Research Analyst Skills
Learn about the skills that will be most essential for Research Analysts in 2024.
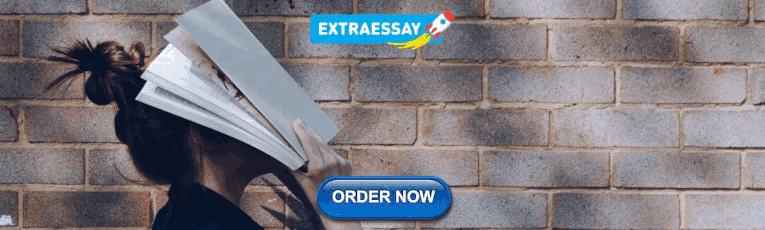
Getting Started as a Research Analyst
- What is a Research Analyst
- How To Become
- Certifications
- Tools & Software
- LinkedIn Guide
- Interview Questions
- Work-Life Balance
- Professional Goals
- Resume Examples
- Cover Letter Examples
What Skills Does a Research Analyst Need?
Find the important skills for any job.
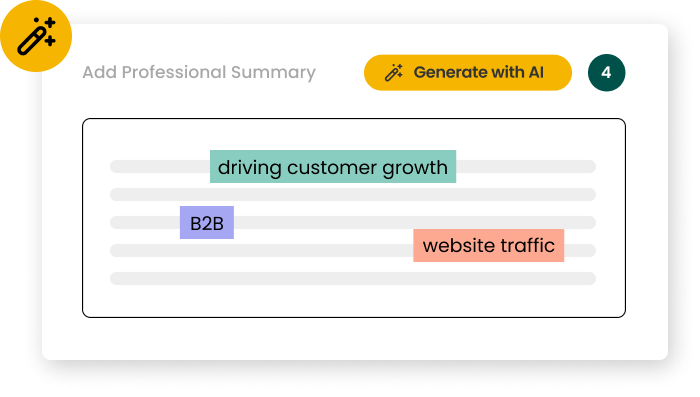
Types of Skills for Research Analysts
Critical thinking and analytical skills, technical proficiency and data management, quantitative research and statistical knowledge, communication and visualization, industry knowledge and business acumen, top hard skills for research analysts.
- Critical Thinking and Analytical Reasoning
- Effective Communication
Attention to Detail
- Problem-Solving
- Adaptability and Flexibility
- Time Management and Prioritization
- Collaboration and Teamwork
- Creativity and Innovation
- Emotional Intelligence
- Persuasion and Negotiation
Top Soft Skills for Research Analysts
- Data Collection and Management
- Statistical Analysis and Quantitative Methods
- Data Visualization and Reporting
- Advanced Excel and Spreadsheet Proficiency
- Database Management and SQL
- Programming Skills in Python or R
- Econometrics and Modeling Techniques
- Machine Learning and Predictive Analytics
- Survey Design and Implementation
- Big Data Analytics Tools (e.g., Hadoop, Spark)
Most Important Research Analyst Skills in 2024
Advanced analytical proficiency, critical thinking and problem solving, technological savvy, effective communication and storytelling, industry-specific knowledge, quantitative research methodologies, adaptability and continuous learning.
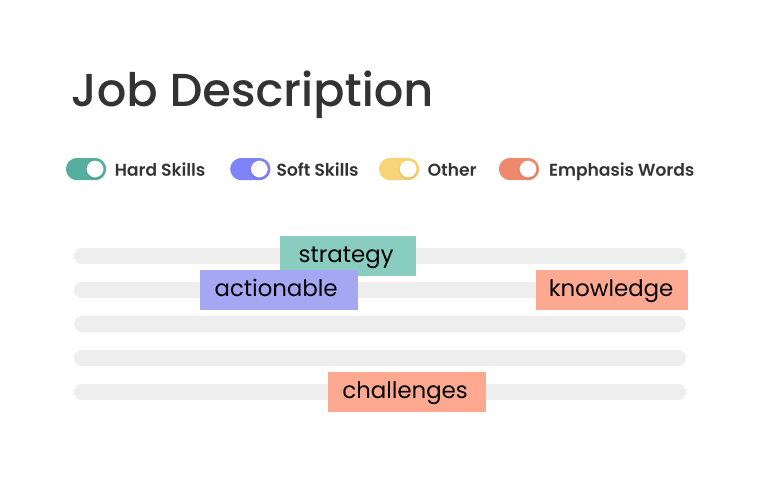
Show the Right Skills in Every Application
Research analyst skills by experience level, important skills for entry-level research analysts, important skills for mid-level research analysts, important skills for senior research analysts, most underrated skills for research analysts, 1. intellectual curiosity, 2. effective communication, 3. active listening, how to demonstrate your skills as a research analyst in 2024, how you can upskill as a research analyst.
- Master Advanced Data Analytics Tools: Invest time in learning cutting-edge data analysis software and platforms that are becoming industry standards, to handle large datasets more efficiently.
- Develop Proficiency in Statistical Programming: Gain expertise in programming languages such as R or Python, which are essential for complex data manipulation and analysis.
- Expand Your Knowledge in Machine Learning: Explore machine learning techniques to uncover deeper insights from data and stay competitive in the field of advanced analytics.
- Enroll in Specialized Research Methodology Courses: Keep abreast of the latest research methodologies by taking courses from accredited institutions or online learning platforms.
- Build a Strong Foundation in Data Ethics: Understand the ethical implications of data handling and analysis to ensure integrity and trustworthiness in your research.
- Participate in Research Forums and Networks: Engage with the research community through forums, webinars, and professional networks to exchange ideas and stay informed about industry trends.
- Seek Opportunities for Collaborative Research: Collaborate with peers from different disciplines to broaden your perspective and enhance your analytical skills.
- Attend Industry-Specific Seminars and Conferences: Stay updated on sector-specific insights and developments by attending relevant events, which can also serve as networking opportunities.
- Focus on Effective Communication of Findings: Improve your ability to communicate complex data and analysis clearly to stakeholders through visualization tools and storytelling techniques.
- Embrace Continuous Learning: Dedicate time to reading academic journals, industry reports, and books to keep your knowledge current and comprehensive.
Skill FAQs for Research Analysts
What are the emerging skills for research analysts today, how can research analysts effectivley develop their soft skills, how important is technical expertise for research analysts.
Research Analyst Education
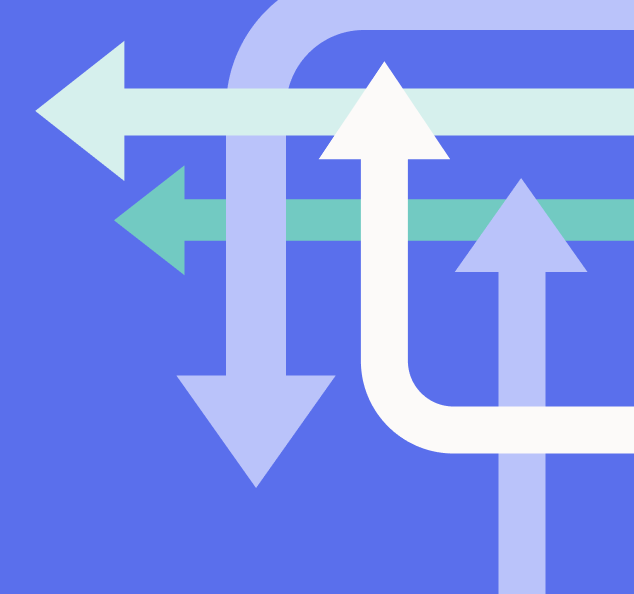
More Skills for Related Roles
Unlocking business insights through data, driving strategic decisions with numbers
Transforming data into insights, driving strategic business decisions and growth
Unearthing insights from data, driving strategic decisions with predictive analytics
Interpreting economic trends, shaping business strategy with insightful analysis
Unearthing insights and data to drive decision-making, shaping the future of research
Driving business growth and efficiency through data-driven insights and strategic analysis
Start Your Research Analyst Career with Teal
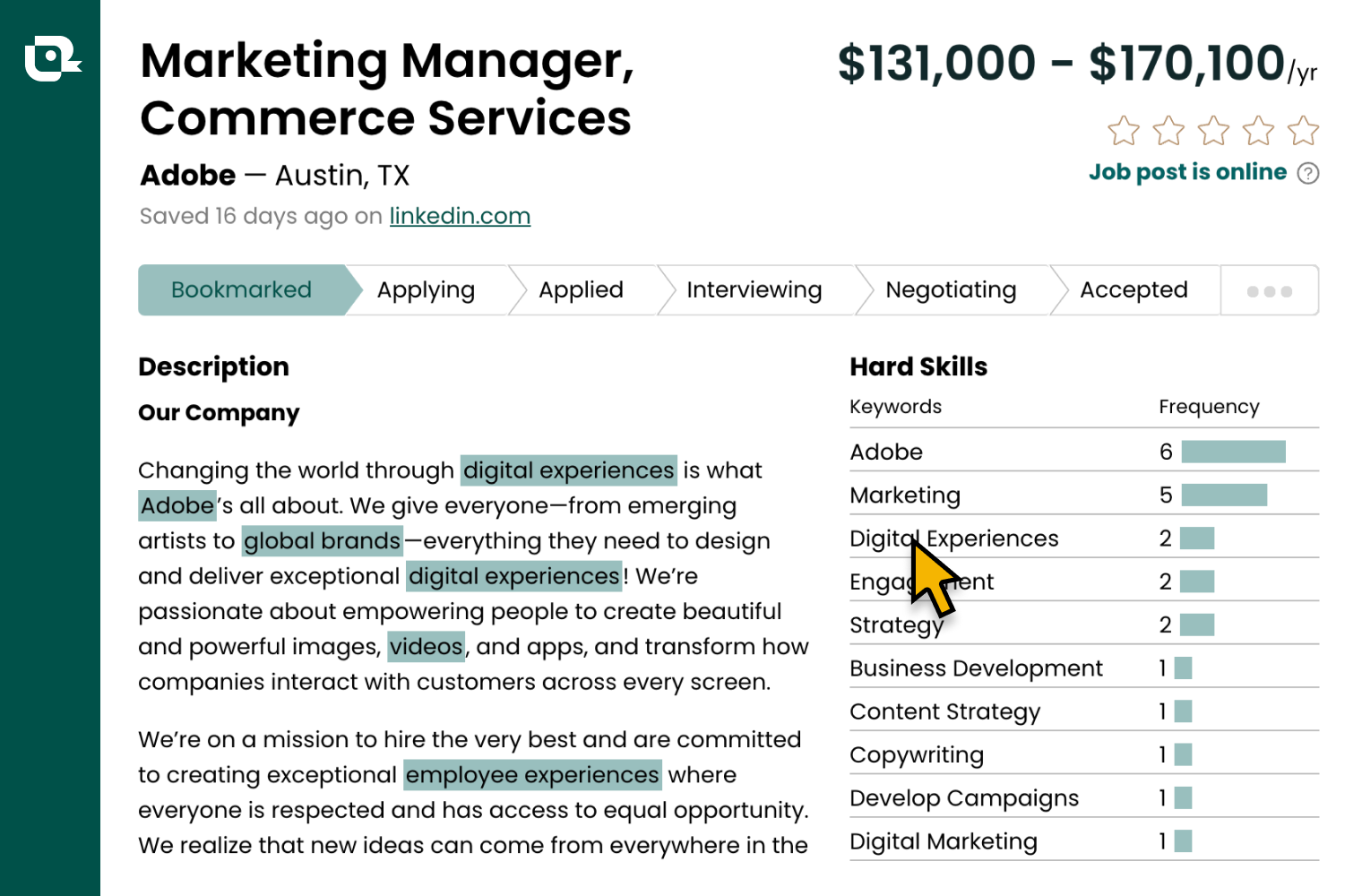
Instant insights, infinite possibilities
What are research skills?
Last updated
26 April 2023
Reviewed by
Short on time? Get an AI generated summary of this article instead
Broadly, it includes a range of talents required to:
Find useful information
Perform critical analysis
Form hypotheses
Solve problems
It also includes processes such as time management, communication, and reporting skills to achieve those ends.
Research requires a blend of conceptual and detail-oriented modes of thinking. It tests one's ability to transition between subjective motivations and objective assessments to ensure only correct data fits into a meaningfully useful framework.
As countless fields increasingly rely on data management and analysis, polishing your research skills is an important, near-universal way to improve your potential of getting hired and advancing in your career.
Make research less tedious
Dovetail streamlines research to help you uncover and share actionable insights
What are basic research skills?
Almost any research involves some proportion of the following fundamental skills:
Organization
Decision-making
Investigation and analysis
Creative thinking
What are primary research skills?
The following are some of the most universally important research skills that will help you in a wide range of positions:
Time management — From planning and organization to task prioritization and deadline management, time-management skills are highly in-demand workplace skills.
Problem-solving — Identifying issues, their causes, and key solutions are another essential suite of research skills.
Critical thinking — The ability to make connections between data points with clear reasoning is essential to navigate data and extract what's useful towards the original objective.
Communication — In any collaborative environment, team-building and active listening will help researchers convey findings more effectively through data summarizations and report writing.
What are the most important skills in research?
Detail-oriented procedures are essential to research, which allow researchers and their audience to probe deeper into a subject and make connections they otherwise may have missed with generic overviews.
Maintaining priorities is also essential so that details fit within an overarching strategy. Lastly, decision-making is crucial because that's the only way research is translated into meaningful action.
- Why are research skills important?
Good research skills are crucial to learning more about a subject, then using that knowledge to improve an organization's capabilities. Synthesizing that research and conveying it clearly is also important, as employees seek to share useful insights and inspire effective actions.
Effective research skills are essential for those seeking to:
Analyze their target market
Investigate industry trends
Identify customer needs
Detect obstacles
Find solutions to those obstacles
Develop new products or services
Develop new, adaptive ways to meet demands
Discover more efficient ways of acquiring or using resources
Why do we need research skills?
Businesses and individuals alike need research skills to clarify their role in the marketplace, which of course, requires clarity on the market in which they function in. High-quality research helps people stay better prepared for challenges by identifying key factors involved in their day-to-day operations, along with those that might play a significant role in future goals.
- Benefits of having research skills
Research skills increase the effectiveness of any role that's dependent on information. Both individually and organization-wide, good research simplifies what can otherwise be unwieldy amounts of data. It can help maintain order by organizing information and improving efficiency, both of which set the stage for improved revenue growth.
Those with highly effective research skills can help reveal both:
Opportunities for improvement
Brand-new or previously unseen opportunities
Research skills can then help identify how to best take advantage of available opportunities. With today's increasingly data-driven economy, it will also increase your potential of getting hired and help position organizations as thought leaders in their marketplace.
- Research skills examples
Being necessarily broad, research skills encompass many sub-categories of skillsets required to extrapolate meaning and direction from dense informational resources. Identifying, interpreting, and applying research are several such subcategories—but to be specific, workplaces of almost any type have some need of:
Searching for information
Attention to detail
Taking notes
Problem-solving
Communicating results
Time management
- How to improve your research skills
Whether your research goals are to learn more about a subject or enhance workflows, you can improve research skills with this failsafe, four-step strategy:
Make an outline, and set your intention(s)
Know your sources
Learn to use advanced search techniques
Practice, practice, practice (and don't be afraid to adjust your approach)
These steps could manifest themselves in many ways, but what's most important is that it results in measurable progress toward the original goals that compelled you to research a subject.
- Using research skills at work
Different research skills will be emphasized over others, depending on the nature of your trade. To use research most effectively, concentrate on improving research skills most relevant to your position—or, if working solo, the skills most likely have the strongest impact on your goals.
You might divide the necessary research skills into categories for short, medium, and long-term goals or according to each activity your position requires. That way, when a challenge arises in your workflow, it's clearer which specific research skill requires dedicated attention.
How can I learn research skills?
Learning research skills can be done with a simple three-point framework:
Clarify the objective — Before delving into potentially overwhelming amounts of data, take a moment to define the purpose of your research. If at any point you lose sight of the original objective, take another moment to ask how you could adjust your approach to better fit the original objective.
Scrutinize sources — Cross-reference data with other sources, paying close attention to each author's credentials and motivations.
Organize research — Establish and continually refine a data-organization system that works for you. This could be an index of resources or compiling data under different categories designed for easy access.
Which careers require research skills?
Especially in today's world, most careers require some, if not extensive, research. Developers, marketers, and others dealing in primarily digital properties especially require extensive research skills—but it's just as important in building and manufacturing industries, where research is crucial to construct products correctly and safely.
Engineering, legal, medical, and literally any other specialized field will require excellent research skills. Truly, almost any career path will involve some level of research skills; and even those requiring only minimal research skills will at least require research to find and compare open positions in the first place.
Should you be using a customer insights hub?
Do you want to discover previous research faster?
Do you share your research findings with others?
Do you analyze research data?
Start for free today, add your research, and get to key insights faster
Editor’s picks
Last updated: 18 April 2023
Last updated: 27 February 2023
Last updated: 22 August 2024
Last updated: 5 February 2023
Last updated: 16 August 2024
Last updated: 9 March 2023
Last updated: 30 April 2024
Last updated: 12 December 2023
Last updated: 11 March 2024
Last updated: 4 July 2024
Last updated: 6 March 2024
Last updated: 5 March 2024
Last updated: 13 May 2024
Latest articles
Related topics, .css-je19u9{-webkit-align-items:flex-end;-webkit-box-align:flex-end;-ms-flex-align:flex-end;align-items:flex-end;display:-webkit-box;display:-webkit-flex;display:-ms-flexbox;display:flex;-webkit-flex-direction:row;-ms-flex-direction:row;flex-direction:row;-webkit-box-flex-wrap:wrap;-webkit-flex-wrap:wrap;-ms-flex-wrap:wrap;flex-wrap:wrap;-webkit-box-pack:center;-ms-flex-pack:center;-webkit-justify-content:center;justify-content:center;row-gap:0;text-align:center;max-width:671px;}@media (max-width: 1079px){.css-je19u9{max-width:400px;}.css-je19u9>span{white-space:pre;}}@media (max-width: 799px){.css-je19u9{max-width:400px;}.css-je19u9>span{white-space:pre;}} decide what to .css-1kiodld{max-height:56px;display:-webkit-box;display:-webkit-flex;display:-ms-flexbox;display:flex;-webkit-align-items:center;-webkit-box-align:center;-ms-flex-align:center;align-items:center;}@media (max-width: 1079px){.css-1kiodld{display:none;}} build next, decide what to build next, log in or sign up.
Get started for free
- Business Essentials
- Leadership & Management
- Credential of Leadership, Impact, and Management in Business (CLIMB)
- Entrepreneurship & Innovation
- Digital Transformation
- Finance & Accounting
- Business in Society
- For Organizations
- Support Portal
- Media Coverage
- Founding Donors
- Leadership Team
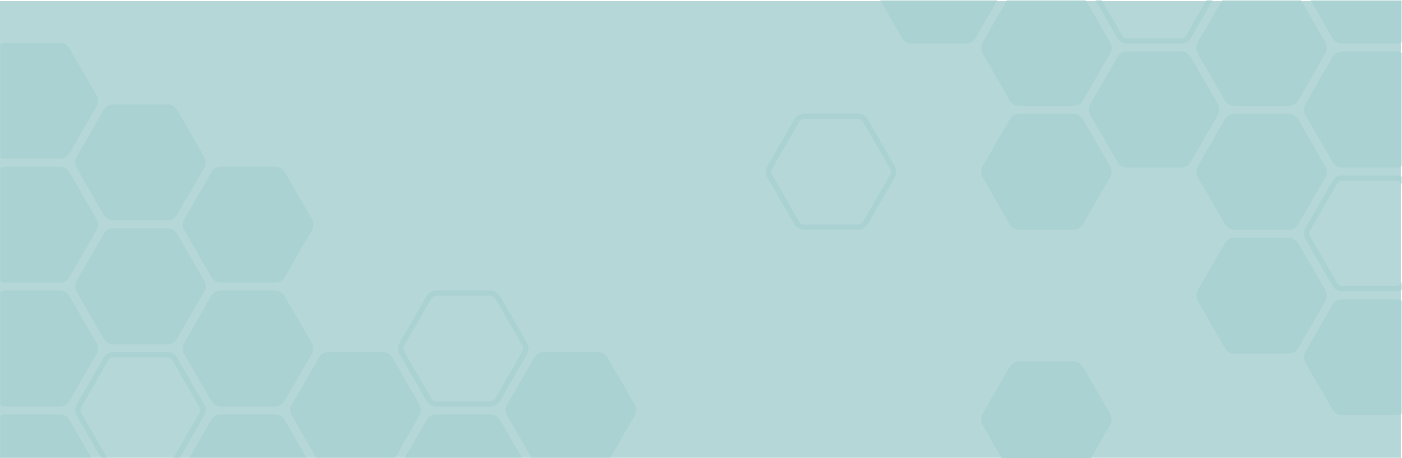
- Harvard Business School →
- HBS Online →
- Business Insights →
Business Insights
Harvard Business School Online's Business Insights Blog provides the career insights you need to achieve your goals and gain confidence in your business skills.
- Career Development
- Communication
- Decision-Making
- Earning Your MBA
- Negotiation
- News & Events
- Productivity
- Staff Spotlight
- Student Profiles
- Work-Life Balance
- AI Essentials for Business
- Alternative Investments
- Business Analytics
- Business Strategy
- Business and Climate Change
- Creating Brand Value
- Design Thinking and Innovation
- Digital Marketing Strategy
- Disruptive Strategy
- Economics for Managers
- Entrepreneurship Essentials
- Financial Accounting
- Global Business
- Launching Tech Ventures
- Leadership Principles
- Leadership, Ethics, and Corporate Accountability
- Leading Change and Organizational Renewal
- Leading with Finance
- Management Essentials
- Negotiation Mastery
- Organizational Leadership
- Power and Influence for Positive Impact
- Strategy Execution
- Sustainable Business Strategy
- Sustainable Investing
- Winning with Digital Platforms
4 Ways to Improve Your Analytical Skills
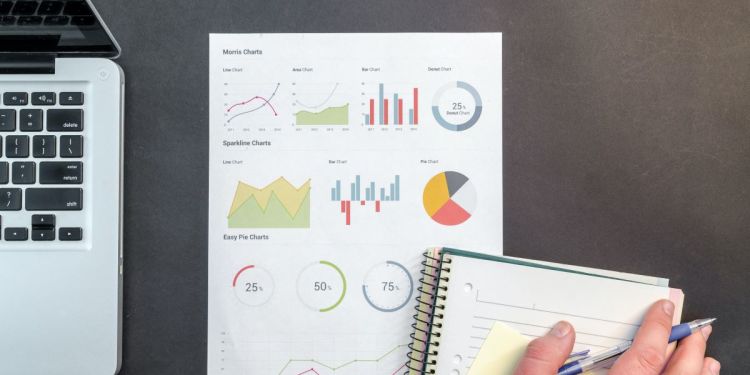
- 07 Jan 2021
Data is ubiquitous. It’s collected at every purchase made, flight taken, ad clicked, and social media post liked—which means it’s never been more crucial to understand how to analyze it.
“Never before has so much data about so many different things been collected and stored every second of every day,” says Harvard Business School Professor Jan Hammond in the online course Business Analytics .
The volume of data you encounter can be overwhelming and raise several questions: Can I trust the data’s source? Is it structured in a way that makes sense? What story does it tell, and what actions does it prompt?
Data literacy and analytical skills can enable you to answer these questions and not only make sense of raw data, but use it to drive impactful change at your organization.
Here’s a look at what it means to be data literate and four ways to improve your analytical skills.
Access your free e-book today.
What Is Data Literacy?
Data literacy is the ability to analyze, interpret, and question data. A dataset is made up of numerous data points that, when viewed together, tell a story.
Before conducting an analysis, it’s important to ensure your data’s quality and structure is in accordance with your organization’s needs.
“In order to transform data into actionable information, you first need to evaluate its quality,” says Professor Dustin Tingley in the Harvard Online course Data Science Principles . “But evaluating the quality of your data is just the first step. You’ll also need to structure your data. Without structure, it’s nearly impossible to extract any information.”
When you’re able to look at quality data, structure it, and analyze it, trends emerge. The next step is to reflect on your analysis and take action.
Tingley shares several questions to ask yourself once you’ve analyzed your dataset: “Did all the steps I took make sense? If so, how should I respond to my analysis? If not, what should I go back and improve?”
For example, you may track users who click a button to download an e-book from your website.
After ensuring your data’s quality and structuring it in a way that makes sense, you begin your analysis and find that a user’s age is positively correlated with their likelihood to click. What story does this trend tell? What does it say about your users, product offering, and business strategy?
To answer these questions, you need strong analytical skills, which you can develop in several ways.
Related: Business Analytics: What It Is & Why It’s Important
How to Improve Your Analytical Skills
Analysis is an important skill to have in any industry because it enables you to support decisions with data, learn more about your customers, and predict future trends.
Key analytical skills for business include:
- Visualizing data
- Determining the relationship between two or more variables
- Forming and testing hypotheses
- Performing regressions using statistical programs, such as Microsoft Excel
- Deriving actionable conclusions from data analysis
If you want to provide meaningful conclusions and data-based recommendations to your team, here are four ways to bolster your analytical skills.
Related: How to Learn Business Analytics Without A Business Background
1. Consider Opposing Viewpoints
While engaging with opposing viewpoints can help you expand your perspective, combat bias, and show your fellow employees their opinions are valued, it can also be a useful way to practice analytical skills.
When analyzing data, it’s crucial to consider all possible interpretations and avoid getting stuck in one way of thinking.
For instance, revisit the example of tracking users who click a button on your site to download an e-book. The data shows that the user’s age is positively correlated with their likelihood to click the button; as age increases, downloads increase, too. At first glance, you may interpret this trend to mean that a user chooses to download the e-book because of their age.
This conclusion, however, doesn’t take into consideration the vast number of variables that change with age. For instance, perhaps the real reason your older users are more likely to download the e-book is their higher level of responsibility at work, higher average income, or higher likelihood of being parents.
This example illustrates the need to consider multiple interpretations of data, and specifically shows the difference between correlation (the trending of two or more variables in the same direction) and causation (when a trend in one variable causes a trend to occur in one or more other variables).
“Data science is built on a foundation of critical thinking,” Tingley says in Data Science Principles . “From the first step of determining the quality of a data source to determining the accuracy of an algorithm, critical thinking is at the heart of every decision data scientists—and those who work with them—make.”
To practice this skill, challenge yourself to question your assumptions and ask others for their opinions. The more you actively engage with different viewpoints, the less likely you are to get stuck in a one-track mindset when analyzing data.
2. Play Games or Brain Teasers
If you’re looking to sharpen your skills on a daily basis, there are many simple, enjoyable ways to do so.
Games, puzzles, and stories that require visualizing relationships between variables, examining situations from multiple angles, and drawing conclusions from known data points can help you build the skills necessary to analyze data.
Some fun ways to practice analytical thinking include:
- Crossword puzzles
- Mystery novels
- Logic puzzles
- Strategic board games or card games
These options can supplement your analytics coursework and on-the-job experience. Some of them also allow you to spend time with friends or family. Try engaging with one each day to hone your analytical mindset.
Related: 3 Examples of Business Analytics in Action
3. Take an Online Analytics Course
Whether you want to learn the basics, brush up on your skills, or expand your knowledge, taking an analytics course is an effective way to improve. A course can enable you to focus on the content you want to learn, engage with the material presented by a professional in the field, and network and interact with others in the data analytics space.
For a beginner, courses like Harvard Online's Data Science Principles can provide a foundation in the language of data. A more advanced course, like Harvard Online's Data Science for Business , may be a fit if you’re looking to explore specific facets of analytics, such as forecasting and machine learning. If you’re interested in hands-on applications of analytical formulas, a course like HBS Online's Business Analytics could be right for you. The key is to understand what skills you hope to gain, then find a course that best fits your needs.
If you’re balancing a full-time job with your analytics education, an online format may be a good choice . It offers the flexibility to engage with course content whenever and wherever is most convenient for you.
An online course may also present the opportunity to network and build relationships with other professionals devoted to strengthening their analytical skills. A community of like-minded learners can prove to be an invaluable resource as you learn and advance your career.
Related: Is An Online Business Analytics Course Worth It?
4. Engage With Data
Once you have a solid understanding of data science concepts and formulas, the next step is to practice. Like any skill, analytical skills improve the more you use them.
Mock datasets—which you can find online or create yourself—present a low-risk option for putting your skills to the test. Import the data into Microsoft Excel, then explore: make mistakes, try that formula you’re unsure of, and ask big questions of your dataset. By testing out different analyses, you can gain confidence in your knowledge.
Once you’re comfortable, engage with your organization’s data. Because these datasets have inherent meaning to your business's financial health, growth, and strategic direction, analyzing them can produce evidence and insights that support your decisions and drive change at your organization.

Investing in Your Data Literacy
As data continues to be one of businesses’ most valuable resources, taking the time and effort to build and bolster your analytical skill set is vital.
“Much more data are going to be available; we’re only seeing the beginning now,” Hammond says in a previous article . “If you don’t use the data, you’re going to fall behind. People that have those capabilities—as well as an understanding of business contexts—are going to be the ones that will add the most value and have the greatest impact.”
Are you interested in furthering your data literacy? Download our Beginner’s Guide to Data & Analytics to learn how you can leverage the power of data for professional and organizational success.

About the Author
How it works
Transform your enterprise with the scalable mindsets, skills, & behavior change that drive performance.
Explore how BetterUp connects to your core business systems.
We pair AI with the latest in human-centered coaching to drive powerful, lasting learning and behavior change.
Build leaders that accelerate team performance and engagement.
Unlock performance potential at scale with AI-powered curated growth journeys.
Build resilience, well-being and agility to drive performance across your entire enterprise.
Transform your business, starting with your sales leaders.
Unlock business impact from the top with executive coaching.
Foster a culture of inclusion and belonging.
Accelerate the performance and potential of your agencies and employees.
See how innovative organizations use BetterUp to build a thriving workforce.
Discover how BetterUp measurably impacts key business outcomes for organizations like yours.
Daring Leadership Institute: a groundbreaking partnership that amplifies Brené Brown's empirically based, courage-building curriculum with BetterUp’s human transformation platform.

- What is coaching?
Learn how 1:1 coaching works, who its for, and if it's right for you.
Accelerate your personal and professional growth with the expert guidance of a BetterUp Coach.
Types of Coaching
Navigate career transitions, accelerate your professional growth, and achieve your career goals with expert coaching.
Enhance your communication skills for better personal and professional relationships, with tailored coaching that focuses on your needs.
Find balance, resilience, and well-being in all areas of your life with holistic coaching designed to empower you.
Discover your perfect match : Take our 5-minute assessment and let us pair you with one of our top Coaches tailored just for you.
Find your coach
-1.png)
Research, expert insights, and resources to develop courageous leaders within your organization.
Best practices, research, and tools to fuel individual and business growth.
View on-demand BetterUp events and learn about upcoming live discussions.
The latest insights and ideas for building a high-performing workplace.
- BetterUp Briefing
The online magazine that helps you understand tomorrow's workforce trends, today.
Innovative research featured in peer-reviewed journals, press, and more.
Founded in 2022 to deepen the understanding of the intersection of well-being, purpose, and performance
We're on a mission to help everyone live with clarity, purpose, and passion.
Join us and create impactful change.
Read the buzz about BetterUp.
Meet the leadership that's passionate about empowering your workforce.

For Business
For Individuals

What are analytical skills? Examples and how to level up
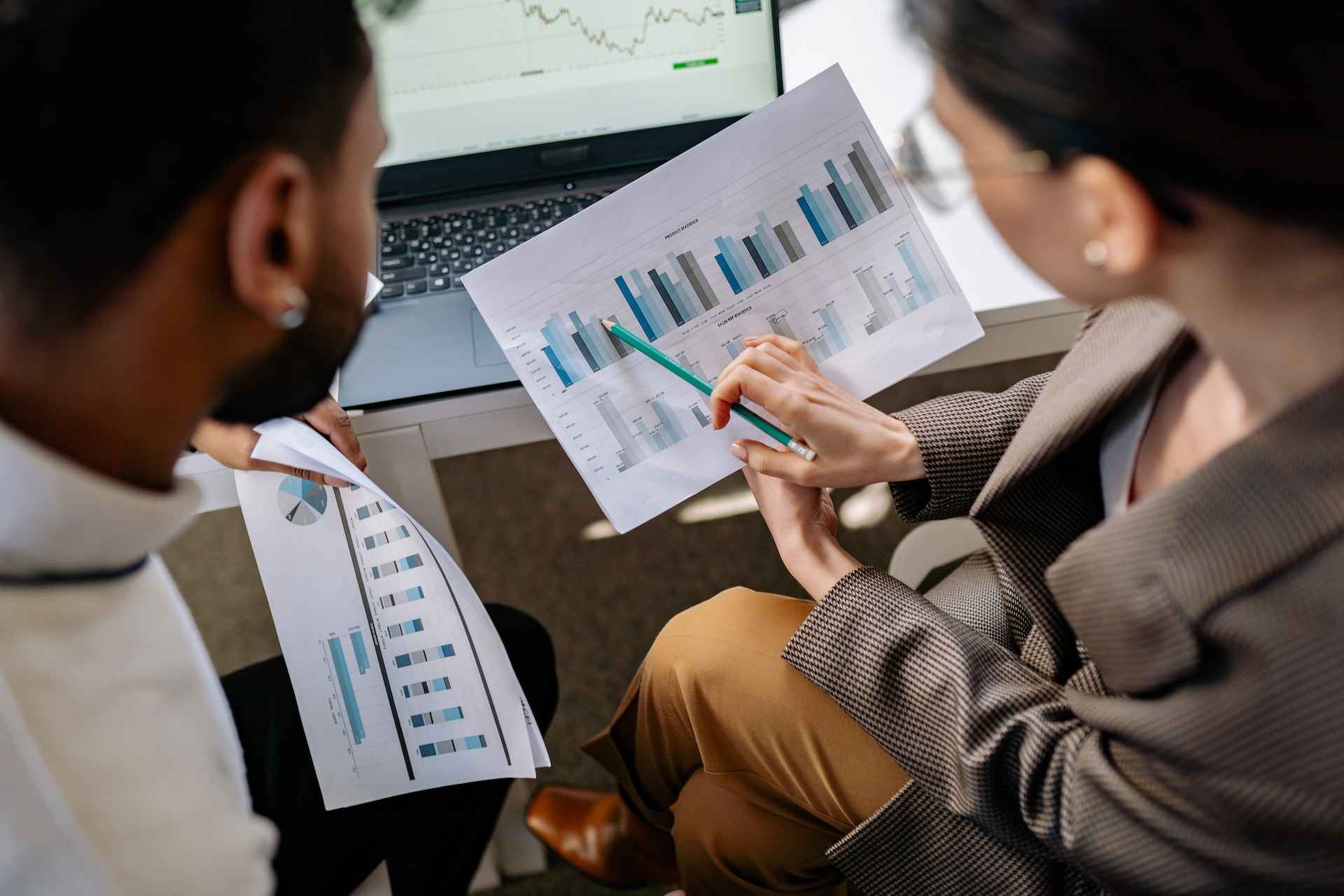
Jump to section
What are analytical skills?
Why are analytical skills important, 9 analytical skills examples, how to improve analytical skills, how to show analytical skills in a job application, the benefits of an analytical mind.
With market forecasts, performance metrics, and KPIs, work throws a lot of information at you.
If you want to stay ahead of the curve, not only do you have to make sense of the data that comes your way — you need to put it to good use. And that requires analytical skills.
You likely use analytical thinking skills every day without realizing it, like when you solve complex problems or prioritize tasks . But understanding the meaning of analysis skills in a job description, why you should include them in your professional development plan, and what makes them vital to every position can help advance your career.
Analytical skills, or analysis skills, are the ones you use to research and interpret information. Although you might associate them with data analysis, they help you think critically about an issue, make decisions , and solve problems in any context. That means anytime you’re brainstorming for a solution or reviewing a project that didn’t go smoothly, you’re analyzing information to find a conclusion. With so many applications, they’re relevant for nearly every job, making them a must-have on your resume.
Analytical skills help you think objectively about information and come to informed conclusions. Positions that consider these skills the most essential qualification grew by 92% between 1980 and 2018 , which shows just how in-demand they are. And according to Statista, global data creation will grow to more than 180 zettabytes by 2025 — a number with 21 zeros. That data informs every industry, from tech to marketing.
Even if you don’t interact with statistics and data on the job, you still need analytical skills to be successful. They’re incredibly valuable because:
- They’re transferable: You can use analysis skills in a variety of professional contexts and in different areas of your life, like making major decisions as a family or setting better long-term personal goals.
- They build agility: Whether you’re starting a new position or experiencing a workplace shift, analysis helps you understand and adapt quickly to changing conditions.
- They foster innovation: Analytical skills can help you troubleshoot processes or operational improvements that increase productivity and profitability.
- They make you an attractive candidate: Companies are always looking for future leaders who can build company value. Developing a strong analytical skill set shows potential employers that you’re an intelligent, growth-oriented candidate.
If the thought of evaluating data feels unintuitive, or if math and statistics aren’t your strong suits, don’t stress. Many examples of analytical thinking skills don’t involve numbers. You can build your logic and analysis abilities through a variety of capacities, such as:
1. Brainstorming
Using the information in front of you to generate new ideas is a valuable transferable skill that helps you innovate at work . Developing your brainstorming techniques leads to better collaboration and organizational growth, whether you’re thinking of team bonding activities or troubleshooting a project roadblock. Related skills include benchmarking, diagnosis, and judgment to adequately assess situations and find solutions.
2. Communication
Becoming proficient at analysis is one thing, but you should also know how to communicate your findings to your audience — especially if they don’t have the same context or experience as you. Strong communication skills like public speaking , active listening , and storytelling can help you strategize the best ways to get the message out and collaborate with your team . And thinking critically about how to approach difficult conversations or persuade someone to see your point relies on these skills.
3. Creativity
You might not associate analysis with your creativity skills, but if you want to find an innovative approach to an age-old problem, you’ll need to combine data with creative thinking . This can help you establish effective metrics, spot trends others miss, and see why the most obvious answer to a problem isn’t always the best. Skills that can help you to think outside the box include strategic planning, collaboration, and integration.

4. Critical thinking
Processing information and determining what’s valuable requires critical thinking skills . They help you avoid the cognitive biases that prevent innovation and growth, allowing you to see things as they really are and understand their relevance. Essential skills to turn yourself into a critical thinker are comparative analysis, business intelligence, and inference.
5. Data analytics
When it comes to large volumes of information, a skilled analytical thinker can sort the beneficial from the irrelevant. Data skills give you the tools to identify trends and patterns and visualize outcomes before they impact an organization or project’s performance. Some of the most common skills you can develop are prescriptive analysis and return on investment (ROI) analysis.
6. Forecasting
Predicting future business, market, and cultural trends better positions your organization to take advantage of new opportunities or prepare for downturns. Business forecasting requires a mix of research skills and predictive abilities, like statistical analysis and data visualization, and the ability to present your findings clearly.
7. Logical reasoning
Becoming a logical thinker means learning to observe and analyze situations to draw rational and objective conclusions. With logic, you can evaluate available facts, identify patterns or correlations, and use them to improve decision-making outcomes. If you’re looking to improve in this area, consider developing inductive and deductive reasoning skills.
8. Problem-solving
Problem-solving appears in all facets of your life — not just work. Effectively finding solutions to any issue takes analysis and logic, and you also need to take initiative with clear action plans . To improve your problem-solving skills , invest in developing visualization , collaboration, and goal-setting skills.
9. Research
Knowing how to locate information is just as valuable as understanding what to do with it. With research skills, you’ll recognize and collect data relevant to the problem you’re trying to solve or the initiative you’re trying to start. You can improve these skills by learning about data collection techniques, accuracy evaluation, and metrics.

You don’t need to earn a degree in data science to develop these skills. All it takes is time, practice, and commitment. Everything from work experience to hobbies can help you learn new things and make progress. Try a few of these ideas and stick with the ones you enjoy:
1. Document your skill set
The next time you encounter a problem and need to find solutions, take time to assess your process. Ask yourself:
- What facts are you considering?
- Do you ask for help or research on your own? What are your sources of advice?
- What does your brainstorming process look like?
- How do you make and execute a final decision?
- Do you reflect on the outcomes of your choices to identify lessons and opportunities for improvement?
- Are there any mistakes you find yourself making repeatedly?
- What problems do you constantly solve easily?
These questions can give insight into your analytical strengths and weaknesses and point you toward opportunities for growth.
2. Take courses
Many online and in-person courses can expand your logical thinking and analysis skills. They don’t necessarily have to involve information sciences. Just choose something that trains your brain and fills in your skills gaps .
Consider studying philosophy to learn how to develop your arguments or public speaking to better communicate the results of your research. You could also work on your hard skills with tools like Microsoft Excel and learn how to crunch numbers effectively. Whatever you choose, you can explore different online courses or certification programs to upskill.
3. Analyze everything
Spend time consciously and critically evaluating everything — your surroundings, work processes, and even the way you interact with others. Integrating analysis into your day-to-day helps you practice. The analytical part of your brain is like a muscle, and the more you use it, the stronger it’ll become.
After reading a book, listening to a podcast, or watching a movie, take some time to analyze what you watched. What were the messages? What did you learn? How was it delivered? Taking this approach to media will help you apply it to other scenarios in your life.
If you’re giving a presentation at work or helping your team upskill , use the opportunity to flex the analytical side of your brain. For effective teaching, you’ll need to process and analyze the topic thoroughly, which requires skills like logic and communication. You also have to analyze others’ learning styles and adjust your teachings to match them.
5. Play games
Spend your commute or weekends working on your skills in a way you enjoy. Try doing logic games like Sudoku and crossword puzzles during work breaks to foster critical thinking. And you can also integrate analytical skills into your existing hobbies. According to researcher Rakesh Ghildiyal, even team sports like soccer or hockey will stretch your capacity for analysis and strategic thinking .
6. Ask questions
According to a study in Tr ends in Cognitive Sciences, being curious improves cognitive function , helping you develop problem-solving skills, retention, and memory. Start speaking up in meetings and questioning the why and how of different decisions around you. You’ll think more critically and even help your team find breakthrough solutions they otherwise wouldn’t.
7.Seek advice
If you’re unsure what analytical skills you need to develop, try asking your manager or colleagues for feedback . Their outside perspective offers insight you might not find within, like patterns in. And if you’re looking for more consistent guidance, talking to a coach can help you spot weaknesses and set goals for the long term.
8. Pursue opportunities
Speak to your manager about participating in special projects that could help you develop and flex your skills. If you’d like to learn about SEO or market research, ask to shadow someone in the ecommerce or marketing departments. If you’re interested in business forecasting, talk to the data analysis team. Taking initiative demonstrates a desire to learn and shows leadership that you’re eager to grow.

