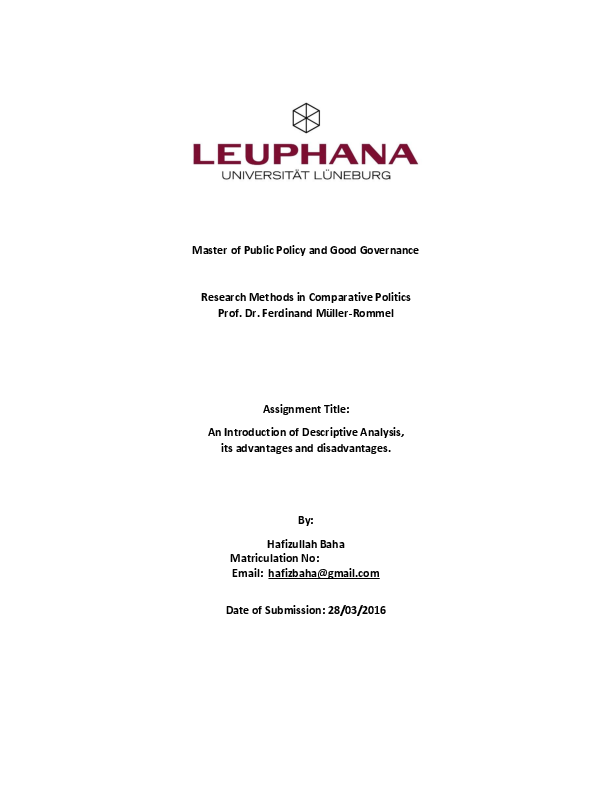
Title : An Introduction on Descriptive Analysis; Its advantages and disadvantages

Research is a crucial tool for leading man towards achieving progress, findings new facts, new concepts and discovering truths which leads to better ways of doing things. In the other words, “research is a diligent search, studious inquiry, investigation, experiment or collection of information, interpretation of facts, revision of existing theories and laws aimed at discovery of new facts and findings” (Adams al.,2007,P.20). Research Begins when researchers discover real world problems and try to answer those problems with the required mechanisms, tools and methods. Therefore, research methods have gained acceptance in all branches of science and disciplines which seek to find the answer for research questions in scientific manner (Ibid). It is believed, if a research does not follow any methodology, it may produce false results. There are different types of research for different disciplines and each discipline is associated with the particular scientific tools. Social sciences are one of those branches of sciences that follow its own research methods, methodologies and tools. Research method in social sciences is a vast topic. This is due to the fact that Social sciences include a great number of disciplines namely; Political Science, International Relations, Sociology, Economics, Anthropology, Social Capital, Education, Management, History, Psychology and so forth. Within each discipline researchers apply different methods and methodologies. The most frequently used methods are laboratory experiments, comparative politics, inferential analysis, descriptive analysis, exploratory research, Analytical Research and Predictive Research. Despite differences in disciplines and methods used in research, most disciplines in social sciences share same features and use same language for interpretation and reporting of their results (Walliman, 2011). It also happens that researchers use different methodologies for the similar type of problem of a discipline, it is as a result of limiting factors such as; cost, time, availability of tools, literature, access to publications and a country’s own peculiarities and circumstances (Adams et al.,2007). Descriptive research is one of the most commonly used type of researches in social sciences. A descriptive research aims to describe a phenomena the ways it is, for example, describing social systems or relationships between events (Adams et al., 2007). This paper attempts to introduce descriptive analysis; its advantages, disadvantages an example of Descriptive Analysis and conclusion. The next section introduces Descriptive Analysis.
Related Papers
Jacob Otachi ORINA
The study sought to establish the influence of governance on corruption levels from the perspective of the Public Service in Kenya. One of the study objectives was to: assess the influence of institutional leadership on corruption levels in the Public Service. A review of literature was done anchored on Principal-Agent Theory. The study adopted both the correlational and descriptive research designs. A study population of 265 institutions (as on 2015) provided a target sample size of 157 institutions. The target respondents in the sampled institutions were public officers who had undergone training on the following disciplines: leadership, integrity, values and principles of the public service and management during the study period (2010-2015). These purposely selected respondents were subjected to questionnaire. To augment data from the questionnaires, 23 key informant interviews were conducted targeting senior officers in the public service, non-state actors and experts. Data collected was analyzed by descriptive and inferential statistics. The overall correlation analysis results showed that there was a significant but negative relationship between institutional leadership and corruption levels as supported by correlation coefficient of-.525. The regression analysis results showed the coefficient of determination R square is .291 and R is .540 at 0.05 level of significance. The coefficient of determination indicates that 29.1% of the variation on corruption level is influenced by institutional leadership. The findings
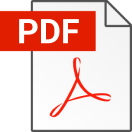
The study sought to establish the influence of governance on corruption levels in the Public Service in Kenya. One of the study objectives was to: assess the influence of stakeholder participation on corruption levels in the Public Service. A review of literature was done anchored on Stakeholder Theory. Further, the empirical review, critique of reviewed literature, a summary and the research gaps were presented. The study adopted both the correlational and descriptive research designs. A study population of 265 institutions (as at 2015) provided a target sample size of 157 institutions where 133 were positive. The target respondents (unit of observation) in the sampled institutions were public officers who had undergone training on the following disciplines: leadership, integrity, values and principles of the public service and management during the study period (2010-2015). These purposely selected respondents were subjected to questionnaire as a primary tool of data collection. To augment data from the questionnaires, 23 key informant interviews were conducted targeting senior officers in the public service, non-state actors and experts. Data collected was analyzed by descriptive and inferential statistics. Data was presented in form of pie charts, graphs, tables and equations. The overall correlation analysis results showed that there was a significant but negative relationship between stakeholder participation and corruption levels as supported by correlation coefficient of -.741. The regression analysis results showed the coefficient of determination R square is 0.548 and R is 0.720 at 0.05 significance level. The coefficient of determination indicates that 54.8% of the variation on corruption level is influenced by stakeholder participation. The findings from the study are to benefit the policy makers, public service, citizens of Kenya and other stakeholders. It also fills the knowledge gap owed to previous little research on the influence of stakeholder participation on corruption levels. The study recommended that the public service should be keen to design policies and implement programs targeted on addressing the specific stakeholder sub constructs (stakeholder voice, openness, and partnership) so as to address the run-away corruption in the public service.
Oirc Journals
Risk is a fact of life in procurement but in spite of this, majority of manufacturing companies give this topic much less attention than it deserves. However, little or no research has been published that specifically addresses the procurement risk and mitigation strategies within the manufacturing sector in Africa land more so in the I Kenyan I manufacturing I firms that is central to delivery of goods and services to its customers. The main purpose of the study was to assess the influence of risk reduction on procurement performance. The study was guided by risk compensation theory. Explanatory research design was adopted. The target population was employees from four manufacturing firms and a sample of 127 respondents were selected using Yamane’s formula from an accessible population of 187. Data was collected through structured questionnaires and was summarized, edited, coded, entered and analyzed using statistical package for social scientists (SPSS). Inferential statistics involved regression analysis. The result was as follows: Based on risk reduction strategy, the correlation result was 0.583 and β = 0.051 at P<0.05. The study concluded that risk reduction was statistically significant and had a positive influence on procurement performance. The study findings rejected the null hypothesis that there is no statistically significant influence of risk reduction strategy on procurement performance. The study recommended policy makers to embrace other risk reduction strategies tools like diversification, underwriting and hedges. The study suggests that a further study be done on specific risk reduction strategies suitable for the manufacturing sector and a further study be done that focuses on specific procurement risks affecting the manufacturing sector and their effect on procurement performance.
