REVIEW article
A systematic review on machine learning methods for root cause analysis towards zero-defect manufacturing.

- 1 Department of Energy Systems, University of Thessaly, Larisa, Greece
- 2 Centre for Research and Technology Hellas, Information Technologies Institute, Thessaloniki, Greece
- 3 Foundation for Research and Technology Hellas (FORTH), Heraklion, Greece
The identification of defect causes plays a key role in smart manufacturing as it can reduce production risks, minimize the effects of unexpected downtimes, and optimize the production process. This paper implements a literature review protocol and reports the latest advances in Root Cause Analysis (RCA) toward Zero-Defect Manufacturing (ZDM). The most recent works are reported to demonstrate the use of machine learning methodologies for root cause analysis in the manufacturing domain. The popularity of these technologies is then summarized and presented in the form of visualizing graphs. This enables us to identify the most popular and prominent methods used in modern industry. Although artificial intelligence gains more and more attraction in smart manufacturing, machine learning methods for root cause analysis seem to be under-explored. The literature survey revealed that only limited reviews are available in the field of RCA towards zero-defect manufacturing using AI and machine learning; thus, it attempts to fill this gap. This work also presents a set of open challenges to determine future developments.
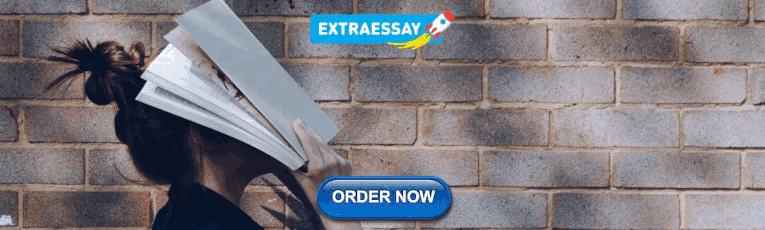
1 Introduction
1.1 motivation and scope.
With the onset of Industry 4.0, manufacturing companies are in need of a continuous upgrade of their manufacturing processes in terms of products and operations to become more competitive. In their effort to provide an optimized production operation and consistent delivery of better products, detecting defects and identifying defect causes upstream becomes a crucial factor for the industry. More specifically, defect detection is used by the industry operators to conduct a quality inspection in the production line, while defect source identification is used to further conduct smart quality control by identifying defects or anomalies per root cause.
Product quality improvement has been the cornerstone of Industry 4.0. The technological advances of the modern industry have brought new challenges in the quality improvement stage, which traditional quality control methodologies cannot handle. New concepts have been born, like the development of policies toward Zero-Defect Manufacturing (ZDM) ( Psarommatis et al., 2020a ), which support the migration to this new era ( Psarommatis et al., 2022 ). ZDM heavily relies on new technologies like virtual metrology, i.e., the ability to inspect product quality from production data without physically measuring it ( Dreyfus et al., 2022 ). An extensive review of ZDM opportunities and shortcomings has been presented in ( Psarommatis et al., 2020b ). ZDM can be further boosted by the integration of numerous Artificial Intelligence (AI) methods into traditional technologies, such as metrology, digital twins, internet of things, computer vision, augmented reality, quality control, and predictive maintenance; at least 15 EU-funded projects (mostly FoF-11 projects) and numerous individual publications may be identified in the literature ( OPTIMAI, 2021–2023 ; Papageorgiou et al., 2021 ).
Defect detection aims to monitor the production line and assess the quality of products. The quality requirements and defect specifications are provided by the end-users on a per-case level. Defect detection is mainly oriented along the following two directions i): defect detection from images, which include photographs, 3D scans, point clouds, and every other data format that can be directly converted into a picture, and ii) anomaly detection, through the analysis of time-series data, feature analysis and discovery of unexpected events. In the era of data-driven smart manufacturing ( Tao et al., 2018 ), defect detection procedures may benefit from AI technologies and transform manual operations into semi- or even fully automatic.
Industry 4.0 takes defect detection to the next level by pursuing not only defects but also their causes. Traditional defect detection targets capturing faults and defects; thus, it fails to provide any information about avoiding recurrence or how the defect is related to production processes. Defects may often go unattended and propagate along multiple production stages before being captured. This leads to taking corrective actions at a later stage, which is probably not related to the defect’s actual cause. A systematic methodology has been realized within smart manufacturing to identify the sources of defects, termed Root Cause Analysis (RCA). This methodology can seek among the production stages and determine the primary cause responsible for delivering defective products. The goal of RCA is obviously to prevent the recurrence of failures. RCA can be thought of as an optimization process in smart manufacturing that focuses on minimizing scrapping yields by addressing “what caused the failure?” rather than “what is the failure?” Thus, RCA can become a key asset to ZDM for industrial applications that involve many sequential and complex processes.
Traditional RCA methodologies such as Pareto Analysis, Fishbone Diagram, and Five-Whys have already been established in manufacturing ( Murugaiah et al., 2010 ; Jayswal et al., 2011 ; Ma et al., 2021 ). However, these methodologies are highly correlated with expertise and knowledge and thus are hindered by biases, individualism (as they cannot be saved or transferred), and time inefficiency. Moreover, the major drawback of traditional methodologies is the under-exploitation of the information that exists in the data from production processes. The large volume of data that is being gathered through production processes in industry 4.0 is considered very important for ZDM. Nevertheless, research questions arise about how these data can be exploited and what are the proper models for processing them, as these data are often multisensorial, multidimensional, and highly non-linear. Machine learning has proved that it can efficiently treat such types of data and thus, should be considered for AI-based RCA.
The aim of this work is to conduct a systematic review survey demonstrating the use of artificial intelligence methods for RCA in smart manufacturing, with a focus on Machine Learning Methods for RCA toward Zero-Defect Manufacturing. A key objective of the paper is to present the employed survey methodology in detail so as to not only present the current state of the art in a repeatable way but also guide readers on how to extend it in a consistent way and according to their needs.
An extensive literature review revealed the existence of only two surveys that regard RCA in manufacturing ( e Oliveira et al., 2022 ; Solé et al., 2017 ), none of which deals with AI technologies. The first survey covers a spectrum of RCA techniques applied in various industrial domains, such as the semiconductor and chemical industries ( e Oliveira et al., 2022 ). According to the survey, the most popular methodologies include association/classification rules, control charts, regression models, and principal component analysis. The second survey follows a different approach and categorizes available methodologies based on causality; deterministic and probabilistic methodologies are thoroughly reviewed, targeting mainly information technology applications ( Solé et al., 2017 ). According to this survey, Bayesian networks are among the most popular learning models in this area. The current paper focuses on the integration of ML methodologies into RCA models applied in smart manufacturing, thus, filling a gap in the current literature. The performed survey covers the most recent advances in a 5-year period, from 2017 up to date.
1.2 Models for ML-based root cause analysis
There is a wide range of AI/ML models that could be involved in the RCA process. These models belong either to the deterministic or the probabilistic group of AI-based methodologies. Each class expresses different attributes, may have different implementations and demonstrates different performance implications ( Solé et al., 2017 ). On the one hand, deterministic models are developed by applying statistical learning techniques, which allow these models to identify patterns in the data automatically. To accomplish that, the models need to be trained on large datasets to unearth boundaries and relationships in the data. The more data are used to train the model, the higher the predictive accuracy. On the other hand, probabilistic models can be constructed hierarchically from data, which allows their wide use for RCA. They enable reasoning behind the uncertainties inherent to most data, thus allowing for fully coherent inferences over complex data structures. Representative probabilistic models are those based on Bayesian Networks and probabilistic Fuzzy Cognitive Maps (FCMs). Since no domain knowledge is available to build these models, the only way is to use learning algorithms that exploit the raw data of the examined system. Various learning algorithms have been developed, oriented on either learning both structures and parameters of the model or just learning the parameters in a given structure.
Deep Learning (DL) is a division of ML which has recently displayed remarkable applicability in a range of different applications, as well as in smart manufacturing. In smart manufacturing, DL has found significant applicability for processing and analyzing big manufacturing data. In most cases, DL networks can be trained using supervised learning with large sets of training data. The most popular DL methods are the following:
1) Deep Neural Networks (DNNs). A DNN resembles an Artificial Neural Network (ANN) with many hidden layers. The difference is in the training process. DNN uses deep learning as a class of machine learning algorithms with the following main aspects: (a) use a cascade of multiple layers of non-linear processing units for feature extraction and transformation, (b) learn in supervised (e.g., classification), and/or unsupervised (e.g., pattern analysis) manners, and (c) learn multiple levels of representations that correspond to different levels of abstraction; the levels form a hierarchy of concepts. DNNs have more than three layers trained to model non-linear problems.
2) Convolutional Neural Networks (CNNs). They are among the most powerful deep learning techniques presenting notable capabilities in analyzing and classifying images. They are mainly employed in image processing applications (semantic segmentation, image classification, instance segmentation, object detection, etc. ). Their neurons architecture is based on the features of images they process (width, height, depth, etc. ). Typical CNNs have a similar structure to ANN and consist of one or more filters (i.e., convolutional layers), followed by aggregation/pooling layers in order to extract features for classification tasks. Since a CNN has similar characteristics to a standard ANN, it uses gradient descent and backpropagation for training tasks, whereas it contains pooling layers along with layers of convolutions. The vector that is sited at the end of the network architecture can deliver the final outputs.
3) Residual Neural Networks (Res-Nets). They are an extension of DNNs. They are highly considered in industrial applications where precision is vital for machinery health-state diagnosis. Res-Nets typically perform better than CNN-based approaches.
4) Recurrent neural networks (RNN). These are ANNs that utilize connections between units in order to form a directed graph along a sequence. RNNs use their internal memory to process such sequences, something that is not met in feed-forward ANNs. However, RNNs suffer from short-term memory and the problem of vanishing gradient during backpropagation. This is resolved by the Long Short-Term Memory (LSTM) algorithm, presented in the following.
5) Long Short-Term Memory networks (LSTMs). LSTMs excel over the original RNN due to their specific cell structure, which allows the algorithm to add or remove information from this cell using entities called gates. These gates control this memorizing process by allowing the model to learn which information to store in the long memory and which to discard. The cell state resembles a conveyor belt. It runs straight down the entire chain, with only some minor linear interactions. Gates are a way to optionally let information through. They are composed of a sigmoid neural net layer and a pointwise multiplication operation. LSTMs have been applied in predictive maintenance and prognostics in manufacturing processes.
DL techniques enable to i) automatically learn from data ii), detect underlying patterns, and eventually, iii) make efficient decisions. With automatic feature learning and high-volume modeling capabilities, DL provides an advanced analytics tool for smart manufacturing in the big data era. It uses a cascade of layers of non-linear processing to learn the representations of data corresponding to different levels of abstraction. The hidden patterns underneath each other are then identified and predicted through end-to-end optimization. Thus, DL offers great potential to boost data-driven manufacturing applications. Several review papers extracted from the related literature show the actual implementations of ML and DL methods in factory operations within the smart manufacturing domain.
2 Systematic literature review
The presented review is based on the “Preferred Reporting Items for Systematic reviews and Meta-Analyses” (PRISMA) principles ( Moher et al., 2010 ). This methodology is globally accepted in the research community as it leads to well-structured article surveys, allowing investigators to perform accurate systematic reviews. According to PRISMA, a predefined set of questions needs to be defined. Then, identified documents are collected, filtered, analyzed, and critically evaluated.
2.1 Research questions
The goals of this review can be outlined as i) to determine what AI-based technologies have been exploited toward RCA within smart manufacturing; ii) to investigate specific applications and find out how these technologies have been implemented; thus, iii) to shed light in current practices so that further improvements may be built. To achieve these goals, the following research questions (RQs) have been posed:
1) What AI algorithms have been employed? This RQ reveals the available tools in the quiver of current smart manufacturing.
2) What is the accommodated industrial application? This RQ reveals the field of application.
3) What is the popularity of each employed methodology? This RQ reveals the trends in the employment of AI for RCA; popular and well-established methods will probably be the foundation for future developments.
Each article found during the search has been reviewed to answer these questions. The answers were compiled in a comprehensive way to give the reader a clear picture of the current state of the art and its potential for future developments.
2.2 Review protocol
The review protocol includes the selection of proper sources, the definition of search terms, and the definition of acceptance/rejection criteria. These are described in the following.
2.2.1 Search sources
Amongst the many available databases and search engines, Google Scholar and Scopus were preferred because i) they are among the most popular in the research society, ii) they provide consistent and reproducible search results, and iii) Google Scholar provides free access, while Scopus is available to most scientists and researchers through institutional subscriptions.
2.2.2 Search terms
Predetermined search terms were employed for searching the most suitable articles; search terms were pursued in title, abstract, and keywords. The literature search strategy was conducted by utilizing the keywords “defect identification,” “root cause analysis,” “deep learning,” “machine learning,” “artificial intelligence,” “industry,” and “manufacturing,” using the depicted query string ( Figure 1 ).
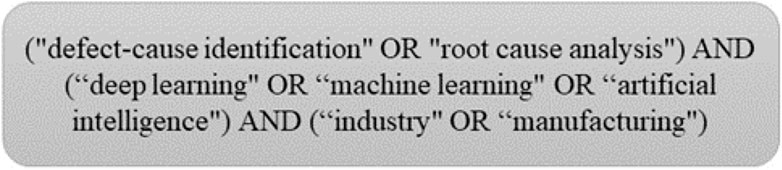
FIGURE 1 . Query string for literature search on RCA.
Raw search returned a vast number of results; thus, the inclusion/exclusion criteria needed to be defined so that only the most relevant works were considered for evaluation. The inclusion/exclusion criteria are outlined in the following paragraphs and summarized in Table 1 in the form of acceptance/rejection rules.
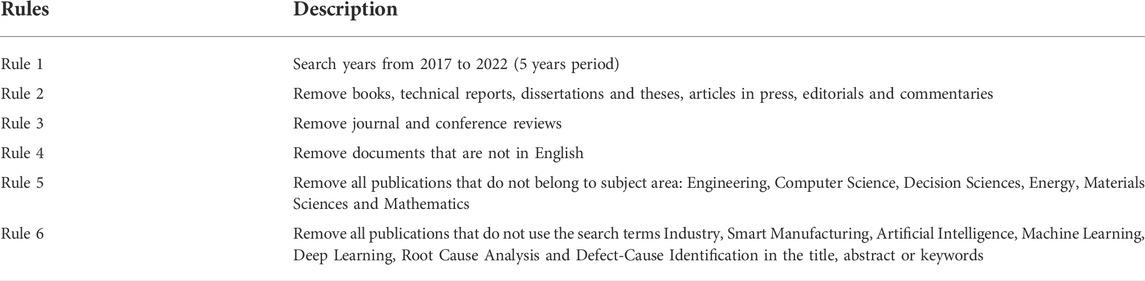
TABLE 1 . Inclusion/Exclusion rules.
2.2.3 Inclusion criteria
This study focuses on ML-based methods for RCA in smart manufacturing. Thus, studies in consideration should include RCA methodologies applied in industrial environments and applications and implementing AI, DL, or ML in any of their flavors. Since the state of the art is investigated, only the latest works within the last 5 years were pursued, namely works published between 1 January 2017, and 15 April 2022 (date of literature search). Only publications in English were considered, as all primary studies are published in English.
The inclusion criteria include: 1) papers published; 2) the process of root cause analysis and defect-cause identification in industry; 3) ML-based algorithms, including both traditional ML and DL techniques incorporated for defect-cause analysis; 4) ML methods for RCA in smart manufacturing.
2.2.4 Exclusion criteria
To reduce the number of articles for investigation and keep this survey within scope, numerous exclusion criteria were set. First, articles published before 2017 were not considered, as they do not reflect the current state of the art. Furthermore, RCA methodologies should be literally based on AI/ML methods; otherwise, they are considered irrelevant. Material of questionable quality content was also neglected; such material includes websites and online material, student theses, book chapters, editorials, commentaries, non-original research articles, protocols, meta-analyses, etc. , as well as all non-peer-reviewed content. Journal and conference reviews were omitted as well. Nevertheless, articles published in languages other than English were eliminated by this search.
2.3 Literature collection
After a thorough review of all mined articles, only those fulfilling the established criteria were considered in the industrial or manufacturing domain. After the adoption of the PRISMA method and only those articles that were explicit about the subject of this short review were retrieved. The overall search process is graphically illustrated in Figure 2 .
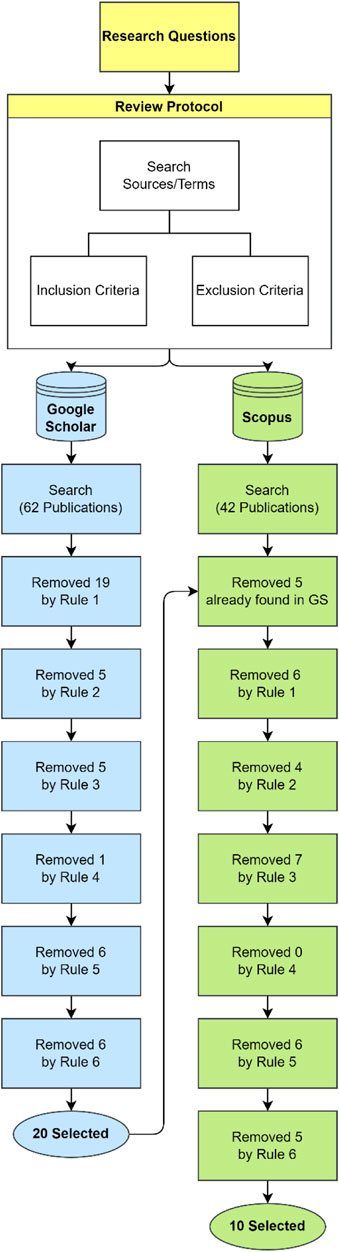
FIGURE 2 . Research screening process.
Finally, 30 research papers were selected for further analysis. The selected articles are listed in Table 2 , along with the year of publication, the method employed, the scope of the study, and the industrial process in which the methodology is applied.
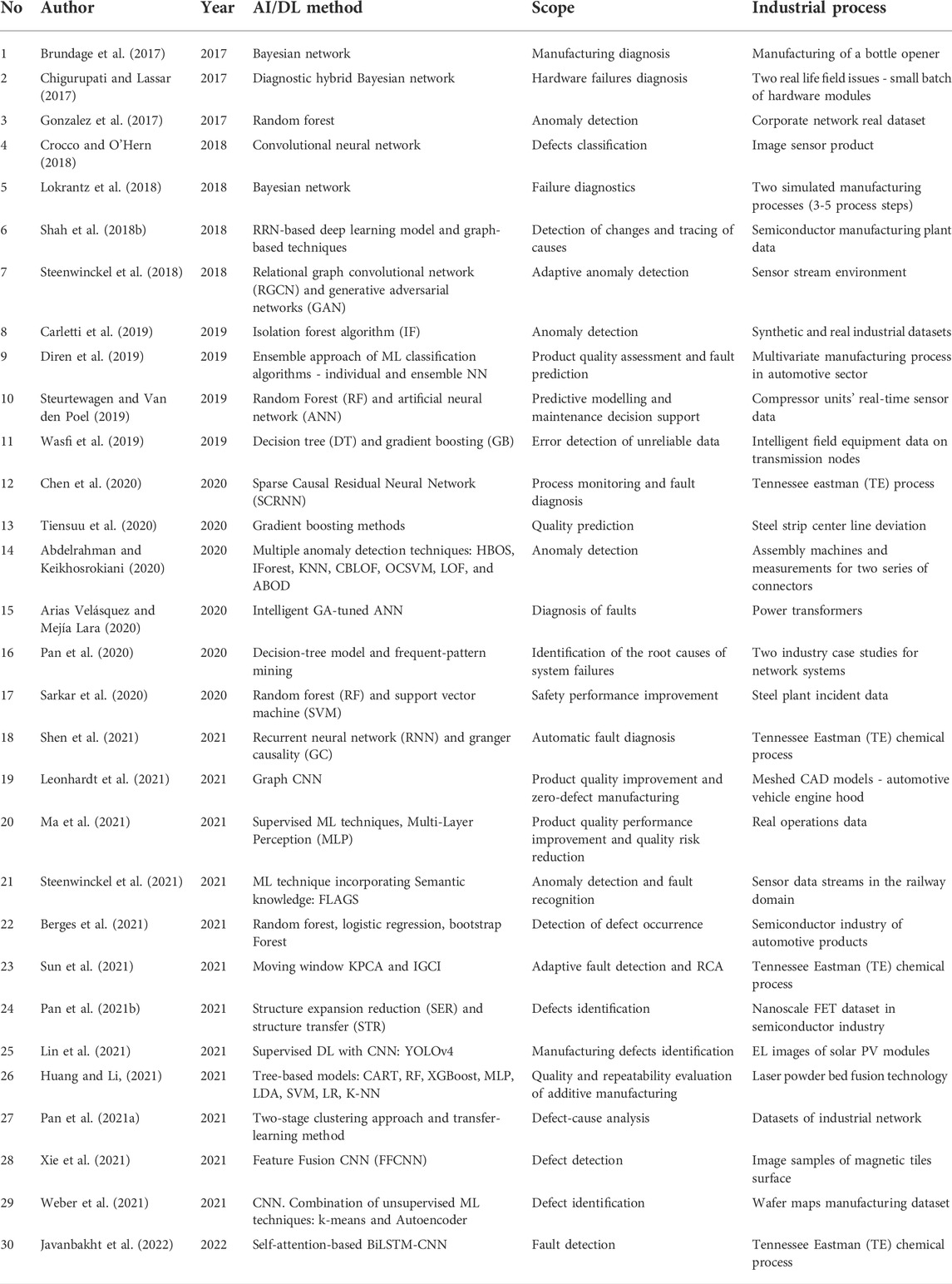
TABLE 2 . Collection of reviewed articles.
The review for AI-based RCA was divided into two families according to the applied models’ specifications.
The first family of AI methods incorporated for automatic RCA is based on probabilistic models, which comprise several techniques, each one having different implementations and certain performance implications. In this category, the main representatives are the Bayesian and Hybrid Bayesian networks. A Diagnostic Hybrid Bayesian Network is built in ( Chigurupati and Lassar, 2017 ) to model the cause-effect relationship between the degradation parameters (cause) and failure modes (effect) that occur in order to capture the cause-symptom relationship within the examined hardware system. The required step of assigning conditional probabilities for building up the Bayesian network topology is accomplished with the deployment of the linearly varying Weibull and Lognormal distributions. This model was applied in two real-life field use cases concerning a small batch of hardware modules. The utilization of a Bayesian Network is also discussed in ( Lokrantz et al., 2018 ) as part of the proposed framework for automatic root cause analysis and failure diagnostics in two simulated manufacturing processes, which consist of three and five process steps. To build the Bayesian network, various algorithms were utilized for structure learning, parameter learning, and inference. Regarding model training on historical data, the inference is conducted on the causal nodes, whereas the root causes of possible new process failures were determined. Finally, the result of inference was given in the form of conditional probabilities of the desired variables. The next use case involves the determination of possible causes in the manufacturing process of a bottle opener, termed the “Lion’s Jaw” ( Brundage et al., 2017 ). This article introduces a framework for the formal, systematic manufacturing diagnosis of problems arising in manufacturing systems. A Bayesian network was selected because it models the cause-effect (causal) relationship between nodes, increasing the system’s accuracy. The required probabilities for model training were obtained from the Simio simulation model ( simi.com ).
The second family of methods reported in this review comprises ML and Artificial Neural Networks, which are referred to as deterministic models since there is no involvement of randomness in calculating the output state of the model. In this regard, an ANN classifier tuned with an intelligent Genetic Algorithm (GA) is proposed in ( Arias Velásquez and Mejía Lara, 2020 ) to improve the root cause analysis and diagnosis of faults in power transformers. Moreover, the authors in ( Ma et al., 2021 ) develop a big-data-driven root cause analysis system utilizing ML techniques. More specifically, they apply K-Nearest Neighbor (K-nn) and Neural Network (NN) classifiers to improve the performance of RCA in their effort to enhance product quality performance and reduce quality risk. The proposed framework comprises three distinct modules: Problem Identification (PI), Root Cause Identification (RCI), and Permanent Corrective Action. In the RCI Module, a supervised ML method is deployed to detect possible root causes of the defined quality problem. Then, the Multi-Layer Perception (MLP) model is employed to define quality problems and identify the root causes of the quality problems.