Shining a spotlight on your analytical skills can help you at any stage of your job search. But since they take many forms, it’s best to be specific and show potential employers exactly why and how they make you a better candidate. Here are a few ways you can showcase them to the fullest:
1. In your cover letter
Your cover letter crafts a narrative around your skills and work experience. Use it to tell a story about how you put your analytical skills to use to solve a problem or improve workflow. Make sure to include concrete details to explain your thought process and solution — just keep it concise. Relate it back to the job description to show the hiring manager or recruiter you have the qualifications necessary to succeed.
2. On your resume
Depending on the type of resume you’re writing, there are many opportunities to convey your analytical skills to a potential employer. You could include them in sections like:
- Professional summary: If you decide to include a summary, describe yourself as an analytical person or a problem-solver, whichever relates best to the job posting.
- Work experience: Describe all the ways your skill for analysis has helped you perform or go above and beyond your responsibilities. Be sure to include specific details about challenges and outcomes related to the role you’re applying for to show how you use those skills.
- Skills section: If your resume has a skill-specific section, itemize the analytical abilities you’ve developed over your career. These can include hard analytical skills like predictive modeling as well as interpersonal skills like communication.
3. During a job interview
As part of your interview preparation , list your professional accomplishments and the skills that helped along the way, such as problem-solving, data literacy, or strategic thinking. Then, pull them together into confident answers to common interview questions using the STAR method to give the interviewer a holistic picture of your skill set.
Developing analytical skills isn’t only helpful in the workplace. It’s essential to life. You’ll use them daily whenever you read the news, make a major purchase, or interact with others. Learning to critically evaluate information can benefit your relationships and help you feel more confident in your decisions, whether you’re weighing your personal budget or making a big career change .
Understand Yourself Better:
Big 5 Personality Test
Elizabeth Perry, ACC
Elizabeth Perry is a Coach Community Manager at BetterUp. She uses strategic engagement strategies to cultivate a learning community across a global network of Coaches through in-person and virtual experiences, technology-enabled platforms, and strategic coaching industry partnerships. With over 3 years of coaching experience and a certification in transformative leadership and life coaching from Sofia University, Elizabeth leverages transpersonal psychology expertise to help coaches and clients gain awareness of their behavioral and thought patterns, discover their purpose and passions, and elevate their potential. She is a lifelong student of psychology, personal growth, and human potential as well as an ICF-certified ACC transpersonal life and leadership Coach.
20 examples of development opportunities that can level up your career
A roadmap for career development: how to set your course, create a networking plan in 7 easy steps, what business acumen is and 9 ways to develop it, discover how to get noticed by upper management at work, are you being passed over for a promotion here’s what to do, 8 examples for setting professional development goals at work, how to pursue jobs versus careers to achieve different goals, professional development is for everyone (we’re looking at you), how to develop critical thinking skills, why we're facing a crisis of imagination, and how to overcome it, 10 essential business skills that make an impact on your career, what are hard skills & examples for your resume, use a personal swot analysis to discover your strengths and weaknesses, 17 essential transferable skills to boost your job search, critical thinking is the one skillset you can't afford not to master, what are metacognitive skills examples in everyday life, stay connected with betterup, get our newsletter, event invites, plus product insights and research..
3100 E 5th Street, Suite 350 Austin, TX 78702
- Platform Overview
- Integrations
- Powered by AI
- BetterUp Lead™
- BetterUp Manage™
- BetterUp Care®
- Sales Performance
- Diversity & Inclusion
- Case Studies
- Why BetterUp?
- About Coaching
- Find your Coach
- Career Coaching
- Communication Coaching
- Personal Coaching
- News and Press
- Leadership Team
- Become a BetterUp Coach
- BetterUp Labs
- Center for Purpose & Performance
- Leadership Training
- Business Coaching
- Contact Support
- Contact Sales
- Privacy Policy
- Acceptable Use Policy
- Trust & Security
- Cookie Preferences
Home › Study Tips › Research Skills: What They Are and How They Benefit You
Research Skills: What They Are and How They Benefit You
- Published May 23, 2024
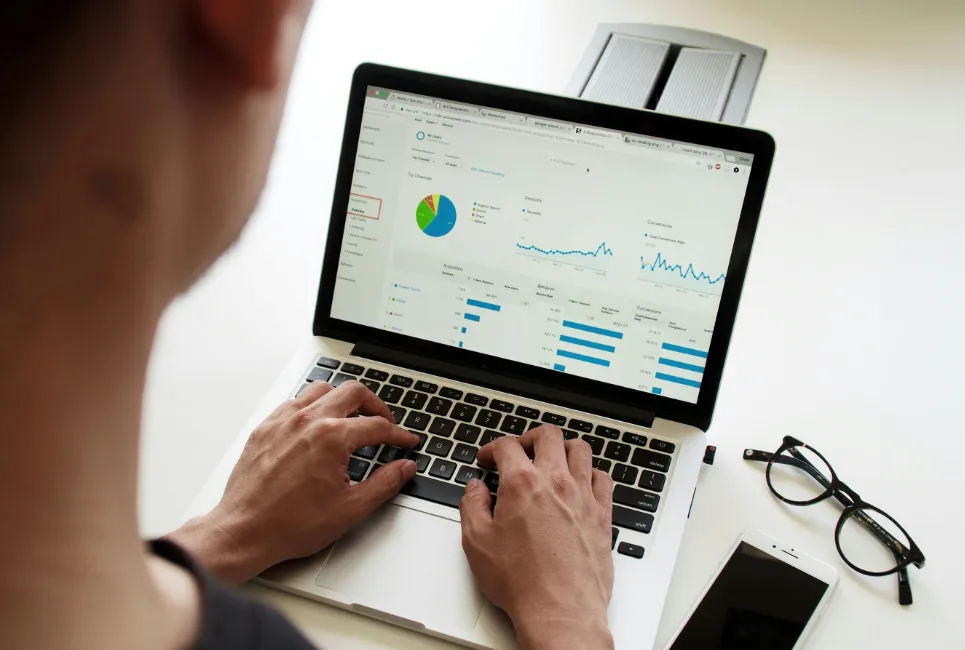
Research skills give you the ability to gather relevant information from different sources and analyse it critically in order to develop a comprehensive understanding of a subject. Thus, research skills are fundamental to academic success.
Developing these skills will improve your studies, helping you understand subjects better and positioning you for academic success.
That said, how can you develop important research skills? This will explore what research skills are, identify the core ones, and explain how you can develop them.
What Are Research Skills?
Research skills are a set of abilities that allow individuals to find and gather reliable information and then evaluate the information to find answers to questions.
Good research skills are important in academic settings, as finding and critically evaluating relevant information can help you gain a deeper understanding of a subject.
These skills are also important in professional and personal settings. When you graduate and are working in a professional capacity, you’ll often need to analyse sets of data to identify issues and determine how to solve them.
In personal contexts, you’ll always need to assess relevant information to make an informed decision. Whether you’re deciding on a major purchase, choosing a healthcare provider, or planning to make an investment, you’ll need to evaluate options to ensure better decision outcomes.
Different Types of Research Skills
Research skills are categorised into different sub-skills. The most common types are:
Quantitative Skills
Quantitative skills refer to the ability to work with numerical data and perform mathematical and statistical analyses to extract meaningful insights and draw conclusions.
When you have quantitative skills, you’ll be able to apply mathematical concepts and operations in research design and data analysis.
You’ll also be proficient in using statistical methods to analyse data and interpreting numerical data to draw meaningful conclusions.
Analytical Skills
Analytical skills refer to the ability to gather data, evaluate it, and draw sound conclusions. When you have analytical skills, you’ll be able to systematically analyse information to reach a reasonable conclusion.
Analytical skills are important in problem-solving. They help you to break down complex problems into more manageable components, think critically about the information at hand, analyse root causes, and develop effective solutions.
Qualitative Skills
Qualitative skills refer to the ability to collect, analyse, and interpret non-numerical data. When you have qualitative skills, you’ll be proficient in observation, interviewing, and other methods for collecting qualitative research data.
You’ll also be able to analyse non-numerical data, such as documents and images, to identify themes, patterns, and meanings.
Research Skills Examples
The core research skills you need for success in academic, professional, and personal contexts include:
Data Collection
Data is at the centre of every research, as data is what you assess to find the answers you seek. Thus, research starts with collecting relevant data.
Depending on the research, there are two broad categories of data you can collect: primary and secondary.
Primary data is generated by the researcher, like data from interviews, observations, or experiments. Secondary data is pre-existing data obtained from different existing databases, like published literature, government reports, etc.
Thus, data collection is more than gathering information from the Internet. Depending on the research, it can require more advanced skills for conducting experiments to generate your own data.
Source Evaluation
When doing research on any subject (especially when using the Internet), you’ll be amazed at the volume of information you’ll find. And a lot is pure garbage that can compromise your research work.
Thus, an important research skill is being able to dig through the garbage to get to the real facts. This is where source evaluation comes in!
Good research skills call for being able to identify biases, assess the authority of the author, and determine the accuracy of information before using it.
Time Management Skills
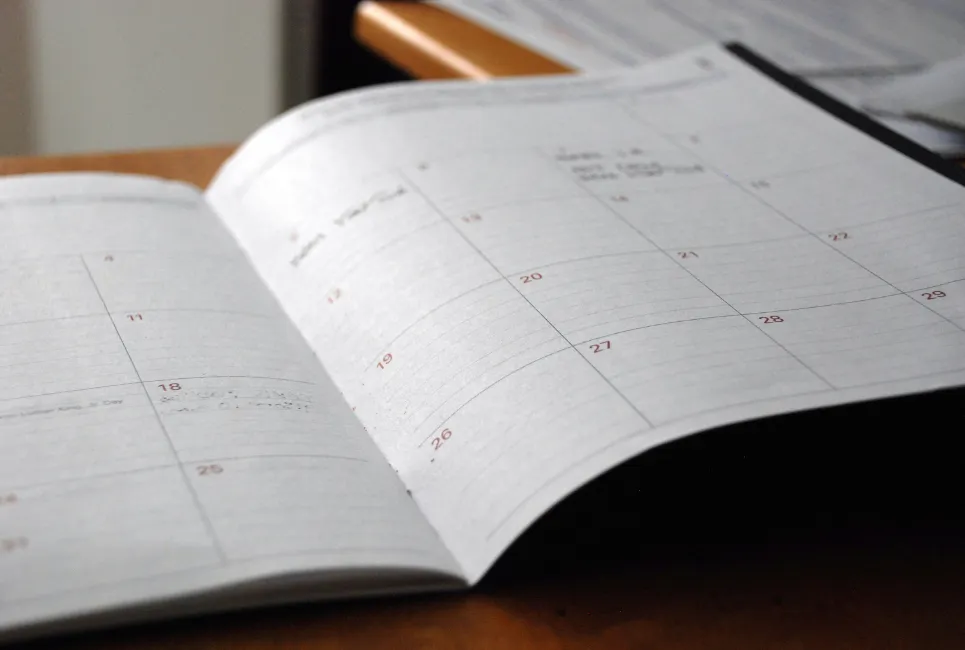
Have you ever felt that there is not enough time in a day for all that you need to do? When you already have so much to do, adding research can be overwhelming.
Good time management skills can help you find the time to do all you need to do, including relevant research work, making it an essential research skill.
Time management allows you to plan and manage your research project effectively. It includes breaking down research tasks into more manageable parts, setting priorities, and allocating time to the different stages of the research.
Communication Skills
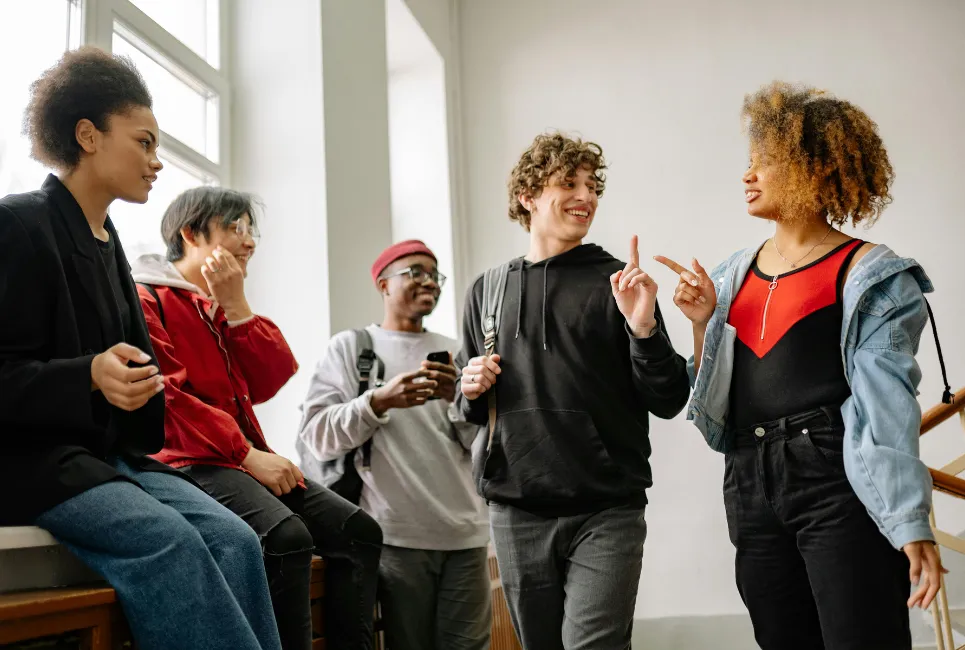
Communication is an important aspect of every research, as it aids in data collection and sharing research findings.
Important communication skills needed in research include active listening, active speaking, interviewing, report writing, data visualisation, and presentation, etc.
For example, when research involves collecting primary data via interviews, you must have sound speaking and listening skills.
When you conclude the research and need to share findings, you’ll need to write a research report and present key findings in easy-to-understand formats like charts.
Attention to Detail
Attention to detail is the ability to achieve thoroughness and accuracy when doing something. It requires focusing on every aspect of the tasks, even small ones.
Anything you miss during your research will affect the quality of your research findings. Thus, the ability to pay close attention to details is an important research skill.
You need attention to detail at every stage of the research process. During data collection, it helps you ensure reliable data.
During analysis, it reduces the risk of error to ensure your results are trustworthy. It also helps you express findings precisely to minimise ambiguity and facilitate understanding.
Note-Taking
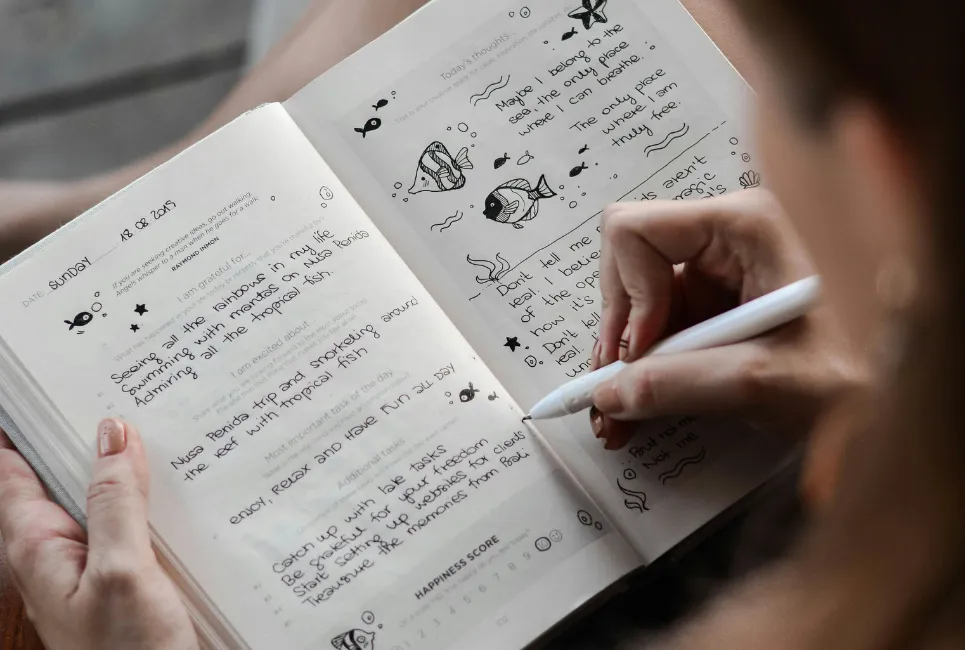
Note-taking is exactly what it sounds like—writing down key information during the research process.
Remember that research involves sifting through and taking in a lot of information. It’s impossible to take in all the information and recall it from memory. This is where note-taking comes in!
Note-taking helps you capture key information, making it easier to remember and utilise for the research later. It also involves writing down where to look for important information.
Critical Thinking
Critical thinking is the ability to think rationally and synthesise information in a thoughtful way. It is an important skill needed in virtually all stages of the research process.
For example, when collecting data, you need critical thinking to assess the quality and relevance of data. It can help you identify gaps in data to formulate your research question and hypothesis.
It can also help you to identify patterns and make reasonable connections when interpreting research findings.
Data Analysis
Data may not mean anything until you analyse it qualitatively or quantitatively (using techniques like Excel or SPSS). For this reason, data analysis analysis is an important research skill.
Researchers need to be able to build hypotheses and test these using appropriate research techniques. This helps to draw meaningful conclusions and gain a comprehensive understanding of research data.
Problem-Solving Skills
Research often involves addressing specific questions and solving problems. For this reason, problem-solving skills are important skills when conducting research.
Problem-solving skills refer to the ability to identify, analyse, and solve problems effectively.
With problem-solving skills, you’ll be able to assess a situation, consider various solutions, and choose the most appropriate course of action toward finding a solution.
Benefits of Research Skills
Research skills have many benefits, including:
Enhances Critical Thinking
Research skills and critical thinking are intertwined such that developing one enhances the other.
Research requires people to question assumptions, evaluate evidence, analyse information, and draw conclusions. These activities require you to think critically about the information at hand. Hence, engaging in research enhances critical thinking.
Develops Problem-Solving Skills
Research helps you acquire a set of critical skills that are directly transferable to problem-solving.
For example, research fosters creative thinking, as it often requires synthesising data from different sources and connecting different concepts. After developing creative thinking via research, you can apply the skill to generate innovative solutions in problem-solving situations.
Helps in Knowledge Acquisition
Engaging in research is a powerful way to acquire knowledge. Research involves exploring new ideas, and this helps you expand your breadth of knowledge.
It also involves applying research methods and methodologies. So, you’ll acquire knowledge about research methods, enhancing your ability to design and conduct studies in your higher education or professional life.
Why Are Research Skills Important?
Strong research skills offer numerous benefits, especially for students’ academic learning and development.
When you develop good research skills, you’ll reap great academic rewards that include:
In-Depth Understanding
Conducting research allows you to delve deep into specific topics, helping you gain a thorough understanding of the subject matter beyond what is covered in standard coursework.
Critical Thinking Development
Research involves critical evaluation of information and making informed decisions. This builds your ability to think critically.
This skill will not only help you solve academic problems better, but it’s also crucial to your personal and professional growth.
Encouragement of Independent Learning
Research encourages independent learning. When you engage in research, you seek answers independently. You take the initiative to find, retrieve, and evaluate information relevant to your research.
That helps you develop self-directed study habits. You’ll be able to take ownership of your education and actively seek out information for a better understanding of the subject matter.
Intellectual Curiosity Development
Research skills encourage intellectual curiosity and a love of learning, as they’ll make you explore topics you find intriguing or important. Thus, you’ll be more motivated to explore topics beyond the scope of your coursework.
Enhanced Communication Skills
Research helps you build better interpersonal skills as well as report-writing skills.
Research helps you sharpen your communication skills when you interact with research subjects during data collection. Communicating research findings to an audience also helps sharpen your presentation skills or report writing skills.
Assistance in Career Preparation
Many professions find people with good research skills. Whether you’ll pursue a career in academia, business, healthcare, or IT, being able to conduct research will make you a valuable asset.
So, researching skills for students prepares you for a successful career when you graduate.
Contribution to Personal Growth
Research also contributes to your personal growth. Know that research projects often come with setbacks, unexpected challenges, and moments of uncertainty. Navigating these difficulties helps you build resilience and confidence.
Acquisition of Time Management Skills
Research projects often come with deadlines. Such research projects force you to set goals, prioritise tasks, and manage your time effectively.
That helps you acquire important time management skills that you can use in other areas of academic life and your professional life when you graduate.
Ways to Improve Research Skills
The ways to improve your research skills involve a combination of learning and practice.
You should consider enrolling in research-related programmes, learning to use data analysis tools, practising summarising and synthesising information from multiple sources, collaborating with more experienced researchers, and more.
Looking to improve your research skills? Read our 11 ways to improve research skills article.
How Can I Learn Research Skills?
You can learn research skills using these simple three-point framework:
Clarifying the Objective
Start by articulating the purpose of your research. Identify the specific question you are trying to answer or the problem you are aiming to solve.
Then, determine the scope of your research to help you stay focused and avoid going after irrelevant information.
Cross-Referencing Sources
The next step is to search for existing research on the topic. Use academic databases, journals, books, and reputable online sources.
It’s important to compare information from multiple sources, taking note of consensus among studies and any conflicting findings.
Also, check the credibility of each source by looking at the author’s expertise, information recency, and reputation of the publication’s outlet.
Organise the Research
Develop a note-taking system to document key findings as you search for existing research. Create a research outline, then arrange your ideas logically, ensuring that each section aligns with your research objective.
As you progress, be adaptable. Be open to refining your research plan as new understanding evolves.
Enrolling in online research programmes can also help you build strong research skills. These programmes combine subject study with academic research project development to help you hone the skills you need to succeed in higher education.
Immerse Education is a foremost provider of online research programmes.
Acquire Research Skills with Immerse Education
Research skills are essential to academic success. They help you gain an in-depth understanding of subjects, enhance your critical thinking and problem-solving skills, improve your time management skills, and more.
In addition to boosting you academically, they contribute to your personal growth and prepare you for a successful professional career.
Thankfully, you can learn research skills and reap these benefits. There are different ways to improve research skills, including enrolling in research-based programmes. This is why you need Immerse Education!
Immerse Education provides participants aged 13-18 with unparalleled educational experience. All our programmes are designed by tutors from top global universities and help prepare participants for future success.
Our online research programme expertly combines subject study with academic research projects to help you gain subject matter knowledge and the important research skills you need to succeed in higher education. With one-on-one tutoring or group sessions from an expert academic from Oxford or Cambridge University and a flexible delivery mode, the programme is designed for you to succeed. Subsequently, enrolling in our accredited Online Research Programme will award students with 8 UCAS points upon completion.
- I'm a Parent
- I'm a Student
- First Name *
- Last Name *
- Which subjects interest you? (Optional) Architecture Artificial Intelligence Banking and Finance Biology Biotechnology Business Management Chemistry Coding Computer Science Computer Science and Artificial Intelligence Creative Writing Creative Writing and Film Criminology Data Science and Analytics Earth Science Economics Encryption and Cybersecurity Engineering English Literature Entrepreneurship Fashion and Design Female Future Leaders Film Studies Fine Arts Global Society and Sustainability Health and Biotechnology History International Relations Law Marketing and Entertainment Mathematics Medicine Medicine and Health Sciences Nanotechnology Natural Sciences Philosophy Philosophy Politics and Economics Physics Psychology Software Development and AI Software Development and Gaming Veterinary Studies Online Research Programme
Secure priority enrolment for our new summer school location with a small refundable deposit.
" * " indicates required fields
Receive priority enrolment for new summer school locations by registering your interest below.
Our programme consultant will contact you to talk about your options.
- Family Name *
- Phone Number
- Yes. See Privacy Policy.
Subject is unavailable at location
You have selected a subject that is not available at the location that you have previously chosen.
The location filter has been reset, and you are now able to search for all the courses where we offer the subject.
Resume Worded | Resume Skills
Skill profile, research analyst, improve your resume's success rate by using these research analyst skills and keywords ..
- Hard Skills and Keywords for your Research Analyst Resume
- ATS Scan : Compare Your Resume To These Skills
- Sample Resume Templates
- How To Add Skills
- Soft Skills for Research Analyst Roles
- Research Analyst More Resume Templates
Resume Skills and Keywords from Related Jobs
Browse skills from similar jobs, frequently asked questions.
- 3. Effective Action Verbs for your Resume
Get a Free Resume Review
Looking for keywords for a specific job search for your job title here., © 2024 resume worded. all rights reserved., research analyst resume keywords and skills (hard skills).
Here are the keywords and skills that appear most frequently on recent Research Analyst job postings. In other words, these are the most sought after skills by recruiters and hiring managers. Go to Sample Templates ↓ below to see how to include them on your resume. Remember that every job is different. Instead of including all keywords on your resume, identify those that are most relevant to the job you're applying to. Use the free Targeted Resume tool to help with this.
- Microsoft Access
- Geographic Information Systems (GIS)
- Data Analysis
- Project Management
- Quantum GIS
- Cartography
- Find out what your resume's missing
- Market Research
- ArcGIS Products
- Project Planning
- Engineering
- Sustainable Development
- R (Programming Language)
- Secondary Research
- Primary Research
- Lead Generation
- Qualitative Research
- Business Analysis
- Quantitative Research
- Financial Analysis
- Customer Relationship Management (CRM)
- Online Research
- Competitive Analysis
- Market Analysis
- Business Strategy
Resume Skills: Data Analysis
- Google Analytics
- Match your resume to these skills
Resume Skills: Research & Analysis
- Consumer Behavior Analysis
- Statistical Analysis
- Quantitative & qualitative research
- Competitive Intelligence
- Data Mining
- Predictive Analytics
Resume Skills: Programming
- Python (Pandas, NumPy, Scikit-learn)
Resume Skills: Research Tools
- Microsoft Office Suite
- Google Research
- Python (NumPy, Pandas)
Resume Skills: Statistics
- Descriptive Analysis
- Regression Analysis
- Correlation
- Statistical Hypothesis Testing
Resume Skills: Predictive Modelling
- Neural Networks
- Decision Trees
- Random Forest
Resume Skills: Tools & Frameworks
- SAS Analytics
Resume Skills: Marketing
- Content Marketing
- Social Media Advertising
- Direct Marketing
Resume Skills: Project Management
- Agile Methodology
- Stakeholder Communication
- Risk Management
Resume Skills: Statistical Modelling
- Hypothesis Testing
- Time Series Analysis
- Cluster Analysis
- Machine Learning
Resume Skills: Databases
- MS SQL Server
Resume Skills: Data Visualization
Resume skills: machine learning.
- Sci-kit Learn
Resume Skills: Operating Systems
Resume skills: research techniques.
- Quantitative Analysis
- Qualitative Analysis
- Data Collection
- Experimental Design
- Survey Design
- Clinical Trial Designs
Resume Skills: Biological Sciences
- Molecular Biology
- Cell Culture
- Genetic Engineering
- Immunology Techniques
Where on my resume do I add these buzzwords? Add keywords directly into your resume's work experiences , education or projects. Alternatively, you can also include a Skills section where you can list your technical skills in order of your proficiency. Only include these technical skills or keywords into your resume if you actually have experience with them.
Does your resume contain all the right skills? Paste in your resume in the AI Resume Scan ↓ section below and get an instant score.
Compare Your Resume To These Research Analyst Skills (ATS Scan)
Paste your resume below and our AI will identify which keywords are missing from your resume from the list above (and what you need to include). Including the right keywords will help you get past Applicant Tracking Systems (i.e. resume screeners) which may scan your resume for keywords to see if you're a match for the job.
Sample Research Analyst Resume Examples: How To Include These Skills
Add keywords directly into your resume's work experiences , education or skills section , like we've shown in the examples below. use the examples below as inspiration..
Where on my resume do I add these buzzwords? Add keywords directly into your resume's work experiences , education or projects. Only include these technical skills or keywords into your resume if you actually have experience with them.
How do I add skills to a Research Analyst resume?
Go through the Research Analyst posting you're applying to, and identify hard skills the company is looking for. For example, skills like Data Analysis, AutoCAD and Geographic Information Systems (GIS) are possible skills. These are skills you should try to include on your resume.
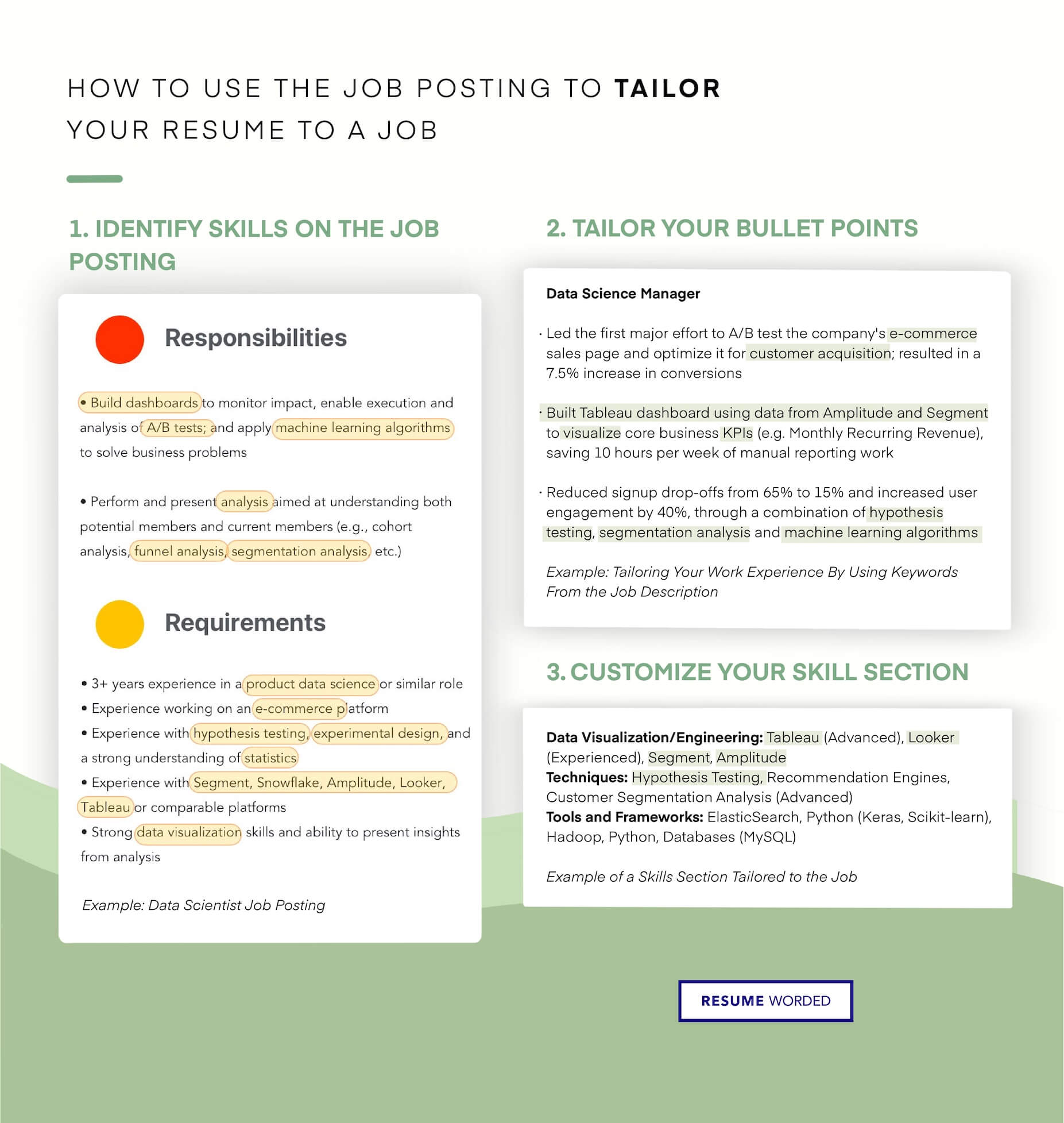
Add other common skills from your industry - such as Microsoft Access, Quantum GIS and Research - into your resume if they're relevant.

Incorporate skills - like SIG, Secondary Research and Online Research - into your work experience too. This shows hiring managers that you have practical experience with these tools, techniques and skills.
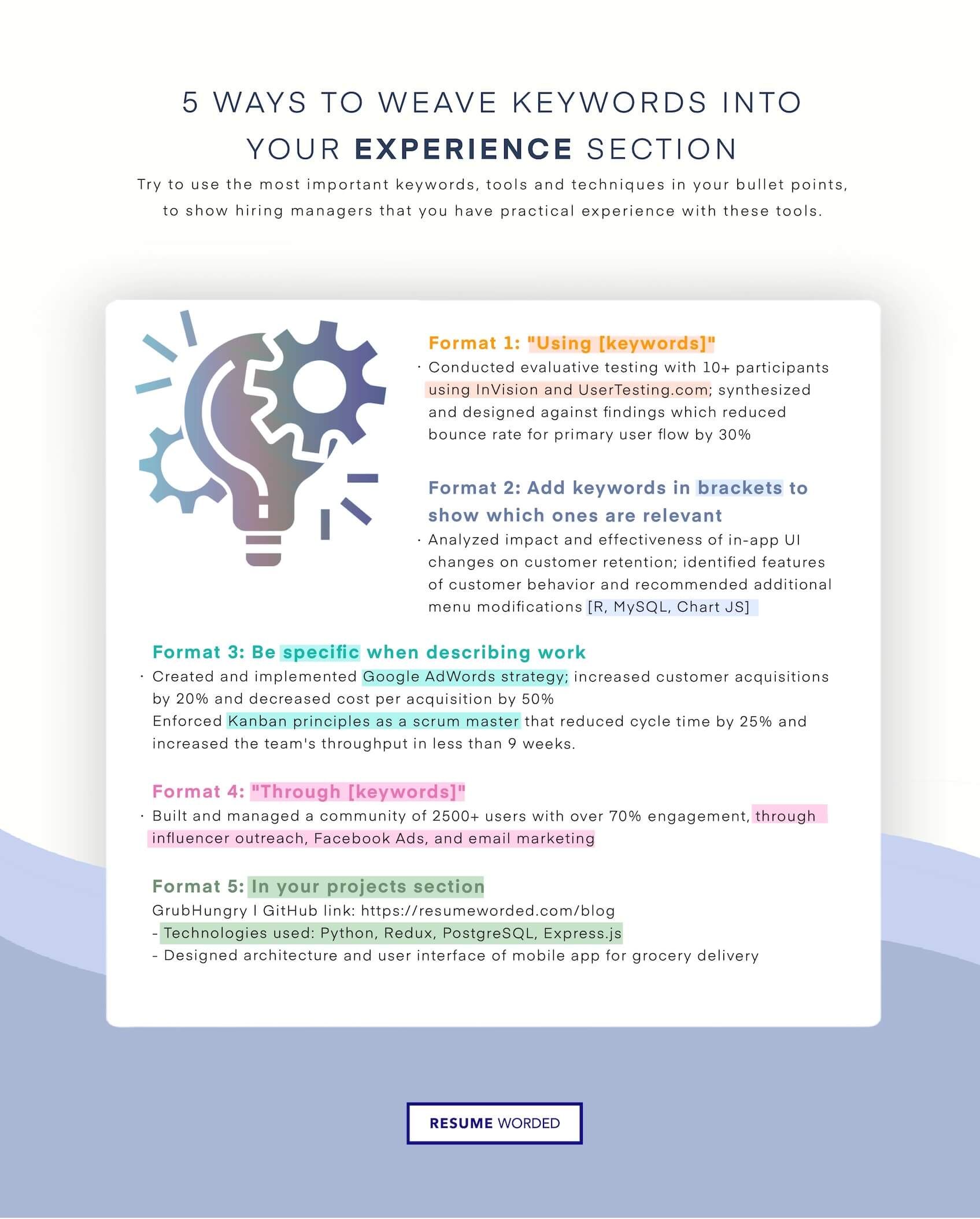
Recruiters want to know that you're the kind of person that can solve new and challenging problems. In your Research Analyst resume, highlight projects that involved problems that were technically complex or mattered to the company.
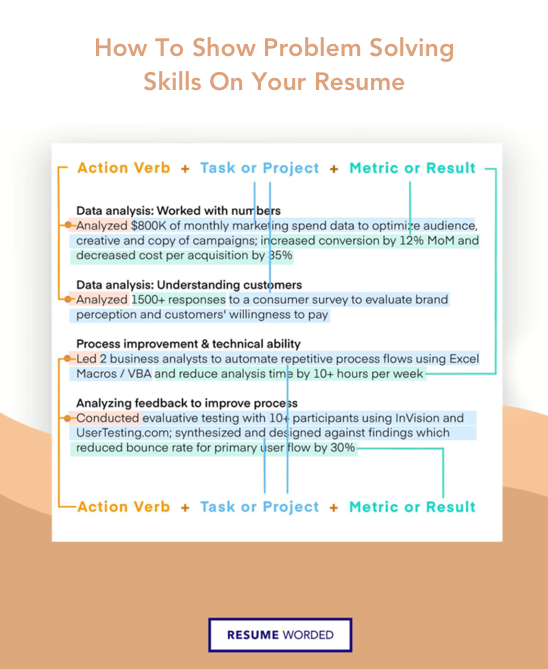
Analytical skills involve your ability to break down a problem and come up with effective solutions. On Research Analyst resumes, hiring managers want to see evidence of how you analyzed quantitative or qualitative data.
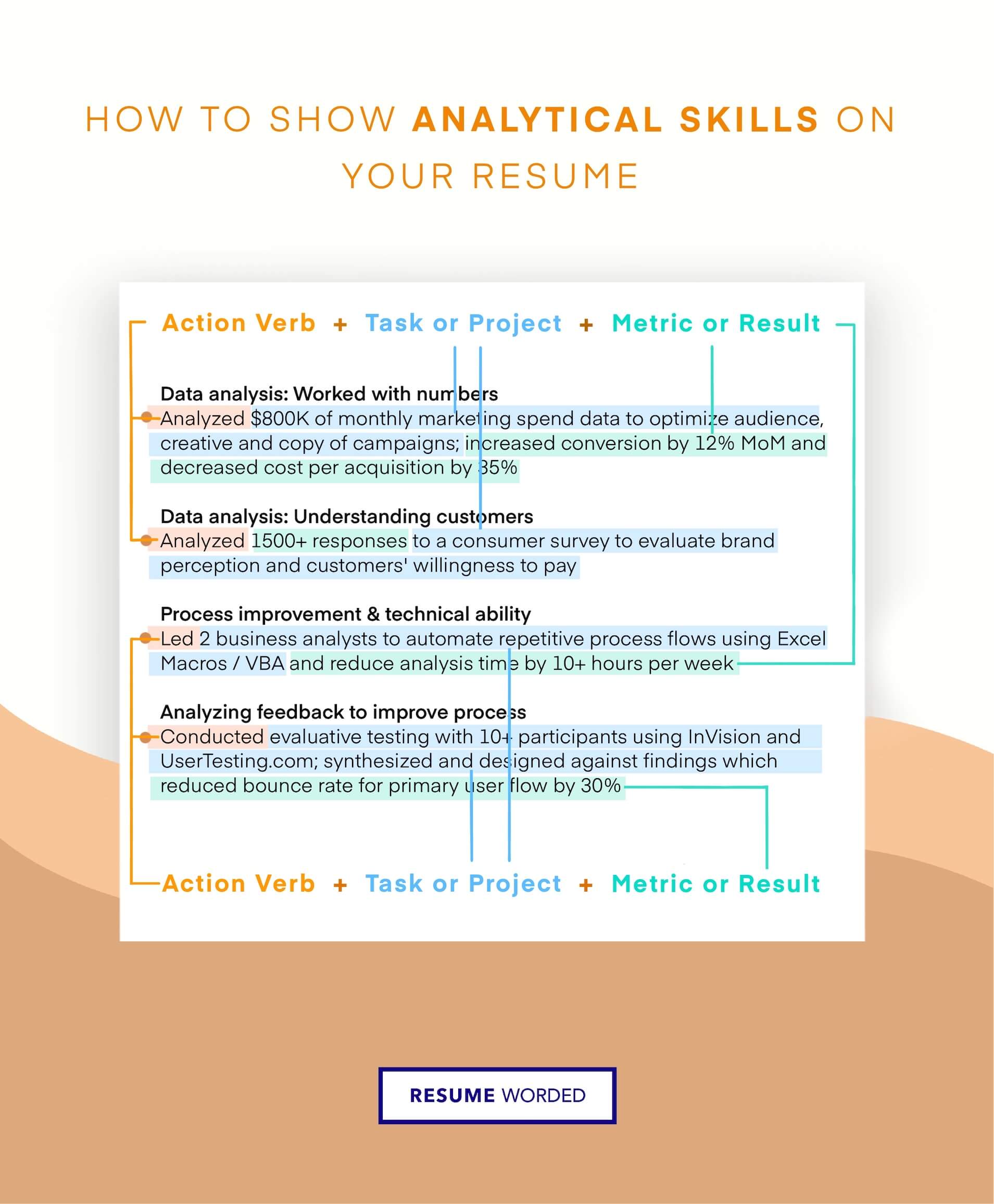
Try to add the exact job title, Research Analyst, somewhere into your resume to get past resume screeners. See the infographic for how to do this.
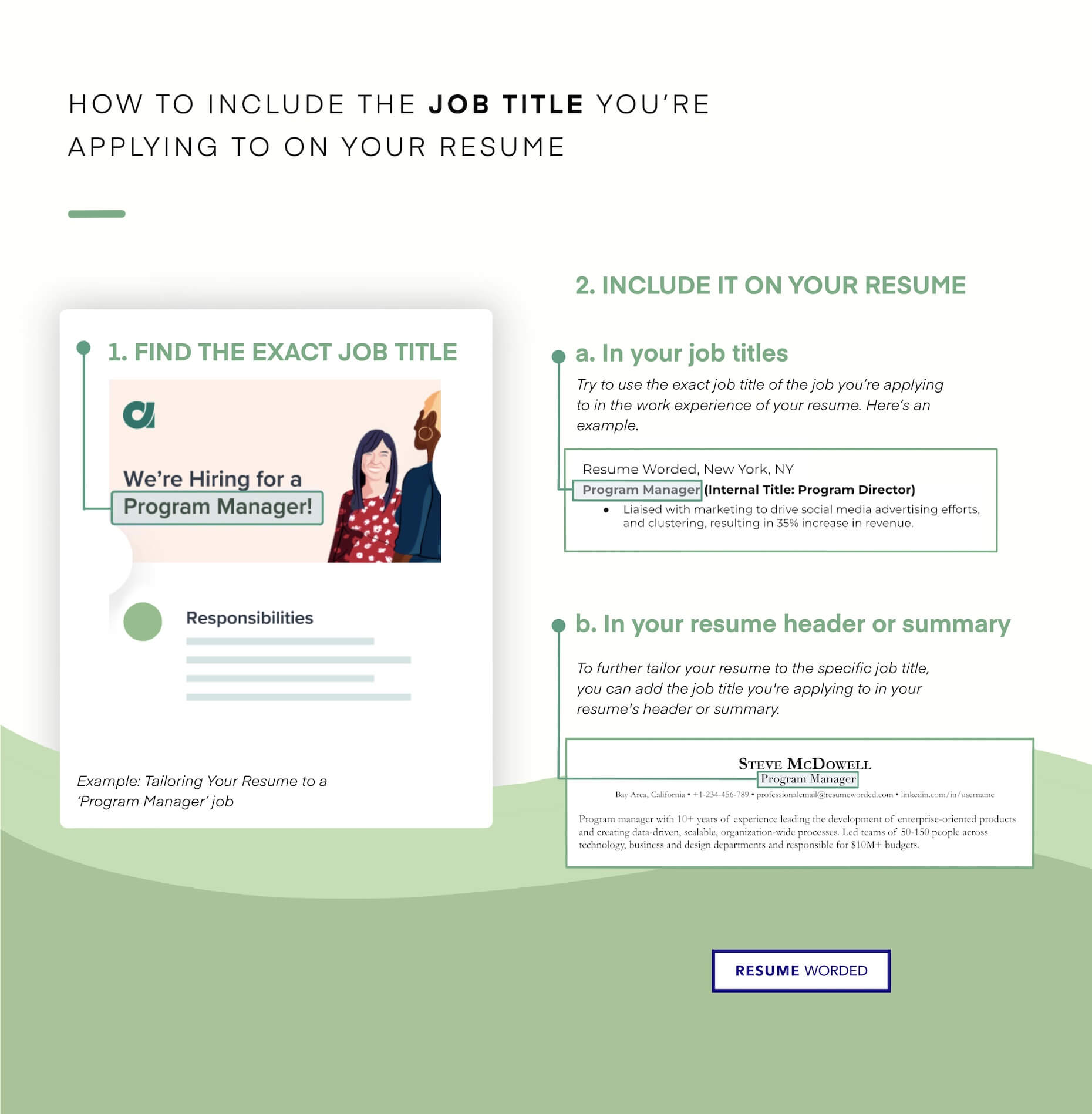
Word Cloud for Research Analyst Skills & Keywords
The following word cloud highlights the most popular keywords that appear on Research Analyst job descriptions. The bigger the word, the more frequently it shows up on employer's job postings. If you have experience with these keywords, include them on your resume.

