Thank you for visiting nature.com. You are using a browser version with limited support for CSS. To obtain the best experience, we recommend you use a more up to date browser (or turn off compatibility mode in Internet Explorer). In the meantime, to ensure continued support, we are displaying the site without styles and JavaScript.
- View all journals
- Explore content
- About the journal
- Publish with us
- Sign up for alerts
- Open access
- Published: 09 October 2023
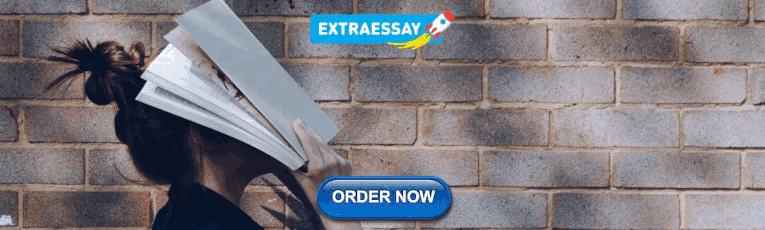
Sign language recognition using the fusion of image and hand landmarks through multi-headed convolutional neural network
- Refat Khan Pathan 1 ,
- Munmun Biswas 2 ,
- Suraiya Yasmin 3 ,
- Mayeen Uddin Khandaker ORCID: orcid.org/0000-0003-3772-294X 4 , 5 ,
- Mohammad Salman 6 &
- Ahmed A. F. Youssef 6
Scientific Reports volume 13 , Article number: 16975 ( 2023 ) Cite this article
16k Accesses
13 Citations
Metrics details
- Computational science
- Image processing
Sign Language Recognition is a breakthrough for communication among deaf-mute society and has been a critical research topic for years. Although some of the previous studies have successfully recognized sign language, it requires many costly instruments including sensors, devices, and high-end processing power. However, such drawbacks can be easily overcome by employing artificial intelligence-based techniques. Since, in this modern era of advanced mobile technology, using a camera to take video or images is much easier, this study demonstrates a cost-effective technique to detect American Sign Language (ASL) using an image dataset. Here, “Finger Spelling, A” dataset has been used, with 24 letters (except j and z as they contain motion). The main reason for using this dataset is that these images have a complex background with different environments and scene colors. Two layers of image processing have been used: in the first layer, images are processed as a whole for training, and in the second layer, the hand landmarks are extracted. A multi-headed convolutional neural network (CNN) model has been proposed and tested with 30% of the dataset to train these two layers. To avoid the overfitting problem, data augmentation and dynamic learning rate reduction have been used. With the proposed model, 98.981% test accuracy has been achieved. It is expected that this study may help to develop an efficient human–machine communication system for a deaf-mute society.
Similar content being viewed by others
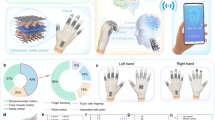
AI enabled sign language recognition and VR space bidirectional communication using triboelectric smart glove
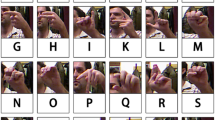
Sign language recognition based on dual-path background erasure convolutional neural network
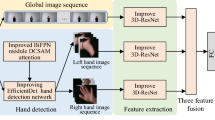
Improved 3D-ResNet sign language recognition algorithm with enhanced hand features
Introduction.
Spoken language is the medium of communication between a majority of the population. With spoken language, it would be workable for a massive extent of the population to impart. Nonetheless, despite spoken language, a section of the population cannot speak with most of the other population. Mute people cannot convey a proper meaning using spoken language. Hard of hearing is a handicap that weakens their hearing and makes them unfit to hear, while quiet is an incapacity that impedes their talking and makes them incapable of talking. Both are just handicapped in their hearing or potentially, therefore, cannot still do many other things. Communication is the only thing that isolates them from ordinary people 1 . As there are so many languages in the world, a unique language is needed to express their thoughts and opinions, which will be understandable to ordinary people, and such a language is named sign language. Understanding sign language is an arduous task, an ability that must be educated with training.
Many methods are available that use different things/tools like images (2D, 3D), sensor data (hand globe 2 , Kinect sensor 3 , neuromorphic sensor 4 ), videos, etc. All things are considered due to the fact that the captured images are excessively noisy. Therefore an elevated level of pre-processing is required. The available online datasets are already processed or taken in a lab environment where it becomes easy for recent advanced AI models to train and evaluate, causing prone to errors in real-life applications with different kinds of noises. Accordingly, it is a basic need to make a model that can deal with noisy images and also be able to deliver positive results. Different sorts of methods can be utilized to execute the classification and recognition of images using machine learning. Apart from recognizing static images, work has been done in depth-camera detecting and video processing 5 , 6 , 7 . Various cycles inserted in the system were created utilizing other programming languages to execute the procedural strategies for the final system's maximum adequacy. The issue can be addressed and deliberately coordinated into three comparable methodologies: initially using static image recognition techniques and pre-processing procedures, secondly by using deep learning models, and thirdly by using Hidden Markov Models.
Sign language guides this part of the community and empowers smooth communication in the community of people with trouble talking and hearing (deaf and dumb). They use hand signals along with facial expressions and body activities to cooperate. Yet, as a global language, not many people become familiar with communication via sign language gestures 8 . Hand motions comprise a significant part of communication through signing vocabulary. At the same time, facial expressions and body activities assume the jobs of underlining the words and phrases communicated by hand motions. Hand motions can be static or dynamic 9 , 10 . There are methodologies for motion discovery utilizing the dynamic vision sensor (DVS), a similar technique used in the framework introduced in this composition. For example, Arnon et al. 11 have presented an event-based gesture recognition system, which measures the event stream utilizing a natively event-based processor from International Business Machines called TrueNorth. They use a temporal filter cascade to create Spatio-temporal frames that CNN executes in the event-based processor, and they reported an accuracy of 96.46%. But in a real-life scenario, corresponding background situations are not static. Therefore the stated power saving process might not work properly. Jun Haeng Lee et al. 12 proposed a motion classification method with two DVSs to get a stereo-vision system. They used spike neurons to handle the approaching occasions with the same real-life issue. Static hand signals are also called hand acts and are framed in different shapes and directions of hands without speaking to any movement data. Dynamic hand motions comprise a sequence of hand stances with related movement information 13 . Using facial expressions, static hand images, and hand signals, communication through signing gives instruments to convey similarly as if communicated in dialects; there are different kinds of communication via gestures as well 14 .
In this work, we have applied a fusion of traditional image processing with extracted hand landmarks and trained on a multi-headed CNN so that it could complement each other’s weights on the concatenation layer. The main objective is to achieve a better detection rate without relying on a traditional single-channel CNN. This method has been proven to work well with less computational power and fewer epochs on medical image datasets 15 . The rest of the paper is divided into multiple sections as literature review in " Literature review " section, materials and methods in " Materials and methods " section with three subsections: dataset description in Dataset description , image pre-processing in " Pre-processing of image dataset " and working procedure in " Working procedure ", result analysis in " Result analysis " section, and conclusion in " Conclusion " section.
Literature review
State-of-the-art techniques centered after utilizing deep learning models to improve good accuracy and less execution time. CNNs have indicated huge improvements in visual object recognition 16 , natural language processing 17 , scene labeling 18 , medical image processing 15 , and so on. Despite these accomplishments, there is little work on applying CNNs to video classification. This is halfway because of the trouble in adjusting the CNNs to join both spatial and fleeting data. Model using exceptional hardware components such as a depth camera has been used to get the data on the depth variation in the image to locate an extra component for correlation, and then built up a CNN for getting the results 19 , still has low accuracy. An innovative technique that does not need a pre-trained model for executing the system was created using a capsule network and versatile pooling 11 .
Furthermore, it was revealed that lowering the layers of CNN, which employs a greedy way to do so, and developing a deep belief network produced superior outcomes compared to other fundamental methodologies 20 . Feature extraction using scale-invariant feature transform (SIFT) and classification using Neural Networks were developed to obtain the ideal results 21 . In one of the methods, the images were changed into an RGB conspire, the data was developed utilizing the movement depth channel lastly using 3D recurrent convolutional neural networks (3DRCNN) to build up a working system 5 , 22 where Canny edge detection oriented FAST and Rotated BRIEF (ORB) has been used. ORB feature detection technique and K-means clustering algorithm used to create the bag of feature model for all descriptors is described, but the plain background, easy to detect edges are totally dependent on edges; if the edges give wrong info, the model may fall accuracy and become the main problem to solve.
In recent years, utilizing deep learning approaches has become standard for improving the recognition accuracy of sign language models. Using Faster Region-based Convolutional Neural Network (Faster-RCNN) 23 , a CNN model is applied for hand recognition in the data image. Rastgoo et al. 24 proposed a method where they cropped an image properly, used fusion between RGB and depth image (RBM), added two noise types (Gaussian noise + salt n paper noise), and prepared the data for training. As a naturally propelled deep learning model, CNNs achieve every one of the three phases with a single framework that is prepared from crude pixel esteems to classifier yields, but extreme computation power was needed. Authors in ref. 25 proposed 3D CNNs where the third dimension joins both spatial and fleeting stamps. It accepts a few neighboring edges as input and performs 3D convolution in the convolutional layers. Along with them, the study reported in 26 followed similar thoughts and proposed regularizing the yields with high-level features, joining the expectations of a wide range of models. They applied the developed models to perceive human activities and accomplished better execution in examination than benchmark methods. But it is not sure it works with hand gestures as they detected face first and thenody movement 27 .
On the other hand, the Microsoft and Leap Motion companies have developed unmistakable approaches to identify and track a user’s hand and body movement by presenting Kinect and the leap motion controller (LMC) separately. Kinect recognizes the body skeleton and tracks the hands, whereas the LMC distinguishes and tracks hands with its underlying cameras and infrared sensors 3 , 28 . Using the provided framework, Sykora et al. 7 utilized the Kinect system to catch the depth data of 10 hand motions to classify them using a speeded-up robust features (SURF) technique that came up to an 82.8% accuracy, but it cannot test on more extensive database and modified feature extraction methods (SIFT, SURF) so it can be caused non-invariant to the orientation of gestures. Likewise, Huang et al. 29 proposed a 10-word-based ASL recognition system utilizing Kinect by tenfold cross-validation with an SVM that accomplished a precision pace of 97% using a set of frame-independent features, but the most significant problem in this method is segmentation.
The literature summarizes that most of the models used in this application either depend on a single variable or require high computational power. Also, their dataset choice for training and validating the model is in plain background, which is easier to detect. Our main aim is to show how to reduce the computational power for training and the dependency of model training on one layer.
Materials and methods
Dataset description.
Using a generalized single-color background to classify sign language is very common. We intended to avoid that single color background and use a complex background with many users’ hand images to increase the detection complexity. That’s why we have used the “ASL Finger Spelling” dataset 30 , which has images of different sizes, orientations, and complex backgrounds of over 500 images per sign (24 sign total) of 4 users (non-native to sign language). This dataset contains separate RGB and depth images; we have worked with the RGB images in this research. The photos were taken in 5 sessions with the same background and lighting. The dataset details are shown in Table 1 , and some sample images are shown in Fig. 1 .
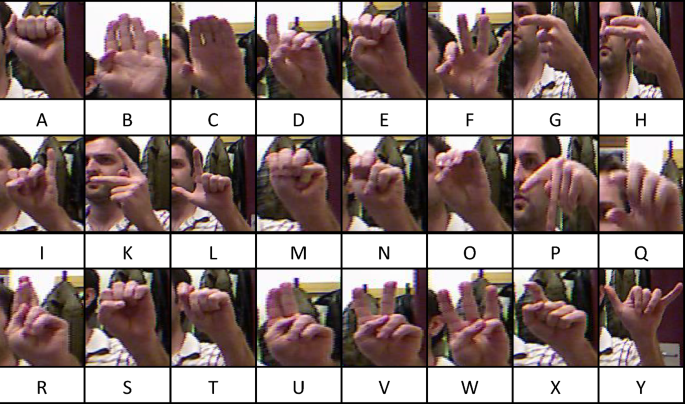
Sample images from a dataset containing 24 signs from the same user.
Pre-processing of image dataset
Images were pre-processed for two operations: preparing the original image training set and extracting the hand landmarks. Traditional CNN has one input data channel and one output channel. We are using two input data channels and one output channel, so data needs to be prepared for both inputs individually.
Raw image processing
In raw image processing, we have converted the images from RGB to grayscale to reduce color complexity. Then we used a 2D kernel matrix for sharpening the images, as shown in Fig. 2 . After that, we resized the images into 50 × 50 pixels for evaluation through CNN. Finally, we have normalized the grayscale values (0–255) by dividing the pixel values by 255, so now the new pixel array contains value ranges (0–1). The primary advantage of this normalization is that CNN works faster in the (0–1) range rather than other limits.
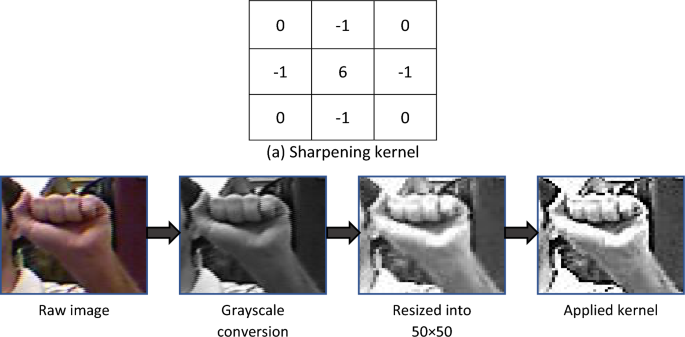
Raw image pre-processing with ( a ) sharpening kernel.
Hand landmark detection
Google’s hand landmark model has an input channel of RGB and an image size of (224 × 224 × 3). So, we have taken the RGB images, converted pixel values into float32, and resized all the images into (256 × 256 × 3). After applying the model, it gives 21 coordinated 3-dimensional points. The landmark detection process is shown in Fig. 3 .
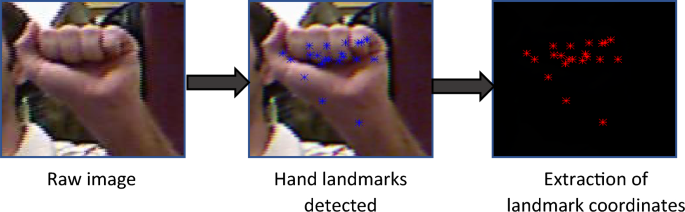
Hand landmarks detection and extraction of 21 coordinates.
Working procedure
The whole work is divided into two main parts, one is the raw image processing, and another one is the hand landmarks extraction. After both individual processing had been completed, a custom lightweight simple multi-headed CNN model was built to train both data. Before processing through a fully connected layer for classification, we merged both channel’s features so that the model could choose between the best weights. This working procedure is illustrated in Fig. 4 .
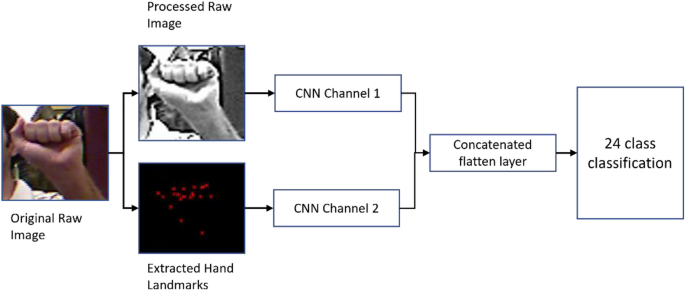
Flow diagram of working procedure.
Model building
In this research, we have used multi-headed CNN, meaning our model has two input data channels. Before this, we trained processed images and hand landmarks with two separate models to compare. Google’s model is not best for “in the wild” situations, so we needed original images to complement the low faults in Google’s model. In the first head of the model, we have used the processed images as input and hand landmarks data as the second head’s input. Two-dimensional Convolutional layers with filter size 50, 25, kernel (3, 3) with Relu, strides 1; MaxPooling 2D with pool size (2, 2), batch normalization, and Dropout layer has been used in the hand landmarks training side. Besides, the 2D Convolutional layer with filter size 32, 64, 128, 512, kernel (3, 3) with Relu; MaxPooling 2D with pool size (2, 2); batch normalization and dropout layer has been used in the image training side. After both flatten layers, two heads are concatenated and go through a dense, dropout layer. Finally, the output dense layer has 24 units with Softmax activation. This model has been compiled with Adam optimizer and MSE loss for 50 epochs. Figure 5 illustrates the proposed CNN architecture, and Table 2 shows the model details.
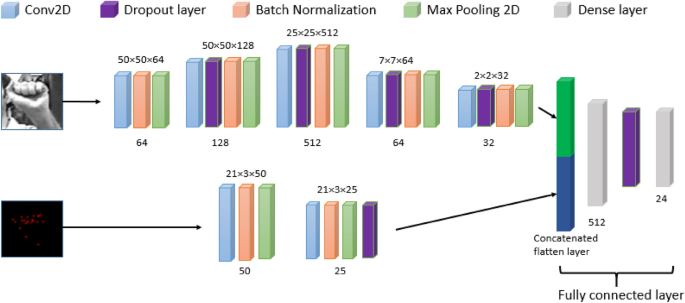
Proposed multi-headed CNN architecture. Bottom values are the number of filters and top values are output shapes.
Training and testing
The input images were augmented to generate more difficulty in training so that the model could not overfit. Image Data Generator did image augmentation with 10° rotation, 0.1 zoom range, 0.1 widths and height shift range, and horizontal flip. Being more conscious about the overfitting issues, we have used dynamic learning rates, monitoring the validation accuracy with patience 5, factor 0.5, and a minimum learning rate of 0.00001. For training, we have used 46,023 images, and for testing, 19,725 images. For 50 epochs, the training vs testing accuracy and loss has been shown in Fig. 6 .
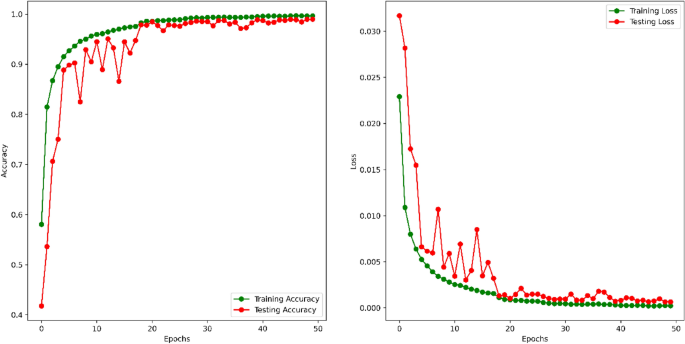
Training versus testing accuracy and loss for 50 epochs.
For further evaluation, we have calculated the precision, recall, and F1 score of the proposed multi-headed CNN model, which shows excellent performance. To compute these values, we first calculated the confusion matrix (shown in Fig. 7 ). When a class is positive and also classified as so, it is called true positive (TP). Again, when a class is negative and classified as so, it is called true negative (TN). If a class is negative and classified as positive, it is called false positive (FP). Also, when a class is positive and classified as not negative, it is called false negative (FN). From these, we can conclude precision, recall, and F1 score like the below:
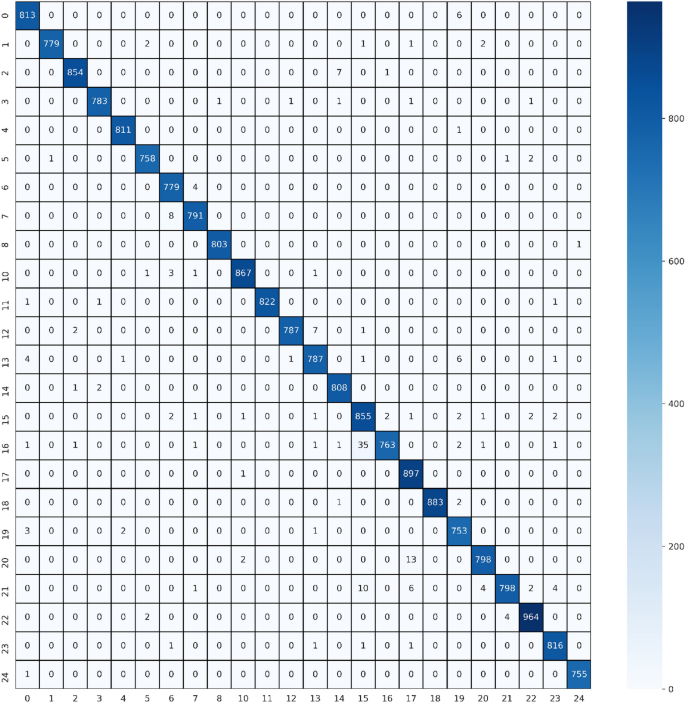
Confusion matrix of the testing dataset. Numerical values in X and Y axis means the sequential letters from A = 0 to Y = 24, number 9 and 25 is missing because dataset does not have letter J and Z.
Precision: Precision is the ratio of TP and total predicted positive observation.
Recall: It is the ratio of TP and total positive observations in the actual class.
F1 score: F1 score is the weighted average of precision and recall.
The Precision, Recall, and F1 score for 24 classes are shown in Table 3 .
Result analysis
In human action recognition tasks, sign language has an extra advantage as it can be used to communicate efficiently. Many techniques have been developed using image processing, sensor data processing, and motion detection by applying different dynamic algorithms and methods like machine learning and deep learning. Depending on methodologies, researchers have proposed their way of classifying sign languages. As technologies develop, we can explore the limitations of previous works and improve accuracy. In ref. 13 , this paper proposes a technique for acknowledging hand motions, which is an excellent part of gesture-based communication jargon, because of a proficient profound deep convolutional neural network (CNN) architecture. The proposed CNN design disposes of the requirement for recognition and division of hands from the captured images, decreasing the computational weight looked at during hand pose recognition with classical approaches. In our method, we used two input channels for the images and hand landmarks to get more robust data, making the process more efficient with a dynamic learning rate adjustment. Besides in ref 14 , the presented results were acquired by retraining and testing the sign language gestures dataset on a convolutional neural organization model utilizing Inception v3. The model comprises various convolution channel inputs that are prepared on a piece of similar information. A capsule-based deep neural network sign posture translator for an American Sign Language (ASL) fingerspelling (posture) 20 has been introduced where the idea concept of capsules and pooling are used simultaneously in the network. This exploration affirms that utilizing pooling and capsule routing on a similar network can improve the network's accuracy and convergence speed. In our method, we have used the pre-trained model of Google to extract the hand landmarks, almost like transfer learning. We have shown that utilizing two input channels could also improve accuracy.
Moreover, ref 5 proposed a 3DRCNN model integrating a 3D convolutional neural network (3DCNN) and upgraded completely associated recurrent neural network (FC-RNN), where 3DCNN learns multi-methodology features from RGB, motion, and depth channels, and FCRNN catch the fleeting data among short video clips divided from the original video. Consecutive clips with a similar semantic significance are singled out by applying the sliding window way to deal with a section of the clips on the whole video sequence. Combining a CNN and traditional feature extractors, capable of accurate and real-time hand posture recognition 26 where the architecture is assessed on three particular benchmark datasets and contrasted and the cutting edge convolutional neural networks. Extensive experimentation is directed utilizing binary, grayscale, and depth data and two different validation techniques. The proposed feature fusion-based CNN 31 is displayed to perform better across blends of approval procedures and image representation. Similarly, fusion-based CNN is demonstrated to improve the recognition rate in our study.
After worldwide motion analysis, the hand gesture image sequence was dissected for keyframe choice. The video sequences of a given gesture were divided in the RGB shading space before feature extraction. This progression enjoyed the benefit of shaded gloves worn by the endorsers. Samples of pixel vectors representative of the glove’s color were used to estimate the mean and covariance matrix of the shading, which was sectioned. So, the division interaction was computerized with no user intervention. The video frames were converted into color HSV (Hue-SaturationValue) space in the color object tracking method. Then the pixels with the following shading were distinguished and marked, and the resultant images were converted to a binary (Gray Scale image). The system identifies image districts compared to human skin by binarizing the input image with a proper threshold value. Then, at that point, small regions from the binarized image were eliminated by applying a morphological operator and selecting the districts to get an image as an applicant of hand.
In the proposed method we have used two-headed CNN to train the processed input images. Though the single image input stream is widely used, two input streams have an advantage among them. In the classification layer of CNN, if one layer is giving a false result, it could be complemented by the other layer’s weight, and it is possible that combining both results could provide a positive outcome. We used this theory and successfully improved the final validation and test results. Before combining image and hand landmark inputs, we tested both individually and acquired a test accuracy of 96.29% for the image and 98.42% for hand landmarks. We did not use binarization as it would affect the background of an image with skin color matched with hand color. This method is also suitable for wild situations as it is not entirely dependent on hand position in an image frame. A comparison of the literature and our work has been shown in Table 4 , which shows that our method overcomes most of the current position in accuracy gain.
Table 5 illustrates that the Combined Model, while having a larger number of parameters and consuming more memory, achieves the highest accuracy of 98.98%. This suggests that the combined approach, which incorporates both image and hand landmark information, is effective for the task when accuracy is priority. On the other hand, the Hand Landmarks Model, despite having fewer parameters and lower memory consumption, also performs impressively with an accuracy of 98.42%. But it has its own error and memory consumption rate in model training by Google. The Image Model, while consuming less memory, has a slightly lower accuracy of 96.29%. The choice between these models would depend on the specific application requirements, trade-offs between accuracy and resource utilization, and the importance of execution time.
This work proposes a methodology for perceiving the classification of sign language recognition. Sign language is the core medium of communication between deaf-mute and everyday people. It is highly implacable in real-world scenarios like communication, human–computer interaction, security, advanced AI, and much more. For a long time, researchers have been working in this field to make a reliable, low cost and publicly available SRL system using different sensors, images, videos, and many more techniques. Many datasets have been used, including numeric sensory, motion, and image datasets. Most datasets are prepared in a good lab condition to do experiments, but in the real world, it may not be a practical case. That’s why, looking into the real-world situation, the Fingerspelling dataset has been used, which contains real-world scenarios like complex backgrounds, uneven image shapes, and conditions. First, the raw images are processed and resized into a 50 × 50 size. Then, the hand landmark points are detected and extracted from these hand images. Making images goes through two processing techniques; now, there are two data channels. A multi-headed CNN architecture has been proposed for these two data channels. Total data has been augmented to avoid overfitting, and dynamic learning rate adjustment has been done. From the prepared data, 70–30% of the train test spilled has been done. With the 30% dataset, a validation accuracy of 98.98% has been achieved. In this kind of large dataset, this accuracy is much more reliable.
There are some limitations found in the proposed method compared with the literature. Some methods might work with low image dataset numbers, but as we use the simple CNN model, this method requires a good number of images for training. Also, the proposed method depends on the hand landmark extraction model. Other hand landmark model can cause different results. In raw image processing, it is possible to detect hand portions to reduce the image size, which may increase the recognition chance and reduce the model training time. Hence, we may try this method in future work. Currently, raw image processing takes a good amount of training time as we considered the whole image for training.
Data availability
The dataset used in this paper (ASL Fingerspelling Images (RGB & Depth)) is publicly available at Kaggle on this URL: https://www.kaggle.com/datasets/mrgeislinger/asl-rgb-depth-fingerspelling-spelling-it-out .
Anderson, R., Wiryana, F., Ariesta, M. C. & Kusuma, G. P. Sign language recognition application systems for deaf-mute people: A review based on input-process-output. Proced. Comput. Sci. 116 , 441–448. https://doi.org/10.1016/j.procs.2017.10.028 (2017).
Article Google Scholar
Mummadi, C. et al. Real-time and embedded detection of hand gestures with an IMU-based glove. Informatics 5 (2), 28. https://doi.org/10.3390/informatics5020028 (2018).
Hickeys Kinect for Windows - Windows apps. (2022). Accessed 01 January 2023. https://learn.microsoft.com/en-us/windows/apps/design/devices/kinect-for-windows
Rivera-Acosta, M., Ortega-Cisneros, S., Rivera, J. & Sandoval-Ibarra, F. American sign language alphabet recognition using a neuromorphic sensor and an artificial neural network. Sensors 17 (10), 2176. https://doi.org/10.3390/s17102176 (2017).
Article ADS PubMed PubMed Central Google Scholar
Ye, Y., Tian, Y., Huenerfauth, M., & Liu, J. Recognizing American Sign Language Gestures from Within Continuous Videos. In 2018 IEEE/CVF Conference on Computer Vision and Pattern Recognition Workshops (CVPRW) , 2145–214509 (IEEE, 2018). https://doi.org/10.1109/CVPRW.2018.00280 .
Ameen, S. & Vadera, S. A convolutional neural network to classify American Sign Language fingerspelling from depth and colour images. Expert Syst. 34 (3), e12197. https://doi.org/10.1111/exsy.12197 (2017).
Sykora, P., Kamencay, P. & Hudec, R. Comparison of SIFT and SURF methods for use on hand gesture recognition based on depth map. AASRI Proc. 9 , 19–24. https://doi.org/10.1016/j.aasri.2014.09.005 (2014).
Sahoo, A. K., Mishra, G. S. & Ravulakollu, K. K. Sign language recognition: State of the art. ARPN J. Eng. Appl. Sci. 9 (2), 116–134 (2014).
Google Scholar
Mitra, S. & Acharya, T. “Gesture recognition: A survey. IEEE Trans. Syst. Man Cybern. Part C 37 (3), 311–324. https://doi.org/10.1109/TSMCC.2007.893280 (2007).
Rautaray, S. S. & Agrawal, A. Vision based hand gesture recognition for human computer interaction: A survey. Artif. Intell. Rev. 43 (1), 1–54. https://doi.org/10.1007/s10462-012-9356-9 (2015).
Amir A. et al A low power, fully event-based gesture recognition system. In 2017 IEEE Conference on Computer Vision and Pattern Recognition (CVPR) , 7388–7397 (IEEE, 2017). https://doi.org/10.1109/CVPR.2017.781 .
Lee, J. H. et al. Real-time gesture interface based on event-driven processing from stereo silicon retinas. IEEE Trans. Neural Netw. Learn Syst. 25 (12), 2250–2263. https://doi.org/10.1109/TNNLS.2014.2308551 (2014).
Article PubMed Google Scholar
Adithya, V. & Rajesh, R. A deep convolutional neural network approach for static hand gesture recognition. Proc. Comput. Sci. 171 , 2353–2361. https://doi.org/10.1016/j.procs.2020.04.255 (2020).
Das, A., Gawde, S., Suratwala, K., & Kalbande, D. Sign language recognition using deep learning on custom processed static gesture images. In 2018 International Conference on Smart City and Emerging Technology (ICSCET) , 1–6 (IEEE, 2018). https://doi.org/10.1109/ICSCET.2018.8537248 .
Pathan, R. K. et al. Breast cancer classification by using multi-headed convolutional neural network modeling. Healthcare 10 (12), 2367. https://doi.org/10.3390/healthcare10122367 (2022).
Article PubMed PubMed Central Google Scholar
Lecun, Y., Bottou, L., Bengio, Y. & Haffner, P. Gradient-based learning applied to document recognition. Proc. IEEE 86 (11), 2278–2324. https://doi.org/10.1109/5.726791 (1998).
Collobert, R., & Weston, J. A unified architecture for natural language processing. In Proceedings of the 25th international conference on Machine learning—ICML ’08 , 160–167 (ACM Press, 2008). https://doi.org/10.1145/1390156.1390177 .
Farabet, C., Couprie, C., Najman, L. & LeCun, Y. Learning hierarchical features for scene labeling. IEEE Trans. Pattern Anal. Mach. Intell. 35 (8), 1915–1929. https://doi.org/10.1109/TPAMI.2012.231 (2013).
Xie, B., He, X. & Li, Y. RGB-D static gesture recognition based on convolutional neural network. J. Eng. 2018 (16), 1515–1520. https://doi.org/10.1049/joe.2018.8327 (2018).
Jalal, M. A., Chen, R., Moore, R. K., & Mihaylova, L. American sign language posture understanding with deep neural networks. In 2018 21st International Conference on Information Fusion (FUSION) , 573–579 (IEEE, 2018).
Shanta, S. S., Anwar, S. T., & Kabir, M. R. Bangla Sign Language Detection Using SIFT and CNN. In 2018 9th International Conference on Computing, Communication and Networking Technologies (ICCCNT) , 1–6 (IEEE, 2018). https://doi.org/10.1109/ICCCNT.2018.8493915 .
Sharma, A., Mittal, A., Singh, S. & Awatramani, V. Hand gesture recognition using image processing and feature extraction techniques. Proc. Comput. Sci. 173 , 181–190. https://doi.org/10.1016/j.procs.2020.06.022 (2020).
Ren, S., He, K., Girshick, R., & Sun, J. Faster r-cnn: Towards real-time object detection with region proposal networks. Adv. Neural Inf. Process Syst. , 28 (2015).
Rastgoo, R., Kiani, K. & Escalera, S. Multi-modal deep hand sign language recognition in still images using restricted Boltzmann machine. Entropy 20 (11), 809. https://doi.org/10.3390/e20110809 (2018).
Jhuang, H., Serre, T., Wolf, L., & Poggio, T. A biologically inspired system for action recognition. In 2007 IEEE 11th International Conference on Computer Vision , 1–8. (IEEE, 2007) https://doi.org/10.1109/ICCV.2007.4408988 .
Ji, S., Xu, W., Yang, M. & Yu, K. 3D convolutional neural networks for human action recognition. IEEE Trans. Pattern Anal. Mach. Intell. 35 (1), 221–231. https://doi.org/10.1109/TPAMI.2012.59 (2013).
Huang, J., Zhou, W., Li, H., & Li, W. sign language recognition using 3D convolutional neural networks. In 2015 IEEE International Conference on Multimedia and Expo (ICME) , 1–6 (IEEE, 2015). https://doi.org/10.1109/ICME.2015.7177428 .
Digital worlds that feel human Ultraleap. Accessed 01 January 2023. Available: https://www.leapmotion.com/
Huang, F., & Huang, S. Interpreting american sign language with Kinect. Journal of Deaf Studies and Deaf Education, [Oxford University Press] , (2011).
Pugeault, N., & Bowden, R. Spelling it out: Real-time ASL fingerspelling recognition. In 2011 IEEE International Conference on Computer Vision Workshops (ICCV Workshops) , 1114–1119 (IEEE, 2011). https://doi.org/10.1109/ICCVW.2011.6130290 .
Rahim, M. A., Islam, M. R. & Shin, J. Non-touch sign word recognition based on dynamic hand gesture using hybrid segmentation and CNN feature fusion. Appl. Sci. 9 (18), 3790. https://doi.org/10.3390/app9183790 (2019).
“ASL Alphabet.” Accessed 01 Jan, 2023. https://www.kaggle.com/grassknoted/asl-alphabet
Download references
Funding was provided by the American University of the Middle East, Egaila, Kuwait.
Author information
Authors and affiliations.
Department of Computing and Information Systems, School of Engineering and Technology, Sunway University, 47500, Bandar Sunway, Selangor, Malaysia
Refat Khan Pathan
Department of Computer Science and Engineering, BGC Trust University Bangladesh, Chittagong, 4381, Bangladesh
Munmun Biswas
Department of Computer and Information Science, Graduate School of Engineering, Tokyo University of Agriculture and Technology, Koganei, Tokyo, 184-0012, Japan
Suraiya Yasmin
Centre for Applied Physics and Radiation Technologies, School of Engineering and Technology, Sunway University, 47500, Bandar Sunway, Selangor, Malaysia
Mayeen Uddin Khandaker
Faculty of Graduate Studies, Daffodil International University, Daffodil Smart City, Birulia, Savar, Dhaka, 1216, Bangladesh
College of Engineering and Technology, American University of the Middle East, Egaila, Kuwait
Mohammad Salman & Ahmed A. F. Youssef
You can also search for this author in PubMed Google Scholar
Contributions
R.K.P and M.B, Conceptualization; R.K.P. methodology; R.K.P. software and coding; M.B. and R.K.P. validation; R.K.P. and M.B. formal analysis; R.K.P., S.Y., and M.B. investigation; S.Y. and R.K.P. resources; R.K.P. and M.B. data curation; S.Y., R.K.P., and M.B. writing—original draft preparation; S.Y., R.K.P., M.B., M.U.K., M.S., A.A.F.Y. and M.S. writing—review and editing; R.K.P. and M.U.K. visualization; M.U.K. and M.B. supervision; M.B., M.S. and A.A.F.Y. project administration; M.S. and A.A.F.Y, funding acquisition.
Corresponding author
Correspondence to Mayeen Uddin Khandaker .
Ethics declarations
Competing interests.
The authors declare no competing interests.
Additional information
Publisher's note.
Springer Nature remains neutral with regard to jurisdictional claims in published maps and institutional affiliations.
Rights and permissions
Open Access This article is licensed under a Creative Commons Attribution 4.0 International License, which permits use, sharing, adaptation, distribution and reproduction in any medium or format, as long as you give appropriate credit to the original author(s) and the source, provide a link to the Creative Commons licence, and indicate if changes were made. The images or other third party material in this article are included in the article's Creative Commons licence, unless indicated otherwise in a credit line to the material. If material is not included in the article's Creative Commons licence and your intended use is not permitted by statutory regulation or exceeds the permitted use, you will need to obtain permission directly from the copyright holder. To view a copy of this licence, visit http://creativecommons.org/licenses/by/4.0/ .
Reprints and permissions
About this article
Cite this article.
Pathan, R.K., Biswas, M., Yasmin, S. et al. Sign language recognition using the fusion of image and hand landmarks through multi-headed convolutional neural network. Sci Rep 13 , 16975 (2023). https://doi.org/10.1038/s41598-023-43852-x
Download citation
Received : 04 March 2023
Accepted : 29 September 2023
Published : 09 October 2023
DOI : https://doi.org/10.1038/s41598-023-43852-x
Share this article
Anyone you share the following link with will be able to read this content:
Sorry, a shareable link is not currently available for this article.
Provided by the Springer Nature SharedIt content-sharing initiative
This article is cited by
- Junming Zhang
- Xiaolong Bu
Scientific Reports (2024)
Boxing behavior recognition based on artificial intelligence convolutional neural network with sports psychology assistant
- Yuanhui Kong
- Zhiyuan Duan
Using LSTM to translate Thai sign language to text in real time
- Werapat Jintanachaiwat
- Kritsana Jongsathitphaibul
- Thitirat Siriborvornratanakul
Discover Artificial Intelligence (2024)
By submitting a comment you agree to abide by our Terms and Community Guidelines . If you find something abusive or that does not comply with our terms or guidelines please flag it as inappropriate.
Quick links
- Explore articles by subject
- Guide to authors
- Editorial policies
Sign up for the Nature Briefing: AI and Robotics newsletter — what matters in AI and robotics research, free to your inbox weekly.