The contribution of another NN for industrial root cause diagnosis is presented in ( Chen et al., 2020 ). The Sparse Causal Residual Neural Network (SCRNN) model is a ML-based method which seeks to figure out the causal relationships and causality lags between multiple variables. It seeks to predict the future state of a target variable using as input the previous state of the multiple time series, behaving in a regressive way, thus directly determining the causal relationship between variables after optimization. SCRNN comprises two modules: Variable selection and Fitting. In the second module, the fault variables are determined, and then the RCA follows by deploying the SCRNN, ending up in the isolation and recovery of the faults.
In the field of manufacturing industry, another ML model was built, which deployed several anomaly detection techniques for improving product quality in two assembly lines ( Abdelrahman and Keikhosrokiani, 2020 ). These techniques include Histogram-Based Outlier Score (HBOS), IForest, K-nn, Cluster-Based Local Outlier Factor (CBLOF), One Class Support Vector Machine (OCSVM), Local Outlier Factor (LOF), and Angular-Based Outlier Detector (ABOD). Their behaviour was assessed through the application of two performance metrics. Among these models, K-nn and ABOD showed the highest performance. The authors performed an RCA using the Pareto chart to identify those variables that cause the anomalies. Also, 2 ML algorithms, namely Random Forest (RF) and Support Vector Machine (SVM) were deployed in ( Sarkar et al., 2020 ) to accurately classify accident reports in a steel plant, using text classification approaches and evaluate their usefulness. The proposed RCA implementation aimed to find hidden causal factors that would help the steel plant company to take proper precautionary measures to minimize injuries, as RCA provides a much deeper insight into the root causes behind the incidents. In the case of RF, such a model was built in ( Steurtewagen and Van den Poel, 2019 ) and further compared with an ANN to determine potential root causes of machinery breakdowns and, more specifically, to identify possible breakage points of compressor units that constitute the examined case study. RCA was performed to implement predictive modelling as well as to accurately predict compressor behaviour based on sensor data. Two more approaches based on RF models were developed in ( Gonzalez et al., 2017 ; Berges et al., 2021 ) on two different case studies. The first deals with the identification of root causes of errors that happen in a network, and are based on a historical dataset of events, while the second refers to the detection of the signals triggering defect occurrence in the semiconductor industry of automotive products.
Isolation Forest (IF) is another ML approach, and it is proposed in ( Carletti et al., 2019 ) for the task of Anomaly Detection. Specifically, the IF algorithm is involved in the Depth-based Isolation Forest Feature Importance (DIFFI) framework, which is proposed for defining and evaluating feature importance in industrial scenarios and further enables simple RCA. IF is part of the isolation procedure, which defines a tree-like model of decisions, called an Isolation Tree, in which each node is linked with a variable, and its children are determined based on a splitting value. According to it, a feature is defined as important for anomaly detection when it can isolate samples, meaning that it can induce isolation of outliers at small depths and does not contribute to the isolation of inliers. RCA can then be performed based on features that are marked as the most relevant by the proposed approach.
Among the frameworks that belong in the same ML and ANN family of methods, a scheme that consists of a moving window using kernel principal component analysis (KPCA) and an information geometric causal inference (IGCI) is reported in ( Sun et al., 2021 ) and concerns the adaptive fault detection and RCA. Another method for fault root diagnosis is based on Recurrent Neural Network (RNN) and Granger Causality (GC), as proposed in ( Shen et al., 2021 ). The framework comprises three steps. First, a Principal Component Analysis is performed to detect faults. Then, Dynamic Time Warping (DTW) is used to group fault candidate variables based on similarity and perform causality testing. Lastly, the combination of RNN and GC models is used to locate the root cause of faults. In addition, a new methodology for adaptive anomaly detection and RCA was explored in ( Steenwinckel et al., 2021 ), using ML along with expert knowledge. The developed Fused-AI interpretable Anomaly Generation System (FLAGS) framework combines the advantages of both data- and knowledge-driven techniques towards optimizing anomaly detection, fault recognition, and RCA, while providing interpretable causes for the occurred anomalies. The proposed methodology was tested using a predictive maintenance case in the railway domain. This method seems to reduce downtime and provide more insight into frequently occurring problems while it gives the operator a new tool to investigate possible errors in the system.
A ML-guided methodology concerning tree-based models (Decision Tree, RF, and XGBoost) for RCA is demonstrated in ( Huang and Li, 2021 ) to identify influencing production parameters for repeatability improvement and quality evaluation of additive manufacturing printed parts of the laser powder bed fusion (L-PBF) technology. An unsupervised RCA method deploying a decision-tree model is also demonstrated in ( Pan et al., 2020 ), which is combined with frequent-pattern mining to cluster the data. Two case studies from the industry are involved, adopting real-world test data from network systems. In ( Wasfi et al., 2019 ), a Decision Tree (DT) algorithm along with a Gradient Boosting (GB) model were selected to implement pattern recognition algorithms, which target the recognition of those transmission nodes characterized by unreliable data. On the other hand, the GB model was proposed in ( Tiensuu et al., 2020 ) to find root causes behind the center line deviation of the steel strips. Using feature extraction and domain knowledge, the authors performed data reduction and new features construction to train the GB model. Their case study showed a correlation of errors between a former procedure (the hot rolling process) and a latter procedure (the RAP-line process).
In addition to individual ML methods, it is recorded a combination of such methods in the related literature. For example, a NN ensemble technique was developed in ( Diren et al., 2019 ) to determine the root cause of uncontrolled situations in a Multivariate Manufacturing Process in the automotive sector. Five different root causes were identified in the process of painting seats, door panels, and bumper modules, paying attention to surface quality and fluidity. In ( Pan et al., 2021a ), another AI method based on ensemble learning is built to facilitate transfer learning in order to select the valuable samples from a source product similar to the target product. The examined case studies refer to two industry designs. The aim of the proposed model is to improve the RCA accuracy on the target product.
Apart from the two AI-based families of methodologies, as reported above, there is one more group of models that has a significant contribution to RCA, demonstrating notable performance. This group includes the DL and CNNs models, which are developed and applied in certain case studies as presented in the following lines. More specifically, the authors in ( Crocco and O’Hern, 2018 ) perform a statistical RCA using CNNs for manufacturing quality improvement of an image sensor array. They investigate defects classification in an image sensor product to their corresponding origin. Root cause failure analysis was performed for all pixels exhibiting failures. In another study, a novel process for RCA utilizing unsupervised machine learning techniques for clustering and a CNN deep learning network for classification is proposed ( Weber et al., 2021 ). The use case concerns a known root-cause for specific defect patterns in wafer maps. For automatic defect identification, an end-to-end CNN architecture is also proposed in ( Xie et al., 2021 ), termed fusion feature CNN (FFCNN), consisting of three modules: feature extraction, feature fusion and decision-making. This intelligent machine-vision-based system was developed to detect surface defects on magnetic tiles during the production stage. AlexNet, VGG-16, and Resnet-50 were investigated for the development of the appropriate network. Another CNN model, namely the BiLSTM-CNN classifier, was built in ( Javanbakht et al., 2022 ) to analyze alarm data of the Tennessee Eastman chemical process. After its training, the neural network was used for online fault detection, identifying the root cause of the alarms by the first five alarms of each fault scenario. Its structure includes six layers; input layer, 1D-CNN, BiLSTM, Self-Attention, Dense layer, and output layer.
In an effort to enhance the detection rate and automatically interpret the cause of an anomaly, the author in ( Steenwinckel et al., 2018 ) adds prior experts’ knowledge into ML systems. One such technique is the Relational Graph Convolutional Network (RGCN), which operates on realistic knowledge graphs, fusing both ML and semantics to improve anomaly detection together with the ability to identify root causes inside a stream of data accurately. In another study, a Graph Convolutional Neural Network (GCNN) is also utilized as a part of the proposed model termed Process Estimator Neural Network (PEN), which was developed to tackle the non-linear issue of the state-sparse model ( Leonhardt et al., 2021 ). PEN is actually a NN that uses a single graph convolution layer followed by two fully connected layers and constitutes a novel RCA methodology targeting modern multistage assembly lines for increasing product quality and implementing zero-defect manufacturing.
In order to perform root cause detection, the authors in ( Shah et al., 2018a ) used an RNN to extract two types of causal relationships (interdependencies and lagged dependencies) among the time-series of the examined system. They used dynamic dependency graphs that have been extracted from multivariate time series data.
A CNN is also utilized in the automatic Segmentation of Cells and Defect Detection (SCDD) system, proposed in ( Lin et al., 2021 ), that aims to visually inspect defects in Electroluminescence (EL) images of single-crystalline silicon solar panels in photovoltaic (PV) industries. ResNet50 was utilized as a classifier, while YOLOv4 contributed as a detector for the panel-based defect detection. By applying cutting-edge deep CNNs, this approach achieves highly accurate defect detection rates from limited training samples of cell images. Finally, two ML models, termed STR (Structure transfer) and SER (Structure Expansion Reduction), and an ensemble (MIX) model were proposed to conduct a failure analysis through defect detection on nanoscale field-effect transistors (FET) of the semiconductor industry ( Pan et al., 2021b ). These ML models were trained on the same two defect datasets (FinFET and GAA-FET), providing notable accuracy in the identification of failures of the devices, guiding the acceleration of the production process.
The taxonomy of employed ML-based models is shown in Figure 3 .
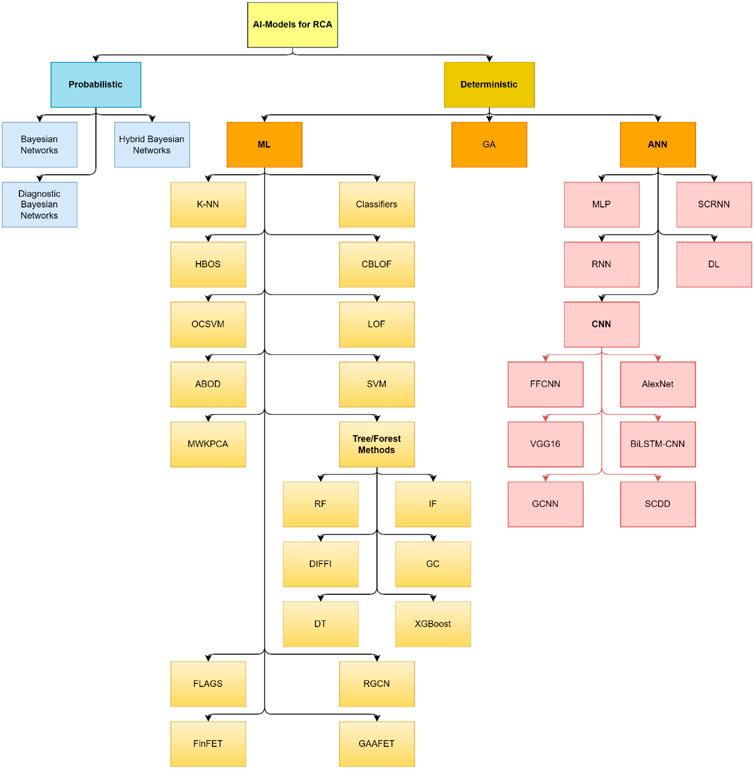
FIGURE 3 . Taxonomy of employed AI-based models for RCA.
To sum up, detecting root causes of defected parts in the production process is a highly demanding task in the manufacturing industry and needs extensive knowledge from experts to perform analyses. However, this demands high costs and offers low flexibility ( Mueller et al., 2018 ). In this direction, ML-based techniques are able to model a vast amount of process data empirically, contributing to an automated root cause analysis, also reducing the costs and the necessary expert knowledge. In contrary to manual RCA which was previously conducted also using predefined root causes as training, ML-based algorithms possess the ability of analyzing complex data of different sources and types providing an automated way for root cause analysis. In that case, the abovementioned literature review brings together a number of ML methods that have been exploited toward the automatic RCA within smart manufacturing.
4 Discussion
4.1 findings.
Through the current systematic review, valuable outcomes have been extracted, as illustrated in the following graphs, and further analyzed, evaluated and discussed, so that certain insights are elicited, highlighting the contribution of ML methods for RCA in smart manufacturing. To begin with, Figure 4 shows the distribution of publications during the investigated period. There is an evident increase in the number of publications, reflecting the growing interest in AI/ML-based RCA. As for the limited number of publications in 2022, this is due to the fact that this review was conducted at the beginning of 2022. However, an increasing tendency in the number of publications is anticipated.
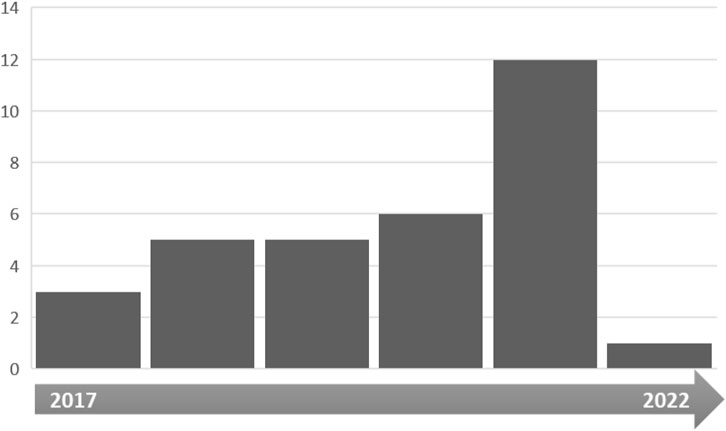
FIGURE 4 . The distribution of publications for 2017-2022.
The literature analysis regarding the utilization of various AI technologies dictates that the deterministic methods (ML-, DL-, and NN-based) is the most popular choice ( Figure 5 ) having 90% preference against the family of probabilistic models. In particular, the ML methods and their combinations are by far the most popular choice (60%), followed by DL and CNNs (30%), whereas the group of probabilistic methods (Bayesian and their Hybrid modules) come as the least employed methodologies for RCA with only 10%. This indicates that the major categories (ML, DL and CNNs) demonstrate their dominant presence in almost all case studies (90%) regarding AI-based RCA in smart manufacturing. This preference is attributed to the efficient learning capacity and performance of ANNs and the adequate performance of the traditional ML technologies for lesser amounts of input data.
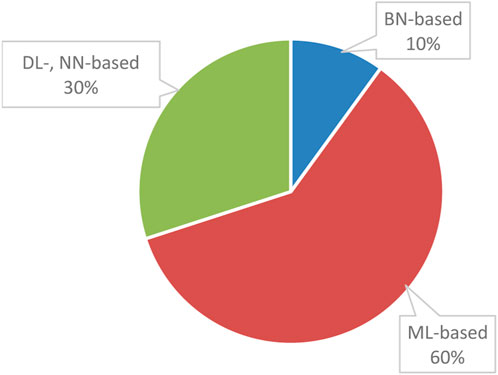
FIGURE 5 . Categorization of AI models employed for RCA.
The distribution of AI-based models exploitation shown in Figure 5 is further quantified in Figure 6 . CNNs are the most popular architecture (23%), as reported in the reviewed literature. Then, ANNs and Tree/Forest-based methods follow with 20% each, while the third best architecture is ML with 17%. Clustering is also well preferred (13%) either in its traditional forms (K-NN etc. ) or combined with some AI architectures. The Bayesian methods also exhibit the same popularity. On the other hand, SVM demonstrates reduced popularity (7%), whereas RNNs, ResNets and GA seem to be the least favored methods (3%). The rest of the examined studies are scattered among other less popular methodologies. This categorization was performed under the consideration that some of the works investigated might employ more than one AI method; thus, the overall sum of the individual ratios exceeds 100%.
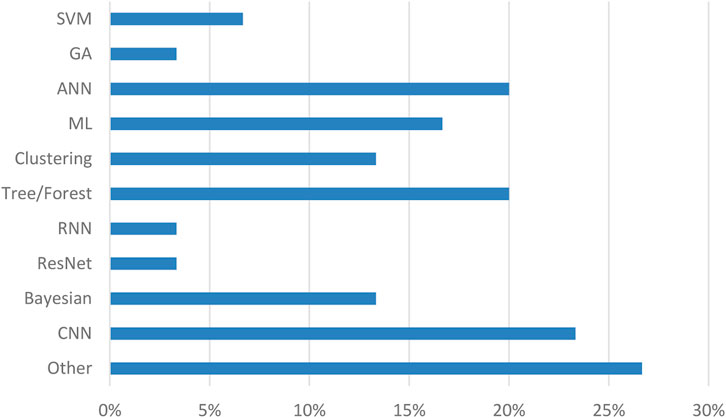
FIGURE 6 . Popularity of AI methodologies.
It is noteworthy that AI methods have been exploited in a wide variety of industrial sectors. Figure 7 shows the distribution in the percentage of all the industrial applications involved, considering the reviewed studies. The manufacturing sector is the leader in the use of AI methods as most reviewed papers (33%) concern some sort of manufacturing process. Quality Control/Assessment, Tennessee Eastman Process, and Data Analytics are equally (13%) employing AI techniques. The semiconductor industry follows (10%) along with Imaging applications (7%). One paper is related to Incident Analysis, targeting accident prevention. The rest of the papers (27%) are scattered among various other industrial sectors. It needs to be mentioned again, that the sum of the partial ratios exceeds 100%, as in some papers, more than one industrial sector is involved.
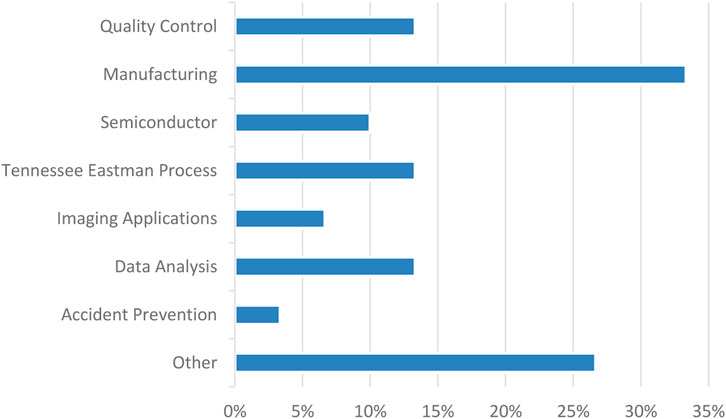
FIGURE 7 . Usage of AI methods in various industrial sectors.
Furthermore, the applications that currently benefit most from the ML-based RCA are summarized in Figure 8 . The majority of the ML-based RCA methods seem to be most embraced by Manufacturing (50%) and Quality Control (27%) applications. Manufacturing is further analyzed and categorized into general product fabrication applications (18%), semiconductor fabrication (14%), hardware and devices manufacturing (14%), and assembly line monitoring (4%).
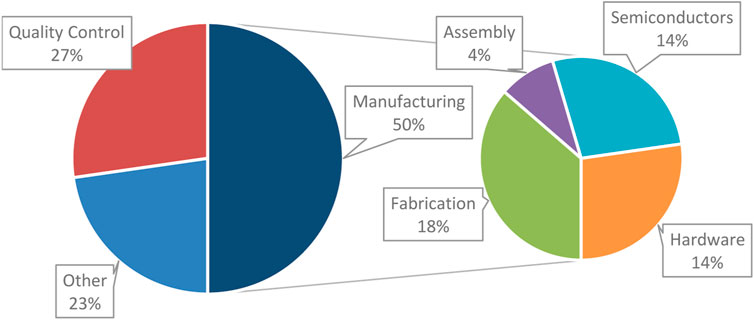
FIGURE 8 . Industrial applications currently using ML-based RCA.
4.2 Lessons learned
This systematic review was conducted to investigate the potential of using AI/ML for RCA in smart manufacturing. An increasing trend is demonstrated ( Figure 4 ) regarding the number of relevant publications in the literature, which proves a growing interest in this area. This review was directed by a set of research questions; the answers to these questions provided insight regarding the developments and popularity of employed methods. First, a taxonomy of AI algorithms and methods has been compiled ( Figure 3 ). This taxonomy lists the available tools, in a manner that considers the relevance among each other. That is, if a tool exhibits weaknesses, a similar one in the list may be exploited to overcome the observed limitations of the previous tool. Second, AI/ML-based RCA is not limited to a few specialized fields, but rather spans a wide range of industrial applications. The heterogeneity of industrial sectors ( Figure 7 ) reveals a growing endeavor for novel AI tools for RCA. Third, Neural Networks in their many variants seem to be the dominant technology in AI-based RCA. This is attributed to their exceptional learning capabilities, their architectural flexibility, and their extraordinary capacity to discover underlying patterns.
4.3 Challenges
Despite the successful use of AI in RCA, there are still challenges to be addressed:
1) Explainability. The domain of AI is considered unclear by humans as regards its behavior, especially in the process of decision-making. This originates from AI models’ high complexity, ambiguity and the increased source of data, along with the inexplicit AI learning methods employed. Hence, the produced results cannot be directly and adequately explained by humans, and this leads to a sort of failure in applying AI models to a number of critical problems, failing overall to configure the best decision-making process. In this direction, Explainable AI (XAI) is a promising domain that allows humans to uncover clarity and reasoning in AI systems in an effort to comprehend AI models’ complex behavior at certain tasks and to arrive at a specific decision. On that basis, the incorporation of XAI into RCA methods would surely strengthen confidence in derived decisions.
2) Quality of Training. The performance of AI algorithms in RCA strongly depends on the availability of high-quality data. However, lack of adequate and suitable sources of data, as well as data scarcity, are common issues in the training process of AI models. Four papers were identified dealing with data for AI-based in RCA, including big-data, feature importance, data streams, etc. Thus, innovative data processing methods (e.g., data augmentation) are highly needed for efficient RCA and should probably be a topic of deeper investigation.
3) Standardization and Interoperability. As demonstrated in the presented graphs, there is a broad range of industrial applications that already benefit from AI-based RCA. Each operator may, of course, use their own protocols and practices. However, the establishment of standard rules and compatible interfaces and protocols would enable broad collaborations and joint efforts.
4) Data Privacy. The progress in AI and especially in DL is the result of a collaboration of multiple contributions, including open-source data offered by the community. Open-source datasets e.g., ImageNet ( Deng et al., 2009 ), played a key role in establishing AI methods and boosted their development and optimization. AI-based RCA can also benefit from such datasets. However, the nature of RCA requires datasets from different production stages that could potentially expose sensitive information about the industrial provider. Thus, obtaining a real industrial benchmark dataset to further evaluate the effectiveness of AI methods for RCA is a real challenge.
5) Security. The industrial environment requires secure transactions. On the one hand, data security would prevent the leakage of sensitive or confidential information. On the other hand, data integrity is also necessary to prevent corruption and information loss. Such issues may be dealt with using current technologies like blockchain, but this certainly is a topic that deserves its own research.
6) New technologies. The development of AI-based methods is progressing at a fast pace and new tools appear every day. Digital twins provide a means to create a digital replica of the manufacturing line, which can be employed to tailor and optimize production parameters without affecting the running production. Digital twins may be exploited towards RCA, but this requires future research on this topic.
4.4 Concluding remarks
AI technologies have demonstrated remarkable efficiency in the implementation of RCA in smart manufacturing. A wide range of AI models is increasingly involved in the manufacturing processes that struggle to provide optimized production operation and consistent delivery of better products. To achieve that, quality inspection and smart quality control become crucial processes in the production line towards conducting detection of defects and defect source identification. This systematic literature review aims to demonstrate the integration of various AI methods into RCA models applied in smart manufacturing, investigating their implementation methodology as well as current practices. To accomplish the goals of this work, the PRISMA method was followed, while proper database sources were selected, including Google Scholar and Scopus, to mine published scientific papers from the year 2017 up to 2022. The followed review protocol also included the search term and the inclusion and exclusion criteria set by the authors. The list of the retrieved papers contains the authors, the year of publication, the AI method employed, the scope of the AI model’s implementation, and the industrial process the AI method was applied in toward defect source identification. The results have shown an increasing trend in the number of publications that report on the contribution of AI methods for RCA in smart manufacturing. The first goal regarding the availability of the ΑΙ tools in the realm of current smart manufacturing was accordingly answered. Based on our study, it was found that several deterministic and probabilistic AI technologies were applied for RCA in industry to identify defect sources in the early stages. Such technologies include ML-, DL- and NN-based models as well as Bayesian and their Hybrid modules. A variety of industrial processes were also reported to comply with the second goal of this review study. These were related to the manufacturing sector, semiconductor industry, data analysis, quality control and several others. Furthermore, this study also revealed the popularity of the AI technologies that have been employed for RCA in smart manufacturing so far. That was the third research question to be answered in this study. The findings showed that CNNs are the most popular architecture, followed by ANNs and Tree/Forest-based methods, while ML, Clustering, and Bayesian come with a smaller percentage in their contribution to RCA in industry 4.0. SVM, RNNs, ResNets, and GA seem to be the least favored methods in the examined field. In addition, this research study pinpoints a number of challenges that need to be considered in the future implementation of AI methodologies in RCA. Among them, explainability, quality of training, interoperability, privacy, and security have a significant share and role when AI-based technologies are exploited towards RCA within smart manufacturing, and thus they need to be further investigated.