Research Analyst Soft Skills
Here are common soft skills that appear on Research Analyst job postings. Unlike hard skills, which refer to tools, software or techniques, soft skills focus on character traits and interpersonal skills. Instead of listing these phrases on your resume, try to show them through your bullet points like in the examples below.
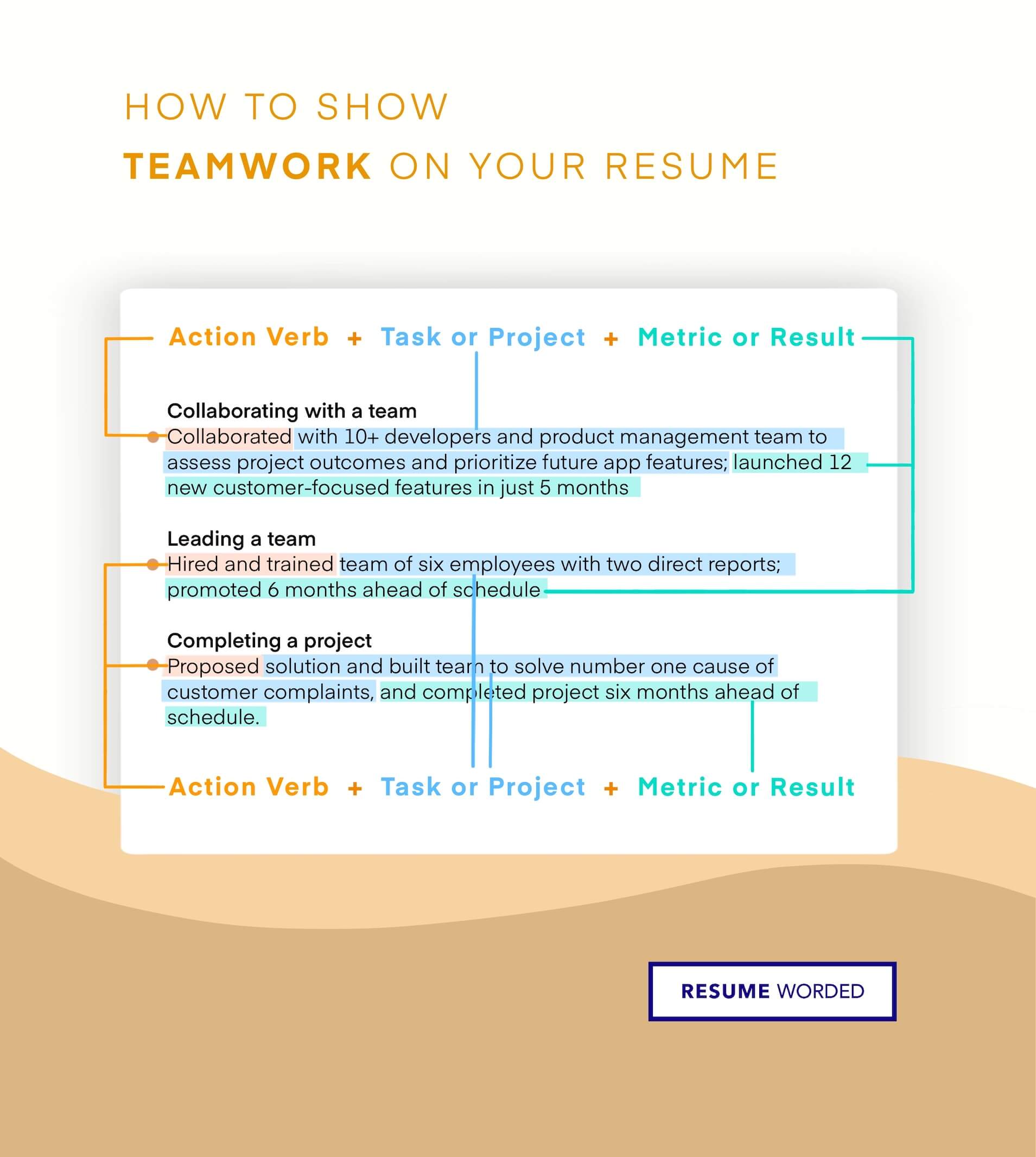
- Public Speaking
Tip: Do not list these words or phrases on your resume, and instead focus on the hard skills we described above.
We also found variations and further specializations to your job title. Browse through the related job titles to find additional keywords that you can include into your resume.
- Data Research
- Data Analytics
- Personal Development
- Spreadsheets
- C (Programming Language)
- Python (Programming Language)
- Data Visualization
- Statistical Data Analysis
- SAS Programming
- Data Science
- Microsoft SQL Server
- Operating Lease
- Quantitative Finance
- Financial Modeling
- Portfolio Optimization
- Statistical Modeling
- Quantitative Analytics
- Derivatives
- Fixed Income
- Portfolio Management
- Asset Management
- Investments
- Investment Research
- Equity Research
- Alternative Investments
- Investment Management
- Mutual Funds
- Marketing Strategy
- Customer Insight
- Digital Marketing
- Social Media Marketing
- Business Development
- Market Intelligence
- Market Access
- Market Risk
- Email Marketing
- Product Marketing
- Equity Valuation
- Fundamental Analysis
- Capital Markets
- Equity Research Analysis
- Corporate Finance
- DCF Valuation
- Financial Markets
- Investment Banking
- Technical Analysis
- Financial Statement Analysis
- Bloomberg Terminal
- Hedge Funds
Get your Resume Instantly Checked, For Free
Upload your resume and we'll spot the issues in it before an actual research analyst recruiter sees it. for free., research analyst resume templates.
Here are examples of proven resumes in related jobs and industries, approved by experienced hiring managers. Use them as inspiration when you're writing your own resume. You can even download and edit the resume template in Google Docs.
Resume Example Clinical Research Assistant
An effective Description of the templates...
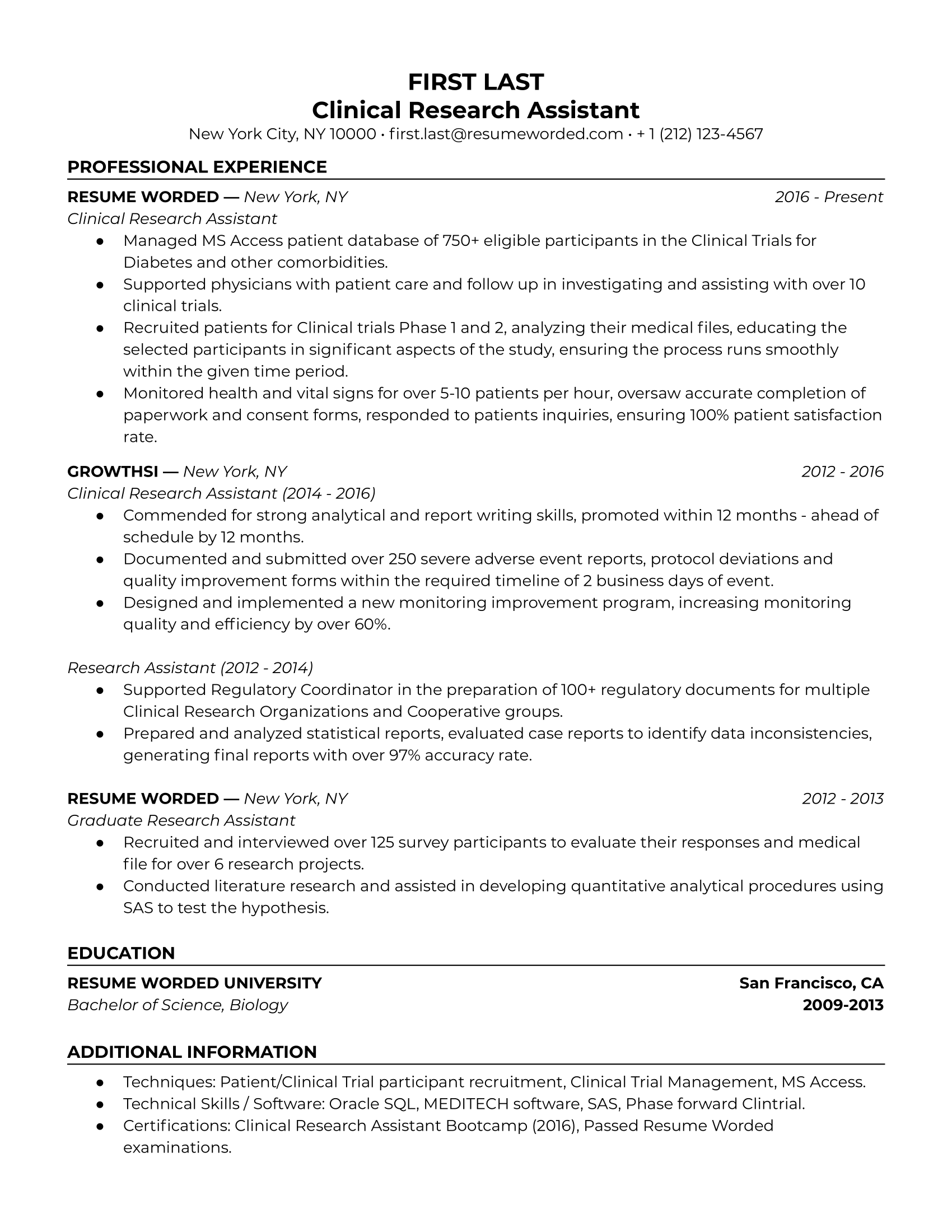
Download this resume template
Clinical research assistants work on clinical trials in hospitals and medical research centers. As a clinical research assistant, you’ll be assisting doctors and senior researchers by recruiting and enrolling research subjects, developing protocols, setting up and managing trials, collecting and analyzing data, and contributing to trial reports, regulatory authority applications, and grant writing. Ideally, you’ll need some experience or a degree in the field of study and clinical trial experience.
Tips on why this template works
focused on clinical research.
When applying for a more specialized position like clinical research assistant, you want to keep your resume as tightly focused as possible. That means prioritizing clinical research experience. It’s fine to include general research experience, but try to tailor your bullet points by including accomplishments relevant to clinical research, like preparing regulatory documents and conducting medical research.
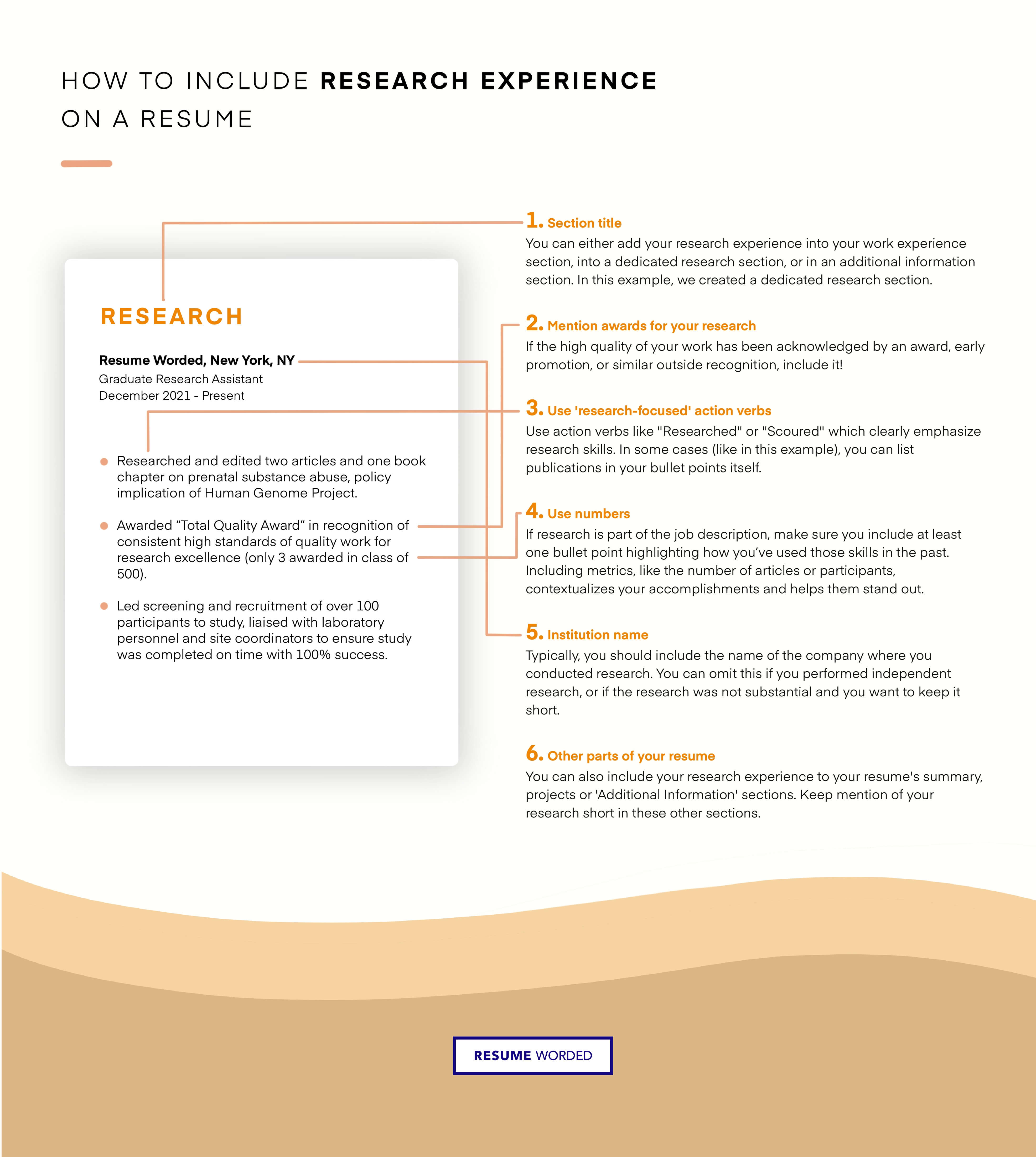
Good use of skills section to highlight research skills and tools
Jobs that require a lot of hard skills, like clinical research, may end up with a larger than usual skills section. Avoid making recruiters’ eyes glaze over by splitting it into subsections, like certifications, techniques, and technical skills. Make sure you’re exclusively listing hard skills — employers want to see things like relevant software skills and clinical trial experience.
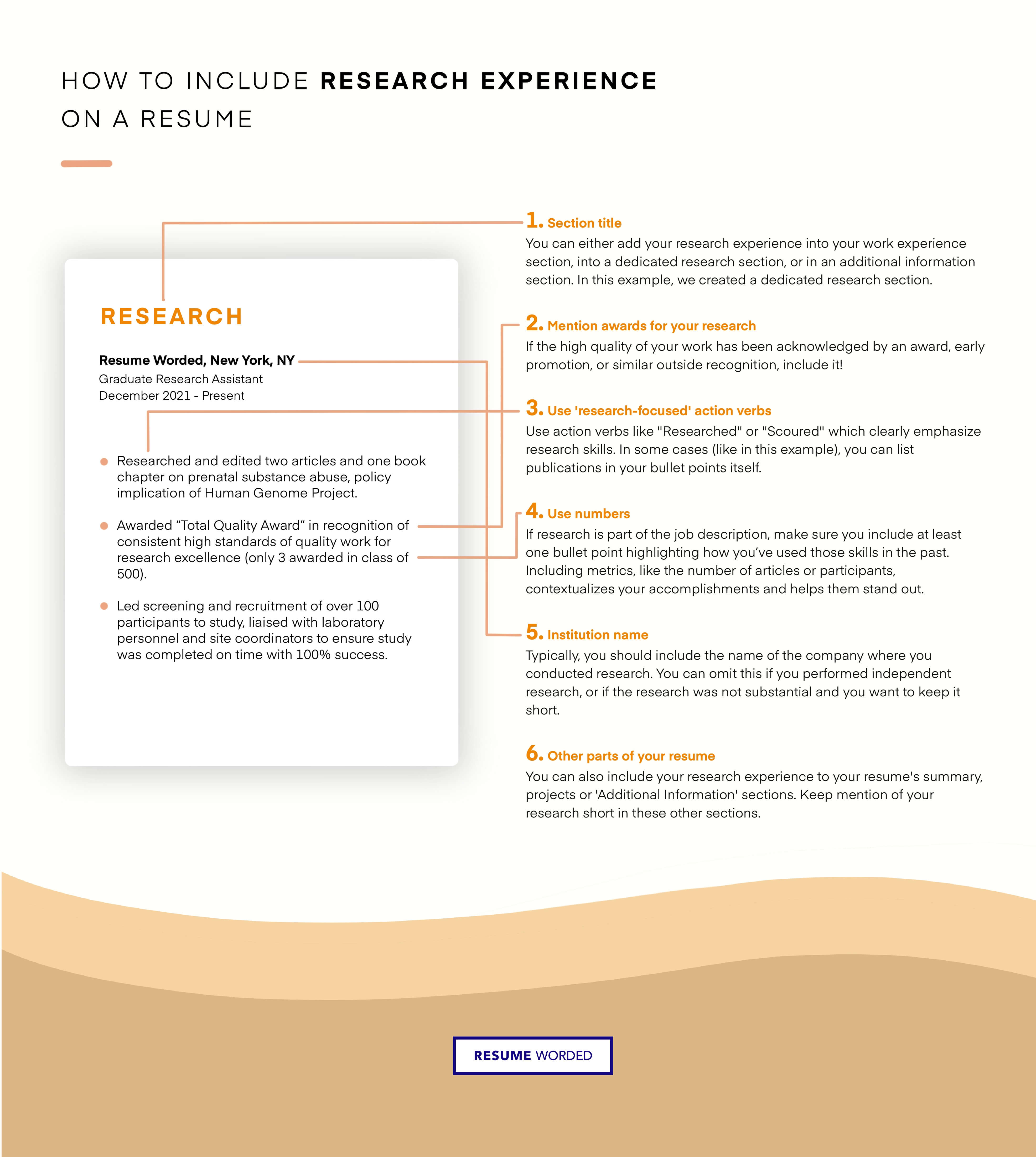
Resume Example Laboratory Research Assistant
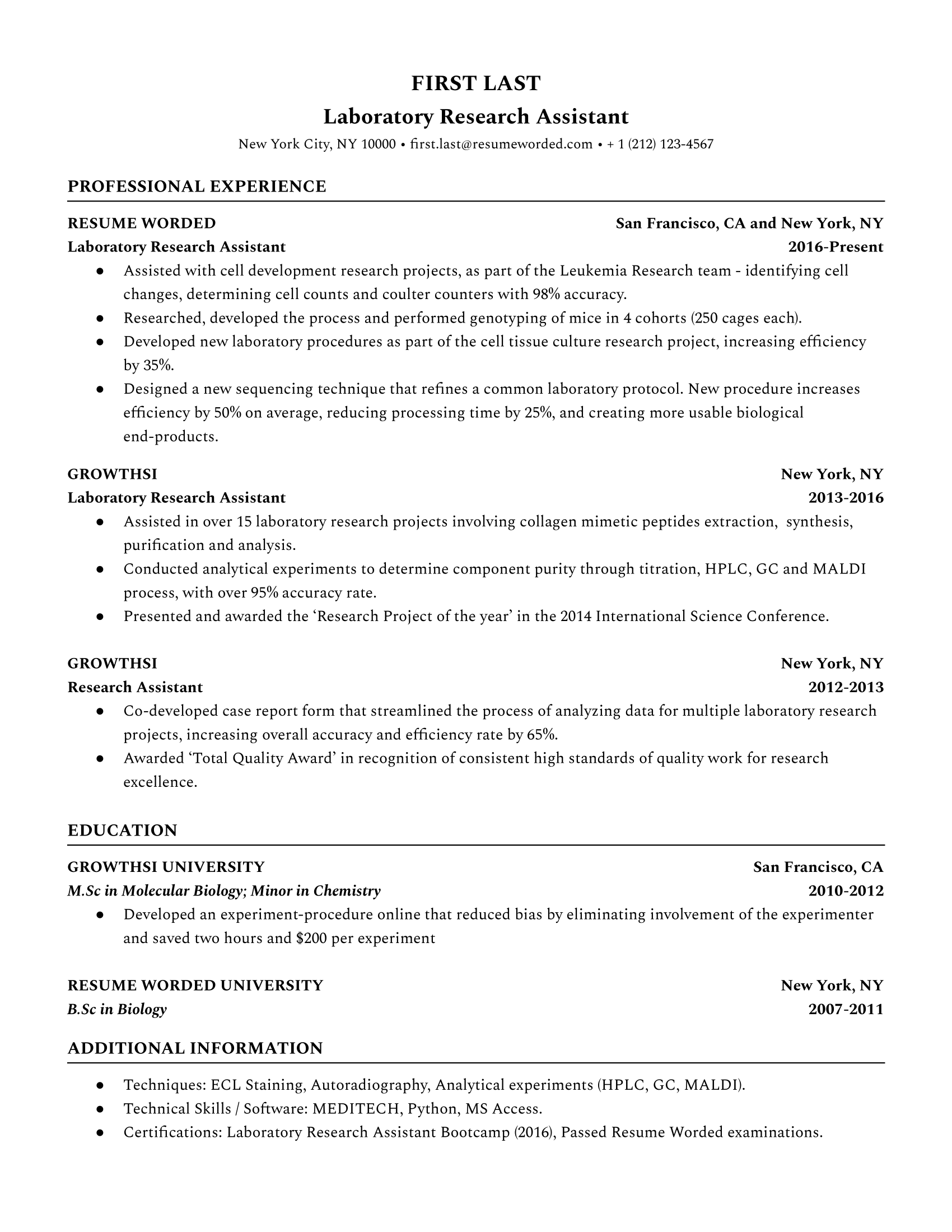
As a laboratory research assistant, you’ll be working in a laboratory environment to design projects, conduct research and experiments, write reports, perform general laboratory maintenance, and assist senior laboratory staff — so make sure to emphasize your technical skills. You may be working for a private laboratory, medical or research facility, or pharmaceutical company. Unlike standard research assistant positions, lab assistants typically work full-time, including weekend and evening shifts.
Bullet points feature strong action verbs
Every bullet point should start with a strong action verb. Remember, your resume is supposed to highlight your accomplishments, not simply list your job duties. As a laboratory research assistant, you’ll want to emphasize your scientific expertise. Verbs like Researched, Designed, and Developed all reflect the skills you’ll be expected to use on the job.
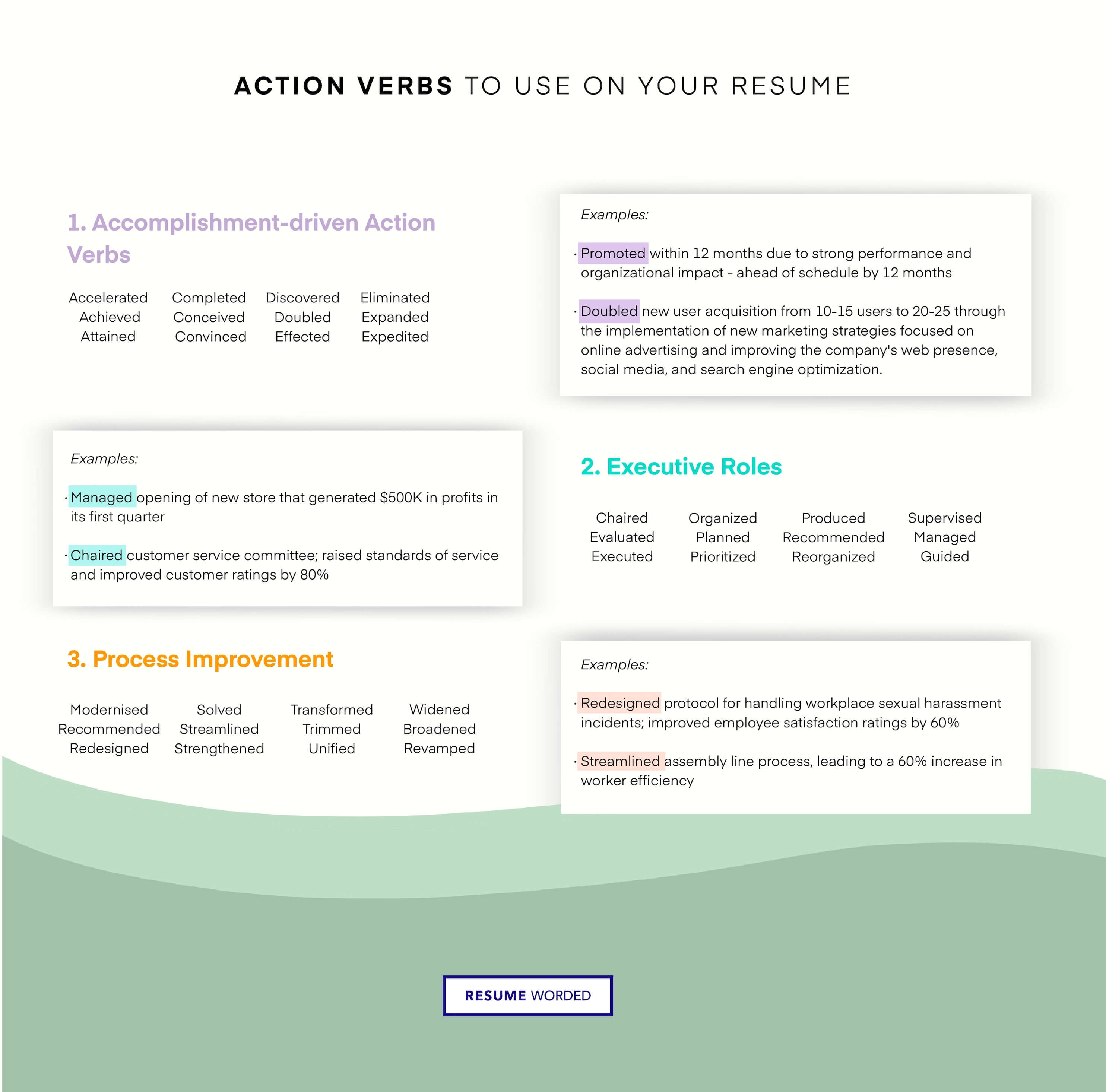
Uses hard numbers and metrics
Including metrics in your bullet points is the best way to demonstrate the outcome of your work. This doesn’t mean every bullet point needs to include numbers but try to quantify your accomplishments whenever possible. If you analyzed data with 98% accuracy, reduced processing times by 25%, or performed research on 1,000 subjects, say exactly that.
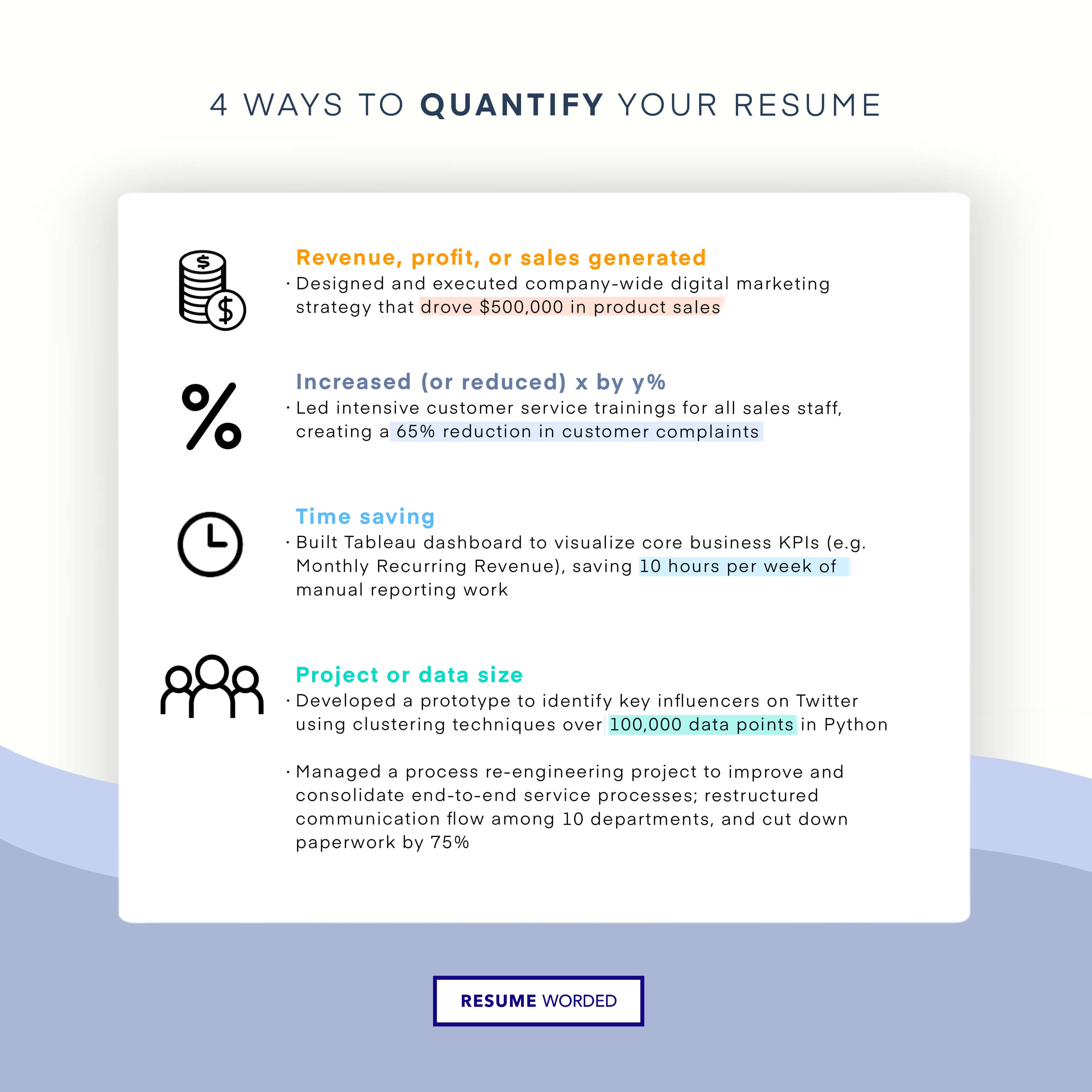
Resume Example Graduate Research Assistant
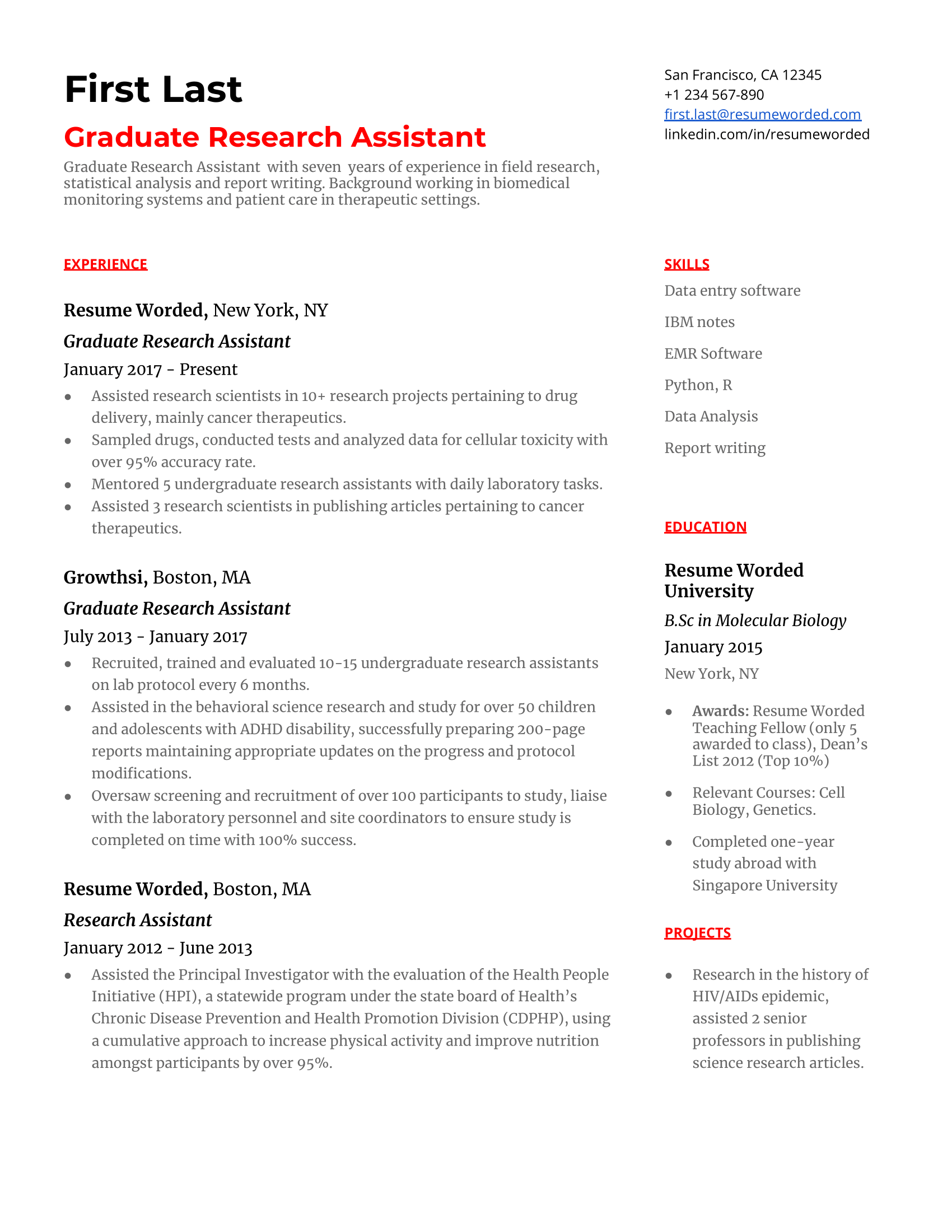
Graduate research assistants are able to work while they study, receiving a tuition reimbursement or stipend as well as valuable experience working in academia. As a graduate research assistant, you’ll have completed an undergraduate degree and be pursuing a master’s degree or PhD. You’ll generally be working closely with a supervisor to support their projects, including conducting research, analyzing data, writing reports, and supervising undergraduate research assistants.
Highlights university research projects
As a graduate research assistant, hiring managers won’t expect you to have extensive paid experience. If you’ve worked on previous research projects as a student, you can list these under your work experience or in an education or projects section. For greater impact, use action verbs and metrics to frame your accomplishments in an action-focused way.
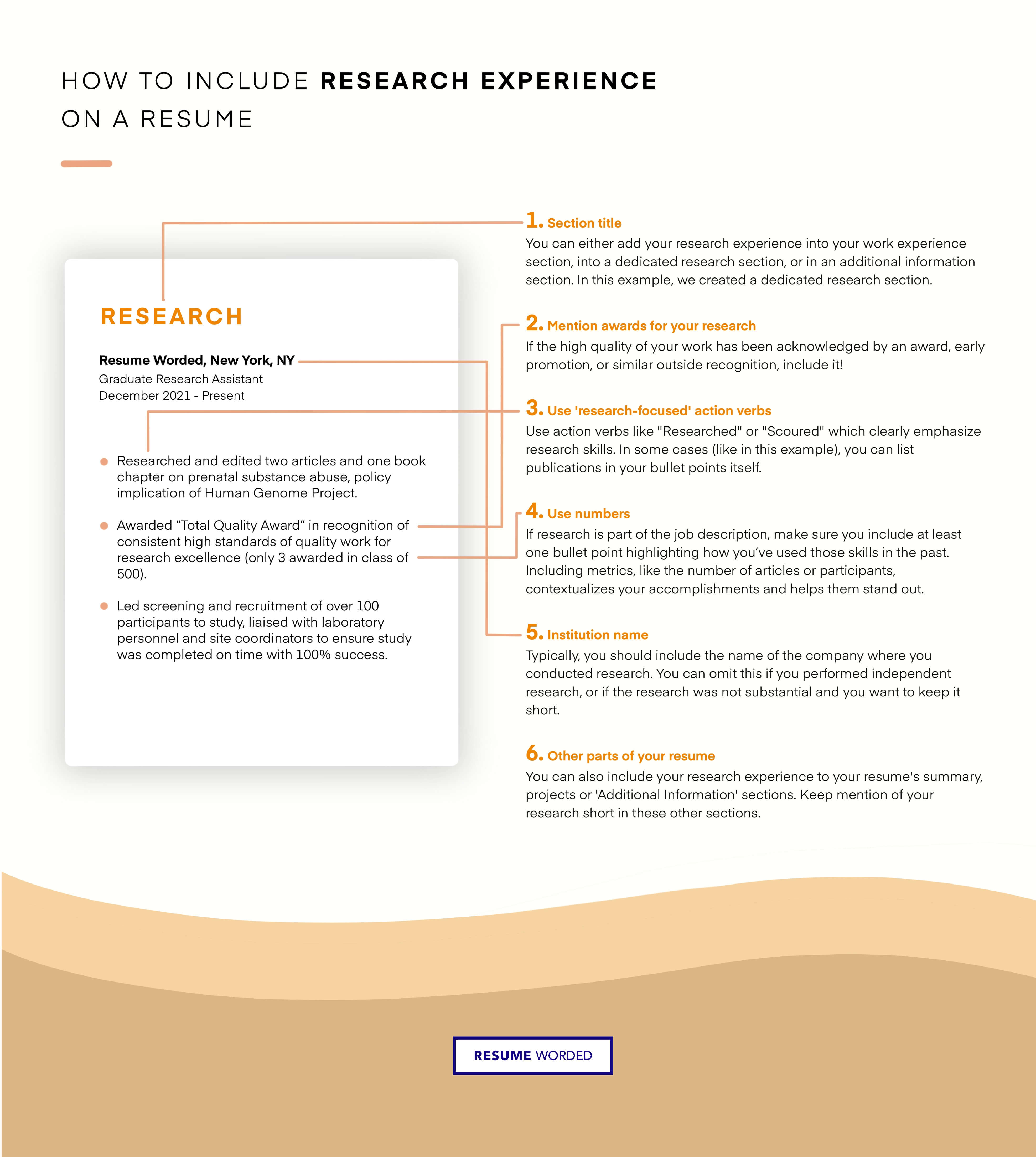
Includes a resume summary focused on graduate research interests and experience
Even as a graduate, you may have significant research experience, especially if you’ve been heavily involved in student research. You can highlight your skills and background with a short resume summary — no more than 100 words — explaining your years of experience, research or educational specialization, and 1-2 of your most impressive accomplishments.
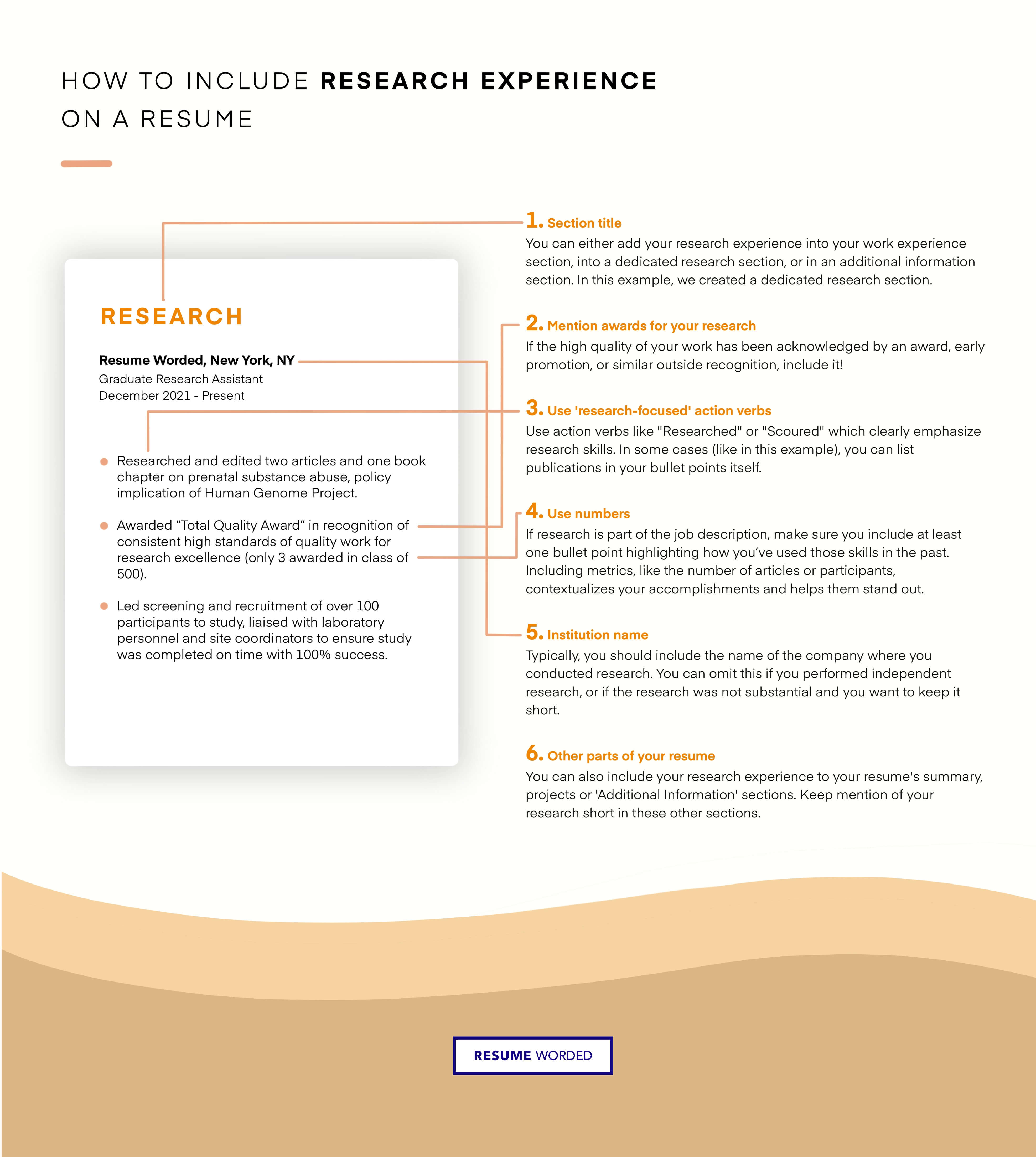
Resume Example Chemistry Research Student
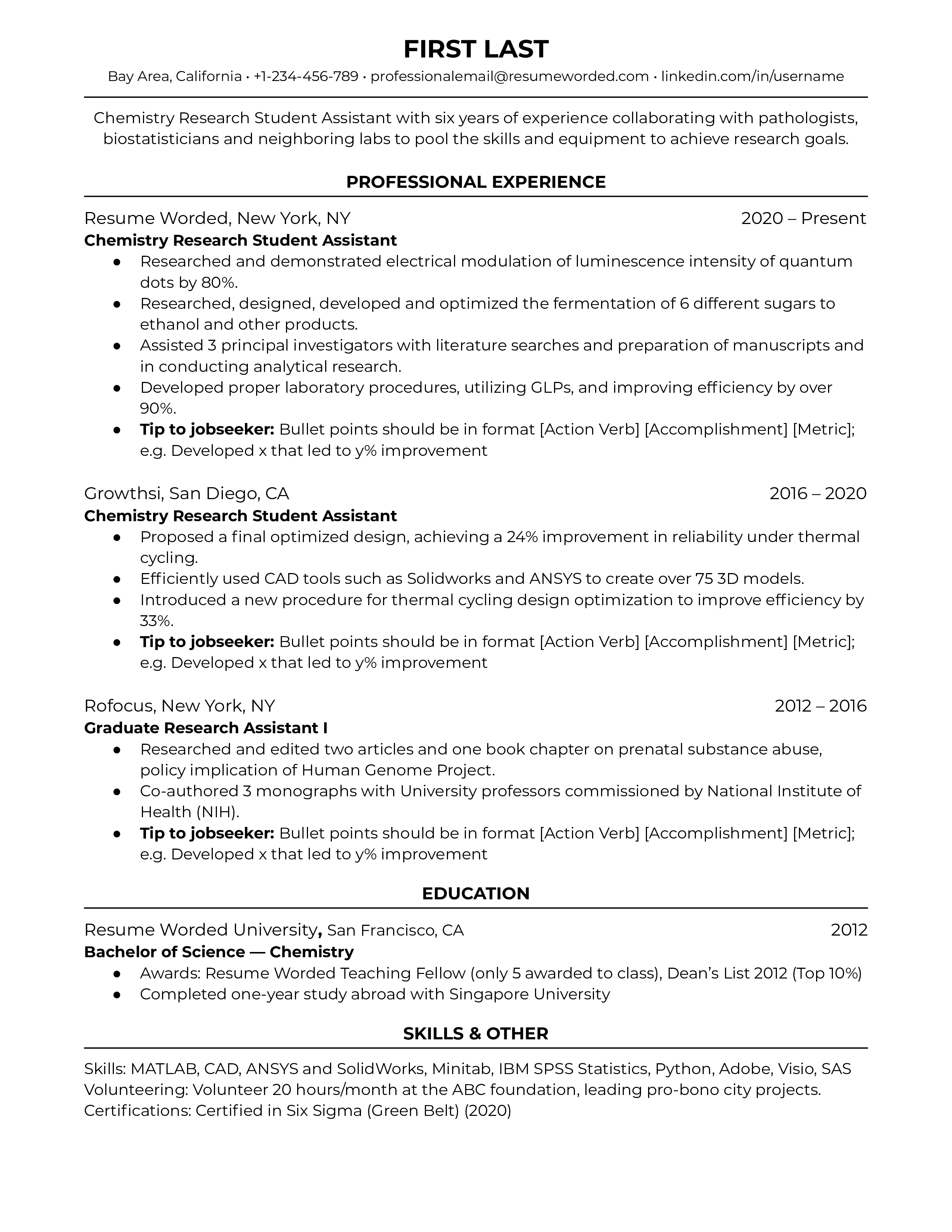
When applying to be a chemistry research student assistant, emphasize your past research experience and chemistry skills in your resume. Chemistry is a specific and precise discipline, and your resume should reflect these qualities. Aim to choose instances that detail your expertise in hands-on lab procedures or with relevant software, as opposed to simply listing out the responsibilities you were assigned. Use strong action verbs and be deliberate with what you include.
Emphasize hard skills with metrics relevant to chemistry
As mentioned above, chemistry is a precise discipline -- you’re often working in the lab with dangerous chemicals or complex equipment. That means that the employers reading your resume -- labs, government agencies, or academic institutions -- are looking for evidence of your experience and skills in those areas. Do your research to find what types of software the job posting notes, whether that’s MATLAB, Solidworks, or ANSYS. Emphasize the hard skills you’ve learned through your past experience with powerful action verbs, and highlight your achievements with quantifiable metrics.
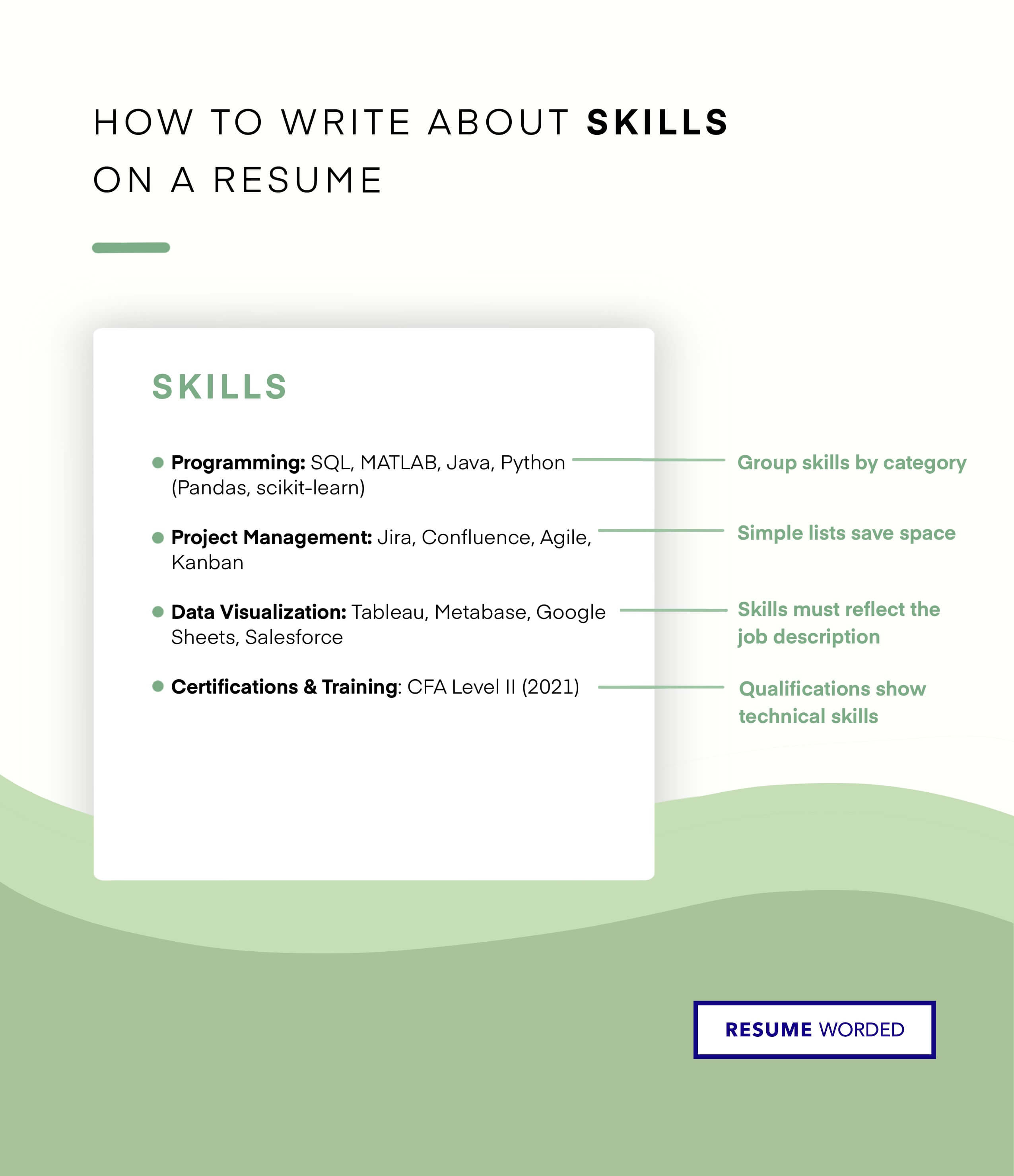
Concise, informational chemistry resume summary
This resume makes great use of a concise, information-packed elevator pitch that is well-written and to the point. Chemistry recruiting managers often don’t have time to carefully read through every detail of your resume, so a resume summary is a great way for them to get a high-level overview of your work history. When brainstorming what to put in your chemistry resume elevator pitch, include your personal strengths as a chemist, or even the types of people you’ve learned to work with (i.e., biostatisticians, pathologists, professors).
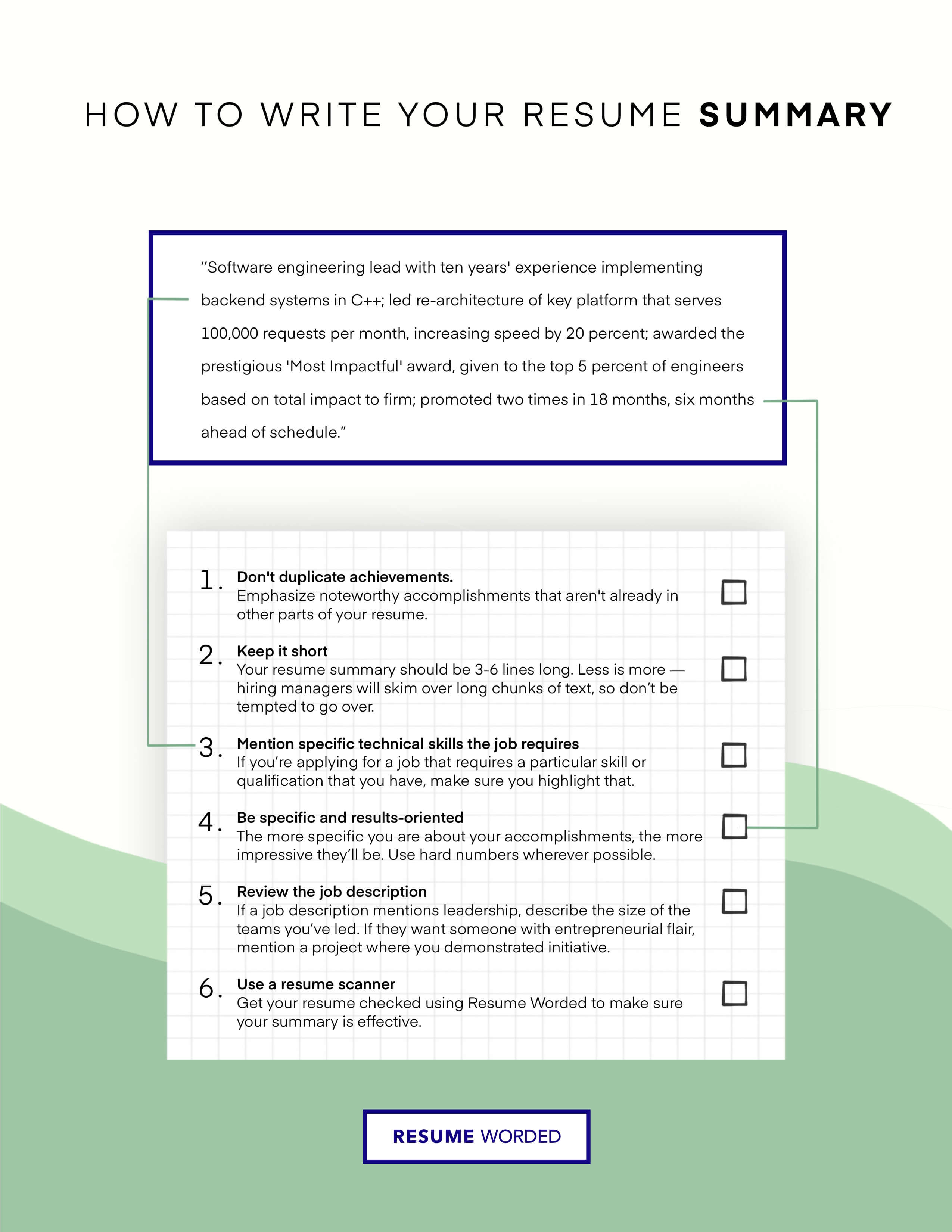
Resume Example Policy and Research Policy Analyst
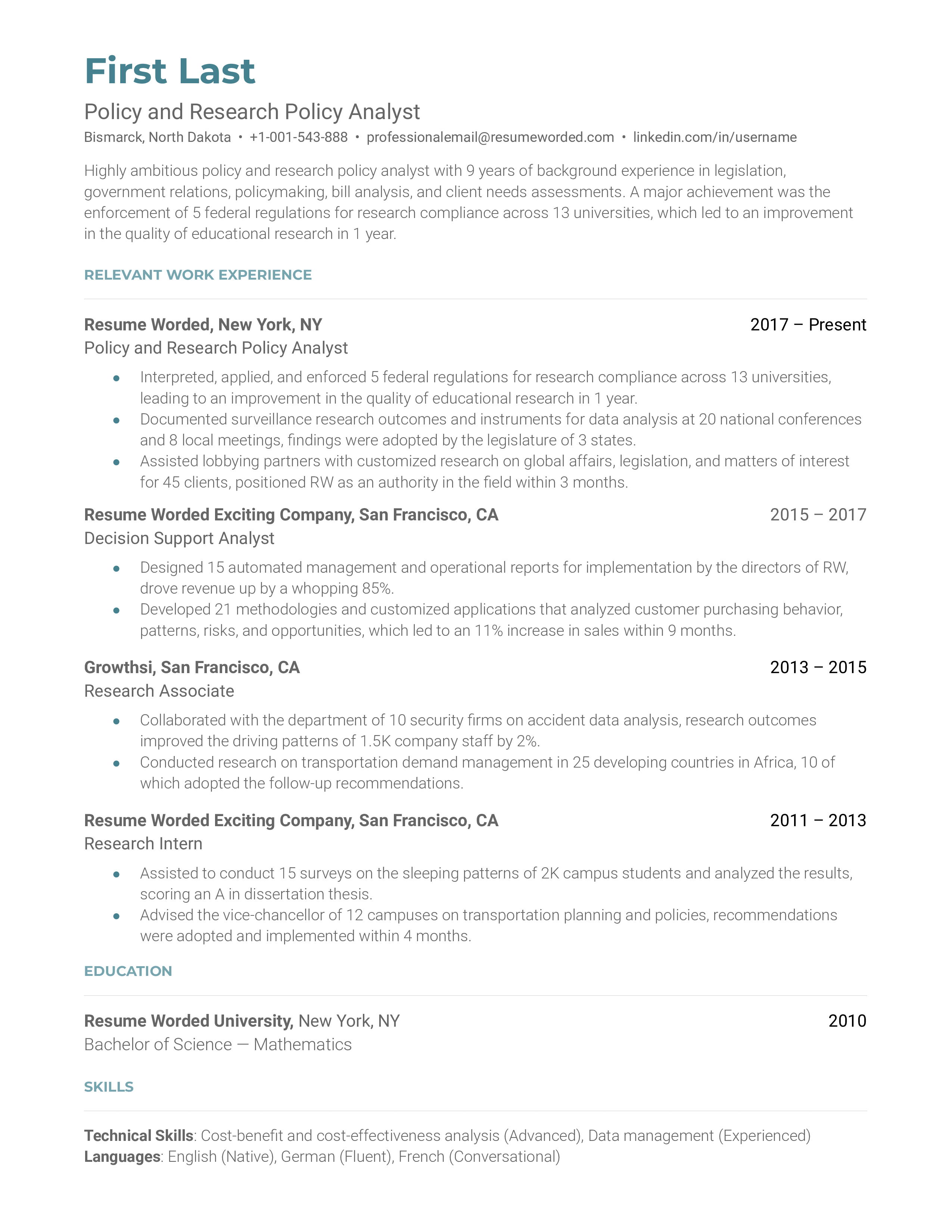
As the title suggests, this position requires an analyst to interrogate current policies or problems, do in-depth research and data analysis around a problem and be able to offer workable solutions. You will most likely work under a senior analyst and possibly as part of a team of analysts. You should therefore be an effective team player. This resume does a great job of highlighting the applicant's extensive research-based background as well as their ability to develop effective solutions. It also does a great job of emphasizing the applicant’s ability to successfully collaborate with colleagues.
Highlight research experience.
You want an employer to be confident in your capabilities to properly research any assigned topic. You also want to make it clear that not only do you have extensive experience as a researcher but you are also capable of synthesizing the research into solutions. So, highlight your crafted solutions - especially those that were adopted.
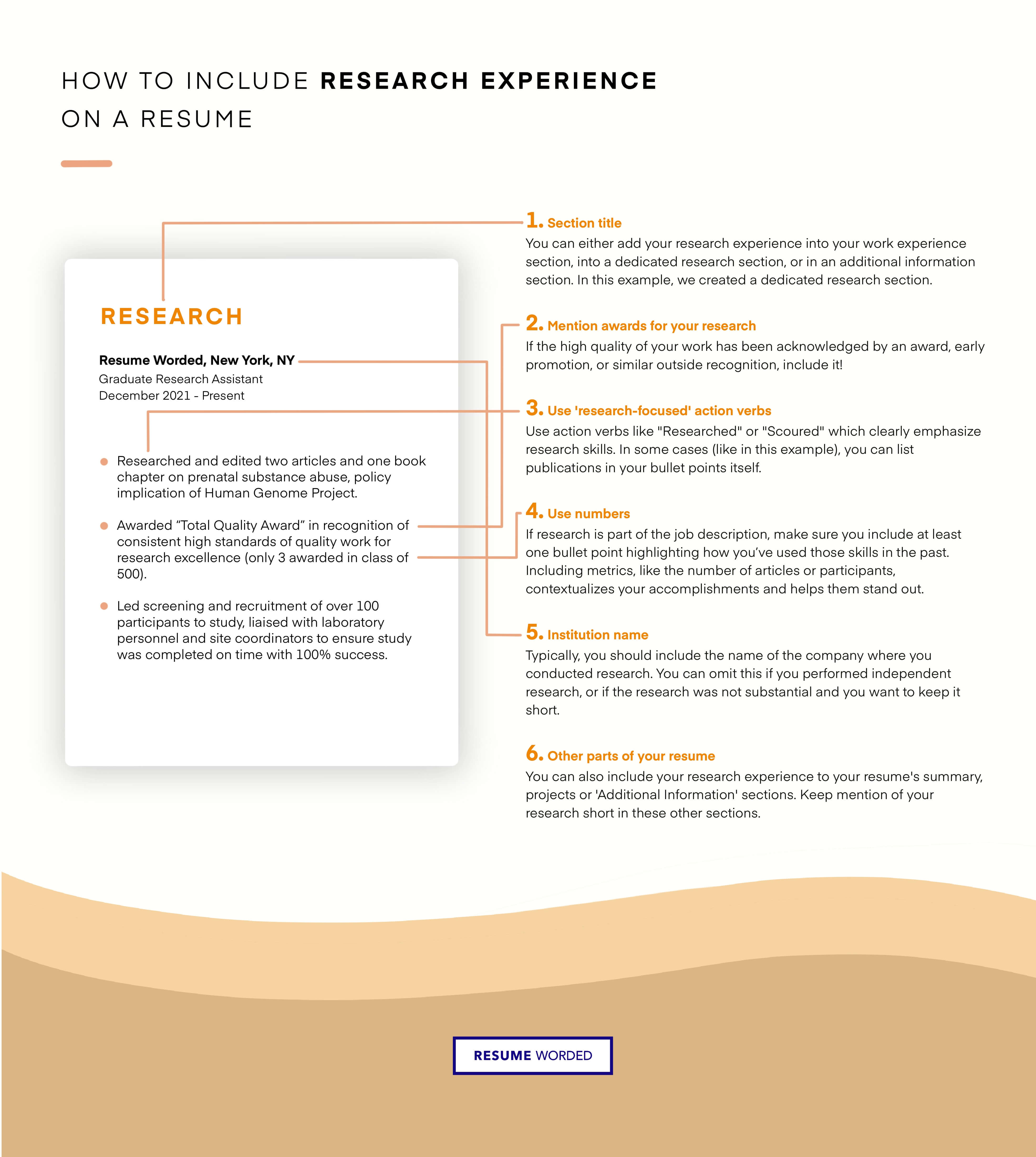
Quantify your value add to employers.
A policy analyst is supposed to create workable solutions that should have positive results. Show your effectiveness by quantifying how your suggestions and policy saved resources, increased revenue, or otherwise benefitted your previous employers.
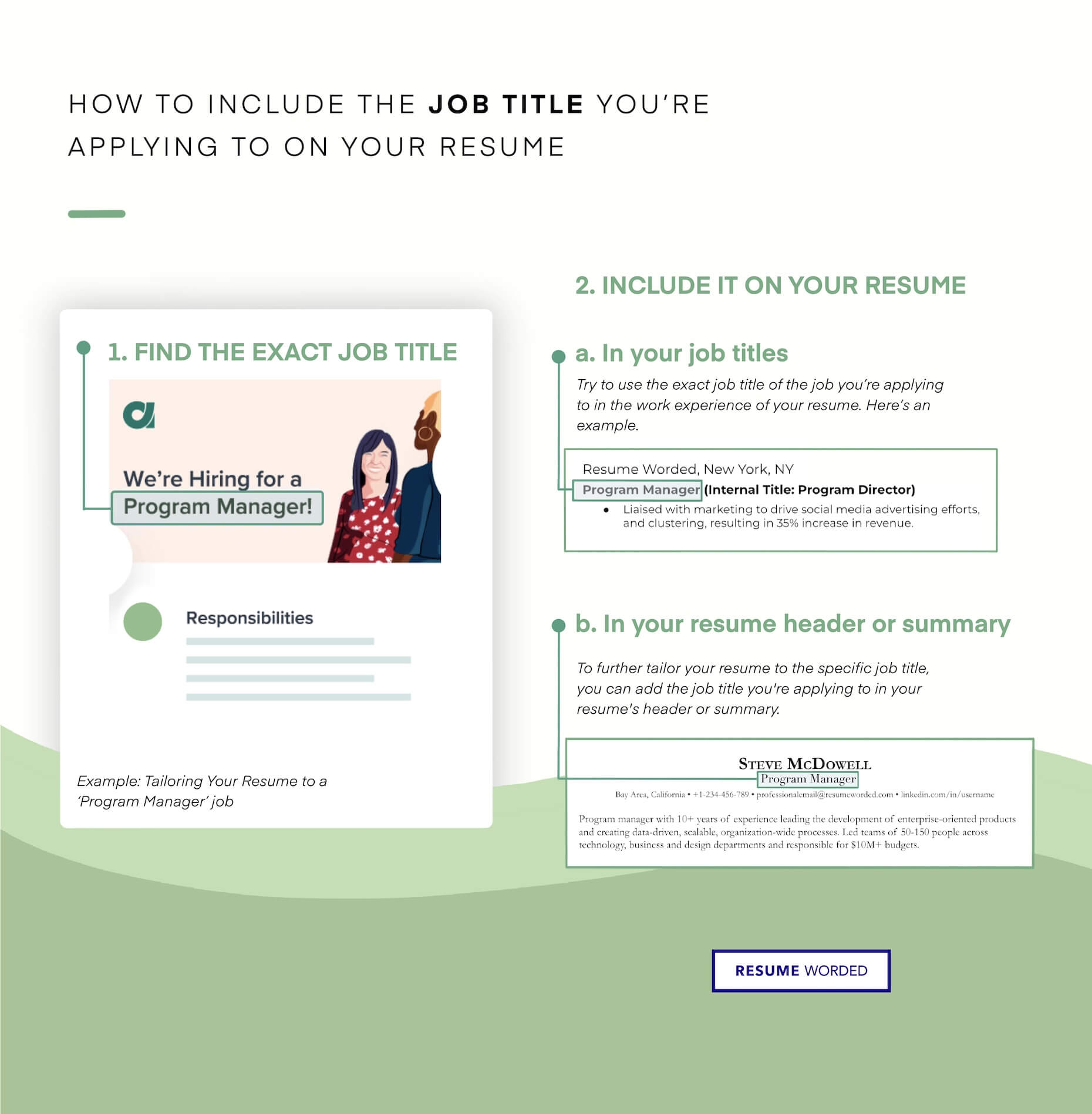
Resume Example Equity Research Associate
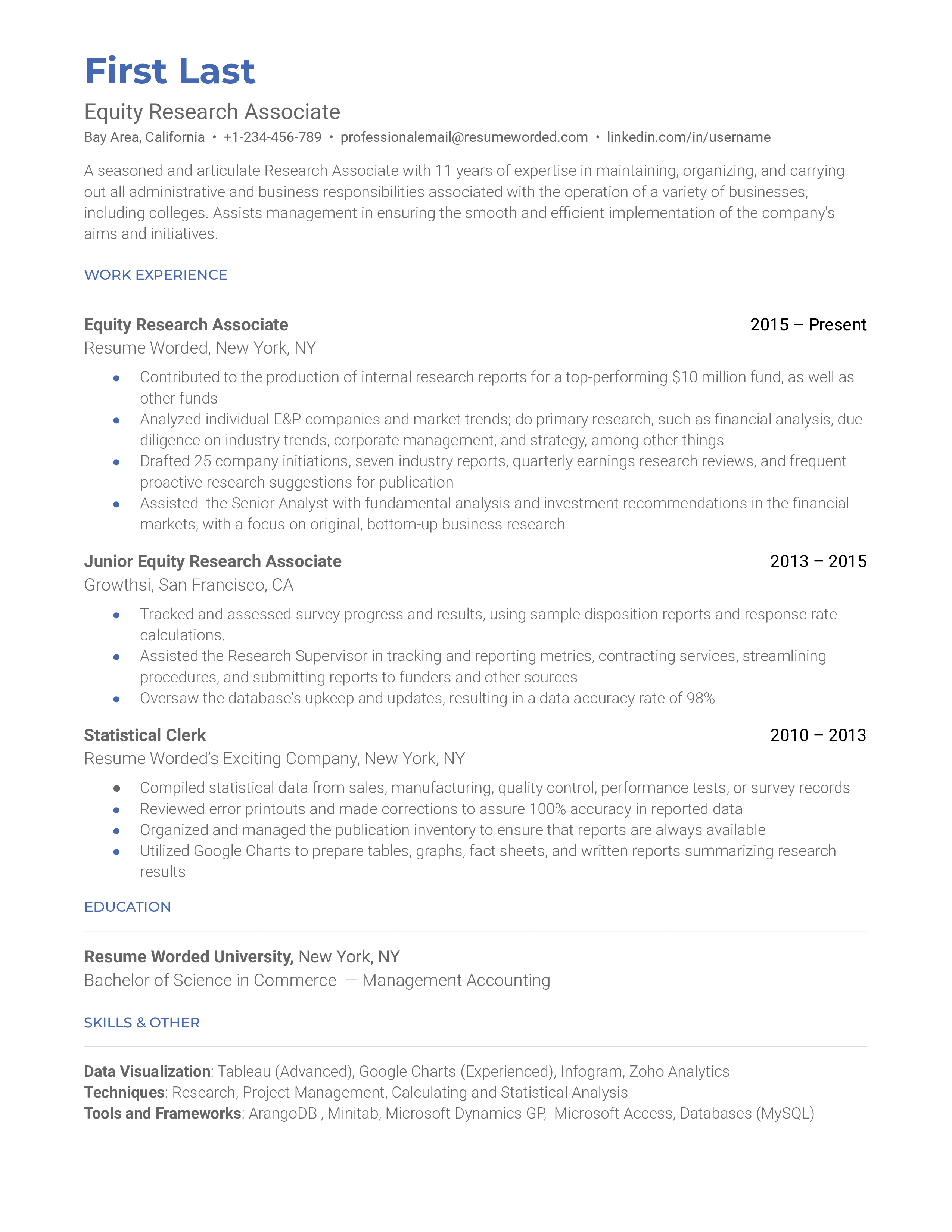
An equity research associate position is commonly an entry-level position in the equity research analyst industry. In this position, you will generally be working under/with a more senior research associate. You will be doing a lot of financial modeling, valuation, and other necessary financial research. Your work will help inform the recommendations the senior analyst will make to the decision-makers. This position has a heavy and varied workload. You will want your resume to show your ability to complete varied research and analysis-related tasks to a high level. If you do not have years of experience, you will also want to clearly list any related educational background and any skills that would enable you to complete expected tasks.
Include research and analysis experience in related industries.
Because this can be an entry related position, you may not always have a lot of equity research experience. So include any positions you have had in related industries. Especially if those positions are research and analysis related.
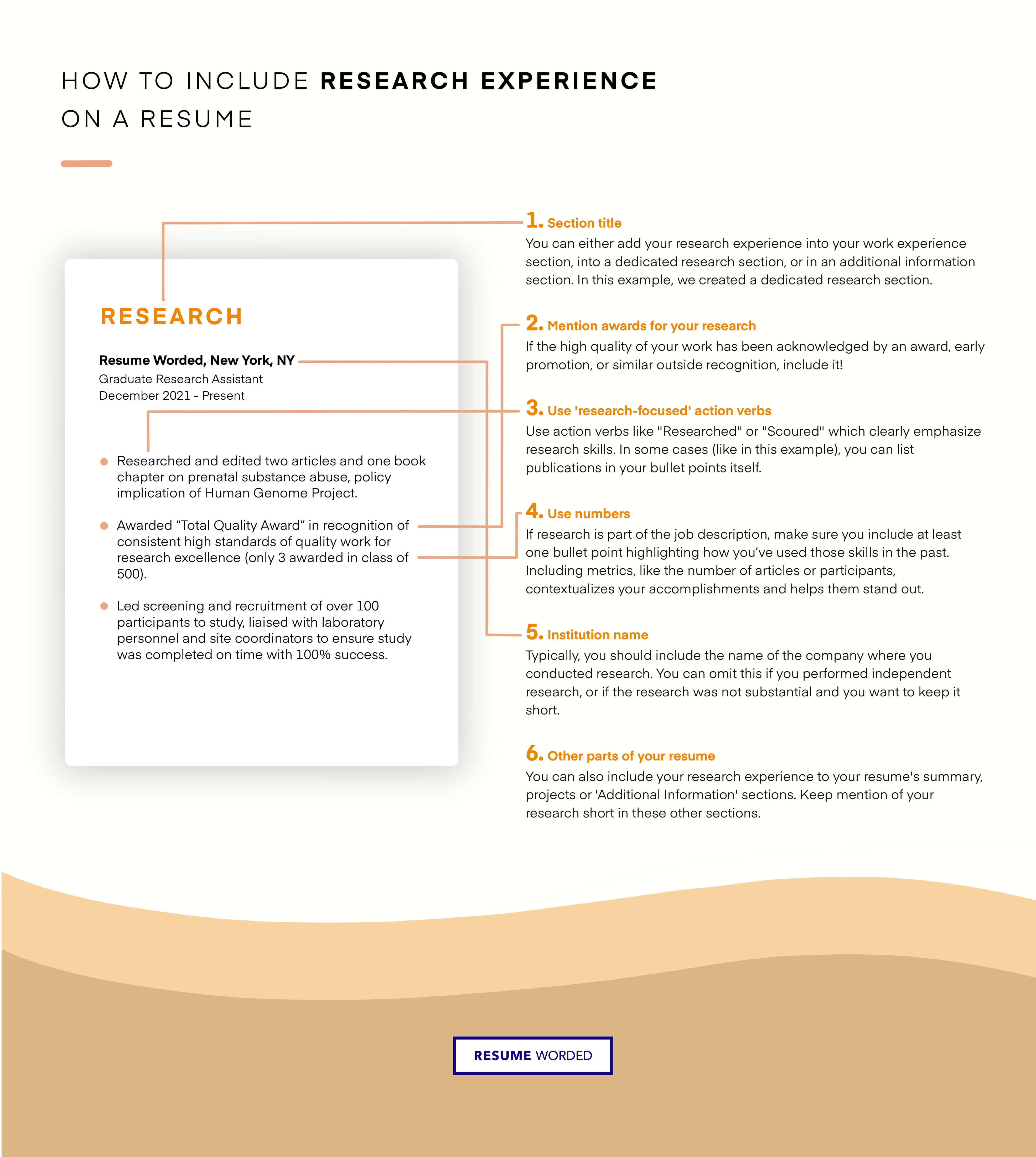
Highlight research and analysis skills and tools.
What you can’t prove in experience, you can prove with your skills list. Ensure you write a comprehensive list of all your learned skills that would be useful in your position. Remember that you can always take courses online to learn new skills to bolster your resume.
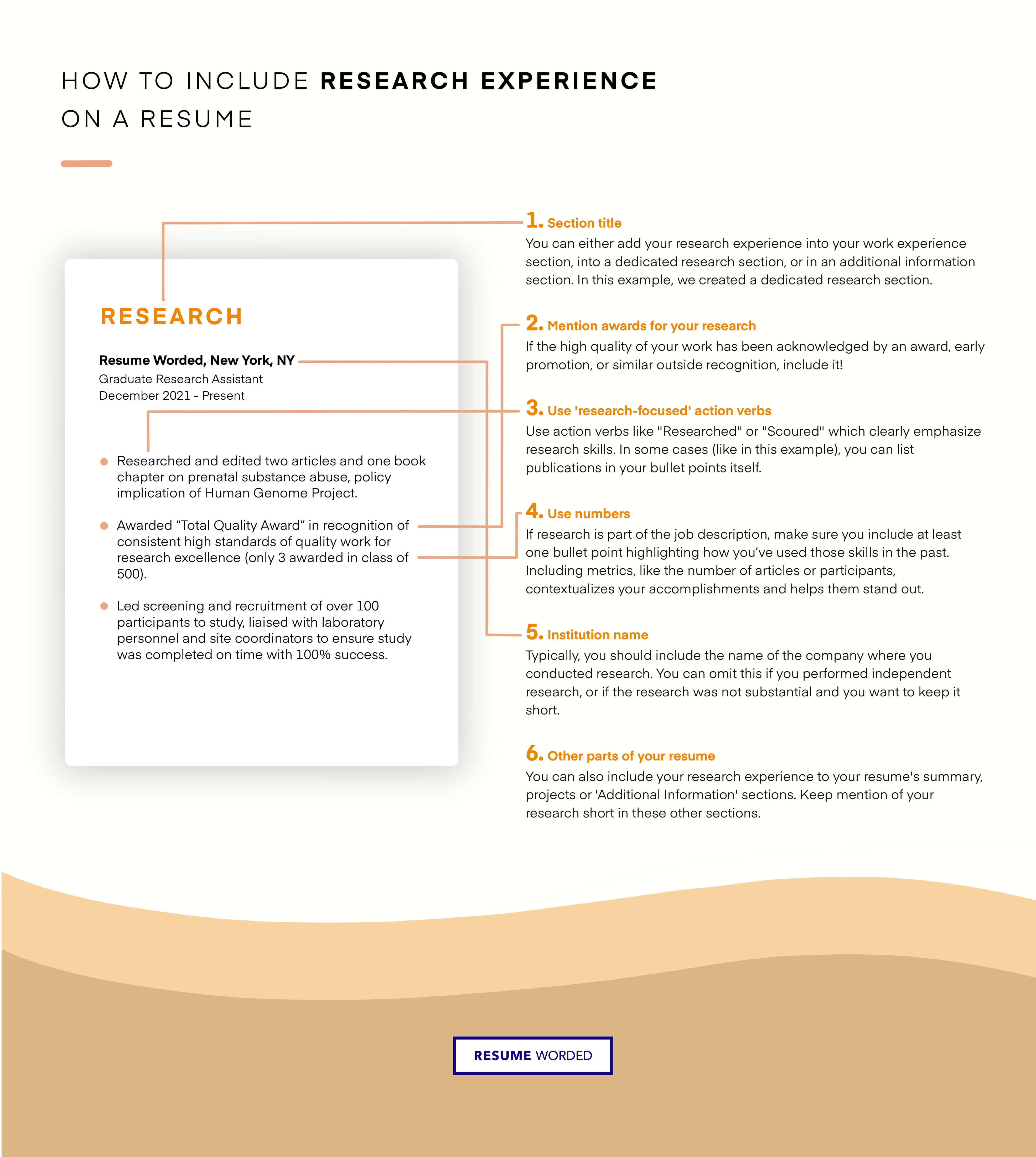
What skills should you add to a Research Analyst resume?
On top Research Analyst resumes, skills like AutoCAD, Microsoft Access, Geographic Information Systems (GIS), Data Analysis, Project Management, Quantum GIS, Cartography and Research appear most often. Depending on the exact role you're applying to, skills like SIG, Secondary Research, Financial Analysis, Online Research and Market Research can also be effective keywords to include on your resume.
What are the differences in resume skills between a Data Research Analyst and a Quantitative Research Analyst?
What are good resume skills to include for different research analyst roles and job titles.
Depending on the specific role you apply to, you may need to emphasize different skill sets. Here are a few examples:
- Quantitative Research Analyst : R (Programming Language), Operating Lease, Quantitative Finance, Quantitative Research and Machine Learning
- Investment Research Analyst : Financial Modeling, Investments, Investment Research, Financial Analysis and Valuation
- Data Research Analyst : Data Research, Data Analysis, Research, Data Mining and Market Research
- Marketing Research Analyst : Google Analytics, Marketing Strategy, Tableau, Survey Design and IBM SPSS
- Market Research Analyst : Secondary Research, Market Analysis, Primary Research, Marketing and Lead Generation
Target your Resume to a Job Description
While the keywords above are a good indication of what skills you need on your resume, you should try to find additional keywords that are specific to the job. To do this, use the free Targeted Resume tool. It analyzes the job you are applying to and finds the most important keywords you need on your resume. It is personalized to your resume, and is the best way to ensure your resume will pass the automated resume filters. Start targeting your resume
Most resumes get auto-rejected because of small, simple errors. These errors are easy to miss but can be costly in your job search. If you want to make sure your resume is error-free, upload it to Score My Resume for a free resume review. You'll get a score so you know where your resume stands, as well as actionable feedback to improve it. Get a free resume review
Scan your skills and keywords.
Creating an account is free and takes five seconds. you'll get instant access to all skills and keywords, plus be able to score your resume against them - no strings attached., choose an option..
- Have an account? Sign in
E-mail Please enter a valid email address This email address hasn't been signed up yet, or it has already been signed up with Facebook or Google login.
Password Show Your password needs to be between 6 and 50 characters long, and must contain at least 1 letter and 1 number. It looks like your password is incorrect.
Remember me
Forgot your password?
Sign up to get access to Resume Worded's Career Coaching platform in less than 2 minutes
Name Please enter your name correctly
E-mail Remember to use a real email address that you have access to. You will need to confirm your email address before you get access to our features, so please enter it correctly. Please enter a valid email address, or another email address to sign up. We unfortunately can't accept that email domain right now. This email address has already been taken, or you've already signed up via Google or Facebook login. We currently are experiencing a very high server load so Email signup is currently disabled for the next 24 hours. Please sign up with Google or Facebook to continue! We apologize for the inconvenience!
Password Show Your password needs to be between 6 and 50 characters long, and must contain at least 1 letter and 1 number.
Receive resume templates, real resume samples, and updates monthly via email
By continuing, you agree to our Terms and Conditions and Privacy Policy .
Lost your password? Please enter the email address you used when you signed up. We'll send you a link to create a new password.
E-mail This email address either hasn't been signed up yet, or you signed up with Facebook or Google. This email address doesn't look valid.
Back to log-in
Find out what keywords recruiters search for. These keywords will help you beat resume screeners (i.e. the Applicant Tracking System).
get a resume score., find out how effective your resume really is. you'll get access to our confidential resume review tool which will tell you how recruiters see your resume..
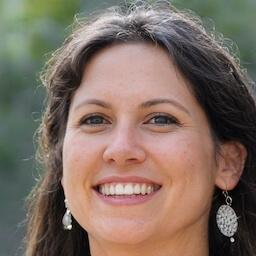
Thank you for the checklist! I realized I was making so many mistakes on my resume that I've now fixed. I'm much more confident in my resume now.
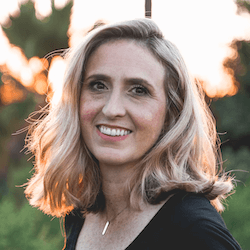
Top 12 Research Specialist Skills to Put on Your Resume
In today's competitive job market, standing out as a research specialist requires a unique blend of skills that showcase your analytical prowess and attention to detail. This article explores the top 12 skills that will make your resume shine, setting you apart from the competition and signaling to potential employers that you are a top-tier candidate for research-intensive roles.
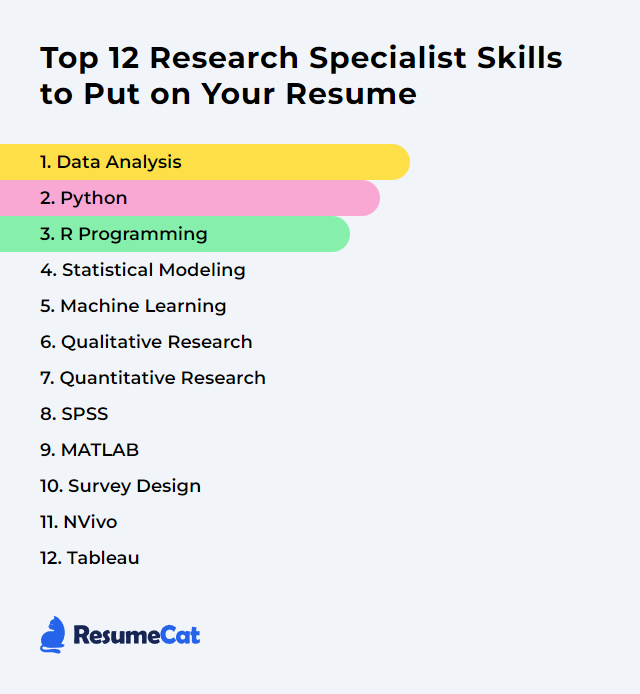
Research Specialist Skills
- Data Analysis
- R Programming
- Statistical Modeling
- Machine Learning
- Qualitative Research
- Quantitative Research
- Survey Design
1. Data Analysis
Data analysis is the process of systematically applying statistical and/or logical techniques to evaluate and interpret data, aiming to extract meaningful insights and conclusions to support decision-making and research findings.
Why It's Important
Data analysis is crucial for a Research Specialist as it enables them to systematically interpret data, uncover patterns, validate hypotheses, and inform decision-making, thereby enhancing the accuracy and credibility of research findings.
How to Improve Data Analysis Skills
Improving data analysis as a Research Specialist involves several key strategies focused on enhancing accuracy, efficiency, and insight generation. Here's a concise guide:
Strengthen Statistical Foundations : Solidify your understanding of statistical methods and principles. Resources like Khan Academy offer free, high-quality tutorials.
Master Data Analysis Tools : Excel in using software specific to your field, such as SPSS, R, or Python. Tutorials on DataCamp can help you advance your skills.
Data Cleaning Techniques : Learn to efficiently clean and preprocess data. The Data Cleaning Guide on KDnuggets provides valuable tips.
Critical Thinking and Problem-Solving : Enhance these skills to interpret data more effectively. Engage with communities on Stack Exchange to see real-world problem-solving.
Stay Updated with Latest Trends : Follow leading data analysis blogs and publications, such as Towards Data Science on Medium, to keep abreast of new techniques and technologies.
Hands-on Practice : Apply your skills on real-world datasets available on platforms like Kaggle to gain practical experience.
Peer Review and Collaboration : Engage in collaborative projects and peer review sessions to gain new insights and feedback. Platforms like GitHub facilitate collaboration and version control for your projects.
By focusing on these areas, you can significantly enhance your data analysis capabilities as a Research Specialist.
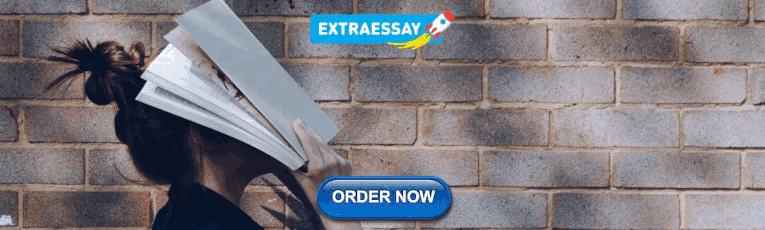
How to Display Data Analysis Skills on Your Resume
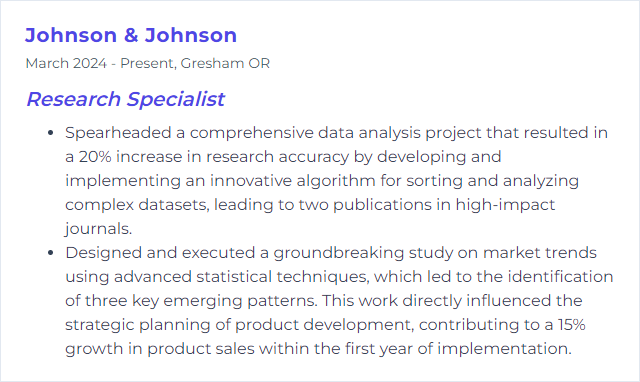
Python is a high-level, versatile programming language favored for its ease of learning and readability, widely used in data analysis, machine learning, web development, and automation.
Python is important for a Research Specialist because it provides powerful libraries for data analysis, visualization, and machine learning, enabling efficient handling of large datasets and complex computations with relatively simple and readable code.
How to Improve Python Skills
Improving your Python skills as a Research Specialist involves a combination of practicing coding, understanding advanced features, and applying Python in your research domain. Here's a concise guide:
Master the Basics : Ensure a strong grasp of Python syntax and basic constructs. Python's official documentation is an excellent starting point.
Learn Data Handling : Master libraries like NumPy for numerical data and Pandas for data manipulation and analysis.
Visualization Skills : Learn to visualize data using libraries such as Matplotlib and Seaborn , crucial for data analysis and presentation.
Statistical and Scientific Computing : Enhance your skills in scientific computing by using SciPy for advanced computations and Statsmodels for statistical modeling.
Machine Learning : If your research involves predictive modeling or analysis, Scikit-learn is a must-learn library. For deep learning, consider TensorFlow or PyTorch .
Practice Regularly : Apply what you've learned in small projects or participate in challenges on platforms like LeetCode or Kaggle .
Collaborate and Share : Use GitHub for version control, collaboration, and showcasing your projects.
Stay Updated and Network : Join Python and research communities on Stack Overflow , Reddit , and LinkedIn to stay updated on latest trends and network with peers.
Continuous Learning : Enroll in advanced Python courses on platforms like Coursera or edX that offer specialized courses in Python for research and data analysis.
By following these steps and leveraging the resources provided, you can significantly improve your Python skills relevant to your role as a Research Specialist.
How to Display Python Skills on Your Resume
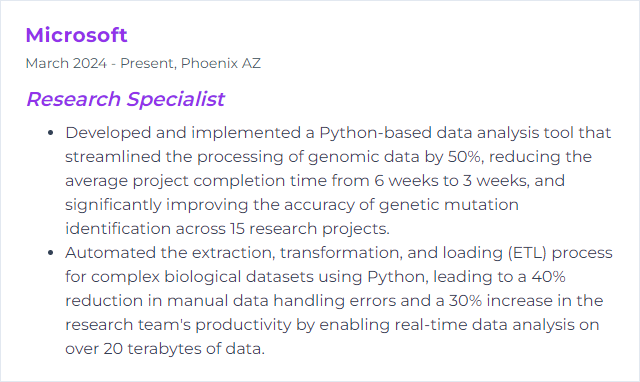
3. R Programming
R Programming is a statistical computing and graphics language used by research specialists for data analysis, statistical modeling, and visualization to support empirical research and decision-making.
R Programming is important for a Research Specialist because it offers a comprehensive statistical analysis toolkit, facilitates data manipulation and visualization, and is highly extensible, enabling the execution of complex data analysis tasks and reproducible research across diverse scientific fields.
How to Improve R Programming Skills
Improving your R programming skills, especially as a Research Specialist, involves a multifaceted approach focusing on advancing your coding proficiency, statistical analysis capabilities, and data visualization skills. Here are concise steps with relevant resources:
Practice Regularly : Consistent practice through solving real-world problems enhances understanding and skill. R for Data Science is an excellent resource to get started.
Learn Data Manipulation : Master data manipulation packages like dplyr and tidyr for efficient data handling. Data Manipulation with R - dplyr provides a comprehensive guide.
Understand Data Visualization : Gain proficiency in ggplot2 for advanced data visualization. The Data Visualization with ggplot2 guide is a great starting point.
Study Statistical Analysis : Deepen your statistical analysis skills within R. The R Book covers a wide range of statistical methods.
Contribute to and Learn from R Community : Engage with the R community through forums like RStudio Community and Stack Overflow . Sharing knowledge and solving others' queries can provide new insights.
Experiment with R Packages : Explore and incorporate different R packages into your work. The CRAN Task Views categorize packages by relevant fields.
Attend Workshops and Webinars : Participate in workshops and webinars for hands-on learning. UseR! Conference is an annual event bringing together R users and developers.
Follow R Blogs and Podcasts : Stay updated with the latest in R by following blogs like R-bloggers and listening to podcasts like The R-Podcast .
Implement Version Control : Learn to use version control systems like Git with R for better project management. Happy Git and GitHub for the useR provides a tailored guide for R users.
Explore Advanced Topics : As you gain proficiency, explore advanced topics like Machine Learning with R or Shiny apps for interactive web applications. Shiny from RStudio offers comprehensive tutorials and examples.
By systematically following these steps and utilizing the provided resources, you'll significantly enhance your R programming skills, making you a more effective and efficient Research Specialist.
How to Display R Programming Skills on Your Resume
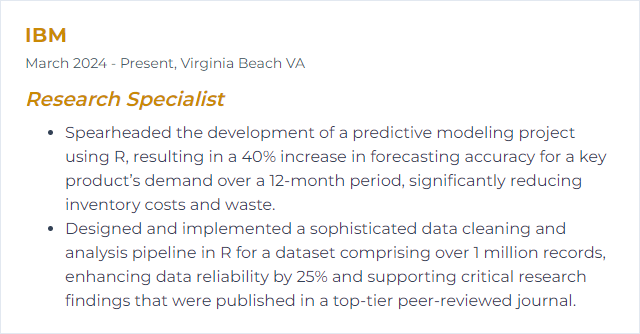
4. Statistical Modeling
Statistical modeling is the process of creating mathematical representations of real-world processes to analyze, explain, and make predictions about data, using statistical methods and assumptions. For a Research Specialist, it serves as a crucial tool for understanding complex relationships, testing hypotheses, and informing decision-making based on empirical evidence.
Statistical modeling is crucial for a Research Specialist as it provides a systematic approach to understanding and interpreting data, allows for the prediction of trends, and aids in making informed decisions based on empirical evidence.
How to Improve Statistical Modeling Skills
Improving statistical modeling, especially for a Research Specialist, involves a combination of enhancing your statistical knowledge, applying best practices in data collection and analysis, and leveraging advanced tools and methodologies. Here are concise steps with resources to guide you:
Enhance Statistical Knowledge : Strengthen your understanding of statistical theories and models. MIT OpenCourseWare offers a comprehensive course on probability and statistics.
Master Statistical Software : Become proficient in statistical software like R or Python for data analysis. DataCamp has courses for R and Python.
Understand Your Data : Ensure a thorough understanding of your data source, quality, and structure. The Data Management Guide by the ICPSR provides excellent best practices .
Model Selection and Validation : Learn about different statistical models and their applicability, including assumptions and limitations. Stanford Online offers a course on Statistical Learning that covers model selection and validation techniques.
Stay Updated on New Techniques : The field of statistics is constantly evolving. Follow journals like The American Statistician to stay updated on new methodologies and applications.
Collaborate and Seek Feedback : Engage with the community through forums like Cross Validated on Stack Exchange to discuss modeling challenges and solutions.
Apply Ethical Practices : Ensure transparency and ethics in your modeling practices. The American Statistical Association provides guidelines on Ethical Statistics Practice .
By following these steps and utilizing the resources provided, you can significantly improve your statistical modeling skills and enhance your research outputs.
How to Display Statistical Modeling Skills on Your Resume
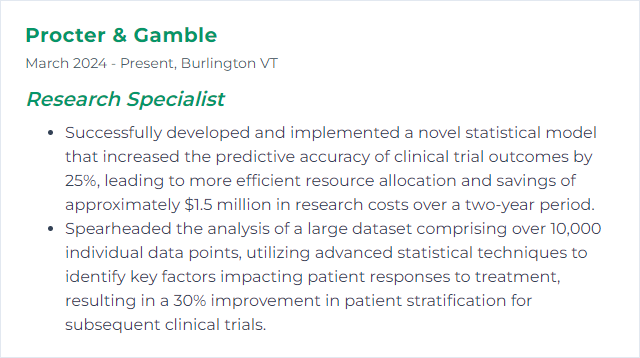
5. Machine Learning
Machine Learning is a subset of artificial intelligence that enables systems to learn and improve from experience without being explicitly programmed, focusing on the development of algorithms that can analyze and make predictions or decisions based on data.
Machine learning is crucial for a Research Specialist as it enables the automated analysis and interpretation of complex data, uncovering insights and patterns that can drive innovation, inform decision-making, and solve intricate problems across various fields.
How to Improve Machine Learning Skills
Improving machine learning involves a multifaceted approach focusing on data quality, model complexity, and continuous evaluation. Here's a concise guide tailored for a Research Specialist seeking to enhance their machine learning projects:
Enhance Data Quality : Ensure high-quality, diverse, and relevant datasets. Utilize techniques like data augmentation, cleaning, and transformation for better model performance. Data Quality Improvement.
Feature Engineering : Extract and select the most informative features. This process can significantly impact the model's performance. Feature Engineering Techniques.
Model Selection and Optimization : Choose the right model based on the problem complexity and data type. Use hyperparameter tuning to find the optimal model settings. Model Selection Guide .
Cross-validation : Employ cross-validation techniques to ensure that the model generalizes well to unseen data. Cross-validation Strategies .
Regularization : Implement regularization methods to prevent overfitting, especially in complex models. Understanding Regularization.
Ensemble Methods : Combine multiple models to improve predictions. Techniques like Bagging, Boosting, and Stacking can lead to better and more robust models. Ensemble Learning Guide.
Continuous Evaluation and Update : Regularly evaluate model performance on new data. Update models and algorithms based on performance metrics and emerging research. Model Evaluation Techniques.
Ethical AI and Bias Mitigation : Address biases in data and model predictions. Ensure your models are fair, ethical, and transparent. Ethical AI Practices .
Stay Updated : Machine learning is a rapidly evolving field. Stay informed about the latest research, tools, and best practices. arXiv and Google Scholar are valuable resources for the latest literature.
Collaborate and Share : Engage with the community through platforms like GitHub for code sharing and Kaggle for competitions and datasets. Collaboration can provide new insights and feedback to refine your projects.
Focusing on these areas will help improve the robustness, accuracy, and efficiency of your machine learning models, ultimately leading to more impactful research outcomes.
How to Display Machine Learning Skills on Your Resume
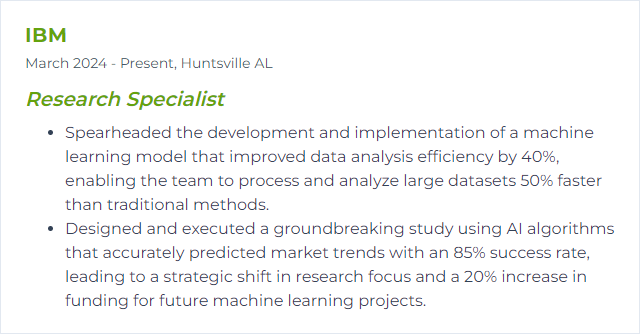
6. Qualitative Research
Qualitative research is an exploratory research methodology that focuses on understanding phenomena from a human perspective. It involves collecting non-numerical data, such as interviews, observations, and text analysis, to gain insights into concepts, experiences, or social contexts. This approach allows a Research Specialist to explore the depth, meaning, and complexity of the subject matter.
Qualitative research is crucial as it provides deep insights into people's attitudes, behaviors, and experiences, enabling a more comprehensive understanding of complex issues. This rich, detailed data supports the development of effective strategies and solutions tailored to specific contexts, essential for a Research Specialist in designing and evaluating interventions.
How to Improve Qualitative Research Skills
Improving qualitative research, particularly for a Research Specialist, involves enhancing the depth, reliability, and applicability of the study. Here are concise strategies:
Define Clear Objectives : Clearly articulate the purpose and objectives of your research. This clarity guides the entire research process (Qualitative Research Guidelines Project).
Ensure Rigorous Methodology : Adopt and stick to a rigorous methodology tailored to your research question. This involves careful selection of participants, data collection methods, and analysis techniques ( SAGE Publications ).
Maintain Ethical Standards : Adhere strictly to ethical guidelines, ensuring participant confidentiality and informed consent ( American Psychological Association ).
Triangulation : Use multiple sources of data or multiple approaches to data collection and analysis to confirm the findings ( Social Research Methods ).
Member Checking : Involve participants in reviewing the findings or interpretation of the data to ensure accuracy and credibility ( The Qualitative Report ).
Peer Debriefing : Engage with peers or mentors in discussions about the research process and findings to challenge assumptions and enhance insights (Research Gate).
Rich, Thick Descriptions : Provide detailed descriptions of the context and participants to allow readers to connect with the situation and assess transferability (SAGE Publications).
Reflexivity : Continuously reflect on and document your biases, perspectives, and influences on the research process ( SAGE Research Methods ).
Continuous Learning : Stay updated with the latest qualitative research methods and theories through professional development and scholarly literature (SAGE Journals).
By integrating these strategies, a Research Specialist can significantly enhance the quality and impact of their qualitative research.
How to Display Qualitative Research Skills on Your Resume
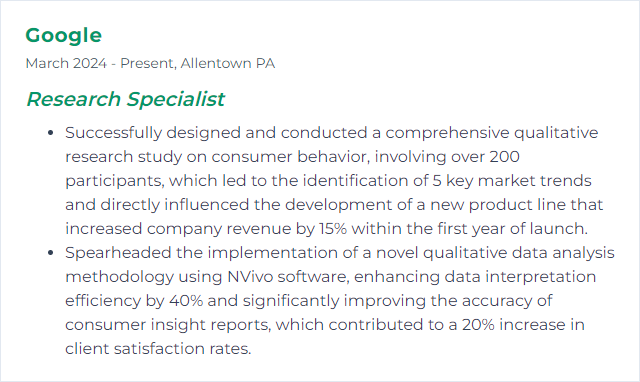
7. Quantitative Research
Quantitative research is a systematic investigation that primarily uses statistical, mathematical, or computational techniques to collect and analyze numerical data, aiming to establish patterns, relationships, or generalizations across a population.
Quantitative research is important because it provides objective, measurable data that can be used to identify patterns, test hypotheses, and make informed decisions. It allows for the statistical analysis of results, ensuring reliability and validity in findings that are essential for evidence-based practice. This is crucial for a Research Specialist who aims to support conclusions with empirical evidence, enabling accurate predictions and effective solutions.
How to Improve Quantitative Research Skills
Improving quantitative research involves several key strategies aimed at enhancing the precision, accuracy, and reliability of your data and analysis. Here's a concise guide:
Define Clear Objectives : Start with a clear and focused research question or hypothesis. This guides your study's direction and helps in selecting appropriate methodologies ( Research Question Importance ).
Ensure Proper Sample Size : Use statistical methods to determine the right sample size for generalizability and power of your study ( Sample Size Calculation ).
Choose Appropriate Tools and Techniques : Select the right statistical tools and data collection methods that align with your research objectives ( Selecting Statistical Tests ).
Maintain Data Integrity : Implement rigorous data collection and entry protocols to avoid errors (Data Integrity Best Practices).
Validate Your Instruments : Ensure your measurement tools are valid and reliable for the constructs you're measuring (Instrument Validation).
Statistical Analysis : Apply appropriate and advanced statistical techniques to analyze your data accurately. Knowledge in software like SPSS, R, or Python can be beneficial (SPSS Tutorials).
Interpret Results Within Context : Analyze and interpret your findings in the context of existing literature, considering limitations and implications ( Data Interpretation ).
Peer Review and Feedback : Before finalizing your research, seek feedback from peers or mentors to identify any overlooked flaws or biases (Peer Review Importance).
Continual Learning : Stay updated with the latest research methodologies and statistical techniques through workshops, courses, and literature ( Coursera Courses ).
Ethical Considerations : Ensure your research adheres to ethical standards, protecting participants' rights and data privacy ( Ethical Guidelines ).
These steps, when meticulously followed, can significantly enhance the quality and impact of quantitative research.
How to Display Quantitative Research Skills on Your Resume
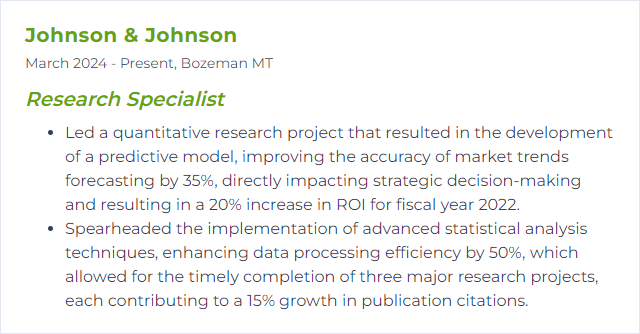
SPSS (Statistical Package for the Social Sciences) is a software tool used by research specialists for statistical analysis, data management, and data documentation, facilitating complex data manipulation and detailed analysis of large datasets.
SPSS is important because it provides comprehensive tools for data analysis, making it easier for Research Specialists to manage, analyze, and interpret complex datasets efficiently, thereby enhancing the accuracy and reliability of research findings.
How to Improve SPSS Skills
To improve your proficiency in SPSS as a Research Specialist, focus on the following concise strategies:
Official IBM SPSS Tutorials : Start with IBM's official SPSS tutorials to understand the basics and advanced features directly from the source.
Online Courses : Enroll in structured online courses from platforms like Coursera or Udemy that offer comprehensive training on SPSS, from beginner to advanced levels.
Practice Datasets : Apply your skills on practice datasets available on sites like Kaggle to get hands-on experience with real-world data.
SPSS Forums and Communities : Join SPSS forums and communities, such as the IBM SPSS Community , to ask questions, share experiences, and learn from other users.
Books and Academic Journals : Refer to specialized SPSS books and academic journals for in-depth understanding and advanced statistical techniques. Titles like "SPSS Statistics for Dummies" and journals from SAGE Publications offer valuable insights.
Automation and Scripting : Learn to automate repetitive tasks using SPSS Syntax or Python integration for efficiency. Resources on SPSS Syntax and Python programming in SPSS can be quite helpful.
Stay Updated : Keep abreast of the latest updates and features in SPSS by checking the official IBM SPSS software announcements .
By systematically pursuing these strategies, you'll significantly improve your SPSS skills, making you more effective and efficient in your role as a Research Specialist.
How to Display SPSS Skills on Your Resume
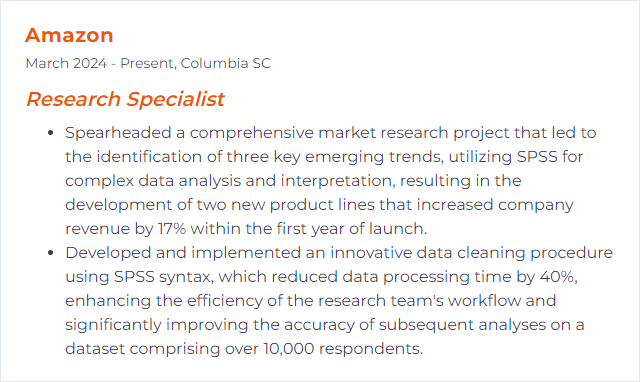
MATLAB is a high-level programming and numerical computing environment used for algorithm development, data analysis, visualization, and mathematical computations, widely utilized in research and engineering projects.
MATLAB is important for a Research Specialist as it provides a versatile environment for numerical computation, data analysis, and visualization, facilitating complex mathematical operations and the development of algorithms critical for research advancements.
How to Improve MATLAB Skills
To improve MATLAB skills as a Research Specialist, focus on these concise strategies:
Official MATLAB Tutorials : Start with MATLAB's official documentation and tutorials for foundational knowledge.
Practice Coding : Apply your skills on Project Euler or MATLAB Cody to solve problems and learn by doing.
Online Courses : Enroll in Coursera or Udemy courses that cater to various skill levels, from beginner to advanced.
Join Forums : Engage with the MATLAB Central community to ask questions, share knowledge, and learn from experts.
Read Books : Consider books like "MATLAB for Engineers" (Holly Moore) for practical applications or "MATLAB Programming for Biomedical Engineers and Scientists" (Andrew P. King, Paul Aljabar) for more specialized knowledge. Check online retailers or libraries for access.
Explore Toolboxes : Experiment with different MATLAB toolboxes relevant to your field to expand your skill set.
Attend Workshops/Seminars : Look for MATLAB workshops or seminars offered by universities, research institutions, or MathWorks Events .
Learn from YouTube : Channels like MATLAB and Engineer Clearly offer tutorials and tips suited for various levels.
By incorporating these strategies, you'll enhance your MATLAB proficiency, enabling you to conduct more efficient and effective research.
How to Display MATLAB Skills on Your Resume
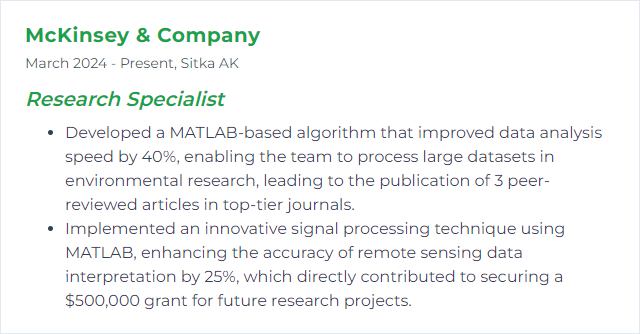
10. Survey Design
Survey design refers to the process of planning and structuring a survey to efficiently gather accurate and relevant data, involving the selection of target populations, question formulation, and choice of delivery method to meet specific research objectives.
Survey design is crucial for a Research Specialist because it ensures the collection of reliable, accurate, and relevant data, enabling valid conclusions and informed decision-making.
How to Improve Survey Design Skills
Improving survey design involves several key strategies aimed at enhancing the quality of data collected and the response rate. Here's a concise guide for a Research Specialist:
Define Clear Objectives : Establish what you aim to learn. This ensures your survey is focused and relevant. Learn more.
Keep it Short and Simple : Respect respondents' time. Shorter surveys typically have higher completion rates. Use simple, direct language. Survey Length Best Practices .
Use Closed-Ended Questions : Whenever possible, use multiple-choice questions instead of open-ended ones to streamline analysis and improve response rates. Closed vs. Open-Ended Questions.
Pilot Your Survey : Conduct a pilot test with a small, similar audience to catch issues before the full launch. Pilot Testing .
Ensure Anonymity and Confidentiality : If responses are anonymous or confidential, make sure to communicate this to participants as it may increase response rate and honesty. Anonymity in Surveys.
Avoid Leading and Loaded Questions : Ensure questions are neutral and unbiased to avoid skewing the data. Question Design Flaws.
Optimize for Mobile Devices : With increasing mobile internet usage, ensure your survey is mobile-friendly. Mobile Surveys .
Use Logical Question Flow : Organize questions logically from general to specific, and group similar topics together to maintain respondent engagement. Survey Flow.
Offer Incentives : If possible, offer incentives to increase participation rates. Ensure incentives are appropriate and ethical. Using Incentives.
Follow Up : Send reminders to those who have not completed the survey to boost response rates. Survey Reminders.
By implementing these strategies, Research Specialists can significantly improve the design of their surveys, leading to higher quality data and insights.
How to Display Survey Design Skills on Your Resume
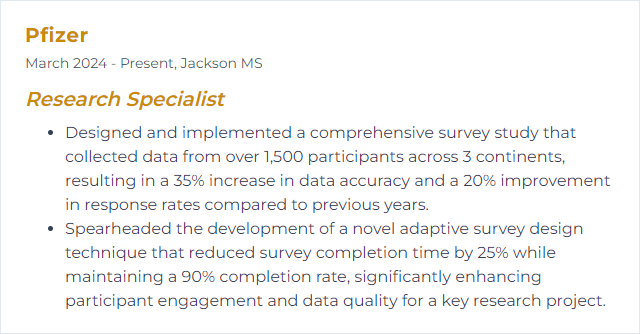
NVivo is a qualitative data analysis (QDA) software designed to help researchers organize, analyze, and find insights in unstructured or qualitative data like interviews, open-ended survey responses, articles, social media, and web content.
NVivo is important for a Research Specialist as it facilitates sophisticated data analysis, enabling the organization, coding, and interpretation of qualitative and mixed-methods research data, thus enhancing the depth, accuracy, and efficiency of research findings.
How to Improve NVivo Skills
Improving your use of NVivo as a Research Specialist involves a blend of enhancing technical skills, staying updated with the latest features, and integrating best practices for qualitative data analysis. Here are concise tips to help you enhance your NVivo proficiency:
Master the Basics and Advanced Features : Start with the basics and progressively dive into advanced features. Online tutorials and webinars can be excellent resources. QSR International, the developer of NVivo, offers free webinars and tutorials that cover both foundational and complex aspects of the software.
Customize Your Workspace : Tailor NVivo’s interface and settings to fit your research needs. This can improve your efficiency and make navigation easier. The NVivo Help Center provides guidance on customization options.
Use Coding Queries Effectively : Develop proficiency in creating and refining coding queries to analyze your data deeply. Understanding the nuances of query options allows you to extract meaningful insights. The NVivo Resource Library includes tutorials and examples on various query types.
Engage with the NVivo Community : Join NVivo forums and user groups to share experiences, ask questions, and learn from other researchers. Engaging with the community can provide you with unique insights and tips. The NVivo Community is a good place to start.
Stay Updated : NVivo regularly updates its software to include new features and improvements. Keeping your software up to date ensures you have access to the latest tools and security features. Check the NVivo Blog for updates and announcements.
Practice Regularly : Like any software, proficiency in NVivo comes with regular use. Set aside time for practice and experimentation with different features and datasets.
Seek Formal Training : If you're looking for structured learning, consider enrolling in NVivo training courses. These can provide comprehensive knowledge and hands-on experience. The NVivo Academy offers official training courses that cater to different expertise levels.
By incorporating these strategies into your research workflow, you can enhance your NVivo skills, leading to more efficient and effective qualitative data analysis.
How to Display NVivo Skills on Your Resume
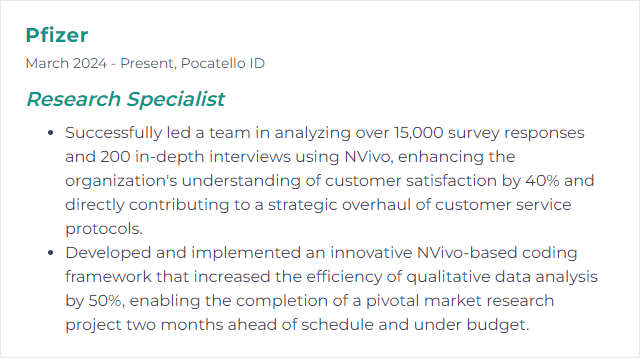
12. Tableau
Tableau is a powerful data visualization tool used by Research Specialists to analyze, visualize, and share insights from complex datasets through interactive dashboards and reports.
Tableau is important for a Research Specialist because it enables efficient data visualization, making complex datasets easier to analyze and interpret. This facilitates the identification of trends and patterns, aiding in evidence-based decision-making.
How to Improve Tableau Skills
Improving your skills in Tableau as a Research Specialist involves focusing on data visualization best practices, mastering Tableau’s advanced features, and staying updated with the latest trends. Here’s a short, concise guide to help you enhance your Tableau proficiency:
Learn the Basics Thoroughly : Ensure you have a solid understanding of Tableau basics. Tableau's own Getting Started resources are an excellent place to begin.
Master Data Visualization Principles : Understanding core data visualization principles can significantly improve how your Tableau dashboards communicate information. Edward Tufte’s book, "The Visual Display of Quantitative Information", is a seminal work in this area.
Advance Your Skills : Dive into advanced features like calculated fields, parameters, and Tableau Prep. Tableau offers advanced training videos that are quite useful.
Incorporate Best Practices : Adopting dashboard design best practices enhances clarity and user experience. Explore Tableau’s Dashboard Design guidelines for insights.
Engage with the Community : The Tableau Community ( Tableau Community Forums ) is a goldmine for tips, tricks, and support from other Tableau users.
Stay Updated : Tableau’s platform evolves rapidly. Keep abreast of new features and updates by regularly checking the Tableau Blog .
Practice Regularly : Apply your skills to real-world datasets. Websites like Kaggle offer datasets you can use to practice and showcase your work.
Seek Feedback : Share your visualizations with peers or on platforms like Tableau Public for feedback and inspiration.
By focusing on these areas, you can significantly improve your Tableau skills and effectiveness as a Research Specialist in visualizing and interpreting data.
How to Display Tableau Skills on Your Resume
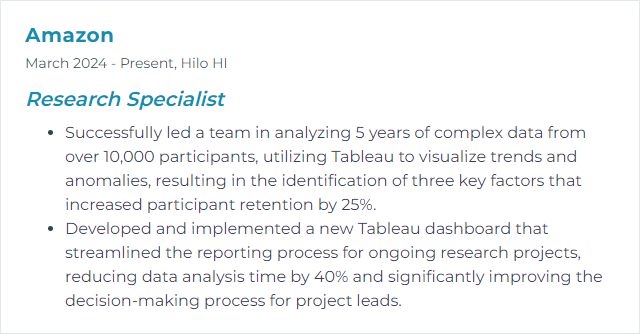
Related Career Skills
- Research and Development Engineer
- Research Nurse
- Research Administrator
- Research Analyst
- Research Associate
- Research Biologist
Explore Jobs
- Jobs Near Me
- Remote Jobs
- Full Time Jobs
- Part Time Jobs
- Entry Level Jobs
- Work From Home Jobs
Find Specific Jobs
- $15 Per Hour Jobs
- $20 Per Hour Jobs
- Hiring Immediately Jobs
- High School Jobs
- H1b Visa Jobs
Explore Careers
- Business And Financial
- Architecture And Engineering
- Computer And Mathematical
Explore Professions
- What They Do
- Certifications
- Demographics
Best Companies
- Health Care
- Fortune 500
Explore Companies
- CEO And Executies
- Resume Builder
- Career Advice
- Explore Majors
- Questions And Answers
- Interview Questions
Can’t keep your job search organized?
Track all your applications in one place with the Zippia extension for Chrome.
Research Analyst skills for your resume and career

