AI in Finance: A Review
36 Pages Posted: 6 Aug 2020 Last revised: 23 Nov 2021
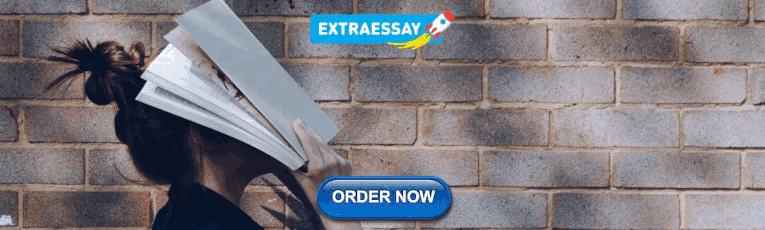
Longbing Cao
Macquarie University - Faculty of Science and Engineering
Date Written: July 10, 2020
The recent booming of AI in FinTech evidences the significant developments and potential of AI for making smart FinTech, economy, finance and society. AI-empowered smart FinTech has emerged as a sexy and increasingly critical area in AI, data science, economics, finance, and other relevant research disciplines and business domains. This trend was built on the long history of AI in finance, and the new-generation AI, data science and machine learning are fundamentally and seamlessly transforming the vision, missions, objectives, paradigms, theories, approaches, tools and social aspects of economics and finance and driving smart FinTech. AI is empowering more personalized and advanced and better, safer and newer mainstream and alternative economic-financial mechanisms, products, models, services, systems, and applications. This review summarizes the lasting research on AI in finance and focuses on creating a comprehensive, multidimensional and economic-financial problem-driven research landscape of the roles, research directions and opportunities of AI in new-generation FinTech and finance.
Keywords: Artificial intelligence, economics, finance, AI in FinTech, FinTech, AI in finance, AI in economics, data science, machine learning, data analytics, advanced analytics
JEL Classification: G001
Suggested Citation: Suggested Citation
Longbing Cao (Contact Author)
Macquarie university - faculty of science and engineering ( email ).
Balaclava Rd North Ryde, 2109 Australia
Do you have a job opening that you would like to promote on SSRN?
Paper statistics, related ejournals, behavioral & experimental finance (editor's choice) ejournal.
Subscribe to this free journal for more curated articles on this topic
Artificial Intelligence eJournal
Subscribe to this fee journal for more curated articles on this topic
Artificial Intelligence - Law, Policy, & Ethics eJournal
Innovation practice ejournal, investing ejournal, information systems ejournal, technology & investing ejournal, decision-making in economics & finance ejournal, decision-making in computational design & technology ejournal.
IEEE Account
- Change Username/Password
- Update Address
Purchase Details
- Payment Options
- Order History
- View Purchased Documents
Profile Information
- Communications Preferences
- Profession and Education
- Technical Interests
- US & Canada: +1 800 678 4333
- Worldwide: +1 732 981 0060
- Contact & Support
- About IEEE Xplore
- Accessibility
- Terms of Use
- Nondiscrimination Policy
- Privacy & Opting Out of Cookies
A not-for-profit organization, IEEE is the world's largest technical professional organization dedicated to advancing technology for the benefit of humanity. © Copyright 2024 IEEE - All rights reserved. Use of this web site signifies your agreement to the terms and conditions.
Applications of Explainable Artificial Intelligence in Finance—a systematic review of Finance, Information Systems, and Computer Science literature
- Open access
- Published: 28 February 2023
- Volume 74 , pages 867–907, ( 2024 )
Cite this article
You have full access to this open access article
- Patrick Weber ORCID: orcid.org/0000-0002-5921-6442 1 ,
- K. Valerie Carl ORCID: orcid.org/0000-0003-4655-1046 1 &
- Oliver Hinz ORCID: orcid.org/0000-0003-4757-0599 1
21k Accesses
41 Citations
7 Altmetric
Explore all metrics
Digitalization and technologization affect numerous domains, promising advantages but also entailing risks. Hence, when decision-makers in highly-regulated domains like Finance implement these technological advances—especially Artificial Intelligence—regulators prescribe high levels of transparency, assuring the traceability of decisions for third parties. Explainable Artificial Intelligence (XAI) is of tremendous importance in this context. We provide an overview of current research on XAI in Finance with a systematic literature review screening 2,022 articles from leading Finance, Information Systems, and Computer Science outlets. We identify a set of 60 relevant articles, classify them according to the used XAI methods and goals that they aim to achieve, and provide an overview of XAI methods used in different Finance areas. Areas like risk management, portfolio optimization, and applications around the stock market are well-researched, while anti-money laundering is understudied. Researchers implement both transparent models and post-hoc explainability, while they recently favored the latter.
Similar content being viewed by others
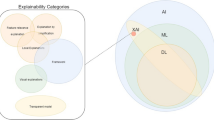
Explainable artificial intelligence (XAI) in finance: a systematic literature review
Artificial Intelligence in Finance and Accounting: Opportunities and Challenges
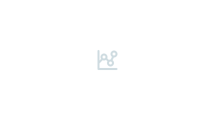
FinBrain 2.0: when finance meets trustworthy AI
Explore related subjects.
- Artificial Intelligence
Avoid common mistakes on your manuscript.
1 Introduction
The Finance industry is under constant development, always using and adapting to new technological opportunities (Gimpel et al. 2018 )—like Artificial Intelligence (AI) and Data Analytics—that shape private and working lives worldwide. Financial institutions benefit from technological advances, recently from AI deployment (Alt et al. 2018 ; Goodell et al. 2021 ): Due to their strong predictive performance, AI-based systems are becoming increasingly crucial for decision-making in various settings and offer a wide range of opportunities for companies to exploit the economic potential of augmentation (Collins et al. 2021 ). In line with this development, academia renewed its interest in researching the application of AI in the financial sector (Cao 2022 ; Padmanabhan et al. 2022 ). The application scenarios in the area of Finance are very diverse and have specific requirements for implementing automated systems. However, they have in common that they illustrate resentment against the black-box nature of AI-based systems, thus limiting their widespread use and hampering exploiting their full potential. This is where Explainable Artificial Intelligence (XAI) methods can help to counteract these adoption and implementation hurdles and exploit automation's full potential in regulated industries like Finance. Therefore, research on XAI in Finance gains interest (Adadi and Berrada 2018 ).
In general, XAI tries to mitigate the problem of non-transparency of AI and provides explanations to make the inner workings of AI models interpretable and easy to understand, as AI-generated rules from data are often unintelligible for humans. This unintelligibility creates a barrier concerning explainability and ultimately hinders the practical deployment of AI models, especially in highly-regulated application domains like Finance. Thus, XAI is a driver of further AI usage and adoption (Adadi and Berrada 2018 ; Janiesch et al. 2021 ; Mirbabaie et al. 2021 ; Sigrist and Hirnschall 2019 ) in particular in these domains as it helps to mitigate the current black-box nature of AI-based systems, i.e., unobservable inner workings.
Therefore it is not surprising that technological development has already found its way into the Finance domain. There are multiple areas of Finance examining and the first already employing XAI techniques, including risk management and portfolio optimization. One application scenario within risk management, which refers to a state of corporate failure (Sigrist and Hirnschall 2019 ), is default prediction. This specific area deals with predicting the probability of lenders committing default by using information such as profiles or loan history. As a mass business, there is a frequent and continuous need to reevaluate the default risk. AI methods support these endeavors, e.g., by assessing enormous amounts of available data and making informed recommendations. However, the reasons for these recommendations are often not transparent or comprehensible, leading to implementation resentment. XAI addresses these reservations by complementing AI-based suggestions with explanations to ensure they are discrimination-free and thoroughly founded, rendering the AI methods usable in the industry (Park et al. 2021 ).
In addition to these adoption hurdles, in a highly-regulated application domain such as Finance, laws and regulations condition the need for using XAI systems, as AI systems often would not comply with the law (Weber et al. 2020 ). Recent law initiatives, e.g., the US Financial Transparency Act of 2021 (FTA) (Maloney 2021 ) or the EU Artificial Intelligence Act (AIA) (European Commission 2021 ), prescribe very high levels of transparency when applying AI-supported decision-making in practice (Hoofnagle 2013 ; Elliott et al. 2021 ) in order to ensure trust in and reliability of these systems. The proposed US FTA gives the following justification: The act aspires to make reported information electronically searchable, to enable the creation of RegTech (i.e., technology supporting regulatory processes) and AI applications, and to decrease regulatory compliance burdens through standards and the enhancement of transparency and accountability. Footnote 1 The proposed EU AIA addresses AI’s risks or negative consequences for individuals or society, stating: “ Rules for AI available in the Union market or otherwise affecting people in the Union should therefore be human centric, so that people can trust that the technology is used in a way that is safe and compliant with the law, including the respect of fundamental rights. ” Regarding transparency, the AIA underlines proportionality, imposing higher transparency requirements on so-called high-risk AI systems such as those in Finance or Healthcare. Besides, the EU General Data Protection Regulation (GDPR) requires that decisions must always be the responsibility of people (Art. 22 GDPR), requiring some trust from employees and their ability to trace decisions made by an AI. Furthermore, some national authorities, e.g., the German Federal Financial Supervisory Authority, enforce additional requirements on the financial industry in particular, e.g., about the traceability of decisions and thus increased transparency (Pasquale 2015 ; BaFin 2018 ), which has always played an essential role (Vishwanath and Kaufmann 1999 ). Accordingly, evaluating XAI systems in Finance is essential for the applicability of such systems in these highly-regulated application domains, as automation aside from XAI models is less feasible from a regulatory point of view.
Nevertheless, research on XAI in Finance is widely dispersed (Elliott et al. 2021 ), making it more difficult for researchers and practitioners to unlock the full potential of XAI in the Finance domain. This paper aims to aggregate this scattered knowledge to provide an overview of the current state of research, including possible future research avenues, and to support practitioners’ implementation of XAI in financial business practice. A structured overview could represent another step towards realizing the full potential of AI-based systems, especially XAI systems, due to prevailing regulations, in the financial industry.
While there is research dealing with AI in Finance, from a more technological perspective in IS and CS, or XAI in general, there is no paper reviewing the current state of research on XAI in Finance in leading international journals and conferences (Arrieta et al. 2019 ). Searching for related research, we were only able to find qualitative literature reviews. These include dealing with aspects of the trustworthiness of AI employment in systemic risk assessment (Daníelsson et al. 2021 ), deep learning and anti-money laundering as a combination of a specific XAI method with a specific area in Finance (Kute et al. 2021 ), the design of smart markets, that, by using computational tools, help human decision-makers make real-time decisions in complex trading environments (Bichler et al. 2010 ), or banking in general (Burgt 2020 ). With our study presenting a systematic literature review (SLR), we follow calls for further research from the academic community concerning the compliance behavior in highly-regulated application domains such as Finance and how researchers, practitioners, and regulators should react to recent developments in AI and XAI (Ciatto et al. 2020 ). The need for this research is deepened by practitioners agreeing on the necessity of trustworthiness of AI in Finance, which is achievable through explainability (IEEE 2021 ). Besides, regulators in many countries demand greater transparency and explainability of decisions in the financial sector by requiring XAI rather than AI-based systems (Kalyanathaya 2022 ). Accordingly, we advance research and practice by reviewing relevant Finance, IS, and CS literature and performing an SLR to aggregate yet disorganized research on XAI in Finance.
With the paper at hand, we contribute to academia’s emerging interest in XAI in Finance research in different dimensions. First, our research offers guidance for scientists to understand the growing emphasis on XAI in Finance research that we also found in other domains (Wells and Bednarz 2021 ; Islam et al. 2022 ). Second, when taking a closer look at the goals these research endeavors and the application of XAI in practice try to achieve, we confirm an imbalance already highlighted by prior research (e.g., Arrieta et al. 2019 ). Third, scientists looking for the distribution of a specific XAI method in Finance may find answers in our results, as we provide an overview of current research on XAI, especially in Finance. As our final set of articles reveals, only a few papers deal with whether the developed or applied XAI methods meet regulatory requirements (except for, e.g., Park et al. 2021 ). This is a prerequisite for the implementation in practice, thus highlighting a critical future research avenue.