International Journal of Strategic Management and Procurement
Performance of microfinance institutions is indicated by contributions to social welfare, job creation, general economic empowerment and improvement of lives of the poor. Despite the interest in the sector and the subsidies that have flowed into some of the mission-oriented MFIs, it seems that most MFIs struggle with the challenge of remaining viable over the long-term. Sensing capabilities could offer a solution to this dilemma through providing a customer management system which incorporates all functional areas of the organization. Thus, the main purpose of the study was to determine effect of sensing capability on performance of micro finance institutions in Eldoret town. This study was guided by resource-based view theory. Explanatory research design was used in this study. The target population for this study comprised of 584 employees drawn from 14 MFIs within Eldoret town. Stratified and simple random sampling technique was used in this study to select a sample of 162 employees. Primary data was obtained from the respondents using questionnaire. This study used questionnaires and interview schedules to collect data from respondents. Quantitative data collected from questionnaires were analysed using descriptive statistical techniques which were the frequencies, mean, standard deviation. Qualitative data collected from interview schedules of senior managers were analysed thematically. The researcher also used inferential statistics of Pearson Product Moment Correlation to show the relationships that exist between the variables and multiple regressions and correlation analysis, the significance of each independent variable was tested at a confidence level of 95%. Analysed data was presented in form of tables, figures and percentages. From the study finding, sensing capability has a significant effect on performance of micro finance institutions in Eldoret town with a beta coefficient of 0.127 and significance of (p<0.05). The study concluded that sensing capabilities about environment is a coping capability mechanism that enables the organization to be competitive.
Danial Zemchal Media Development in Tigray
Danial Zemchal
This paper comprises an ongoing MA Thesis research project titled “Assessment of Media Development in Tigray”. The main focus of this investigation concentrates on measuring the media development based on the UNESCO’s Media Development Measures. The pillars of the assessment are the system of regulation and practice in relation to freedom of expression, transparency of media ownership and concentration, diversity and plurality of the media, media as a platform of public discourse, professional capacity building as well as capacity of media infrastructure including its inclusive access to the marginalized society. It also examines the relationship among the media development measures through statistical Measure, SPSS. The research project which spotlight in examining the media development context in Tigray began in October 2018 and lasts in July 2019. A combination of quantitative questionnaire survey, qualitative; in-depth personal interview and focus group discussion are employed. Professionals in media firms in Tigray, higher education journalism and communication schools, democratic institutions; human right office, ombudsman office, civic and civil societies, Tigray, Kunama and Irob ethnicity communities are subjects of the research. The research project is currently progressed the quantitative and qualitative data collection process and analysis and presentation will be followed.
Assessment of Media Development in Tigray
International Journal of Scientific and Technological Research
Loading Preview
Sorry, preview is currently unavailable. You can download the paper by clicking the button above.
RELATED PAPERS
Cabdi Ciise
Begna Olira
Qeyliye Jama
Elisha Chimusoro
A Simple Guide to Research writing
Dr. David Annan
Effects of Terrorism on the Political Economy: Case Study of Garissa County, Kenya
George Nyongesa
European Journal of Business and Management
N. Marangu , ROBERT EGESSA
IOSR Journals
Janet Manyasi , Mathew Egessa
Mathew Egessa
Diane Mortel
Basheer Salaytah
Fernando Almeida
Nagalaxmi Lucky
Relationship between Corporate Governance and Information Security Governance Effectiveness in United States Corporations
Dr. Robert E Davis
Abdulwasiu K Ayoku
Lillian Otieno-Omutoko
lillian omutoko
Miguel Centellas
Faie Faiezah
kavita vadrale
American Based Research Journal
American Journal
JASH MATHEW
Othniel Kighir
Jacintho Del Vecchio Junior
Parvez Alam
Jara Mi Serino
International Journal of Social Science and Humanities Research
Conrad O Mogaka
Masters Degree Thesis
Stephen Baguma
Wossen Mesele
Open Access Publishing Group
oirc journals
Mnaka Godfrey
AFRICA NAZARENE UNIVERSITY
Edwin Onyango
International Journal of Academics & Research, IJARKE Journals
RELATED TOPICS
- We're Hiring!
- Help Center
- Find new research papers in:
- Health Sciences
- Earth Sciences
- Cognitive Science
- Mathematics
- Computer Science
- Academia ©2024
Information
- Author Services
Initiatives
You are accessing a machine-readable page. In order to be human-readable, please install an RSS reader.
All articles published by MDPI are made immediately available worldwide under an open access license. No special permission is required to reuse all or part of the article published by MDPI, including figures and tables. For articles published under an open access Creative Common CC BY license, any part of the article may be reused without permission provided that the original article is clearly cited. For more information, please refer to https://www.mdpi.com/openaccess .
Feature papers represent the most advanced research with significant potential for high impact in the field. A Feature Paper should be a substantial original Article that involves several techniques or approaches, provides an outlook for future research directions and describes possible research applications.
Feature papers are submitted upon individual invitation or recommendation by the scientific editors and must receive positive feedback from the reviewers.
Editor’s Choice articles are based on recommendations by the scientific editors of MDPI journals from around the world. Editors select a small number of articles recently published in the journal that they believe will be particularly interesting to readers, or important in the respective research area. The aim is to provide a snapshot of some of the most exciting work published in the various research areas of the journal.
Original Submission Date Received: .
- Active Journals
- Find a Journal
- Proceedings Series
- For Authors
- For Reviewers
- For Editors
- For Librarians
- For Publishers
- For Societies
- For Conference Organizers
- Open Access Policy
- Institutional Open Access Program
- Special Issues Guidelines
- Editorial Process
- Research and Publication Ethics
- Article Processing Charges
- Testimonials
- Preprints.org
- SciProfiles
- Encyclopedia
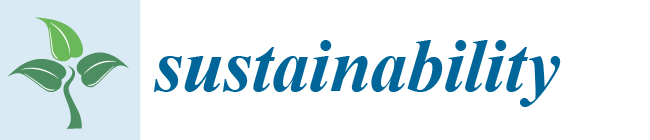
Article Menu
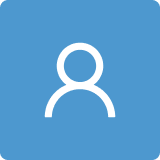
- Subscribe SciFeed
- Recommended Articles
- Google Scholar
- on Google Scholar
- Table of Contents
Find support for a specific problem in the support section of our website.
Please let us know what you think of our products and services.
Visit our dedicated information section to learn more about MDPI.
JSmol Viewer
A quantitative analysis of the complex response relationship between urban green infrastructure (ugi) structure/spatial pattern and urban thermal environment in shanghai.
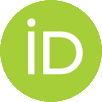
1. Introduction
2. materials and methods, 2.1. study area, 2.2. data sources, 2.3. methods, 2.3.1. data preprocessing, 2.3.2. lst retrieval and generation of thermally sharpened products, 2.3.3. extraction of main variables/indicators of built environment affecting lst, 2.3.4. statistical analysis, 3.1. the relationship between ugi and lst under different spatial stratification, 3.2. response of lst to ugi pattern in uths range, 3.3. quantitative model analysis of pattern response relationship between lst and ugi, 4. discussion, 4.1. improving ugi spatial pattern to mitigate uhi effect, 4.2. improving ecological building design to enhance urban sustainability, 5. conclusions, author contributions, institutional review board statement, informed consent statement, data availability statement, conflicts of interest.