A research analyst needs a mix of hard and soft skills. For hard skills, understanding data analysis, managing research projects, and mastering data collection and analysis are key. Proficiency in tools like PowerPoint, Python, and SAS is also important. As Professor Pooyan Kazemian Ph.D. from Case Western Reserve University points out, "a working knowledge of mathematical modeling, optimization, statistical analysis, machine learning, and programming languages such as R and Python are fundamental technical skills needed for most OR Analyst positions."
On the other hand, soft skills are equally important for a research analyst. Strong communication skills are necessary to portray findings and suggestions effectively. Being client-focused and demonstrating a commitment to fulfilling client needs is also crucial. As Professor Fronefield Crawford from Franklin and Marshall College puts it, "one's first job out of college may not be as important as the job one eventually will have after applying the various skills and knowledge learned in a challenging academic major."
15 research analyst skills for your resume and career
1. data analysis.
Data analysis is the process of inspecting, cleansing, transforming, and modeling data to discover useful information, inform conclusions, and support decision-making. Research analysts use data analysis to prepare and refine audit work programs, summarize research activities, and conduct statistical analysis. They also use it to identify trends across different data sources and support business development. As John Lyden , Professor and Chair of Religious Studies at the University of Nebraska - Omaha, puts it, "Data analysis and interpretation are highly valued skills in research analysts. Employers will include those in higher education, government, non-profits, research institutes, and museums."
- Prepared data analysis for completed test records/ participants along with assisting in the preparation and refinement of audit work programs.
- Provided data analysis summarizing research activities and results, and transplant outcomes analysis for IRB submissions and insurance re-certifications.
2. Data Collection
Data collection is the process of gathering information from various sources to build a database or data set. Research analysts use data collection to manage projects, analyze data, and prepare reports. They also design surveys, supervise data collection, and train others in research methodology. As Harriet Phinney Ph.D., Associate Professor at Seattle University, puts it, "Empirical data collection: Research skills for collecting original data, analyzing the data, writing up the information collected, and presenting it in a professional manner."
- Managed the execution of primary research projects from questionnaire development, supervised data collection and data tabulation, and prepared reports.
- Redesigned data collection forms used by the director services contractor to submit service delivery information required by the federal funding agency.
3. Research Projects
Research projects are in-depth investigations into a specific topic or issue. Research analysts use research projects to gather information, identify patterns, and draw conclusions. They manage these projects from start to finish, including developing specifications, collecting data, and writing reports. They also collaborate with others, provide technical assistance, and manage teams. For instance, a research analyst might lead a project to evaluate the effectiveness of a state's public policy implementation, or assist senior analysts with equity research projects across various industries.
- Completed series of research projects which allowed company to redesign suture packaging, eliminating waste which created significant savings.
- Managed qualitative and quantitative research projects, as well as writing and editing reports utilizing primary and secondary data.
4. PowerPoint
PowerPoint is a presentation software used to create slide shows. Research analysts use PowerPoint to prepare presentations for meetings, reports, and briefings. They create charts and summaries using the software, and then present their findings to colleagues, executives, or government officials. They also use PowerPoint to support sales efforts, create client-ready presentations, and produce monthly and quarterly reports.
- Assisted economists in preparing PowerPoint presentations, vulnerability monitors, economic outlook assessments, and staff reports for their respective countries.
- Provide Russian language translation support and specialized assistance * Produce written reports and PowerPoint presentations in support of customer requirements
5. Market Research
Market research is the process of gathering and analyzing data about a target market. Research analysts use market research to support various business functions such as sales, marketing, and business development. They conduct qualitative and quantitative studies, analyze data, and provide recommendations for improvement. They also use market research to identify and exploit pricing inefficiencies, understand the product value proposition, and capture information on international corporations for B2B marketing.
- Conducted and analyzed qualitative and quantitative market research for national advertising clients of The Martin Agency and other leading advertising agencies.
- Market Research project experience includes Market Sizing and Forecasting, Customer Segmentation, New Product Concept Testing, Customer Satisfaction-Importance Analysis.
Python is a popular programming language used for various tasks like data analysis, automated reporting, and data cleaning. Research analysts use Python to extract data, perform statistical analysis, and create scripts for data storage and cleaning. They also use it to build company websites and analyze user engagement on web platforms. As Associate Professor Autumn Mathias Ph.D., LCSW from Elms College puts it, "quantitative research skills in particular are advantageous for many positions. This includes attaining coding skills and learning coding languages such as Python, R, and Java."
- Performed data extraction and statistical analysis of legal texts using custom designed and developed Python code.
- Performed statistical analyses on environmental data using Python and R statistical packages.
Choose from 10+ customizable research analyst resume templates
7. data entry.
Data entry is the process of inputting information into a computer system or database. Research analysts use data entry to process information, verify data, and update records. They enter customer information, research data, and statistical analysis results into spreadsheets and databases. They also use data entry to compare and resolve discrepancies, and to generate and track data clarifications and queries.
- Processed third party authorizations and customer address and telephone update requests through daily data entry.
- Performed data entry into spreadsheets and database of information located online for various computer products.
8. Statistical Analysis
Statistical analysis is the process of collecting and analyzing data to identify patterns and trends. Research analysts use statistical analysis to ensure the integrity of their data, create models, and identify factors that affect certain outcomes. They also use it to validate the reliability of new measures, manage data, and provide recommendations to clients. For example, they might use statistical analysis to understand the impact of management development on employee performance or identify the factors affecting the cost of switching to renewable electricity.
- Employed quality control procedures, including statistical analysis of index levels, used to ensure integrity of data that was disseminated.
- Performed statistical analysis and econometric modeling on project supporting the Social Security Administration on improving their modeling techniques regarding payment outcomes.
9. Healthcare
Healthcare is the act of taking preventative or necessary medical procedures to improve a person's well-being. Research analysts use healthcare in various ways, such as analyzing financial statements of healthcare companies, researching and updating information about healthcare providers, and conducting interviews with healthcare professionals. They also use it to develop policy recommendations to make healthcare more affordable, and to perform strategic and operational assessments for clients across the healthcare continuum.
- Determined prior authorizations for medication, Researched questions and clarified issues with patient claims and collaborated with pharmacies and healthcare provider.
- Persuaded Board of Directors and senior management to implement proposals for out-of-the-box innovation that influence innovation in healthcare and wellness initiatives.
SAS, or Statistical Analysis System, is a software suite used for data management, predictive analytics, and business intelligence. Research analysts use SAS to develop statistical models, forecast data, and create reports. They also use it to streamline operations, manage data, and perform statistical analyses. For example, a research analyst might use SAS to analyze data from internal company sources to troubleshoot problems, or to prepare reports on various indexes to support trading operations.
- Developed software to readily obtain information from several internal company sources for troubleshooting metering/billing problems using SAS and Visual Basic.
- Developed statistical models using SAS to forecast various indexes to support the mortgage-backed securities derivatives trading operations
11. Visualization
Visualization is the process of creating images or diagrams to help people better understand data. Research analysts use visualization to present complex information in a clear and simple way. They might use tools like Tableau or ArcGIS to create reports that are easy to understand. For example, they might use visualization to show how people use their cell phones or to help managers make better decisions about business strategy.
- Authored and delivered presentation introducing Tableau to the advertising sales team, allowing exposure to improvements in data visualization.
- Launched data visualization with Tableau environments.
12. Research Data
Research data is information collected and analyzed to answer a specific question or solve a problem. Research analysts use research data in various ways. They may travel to collect data, design frameworks for it, or analyze it to prepare presentations. They might also use it to develop financial models, update databases, or conduct statistical analyses. Research data can come from many sources, including surveys, scientific laboratory analyses, or online searches. Analysts use this data to gain insight into various fields, such as real estate, healthcare, or the entertainment industry.
- Traveled to elementary schools to collect qualitative research data for use in a national study over early childhood language development.
- Designed new preparedness research frameworks, preparedness research data systems in alignment with currently addressed national and state research agendas.
13. Analyze Data
Analyzing data is the process of examining information to identify patterns, trends, and correlations. Research analysts use this skill to manage data from various sources, such as biological, psychological, or clinical aspects of a subject. They also use statistical methods to analyze data, collaborate with other team members, and identify trends and patterns. As Shiri Noy Ph.D. , Assistant Professor at Denison University, points out, "Knowing how to collect, systematize, and analyze data. is something that is very appealing to employers. Having employees that can distinguish patterns and highlight key issues is highly prized."
- Manage and analyze data collected from biological, psychological, sociopolitical, and clinical aspects of substance use and related behavior.
- Worked cohesively and collaboratively with other team members to effectively analyze data from various energy assets and meet project deadlines.
SPSS is a software package used for statistical analysis. Research analysts use SPSS to determine correlations, conduct regression analyses, and perform data quality controls. They also use it to aggregate data and conduct various statistical tests to identify patterns of significance. For example, a research analyst might use SPSS to analyze data on market penetration and advertising rates, or to identify relationships between clients' nationalities and price expectations.
- Leveraged SPSS to determine statistically insignificant correlation between consumers' nationalities and price expectations on certain goods.
- Conducted statistical and regression analyses to determine relationships between advertising rates and market penetration using SPSS
SQL, or Structured Query Language, is a programming language used to manage and manipulate data in relational database management systems. Research analysts use SQL to extract and analyze data from databases. They create queries using SQL commands like "select", "join", and "where" to pull specific data and compile it into reports. For example, they might use SQL to track customer complaints, identify trends in claims data, or manage customer relationships. They also use SQL to optimize data storage and accessibility.
- Managed SQL Server customer complaint database; developed reports documenting quality and complaint trends.
- Developed an algorithm in SQL queries based on specialty pharmacy claims for early patient identification to increase enrolled members and revenue.
12 Research Analyst Resume Examples
Build a professional research analyst resume in minutes. Browse through our resume examples to identify the best way to word your resume. Then choose from 12 + resume templates to create your research analyst resume.
What skills help Research Analysts find jobs?
Tell us what job you are looking for, we’ll show you what skills employers want. Get Started
What type of skills will young Research Analysts need?
Business Instructor , San Francisco State University
What soft skills should all Research Analysts possess?
Professor, History Department , Humboldt State University
What skills stand out on Research Analyst resumes?
Associate Professor , Virginia Commonwealth University
What Research Analyst skills would you recommend for someone trying to advance their career?
Lecturer , Benjamin Franklin Institute of Technology
List of research analyst skills to add to your resume