Practitioners, such as legislators, regulators, and Finance managers, benefit from our research, as we provide basics of XAI methods employed in Finance, a yet dispersed research area. Looking for existing XAI applications in their specific Finance areas, Finance managers may consult our study to learn about ready-to-employ solutions to add transparency to their AI-based systems. Alternatively, existing knowledge about XAI models and possible application scenarios in the company can be matched. Practitioners can use our study to gain a quick overview of already acceptable methods from a regulatory point of view. Policymakers may respond to the shift from transparent models to post-hoc explainability by designing corresponding laws and regulatory requirements. Finally, practitioners may infer trends in XAI in Finance to prepare their employees for future challenges through specialized XAI trainings.
The remainder of this study is structured as follows. Next, we present a section about related literature introducing the areas of AI and XAI on the one hand and the Finance domain (i.e., technological influences and areas where AI and XAI are applicable) on the other. In this way, we establish common ground for the characteristics of different XAI models, reconvene why the explainability of AI is crucial for decision-making, describe the main concepts of how to achieve it, and introduce the possibilities for usage of (X)AI-based systems in Finance. Then, we give an overview of our research method, followed by a presentation of our results. We conclude with a discussion of the theoretical and managerial implications of our research next to limitations and avenues for future research.
2 Related literature
2.1 artificial intelligence.
AI is a key technology of the 21st century which is applied in various areas, e.g., search engines, voice recognition, and interactive interpreters (Russell et al. 2016 ). Starting from the mid of last century (McCarthy et al. 1955 ), benefiting from Big Data, cheap computing power and storage, and improved algorithms, AI faces unseen opportunities nowadays. It is expected to largely change society through productivity-enhancing automation and job replacement. Footnote 2
In research, AI is considered a subarea of Computer Science (CS) (Shapiro 1992 ). Still, consensus lacks a single AI definition, as intelligence itself is not adequately defined (Legg and Hutter 2007 ; Rzepka and Berger 2018 ). Thus, there are numerous definitions of AI. The founders of AI gave the first definition as “ making a machine behave in ways that would be called intelligent if a human were so behaving ” (McCarthy et al. 1955 , p. 11). More recently, Russell et al. ( 2016 ) systematized eight currently discussed definitions by seeking human intelligence or rationality and focusing on either thinking or acting. Recent definitions emphasize intelligent agents interacting with their environment and pre-set goals (Russell et al. 2016 ). AI is also influenced by other disciplines (Shapiro 1992 ), e.g., mathematics, economics, and psychology (Russell et al. 2016 ).
Although there is a plethora of AI research (e.g., Abdel-Karim et al. 2021 ; Cao 2020 , 2022 ; Goodell et al. 2021 ; Martin 2019 ; Rai et al. 2019 ; Zheng et al. 2019 ), practical implementation lags due to the so-called black-box nature of most AI-based systems, thus missing to unlock the full potential of AI implementation. The black-box nature refers to a lack of explainability and interpretability of AI-based systems, primarily arising from the opacity of many of today's AI-based systems. Hence, the nature of inputs and outputs can be observed and understood chiefly, but not the exact processing steps in between—the black box. Accordingly, users or programmers of these systems cannot determine what influence which variable had on the decision or how this decision arose from the input variables. This is difficult for traceability, which is partly mandatory for regulatory purposes, but also the trust in such systems. XAI, a sub-class of AI systems, counteracts the general black-box nature of AI-based systems (Doran et al. 2017 ; Rosenfeld and Richardson 2019 ; Arrieta et al. 2019 ; Ciatto et al. 2020 ; Sanneman and Shah 2020 ; Verhagen et al. 2021 ).
2.2 Explainable Artificial Intelligence
In our work, we follow the definition of XAI given by Arrieta et al. ( 2019 ), that states as follows: “ Given an audience, an explainable Artificial Intelligence is one that produces details or reasons to make its functioning clear or easy to understand.” (Arrieta et al. 2019 , p. 6) . Thereby, we impose ease of understanding of AI for humans and use targeted explanation techniques such as XAI. In general, we can view explainability as a vital attribute of AI models, which indicates any procedure that intends to clarify its inner workings. This is predominantly known as understandability in the XAI literature and denotes specifically the model characteristics that help a human understand how the AI system works (Montavon et al. 2018 ). Additionally, this is tied to interpretability , which we can define as the ability to provide a model with meaning in understandable terms to humans through, e.g., transparency, if an AI model is by itself understandable. Thus, explainability is twofold, leading to model explainability, i.e., the ability of the system to reveal its inner workings, and human understandability, i.e., the capability of humans to understand the factors and the knowledge contained within an AI model. Employing XAI can lead to increased trust, e.g., by consumers and employees, and accountability in deployed AI models (Rai et al. 2019 ; Martin 2019 ; Elliott et al. 2021 ). Increased trust ultimately results in more widespread adoption of AI applications (Adadi and Berrada 2018 ; Janiesch et al. 2021 ; Mirbabaie et al. 2021 ; Sigrist and Hirnschall 2019 ). Especially in highly-regulated application domains or in environments with highly consequential decisions—like Finance, Healthcare, and Automotive—this requirement can be crucial for a successful application. Nevertheless, these industries are predestined for the application of AI systems due to the vast amounts of available data and automatable processes. In this vein, the need for XAI emerged, as it supports the evaluation and justification of AI systems (Zheng et al. 2019 ; Meske et al. 2022 ). Recent calls for, e.g., trustworthy (Thiebes et al. 2021 ) or sustainable AI (Bawack et al. 2022 ) underline the need for an exaggerated concept of AI.
Next to defining XAI, it is essential to reconvene various goals of explainability of AI evolved from the literature. In general, all these goals help to understand why the explainability of AI is needed and performed, especially when acting in a highly-regulated application domain such as Finance (Cao 2022 ). Thus, we follow an established nomenclature of XAI goals (Arrieta et al. 2019 ) and include the following nine goals: trustworthiness , causality , transferability , informativeness , confidence , fairness , accessibility , interactivity, and privacy awareness . These goals, presented in Table 1 , relate to what XAI aspires to compel.
Finally, it is crucial to inspect how we can achieve explainability. The first important distinction is between understandable models by design and so-called black-box models, which require external XAI techniques to enable human understanding. Thus, we differentiate between transparent models and post-hoc explanation techniques . Footnote 3
Transparent models must satisfy specific properties to be explainable to decision-makers. First, they are decomposable in that each part is fully explainable or interpretable by design, e.g., input parameters or computations. For instance, complex and non-interpretable input features would fail this criterion, making an AI model less understandable (Lou et al. 2012 ). Second, transparent models should satisfy algorithmic transparency, i.e., a decision-maker’s need to understand the process of the model to produce its output derived from its inputs. Thus, the decision-maker knows how the model would react in any given situation. Commonly used transparent models are linear/logistic regression, decision trees, k-nearest neighbors, rule-based learners, general additive models, or Bayesian models. 3 To give an example, decision trees consist of a hierarchical structure for decision-making, primarily supporting classification problems. Decision trees display different features and feature values on each tree level. Generally, they are easy to understand for a human decision-maker as they easily represent input features and the output variable. Figure 1 provides an exemplary decision tree for forecasting bank loans loss-given-default. The tree reflects a recovery horizon of 24 months and estimates the expected recovery rate (RR), depending on features like the loan size (Debt) and the age of the client’s relationship with the bank (AoR) measured in months.
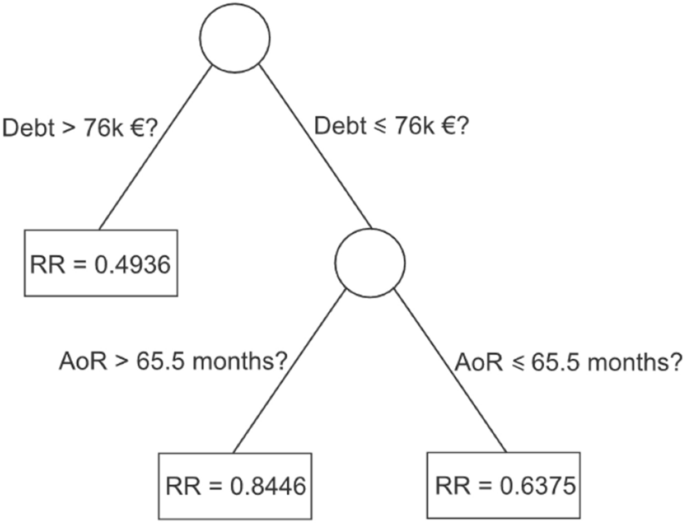
Example decision tree (Bastos 2010 , p. 2514)
When AI models do not satisfy the properties of transparent models, post-hoc explainability methods come into place. These methods provide interpretable information for decision-makers to understand the AI model’s process of producing its output for a given input. The literature (e.g., Arrieta et al. 2019 ) distinguishes between two different post-hoc explainability methods: model-agnostic and model-specific . 3 Model-agnostic methods can be applied to any given AI model. Such methods can be classified into three categories: explanation by simplification, feature relevance explanation, and visual explanation. One example of explanation by simplification is local explanation techniques such as Local Interpretable Model-Agnostic Explanations (LIME) and all its variants. LIME is a rule extraction technique and builds locally linear models around the prediction of the model that it tries to explain (Ribeiro et al. 2016 ). Other well-known model-agnostic techniques, such as Shapley Additive explanations (SHAP), fall within the feature relevance explanation. With SHAP, the authors offer a way to measure the influence of relevant input features by calculating a feature importance score for each particular prediction (Lundberg and Lee 2017 ). Although LIME and SHAP may be visualized, it is not their defining characteristic, leading to the third category: visualization techniques such as Individual Conditional Expectation (ICE) (Goldstein et al. 2015 ). ICE allows graphing the functional relationship between the predicted response and the feature for individual observations. For instance, Fernández ( 2020 ) predicts bank solvency in the United States and uses ICE plots for sensitivity analysis. Figure 2 shows the individual observations, plotting the mortgage rate on the x-axis and the bank solvency (measured as the level of regulatory capital among risk-weighted assets) on the y-axis. From here, one can deduce two breakpoints in solvency: With rising mortgage rates, there are two drops in bank solvency, one at mortgage rates of 5% and the other at about 8.5%.
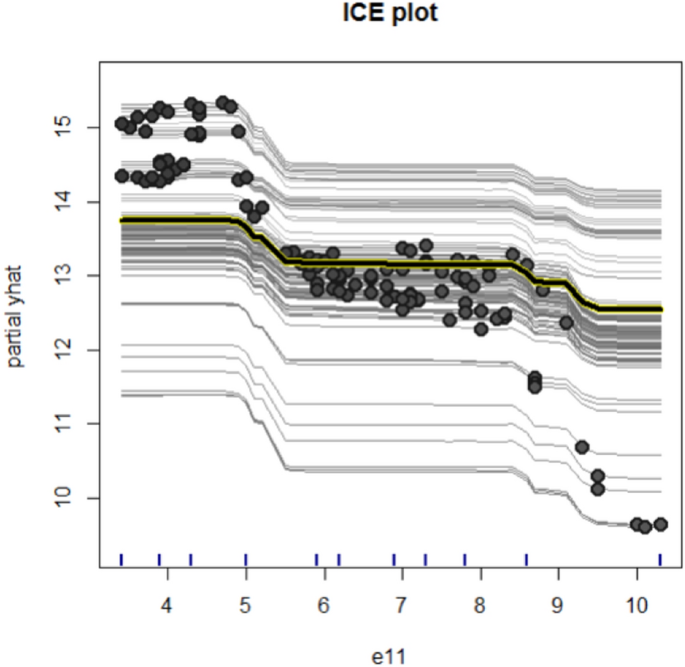
Example ICE plot (Fernández 2020 , p. 145)
These techniques help visualize the model parameters of any supervised AI algorithm. However, visualizations are less common model-agnostic explanation techniques since ensuring their applicability to any AI model’s inner structure is challenging.