- Santamouris, M. Recent progress on urban overheating and heat island research. Integrated assessment of the energy, environmental, vulnerability and health impact. Synergies with the global climate change. Energy Build. 2020; 207, 109482. [ Google Scholar ]
- Voogt, J.A. Urban heat island: Causes and consequences of global environmental change. In Encyclopaedia of Global Environmental Change ; Wiley: Chichester, UK, 2002; Volume 3, pp. 660–666. [ Google Scholar ]
- Oke, T.R. Boundary Layer Climates ; Routledge: London, UK, 2002. [ Google Scholar ]
- Arnfield, A.J. Two decades of urban climate research: A review of turbulence, exchanges of energy and water, and the urban heat island. Int. J. Climatol. 2003 , 23 , 1–26. [ Google Scholar ] [ CrossRef ]
- Zhao, Z.-Q.; He, B.-J.; Li, L.-G.; Wang, H.-B.; Darko, A. Profile and concentric zonal analysis of relationships between land use/land cover and land surface temperature: Case study of Shenyang, China. Energy Build. 2017 , 155 , 282–295. [ Google Scholar ] [ CrossRef ]
- UN-Habitat. World Cities Report 2022: Envisaging the Future of Cities ; UN-Habitat: Nairobi, Kenya, 2022. [ Google Scholar ]
- Zhao, L.; Lee, X.; Smith, R.B.; Oleson, K. Strong contributions of local background climate to urban heat islands. Nature 2014 , 511 , 216–219. [ Google Scholar ] [ CrossRef ] [ PubMed ]
- Leal Filho, W.; Echevarria Icaza, L.; Neht, A.; Klavins, M.; Morgan, E.A. Coping with the impacts of urban heat islands. A literature based study on understanding urban heat vulnerability and the need for resilience in cities in a global climate change context. J. Clean. Prod. 2018 , 171 , 1140–1149. [ Google Scholar ] [ CrossRef ]
- Singh, N.; Singh, S.; Mall, R.K. Urban ecology and human health: Implications of urban heat island, air pollution and climate change nexus. In Urban Ecology ; Elsevier: Amsterdam, The Netherlands, 2020; pp. 317–334. [ Google Scholar ]
- Patz, J.A.; Campbell-Lendrum, D.; Holloway, T.; Foley, J.A. Impact of regional climate change on human health. Nature 2005 , 438 , 310–317. [ Google Scholar ] [ CrossRef ]
- Gasparrini, A.; Guo, Y.; Sera, F.; Vicedo-Cabrera, A.M.; Huber, V.; Tong, S.; de Sousa Zanotti Stagliorio Coelho, M.; Nascimento Saldiva, P.H.; Lavigne, E.; Matus Correa, P.; et al. Projections of temperature-related excess mortality under climate change scenarios. Lancet Planet Health 2017 , 1 , e360–e367. [ Google Scholar ] [ CrossRef ] [ PubMed ]
- Balling, R.C.; Gober, P.; Jones, N. Sensitivity of residential water consumption to variations in climate: An intraurban analysis of Phoenix, Arizona. Water Resour. Res. 2008 , 44 . [ Google Scholar ] [ CrossRef ]
- Wang, C.; Ren, Z.; Guo, Y.; Zhang, P.; Hong, S.; Ma, Z.; Hong, W.; Wang, X. Assessing urban population exposure risk to extreme heat: Patterns, trends, and implications for climate resilience in China (2000–2020). Sustain. Cities Soc. 2024 , 103 , 105260. [ Google Scholar ] [ CrossRef ]
- Buchin, O.; Hoelscher, M.-T.; Meier, F.; Nehls, T.; Ziegler, F. Evaluation of the health-risk reduction potential of countermeasures to urban heat islands. Energy Build. 2016 , 114 , 27–37. [ Google Scholar ] [ CrossRef ]
- Tan, J.; Zheng, Y.; Tang, X.; Guo, C.; Li, L.; Song, G.; Zhen, X.; Yuan, D.; Kalkstein, A.J.; Li, F. The urban heat island and its impact on heat waves and human health in Shanghai. Int. J. Biometeorol. 2010 , 54 , 75–84. [ Google Scholar ] [ CrossRef ]
- Memon, R.A.; Leung, D.Y.; Chunho, L. A review on the generation, determination and mitigation of urban heat island. J. Environ. Sci. (China) 2008 , 20 , 120–128. [ Google Scholar ]
- Xu, C.; Chen, G.; Huang, Q.; Su, M.; Rong, Q.; Yue, W.; Haase, D. Can improving the spatial equity of urban green space mitigate the effect of urban heat islands? An empirical study. Sci. Total. Environ. 2022 , 841 , 156687. [ Google Scholar ] [ CrossRef ]
- Wang, Y.; Bakker, F.; de Groot, R.; Wörtche, H. Effect of ecosystem services provided by urban green infrastructure on indoor environment: A literature review. Build. Environ. 2014 , 77 , 88–100. [ Google Scholar ] [ CrossRef ]
- Sun, R.; Xie, W.; Chen, L. A landscape connectivity model to quantify contributions of heat sources and sinks in urban regions. Landsc. Urban Plan. 2018 , 178 , 43–50. [ Google Scholar ] [ CrossRef ]
- Young, R.; Zanders, J.; Lieberknecht, K.; Fassman-Beck, E. A comprehensive typology for mainstreaming urban green infrastructure. J. Hydrol. 2014 , 519 , 2571–2583. [ Google Scholar ] [ CrossRef ]
- Cameron, R.W.F.; Blanuša, T.; Taylor, J.E.; Salisbury, A.; Halstead, A.J.; Henricot, B.; Thompson, K. The domestic garden–Its contribution to urban green infrastructure. Urban For. Urban Green. 2012 , 11 , 129–137. [ Google Scholar ] [ CrossRef ]
- Liu, O.Y.; Russo, A. Assessing the contribution of urban green spaces in green infrastructure strategy planning for urban ecosystem conditions and services. Sustain. Cities Soc 2021 , 68 . [ Google Scholar ] [ CrossRef ]
- Zhang, S.; Muñoz Ramírez, F. Assessing and mapping ecosystem services to support urban green infrastructure: The case of Barcelona, Spain. Cities 2019 , 92 , 59–70. [ Google Scholar ] [ CrossRef ]
- Shen, J.; Peng, Z.; Wang, Y. From GI, UGI to UAGI: Ecosystem service types and indicators of green infrastructure in response to ecological risks and human needs in global metropolitan areas. Cities 2023 , 134 , 104176. [ Google Scholar ] [ CrossRef ]
- Amorim, J.H.; Engardt, M.; Johansson, C.; Ribeiro, I.; Sannebro, M. Regulating and cultural ecosystem services of urban green infrastructure in the nordic countries: A systematic review. Int. J. Environ. Res. Public Health 2021 , 18 , 1219. [ Google Scholar ] [ CrossRef ]
- Kumar, P. The Economics of Ecosystems and Biodiversity: Ecological and Economic Foundations ; Routledge: London, UK, 2012. [ Google Scholar ]
- Maes, J.; Liquete, C.; Teller, A.; Erhard, M.; Paracchini, M.L.; Barredo, J.I.; Grizzetti, B.; Cardoso, A.; Somma, F.; Petersen, J.-E. An indicator framework for assessing ecosystem services in support of the EU Biodiversity Strategy to 2020. Ecosyst. Serv. 2016 , 17 , 14–23. [ Google Scholar ] [ CrossRef ]
- Müller, N.; Kuttler, W.; Barlag, A.-B. Counteracting urban climate change: Adaptation measures and their effect on thermal comfort. Theor. Appl. Climatol. 2013 , 115 , 243–257. [ Google Scholar ] [ CrossRef ]
- Balany, F.; Ng, A.W.M.; Muttil, N.; Muthukumaran, S.; Wong, M.S. Green Infrastructure as an Urban Heat Island Mitigation Strategy—A Review. Water 2020 , 12 , 3577. [ Google Scholar ] [ CrossRef ]
- Hu, L.; Li, Q. Greenspace, bluespace, and their interactive influence on urban thermal environments. Environ. Res. Lett. 2020 , 15 , 034041. [ Google Scholar ] [ CrossRef ]
- Li, F. Planning Green Space for Climate Change Adaptation and Mitigation: A Review of Green Space in the Central City of Beijing. Urban Reg. Plan. 2018 , 3 . [ Google Scholar ] [ CrossRef ]
- Onishi, A.; Cao, X.; Ito, T.; Shi, F.; Imura, H. Evaluating the potential for urban heat-island mitigation by greening parking lots. Urban For. Urban Green. 2010 , 9 , 323–332. [ Google Scholar ] [ CrossRef ]
- Wang, C.; Ren, Z.; Du, Y.; Guo, Y.; Zhang, P.; Wang, G.; Hong, S.; Ma, Z.; Hong, W.; Li, T. Urban vegetation cooling capacity was enhanced under rapid urbanization in China. J. Clean. Prod. 2023 , 425 , 138906. [ Google Scholar ] [ CrossRef ]
- Tan, J.; Zheng, Y.; Song, G.; Kalkstein, L.S.; Kalkstein, A.J.; Tang, X. Heat wave impacts on mortality in Shanghai, 1998 and 2003. Int. J. Biometeorol. 2007 , 51 , 193–200. [ Google Scholar ] [ CrossRef ]
- Zhou, H.; Gao, Y.; Ge, W.; Li, T. The Research on the Relationship Between the Urban Expansion and the Change of the Urban Heat Island Distribution in Shanghai Area. Ecol. Environ. 2008 , 17 , 163–168. [ Google Scholar ]
- Zhang, H.; Qi, Z.-f.; Ye, X.-y.; Cai, Y.-b.; Ma, W.-c.; Chen, M.-n. Analysis of land use/land cover change, population shift, and their effects on spatiotemporal patterns of urban heat islands in metropolitan Shanghai, China. Appl. Geogr. 2013 , 44 , 121–133. [ Google Scholar ] [ CrossRef ]
- Li, Y.-y.; Zhang, H.; Kainz, W. Monitoring patterns of urban heat islands of the fast-growing Shanghai metropolis, China: Using time-series of Landsat TM/ETM+ data. Int. J. Appl. Earth Obs. Geoinf. 2012 , 19 , 127–138. [ Google Scholar ] [ CrossRef ]
- Milard, D. Urban Energy and Environmental Policy: The Case of Shanghai since the 2000’s. 2017. Available online: https://www.academia.edu/110727076/Urban_energy_and_environmental_policy_the_case_of_Shanghai_since_the_2000_s (accessed on 1 June 2017).