The most important skills for a research analyst resume and required skills for a research analyst to have include:
- Data Analysis
- Data Collection
- Research Projects
- Market Research
- Statistical Analysis
- Visualization
- Research Data
- Analyze Data
- Customer Service
- Data Management
- Strong Analytical
- Real Estate
- Project Management
- Research Studies
- Research Findings
- Financial Statements
- Research Reports
- Literature Reviews
- Quantitative Research
- Quantitative Data
- Qualitative Data
- Government Agencies
- Qualitative Research
- Research Support
- Financial Analysis
- Business Development
- Primary Research
- Quantitative Analysis
- Financial Models
- Pivot Tables
- Due Diligence
- In-Depth Research
- Survey Data
- Media Research
- Research Results
Updated June 25, 2024
Editorial Staff
The Zippia Research Team has spent countless hours reviewing resumes, job postings, and government data to determine what goes into getting a job in each phase of life. Professional writers and data scientists comprise the Zippia Research Team.
Research Analyst Related Skills
- Analyst Skills
- Analyst Internship Skills
- Analyst Sales Skills
- Associate Analyst Skills
- Data Analyst Skills
- Data Analyst Internship Skills
- Data Research Analyst Skills
- Junior IS Analyst Skills
- Market Analyst Skills
- Market Analyst Internship Skills
- Market Research Analyst Skills
- Market Research Internship Skills
- Marketing Research Analyst Skills
- Marketing Research Internship Skills
- Operations Research Analyst Skills
Research Analyst Related Careers
- Analyst Internship
- Analyst Sales
- Associate Analyst
- Data Analyst
- Data Analyst Internship
- Data Research Analyst
- Junior IS Analyst
- Market Analyst
- Market Analyst Internship
- Market Research Analyst
- Market Research Internship
- Marketing Research Analyst
- Marketing Research Internship
- Operations Research Analyst
Research Analyst Related Jobs
- Analyst Jobs
- Analyst Internship Jobs
- Analyst Sales Jobs
- Associate Analyst Jobs
- Data Analyst Jobs
- Data Analyst Internship Jobs
- Data Research Analyst Jobs
- Junior IS Analyst Jobs
- Market Analyst Jobs
- Market Analyst Internship Jobs
- Market Research Analyst Jobs
- Market Research Internship Jobs
- Marketing Research Analyst Jobs
- Marketing Research Internship Jobs
- Operations Research Analyst Jobs
Research Analyst Jobs By Location
- Arlington Research Analyst Jobs
- Bossier City Research Analyst Jobs
- College Park Research Analyst Jobs
- Dallas Research Analyst Jobs
- Hawaiian Gardens Research Analyst Jobs
- Jacksonville Research Analyst Jobs
- Kings Park West Research Analyst Jobs
- New Haven Research Analyst Jobs
- New York Research Analyst Jobs
- Olive Branch Research Analyst Jobs
- Pittsford Research Analyst Jobs
- Rock Island Research Analyst Jobs
- Seattle Research Analyst Jobs
- Syosset Research Analyst Jobs
- West Haven Research Analyst Jobs
- Zippia Careers
- Business and Financial Industry
- Research Analyst
- Research Analyst Skills
Browse business and financial jobs
- Costs, Scholarships & Aid
- Campus Life
- Faculty & Staff
- Family & Visitors
- DFW Community
- Galaxy Login
- Academic Calendar
- Human Resources
- Accessibility
Doctor of Philosophy in Data Science and Statistics
Program description.
The Data Science and Statistics PhD degree curriculum at The University of Texas at Dallas offers extensive coursework and intensive research experience in theory, methodology and applications of statistics. During their study, PhD students acquire the necessary skills to prepare them for careers in academia or in fields that require sophisticated data analysis skills.
The PhD program is designed to accommodate the needs and interests of the students. The student must arrange a course program with the guidance and approval of the graduate advisor. Adjustments can be made as the student’s interests develop and a specific dissertation topic is chosen.
Some of the broad research areas represented in the department include: probability theory, stochastic processes, statistical inference, asymptotic theory, statistical methodology, time series analysis, Bayesian analysis, robust multivariate statistical methods, nonparametric methods, nonparametric curve estimation, sequential analysis, biostatistics, statistical genetics, and bioinformatics.
Career Opportunities
Statisticians generally find employment in fields where there is a need to collect, analyze and interpret data — including pharmaceutical, banking and insurance industries, and government — and also in academia. The job of a statistician consistently appears near the top in the rankings of 200 jobs by CareerCast’s Jobs Rated Almanac based upon factors such as work environment, income, hiring outlook and stress.
For more information about careers in statistics, view the career page of American Statistical Association. UT Dallas PhD graduates are currently employed as statisticians, biostatisticians, quantitative analysts, managers, and so on, and also as faculty members in universities.
The NSM Career Success Center is an important resource for students pursuing STEM and healthcare careers. Career professionals are available to provide strategies for mastering job interviews, writing professional cover letters and resumes and connecting with campus recruiters, among other services.
Marketable Skills
Review the marketable skills for this academic program.
Application Deadlines and Requirements
The university application deadlines apply with the exception that, for the upcoming Fall term, all application materials must be received by December 15 for first-round consideration of scholarships and fellowships. See the Department of Mathematical Sciences graduate programs website for additional information.
Visit the Apply Now webpage to begin the application process.
Contact Information
For more information, contact [email protected]
School of Natural Sciences and Mathematics The University of Texas at Dallas 800 W. Campbell Road Richardson, TX 75080-3021 Phone: 972-883-2416
nsm.utdallas.edu
Request More Information
Contact Email
We have received your request for more information, and thank you for your interest! We are excited to get to know you and for you to explore UT Dallas. You’ll begin receiving emails and information about our beautiful campus, excellent academic programs and admission processes. If you have any questions, email [email protected].
The University of Texas at Dallas respects your right to privacy . By submitting this form, you consent to receive emails and calls from a representative of the University.
* Required Field
800 W. Campbell Road Richardson, Texas 75080-3021
972-883-2111
Copyright Information
© The University of Texas at Dallas
Questions or comments about this page?
Stay Connected with UT Dallas
- Emergency Preparedness
- Campus Carry
- Campus Police
- Required links
- Tobacco-Free Campus
- Texas Veterans Portal
- Work at UT Dallas
- Nondiscrimination Policy
- Title IX Initiatives
- Student Achievements
- HEERF Reporting
- Counseling/Mental Health
- Hazing Prevention
- Public Course and Syllabus Information
- Privacy Policy
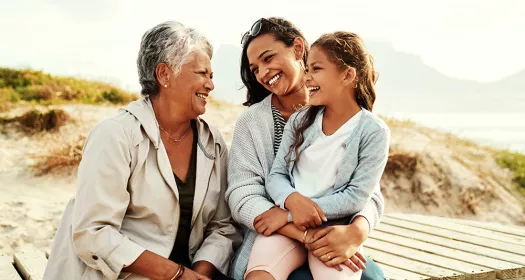
Working together, we can reimagine medicine to improve and extend people’s lives.
Principal Data Scientist Imaging and Vision AI Researcher
About the role.
Key responsibilities
- Lead AI research projects independently, focusing on imaging and vision applications within pharmaceutical research and development using scientific, rigorous, and reproducible methodology
- Develop and implement state-of-the-art AI algorithms for image processing, analysis, segmentation, and interpretation, focusing on data modalities such as MRI, histopathology, echocardiograms, lab animal video data or other image modalities used in biological research.
- Collaborate with other AI specialists, data architects, software engineers, business analysts, user interface designers within RX on products in the Image/Vision domain
- Act as an Imaging and data science subject matter expert, to help commodify AI and data analytics expertise and assets into impactful analysis workflows and software products, and to help make our research data FAIR
- Engage with diverse stakeholders in clinical as well as non-clinical settings
- Serve as an ambassador for AI/Data Science by presenting and publishing articles.
- Build a bridge between academia, technology, and industry partners.
- Keep ahead of latest developments in the field and mentor associates .
- Be a part of a truly unique organization, work with an inter-disciplinary team of highly accomplished scientists and push the AI frontiers for disease understanding and drug discovery.
This opportunity is located at the Novartis Cambridge (MA) site and will not have the ability to be located remotely. Relocation assistance may be available.
The pay range for this position at commencement of employment is expected to be between $144,000 and $216,000 per year; however, while salary ranges are effective from 1/1/24 through 12/31/24, fluctuations in the job market may necessitate adjustments to pay ranges during this period. Further, final pay determinations will depend on various factors, including, but not limited to geographical location, experience level, knowledge, skills and abilities. The total compensation package for this position may also include other elements, including a sign-on bonus, restricted stock units, and discretionary awards in addition to a full range of medical, financial, and/or other benefits (including 401(k) eligibility and various paid time off benefits, such as vacation, sick time, and parental leave), dependent on the position offered. Details of participation in these benefit plans will be provided if an employee receives an offer of employment. If hired, employee will be in an “at-will position” and the Company reserves the right to modify base salary (as well as any other discretionary payment or compensation program) at any time, including for reasons related to individual performance, Company or individual department/team performance, and market factors.
The Novartis Group of Companies are Equal Opportunity Employers and take pride in maintaining a diverse environment. We do not discriminate in recruitment, hiring, training, promotion or other employment practices for reasons of race, color, religion, gender, national origin, age, sexual orientation, gender identity or expression, marital or veteran status, disability, or any other legally protected status. We are committed to building diverse teams, representative of the patients and communities we serve, and we strive to create an inclusive workplace that cultivates bold innovation through collaboration and empowers our people to unleash their full potential.
Role Requirements
- Ph.D. in Computer Science, AI, Machine Learning, or a related field with a focus on imaging and vision applications.
- Minimum of 5 y ears of experience in AI research, specifically in imaging and vision applications.
- Applied knowledge of image/vision data modalities used in biological research or clinical settings
- Minimum of 4 y ears of relevant publication track record in machine learning applications and AI/ML methods
- Proven experience in leading AI research projects independently.
- Strong understanding of advanced machine learning algorithms and AI systems.
- Extensive experience in using AI for image analysis, particularly in a pharmaceutical setting.
- Proficient in programming in AI platforms such as TensorFlow, PyTorch, or Keras.
- Strong ability to communicate technical concepts to a variety of audiences, including scientists, engineers, and non-technical stakeholders.
- Familiarity with pharmaceutical research processes and requirements.
- Strong problem-solving skills and the ability to think creatively and innovatively.
Why Novartis? 766 million lives were touched by Novartis medicines in 2021, and while we’re proud of this, we know there is so much more we could do to help improve and extend people’s lives. We believe new insights, perspectives and ground-breaking solutions can be found at the intersection of medical science and digital innovation. That a diverse, equitable and inclusive environment inspires new ways of working. We believe our potential can thrive and grow in an unbossed culture underpinned by integrity, curiosity and flexibility. And we can reinvent what's possible, when we collaborate with courage to aggressively and ambitiously tackle the world’s toughest medical challenges. Because the greatest risk in life, is the risk of never trying! Imagine what you could do here at Novartis! Commitment to Diversity & Inclusion: Novartis is committed to building an outstanding, inclusive work environment and diverse teams representative of the patients and communities we serve. Accessibility and Reasonable Accommodations: Individuals in need of a reasonable accommodation due to a medical condition or disability for any part of the application process, or to perform the essential functions of a position, please send an e-mail to tas.nacomms@novartis.com or call +1 (877)395-2339 and let us know the nature of your request and your contact information. Please include the job requisition number in your message. Join our Novartis Network : If this role is not suitable to your experience or career goals but you wish to stay connected to hear more about Novartis and our career opportunities, join the Novartis Network here: https://talentnetwork.novartis.com/network
Why Novartis: Helping people with disease and their families takes more than innovative science. It takes a community of smart, passionate people like you. Collaborating, supporting and inspiring each other. Combining to achieve breakthroughs that change patients’ lives. Ready to create a brighter future together? https://www.novartis.com/about/strategy/people-and-culture
Join our Novartis Network: Not the right Novartis role for you? Sign up to our talent community to stay connected and learn about suitable career opportunities as soon as they come up: https://talentnetwork.novartis.com/network
Benefits and Rewards: Read our handbook to learn about all the ways we’ll help you thrive personally and professionally: https://www.novartis.com/careers/benefits-rewards
EEO Statement:
The Novartis Group of Companies are Equal Opportunity Employers who are focused on building and advancing a culture of inclusion that values and celebrates individual differences, uniqueness, backgrounds and perspectives. We do not discriminate in recruitment, hiring, training, promotion or other employment practices for reasons of race, color, religion, sex, national origin, age, sexual orientation, gender identity or expression, marital or veteran status, disability, or any other legally protected status. We are committed to fostering a diverse and inclusive workplace that reflects the world around us and connects us to the patients, customers and communities we serve.
Accessibility & Reasonable Accommodations
The Novartis Group of Companies are committed to working with and providing reasonable accommodation to individuals with disabilities. If, because of a medical condition or disability, you need a reasonable accommodation for any part of the application process, or to perform the essential functions of a position, please send an e-mail to [email protected] or call +1(877)395-2339 and let us know the nature of your request and your contact information. Please include the job requisition number in your message.
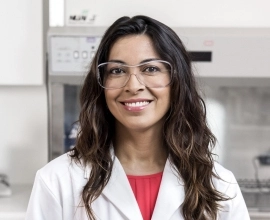
Analytical Skills
An individual’s ability to identify a problem, investigate to find out relevant facts, and find a logical solution
What are Analytical Skills?
Analytical skills refer to an individual’s ability to identify a problem, investigate to find out relevant facts, and find a logical solution. They are considered important skills in many different fields; however, it is especially important in finance when evaluating financial securities .
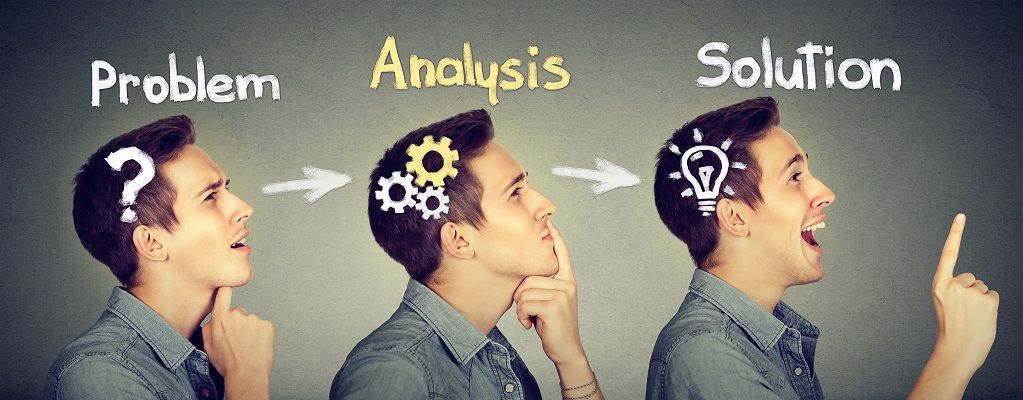
Analytical skills are the ability to collect information and to thoroughly analyze that information. They refer to problem-solving skills and making a decision based on insights drawn from the collected information.
Analytical skills are used when detecting patterns, brainstorming ideas, observing data, collecting data, interpreting data, integrating new information, synthesizing information, and making decisions based on the situation.
Types of Analytical Skills
The five types of skills that are crucial to success in many different jobs and industries are:
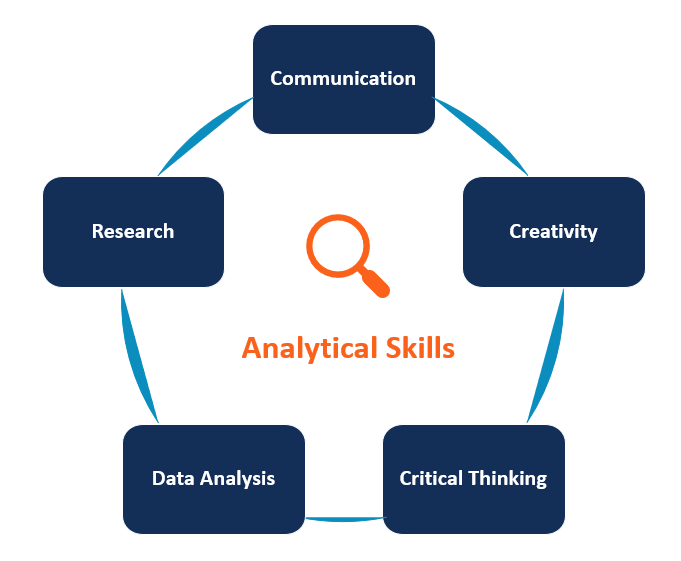
1. Communication
An underrated skill is the ability to communicate the analysis that has been conducted. Being able to identify problems and find solutions is useless if the conclusions and findings cannot be effectively communicated to decision-makers.
Findings from the analysis may need to be presented orally through a presentation or a meeting. The analysis may need to be presented in written form, either through a report or email. Therefore, communication skills are a critically important skill when developing overall analytical skills.
2. Creativity
Another important skill is the ability to think creatively. Many problems require “ out-of-the-box ” thinking in order to find the optimal solution. The obvious solution may not always be the most effective, and therefore, it is important to be able to think of creative solutions.
3. Critical Thinking
Critical thinking is exceptionally important to obtaining strong overall analytical skills. It refers to the objective analysis of a complex problem to form a judgment.
Effective critical thinkers are able to think clearly and rationally and understand the logical relations between data points and ideas. To find rational solutions, critical thinking is a very important skill to master.
4. Data Analysis
Data is very important in all disciplines, specifically, the ability to analyze large volumes of data and identify patterns and trends. Therefore, it is increasingly vital to understand the discipline of statistics and quantitative analysis . Data analysis is becoming more prominent within finance, as trends such as technical trading and quant-investing continue to grow in popularity.
Quantitative analysis requires identifying patterns in data but also being able to define the findings to decision-makers, which ties into the communication aspect of analytical skills.
5. Research
Applying analytical skills requires patience and curiosity. If a problem is solved quickly, without considering all relevant information, then it is highly unlikely that it will be the most effective solution. Therefore, coming up with an effective solution requires taking the time to learn more about the problem before attempting to solve it.
Research requires collecting information and relevant data, then compiling it before analyzing it. Collecting data and thoroughly researching a topic is important for being able to apply strong analytical skills.
Importance of Analytical Skills
Analytical skills are important because they allow people to find solutions to various problems and make concrete decisions and action plans to solve those problems. As mentioned earlier, they are important skills in all disciplines, ranging from data science, project management, marketing, law, medicine, scientific research, accounting, etc.
Analytical skills are exceptionally important in finance. Financial analysts must be able to synthesize large volumes of data, analyze the data, and find a solution to various problems. For example, consider an equity research analyst for an investment fund. The problem that needs to be solved is finding the ideal equity investment that fits the portfolio’s philosophy.
In order for the analyst to solve such a problem, they must take financial information from reports of companies, pricing information, information on the current portfolio, and other relevant data points.
Next, the analyst must analyze the information and find an ideal equity investment with an optimal return and risk profile, as well as an investment that aligns with the fund’s investing philosophy. Lastly, the analyst must be able to communicate their findings to the portfolio manager, so that an investment decision can be made in a timely manner.
More Resources
CFI now offers the Business Essentials Bundle with courses on Microsoft Excel, Word, and PowerPoint, business communication, data visualization, and an understanding of corporate strategy. To keep learning, we suggest these resources:
- Adult Learning Theory
- Emotional Intelligence
- Credit Analyst Skills
- Vocational Degree
Take the FREE DISC Assessment and take the FREE Personality Testing Using DISC online course through Getting People Right.
See also our management & strategy resources .
- Share this article
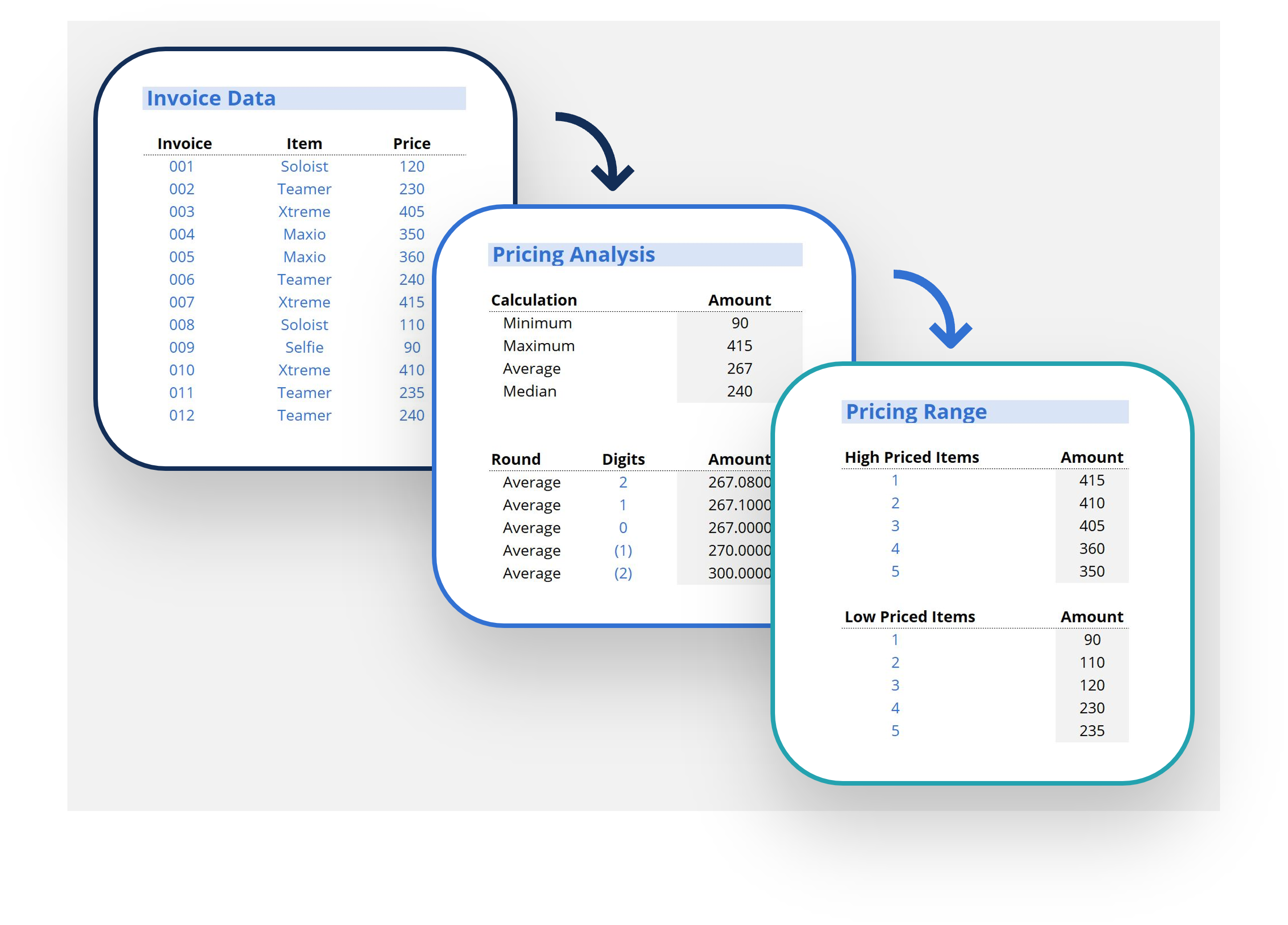
Create a free account to unlock this Template
Access and download collection of free Templates to help power your productivity and performance.
Already have an account? Log in
Supercharge your skills with Premium Templates
Take your learning and productivity to the next level with our Premium Templates.
Upgrading to a paid membership gives you access to our extensive collection of plug-and-play Templates designed to power your performance—as well as CFI's full course catalog and accredited Certification Programs.
Already have a Self-Study or Full-Immersion membership? Log in
Access Exclusive Templates
Gain unlimited access to more than 250 productivity Templates, CFI's full course catalog and accredited Certification Programs, hundreds of resources, expert reviews and support, the chance to work with real-world finance and research tools, and more.
Already have a Full-Immersion membership? Log in
- Cette page n'est pas disponible en Français
Measuring the demand for AI skills in the United Kingdom
- United Kingdom
- Artificial Intelligence
- Future of work
- Digital transformation
- AI and work