To sum up, many AI technologies have already been successfully incorporated within the framework for RCA toward zero-defect manufacturing. In fact, there is a growing interest in further development, as implied by the number of publications per year. To accomplish an efficient literature survey, a set of research questions were initially posed. Then, a systematic literature review protocol was properly chosen to answer the posed queries. The conducted survey was focused on the most popular online, well-respected, and highly available databases, covering a wide range of disciplines, from the theoretical to the applied. Taking a close look at the produced outcomes, it is noted that ML and DL have shown a major contribution to RCA for smart industry applications. Seeking further, it seems that Neural Networks in their many variants (ANNs, CNNs, RNNs, etc. ) are the current trend for automatic RCA in smart manufacturing using AI models. However, despite the current success, there are still open issues regarding explainability, data quality, standardization, data security, integrity, etc. These challenges deserve papers of their own and will be dealt with in future extensions of this work.
Author contributions
KP laid the foundation and coordinated with the team to bring out the paper’s content. His significant contributions are establishing the Introduction , AI-based models, systematic literature review, Results , and Discussion of this review paper. AR and TT worked on a systematic literature review and wrote the Results and Discussion of this review paper. The sections Discussion of Results and Conclusion are contributed significantly by EP, TT, and KP. EP made all the edits, referencing, abstract, conclusion, and communication with the group and brought the contents of the manuscript to the current shape. TT has greatly contributed during the conceptualizing phase, and his contributions are greatly in articulating the contents of this manuscript and performing the revisions. ND, DT, and GM have contributed to editing. All authors are involved in discussing the contents/design/flow of the paper. All authors contributed to the article and approved the submitted version.
This work has been supported by the European Commission through project OPTIMAI, funded by the European Union (H2020-NMBP-TR-IND-2020-singlestage, Topic: DT-FOF-11-2020, GA 958264).
Conflict of interest
The authors declare that the research was conducted in the absence of any commercial or financial relationships that could be construed as a potential conflict of interest.
Publisher’s note
All claims expressed in this article are solely those of the authors and do not necessarily represent those of their affiliated organizations, or those of the publisher, the editors and the reviewers. Any product that may be evaluated in this article, or claim that may be made by its manufacturer, is not guaranteed or endorsed by the publisher.
Author disclaimer
The opinions expressed in this paper are those of the authors and do not necessarily reflect the views of the European Commission.
Abdelrahman, O., and Keikhosrokiani, P. (2020). Assembly line anomaly detection and root cause analysis using machine learning. IEEE Access 8, 189661–189672. doi:10.1109/access.2020.3029826
CrossRef Full Text | Google Scholar
Arias Velásquez, R. M., and Mejía Lara, J. V. (2020). Root cause analysis improved with machine learning for failure analysis in power transformers. Eng. Fail. Anal. 115, 104684. doi:10.1016/j.engfailanal.2020.104684
Berges, C., Bird, J., Shroff, M. D., Rongen, R., and Smith, C. (2021). “Data analytics and machine learning: Root-cause problem-solving approach to prevent yield loss and quality issues in semiconductor industry for automotive applications,” in 2021 IEEE International Symposium on the Physical and Failure Analysis of Integrated Circuits (IPFA) , Singapore, Singapore ( IEEE ) Available at: https://ieeexplore.ieee.org/document/9617238/ .(Accessed on April 18, 2022). doi:10.1109/IPFA53173.2021.9617238
Brundage, M. P., Kulvatunyou, B., Ademujimi, T., and Rakshith, B. (2017). “Smart manufacturing through a framework for a knowledge-based diagnosis system.” in Manufacturing Equipment and Systems , 3. Los Angeles, California, USA : American Society of Mechanical Engineers ASME . Available at: https://asmedigitalcollection.asme.org/MSEC/proceedings/MSEC2017/50749/Los%20Angeles,%20California,%20USA/269585 .
Google Scholar
Carletti, M., Masiero, C., Beghi, A., and Susto, G. A. (2019). “Explainable machine learning in industry 4.0: Evaluating feature importance in anomaly detection to enable root cause analysis,” in 2019 IEEE International Conference on Systems, Man and Cybernetics (SMC) , Bari, Italy ( IEEE ). Available at: https://ieeexplore.ieee.org/document/8913901/ (Accessed on Feb 18, 2022). doi:10.1109/SMC.2019.8913901
Chen, J., Zhao, C., and Sun, Y. (2020). “Sparse causal residual neural network for linear and nonlinear concurrent causal inference and root cause diagnosis,” in 2020 16th International Conference on Control, Automation, Robotics and Vision (ICARCV) , Shenzhen, China ( IEEE ). Available at: https://ieeexplore.ieee.org/document/9305508/ .(Accessed on Feb 18, 2022). doi:10.1109/ICARCV50220.2020.9305508
Chigurupati, A., and Lassar, N. (2017). “Root cause analysis using artificial intelligence,” in 2017 Annual Reliability and Maintainability Symposium (RAMS) , Orlando, FL, USA , 23-26 January 2017 ( IEEE ). Available at: http://ieeexplore.ieee.org/document/7889651/ .(Accessed on Feb 16, 2022). doi:10.1109/RAM.2017.7889651
Crocco, J. D., and O’Hern, J. R. P. R. (2018). Manufacturing quality improvement through statistical root cause analysis using convolution neural networks. US 2018/0293722 A Available at: https://patentimages.storage.googleapis.com/ae/88/9b/7085b4580621de/US20180293722A1.pdf .(Accessed on Oct 11, 2022).
Deng, J., Dong, W., Socher, R., Li, L. J., Li, K., and Fei-Fei, L. (2009). “ImageNet: A large-scale hierarchical image database,” in 2009 IEEE Conference on Computer Vision and Pattern Recognition , Miami, FL, USA ( IEEE ), 248–255. doi:10.1109/CVPR.2009.5206848
Diren, D. D., Boran, S., Selvi, I. H., and Hatipoglu, T. (2019). “Root cause detection with an ensemble machine learning approach in the multivariate manufacturing process,” in Industrial engineering in the big data era . Editors F. Calisir, E. Cevikcan, and H. Camgoz Akdag (Cham: Springer International Publishing ), 163–174. (Lecture Notes in Management and Industrial Engineering). Available at: http://link.springer.com/10.1007/978-3-030-03317-0_14 .(Accessed on April 18, 2022).
Dreyfus, P. A., Psarommatis, F., May, G., and Kiritsis, D. (2022). Virtual metrology as an approach for product quality estimation in industry 4.0: A systematic review and integrative conceptual framework. Int. J. Prod. Res. 60 (2), 742–765. doi:10.1080/00207543.2021.1976433
e Oliveira, E., Miguéis, V. L., and Borges, J. L. (2022). Automatic root cause analysis in manufacturing: An overview & conceptualization. J. Intell. Manuf . Available at: https://link.springer.com/10.1007/s10845-022-01914-3 .(Accessed on April 14, 2022). doi:10.1007/s10845-022-01914-3
Gonzalez, J. M. N., Jimenez, J. A., Lopez, J. C. D., and Parada, G. H. A. (2017). Root cause analysis of network failures using machine learning and summarization techniques. IEEE Commun. Mag. 55 (9), 126–131. doi:10.1109/mcom.2017.1700066
Huang, D. J., and Li, H. (2021). A machine learning guided investigation of quality repeatability in metal laser powder bed fusion additive manufacturing. Mater. Des. 203, 109606. doi:10.1016/j.matdes.2021.109606
Javanbakht, N., Neshastegaran, A., and Izadi, I. (2022). Alarm-based root cause analysis in industrial processes using deep learning. Available at: https://arxiv.org/abs/2203.11321 .(Accessed on April 18, 2022).
Jayswal, A., Li, X., Zanwar, A., Lou, H. H., and Huang, Y. (2011). A sustainability root cause analysis methodology and its application. Comput. Chem. Eng. 35 (12), 2786–2798. doi:10.1016/j.compchemeng.2011.05.004
Leonhardt, V., Claus, F., and Garth, C. (2021). Pen: Process Estimator neural Network for root cause analysis using graph convolution. J. Manuf. Syst. 62, 886–902. doi:10.1016/j.jmsy.2021.11.008
Lin, H. H., Dandage, H. K., Lin, K. M., Lin, Y. T., and Chen, Y. J. (2021). Efficient cell segmentation from electroluminescent images of single-crystalline silicon photovoltaic modules and cell-based defect identification using deep learning with pseudo-colorization. Sensors 21 (13), 4292. doi:10.3390/s21134292
PubMed Abstract | CrossRef Full Text | Google Scholar
Lokrantz, A., Gustavsson, E., and Jirstrand, M. (2018). Root cause analysis of failures and quality deviations in manufacturing using machine learning. Procedia CIRP 72, 1057–1062. doi:10.1016/j.procir.2018.03.229
Ma, Q., Li, H., and Thorstenson, A. (2021). A big data-driven root cause analysis system: Application of Machine Learning in quality problem solving. Comput. Industrial Eng. 160, 107580. doi:10.1016/j.cie.2021.107580
Moher, D., Liberati, A., Tetzlaff, J., and Altman, D. G. (2010). Preferred reporting items for systematic reviews and meta-analyses: The PRISMA statement. Int. J. Surg. 8 (5), 336–341. doi:10.1016/j.ijsu.2010.02.007
Mueller, T., Greipel, J., Weber, T., and Schmitt, R. H. (2018). Automated root cause analysis of non-conformities with machine learning algorithms. J. Mach. Eng. 18, 60–72. doi:10.5604/01.3001.0012.7633
Murugaiah, U., Benjamin, S. J., Marathamuthu, M. S., and Muthaiyah, S. (2010). Scrap loss reduction using the 5-whys analysis. Int. J. Qual. Reliab. Manag. 27 (5), 527–540. doi:10.1108/02656711011043517
OPTIMAI (2021–2023). OPTIMAI project. Available from: https://optimai.eu/ . GA 958264.
PubMed Abstract | Google Scholar
Pan, J., Low, K. L., Ghosh, J., Jayavelu, S., Ferdaus, M. M., and Lim, S. Y. (2021). Transfer learning-based artificial intelligence-integrated physical modeling to enable failure analysis for 3 nanometer and smaller silicon-based CMOS transistors. ACS Appl. Nano Mat. 4 (7), 6903–6915. doi:10.1021/acsanm.1c00960
Pan, R., Li, X., and Chakrabarty, K. (2021). “Unsupervised root-cause analysis with transfer learning for integrated systems,” in 2021 IEEE 39th VLSI Test Symposium (VTS) , San Diego, CA, USA . ( IEEE ). Available from: https://ieeexplore.ieee.org/document/9441030/ .(Accessed on April 18, 2022). doi:10.1109/VTS50974.2021.9441030
Pan, R., Zhang, Z., Li, X., Chakrabarty, K., and Gu, X. (2020). “Unsupervised root-cause analysis for integrated systems,” in 2020 IEEE International Test Conference (ITC) , Washington, DC, USA ( IEEE ). Available from: https://ieeexplore.ieee.org/document/9325268/ .(Accessed on April 18, 2022). doi:10.1109/ITC44778.2020.9325268
Papageorgiou, E., Theodosiou, T., Papageorgiou, K., Casanovas, P., Charalampous, P., and Dimitriou, N. (2021). State of the art survey. Available from: https://optimai.eu/wp-content/uploads/2021/07/OPTIMAI-D2.3-State-of-Art_V2.0_FINAL_SUBMISSION.pdf .
Psarommatis, F., May, G., Dreyfus, P. A., and Kiritsis, D. (2020). Zero defect manufacturing: State-of-the-art review, shortcomings and future directions in research. Int. J. Prod. Res. 58 (1), 1–17. doi:10.1080/00207543.2019.1605228
Psarommatis, F., Prouvost, S., May, G., and Kiritsis, D. (2020). Product quality improvement policies in industry 4.0: Characteristics, enabling factors, barriers, and evolution toward zero defect manufacturing. Front. Comput. Sci. 2, 26. doi:10.3389/fcomp.2020.00026
Psarommatis, F., Sousa, J., Mendonça, J. P., and Kiritsis, D. (2022). Zero-defect manufacturing the approach for higher manufacturing sustainability in the era of industry 4.0: A position paper. Int. J. Prod. Res. 60 (1), 73–91. doi:10.1080/00207543.2021.1987551
Sarkar, S., Ejaz, N., Kumar, M., and Maiti, J. (2020). “Root cause analysis of incidents using text clustering and classification algorithms,” in Proceedings of ICETIT 2019. Lecture notes in electrical engineering . Editors P. K. Singh, B. K. Panigrahi, N. K. Suryadevara, S. K. Sharma, and A. P. Singh (Cham: Springer International Publishing ), 605, 707–718. Available at: http://link.springer.com/10.1007/978-3-030-30577-2_63 .(Accessed on April 18, 2022).
Shah, S. Y., Dang, X. H., and Zerfos, P. (2018). “Root cause detection using dynamic dependency graphs from time series data,” in 2018 IEEE International Conference on Big Data (Big Data) , Seattle, WA, USA ( IEEE ), 1998–2003. doi:10.1109/BigData.2018.8622059
Shah, S. Y., Dang, X. H., and Zerfos, P. (2018). “Root cause detection using dynamic dependency graphs from time series data,” in 2018 IEEE International Conference on Big Data (Big Data) , Seattle, WA, USA ( IEEE ). Available at: https://ieeexplore.ieee.org/document/8622059/ .(Accessed on April 4, 2022). doi:10.1109/BigData.2018.8622059
Shen, G., Wang, P., Hu, K., and Ye, Q. (2021). “fault root cause diagnosis method based on recurrent neural network and granger causality,” in 2021 CAA Symposium on Fault Detection, Supervision, and Safety for Technical Processes (SAFEPROCESS) , Chengdu, China ( IEEE ). Available at: https://ieeexplore.ieee.org/document/9693579/ . doi:10.1109/SAFEPROCESS52771.2021
Solé, M., Muntés-Mulero, V., Rana, A. I., and Estrada, G. (2017). Survey on models and techniques for root-cause analysis. Available at: https://arxiv.org/abs/1701.08546 .(Accessed on April 14, 2022).
Steenwinckel, B. (2018). “Adaptive anomaly detection and root cause analysis by fusing semantics and machine learning,” in The semantic web: ESWC 2018 satellite events Lecture notes in computer science . Editors A. Gangemi, A. L. Gentile, A. G. Nuzzolese, S. Rudolph, M. Maleshkova, and H. Paulheim (Cham: Springer International Publishing ). Available at: http://link.springer.com/10.1007/978-3-319-98192-5_46 . doi:10.23919/PCICEurope46863.2019.9011628
Steenwinckel, B., De Paepe, D., Vanden Hautte, S., Heyvaert, P., Bentefrit, M., and Moens, P. (2021). Flags: A methodology for adaptive anomaly detection and root cause analysis on sensor data streams by fusing expert knowledge with machine learning. Future Gener. Comput. Syst. 116, 30–48. doi:10.1016/j.future.2020.10.015
Steurtewagen, B., and Van den Poel, D. (2019). “Root cause analysis of compressor failure by machine learning,” in 2019 Petroleum and Chemical Industry Conference Europe (PCIC EUROPE) , Paris, France ( IEEE ). Available at: https://ieeexplore.ieee.org/document/9011628/ .
Sun, Y., Qin, W., Zhuang, Z., and Xu, H. (2021). An adaptive fault detection and root-cause analysis scheme for complex industrial processes using moving window KPCA and information geometric causal inference. J. Intell. Manuf. 32 (7), 2007–2021. doi:10.1007/s10845-021-01752-9
Tao, F., Qi, Q., Liu, A., and Kusiak, A. (2018). Data-driven smart manufacturing. J. Manuf. Syst. 48, 157–169. doi:10.1016/j.jmsy.2018.01.006
Tiensuu, H., Tamminen, S., Haapala, O., and Röning, J. (2020). Intelligent methods for root cause analysis behind the center line deviation of the steel strip. Open Eng. 10 (1), 386–393. doi:10.1515/eng-2020-0041
Wasfi, B., Alqasimi, M., Al Kadem, M., and Alghamdi, A. (2019). “Innovative machine learning method to locate the root cause of the unreliable data coming from intelligent field equipment,” in Abu Dhabi International Petroleum Exhibition & Conference , Abu Dhabi, UAE , November 12, 2019 . Available at: https://onepetro.org/SPEADIP/proceedings/19ADIP/2-19ADIP/Abu%20Dhabi,%20UAE/216802 .doi:10.2118/197270-MS
Weber, C., Tripuramallu, A., Czerner, P., and Fathi, M. (2021). “Clustering wafer defect patterns within the semiconductor industry based on wafer Maps, using an agile unsupervised deep learning approach,” in 2021 IEEE International Conference on Systems, Man, and Cybernetics (SMC) [Internet] , Melbourne, Australia ( IEEE ). Available at: https://ieeexplore.ieee.org/document/9658907/ . doi:10.1109/SMC52423.2021.9658907
Xie, L., Xiang, X., Xu, H., Wang, L., Lin, L., and Yin, G. (2021). FFCNN: A deep neural network for surface defect detection of magnetic tile. IEEE Trans. Ind. Electron. 68 (4), 3506–3516. doi:10.1109/tie.2020.2982115
Keywords: smart manufacturing (Industry 4.0), root cause analysis, defect identification, artificial intelligence, deep learning, machine learning
Citation: Papageorgiou K, Theodosiou T, Rapti A, Papageorgiou EI, Dimitriou N, Tzovaras D and Margetis G (2022) A systematic review on machine learning methods for root cause analysis towards zero-defect manufacturing. Front. Manuf. Technol. 2:972712. doi: 10.3389/fmtec.2022.972712
Received: 18 June 2022; Accepted: 14 October 2022; Published: 28 October 2022.
Reviewed by:
Copyright © 2022 Papageorgiou, Theodosiou, Rapti, Papageorgiou, Dimitriou, Tzovaras and Margetis. This is an open-access article distributed under the terms of the Creative Commons Attribution License (CC BY). The use, distribution or reproduction in other forums is permitted, provided the original author(s) and the copyright owner(s) are credited and that the original publication in this journal is cited, in accordance with accepted academic practice. No use, distribution or reproduction is permitted which does not comply with these terms.
*Correspondence: Elpiniki I. Papageorgiou, [email protected]
Disclaimer: All claims expressed in this article are solely those of the authors and do not necessarily represent those of their affiliated organizations, or those of the publisher, the editors and the reviewers. Any product that may be evaluated in this article or claim that may be made by its manufacturer is not guaranteed or endorsed by the publisher.

An official website of the United States government
The .gov means it’s official. Federal government websites often end in .gov or .mil. Before sharing sensitive information, make sure you’re on a federal government site.
The site is secure. The https:// ensures that you are connecting to the official website and that any information you provide is encrypted and transmitted securely.
- Publications
- Account settings
- My Bibliography
- Collections
- Citation manager
Save citation to file
Email citation, add to collections.
- Create a new collection
- Add to an existing collection
Add to My Bibliography
Your saved search, create a file for external citation management software, your rss feed.
- Search in PubMed
- Search in NLM Catalog
- Add to Search
Successful risk assessment may not always lead to successful risk control: A systematic literature review of risk control after root cause analysis
Affiliation.
- 1 University of Cambridge Engineering Design Centre.
- PMID: 22359258
- DOI: 10.1002/jhrm.20090
Root cause analysis is perhaps the most widely used tool in healthcare risk management, but does it actually lead to successful risk control? Are there categories of risk control that are more likely to be effective? And do healthcare risk managers have the tools they need to support the risk control process? This systematic review examines how the healthcare sector translates risk analysis to risk control action plans and examines how to do better. It suggests that the hierarchy of risk controls should inform risk control action planning and that new tools should be developed to improve the risk control process.
© 2012 American Society for Healthcare Risk Management of the American Hospital Association.
PubMed Disclaimer
Similar articles
- Learning from Adverse Events in Obstetrics: Is a Standardized Computer Tool an Effective Strategy for Root Cause Analysis? Murray-Davis B, McDonald H, Cross-Sudworth F, Ahmed R, Simioni J, Dore S, Marrin M, DeSantis J, Leyland N, Gardosi J, Hutton E, McDonald S. Murray-Davis B, et al. J Obstet Gynaecol Can. 2015 Aug;37(8):728-735. doi: 10.1016/S1701-2163(15)30178-X. J Obstet Gynaecol Can. 2015. PMID: 26474230
- Systematic approaches to adverse events in obstetrics, Part II: Event analysis and response. Pettker CM. Pettker CM. Semin Perinatol. 2017 Apr;41(3):156-160. doi: 10.1053/j.semperi.2017.03.004. Epub 2017 May 22. Semin Perinatol. 2017. PMID: 28545653 Review.
- Root Cause Analysis, Part 1: Nonsterile Compounding. Cabaleiro J, Jackson K. Cabaleiro J, et al. Int J Pharm Compd. 2015 Nov-Dec;19(6):465-9. Int J Pharm Compd. 2015. PMID: 26891560
- Failure mode and effects analysis: too little for too much? Dean Franklin B, Shebl NA, Barber N. Dean Franklin B, et al. BMJ Qual Saf. 2012 Jul;21(7):607-11. doi: 10.1136/bmjqs-2011-000723. Epub 2012 Mar 23. BMJ Qual Saf. 2012. PMID: 22447819
- Approaches to formalization of the informal waste sector into municipal solid waste management systems in low- and middle-income countries: Review of barriers and success factors. Aparcana S. Aparcana S. Waste Manag. 2017 Mar;61:593-607. doi: 10.1016/j.wasman.2016.12.028. Epub 2016 Dec 27. Waste Manag. 2017. PMID: 28038906 Review.
- Pharmaceutical Interventions for Inpatients with Liver Cirrhosis and Liver Transplantation: A Systematic Review of Experimental Studies. Jibai N, Koch A, Ulmer TF, Erdmann P, Koeck JA, Eisert A. Jibai N, et al. J Clin Med. 2023 Nov 10;12(22):7030. doi: 10.3390/jcm12227030. J Clin Med. 2023. PMID: 38002644 Free PMC article. Review.
- What can Safety Cases offer for patient safety? A multisite case study. Liberati EG, Martin GP, Lamé G, Waring J, Tarrant C, Willars J, Dixon-Woods M. Liberati EG, et al. BMJ Qual Saf. 2024 Feb 19;33(3):156-165. doi: 10.1136/bmjqs-2023-016042. BMJ Qual Saf. 2024. PMID: 37734957 Free PMC article.
- Exploring the "Black Box" of Recommendation Generation in Local Health Care Incident Investigations: A Scoping Review. Lea W, Lawton R, Vincent C, O'Hara J. Lea W, et al. J Patient Saf. 2023 Dec 1;19(8):553-563. doi: 10.1097/PTS.0000000000001164. Epub 2023 Sep 15. J Patient Saf. 2023. PMID: 37712844 Free PMC article. Review.
- Systems-based investigation of patient safety incidents. Weaver S, Stewart K, Kay L. Weaver S, et al. Future Healthc J. 2021 Nov;8(3):e593-e597. doi: 10.7861/fhj.2021-0147. Future Healthc J. 2021. PMID: 34888447 Free PMC article.
- Adverse events reporting by obstetric units in Norway as part of their quality assurance and patient safety work: an analysis of practice. Johansen LT, Braut GS, Acharya G, Andresen JF, Øian P. Johansen LT, et al. BMC Health Serv Res. 2021 Sep 8;21(1):931. doi: 10.1186/s12913-021-06956-6. BMC Health Serv Res. 2021. PMID: 34493278 Free PMC article.
Publication types
- Search in MeSH
LinkOut - more resources
Full text sources.
- Ovid Technologies, Inc.
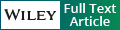
- Citation Manager
NCBI Literature Resources
MeSH PMC Bookshelf Disclaimer
The PubMed wordmark and PubMed logo are registered trademarks of the U.S. Department of Health and Human Services (HHS). Unauthorized use of these marks is strictly prohibited.