- Gielen, D.; Chen, C. The CO 2 emission reduction benefits of Chinese energy policies and environmental policies: A case study for Shanghai, period 1995–2020. Ecol. Econom. 2001 , 39 , 257–270. [ Google Scholar ] [ CrossRef ]
- Harlan, S.L.; Ruddell, D.M. Climate change and health in cities: Impacts of heat and air pollution and potential co-benefits from mitigation and adaptation. Curr. Opin. Environ. Sustain. 2011 , 3 , 126–134. [ Google Scholar ] [ CrossRef ]
- Shi, C.; Guo, N.; Gao, X.; Wu, F. How carbon emission reduction is going to affect urban resilience. J. Clean. Prod. 2022 , 372 , 133737. [ Google Scholar ] [ CrossRef ]
- Gómez-Baggethun, E.; Barton, D.N. Classifying and valuing ecosystem services for urban planning. Ecol. Econ. 2013 , 86 , 235–245. [ Google Scholar ] [ CrossRef ]
- Shanghai Bureau of Statistics. Shanghai Statistical Yearbook (2021). 2021; p. 2. Available online: https://tjj.sh.gov.cn/tjnj/20220309/0e01088a76754b448de6d608c42dad0f.html (accessed on 1 January 2022).
- Han, J.; Zhao, X.; Zhang, H.; Liu, Y. Analyzing the Spatial Heterogeneity of the Built Environment and Its Impact on the Urban Thermal Environment—Case Study of Downtown Shanghai. Sustainability 2021 , 13 , 11302. [ Google Scholar ] [ CrossRef ]
- Shanghai Municipal Statistics Bureau (SMSB); Survey Office of the National Bureau of Statistics in Shanghai (SONBS-SH). Shanghai Statistical Yearbook-2022 ; China Statistics Press: Beijing, China, 2021. [ Google Scholar ]
- Zhang, H.; Han, J.-J.; Zhou, R.; Zhao, A.-L.; Zhao, X.; Kang, M.-Y. Quantifying the relationship between land parcel design attributes and intra-urban surface heat island effect via the estimated sensible heat flux. Urban Clim. 2022 , 41 , 101030. [ Google Scholar ] [ CrossRef ]
- Zhao, M.; Cai, H.; Qiao, Z.; Xu, X. Influence of urban expansion on the urban heat island effect in Shanghai. Int. J. Geogr. Inf. Sci. 2016 , 30 , 2421–2441. [ Google Scholar ] [ CrossRef ]
- Li, J.-j.; Wang, X.-r.; Wang, X.-j.; Ma, W.-c.; Zhang, H. Remote sensing evaluation of urban heat island and its spatial pattern of the Shanghai metropolitan area, China. Ecol. Complex. 2009 , 6 , 413–420. [ Google Scholar ] [ CrossRef ]
- Li, J.; Song, C.; Cao, L.; Zhu, F.; Meng, X.; Wu, J. Impacts of landscape structure on surface urban heat islands: A case study of Shanghai, China. Remote Sens. Environ. 2011 , 115 , 3249–3263. [ Google Scholar ] [ CrossRef ]
- Shanghai Municipal Afforestation Administration. Plan Report for Shanghai Greening System (2002–2020). 2002. Available online: https://www.yuanlin.com/rules/html/detail/2006-4/462.html (accessed on 3 February 2022).
- Zhang, H.; Li, T.t.; Liu, Y.; Han, J.j.; Guo, Y.j. Understanding the contributions of land parcel features to intra-surface urban heat island intensity and magnitude: A study of downtown Shanghai, China. Land Degrad. Dev. 2020 , 32 , 1353–1367. [ Google Scholar ] [ CrossRef ]
- Müller-Wilm, U.; Devignot, O.; Pessiot, L. Sen2Cor Configuration and User Manual. S2-PDGS-MPC-L2A-SUM-V2.4. 2017. Available online: https://step.esa.int/thirdparties/sen2cor/2.4.0/Sen2Cor_240_Documenation_PDF/S2-PDGS-MPC-L2A-SUM-V2.4.0.pdf (accessed on 1 June 2022).
- Feng, L.; Zhao, M.; Zhou, Y.; Zhu, L.; Tian, H. The seasonal and annual impacts of landscape patterns on the urban thermal comfort using Landsat. Ecol. Indic. 2020 , 110 , 105798. [ Google Scholar ] [ CrossRef ]
- Yue, W.; Qiu, S.; Xu, H.; Xu, L.; Zhang, L. Polycentric urban development and urban thermal environment: A case of Hangzhou, China. Landsc. Urban Plan. 2019 , 189 , 58–70. [ Google Scholar ] [ CrossRef ]
- Xu, X.; Liu, Q.; Chen, J. Synchronous retrieval of land surface temperature and emissivity. Sci. China Ser. D Earth Sci. 1998 , 41 , 658–668. [ Google Scholar ] [ CrossRef ]
- Santamouris, M.; Synnefa, A.; Karlessi, T. Using advanced cool materials in the urban built environment to mitigate heat islands and improve thermal comfort conditions. Solar Energy 2011 , 85 , 3085–3102. [ Google Scholar ] [ CrossRef ]
- Næss, P. Built environment, causality and urban planning. Plan. Theory Pract. 2016 , 17 , 52–71. [ Google Scholar ] [ CrossRef ]
- McGarigal, K.; Cushman, S.A.; Ene, E. FRAGSTATS v4: Spatial Pattern Analysis Program for Categorical and Continuous Maps. Computer Software Program Produced by the Authors at the University of Massachusetts Amherst. 2012. Available online: http://www.umass.edu/landeco/research/fragstats/fragstats.html (accessed on 26 July 2018).