Cite this content as:
This paper estimates the artificial intelligence-hiring intensity of occupations/industries (i.e. the share of job postings related to AI skills) in the United Kingdom during 2012-22. The analysis deploys a natural language processing algorithm (NLP) on online job postings, collected by Lightcast, which provides timely and detailed insights into labour demand for different professions. The key contribution of the study lies in the design of the classification rule identifying jobs as AI-related which, contrary to the existing literature, goes beyond the simple use of keywords. Moreover, the methodology allows for comparisons between data-hiring intensive jobs, defined as the share of jobs related to data production tasks, and AI-hiring intensive jobs. Estimates point to a rise in the economy-wide AI-hiring intensity in the United Kingdom over the past decade but to fairly small levels (reaching 0.6% on average over the 2017-22 period). Over time, the demand for AI-related jobs has spread outside the traditional Information, Communication and Telecommunications industries, with the Finance and Insurance industry increasingly demanding AI skills. At a regional level, the higher demand for AI-related jobs is found in London and research hubs. At the occupation level, marked changes in the demand for AI skills are also visible. Professions such as data scientist, computer scientist, hardware engineer and robotics engineer are estimated to be the most AI-hiring intense occupations in the United Kingdom. The data and methodology used allow for the exploration of cross-country estimates in the future.
In the same series

Related publications

- How's Life in United Kingdom? 30 July 2024

- Open access
- Published: 10 September 2024
Analysis of virtual standardized patients for assessing clinical fundamental skills of medical students: a prospective study
- Xinyu Zhang ORCID: orcid.org/0009-0000-2893-3986 1 , 2 na1 ,
- Duo Zeng 2 na1 ,
- Xiandi Wang 2 ,
- Yaoyu Fu ORCID: orcid.org/0000-0002-5890-4745 3 ,
- Ying Han 2 ,
- Manqing He 2 ,
- Xiaoling Chen 2 &
- Dan Pu 1 , 2
BMC Medical Education volume 24 , Article number: 981 ( 2024 ) Cite this article
Metrics details
History-taking is an essential clinical competency for qualified doctors. The limitations of the standardized patient (SP) in taking history can be addressed by the virtual standardized patient (VSP). This paper investigates the accuracy of virtual standardized patient simulators and evaluates the applicability of the improved system’s accuracy for diagnostic teaching support and performance assessment.
Data from the application of VSP to medical residents and students were gathered for this prospective study. In a human–machine collaboration mode, students completed exams involving taking SP histories while VSP provided real-time scoring. Every participant had VSP and SP scores. Lastly, using the voice and text records as a guide, the technicians will adjust the system’s intention recognition accuracy and speech recognition accuracy.
The research revealed significant differences in scoring across several iterations of VSP and SP ( p < 0.001). Across various clinical cases, there were differences in application accuracy for different versions of VSP ( p < 0.001). Among training groups, the diarrhea case showed significant differences in speech recognition accuracy ( Z = -2.719, p = 0.007) and intent recognition accuracy ( Z = -2.406, p = 0.016). Scoring and intent recognition accuracy improved significantly after system upgrades.
VSP has a comprehensive and detailed scoring system and demonstrates good scoring accuracy, which can be a valuable tool for history-taking training.
Peer Review reports
History-taking is an essential skill for becoming a competent doctor, and it is a fundamental component of work in various medical fields [ 1 ]. History-taking typically includes general data, chief complaints, present history, past medical history, family history, social history, and review of systems, etc. Since it directs subsequent exams, diagnosis, and treatment choices, gathering patient histories is the first and most important stage in identifying medical conditions [ 2 ]. Therefore, it is vital to provide medical professionals with training in history-taking before they engage in clinical practice [ 3 , 4 , 5 , 6 ].
Currently, the most common method for teaching history-taking skills combines theoretical instruction with simulation-based education, with Standardized Patients (SP) as the primary method of simulation. SP are individuals who have undergone standardized and systematic training to accurately, realistically, and consistently portray the characteristics, psychosocial features, and emotional responses required for specific medical cases [ 7 , 8 ]. Doctor-patient communication encompasses both verbal and non-verbal components, which are equally important [ 2 , 9 ]. During the diagnostic process, doctors collect information from patients by observing their facial expressions and body language. Similarly, doctors use body language and facial expressions to encourage and ensure patient comfort [ 10 , 11 ]. The United States first used SP for clinical teaching in the 1960s, and China adopted the practice in the 1990s [ 12 ]. SP teaching is a valuable bridge between theoretical instruction and clinical practice. It not only facilitates the simulation of authentic medical scenarios without ethical concerns but also boosts student engagement, enhances clinical communication skills, supports the acquisition of medical knowledge, and promotes a deeper grasp of abstract concepts [ 13 ].
However, the use of SP in medical education has its own challenges. The training process for SP is rigorous, time-consuming, and resource-intensive. Consequently, the availability of qualified SP is limited [ 14 , 15 ]. In the process of SP teaching evaluation, the influence of subjective factors cannot be avoided [ 16 ]. The lengthy and strict training process, resulting in the scarcity of SP, makes it challenging to implement one-on-one history-taking training effectively. To address these limitations of SP, virtual standardized patient (VSP) offers a potential solution. As early as the early twenty-first century, research suggested using computers to aid in history-taking exercises [ 17 , 18 , 19 , 20 ], but VSP has not become widely adopted. Implementing VSP in history-taking instruction can effectively address the limitations found in SP. It reduces the lengthy training time and costs associated with training SP, allows for repetitive training [ 21 , 22 ], and facilitates the assessment of teaching effectiveness [ 23 ], thereby boosting student confidence [ 24 , 25 ]. It reduces the potential subjectivity of both instructors and SP, enabling a more objective and standardized evaluation [ 23 ].
We developed a VSP according to the needs, using speech recognition technology, intention recognition technology, and automatic scoring. VSP initially used sentence similarity matching and then improved to intention recognition. The article aims to explore the accuracy of VSP and assess whether the upgraded system’s accuracy can be applied to diagnostic teaching assistance and performance evaluation. This research highlights certain limitations in SP physician training and examines the application accuracy of our independently developed VSP. The goal is to establish a foundation for more effective teaching strategies.
This study utilizes a virtual standardized patient history-taking system jointly developed by our institution and Shanghai Chuxin Medical Technology Co., Ltd., based on speech recognition and intent recognition technology. The system operates in both a human–computer dialogue mode and a human–computer collaborative mode, as detailed in Fig. 1 . This research employs the latter.
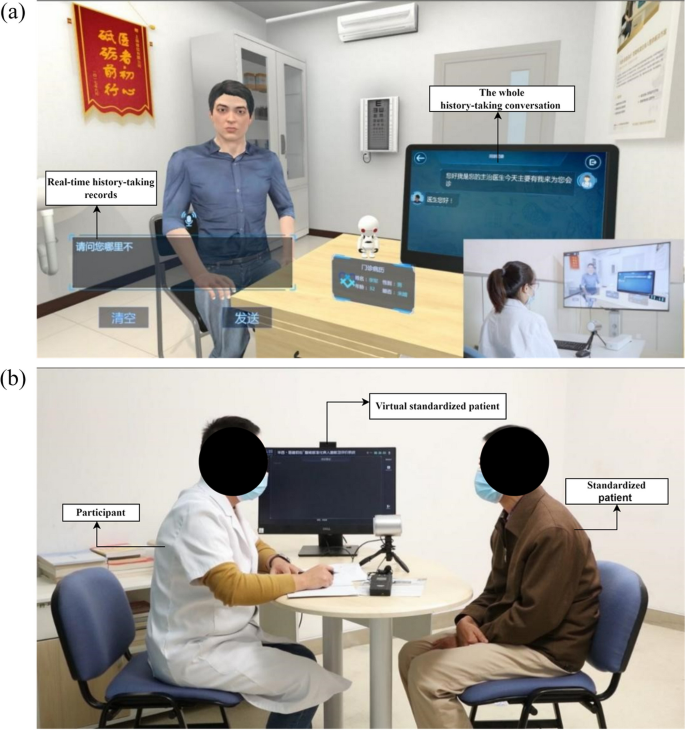
Human–computer dialogue mode and human–computer collaborative mode of VSP a human–computer dialogue mode; b human–computer collaborative mode
The system first converts the spoken dialogue into text. Subsequently, the sentences are dissected, breaking them down into phrases. Following part-of-speech recognition and classification, these sentences are compared to the intent templates kept in the intent library to provide assessments and comments. After gathering all the data, the system performs self-learning to adjust the corpus [ 26 ]. The specific process is illustrated in Fig. 2 .
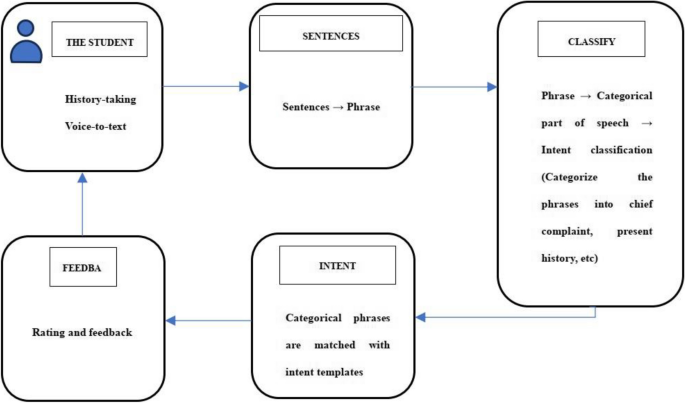
The specific process of the virtual standardized patient history-taking system based on speech recognition technology and artificial intelligence
VSP underwent a general system replacement during the experiment, with VSP 1.0 being the old version and VSP 2.0 and VSP 3.0 being the new version. VSP 1.0 compares sentences and keywords with standard statements, for the old version of the system. It is considered the same statement when the sentences are similar to the standard statements [ 27 ]. For the updated version of the system, VSP 2.0 divides the sentences into phrases, categorizes the phrases, and matches the intent templates of the phrases [ 28 ]. VSP 3.0 is the version of VSP 2.0 self-learning optimization [ 29 , 30 , 31 , 32 ]. The differences between the old and new versions are shown in Fig. 3 .
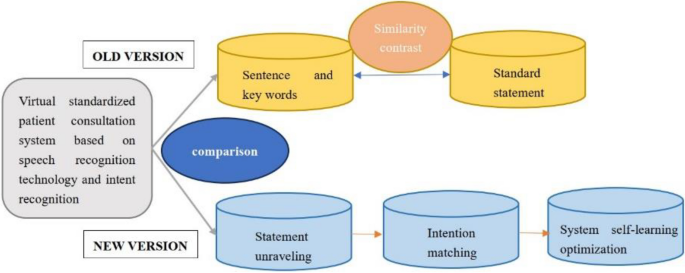
Comparison of old and new VSP
Design, setting and subjects
We adopted a prospective study design. The Biomedical Ethics Committee of West China Hospital, Sichuan University approved this study (Approval 2019 No.1071). In this study, we applied different versions of VSP to assess the clinical performance of medical students with no prior clinical experience and residents, using a human–computer collaborative model for evaluation. In the study population, clinical medical students were recruited from the annual diagnostics course, while residents were selected from the enhanced training sessions, which covered three years. Participants willing to use VSP are recruited from these two courses, and the scoring results of history-taking can be compared with those of SP. Informed consent was obtained from all participants before the tests, and they were informed that the results of this study would not affect their final course grades. All participants had previously received theoretical instruction in medical history taking.
Measurements
In this study, the accuracy of the VSP application was determined. The application accuracy included speech recognition accuracy, intention recognition accuracy, and scoring accuracy. Speech recognition accuracy is the ratio of correctly recognized characters to the total number of characters. Intent recognition accuracy is the ratio of correctly matched phrases to the total number of phrases. The system automatically determines the accuracy of speech recognition and intent recognition, and it separates intent matches with a probability of less than 80%. The results were reviewed manually by two technicians. If their results differed, a third technician made the final decision. The score consisted of the content of history-taking and the skill of history-taking, with a total of 70 scoring points, and the total score of each scoring point was different. The Department of Diagnostics’ multidisciplinary team established the scoring scale, which has been validated and applied for many years. The scale underwent minor modifications based on actual conditions to ensure quality control. Because SP is highly trained and experienced, we calculated the VSP’s scoring accuracy using its scores as the gold standard. Scoring accuracy was calculated as the ratio of the number of scoring points with the same VSP and SP scores to the total score points.
In this study, the participants randomly selected one case from the four cases (diarrhea, syncope, palpitation, cough) during the assessment process. Throughout the assessment, SP acted as a patient and interacted with participants performing the role of doctors. The VSP was placed next to it without responding, collecting information for real-time scoring. As a result, two sets of scores were obtained from SP and VSP. After the examination, participants were invited to complete relevant questionnaires voluntarily (results are shown in the appendices). Following history-taking training with SP, both SP and VSP scores are simultaneously obtained, and participants provide feedback about the VSP after comparing these scores. Speech and intent recognition employ mature commercial technologies, with accuracy automatically generated by the system upon the corpus. Lastly, technicians reviewed the texts and recordings to assess and adjust the accuracy provided by the system. See Fig. 4 for details.
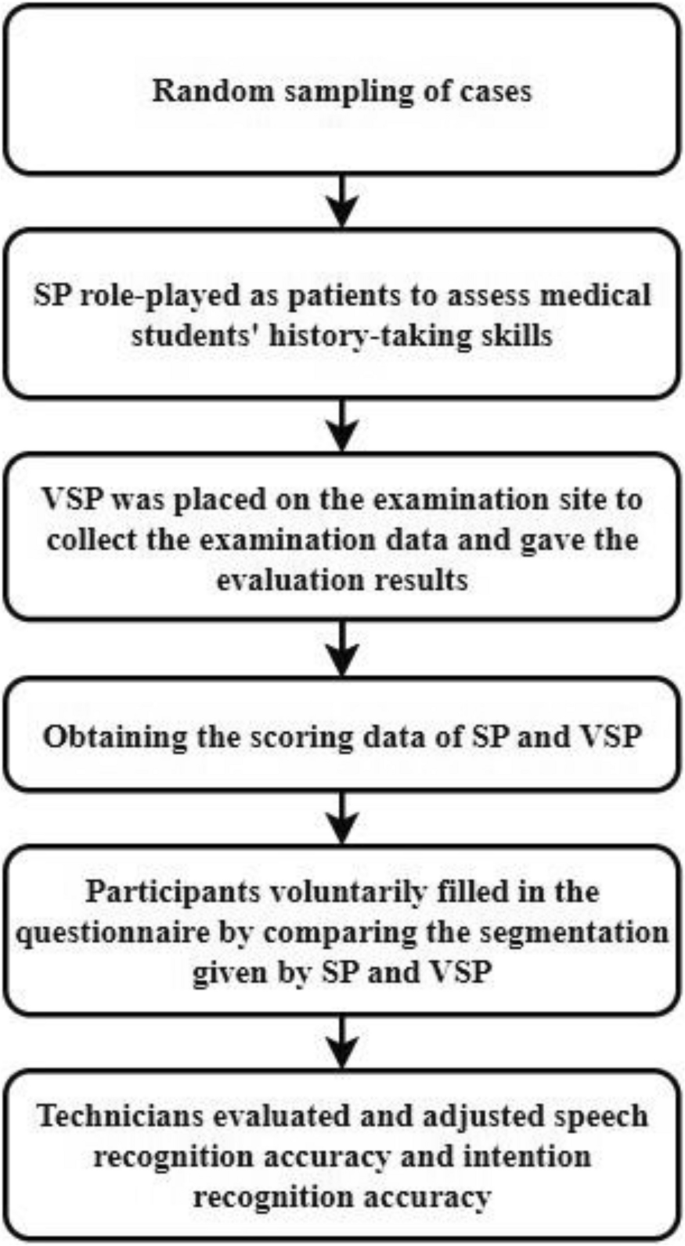
The procedure of the study
Data analysis
Since the data did not meet the normal distribution, we used the Wilcoxon rank sum test when comparing the SP and VSP scores. Analysis of variance (ANOVA) was used to test the significance of the differences in the accuracy of scoring, speech recognition, and intent recognition of VSP across different VSP versions in various cases. Kruskal–Wallis one-way ANOVA tests were used for pairwise comparisons, with post hoc analysis using the Bonferroni correction. The independent t -test was used to compare the accuracy of scoring, speech recognition, and intent recognition of VSP 3.0 in different cases between medical students and residents. p < 0.05 was considered statistical significance.
Demographic characteristics
A total of 502 students participated in the study over the three years. Of these, 476 students were included in the final analysis, as 26 students’ data were not recorded due to VSP 1.0 system problems. Among the included participants, 89 medical students used VSP 1.0, 129 used VSP 2.0, and 104 used VSP 3.0. The 154 residents used VSP 3.0. Statistics were based on different versions and randomly selected cases, as shown in Table 1 :
The medical students who used different versions of VSP are at similar stages of learning history-taking, with comparable ages. Residents have more clinical experience than medical students in the same stage of training.
Comparison of history-taking scores given by SP and VSP
The t-test revealed significant differences in the scores given by SP and VSP for both medical students ( Z = -8.194, p < 0.05; Z = -9.864, p < 0.05; Z = -8.867, p < 0.05) and residents ( Z = -10.773, p < 0.05). Generally, VSP scores were lower than SP scores. The score distribution was skewed, mainly in the high-score range, as shown in Table 2. .
Comparison of VSP application accuracy
Our study examined four distinct medical scenarios, comparing the application accuracy of various VSP versions and determining whether there are variations in accuracy while using VSP 3.0 with medical students and residents.
Comparison of VSP application accuracy in diarrhea cases
The results indicated significant differences in the application accuracy ( H = 42.424, p < 0.001; H = 27.220, p < 0.001; H = 44.135, p < 0.001) among the three versions of the VSP system. Multiple mean comparisons revealed significant differences in scoring accuracy between VSP 1.0 and VSP 2.0 ( p < 0.001), VSP 1.0 and VSP 3.0 ( p < 0.001). The speech recognition accuracy between VSP 1.0 and VSP 3.0 ( p < 0.001), and VSP 2.0 and VSP 3.0 ( p < 0.001) was significantly different. Intent recognition accuracy was significantly different between VSP 1.0 and VSP 2.0 ( p < 0.001), VSP 1.0 and VSP 3.0 ( p < 0.001). The results are presented in Table 3 .
When instructing medical students and residents in history-taking using VSP 3.0, a t-test was employed to determine whether there were any significant differences in application accuracy between the two groups. The results showed significant differences in speech recognition accuracy ( Z = -2.719, p = 0.007) and intent recognition accuracy ( Z = -2.406, p = 0.016). The results are presented in Table 4 .
Comparison of VSP application accuracy in syncope cases
There were significant differences in the application accuracy ( H = 34.506, p < 0.001; H = 27.233, p < 0.001; H = 38.485, p < 0.001). Multiple mean comparison results showed significant differences in scoring accuracy between VSP 1.0 and VSP 2.0 ( p < 0.001), as well as between VSP 1.0 and VSP 3.0 ( p < 0.001). Significant differences were observed in speech recognition accuracy between VSP 1.0 and VSP 2.0 ( p < 0.001), between VSP 1.0 and VSP 3.0 ( p = 0.016), and between VSP 2.0 and VSP 3.0 ( p = 0.019). Intent recognition accuracy exhibited significant differences between VSP 1.0 and VSP 2.0 ( p < 0.001), between VSP 1.0 and VSP 3.0 ( p < 0.001), and between VSP 2.0 and VSP 3.0 ( p = 0.036). The results are presented in Table 5 .
There were no significant differences in application accuracy ( Z = -0.426, p = 0.670; Z = -0.216, p = 0.829; Z = -0.035, p = 0.972) between medical students and residents using VSP 3.0 in syncope cases. The results are presented in Table 6 .
Comparison of VSP application accuracy in palpitation cases
There were significant differences in the application accuracy ( H = 71.858, p < 0.001; H = 23.986, p < 0.001; H = 77.121, p < 0.001). Multiple mean comparison results showed significant differences in scoring accuracy between VSP 1.0 and VSP 2.0 ( p < 0.001), as well as between VSP 1.0 and VSP 3.0 ( p < 0.001). Significant differences were observed in speech recognition accuracy between VSP 1.0 and VSP 2.0 ( p = 0.011), VSP 1.0 and VSP 3.0 ( p < 0.001), and between VSP 2.0 and VSP 3.0 ( p = 0.035). Intent recognition accuracy exhibited significant differences between VSP 1.0 and VSP 2.0 ( p < 0.001), VSP 1.0 and VSP 3.0 ( p < 0.001), and between VSP 2.0 and VSP 3.0 ( p = 0.033). The results are presented in Table 7 .
The results showed no significant differences in application accuracy ( t = 1.055, p = 0.132; t = 0.138, p = 0.068; t = -0.872, p = 0.557) when using VSP 3.0 for teaching medical students and residents in palpitation cases. The results are presented in Table 8 .
Comparison of VSP application accuracy in cough cases
There were significant differences in the application accuracy ( H = 40.521, p < 0.001; H = 18.961, p < 0.001; F = 235.851, p < 0.001). Multiple mean comparison results indicated significant differences in scoring accuracy between VSP 1.0 and VSP 2.0 ( p < 0.001), as well as between VSP 1.0 and VSP 3.0 ( p < 0.001). Significant differences were observed in speech recognition accuracy between VSP 1.0 and VSP 2.0 ( p < 0.001), as well as between VSP 1.0 and VSP 3.0 ( p = 0.011). Intent recognition accuracy exhibited significant differences between VSP 1.0 and VSP 2.0 ( p < 0.001), between VSP 1.0 and VSP 3.0 ( p < 0.001), and between VSP 2.0 and VSP 3.0 ( p < 0.001). The results are presented in Table 9 .
There were no significant differences in application accuracy ( t = 0.276, p = 0.241; t = -4.933, p = 0.186; t = -0.486, p = 0.309) when using VSP 3.0 for teaching medical students and residents in cough cases. The results are presented in Table 10 .
Changes in the accuracy of different cases
We conducted an analysis and comparison of scoring accuracy, speech recognition accuracy, and intent recognition accuracy (Fig. 5 ). Results showed that both scoring accuracy and intent recognition accuracy increased with the upgrade of the VSP version, and the standard deviation decreased. However, the trend in speech recognition accuracy varied depending on the cases. In the VSP 1.0 version, the syncope cases showed the best accuracy in speech recognition and intent recognition, followed by diarrhea, palpitations, and coughing. In the VSP 2.0 and VSP 3.0 versions, scoring and intent recognition accuracy were nearly identical for all four cases.
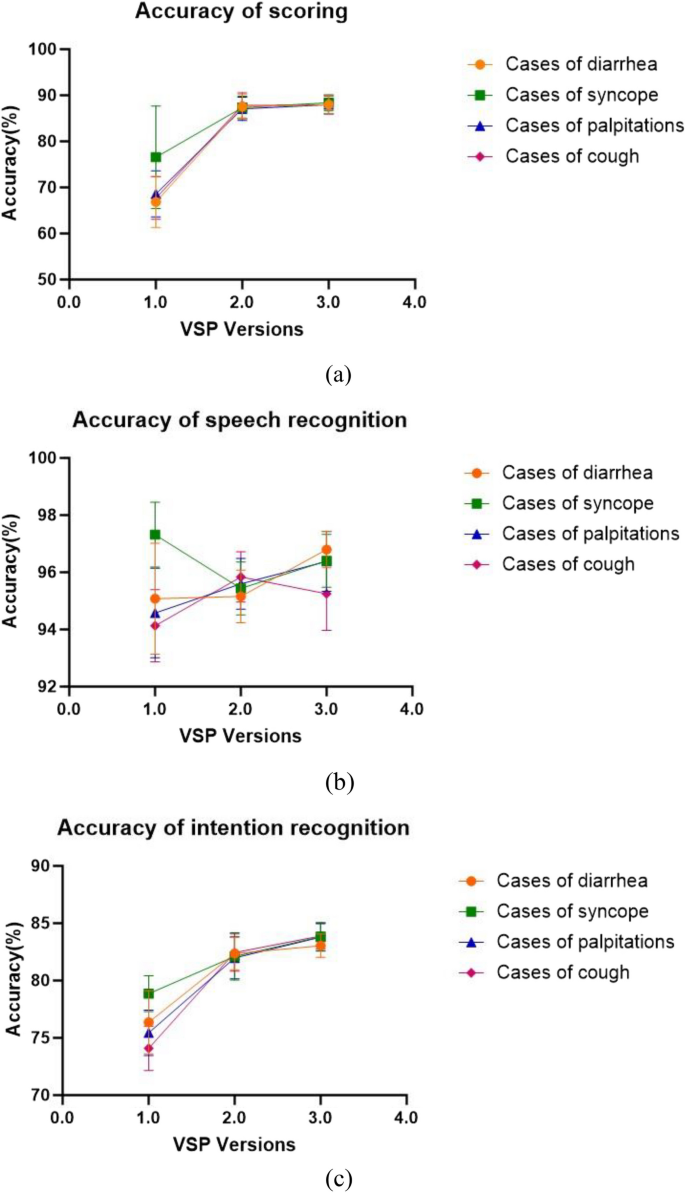
The accuracy trend chart and the maximum accuracy was 100%. a is the accuracy of scoring; b is the accuracy of speech recognition; c is the accuracy of intention recognition
We explore the accuracy of our self-developed VSP simulator for assessing history-taking skills. While intent recognition and score accuracy have increased after updates and optimizations, speech recognition accuracy has continuously maintained a high level. The VSP application accuracy has stabilized after optimization and updating, continuously reaching high levels in various scenarios. The application accuracy of VSP does not vary depending on the population.
It is clear from the statistics in Table 2. that VSP scores are generally lower than SP scores. This finding aligns with the results of a study by Fink and others [ 33 ]. Fink et al. attributed this to the lower subjects’ interest, reduced appraisal of motivational value, and decreased quantity of evidence generation reported for VPs. However, our VSP did not engage in human–computer dialogue, so we believe the reason is different. Based on the analysis of this study, the reasons may be attributed to the overall operational processes and assessment methods of the VSP system. The accuracy of the system’s voice recognition and intention recognition might have affected the scoring accuracy since VSP will first translate the speech into text, then perform intention recognition, and finally provide the score. The lower score of VSP compared to SP may result from the recognition and classification error of speech and intention. Therefore, our study further explored the scoring accuracy, speech recognition accuracy, and intent recognition accuracy of VSP.
Considering the potential confounding effects of different case content, we conducted separate analyses of scoring accuracy, speech recognition accuracy, and intent recognition accuracy for each of the four cases: diarrhea, syncope, palpitations, and cough. All versions of VSP used these same four cases. We analyzed the scoring accuracy, speech recognition accuracy, and intent recognition accuracy of different VSP versions in these cases. The results all showed significant differences.
We also looked at any discrepancies in accuracy between medical students and residents using VSP 3.0. Among the results of comparing different groups, significant differences were observed only in the case of diarrhea, where speech recognition accuracy and intent recognition accuracy showed differences. The reason may be that medical students and residents conducted history-taking in different ways. Medical students, lacking clinical experience, tend to follow a standardized model provided by professors, making their approach easily recognizable by the system. In contrast, residents have some clinical experience, which leads to various inquiry styles that pose certain identification challenges. Furthermore, there are regional and dialectal accent variances in Chinese, which contributes to some degree of mistakes in the voice recognition system.
Based on the scoring accuracy data, the latest version of VSP achieved an accuracy rate of 85.40–89.62%, which aligns with similar research findings. In a study by William and colleagues [ 34 ] response accuracy ranged from 84 to 88%, and in Maicher et al.’s study [ 35 ], response accuracy ranged from 79 to 86%. The construction of this system has been relatively successful. However, future work should focus on enriching the synonym database and improving accuracy. The results of pairwise comparisons indicate a significant improvement in scoring accuracy with the newer system versions, i.e. VSP 2.0 and VSP 3.0. However, when compared with VSP 2.0, VSP 3.0 showed no improvement in scoring accuracy, indicating that the system’s self-learning functionality has a limited impact on enhancing scoring accuracy. The reason might be insufficient data in the collected corpus and insufficient time for the machine’s self-learning. It remains uncertain whether the self-learning feature of VSP has any impact on scoring accuracy. Further research is needed to confirm whether the self-learning functionality of VSP affects scoring accuracy.
In the four medical cases, speech recognition accuracy is relatively high. After pairwise comparisons, no specific patterns causing significant differences in speech recognition accuracy were observed in the data. There are possible reasons for this phenomenon. Firstly, regional and ethnic differences may contribute to distinct accents, especially when the system is designed for standard Mandarin. Secondly, speaking at a fast pace could cause the system to have difficulty accurately capturing the spoken words. Lastly, the system may fail to recognize or accurately identify sentence breaks, which could also be a contributing factor. When speech-to-text conversion fails to accurately convey the intended meaning, the system responds with errors or fails to respond. This aligns with the findings of Kammoun et al. [ 36 ], whose system automatically moves to the next section if it cannot accurately recognize the speech. In the future, adjustments can be made to the system to optimize the speech recognition section by customizing response time intervals for each individual.
Overall, intent recognition accuracy has been consistently improving. This suggests that VSP 2.0 successfully addressed the issue of intent recognition accuracy compared to VSP 1.0, and its self-learning feature provides an advantage in enhancing intent recognition accuracy. Based on the research results, it can be inferred that VSP’s intent recognition accuracy does not vary with different experience groups. Future research should include a more diverse range of participants to validate these findings.
The findings (Fig. 5 ) indicate that scoring accuracy and intent recognition accuracy improve with the upgrade of the VSP version. However, speech recognition accuracy varies across different cases. This discrepancy can be attributed to factors mentioned earlier, such as the subject’s accent, speaking speed, and sentence breakage, posing challenges for VSP recognition. In VSP 1.0, there was a notable standard deviation in application accuracy, with diarrhea cases showing the highest accuracy. This variation may be linked to VSP 1.0’s slight instability and differing word recognition accuracy across cases. The scoring process involves speech recognition followed by intent recognition, leading to relatively consistent results in scoring accuracy, speech recognition accuracy, and intent recognition accuracy in VSP 1.0.
This study only utilized the system’s examination mode, focusing solely on speech conversion and score feedback. The system we employed has an additional human–computer interaction mode that can be used for student history-taking training, which we did not explore in this study. Moreover, the system’s comprehension of voice text and response accuracy were not examined in this study. These aspects can be studied in future research. Furthermore, we only examined a few key metrics, including VSP score accuracy, speech recognition accuracy, and intent recognition accuracy, without discussing all the metrics. Additionally, this study only included medical students and residents. Extra variables for various demographics should be considerate for analysis to investigate the correctness of the application of VSP in various population groupings.
VSP proves to be a feasible way to train history-taking skills. This study describes the scoring process of our self-developed VSP and reveals its commendable application accuracy. The upgrading and the self-learning function of the system have played a role in improving the stability and accuracy of VSP. At this point, the accuracy of VSP 3.0 has reached the level required for the history-taking training auxiliary tool, opening up possibilities for integrating diagnostic training tools into clinical education, and effectively addressing the shortage of opportunities for students in SP training. In the future, continuous optimization of VSP will position it as a reliable training and assessment tool, fostering students’ independent learning abilities in classroom teaching.
Availability of data and materials
The datasets used and analyzed during the current study are available from the corresponding author upon reasonable request.
Tawanwongsri W, Phenwan T. Reflective and feedback performances on Thai medical students’ patient history-taking skills. BMC Med Educ. 2019;19(1):141.
Article Google Scholar
Vogel D, Meyer M, Harendza S. Verbal and non-verbal communication skills including empathy during history taking of undergraduate medical students. BMC Med Educ. 2018;18(1):157.
Alharbi L, Almoallim H. History-Taking Skills in Rheumatology. In: Almoallim H, Cheikh M, editors. Skills in Rheumatology. Singapore: Springer; 2021. p. 3–16.
Chapter Google Scholar
Kantar A, Marchant JM, Song WJ, Shields MD, Chatziparasidis G, Zacharasiewicz A, Moeller A, Chang AB. History taking as a diagnostic tool in children with chronic cough. Front Pediatr. 2022;10:850912.
Steinkellner C, Schlömmer C, Dünser M. Medical history taking and clinical examination in emergency and intensive care medicine. Med Klin Intensivmed Notfmed. 2020;115(7):530–8.
Altshuler L, Wilhite JA, Hardowar K, Crowe R, Hanley K, Kalet A, Zabar S, Gillespie C, Ark T. Understanding medical student paths to communication skills expertise using latent profile analysis. Med Teach. 2023;45(10):1140–7.
Barrows HS. An overview of the uses of standardized patients for teaching and evaluating clinical skills. AAMC. Acad Med. 1993;68(6):443–51. Discussion 451–443.
Gillette C, Stanton RB, Rockich-Winston N, Rudolph M, Anderson HG Jr. Cost-effectiveness of using standardized patients to assess student-pharmacist communication skills. Am J Pharm Educ. 2017;81(10):73–9.
Bagacean C, Cousin I, Ubertini A-H, El Yacoubi El Idrissi M, Bordron A, Mercadie L, Garcia LC, Ianotto J-C, De Vries P, Berthou C. Simulated patient and role play methodologies for communication skills and empathy training of undergraduate medical students. BMC Med Educ. 2020;20(1):491.
Ishikawa H, Hashimoto H, Kinoshita M, Fujimori S, Shimizu T, Yano E. Evaluating medical students’ non-verbal communication during the objective structured clinical examination. Med Educ. 2006;40(12):1180–7.
Mehrabian A, Ferris SR. INFERENCE OF ATTITUDES FROM NONVERBAL COMMUNICATION IN 2 CHANNELS. J Consult Psychol. 1967;31(3):248–52.
Stillman PL, Sawyer WD. A new program to enhance the teaching and assessment of clinical skills in the People’s Republic of China. Acad Med. 1992;67(8):495–9.
Liu T, Luo J, He H, Zheng J, Zhao J, Li K. History-taking instruction for baccalaureate nursing students by virtual patient training: A retrospective study. Nurse Educ Today. 2018;71:97–104.
Aranda JH, Monks SM. Roles and Responsibilities of the Standardized Patient Director in Medical Simulation. StatPearls. Treasure Island (FL): StatPearls Publishing LLC; 2023.
Google Scholar
Zhang S, Soreide KK, Kelling SE, Bostwick JR. Quality assurance processes for standardized patient programs. Curr Pharm Teach Learn. 2018;10(4):523–8.
Du J, Zhu X, Wang J, Zheng J, Zhang X, Wang Z, Li K. History-taking level and its influencing factors among nursing undergraduates based on the virtual standardized patient testing results: cross sectional study. Nurse Educ Today. 2022;111:105312.
Edelstein RA, Reid HM, Usatine R, Wilkes MS. A comparative study of measures to evaluate medical students’ performance. Acad Med. 2000;75(8):825–33.
Guagnano MT, Merlitti D, Manigrasso MR, Pace-Palitti V, Sensi S. New medical licensing examination using computer-based case simulations and standardized patients. Acad Med. 2002;77(1):87–90.
Hawkins R, MacKrell Gaglione M, LaDuca T, Leung C, Sample L, Gliva-McConvey G, Liston W, De Champlain A, Ciccone A. Assessment of patient management skills and clinical skills of practising doctors using computer-based case simulations and standardised patients. Med Educ. 2004;38(9):958–68.
Maicher KR, Stiff A, Scholl M, White M, Fosler-Lussier E, Schuler W, Serai P, Sunder V, Forrestal H, Mendella L, et al. Artificial intelligence in virtual standardized patients: combining natural language understanding and rule based dialogue management to improve conversational fidelity. Med Teach. 2023;45(3):279–85.
Hauze SW, Hoyt HH, Frazee JP, Greiner PA, Marshall JM. Enhancing nursing education through affordable and realistic holographic mixed reality: the virtual standardized patient for clinical simulation. Adv Exp Med Biol. 2019;1120:1–13.
Kelly S, Smyth E, Murphy P, Pawlikowska T. A scoping review: virtual patients for communication skills in medical undergraduates. BMC Med Educ. 2022;22(1):429.
Maicher KR, Zimmerman L, Wilcox B, Liston B, Cronau H, Macerollo A, Jin L, Jaffe E, White M, Fosler-Lussier E, et al. Using virtual standardized patients to accurately assess information gathering skills in medical students. Med Teach. 2019;41(9):1053–9.
Borja-Hart NL, Spivey CA, George CM. Use of virtual patient software to assess student confidence and ability in communication skills and virtual patient impression: A mixed-methods approach. Curr Pharm Teach Learn. 2019;11(7):710–8.
Quail M, Brundage SB, Spitalnick J, Allen PJ, Beilby J. Student self-reported communication skills, knowledge and confidence across standardised patient, virtual and traditional clinical learning environments. BMC Med Educ. 2016;16:73.
Campillos-Llanos L, Thomas C, Bilinski É, Neuraz A, Rosset S, Zweigenbaum P. Lessons Learned from the Usability Evaluation of a Simulated Patient Dialogue System. J Med Syst. 2021;45(7):69.
Sun Y, Shuohuan W, Yukun L, Shikun F, Xuyi C, Han Z, et al. ERNIE: enhanced representation through knowledge integration [Internet]. arXiv [Preprint]. 2019. Available from: https://arxiv.org/abs/1904.09223 .
Huang L, Hu J, Cai Q, Fu G, Bai Z, Liu Y, et al. The performance evaluation of artificial intelligence ERNIE bot in Chinese National Medical Licensing Examination. Postgrad Med J. 2024:qgae062.
Han H. The application of the mask detection based on automatic machine learning. Proceedings of SPIE; 2022, 12287. p. 124–9.
Wang T, Wu Y. Design and practice of teaching demonstration system for water quality prediction experiment based on EasyDL. In Proceedings of the 2023 7th International Conference on Electronic Information Technology and Computer Engineering; 2023. p. 1369–74.
Zhao Y, Wang P. The Prediction and Investigation of Factors in the Adaptability Level of Online Learning Based on AutoML and K-Means Algorithm. In 2024 IEEE 2nd International Conference on Control, Electronics and Computer Technology (ICCECT); 2024. p. 1313–9.
Zhou Z. Automatic machine learning-based data analysis for video game industry. In 2022 2nd International Conference on Computer Science, Electronic Information Engineering and Intelligent Control Technology (CEI); 2022. p. 732–7.
Fink MC, Reitmeier V, Stadler M, Siebeck M, Fischer F, Fischer MR. Assessment of diagnostic competences with standardized patients versus virtual patients: experimental study in the context of history taking. J Med Internet Res. 2021;23(3):e21196.
Bond WF, Lynch TJ, Mischler MJ, Fish JL, McGarvey JS, Taylor JT, Kumar DM, Mou KM, Ebert-Allen RA, Mahale DN, et al. Virtual standardized patient simulation: case development and pilot application to high-value care. Simul Healthc. 2019;14(4):241–50.
Maicher K, Danforth D, Price A, Zimmerman L, Wilcox B, Liston B, Cronau H, Belknap L, Ledford C, Way D, et al. Developing a conversational virtual standardized patient to enable students to practice history-taking skills. Simul Healthc. 2017;12(2):124–31.
Kammoun A, Slama R, Tabia H, Ouni T, Abid M. Generative adversarial networks for face generation: a survey. ACM Comput Surv. 2022;55:1–37.
Download references
Acknowledgements
We would like to thank all the participants and colleagues involved in this study.
This study was supported by a Research project of the New Century Higher Education Teaching Reform Project (the ninth phase) of Sichuan University (SCU9334), the Medical Simulation Education Research Project of the National Center for Medical Education Development (2021MNYB04), and the Experimental Technology Research Program of Sichuan University (SCU2023005).
Author information
Xinyu Zhang and Duo Zeng contributed equally to this work.
Authors and Affiliations
The Chinese Cochrane Center, West China Hospital, Sichuan University, Chengdu, Sichuan Province, 610041, People’s Republic of China
Xinyu Zhang & Dan Pu
West China Medical Simulation Center, West China Hospital, Sichuan University, Chengdu, Sichuan Province, 610041, People’s Republic of China
Xinyu Zhang, Duo Zeng, Xiandi Wang, Ying Han, Manqing He, Xiaoling Chen & Dan Pu
West China Biomedical Big Data Center, West China Hospital, Sichuan University, Chengdu, Sichuan Province, 610041, People’s Republic of China
You can also search for this author in PubMed Google Scholar
Contributions
XZ, DZ, and DP conceptualized the study. MH, YH, XC, and XW implemented the experiment. DZ and XZ collected and analyzed the data. XZ, DZ, and XW wrote the original draft. YF revised and polished the manuscript. All authors contributed to revising the manuscript and reviewing the manuscript.
Corresponding author
Correspondence to Dan Pu .
Ethics declarations
Ethics approval and consent to participate.
The study protocol was approved by the University of West China Hospital of Sichuan University Research Ethics Board (Approval 2019 No.1071). Each participant provided written consent before entering the study. The study was designed and conducted according to the Declaration of Helsinki and China’s competent laws and regulations.
Consent for publication
Not applicable.
Competing interests
The authors declare no competing interests.
Additional information
Publisher’s note.
Springer Nature remains neutral with regard to jurisdictional claims in published maps and institutional affiliations.
Supplementary Information
Supplementary material 1., rights and permissions.
Open Access This article is licensed under a Creative Commons Attribution-NonCommercial-NoDerivatives 4.0 International License, which permits any non-commercial use, sharing, distribution and reproduction in any medium or format, as long as you give appropriate credit to the original author(s) and the source, provide a link to the Creative Commons licence, and indicate if you modified the licensed material. You do not have permission under this licence to share adapted material derived from this article or parts of it. The images or other third party material in this article are included in the article’s Creative Commons licence, unless indicated otherwise in a credit line to the material. If material is not included in the article’s Creative Commons licence and your intended use is not permitted by statutory regulation or exceeds the permitted use, you will need to obtain permission directly from the copyright holder. To view a copy of this licence, visit http://creativecommons.org/licenses/by-nc-nd/4.0/ .
Reprints and permissions
About this article
Cite this article.
Zhang, X., Zeng, D., Wang, X. et al. Analysis of virtual standardized patients for assessing clinical fundamental skills of medical students: a prospective study. BMC Med Educ 24 , 981 (2024). https://doi.org/10.1186/s12909-024-05982-2
Download citation
Received : 25 February 2024
Accepted : 03 September 2024
Published : 10 September 2024
DOI : https://doi.org/10.1186/s12909-024-05982-2
Share this article
Anyone you share the following link with will be able to read this content:
Sorry, a shareable link is not currently available for this article.
Provided by the Springer Nature SharedIt content-sharing initiative
- Virtual standardized patient
- Standardized patient
- Simulation-based education
- Clinical skills
- History-taking
BMC Medical Education
ISSN: 1472-6920
- General enquiries: [email protected]
Thank you for visiting nature.com. You are using a browser version with limited support for CSS. To obtain the best experience, we recommend you use a more up to date browser (or turn off compatibility mode in Internet Explorer). In the meantime, to ensure continued support, we are displaying the site without styles and JavaScript.
- View all journals
- Explore content
- About the journal
- Publish with us
- Sign up for alerts
- Open access
- Published: 08 September 2024
Longitudinal analysis of teacher self-efficacy evolution during a STEAM professional development program: a qualitative case study
- Haozhe Jiang ORCID: orcid.org/0000-0002-7870-0993 1 ,
- Ritesh Chugh ORCID: orcid.org/0000-0003-0061-7206 2 ,
- Xuesong Zhai ORCID: orcid.org/0000-0002-4179-7859 1 , 3 nAff7 ,
- Ke Wang 4 &
- Xiaoqin Wang 5 , 6
Humanities and Social Sciences Communications volume 11 , Article number: 1162 ( 2024 ) Cite this article
Metrics details
Despite the widespread advocacy for the integration of arts and humanities (A&H) into science, technology, engineering, and mathematics (STEM) education on an international scale, teachers face numerous obstacles in practically integrating A&H into STEM teaching (IAT). To tackle the challenges, a comprehensive five-stage framework for teacher professional development programs focussed on IAT has been developed. Through the use of a qualitative case study approach, this study outlines the shifts in a participant teacher’s self-efficacy following their exposure to each stage of the framework. The data obtained from interviews and reflective analyses were analyzed using a seven-stage inductive method. The findings have substantiated the significant impact of a teacher professional development program based on the framework on teacher self-efficacy, evident in both individual performance and student outcomes observed over eighteen months. The evolution of teacher self-efficacy in IAT should be regarded as an open and multi-level system, characterized by interactions with teacher knowledge, skills and other entrenched beliefs. Building on our research findings, an enhanced model of teacher professional learning is proposed. The revised model illustrates that professional learning for STEAM teachers should be conceived as a continuous and sustainable process, characterized by the dynamic interaction among teaching performance, teacher knowledge, and teacher beliefs. The updated model further confirms the inseparable link between teacher learning and student learning within STEAM education. This study contributes to the existing body of literature on teacher self-efficacy, teacher professional learning models and the design of IAT teacher professional development programs.
Similar content being viewed by others
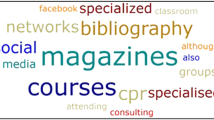
Primary and secondary school teachers’ perceptions of their social science training needs
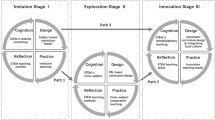
Investigating how subject teachers transition to integrated STEM education: A hybrid qualitative study on primary and middle school teachers
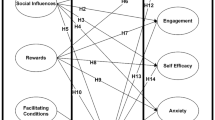
Exploring the factors affecting elementary mathematics teachers’ innovative behavior: an integration of social cognitive theory
Introduction.
In the past decade, there has been a surge in the advancement and widespread adoption of Science, Technology, Engineering, and Mathematics (STEM) education on a global scale (Jiang et al. 2021 ; Jiang et al. 2022 ; Jiang et al. 2023 ; Jiang et al. 2024a , b ; Zhan et al. 2023 ; Zhan and Niu 2023 ; Zhong et al. 2022 ; Zhong et al. 2024 ). Concurrently, there has been a growing chorus of advocates urging the integration of Arts and Humanities (A&H) into STEM education (e.g., Alkhabra et al. 2023 ; Land 2020 ; Park and Cho 2022 ; Uştu et al. 2021 ; Vaziri and Bradburn 2021 ). STEM education is frequently characterized by its emphasis on logic and analysis; however, it may be perceived as deficient in emotional and intuitive elements (Ozkan and Umdu Topsakal 2021 ). Through the integration of Arts and Humanities (A&H), the resulting STEAM approach has the potential to become more holistic, incorporating both rationality and emotional intelligence (Ozkan and Umdu Topsakal 2021 ). Many studies have confirmed that A&H can help students increase interest and develop their understanding of the contents in STEM fields, and thus, A&H can attract potential underrepresented STEM learners such as female students and minorities (Land 2020 ; Park and Cho 2022 ; Perignat and Katz-Buonincontro 2019 ). Despite the increasing interest in STEAM, the approaches to integrating A&H, which represent fundamentally different disciplines, into STEM are theoretically and practically ambiguous (Jacques et al. 2020 ; Uştu et al. 2021 ). Moreover, studies have indicated that the implementation of STEAM poses significant challenges, with STEM educators encountering difficulties in integrating A&H into their teaching practices (e.g., Boice et al. 2021 ; Duong et al. 2024 ; Herro et al. 2019 ; Jacques et al. 2020 ; Park and Cho 2022 ; Perignat and Katz-Buonincontro 2019 ). Hence, there is a pressing need to provide STEAM teachers with effective professional training.
Motivated by this gap, this study proposes a novel five-stage framework tailored for teacher professional development programs specifically designed to facilitate the integration of A&H into STEM teaching (IAT). Following the establishment of this framework, a series of teacher professional development programs were implemented. To explain the framework, a qualitative case study is employed, focusing on examining a specific teacher professional development program’s impact on a pre-service teacher’s self-efficacy. The case narratives, with a particular focus on the pre-service teacher’s changes in teacher self-efficacy, are organized chronologically, delineating stages before and after each stage of the teacher professional development program. More specifically, meaningful vignettes of the pre-service teacher’s learning and teaching experiences during the teacher professional development program are offered to help understand the five-stage framework. This study contributes to understanding teacher self-efficacy, teacher professional learning model and the design of IAT teacher professional development programs.
Theoretical background
The conceptualization of steam education.
STEM education can be interpreted through various lenses (e.g., Jiang et al. 2021 ; English 2016 ). As Li et al. (2020) claimed, on the one hand, STEM education can be defined as individual STEM disciplinary-based education (i.e., science education, technology education, engineering education and mathematics education). On the other hand, STEM education can also be defined as interdisciplinary or cross-disciplinary education where individual STEM disciplines are integrated (Jiang et al. 2021 ; English 2016 ). In this study, we view it as individual disciplinary-based education separately in science, technology, engineering and mathematics (English 2016 ).
STEAM education emerged as a new pedagogy during the Americans for the Arts-National Policy Roundtable discussion in 2007 (Perignat and Katz-Buonincontro 2019 ). This pedagogy was born out of the necessity to enhance students’ engagement, foster creativity, stimulate innovation, improve problem-solving abilities, and cultivate employability skills such as teamwork, communication and adaptability (Perignat and Katz-Buonincontro 2019 ). In particular, within the framework of STEAM education, the ‘A’ should be viewed as a broad concept that represents arts and humanities (A&H) (Herro and Quigley 2016 ; de la Garza 2021 , Park and Cho 2022 ). This conceptualization emphasizes the need to include humanities subjects alongside arts (Herro and Quigley 2016 ; de la Garza 2021 ; Park and Cho 2022 ). Sanz-Camarero et al. ( 2023 ) listed some important fields of A&H, including physical arts, fine arts, manual arts, sociology, politics, philosophy, history, psychology and so on.
In general, STEM education does not necessarily entail the inclusion of all STEM disciplines collectively (Ozkan and Umdu Topsakal 2021 ), and this principle also applies to STEAM education (Gates 2017 ; Perignat and Katz-Buonincontro 2019 ; Quigley et al. 2017 ; Smith and Paré 2016 ). As an illustration, Smith and Paré ( 2016 ) described a STEAM activity in which pottery (representing A&H) and mathematics were integrated, while other STEAM elements such as science, technology and engineering were not included. In our study, STEAM education is conceptualized as an interdisciplinary approach that involves the integration of one or more components of A&H into one or more STEM school subjects within educational activities (Ozkan and Umdu Topsakal 2021 ; Vaziri and Bradburn 2021 ). Notably, interdisciplinary collaboration entails integrating one or more elements from arts and humanities (A&H) with one or more STEM school subjects, cohesively united by a shared theme while maintaining their distinct identities (Perignat and Katz-Buonincontro 2019 ).
In our teacher professional development programs, we help mathematics, technology, and science pre-service teachers integrate one component of A&H into their disciplinary-based teaching practices. For instance, we help mathematics teachers integrate history (a component of A&H) into mathematics teaching. In other words, in our study, integrating A&H into STEM teaching (IAT) can be defined as integrating one component of A&H into the teaching of one of the STEM school subjects. The components of A&H and the STEM school subject are brought together under a common theme, but each of them remains discrete. Engineering is not taught as an individual subject in the K-12 curriculum in mainland China. Therefore, A&H is not integrated into engineering teaching in our teacher professional development programs.
Self-efficacy and teacher self-efficacy
Self-efficacy was initially introduced by Bandura ( 1977 ) as a key concept within his social cognitive theory. Bandura ( 1997 ) defined self-efficacy as “people’s beliefs about their capabilities to produce designated levels of performance that exercise influence over events that affect their lives” (p. 71). Based on Bandura’s ( 1977 ) theory, Tschannen-Moran et al. ( 1998 ) defined the concept of teacher self-efficacy Footnote 1 as “a teacher’s belief in her or his ability to organize and execute the courses of action required to successfully accomplish a specific teaching task in a particular context” (p. 233). Blonder et al. ( 2014 ) pointed out that this definition implicitly included teachers’ judgment of their ability to bring about desired outcomes in terms of students’ engagement and learning. Moreover, OECD ( 2018 ) defined teacher self-efficacy as “the beliefs that teachers have of their ability to enact certain teaching behavior that influences students’ educational outcomes, such as achievement, interest, and motivation” (p. 51). This definition explicitly included two dimensions: teachers’ judgment of the ability related to their teaching performance (i.e., enacting certain teaching behavior) and their influence on student outcomes.
It is argued that teacher self-efficacy should not be regarded as a general or overarching construct (Zee et al. 2017 ; Zee and Koomen 2016 ). Particularly, in the performance-driven context of China, teachers always connect their beliefs in their professional capabilities with the educational outcomes of their students (Liu et al. 2018 ). Therefore, we operationally conceptualize teacher self-efficacy as having two dimensions: self-efficacy in individual performance and student outcomes (see Table 1 ).
Most importantly, given its consistent association with actual teaching performance and student outcomes (Bray-Clark and Bates 2003 ; Kelley et al. 2020 ), teacher self-efficacy is widely regarded as a pivotal indicator of teacher success (Kelley et al. 2020 ). Moreover, the enhancement of teaching self-efficacy reflects the effectiveness of teacher professional development programs (Bray-Clark and Bates 2003 ; Kelley et al. 2020 ; Wong et al. 2022 ; Zhou et al. 2023 ). For instance, Zhou et al. ( 2023 ) claimed that in STEM teacher education, effective teacher professional development programs should bolster teachers’ self-efficacy “in teaching the content in the STEM discipline” (p. 2).
It has been documented that teachers frequently experience diminished confidence and comfort when teaching subject areas beyond their expertise (Kelley et al. 2020 ; Stohlmann et al. 2012 ). This diminished confidence extends to their self-efficacy in implementing interdisciplinary teaching approaches, such as integrated STEM teaching and IAT (Kelley et al. 2020 ). For instance, Geng et al. ( 2019 ) found that STEM teachers in Hong Kong exhibited low levels of self-efficacy, with only 5.53% of teachers rating their overall self-efficacy in implementing STEM education as higher than a score of 4 out of 5. Additionally, Hunter-Doniger and Sydow ( 2016 ) found that teachers may experience apprehension and lack confidence when incorporating A&H elements into the classroom context, particularly within the framework of IAT. Considering the critical importance of teacher self-efficacy in STEM and STEAM education (Kelley et al. 2020 ; Zakariya, 2020 ; Zhou et al. 2023 ), it is necessary to explore effective measures, frameworks and teacher professional development programs to help teachers improve their self-efficacy regarding interdisciplinary teaching (e.g., IAT).
Teacher professional learning models
The relationship between teachers’ professional learning and students’ outcomes (such as achievements, skills and attitudes) has been a subject of extensive discussion and research for many years (Clarke and Hollingsworth 2002 ). For instance, Clarke and Hollingsworth ( 2002 ) proposed and validated the Interconnected Model of Professional Growth, which illustrates that teacher professional development is influenced by the interaction among four interconnected domains: the personal domain (teacher knowledge, beliefs and attitudes), the domain of practice (professional experimentation), the domain of consequence (salient outcomes), and the external domain (sources of information, stimulus or support). Sancar et al. ( 2021 ) emphasized that teachers’ professional learning or development never occurs independently. In practice, this process is inherently intertwined with many variables, including student outcomes, in various ways (Sancar et al. 2021 ). However, many current teacher professional development programs exclude real in-class teaching and fail to establish a comprehensive link between teachers’ professional learning and student outcomes (Cai et al. 2020 ; Sancar et al. 2021 ). Sancar et al. ( 2021 ) claimed that exploring the complex relationships between teachers’ professional learning and student outcomes should be grounded in monitoring and evaluating real in-class teaching, rather than relying on teachers’ self-assessment. It is essential to understand these relationships from a holistic perspective within the context of real classroom teaching (Sancar et al. 2021 ). However, as Sancar et al. ( 2021 ) pointed out, such efforts in teacher education are often considered inadequate. Furthermore, in the field of STEAM education, such efforts are further exacerbated.
Cai et al. ( 2020 ) proposed a teacher professional learning model where student outcomes are emphasized. This model was developed based on Cai ( 2017 ), Philipp ( 2007 ) and Thompson ( 1992 ). It has also been used and justified in a series of teacher professional development programs (e.g., Calabrese et al. 2024 ; Hwang et al. 2024 ; Marco and Palatnik 2024 ; Örnek and Soylu 2021 ). The model posits that teachers typically increase their knowledge and modify their beliefs through professional teacher learning, subsequently improving their classroom instruction, enhancing teaching performance, and ultimately fostering improved student learning outcomes (Cai et al. 2020 ). Notably, this model can be updated in several aspects. Firstly, prior studies have exhibited the interplay between teacher knowledge and beliefs (e.g., Basckin et al. 2021 ; Taimalu and Luik 2019 ). This indicates that the increase in teacher knowledge and the change in teacher belief may not be parallel. The two processes can be intertwined. Secondly, the Interconnected Model of Professional Growth highlights that the personal domain and the domain of practice are interconnected (Clarke and Hollingsworth 2002 ). Liu et al. ( 2022 ) also confirmed that improvements in classroom instruction may, in turn, influence teacher beliefs. This necessitates a reconsideration of the relationships between classroom instruction, teacher knowledge and teacher beliefs in Cai et al.’s ( 2020 ) model. Thirdly, the Interconnected Model of Professional Growth also exhibits the connections between the domain of consequence and the personal domain (Clarke and Hollingsworth 2002 ). Hence, the improvement of learning outcomes may signify the end of teacher learning. For instance, students’ learning feedback may be a vital source of teacher self-efficacy (Bandura 1977 ). Therefore, the improvement of student outcomes may, in turn, affect teacher beliefs. The aforementioned arguments highlight the need for an updated model that integrates Cai et al.’s ( 2020 ) teacher professional learning model with Clarke and Hollingsworth’s ( 2002 ) Interconnected Model of Professional Growth. This integration may provide a holistic view of the teacher’s professional learning process, especially within the complex contexts of STEAM teacher education.
The framework for teacher professional development programs of integrating arts and humanities into STEM teaching
In this section, we present a framework for IAT teacher professional development programs, aiming to address the practical challenges associated with STEAM teaching implementation. Our framework incorporates the five features of effective teacher professional development programs outlined by Archibald et al. ( 2011 ), Cai et al. ( 2020 ), Darling-Hammond et al. ( 2017 ), Desimone and Garet ( 2015 ) and Roth et al. ( 2017 ). These features include: (a) alignment with shared goals (e.g., school, district, and national policies and practice), (b) emphasis on core content and modeling of teaching strategies for the content, (c) collaboration among teachers within a community, (d) adequate opportunities for active learning of new teaching strategies, and (e) embedded follow-up and continuous feedback. It is worth noting that two concepts, namely community of practice and lesson study, have been incorporated into our framework. Below, we delineate how these features are reflected in our framework.
(a) The Chinese government has issued a series of policies to facilitate STEAM education in K-12 schools (Jiang et al. 2021 ; Li and Chiang 2019 ; Lyu et al. 2024 ; Ro et al. 2022 ). The new curriculum standards released in 2022 mandate that all K-12 teachers implement interdisciplinary teaching, including STEAM education. Our framework for teacher professional development programs, which aims to help teachers integrate A&H into STEM teaching, closely aligns with these national policies and practices supporting STEAM education in K-12 schools.
(b) The core content of the framework is IAT. Specifically, as A&H is a broad concept, we divide it into several subcomponents, such as history, culture, and visual and performing arts (e.g., drama). We are implementing a series of teacher professional development programs to help mathematics, technology and science pre-service teachers integrate these subcomponents of A&H into their teaching Footnote 2 . Notably, pre-service teachers often lack teaching experience, making it challenging to master and implement new teaching strategies. Therefore, our framework provides five step-by-step stages designed to help them effectively model the teaching strategies of IAT.
(c) Our framework advocates for collaboration among teachers within a community of practice. Specifically, a community of practice is “a group of people who share an interest in a domain of human endeavor and engage in a process of collective learning that creates bonds between them” (Wenger et al. 2002 , p. 1). A teacher community of practice can be considered a group of teachers “sharing and critically observing their practices in growth-promoting ways” (Näykki et al. 2021 , p. 497). Long et al. ( 2021 ) claimed that in a teacher community of practice, members collaboratively share their teaching experiences and work together to address teaching problems. Our community of practice includes three types of members. (1) Mentors: These are professors and experts with rich experience in helping pre-service teachers practice IAT. (2) Pre-service teachers: Few have teaching experience before the teacher professional development programs. (3) In-service teachers: All in-service teachers are senior teachers with rich teaching experience. All the members work closely together to share and improve their IAT practice. Moreover, our community includes not only mentors and in-service teachers but also pre-service teachers. We encourage pre-service teachers to collaborate with experienced in-service teachers in various ways, such as developing IAT lesson plans, writing IAT case reports and so on. In-service teachers can provide cognitive and emotional support and share their practical knowledge and experience, which may significantly benefit the professional growth of pre-service teachers (Alwafi et al. 2020 ).
(d) Our framework offers pre-service teachers various opportunities to engage in lesson study, allowing them to actively design and implement IAT lessons. Based on the key points of effective lesson study outlined by Akiba et al. ( 2019 ), Ding et al. ( 2024 ), and Takahashi and McDougal ( 2016 ), our lesson study incorporates the following seven features. (1) Study of IAT materials: Pre-service teachers are required to study relevant IAT materials under the guidance of mentors. (2) Collaboration on lesson proposals: Pre-service teachers should collaborate with in-service teachers to develop comprehensive lesson proposals. (3) Observation and data collection: During the lesson, pre-service teachers are required to carefully observe and collect data on student learning and development. (4) Reflection and analysis: Pre-service teachers use the collected data to reflect on the lesson and their teaching effects. (5) Lesson revision and reteaching: If needed, pre-service teachers revise and reteach the lesson based on their reflections and data analysis. (6) Mentor and experienced in-service teacher involvement: Mentors and experienced in-service teachers, as knowledgeable others, are involved throughout the lesson study process. (7) Collaboration on reporting: Pre-service teachers collaborate with in-service teachers to draft reports and disseminate the results of the lesson study. Specifically, recognizing that pre-service teachers often lack teaching experience, we do not require them to complete all the steps of lesson study independently at once. Instead, we guide them through the lesson study process in a step-by-step manner, allowing them to gradually build their IAT skills and confidence. For instance, in Stage 1, pre-service teachers primarily focus on studying IAT materials. In Stage 2, they develop lesson proposals, observe and collect data, and draft reports. However, the implementation of IAT lessons is carried out by in-service teachers. This approach prevents pre-service teachers from experiencing failures due to their lack of teaching experience. In Stage 3, pre-service teachers implement, revise, and reteach IAT lessons, experiencing the lesson study process within a simulated environment. In Stage 4, pre-service teachers engage in lesson study in an actual classroom environment. However, their focus is limited to one micro-course during each lesson study session. It is not until the fifth stage that they experience a complete lesson study in an actual classroom environment.
(e) Our teacher professional development programs incorporate assessments specifically designed to evaluate pre-service teachers’ IAT practices. We use formative assessments to measure their understanding and application of IAT strategies. Pre-service teachers receive ongoing and timely feedback from peers, mentors, in-service teachers, and students, which helps them continuously refine their IAT practices throughout the program. Recognizing that pre-service teachers often have limited contact with real students and may not fully understand students’ learning needs, processes and outcomes, our framework requires them to actively collect and analyze student feedback. By doing so, they can make informed improvements to their instructional practice based on student feedback.
After undergoing three rounds of theoretical and practical testing and revision over the past five years, we have successfully finalized the optimization of the framework design (Zhou 2021 ). Throughout each cycle, we collected feedback from both participants and researchers on at least three occasions. Subsequently, we analyzed this feedback and iteratively refined the framework. For example, we enlisted the participation of in-service teachers to enhance the implementation of STEAM teaching, extended practice time through micro-teaching sessions, and introduced a stage of micro-course development within the framework to provide more opportunities for pre-service teachers to engage with real teaching situations. In this process, we continuously improved the coherence between each stage of the framework, ensuring that they mutually complement one another. The five-stage framework is described as follows.
Stage 1 Literature study
Pre-service teachers are provided with a series of reading materials from A&H. On a weekly basis, two pre-service teachers are assigned to present their readings and reflections to the entire group, followed by critical discussions thereafter. Mentors and all pre-service teachers discuss and explore strategies for translating the original A&H materials into viable instructional resources suitable for classroom use. Subsequently, pre-service teachers select topics of personal interest for further study under mentor guidance.
Stage 2 Case learning
Given that pre-service teachers have no teaching experience, collaborative efforts between in-service teachers and pre-service teachers are undertaken to design IAT lesson plans. Subsequently, the in-service teachers implement these plans. Throughout this process, pre-service teachers are afforded opportunities to engage in lesson plan implementation. Figure 1 illustrates the role of pre-service teachers in case learning. In the first step, pre-service teachers read about materials related to A&H, select suitable materials, and report their ideas on IAT lesson design to mentors, in-service teachers, and fellow pre-service teachers.
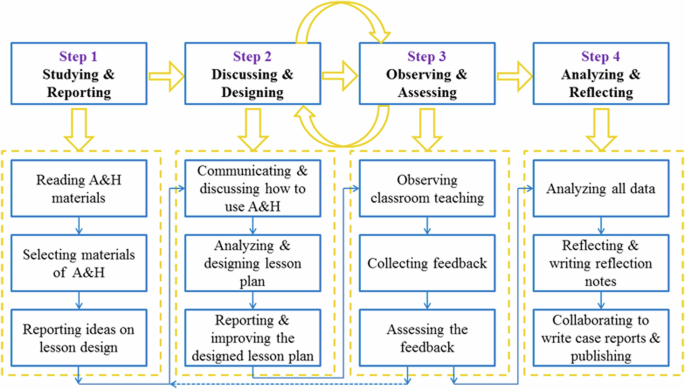
Note: A&H refers to arts and humanities.
In the second step, they liaise with the in-service teachers responsible for implementing the lesson plan, discussing the integration of A&H into teaching practices. Pre-service teachers then analyze student learning objectives aligned with curriculum standards, collaboratively designing the IAT lesson plan with in-service teachers. Subsequently, pre-service teachers present lesson plans for feedback from mentors and other in-service teachers.
In the third step, pre-service teachers observe the lesson plan’s implementation, gathering and analyzing feedback from students and in-service teachers using an inductive approach (Merriam 1998 ). Feedback includes opinions on the roles and values of A&H, perceptions of the teaching effect, and recommendations for lesson plan implementation and modification. The second and third steps may iterate multiple times to refine the IAT lesson plan. In the fourth step, pre-service teachers consolidate all data, including various versions of teaching instructions, classroom videos, feedback, and discussion notes, composing reflection notes. Finally, pre-service teachers collaborate with in-service teachers to compile the IAT case report and submit it for publication.
Stage 3 Micro-teaching
Figure 2 illustrates the role of pre-service teachers in micro-teaching. Before entering the micro-classrooms Footnote 3 , all the discussions and communications occur within the pre-service teacher group, excluding mentors and in-service teachers. After designing the IAT lesson plan, pre-service teachers take turns implementing 40-min lesson plans in a simulated micro-classroom setting. Within this simulated environment, one pre-service teacher acts as the teacher, while others, including mentors, in-service teachers, and other fellow pre-service teachers, assume the role of students Footnote 4 . Following the simulated teaching, the implementer reviews the video of their session and self-assesses their performance. Subsequently, the implementer receives feedback from other pre-service teachers, mentors, and in-service teachers. Based on this feedback, the implementer revisits steps 2 and 3, revising the lesson plan and conducting the simulated teaching again. This iterative process typically repeats at least three times until the mentors, in-service teachers, and other pre-service teachers are satisfied with the implementation of the revised lesson plan. Finally, pre-service teachers complete reflection notes and submit a summary of their reflections on the micro-teaching experience. Each pre-service teacher is required to choose at least three topics and undergo at least nine simulated teaching sessions.