Model-specific methods can only be applied to their respective category of AI models such as, e.g., ensembles and multiple classifier systems, support vector machines (SVMs), and neural networks. 3 For the latter, simplification techniques like DeepLIFT compute importance scores for multi-layer neural networks. With this approach, one can compare various neuron activations to a reference activation and assign a score based on the computed difference (Shrikumar et al. 2017 ).
2.3 Technological advances in Finance
Finance is a broad field of applications with very diverse requirements for technical systems and automation, mainly due to regulatory and legal requirements. Still, the domain continuously uses and adapts to new technological opportunities (Gimpel et al. 2018 ; Zheng et al. 2019 ), like AI and XAI. This phenomenon is also referred to as FinTech, i.e., financial technology. Previously, research emphasized the application of AI in Finance (e.g., Cao 2020 , 2022 ; Goodell et al. 2021 ; Zheng et al. 2019 ). Cao ( 2020 , 2022 ) evaluates the challenges of financial businesses and provides a comprehensive overview of solutions through classic and modern AI in Finance and economics. Goodell et al. ( 2021 ) present a holistic retrospection of the extant literature on AI application in Finance through co-citation and bibliometric-coupling analyses of 283 articles for the last three decades. They derive the following thematic clusters: financial distress and corporate failure, algorithmic and high-frequency trading, forecasting and predictive analysis, text mining and sentiment analysis, financial fraud, pricing and valuation, scheduling, and investor behavior and trade classification. Zheng et al. ( 2019 ) specifically analyze financial intelligence, which they define as FinTech 3.0, i.e., the third stage of technological advancements in Finance after computers (FinTech 1.0) and the internet (FinTech 2.0). Financial intelligence achieves “ intelligent and accurate calculation responsibility and (…) lead[s] the overall change in the financial industry ” (Zheng et al. 2019 , p. 914). They describe the AI key application areas of wealth management, risk management, financial security, financial consulting, and blockchain. While there is a considerable amount of publications aggregating research on AI in Finance, there is no paper reviewing the current state of research on XAI in Finance. Due to the high regulations applying to domains like Finance and practical requirements, e.g., for the transparency of decisions, XAI can play a decisive role in augmenting processes in such domains. Hence, it is necessary to discuss XAI related to the different application areas in Finance. Research divides Finance into several areas (e.g., Huang et al. 2020 ; Hentzen et al. 2021 ; Goodell et al. 2021 ). Particularly pivotal and commonly discussed for AI deployment are the Finance areas of risk management, stock market, portfolio optimization, anti-money laundering, and electronic financial transaction classification.
Risk management (e.g., default and bankruptcy prediction, fraud detection) is concerned with identifying, measuring, and controlling financial risks (Zheng et al. 2019 ). Financial institutions continuously perform it, and regulators require it (Adams and Hagras 2020 ). Default and bankruptcy prediction refers to a state of (corporate) failure (Sigrist and Hirnschall 2019 ). Default prediction is concerned with predicting the probability of debtors, e.g., credit card holders and financial institutions, to commit default using available information, e.g., profiles, loan history, and repayment history. Bankruptcy prediction deals with publicly available information and derives, e.g., accounting ratios to determine the likelihood of a company going bankrupt, a valuable information for potential investors and current creditors (Sigrist and Hirnschall 2019 ; Zheng et al. 2019 ). Fraud detection involves uncovering unauthorized (fraudulent) transactions on accounts (Jarovsky et al. 2018 ). AI methods support these endeavors by assessing numerous cases, e.g., credit card applications, or enormous amounts of available data, e.g., in the case of public companies, and suggesting possible actions, like approving or denying credit card applications or loans. XAI complements these suggestions with explanations to ensure they are discrimination-free and thoroughly founded, rendering the AI methods usable in the industry (Park et al. 2021 ). More precisely, in the case of default prediction, e.g., a gradient tree-boosted model may get employed (Sigrist and Hirnschall 2019 ). This model performs a binary classification by assigning loans to small and medium-sized enterprises into two categories: defaults and non-defaults. Among approximately 50 different predictor variables, this AI model uses the number of days of delay until repayment (delay days) to predict rare events, such as loan defaults. Here, the authors introduce two model-agnostic post-hoc explainability tools for the interpretation of the AI model (i.e., two XAI models): variable importance measures (feature relevance explanation) and partial dependence plots (visual explanation). Variable importance measures quantify and illustrate single variables’ importance for the prediction (Sigrist and Hirnschall 2019 ). Further, partial dependence plots (see Fig. 3 ) visualize the model by showing how a single variable influences the prediction outcome aggregated for several observations. For instance, plotting the age of the accounting data provided by a company (age accounting data) or the delay days against default probability illustrates the model. As Fig. 3 shows, a lower age accounting data and fewer delay days are related to a lower default probability. Additionally, the linear relationship in the case of age accounting data and the non-linear relationship in the case of delay days is recognizable. These illustrations help overcoming the black-box nature of AI-based systems.
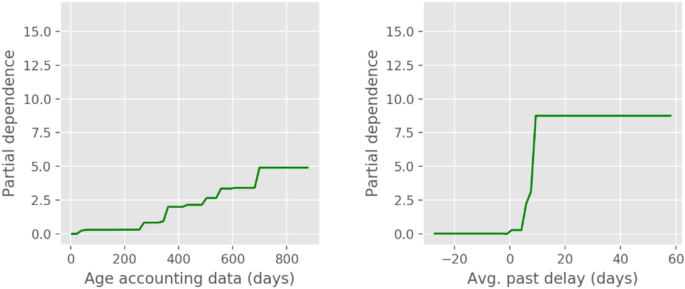
Example partial dependence plots (Sigrist and Hirnschall 2019 , p. 28)
Trading in the stock market benefits from AI usage, as the latter is expected to impede emotionally, and thus irrational, investment decisions and find patterns beyond human recognizability (Ferreira et al. 2021 ). Price prediction estimates the future value of a specific stock, option, or index. To achieve this, human and AI predictors use various input factors from within the stock market, e.g., past prices and trading volumes, and outside of it, e.g., press releases, annual reports, and social media (Ito et al. 2021 ). XAI supports this automated process by delivering explanations to highlight on which specific input variables investment decisions or price predictions are based. To give another example of the application of XAI from the area of trading in the stock market, we closer inspect technical analysts . They rely in their trading strategies on historical charts and heuristic approaches, trying to extrapolate future asset prices, as opposed to fundamentalists , who strictly base their trading behavior on asset prices derived from efficient market hypothesis’ fundamental values (Brock and Hommes 1998 ). Technical analysts’ behavior provides ground for applying reinforcement learning to retrieve the used “rules-of-thumb” (rule-base learners). This refers to determining “the amount of relevant historical information as well as the optimal parameters of the technical rules employed” (Bekiros 2010 , p. 1154), as a common problem discussed with the technical analysts’ approach is the lack thereof. Here, using AI that is by design interpretable, as its outcome consists of parameters of fuzzy inference rules (transparent XAI model), supports the cause of XAI (Bekiros 2010 ).
Portfolio optimization deals with asset allocation, which is considered one of the most relevant research fields in asset management (Zhang et al. 2020 a). It refers to finding a set of investment assets that fulfills the needs of a particular investor while usually simultaneously maximizing a particular goal (Ferreira et al. 2021 ). Advanced models allow investors to include their expectations and confidence level, while Big Data enables the incorporation of, e.g., market sentiments or other macroeconomic factors such as gross domestic product growth or inflation (Zhu et al. 2020 ). AI models help solve this complex problem by automatically deriving conclusions from these large amounts of data with multiple input variables. At the same time, XAI adds interpretability to these conclusions, e.g., a portfolio output, to narrow down the relevant factors for specific asset allocations.
Money laundering refers to techniques of “ hiding proceeds of crime ” (Levi and Reuter 2006 , p. 289). Anti-money laundering measures are, e.g., know-your-customer requirements that ensure that banks verify their customers' identity by checking government-issued IDs. The application of AI models may improve this process by handling large amounts of data and detecting anomalies, thus revealing potential money laundering cases. However, as money laundering suspicions can prevent customers from financial transactions, interpretability in the form of XAI for these automatically generated suspicions is needed (Kute et al. 2021 ). The mere results of an AI model are not sufficient in this case. Instead, explanations for the decisions are crucial.
Electronic financial transaction classification is concerned with classifying transactions that bank account holders perform into categories, e.g., groceries or transportation (Maree et al. 2020 ). While less regulated than others, this area is of pivotal importance for AI applications in Finance. Hence, we include this area in our consideration. Financial institutions may use this classification to present value-adding products to customers like digital financial advisors. Individuals’ electronic financial transactions are highly personal (Achituve et al. 2019 ). Thus, applications based on these transactions include the risk of privacy intrusions and discrimination. Misclassification of transactions could also impede the usability, and thus profitability, of a digital financial advisor, which determines the need for explainability.
To sum up, Finance is a highly-regulated application domain with a tremendous need for explanations when employing AI techniques (Zheng et al. 2019 ). Regulators and supervisors require banks to illuminate their activities when using AI (Burgt 2020 ; Weber et al. 2020 ; Adams and Hagras 2020 ), hence requiring the deployment of XAI models in specific application scenarios.
The conducted SLR aims to provide a comprehensive overview of previously dispersed research and a research agenda for XAI in Finance. This contributes to the goal of anchoring and facilitating XAI usage in the financial sector, thereby advancing theory and practice alike. In the following, we describe the methodology and employed search strategy for our SLR.
SLRs present the current state of research on a particular topic by aggregating prior research results. In this vein, SLRs also uncover less researched areas of the topic under investigation (e.g., Kitchenham et al. 2011 ; Okoli and Schabram 2010 ; Snyder 2019 ). Consequently, SLRs aim to aggregate the extracted research outcomes of previously conducted studies and their propositions (Okoli and Schabram 2010 ; Kitchenham et al. 2011 ). Thus, SLRs cluster the current state of research according to predefined criteria and thereby contribute to the conceptualization of the topic under investigation (Paré et al. 2015 ; Snyder 2019 ). Derived results of an SLR target researchers and practitioners (Kitchenham et al. 2011 ; van Aaken and Buchner 2020 ). Researchers benefit from a comprehensive overview of previous research and the developed future avenues for research, whereas practitioners can employ the derived overview as a guideline for their operations (Kitchenham et al. 2011 ; Snyder 2019 ).
We designed our SLR according to well-established guidelines in IS research, specifically those by Kitchenham ( 2004 ). By following these guidelines, we ensured a transparent and reproducible process, providing trustable, reliable, and rigorous research outcomes. Besides, we employed a detailed review protocol to ensure further transparency and reproducibility. The review protocol covered the whole process from conceptualization to the actual search and evaluation of results. It provided detailed information on the conducted search, inclusion and exclusion criteria, search strategy, and analysis procedure.
The initial search for articles employed three search term domains connected by the operator “AND” to ensure the fit with the topic under investigation. One domain aimed at explainability, one fostered AI implementation, and a third one ensured the applicability to the financial domain (see Table 2 ). This helped us to limit the number of retrieved articles and ensured the relevance of the derived studies. We searched the title and abstract for the chosen search terms to provide a broad set of relevant prior research. Nevertheless, an SLR does not aim at providing an exhaustive overview of prior research on a particular topic but rather guidance on the previously conducted research. Summing up, the search focused on publications addressing XAI in the financial context while excluding prior research emphasizing AI or other application scenarios (e.g., Healthcare, Automotive).