Recent progress in sign language recognition: a review
- Original Paper
- Published: 21 October 2023
- Volume 34 , article number 127 , ( 2023 )
Cite this article
- Aamir Wali ORCID: orcid.org/0000-0002-5314-6113 1 ,
- Roha Shariq 1 ,
- Sajdah Shoaib 1 ,
- Sukhan Amir 1 &
- Asma Ahmad Farhan 1
851 Accesses
Explore all metrics
Sign language is a predominant form of communication among a large group of society. The nature of sign languages is visual, making them distinct from spoken languages. Unfortunately, very few able people can understand sign language making communication with the hearing-impaired infeasible. Research in the field of sign language recognition (SLR) can help reduce the barrier between deaf and able people. Despite having tremendous advances in SLR, unfortunately, this form of recognition is still at least a decade behind speech recognition. There has been a gradual transition from static to isolated to continuous SLR, but still the research is scattered, limited to very small vocabularies, and only suitable for tailor-made conditions. This paper aims to compile recent progress in SLR and presents a comprehensive review of the emerging SLR frameworks and algorithms. We have categorized SLR based on the unit of written text, i.e., letters or alphabets, words and sentences. This review also includes a study-wise summary of the datasets used in different research conducted during the last few years. We identify state-of-the-art techniques for each category. We also suggest novel research directions for future work, and highlight several primary factors contributing to SLR’s inability to achieve improved practical outcomes.
This is a preview of subscription content, log in via an institution to check access.
Access this article
Subscribe and save.
- Get 10 units per month
- Download Article/Chapter or eBook
- 1 Unit = 1 Article or 1 Chapter
- Cancel anytime
Price excludes VAT (USA) Tax calculation will be finalised during checkout.
Instant access to the full article PDF.
Rent this article via DeepDyve
Institutional subscriptions
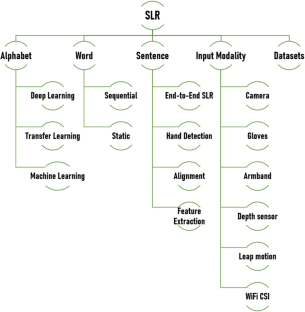
Similar content being viewed by others
An Investigation and Observational Remarks on Conventional Sign Language Recognition
A Survey on Dynamic Sign Language Recognition
A Systematic Study of Sign Language Recognition Systems Employing Machine Learning Algorithms
Bromwich, M.: 360 million people worldwide suffer disabling hearing loss. https://www.shoebox.md/360-million-people-worldwide-suffer-disabling-hearing-loss/ (2022). Accessed 09 Apr 2022
UN, U.N.: International day of sign languages. https://www.un.org/en/observances/sign-languages-day . Accessed 09 Apr 2022
Sign language. https://en.wikipedia.org/wiki/Sign_language . Accessed 09 Apr 2022
Zhu, Q., Li, J., Yuan, F., Gan, Q.: Multi-scale temporal network for continuous sign language recognition (2022). arXiv preprint arXiv:2204.03864
Adaloglou, N.M., et al.: A comprehensive study on deep learning-based methods for sign language recognition. IEEE Trans. Multimed. (2021). https://doi.org/10.1109/TMM.2021.3070438
Article Google Scholar
Rastgoo, R., Kiani, K., Escalera, S.: Sign language recognition: a deep survey. Expert Syst. Appl. 164 , 113794 (2021)
Recent advances in sign language recognition using deep learning techniques
El-Alfy, E.-S.M., Luqman, H.: A comprehensive survey and taxonomy of sign language research. Eng. Appl. Artif. Intell. 114 , 105198 (2022)
Gaikwad, R.S., Admuthe, L.S.: A review of various sign language recognition techniques. Model. Simul. Optim. 8 , 111–126 (2022)
Google Scholar
Arab sign language recognition with convolutional neural networks. IEEE
Sign language recognition using convolutional neural networks. Springer
Shahzad, A., Wali, A.: Computerization of off-topic essay detection: a possibility? Educ. Inf. Technol. 27 (4), 5737–5747 (2022)
Jiang, Z., Zaheer, W., Wali, A., Gilani, S.: Visual sentiment analysis using data-augmented deep transfer learning techniques. Multimed. Tools Appl. 8 , 1–17 (2023)
Yan, C., Gong, B., Wei, Y., Gao, Y.: Deep multi-view enhancement hashing for image retrieval. IEEE Trans. Pattern Anal. Mach. Intell. 43 (4), 1445–1451 (2020)
A new benchmark on american sign language recognition using convolutional neural network. IEEE
Wali, A., Saeed, M.: m-calp-yet another way of generating handwritten data through evolution for pattern recognition. Biosystems 175 , 24–29 (2019)
Wali, A.: Ca-nn: a cellular automata neural network for handwritten pattern recognition. Nat. Comput. 20 , 1–8 (2022)
Wali, A., Ahmad, M., Naseer, A., Tamoor, M., Gilani, S.: Stynmedgan: medical images augmentation using a new GAN model for improved diagnosis of diseases. J. Intell. Fuzzy Syst. 26 , 1–18 (2023)
Xu, Y., Wali, A.: Handwritten pattern recognition using birds-flocking inspired data augmentation technique. IEEE Access (2023)
Mannan, A., et al.: Hypertuned deep convolutional neural network for sign language recognition. Comput. Intell. Neurosci. (2022)
Sign language recognition using deep learning on custom processed static gesture images. IEEE
Mannan, A. et al.: Hypertuned deep convolutional neural network for sign language recognition. Comput. Intell. Neurosci. (2022)
Kasapbaşi, A., Elbushra, A.E.A., Omar, A.-H., Yilmaz, A.: Deepaslr: a CNN based human computer interface for American sign language recognition for hearing-impaired individuals. Comput. Methods Programs Biomed. Update 2 , 100048 (2022)
Zakariah, M., Alotaibi, Y.A., Koundal, D., Guo, Y., Mamun Elahi, M.: Sign language recognition for Arabic alphabets using transfer learning technique. Comput. Intell. Neurosci. (2022)
Thakur, A., Budhathoki, P., Upreti, S., Shrestha, S., Shakya, S.: Real time sign language recognition and speech generation. J. Innov. Image Process. 2 (2), 65–76 (2020)
Yirtici, T., Yurtkan, K.: Regional-CNN-based enhanced Turkish sign language recognition. Signal Image Video Process. 8 , 1–7 (2022)
Sahoo, A.K., Mishra, G.S., Ravulakollu, K.K.: Sign language recognition: state of the art. ARPN J. Eng. Appl. Sci. 9 (2), 116–134 (2014)
Hussain, M.J., et al.: Intelligent sign language recognition system for e-learning context (2022)
American sign language identification using hand trackpoint analysis. Springer
Shah, F., et al.: Sign language recognition using multiple kernel learning: a case study of Pakistan sign language. IEEE Access 9 , 67548–67558 (2021)
Katoch, S., Singh, V., Tiwary, U.S.: Indian sign language recognition system using surf with SVM and CNN. Array 14 , 100141 (2022)
Word-level deep sign language recognition from video: a new large-scale dataset and methods comparison
Context matters: self-attention for sign language recognition. IEEE
Connectionist temporal classification: labelling unsegmented sequence data with recurrent neural networks
Töngi, R.: Application of transfer learning to sign language recognition using an inflated 3d deep convolutional neural network (2021). arXiv preprint arXiv:2103.05111
Sharma, S., Singh, S.: Vision-based hand gesture recognition using deep learning for the interpretation of sign language. Expert Syst. Appl. 182 , 115657 (2021)
Lim, K.M., Tan, A.W.C., Lee, C.P., Tan, S.C.: Isolated sign language recognition using convolutional neural network hand modelling and hand energy image. Multimed. Tools Appl. 78 (14), 19917–19944 (2019)
Sincan, O.M., Keles, H.Y.: Using motion history images with 3d convolutional networks in isolated sign language recognition. IEEE Access 10 , 18608–18618 (2022)
Venugopalan, A., Reghunadhan, R.: Applying hybrid deep neural network for the recognition of sign language words used by the deaf covid-19 patients. Arab. J. Sci. Eng. 8 , 1–14 (2022)
Boukdir, A., Benaddy, M., Ellahyani, A., Meslouhi, O.E., Kardouchi, M.: Isolated video-based Arabic sign language recognition using convolutional and recursive neural networks. Arab. J. Sci. Eng. 47 (2), 2187–2199 (2022)
Sign pose-based transformer for word-level sign language recognition
Yan, C., et al.: Task-adaptive attention for image captioning. IEEE Trans. Circuits Syst. Video Technol. 32 (1), 43–51 (2021)
Yan, C., et al.: Age-invariant face recognition by multi-feature fusionand decomposition with self-attention. ACM Trans. Multimed. Comput. Commun. Appl. 18 (1), 1–18 (2022)
Vaswani, A., et al.: Attention is all you need. Adv. Neural Inf. Process. Syst. 30 , 50 (2017)
Rastgoo, R., Kiani, K., Escalera, S.: Hand sign language recognition using multi-view hand skeleton. Expert Syst. Appl. 150 , 113336 (2020)
Hamza, H.M., Wali, A.: Pakistan sign language recognition: leveraging deep learning models with limited dataset. Mach. Vis. Appl. 34 (5), 71 (2023)
More, V., Sangamnerkar, S., Thakare, V., Mane, D., Dolas, R.: Sign language recognition using image processing. J. NX 8 , 85–87 (2021)
Kumar, A.R., Bhavana, T., Sri, P.M.: A deep neural framework for continuous sign language recognition by iterative training. J. Algebraic Stat. 13 (3), 4574–4584 (2022)
Wang, F., Song, Y., Zhang, J., Han, J., Huang, D.: Temporal unet: sample level human action recognition using wifi (2019). arXiv preprint arXiv:1904.11953
Rastgoo, R., Kiani, K., Escalera, S.: Real-time isolated hand sign language recognition using deep networks and SVD. J. Ambient. Intell. Humaniz. Comput. 13 (1), 591–611 (2022)
Tolentino, L.K.S., et al.: Static sign language recognition using deep learning. Int. J. Mach. Learn. Comput 9 (6), 821–827 (2019)
European Language Resources Association (ELRA). Sign language recognition with transformer networks
ML based sign language recognition system. IEEE
Transferring cross-domain knowledge for video sign language recognition
Wadhawan, A., Kumar, P.: Deep learning-based sign language recognition system for static signs. Neural Comput. Appl. 32 (12), 7957–7968 (2020)
Better sign language translation with STMC-transformer
Deep sign: hybrid CNN-HMM for continuous sign language recognition
Real-time sign language detection using human pose estimation. Springer
Liao, Y., Xiong, P., Min, W., Min, W., Lu, J.: Dynamic sign language recognition based on video sequence with BLSTM-3d residual networks. IEEE Access 7 , 38044–38054 (2019)
Gao, L., et al.: RNN-transducer based Chinese sign language recognition. Neurocomputing 434 , 45–54 (2021)
Spatial-temporal multi-cue network for continuous sign language recognition, vol. 34
Aditya, W., et al.: Novel spatio-temporal continuous sign language recognition using an attentive multi-feature network. Sensors 22 (17), 6452 (2022)
Venugopalan, A., Reghunadhan, R.: Applying deep neural networks for the automatic recognition of sign language words: a communication aid to deaf agriculturists. Expert Syst. Appl. 185 , 115601 (2021)
Fully convolutional networks for continuous sign language recognition. Springer
Kumar, E.K., Kishore, P., Kumar, M.T.K., Kumar, D.A.: 3d sign language recognition with joint distance and angular coded color topographical descriptor on a 2-stream CNN. Neurocomputing 372 , 40–54 (2020)
Khan, R. Sign Language Recognition from a webcam video stream. Master’s thesis, Technische Universität München (2022)
Deep high-resolution representation learning for human pose estimation
Wen, F., Zhang, Z., He, T., Lee, C.: Ai enabled sign language recognition and VR space bidirectional communication using triboelectric smart glove. Nat. Commun. 12 (1), 1–13 (2021)
MyoSign: enabling end-to-end sign language recognition with wearables
Cerna, L.R., Cardenas, E.E., Miranda, D.G., Menotti, D., Camara-Chavez, G.: A multimodal libras-ufop Brazilian sign language dataset of minimal pairs using a microsoft kinect sensor. Expert Syst. Appl. 167 , 114179 (2021)
Mirza, S.F., Al-Talabani, A.K.: Efficient kinect sensor-based Kurdish sign language recognition using echo system network. ARO Sci. J. Koya Univ. 9 (2), 1–9 (2021)
Mittal, A., Kumar, P., Roy, P.P., Balasubramanian, R., Chaudhuri, B.B.: A modified LSTM model for continuous sign language recognition using leap motion. IEEE Sens. J. 19 (16), 7056–7063 (2019)
Lee, C.K., et al.: American sign language recognition and training method with recurrent neural network. Expert Syst. Appl. 167 , 114403 (2021)
Chong, T.-W., Lee, B.-G.: American sign language recognition using leap motion controller with machine learning approach. Sensors 18 (10), 3554 (2018)
Pereira-Montiel, E., et al.: Automatic sign language recognition based on accelerometry and surface electromyography signals: a study for Colombian sign language. Biomed. Signal Process. Control 71 , 103201 (2022)
Visual alignment constraint for continuous sign language recognition
Adaloglou, N., et al.: A comprehensive study on deep learning-based methods for sign language recognition. IEEE Trans. Multimed. 24 , 1750–1762 (2021)
Sign language production: a review
Bazarevsky, V. et al. Blazepose: on-device real-time body pose tracking (2020). arXiv preprint arXiv:2006.10204
Hrúz, M., et al.: One model is not enough: ensembles for isolated sign language recognition. Sensors 22 (13), 5043 (2022)
Zhou, Z., Tam, V.W., Lam, E.Y.: A cross-attention Bert-based framework for continuous sign language recognition. IEEE Signal Process. Lett. 29 , 1818–1822 (2022)
Lugaresi, C. et al.: Mediapipe: a framework for building perception pipelines (2019). arXiv preprint arXiv:1906.08172
Subramanian, B., et al.: An integrated mediapipe-optimized GRU model for Indian sign language recognition. Sci. Rep. 12 (1), 1–16 (2022)
Bidirectional Skeleton-Based Isolated Sign Recognition using Graph Convolution Networks
Download references
This research did not receive any specific grant from funding agencies in the public, commercial, or not-for-profit sectors.
Author information
Authors and affiliations.
FAST School of Computing, National University of Computer and Emerging Science, 852-B, Faisal Town, Lahore, Pakistan
Aamir Wali, Roha Shariq, Sajdah Shoaib, Sukhan Amir & Asma Ahmad Farhan
You can also search for this author in PubMed Google Scholar
Corresponding author
Correspondence to Aamir Wali .
Ethics declarations
Conflict of interest.
The authors declare that they have no relevant financial or non-financial interests to disclose. There is no personal relationship that could influence the work reported in this paper. No funding was received for conducting this study. The authors have no conflicts of interest to declare that are relevant to the content of this article.
Compliance with Ethical Standards
This statement is to certify that the author list is correct. The Authors also confirm that this research has not been published previously and that it is not under consideration for publication elsewhere. On behalf of all Co-Authors, the Corresponding Author shall bear full responsibility for the submission. There is no conflict of interest. This research did not involve any human participants and/or animals.
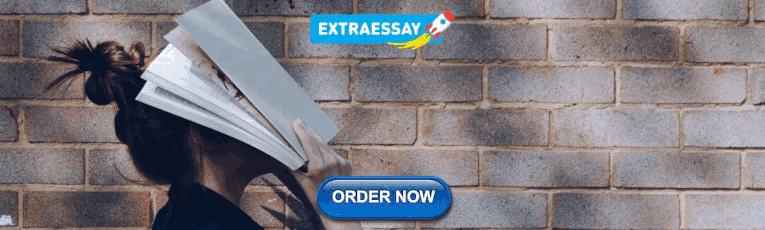
Additional information
Publisher's note.
Springer Nature remains neutral with regard to jurisdictional claims in published maps and institutional affiliations.
Rights and permissions
Springer Nature or its licensor (e.g. a society or other partner) holds exclusive rights to this article under a publishing agreement with the author(s) or other rightsholder(s); author self-archiving of the accepted manuscript version of this article is solely governed by the terms of such publishing agreement and applicable law.
Reprints and permissions
About this article
Wali, A., Shariq, R., Shoaib, S. et al. Recent progress in sign language recognition: a review. Machine Vision and Applications 34 , 127 (2023). https://doi.org/10.1007/s00138-023-01479-y
Download citation
Received : 14 November 2022
Revised : 13 August 2023
Accepted : 11 September 2023
Published : 21 October 2023
DOI : https://doi.org/10.1007/s00138-023-01479-y
Share this article
Anyone you share the following link with will be able to read this content:
Sorry, a shareable link is not currently available for this article.
Provided by the Springer Nature SharedIt content-sharing initiative
- Sign language recognition
- Transformer
- Gesture recognition
- Continuous SLR
- Isolated SLR
- Find a journal
- Publish with us
- Track your research
Information
- Author Services
Initiatives
You are accessing a machine-readable page. In order to be human-readable, please install an RSS reader.
All articles published by MDPI are made immediately available worldwide under an open access license. No special permission is required to reuse all or part of the article published by MDPI, including figures and tables. For articles published under an open access Creative Common CC BY license, any part of the article may be reused without permission provided that the original article is clearly cited. For more information, please refer to https://www.mdpi.com/openaccess .
Feature papers represent the most advanced research with significant potential for high impact in the field. A Feature Paper should be a substantial original Article that involves several techniques or approaches, provides an outlook for future research directions and describes possible research applications.
Feature papers are submitted upon individual invitation or recommendation by the scientific editors and must receive positive feedback from the reviewers.
Editor’s Choice articles are based on recommendations by the scientific editors of MDPI journals from around the world. Editors select a small number of articles recently published in the journal that they believe will be particularly interesting to readers, or important in the respective research area. The aim is to provide a snapshot of some of the most exciting work published in the various research areas of the journal.
Original Submission Date Received: .
- Active Journals
- Find a Journal
- Proceedings Series
- For Authors
- For Reviewers
- For Editors
- For Librarians
- For Publishers
- For Societies
- For Conference Organizers
- Open Access Policy
- Institutional Open Access Program
- Special Issues Guidelines
- Editorial Process
- Research and Publication Ethics
- Article Processing Charges
- Testimonials
- Preprints.org
- SciProfiles
- Encyclopedia
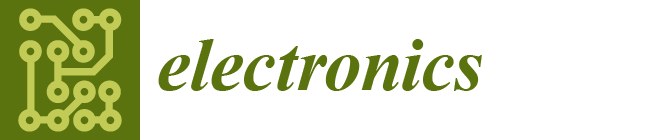
Article Menu
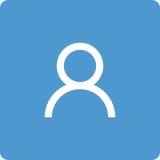
- Subscribe SciFeed
- Recommended Articles
- Google Scholar
- on Google Scholar
- Table of Contents
Find support for a specific problem in the support section of our website.
Please let us know what you think of our products and services.
Visit our dedicated information section to learn more about MDPI.
JSmol Viewer
Deepsign: sign language detection and recognition using deep learning.