Stage 4 Micro-course development
While pre-service teachers may not have the opportunity to execute the whole lesson plans in real classrooms, they can design and create five-minute micro-courses Footnote 5 before class, subsequently presenting these videos to actual students. The process of developing micro-courses closely mirrors that of developing IAT cases in the case learning stage (see Fig. 1 ). However, in Step 3, pre-service teachers assume dual roles, not only as observers of IAT lesson implementation but also as implementers of a five-minute IAT micro-course.
Stage 5 Classroom teaching
Pre-service teachers undertake the implementation of IAT lesson plans independently, a process resembling micro-teaching (see Fig. 2 ). However, pre-service teachers engage with real school students in partner schools Footnote 6 instead of simulated classrooms. Furthermore, they collect feedback not only from the mentors, in-service teachers, and fellow pre-service teachers but also from real students.
To provide our readers with a better understanding of the framework, we provide meaningful vignettes of a pre-service teacher’s learning and teaching experiences in one of the teacher professional development programs based on the framework. In addition, we choose teacher self-efficacy as an indicator to assess the framework’s effectiveness, detailing the pre-service teacher’s changes in teacher self-efficacy.
Research design
Research method.
Teacher self-efficacy can be measured both quantitatively and qualitatively (Bandura 1986 , 1997 ; Lee and Bobko 1994 ; Soprano and Yang 2013 ; Unfried et al. 2022 ). However, researchers and theorists in the area of teacher self-efficacy have called for more qualitative and longitudinal studies (Klassen et al. 2011 ). As some critiques stated, most studies were based on correlational and cross-sectional data obtained from self-report surveys, and qualitative studies of teacher efficacy were overwhelmingly neglected (Henson 2002 ; Klassen et al. 2011 ; Tschannen-Moran et al. 1998 ; Xenofontos and Andrews 2020 ). There is an urgent need for more longitudinal studies to shed light on the development of teacher efficacy (Klassen et al. 2011 ; Xenofontos and Andrews 2020 ).
This study utilized a longitudinal qualitative case study methodology to delve deeply into the context (Jiang et al. 2021 ; Corden and Millar 2007 ; Dicks et al. 2023 ; Henderson et al. 2012 ; Matusovich et al. 2010 ; Shirani and Henwood 2011 ), presenting details grounded in real-life situations and analyzing the inner relationships rather than generalize findings about the change of a large group of pre-service teachers’ self-efficacy.
Participant
This study forms a component of a broader multi-case research initiative examining teachers’ professional learning in the STEAM teacher professional development programs in China (Jiang et al. 2021 ; Wang et al. 2018 ; Wang et al. 2024 ). Within this context, one participant, Shuitao (pseudonym), is selected and reported in this current study. Shuitao was a first-year graduate student at a first-tier Normal university in Shanghai, China. Normal universities specialize in teacher education. Her graduate major was mathematics curriculum and instruction. Teaching practice courses are offered to students in this major exclusively during their third year of study. The selection of Shuitao was driven by three primary factors. Firstly, Shuitao attended the entire teacher professional development program and actively engaged in nearly all associated activities. Table 2 illustrates the timeline of the five stages in which Shuitao was involved. Secondly, her undergraduate major was applied mathematics, which was not related to mathematics teaching Footnote 7 . She possessed no prior teaching experience and had not undergone any systematic study of IAT before her involvement in the teacher professional development program. Thirdly, her other master’s courses during her first two years of study focused on mathematics education theory and did not include IAT Footnote 8 . Additionally, she scarcely participated in any other teaching practice outside of the teacher professional development program. As a pre-service teacher, Shuitao harbored a keen interest in IAT. Furthermore, she discovered that she possessed fewer teaching skills compared to her peers who had majored in education during their undergraduate studies. Hence, she had a strong desire to enhance her teaching skills. Consequently, Shuitao decided to participate in our teacher professional development program.
Shuitao was grouped with three other first-year graduate students during the teacher professional development program. She actively collaborated with them at every stage of the program. For instance, they advised each other on their IAT lesson designs, observed each other’s IAT practice and offered constructive suggestions for improvement.
Research question
Shuitao was a mathematics pre-service teacher who participated in one of our teacher professional development programs, focusing on integrating history into mathematics teaching (IHT) Footnote 9 . Notably, this teacher professional development program was designed based on our five-stage framework for teacher professional development programs of IAT. To examine the impact of this teacher professional development program on Shuitao’s self-efficacy related to IHT, this case study addresses the following research question:
What changes in Shuitao’s self-efficacy in individual performance regarding integrating history into mathematics teaching (SE-IHT-IP) may occur through participation in the teacher professional development program?
What changes in Shuitao’s self-efficacy in student outcomes regarding integrating history into mathematics teaching (SE-IHT-SO) may occur through participation in the teacher professional development program?
Data collection and analysis
Before Shuitao joined the teacher professional development program, a one-hour preliminary interview was conducted to guide her in self-narrating her psychological and cognitive state of IHT.
During the teacher professional development program, follow-up unstructured interviews were conducted once a month with Shuitao. All discussions in the development of IHT cases were recorded, Shuitao’s teaching and micro-teaching were videotaped, and the reflection notes, journals, and summary reports written by Shuitao were collected.
After completing the teacher professional development program, Shuitao participated in a semi-structured three-hour interview. The objectives of this interview were twofold: to reassess her self-efficacy and to explore the relationship between her self-efficacy changes and each stage of the teacher professional development program.
Interview data, discussions, reflection notes, journals, summary reports and videos, and analysis records were archived and transcribed before, during, and after the teacher professional development program.
In this study, we primarily utilized data from seven interviews: one conducted before the teacher professional development program, five conducted after each stage of the program, and one conducted upon completion of the program. Additionally, we reviewed Shuitao’s five reflective notes, which were written after each stage, as well as her final summary report that encompassed the entire teacher professional development program.
Merriam’s ( 1998 ) approach to coding data and inductive approach to retrieving possible concepts and themes were employed using a seven-stage method. Considering theoretical underpinnings in qualitative research is common when interpreting data (Strauss and Corbin 1990 ). First, a list based on our conceptual framework of teacher self-efficacy (see Table 1 ) was developed. The list included two codes (i.e., SE-IHT-IP and SE-IHT-SO). Second, all data were sorted chronologically, read and reread to be better understood. Third, texts were coded into multi-colored highlighting and comment balloons. Fourth, the data for groups of meanings, themes, and behaviors were examined. How these groups were connected within the conceptual framework of teacher self-efficacy was confirmed. Fifth, after comparing, confirming, and modifying, the selective codes were extracted and mapped onto the two categories according to the conceptual framework of teacher self-efficacy. Accordingly, changes in SE-IHT-IP and SE-IHT-SO at the five stages of the teacher professional development program were identified, respectively, and then the preliminary findings came (Strauss and Corbin 1990 ). In reality, in Shuitao’s narratives, SE-IHT-IP and SE-IHT-SO were frequently intertwined. Through our coding process, we differentiated between SE-IHT-IP and SE-IHT-SO, enabling us to obtain a more distinct understanding of how these two aspects of teacher self-efficacy evolved over time. This helped us address the two research questions effectively.
Reliability and validity
Two researchers independently analyzed the data to establish inter-rater reliability. The inter-rater reliability was established as kappa = 0.959. Stake ( 1995 ) suggested that the most critical assertions in a study require the greatest effort toward confirmation. In this study, three methods served this purpose and helped ensure the validity of the findings. The first way to substantiate the statement about the changes in self-efficacy was by revisiting each transcript to confirm whether the participant explicitly acknowledged the changes (Yin 2003 ). Such a check was repeated in the analysis of this study. The second way to confirm patterns in the data was by examining whether Shuitao’s statements were replicated in separate interviews (Morris and Usher 2011 ). The third approach involved presenting the preliminary conclusions to Shuitao and affording her the opportunity to provide feedback on the data and conclusions. This step aimed to ascertain whether we accurately grasped the true intentions of her statements and whether our subjective interpretations inadvertently influenced our analysis of her statements. Additionally, data from diverse sources underwent analysis by at least two researchers, with all researchers reaching consensus on each finding.
As each stage of our teacher professional development programs spanned a minimum of three months, numerous documented statements regarding the enhancement of Shuitao’s self-efficacy regarding IHT were recorded. Notably, what we present here offers only a concise overview of findings derived from our qualitative analysis. The changes in Shuitao’s SE-IHT-IP and SE-IHT-SO are organized chronologically, delineating the period before and during the teacher professional development program.
Before the teacher professional development program: “I have no confidence in IHT”
Before the teacher professional development program, Shuitao frequently expressed her lack of confidence in IHT. On the one hand, Shuitao expressed considerable apprehension about her individual performance in IHT. “How can I design and implement IHT lesson plans? I do not know anything [about it]…” With a sense of doubt, confusion and anxiety, Shuitao voiced her lack of confidence in her ability to design and implement an IHT case that would meet the requirements of the curriculum standards. Regarding the reasons for her lack of confidence, Shuitao attributed it to her insufficient theoretical knowledge and practical experience in IHT:
I do not know the basic approaches to IHT that I could follow… it is very difficult for me to find suitable historical materials… I am very confused about how to organize [historical] materials logically around the teaching goals and contents… [Furthermore,] I am [a] novice, [and] I have no IHT experience.
On the other hand, Shuitao articulated very low confidence in the efficacy of her IHT on student outcomes:
I think my IHT will have a limited impact on student outcomes… I do not know any specific effects [of history] other than making students interested in mathematics… In fact, I always think it is difficult for [my] students to understand the history… If students cannot understand [the history], will they feel bored?
This statement suggests that Shuitao did not fully grasp the significance of IHT. In fact, she knew little about the educational significance of history for students, and she harbored no belief that her IHT approach could positively impact students. In sum, her SE-IHT-SO was very low.
After stage 1: “I can do well in the first step of IHT”
After Stage 1, Shuitao indicated a slight improvement in her confidence in IHT. She attributed this improvement to her acquisition of theoretical knowledge in IHT, the approaches for selecting history-related materials, and an understanding of the educational value of history.
One of Shuitao’s primary concerns about implementing IHT before the teacher professional development program was the challenge of sourcing suitable history-related materials. However, after Stage 1, Shuitao explicitly affirmed her capability in this aspect. She shared her experience of organizing history-related materials related to logarithms as an example.
Recognizing the significance of suitable history-related materials in effective IHT implementation, Shuitao acknowledged that conducting literature studies significantly contributed to enhancing her confidence in undertaking this initial step. Furthermore, she expressed increased confidence in designing IHT lesson plans by utilizing history-related materials aligned with teaching objectives derived from the curriculum standards. In other words, her SE-IHT-IP was enhanced. She said:
After experiencing multiple discussions, I gradually know more about what kinds of materials are essential and should be emphasized, what kinds of materials should be adapted, and what kinds of materials should be omitted in the classroom instructions… I have a little confidence to implement IHT that could meet the requirements [of the curriculum standards] since now I can complete the critical first step [of IHT] well…
However, despite the improvement in her confidence in IHT following Stage 1, Shuitao also expressed some concerns. She articulated uncertainty regarding her performance in the subsequent stages of the teacher professional development program. Consequently, her confidence in IHT experienced only a modest increase.
After stage 2: “I participate in the development of IHT cases, and my confidence is increased a little bit more”
Following Stage 2, Shuitao reported further increased confidence in IHT. She attributed this growth to two main factors. Firstly, she successfully developed several instructional designs for IHT through collaboration with in-service teachers. These collaborative experiences enabled her to gain a deeper understanding of IHT approaches and enhance her pedagogical content knowledge in this area, consequently bolstering her confidence in her ability to perform effectively. Secondly, Shuitao observed the tangible impact of IHT cases on students in real classroom settings, which reinforced her belief in the efficacy of IHT. These experiences instilled in her a greater sense of confidence in her capacity to positively influence her students through her implementation of IHT. Shuitao remarked that she gradually understood how to integrate suitable history-related materials into her instructional designs (e.g., employ a genetic approach Footnote 10 ), considering it as the second important step of IHT. She shared her experience of developing IHT instructional design on the concept of logarithms. After creating several iterations of IHT instructional designs, Shuitao emphasized that her confidence in SE-IHT-IP has strengthened. She expressed belief in her ability to apply these approaches to IHT, as well as the pedagogical content knowledge of IHT, acquired through practical experience, in her future teaching endeavors. The following is an excerpt from the interview:
I learned some effective knowledge, skills, techniques and approaches [to IHT]… By employing these approaches, I thought I could [and] I had the confidence to integrate the history into instructional designs very well… For instance, [inspired] by the genetic approach, we designed a series of questions and tasks based on the history of logarithms. The introduction of the new concept of logarithms became very natural, and it perfectly met the requirements of our curriculum standards, [which] asked students to understand the necessity of learning the concept of logarithms…
Shuitao actively observed the classroom teaching conducted by her cooperating in-service teacher. She helped her cooperating in-service teacher in collecting and analyzing students’ feedback. Subsequently, discussions ensued on how to improve the instructional designs based on this feedback. The refined IHT instructional designs were subsequently re-implemented by the in-service teacher. After three rounds of developing IHT cases, Shuitao became increasingly convinced of the significance and efficacy of integrating history into teaching practices, as evidenced by the following excerpt:
The impacts of IHT on students are visible… For instance, more than 93% of the students mentioned in the open-ended questionnaires that they became more interested in mathematics because of the [historical] story of Napier… For another example, according to the results of our surveys, more than 75% of the students stated that they knew log a ( M + N ) = log a M × log a N was wrong because of history… I have a little bit more confidence in the effects of my IHT on students.
This excerpt highlights that Shuitao’s SE-IHT-SO was enhanced. She attributed this enhancement to her realization of the compelling nature of history and her belief in her ability to effectively leverage its power to positively influence her students’ cognitive and emotional development. This also underscores the importance of reinforcing pre-service teachers’ awareness of the significance of history. Nonetheless, Shuiato elucidated that she still retained concerns regarding the effectiveness of her IHT implementation. Her following statement shed light on why her self-efficacy only experienced a marginal increase in this stage:
Knowing how to do it successfully and doing it successfully in practice are two totally different things… I can develop IHT instructional designs well, but I have no idea whether I can implement them well and whether I can introduce the history professionally in practice… My cooperation in-service teacher has a long history of teaching mathematics and gains rich experience in educational practices… If I cannot acquire some required teaching skills and capabilities, I still cannot influence my students powerfully.
After stage 3: “Practice makes perfect, and my SE-IHT-IP is steadily enhanced after a hit”
After successfully developing IHT instructional designs, the next critical step was the implementation of these designs. Drawing from her observations of her cooperating in-service teachers’ IHT implementations and discussions with other pre-service teachers, Shuitao developed her own IHT lesson plans. In Stage 3, she conducted simulated teaching sessions and evaluated her teaching performance ten times Footnote 11 . Shuitao claimed that her SE-IHT-IP steadily improved over the course of these sessions. According to Shuitao, two main processes in Stage 3 facilitated this steady enhancement of SE-IHT-IP.
On the one hand, through the repeated implementation of simulated teaching sessions, Shuitao’s teaching proficiency and fluency markedly improved. Shuitao first described the importance of teaching proficiency and fluency:
Since the detailed history is not included in our curriculum standards and textbooks, if I use my historical materials in class, I have to teach more contents than traditional teachers. Therefore, I have to teach proficiently so that teaching pace becomes a little faster than usual… I have to teach fluently so as to use each minute efficiently in my class. Otherwise, I cannot complete the teaching tasks required [by curriculum standards].
As Shuitao said, at the beginning of Stage 3, her self-efficacy even decreased because she lacked teaching proficiency and fluency and was unable to complete the required teaching tasks:
In the first few times of simulated teaching, I always needed to think for a second about what I should say next when I finish one sentence. I also felt very nervous when I stood in the front of the classrooms. This made my narration of the historical story between Briggs and Napier not fluent at all. I paused many times to look for some hints on my notes… All these made me unable to complete the required teaching tasks… My [teaching] confidence took a hit.
Shuitao quoted the proverb, “practice makes perfect”, and she emphasized that it was repeated practice that improved her teaching proficiency and fluency:
I thought I had no other choice but to practice IHT repeatedly… [At the end of Stage 3,] I could naturally remember most words that I should say when teaching the topics that I selected… My teaching proficiency and fluency was improved through my repeated review of my instructional designs and implementation of IHT in the micro-classrooms… With the improvement [of my teaching proficiency and fluency], I could complete the teaching tasks, and my confidence was increased as well.
In addition, Shuitao also mentioned that through this kind of self-exploration in simulated teaching practice, her teaching skills and capabilities (e.g., blackboard writing, abilities of language organization abilities, etc.) improved. This process was of great help to her enhancement of SE-IHT-IP.
On the other hand, Shuitao’s simulated teaching underwent assessment by herself, with mentors, in-service teachers and fellow pre-service teachers. This comprehensive evaluation process played a pivotal role in enhancing her individual performance and self-efficacy. Reflecting on this aspect, Shuitao articulated the following sentiments in one of her reflection reports:
By watching the videos, conducting self-assessment, and collecting feedback from others, I can understand what I should improve or emphasize in my teaching. [Then,] I think my IHT can better meet the requirements [of curriculum standards]… I think my teaching performance is getting better and better.
After stage 4: “My micro-courses influenced students positively, and my SE-IHT-SO is steadily enhanced”
In Stage 4, Shuitao commenced by creating 5-min micro-course videos. Subsequently, she played these videos in her cooperating in-service teachers’ authentic classroom settings and collected student feedback. This micro-course was played at the end of her cooperating in-service teachers’ lesson Footnote 12 . Shuitao wrote in her reflections that this micro-course of logarithms helped students better understand the nature of mathematics:
According to the results of our surveys, many students stated that they knew the development and evolution of the concept of logarithms is a long process and many mathematicians from different countries have contributed to the development of the concept of logarithms… This indicated that my micro-course helped students better understand the nature of mathematics… My micro-course about the history informed students that mathematics is an evolving and human subject and helped them understand the dynamic development of the [mathematics] concept…
Meanwhile, Shuitao’s micro-course positively influenced some students’ beliefs towards mathematics. As evident from the quote below, integrating historical context into mathematics teaching transformed students’ perception of the subject, boosting Shuitao’s confidence too.
Some students’ responses were very exciting… [O]ne [typical] response stated, he always regarded mathematics as abstract, boring, and dreadful subject; but after seeing the photos of mathematicians and great men and learning the development of the concept of logarithms through the micro-course, he found mathematics could be interesting. He wanted to learn more the interesting history… Students’ such changes made me confident.
Furthermore, during post-class interviews, several students expressed their recognition of the significance of the logarithms concept to Shuitao, attributing this realization to the insights provided by prominent figures in the micro-courses. They also conveyed their intention to exert greater effort in mastering the subject matter. This feedback made Shuitao believe that her IHT had the potential to positively influence students’ attitudes towards learning mathematics.
In summary, Stage 4 marked Shuitao’s first opportunity to directly impact students through her IHT in authentic classroom settings. Despite implementing only brief 5-min micro-courses integrating history during each session, the effectiveness of her short IHT implementation was validated by student feedback. Shuitao unequivocally expressed that students actively engaged with her micro-courses and that these sessions positively influenced them, including attitudes and motivation toward mathematics learning, understanding of mathematics concepts, and beliefs regarding mathematics. These collective factors contributed to a steady enhancement of her confidence in SE-IHT-SO.
After stage 5: “My overall self-efficacy is greatly enhanced”
Following Stage 5, Shuitao reported a significant increase in her overall confidence in IHT, attributing it to gaining mastery through successful implementations of IHT in real classroom settings. On the one hand, Shuitao successfully designed and executed her IHT lesson plans, consistently achieving the teaching objectives mandated by curriculum standards. This significantly enhanced her SE-IHT-IP. On the other hand, as Shuitao’s IHT implementation directly influenced her students, her confidence in SE-IHT-SO experienced considerable improvement.
According to Bandura ( 1997 ), mastery experience is the most powerful source of self-efficacy. Shuitao’s statements confirmed this. As she claimed, her enhanced SE-IHT-IP in Stage 5 mainly came from the experience of successful implementations of IHT in real classrooms:
[Before the teacher professional development program,] I had no idea about implementing IHT… Now, I successfully implemented IHT in senior high school [classrooms] many times… I can complete the teaching tasks and even better completed the teaching objectives required [by the curriculum standards]… The successful experience greatly enhances my confidence to perform well in my future implementation of IHT… Yeah, I think the successful teaching practice experience is the strongest booster of my confidence.
At the end of stage 5, Shuitao’s mentors and in-service teachers gave her a high evaluation. For instance, after Shuitao’s IHT implementation of the concept of logarithms, all mentors and in-service teachers consistently provided feedback that her IHT teaching illustrated the necessity of learning the concept of logarithms and met the requirements of the curriculum standards very well. This kind of verbal persuasion (Bandura 1997 ) enhanced her SE-IHT-IP.
Similarly, Shuitao’s successful experience of influencing students positively through IHT, as one kind of mastery experience, powerfully enhanced her SE-IHT-SO. She described her changes in SE-IHT-SO as follows:
I could not imagine my IHT could be so influential [before]… But now, my IHT implementation directly influenced students in so many aspects… When I witnessed students’ real changes in various cognitive and affective aspects, my confidence was greatly improved.
Shuitao described the influence of her IHT implementation of the concept of logarithms on her students. The depiction is grounded in the outcomes of surveys conducted by Shuitao following her implementation. Shuitao asserted that these results filled her with excitement and confidence regarding her future implementation of IHT.
In summary, following Stage 5 of the teacher professional development program, Shuitao experienced a notable enhancement in her overall self-efficacy, primarily attributed to her successful practical experience in authentic classroom settings during this stage.
A primary objective of our teacher professional development programs is to equip pre-service teachers with the skills and confidence needed to effectively implement IAT. Our findings show that one teacher professional development program, significantly augmented a participant’s TSE-IHT across two dimensions: individual performance and student outcomes. Considering the pressing need to provide STEAM teachers with effective professional training (e.g., Boice et al. 2021 ; Duong et al. 2024 ; Herro et al. 2019 ; Jacques et al. 2020 ; Park and Cho 2022 ; Perignat and Katz-Buonincontro 2019 ), the proposed five-stage framework holds significant promise in both theoretical and practical realms. Furthermore, this study offers a viable solution to address the prevalent issue of low levels of teacher self-efficacy in interdisciplinary teaching, including IAT, which is critical in STEAM education (Zhou et al. 2023 ). This study holds the potential to make unique contributions to the existing body of literature on teacher self-efficacy, teacher professional learning models and the design of teacher professional development programs of IAT.
Firstly, this study enhances our understanding of the development of teacher self-efficacy. Our findings further confirm the complexity of the development of teacher self-efficacy. On the one hand, the observed enhancement of the participant’s teacher self-efficacy did not occur swiftly but unfolded gradually through a protracted, incremental process. Moreover, it is noteworthy that the participant’s self-efficacy exhibited fluctuations, underscoring that the augmentation of teacher self-efficacy is neither straightforward nor linear. On the other hand, the study elucidated that the augmentation of teacher self-efficacy constitutes an intricate, multi-level system that interacts with teacher knowledge, skills, and other beliefs. This finding resonates with prior research on teacher self-efficacy (Morris et al. 2017 ; Xenofontos and Andrews 2020 ). For example, our study revealed that Shuitao’s enhancement of SE-IHT-SO may always be interwoven with her continuous comprehension of the significance of the A&H in classroom settings. Similarly, the participant progressively acknowledged the educational value of A&H in classroom contexts in tandem with the stepwise enhancement of SE-IHT-SO. Factors such as the participant’s pedagogical content knowledge of IHT, instructional design, and teaching skills were also identified as pivotal components of SE-IHT-IP. This finding corroborates Morris and Usher ( 2011 ) assertion that sustained improvements in self-efficacy stem from developing teachers’ skills and knowledge. With the bolstering of SE-IHT-IP, the participant’s related teaching skills and content knowledge also exhibited improvement.
Methodologically, many researchers advocate for qualitative investigations into self-efficacy (e.g., Philippou and Pantziara 2015; Klassen et al. 2011 ; Wyatt 2015 ; Xenofontos and Andrews 2020 ). While acknowledging limitations in sample scope and the generalizability of the findings, this study offers a longitudinal perspective on the stage-by-stage development of teacher self-efficacy and its interactions with different factors (i.e., teacher knowledge, skills, and beliefs), often ignored by quantitative studies. Considering that studies of self-efficacy have been predominantly quantitative, typically drawing on survey techniques and pre-determined scales (Xenofontos and Andrews, 2020 ; Zhou et al. 2023 ), this study highlights the need for greater attention to qualitative studies so that more cultural, situational and contextual factors in the development of self-efficacy can be captured.
Our study provides valuable practical implications for enhancing pre-service teachers’ self-efficacy. We conceptualize teacher self-efficacy in two primary dimensions: individual performance and student outcomes. On the one hand, pre-service teachers can enhance their teaching qualities, boosting their self-efficacy in individual performance. The adage “practice makes perfect” underscores the necessity of ample teaching practice opportunities for pre-service teachers who lack prior teaching experience. Engaging in consistent and reflective practice helps them develop confidence in their teaching qualities. On the other hand, pre-service teachers should focus on positive feedback from their students, reinforcing their self-efficacy in individual performance. Positive student feedback serves as an affirmation of their teaching effectiveness and encourages continuous improvement. Furthermore, our findings highlight the significance of mentors’ and peers’ positive feedback as critical sources of teacher self-efficacy. Mentors and peers play a pivotal role in the professional growth of pre-service teachers by actively encouraging them and recognizing their teaching achievements. Constructive feedback from experienced mentors and supportive peers fosters a collaborative learning environment and bolsters the self-confidence of pre-service teachers. Additionally, our research indicates that pre-service teachers’ self-efficacy may fluctuate. Therefore, mentors should be prepared to help pre-service teachers manage teaching challenges and setbacks, and alleviate any teaching-related anxiety. Mentors can help pre-service teachers build resilience and maintain a positive outlook on their teaching journey through emotional support and guidance. Moreover, a strong correlation exists between teacher self-efficacy and teacher knowledge and skills. Enhancing pre-service teachers’ knowledge base and instructional skills is crucial for bolstering their overall self-efficacy.
Secondly, this study also responds to the appeal to understand teachers’ professional learning from a holistic perspective and interrelate teachers’ professional learning process with student outcome variables (Sancar et al. 2021 ), and thus contributes to the understanding of the complexity of STEAM teachers’ professional learning. On the one hand, we have confirmed Cai et al.’s ( 2020 ) teacher professional learning model in a new context, namely STEAM teacher education. Throughout the teacher professional development program, the pre-service teacher, Shuitao, demonstrated an augmentation in her knowledge, encompassing both content knowledge and pedagogical understanding concerning IHT. Moreover, her beliefs regarding IHT transformed as a result of her engagement in teacher learning across the five stages. This facilitated her in executing effective IHT teaching and improving her students’ outcomes. On the other hand, notably, in our studies (including this current study and some follow-up studies), student feedback is a pivotal tool to assist teachers in discerning the impact they are effectuating. This enables pre-service teachers to grasp the actual efficacy of their teaching efforts and subsequently contributes significantly to the augmentation of their self-efficacy. Such steps have seldom been conducted in prior studies (e.g., Cai et al. 2020 ), where student outcomes are often perceived solely as the results of teachers’ instruction rather than sources informing teacher beliefs. Additionally, this study has validated both the interaction between teaching performance and teacher beliefs and between teacher knowledge and teacher beliefs. These aspects were overlooked in Cai et al.’s ( 2020 ) model. More importantly, while Clarke and Hollingsworth’s ( 2002 ) Interconnected Model of Professional Growth illustrates the connections between the domain of consequence and the personal domain, as well as between the personal domain and the domain of practice, it does not adequately clarify the complex relationships among the factors within the personal domain (e.g., the interaction between teacher knowledge and teacher beliefs). Therefore, our study also supplements Clarke and Hollingsworth’s ( 2002 ) model by addressing these intricacies. Based on our findings, an updated model of teacher professional learning has been proposed, as shown in Fig. 3 . This expanded model indicates that teacher learning should be an ongoing and sustainable process, with the enhancement of student learning not marking the conclusion of teacher learning, but rather serving as the catalyst for a new phase of learning. In this sense, we advocate for further research to investigate the tangible impacts of teacher professional development programs on students and how those impacts stimulate subsequent cycles of teacher learning.
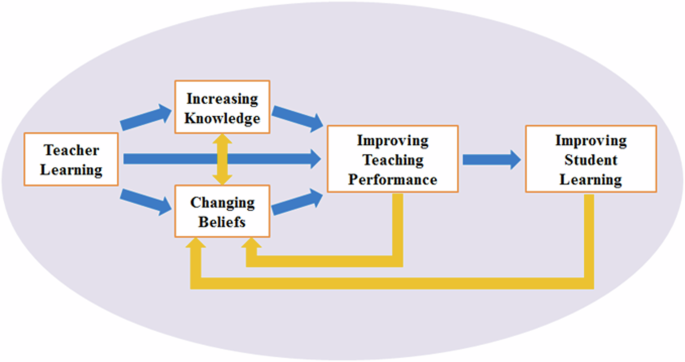
Note: Paths in blue were proposed by Cai et al. ( 2020 ), and paths in yellow are proposed and verified in this study.
Thirdly, in light of the updated model of teacher professional learning (see Fig. 3 ), this study provides insights into the design of teacher professional development programs of IAT. According to Huang et al. ( 2022 ), to date, very few studies have set goals to “develop a comprehensive understanding of effective designs” for STEM (or STEAM) teacher professional development programs (p. 15). To fill this gap, this study proposes a novel and effective five-stage framework for teacher professional development programs of IAT. This framework provides a possible and feasible solution to the challenges of STEAM teacher professional development programs’ design and planning, and teachers’ IAT practice (Boice et al. 2021 ; Herro et al. 2019 ; Jacques et al. 2020 ; Park and Cho 2022 ; Perignat and Katz-Buonincontro 2019 ).
Specifically, our five-stage framework incorporates at least six important features. Firstly, teacher professional development programs should focus on specific STEAM content. Given the expansive nature of STEAM, teacher professional development programs cannot feasibly encompass all facets of its contents. Consistent with recommendations by Cai et al. ( 2020 ), Desimone et al. ( 2002 ) and Garet et al. ( 2001 ), an effective teacher professional development program should prioritize content focus. Our five-stage framework is centered on IAT. Throughout an 18-month duration, each pre-service teacher is limited to selecting one subcomponent of A&H, such as history, for integration into their subject teaching (i.e., mathematics teaching, technology teaching or science teaching) within one teacher professional development program. Secondly, in response to the appeals that teacher professional development programs should shift from emphasizing teaching and instruction to emphasizing student learning (Cai et al. 2020 ; Calabrese et al. 2024 ; Hwang et al. 2024 ; Marco and Palatnik 2024 ; Örnek and Soylu 2021 ), our framework requires pre-service teachers to pay close attention to the effects of IAT on student learning outcomes, and use students’ feedback as the basis of improving their instruction. Thirdly, prior studies found that teacher education with a preference for theory led to pre-service teachers’ dissatisfaction with the quality of teacher professional development program and hindered the development of pre-service teachers’ teaching skills and teaching beliefs, which also widened the gap between theory and practice (Hennissen et al. 2017 ; Ord and Nuttall 2016 ). In this regard, our five-stage framework connects theory and teaching practice closely. In particular, pre-service teachers can experience the values of IAT not only through theoretical learning but also through diverse teaching practices. Fourthly, we build a teacher community of practice tailored for pre-service teachers. Additionally, we aim to encourage greater participation of in-service teachers in such teacher professional development programs designed for pre-service educators in STEAM teacher education. By engaging in such programs, in-service teachers can offer valuable teaching opportunities for pre-service educators and contribute their insights and experiences from teaching practice. Importantly, pre-service teachers stand to gain from the in-service teachers’ familiarity with textbooks, subject matter expertise, and better understanding of student dynamics. Fifthly, our five-stage framework lasts for an extended period, spanning 18 months. This duration ensures that pre-service teachers engage in a sustained and comprehensive learning journey. Lastly, our framework facilitates a practical understanding of “integration” by offering detailed, sequential instructions for blending two disciplines in teaching. For example, our teacher professional development programs prioritize systematic learning of pedagogical theories and simulated teaching experiences before pre-service teachers embark on real STEAM teaching endeavors. This approach is designed to mitigate the risk of unsuccessful experiences during initial teaching efforts, thereby safeguarding pre-service teachers’ teacher self-efficacy. Considering the complexity of “integration” in interdisciplinary teaching practices, including IAT (Han et al. 2022 ; Ryu et al. 2019 ), we believe detailed stage-by-stage and step-by-step instructions are crucial components of relevant pre-service teacher professional development programs. Notably, this aspect, emphasizing structural instructional guidance, has not been explicitly addressed in prior research (e.g., Cai et al. 2020 ). Figure 4 illustrates the six important features outlined in this study, encompassing both established elements and the novel addition proposed herein, describing an effective teacher professional development program.
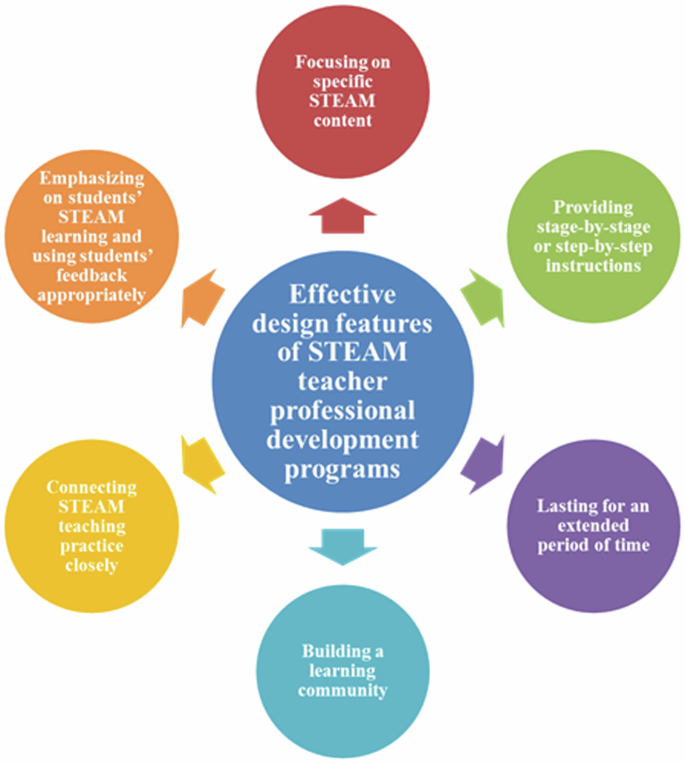
Note: STEAM refers to science, technology, engineering, arts and humanities, and mathematics.
The successful implementation of this framework is also related to the Chinese teacher education system and cultural background. For instance, the Chinese government has promoted many university-school collaboration initiatives, encouraging in-service teachers to provide guidance and practical opportunities for pre-service teachers (Lu et al. 2019 ). Influenced by Confucian values emphasizing altruism, many experienced in-service teachers in China are eager to assist pre-service teachers, helping them better realize their teaching career aspirations. It is reported that experienced in-service teachers in China show significantly higher motivation than their international peers when mentoring pre-service teachers (Lu et al. 2019 ). Therefore, for the successful implementation of this framework in other countries, it is crucial for universities to forge close collaborative relationships with K-12 schools and actively involve K-12 teachers in pre-service teacher education.
Notably, approximately 5% of our participants dropped out midway as they found that the IAT practice was too challenging or felt overwhelmed by the number of required tasks in the program. Consequently, we are exploring options to potentially simplify this framework in future iterations.
Without minimizing the limitations of this study, it is important to recognize that a qualitative longitudinal case study can be a useful means of shedding light on the development of a pre-service STEAM teacher’s self-efficacy. However, this methodology did not allow for a pre-post or a quasi-experimental design, and the effectiveness of our five-stage framework could not be confirmed quantitatively. In the future, conducting more experimental or design-based studies could further validate the effectiveness of our framework and broaden our findings. Furthermore, future studies should incorporate triangulation methods and utilize multiple data sources to enhance the reliability and validity of the findings. Meanwhile, owing to space limitations, we could only report the changes in Shuitao’s SE-IHT-IP and SE-IHT-SO here, and we could not describe the teacher self-efficacy of other participants regarding IAT. While nearly all of the pre-service teachers experienced an improvement in their teacher self-efficacy concerning IAT upon participating in our teacher professional development programs, the processes of their change were not entirely uniform. We will need to report the specific findings of these variations in the future. Further studies are also needed to explore the factors contributing to these variations. Moreover, following this study, we are implementing more teacher professional development programs of IAT. Future studies can explore the impact of this framework on additional aspects of pre-service STEAM teachers’ professional development. This will help gain a more comprehensive understanding of its effectiveness and potential areas for further improvement. Additionally, our five-stage framework was initially developed and implemented within the Chinese teacher education system. Future research should investigate how this framework can be adapted in other educational systems and cultural contexts.
The impetus behind this study stems from the burgeoning discourse advocating for the integration of A&H disciplines into STEM education on a global scale (e.g., Land 2020 ; Park and Cho 2022 ; Uştu et al. 2021 ; Vaziri and Bradburn 2021 ). Concurrently, there exists a pervasive concern regarding the challenges teachers face in implementing STEAM approaches, particularly in the context of IAT practices (e.g., Boice et al. 2021 ; Herro et al. 2019 ; Jacques et al. 2020 ; Park and Cho 2022 ; Perignat and Katz-Buonincontro 2019 ). To tackle this challenge, we first proposed a five-stage framework designed for teacher professional development programs of IAT. Then, utilizing this innovative framework, we implemented a series of teacher professional development programs. Drawing from the recommendations of Bray-Clark and Bates ( 2003 ), Kelley et al. ( 2020 ) and Zhou et al. ( 2023 ), we have selected teacher self-efficacy as a key metric to examine the effectiveness of the five-stage framework. Through a qualitative longitudinal case study, we scrutinized the influence of a specific teacher professional development program on the self-efficacy of a single pre-service teacher over an 18-month period. Our findings revealed a notable enhancement in teacher self-efficacy across both individual performance and student outcomes. The observed enhancement of the participant’s teacher self-efficacy did not occur swiftly but unfolded gradually through a prolonged, incremental process. Building on our findings, an updated model of teacher learning has been proposed. The updated model illustrates that teacher learning should be viewed as a continuous and sustainable process, wherein teaching performance, teacher beliefs, and teacher knowledge dynamically interact with one another. The updated model also confirms that teacher learning is inherently intertwined with student learning in STEAM education. Furthermore, this study also summarizes effective design features of STEAM teacher professional development programs.
Data availability
The datasets generated and/or analyzed during this study are not publicly available due to general data protection regulations, but are available from the corresponding author on reasonable request.
In their review article, Morris et al. ( 2017 ) equated “teaching self-efficacy” and “teacher self-efficacy” as synonymous concepts. This perspective is also adopted in this study.
An effective teacher professional development program should have specific, focused, and clear content instead of broad and scattered ones. Therefore, each pre-service teacher can only choose to integrate one subcomponent of A&H into their teaching in one teacher professional development program. For instance, Shuitao, a mathematics pre-service teacher, participated in one teacher professional development program focused on integrating history into mathematics teaching. However, she did not explore the integration of other subcomponents of A&H into her teaching during her graduate studies.
In the micro-classrooms, multi-angle, and multi-point high-definition video recorders are set up to record the teaching process.
In micro-teaching, mentors, in-service teachers, and other fellow pre-service teachers take on the roles of students.
In China, teachers can video record one section of a lesson and play them in formal classes. This is a practice known as a micro-course. For instance, in one teacher professional development program of integrating history into mathematics teaching, micro-courses encompass various mathematics concepts, methods, ideas, history-related material and related topics. Typically, teachers use these micro-courses to broaden students’ views, foster inquiry-based learning, and cultivate critical thinking skills. Such initiatives play an important role in improving teaching quality.
Many university-school collaboration initiatives in China focus on pre-service teachers’ practicum experiences (Lu et al. 2019 ). Our teacher professional development program is also supported by many K-12 schools in Shanghai. Personal information in videos is strictly protected.
In China, students are not required to pursue a graduate major that matches their undergraduate major. Most participants in our teacher professional development programs did not pursue undergraduate degrees in education-related fields.
Shuitao’s university reserves Wednesday afternoons for students to engage in various programs or clubs, as classes are not scheduled during this time. Similarly, our teacher professional development program activities are planned for Wednesday afternoons to avoid overlapping with participants’ other coursework commitments.
History is one of the most important components of A&H (Park and Cho 2022 ).
To learn more about genetic approach (i.e., genetic principle), see Jankvist ( 2009 ).
For the assessment process, see Fig. 2 .
Shuitao’s cooperating in-service teacher taught the concept of logarithms in Stage 2. In Stage 4, the teaching objective of her cooperating in-service teacher’s review lesson was to help students review the concept of logarithms to prepare students for the final exam.
Akiba M, Murata A, Howard C, Wilkinson B, Fabrega J (2019) Race to the top and lesson study implementation in Florida: District policy and leadership for teacher professional development. In: Huang R, Takahashi A, daPonte JP (eds) Theory and practice of lesson study in mathematics, pp. 731–754. Springer, Cham. https://doi.org/10.1007/978-3-030-04031-4_35
Alkhabra YA, Ibrahem UM, Alkhabra SA (2023) Augmented reality technology in enhancing learning retention and critical thinking according to STEAM program. Humanit Soc Sci Commun 10:174. https://doi.org/10.1057/s41599-023-01650-w
Article Google Scholar
Alwafi EM, Downey C, Kinchin G (2020) Promoting pre-service teachers’ engagement in an online professional learning community: Support from practitioners. J Professional Cap Community 5(2):129–146. https://doi.org/10.1108/JPCC-10-2019-0027
Archibald S, Coggshall JG, Croft A, Goe L (2011) High-quality professional development for all teachers: effectively allocating resources. National Comprehensive Center for Teacher Quality, Washington, DC
Google Scholar
Bandura A (1977) Self-efficacy: Toward a unifying theory of behavioral change. Psychological Rev 84:191–215. https://doi.org/10.1016/0146-6402(78)90002-4
Article CAS Google Scholar
Bandura A (1986) The explanatory and predictive scope of self-efficacy theory. J Soc Clin Psychol 4:359–373. https://doi.org/10.1521/jscp.1986.4.3.359
Bandura A (1997) Self-efficacy: The exercise of control. Freeman, New York
Basckin C, Strnadová I, Cumming TM (2021) Teacher beliefs about evidence-based practice: A systematic review. Int J Educ Res 106:101727. https://doi.org/10.1016/j.ijer.2020.101727
Bray-Clark N, Bates R (2003) Self-efficacy beliefs and teacher effectiveness: Implications for professional development. Prof Educ 26(1):13–22
Blonder R, Benny N, Jones MG (2014) Teaching self-efficacy of science teachers. In: Evans R, Luft J, Czerniak C, Pea C (eds), The role of science teachers’ beliefs in international classrooms: From teacher actions to student learning, Sense Publishers, Rotterdam, Zuid-Holland, pp. 3–16
Boice KL, Jackson JR, Alemdar M, Rao AE, Grossman S, Usselman M (2021) Supporting teachers on their STEAM journey: A collaborative STEAM teacher training program. Educ Sci 11(3):105. https://doi.org/10.3390/educsci11030105
Cai J (2017) Longitudinally investigating the effect of teacher professional development on instructional practice and student learning: A focus on mathematical problem posing. The University of Delaware, Newark, DE
Cai J, Chen T, Li X, Xu R, Zhang S, Hu Y, Zhang L, Song N (2020) Exploring the impact of a problem-posing workshop on elementary school mathematics teachers’ conceptions on problem posing and lesson design. Int J Educ Res 102:101404. https://doi.org/10.1016/j.ijer.2019.02.004
Calabrese JE, Capraro MM, Viruru R (2024) Semantic structure and problem posing: Preservice teachers’ experience. School Sci Math. https://doi.org/10.1111/ssm.12648
Clarke D, Hollingsworth H (2002) Elaborating a model of teacher professional growth. Teach Teach Educ 18(8):947–967. https://doi.org/10.1016/S0742-051X(02)00053-7
Corden A, Millar J (2007) Time and change: A review of the qualitative longitudinal research literature for social policy. Soc Policy Soc 6(4):583–592. https://doi.org/10.1017/S1474746407003910
Darling-Hammond L, Hyler ME, Gardner M (2017) Effective teacher professional development. Learning Policy Institute, Palo Alto, CA
Book Google Scholar
de la Garza A (2021) Internationalizing the curriculum for STEAM (STEM+ Arts and Humanities): From intercultural competence to cultural humility. J Stud Int Educ 25(2):123–135. https://doi.org/10.1177/1028315319888468
Article MathSciNet Google Scholar
Desimone LM, Garet MS (2015) Best practices in teachers’ professional development in the United States. Psychol, Soc, Educ 7(3):252–263
Desimone LM, Porter AC, Garet MS, Yoon KS, Birman BF (2002) Effects of professional development on teachers’ instruction: Results from a three-year longitudinal study. Educ Evaluation Policy Anal 24(2):81–112. https://doi.org/10.3102/01623737024002081
Dicks SG, Northam HL, van Haren FM, Boer DP (2023) The bereavement experiences of families of potential organ donors: a qualitative longitudinal case study illuminating opportunities for family care. Int J Qualitative Stud Health Well-being 18(1):1–24. https://doi.org/10.1080/17482631.2022.2149100
Ding M, Huang R, Pressimone Beckowski C, Li X, Li Y (2024) A review of lesson study in mathematics education from 2015 to 2022: implementation and impact. ZDM Math Educ 56:87–99. https://doi.org/10.1007/s11858-023-01538-8
Duong NH, Nam NH, Trung TT (2024) Factors affecting the implementation of STEAM education among primary school teachers in various countries and Vietnamese educators: comparative analysis. Education 3–13. https://doi.org/10.1080/03004279.2024.2318239
English LD (2016) STEM education K-12: Perspectives on integration. Int J STEM Educ 3:3. https://doi.org/10.1186/s40594-016-0036-1
Garet MS, Porter AC, Desimone L, Birman BF, Yoon KS (2001) What makes professional development effective? Results from a national sample of teachers. Am Educ Res J 38(4):915–945. https://doi.org/10.3102/00028312038004915
Gates AE (2017) Benefits of a STEAM collaboration in Newark, New Jersey: Volcano simulation through a glass-making experience. J Geosci Educ 65(1):4–11. https://doi.org/10.5408/16-188.1
Geng J, Jong MSY, Chai CS (2019) Hong Kong teachers’ self-efficacy and concerns about STEM education. Asia-Pac Educ Researcher 28:35–45. https://doi.org/10.1007/s40299-018-0414-1
Han J, Kelley T, Knowles JG (2022) Building a sustainable model of integrated stem education: investigating secondary school STEM classes after an integrated STEM project. Int J Technol Design Educ. https://doi.org/10.1007/s10798-022-09777-8
Henderson S, Holland J, McGrellis S, Sharpe S, Thomson R (2012) Storying qualitative longitudinal research: Sequence, voice and motif. Qualitative Res 12(1):16–34. https://doi.org/10.1177/1468794111426232
Hennissen P, Beckers H, Moerkerke G (2017) Linking practice to theory in teacher education: A growth in cognitive structures. Teach Teach Educ 63:314–325. https://doi.org/10.1016/j.tate.2017.01.008
Henson RK (2002) From adolescent angst to adulthood: Substantive implications and measurement dilemmas in the development of teacher efficacy research. Educ Psychologist 37:137–150. https://doi.org/10.1207/S15326985EP3703_1
Herro D, Quigley C (2016) Innovating with STEAM in middle school classrooms: remixing education. Horizon 24(3):190–204. https://doi.org/10.1108/OTH-03-2016-0008
Herro D, Quigley C, Cian H (2019) The challenges of STEAM instruction: Lessons from the field. Action Teach Educ 41(2):172–190. https://doi.org/10.1080/01626620.2018.1551159
Huang B, Jong MSY, Tu YF, Hwang GJ, Chai CS, Jiang MYC (2022) Trends and exemplary practices of STEM teacher professional development programs in K-12 contexts: A systematic review of empirical studies. Comput Educ 189:104577. https://doi.org/10.1016/j.compedu.2022.104577
Hunter-Doniger T, Sydow L (2016) A journey from STEM to STEAM: A middle school case study. Clearing House 89(4-5):159–166. https://doi.org/10.1080/00098655.2016.1170461
Hwang S, Xu R, Yao Y, Cai J (2024) Learning to teach through problem posing: A teacher’s journey in a networked teacher−researcher partnership. J Math Behav 73:101120. https://doi.org/10.1016/j.jmathb.2023.101120
Jacques LA, Cian H, Herro DC, Quigley C (2020) The impact of questioning techniques on STEAM instruction. Action Teach Educ 42(3):290–308. https://doi.org/10.1080/01626620.2019.1638848
Jankvist UT (2009) A categorization of the “whys” and “hows” of using history in mathematics education. Educ Stud Math 71:235–261. https://doi.org/10.1007/s10649-008-9174-9
Jiang H, Chugh R, Turnbull D, Wang X, Chen S (2023) Modeling the impact of intrinsic coding interest on STEM career interest: evidence from senior high school students in two large Chinese cities. Educ Inf Technol 28:2639–2659. https://doi.org/10.1007/s10639-022-11277-0
Jiang H, Chugh R, Turnbull D, Wang X, Chen S (2024a) Exploring the effects of technology-related informal mathematics learning activities: A structural equation modeling analysis. Int J Sci Math Educ . Advance online publication. https://doi.org/10.1007/s10763-024-10456-4
Jiang H, Islam AYMA, Gu X, Guan J (2024b) How do thinking styles and STEM attitudes have effects on computational thinking? A structural equation modeling analysis. J Res Sci Teach 61:645–673. https://doi.org/10.1002/tea.21899
Jiang H, Turnbull D, Wang X, Chugh R, Dou Y, Chen S (2022) How do mathematics interest and self-efficacy influence coding interest and self-efficacy? A structural equation modeling analysis. Int J Educ Res 115:102058. https://doi.org/10.1016/j.ijer.2022.102058
Jiang H, Wang K, Wang X, Lei X, Huang Z (2021) Understanding a STEM teacher’s emotions and professional identities: A three-year longitudinal case study. Int J STEM Educ 8:51. https://doi.org/10.1186/s40594-021-00309-9
Kelley TR, Knowles JG, Holland JD, Han J (2020) Increasing high school teachers self-efficacy for integrated STEM instruction through a collaborative community of practice. Int J STEM Educ 7:14. https://doi.org/10.1186/s40594-020-00211-w
Klassen RM, Tze VMC, Betts SM, Gordon KA (2011) Teacher efficacy research 1998–2009: Signs of progress or unfulfilled promise? Educ Psychol Rev 23(1):21–43. https://doi.org/10.1007/s10648-010-9141-8
Land M (2020) The importance of integrating the arts into STEM curriculum. In: Stewart AJ, Mueller MP, Tippins DJ (eds), Converting STEM into STEAM programs, pp. 11–19. Springer. https://doi.org/10.1007/978-3-030-25101-7_2
Lee C, Bobko P (1994) Self-efficacy beliefs: Comparison of five measures. J Appl Psychol 79(3):364–369. https://doi.org/10.1037/0021-9010.79.3.364
Li W, Chiang FK (2019) Preservice teachers’ perceptions of STEAM education and attitudes toward STEAM disciplines and careers in China. In: Sengupta P, Shanahan MC, Kim B, (eds), Critical, transdisciplinary and embodied approaches in STEM education. Springer. https://doi.org/10.1007/978-3-030-29489-2_5
Liu M, Zwart R, Bronkhorst L, Wubbels T (2022) Chinese student teachers’ beliefs and the role of teaching experiences in the development of their beliefs. Teach Teach Educ 109:103525. https://doi.org/10.1016/j.tate.2021.103525
Liu S, Xu X, Stronge J (2018) The influences of teachers’ perceptions of using student achievement data in evaluation and their self-efficacy on job satisfaction: evidence from China. Asia Pac Educ Rev 19:493–509. https://doi.org/10.1007/s12564-018-9552-7
Long T, Zhao G, Yang X, Zhao R, Chen Q (2021) Bridging the belief-action gap in a teachers’ professional learning community on teaching of thinking. Professional Dev Educ 47(5):729–744. https://doi.org/10.1080/19415257.2019.1647872
Lu L, Wang F, Ma Y, Clarke A, Collins J (2019) Exploring Chinese teachers’ commitment to being a cooperating teacher in a university-government-school initiative for rural practicum placements. In: Liu WC, Goh CCM (eds), Teachers’ perceptions, experience and learning, pp. 33–54. Routledge, London
Lyu S, Niu S, Yuan J, Zhan Z (2024) Developing professional capital through technology-enabled university-school-enterprise collaboration: an innovative model for C-STEAM preservice teacher education in the Greater Bay area. Asia Pacific J Innov Entrepreneurship. https://doi.org/10.1108/APJIE-01-2024-0014
Marco N, Palatnik A (2024) From “learning to variate” to “variate for learning”: Teachers learning through collaborative, iterative context-based mathematical problem posing. J Math Behav 73:101119. https://doi.org/10.1016/j.jmathb.2023.101119
Merriam SB (1998) Qualitative research and case study applications in education. Jossey-Bass Publishers, Hoboken, New Jersey
Morris DB, Usher EL (2011) Developing teaching self-efficacy in research institutions: A study of award-winning professors. Contemp Educ Psychol 36(3):232–245. https://doi.org/10.1016/j.cedpsych.2010.10.005
Morris DB, Usher EL, Chen JA (2017) Reconceptualizing the sources of teaching self-efficacy: A critical review of emerging literature. Educ Psychol Rev 29(4):795–833. https://doi.org/10.1007/s10648-016-9378-y
Matusovich HM, Streveler RA, Miller RL (2010) Why do students choose engineering? A qualitative, longitudinal investigation of students’ motivational values. J Eng Educ 99(4):289–303. https://doi.org/10.1002/j.2168-9830.2010.tb01064.x
Näykki P, Kontturi H, Seppänen V, Impiö N, Järvelä S (2021) Teachers as learners–a qualitative exploration of pre-service and in-service teachers’ continuous learning community OpenDigi. J Educ Teach 47(4):495–512. https://doi.org/10.1080/02607476.2021.1904777
OECD (2018) Teaching and learning international survey (TALIS) 2018 conceptual framework. OECD, Paris
Örnek T, Soylu Y (2021) A model design to be used in teaching problem posing to develop problem-posing skills. Think Skills Creativity 41:100905. https://doi.org/10.1016/j.tsc.2021.100905
Ord K, Nuttall J (2016) Bodies of knowledge: The concept of embodiment as an alternative to theory/practice debates in the preparation of teachers. Teach Teach Educ 60:355–362. https://doi.org/10.1016/j.tate.2016.05.019
Ozkan G, Umdu Topsakal U (2021) Investigating the effectiveness of STEAM education on students’ conceptual understanding of force and energy topics. Res Sci Technol Educ 39(4):441–460. https://doi.org/10.1080/02635143.2020.1769586
Park W, Cho H (2022) The interaction of history and STEM learning goals in teacher-developed curriculum materials: opportunities and challenges for STEAM education. Asia Pacific Educ Rev. https://doi.org/10.1007/s12564-022-09741-0
Perignat E, Katz-Buonincontro J (2019) STEAM in practice and research: An integrative literature review. Think Skills Creativity 31:31–43. https://doi.org/10.1016/j.tsc.2018.10.002
Philipp RA (2007) Mathematics teachers’ beliefs and affect. In: Lester Jr FK, (ed), Second handbook of research on mathematics teaching and learning, pp. 257–315. Information Age, Charlotte, NC
Quigley CF, Herro D, Jamil FM (2017) Developing a conceptual model of STEAM teaching practices. Sch Sci Math 117(1–2):1–12. https://doi.org/10.1111/ssm.12201
Ro S, Xiao S, Zhou Z (2022) Starting up STEAM in China: A case study of technology entrepreneurship for STEAM education in China. In: Ray P, Shaw R (eds), Technology entrepreneurship and sustainable development, pp. 115–136. Springer. https://doi.org/10.1007/978-981-19-2053-0_6
Roth KJ, Bintz J, Wickler NI, Hvidsten C, Taylor J, Beardsley PM, Wilson CD (2017) Design principles for effective video-based professional development. Int J STEM Educ 4:31. https://doi.org/10.1186/s40594-017-0091-2
Article PubMed PubMed Central Google Scholar
Ryu M, Mentzer N, Knobloch N (2019) Preservice teachers’ experiences of STEM integration: Challenges and implications for integrated STEM teacher preparation. Int J Technol Des Educ, 29:493–512. https://doi.org/10.1007/s10798-018-9440-9
Sancar R, Atal D, Deryakulu D (2021) A new framework for teachers’ professional development. Teach Teach Educ 101:103305. https://doi.org/10.1016/j.tate.2021.103305
Sanz-Camarero R, Ortiz-Revilla J, Greca IM (2023) The place of the arts within integrated education. Arts Educ Policy Rev. https://doi.org/10.1080/10632913.2023.2260917
Shirani F, Henwood K (2011) Continuity and change in a qualitative longitudinal study of fatherhood: relevance without responsibility. Int J Soc Res Methodol 14(1):17–29. https://doi.org/10.1080/13645571003690876
Smith CE, Paré JN (2016) Exploring Klein bottles through pottery: A STEAM investigation. Math Teach 110(3):208–214. https://doi.org/10.5951/mathteacher.110.3.0208
Soprano K, Yang L (2013) Inquiring into my science teaching through action research: A case study on one pre-service teacher’s inquiry-based science teaching and self-efficacy. Int J Sci Math Educ 11(6):1351–1368. https://doi.org/10.1007/s10763-012-9380-x
Stake RE (1995) The art of case study research. Sage Publication, Thousand Oaks, California
Stohlmann M, Moore T, Roehrig G (2012) Considerations for teaching integrated STEM education. J Pre Coll Eng Educ Res 2(1):28–34. https://doi.org/10.5703/1288284314653
Strauss AL, Corbin JM (1990) Basics of qualitative research. Sage Publications, Thousand Oaks, California
Taimalu M, Luik P (2019) The impact of beliefs and knowledge on the integration of technology among teacher educators: A path analysis. Teach Teach Educ 79:101–110. https://doi.org/10.1016/j.tate.2018.12.012
Takahashi A, McDougal T (2016) Collaborative lesson research: Maximizing the impact of lesson study. ZDM Math Educ 48:513–526. https://doi.org/10.1007/s11858-015-0752-x
Thompson AG (1992) Teachers’ beliefs and conceptions: A synthesis of the research. In: Grouws DA (ed), Handbook of research on mathematics teaching and learning, pp. 127–146. Macmillan, New York
Tschannen-Moran M, Woolfolk Hoy A, Hoy WK (1998) Teacher efficacy: Its meaning and measure. Rev Educ Res 68:202–248. https://doi.org/10.3102/00346543068002202
Unfried A, Rachmatullah A, Alexander A, Wiebe E (2022) An alternative to STEBI-A: validation of the T-STEM science scale. Int J STEM Educ 9:24. https://doi.org/10.1186/s40594-022-00339-x
Uştu H, Saito T, Mentiş Taş A (2021) Integration of art into STEM education at primary schools: an action research study with primary school teachers. Syst Pract Action Res. https://doi.org/10.1007/s11213-021-09570-z
Vaziri H, Bradburn NM (2021) Flourishing effects of integrating the arts and humanities in STEM Education: A review of past studies and an agenda for future research. In: Tay L, Pawelski JO (eds), The Oxford handbook of the positive humanities, pp. 71–82. Oxford University Press, Oxford
Wang K, Wang X, Li Y, Rugh MS (2018) A framework for integrating the history of mathematics into teaching in Shanghai. Educ Stud Math 98:135–155. https://doi.org/10.1007/s10649-018-9811-x
Wang Z, Jiang H, Jin W, Jiang J, Liu J, Guan J, Liu Y, Bin E (2024) Examining the antecedents of novice stem teachers’ job satisfaction: The roles of personality traits, perceived social support, and work engagement. Behav Sci 14(3):214. https://doi.org/10.3390/bs14030214
Wenger E, McDermott R, Snyder WM (2002) Cultivating communities of practice. Harvard Business School Press, Boston, MA
Wong JT, Bui NN, Fields DT, Hughes BS (2022) A learning experience design approach to online professional development for teaching science through the arts: Evaluation of teacher content knowledge, self-efficacy and STEAM perceptions. J Sci Teacher Educ. https://doi.org/10.1080/1046560X.2022.2112552
Wyatt M (2015) Using qualitative research methods to assess the degree of fit between teachers’ reported self-efficacy beliefs and their practical knowledge during teacher education. Aust J Teach Educ 40(1):117–145
Xenofontos C, Andrews P (2020) The discursive construction of mathematics teacher self-efficacy. Educ Stud Math 105(2):261–283. https://doi.org/10.1007/s10649-020-09990-z
Yin R (2003) Case study research: Design and methods. Sage Publications, Thousand Oaks, California
Zakariya YF (2020) Effects of school climate and teacher self-efficacy on job satisfaction of mostly STEM teachers: a structural multigroup invariance approach. Int J STEM Educ 7:10. https://doi.org/10.1186/s40594-020-00209-4
Zee M, de Jong PF, Koomen HM (2017) From externalizing student behavior to student-specific teacher self-efficacy: The role of teacher-perceived conflict and closeness in the student–teacher relationship. Contemp Educ Psychol 51:37–50. https://doi.org/10.1016/j.cedpsych.2017.06.009
Zee M, Koomen HM (2016) Teacher self-efficacy and its effects on classroom processes, student academic adjustment, and teacher well-being: A synthesis of 40 years of research. Rev Educ Res 86(4):981–1015. https://doi.org/10.3102/0034654315626801
Zhan Z, Li Y, Mei H, Lyu S (2023) Key competencies acquired from STEM education: gender-differentiated parental expectations. Humanit Soc Sci Commun 10:464. https://doi.org/10.1057/s41599-023-01946-x
Zhan Z, Niu S (2023) Subject integration and theme evolution of STEM education in K-12 and higher education research. Humanit Soc Sci Commun 10:781. https://doi.org/10.1057/s41599-023-02303-8
Zhong B, Liu X, Li X (2024) Effects of reverse engineering pedagogy on students’ learning performance in STEM education: The bridge-design project as an example. Heliyon 10(2):e24278. https://doi.org/10.1016/j.heliyon.2024.e24278
Zhong B, Liu X, Zhan Z, Ke Q, Wang F (2022) What should a Chinese top-level design in STEM Education look like? Humanit Soc Sci Commun 9:261. https://doi.org/10.1057/s41599-022-01279-1
Zhou B (2021) Cultivation of Ed. M. to bring up “famous subject teachers”: practical exploration and policy suggestions. Teach Educ Res 33(5):19–26. https://doi.org/10.13445/j.cnki.t.e.r.2021.05.003
Zhou X, Shu L, Xu Z, Padrón Y (2023) The effect of professional development on in-service STEM teachers’ self-efficacy: a meta-analysis of experimental studies. Int J STEM Educ 10:37. https://doi.org/10.1186/s40594-023-00422-x
Download references
Acknowledgements
This research is funded by 2021 National Natural Science Foundation of China (Grant No.62177042), 2024 Zhejiang Provincial Natural Science Foundation of China (Grant No. Y24F020039), and 2024 Zhejiang Educational Science Planning Project (Grant No. 2024SCG247).
Author information
Xuesong Zhai
Present address: School of Education, City University of Macau, Macau, China
Authors and Affiliations
College of Education, Zhejiang University, Hangzhou, China
Haozhe Jiang & Xuesong Zhai
School of Engineering and Technology, CML‑NET & CREATE Research Centres, Central Queensland University, North Rockhampton, QLD, Australia
Ritesh Chugh
Hangzhou International Urbanology Research Center & Zhejiang Urban Governance Studies Center, Hangzhou, China
Department of Teacher Education, Nicholls State University, Thibodaux, LA, USA
School of Mathematical Sciences, East China Normal University, Shanghai, China
Xiaoqin Wang
College of Teacher Education, Faculty of Education, East China Normal University, Shanghai, China
You can also search for this author in PubMed Google Scholar
Contributions
Conceptualization - Haozhe Jiang; methodology - Haozhe Jiang; software - Xuesong Zhai; formal analysis - Haozhe Jiang & Ke Wang; investigation - Haozhe Jiang; resources - Haozhe Jiang, Xuesong Zhai & Xiaoqin Wang; data curation - Haozhe Jiang & Ke Wang; writing—original draft preparation - Haozhe Jiang & Ritesh Chugh; writing—review and editing - Ritesh Chugh & Ke Wang; visualization - Haozhe Jiang, Ke Wang & Xiaoqin Wang; supervision - Xuesong Zhai & Xiaoqin Wang; project administration - Xuesong Zhai & Xiaoqin Wang; and funding acquisition - Xuesong Zhai & Xiaoqin Wang. All authors have read and agreed to the published version of the manuscript.
Corresponding author
Correspondence to Xuesong Zhai .
Ethics declarations
Competing interests.
The authors declare no competing interests.
Ethical approval
This research was approved by the Committee for Human Research of East China Normal University (Number: HR 347-2022). The procedures used in this study adhere to the tenets of the Declaration of Helsinki.
Informed consent
Written informed consent was obtained from all participants in this study before data collection.
Additional information
Publisher’s note Springer Nature remains neutral with regard to jurisdictional claims in published maps and institutional affiliations.
Rights and permissions
Open Access This article is licensed under a Creative Commons Attribution-NonCommercial-NoDerivatives 4.0 International License, which permits any non-commercial use, sharing, distribution and reproduction in any medium or format, as long as you give appropriate credit to the original author(s) and the source, provide a link to the Creative Commons licence, and indicate if you modified the licensed material. You do not have permission under this licence to share adapted material derived from this article or parts of it. The images or other third party material in this article are included in the article’s Creative Commons licence, unless indicated otherwise in a credit line to the material. If material is not included in the article’s Creative Commons licence and your intended use is not permitted by statutory regulation or exceeds the permitted use, you will need to obtain permission directly from the copyright holder. To view a copy of this licence, visit http://creativecommons.org/licenses/by-nc-nd/4.0/ .
Reprints and permissions
About this article
Cite this article.
Jiang, H., Chugh, R., Zhai, X. et al. Longitudinal analysis of teacher self-efficacy evolution during a STEAM professional development program: a qualitative case study. Humanit Soc Sci Commun 11 , 1162 (2024). https://doi.org/10.1057/s41599-024-03655-5
Download citation
Received : 27 April 2024
Accepted : 12 August 2024
Published : 08 September 2024
DOI : https://doi.org/10.1057/s41599-024-03655-5
Share this article
Anyone you share the following link with will be able to read this content:
Sorry, a shareable link is not currently available for this article.
Provided by the Springer Nature SharedIt content-sharing initiative
Quick links
- Explore articles by subject
- Guide to authors
- Editorial policies