In order to do justice to the topic at hand, we included the top-ranked (A + and A) Finance journals according to the German Academic Association of Business Research (VHB) in the search. Further, we included the eight Information Systems (IS) basket journals (i.e., European Journal of Information Systems, Information Systems Journal, Information Systems Research, Journal of AIS, Journal of Information Technology, Journal of MIS, Journal of Strategic Information Systems, MIS Quarterly), and the top CS journals according to the Scimagor Journal Ranking. We included CS as a research area to capture a more technical angle to the topic and IS as research on the intersection of technology and practical implementation. Besides, the search process also covered several databases (i.e., ACM Digital Library, AIS eLibrary, IEEE Xplore) related to IS and CS research to provide an extensive overview of current research findings, thus following similar SLRs (e.g., Bouncken et al. 2015 ; Sageder et al. 2018 ; Malinova and Mendling 2021 ). We included peer-reviewed publications in leading international journals and conference proceedings to ensure high-quality standards. Besides, we limited the search to publications since 2010 to provide a more recent overview of current research in a rapidly changing environment of XAI research endeavors. The same search strategy (i.e., search terms and criteria) is independently applied to each outlet and database. The search took place from October 2021 to January 2022 to provide the most recent picture of current research, up to and including the year 2021. The initial search yielded 2,022 results. Upon excluding duplicates, the set consisted of 2,000 results. Figure 4 visualizes the steps of our SLR process and provides additional information on the particular quantities (i.e., initially, 545 results from Finance, 870 results from IS, and 607 results from CS).
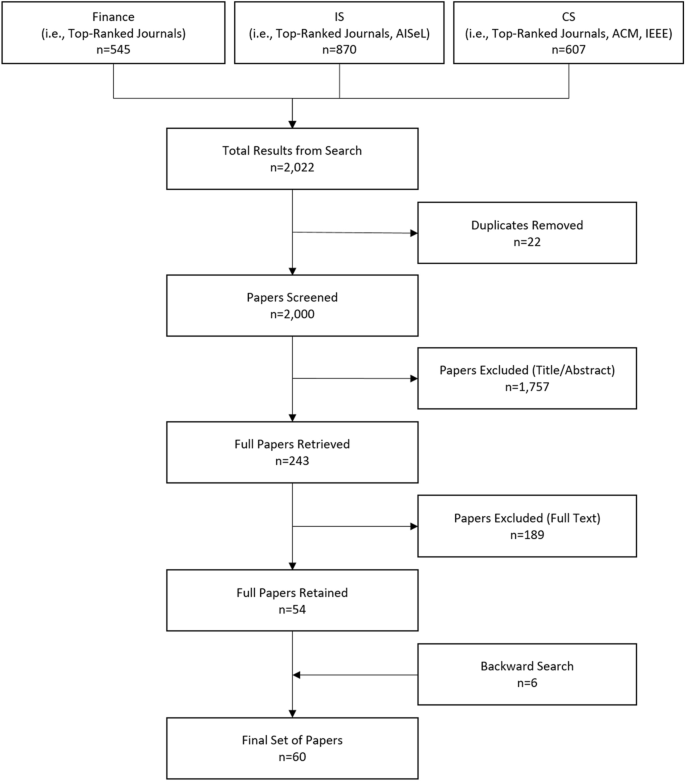
Visualization of the SLR process
The screening process employed a set of exclusion criteria to ensure the relevance of the results and the focus on the research goal. Besides publication date and focus on peer-reviewed outlets, we defined the following rigid exclusion criteria Footnote 4 to filter out irrelevant publications:
The paper deals with Finance but not with XAI.
The paper deals with XAI but not with Finance.
The paper deals with AI and Finance but not with XAI.
The screening process consisted of several steps. First, we checked the result set for relevance to the research project and the criteria utilizing their titles, abstracts, and keywords. In questionable cases, we also screened additional publication sections like the introduction and conclusion. We excluded all articles with a misfit concerning research goals and search criteria, in our case, 1,757 publications. This led to 243 publications relevant to the following screening step. Within the next step, we evaluated these publications in detail according to their full texts. Fifty-four publications finally matched the search criteria and research goals. The far-reaching search caused relatively high exclusion rates during the process. However, conducting a more comprehensive search was necessary to provide an overview of the intersection of XAI and Finance. After screening the derived publications for relevance, we browsed these retained publications for key publications (i.e., backward search). The backward search yielded another six relevant publications. Hence, the final set featured a total of 60 articles (see Appendix 1 for the complete list).
The last step of the SLR covered the coding of the final publication set. We coded according to publication details (i.e., publication, year, methodology) and the thematic focus and application (i.e., XAI method and goal, area of Finance). To the best of our knowledge, no suitable coding scheme is available. Thus, we developed a multiple classification-coding scheme (i.e., coders can assign one publication to several classifications). The developed coding scheme spanned a matrix that connects the XAI application and the area of Finance (according to the presented nomenclatures). However, the developed coding scheme should ensure an iterative, discursive process instead of being a rigid scheme. Three coders independently performed the coding, discussing possible deviations and settling them in mutual agreement.
4.1 General overview
We present the publication trend of XAI research in Finance in Fig. 5 . The graph shows the number of articles published in the respective year. Figure 5 indicates that XAI research in Finance was already relevant a decade ago, followed by a paucity around 2013 and 2014. We find the most articles for 2020 with n = 17 articles, followed by 2021 with the second-most n = 16 articles. Thus, the two recent years account for over 55% of the articles we found, indicating this research area to be of considerable growth. We assume this to manifest the current AI and XAI trend in research (Collins et al. 2021 ), e. g. due to rising transparency needs by policymakers, we also find in other domains regarding XAI (Wells and Bednarz 2021 ; Islam et al. 2022 ).
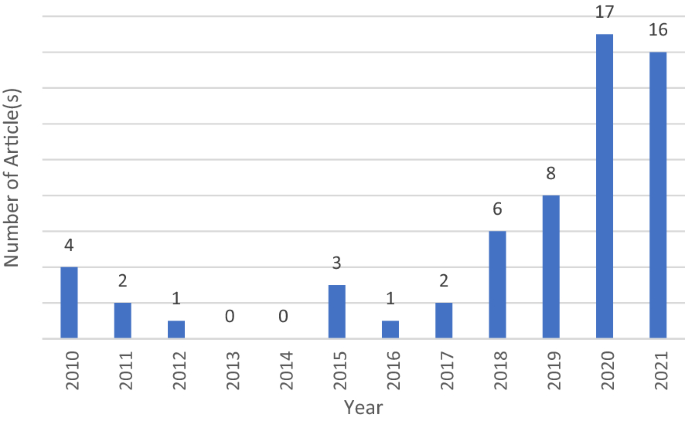
Publication trend of XAI research in Finance ( n = 60)
In Fig. 6 , we provide an overview of the methods used in XAI research in Finance. Footnote 5 The majority of the reviewed articles present theoretical models, which are subsequently applied to real market datasets ( n = 32). For instance, Liu et al. ( 2021 ) propose a logistic regression model empowered with cross-features generated through deep neural networks, which they apply to multiple public and business datasets from real-world credit risk assessment applications. The runner-up method is the experiment performed by n = 18 articles in our subset. For instance, Ghandar and Michalewicz ( 2011 ) experiment with multi-objective evolutionary algorithms for stock price prediction to pursue the XAI goals of informativeness and confidence. Least used are the methods of qualitative literature review and case study, which are only employed by two research teams each, e.g., a case study by Mayer et al. ( 2020 ), who conduct an in-depth case study of a large German bank implementing an AI system supporting credit risk decisions.
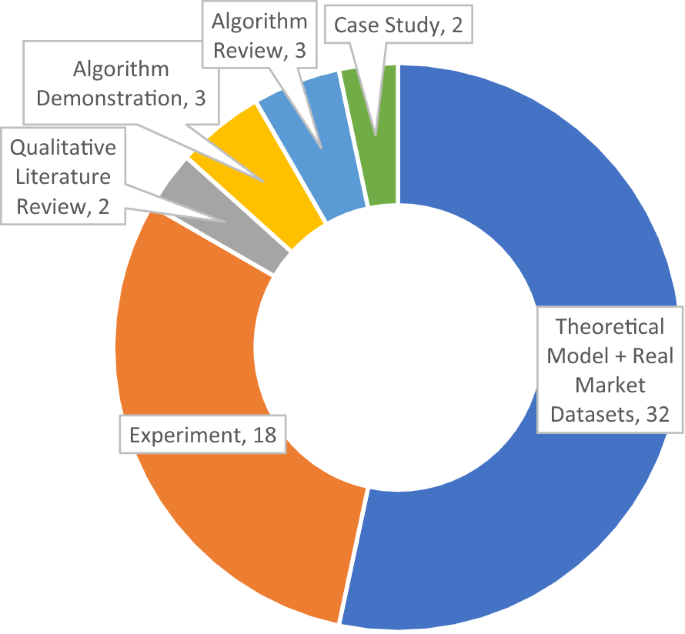
Methodological overview of XAI research in Finance ( n = 60)
We figure this focus on theoretical models with real market datasets and experiments to be an expression of the data-driven characteristic of AI and, subsequently, XAI. Most researchers employed either real or experiment-crafted datasets to present their XAI-related results in Finance. This adds to the practical applicability of the study at hand, as it shows Finance practitioners that XAI is already well-connected to, e.g., real market datasets and is thus more readily available for implementation in their specific Finance areas.
We find an imbalance in the disciplines from which the reviewed research articles originated. As Fig. 7 shows in the first bar, roughly a quarter ( n = 13) of the sample is published in Finance literature such as the Journal of Banking and Finance, while the majority ( n = 47) is based in the IS and CS disciplines with research outlets like Information Systems Research. This paucity of XAI research in Finance literature we found in our sample fosters further inspection. We conclude there is a further need for the Finance discipline to engage with research on XAI due to the specific requirements of this industry for such systems. Accordingly, it is insufficient if only more technically oriented disciplines, i.e., IS and CS, deal with it. Instead, the view of the application-specific discipline, i.e., Finance, is also valuable and essential. Besides, due to high regulatory requirements in this industry, law researchers should respond to law initiatives and laws such as the US FTA, the EU AIA, and the GDPR next to scientific calls for transparency in Finance (Vishwanath and Kaufmann 1999 ; Pasquale 2015 ; Weber et al. 2020 ) and deepen their research on XAI in regulated domains. We find a balanced distribution by looking at the publication form the authors in our subsample chose. Half of the articles were published in journals ( n = 28), while the other half were introduced at conferences ( n = 32) , as the second bar of Fig. 7 shows. This balance implies that XAI in Finance is an established research field future scientists might want to look at, as it is well-represented in both research trends capturing conferences and basic research advancing journals. In the lower bar of Fig. 7 , we can see the distribution of the rankings the respective publication outlets received. We adopt the rating provided by the VHB, ranking outlets from A + (best quality and impact) to D (lower quality and impact), considering all discipline ratings. Footnote 6 We find only one article with the highest rank A + , while a fifth of our subsample’s articles were published in outlets receiving the second-best rank, A ( n = 11). Interestingly, researchers publish most articles in journals or conference proceedings that received no rating (n.r.) by the VHB ( n = 45). This fact may show that XAI in Finance is yet predominantly concerned with an application point of view, thus, possibly neglecting the theory-building and development part of research that could further pave the way for realizing the full potential of XAI in Finance. Additionally, research is driven by non-management disciplines such as IS and CS.