1. Introduction
2. related work, 3. methodology, proposed lstm-gru-based model.
- The feature vectors are extracted using InceptionResNetV2 and passed to the model. Here, the video frames are classified into objects with InceptionResNet-2; then, the task is to create key points stacked for video frames;
- The first layer of the neural network is composed of a combination of LSTM and GRU. This composition can be used to capture the semantic dependencies in a more effective way;
- The dropout is used to reduce overfitting and improve the model’s generalization ability;
- The final output is obtained through the ‘softmax’ function.
- The LSTM layer of 1536 units, 0.3 dropouts, and a kernel regularizer of ′l2′ receive data from the input layer;
- Then, the data are passed from the GRU layer using the same parameters;
- Results are passed to a fully connected dense layer;
- The output is fed to the dropout layer, with an effective value of 0.3.
4. Experiments and Results
4.1. dataset, 4.2. results, 5. discussion and limitations, 6. conclusions, author contributions, data availability statement, acknowledgments, conflicts of interest.
- Ministry of Statistics & Programme Implementation. Available online: https://pib.gov.in/PressReleasePage.aspx?PRID=1593253 (accessed on 5 January 2022).
- Manware, A.; Raj, R.; Kumar, A.; Pawar, T. Smart Gloves as a Communication Tool for the Speech Impaired and Hearing Impaired. Int. J. Emerg. Technol. Innov. Res. 2017 , 4 , 78–82. [ Google Scholar ]
- Wadhawan, A.; Kumar, P. Sign language recognition systems: A decade systematic literature review. Arch. Comput. Methods Eng. 2021 , 28 , 785–813. [ Google Scholar ] [ CrossRef ]
- Papastratis, I.; Chatzikonstantinou, C.; Konstantinidis, D.; Dimitropoulos, K.; Daras, P. Artificial Intelligence Technologies for Sign Language. Sensors 2021 , 21 , 5843. [ Google Scholar ] [ CrossRef ] [ PubMed ]
- Nandy, A.; Prasad, J.; Mondal, S.; Chakraborty, P.; Nandi, G. Recognition of Isolated Indian Sign Language Gesture in Real Time. Commun. Comput. Inf. Sci. 2010 , 70 , 102–107. [ Google Scholar ]
- Mekala, P.; Gao, Y.; Fan, J.; Davari, A. Real-time sign language recognition based on neural network architecture. In Proceedings of the IEEE 43rd Southeastern Symposium on System Theory, Auburn, AL, USA, 14–16 March 2011. [ Google Scholar ]
- Chen, J.K. Sign Language Recognition with Unsupervised Feature Learning ; CS229 Project Final Report; Stanford University: Stanford, CA, USA, 2011. [ Google Scholar ]
- Sharma, M.; Pal, R.; Sahoo, A. Indian sign language recognition using neural networks and KNN classifiers. J. Eng. Appl. Sci. 2014 , 9 , 1255–1259. [ Google Scholar ]
- Agarwal, S.R.; Agrawal, S.B.; Latif, A.M. Article: Sentence Formation in NLP Engine on the Basis of Indian Sign Language using Hand Gestures. Int. J. Comput. Appl. 2015 , 116 , 18–22. [ Google Scholar ]
- Wazalwar, S.S.; Shrawankar, U. Interpretation of sign language into English using NLP techniques. J. Inf. Optim. Sci. 2017 , 38 , 895–910. [ Google Scholar ] [ CrossRef ]
- Shivashankara, S.; Srinath, S. American Sign Language Recognition System: An Optimal Approach. Int. J. Image Graph. Signal Process. 2018 , 10 , 18–30. [ Google Scholar ]
- Camgoz, N.C.; Hadfield, S.; Koller, O.; Ney, H.; Bowden, R. Neural Sign Language Translation. In Proceedings of the IEEE Conference on Computer Vision and Pattern Recognition (CVPR) 2018, Salt Lake City, UT, USA, 18–22 June 2018; IEEE: Piscataway, NJ, USA, 2018. [ Google Scholar ]
- Muthu Mariappan, H.; Gomathi, V. Real-Time Recognition of Indian Sign Language. In Proceedings of the International Conference on Computational Intelligence in Data Science, Haryana, India, 6–7 September 2019. [ Google Scholar ]
- Mittal, A.; Kumar, P.; Roy, P.P.; Balasubramanian, R.; Chaudhuri, B.B. A Modified LSTM Model for Continuous Sign Language Recognition Using Leap Motion. IEEE Sens. J. 2019 , 19 , 7056–7063. [ Google Scholar ] [ CrossRef ]
- De Coster, M.; Herreweghe, M.V.; Dambre, J. Sign Language Recognition with Transformer Networks. In Proceedings of the Conference on Language Resources and Evaluation (LREC 2020), Marseille, France, 13–15 May 2020; pp. 6018–6024. [ Google Scholar ]
- Jiang, S.; Sun, B.; Wang, L.; Bai, Y.; Li, K.; Fu, Y. Skeleton aware multi-modal sign language recognition. In Proceedings of the IEEE/CVF Conference on Computer Vision and Pattern Recognition, Nashville, TN, USA, 21–24 June 2021; pp. 3413–3423. [ Google Scholar ]
- Liao, Y.; Xiong, P.; Min, W.; Min, W.; Lu, J. Dynamic Sign Language Recognition Based on Video Sequence with BLSTM-3D Residual Networks. IEEE Access 2019 , 7 , 38044–38054. [ Google Scholar ] [ CrossRef ]
- Adaloglou, N.; Chatzis, T. A Comprehensive Study on Deep Learning-based Methods for Sign Language Recognition. IEEE Trans. Multimed. 2022 , 24 , 1750–1762. [ Google Scholar ] [ CrossRef ]
- Aparna, C.; Geetha, M. CNN and Stacked LSTM Model for Indian Sign Language Recognition. Commun. Comput. Inf. Sci. 2020 , 1203 , 126–134. [ Google Scholar ] [ CrossRef ]
- Szegedy, C.; Ioffe, S.; Vanhoucke, V.; Alemi, A.A. Inception-v4, Inception-ResNet and the Impact of Residual Connections on Learning. arXiv 2016 , arXiv:1602.07261. [ Google Scholar ]
- Yang, D.; Martinez, C.; Visuña, L.; Khandhar, H.; Bhatt, C.; Carretero, J. Detection and Analysis of COVID-19 in medical images using deep learning techniques. Sci. Rep. 2021 , 11 , 19638. [ Google Scholar ] [ CrossRef ] [ PubMed ]
- Likhar, P.; Bhagat, N.K.; Rathna, G.N. Deep Learning Methods for Indian Sign Language Recognition. In Proceedings of the 2020 IEEE 10th International Conference on Consumer Electronics (ICCE-Berlin), Berlin, Germany, 9–11 November 2020; pp. 1–6. [ Google Scholar ] [ CrossRef ]
- Hochreiter, S.; Schmidhuber, J. Long Short-term Memory. Neural Comput. 1997 , 9 , 1735–1780. [ Google Scholar ] [ CrossRef ] [ PubMed ]
- Le, X.-H.; Hung, V.; Ho, G.L.; Sungho, J. Application of Long Short-Term Memory (LSTM) Neural Network for Flood Forecasting. Water 2019 , 11 , 1387. [ Google Scholar ] [ CrossRef ] [ Green Version ]
- Yan, S. Understanding LSTM and Its Diagrams. Available online: https://medium.com/mlreview/understanding-lstm-and-its-diagrams-37e2f46f1714 (accessed on 19 January 2022).
- Chen, J. CS231A Course Project Final Report Sign Language Recognition with Unsupervised Feature Learning. 2012. Available online: http://vision.stanford.edu/teaching/cs231a_autumn1213_internal/project/final/writeup/distributable/Chen_Paper.pdf (accessed on 15 March 2022).
Click here to enlarge figure
Author | Methodology | Dataset | Accuracy |
---|---|---|---|
Mittal et al. (2019) [ ] | 2D-CNN and Modified LSTM, with Leap motion sensor | ASL | 89.50% |
Aparna and Geetha (2019) [ ] | CNN and 2layer LSTM | Custom Dataset (6 signs) | 94% |
Jiang et al. (2021) [ ] | 3DCNN with SL-GCN using RGB-D modalities | AUTSL | 98% |
Liao et al. (2019) [ ] | 3D- ConvNet with BLSTM | DEVISIGN_D | 89.8% |
Adaloglou et al. (2021) [ ] | Inflated 3D ConvNet with BLSTM | RGB + D | 89.74% |
IISL2020 (Our Dataset) | AUTSL | GSL | |||||||
---|---|---|---|---|---|---|---|---|---|
Model | Precision | Recall | F1-Score | Precision | Recall | F1-Score | Precision | Recall | F1-Score |
GRU-GRU | 0.92 | 0.90 | 0.90 | 0.93 | 0.90 | 0.90 | 0.93 | 0.92 | 0.93 |
LSTM-LSTM | 0.96 | 0.96 | 0.95 | 0.89 | 0.89 | 0.89 | 0.90 | 0.89 | 0.89 |
GRU-LSTM | 0.91 | 0.89 | 0.89 | 0.90 | 0.89 | 0.89 | 0.91 | 0.90 | 0.90 |
LSTM-GRU | 0.97 | 0.97 | 0.97 | 0.95 | 0.94 | 0.95 | 0.95 | 0.94 | 0.94 |
MDPI stays neutral with regard to jurisdictional claims in published maps and institutional affiliations. |
Share and Cite
Kothadiya, D.; Bhatt, C.; Sapariya, K.; Patel, K.; Gil-González, A.-B.; Corchado, J.M. Deepsign: Sign Language Detection and Recognition Using Deep Learning. Electronics 2022 , 11 , 1780. https://doi.org/10.3390/electronics11111780
Kothadiya D, Bhatt C, Sapariya K, Patel K, Gil-González A-B, Corchado JM. Deepsign: Sign Language Detection and Recognition Using Deep Learning. Electronics . 2022; 11(11):1780. https://doi.org/10.3390/electronics11111780
Kothadiya, Deep, Chintan Bhatt, Krenil Sapariya, Kevin Patel, Ana-Belén Gil-González, and Juan M. Corchado. 2022. "Deepsign: Sign Language Detection and Recognition Using Deep Learning" Electronics 11, no. 11: 1780. https://doi.org/10.3390/electronics11111780
Article Metrics
Article access statistics, further information, mdpi initiatives, follow mdpi.
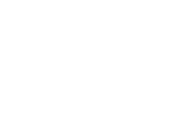
Subscribe to receive issue release notifications and newsletters from MDPI journals
- Architecture and Design
- Asian and Pacific Studies
- Business and Economics
- Classical and Ancient Near Eastern Studies
- Computer Sciences
- Cultural Studies
- Engineering
- General Interest
- Geosciences
- Industrial Chemistry
- Islamic and Middle Eastern Studies
- Jewish Studies
- Library and Information Science, Book Studies
- Life Sciences
- Linguistics and Semiotics
- Literary Studies
- Materials Sciences
- Mathematics
- Social Sciences
- Sports and Recreation
- Theology and Religion
- Publish your article
- The role of authors
- Promoting your article
- Abstracting & indexing
- Publishing Ethics
- Why publish with De Gruyter
- How to publish with De Gruyter
- Our book series
- Our subject areas
- Your digital product at De Gruyter
- Contribute to our reference works
- Product information
- Tools & resources
- Product Information
- Promotional Materials
- Orders and Inquiries
- FAQ for Library Suppliers and Book Sellers
- Repository Policy
- Free access policy
- Open Access agreements
- Database portals
- For Authors
- Customer service
- People + Culture
- Journal Management
- How to join us
- Working at De Gruyter
- Mission & Vision
- De Gruyter Foundation
- De Gruyter Ebound
- Our Responsibility
- Partner publishers