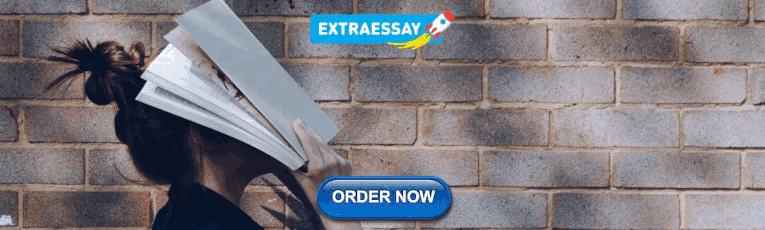
IMAGES
VIDEO
COMMENTS
The Most Important Research Skills (With Examples)
Top Skills for Research Analysts in 2024 (+Most ...
What Are Analytical Skills? Definition, Examples and Tips
What Are Research Skills? Types, Benefits, & Examples
Research Skills: What They Are and Why They're Important
4 Ways to Improve Your Analytical Skills - HBS Online
What Are Analytical Skills? 9 Examples & Tips to Improve
Research Skills: What They Are and How They Benefit You
How to Become a Research Analyst: A 2024 Guide
By following these steps and consistently practicing, you'll enhance your R skills and become more proficient in data analysis and research. How to Display R Skills on Your Resume 3. SQL. SQL (Structured Query Language) is a programming language used by Research Analysts to retrieve, manipulate, and analyze data stored in relational databases.
Go through the Research Analyst posting you're applying to, and identify hard skills the company is looking for. For example, skills like Data Analysis, AutoCAD and Geographic Information Systems (GIS) are possible skills. These are skills you should try to include on your resume. Expand. 2.
45 Examples of Analytical Skills in the Workplace
How to Display Survey Design Skills on Your Resume. 11. NVivo. NVivo is a qualitative data analysis (QDA) software designed to help researchers organize, analyze, and find insights in unstructured or qualitative data like interviews, open-ended survey responses, articles, social media, and web content.
Research Skills for Your Resume: 40 Examples
Research Analyst - Overview, Types, Skills Needed
15 Research Analyst Skills For Your Resume
Analytical skills (With examples for career success)
Analytical and Research Skills. Being able to objectively analyse an issue or problem, and critically appraise different aspects or solutions. This includes being able to reason well, define and review the work of others, apply theories or abstract concepts and explain your thinking methodically to others. This skill may also be described as ...
9 Essential Data Analyst Skills: A Comprehensive Career ...
For additional information or for more information regarding administrative policies such as complaints and refunds, please contact Martha Leon at 212-903-8173. Register for AMA's Analytical skills training courses and develop the basic analytical skills to conduct research and perform analysis.
11 Jobs Involving Research and Analysis (With Salaries)
Program Description The Data Science and Statistics PhD degree curriculum at The University of Texas at Dallas offers extensive coursework and intensive research experience in theory, methodology and applications of statistics. During their study, PhD students acquire the necessary skills to prepare them for careers in academia or in fields that require sophisticated data analysis […]
2023 New York State Offshore Wind Skills Analysis [PDF] - This report is designed to build on past New York State analyses by answering several follow-on questions emerging from industry stakeholders that are foundational to developing a New York State offshore wind workforce. This report helps inform strategic policy, funding, and programmatic ...
Key responsibilities Lead AI research projects independently, focusing on imaging and vision applications within pharmaceutical research and development using scientific, rigorous, and reproducible methodologyDevelop and implement state-of-the-art AI algorithms for image processing, analysis, segmentation, and interpretation, focusing on data modalities such as MRI, histopathology ...
Analytical Skills - Definition, Types, Importance
Over time, the demand for AI-related jobs has spread outside the traditional Information, Communication and Telecommunications industries, with the Finance and Insurance industry increasingly demanding AI skills. At a regional level, the higher demand for AI-related jobs is found in London and research hubs.
Background History-taking is an essential clinical competency for qualified doctors. The limitations of the standardized patient (SP) in taking history can be addressed by the virtual standardized patient (VSP). This paper investigates the accuracy of virtual standardized patient simulators and evaluates the applicability of the improved system's accuracy for diagnostic teaching support and ...
The relationship between teachers' professional learning and students' outcomes (such as achievements, skills and attitudes) has been a subject of extensive discussion and research for many ...