- Research article
- Open access
- Published: 05 June 2020
Our first review: an evaluation of effectiveness of root cause analysis recommendations in Hong Kong public hospitals
- Yick Ting A Kwok 1 , 2 ,
- Alastair PY Mah 1 , 3 , 4 &
- Katherine MC Pang 1 , 3
BMC Health Services Research volume 20 , Article number: 507 ( 2020 ) Cite this article
9968 Accesses
14 Citations
11 Altmetric
Metrics details
To evaluate the effectiveness of root cause analysis (RCA) recommendations and propose possible ways to enhance its quality in Hong Kong public hospitals.
A retrospective cross-sectional study was performed across 43 public hospitals and institutes in Hong Kong, reviewing RCA reports of all Sentinel Events and Serious Untoward Events within a two-year period. The incident nature, types of root causes and strengths of recommendations were analysed. The RCA recommendations were categorised as ‘strong’, ‘medium’ or ‘weak’ strengths utilizing the US’s Veteran Affairs National Center for Patient Safety action hierarchy.
A total of 214 reports from October 2016 to September 2018 were reviewed. These reports generated 504 root causes, averaging 2.4 per RCA report, and comprising 249 (49%) system, 233 (46%) staff behavioural and 22 (4%) patient factors. There were 760 recommendations identified in the RCA reports with an average of 3.6 per RCA. Of these, 18 (2%) recommendations were rated strong, 116 (15%) medium and 626 (82%) weak. Most recommendations were related to ‘training and education’ (466, 61%), ‘additional study/review’ (104, 14%) and ‘review/enhancement of policy/guideline’ (39, 5%).
Conclusions
This study provided insights about the effectiveness of RCA recommendations across all public hospitals in Hong Kong. The results showed a high proportion of root causes were attributed to staff behavioural factors and most of the recommendations were weak. The reasons include the lack of training, tools and expertise, appropriateness of panel composition, and complicated processes in carrying out large scale improvements. The Review Team suggested conducting regular RCA training, adopting easy-to-use tools, enhancing panel composition with human factors expertise, promoting an organization-wide safety culture to staff and aggregating analysis of incidents as possible improvement actions.
Peer Review reports
Strengths and limitations of this study
This study is the first study analyzing the root causes and evaluating the effectiveness of RCA recommendations in Hong Kong public hospitals.
This study used a robust methodology to analyse the root causes and recommendations of RCA reports.
This study highlighted the key factors contributing to the distribution of strengths of recommendations.
The study only reviewed the strengths of recommendations but not the work progresses, feasibilities and outcome measures.
There were no other local data available to benchmark the results.
Ensuring patient safety is always a major challenge for healthcare organisations. With the arousal of awareness in patient safety since the release of the Institute of Medicine report in 1999, initiatives in patient safety improvement have been launched in countries all over the world [ 1 , 2 , 3 , 4 ]. These patient safety initiatives may have arisen from recommendations identified through root cause analysis (RCA) which identified system vulnerabilities from past clinical incidents.
RCA is an investigation methodology commonly used in healthcare organisations aiming to learn from system failures of clinical incidents, and identify patient safety improvement initiatives leading to elimination or control of the risks leading to such events [ 5 , 6 , 7 , 8 ]. It analyses incidents retrospectively in a systematic approach under a just and fair environment. The products of an RCA are the contributory factors proximally leading to the incident, the root causes about latent factors from the system perspective and the action plans to prevent recurrence of similar incidents in the future [ 8 , 9 , 10 , 11 , 12 ].
Many hospitals use RCA to investigate adverse events, with an example being the U.S. Joint Commission requiring incidents to be systematically investigated [ 13 ]. Although organisations might have put vast resources, in terms of time and manpower, [ 10 , 14 ] in conducting RCAs, the number of preventable clinical incidents did not seem to reduce as would have expected. Researchers speculated that this might be due to the low effectiveness of RCA recommendations, and studies evaluating the strengths of recommendations found a high proportion of weak recommendations were put forward [ 10 , 14 , 15 ]. The low RCA effectiveness was widely discussed and suggested to be caused by the limited focus of weak recommendations that tackle superficial issues, mainly attempting to modify human behaviour which is believed to be difficult to sustain and cannot effectively eliminate the inherent latent risks at the system level [ 10 , 12 , 16 , 17 ]. The identified factors preventing the development of stronger recommendations include the flawed design and context of RCA, the lack of RCA training and expertise, poor leadership and the loose mechanisms in promoting a safety culture [ 10 , 14 , 16 , 18 , 19 ].
In Hong Kong, all 43 public hospitals and institutes are established under the Hong Kong Hospital Authority (HA). Sentinel Events (SEs) are mandatory reporting incidents for HA hospitals since 2007 [ 20 ]. The original definition of Sentinel Events was adopted from the Joint Commission on Accreditation of Healthcare Organisations in the US, and the list of SEs was referenced to the Australia’s Sentinel Events categories [ 21 ]. For these public hospitals, RCAs must be conducted to investigate the root causes of all SEs and provide corresponding recommendations. RCA panels have to be formed and comprise of members from the involved hospitals or institutes, technical experts with relevant clinical expertise, as well as representatives from the Hospital Authority Head Office (HAHO). In 2010, the HA further mandated performing RCA for events called Serious Untoward Events (SUEs), incidents that could have the potential for serious patient harm once occurred [ 20 ]. For SUEs, the RCA panel would comprise members nominated by the involved hospitals or institutes. An RCA report template is provided by HAHO to facilitate RCA panels to document their investigation results. The identities of involved staff and patients are not disclosed in any RCA reports to maintain confidentiality and reduce blame on individuals. According to the HA SE and SUE Policy, RCA reports have to be submitted to the HAHO for record within 8 weeks. A meeting between hospital representatives and the Quality and Safety Division of the HAHO is conducted every 6 months to follow up on the work progress of recommendations.
From October 2007 till September 2019, 445 SEs and 876 SUEs were reported in the 43 public hospitals and institutes of the HA [ 20 , 22 ]. With RCA having been introduced in the HA for more than a decade, there has been doubts within the organisation about the effectiveness of RCA in promoting patient safety and its sustainability. Since no corresponding research study in evaluating the effectiveness of RCA recommendations has ever been conducted in Hong Kong, the objectives of this study were to evaluate the effectiveness of RCA recommendations and propose suggestions for improving the quality of incident investigations in Hong Kong.
Study design
A retrospective study of RCA reports of all SEs and SUEs reported in the HA across a 2 year period was undertaken by an RCA Reports Review Team (the Review Team). A recent timeframe was chosen for review to gain a cross-sectional understanding of the quality of contemporary reports and recommend areas for improvement. The incident nature, types of root causes and strengths of recommendations were analysed. The root causes (in some RCA reports they were referred to as ‘contributory factors’, and for simplification purposes these are also regarded as ‘root causes’ in this study) of the events were reviewed and categorised according to the Contributory Factors Classification Framework of the National Patient Safety Agency (NPSA) of the United Kingdom (UK) [ 23 ]. Other classification methods like the Human Factors Analysis Classification System (HFACS) and Conceptual Framework for the International Classification for Patient Safety of the World Health Organisation (WHO) were considered for analysis but were not chosen [ 13 , 24 ]. The HFACS was not chosen because some of the root causes were difficult to classify in a trial analysis using some HA RCA reports due to the vague description of the root causes. The NPSA method is more comprehensive in each category with explanation than the WHO Framework, thus the former method is selected.
In evaluating the strengths of RCA recommendations, the action hierarchy of the United States of America’s Veteran Affairs National Center for Patient Safety was selected as this method is widely accepted internationally [ 12 ]. In our study, some local adaptations were made, for example, recommendation types from Hibbert’s study were included for easier classification of the action hierarchy [ 10 ]. In the evaluation, a ‘strong’, ‘medium’ or ‘weak’ strength is given to each recommendation by the Review Team. ‘Strong’ recommendations indicate the recommendations focus on system changes and are more sustainable while ‘weak’ recommendations are focusing on human behavioural change and are less effective in eliminating the risks. The ‘medium’ recommendations, e.g. a checklist, have elements of system improvement but still rely on human compliance to be effective.
The research was done without patient or public involvement.
Data collection
All SE and SUE RCA reports conducted from October 2016 to September 2018 in the 43 public hospitals and institutes under the governance of the HA in Hong Kong were reviewed.
The RCA reports review team
The Review Team comprised of 3 reviewers, in which 2 were the primary reviewers (YK and AM) and the remaining was the secondary reviewer (MP). The primary reviewers first analysed the root causes and recommendations from RCA reports independently. The classifications of root causes and recommendations were finalised if the two sets of results between the primary reviewers were the same. For results that had discrepancy, the primary reviewers would discuss the results for a consensus. Otherwise, the secondary reviewer would make the final decision on the classification if a mutually agreed decision between the primary reviewers could not be made. A descriptive analysis was then conducted to evaluate the types of root causes and strengths and types of recommendations.
Statistical analysis
Cohen’s kappa coefficients, calculated by SPSS version 21, were used to measure the inter-rater reliability of the reviewers [ 25 ].
Ethics approval and consent to participate
Approval from the Clinical Research Ethics Committee, Kowloon Central Cluster, which is the governing committee in research ethics for the Hospital Authority Head Office, was obtained.
A total of 214 SEs and SUEs from October 2016 to September 2018 were reported in the 43 public hospitals and institutes of the HA. The distribution of event types is listed in Table 1 . Nearly two-thirds (137, 64%) of the events were SUEs related to ‘medication error which could have led to death or permanent harm’, followed by 29 (14%) SEs related to ‘retained instruments or other material after surgery / interventional procedure’. There was no SE related to ‘medication error resulting in major permanent loss of function or death’ in the review period.
These RCA reports generated 504 root causes, averaging 2.35 per RCA report. The distribution of root causes by types is listed in Table 2 . Kappa values between the primary reviewers (YK and AM) was 0.637 and the primary reviewers and secondary reviewer separately was 0.765 (YK and MP) and 0.806 (AM and MP). The Kappa values indicated that there was a moderate to strong strength of agreement [ 25 ]. In the Task Factor of the NPSA method, the Review Team separately extracted one sub-factor related to policy or guideline adherence in Task Factor for analysis. This sub-factor, which is related to staff’s non-compliance with the existing policy or guidelines (the ‘violation’ root causes), was the most common factor amongst the root causes (152, 30%), followed by education and training (87, 17%) and staff factor (81, 16%). According to the nature of the 9 different factors in the NPSA method, the Review Team has grouped these factors into three major groups, namely the Patient Factors, the Staff Behavioural Factors and the System Factors. Since in error taxonomy, ‘violation’ is a type of human failure, the Review Team thus grouped the ‘violation’ factor into the Staff Behavioural Factors Group. The distribution of root causes according to our grouping was 49% (249/504), 46% (233/504) and 4% (22/504) in System Factors, Staff Behavioural Factors and Patient Factors respectively.
The Review Team identified 760 recommendations, with an average of 3.6 per RCA. The distribution of recommendations is listed in Table 3 . Kappa values between the primary reviewers (YK and AM) was 0.647 and between the primary reviewers and secondary reviewer separately was 0.730 (YK and MP) and 0.842 (AM and MP). The Kappa values indicated that there was a good strength of agreement [ 25 ]. Three RCAs did not provide any recommendations, all were SEs related to ‘death of an inpatient from suicide (including home leave)’. For the 760 recommendations, 18 (2%), 116 (15%) and 626 (82%) of them had strong, medium and weak strengths respectively. Most of the recommendations were ‘training and education (include counselling)’ (466, 61%), ‘additional study/review’ (104, 14%) and ‘review/enhancement of policy/guideline’ (39, 5%). The first two categories of recommendations, which were categorised with weak strength, had contributed to 75% of all recommendations.
This study is the first research in Hong Kong in understanding the effectiveness of RCA since its mandatory use for investigating SEs in 2007. The results of this study, especially in the categorization of root causes and recommendations, can provide meaningful information for the Hong Kong in improving its quality in incident investigations and subsequent risk mitigation actions.
One of the major challenges for the RCA panels is to identify the system vulnerabilities contributing to the underlying latent failures of the organisation [ 14 , 16 ]. According to the results by our grouping, about 46% of the root causes were identified to be related to staff behavioural factors, for example, violation, lack of vigilance and lapse of concentration. In some RCA reports, the Review Team noticed that only staff behavioural factors were identified and no other system factors had been identified. In fact, human failures like slips, lapse and mistakes are normal human behaviour and can be difficult to eliminate [ 26 ]. The identification of such root causes is only superficial and only demonstrates that humans are imperfect, but is not meaningful in solving the problem [ 14 ]. The large proportion of staff behavioural factors suggests that the RCA panels were not able to recognize different aspects of systems issues such as equipment and workflow design flaws, poor usability of system interface, work overload and inadequate safety culture [ 10 , 14 , 16 , 18 ]. This observation in the HA can be explained by the reason that the last corporate-led RCA training in the HA was conducted in 2009 and most RCA members have not been formally trained in RCA investigations in the past decade given the lack of training opportunities. This is especially true for the clinical experts who are invited to join the RCA according to their respective clinical expertise. These experts, generally with more than 10 years of clinical experience, would have limited understanding in RCA, safety systems knowledge and improvement science, as these have only taught in the medical undergraduate curriculum in the recent decade in Hong Kong [ 27 ].
The ‘violation’ root causes likely demonstrates the misconception to the term ‘violation’ amongst RCA panels in our review. From a human factors perspective, ‘violations’ are deviations from safe operating practices, procedures, standards, or rules, and have to be deliberately performed by the staff [ 26 ]. The Review Team noticed many RCA reports concluded that the staff had violated the policy or guideline, which was contributed by the causal factors of staff ‘having forgotten to perform a checking step’ or ‘not being aware of the situation’. There was no further investigation on the reasons for ‘violation’ nor was evidence of the staff’s intention to deliberately violate the rules provided. This observation is of particular importance as such misconception in ‘violation’ might have led to an unfair judgement being made to the involved staff. It is important that all RCA panel members buy into the purpose and principles behind an RCA as an incident investigation method, and to identify what is wrong at the system level and promote learning and sharing [ 28 ]. Tools like the Culpability Decision Tree or its recently adapted version, Just Culture Guide by the UK National Health Service, would be helpful in facilitating RCA panels to differentiate violations from other factors causing the staff not to follow the policy or guidelines and bringing a non-blame culture to the organisation [ 29 , 30 ].
At the HA public hospitals, ‘5 whys’ and fishbone diagram are the commonest tools used to identify root causes. Though easy to use, both techniques have their drawbacks and RCA panel members have to use these techniques with caution [ 31 , 32 ]. Other incident investigation and human factors tools and techniques including fault tree analysis, cognitive walkthrough, task analysis, heuristic evaluation techniques and interview question bank, are very useful to facilitate investigation on the evaluation of workflow, equipment and user interface and support data analysis and should be considered in the investigation process [ 12 , 33 , 34 , 35 , 36 , 37 , 38 ]. Currently, the HA RCA report template does not provide any of the above tools for the RCA panel to make reference. In many studies and organisations’ incident management guide, human factors considerations are key components in conducting a robust RCA while quality improvement expertise is vital to effective implementation and process monitoring of action plans. It is advised that RCA members should be formally trained in human factors to support incident investigation and identification of system issues, make use of different tools and techniques to facilitate the investigation and analysis, and understand improvement science to implement action plans effectively [ 10 , 12 , 14 , 16 , 28 , 37 , 38 , 39 , 40 ].
The study results showed that most of the recommendations were weak. Observations of high proportion of weak RCA recommendations have been reported in other studies using similar methodologies [ 10 , 14 , 15 ]. The Review Team noticed that in most RCAs, when a root cause of staff behavioural factor was identified, the corresponding recommendations would generally be to share the incident in department meetings, re-educate the involved staff or enhance their awareness through one-off training. These are weak recommendations as they attempt to change the human behaviour but do not treat the underlying ‘why’ problems [ 10 , 16 ]. The Review Team also noticed some recommendations were not clearly linked to the root causes. For example, in an incident of wrong dose of Gentamicin administration, one of the root causes was the unclear content of verbal communication among nurse, dispenser and pharmacist. The report did not mention how ‘unclear’ the communication was while the corresponding recommendations were to share the incident in a training forum and in the nurses’ meeting. The Review Team believed that the RCA panel in this incident should further elaborate how and why the communication had broken down and a specific enhancement in that particular communication process between the staff would be a more appropriate recommendation rather than solely sharing the incident with staff. Indeed, sharing of incidents and their findings is in the regular incident management process and should not be a specific recommendation [ 37 , 38 ]. The recommendations written in RCA reports should be actions inducing systems changes [ 8 ]. If training is identified as a recommendation at last by the RCA panel like the example above, the training should explain the risks and consequences of not communicating effectively with other staff during a procedure, and teach the necessary knowledge and skills required to address this [ 41 ].
Other system factor root causes were also found to propose weak recommendations in our review. Studies suggested that the tendency of RCA panels to propose weak recommendations is generally caused by the lack of understanding in RCA, limited knowledge in the hierarchy of controls and human factors [ 10 , 14 , 16 , 28 ]. The RCA panels might perceive the investigation to be restricted to within the department such that organisational issues at a broader level were ignored for discussion. Within such confines, the choices of recommendations implementable solely at the departmental level become limited and additional staff training or putting more reminders has been the prominent actions arising from RCAs [ 19 ]. Stronger recommendations are also known to be costly and require more attention and monitoring to complete [ 37 , 38 , 42 ]. Though the RCA Panels are nominated directly by Hospital Chief Executives in the HA, political considerations for driving organisational change are often required for stronger recommendations, and those affecting fundamental systems of the whole HA may be difficult to ignore. For example, all hospitals and institutes in the HA use the same electronic clinical management system for patient documentation and management. When an RCA panel has identified a loophole in the electronic clinical management system, instead of directly asking the Information Technology Department to make the corrective actions, the proposed actions have to go through a series of processes including a number of platforms at hospital and corporate levels for stakeholder consultation, seek approval from different hospital and organisational committees, and lastly conduct a series of feasibility tests by software technicians. This process usually takes months if not years to complete. During such processes, hospital staff may question the RCA panel’s capability as they believe that the panel has not identified the appropriate root causes, and some observers may still assign blame to the involved staff as this is more visible [ 16 , 19 ].
In addition, staff may blame the RCA panel for introducing new or perceived unrealistic changes at the organisational level for one single adverse event which they believe to be contributed to by a staff’s mistake [ 17 ]. The organisation might also have difficulty in assessing the vulnerability of the system by one single event [ 16 ]. The RCAs investigating individual, similar incidents during this review were found to produce inconsistent recommendations. An example would be in incidents where known drugs that cause allergic reactions to patients were prescribed and administered. The causes were found to be due to the input of the allergen in the “free text” section of the electronic medication management system, and thus could not automatically check cross-sensitivity of the drugs to alert staff. However, in some reports the recommendations were to provide training or reinforce staff compliance in checking allergy histories, while some reports suggested converting the free text allergy entries into structured entries to enable drug allergy checking by the electronic system. These inconsistent recommendations may be a product of multiple RCAs being conducted for individual events that have similar causes rather than being collectively reviewed and identifying underlying themes. Such variations in recommendations could affect staff’s impression about the quality of RCA as a whole.
These anticipated difficulties and conflicts in proposing system modifying recommendations may encourage the shift to weaker recommendations to avoid the RCA panel being held responsible and taking up the role in the complicated consultation process [ 19 ]. Recommendations like training and education or ‘to explore the feasibility of implementing an action plan or revising the practice’ have become common, yet are considered weak as the feasibility in implementing the actions are not yet certain or concrete. Even though stronger recommendations leading to some system changes have been suggested, they are usually limited to the departmental level and do not reach the whole organisation. Similar incidents therefore still recur in the same facility.
The results provided insights to the Review Team in proposing suggestions to enhance RCA quality in public hospitals and other healthcare organisations. First, regular training for RCA panel members must be conducted, and systems thinking and human factors should be an essential component of the training. The correct concept of ‘violations’, or in general the human error model must be understood, and available RCA tools should be promoted and panelists trained in their application [ 43 ]. References of investigation tools can also be added to the existing HA RCA report template to facilitate ease of access and serves as a prompt for use. Promotion of no-blame culture to all hospital staff must also be carried out to encourage staff in focusing on system issues instead of blaming individuals [ 16 ]. Skills in writing RCA reports have to be developed with practice so that the root causes and recommendations can be written more specifically and enable a new reader to immediately understand the issues and solutions [ 18 ].
Second, members with human factors expertise should be invited to join RCA panels as it may help shift any focus on blaming individuals to identifying systems and design flaws. This expertise can also support the design of patient safety initiatives [ 10 , 16 , 43 ]. Inviting staff who understand the involved workflows can also help the RCA panel quickly understand the detailed nuances of the situation [ 12 ]. Training a core group of staff specialized in incident investigations within the organisation would be a possible solution to solve the RCA expertise and sustainability problems. This core group of “specialists” can retain their knowledge and skills in RCA and they would have more opportunities to participate in an RCA [ 18 ]. From a broader perspective, an independent institution as a third party, with positioned similarly to the National Aeronautics and Space Administration of the US or the Healthcare Safety Investigation Branch of the UK, [ 44 , 45 ] in the territory could be developed and hospitals can draw on expertise from this institution to support incident investigations as an independent party. The advantages of these independent institutions include minimising internal conflict, promoting unbiasedness, accuracy and credibility of RCA findings, and separate incident investigations and learning from violation disciplinary actions [ 46 ].
Third, the HA must promote a safety culture to all staff, which includes understanding the goals of a RCA, and reduce the barriers of RCA panels in proposing stronger recommendations focusing on organisational changes [ 42 ]. Fourth, the HA should consider aggregating analysis of incidents to counter the inconsistencies that will inevitably arise from multiple RCAs investigating similar issues [ 6 , 10 , 16 , 37 , 38 ]. By implementing these suggestions, the Review Team believes that incident investigations and improvements arising from them will become more effective in the organisation. The root causes will be more focused on system defects and a higher proportion of strong or medium recommendations would be anticipated. Last but not least, Australia has reviewed its SE list in 2017 and launched a second version in December 2018 [ 47 , 48 ]. With the current SE and SUE lists being implemented in Hong Kong for about a decade, the list should be reviewed to ensure it aligns with the goal of effectively monitoring and preventing serious adverse patient harm events.
This study has two limitations. First, the study only reviewed the strengths of recommendations but not the work progresses, feasibilities and outcomes of the recommendations. Due to the boundary of action hierarchy in categorizing recommendations of ‘additional study / analysis’ as weak, the Review Team believed that there will be more strong or medium actions taken when the recommendations are studied to be feasible. Though the HAHO follow up on the work progress of RCA recommendations every 6 months, the outcomes are not evaluated. The Review Team believes that incorporating measures of success in RCA Reports would be worthwhile to determine if the recommendations are effective. Second, this study is the first study in the HA to review RCA effectiveness. There was no other local data available for benchmarking. If improvements like conducting RCA training and improving the human factors expertise of RCA panel members are implemented, a follow-up study would be beneficial.
This study provided insights to the HA about the effectiveness of RCA recommendations in the organisation. The results showed a high proportion of root causes were staff behavioural factors and most of the recommendations were weak. The reasons behind were the lack of training to RCA panel members especially in systems thinking and human factors knowledge, skills and tools, the lack of members with human factors expertise and staff who understand the clinical workflow in the RCA panel composition, and the complicated process in carrying out large scale improvements across the organisation. The Review Team suggested conducting regular RCA training, implementing easy-to-use RCA tools, inviting members with human factors expertise to the RCA panel, promoting a safety culture to staff in all public hospitals and aggregating analysis of incidents, to be possible actions adopted by the organisation’s management. A follow-up study could be considered after conducting the improvement actions. The HA should also consider reviewing the SE and SUE list to ensure serious adverse patient harm events are effectively monitored and prevented.
Availability of data and materials
The data that support the findings of this study are available from the Hong Kong Hospital Authority but restrictions apply to the availability of these data, which were used only for the current study, and so are not publicly available. Data are however available from the authors upon reasonable request and with permission of the Hospital Authority.
Abbreviations
Hospital Authority
Hospital Authority Head Office
Human Factors Analysis Classification System
National Patient Safety Agency
Root Cause Analysis
Sentinel Event
Serious Untoward Event
United Kingdom
World Health Organisation
Institute of Medicine. To err is human: building a safer Health system. Washington, DC: National Academy Press; 1999.