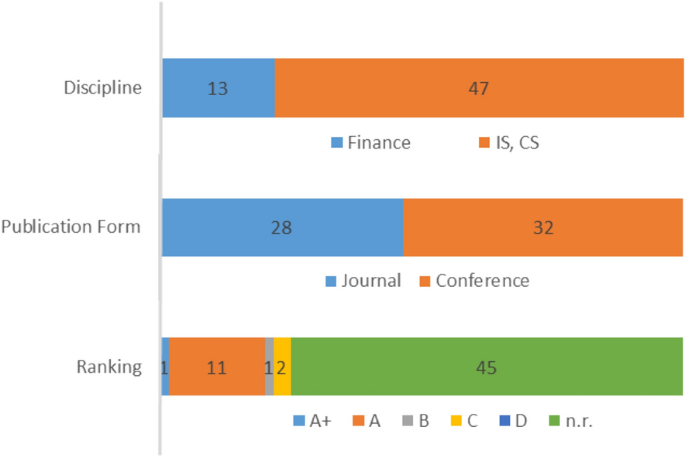
Overview of publication disciplines, forms, and ratings ( n = 60)
4.2 Overview concerning XAI goals and methods
We research which goals XAI in our subsample pursuits. For this purpose, our coding scheme grounds on the well-accepted nomenclature of XAI goals by Arrieta et al. ( 2019 ) and considers the nine goals of XAI mentioned above: trustworthiness , causality , transferability , informativeness , confidence , fairness , accessibility , interactivity, and privacy awareness . Table 3 provides an overview of the respective articles dealing with specific XAI goals. As the literature indicates (e.g., Arrieta et al. 2019 ), most XAI papers deal with the goal of informativeness, while the least deal with privacy awareness. We confirm these results for our sample of papers. Most of our sample ( n = 43) deals with informativeness, while only two articles target the XAI goal of privacy awareness. This paucity of research with the goal of privacy awareness is somewhat interesting, as laws, e.g., the GDPR, emphasize privacy and the right to informational self-determination, especially in industries like the financial sector. Future research has to unravel this mismatch and identify potential reasons for it.
Next to the goals of XAI, we take a closer look at the XAI methods used in the reviewed sample. As for the list of XAI goals, we base our coding of XAI methods on prior literature (e.g., Arrieta et al. 2019 ). We distinguish between transparent AI models, which are interpretable without any further additions, and so-called post-hoc explainability, which complements existing AI models to create or improve their interpretability. For the latter, there is model-agnostic explainability on the one side, providing explainability regardless of the model, and model-specific explainability on the other, which improves explainability for distinct AI models (Arrieta et al. 2019 ).
Table 4 provides an overview of the reviewed articles and their XAI methods. Multi-method usage is possible, while six papers do not employ methods (Ghandar and Michalewicz 2011 ; Renault 2017 ; Oppold and Herschel 2019 ; Koshiyama et al. 2020 ; Daníelsson et al. 2021 ; Ge et al. 2021 ), but only deal with XAI from a high-level perspective considering goals. Thus, they are not included in Table 4 but in Table 3 . The results indicate that roughly the same number of papers use transparent models ( n = 26) or introduce XAI in the form of post-hoc explainability ( n = 32). This balance represents researchers’ balanced preferences towards both kinds of XAI, transparent models next to post-hoc explainability, highlighting XAI in Finance as a well-recognized, established research stream. Additionally, we observe articles employing both kinds of XAI, thus comparing, e.g., neural networks and logistic regression (Adams and Hagras 2020 ), showing academia targeting both kinds of XAI individually and their combination and comparison. Decision trees are the most used method ( n = 12) when only looking at transparent models, while general additive and Bayesian models are not used. Related studies (e.g., Arrieta et al. 2019 ; Chou 2019 ) confirm decision trees to be well-employed in XAI research, but we find a different picture regarding general additive models. Relevant research concerning XAI in the form of general additive models in Finance (Berg 2007 ; Taylan et al. 2007 ; Calabrese 2012 ) did not appear in our search. This fact eventually hints at possible limitations of this study and sparks further research as to why there has been no research on general additive models in Finance in top-tiered outlets since 2010. Looking at methods including post-hoc explainability ( n = 37), we find roughly two-fifths being model-agnostic ( n = 16) and three-fifths being model-specific ( n = 21). Most model-specific methods deal with multi-layer neural networks ( n = 14), while least model-specific methods focus on recurrent neural networks ( n = 1) and none on SVMs. Among model-agnostic methods, an equal number of articles deal with explanation by simplification and feature relevance explanation ( n = 5).
In Fig. 8 , we provide an overview of the publication trend of XAI research in Finance grouped by explainability type. Research trends change over time regarding transparent models (blue) or post-hoc explainability (orange). Some papers employ both types of XAI, while others employ neither ( n = 6), focusing on goals instead (see Table 3 ). While up to 2018, except for 2012, there has been more, or at least as much, research applying transparent models compared to post-hoc explainability, this trend recently shifted. If we consider the research-intense years of 2019–2021, the volume of research and its distribution changed. Research employing post-hoc explainability surpassed the former more popular transparent models by far with, e.g., almost four times as many papers dealing with post-hoc explainability ( n = 11) than with transparent models ( n = 3) in 2021. This development is in line with the general development of AI-based systems, where complex AI methods such as deep neural networks are more frequently used for decision-making.
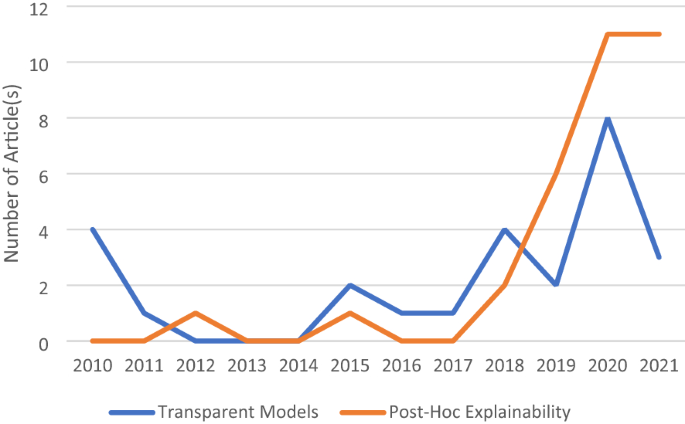
Publication trend of XAI research in Finance regarding transparent models and post-hoc explainability ( n = 60)
Table 5 gives an overview of XAI methods concerning their application areas in Finance. This overview allows us to indicate blind spots, deriving fruitful avenues for future research, especially from a Finance point of view. From Table 5 , we can infer underrepresented research areas in Finance, such as anti-money laundering. Up-and-coming scientists may evaluate the application of other XAI methods, e.g., rule-based learners or SVMs, to these areas to advance research and help practitioners employ AI models complemented with explainability. Of course, it is mandatory to investigate the absence of XAI methods applications in Finance areas derived from Table 5 . Some combinations might be less than others or not fruitful at all. For instance, new researchers undertaking projects in this space should take a closer look at the Finance area of electronic financial transaction classification and try to apply XAI methods such as logistic/linear regression or visual explanation. Thus, they will advance research by supplementing the current research landscape with new insights or providing evidence for the impossibility of such an XAI method and Finance area combination. Furthermore, we may spot areas of Finance, which are already well-researched, such as risk management and portfolio optimization. These areas may benefit from applying new yet unused XAI methods, which we do not list in our overview in Table 5 , or from comparing research between the methods mentioned above to acquire a broader perspective on the subject at hand, e.g., comparing transparent models and post-hoc explainability.
Furthermore, we discern that research tackled some, but not all, of the most pressing issues in Finance. Risk management is central to the Finance industry in many respects and essential for the successful continuation of a wide variety of agents. Hence, it is not surprising that prior research put much effort into researching the application of XAI in this Finance area due to its centrality to the domain (Adams and Hagras 2020 ). The same applies to the stock market and portfolio optimization, building blocks upon which the success of many agents in the domain of Finance grounds (Zhang et al. 2020 a). Besides, anti-money laundering is one of the most pressing issues threatening financial systems (Kute et al. 2021 ). However, research so far has only rarely tackled this issue, posing the question of why that is the case. Classifying electronic financial transactions has recently become a differentiator in customers' eyes. Therefore, agents in the financial domain renewed their interest in this area (Maree et al. 2020 ).
Hence, prior research focused on more critical and also predestinated Finance areas and evaluated the application of at least one XAI method per area. However, there is still an imbalance urging research attention in the future on the one hand concerning the application of more diverse XAI methods to several areas. On the other hand, future research should assess less urgent but still promising areas in Finance for possible application of XAI methods.
5 Conclusion and future research avenues
To exploit AI applications' full potential in Finance, XAI methods' employment seems promising. Using XAI instead of AI is decisive to ensure the necessary level of transparency and traceability required by regulatory and legal entities and achieve the trust needed for such systems to be deployed. However, research on XAI in Finance is highly dispersed over application areas and research methods (see Table 4 ). Frequently, researchers only investigated applying a particular XAI method to a specific use case. In this way, however, research takes place very isolated. This study provides an overview of previous research and aggregates prior research results to help exploit the full potential of XAI. Thus, we identify future research directions. To the best of our knowledge, there is no research reviewing the application of XAI in Finance on a broader level, albeit the necessity to implement XAI methods to ensure legally binding traceability and transparency of decisions made by the system in the financial industry. We are the first to provide an SLR and thus advance the fields of Finance, IS, and CS. This way, we contribute to conceptualizing the topic under investigation and answer respective calls for research. This paper aims to guide future research and to be a thorough approach to the subject. To achieve this goal, we reviewed relevant Finance, IS, and CS journals next to important databases, analyzing 60 articles in detail to acquire an overview of recent research (year 2010 to year 2021) dealing with this topic. We discuss our results by summarizing the implications for both researchers and practitioners. Finally, we present the limitations of our study and avenues for future research regarding XAI in Finance.
5.1 Theoretical implications
Our research contributes to academia’s emerging interest in XAI in Finance research (see Fig. 5 ). In this vein, we provide an easy-to-follow, low-threshold, comprehensive overview for interested scholars willing to get acquainted with XAI in Finance. We provide basics of XAI method application in Finance, next to areas of Finance where XAI employment is likely fruitful and pressing. Finally, we present research trends and cluster research and discuss the combinations of XAI methods and areas of Finance existing research focused. Due to the recent increase in publications, a reasonable amount of overlap between specific research streams emerged. In a perfect world, these scientists consolidate their work and progress together, rather than in parallel, into the future.
Our research offers broad guidance for scientists to understand the growing emphasis on XAI in Finance research, which we also found in other domains (Wells and Bednarz 2021 ; Islam et al. 2022 ). When looking at the disciplines that focused on XAI in Finance, we found a minority of research rooted in the Finance discipline. Thus, we especially encourage Finance researchers to evaluate how XAI may benefit their field of application. As most articles were published in outlets without a rating by VHB, we conclude that research predominantly took an application point of view, thus, possibly, neglecting the theory-building and development part of research. We highly encourage XAI and Finance researchers to consider engaging in theory building and development regarding XAI in Finance in high-ranked outlets.
When taking a closer look at the goals these papers try to achieve with the application of XAI, we notice an imbalance already highlighted by prior research (e.g., Arrieta et al. 2019 ). The reviewed articles mainly focus on the goals of informativeness and confidence but rather neglect the goals of, e.g., privacy awareness, accessibility, and causality. Hence, research pursuing these goals might be a fruitful avenue for future research, especially as laws, e.g., the GDPR, emphasize privacy and the right to informational self-determination. Future research has to unravel this mismatch and identify potential reasons for it.
Besides, theorists may benefit from our study employing it as an overview of current research on XAI and especially in Finance. While there is research dealing with AI in IS and CS (e.g., Abdel-Karim et al. 2021 ; Martin 2019 ; Rai et al. 2019 ) or Finance (e.g., Cao 2020 , 2022 ; Goodell et al. 2021 ; Zheng et al. 2019 ) or XAI in general (e.g., Arrieta et al. 2019 ; Ciatto et al. 2020 ; Doran et al. 2017 ; Rosenfeld and Richardson 2019 ; Sanneman and Shah 2020 ; Verhagen et al. 2021 ), so far, there is no paper reviewing the current state of research on XAI in Finance in leading international journals and conferences. Scientists looking for the distribution of a specific XAI method in Finance find answers in our provided results. For instance, they may spot decision trees and multi-layer neural networks as well-researched XAI method applications in Finance or find an indication for the underrepresentation of, e.g., Bayesian models. Additionally, we provide interested researchers with a set of studies employing both kinds of XAI, transparent models next to post-hoc explainability, in the area of Finance, thus highlighting these comparing works.