Your purchase has been completed. Your documents are now available to view.
Sign language identification and recognition: A comparative study
Sign Language (SL) is the main language for handicapped and disabled people. Each country has its own SL that is different from other countries. Each sign in a language is represented with variant hand gestures, body movements, and facial expressions. Researchers in this field aim to remove any obstacles that prevent the communication with deaf people by replacing all device-based techniques with vision-based techniques using Artificial Intelligence (AI) and Deep Learning. This article highlights two main SL processing tasks: Sign Language Recognition (SLR) and Sign Language Identification (SLID). The latter task is targeted to identify the signer language, while the former is aimed to translate the signer conversation into tokens (signs). The article addresses the most common datasets used in the literature for the two tasks (static and dynamic datasets that are collected from different corpora) with different contents including numerical, alphabets, words, and sentences from different SLs. It also discusses the devices required to build these datasets, as well as the different preprocessing steps applied before training and testing. The article compares the different approaches and techniques applied on these datasets. It discusses both the vision-based and the data-gloves-based approaches, aiming to analyze and focus on main methods used in vision-based approaches such as hybrid methods and deep learning algorithms. Furthermore, the article presents a graphical depiction and a tabular representation of various SLR approaches.
1 Introduction
Based on the World Health Organization (WHO) statistics, there are over 360 million people with hearing loss disability (WHO 2015 [ 1 , 2 ]). This number has increased to 466 million by 2020, and it is estimated that by 2050 over 900 million people will have hearing loss disability. According to the world federation of deaf people, there are about 300 sign languages (SLs) used around the world. SL is the bridge for communication between deaf and normal people. It is defined as a mode of interaction for the hard of hearing people through a collection of hand gestures, postures, movements, and facial expressions or movements which correspond to letters and words in our real life. To communicate with deaf people, an interpreter is needed to translate real-world words and sentences. So, deaf people can understand us or vice versa . Unfortunately, deaf people do not have a written form and have a huge lack of electronic resources. The most common SLs are American Sign Language (ASL) [ 3 ], Spanish Sign Language (SSL) [ 4 ], Australian Sign Language (AUSLAN) [ 5 ], and Arabic Sign Language (ArSL) [ 6 ]. Some of these societies use only one hand for sign languages such as USA, France, and Russia, while others use two-hands like UK, Turkey, and Czech Republic.
The need for an organized and unified SL was first discussed in World Sign Congress in 1951. The British Deaf Association (BDA) Published a book named Gestuno [ 7 ]. Gestuno is an International SL for the Deaf which contains a vocabulary list of about 1,500 signs. The name “Gestuno” was chosen referencing gesture and oneness. This language arises in the Western and Middle Eastern languages. Gestuno is considered a pidgin of SLs with limited lexicons. It was established in different countries such as US, Denmark, Italy, Russia, and Great Britain, in order to cover the international meetings of deaf people. Although, Gestuno cannot be considered as a language due to several reasons. First, no children or ordinary people grow up using this global language. Second, it has no unified grammar (their book contains only a collection of signs without any grammar). Third, there are a fewer number of specialized people who are fluent or professional in practicing this language. Last, it is not used daily in any single country and it is not likely that people replace their national SL with this international one [ 8 ].
ASL has many linguistics that is difficult to be understood by researchers who are interested in technology, so experts of SLs are needed to facilitate these difficulties. SL has many building blocks that are known as phonological features. These features are represented as hand gestures, facial expressions, and body movements. Each one of these three phonological features has its own shape which differs and varies from one sign to another one. A word/an expression may have similar phonological features in different SLs. For example, the word “drink” could be represented similarly in the three languages ASL, ArSL, and SSL [ 16 ]. On the other hand, a word/an expression may have different phonological features in different SLs. For example, the word “Stand” in American and the word “يقف” (stand) in Arabic are represented differently in the two SLs. The process of understanding a SL by a machine is called Sign Language Processing (SLP) [ 9 ]. Many research problems are suggested in this domain such as Sign Language Recognition (SLR), Sign Language Identification (SLID), Sign Language Synthesis, and Sign Language Translation [ 10 ]. This article covers the first two tasks: SLR and SLID.
SLR basically depends on what is the translation of any hand gesture and posture included in SL, and continues/deals from sign gesture until the step of text generation to the ordinary people to understand deaf people. To detect any sign, a feature extraction step is a crucial phase in the recognition system. It plays the most important role in sign recognition. They must be unique, normalized, and preprocessed. Many algorithms have been suggested to solve sign recognition ranging from traditional machine learning (ML) algorithms to deep learning algorithms as we shall discuss in the upcoming sections. On the other hand, few researchers have focused on SLID [ 11 ]. SLID is the task of assigning a language when given a collection of hand gestures, postures, movements, and facial expressions or movements. The term “SLID” raised in the last decade as a result of many attempts to globalize and identify a global SL. The identification process is considered as a multiclass classification problem. There are many contributions in SLR with prior surveys. The latest survey was a workshop [ 12 , 13 ]. To the best of our knowledge, no prior works have surveyed SLID in previous Literature. This shortage was due to the need for experts who can explain and illustrate many different SLs to researchers. Also, this shortage due to the distinction between any SL and its spoken language [ 8 , 14 ] (i.e., ASL is not a manual form of English and does not have a unified written form).
Although many SLR models have been developed, to the best of our knowledge, none of them can be used to recognize multiple SLs. At the same time, in recent decades, the need for a reliable system that could interact and communicate with people from different nations with different SLs is of great necessity [ 15 ]. COVID-19 Coronavirus is a global pandemic that forced a huge percentage of employees to work and contact remotely. Deaf people need to contact and attend online meetings using different platforms such as Zoom, Microsoft Team, and Google Meeting rooms. So, we need to identify and globalize a unique SL as excluding deaf people and discarding their attendance will affect the whole work progress and damage their psyche which emphasizes the principle of “nothing about us without us.” Also, SL occupies a big space of all daily life activities such as TV sign translators, local conferences sign translators, and international sign translators which is a big issue to translate all conference’s points to all deaf people from different nations, as every deaf person requires a translator of their own SL to translate and communicate with him. In Deaflympics 2010, many deaf athletics were invited for this international Olympics. They need to interact and communicate with each other or even with anybody in their residence [ 16 ]. Building an interactive unified recognizer system is a challenge [ 11 ] as there are many words/expressions with the same sign in different languages, other words/expressions with different signs in the different languages, and other words/expressions could be expressed using the hands beside the movements of the eyebrows, mouth, head, shoulders, and eye gaze. For example, in ASL, raised eyebrows indicate an open-ended question, and furrowed eyebrows indicate a yes/no question. SLs could also be modified by mouth movements. For example, expressing the sign CUP with different mouth positions may indicate cup size, also body movements which may be included while expressing any SL provides different meanings. SLID will help in breaking down all these barriers for SL across the world.
Traditional machine and deep learning algorithms were applied to different SLs to recognize and detect signs. Most proposed systems achieved promising results and indicated significant improvements in SL recognition accuracy. According to higher results in SLR on different SLs, a new task of SLID arises to achieve more stability and facility in deaf and ordinary people communication. SLID has many subtasks starting from image preprocessing, segmentation, feature extraction, and image classification. Most proposed models for recognition were applied to a single dataset, whereas the proposed SLID was applied to more than one SL dataset [ 11 ]. SLID inherits all SLR challenges, such as background and illumination variance [ 17 ], also skin detection and hands segmentation using both static and dynamic gestures. Challenges are doubled and maximized in SLID as many characters and words in different signs share the same hand’s gestures, body movements, and so on, but may differ by considering facial expressions. For example, in ASL, raised eyebrows indicate an open-ended question, and furrowed eyebrows indicate a yes/no question, SL could also be modified by mouth movements.
Despite the need for interdisciplinary learning and knowledge of sign linguistics, most existing research does not go in depth but tackles the most important topics and separate portions. In this survey, we will introduce three important questions – (1) Why SLID is important? (2) What are the challenges to solve SLID? (3) what is the most used sign language for identifications and why? A contact inequality of SLs arises from this communication, whether it is in an informal personal context or in a formal international context. Deaf people have therefore used a kind of auxiliary gestural system for international communication at sporting or cultural events since the early 19th century [ 18 ]. Spoken languages like English are the most used language between all countries and many people thought it is a globally spoken language. Unfortunately, it is like all local languages. On the other side, for deaf people, many of them thought that ASL is the universal SL.
Furthermore, this article compares the different machine and deep learning models applied on different datasets, identifies best deep learning parameters such as, neural network, activation function, number of Epochs, best optimization functions, and so on, and highlights the main state of the art contributions in SLID. It also covers the preprocessing steps required for sign recognition, the devices used for this task, and the used techniques. The article tries to answer the questions: Which algorithms and datasets had achieved high accuracy? What are the main sub-tasks that every paper seeks to achieve? Are they successful in achieving the main goal or not?
This survey will also be helpful in our next research which will be about SLID, which requires deep understanding of more trending techniques and procedures used in SLR and SLID. Also, the survey compares the strengths and weaknesses of different algorithms and preprocessing steps to recognize signs in different SLs. Furthermore, it will be helpful to other researchers to be more aware of SL techniques.
The upcoming sections are arranged as follows: Datasets of different SLs are described in Section 2 . The preprocessing steps for these datasets, that are prerequisite for all SL aspects, and the required devices will be discussed in Section 3 . Section 4 includes the applied techniques for SL. Section 5 comprehensively compares the results and the main contributions of these addressed models. Finally, the conclusion and the future work will be discussed in Section 6 .
In this section, we discuss many datasets that had been used in different SL aspects such as skin and body detection, image segmentation, feature extraction, gesture recognition, and sign identification for more advanced approaches. For each dataset, we try to explore the structure of the dataset, the attributes with significant effects in the training and testing processes, the advantages and disadvantages of the dataset, and the content of the dataset (images, videos, or gloves). Also, we try to compare the accuracies of the dataset when applying different techniques to it [ 19 ]. Table 1 summarizes the results of these comparisons.
A comparison between different datasets
Name | Devices | No. of participants | Supported language | Size | Content (image/video/gloves) |
---|---|---|---|---|---|
RWTH German fingerspelling [ ] | Webcam | 20 | GSL | 1,400 (images) | |
RWTH-BOSTON-104 [ , ] | Digital camera [black – white] | Not mentioned | ASL | 201 (videos) | Video |
CORPUS-NGT [ ] | Digital camera | 92 | NSL | 72 h | Video |
CopyCat games [ ] | Gloves with accelerometer | 30 | ASL | 5,829 (phrases) | Gloves |
Multiple dataset [ ] | DG5-VHand data gloves and Polhemus G4 | Not mentioned | ArSL | 40 (phrases) | Gloves |
ArSL dataset [ ] | Digital camera | Not mentioned | ArSL | 20 (words) | Video |
SIGNUM [ ] | Digital camera | 25 | DGS | 455 (signs)/19k (sentences) | Video |
ISL-HS [ ] | Apple iPhone-7 | 6 | ISL | 468 videos [58,114 images] | Image/video |
Camgoz et al. [ ] | Microsoft Kinect v2 sensor | 10 | TSL | 855 (signs) | Video |
SMILE [ ] | Microsoft Kinect, 2 video cameras and 3 webcams | 60 learners and signers | SGSL | 100 (signs) | Video |
Gebre et al. [ ] | Digital camera | 9 (British) | BSL/GSL | ∼16 h | Video |
10 (Greek) | |||||
Adaloglou et al. [ ] | Intel RealSense D435 RGB + D camera | 7 (Native Greek signers) | GSL | 6.44 h | Video |
Sahoo [ ] | Sony digital camera | 100 users | ISL | 5,000 images | Image |
RKS-PERSIANSIGN [ ] | Digital camera | 10 | PSL | 10,000 RGB videos | Video |
MS-ASL [ ] | — | 100 | ASL | 25,000 videos | Video |
WASL [ ] | — | 119 | ASL | 21,083 videos | Video |
AUTSL [ ] | Microsoft Kinect v2 | 43 | TSL | 38,336 videos | Video |
KArSL [ ] | Multi-modal Microsoft Kinect V2 | 3 | ArSL | 75,300 videos | Video |
Breland [ ] | Raspberry pi with a thermal camera | — | — | 3,200 images | Image |
Mittal et al. [ ] | Leap motion sensor | 6 | ISL | 3,150 signs | Video |
ASL: American Sign Language, DGS: German Sign Language, NSL: Netherlands Sign Language, TSL: Turkish Sign Language, LSF: French Sign Language, ISL: Irish Sign Language, SGSL: Swiss German Sign Language, ISL: Indian Sign Language, PSL: Persian Sign Language.
CopyCate Game [ 20 ]: a dataset that was collected from deaf children for educational adventure game purpose. These games facilitate interaction with computers using gesture recognition technology. Children wear two colored gloves (red and purple), one glove on each hand. They had collected about 5,829 phrases over 4 phases, with a total number of 9 deployments, each phrase has about 3, 4, or 5 signs taken from a vocabulary token of about 22 signs which is a list of adjectives, objects, prepositions, and subjects. The phrases have the following format:
[adjective1] subject preposition [adjective2] object.
Some disadvantages of this dataset are library continuity, sensor changes, varied environments, data integrity, and sign variation. Another disadvantage and disability are wearing gloves because users must interact with systems using gloves. On the other hand, it has the advantage of integrating new data they gathered from other deployments into its libraries.
Multiple Dataset [ 25 ]: Collected two datasets of ArSL, consisting of 40 phrases with 80-word lexicon, each phrase was repeated 10 times, using DG5-Vhand data glove with five sensors on each finger with an embedded accelerometer. It was collected using two Polhemus G4 motion trackers providing six different measurements. Dataset (Number.2) was collected using a digital camera without wearing gloves for capturing signs.
ArSL Dataset [ 26 ]: Digital cameras are used to capture signer’s gestures, then videos are stored as a AVI video format to be analyzed later. Data were captured from deaf volunteers to generate samples for training and testing the model. It consists of 20 lexicons, with 45 repetitions for every word, 20 for training and 18 for testing. All signer’s hands are bare, and no wearable gloves are required. Twenty-five frames are captured per second with a resolution of 640 × 480.
Weather Dataset [ 40 ]: A continuous SL composed of three state-of-the-art datasets for the SL recognition purpose: RWTH-PHOENIX-Weather 2012, RWTH-PHOENIX-Weather 2014, and SIGNUM.
SIGNUM [ 27 ]: It is used for pattern recognition and SL recognition tasks. A video database is collected from daily life activities and sentences like going to the cinema, ride a bus, and so on. Signer’s gestures were captured by digital cameras.
CORPUS-NGT [ 24 ]: Great and huge efforts are done to collect and record videos of the SL of Netherlands (Nederlandes Gebarentaal: NGT), providing global access to this corpus for all researchers and sign language studies. About 100 native signers of different ages participated in collecting and recording signs for about 72 h. It provided annotation or translation of some of these signs.
RWTH German fingerspelling [ 21 ]: A German SL dataset is collected from 20 participants, producing about 1,400 image sequences. Each participant was asked to record every sign twice on different days by different cameras (one webcam and one camcorder) without any background limitations or restrictions on wearing clothes while gesturing. Dataset contains about 35 gestures with video sequences of alphabets and first 5 numbers (1–5).
RWTH-BOSTON-104: A dataset published by the national center of SL and gesture resources by Boston University. Four cameras were used to capture signs, three of them are white/black cameras and one is a color camera. Two white/black cameras in front of the signers to form stereo, another camera on the side of the signer, and the colored camera was focused between the stereo cameras. It consists of 201 annotated videos, about 161 videos are used for training and about 40 for testing. Captured movies of sentences consist of 30 fps [312 × 242] using only the upper center [195 × 165].
Oliveira et al. [ 28 ]: An Irish SL dataset captured human subjects using handshapes and movements, producing 468 videos. It is represented as two datasets which were employed for static (each sign language is transferred by a single frame) and dynamic (each sign is expressed by different frames and dimensions) Irish Sign Language recognition.
ISL-HS consists of 486 videos that captured 6 persons performing Irish SL with a rotating hand while signing each letter. Only arms and hands are considered in the frames. Also, videos whose background was removed by thresholding were provided. Further, 23 labels are considered, excluding j, x, and z letters, because they require hand motion which is out of the research area of the framework [ 41 ].
Camgoz et al. [ 29 ]: It presented the Turkish sign language. It was recorded using the state-of-the-art Microsoft Kinect v2 sensor. This dataset contains about 855 signs from everyday life domains from different fields such as finance and health. It has about 496 samples in health domain, about 171 samples in finance domain, and the remaining signs (about 181) are commonly used signs in everyday life. Each sign was captured by 10 users and was repeated 6 times, each user was asked to perform about 30–70 signs.
SMILE [ 30 ]: It prepared an assessment system for lexical signs of Swiss German Sign Language that relies on SLR. The aim of this assessment system is to give adult L2 (learners of the sign language) ofDSGS feedback on the correctness of the manual parameters such as hand position, shape, movement, and location. As an initial step, the system will have feedback for a subset of DSGS vocabulary production of 100 lexical words, to provide the SLR as a component of the assessment systems a huge dataset of 100 items was recorded with the aid of 11 adult L1 signers and 19 adult L2 learners of DSGS.
Most SLR techniques begin with extracting the upper body pose information, which is a challenge task due to the difference between signer and background color, another challenge is motion blur. To overcome all these challenges, they used a diverse set of visual sensors including high-speed and high-resolution GoPro video cameras, and a Microsoft Kinect V2 depth sensor.
Gebre et al. [ 11 ]: Dataset includes two languages British and Greek sign language which are available on Dicta-Sign corpus. The corpus has recordings for 4 sign languages with at least 14 signers per language and approximately 2 h using the same material across languages, from this selection, two languages BL and GL were selected. The priority of the signer’s selection was based on their skin’s color difference from background color. About 95% F1 score of accuracy was achieved.
Sahoo [ 32 ]: About 5,000 images of digital numbers (0,1,2…9) from 100 users (31 were female and 69 were male) were collected. Each signer was asked to repeat each character 5 times. Sony digital camera with resolution up to 16.1MP is used. Image format is JPEG with a resolution of 4,608 × 3,456 of the captured images. Image resolution was resized to 200 × 300. Finally, the dataset was divided into two groups for training and testing.
RKS-PERSIANSIGN [ 33 ] include a large dataset of 10 contributors with different backgrounds to produce 10,000 videos of Persian sign language (PSL), containing 100 videos for each PSL word, using the most commonly used words in daily communication of people.
Joze and Koller [ 34 ] proposed a large-scale dataset for understanding ASL including about 1,000 signs registered over 200 signers, comprising over 25,000 videos.
WASL [ 35 ] constructed a wide scale ASL dataset from authorized websites such as ASLU and ASL_LEX. Also, data were collected from YouTube based on clear titles that describe signs. About 21,083 videos were accessed from 20 different websites. Dataset was performed by 119 signers, producing only one video for every sign.
AUTSL [ 36 ] presented a new large-scale multi-modal dataset for Turkish Sign Language dataset (AUTSL). 226 signs were captured by 43 different signers, producing 38,336 isolated sign videos. Some samples of videos containing a wide variety of background recorded in indoor and outdoor environments.
KArSL [ 37 ], a comprehensive benchmark for ArSL containing 502 signs recorded by 3 different signers. Each sign was repeated 50 times by each signer, using Microsoft Kinect V2 for sign recording.
Daniel [ 38 ] used Raspberry pi with a thermal camera to produce 3,200 images with low resolution of 32 × 32 pixel. Each sign has 320 thermal images, so we conclude capturing images of about 10 signs.
Mittal et al. [ 39 ] created an ISL dataset recorded by six participants. The dataset contains 35 sign words, each word was repeated at least 15 times by each participant, so the size of the dataset is 3,150 (35 × 15 × 6).
3 Preprocessing steps
Two main preprocessing steps are required for different sign language processing tasks: segmentation and filtration. These tasks include the following subtasks: skin detection, handshape detection, feature extraction, image/video segmentation, gesture recognition, and so on. In this section, we shall briefly discuss all these subtasks. Figure 1 shows the sequence of different preprocessing steps that are almost required for different SL models. Each model usually starts with a signer’s image, applying color space conversion. Non-skin images are rejected, while other images continue the processing by applying image morphology (erosion and dilation) for noise reduction. Each image is validated by checking whether it has a hand or not. If yes, then the Region-of-Interest (ROI) is detected using hand mask images and segment fingers using defined algorithms. Image enhancement such as image filtering, data augmentation, and some other algorithms could be used to detect edges.