Google Scholar
Altman DE, Clancy C, Blendon RJ. Improving patient safety – five years after the IOM report. N Engl J Med. 2004;351:2041–3.
Article CAS PubMed Google Scholar
Pronovost PJ, Cleeman JI, Wright D, et al. Fifteen years after to err is human: a success story to learn from. BMJ Q&S. 2016;25:396–9.
Stelfox HT, Palmisani S, Scurlock C, et al. The “to err is human” report and the patient safety literature. Qual Saf Health Care. 2006;15:174–8.
Article CAS PubMed PubMed Central Google Scholar
Agency for Healthcare Research and Quality. Root Cause Analysis. Rockville: Patient Safety Network; 2019. [updated 2019; cited 2020 Jan 21]. Available from https://psnet.ahrq.gov/primers/primer/10/root-cause-analysis .
Hooker AB, Etman A, Westra M, et al. Aggregate analysis of sentinel events as a strategic tool in safety management can contribute to the improvement of healthcare safety. Int J Qual Health Care. 2019;31:110–6.
Article PubMed Google Scholar
Nicolini D, Waring J, Mengis J. Policy and practice in the use of root cause analysis to investigate clinical adverse events: mind the gap. Soc Sci Med. 2011;73:217–25.
Charles R, Hood B, Derosier JM, et al. How to perform a root cause analysis for workup and future prevention of medical errors: a review. Patient Safe Surg. 2016;10:20.
Cerniglia-Lowensen J. Learning from mistakes and near mistakes: using root cause analysis as a risk management tool. J Radiol Nurs. 2015;34:4–7.
Article Google Scholar
Hibbert PD, Thomas MJW, Deakin A, et al. Are root cause analyses recommendations effective and sustainable? An observational study. Int J Qual Health Care. 2018;30:124–31.
National Health Services. Root cause analysis using five whys. London: National Health Services Improvement; 2018. [cited 2020 Jan 21]. Available from https://improvement.nhs.uk/resources/root-cause-analysis-using-five-whys/ .
National Patient Safety Foundation. RCA 2 : improving root cause analyses and actions to prevent harm. Boston: National Patient Safety Foundation; 2016.
Diller T, Helmrich G, Dunning S, et al. The human factors analysis classification system (HFACS) applied to Health care. Am J Med Qual. 2014;29:181–90.
Kellogg KM, Hettinger Z, Shah M, et al. Our current approach to root cause analysis: is it contributing to our failure to improve patient safety? BMJ Qual Saf. 2017;26:381–7.
PubMed Google Scholar
Taitz J, Genn K, Brooks V, et al. System-wide learning from root cause analysis: a report from the New South Wales root cause analysis review committee. Qual Saf Health Care. 2010;19:e63.
Peerally MF, Carr S, Waring J, et al. The problem with root cause analysis. BMJ Qual Saf. 2017;26:417–22.
Wu AW, Lipshutz AK, Pronovost PJ. Effectiveness and efficiency of root cause analysis in medicine. JAMA. 2008;299:685–7.
Bowie P, Skinner J, de Wet C. Training health care professionals in root cause analysis: a cross-sectional study of post-training experiences, benefits and attitude. BMC Health Serv Res. 2013;13:50.
Nicolini D, Waring J, Mengis J. The challenges of undertaking root cause analysis in health care: a qualitative study. J Health Serv Res Policy. 2011;16(Suppl 1):34–41.
Hospital Authority. HA Sentinel & Serious Untoward Events Annual Report (Oct 2018 – Sep 2019). Hong Kong: Patient Safety and Risk Management Department; 2020.
Australian Institute of Health and Welfare and the Australian Commission on Safety and Quality in Health Care. Sentinel events in Australian public hospitals 2004–05. Canberra: Australian Institute of Health and Welfare; 2007.
Hospital Authority. HA Sentinel & Serious Untoward Events Annual Report (Oct 2009 – Sep 2010). Hong Kong: Patient Safety and Risk Management Department; 2011.
National Health Service England Patient Safety Domain. Serious Incident Framework. Supporting Learning to Prevent Recurrence. London: National Health Service England; 2015.
World Health Organization, WHO Patient Safety. Conceptual Framework for the International Classification for Patient Safety. Geneva: World Health Organization; 2009.
McHugh ML. Interrater reliability: the kappa statistic. Biochem Med (Zagreb). 2012;22:276–82.
Reason J. Understanding adverse events: human factors. Qual Health Care. 1995;4:80–9.
Leung GKK, Patil NG. Patient safety in the undergraduate curriculum: medical students' perception. Hong Kong Med J. 2010;16:101–5.
CAS PubMed Google Scholar
National Health Service Improvement. The future of NHS patient safety investigations. London: National Patient Safety Agency; 2018.
National Health Service England and National Health Service Improvement. A just culture guide. London: National Health Service England and National Health Service Improvement; 2018.
Reason J. Managing the risks of organizational accidents. Hampshire: Ashgate Publishing Limited; 1997.
Card AJ. The problem with ‘5 whys’. BMJ Qual Saf. 2017;26:671–7.
UKEssays. Causes and Effect Diagrams in Quality Management. 2018 [cited 2020 Jan 21]. Available from https://www.ukessays.com/essays/engineering/cause-effect-diagram.php?vref=1 .
Abecassis ZA, McElroy LM, Patel RM, et al. Applying fault tree analysis to the prevention of wrong site surgery. J Surg Res. 2015;193:88–94.
Reason J. Combating omission errors through task analysis and good reminders. Qual Saf Health Care. 2002;11:40–4.
Zhang J, Johnson TR, Patel VL, et al. Using usability heuristics to evaluate patient safety of medical devices. J Biomed Info. 2003;36:23–30.
Fidler R, Bond R, Finlay D, et al. Human factors approach to evaluate the user interface of physiologic monitoring. J Electrocardiol. 2015;48:982–7.
Health Q. Best practice guide to clinical incident management. State of Queensland: Queensland Health; 2014.
Parties IAC. Canadian incident analysis framework. Edmonton: Canada Patient Safety Institute; 2012.
Chartered Institute of Ergonomics and Human Factors. White paper on human factors for Health and social care. Birmingham: Chartered Institute of Ergonomics and Human Factors; 2018.
Trbovich P, Shojania KG. Root-cause analysis: swatting at mosquitoes versus draining the swamp. BMJ Qual Saf. 2017;26:350–3.
Boholm A. Lessons of success and failure: practicing risk communication at government agencies. Saf Sci. 2019;118:158–67.
Calcia M. Why adverse incidents are an opportunity for learning. BMJ. 2016;355:i5069.
Reason J. Human error: models and management. BMJ. 2000;320:768–70.
Aviation Safety Reporting System. Program Briefing [Internet]. California: National Aeronautics and Space Administration; [cited 2020 Jan 27]. Available from https://asrs.arc.nasa.gov/overview/summary.html .
Healthcare Safety Investigation Branch. About us. London: Healthcare Safety Investigation Branch; [cited 2020 Jan 27]. Available from https://www.hsib.org.uk/ .
Dempsey PS. Independence of aviation safety investigation authorities: keeping the foxes from the henhouse. J Air L & Com. 2010;75:223–84.
Australian Commission on Safety and Quality in Health Care. Australian sentinel events list [Internet]. Sydney: Australian Commission on Safety and Quality in Health Care; [cited 2020 Jan 27] Available from https://www.safetyandquality.gov.au/our-work/indicators/australian-sentinel-events-list .
Victorian Government. Victorian sentinel event guide. Melbourne: Victorian Government; 2019.
Download references
Acknowledgements
The authors would like to thank the members of HA RCA Review Workgroup, in particular Dr. Ngai Chuen Sin and Dr. Gladys Kwan, for their support in conducting this study, and Professor Jeffrey Braithwaite and Associate Professor Peter Hibbert for their advice during the final stages of drafting.
This research received no specific grant from any funding agency in the public, commercial or not-for-profit sectors.
Author information
Authors and affiliations.
Root Cause Analysis Review Workgroup, Hospital Authority, Kowloon, Hong Kong
Yick Ting A Kwok, Alastair PY Mah & Katherine MC Pang
Quality and Safety Division, New Territories West Cluster, Hospital Authority, New Territories, Hong Kong
Yick Ting A Kwok
Patient Safety and Risk Management Department, Quality and Safety Division, Head Office, Hospital Authority, Kowloon, Hong Kong
Alastair PY Mah & Katherine MC Pang
School of Medicine, Faculty of Health, Deakin University, Geelong, Australia
Alastair PY Mah
You can also search for this author in PubMed Google Scholar
Contributions
All authors contributed to the design. YK and AM were the primary reviewers. MP was the secondary reviewer. YK and AM were contributors of the manuscript. All authors read and approved the final manuscript.
Corresponding author
Correspondence to Alastair PY Mah .
Ethics declarations
Consent for publication.
Not applicable.
Competing interests
None declared.
Additional information
Publisher’s note.
Springer Nature remains neutral with regard to jurisdictional claims in published maps and institutional affiliations.
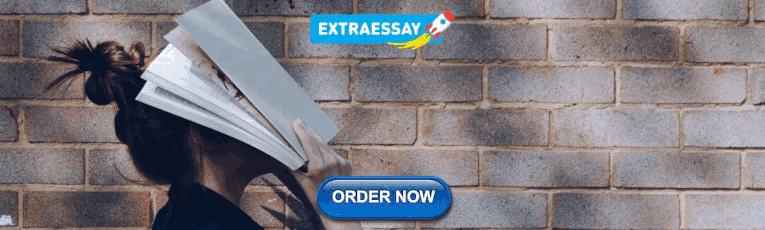
Rights and permissions
Open Access This article is licensed under a Creative Commons Attribution 4.0 International License, which permits use, sharing, adaptation, distribution and reproduction in any medium or format, as long as you give appropriate credit to the original author(s) and the source, provide a link to the Creative Commons licence, and indicate if changes were made. The images or other third party material in this article are included in the article's Creative Commons licence, unless indicated otherwise in a credit line to the material. If material is not included in the article's Creative Commons licence and your intended use is not permitted by statutory regulation or exceeds the permitted use, you will need to obtain permission directly from the copyright holder. To view a copy of this licence, visit http://creativecommons.org/licenses/by/4.0/ . The Creative Commons Public Domain Dedication waiver ( http://creativecommons.org/publicdomain/zero/1.0/ ) applies to the data made available in this article, unless otherwise stated in a credit line to the data.
Reprints and permissions
About this article
Cite this article.
Kwok, Y.A., Mah, A.P. & Pang, K.M. Our first review: an evaluation of effectiveness of root cause analysis recommendations in Hong Kong public hospitals. BMC Health Serv Res 20 , 507 (2020). https://doi.org/10.1186/s12913-020-05356-6
Download citation
Received : 20 February 2020
Accepted : 24 May 2020
Published : 05 June 2020
DOI : https://doi.org/10.1186/s12913-020-05356-6
Share this article
Anyone you share the following link with will be able to read this content:
Sorry, a shareable link is not currently available for this article.
Provided by the Springer Nature SharedIt content-sharing initiative
- Root cause analysis
- Incident investigation
- Patient harm
- Patient safety
- Sentinel events
BMC Health Services Research
ISSN: 1472-6963
- General enquiries: [email protected]
Have a language expert improve your writing
Run a free plagiarism check in 10 minutes, generate accurate citations for free.
- Knowledge Base
- Starting the research process
- What Is Root Cause Analysis? | Definition & Examples
What Is Root Cause Analysis? | Definition & Examples
Published on January 6, 2023 by Tegan George . Revised on November 17, 2023.
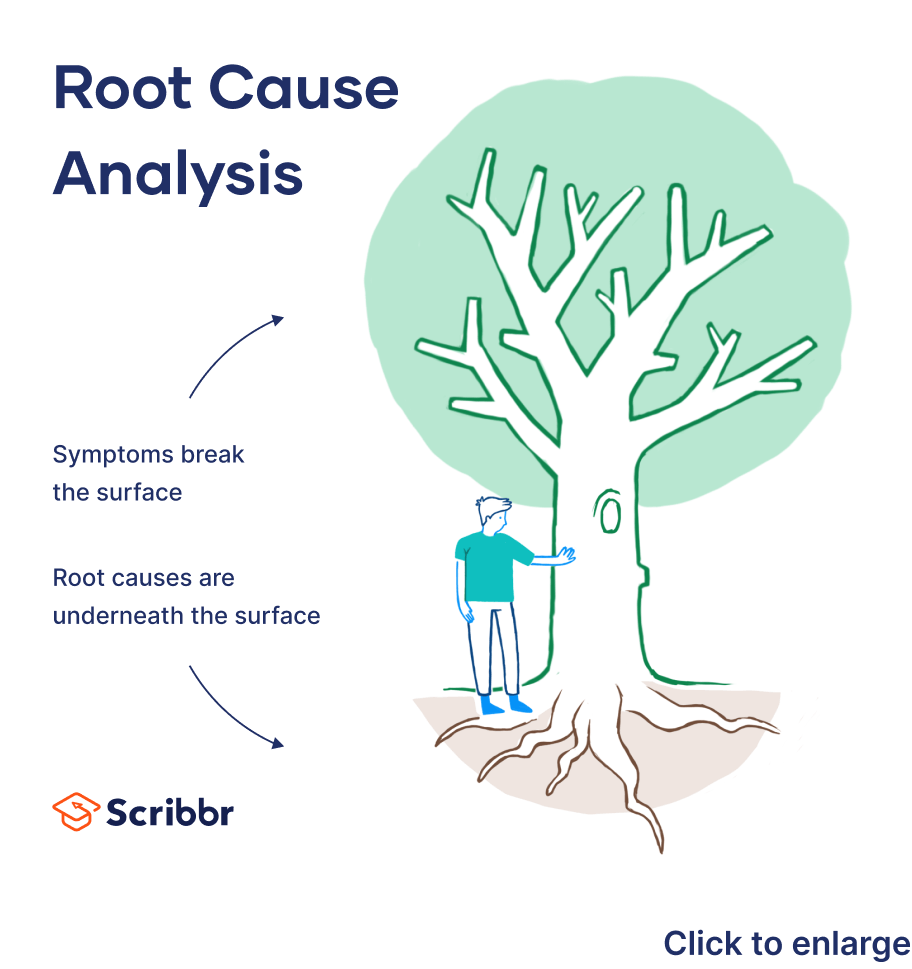
Root cause analysis is a problem-solving approach that uses the analogy of roots and blooms to model cause-and-effect relationships. Rather than focusing on what’s above the surface, root cause analysis troubleshoots solutions to problems by analyzing what is causing them. Note Similarly to exploratory research , it’s important to remember that root cause analysis does not provide solutions to problems. Rather, it’s one method within a larger problem-solving landscape.
Root cause analysis is a form of quality management, often used in organizational management, quality control, and in healthcare fields like nursing. Root cause analysis can be a helpful study tool for students, too, when used for brainstorming or memorization exercises.
Table of contents
Root cause analysis template, the “5 whys” of root cause analysis, advantages and disadvantages of root cause analysis, other interesting articles, frequently asked questions.
It’s easy to draw root cause analysis charts by hand, on a whiteboard or a big piece of paper. Many people use fishbone diagrams as well, or you can download our template below.
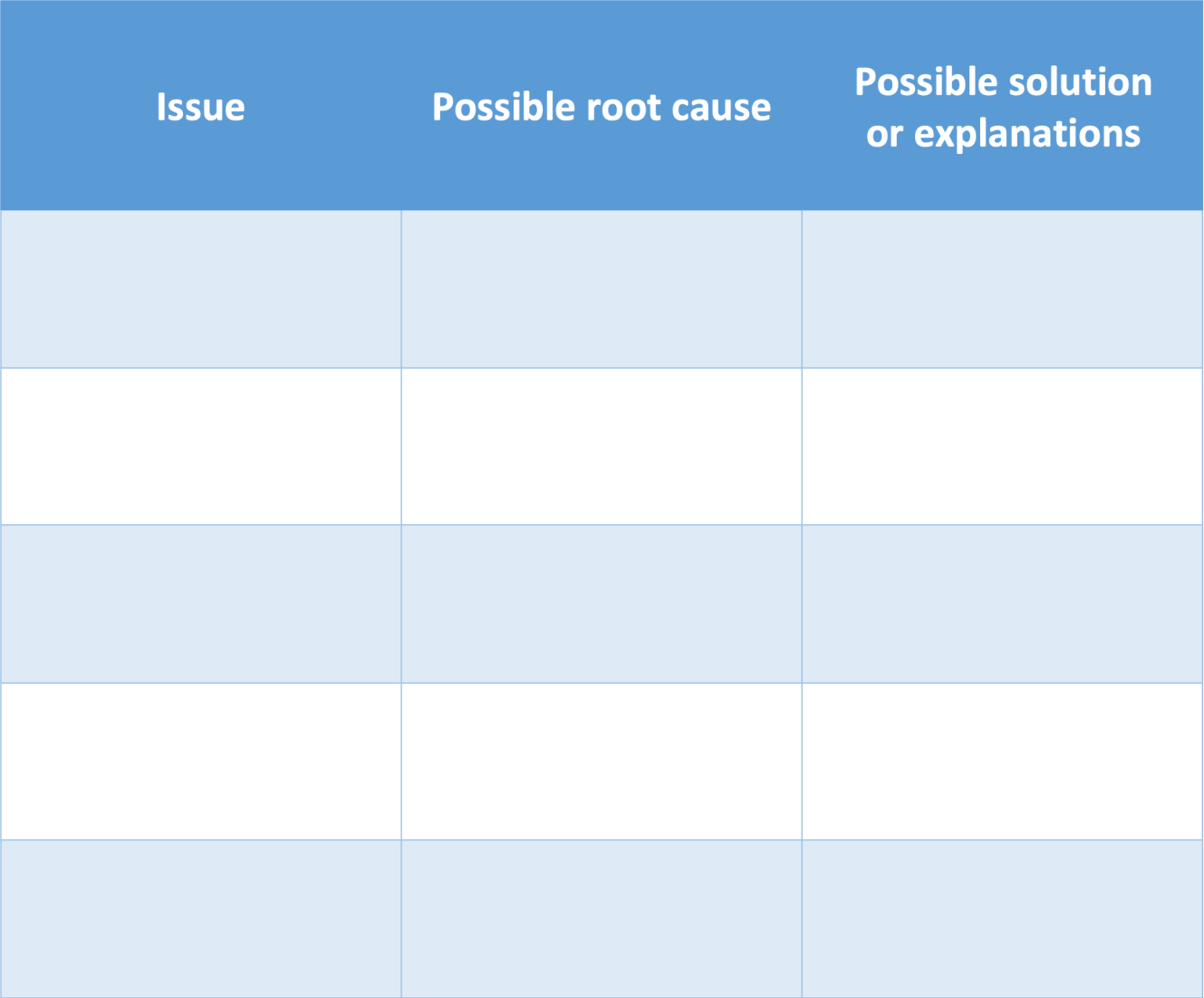
Here's why students love Scribbr's proofreading services
Discover proofreading & editing
One of the most common ways to conduct root cause analysis is using the “5 Whys” method, popular in lean management. The 5 Whys are an interconnected method of analysis: after defining your problem, you ask “why?” and answer as concisely as possible. The first “why” often leads to the second, which leads to the third, etc.
In short, you continue to ask “why” until the answer provided is no longer a contributor to the broader issue, but a possible solution to that issue. In other words, as you strategize, you’ll sense it’s time to stop when a provided answer has the potential to stop the whole problem from occurring, rather than only one aspect of that problem. This often takes 3-5 “whys” but can definitely stretch out for longer.
You can use this template to map out your whys.
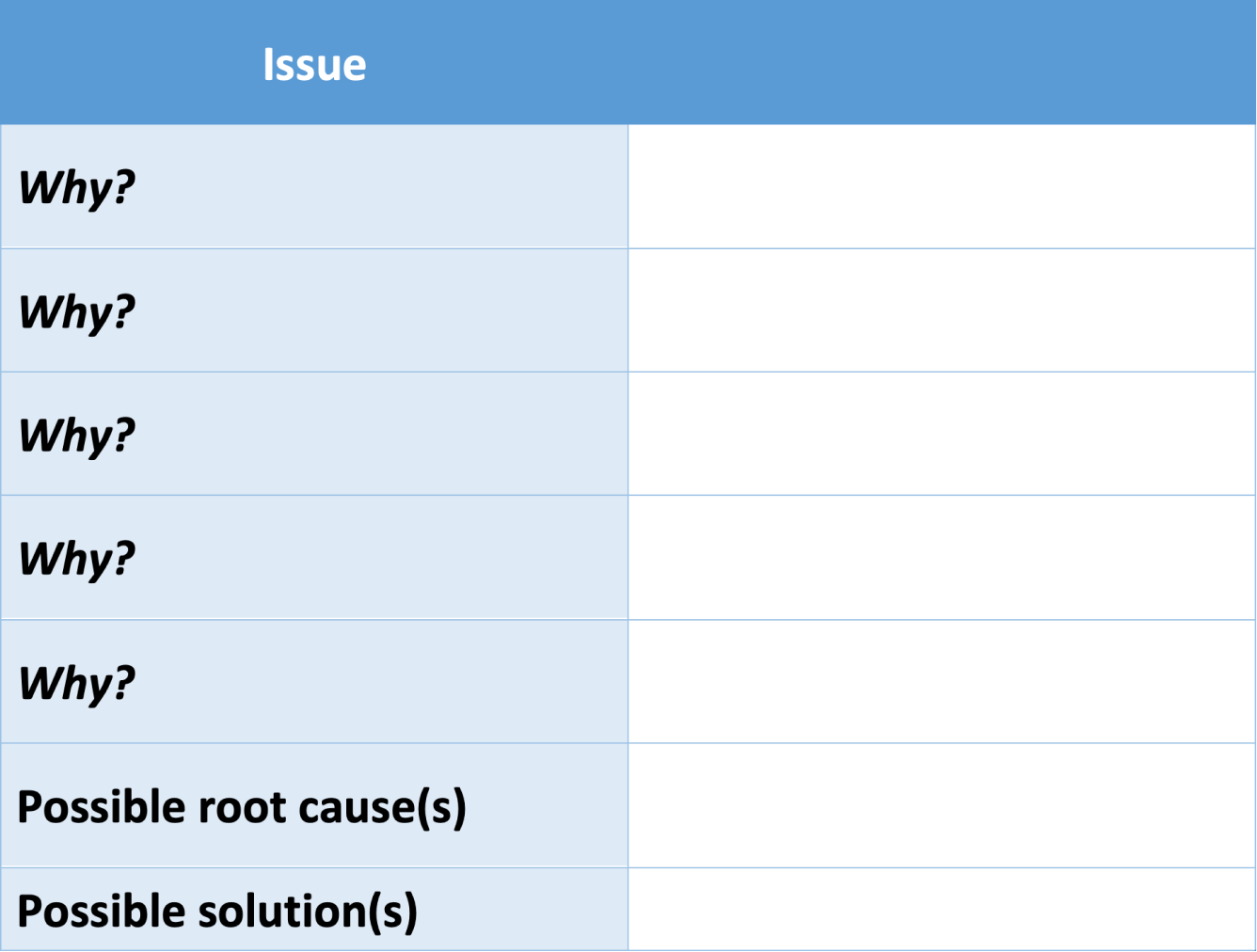
Root cause analysis is a great way to organize your thoughts, but its simplicity leads to a few downsides.
- Great brainstorming tool for individual or group projects.
- Can help identify causal relationships and clarify relationships between variables .
- “5 whys” system can help simplify complex issues and drive possible solutions.
Disadvantages
- Can be overly simplistic, not leaving much room for nuance or variations.
- Path dependence can occur if the wrong question is asked, leading to incorrect conclusions.
- Cannot provide answers, only suggestions, so best used in the exploratory research phase .
If you want to know more about the research process , methodology , research bias , or statistics , make sure to check out some of our other articles with explanations and examples.
Methodology
- Sampling methods
- Simple random sampling
- Stratified sampling
- Cluster sampling
- Likert scales
- Reproducibility
Statistics
- Null hypothesis
- Statistical power
- Probability distribution
- Effect size
- Poisson distribution
Research bias
- Optimism bias
- Cognitive bias
- Implicit bias
- Hawthorne effect
- Anchoring bias
- Explicit bias
There are several common tools used for root cause analysis , the most popular of which include fishbone diagrams , scatterplots, and the “5 whys.”