With our overview of XAI methods and areas of Finance, we identified blind spots for future research. We provide a summary of XAI methods, which are less researched than others. Future researchers could focus on these underrepresented areas, e.g., k-nearest neighbors or SVMs, to broaden our understanding of applicable XAI methods in Finance, albeit having to investigate the reason for this research paucity first. Similarly, we point out fewer explored still pressing areas of Finance that could benefit from additional research on XAI method applicability to provide a better understanding of (potential) XAI method employment in Finance, e.g., anti-money laundering. Besides, new researchers undertaking projects should take a closer look at the Finance area of electronic financial transaction classification and try to apply XAI methods such as logistic/linear regression or visual explanation. Thus, they will advance research by supplementing the current research landscape with new insights or providing evidence for the impossibility of such an XAI method and Finance area combination. XAI applications in the Finance area of risk management, especially default and bankruptcy prediction, deserve further research, as financial institutions perform them continuously due to regulatory requirements (Adams and Hagras 2020 ). In addition, future research should evaluate less pressing Finance areas for the potential application of XAI in future. Still, applying XAI models to less pressing and therefore less present Finance areas could be rewarding for the domain concerning a more comprehensive understanding.
When analyzing the contributions of the final set of papers, most models can visibly outperform current industry practices and offer transparency and traceability of decisions, hence fulfilling the requirements for automating processes in Finance. Nevertheless, very few papers deal with whether the developed or applied methods and models actually meet regulatory requirements and can thus be implemented in practice (except for, e.g., Park et al. 2021 ). Especially in a highly-regulated application domain like Finance with legal requirements for using AI-based systems in practice, such an assessment should be part of the individual evaluations. Accordingly, this represents an important future research endeavor to examine and consider compliance with existing laws and regulations during the development and testing of methodologies. Summing up, we provide a research agenda for future XAI research in Finance incorporating different approaches to the issue.
Finally, our research results also emphasize the need for XAI compared to mere AI deployment in Finance. However, one of the problems targeted by XAI is not new to research. One may also interpret XAI as a means of information asymmetry reduction. Information asymmetry refers to a state where a party has less or other information than their counterpart in a (planned) mutual relationship or contract (Akerlof 1970 ). AI implementation might create such a situation between the AI user and the AI tool when the user knows less or nothing about the inner mechanisms of an applied AI tool. XAI may educate the user with such knowledge, thus helping reduce the aforementioned information asymmetry. In Finance, this helps increase trust and understandability of AI systems, ultimately leading to higher adoption rates. Currently, multiple XAI methods apply to areas of Finance such as risk management and the stock market. Hence, we conclude higher relevance of information asymmetries and needs for information asymmetry reduction in these areas.
5.2 Managerial implications
Interested readers from business practice may also thrive by consulting our study. Our review provides an easy-to-follow, low-threshold, comprehensive overview for interested practitioners willing to get acquainted with XAI in Finance. Legislators, regulators, and Finance managers seeking to educate themselves benefit from our research, as we provide the basics of XAI methods employed in Finance. Additionally, we identify application areas in Finance where XAI employment is likely to be fruitful. We furthermore present research trends and cluster existing research, next to discussing the combinations of XAI methods and areas of Finance researched so far. As academia preferred the methods of real market datasets and experiments, it adds to this study’s practical applicability, showing practitioners that XAI is already well-connected to, e.g., real market datasets and thus more readily available for implementation.
Research on XAI in Finance is dispersed and thus makes it difficult for practitioners to get an overview of possibly suitable XAI methods for a specific application problem or to fathom possible areas of application for XAI methods. Our study maps XAI methods and areas of Finance, thus providing easy access to the deployment of XAI methods in practice. Hence, Finance managers planning to adopt XAI might consider our work an initial overview. Looking for existing XAI applications in their specific Finance areas, they may consult our study to learn about ready-to-employ solutions to add transparency and explainability to their yet black-box nature AI-based systems or not-yet automated processes. Alternatively, existing knowledge in the company about specific XAI models can be used, and possible application scenarios can be identified.
Accordingly, practitioners can use our study as a concrete guideline on how XAI can be implemented in Finance and thus gain a quick overview. This study aggregates yet scattered research on XAI in Finance to provide more copious and easier access to XAI in Finance, and thus brings us one step closer to unlocking the full potential of such systems in this industrial sector. In addition to providing general ideas for implementing XAI systems, the paper helps as a tangible overview of which financial sectors already have solutions that managers can consider in practice. In this vein, this study should anchor XAI usage in Finance.
Currently, practitioners lack concrete guidance on the legally compliant application of AI and XAI methods in Finance (Weber et al. 2020 ). Due to the highly-regulated application domain of Finance and its specific requirements for transparency and traceability of decisions and processes, practitioners face a very complex decision-making situation when they want to implement such methods in practice. Accordingly, this paper supports them in identifying already acceptable methods from a regulatory point of view, especially by motivating future research in this field of application. For instance, they could inspect the literature we presented and filter for works employing the method of case studies in order to observe what kinds of XAI were already applied in real Finance business cases.
Moreover, and most importantly, this study supports policymakers in establishing more specific regulations and prerequisites for using AI-based systems in Finance. As we showed in Fig. 8 , research regarding XAI in Finance recently shifted from mainly incorporating explainability by design (transparent models) to mainly applying explanations through external XAI techniques (post-hoc explainability). Legislators and regulators may want to respond to this trend by designing laws and regulatory requirements concerning this type of XAI techniques. For instance, post-hoc explainability methods are independent of the AI models they explain, which might require a distinct legislative approach for the former than for the latter.
Finally, practitioners may infer current or future trends in XAI in Finance research to prepare their businesses for future challenges. Practitioners may employ our overview as a guideline for their yet-to-come operations. For instance, they could enhance their employees’ technical skills by educating them about XAI methods from our aggregated research endeavors, e.g., decision trees, a rather widely applied XAI method in Finance, or ensembles and multiple classifier systems, a yet relatively rarely used XAI method in Finance.
5.3 Future research
The results reveal several further research avenues worth evaluating: We highly encourage future scientists to further enrich the research on XAI application in Finance. More precisely, forthcoming researchers should focus on underrepresented areas
of XAI goals, i.e., causality, accessibility, and privacy awareness,
of XAI method employment, i.e., k-nearest neighbors, general additive models, Bayesian models, ensembles and multiple classifier systems, SVMs, recurrent neural networks, and the comparison of transparent models and post-hoc explainability, and
of Finance, i.e., anti-money laundering and electronic financial transaction classification as well as less pressing Finance areas,
and especially their various combinations. It is essential and beneficial to have a broad understanding of XAI in Finance regarding specific goals, methods, and areas. Nevertheless, a broad understanding of the subject is important and should be a step for future research. Goals that have not yet been researched more intensively in the context of XAI in Finance nevertheless represent important fields of action for this domain. Particularly in Finance—and the correspondingly sensitive data—e.g., privacy awareness is of decisive importance and may even be required by regulation. There is a plethora of XAI methods applicable to the domain of Finance. However, most research focuses on only a few of these methods. Still, future research should exploit the versatility of established XAI methods. Only in this way can the potential of different methodologies be evaluated and thus also exploited. In addition, this study only examined Finance areas pressing and predestined for applying XAI in Finance or even requiring explainability. Accordingly, it makes sense to extend the focus of previous research to the yet understudies pressing areas as well as further Finance areas not yet that present in the scholarly discussion.
To ensure the practical relevance of research, researchers should focus on regulatory compliance of existing and new XAI methods in Finance. Only in this way can one exploit the potential of XAI in Finance. The practical use of such systems is highly regulated. Therefore, compliance is crucial for such systems' practical relevance. So far, there is hardly any discussion of the results in the context of regulatory compliance—a gap researchers should close with future studies.
Despite our best efforts, the findings and implications of our SLR are not without limitations, thus motivating future research. Although we tried to base our literature search on a broad variety of databases, there is still the possibility of researching more outlets, varying keywords, and including a broader publication date range to receive an even richer set of results (e.g., Berg 2007 ; Taylan et al. 2007 ). Even then, an exhaustive SLR remains impossible. We focused on recent research from the last decade to retrieve articles relevant to contemporary researchers and practitioners. Nevertheless, broadening and updating the SLR over time is an important avenue for future research.
The focus on XAI application in specific Finance areas may impose another limitation. However, this paper emphasized once again that even a highly-regulated application domain, such as the financial industry, does not represent a uniform field of application but rather many different areas with different requirements belonging to this industry. Accordingly, our research should motivate future scientists to perform similar analyses of the applicability of XAI methods in other areas of Finance and industries, including Healthcare and Automotive, and thus pave the way for a further spread of XAI in the most diverse industries with different requirements.
In conclusion, our study contributes to literature and practice by providing an easy-to-follow, low-threshold, comprehensive overview of previous works and a research agenda for future XAI research in Finance.
Data availability
The data sets used in our publication are not publicly available. Still, interested readers may get access to the data sets used for coding by contacting the authors.
Note, that this does not decrease regulatory compliance requirements (e.g., transparency, accountability) themselves. The decrease affects the burdens imposed by the requirements on the private sector through harmonization and standardized business reporting.
For further information see Russell et al. ( 2016 ).
For further information and an explanation of each of the models see Arrieta et al. ( 2019 ).
We applied these criteria in each of the screening steps to ensure the consistent pursuing of the research goal.
We chose to include qualitative literature reviews in our sample, as it presents a broader picture of the relevant literature. Also, in this, we follow other SLRs (e.g., Bouncken et al. 2015 ; Sageder et al. 2018 ; Malinova and Mendling 2021 ).
https://vhbonline.org/en/vhb4you/vhb-jourqual/vhb-jourqual-3/complete-list .