- Geladi, P.; Kowalski, B. Partial Least-Squares Regression: A Tutorial. Anal. Chim. Acta 1986 , 185 , 1–17. [ Google Scholar ] [ CrossRef ]
- Wold, H. Soft Modelling by Latent Variables: The Non-Linear Iterative Partial Least Squares (NIPALS) Approach. J. Appl. Probab. 2017 , 12 , 117–142. [ Google Scholar ] [ CrossRef ]
- Wehrens, R.; Mevik, B.-H. The pls package: Principal component and partial least squares regression in R. J. Stat. Softw. 2007 , 18 , 1–23. [ Google Scholar ]
- Wu, C.; Li, J.; Wang, C.; Song, C.; Haase, D.; Breuste, J.; Finka, M. Estimating the Cooling Effect of Pocket Green Space in High Density Urban Areas in Shanghai, China. Front. Environ. Sci. 2021 , 9 , 657969. [ Google Scholar ] [ CrossRef ]
- Liu, J.; Zhang, L.; Zhang, Q.; Zhang, G.; Teng, J. Predicting the surface urban heat island intensity of future urban green space development using a multi-scenario simulation. Sustain. Cities Soc. 2021 , 66 , 102698. [ Google Scholar ] [ CrossRef ]
- Jones, L.; Anderson, S.; Læssøe, J.; Banzhaf, E.; Jensen, A.; Bird, D.N.; Miller, J.; Hutchins, M.G.; Yang, J.; Garrett, J.; et al. A typology for urban Green Infrastructure to guide multifunctional planning of nature-based solutions. Nat.-Based Solut. 2022 , 2 , 100041. [ Google Scholar ] [ CrossRef ]
- Adegun, O.B.; Ikudayisi, A.E.; Morakinyo, T.E.; Olusoga, O.O. Urban green infrastructure in Nigeria: A review. Sci. Afr. 2021 , 14 , e01044. [ Google Scholar ] [ CrossRef ]
- Sodoudi, S.; Zhang, H.; Chi, X.; Müller, F.; Li, H. The influence of spatial configuration of green areas on microclimate and thermal comfort. Urban For. Urban Green. 2018 , 34 , 85–96. [ Google Scholar ] [ CrossRef ]
- Lai, D.; Liu, Y.; Liao, M.; Yu, B. Effects of different tree layouts on outdoor thermal comfort of green space in summer Shanghai. Urban Clim. 2023 , 47 , 101398. [ Google Scholar ] [ CrossRef ]
- Yu, K.; Chen, Y.; Wang, D.; Chen, Z.; Gong, A.; Li, J. Study of the Seasonal Effect of Building Shadows on Urban Land Surface Temperatures Based on Remote Sensing Data. Remote Sens. 2019 , 11 , 497. [ Google Scholar ] [ CrossRef ]
- Han, Y.; Taylor, J.E.; Pisello, A.L. Toward mitigating urban heat island effects: Investigating the thermal-energy impact of bio-inspired retro-reflective building envelopes in dense urban settings. Energy Build. 2015 , 102 , 380–389. [ Google Scholar ] [ CrossRef ]
- He, B.-J.; Zhao, Z.-Q.; Shen, L.-D.; Wang, H.-B.; Li, L.-G. An approach to examining performances of cool/hot sources in mitigating/enhancing land surface temperature under different temperature backgrounds based on landsat 8 image. Sustain. Cities Soc. 2019 , 44 , 416–427. [ Google Scholar ] [ CrossRef ]
- He, B.-J. Towards the next generation of green building for urban heat island mitigation: Zero UHI impact building. Sustain. Cities Soc. 2019 , 50 , 101647. [ Google Scholar ] [ CrossRef ]
- Cui, Y.-q.; Zheng, H.-C. Impact of Three-Dimensional Greening of Buildings in Cold Regions in China on Urban Cooling Effect. Procedia Eng. 2016 , 169 , 297–302. [ Google Scholar ] [ CrossRef ]
- Shi, D.; Song, J.; Huang, J.; Zhuang, C.; Guo, R.; Gao, Y. Synergistic cooling effects (SCEs) of urban green-blue spaces on local thermal environment: A case study in Chongqing, China. Sustain. Cities Soc. 2020 , 55 , 102065. [ Google Scholar ] [ CrossRef ]
- Völker, S.; Baumeister, H.; Claßen, T.; Hornberg, C.; Kistemann, T. Evidence for the temperature-mitigating capacity of urban blue space—A health geographic perspective. Erdkunde 2013 , 67 , 355–371. [ Google Scholar ] [ CrossRef ]
- Georgescu, M.; Morefield, P.E.; Bierwagen, B.G.; Weaver, C.P. Urban adaptation can roll back warming of emerging megapolitan regions. Proc. Natl. Acad. Sci. USA 2014 , 111 , 2909–2914. [ Google Scholar ] [ CrossRef ] [ PubMed ]
- Tian, Y.; Zhou, W. The effect of urban 2D and 3D morphology on air temperature in residential neighborhoods. Landsc. Ecol. 2019 , 34 , 1161–1178. [ Google Scholar ] [ CrossRef ]
- Javadi, R.; Nasrollahi, N. Urban green space and health: The role of thermal comfort on the health benefits from the urban green space; a review study. Build. Environ. 2021 , 202 , 108039. [ Google Scholar ] [ CrossRef ]
- Oliveira, S.; Andrade, H.; Vaz, T. The cooling effect of green spaces as a contribution to the mitigation of urban heat: A case study in Lisbon. Build. Environ. 2011 , 46 , 2186–2194. [ Google Scholar ] [ CrossRef ]
- Theeuwes, N.E.; Steeneveld, G.J.; Ronda, R.J.; Heusinkveld, B.G.; van Hove, L.W.A.; Holtslag, A.A.M. Seasonal dependence of the urban heat island on the street canyon aspect ratio. Q. J. R. Meteorol. Soc. 2014 , 140 , 2197–2210. [ Google Scholar ] [ CrossRef ]
- Park, Y.; Guldmann, J.-M.; Liu, D. Impacts of tree and building shades on the urban heat island: Combining remote sensing, 3D digital city and spatial regression approaches. Comput. Environ. Urban Syst. 2021 , 88 , 101655. [ Google Scholar ] [ CrossRef ]
- Cao, Q.; Liu, Y.; Georgescu, M.; Wu, J. Impacts of landscape changes on local and regional climate: A systematic review. Landsc. Ecol. 2020 , 35 , 1269–1290. [ Google Scholar ] [ CrossRef ]
- Yang, J.; Yang, Y.; Sun, D.; Jin, C.; Xiao, X. Influence of urban morphological characteristics on thermal environment. Sustain. Cities Soc. 2021 , 72 , 103045. [ Google Scholar ] [ CrossRef ]
- Shashua-Bar, L.; Tzamir, Y.; Hoffman, M.E. Thermal effects of building geometry and spacing on the urban canopy layer microclimate in a hot-humid climate in summer. Int. J. Climatol. 2004 , 24 , 1729–1742. [ Google Scholar ] [ CrossRef ]
Click here to enlarge figure
Data | Description |
---|---|
Landsat-8/9 OLI/TIRS images | Among the available high-quality cloud-free images collected in the summer of 2013–2022, considering the time span and interval of the whole study period, five phases of images were selected in this study: 29 August 2013, 3 August 2015, 24 August 2017, 16 August 2020, and 14 August 2010. These satellite images were downloaded via (accessed on 1 June 2023). |
Sentinel-1/2 images | Sentinel is a series of Earth observation satellites launched by the Copernicus Program of the European Space Agency (ESA). Three images dated 24 February 2020 16 August 2020, 23 February 2020, and 16 August 2020 were downloaded from the Open port provided by the European Space Agency ( , accessed on 1 June 2023). |