A fishbone diagram is a method that can be used to conduct root cause analysis.
Cite this Scribbr article
If you want to cite this source, you can copy and paste the citation or click the “Cite this Scribbr article” button to automatically add the citation to our free Citation Generator.
George, T. (2023, November 17). What Is Root Cause Analysis? | Definition & Examples. Scribbr. Retrieved August 5, 2024, from https://www.scribbr.com/research-process/root-cause-analysis/
Is this article helpful?
EXTERNAL PUBLICATIONS
- FABIG -
- Home -
- External Publications -
HSE CRR 325/2001
Root causes analysis: literature review, fabig members: log-in to access all fabig resources log in.
Typically an incident report will place emphasis on developing a description of the consequences rather than causes of the incident, explaining what happened, but not why it happened. It is only by adopting investigation techniques that explicitly identify root causes, ie the reasons why an incident occurred, that organisations may learn from past failures and avoid similar incidents in the future. Root causes analysis is simply a tool designed to help incident investigators determine what, how and most importantly, why an incident occurred.
Based on this literature review it is apparent that there are three key components that need to be applied to ensure effective root causes analysis incident investigation. These are a method of describing and schematically representing the incident sequence and its contributing conditions; a method of identifying the critical events or active failures and conditions in the incident sequence, and based on this identification; a method for systematically investigating the management and organisational factors that allowed the active failures to occur, ie a method for root causes analysis. In selecting or developing a root causes analysis method, the analyst needs to consider whether the method specifically facilitates the identification of safety management and organisational inadequacies and oversights which relate to their own operations. The method needs to identify those factors that exert control over the design, development, maintenance and review of their risk control systems and procedures.
This report and the work it describes were funded by the Health and Safety Executive (HSE) Its contents, including any opinions and/or conclusions expressed, are those of the authors alone and do not necessarily reflect HSE policy.
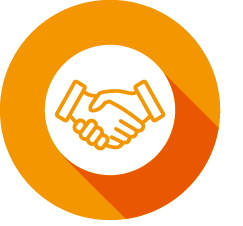
Corporate Membership
Do you have a question to get in touch please, keep up-to-date with the latest fabig news and events.
This resource is only available to FABIG members. Become a member!
Session expired
Your session has expired. Please log in again. LOGIN
Information
- Author Services
Initiatives
You are accessing a machine-readable page. In order to be human-readable, please install an RSS reader.
All articles published by MDPI are made immediately available worldwide under an open access license. No special permission is required to reuse all or part of the article published by MDPI, including figures and tables. For articles published under an open access Creative Common CC BY license, any part of the article may be reused without permission provided that the original article is clearly cited. For more information, please refer to https://www.mdpi.com/openaccess .
Feature papers represent the most advanced research with significant potential for high impact in the field. A Feature Paper should be a substantial original Article that involves several techniques or approaches, provides an outlook for future research directions and describes possible research applications.
Feature papers are submitted upon individual invitation or recommendation by the scientific editors and must receive positive feedback from the reviewers.
Editor’s Choice articles are based on recommendations by the scientific editors of MDPI journals from around the world. Editors select a small number of articles recently published in the journal that they believe will be particularly interesting to readers, or important in the respective research area. The aim is to provide a snapshot of some of the most exciting work published in the various research areas of the journal.
Original Submission Date Received: .
- Active Journals
- Find a Journal
- Proceedings Series
- For Authors
- For Reviewers
- For Editors
- For Librarians
- For Publishers
- For Societies
- For Conference Organizers
- Open Access Policy
- Institutional Open Access Program
- Special Issues Guidelines
- Editorial Process
- Research and Publication Ethics
- Article Processing Charges
- Testimonials
- Preprints.org
- SciProfiles
- Encyclopedia
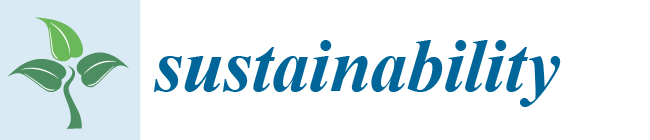
Article Menu
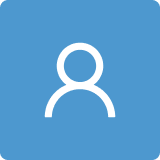
- Subscribe SciFeed
- Recommended Articles
- Google Scholar
- on Google Scholar
- Table of Contents
Find support for a specific problem in the support section of our website.
Please let us know what you think of our products and services.
Visit our dedicated information section to learn more about MDPI.
JSmol Viewer
An influencing factors analysis of road traffic accidents based on the analytic hierarchy process and the minimum discrimination information principle.
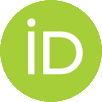
1. Introduction
2. literature review, 2.1. study on road traffic accident influencing factors, 2.1.1. research on human-related factors, 2.1.2. research on vehicle-related factors, 2.1.3. research on road-related factors, 2.1.4. research on environment-related factors, 2.2. influencing factors analysis based on the analytic hierarchy process, 3.1. the subjective data, 3.2. the objective data, 4.1. analytic hierarchy process calculation steps, 4.2. principle of minimum discrimination information, 5. hierarchical model and weight calculation of influencing factors, 5.1. hierarchical model of road traffic accident influencing factors, 5.2. weight calculation of road traffic accident influencing factors, 5.2.1. analytic hierarchy process determines the subjective weight, 5.2.2. data normalization determines the objective weight, 5.2.3. the principle of minimum discrimination information determines the comprehensive weight, 5.2.4. weight, its rank, and weight difference of road traffic accident influencing factors, 6. discussion, 6.1. hierarchical model of influencing factors, 6.2. subjective and objective weight difference, 6.3. causative factors, 6.3.1. first-level causative factors, 6.3.2. second-level causative factors, 6.3.3. third-level causative factors, 7. conclusions, author contributions, institutional review board statement, informed consent statement, data availability statement, conflicts of interest.
- WHO. Global Health Estimates 2019: Deaths by Cause, Age, Sex, by Country and by Region, 2000–2019 ; WHO: Geneva, Switzerland, 2019. [ Google Scholar ]
- WHO. Powered Two-and Three-Wheeler Safety: A Road Safety Manual for Decisionmakers and Practitioners ; WHO: Geneva, Switzerland, 2022. [ Google Scholar ]
- Zhang, H.; Wu, C.; Yan, X.; Qiu, T.Z. The effect of fatigue driving on car following behavior. Transp. Res. Part F 2016 , 43 , 80–89. [ Google Scholar ] [ CrossRef ]
- Chen, Y.; Wang, K.; Lu, J.J. Feature selection for driving style and skill clustering using naturalistic driving data and driving behavior questionnaire. Accid. Anal. Prev. 2023 , 185 , 107022. [ Google Scholar ] [ CrossRef ] [ PubMed ]
- Rodwell, D.; Watson-Brown, N.; Bates, L. Perceptions of novice driver education needs; Development of a scale based on the Goals for driver education using young driver and parent samples. Accid. Anal. Prev. 2023 , 191 , 107190. [ Google Scholar ] [ CrossRef ] [ PubMed ]
- Verhaegen, P. Liability of older drivers in collisions. Ergonomics 1995 , 38 , 499–507. [ Google Scholar ] [ CrossRef ]
- Pitta, L.S.R.; Quintas, J.L.; Trindade, I.O.A.; Belchior, P.; da Silva Duarte Gameiro, K.; Gomes, C.M.; Nóbrega, O.T.; Camargos, E.F. Older drivers are at increased risk of fatal crash involvement: Results of a systematic review and meta-analysis. Arch. Gerontol. Geriatr. 2021 , 95 , 104414. [ Google Scholar ] [ CrossRef ]
- Yu, Z.; Qu, W.; Ge, Y. Trait anger causes risky driving behavior by influencing executive function and hazard cognition. Accid. Anal. Prev. 2022 , 177 , 106824. [ Google Scholar ] [ CrossRef ] [ PubMed ]
- Su, Z.; Woodman, R.; Smyth, J.; Elliott, M. The relationship between aggressive driving and driver performance: A systematic review with meta-analysis. Accid. Anal. Prev. 2023 , 183 , 106972. [ Google Scholar ] [ CrossRef ]
- Abdel-Aty, M.A.; Abdelwahab, H.T. Exploring the relationship between alcohol and the driver characteristics in motor vehicle accidents. Accid. Anal. Prev. 2000 , 32 , 473–482. [ Google Scholar ] [ CrossRef ]
- Escamilla, C.; Bele, M.A.; Picó, A.; Rojo, J.M.; Mateu-Moll, J. A psychological profile of drivers convicted of driving under the influence of alcohol. Transp. Res. Part F 2023 , 95 , 380–390. [ Google Scholar ] [ CrossRef ]
- Strohl, K.P.; Blatt, J.; Council, F.; Georges, K.; Kiley, J.; Kurrus, R.; MacCartt, A.T.; Merritt, S.L.; Pack, A.I.; Rogus, S.; et al. Drowsy Driving and Automobile Crashes: Reports and Recommendations ; DOT HS 1998, 808 707, III-30; National Center on Sleep Disorders Research & National Highway Traffic Safety Administration: Washington, DC, USA, 1998. [ Google Scholar ]
- Watling, C.N.; Home, M. Hazard perception performance and visual scanning behaviours: The effect of sleepiness. Transp. Res. Part F 2022 , 90 , 243–251. [ Google Scholar ] [ CrossRef ]
- Tefft, B.C. Prevalence of motor vehicle crashes involving drowsy drivers, United States, 1999–2008. Accid. Anal. Prev. 2012 , 45 , 180–186. [ Google Scholar ] [ CrossRef ] [ PubMed ]
- Shao, Y.; Shi, X.; Zhang, Y.; Zhang, Y.; Xu, Y.; Chen, W.; Ye, Z. Adaptive forward collision warning system for hazmat truck drivers: Considering differential driving behavior and risk levels. Accid. Anal. Prev. 2023 , 191 , 107221. [ Google Scholar ] [ CrossRef ] [ PubMed ]
- Palk, G.; Freeman, J.; Kee, A.G.; Steinhardt, D.; Davey, J. The prevalence and characteristics of self-reported dangerous driving behaviours among a young cohort. Transp. Res. Part F 2011 , 14 , 147–154. [ Google Scholar ] [ CrossRef ]
- Zhu, T.; Qin, D.; Jia, W. Examining the associations between urban bus drivers’ rule violations and crash frequency using observational data. Accid. Anal. Prev. 2023 , 187 , 107074. [ Google Scholar ] [ CrossRef ] [ PubMed ]
- Ospina-Mateus, H.; Quintana Jiménez, L.; López-Valdés, F.J. Analyzing traffic conflicts and the behavior of motorcyclists at unsignalized three-legged and four-legged intersections in Cartagena, Colombia. Accid. Anal. Prev. 2023 , 191 , 107222. [ Google Scholar ] [ CrossRef ] [ PubMed ]
- Zhang, F.; Ji, Y.; Lv, H.; Ma, X. Analysis of factors influencing delivery e-bikes’ red-light running behavior: A correlated mixed binary logit approach. Accid. Anal. Prev. 2021 , 152 , 105977. [ Google Scholar ] [ CrossRef ] [ PubMed ]
- Oviedo-Trespalacios, O.; Rubie, E.; Haworth, N. Risky business: Comparing the riding behaviours of food delivery and private bicycle riders. Accid. Anal. Prev. 2022 , 177 , 106820. [ Google Scholar ] [ CrossRef ] [ PubMed ]
- Wang, X.; Chen, J.; Quddus, M.; Zhou, W.; Shen, M. Influence of familiarity with traffic regulations on delivery riders’ e-bike crashes and helmet use: Two mediator ordered logit models. Accid. Anal. Prev. 2021 , 159 , 106277. [ Google Scholar ] [ CrossRef ] [ PubMed ]
- Qian, Q.; Shi, J. Comparison of injury severity between E-bikes-related and other two-wheelers-related accidents: Based on an accident dataset. Accid. Anal. Prev. 2023 , 190 , 107189. [ Google Scholar ] [ CrossRef ]
- Jensen, S.U. Pedestrian safety in Denmark. Transp. Res. Rec. 1999 , 1674 , 61–69. [ Google Scholar ] [ CrossRef ]
- Haleem, K.; Alluri, P.; Gan, A. Analyzing pedestrian crash injury severity at signalized and non-signalized locations. Accid. Anal. Prev. 2015 , 81 , 14–23. [ Google Scholar ] [ CrossRef ] [ PubMed ]
- Zeng, Q.; Wang, Q.; Zhang, K.; Wong, S.C.; Xu, P. Analysis of the injury severity of motor vehicle–pedestrian crashes at urban intersections using spatiotemporal logistic regression models. Accid. Anal. Prev. 2023 , 189 , 107119. [ Google Scholar ] [ CrossRef ] [ PubMed ]
- Blower, D.; Green, P.; Matteson, A. Condition of trucks and truck crash involvement: Evidence from the large truck crash causation study. Transp. Res. Rec. J. Transp. Res. Board 2010 , 2194 , 21–28. [ Google Scholar ] [ CrossRef ]
- Schoor, O.V.; Niekerk, J.L.; Grobbelaar, B. Mechanical failures as a contributing cause to motor vehicle accidents—South Africa. Accid. Anal. Prev. 2001 , 33 , 713–721. [ Google Scholar ] [ CrossRef ] [ PubMed ]
- Solah, M.S.; Hamzah, A.; Ariffin, A.H.; Paiman, N.F.; Hamid, I.A.; Wahab, M.A.F.A.; Jawi, Z.M.; Osman, M.R. Private vehicle roadworthiness in Malaysia from the vehicle inspection perspective article history. J. Soc. Automot. Eng. Malays. 2017 , 1 , 262–271. [ Google Scholar ]
- Haq, M.T.; Ampadu, V.-M.K.; Ksaibati, K. An investigation of brake failure related crashes and injury severity on mountainous roadways in Wyoming. J. Saf. Res. 2023 , 84 , 7–17. [ Google Scholar ] [ CrossRef ] [ PubMed ]
- Quimby, A.R. Comparing UK and European drivers on speed and speeding issues: Some results from SARTRE 3 survey. In Behavioural Research in Road Safety: Fifteenth Seminar ; Department for Transport: London, UK, 2005; pp. 49–68. ISBN 1904763618. [ Google Scholar ]
- Varet, F.; Apostolidis, T.; Granié, M.-A. Social value, normative features and gender differences associated with speeding and compliance with speed limits. J. Saf. Res. 2023 , 84 , 182–191. [ Google Scholar ] [ CrossRef ] [ PubMed ]
- Hu, W.; Cicchino, J.B. Effects of a rural speed management pilot program in Bishopville, Maryland, on public opinion and vehicle speeds. J. Saf. Res. 2023 , 85 , 278–286. [ Google Scholar ] [ CrossRef ]
- Wang, Y.; Tu, H.; Sze, N.N.; Li, H.; Ruan, X. A novel traffic conflict risk measure considering the effect of vehicle weight. J. Saf. Res. 2022 , 80 , 1–13. [ Google Scholar ] [ CrossRef ]
- Bunn, T.L.; Liford, M.; Turner, M.; Bush, A. Driver injuries in heavy vs. light and medium truck local crashes, 2010–2019. J. Saf. Res. 2022 , 83 , 26–34. [ Google Scholar ] [ CrossRef ]
- Afghari, A.P.; Vos, J.; Farah, H.; Papadimitriou, E. “I did not see that coming”: A latent variable structural equation model for understanding the effect of road predictability on crashes along horizontal curves. Accid. Anal. Prev. 2023 , 187 , 107075. [ Google Scholar ] [ CrossRef ] [ PubMed ]
- Elvik, R. International transferability of accident modification functions for horizontal curves. Accid. Anal. Prev. 2013 , 59 , 487–496. [ Google Scholar ] [ CrossRef ] [ PubMed ]
- Wen, H.; Ma, Z.; Chen, Z.; Luo, C. Analyzing the impact of curve and slope on multi-vehicle truck crash severity on mountainous freeways. Accid. Anal. Prev. 2023 , 181 , 106951. [ Google Scholar ] [ CrossRef ] [ PubMed ]
- Ma, Y.; Wang, F.; Chen, S.; Xing, G.; Xie, Z.; Wang, F. A dynamic method to predict driving risk on sharp curves using multi-source data. Accid. Anal. Prev. 2023 , 191 , 107228. [ Google Scholar ] [ CrossRef ] [ PubMed ]
- Ma, Z.; Lu, X.; Chien, S.I.-J.; Hu, D. Investigating factors influencing pedestrian injury severity at intersections. Traffic Inj. Prev. 2018 , 19 , 159–164. [ Google Scholar ] [ CrossRef ] [ PubMed ]
- Das, S.; Dutta, A.; Geedipally, S.R. Applying bayesian data mining to measure the effect of vehicular defects on crash severity. J. Transp. Saf. Secur. 2021 , 13 , 605–621. [ Google Scholar ] [ CrossRef ]
- DiLorenzo, T.; Yu, X. Use of ice detection sensors for improving winter road safety. Accid. Anal. Prev. 2023 , 191 , 107197. [ Google Scholar ] [ CrossRef ]
- Abdel-Aty, M.; Devarasetty, P.C.; Pande, A. Safety evaluation of multilane arterials in Florida. Accid. Anal. Prev. 2009 , 41 , 777–788. [ Google Scholar ] [ CrossRef ] [ PubMed ]
- Zhang, Z.; Akinci, B.; Qian, S. Inferring heterogeneous treatment effects of work zones on crashes. Accid. Anal. Prev. 2022 , 177 , 106811. [ Google Scholar ] [ CrossRef ]
- Islam, M.; Hosseini, P.; Jalayer, M. An analysis of single-vehicle truck crashes on rural curved segments accounting for unobserved heterogeneity. J. Saf. Res. 2022 , 80 , 148–159. [ Google Scholar ] [ CrossRef ]
- Uddin, M.; Huynh, N. Injury severity analysis of truck-involved crashes under different weather conditions. Accid. Anal. Prev. 2020 , 141 , 105529. [ Google Scholar ] [ CrossRef ]
- Yasanthi, R.G.N.; Babak Mehran, B.; Alhajyaseen, W.K.M. A reliability-based weather-responsive variable speed limit system to improve the safety of rural highways. Accid. Anal. Prev. 2022 , 177 , 106831. [ Google Scholar ] [ CrossRef ] [ PubMed ]
- Abdel-Atya, M.; Al-Ahad, E.; Huang, H.; Choic, K. A study on crashes related to visibility obstruction due to fog and smoke. Accid. Anal. Prev. 2011 , 43 , 1730–1737. [ Google Scholar ] [ CrossRef ] [ PubMed ]
- Batouli, G.; Guo, M.; Janson, B.; Marshall, W. Analysis of pedestrian-vehicle crash injury severity factors in Colorado 2006–2016. Accid. Anal. Prev. 2020 , 148 , 105782. [ Google Scholar ] [ CrossRef ] [ PubMed ]
- AlGhamdi, A.S. Experimental evaluation of fog warning system. Accid. Anal. Prev. 2007 , 39 , 1065–1072. [ Google Scholar ] [ CrossRef ] [ PubMed ]
- Bee, F. At Least 40 Vehicles Crash in Dense Fog on Highway 198. Available online: http://www.fresnobee.com/news/local/article129797864.html (accessed on 19 July 2017).
- Das, A.; Ali Ghasemzadeh, A.; Ahmed, M.M. Analyzing the effect of fog weather conditions on driver lane-keeping performance using the SHRP2 naturalistic driving study data. J. Saf. Res. 2019 , 68 , 71–80. [ Google Scholar ] [ CrossRef ]
- Saaty, T.L. A scaling method for priorities in hierarchical structures. J. Math. Psychol. 1977 , 15 , 234–281. [ Google Scholar ] [ CrossRef ]
- Huang, Y.; Bian, L. A Bayesian network and analytic hierarchy process based personalized recommendations for tourist attractions over the Internet. Expert Syst. Appl. 2009 , 36 , 933–943. [ Google Scholar ] [ CrossRef ]
- Ma, H.; Li, S.; Chan, C.-S. Analytic Hierarchy Process (AHP)-based assessment of the value of non- World Heritage Tulou: A case study of Pinghe County, Fujian Province. Tour. Manag. Perspect. 2018 , 26 , 67–77. [ Google Scholar ] [ CrossRef ]
- Badea, A.; Prostean, G.; Goncalves, G.; Allaoui, H. Assessing risk factors in collaborative supply chain with the analytic hierarchy process (AHP). Procedia-Soc. Behav. Sci. 2014 , 124 , 114–123. [ Google Scholar ] [ CrossRef ]
- Ignaccolo, M.; Inturri, G.; García-Melón, M.; Giuffrida, N.; Le Pira, M.; Torrisi, V. Combining Analytic Hierarchy Process (AHP) with role-playing games for stakeholder engagement in complex transport decisions. Transp. Res. Procedia 2017 , 27 , 500–507. [ Google Scholar ] [ CrossRef ]
- Ha, J.S.; Seong, P.H. A method for risk-informed safety significance categorization using the analytic hierarchy process and bayesian belief networks. Reliab. Eng. Syst. Saf. 2004 , 83 , 1–15. [ Google Scholar ] [ CrossRef ]
- Abrahamsen, E.B.; Milazzo, M.F.; Selvik, J.T.; Asche, F.; Abrahamsen, H.B. Prioritising investments in safety measures in the chemical industry by using the Analytic Hierarchy Process. Reliab. Eng. Syst. Saf. 2020 , 198 , 106811. [ Google Scholar ] [ CrossRef ]
- Guo, X.; Kapucu, M. Assessing social vulnerability to earthquake disaster using rough analytic hierarchy process method: A case study of Hanzhong City, China. Saf. Sci. 2020 , 125 , 104625. [ Google Scholar ] [ CrossRef ]
- Zhao, D.; Wang, Z.-R.; Song, Z.-Y.; Guo, P.-K.; Cao, X.-Y. Assessment of domino effects in the coal gasification process using Fuzzy Analytic Hierarchy Process and Bayesian Network. Saf. Sci. 2020 , 130 , 104888. [ Google Scholar ] [ CrossRef ]
- Kumar, A.; Sinha, P.K. Human error control in railways. Jordan J. Mech. Ind. Eng. 2008 , 2 , 183–190. [ Google Scholar ]
- Larue, G.S.; Naweed, A.; Rodwell, D. The road user, the pedestrian, and me: Investigating the interactions, errors and escalating risks of users of fully protected level crossings. Saf. Sci. 2018 , 110 , 80–88. [ Google Scholar ] [ CrossRef ]
- Sangiorgio, V.; Mangini, A.M.; Precchiazzi, I. A new index to evaluate the safety performance level of railway transportation systems. Saf. Sci. 2020 , 131 , 104921. [ Google Scholar ] [ CrossRef ]
- Paltrinieri, N.; Landucci, G.; Molag, M.; Bonvicini, S.; Spadoni, G.; Cozzani, V. Risk reduction in road and rail LPG transportation by passive fire protection. J. Hazard Mater. 2009 , 167 , 332–344. [ Google Scholar ] [ CrossRef ] [ PubMed ]
- Mearns, K.; Yule, S. The role of national culture in determining safety performance: Challenges for the global oil and gas industry. Saf. Sci. 2009 , 47 , 777–785. [ Google Scholar ] [ CrossRef ]
- Ghaleh, S.; Omidvari, M.; Nassiri, P.; Momeni, M.; Lavasani, S.M.M. Pattern of safety risk assessment in road fleet transportation of hazardous materials (oil materials). Saf. Sci. 2019 , 116 , 1–12. [ Google Scholar ] [ CrossRef ]
- Karahalios, H. The contribution of risk management in ship management: The case of ship collision. Saf. Sci. 2014 , 63 , 104–114. [ Google Scholar ] [ CrossRef ]
- Yoo, K.E.; Choi, Y.C. Analytic hierarchy process approach for identifying relative importance of factors to improve passenger security checks at airports. J. Air Transp. Manag. 2006 , 12 , 135–142. [ Google Scholar ] [ CrossRef ]
- Manca, D.; Brambilla, S. A methodology based on the Analytic Hierarchy Process for the quantitative assessment of emergency preparedness and response in road tunnels. Transp. Policy 2011 , 18 , 657–664. [ Google Scholar ] [ CrossRef ]
- Ahlström, C.; Raimondas Zemblys, R.; Finér, S.; Kircher, K. Alcohol impairs driver attention and prevents compensatory strategies. Accid. Anal. Prev. 2023 , 184 , 107010. [ Google Scholar ] [ CrossRef ]
- Schlueter, D.A.; Austerschmidt, K.L.; Schulz, P.; Beblo, T.; Driessen, M.; Kreisel, S.; Toepper, M. Overestimation of on-road driving performance is associated with reduced driving safety in older drivers. Accid. Anal. Prev. 2023 , 187 , 107086. [ Google Scholar ] [ CrossRef ]
- Hassan, A.; Lee, C.; Cramer, K.; Lafreniere, K. Analysis of driver characteristics, self-reported psychology measures and driving performance measures associated with aggressive driving. Accid. Anal. Prev. 2023 , 188 , 107097. [ Google Scholar ] [ CrossRef ]
- National Bureau of Statistics of China. Available online: https://www.stats.gov.cn/sj/ndsj/2023/indexch.htm (accessed on 13 June 2024).