A flowchart that demonstrates the different image preprocessing steps.
Skin-detection: It is the process of separating the skin color from the non-skin color. Ref. [ 42 ] approved that it is not possible to provide a uniform method for detection and segmentation of human skin as it varies from one person to another. RGB is a widely used color mode, but it is not preferred in skin detection because of its chrominance and luminance and its non-uniform characteristics. Skin detection is applied on HSV (HUE, and Saturation Values) images and YCbCr.
ROI [ 42 ]: It is focused on detecting [ 43 ] hand gestures and extracting the most interesting points. The hand region is detected using skin-detection from the original image using some defined masks and filters as shown in Figure 2 .
![sign language detection research paper Figure 2
A proposed hand gesture recognition system using ROI [64].](https://www.degruyter.com/document/doi/10.1515/comp-2022-0240/asset/graphic/j_comp-2022-0240_fig_002.jpg)
A proposed hand gesture recognition system using ROI [ 64 ].
Image resize: It is the process of resizing images by either expanding or decreasing image size. Ref. [ 44 ] applied an interpolation algorithm that changes the image accuracy from one to another. Bicubic, a new pixel B (r^’, c^’) is formed by interpolating the nearest 4 × 4.
Ref. [ 45 ] proposed a promising skin-color detection algorithm, giving the best results even with complex backgrounds. Starting with acquiring an image from the video input stream, then adjusting image size, converting an image from RGB color space to YCbCr space (also denoting that YCbCr space is the most suitable one for skin color detection), and finally identifying color based on different values of threshold [ 46 , 47 ] and marking the detected skin with white color, otherwise with black color. Figure 1 includes a sub-flowchart that illustrates this algorithm.
In Ref. [ 48 ], binarization of images from RGB color mode to black and white color mode using Ostu’s algorithm of global thresholding was performed, images captured were then resized to 260 × 260 pixels for width and height and then Ostu’s method was used to convert the image. Ref. [ 49 ] applied new technique for feature extraction known as 7Hu moments invariant, which are used as a feature vector of algebraic functions. Their values are invariant because of the change in size, rotation, and translation. 7Hu moments were developed by Mark Hu in 1961. Structural shape descriptors [ 23 ] are proposed in five terms, aspect ratio, solidity, elongation, speediness, and orientation.
Ref. [ 50 ] used a face region skin detector which includes eyes and mouth which are non-skin non-smooth regions, which affect and decrease the accuracy.
A window of 10 × 10 around centered pixel of signer’s face is used to detected skin, but it is not accurate because in most cases it detects nose as it suffers from high illumination conditions [ 51 ].
Image segmentation: It refers to the extraction of hands from video frames or images. Either background technique or skin detector algorithm is applied first to detect skin of signer and then segmentation algorithm is applied [ 52 ]. Ref. [ 53 ] applied skin color segmentation using Artificial Neural Network (ANN), features extracted from left and right hands are used for neural network model with average recognition of 92.85%.
Feature extraction: It is the process of getting most important data-items or most interesting points of segmented image or gesture. Ref. [ 25 ] applied two techniques for feature extraction including window-based statistical feature and 2D discrete cosine transform (DCT) transformation. Ref. [ 48 ] applied five types of feature extraction including fingertip finder, elongatedness, eccentricity, pixel segmentation, and rotation. Figures 3 and 4 depict a promising accuracy in percentage of different feature extraction algorithms. Figure 3 illustrates the strength combining different three feature extraction algorithms: pixel segmentation, eccentricity, and elongatedness and fingertip and applying each one individually. Combined algorithms have largest accuracy with 99.5%.

Average recognition rate (%) for each feature extraction algorithm and percentage of combining them.

Comparison of different feature algorithms.
Tracking: Tracking body parts facilitate the SLR process. How important are accurate tracking of body parts and its movements? How accurate are its contribution to SLR? And how does the comparison and differences occur to just use the tracked image for feature extraction. Hand Tracking: hands of the signer convey most of the recognition of signs in most SL. Ref. [ 27 ] employed a free tracking system that is based on dynamic programming tracking (DPT). Tracking facial landmarks: introduced Active Appearance Models (AAMs) which was then reformulated by Matthews et al. [ 54 ].
4 Required devices
In the last decade, researchers depended on electronic devices to detect and recognize hand position and its gestures, because of many reasons [ 55 ]. One of them is SLR using signer independent or signer dependent. Signer dependent is the main core of any SLR system, as the signer performs both training and testing phases. So, this type affects the recognition rate positively. On the other side, signer independence is a challenging phase, as signers perform only the training phase, not admitted in the testing phase. This discarding is a challenge in adapting the system to accept another signer. The target of SL systems can be achieved by (I) image-based approach [ 56 ] or (II) glove-based approach based on sensors as shown in Figure 5 or (III) a new method for gesture recognition called virtual button [ 57 ].

Cyber-glove.
One of the disadvantages of data-gloves or electronic devices mainly are, data-gloves gives accurate information but with little information, the more advanced technology of sensors used, the more the cost, finally the data-gloves must be on-off-on each time of hand gesture recognition, which adds more obstacles with people who do not or are not aware of communication with this technology especially when they are in public places. Below is a short description of most used devices for SLR.
Tilt sensor: It is a device that produces an electrical signal that varies with an angular movement, used to measure slope and tilt with a limited range of motion.
Accelerometer sensor: It measures 3-axis acceleration caused by gravity and motion; in another word it is used to measure the rate of change of velocity.
Flex sensor: It is a very thin and lightweight electric device, used for the measurement of bending or deflection. Usually is stocked to the surface of fingers and the resistance of the sensor varied by bending the surface.
Motion (proximity) sensor: It is an electrical device which utilizes a sensor to capture motion, or it is used to detect the presence of objects without any physical contact.

Different sensor types attached to hand gloves.
As previously illustrated all these kinds of sensors used to measure the bend angles of fingers, the orientation or direction of the rest, abduction, and adduction between fingers. These sensors give an advantage over vison-based systems. Sensors can directly report with required data without any preprocessing steps for feature extraction (pending degree, orientation, etc.) in terms of voltage values to the system, but on the other side, vision-based systems require to apply tracking and feature extraction algorithms. But ref. [ 58 ] mentioned that using data-gloves and sensors do not provide the naturalness of HCI systems.
As a part of electronic devices which may have built-in sensors, there are two devices widely used in many fields, infra-red sensors such as Microsoft Kinect and Leap-Motion devices as shown in Figure 7 ( Table 2 ).

Digital devices with built-in sensors used to capture dynamic gestures of human expressions.
Most widely used electronic devices for hand gesture recognition
Author (year) | Devices | Techniques | Dataset | No. of hands | Accuracy (%) | ||
---|---|---|---|---|---|---|---|
Number | Alphabet | Word/Phrases | |||||
Hussain [ ], 1999 | Colored glove [red and blue] | ANN | — | ✓(ASL) | — | Two | 95.57 |
Oz and Leu [ ], 2011 | CyberGlove [18 sensors] | ANN | — | — | ✓(ASL) | Right hand | 98 |
Kadous [ ], 2014 | Glove [Flex sensors] | Matching | — | ✓(ASL) | — | Right hand | — |
Kadous [ ], 1996 | PowerGlove | IBL and DTL | ✓ | ✓ | ✓(AUSLAN) | — | 80 |
Tubaiz et al. [ ], 2015 | DG5-VHand data gloves | — | — | — | ✓ (ArSL) | — | 98.9% |
Daniel [ ], 2021 | Raspberry PI and Omron D6T thermal camera | CNN | ✓ | — | — | — | |
Mittal et al. [ ], 2019 | Leap motion sensor | LSTM | — | — | ✓(ISL) | Two | 72.3 (sentences) and 89.5% (words) |
Rosero-Montalvo et al. [ ], 2018 | Gloves with flex sensors | KNN | ✓ | — | — | Right hand | 85 |
Chen et al. [ ], 2020 | Myo armband | CNN | — | — | — | Right hand | 98.81 |
ANFIS: Adaptive Neuro-Fuzzy Inference system, MSL: Malaysian Sign Language, IBL: instance-based learning, DTL: decision-tree learning, ISL: Indian Sign Language.
Bold indicated the highest accuracy of using electronic devices for hand gesture recognition.
Vision-based approach : The great development in computer techniques and ML algorithms motivate many researchers to depend on vision-based methodology. A camera is used to capture images and then process to detect the most important features for recognition purposes. Most researchers prefer vision-based method because of its framework’s adaptability, the involvement of facial expression, body movements, and lips perusing. So, this approach required only a Camera to capture a person’s movements with a clear background without any gadgets. Previous gloves required an accompanying camera to register the gesture but does not work well in lightning conditions.
Virtual Button approach [ 57 ]: Depends on a virtual button generated by the system and receives hand’s motion and gesture by holding and discharging individually. This approach is not effective for recognizing SL because every sign language required utilization of all hand’s fingers and it also cannot be practical for real life communication.
4.1 Methodology and applied techniques
Many datasets were used in SL recognition, some of these datasets are based on the approaches of vision and some are based on the approach of soft computing like ANN, Fuzzy Logic, Genetic Algorithms, and others like Principal Component Analysis (PCA) and deep learning like Convolutional Neural Network (CNN).
Also, many algorithms and techniques were applied to recognize SLs and identify different languages with variance accuracy. Some of these techniques are classical algorithms and others are deep learning which has become the heading technique for most AI problems and overshadowing classical ML. A clear reason for depending on deep learning is that it had repeatedly demonstrated high quality results on a wide variety of tasks, especially those with big datasets.
Traditional ML algorithms are a set of algorithms that use training data to learn, then apply what they had learned to make informed decisions. Among traditional algorithms, there are classification and clustering algorithms used for SLR and SLID. Deep learning is considered as an evolution of ML, it uses programmable neural networks which make decisions without human intervention.
5 Methodology and applied techniques
K-Nearest Neighbors (KNN): It is one of the traditional ML algorithms used for classification and regression problems. Many researchers applied KNN on different SL datasets, but accuracy was lower than expected. Ref. [ 65 ] achieved results of 28.6% accuracy when applying KNN with PCA for dimensionality reduction. Other researchers merged some preprocessing steps for better accuracies. Although KNN indicate lower accuracy for image classification problems, some researchers recommended using KNN because of its ease of use, implementation, and fewer steps. Table 3 discusses some of the KNN algorithms applied on different datasets.
KNN classification comparison on different datasets
Author (year) | Technique | Gesture type | -Value | Accuracy (%) | Notes |
---|---|---|---|---|---|
Jadhav et al. [ ], 2017 | KNN | HGR | — | Template matching technique was applied with best recognition of hand's gestures, then used KNN for time reduction | |
Dewinta and Heryadi [ ], 2015 | KNN | ASL | 3 | 99.8 | KNN classifier used to detect and recognize ASL, giving promising accuracy with = 3 and lower accuracy with = 5, which is 28.6% |
Utaminingrum [ ], 2018 | KNN | ASL | 1 | 94:95 | Applied KNN with SMART technique used to improve weights and get best accuracy |
Tubaiz et al. [ ], 2015 | MKNN | ArSL | 3 | 98.9 | Recognize Arabic sign language based on two DG5-VHand data gloves electronic device. About 80-words lexicon were used to build up 40 sentences |
Patel [ ], 2017 | KNN | ISL | 1 | 82 | Used MATLAB functions to convert captured hand gestures into text and speech based on classification algorithms (PNN and KNN) to recognize ISL alphabets |
Saggio et al. [ ], 2010 | KNN | ISL | — | 96.6 | Proposed a wearable electronic device to recognize 10 gestures of Italian sign language. KNN and CNN were applied with accuracy of 96.6 and 98%, respectively |
CNN | 98 | ||||
Sahoo [ ], 2021 | KNN | ISL | 1 | 98.36 | Performed classification of a standard ISL dataset (containing 10 static digit numbers) using two different classifiers (KNN and Naïve Bayes). Accuracy produced by KNN is higher than Naïve Bayes |
Naïve Bayes | 97.79 | ||||
Saggio [ ], 2021 | KNN | — | — | 97.12 | Proposed a KNN classifier to analyze input video and extract the vocabulary of 20 gestures. Using a hand gesture dataset for training and testing, they got an overall accuracy of 97% |
Bold indicated the highest accuracy using KNN algorithm using different K-values.
Dewinta and Heryadi [ 65 ] classified ASL dataset using KNN classifier, varying the value of K = 3, 5, 7, 9, and 11. The highest accuracy was 99.8% using K = 3, while the worst accuracy was achieved by setting K = 5 using PCA for dimensionality reduction.
Fitri [ 66 ] proposed a framework using Simple Multi Attribute Rating Technique (SMART) weighting and KNN classifier. SMART was used to optimize and enhance accuracy of KNN classifier. The accuracy varied from 94 to 96% according to some lightening conditions. The accuracy decreases when lighting decreases and vice versa ( Figure 8 ).

Sign languages-based approaches.
According to Figure 9 it is clear that different algorithms preferred that K -values should not be static to its default value which equals “1”, but varying K -values to 1, 3, 5 or any odd number will result in good results. With K = 3, most researchers get the best accuracy.