Land use map | This map of land use cover in 2013 was originally generated using an object-oriented classification method based on orthophose-corrected high-resolution Quickbird satellite imagery. Based on the field investigation data, the classified products were further manually corrected and verified, and resampled to the TIF grid (1 m resolution), with an overall correction accuracy of 91.1% [ ]. |
Building profile data | The building outline is a high-resolution Quickbird satellite image using orthographic correction, and outside the range is manually drawn using the 91 Weitu Map. |
Digital city thematic products | Commercial thematic layers contain specific land use covers, such as buildings, warehouses, industrial parks, transportation lines, vegetated areas, and bodies of water. (Beijing Digital Space Technology Co., Ltd., Beijing, China) |
Baidu map | Baidu Maps Baidu web products, including high-resolution satellite images (still/no historical review), thematic features (such as buildings, roads, traffic lines, etc.), and street views with retrospective photos. |
91Weitu Map | The online high-resolution satellite image and city digital thematic service layer products operated by Beijing Qianfan World View Company ( , accessed on 20 June 2023). |
Tianditu map | operated by the National Platform for Common Geospatial Information Services ( , accessed on 20 June 2023) |
Ground truth data | Collected in 8 annual field surveys conducted between 2013 and 2020, with intervals of 3–6 months, focusing on the land use type and development pattern of each typical sample area, building height was measured on-site using the Edkors™ model AS1000H handheld height finder (Changzhou Edkors Instrument Co., LTD, Changzhou, China) . |
Dimension | Indicator Name | Formula | Meaning |
---|---|---|---|
Building index | Proportion of impervious surface area | The proportion of surfaces in a given area that are artificially constructed or artificially enclosed by buildings, roads, sidewalks, etc. | |
Building height (BH) | / | The vertical height of a building usually indicates the distance from the outdoor floor to the roof of the building. | |
UGI index | Class area (CA) | It can directly reflect the size of different landscape element types. | |
percentage of landscape (PLAND) | The relative percentage of a certain patch type in the total landscape area can be used to judge landscape dominance. | ||
largest patch index (LPI) | The maximum continuous patch area as a percentage of the entire landscape area. | ||
patch density (PD) | It reflects the degree of fragmentation and spatial heterogeneity of landscape segmentation. | ||
CLUMPY | | It reflects the aggregation and dispersion of patches in the landscape, and the value is between −1 and 1. | |
COHESION | Represents the distance and arrangement pattern of patches in the landscape, reflecting the continuity. | ||
Aggregation Index (Al) | AI ∈ (0,100). AI examined the connectivity between patches of each landscape type. | ||
Splitting Index (SPLIT) | SPLIT is the sum of the square of the total landscape area divided by the square of the patch area. | ||
Landscape Shape Index (LSI) | Reflects the complexity of landscape structure; that is, the larger the value, the more complex the shape. |
Type | Whole Area = Core Area + Buffer Zone | Core Area | Buffer Area | ||||||
---|---|---|---|---|---|---|---|---|---|
Impervious Surface Area (%) | Building Area (%) | UGI Area (%) | Impervious Surface Area (%) | Building Area (%) | UGI Area (%) | Impervious Surface Area (%) | Building Area (%) | UGI Area (%) | |
C1 | 98.95 ± 0.91 | 34.62 ± 10.97 | 1.05 ± 0.91 | 98.28 ± 1.18 | 21.2 ± 5.39 | 1.72 ± 1.18 | 98.98 ± 0.89 | 35.05 ± 11.03 | 1.02 ± 0.89 |
C2 | 93.20 ± 4.58 | 24.86 ± 9.60 | 6.80 ± 4.58 | 72.03 ± 13.52 | 12.8 ± 8.38 | 25.82 ± 15.16 | 94.62 ± 4.91 | 27.72 ± 7.43 | 5.38 ± 4.91 |
C3 | 89.83 ± 8.29 | 23.22 ± 8.22 | 10.17 ± 8.29 | 85.73 ± 7.58 | 16.1 ± 4.78 | 14.27 ± 7.58 | 89.63 ± 10.48 | 23.38 ± 9.15 | 10.37 ± 10.48 |
C4 | 93.75 ± 3.29 | 21.30 ± 3.23 | 6.25 ± 3.29 | 91.84 ± 3.59 | 16.6 ± 4.55 | 8.16 ± 3.59 | 93.98 ± 3.26 | 21.73 ± 3.18 | 6.02 ± 3.26 |
C5 | 83.78 ± 15.02 | 19.27 ± 8.15 | 15.54 ± 14.93 | 53.88 ± 14.60 | 6.22 ± 6.48 | 52.27 ± 13.85 | 88.27 ± 15.01 | 21.28 ± 7.75 | 11.20 ± 14.93 |
Entirety | 89.14 ± 11.73 | 22.61 ± 9.20 | 10.56 ± 11.54 | 69.51 ± 20.05 | 11.3 ± 8.20 | 32.64 ± 8.20 | 91.51 ± 11.30 | 24.36 ± 8.56 | 8.26 ± 11.19 |
Constant | 49.145 | 0.443 | 110.953 | 0.000 | 45.634 | 1.364 | 33.465 | 0.000 | ||
CA | − | − | − | − | − | −5.452 | 0.989 | −5.515 | 0.000 | 3.152 |
IS | 0.000 | 0.000 | 12.289 | 0.000 | 1.348 | 0.000 | 0.000 | 9.747 | 0.000 | 8.184 |
PD | − | − | − | − | − | 0.407 | 0.165 | 2.463 | 0.015 | 1.616 |
LPI | − | − | − | − | − | −0.642 | 0.217 | −2.961 | 0.004 | 16.625 |
Cohesion | − | − | − | − | − | 0.000 | 0.000 | 5.176 | 0.000 | 20.986 |
Height | −0.903 | 0.114 | −7.949 | 0.000 | 1.300 | −0.647 | 0.102 | −6.356 | 0.000 | 1.583 |
SPLIT | 0.000 | 0.000 | 2.067 | 0.040 | 1.042 | − | − | − | − | − |
S | 1.48198 | 1.20508 | ||||||||
R-sq | 53.94% | 74.14% | ||||||||
R-sq(adj) | 53.02% | 73.08% | ||||||||
R-sq(pred) | 51.53% | 70.40% | ||||||||
Constant | 50.947 | 1.232 | 41.353 | 0.000 | 51.06 | 1.57 | 32.61 | 0.000 | ||
CA | −5.052 | 1.128 | −4.480 | 0.000 | 3.264 | −6.53 | 1.14 | −5.75 | 0.000 | 3.15 |
IS | 0.000 | 0.000 | 8.856 | 0.000 | 7.916 | 0.000 | 0.000 | 9.39 | 0.000 | 8.18 |
PD | − | − | − | − | − | 0.387 | 0.190 | 2.04 | 0.043 | 1.62 |
LPI | −0.428 | 0.235 | −1.822 | 0.070 | 15.508 | −0.396 | 0.249 | −1.59 | 0.114 | 16.63 |
Cohesion | 0.000 | 0.000 | 4.584 | 0.000 | 15.968 | 0.000 | 0.000 | 4.15 | 0.000 | 20.99 |
Height | −0.855 | 0.113 | −7.575 | 0.000 | 1.545 | −0.920 | 0.117 | −7.87 | 0.000 | 1.58 |
SPLIT | 0.000 | 0.000 | 1.774 | 0.078 | 1.175 | − | − | − | − | − |
S | 1.35071 | 1.38388 | ||||||||
R-sq | 69.24% | 70.63% | ||||||||
R-sq(adj) | 67.98% | 69.43% | ||||||||
R-sq(pred) | 63.08% | 66.30% | ||||||||
Constant | 49.731 | 0.788 | 63.15 | 0.000 | ||||||
CA | −1.923 | 0.674 | −2.85 | 0.005 | 2.04 | |||||
IS | 0.000 | 0.000 | 12.36 | 0.000 | 5.27 | |||||
PD | − | − | − | − | − | |||||
LPI | − | − | − | − | − | |||||