Abdel-Karim BM, Pfeuffer N, Hinz O (2021) Machine learning in information systems-a bibliographic review and open research issues. Electron Mark 31:643–670. https://doi.org/10.1007/s12525-021-00459-2
Article Google Scholar
Achituve I, Kraus S, Goldberger J (2019) Interpretable online banking fraud detection based on hierarchical attention mechanism. In: 2019 IEEE 29th international workshop on machine learning for signal processing (MLSP), pp 1–6. https://doi.org/10.1109/MLSP.2019.8918896
Adadi A, Berrada M (2018) Peeking inside the black-box: a survey on explainable artificial intelligence (XAI). IEEE Access 6:52138–52160. https://doi.org/10.1109/ACCESS.2018.2870052
Adams J, Hagras H (2020) A type-2 fuzzy logic approach to explainable AI for regulatory compliance, fair customer outcomes and market stability in the global financial sector. In: 2020 IEEE international conference on fuzzy systems (FUZZ-IEEE), pp 1–8. https://doi.org/10.1109/FUZZ48607.2020.9177542
Akerlof GA (1970) Quality uncertainty and the market mechanism. Quart J Econ 84:488–500
Alam TM, Shaukat K, Hameed IA et al (2020) An investigation of credit card default prediction in the imbalanced datasets. IEEE Access 8:201173–201198. https://doi.org/10.1109/ACCESS.2020.3033784
Alt R, Beck R, Smits MT (2018) FinTech and the transformation of the financial industry. Electron Mark 28:235–243. https://doi.org/10.1007/s12525-018-0310-9
Ariza-Garzón MJ, Arroyo J, Caparrini A, Segovia-Vargas M-J (2020) Explainability of a machine learning granting scoring model in peer-to-peer lending. IEEE Access 8:64873–64890. https://doi.org/10.1109/ACCESS.2020.2984412
Arrieta AB, Díaz-Rodríguez N, Del Ser J, et al. (2019) Explainable artificial intelligence (XAI): concepts, taxonomies, opportunities and challenges toward responsible AI. arXiv:191010045 [cs]. https://doi.org/10.1016/j.inffus.2019.12.012
Attanasio G, Cagliero L, Baralis E (2020) Leveraging the explainability of associative classifiers to support quantitative stock trading. In: Proceedings of the sixth international workshop on data science for macro-modeling. ACM, New York, NY, USA, pp 1–6. https://doi.org/10.1145/3401832.3402679
BaFin (2018) Big Data trifft auf künstliche Intelligenz. https://www.bafin.de/SharedDocs/Downloads/DE/dl_bdai_studie.html
Bastos JA (2010) Forecasting bank loans loss-given-default. J Bank Finance 34:2510–2517. https://doi.org/10.1016/j.jbankfin.2010.04.011
Bawack RE, Wamba SF, Carillo KDA, Akter S (2022) Artificial intelligence in e-commerce: a bibliometric study and literature review. Electron Mark 32: 297–338. https://doi.org/10.1007/s12525-022-00537-z
Bekiros SD (2010) Heterogeneous trading strategies with adaptive fuzzy actor-critic reinforcement learning: a behavioral approach. J Econom Dyn Control 34:1153–1170. https://doi.org/10.1016/j.jedc.2010.01.015
Berg D (2007) Bankruptcy prediction by generalized additive models. Appl Stoch Mod Bus Ind 23:129–143. https://doi.org/10.1002/asmb.658
Bichler M, Gupta A, Ketter W (2010) Research commentary—designing smart markets. Inf Syst Res 21:688–699. https://doi.org/10.1287/isre.1100.0316
Bouncken RB, Gast J, Kraus S, Bogers M (2015) Coopetition: a systematic review, synthesis, and future research directions. Rev Manag Sci 9:577–601. https://doi.org/10.1007/s11846-015-0168-6
Brock WA, Hommes CH (1998) Heterogeneous beliefs and routes to chaos in a simple asset pricing model. J Econ Dyn Control 22:1235–1274. https://doi.org/10.1016/S0165-1889(98)00011-6
Butaru F, Chen Q, Clark B et al (2016) Risk and risk management in the credit card industry. J Bank Finance 72:218–239. https://doi.org/10.1016/j.jbankfin.2016.07.015
Calabrese R (2012) Estimating bank loans loss given default by generalized additive models. UCD Geary Institute Discussion Paper Series, WP2012/24
Cao L (2022) AI in finance: challenges, techniques, and opportunities. ACM Comput Surv 55:1–38. https://doi.org/10.1145/3502289
Cao L (2020) AI in finance: a review. SSRN, Rochester, NY. https://doi.org/10.2139/ssrn.3647625
Carta SM, Consoli S, Piras L et al (2021) Explainable machine learning exploiting news and domain-specific lexicon for stock market forecasting. IEEE Access 9:30193–30205. https://doi.org/10.1109/ACCESS.2021.3059960
Chen W, Liu Y, Xiang G et al (2012) A three-stage data mining model for reject inference. In: 2012 fifth international conference on business intelligence and financial engineering, pp 34–38. https://doi.org/10.1109/BIFE.2012.15
Cheong M-S, Wu M-C, Huang S-H (2021) Interpretable stock anomaly detection based on spatio-temporal relation networks with genetic algorithm. IEEE Access 9:68302–68319. https://doi.org/10.1109/ACCESS.2021.3077067
Chou T-N (2019) An explainable hybrid model for bankruptcy prediction based on the decision tree and deep neural network. In: 2019 IEEE 2nd international conference on knowledge innovation and invention (ICKII), pp 122–125. https://doi.org/10.1109/ICKII46306.2019.9042639
Ciatto G, Schumacher MI, Omicini A, Calvaresi D (2020) Agent-based explanations in AI: towards an abstract framework. In: International workshop on explainable, transparent autonomous Agents and multi-agent systems. Springer, pp 3–20. https://doi.org/10.1007/978-3-030-51924-7_1
Collins C, Dennehy D, Conboy K, Mikalef P (2021) Artificial Intelligence in information systems research: a systematic literature review and research agenda. Int J Inf Manag 60:102383. https://doi.org/10.1016/j.ijinfomgt.2021.102383
European Commission (2021) Proposal for a regulation of the european parliament and of the council laying down harmonised rules on artificial intelligence (Artificial intelligence act) and amending certain union legislative acts
Cong L, Tang K, Wang J, Zhang Y (2020) AlphaPortfolio for investment and economically interpretable AI. SSRN Journal. https://doi.org/10.2139/ssrn.3554486
Daníelsson J, Macrae R, Uthemann A (2021) Artificial intelligence and systemic risk. J Bank Finance. https://doi.org/10.1016/j.jbankfin.2021.106290
Dastile X, Celik T (2021) Making deep learning-based predictions for credit scoring explainable. IEEE Access 9:50426–50440. https://doi.org/10.1109/ACCESS.2021.3068854
Dattachaudhuri A, Biswas S, Sarkar S et al (2020a) Transparent neural based expert system for credit risk (TNESCR): an automated credit risk evaluation system. In: 2020 international conference on computational performance evaluation (ComPE), pp 13–17. https://doi.org/10.1109/ComPE49325.2020.9199998
Dattachaudhuri A, Biswas S, Sarkar S, Boruah AN (2020b) Transparent decision support system for credit risk evaluation: an automated credit approval system. In: 2020 IEEE-HYDCON, pp 1–5. https://doi.org/10.1109/HYDCON48903.2020.9242905
Doran D, Schulz S, Besold TR (2017) What does explainable AI really mean? A new conceptualization of perspectives. arXiv preprint arXiv:171000794. https://doi.org/10.48550/arXiv.1710.00794
Duan J, Zeng J (2015) Forecasting stock return using multiple information sources based on rules extraction. In: 2015 12th international conference on fuzzy systems and knowledge discovery (FSKD), pp 1183–1188. https://doi.org/10.1109/FSKD.2015.7382110
Elliott K, Price R, Shaw P et al (2021) Towards an equitable digital society: artificial intelligence (AI) and corporate digital responsibility (CDR). Society 58:179–188. https://doi.org/10.1007/s12115-021-00594-8
Ferdaus MM, Chakrabortty RK, Ryan MJ (2021) Multiobjective automated type-2 parsimonious learning machine to forecast time-varying stock indices online. IEEE Trans Syst Man Cybern Syst. https://doi.org/10.1109/TSMC.2021.3061389
Fernández JAF (2020) United States banking stability: an explanation through machine learning. Banks Bank Syst 15:137–149
Ferreira FG, Gandomi AH, Cardoso RT (2021) Artificial intelligence applied to stock market trading: a review. IEEE Access 9:30898–30917. https://doi.org/10.1109/ACCESS.2021.3058133
Ge R, Zheng Z, Tian X, Liao L (2021) Human-robot interaction: when investors adjust the usage of robo-advisors in peer-to-peer lending. Inf Syst Res 32:774–785. https://doi.org/10.1287/isre.2021.1009
Ghandar A, Michalewicz Z (2011) An experimental study of multi-objective evolutionary algorithms for balancing interpretability and accuracy in fuzzy rulebase classifiers for financial prediction. In: 2011 IEEE symposium on computational intelligence for financial engineering and economics (CIFEr), pp 1–6. https://doi.org/10.1109/CIFER.2011.5953570
Gimpel H, Rau D, Röglinger M (2018) Understanding fintech start-ups–a taxonomy of consumer-oriented service offerings. Electron Mark 28:245–264. https://doi.org/10.1007/s12525-017-0275-0
Goldstein A, Kapelner A, Bleich J, Pitkin E (2015) Peeking inside the black box: visualizing statistical learning with plots of individual conditional expectation. J Comp Graphical Stat 24:44–65. https://doi.org/10.1080/10618600.2014.907095
Goodell JW, Kumar S, Lim WM, Pattnaik D (2021) Artificial intelligence and machine learning in finance: identifying foundations, themes, and research clusters from bibliometric analysis. J Behav Exp Finance 32:100577. https://doi.org/10.1016/j.jbef.2021.100577
Guo M, Pan X, Song S et al (2021) Deep convolutional fuzzy systems of stock value prediction based on AFS theory. In: 2021 40th chinese control conference (CCC), pp 2448–2453. https://doi.org/10.23919/CCC52363.2021.9550275
Hayashi Y, Tanaka Y, Yukita S et al (2015) Three-MLP Ensemble Re-RX algorithm and recent classifiers for credit-risk evaluation. In: 2015 international joint conference on neural networks (IJCNN), pp 1–8. https://doi.org/10.1109/IJCNN.2015.7280388
Hentzen JK, Hoffmann A, Dolan R, Pala E (2021) Artificial intelligence in customer-facing financial services: a systematic literature review and agenda for future research. Int J Bank Mark 40:1299–1336. https://doi.org/10.1108/IJBM-09-2021-0417
Hoofnagle CJ (2013) How the fair credit reporting act regulates big data. SSRN, Rochester, NY
Horel E, Mison V, Xiong T et al. (2018) Sensitivity based neural networks explanations. arXiv:181201029 [cs, stat]. https://doi.org/10.48550/arXiv.1812.01029
Huang J, Chai J, Cho S (2020) Deep learning in finance and banking: a literature review and classification. Frontiers Bus Res China 14:13. https://doi.org/10.1186/s11782-020-00082-6
IEEE (2021) IEEE finance playbook version 1.0 trusted data and artificial intelligence systems (AIS) for financial services
Islam MR, Ahmed MU, Barua S, Begum S (2022) A systematic review of explainable artificial Intelligence in terms of different application domains and tasks. Appl Sci 12:1353. https://doi.org/10.3390/app12031353
Ito K, Minami K, Imajo K, Nakagawa K (2021) Trader-company method: a metaheuristics for interpretable stock price prediction. In: Proceedings of the 20th international conference on autonomous agents and multiagent systems. International foundation for autonomous agents and multiagent systems, Richland, SC, pp 656–664. https://doi.org/10.48550/arXiv.2012.10215
Janiesch C, Zschech P, Heinrich K (2021) Machine learning and deep learning. Electron Mark 31:685–695. https://doi.org/10.1007/s12525-021-00475-2
Jarovsky A, Milo T, Novgorodov S, Tan W-C (2018) GOLDRUSH: rule sharing system for fraud detection. Proc VLDB Endow 11:1998–2001. https://doi.org/10.14778/3229863.3236244
Kalyanathaya KP (2022) A literature review and research agenda on explainable artificial intelligence (XAI). SSRN, Rochester, NY
Book Google Scholar
Kamalloo E, Abadeh MS (2010) An artificial immune system for extracting fuzzy rules in credit scoring. In: IEEE congress on evolutionary computation, pp 1–8. https://doi.org/10.1109/CEC.2010.5586346
Khandani AE, Kim AJ, Lo AW (2010) Consumer credit-risk models via machine-learning algorithms. J Bank Finance 34:2767–2787. https://doi.org/10.1016/j.jbankfin.2010.06.001
Kim J, Bae JK, Delen D (2011) Understading black boxes: knowledge induction from models. PACIS 2011 Proceedings
Kitchenham B, Budgen D, Brereton OP (2011) Using mapping studies as the basis for further research–a participant-observer case study. Inf Softw Technol 53:638–651. https://doi.org/10.1016/j.infsof.2010.12.011