- Saaty, T.L. How to make a decision: The analytic hierarchy process. Eur. J. Oper. Res. 1990 , 48 , 9–26. [ Google Scholar ] [ CrossRef ]
- Saaty, T.L. Multicriteria Decision Making: The Analytic Hierarchy Process ; McGraw-Hill, RSW Publishing: Pittsburgh, PA, USA, 1980. [ Google Scholar ]
- Chang, L.; Chen, W. Data mining of tree-based models to analyze freeway accident frequency. J. Saf. Res. 2005 , 36 , 365–375. [ Google Scholar ] [ CrossRef ]
- Naik, B.; Tung, L.W.; Zhao, S.; Khattak, A.J. Weather impacts on single-vehicle truck crash injury severity. J. Saf. Res. 2016 , 58 , 57–65. [ Google Scholar ] [ CrossRef ]
- Knapp, K.; Kroeger, D.; Giese, K. Mobility and Safety Impacts of Winter Storm Events in a Freeway Environment ; Center for Transportation Research and Education, Iowa State University: Ames, IA, USA, 2000. [ Google Scholar ]
- Claret, P.L.; del Castillo, J.D.; Moleón, J.J.; Cavanillas, A.B.; Martín, M.G.; Vargas, R.G. Age and sex differences in the risk of causing vehicle collisions in Spain, 1990 to 1999. Accid. Anal. Prev. 2003 , 35 , 261–272. [ Google Scholar ] [ CrossRef ] [ PubMed ]
- Md Isa, M.H.; Abu Bakar, S.; Hamzah, A.; Ariffin, A.H.; Mohd Nazri, N.N.; Mohamad Hashim, M.S. Investigating motorcycle turn signal behaviors in mixed- traffic environments. In Recent Trends in Manufacturing and Materials towards Industry 4.0 ; Springer: Singapore, 2021; pp. 711–722. [ Google Scholar ]
- Clarke, D.D.; Ward, P.; Bartle, C.; Truman, W. Killer crashes: Fatal road traffic accidents in the UK. Accid. Anal. Prev. 2010 , 42 , 764–770. [ Google Scholar ] [ CrossRef ] [ PubMed ]
- Park, J.; Abdel-Aty, M.; Wang, J.H. Time series trends of the safety effects of pavement resurfacing. Accid. Anal. Prev. 2017 , 101 , 78–86. [ Google Scholar ] [ CrossRef ] [ PubMed ]
- Zhai, X.; Huang, H.; Sze, N.N.; Song, Z.; Hon, K.K. Diagnostic analysis of the effects of weather condition on pedestrian crash severity. Accid. Anal. Prev. 2019 , 122 , 318–324. [ Google Scholar ] [ CrossRef ]
- Retting, R.A.; Weinstein, H.B.; Solomon, M.G. Analysis of motor-vehicle crashes at stop signs in four US cities. J. Saf. Res. 2003 , 34 , 485–489. [ Google Scholar ] [ CrossRef ] [ PubMed ]
- Xie, X.; Nikitas, A.; Liu, H. A study of fatal pedestrian crashes at rural low-volume road intersections in southwest China. Traffic. Inj. Prev. 2018 , 19 , 298–304. [ Google Scholar ] [ CrossRef ]
- Zhang, Q.; Xu, L.; Yan, Y.; Li, G.; Qiao, D.; Tian, J. Distracted driving behavior in patients with insomnia. Accid. Anal. Prev. 2023 , 183 , 106971. [ Google Scholar ] [ CrossRef ]
Attribute | Category | Quantity | Attribute | Category | Quantity |
---|---|---|---|---|---|
Motor vehicle drivers in bad condition | Fatigue driving | 7 | Driving experience of motor vehicle drivers | ≤5 years | 16 |
Drunk driving | 9 | 6~8 years | 20 | ||
Emotional driving | 10 | 9~14 years | 23 | ||
Motor vehicle drivers’ misconduct | Driving without a license | 6 | 15~19 years | 13 | |
Illegal U-turn | 5 | >20 years | 15 | ||
Illegal overtaking | 8 | Age of motor vehicle drivers | ≤25 years old | 12 | |
Illegal lane change | 16 | 26~30 years old | 14 | ||
Traffic signal violation | 10 | 31~40 years old | 29 | ||
Failure to maintain a safe distance | 10 | 41~50 years old | 23 | ||
Not yielding to pedestrians at zebra crossings | 9 | 50~60 years old | 6 | ||
Untimely braking | 37 | >60 years old | 5 | ||
Non-motor vehicle driver factors | Swerve | 10 | Pedestrian and passenger factors | Illegal crossing lanes | 5 |
Crossing the road | 12 | Illegally crossing the traffic barrier | 7 | ||
No safety helmet | 22 | Traffic signal violation | 4 | ||
Occupying motor vehicle lanes | 4 | Not observing traffic environment | 11 |
Attribute | Category | Quantity | Attribute | Category | Quantity |
---|---|---|---|---|---|
Vehicle safety condition | Tire burst | 4 | Vehicle safety hazard | Overloaded | 5 |
Steering failure | 6 | Over speed | 18 | ||
Brake failure | 13 | Large truck | 22 |
Attribute | Category | Quantity | Attribute | Category | Quantity |
---|---|---|---|---|---|
Pavement condition | Dry | 61 | Road section | Flat straight section | 55 |
Wet and slippery | 38 | Uphill and downhill section | 10 | ||
Construction situation | Road construction | 14 | Sharp turn section | 8 | |
No road construction | 87 | Intersection | 28 | ||
Traffic sign | There are traffic signals or lines | 79 | |||
Lack of traffic signals | 22 |
Attribute | Category | Quantity | Attribute | Category | Quantity |
---|---|---|---|---|---|
Weather condition | Clear Weather | 26 | Sight condition | Day | 75 |
Overcast sky | 33 | Lighting at night | 11 | ||
Rainy and snowy day | 34 | No lighting at night | 15 | ||
Foggy weather | 8 | Visibility less than 100 m | 8 |
Scale | Degree of Importance |
---|---|
1 | Equally important |
3 | Moderately important |
5 | Strongly important |
7 | Very strongly important |
9 | Extremely important |
2, 4, 6, 8 | Intermediate values |
1 | 2 | 3 | 4 | 5 | 6 | 7 | 8 | 9 | 10 | |
---|---|---|---|---|---|---|---|---|---|---|
0 | 0 | 0.58 | 0.90 | 1.12 | 1.24 | 1.32 | 1.41 | 1.45 | 1.49 |
Result | First-Level Influencing Factors | Second-Level Influencing Factors | Third-Level Influencing Factors | Selected Studies |
---|---|---|---|---|
Urban road traffic accidents | Human factor U1 | Motor vehicle drivers’ bad condition U11 | Inexperience U111 | [ , ] |
Old and infirm U112 | [ , ] | |||
Emotional driving U113 | [ , ] | |||
Drunk driving U114 | [ , ] | |||
Fatigue driving U115 | [ , , ] | |||
Motor vehicle drivers’ misconduct U12 | Driving without a license U121 | [ ] | ||
Illegal U-turn U122 | [ ] | |||
Illegal overtaking U123 | [ ] | |||
Illegal lane change U124 | [ ] | |||
Traffic signal violation U125 | [ ] | |||
Failure to maintain a safe distance U126 | [ ] | |||
Not yielding to pedestrians at zebra crossings U127 | [ ] | |||
Untimely braking U128 | [ ] | |||
Non-motor vehicle drivers’ unsafe behavior U13 | Swerve U131 | [ ] | ||
Crossing the road U132 | [ , ] | |||
No safety helmet U133 | [ , ] | |||
Occupy motor vehicle lanes U134 | [ ] | |||
Unsafe behavior by pedestrians and passengers U14 | Illegal crossing lanes U141 | [ ] | ||
Illegally crossing the traffic barrier U142 | [ ] | |||
Traffic signal violation U143 | [ ] | |||
Not observing traffic environment U144 | [ , ] | |||
Vehicle factor U2 | Safety condition U21 | Tire burst U211 | [ , ] | |
Steering failure U212 | [ ] | |||
Brake failure U213 | [ ] | |||
Safety hazard U22 | Over speed U221 | [ , , ] | ||
Overloaded U222 | [ ] | |||
Large truck U223 | [ ] | |||
Road factor U3 | Road section U31 | Uphill and downhill section U311 | [ ] | |
Sharp turn section U312 | [ , ] | |||
Intersection U313 | [ , ] | |||
Road condition U32 | Slippery road U321 | [ ] | ||
Pavement construction U322 | [ , ] | |||
Traffic sign problem U323 | [ , ] | |||
Environment factor U4 | Weather condition U41 | Rain and snow U411 | [ , ] | |
Foggy U412 | [ , ] | |||
Sight condition U42 | No lighting at night U421 | [ , ] | ||
Visibility below 100 m U422 | [ , , , ] |
U1 | U2 | U3 | U4 | |
---|---|---|---|---|
U1 | 1 | 3 | 2 | 2 |
U2 | 1/3 | 1 | 1/3 | 1 |
U3 | 1/2 | 3 | 1 | 4 |
U4 | 1/2 | 1 | 1/4 | 1 |
Judgment Matrixes | CI | RI | CR | |
---|---|---|---|---|
U11–U14 | 4.233 | 0.078 | 0.9 | 0.086 |
U21–U22 | 2 | 0 | 0 | 0 |
U31–U32 | 2 | 0 | 0 | 0 |
U41–U42 | 2 | 0 | 0 | 0 |
U111–U115 | 5.306 | 0.076 | 1.12 | 0.068 |
U121–U128 | 8.949 | 0.136 | 1.41 | 0.096 |
U131–U134 | 4.184 | 0.061 | 0.9 | 0.068 |
U141–U144 | 4.121 | 0.04 | 0.9 | 0.045 |
U211–U213 | 3.054 | 0.027 | 0.58 | 0.046 |
U221–U223 | 3.054 | 0.027 | 0.58 | 0.046 |
U311–U313 | 3.094 | 0.047 | 0.58 | 0.081 |
U321–U323 | 3.104 | 0.052 | 0.58 | 0.089 |
U411–U412 | 2 | 0 | 0 | 0 |
U421–U422 | 2 | 0 | 0 | 0 |
First-Level Factors | Subjective (Global) Weight | Objective (Global) Weight | Comprehensive Weight | First-Level Global Weight | Rank | Weight Difference |
---|---|---|---|---|---|---|
U1 | 0.405 | 0.468 | 0.437 | 0.437 | 1 | −0.063 |
U2 | 0.126 | 0.143 | 0.135 | 0.135 | 4 | −0.017 |
U3 | 0.340 | 0.252 | 0.294 | 0.294 | 2 | 0.088 |
U4 | 0.129 | 0.137 | 0.134 | 0.134 | 3 | −0.008 |
Second-Level Factors | Subjective Weight | Subjective Global Weight | Objective Weight | Objective Global Weight | Comprehensive Weight | Second-Level Global Weight | Rank | Weight Difference |
---|---|---|---|---|---|---|---|---|
U11 | 0.194 | 0.078 | 0.211 | 0.099 | 0.202 | 0.089 | 6 | −0.021 |
U12 | 0.429 | 0.174 | 0.453 | 0.212 | 0.441 | 0.193 | 1 | −0.038 |
U13 | 0.230 | 0.093 | 0.215 | 0.101 | 0.223 | 0.098 | 4 | −0.008 |
U14 | 0.147 | 0.060 | 0.121 | 0.057 | 0.134 | 0.058 | 8 | 0.003 |
U21 | 0.333 | 0.042 | 0.338 | 0.048 | 0.336 | 0.045 | 10 | −0.006 |
U22 | 0.667 | 0.084 | 0.662 | 0.095 | 0.664 | 0.089 | 5 | −0.011 |
U31 | 0.4 | 0.136 | 0.383 | 0.097 | 0.392 | 0.115 | 3 | 0.039 |
U32 | 0.6 | 0.204 | 0.617 | 0.155 | 0.608 | 0.179 | 2 | 0.049 |
U41 | 0.667 | 0.086 | 0.646 | 0.088 | 0.656 | 0.088 | 7 | −0.002 |
U42 | 0.333 | 0.043 | 0.354 | 0.048 | 0.344 | 0.046 | 9 | −0.005 |
Third-Level Factors | Subjective Weight | Subjective Global Weight | Objective Weight | Objective Global Weight | Comprehensive Weight | Third-Level Global Weight | Rank | Weight Difference |
---|---|---|---|---|---|---|---|---|
U111 | 0.372 | 0.029 | 0.340 | 0.034 | 0.356 | 0.032 | 11 | −0.005 |
U112 | 0.110 | 0.009 | 0.106 | 0.011 | 0.109 | 0.010 | 34 | −0.002 |
U113 | 0.226 | 0.018 | 0.213 | 0.021 | 0.220 | 0.019 | 21 | −0.003 |
U114 | 0.146 | 0.011 | 0.191 | 0.019 | 0.167 | 0.015 | 26 | −0.008 |
U115 | 0.146 | 0.011 | 0.149 | 0.015 | 0.148 | 0.013 | 28 | −0.004 |
U121 | 0.072 | 0.013 | 0.059 | 0.013 | 0.066 | 0.013 | 30 | 0 |
U122 | 0.087 | 0.015 | 0.050 | 0.011 | 0.066 | 0.013 | 29 | 0.004 |
U123 | 0.085 | 0.015 | 0.079 | 0.017 | 0.083 | 0.016 | 24 | −0.002 |
U124 | 0.173 | 0.030 | 0.158 | 0.034 | 0.167 | 0.032 | 10 | −0.004 |
U125 | 0.128 | 0.022 | 0.099 | 0.021 | 0.114 | 0.022 | 17 | 0.001 |
U126 | 0.116 | 0.020 | 0.099 | 0.021 | 0.108 | 0.021 | 18 | −0.001 |
U127 | 0.099 | 0.017 | 0.089 | 0.019 | 0.095 | 0.018 | 22 | −0.002 |
U128 | 0.241 | 0.042 | 0.366 | 0.078 | 0.300 | 0.058 | 4 | −0.036 |
U131 | 0.217 | 0.020 | 0.208 | 0.021 | 0.213 | 0.021 | 19 | −0.001 |
U132 | 0.258 | 0.024 | 0.25 | 0.025 | 0.254 | 0.025 | 16 | −0.001 |
U133 | 0.434 | 0.040 | 0.458 | 0.046 | 0.446 | 0.044 | 7 | −0.006 |
U134 | 0.091 | 0.009 | 0.083 | 0.008 | 0.087 | 0.009 | 36 | 0.001 |
U141 | 0.160 | 0.010 | 0.185 | 0.011 | 0.173 | 0.010 | 32 | −0.001 |
U142 | 0.227 | 0.013 | 0.259 | 0.015 | 0.243 | 0.014 | 27 | −0.002 |
U143 | 0.160 | 0.010 | 0.148 | 0.008 | 0.154 | 0.009 | 35 | 0.002 |
U144 | 0.453 | 0.027 | 0.407 | 0.023 | 0.430 | 0.025 | 15 | 0.004 |
U211 | 0.184 | 0.008 | 0.174 | 0.008 | 0.179 | 0.008 | 37 | 0 |
U212 | 0.232 | 0.010 | 0.261 | 0.013 | 0.246 | 0.011 | 31 | −0.003 |
U213 | 0.584 | 0.025 | 0.565 | 0.027 | 0.575 | 0.026 | 13 | −0.002 |
U221 | 0.396 | 0.033 | 0.4 | 0.038 | 0.398 | 0.036 | 8 | −0.005 |
U222 | 0.105 | 0.009 | 0.111 | 0.011 | 0.108 | 0.010 | 33 | −0.002 |
U223 | 0.499 | 0.042 | 0.489 | 0.046 | 0.494 | 0.044 | 6 | −0.004 |
U311 | 0.225 | 0.031 | 0.217 | 0.021 | 0.221 | 0.025 | 14 | 0.010 |
U312 | 0.165 | 0.023 | 0.174 | 0.017 | 0.170 | 0.020 | 20 | 0.006 |
U313 | 0.610 | 0.083 | 0.609 | 0.059 | 0.609 | 0.070 | 3 | 0.024 |
U321 | 0.550 | 0.112 | 0.514 | 0.080 | 0.532 | 0.095 | 1 | 0.032 |
U322 | 0.189 | 0.039 | 0.189 | 0.029 | 0.189 | 0.034 | 9 | 0.01 |
U323 | 0.261 | 0.053 | 0.297 | 0.046 | 0.279 | 0.050 | 5 | 0.007 |
U411 | 0.8 | 0.069 | 0.810 | 0.071 | 0.805 | 0.071 | 2 | −0.002 |
U412 | 0.2 | 0.017 | 0.191 | 0.017 | 0.195 | 0.017 | 23 | 0 |
U421 | 0.667 | 0.029 | 0.652 | 0.032 | 0.659 | 0.030 | 12 | −0.003 |
U422 | 0.333 | 0.014 | 0.348 | 0.017 | 0.341 | 0.016 | 25 | −0.003 |
The statements, opinions and data contained in all publications are solely those of the individual author(s) and contributor(s) and not of MDPI and/or the editor(s). MDPI and/or the editor(s) disclaim responsibility for any injury to people or property resulting from any ideas, methods, instructions or products referred to in the content. |
Share and Cite
Zeng, Y.; Qiang, Y.; Zhang, N.; Yang, X.; Zhao, Z.; Wang, X. An Influencing Factors Analysis of Road Traffic Accidents Based on the Analytic Hierarchy Process and the Minimum Discrimination Information Principle. Sustainability 2024 , 16 , 6767. https://doi.org/10.3390/su16166767
Zeng Y, Qiang Y, Zhang N, Yang X, Zhao Z, Wang X. An Influencing Factors Analysis of Road Traffic Accidents Based on the Analytic Hierarchy Process and the Minimum Discrimination Information Principle. Sustainability . 2024; 16(16):6767. https://doi.org/10.3390/su16166767
Zeng, Youzhi, Yongkang Qiang, Ning Zhang, Xiaobao Yang, Zhenjun Zhao, and Xiaoqiao Wang. 2024. "An Influencing Factors Analysis of Road Traffic Accidents Based on the Analytic Hierarchy Process and the Minimum Discrimination Information Principle" Sustainability 16, no. 16: 6767. https://doi.org/10.3390/su16166767
Article Metrics
Article access statistics, further information, mdpi initiatives, follow mdpi.
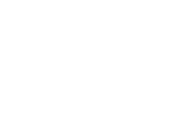
Subscribe to receive issue release notifications and newsletters from MDPI journals

An official website of the United States government
The .gov means it’s official. Federal government websites often end in .gov or .mil. Before sharing sensitive information, make sure you’re on a federal government site.
The site is secure. The https:// ensures that you are connecting to the official website and that any information you provide is encrypted and transmitted securely.
- Publications
- Account settings
Preview improvements coming to the PMC website in October 2024. Learn More or Try it out now .
- Advanced Search
- Journal List
- Health Sci Rep
- v.7(7); 2024 Jul
- PMC11211207
Root cause analysis and strategies for reducing falls among inpatients in healthcare facilities: A narrative review
Parvin lakbala.
1 Department of Health Services Management, Hormozgan University of Medical Sciences, Bandar Abbas Iran
Najmeh Bordbar
2 Health Human Resources Research Centre, School of Management and Medical Information Sciences, Shiraz University of Medical Sciences, Shiraz Iran
Yadolah Fakhri
3 Department of Environmental Health Engineering, Hormozgan University of Medical Sciences, Bandar Abbas Iran
Associated Data
The data that support the findings of this study are available from the corresponding author upon reasonable request.
Background and Aims
Root Cause Analysis (RCA) is a systematic process which can be applied to analyze fall incidences in reactive manner to identify contributing factors and propose actions for preventing future falls. To better understand cause of falls and effective interventions for their reduction we conducted a narrative review of RCA and Strategies for Reducing Falls among Inpatients in Healthcare Facilities.
In this narrative review, databases including Scopus, ISI Web of Science, Cochrane, and PubMed were searched to obtain the related literature published. Databases were searched from January 2005 until the end of March 2023. The Joanna Briggs Institute (JBI) tool was used for quality assessment of articles. To analyze the data, a five‐stage framework analysis method was utilized.
Seven articles that fulfilled the inclusion criteria were identified for this study. All of the selected studies were interventional in nature and employed the RCA method to ascertain the underlying causes of inpatient falls. The root causes discovered for falls involved patient‐related factors (37.5%), environmental factors (25%), organizational and process factors (19.6%), staff and communication factors (17.9%). Strategies to reduce falls involved environmental measures and physical protection (29.4%), identifying, and displaying the causes of risk (23.5%), education and culturalization (21.6%), standard fall risk assessment tool (13.7%), and supervision and monitoring (11.8%).
the findings identify the root causes of falls in inpatient units and provide guidance for successful action plan execution. Additionally, it emphasizes the importance of considering the unique characteristics of healthcare organizations and adapting interventions accordingly for effectiveness in different settings.
1. INTRODUCTION
Falls are a primary cause of avoidable injury in hospital and outpatient settings, 1 and can result in reduced mobility, functionality, and engagement in everyday tasks. Additionally, falls can contribute to longer hospital stays 2 , 3 , 4 and increased mortality rates. 2 , 3 , 5 , 6 These incidents primarily affect frail patients, many of whom have dementia. 7 Research indicates that the occurrence of falls can range from 1.7 to 16.9 falls per 1,00 patient days. 8 , 9 Among patients over the age of 65, approximately one‐third experience at least one fall per year. 10 Furthermore, 20%–30% of falls result in moderate to severe injuries, 11 such as skin excoriation, fractures, dislocations, and head trauma. Various factors contribute to the risk of falls, including age, gender, comorbidities, physical and psychosocial impairments, and medication usage. These risk factors are applicable to both the general population and the elderly. 12 , 13 Falls indeed create a substantial financial burden on the U.S. health systems, with an estimated $754 million in 2015 spent on medical expenses associated with fall‐related fatalities. 14 Furthermore, approximately 43% of these incidents result in harm to the patient. 15 , 16 Approximately 14% of falls that occur in hospitals can be categorized as accidental due to environmental factors, such as slipping on a wet floor. Another 8% of falls are considered unpredictable, resulting from sudden disturbances in the patient's physical condition that affect their balance. The majority, accounting for 78% of falls, are deemed predictable. 17 Potential factors that may contribute to falls include the introduction of new medications and alterations in medication dosage. 18
Root cause analysis (RCA) was introduced in the healthcare industry nearly two decades ago. It is a methodical and structured approach employed to ascertain the fundamental factors that contribute to variations in performance. RCA serves as a widely accepted and standardized technique for pinpointing the causes of medical errors, thereby empowering healthcare establishments to devise strategies aimed at mitigating future errors. 19 RCA has been widely employed within healthcare settings, particularly in hospitals, to systematically address the issue of falls. 20 The primary objective of RCA is to identify and rectify the underlying cause of falls, thereby mitigating the likelihood of recurrence. 21 It is important to note that the primary objective of the RCA process is not to assign blame to individuals, but rather to identify shortcomings in systemic processes, with the ultimate goal of preventing harm to patients, minimizing the occurrence of adverse events, and rectifying deficiencies. 22
Studies have shown that implementing a fall prevention program based on RCA can lead to positive outcomes for inpatients and enhance patient safety through subsequent interventions. 23 , 24 While single and multi‐component interventions are planned to reduce hospital falls, data on successful prevention programs are limited. 20 On the other hand, narrative reviews play a crucial role in presenting a descriptive summary of a topic and conducting a subjective analysis of the literature. They are essential for synthesizing complex research evidence in a detailed and nuanced manner, particularly for topics requiring comprehensive analysis. Narrative reviews offer a flexible and practical approach to synthesizing diverse literature, making them valuable tools. 25 , 26 Therefore, the aim of this narrative review study was to identify the causes of falls and strategies for their reduction within inpatient healthcare settings through RCA.