Cohesion | 0.000 | 0.000 | 3.53 | 0.001 | 7.04 | |||||
Height | −0.4381 | 0.079 | −5.54 | 0.000 | 1.32 | |||||
SPLIT | − | − | − | − | − | |||||
S | 1.023 | |||||||||
R-sq | 72.84% | |||||||||
R-sq(adj) | 72.11% | |||||||||
R-sq(pred) | 70.89% |
Effect Term | LST2022 | LST2020 | LST2017 | LST2015 | LST2013 | ||||||
---|---|---|---|---|---|---|---|---|---|---|---|
Coef | S − Coef | Coef | S − Coef | Coef | S − Coef | Coef | S − Coef | Coef | S − Coef | ||
Constant | 51.77 | 49.61 | 53.71 | 55.09 | 53.42 | ||||||
Main effect | CA | −1.18 | −0.13 | −1.28 | −0.12 | −1.45 | −0.13 | −1.74 | −0.14 | −0.93 | −0.10 |
IS | 0.17 | 0.16 | 0.17 | 0.18 | 0.13 | ||||||
PD | 0.11 | 0.05 | 0.12 | 0.04 | 0.13 | 0.05 | 0.16 | 0.05 | 0.09 | 0.04 | |
PLAND | −0.54 | −0.10 | −0.66 | −0.11 | −0.69 | −0.11 | −0.85 | −0.12 | −0.54 | −0.10 | |
LPI | −0.05 | −0.04 | −0.09 | −0.06 | −0.08 | −0.05 | −0.10 | −0.06 | −0.09 | −0.07 | |
Cohesion | 0.02 | −0.02 | −0.04 | ||||||||
AI | 0.07 | 0.03 | 0.06 | 0.05 | −0.01 | ||||||
Height | −0.57 | −0.47 | −0.49 | −0.35 | −0.65 | −0.43 | −0.74 | −0.44 | −0.25 | −0.20 | |
LSI | −0.07 | −0.25 | −0.07 | −0.20 | −0.08 | −0.23 | −0.09 | −0.24 | −0.04 | −0.14 | |
SPLIT | 0.04 | 0.04 | 0.04 | 0.05 | 0.04 | ||||||
Interaction | PD × SPLIT × LSI | 0.04 | 0.05 | 0.04 | 0.05 | 0.05 | |||||
effect | CA × Cohesion × AI × LPI | −0.11 | −0.11 | −0.11 | −0.12 | −0.10 | |||||
IS × Height | −0.05 | −0.01 | −0.04 | −0.03 | 0.03 | ||||||
IS × Height × PD × SPLIT × LSI | 0.03 | 0.04 | 0.04 | 0.04 | 0.04 | ||||||
PLAND × PD × SPLIT × LSI | 0.05 | 0.05 | 0.05 | 0.06 | 0.05 | ||||||
IS × Height × PLAND | −0.03 | 0.01 | −0.02 | −0.01 | 0.03 | ||||||
PLAND × CA × Cohesion × AI × LPI | −0.11 | −0.11 | −0.11 | −0.12 | −0.10 | ||||||
F | 15.77 | 19.33 | 16.67 | 23.81 | 20.72 | ||||||
R | 0.673 | 0.652 | 0.670 | 0.699 | 0.749 |
Effect Term | LST2022 | LST2020 | LST2017 | LST2015 | LST2013 | ||||||
---|---|---|---|---|---|---|---|---|---|---|---|
Coef | S − Coef | Coef | S − Coef | Coef | S − Coef | Coef | S − Coef | Coef | S − Coef | ||
Constant | 52.01 | 50.88 | 55.05 | 56.52 | 54.84 | ||||||
CA | −0.93 | −0.10 | −0.72 | −0.07 | −0.49 | −0.04 | −0.95 | −0.07 | −0.12 | −0.01 | |
Main effect | IS | 0.26 | 0.36 | 0.42 | 0.39 | 0.39 | |||||
PD | −0.05 | −0.02 | −0.27 | −0.10 | −0.44 | −0.15 | −0.34 | −0.11 | −0.40 | −0.17 | |
PLAND | −0.70 | −0.13 | −1.09 | −0.18 | −1.26 | −0.19 | −1.39 | −0.19 | −1.05 | −0.19 | |
LPI | −0.06 | −0.05 | −0.12 | −0.08 | −0.12 | −0.07 | −0.14 | −0.08 | −0.13 | −0.10 | |
Cohesion | 0.04 | 0.04 | 0.08 | 0.06 | 0.04 | ||||||
AI | 0.09 | 0.09 | 0.14 | 0.12 | 0.09 | ||||||
Height | −0.64 | −0.53 | −0.76 | −0.54 | −0.98 | −0.65 | −1.06 | −0.63 | −0.56 | −0.45 | |
LSI | −0.07 | −0.24 | −0.08 | −0.23 | −0.09 | −0.26 | −0.11 | −0.27 | −0.05 | −0.18 | |
SPLIT | 0.02 | 0.04 | 0.03 | 0.03 | 0.04 | ||||||
PD × SPLIT × LSI | 0.02 | 0.04 | 0.04 | 0.04 | 0.05 | ||||||
Interaction | CA × Cohesion × AI × LPI | −0.09 | −0.08 | −0.06 | −0.08 | −0.05 | |||||
effect | IS × Height | −0.02 | 0.03 | 0.02 | 0.02 | 0.07 | |||||
IS × Height × PD × SPLIT × LSI | 0.01 | 0.03 | 0.03 | 0.03 | 0.04 | ||||||
PLAND × PD × SPLIT × LSI | 0.03 | 0.05 | 0.05 | 0.05 | 0.06 | ||||||
IS × Height × PLAND | 0.02 | 0.09 | 0.10 | 0.08 | 0.14 | ||||||
PLAND × CA × Cohesion × AI × LPI | −0.09 | −0.09 | −0.07 | −0.09 | −0.06 | ||||||
F | 19.69 | 39.29 | 33.05 | 43.47 | 48.57 | ||||||
R | 0.692 | 0.634 | 0.651 | 0.672 | 0.722 |
Effect Term | LST2022 | LST2020 | LST2017 | LST2015 | LST2013 | ||||||
---|---|---|---|---|---|---|---|---|---|---|---|
Coef | S − Coef | Coef | S − Coef | Coef | S − Coef | Coef | S − Coef | Coef | S − Coef | ||
Constant | 52.54 | 47.90 | 52.56 | 51.97 | 51.66 | ||||||
Main effect | CA | −0.51 | −0.04 | −1.70 | −0.13 | −1.52 | −0.11 | −2.26 | −0.16 | −0.50 | −0.05 |
IS | 0.47 | 0.73 | 0.71 | 0.75 | 0.63 | ||||||
PD | −0.23 | −0.08 | 0.03 | 0.01 | −0.18 | −0.06 | 0.15 | 0.05 | −0.09 | −0.04 | |
PLAND | −0.77 | −0.14 | −0.73 | −0.12 | −0.82 | −0.14 | −0.72 | −0.11 | −0.62 | −0.13 | |
LPI | −0.09 | −0.07 | −0.01 | −0.01 | 0.03 | 0.03 | −0.02 | −0.02 | |||
Cohesion | 0.19 | 0.36 | 0.36 | 0.39 | 0.28 | ||||||
AI | 0.01 | −0.01 | 0.02 | 0.01 | 0.02 | ||||||
Height | −0.94 | −0.52 | −0.94 | −0.49 | −1.08 | −0.55 | −1.15 | −0.55 | −0.61 | −0.38 | |
LSI | −0.03 | −0.08 | −0.03 | −0.07 | −0.05 | −0.11 | −0.05 | −0.11 | −0.02 | −0.05 | |
SPLIT | 0.10 | 0.11 | 0.13 | 0.11 | 0.06 | ||||||
Interaction | PD × SPLIT × LSI | 0.03 | 0.02 | 0.01 | 0.01 | ||||||
effect | CA × Cohesion × AI × LPI | −0.11 | −0.10 | −0.09 | −0.11 | −0.10 | |||||
IS × Height | −0.03 | −0.03 | −0.04 | −0.05 | 0.03 | ||||||
IS × Height × PD × SPLIT × LSI | −0.01 | −0.06 | −0.04 | −0.04 | −0.02 | ||||||
PLAND × PD × SPLIT × LSI | 0.03 | 0.02 | 0.04 | 0.04 | 0.04 | ||||||
IS × Height × PLAND | 0.13 | 0.30 | 0.26 | 0.30 | 0.30 | ||||||
PLAND × CA × Cohesion × AI × LPI | −0.09 | −0.05 | −0.04 | −0.06 | −0.06 | ||||||
F | 25.95 | 54.73 | 42.29 | 46.17 | 53.60 | ||||||
R | 0.576 | 0.753 | 0.727 | 0.718 | 0.751 |
The statements, opinions and data contained in all publications are solely those of the individual author(s) and contributor(s) and not of MDPI and/or the editor(s). MDPI and/or the editor(s) disclaim responsibility for any injury to people or property resulting from any ideas, methods, instructions or products referred to in the content. |
Share and Cite
Guan, Z.; Zhang, H. A Quantitative Analysis of the Complex Response Relationship between Urban Green Infrastructure (UGI) Structure/Spatial Pattern and Urban Thermal Environment in Shanghai. Sustainability 2024 , 16 , 6886. https://doi.org/10.3390/su16166886
Guan Z, Zhang H. A Quantitative Analysis of the Complex Response Relationship between Urban Green Infrastructure (UGI) Structure/Spatial Pattern and Urban Thermal Environment in Shanghai. Sustainability . 2024; 16(16):6886. https://doi.org/10.3390/su16166886
Guan, Zhenru, and Hao Zhang. 2024. "A Quantitative Analysis of the Complex Response Relationship between Urban Green Infrastructure (UGI) Structure/Spatial Pattern and Urban Thermal Environment in Shanghai" Sustainability 16, no. 16: 6886. https://doi.org/10.3390/su16166886
Article Metrics
Article access statistics, further information, mdpi initiatives, follow mdpi.