Different KNN results based on change in K -values.
Jadhav et al. [ 67 ] proposed a framework based on KNN for recognizing sign languages. The unique importance of this framework allowed users to define their own sign language. In his system, users must store their signs first in database, after that he can use these signs while communicating with others. While communicating with another person using those stored signs, the opposite person can see the signs and its meaning. This framework suggested using real time sign recognition. The framework is based on three main steps:
Skin detection, he created a “skin detector” method for converting images from BGR format to HSV format. He used an interpolation algorithm for shadow detection from the images and fills it with continuous dots using “FillHoles” method. Another method called “ DetectAndRecognize ” takes the fill hole image as input for detection and recognition and calculates the contours which detect the edges of the signs. Title Blob detection – have two methods “FillHoles” and “DetectAndRecognize” methods.
Umang [ 68 ] applied KNN and PNN as a classification technique to recognize ISL alphabets. 7Hu moments were used for feature extraction. 82% is the approximate accuracy they achieved, using KNN built-in function in MATLAB with default K = 1.
Hidden Markov Model (HMM): Based on our review, HMM was one of the strongly recommended approaches for SL problems. Hybridization of HMM with CNN provided high accuracy with huge datasets. HMM is the most widely used technique for speech recognition and SL problems for both vision-based and data-gloves-based approach. Table 4 discusses some of the HMMs models applied on different datasets.
HMM comparison on different datasets
Author (year) | Technique | Gesture type | Accuracy (%) | Notes |
---|---|---|---|---|
Parcheta and Martínez-Hinarejos [ ], 2017 | HMM | SSL | 87.4 | Compared two results of using KNN + DTW which produced accuracy of 88.4% but recognition process speed was very high of 9,383 compared to HMM which was 519 to recognize Spanish sign language |
Starner et al. [ ], 1998 | HMM | ASL | 98 | Recognized ASL based on HMM using two techniques based on camera mounted on a desk with result of 98% accuracy, and the second used camera stuck to a user’s cap producing 92% accuracy |
Youssif et al. [ ], 2011 | HMM | ArSL | 82.22 | Used their own captured dataset from deaf people to represent 20 Arabic words. Applying HMM to recognize and detect each word with 6 models and different Gaussian mixtures. Average accuracy is 82.22% |
Roy et al. [ ], 2021 | HMM | ASL | 77.75 | Proposed HMM model to track hand motion in videos. Using camshift for hand tracking, accuracy of 77.75 % was achieved using 91 ASL hand gestures. Although this result is low, the author considered it very high as it contains more than double gestures with respect to existing approaches |
Ghanbari Azar and Seyedarabi [ ], 2019 | HMM | PeSL | 97.48 | Dataset of 1200 videos captured based on 20 dynamic signs using 12 participants. HMM was used as a classifier with Gaussian density function as for observations. An average accuracy of 97.48% was obtained using both signer dependent and independent |
Parcheta and Martínez-Hinarejos [ 71 ] used an optimized sensor called “leap motion” that we presented previously. This leap device was used to capture 3D information of hands gestures. He applied one of the two available types of HMM which is discrete and continuous. Continuous HMM was used for gesture recognition. Hidden Markov Model Toolkit ( HTK) was used to interact and interpret HMMs. He tried to recognize about 91 gestures collected using the aforementioned device by partitioning data into four parts, training HMM topologies through some defined models and producing accuracy of 87.4% for gesture recognition.
Starner [ 73 ] proposed a real-time HMM-based system to recognize sentences of ASL consisting of 40-word lexicon and capturing users’ gestures using cameras mounted to a desk, producing 92% accuracy. The second camera was mounted to a cap of the user, producing 98% accuracy. This paper proved that vision-based approach is more useful than glove-based approaches.
Ref. [ 34 ] provides systems based on HMM to recognize real-time ArSL. The system is a signer-independent, removing all barriers to communicate with deaf people. They built their own dataset to recognize 20 Arabic isolated words. They used 6 HMM models with different number of states and different Gaussian mixtures per state. The best accuracy was 82.2%.
Oliveira et al. [ 28 ] built a framework for static and dynamic sign language.
Hand Segmentation used OpenPose [ 73 ] detector trained on the dataset, getting high results for hand segmentation among all evaluated detectors (HandSegNet [ 54 ] and hand detector [ 74 ]). The right hand is detected by applying a forward feed neural network based on VGG-19, and the left image is detected by flipping the image and applying the previous steps again.
Static Signs consists of 2D convolution which contains the features, first layer tends to know more about the basic feature’s pixels like lines and corners. Each input frame is convolved with more than 32 filters to cover the network’s scope which is narrower at the beginning. The model is interested in fewer features.
Dynamic Sign Language Model: It is concerned with two key-points. First, it considered three dimensions of the layer for temporal dimension. It extends over the temporal dimension; this is useful in sign language recognition because it helps to model the local variations that describe the trajectory of gesture during its movement. Briefly, a dynamic model is implemented on a single frame followed by the gesture of each sequence.
For static results of ISL recognition, this paper achieves an accuracy of 0.9998 for cropped frames whereas it achieves an accuracy of 0.9979 for original frame, while for dynamic results, categorical accuracy is considered for each class. Classifier model was trained and tested on 8 classes, its accuracy was not high as it ranges between 0.66 and 0.76 for different streams.
Binyam Gebrekidan Gebre [ 11 ] proposed a method that gathers two methods of Stokoe’s and H-M model as they assumed that features extracted from frames are independent of each other, But Gebre assumes that sign’s features will be extracted from two frames. The next and previous one to get a hand or any movement. He Proposed an ideal SLID, the system subcomponents are: (1) skin detection, (2) feature extraction, (3) modeling, and (4) identification. For a modeling step, it used a random forest algorithm which generates many decision tree classifiers and aggregates their results. Extracted features include high performance, flexibility, and stability. He achieved about 95% F1 score of accuracy.
Invariant features [ 75 ] consists of three stages, namely, a training phase, a testing phase, and a recognition phase. The parameters of 7Hu invariant moment and structural shape descriptors which are created to form a new feature vector to recognize the sign are combined and then MSVM is applies for training the recognized signs of ISL. The effectiveness of the proposed method is validated on a dataset of 720 images with a recognition rate of 96%.
CNN [ 76 ] Kang et al. used CNN, specifically caffe implementation network (CaffeNet), consisting of 5 convolution layers, 3 max-pooling layers, and 3 fully connected layers.
FFANN [ 17 , 77 ] was used in ref. [ 48 ] achieving an average accuracy of 94.32% using convex hull eccentricity, elongatedness, pixel segmentation, and rotation for American number, and alphabets recognition of about 37 signs, whereas ref. [ 49 ] applied FFANN on facial and hand gestures of 11 signs, with an average accuracy of 93.6% depending on automatic gesture area segmentation and orientation normalization. Ref. [ 78 ] also used FFANN for Bengali alphabet with 46 signs achieving an accuracy of 88.69% for testing result depending on Fingertip finder algorithm with multilayered feedforward, back propagation training.
Effective ML algorithms were used to achieve high accuracy, but deep learning algorithms indicate more accurate results. Deep learning types vary between unsupervised pre-trained networks, CNN, recurrent neural network, and recursive neural network which encourage more people to do more research, share, and compare their results. We will compare between types of deep learning algorithms and used parameters, to determine which activation function is the best? How to test and train the model?
Ref. [ 44 ] applied two CNN models on 24 letters of ASL with 10 images per letter, image size is 227 × 227 which is resized using the Bicubic interpolation method. The images were trained using 4 CNNs with 20 layers in each CNN. Each model had a different activation function and a different optimization algorithm. PReLU and ReLU were used in model 1 and model 2, respectively. Accuracy for model 1 is 99.3% as it was able to recognize all 24 letters, but the accuracy of model 2 was 83.33% as it recognizes only 20 letters of all the 24 letters.
Ref. [ 81 ] used deep learning algorithms to identify SL using three publicly available datasets. Also introduced a new public large-scale dataset for Greek sign language RGB + D, providing two CTC variations that were mostly used in other application fields EnCTC and StimCTC. Each frame was resized from 256 × 256 to 224 × 224. The models are trained using Adam optimizer, and initial learning rate of 0.0001 was reduced to 0.00001.
Ref. [ 82 ] proposed a deep learning model consisting of CNN (inception model) and RNN to capture images of ASL, this dataset consists of 2,400 images, divided into 1,800 images for training and the remaining for testing. CNN extracts feature from the frames, using two major approaches for classification as SoftMax layer and the pool layer. After retraining the model using the inception model, the extracted features were passed to RNN using LSTM Model.
Ref. [ 87 ] studied the effect of data-augmentation on deep learning algorithms, achieving an accuracy of 97.12% which is higher than that of the model before applying data augmentation by about 4%. Dataset consists of 10 static gestures to recognize, each class has 800 images for training and 160 for testing, resulting in 8,000 images for training and 1,600 for testing. This algorithm overcomes both SVM and KNN as shown in Figure 10 , while being applied on the same dataset ( Tables 5 and 6 ).

Traditional and deep learning algorithm results applied on the same dataset.
Comparison of different machine learning algorithms based on different datasets
Author (year) | Technique | Gesture type | Accuracy (%) | Notes |
---|---|---|---|---|
Tu et al. [ ], 2013 | FFANN | HGR and face recognition | 93.6 | Automatic gesture segmentation and orientation normalization of hand were used |
Islam et al. [ ], 2017 | FFANN | ASL and numeric numbers | 94.32 | real time recognition system using of 37 signs of numeric and alphabetic American characters |
Dixit and Jalal [ ], 2013 | MSVM | ISL | Recognition of Indian sign language using 7Hu invariant moment and structural face descriptors, combining them to for new feature for sign recognition | |
Kang [ ], 2015 | CNN | Finger spelling | — | Real time sign language finger spelling recognition system using CNN |
Utaminingrum [ ], 2019 | KNN | Alphabets | 96 | KNN classifier with = 1 applied to a dataset of captured alphabets which were preprocessed to enhance images and detect hands using skin color algorithms, producing different accuracies of 94, 95, and 96% for dark, normal, and light images, respectively |
Kamruzzaman [ ], 2020 | CNN | ArSL | 90 | Used CNN as a deep learning model to classify ArSL alphabets of 31 letters. Also, the proposed model produces a speech for the recognized letter |
Varsha and Nair [ ], 2021 | CNN | ISL | 93 | Applied CNN model (inception model V3) on ISL, which receives its input as an image, achieving an average accuracy of 93% |
Comparison of deep learning of different sign language datasets focusing on technical parameters such as activation and optimization function, learning rate, and so on
Author | Deep learning algorithm | Dataset | Activation function | Learning rate (LR) | Epochs | Optimization function | Loss error | Accuracy (%) |
---|---|---|---|---|---|---|---|---|
Raheem and Abdulwahhab [ ] | CNN | 240 images of ASL | PReLU | 0.01 | 100 | SGDM | 0.3274 | 99.3 |
ReLU | 0.01 | 100 | RMSProp | 0.485 | 83.33 | |||
Adaloglou et al. [ ] | GoogLeNet + TConvs | — | — | 0.0001:0.00001 | 10:25 | ADAM optimizer | — | — |
Bantupalli and Xie [ ] | CNN + LSTM | 2,400 images of ASL | SoftMax and pool layer | — | 10 | ADAM optimizer | 0.3 | 91 |
Islam et al. [ ] | CNN | — | ReLU & pool layer | 0.001 | 60 | SGD optimizer | — | 97.12 |
Neethu et al. [ ] | CNN | 1,600 images | Average and max pool layer | — | — | — | — | 96.2 |
Tolentino et al. [ ] | CNN | ASL letters and [1–10] digits | SoftMax and max pool layer | 0.01 | 50 | SGD optimizer | — | 93.67 |
Wangchuk et al. [ ] | CNN | 20,000 images of BSL digits [0–9] | VGGNet [ReLU & max pool] | — | 34 | ADAM optimizer | 0.0021 | 97.62 |
Tolentino et al. [ ] | CNN | 35,000 images of ISL | ReLU | — | 100 | ADAM optimizer | — | |
Ferreira et al. [ ] | CNN | 1,400 images of ASL | SoftMax | 0.001 | — | SGD optimizer | — | 93.17 |
Elboushaki et al. [ ] | MultiD-CNN | isoGD | ReLU | 0.001 | — | ADAM optimizer | — | 72.53 |
SKIG | ||||||||
NATOPS | 95.87 | |||||||
SBU | 97.51 | |||||||
Kopuklu et al. [ ] | CNN | EgoGesture | Average pooling and SoftMax | 0.001 | — | SGD optimizer | — | 94.03 |
Yuxiao et al. [ ] | DG-STA | DHG-14/28 | Softmax | 0.001 | — | ADAM optimizer | — | 91.9 |
Breland et al. [ ] | CNN | Thermal dataset | ReLU | 0.005 | 20 | ADAM optimizer | — |
ISL: Indian Sign Language.
Bold indicates highest results of applying different CNN models on various SL datasets.
Ref. [ 43 ] uses CNN for hand gesture classification. First, the author used the algorithm of connected components analysis to select and segment hands from the image dataset using masks and filters, finger cropping, and segmentation. The author also used Adaptive Histogram equalization (AHE) for image enhancement to improve image contrast. CNN algorithm’s accuracy was 96.2% which is higher than SVM classification algorithm applied by the author to achieve an accuracy of 93.5%. The following table illustrates this difference. Also, recognition time using CNN (0.356 s) is lower than SVM (0.647 s). As shown in Table 7 , CNN exceeds SVM in different measurements like sensitivity, specificity, and accuracy.
Distinction between CNN and SVM on different measurements
Performance analysis parameters (%) | CNN/SVM classification approach | Connected component analysis? CNN/SVM classification approach | AHE? connected component analysis? CNN/SVM classification approach |
---|---|---|---|
Sensitivity | 91.5/89.1 | 96.8/90.5 | 98.1/92.1 |
Specificity | 82.7/78.7 | 89.2/87.5 | 93.4/89.9 |
Accuracy | 91.6/87.5 | 94.8/91.6 | 96.2/93.5 |
Recognition rate | 90.7/88.2 | 96.2/90.5 | 98.7/91.5 |
Ref. [ 88 ] implemented training and testing using CNN by Keras and TensorFlow using SGD algorithm as its optimizer, having a learning rate of 0.01. The number of epochs is equal to 50 with a batch size of 500. Dataset has a set of static signs of letters, digits, and some words then resize of words to 50 × 50. Each class contains 1,200 images. The overall average accuracy of the system was 93.67%, of which 90.04, 93.44, and 97.52% for ASL alphabets, number recognition, and static word recognition, respectively. Tests were applied on 6 persons who were signer’s interpreters and 24 students without any knowledge of using sign language ( Figure 11 ).