2. METHODOLOGY
2.1. search strategy.
In this narrative review, databases including Scopus, ISI Web of Science, Cochrane, and PubMed were searched to obtain the related literature published. Databases were searched from January 2005 until the end of March 2023. The search strategy included the following keywords: ‘RCA,’ ‘Root Cause Analysis,’ Falls, ‘Patient falls,’ ‘Reduce Falls,’ ‘Preventable Falls,’ ‘Decreasing Falls,’ ‘Patient safety,’ Safety, Patient, Delivery, Problems.” In order to increase sensitivity (to increase the selection of related articles), the researcher searched several databases with relatively common terms and synonymous words using the “OR” operator in the titles and abstracts of articles. In addition, to increase the specificity (to reduce the selection of irrelevant articles), we used “AND” operator. The search strategy is given in Table 1 . In addition, to ensure a comprehensive search of articles, the references of selected articles were also reviewed.
The search strategy.
Scopus, ISI Web of Science, Cochrane, and PubMed | |
Language (English) and only original research | |
January 2005 ‐ March 2023 | |
: #1 AND #2, #1 AND #3 | |
#1 | “RCA” OR “root cause analysis” |
#2 | “Patient safety” OR “delivery” OR “safety” OR “patient” OR “Problems” |
#3 | “falls” OR “reduce* falls” OR “decreasing falls” OR “patient falls” OR “preventable falls” |
2.2. Inclusion and exclusion criteria
The following criteria were used as guidelines for screening articles. The inclusion criteria for this study focused on selecting original English articles that employed RCA methods and were interventional in nature. Articles from Journals that did not have a rigorous evaluation process, book reviews, opinion articles, review articles, and letters to editor were exclusion criteria.
2.3. Study selection
The studies obtained from the search were screened by two reviewers (P.L and N.B) separately in three stages (title, abstract and full text), and the result was based on the agreement of these two reviewers. Third reviewer, Y.F., helped to reach a consensus regarding the disagreement. Screening was performed using Endnote v.8.
2.4. Critical appraisal
The Joanna Briggs Institute (JBI) tool was used for quality assessment of articles. We used the JBI Statement, which has a 9‐item checklist, to ensure transparent reporting of studies. Each study was assessed by indicating “Yes,” “No,” “Unclear” or “Not applicable” for each item on the checklist. 27 To prevent bias, the assessments were performed by two reviewers (Parvin Lakbala and Najmeh Bordbar), and a consensus was reached through Third reviewer, Yadolah Fakhri.
2.5. Data extraction
According to the screened studies, the data were extracted to achieve the research goals and questions. For this purpose, first the data extraction form including the names of authors of the article, year, country of study, health setting, health units, type of study, study design, RCA participants, intervention period, fall reduction, fall causes and reducing strategies was designed. In the next step, one of the authors (Parvin Lakbala) extracted the data from the selected articles and the second author (Najmeh Bordbar) reviewed the data. This form was designed and completed for each article in Excel 2013.
2.6. Data synthesis
To analyze the data, a five‐stage framework analysis method was utilized. In the first stage, the researcher repeatedly read extracted data from the included articles to become familiar with the data. The second stage involved forming repetitive ideas into groups of similar themes to identify a thematic framework. The third stage, indexing, focused on identifying units or clusters of data related to specific themes. Following indexing, the data were summarized in a table of themes based on the thematic framework. In the fifth stage, the data were combined, and maps and interpretations were used to clarify concepts, illustrate relationships between concepts, characterize the phenomenon, and provide explanations and suggestions. 28 To ensure dependability, two members of the research team (Parvin Lakbala and Najmeh Bordbar) individually analyzed the contents and discussed the issues to reach a consensus. Ultimately, at the end of this process, the causes of falls and strategies for their reduction were identified.
In the initial search, 255 articles were found and after removing duplicate and irrelevant articles, the abstract of 58 articles was reviewed. At this stage, 36 articles were deleted since they did not meet the inclusion criteria. Finally, after reading the full text of 22 articles, only seven articles were eligible for inclusion (Figure 1 ).

PRISMA Flow diagram for article selection.
Table 2 presents the characteristics of the seven included studies. Studies were conducted in the United States 6 and Saudi Arabia, 1 published between 2008 and 2022. Four studies were conducted in a general hospital (inpatient units), 29 , 31 , 33 , 34 1 study in a children's hospital (mother and baby unit), 30 1 study in an acute care hospital (inpatient behavioral unit), 23 and 1 study in a level one trauma center (emergency department). 32 All studies were interventional and used the RCA method to identify the causes of inpatients' falls. 23 , 29 , 30 , 31 , 32 , 33 , 34 Most studies had established interdisciplinary quality improvement teams and committees to identify causes of falls, 23 , 29 , 30 , 31 , 33 , 34 and only one study had revised an existing risk assessment tool. 32 Further details of RCA participants and interventional period are described in Table 2 .
Study characteristics of included studies.
First author (year) | Country of study | Setting | Unit | Type of study | Study design | RCA participants | intervention period |
---|---|---|---|---|---|---|---|
Wilson et al. | USA | Mackenzie Health (MH) | all inpatient units | organized an interprofessional falls quality aim committee | Interventional/RCA |
| 1 year |
Whatley et al. | USA | Children's Hospital at Dartmouth‐Hitchcock Medical Center | mother and baby unit | convened an interprofessional team | Interventional/RCA |
| 3 years |
Ocker et al. | USA | an acute care hospital | adult inpatient behavioral health unit | established a unit‐based QI team | Interventional/RCA |
| Six months |
Kuwaiti & Subbarayalu | Saudi Arabia | King Fahd Hospital of the University | All inpatient units except outpatients, emergency room, obstetric, pediatric, all procedural areas, operating room, and perioperative services | formed a multidisciplinary team | Interventional/RCA |
| 1 year |
Alexander et al. | USA | Hartford Hospital a Level I Trauma Center | emergency department | revise the existing fall risk tool | Interventional/RCA |
| 1 year |
Weinberg et al. | USA | Staten Island University Hospital | all inpatient units | appointed hospital falls committee (HFC) | Interventional/RCA |
| Four‐year |
Ruddick et al. | USA | 11 hospitals | all inpatient units | conducted the hospitals' interdisciplinary team (IDT) | Interventional/RCA |
| 6 months |
The root causes discovered for falls involved patient‐related factors (37.5%), environmental factors (25%), organizational and process factors (19.6%), staff and communication factors (17.9%). Table 3 displays the specific categories for the reported root causes. Causes such as medication/sedation, inappropriate footwear, unexpected movement, gait and balance limitations, patients under the influence of alcohol, and cognitive impairments (confusion, disorientation, memory deficits, and dizziness) were identified with higher frequency as contributing patient‐related factors to falls. 23 , 29 , 30 , 32 , 34 Various environmental factors have been identified in studies as contributors to falls. These include issues with toilets, tubs, and showers, equipment that poses tripping hazards, a noisy environment, wet floors, cold room temperature, bed/side rails, call lights not being used, crowded rooms, flooring problems, and poor lighting. 23 , 29 , 30 , 34 The study identified organizational and process factors contributing to falls, including the absence of hourly rounding, improper use of bed alarms, tab alarms, or call bells, an incomplete fall risk assessment tool, and the lack of routine falls risk assessments at admission. 30 , 31 , 32 , 34 Staff and communication factors can contribute to falls. Some causes related to these factors include a lack of team communication regarding fall risk assessment, inadequate staff accountability to follow fall prevention protocols, and inadequate safety awareness by staff. 23 , 29 , 30 , 31 , 33
Causes of falls occurring in inpatient units.
| ||
| ||
| ||
|
Table 4 outlines the strategies that were reported in included studies to reduce falls. These strategies involved environmental measures and physical protection (29.4%), identifying, and displaying the causes of risk (23.5%), education and culturalization (21.6%), standard fall risk assessment tool (13.7%), and supervision and monitoring (11.8%). No strategies were reported to have resulted in a negative outcome (Appendix A1 ).
Strategies used to reduce falls in included studies.
Categories | Strategies | Frequency N (%) |
---|---|---|
| 15 (29.4) | |
| 12 (23.5) | |
| 11 (21.6) | |
| 7 (13.7) | |
| 6 (11.8) |
4. DISCUSSION
Patient safety involves creating a safe environment and assessing the likelihood of incidents such as falls, medication errors, and infections. On the other hand, RCA is a process that analyzes incidents in a reactive manner to identify contributing factors and propose actions for mitigation. 35 But like any other method, they are not without limitations. RCA lacks a systemic perspective and implies a single, linear cause. Their inability to see the nonlinear causal mechanism among cause and effect relationships limits them to finding only one absolute root cause. 36 , 37 The aim of this study was to identify the causes of falls and effective interventions for their reduction within inpatient healthcare settings through RCA. Indeed, since the included studies were conducted in various treatment facilities and units, it is expected that the causes of falls and the interventions and strategies employed to reduce them would vary.
The studies consistently found that factors related to the patient were the most frequently reported causes of falls. These factors played a significant role in contributing to fall incidents across the various studies. It's important to consider the results of these studies that highlight medication/sedation as a common cause of falls. According to Najafpour et al's case control study, a combination of patient‐related factors and medication history taken into account as risk factors for falls in hospital in‐patients. 38 In the study by Cioce et al, diuretic drugs were reported as the most common cause of falls with a frequency of 93.33%. To reduce the risk of falls associated with these drugs, recommend rescheduling their administration. 4 In the study by Santana et al., it was found that 74.8% of fall patients had been prescribed sedatives, psychoactive, or antihypertensive drugs. 39 According to Ribeiro et al's review, the use of central nervous system drugs increases the risk of inpatient falls, including anxiolytics, hypnotics, sedatives, antipsychotics, opioids, antiepileptics, and antidepressants. 40 Various medications are linked to a notable risk of falls, known as “fall risk increasing drugs.” Managing drug‐induced falls is crucial for fall prevention, emphasizing the importance of considering the risk level of medications to optimize drug therapy in clinical settings. 41 , 42 In the study by Weinberg et al., specific interventions were implemented to address falls related to medication use. One intervention involved restricting the use of diphenhydramine and hydroxyzine in patients aged 65 and above. Another intervention focused on modifying the administration protocol for furosemide. To reduce the need for nighttime voiding, furosemide was administered 3 h earlier, at 6:00 P.M. These interventions were aimed at minimizing the potential side effects and risks associated with these medications, ultimately reducing the likelihood of falls in the targeted patient population. 33
The studies found that environmental measures and physical protection were the most frequently reported strategies. Addressing these factors is crucial in reducing the risk of falls and promoting a safer environment. In the study conducted by Whatley et al., they implemented measures to reduce the potential injuries caused by falls. These measures included considering the type and height of the mother's bed, implementing physical preventive interventions such as having bed rails up, removing tripping hazards. These interventions aimed to create a safer environment and minimize the risk of falls and subsequent injuries. 30 Additionally, high‐risk patients were offered assisted toileting every 2 h during the day and whenever awake at night. This intervention aimed to address the increased risk of falls associated with the need for frequent toileting. 33 In the study conducted by Ocker et al., they implemented various measures to address fall risk among patients. Firstly, they identified all patients at risk for falls by providing them with a yellow fall‐alert bracelet and placing signs on the doors of their rooms. Additionally, they provided warm sweat suits as an alternative to traditional patient gowns, aiming to discourage the excessive use of blankets during patient ambulation, which can increase the risk of falls. Furthermore, wireless bed and chair alarms were purchased and utilized for impulsive patients to provide timely alerts and prevent falls. 23 In the Kuwaiti and Subbarayalu study, they implemented bed alarm systems and utilized orange stickers/fall risk signs in common fall risk zones within the hospital. These measures were implemented with the goal of reducing falls among patients. Bed alarm systems are designed to alert healthcare providers when a patient attempts to leave the bed without assistance, helping to prevent falls. The use of orange stickers or fall risk signs in specific areas serves as a visual reminder for both patients and staff to exercise caution and take necessary precautions in those areas. By implementing these strategies, the study aimed to enhance patient safety and minimize the incidence of falls in the hospital setting. 31 In the study by Boot et al., they developed an assessment tool to identify high‐risk patients, used wristbands to identify those at risk of falling, and emphasized increased observation for patients prone to falls. 43
Identifying and displaying the causes of risk were the second frequently reported strategies. patients and their family members, as well as staff, represent useful information toward finding the cause of initial falls across RCA process that can be used to prevent subsequent falls. 29 , 32 , 33 , 34 In this regard, various intervention initiatives were implemented, including placing notices on unit dashboards to highlight the reduction of falls, 32 highlighting patients' fall risks on nurses' station whiteboards for easy visibility, incorporating fall risk levels and factors into patient handoff, 23 holding daily contests for the lowest number of consecutive fall‐free days, 33 implementing electronic dashboards to display real‐time unit‐specific falls rates, and soliciting staff feedback on barriers to falls prevention. 29 Ineffective communication among healthcare team members can result in important information about a patient's fall risk assessment not being properly shared, leading to a lack of awareness and failure to implement preventive measures. When staff members do not feel accountable or responsible for adhering to fall prevention protocols, there is a higher likelihood of gaps in implementing preventive measures, increasing the risk of falls.
Additionally, a lack of sufficient training or education on fall prevention strategies and maintaining a safe environment can contribute to inadequate safety. By prioritizing education, culturalization, and clarity, healthcare organizations can enhance staff knowledge, promote cultural sensitivity, and improve communication practices. These efforts contribute to a safer environment, reduced falls, and improved patient safety outcomes. staff training, 23 , 29 , 30 , 31 , 32 , 33 , 34 In the study by Paulino et al., it was found that aspects related to the health work process, such as teamwork, professional skills, and nursing care execution, are the main contributing factors to falls. Effective teamwork, proper skills and training, and adherence to protocols are crucial in preventing falls and improving patient safety. 44 In a review study conducted by Heng et al., it was found that hospital falls prevention interventions that include patient education can effectively reduce falls and associated injuries like bruising, lacerations, or fractures. The success of these interventions is influenced by factors such as the design, delivery method, and quality of educational programs. Well‐designed education programs have shown to improve patients' knowledge and self‐perception of risk, empowering them to take proactive measures to reduce their risk of falling while in the hospital. 45 One study found that several key factors were identified as helpful in promoting effective patient education. These included providing individualized and consistent education, using small interactive groups, and adopting a patient‐centered multi‐factorial approach. 46 The findings of a systematic review and meta‐analysis demonstrated that educating patients and staff significantly reduces the occurrence of bed falls in the hospital setting. This highlights the effectiveness of training interventions in preventing such incidents and promoting patient safety. 47 In addition, according to clinical practice guidelines patients and residents should be screened for fall risks upon admission to the hospital. Multifactorial interventions based on individual risk factors are strongly recommended in hospitals, as they significantly reduce falls. Patients at risk of falling should receive training and advice on fall prevention measures. Caregivers should also receive active educational interventions to increase their knowledge and prevent residents from falling. 48
5. CONCLUSION
Multiple factors, such as patient‐related, environmental, organizational and process, staff and communication factors, contribute to the occurrence of falls in hospitalized patients. Identifying and addressing these factors is essential for preventing falls and ensuring patient safety in healthcare settings. Each healthcare organization has its own unique characteristics and processes, so while certain solutions and strategies have been effective in reducing patient falls in one facility, they may not work the same way in another organization. It is important to consider the specific context and adapt interventions accordingly to ensure effectiveness in different healthcare settings.
6. LIMITATIONS
This study has some limitations. As mentioned, due to the limitations of the RCA method, including only studies that used the RCA was one of the major limitations of this study. Secondly, it is difficult to determine which actions were responsible for the reduction in falls, as multiple interventions were implemented simultaneously. finally, detailed information about the characteristics of the population studied could not be obtained. These limitations should be considered when interpreting the study's findings.
AUTHOR CONTRIBUTIONS
Parvin Lakbala designed the study and its overall methodology, contributed in data analysis and edited the article. Najmeh Bordbar contributed in data gathering, data analysis and prepared the initial draft of the article and also edited the article. Yadolah Fakhri contributed in the methodology of the study and edited the article. All authors read and approved the final manuscript.
CONFLICT OF INTEREST STATEMENT
The authors declare no conflict of interest.
TRANSPARENCY STATEMENT
The lead author Najmeh Bordbar affirms that this manuscript is an honest, accurate, and transparent account of the study being reported; that no important aspects of the study have been omitted; and that any discrepancies from the study as planned (and, if relevant, registered) have been explained.
APPENDIX A.
Table A1
Causes of falls and strategies used to reduce falls in included studies.
First author (year) | Fall causes | Falls reducing strategies | Reported fall reduction |
---|---|---|---|
Wilson et al. |
|
| from 2.03 to 1.12 per 1000 patient‐days |
Whatley et al. |
|
| from 71.8 to 15.5 per 10,000 births |
Ocker et al. |
|
| from 5.43 to 0 per 1000 patient‐days |
Kuwaiti & Subbarayalu |
|
| From 6.57 to 1.91 per 1000 patient‐days |
Alexander et al. |
|
| Not mentioned |
Weinberg et al. |
|
| From 3.9 to 1.1 per 1000 patient‐days |
Ruddick et al. |
|
| from 133.9 to 73.05 per 1000 patient‐days |
Lakbala P, Bordbar N, Fakhri Y. Root cause analysis and strategies for reducing falls among inpatients in healthcare facilities: a narrative review . Health Sci Rep . 2024; 7 :e2216. 10.1002/hsr2.2216 [ CrossRef ] [ Google Scholar ]
DATA AVAILABILITY STATEMENT
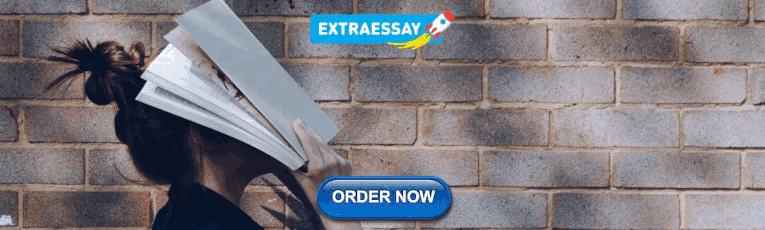
IMAGES
COMMENTS
Root cause analysis (RCA) emerged in the health care field almost 20 years ago. This technique is used worldwide to understand the remote and direct factors favouring the occurrence of an avoidable adverse event (AAE) [ 1 ], and improvement of patient safety [ 2 ]. Three studies have analysed the utility and limitations of this technique [ 3, 4 ...
Root cause analysis with corrective measures: A root cause analysis was performed, and measures were taken to avoid this problem. As the Institute of Safe Medication Practices recommended, magnesium sulfate was marked as a high-alert medication. ... Clinicians should remember to perform a final bedside evaluation and review discharge ...
Root Cause Analysis (RCA) is an analytical tool used by hospitals in quality improvement and patient safety efforts. While hospitals have widely embraced RCA, the effectiveness of the RCA process has been questioned in recent years. Based on a literature review and feedback from practicing administrators, this paper identifies current barriers ...
Searches for "root cause analysis" and "root cause analyses" were run from database inception to September 2007. We reviewed abstracts to obtain a general understanding of the published literature on RCA (Figure 1, right). Articles not in English and articles that did not directly address RCA were excluded from further review.
Root Cause Analysis Using the Prevention and Recovery Information System for Monitoring and Analysis Method in Healthcare Facilities: A Systematic Literature Review ... This is the first systematic literature review giving an overview of the use of the PRISMA-method in healthcare facilities. An experienced librarian (R.O.) assisted in the ...
Root cause analysis (RCA) emerges as a crucial tool in deciphering the fundamental factors that contribute to crisis occurrences. RCA is a methodical process that involves identifying ...
This literature review also focused on root cause analysis of production disturbances in the context of production systems. In this study, "production systems" (or "manufacturing systems") refer to manufacturing companies' industrial processes for transforming raw materials into finished goods.
Searches for "root cause analysis" and "root cause analyses" were run from database inception to September 2007. We reviewed abstracts to obtain a general understanding of the published literature on RCA (Figure 1, right). Articles not in English and articles that did not directly address RCA were excluded from further review.
Root Cause Analysis LiteratureReview Page 1 of 14. Literature Review 2017 . Informing best practice in the conduct of a Root Cause Analysis: A literature review . This document should be cited as: Yap, G. & Melder, A. 2017. Informing best practice in conducting a Root Cause Analysis: A literature review.
Root Cause Analysis Using the Prevention and Recovery Information System for Monitoring and Analysis Method in Healthcare Facilities: A Systematic Literature Review J Patient Saf. 2022 Jun 1;18(4) :342-350. doi ... The aims of this systematic review are to map the use of the PRISMA-method in healthcare facilities worldwide, to assess the ...
This paper implements a literature review protocol and reports the latest advances in Root Cause Analysis (RCA) toward Zero-Defect Manufacturing (ZDM). The most recent works are reported to demonstrate the use of machine learning methodologies for root cause analysis in the manufacturing domain. ... The inclusion criteria include: 1) papers ...
Explore the latest full-text research PDFs, articles, conference papers, preprints and more on ROOT CAUSE ANALYSIS. Find methods information, sources, references or conduct a literature review on ...
The "to err is human" report and the patient safety literature. Qual Saf Health Care. 2006; 15:174-178. [PMC free article] [Google ... Genn K, Brooks V, et al. System-wide learning from root cause analysis: a report from the New South Wales root cause analysis review committee. Qual Saf Health Care. 2010; 19:e63. [Google ...
Root cause analysis is perhaps the most widely used tool in healthcare risk management, but does it actually lead to successful risk control? ... Successful risk assessment may not always lead to successful risk control: A systematic literature review of risk control after root cause analysis J Healthc Risk Manag. 2012;31(3):6-12. doi: 10.1002 ...
Root cause analysis is an integral part of the large TQM toolbox of problem analysis, problem-solving and improvement tools (Andersen & Fagerhaug, 2006:12). This chapter is the last of three that document a literature review that covers the central concepts used in this study. It provides a detailed overview of the key concepts and processes ...
Background To evaluate the effectiveness of root cause analysis (RCA) recommendations and propose possible ways to enhance its quality in Hong Kong public hospitals. Methods A retrospective cross-sectional study was performed across 43 public hospitals and institutes in Hong Kong, reviewing RCA reports of all Sentinel Events and Serious Untoward Events within a two-year period. The incident ...
Root cause analysis is a problem-solving approach that uses the analogy of roots and blooms to model cause-and-effect relationships. Rather than focusing on what's above the surface, root cause analysis troubleshoots solutions to problems by analyzing what is causing them. Note. Similarly to exploratory research, it's important to remember ...
Objectives: The aim of this systematic review was to consolidate studies to determine whether Root Cause Analysis (RCA) is an adequate method to decrease recurrence of avoidable adverse events (AAE).
The effectiveness of root cause analysis: what does the literature tell us? The Joint Commission Journal on Quality and Patient Safety 2008; 34:391-8. 10.1016/S1553-7250(08) ... a systematic literature review of risk control after root cause analysis. J Healthc Risk Manag 2012; 31:6-12. 10.1002/jhrm.20090 [Google Scholar] 51. Peerally MF ...
Since 5 Whys analysis has been one of the more pervasive tools in. use, this study sought to add to the root cause analysis body of knowledge by. investigating tool support factors. While studied in conjunction with 5 Whys, the support. variables lend themselves to other root cause analysis tools as well.
Root Causes Analysis : Literature Review. Get Textbooks on Google Play. Rent and save from the world's largest eBookstore.
Root causes analysis is simply a tool designed to help incident investigators determine what, how and most importantly, why an incident occurred. Based on this literature review it is apparent that there are three key components that need to be applied to ensure effective root causes analysis incident investigation. These are a method of ...
Safe traffic is an important part of sustainable transportation. Road traffic accidents lead to a large number of casualties and property losses every year. Current research mainly studies some types of traffic accidents and ignores other types of traffic accidents; therefore, taking various types of road traffic accidents as a whole, an overall study of their influencing factors is urgently ...
Root Cause Analysis (RCA) is a systematic process which can be applied to analyze fall incidences in reactive manner to identify contributing factors and propose actions for preventing future falls. To better understand cause of falls and effective interventions for their reduction we conducted a narrative review of RCA and Strategies for ...