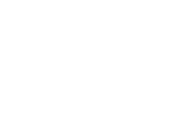
Subscribe to receive issue release notifications and newsletters from MDPI journals
An Overview of Quantitative Research Methods
- August 2023
- INTERNATIONAL JOURNAL OF MULTIDISCIPLINARY RESEARCH AND ANALYSIS 06(08)

- Veritas University College
Discover the world's research
- 25+ million members
- 160+ million publication pages
- 2.3+ billion citations

- Christofer D. Sta. Ana
- Isaiah M. Makhetha

- James C. Royo
- Regine L. Generalao
- Muhammad Ali Zia
- Abdul Rasheed
- Mirana Hanathasia
- Annisa Fitriana Lestari
- Betül Özcan Dost

- David E. McNabb
- H. Johnson Nenty
- Clifford Woody

- Annie W. Ward
- Connie B. Comer
- EDUC PSYCHOL MEAS
- Kenneth D. Hopkins

- Alan Bryman
- Robert E. Stake
- A Thornhill
- Recruit researchers
- Join for free
- Login Email Tip: Most researchers use their institutional email address as their ResearchGate login Password Forgot password? Keep me logged in Log in or Continue with Google Welcome back! Please log in. Email · Hint Tip: Most researchers use their institutional email address as their ResearchGate login Password Forgot password? Keep me logged in Log in or Continue with Google No account? Sign up
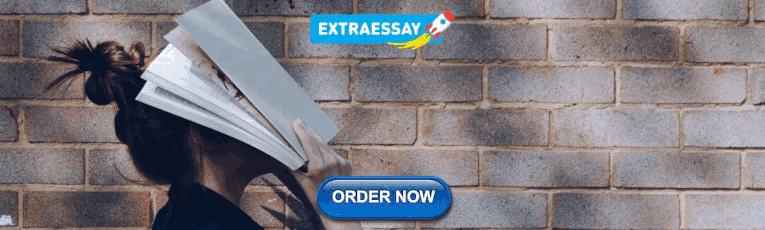
IMAGES
COMMENTS
portant role that descriptive analysis plays in the scientific process in general and education research in particular. It describes how quantitative descriptive analysis can stand on its own as a complete research product or be a component of causal research. Chapter 2. Approaching Descriptive Analysis.
It is important to know w hat kind of data you are planning to collect or analyse as this w ill. affect your analysis method. A 12 step approach to quantitative data analysis. Step 1: Start with ...
methods, so that articles and reports can be evaluated by researchers in different countries. This part of statistics, whose objective is to synthesize, organized and make the presentation of ...
Numeric data collected in a research project can be analysed quantitatively using statistical tools in two different ways. Descriptive analysis refers to statistically describing, aggregating, and presenting the constructs of interest or associations between these constructs.Inferential analysis refers to the statistical testing of hypotheses (theory testing).
Quantitative data analysis is a systematic process of both collecting and evaluating measurable. and verifiable data. It contains a statistical mechanism of assessing or analyzing quantitative ...
can also be used to look at associations or relationship between variables. Quantitative research studies can be placed into one of five categories, although some categories do vary 156 Chapter 6: Quantitative Research Designs: Experimental, Quasi-Experimental, and Descriptive 9781284126464_CH06_PASS02.indd 156 12/01/17 2:53 pm
Descriptive analysis identifies patterns in data to answer questions about who, what, where, when, and to what extent. This guide describes how to more effectively approach, conduct, and communicate quantitative descriptive analysis. The primary audience for this guide includes members of the research community who conduct and publish both ...
Descriptive statistics, such as means and percentages, describe data obtained from empirical observations and measurements, whereas inferen tial statistics are used to make infer ences or draw conclusions about a population, given the data were actu ally obtained for a sample. This article briefly discusses common descriptive data analysis ...
5.1 Introduction. This chapter provides a descriptive analysis of the quantitative data and is divided into five sections. The first section presents the preliminary consideration of data, showing the response rate and the process of data screening and cleaning. The second section deals with the demographic profiles of the respondents.
Given the importance of descriptive questions like this, the purpose of this chapter is to provide principles of sophisticated descriptive analysis in the context of quantitative higher education research, provide relevant, real-world examples of sophisticated descriptions in higher education literature, and demonstrate an application of ...
based decisions rather than exact mathematical proof.The quantitative research processThis guide focuses on descriptive statistics and statistical testing as these are the c. mmon forms of quantitative data analysis required at the university and research level. It is assumed that dat. ollowing stages:Define your aim and research questionsCarry ...
There are 3 main types of descriptive statistics: The distribution concerns the frequency of each value. The central tendency concerns the averages of the values. The variability or dispersion concerns how spread out the values are. You can apply these to assess only one variable at a time, in univariate analysis, or to compare two or more, in ...
duals, conditions, or events. Two commonly used quantitative, non-experimental, descriptive research designs are observational. research and survey res. arch.Observational Research. Some of you may be thinking that this sounds more like a qualitative research d.
Miksza, Peter, and Kenneth Elpus, 'Descriptive Analysis', Design and Analysis for Quantitative Research in Music Education (New York, 2018; online edn, ... This PDF is available to Subscribers Only. View Article Abstract & Purchase Options. For full access to this pdf, sign in to an existing account, or purchase an annual subscription. ...
Descriptive statistics can help in summarizing data in the form of simple quantitative measures such as percentages or means or in the form of visual summaries such as histograms and box plots. Descriptive statistics can be used to describe a single variable (univariate analysis) or more than one variable (bivariate/multivariate analysis).
Descriptive research methods. Descriptive research is usually defined as a type of quantitative research, though qualitative research can also be used for descriptive purposes. The research design should be carefully developed to ensure that the results are valid and reliable.. Surveys. Survey research allows you to gather large volumes of data that can be analyzed for frequencies, averages ...
PDF | On May 20, 2019, Sohil Sharma published Descriptive Research Designs | Find, read and cite all the research you need on ResearchGate. ... A Quantitative Analysis. Article. May 2024;
(Lans & Van Der Voordt, 2002). Descriptive analysis is considered to be expansive than other quantitative methods and It gives a broader picture of an event or phenomenon. Descriptive Analysis can use many number of variables or even a single number of variable to conduct a descriptive study.
The term descriptive research then, refers to research questions, design of the research and data analysis that would be conducted on that topic. It is called an observational research method because none of the variables that are part of the research study are influenced in any capacity. ... Descriptive research is a quantitative research ...
The method also introduced several concepts new to descriptive testing including individual data rather than consensus opinion and graphic line scaling. QDA is consumer oriented in panel selection, training, and attribute language, and is useful for several product development and marketing activities including Product Optimization research.
Quantitative research explains phenomena by collecting numerical unchanging d etailed data t hat. are analyzed using mathematically based methods, in particular statistics that pose questions of ...
Reporting Quantitative Research in Psychology: How to meet APA Style Journal Article Reporting Standards by Harris Cooper This updated edition offers practical guidance for understanding and implementing APA Style Journal Article Reporting Standards (JARS) and Meta‑Analysis Reporting Standards (MARS) for quantitative research. These standards provide the essential information researchers ...
The designs. in this chapter are survey design, descriptive design, correlational design, ex-. perimental design, and causal-comparative design. As we address each research. design, we will learn ...
The urban heat island (UHI) effect has evolved into one of the key environmental problems affecting the urban ecological environment and sustainable development. Based on 52 Urban Thermal Heat spots (UTHSs) with significant differences between land use structure and urban green infrastructure (UGI) spatial layout within the influence range of UHI in Shanghai, Landsat-8/9 satellite images were ...
quantitative research are: Describing a problem statement by presenting the need for an explanation of a variable's relationship. Offering literature, a significant function by answering research ...