Performance measure before and after applying data augmentation.
Ref. [ 89 ] applied CNN algorithm on Bhutanese Sign Language digits recognition, collected dataset of 20,000 images of digits [0–9] from 21 students, each student was asked to capture 10 images per class. Images and videos were captured from different angles, directions, different backgrounds, and lighting conditions. Images were scaled to 64 × 64. TensorFlow was used as a deep learning library. Comparison with traditional ML was done and approved the superiority of deep learning CNN to SVM and KNN algorithms with average accuracy of 97.62% for CNN, 78.95% for KNN, and 70.25% for SVM, with lower testing time for CNN ( Figure 11 ).
Ref. [ 90 ] applied CNN algorithm on ISL dataset which consists of distinct 100 images, generating 35,000 images of both colored and grayscale image types. The dataset includes digits [0–10] and 23 alphabets and about 67 most common words. Original image size of 126 × 126 × 16 was reduced to 63 × 63 × 16 using kernel filter of size 2. Many optimizers were applied such as ADAM, SGD, Adagrad, AdaDelta, RMSprop, and SGD. Using ADAM optimizer he achieved the best result of 99.17% and 98.8% for training and validation, respectively. Also, the proposed model accuracy exceeds other classifiers such as KNN (95.95%), SVM (97.9%), and ANN (98%).
6 Conclusion and future work
The variety of sign language datasets, which includes different gestures, leads to different accuracies as we had discussed based on review of previous literature. This survey showed that different datasets have been used in the training and testing of SLR systems. It compared between vison-based approach and glove-based approach, showed the advantages and the disadvantages of both, illustrated the difference between signer dependent and signer independent, and addressed the basic preprocessing steps such as skin detector, image segmentation, hand tracking, feature extraction, and hand’s gesture classification.
The survey also compares some ML techniques with the most used deep learning algorithm (CNN), showing that deep learning results exceed traditional ML. Some glove-based systems outperform deep learning algorithms due to the accurate signals that researchers get while feature extraction, while using deep learning their features get during model training which is not accurate as the gloves-based systems. According to this previous issue, we need to get rid of any obstacles (gloves, sensors, and leap devices) or any electronic device that may restrict user interaction with the system. Many trials had been done but with less accuracy.
Few researchers are working to solve SLID, although it is important for having a comprehensive SLR system. Including ArSL in our future work will be a challenging task. Also, trying to wear-off any gloves or any electric based systems will give user more comfort while communicating with others.
Conflict of interest: Authors state no conflict of interest.
Data availability statement: Data sharing is not applicable to this article as no new data were created or analyzed in this study.
[1] R. Kushalnagar, “Deafness and Hearing Loss,” Web Accessibility. Human–Computer Interaction Series, Y. Yesilada, S. Harper, eds, London, Springer, 2019. 10.1007/978-1-4471-7440-0_3 Search in Google Scholar
[2] World Federation of the Deaf. Our Work, 2018. http://wfdeaf.org/our-work/Accessed 2019–03–26. Search in Google Scholar
[3] S. Wilcox and J. Peyton, “American Sign Language as a foreign language,” CAL. Dig., pp. 159–160, 1999. Search in Google Scholar
[4] M. del Carmen Cabeza-Pereiro, J. M. Garcia-Miguel, C. G. Mateo, and J. L. A. Castro, “CORILSE: a Spanish sign language repository for linguistic analysis,” Proceedings of the Tenth International Conference on Language Resources and Evaluation (LREC'16), 2016, May, pp. 1402–1407. Search in Google Scholar
[5] T. Johnston and A. Schembri, Australian Sign Language (Auslan): An Introduction to Sign Language Linguistics, Cambridge, UK, Cambridge University Press, 2007. ISBN 9780521540568. 10.1017/CBO9780511607479 . Search in Google Scholar
[6] M. Abdel-Fattah, “Arabic Sign Language: A Perspective,” J. Deaf. Stud. Deaf. Educ., vol. 10, no. 2, 2005, pp. 212–221. 10. 212-21. 10.1093/deafed/eni007. Search in Google Scholar
[7] J. V. Van Cleve, Gallaudet Encyclopedia of Deaf People and Deafness, Vol 3, New York, New York, McGraw-Hill Company, Inc., 1987, pp. 344–346. Search in Google Scholar
[8] D. Cokely, Charlotte Baker-Shenk, American Sign Language, Washington, Gallaudet University Press, 1981. Search in Google Scholar
[9] U. Shrawankar and S. Dixit, Framing Sentences from Sign Language Symbols using NLP, In IEEE conference, 2016, pp. 5260–5262. Search in Google Scholar
[10] N. El-Bendary, H. M. Zawbaa, M. S. Daoud, A. E. Hassanien, K. Nakamatsu, “ArSLAT: Arabic Sign Language Alphabets Translator,” 2010 International Conference on Computer Information Systems and Industrial Management Applications (CISIM), Krackow, 2010, pp. 590–595. 10.1109/CISIM.2010.5643519 Search in Google Scholar
[11] B. G. Gebre, P. Wittenburg, and T. Heskes, “Automatic sign language identification,” 2013 IEEE International Conference on Image Processing, Melbourne, VIC, 2013, pp. 2626–2630. 10.1109/ICIP.2013.6738541 Search in Google Scholar
[12] D. Bragg, O. Koller, M. Bellard, L. Berke, P. Boudreault, A. Braffort, et al., “Sign language recognition, generation, and translation: an interdisciplinary perspective,” The 21st International ACM SIGACCESS Conference on Computers and Accessibility (ASSETS ’19), New York, NY, USA, Association for Computing Machinery, 2019, pp. 16–31. 10.1145/3308561.3353774 Search in Google Scholar
[13] R. Rastgoo, K. Kiani, and S. Escalera, “Sign language recognition: A deep survey,” Expert. Syst. Appl., vol. 164, 113794, 2020. 10.1016/j.eswa.2020.113794 Search in Google Scholar
[14] A. Sahoo, G. Mishra, and K. Ravulakollu, “Sign language recognition: State of the art,” ARPN J. Eng. Appl. Sci., vol. 9, pp. 116–134, 2014. Search in Google Scholar
[15] A. Karpov, I. Kipyatkova, and M. Železný, “Automatic technologies for processing spoken sign languages,” Proc. Computer Sci., vol. 81, pp. 201–207, 2016. 10.1016/j.procs.2016.04.050 . Search in Google Scholar
[16] F. Chou and Y. Su, “An encoding and identification approach for the static sign language recognition,” 2012 IEEE/ASME International Conference on Advanced Intelligent Mechatronics (AIM), Kachsiung, 2012, pp. 885–889. 10.1109/AIM.2012.6266025 Search in Google Scholar
[17] https://en.wikipedia.org/wiki/Feedforward_neural_network. Search in Google Scholar
[18] https://www.deafwebsites.com/sign-language/sign-language-other-cultures.html. Search in Google Scholar
[19] D. Santiago, I. Benderitter, and C. García-Mateo, Experimental Framework Design for Sign Language Automatic Recognition, 2018, pp. 72–76. 10.21437/IberSPEECH.2018-16. Search in Google Scholar
[20] Z. Zafrulla, H. Brashear, P. Yin, P. Presti, T. Starner, and H. Hamilton, “American sign language phrase verification in an educational game for deaf children,” IEEE, pp. 3846–3849, 2010, 10.1109/ICPR.2010.937 . Search in Google Scholar
[21] K. B. Shaik, P. Ganesan, V. Kalist, B. S. Sathish, and J. M. M. Jenitha, “Comparative study of skin color detection and segmentation in HSV and YCbCr color space,” Proc. Computer Sci., vol. 57, pp. 41–48, 2015. 10.1016/j.procs.2015.07.362 . Search in Google Scholar
[22] P. Dreuw, D. Rybach, T. Deselaers, M. Zahedi, and H. Ney, “Speech Recognition Techniques for a Sign Language Recognition System,” ICSLP, Antwerp, Belgium, August. Best Paper Award, 2007a. 10.21437/Interspeech.2007-668 Search in Google Scholar
[23] K. Dixit and A. S. Jalal, “Automatic Indian Sign Language recognition system,” 2013 3rd IEEE International Advance Computing Conference (IACC), Ghaziabad, 2013, pp. 883–887. 10.1109/IAdCC.2013.6514343 . Search in Google Scholar
[24] I. Z. Onno Crasborn and J. Ros, “Corpus-NGT. An open access digital corpus of movies with annotations of Sign Language of the Netherlands,” Technical Report, Centre for Language Studies, Radboud University Nijmegen, 2008. http://www.corpusngt.nl. Search in Google Scholar
[25] M. Hassan, K. Assaleh, and T. Shanableh, “Multiple proposals for continuous arabic sign language recognition,” Sensing Imaging, vol. 20, no. 1. pp. 1–23, 2019. 10.1007/s11220-019-0225-3 Search in Google Scholar
[26] A. Youssif, A. Aboutabl, and H. Ali, “Arabic sign language (ArSL) recognition system using HMM,” Int. J. Adv. Computer Sci. Appl., vol. 2, 2011. 10.14569/IJACSA.2011.021108 . Search in Google Scholar
[27] O. Koller, J. Forster, and H. Ney, “Continuous sign language recognition: Towards large vocabulary statistical recognition systems handling multiple signers,” Computer Vis. Image Underst., vol. 141, pp. 108–125, 2015. 10.1016/j.cviu.2015.09.013 . Search in Google Scholar
[28] M. Oliveira, H. Chatbri, Y. Ferstl, M. Farouk, S. Little, N. OConnor, et al., “A dataset for Irish sign language recognition,” Proceedings of the Irish Machine Vision and Image Processing Conference (IMVIP), vol. 8, 2017. Search in Google Scholar
[29] N. C. Camgoz, A. A. Kindiroğlu, S. Karabüklü, M. Kelepir, A. S. Ozsoy, and L. Akarun, BosphorusSign: a Turkish sign language recognition corpus in health and finance domains. In Proceedings of the Tenth International Conference on Language Resources and Evaluation (LREC’16), 2016, pp. 1383–1388. Search in Google Scholar
[30] S. Ebling, N. C. Camgöz, P. B. Braem, K. Tissi, S. Sidler-Miserez, S. Stoll, and M. Magimai-Doss, “SMILE Swiss German sign language dataset,” Proceedings of the 11th International Conference on Language Resources and Evaluation (LREC) 2018, University of Surrey, 2018. Search in Google Scholar
[31] N. M. Adaloglou, T. Chatzis, I. Papastratis, A. Stergioulas, G. T. Papadopoulos, V. Zacharopoulou, and P. Daras none, “A comprehensive study on deep learning-based methods for sign language recognition,” IEEE Trans. Multimedia, pp. 1, 2021. 10.1109/tmm.2021.3070438 . Search in Google Scholar
[32] A. Sahoo, “Indian sign language recognition using neural networks and kNN classifiers,” J. Eng. Appl. Sci., vol. 9, pp. 1255–1259, 2014. Search in Google Scholar
[33] R. Rastgoo, K. Kiani, and S. Escalera, “Hand sign language recognition using multi-view hand skeleton,” Expert. Syst. Appl., vol. 150, p. 113336, 2020a. 10.1016/j.eswa.2020.113336 Search in Google Scholar
[34] H. R. V. Joze and O. Koller, “MS-ASL: A large-scale dataset and benchmark for understanding American sign language. arXiv preprint arXiv:1812.01053,” arXiv 2018, arXiv:1812.01053. Search in Google Scholar
[35] D. Li, C. Rodriguez, X. Yu, and H. Li, “Word-level deep sign language recognition from video: A new large-scale dataset and methods comparison,” Proceedings of the IEEE/CVF Winter Conference on Applications of Computer Vision, Snowmass Village, CO, USA, 1–5 March 2020, pp. 1459–1469. 10.1109/WACV45572.2020.9093512 Search in Google Scholar
[36] O. M. Sincan and H. Y. Keles, “AUTSL: A large-scale multi-modal Turkish sign language dataset and baseline methods,” IEEE Access, vol. 8, pp. 181340–181355, 2020. 10.1109/ACCESS.2020.3028072 Search in Google Scholar
[37] A. A. I. Sidig, H. Luqman, S. Mahmoud, and M. Mohandes, “KArSL: Arabic sign language database,” ACM Trans. Asian Low-Resour. Lang. Inf. Process, vol. 20, pp. 1–19, 2021. 10.1145/3423420 Search in Google Scholar
[38] D. S. Breland, S. B. Skriubakken, A. Dayal, A. Jha, P. K. Yalavarthy, and L. R. Cenkeramaddi, “Deep learning-based sign language digits recognition from thermal images with edge computing system,” IEEE Sens. J., vol. 21, no. 9. pp. 10445–10453, 2021. 10.1109/JSEN.2021.3061608 Search in Google Scholar
[39] A. Mittal, P. Kumar, P. P. Roy, R. Balasubramanian, and B. B. Chaudhuri, “A modified LSTM model for continuous sign language recognition using leap motion,” IEEE Sens. J., vol. 19, no. 16. pp. 7056–7063, 2019. 10.1109/jsen.2019.2909837 . Search in Google Scholar
[40] O. Koller, S. Zargaran, H. Ney, and R. Bowden, “Deep sign: enabling robust statistical continuous sign language recognition via hybrid CNN-HMMs,” Int. J. Comput. Vis., vol. 126, pp. 1311–1325, 2018. 10.1007/s11263-018-1121-3 Search in Google Scholar
[41] I. Hernández, Automatic Irish sign language recognition, Trinity College, Diss. Thesis of Master of Science in Computer Science (Augmented and Virtual Reality), University of Dublin, 2018. Search in Google Scholar
[42] P. S. Neethu, R. Suguna, and D. Sathish, “An efficient method for human hand gesture detection and recognition using deep learning convolutional neural networks,” Soft Comput., vol. 24, pp. 15239–15248, 2020. 10.1007/s00500-020-04860-5 . Search in Google Scholar
[43] C. D. D. Monteiro, C. M. Mathew, R. Gutierrez-Osuna, F. Shipman, Detecting and identifying sign languages through visual features, 2016 IEEE International Symposium on Multimedia (ISM), 2016. 10.1109/ism.2016.0063 . Search in Google Scholar
[44] F. Raheem and A. A. Abdulwahhab, “Deep learning convolution neural networks analysis and comparative study for static alphabet ASL hand gesture recognition,” Xi'an Dianzi Keji Daxue Xuebao/J. Xidian Univ., vol. 14, pp. 1871–1881, 2020. 10.37896/jxu14.4/212 . Search in Google Scholar
[45] A. Kumar and S. Malhotra, Real-Time Human Skin Color Detection Algorithm Using Skin Color Map, 2015. Search in Google Scholar
[46] Y. R. Wang, W. H. Li and L. Yang, “A Novel real time hand detection based on skin color,” 17th IEEE International Symposium on Consumer Electronics (ISCE), 2013, pp. 141–142. 10.1109/ISCE.2013.6570151 Search in Google Scholar
[47] K. Sheth, N. Gadgil, and P. R. Futane, “A Hybrid hand detection algorithm for human computer interaction using skin color and motion cues,” Inter. J. Computer Appl., vol. 84, no. 2. pp. 14–18, December 2013. 10.5120/14548-2636 Search in Google Scholar
[48] M. M. Islam, S. Siddiqua, and J. Afnan, “Real time hand gesture recognition using different algorithms based on American sign language,” 2017 IEEE International Conference on Imaging, Vision & Pattern Recognition (icIVPR), 2017. 10.1109/icivpr.2017.7890854 . Search in Google Scholar
[49] Y.-J. Tu, C.-C. Kao, and H.-Y. Lin, “Human computer interaction using face and gesture recognition,” 2013 Asia-Pacific Signal and Information Processing Association Annual Summit and Conference, 2013. 10.1109/apsipa.2013.6694276 . Search in Google Scholar
[50] M. Kawulok, “Dynamic skin detection in color images for sign language recognition,” Image Signal. Process, vol. 5099, pp. 112–119, 2008. 10.1007/978-3-540-69905-7_13 Search in Google Scholar
[51] S. Bilal, R. Akmeliawati, M. J. E. Salami, and A. A. Shafie, “Dynamic approach for real-time skin detection,” J. Real-Time Image Proc., vol. 10, no. 2. pp. 371–385, 2015. 10.1007/s11554-012-0305-2 Search in Google Scholar
[52] N. Ibrahim, H. Zayed, and M. Selim, “An automatic arabic sign language recognition system (ArSLRS),” J. King Saud. Univ. – Computer Inf. Sci., Vol. 30, no. 4, October 2018, Pages 470–477. 10.1016/j.jksuci.2017.09.007 . Search in Google Scholar
[53] M. P. Paulraj, S. Yaacob, Z. Azalan, M. Shuhanaz, and R. Palaniappan, A Phoneme-based Sign Language Recognition System Using Skin Color Segmentation, 2010, pp. 1–5. 10.1109/CSPA.2010.5545253 . Search in Google Scholar
[54] T. Simon, H. Joo, I. Matthews, and Y. Sheikh, “Hand Keypoint Detection in Single Images Using Multiview Bootstrapping” 2017 IEEE Conference on Computer Vision and Pattern Recognition (CVPR), 2017, pp. 4645–4653. doi: 10.1109/CVPR.2017.494. 10.1109/CVPR.2017.494 Search in Google Scholar
[55] R. Akmeliawati, “Real-time Malaysian sign language translation using colour segmentation and neural network”, Proc. of the IEEE International Conference on Instrumentation and Measurement Technology 2007, Warsaw, 2007, pp. 1–6. 10.1109/IMTC.2007.379311 Search in Google Scholar
[56] J. Lim, D. Lee, and B. Kim, “Recognizing hand gesture using wrist shapes,” 2010 Digest of Technical Papers of the International Conference on Consumer Electronics (ICCE), Las Vegas, 2010, pp. 197–198. Search in Google Scholar
[57] O.Al-Jarrah and A. Halawani, “Recognition of gestures in Arabic sign language using neuro-fuzzy systems,” Artif. Intell., vol. 133, pp. 117–138, 2001. 10.1016/S0004-3702(01)00141-2 . Search in Google Scholar
[58] M. A. Hussain, Automatic recognition of sign language gestures, Master’s Thesis. Jordan University of Science and Technology, Irbid, 1999. Search in Google Scholar
[59] C. Oz and M. C. Leu, “American sign language word recognition with a sensory glove using artifcial neural networks,” Eng. Appl. Artifcial Intell., vol. 24, no. 7. pp. 1204–1213, Oct. 2011. Search in Google Scholar
[60] M. W. Kadous, “Machine recognition of Auslan signs using PowerGloves: Towards large-lexicon recognition of sign language,” Proceedings of the Workshop on the Integration of Gesture in Language and Speech, Wilmington, DE, USA, 1996, pp. 165–174. Search in Google Scholar
[61] N. Tubaiz, T. Shanableh, and K. Assaleh, “Glove-based continuous Arabic sign language recognition in user-dependent mode,” IEEE Trans. Human-Mach. Syst., vol. 45, no. 4. pp. 526–533, 2015. 10.1109/THMS.2015.2406692 Search in Google Scholar
[62] P. D. Rosero-Montalvo, P. Godoy-Trujillo, E. Flores-Bosmediano, J. Carrascal-Garcia, S. Otero-Potosi, H. Benitez-Pereira, et al., “Sign language recognition based on intelligent glove using machine learning techniques,” 2018 IEEE Third Ecuador Technical Chapters Meeting (ETCM), 2018. 10.1109/etcm.2018.8580268 . Search in Google Scholar
[63] L. Chen, J. Fu, Y. Wu, H. Li, and B. Zheng, “Hand gesture recognition using compact CNN via surface electromyography signals,” Sensors, vol. 20, no. 3. p. 672, 2020. 10.3390/s20030672 . Search in Google Scholar PubMed PubMed Central
[64] D. Aryanie and Y. Heryadi, “American sign language-based finger-spelling recognition using k-Nearest Neighbors classifier.” 2015 3rd International Conference on Information and Communication Technology (ICoICT), 2015, pp. 533–536. 10.1109/ICoICT.2015.7231481 Search in Google Scholar
[65] F. Utaminingrum, I. Komang Somawirata, and G. D. Naviri, “Alphabet sign language recognition using K-nearest neighbor optimization,” JCP, vol. 14, no. 1. pp. 63–70, 2019. 10.17706/jcp.14.1.63-70 Search in Google Scholar
[66] A. Jadhav, G. Tatkar, G. Hanwate, and R. Patwardhan, “Sign language recognition,” Int. J. Adv. Res. Comput. Sci. Softw. Eng., vol. 7, pp. 109–115, no. 3, 2017. 10.23956/ijarcsse/V7I3/0127 Search in Google Scholar
[67] U. Patel and A. G. Ambekar, "Moment Based Sign Language Recognition for Indian Languages," 2017 International Conference on Computing, Communication, Control and Automation (ICCUBEA), 2017, pp. 1–6. 10.1109/ICCUBEA.2017.8463901 . Search in Google Scholar
[68] G. Saggio, P. Cavallo, M. Ricci, V. Errico, J. Zea, and M. E. Benalcázar, “Sign language recognition using wearable electronics: implementing k-Nearest Neighbors with dynamic time warping and convolutional neural network algorithms,” Sensors, vol. 20, no. 14. p. 3879, 2020. 10.3390/s20143879 . Search in Google Scholar PubMed PubMed Central
[69] A. K. Sahoo, “Indian sign language recognition using machine learning techniques,” Macromol. Symp., vol. 397, no. 1. p. 2000241, 2021. 10.1002/masy.202000241 . Search in Google Scholar
[70] Z. Parcheta and C.-D. Martínez-Hinarejos, “Sign language gesture recognition using HMM,” in Pattern Recognition and Image Analysis. Lecture Notes in Computer Science 2017. L. Alexandre, J. Salvador Sánchez, J. Rodrigues, (Eds), IbPRIA, vol. 10255, Cham: Springer, pp. 419–426, 2017. 10.1007/978-3-319-58838-4_46 . Search in Google Scholar
[71] T. Starner, J. Weaver, and A. Pentland, “Real-time American sign language recognition using desk and wearable computer-based video,” IEEE Trans. Pattern Anal. Mach. Intellig., vol. 20, no. 12. pp. 1371–1375, 1998. 10.1109/34.735811 Search in Google Scholar
[72] C. Zimmermann and T. Brox, “Learning to estimate 3D hand pose from single RGB images,” 2017 IEEE International Conference on Computer Vision (ICCV), 2017, pp. 4913–4921. 10.1109/ICCV.2017.525 Search in Google Scholar
[73] D. Victor, Real-Time Hand Tracking Using SSD on TensorFlow, GitHub Repository, 2017. Search in Google Scholar
[74] K. Dixit and A. S. Jalal, “Automatic Indian sign language recognition system,” 2013 3rd IEEE International Advance Computing Conference (IACC), 2013. 10.1109/iadcc.2013.6514343 . Search in Google Scholar
[75] B. Kang, S. Tripathi, and T. Nguyen, “Real-time sign language fingerspelling recognition using convolutional neural networks from depth map,” 3rd IAPR Asian Conference on Pattern Recognition, Kuala Lumpur, Malaysia, 2015. 10.1109/acpr.2015.7486481 . Search in Google Scholar
[76] https://en.wikipedia.org/wiki/Backpropagation. Search in Google Scholar
[77] A. M. Jarman, S. Arshad, N. Alam, and M. J. Islam, “An automated bengali sign language recognition system based on fingertip finder algorithm,” Int. J. Electron. Inform., vol. 4, no. 1. pp. 1–10, 2015. Search in Google Scholar
[78] P. P. Roy, P. Kumar, and B. -G. Kim, “An efficient sign language recognition (SLR) system using camshift tracker and hidden markov model (HMM),” SN Computer Sci., vol. 2, 79, no. 2, 2021. 10.1007/s42979-021-00485-z . Search in Google Scholar
[79] S. Ghanbari Azar and H. Seyedarabi, “Trajectory-based recognition of dynamic persian sign language using hidden Markov Model,” arXiv e-prints, p. arXiv-1912, 2019. Search in Google Scholar
[80] N. M. Adaloglou, T. Chatzis, I. Papastratis, A. Stergioulas, G. T. Papadopoulos, V. Zacharopoulou, and P. Daras, “A Comprehensive Study on Deep Learning-based Methods for Sign Language Recognition,” IEEE Transactions on Multimedia, p. 1, 2021. 10.1109/tmm.2021.3070438 . Search in Google Scholar
[81] K. Bantupalli and Y. Xie, “American sign language recognition using deep learning and computer vision,” 2018 IEEE International Conference on Big Data (Big Data), Seattle, WA, USA, 2018, pp. 4896–4899. 10.1109/BigData.2018.8622141 . Search in Google Scholar
[82] F. Utaminingrum, I. Komang Somawirata, and G. D. Naviri, “Alphabet sign language recognition using K-nearest neighbor optimization,” J. Comput., vol. 14, no. 1. pp. 63–70, 2019. 10.17706/jcp.14.1.63-70 Search in Google Scholar
[83] M. M. Kamruzzaman, “Arabic sign language recognition and generating Arabic speech using convolutional neural network,” Wirel. Commun. Mob. Comput., vol. 2020, pp. 1–9, 2020. 10.1155/2020/3685614 . Search in Google Scholar
[84] M. Varsha and C. S. Nair, “Indian sign language gesture recognition using deep convolutional neural network,” 2021 8th International Conference on Smart Computing and Communications (ICSCC), IEEE, 2021. 10.1109/ICSCC51209.2021.9528246 Search in Google Scholar
[85] M. Z. Islam, M. S. Hossain, R. ul Islam, and K. Andersson, “Static hand gesture recognition using convolutional neural network with data augmentation,” 2019 Joint 8th International Conference on Informatics, Electronics & Vision (ICIEV) and 2019 3rd International Conference on Imaging, Vision & Pattern Recognition (icIVPR), Spokane, WA, USA, 2019, pp. 324–329. 10.1109/ICIEV.2019.8858563 . Search in Google Scholar
[86] L. K. S. Tolentino, R. O. Serfa Juan, A. C. Thio-ac, M. A. B. Pamahoy, J. R. R. Forteza, and X. J. O. Garcia, “Static sign language recognition using deep learning,” Int. J. Mach. Learn. Comput., vol. 9, no. 6. pp. 821–827, 2019. 10.18178/ijmlc.2019.9.6.879 Search in Google Scholar
[87] K. Wangchuk, P. Riyamongkol, and R. Waranusast, “Real-time Bhutanese sign language digits recognition system using convolutional neural network,” ICT Exp., vol. 7, no. 2, pp. 215–220, 2020. 10.1016/j.icte.2020.08.002 . Search in Google Scholar
[88] L. K. Tolentino, R. Serfa Juan, A. Thio-ac, M. Pamahoy, J. Forteza, and X. Garcia, “Static sign language recognition using deep learning,” Int. J. Mach. Learn. Comput., vol. 9, pp. 821–827, 2019. 10.18178/ijmlc.2019.9.6.879 . Search in Google Scholar
[89] P. M. Ferreira, J. S. Cardoso, and A. Rebelo, “Multimodal Learning for Sign Language Recognition,” Pattern Recognition and Image Analysis. IbPRIA 2017. Lecture Notes in Computer Science(), L. Alexandre, J. Salvador Sánchez, and J. Rodrigues, (eds), vol. 10255, Cham, Springer, 2017. 10.1007/978-3-319-58838-4_35 . Search in Google Scholar
[90] A. Elboushaki, R. Hannane, A. Karim, and L. Koutti, “MultiD-CNN: a multi-dimensional feature learning approach based on deep convolutional networks for gesture recognition in RGB-D image sequences,” Expert. Syst. Appl., vol. 139, p. 112829, 2019. 10.1016/j.eswa.2019.112829 . Search in Google Scholar
[91] O. Kopuklu, A. Gunduz, N. Kose, and G. Rigoll, “Real-time hand gesture detection and classification using convolutional neural networks,” 2019 14th IEEE International Conference on Automatic Face & Gesture Recognition (FG 2019), 2019. 10.1109/fg.2019.8756576 . Search in Google Scholar
[92] Ch. Yuxiao, L. Zhao, X. Peng, J. Yuan, and D. Metaxas, Construct Dynamic Graphs for Hand Gesture Recognition Via Spatial-temporal Attention, UK, 2019, pp. 1–13. https://bmvc2019.org/wp-content/uploads/papers/0281-paper.pdf. Search in Google Scholar
[93] A. Z. Shukor, M. F. Miskon, M. H. Jamaluddin, F. Bin Ali, M. F. Asyraf, and M. B. Bin Bahar., “A new data glove approach for malaysian sign language detection,” Procedia Computer Science, vol. 76, pp. 60–67, 2015, 10.1016/j.procs.2015.12.276 . Search in Google Scholar
© 2022 Ahmed Sultan et al ., published by De Gruyter
This work is licensed under the Creative Commons Attribution 4.0 International License.
- X / Twitter
Supplementary Materials
Please login or register with De Gruyter to order this product.
Journal and Issue
Articles in the same issue.
Subscribe to the PwC Newsletter
Join the community, add a new evaluation result row, sign language recognition.
73 papers with code • 15 benchmarks • 23 datasets
Sign Language Recognition is a computer vision and natural language processing task that involves automatically recognizing and translating sign language gestures into written or spoken language. The goal of sign language recognition is to develop algorithms that can understand and interpret sign language, enabling people who use sign language as their primary mode of communication to communicate more easily with non-signers.
( Image credit: Word-level Deep Sign Language Recognition from Video: A New Large-scale Dataset and Methods Comparison )

Benchmarks Add a Result
Trend | Dataset | Best Model | -->Paper | Code | Compare |
---|---|---|---|---|---|
SlowFastSign | -->|||||
SlowFastSign | -->|||||
SlowFastSign | -->|||||
STF+LSTM | -->|||||
NLA-SLR | -->|||||
SignBERT | -->|||||
SPOTER | -->|||||
StepNet | -->|||||
SignBERT+ | -->|||||
mVITv2-S | -->|||||
3D-DCNN + ST-MGCN | -->|||||
Skeleton Image Representation | -->|||||
Skeleton Image Representation | -->|||||
StepNet | -->|||||
HWGAT | -->

Latest papers
Signclip: connecting text and sign language by contrastive learning.

We present SignCLIP, which re-purposes CLIP (Contrastive Language-Image Pretraining) to project spoken language text and sign language videos, two classes of natural languages of distinct modalities, into the same space.
MASA: Motion-aware Masked Autoencoder with Semantic Alignment for Sign Language Recognition
To this end, we propose a Motion-Aware masked autoencoder with Semantic Alignment (MASA) that integrates rich motion cues and global semantic information in a self-supervised learning paradigm for SLR.
Multi-Stream Keypoint Attention Network for Sign Language Recognition and Translation
The resulting framework is denoted as MSKA-SLR, which is expanded into a sign language translation (SLT) model through the straightforward addition of an extra translation network.
Optimizing Hand Region Detection in MediaPipe Holistic Full-Body Pose Estimation to Improve Accuracy and Avoid Downstream Errors
This paper addresses a critical flaw in MediaPipe Holistic's hand Region of Interest (ROI) prediction, which struggles with non-ideal hand orientations, affecting sign language recognition accuracy.
CorrNet+: Sign Language Recognition and Translation via Spatial-Temporal Correlation
In specific, CorrNet+ employs a correlation module and an identification module to build human body trajectories.
Improving Continuous Sign Language Recognition with Adapted Image Models
Besides, fully fine-tuning the model easily forgets the generic essential knowledge acquired in the pretraining stage and overfits the downstream data.
Transfer Learning for Cross-dataset Isolated Sign Language Recognition in Under-Resourced Datasets
Sign language recognition (SLR) has recently achieved a breakthrough in performance thanks to deep neural networks trained on large annotated sign datasets.
Dynamic Spatial-Temporal Aggregation for Skeleton-Aware Sign Language Recognition
Current methods utilize spatial graph modules and temporal modules to capture spatial and temporal features, respectively.
TCNet: Continuous Sign Language Recognition from Trajectories and Correlated Regions
A key challenge in continuous sign language recognition (CSLR) is to efficiently capture long-range spatial interactions over time from the video input.
Towards Online Sign Language Recognition and Translation
Our approach comprises three phases: 1) developing a sign language dictionary encompassing all glosses present in a target sign language dataset; 2) training an isolated sign language recognition model on augmented signs using both conventional classification loss and our novel saliency loss; 3) employing a sliding window approach on the input sign sequence and feeding each sign clip to the well-optimized model for online recognition.
Academia.edu no longer supports Internet Explorer.
To browse Academia.edu and the wider internet faster and more securely, please take a few seconds to upgrade your browser .
Enter the email address you signed up with and we'll email you a reset link.
- We're Hiring!
- Help Center
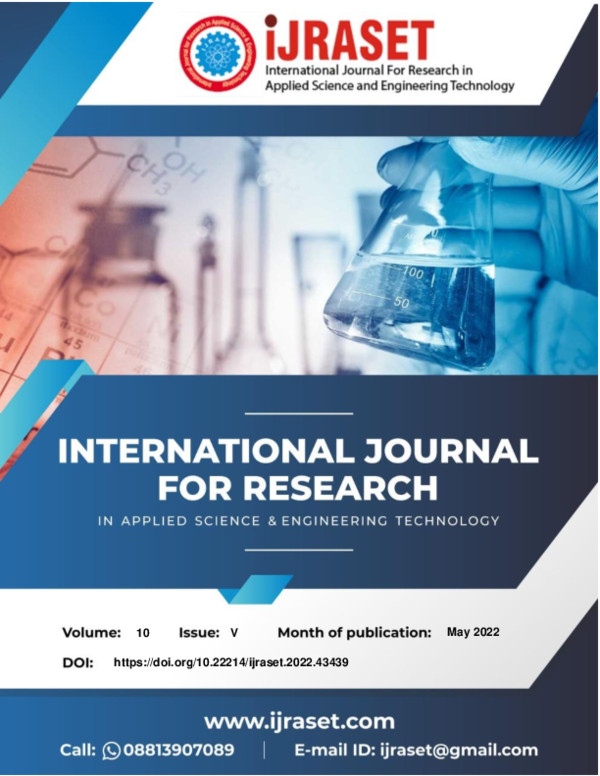
Real-Time Sign Language Detection using TensorFlow, OpenCV and Python

Related Papers
International Journal for Research in Applied Science & Engineering Technology (IJRASET)
IJRASET Publication
Sign Language is globally used by more than 70 million impaired people to communicate and is characterized by fast, highly articulate motion of hand gesture which is difficult for verbal speakers to understand. This limitation combined with the lack of knowledge about sign language by verbal speakers creates a separation where both parties are unable to effectively communicate, to overcome this limitation we propose a new method for sign language recognition using OpenCV (A python library) which is used for pre-processing images and extracting different skin toned hands from the background. In this method hand gesture are used to make signs which are detected by YOLOv5 algorithm for object detection which is the fastest algorithm till date while Convolutional-Neural-Networks (CNN) are used for training gesture and to classify the images, and further we proposed a system which translates speech into sign language so that the words of the verbal speaker can be transmitted to the deaf/mute. This automated system first detects speech using the JavaScript Web-Speech API and converts it into text because the recognized text is processed using the Natural Language Toolkit and aligns token text with the sign language library (sign language videos) videos according to well-known text and finally shows a compiled output which is displayed through avatar animation for a deaf / dumb person. The proposed system has various advantages like Portability, User-friendly Interface and Voice Module. The software is also very cost-effective which only needs a laptop camera or webcam and hand gesture, system accuracy is compared to high-quality methods and is found to be the best.
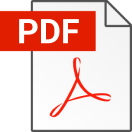
Rising incidents of visual and hearing imparity is a matter of global concern. India itself has around 12 million visually impaired people and over 21 million people are either blind or dead or both. For the blind people, there are various solutions existing such as eye-donation, and hearing aid for the deaf but not everyone can afford it. The purpose of our project is to provide an effective method of communication between the natural people and the impaired people. According to a research article in the "Global World" on January 4,2017 with a deaf community of millions, hearing India is only just beginning to sign. So, to address this problem, we are coming forth with a model based on modern and advanced technologies like machine learning, image processing, artificial intelligence to provide a potential solution and bridge the gap of communication. The sign method is the most accepted method as a means of communication to impaired people. The model will give out the output in the form of text and voice in regional as well as English languages so it can have an effect on the vast majority of the population in rural as well as urban India. This project will definitely provide accessibility, convenience, safety to our visually impaired brothers and sisters who are looked upon by the society just because of their disability.
IJRASET Publication , Palash Dubey
Communication is very imperative for daily life. Normal people use verbal language for communication while people with disabilities use sign language for communication. Sign language is a way of communicating by using the hand gestures and parts of the body instead of speaking and listening. As not all people are familiar with sign language, there lies a language barrier. There has been much research in this field to remove this barrier. There are mainly 2 ways in which we can convert the sign language into speech or text to close the gap, i.e. , Sensor based technique,and Image processing. In this paper we will have a look at the Image processing technique, for which we will be using the Convolutional Neural Network (CNN). So, we have built a sign detector, which will recognise the sign numbers from 1 to 10. It can be easily extended to recognise other hand gestures including alphabets (A-Z) and expressions. We are creating this model based on Indian Sign Language(ISL).
Communication is the method of sharing or exchanging information, ideas or feelings. To have a communication between two people, both of them need to have knowledge and understanding of a common language. But in the case of deaf and dumb people, the means they use for communicating is different from that of normal people. Deaf is not able to hear and dumb is not able to speak. They communicate using sign language among themselves and with normal people but normal people don't take seriously the importance of sign languages. Not everyone has the knowledge and understanding of sign language which make the communication difficult between a normal person and a deaf and dumb person. For overcoming this barrier,a model can be build based on machine learning. A model can be trained to recognize different gestures of sign language and translate them into English language. This will help a lot of people in communicating with deaf and dumb people with ease. A real time ML based system is built for the real time sign language detection with TensorFlow object detection in this paper. The major purpose of this project is to build a system for the differently abled people to communicate with others easily and efficiently.
Deaf and dumb persons use sign language to communicate with other people in their society. Because sign language is the only means of communication for persons who are deaf or hard of hearing, it is mostly utilized by them. Ordinary folks are unfamiliar with this language. A real-time sign language recognition system has been developed in this article to allow those who do not know sign language to communicate with hearing-impaired people more readily. In this case, we employed American Sign Language. We have used American Sign Language in this paper. We introducing the development and implementation of an American Sign Language (ASL) derived from convolutional neural network. Deep Learning Method is used to train a classifier to recognize Sign Language and Convolutional Neural Network (CNN) is used to extract features from the images. We have also used Text-To-Speech Synthesis to convert the detected output into speech format. With use of MATLAB function the obtained text is converted into voice. In our system we are converting text to speech in Hindi language. Therefore hand gesture made by deaf and dumb people has been anatomized and restated into text and voice for better communication.
Sign language is one of the oldest and most natural forms of language for communication , but since most people do not know sign language and interpreters are very difficult to come by, we have come up with a real time method using neural networks for fingerspelling based American sign language. In our method, the hand is first passed through a filter and after the filter is applied the hand is passed through a classifier which predicts the class of the hand gestures. Our method provides 95.7% accuracy for the 26 letters of the alphabet.
Sign language is a way of communicating using hand gestures, movements and facial expressions, instead of spoken words. It is the medium of communication used by people who are deaf or have hearing impairments to exchange information between their own community and with normal people. In order to bridge the communication gap between people with hearing and speaking disabilities and people who do not use sign language, a lot of research work using machine learning algorithms has been done. Hence, Sign language translator came into picture. Sign Language Translators are generally used to interpret signs and gestures from deaf and hard hearing people and convert them into text.
Sign language is a remarkable development that has evolved over time. Unfortunately, there are some disadvantages associated with this language. When conversing with a speech disabled person, not everyone understands how to interpret sign language. Without an interpreter, communication is difficult. We need a product that is both adaptable and robust. We need to transform sign language so that it can be understood by common people and the differently abled can communicate without hurdles.
A large number of deaf and mute people are present around the world and communicating with them is a bit difficult at times; because not everyone can understand Sign language(a system of communication using visual gestures and signs). In addition, there is a lack of official sign language interpreters. In India, the official number of approved sign language interpreters is only 250[1]. This makes communication with deaf and mute people very difficult. The majority of deaf and dumb teaching methods involve accommodating them to people who do not have disabilities-while discouraging the use of sign language. There is a need to encourage the use of sign language. People communicate with each other in sign language by using hand and finger gestures. The language serves its purpose by bridging the gap between the deaf-mute and speaking communities. With recent technological developments, sign language identification is a hard subject in the field of computer vision that has room for further progress. In this project, we propose an optimal recognition engine whose main objective is to translate static American Sign Language alphabets, numbers, and words into human and machine understandable English script and the other way around. Using Neural Networks, we offer a machine learning-based technique for identifying American Sign Language.
The main objective is to extend the reliability of the motor application by using the recent technology advancement. This work makes sure the continuous monitoring of induction motor. By ensuring the system reliability abnormality are easily identified and simply rectified. As induction machine are used nearly 90% in industries, the economic data monitoring is required. The productivity of industries may be increased by doing the preventive maintenance of induction machine. By taking fortification the failure of system and value of mental attitude power motor is protected. The main goal of the server is to provide fast and relevant information about the real word objective and application.
Loading Preview
Sorry, preview is currently unavailable. You can download the paper by clicking the button above.
RELATED PAPERS
International Journal for Innovation Education and Research
Zerihun Tesfaye
International Journal for Research in Applied Science and Engineering Technology
Ketaki Dhotre
Bhagyashri Patle
- We're Hiring!
- Help Center
- Find new research papers in:
- Health Sciences
- Earth Sciences
- Cognitive Science
- Mathematics
- Computer Science
- Academia ©2024
IEEE Account
- Change Username/Password
- Update Address
Purchase Details
- Payment Options
- Order History
- View Purchased Documents
Profile Information
- Communications Preferences
- Profession and Education
- Technical Interests
- US & Canada: +1 800 678 4333
- Worldwide: +1 732 981 0060
- Contact & Support
- About IEEE Xplore
- Accessibility
- Terms of Use
- Nondiscrimination Policy
- Privacy & Opting Out of Cookies
A not-for-profit organization, IEEE is the world's largest technical professional organization dedicated to advancing technology for the benefit of humanity. © Copyright 2024 IEEE - All rights reserved. Use of this web site signifies your agreement to the terms and conditions.
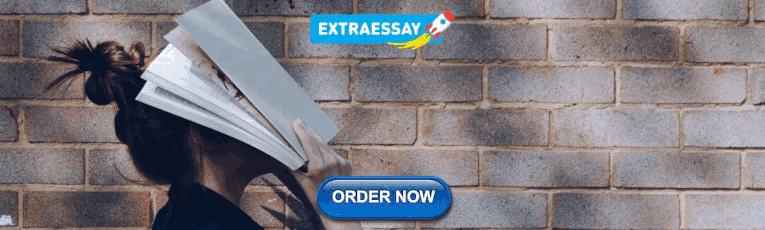
IMAGES
VIDEO
COMMENTS
The three countries play a key role in advancing sign language recognition research, with India leading worldwide. India led with 123 publications over the past two decades, covering 15.4%of the total global publications. ... and joining the edges. Edge detection techniques reviewed in this paper are Robert edge detector, Sobel edge detector ...
This paper focuses on the evolution of a real-time sign language detection model using computer vision, machine learning, and deep learning. ... vision research is the recognition of visual sign ...
Sign Language Recognition is a breakthrough for communication among deaf-mute society and has been a critical research topic for years. ... paper is divided ... Sign Language Detection Using SIFT ...
In the paper by MD Nafis Saiful et al [28], the proposed approach employs deep learning to detect sign language, aiming to bridge communication barriers between the hearing and deaf communities ...
People with hearing impairments are found worldwide; therefore, the development of effective local level sign language recognition (SLR) tools is essential. We conducted a comprehensive review of automated sign language recognition based on machine/deep learning methods and techniques published between 2014 and 2021 and concluded that the current methods require conceptual classification to ...
Sign language is a predominant form of communication among a large group of society. The nature of sign languages is visual, making them distinct from spoken languages. Unfortunately, very few able people can understand sign language making communication with the hearing-impaired infeasible. Research in the field of sign language recognition (SLR) can help reduce the barrier between deaf and ...
The remainder of this paper is organized as follows. Section 2 includes a brief review of Deep Learning algorithms. Section 3 presents a taxonomy of the sign language recognition area. Hand sign language, face sign language, and human sign language literature are reviewed in Sections 4, 5, and 6, respectively.Section 7 presents the recent models in continuous sign language recognition.
2. Paper. Code. **Sign Language Recognition** is a computer vision and natural language processing task that involves automatically recognizing and translating sign language gestures into written or spoken language. The goal of sign language recognition is to develop algorithms that can understand and interpret sign language, enabling people ...
This paper aims to analyse the research published on intelligent systems in sign language recognition over the past two decades. A total of 649 publications related to decision support and ...
Section 3 describes the methodology for implementing an isolated SLR system for real-time sign language detection and recognition, which involves pre-processing, feature extraction, training, and testing steps. This research paper proposes a feedback-based learning methodology using these options, based on a combination of LSTM and GRU: (1) a ...
Sign Language (SL) is the main language for handicapped and disabled people. ... Many research problems are suggested in this domain such as Sign Language Recognition ... and M. B. Bin Bahar., "A new data glove approach for malaysian sign language detection," Procedia Computer Science, vol. 76, pp. 60-67, 2015, 10.1016/j.procs.2015.12.276 ...
Abstract: Sign Language Detection has become crucial and effective for humans and research in this area is in progress and is one of the applications of Computer Vision. Earlier works included detection using static signs with the help of a simple deep learning-based Convolutional Neural Network. This proposal is based on continuous detection of image frames in real-time using action detection ...
The real-time sign language recognition system is developed for recognizing the gestures of Indian Sign Language (ISL). Generally, sign languages consist of hand gestures. For recognizing the signs, the Regions of Interest (ROI) are identified and tracked using the skin segmentation feature of OpenCV. Then by using [1] Media Pipe, it captures the landmarks of the hands and the key points of ...
229. 10 Jan 2024. Paper. Code. **Sign Language Recognition** is a computer vision and natural language processing task that involves automatically recognizing and translating sign language gestures into written or spoken language. The goal of sign language recognition is to develop algorithms that can understand and interpret sign language ...
double-handed gestures but they are not real-time. In this paper, we propose a method to create an Indian Sign Language dataset using a webcam and then using transfer learning, train a TensorFlow model to create a real-time Sign Language Recognition system. The system achieves a good level of accuracy even with a limited size dataset. Keywords:
This paper presents an innovative approach for sign language recognition and conversion to text using a custom dataset containing 15 different classes, each class containing 70-75 different images.
MIE324 Final Report: Sign Language Recognition Anna Deza (1003287855) and Danial Hasan (1003132228) Decemeber 2nd 2018 Word Count: 1993 Penalty: 0% 1 Introduction The goal of this project was to build a neural network able to classify which letter of the American Sign Language (ASL) alphabet is being signed, given an image of a signing hand.
Academia.edu is a platform for academics to share research papers. Real-Time Sign Language Detection using TensorFlow, OpenCV and Python ... 45.98; SJ Impact Factor: 7.538 Volume 10 Issue V May 2022- Available at www.ijraset.com Real-Time Sign Language Detection using TensorFlow, OpenCV and Python Prashant Verma1, Khushboo Badli2 1, 2 Student ...
Sign language is used by speech and hearing-impaired people as a method of communication. There are thousands of sign languages used all around the globe. Understanding sign language is a challenging task for a normal person. At present, speech and hearing-impaired people depend on human translators to make this task easier, but it is not always feasible to have a human translator. The ...
YOLOv8 gives a quicker minimu m point which is around the 40th epoc h mark; contr asting that, YOLOv5. res ults give that point around af ter the 50th epoch, pr esumably around the 60th epoch or ...
A. Methods of Hand-Gesture Recognition in Sign Language Recognition (survey):- Given paper focused on methods used in the prior Sign Language Recognition systems. Based on our review, HMM-based approaches have been extensively explored in prior research, including its modifications. Deep
ArSL there are 70% of sign language recognition systems. who achieved a verage accuracy of greater than 90%, while. 23% of the systems have accuracy be tween 80 and 89%. There are only 7% systems ...
International Journal of Research Publication and Reviews, Vol 4, no 4, pp 2495-2499, April 2023 International Journal of Research Publication and Reviews Journal homepage: www.ijrpr.com ISSN 2582-7421 Real Time Sign Language Detection Using Yolov5 Algorithm Mr. D. Chiranjeevulu1, J. Tejasri2, I. Sasi Kala3, K. Hemanth4, N. Rakesh5