Kitchenham B (2004) Procedures for performing systematic reviews. computer science department, Keele University (TR/SE-0401) and national ICT Australia Ltd. (0400011T.1)
Kong K, Liu R, Zhang Y, Chen Y (2020) Predicting liquidity ratio of mutual funds via ensemble learning. In: 2020 IEEE international conference on big data (Big Data), pp 5441–5450. https://doi.org/10.1109/BigData50022.2020.9378486
Koshiyama A, Firoozye N, Treleaven P (2020) Algorithms in future capital markets: a survey on AI, ML and associated algorithms in capital markets. In: Proceedings of the first ACM international conference on AI in finance. ACM, New York, NY, USA, pp 1–8. https://doi.org/10.1145/3383455.3422539
Kraus M, Feuerriegel S, Oztekin A (2020) Deep learning in business analytics and operations research: models, applications and managerial implications. Eur J Oper Res 281:628–641. https://doi.org/10.1016/j.ejor.2019.09.018
Kute DV, Pradhan B, Shukla N, Alamri A (2021) Deep learning and explainable artificial intelligence techniques applied for detecting money laundering–a critical review. IEEE Access 9:82300–82317. https://doi.org/10.1109/ACCESS.2021.3086230
Lee J, Youn HL, Stevens N et al (2021) FedNLP: An interpretable NLP system to decode federal reserve communications. In: Proceedings of the 44th international ACM SIGIR conference on research and development in information retrieval. ACM, New York, NY, USA, pp 2560–2564. https://doi.org/10.1145/3404835.3462785
Legg S, Hutter M (2007) Universal intelligence: a definition of machine intelligence. Mind Mach 17:391–444. https://doi.org/10.1007/s11023-007-9079-x
Levi M, Reuter P (2006) Money laundering. Crime Justice 34:289–375. https://doi.org/10.1086/501508
Li Z, Liu G, Wang S et al (2018) Credit card fraud detection via kernel-based supervised hashing. In: 2018 IEEE smartworld, ubiquitous intelligence computing, advanced trusted computing, scalable computing communications, cloud big data computing, internet of people and smart city innovation (SmartWorld/SCALCOM/UIC/ATC/CBDCom/IOP/SCI), pp 1249–1254. https://doi.org/10.1109/SmartWorld.2018.00217
Liu Q, Liu Z, Zhang H et al (2021) Mining cross features for financial credit risk assessment. In: Proceedings of the 30th ACM international conference on information and knowledge management. ACM, New York, NY, USA, pp 1069–1078. https://doi.org/10.1145/3459637.3482371
Lou Y, Caruana R, Gehrke J (2012) Intelligible models for classification and regression. In: KDD'12. https://doi.org/10.1145/2339530.2339556
Lundberg SM, Lee S-I (2017) A unified approach to interpreting model predictions. In: Advances in neural information processing systems. Curran Associates, Inc. https://doi.org/10.5555/3295222.3295230
Lusinga M, Mokoena T, Modupe A, Mariate V (2021) Investigating statistical and machine learning techniques to improve the credit approval process in developing countries. In: 2021 IEEE AFRICON, pp 1–6. https://doi.org/10.1109/AFRICON51333.2021.9570906
Malinova M, Mendling J (2021) Cognitive diagram understanding and task performance in systems analysis and design. MIS Q 45:2101–2157. https://doi.org/10.25300/MISQ/2021/15262
Maloney CB (2021) H.R.2989-117th Congress (2021–2022): Financial transparency act of 2021. https://www.congress.gov/bill/117th-congress/house-bill/2989
Maree C, Modal JE, Omlin CW (2020) Towards responsible AI for financial transactions. In: 2020 IEEE symposium series on computational intelligence (SSCI), pp 16–21. https://doi.org/10.1109/SSCI47803.2020.9308456
Mariotti E, Alonso JM, Confalonieri R (2021) A framework for analyzing fairness, accountability, transparency and ethics: a use-case in banking services. In: 2021 IEEE international conference on fuzzy systems (FUZZ-IEEE), pp 1–6. https://doi.org/10.1109/FUZZ45933.2021.9494481
Martin K (2019) Designing ethical algorithms. MIS Q Exec 18:129–142. https://doi.org/10.2139/ssrn.3056692
Mayer A-S, Strich F, Fiedler M (2020) Unintended consequences of introducing AI systems for decision making. MIS Q Exec. https://doi.org/10.17705/2msqe.00036
McCarthy J, Minsky ML, Rochester N, Shannon CE (1955) A proposal for the dartmouth summer research project on artificial intelligence, August 31, 1955 (reprint in 2006). AI Magazine 27:12
Meske C, Bunde E, Schneider J, Gersch M (2022) Explainable artificial intelligence: objectives, stakeholders, and future research opportunities. Inf Syst Manag 39:53–63. https://doi.org/10.1080/10580530.2020.1849465
Mirbabaie M, Brünker F, Möllmann Frick NRJ, Stieglitz S (2021) The rise of artificial intelligence–understanding the AI identity threat at the workplace. Electron Mark 32: 73–99. https://doi.org/10.1007/s12525-021-00496-x
Montavon G, Samek W, Müller K-R (2018) Methods for interpreting and understanding deep neural networks. Digit Signal Process 73:1–15. https://doi.org/10.1016/j.dsp.2017.10.011
Nian K, Coleman TF, Li Y (2021) Learning sequential option hedging models from market data. J Bank Finance 133:106277. https://doi.org/10.1016/j.jbankfin.2021.106277
Okoli C, Schabram K (2010) A guide to conducting a systematic literature review of information systems research. SSRN J. https://doi.org/10.2139/ssrn.1954824
Oppold S, Herschel M (2019) LuPe: a system for personalized and transparent data-driven decisions. In: Proceedings of the 28th ACM international conference on information and knowledge management. ACM, New York, NY, USA, pp 2905–2908. https://doi.org/10.1145/3357384.3357857
Padmanabhan B, Fang X, Sahoo N, Burton-Jones A (2022) Machine learning in information systems research. MIS Q 46:iii–xix
Google Scholar
Paré G, Trudel M-C, Jaana M, Kitsiou S (2015) Synthesizing information systems knowledge: a typology of literature reviews. Inf Manag 52:183–199. https://doi.org/10.1016/j.im.2014.08.008
Park MS, Son H, Hyun C, Hwang HJ (2021) Explainability of machine learning models for bankruptcy prediction. IEEE Access 9:124887–124899. https://doi.org/10.1109/ACCESS.2021.3110270
Pasquale F (2015) The black box society: the secret algorithms that control money and information. Harvard University Press, Cambridge, Massachusetts, London, England
Rai A, Constantinides P, Sarker S (2019) Next generation digital platforms: toward human-AI hybrids. MIS Q 43:iii–ix
Rajab S, Sharma V (2019) An Interpretable neuro-fuzzy approach to stock price forecasting. Soft Comput 23:921–936. https://doi.org/10.1007/s00500-017-2800-7
Renault T (2017) Intraday online investor sentiment and return patterns in the U.S. Stock Market J Bank Finance 84:25–40. https://doi.org/10.1016/j.jbankfin.2017.07.002
Ribeiro MT, Singh S, Guestrin C (2016) Why should i trust you? Explaining the predictions of any classifier. In: Proceedings of the 22nd ACM SIGKDD international conference on knowledge discovery and data mining. ACM, New York, NY, USA, pp 1135–1144. https://doi.org/10.1145/2939672.2939778
Rosati R, Romeo L, Goday CA et al (2020) Machine learning in capital markets: decision support system for outcome analysis. IEEE Access 8:109080–109091. https://doi.org/10.1109/ACCESS.2020.3001455
Rosenfeld A, Richardson A (2019) Explainability in human-agent systems. Auton Agents Multi-Agent Syst 33:673–705. https://doi.org/10.1007/s10458-019-09408-y
Russell SJ, Norvig P, Davis E (2016) Artificial intelligence: a modern approach. Pearson, Malaysia
Rzepka C, Berger B (2018) User interaction with ai-enabled systems: a systematic review of IS research. In: 39th International conference on information systems, San Francisco 2018
Saeed SK, Hagras H (2018) Adaptive type-2 fuzzy logic based system for fraud detection in financial applications. In: 2018 10th computer science and electronic engineering (CEEC), pp 15–18. https://doi.org/10.1109/CEEC.2018.8674217
Sageder M, Mitter C, Feldbauer-Durstmüller B (2018) Image and reputation of family firms: a systematic literature review of the state of research. Rev Manag Sci 12:335–377. https://doi.org/10.1007/s11846-016-0216-x
Sanneman L, Shah JA (2020) A situation awareness-based framework for design and evaluation of explainable AI. In: International workshop on explainable, transparent autonomous agents and multi-agent systems. Springer, pp 94–110. https://doi.org/10.1007/978-3-030-51924-7_6
Sanz JA, Bernardo D, Herrera F et al (2015) A compact evolutionary interval-valued fuzzy rule-based classification system for the modeling and prediction of real-world financial applications with imbalanced data. IEEE Trans Fuzzy Syst 23:973–990. https://doi.org/10.1109/TFUZZ.2014.2336263
Schnaubelt M, Fischer TG, Krauss C (2020) Separating the signal from the noise–financial machine learning for twitter. J Econom Dyn Control 114:103895. https://doi.org/10.1016/j.jedc.2020.103895
Shapiro SC (1992) Artificial intelligence. Encycl Artif Intell 1:54–57
Shi L, Teng Z, Wang L et al (2019) DeepClue: visual interpretation of text-based deep stock prediction. IEEE Trans Knowl Data Eng 31:1094–1108. https://doi.org/10.1109/TKDE.2018.2854193
Shrikumar A, Greenside P, Shcherbina A, Kundaje A (2017) Not just a black box: learning important features through propagating activation differences. arXiv:160501713 [cs]. https://doi.org/10.48550/arXiv.1605.01713
Sigrist F, Hirnschall C (2019) Grabit: gradient tree-boosted tobit models for default prediction. J Bank Finance 102:177–192. https://doi.org/10.1016/j.jbankfin.2019.03.004
Snyder H (2019) Literature review as a research methodology: an overview and guidelines. J Bus Res 104:333–339. https://doi.org/10.1016/j.jbusres.2019.07.039
Taylan P, Weber G-W, Beck A (2007) New approaches to regression by generalized additive models and continuous optimization for modern applications in finance, science and technology. Optim 56:675–698. https://doi.org/10.1080/02331930701618740
Thiebes S, Lins S, Sunyaev A (2021) Trustworthy artificial intelligence. Electron Mark 31:447–464. https://doi.org/10.1007/s12525-020-00441-4
van Aaken D, Buchner F (2020) Religion and CSR: a systematic literature review. J Bus Econ 90:917–945. https://doi.org/10.1007/s11573-020-00977-z
van der Burgt J (2020) Explainable AI in banking. J Digit Bank 4:344–350
Verhagen RS, Neerincx MA, Tielman ML (2021) A two-dimensional explanation framework to classify AI as incomprehensible, interpretable, or understandable. In: International workshop on explainable, transparent autonomous agents and multi-agent systems. Springer, pp 119–138. https://doi.org/10.1007/978-3-030-82017-6_8
Vishwanath T, Kaufmann D (1999) Towards transparency in finance and governance. SSRN, Rochester, NY
Wang X, Zhang Y, Chen Y (2020) A novel lasso regression model for sector rotation trading strategies with economy-policy cycles. In: 2020 IEEE international conference on big data (Big Data), pp 5473–5479. https://doi.org/10.1109/BigData50022.2020.9377759
Weber P, Bauer K, Hinz O (2020) KI in der finanzbranche: im spannungsfeld zwischen technologischer innovation und regulatorischer anforderung. efl White Paper Series & SAFE Working Paper Series. https://www.eflab.de/fileadmin/Redakteure/Reports/efl_whitepaper_pub_14122020.pdf
Wells L, Bednarz T (2021) Explainable AI and reinforcement learning—a systematic review of current approaches and trends. Front Artif Intell. https://doi.org/10.3389/frai.2021.550030
Wu Q, Yan X (2019) Capturing deep tail risk via sequential learning of quantile dynamics. J Econom Dyn Control 109:103771. https://doi.org/10.1016/j.jedc.2019.103771
Xu P, Ding Z, Pan M (2017) An improved credit card users default prediction model based on RIPPER. In: 13th International conference on natural computation, fuzzy systems and knowledge discovery (ICNC-FSKD), pp 1785–1789. https://doi.org/10.1109/FSKD.2017.8393037
Yang L, Zhang Z, Xiong S et al (2018) Explainable text-driven neural network for stock prediction. In: 5th IEEE International conference on cloud computing and intelligence systems (CCIS). IEEE, pp 441–445. https://doi.org/10.1109/CCIS.2018.8691233
Zhang Z, Zohren S, Roberts S (2019) DeepLOB: deep convolutional neural networks for limit order books. IEEE Trans Signal Process 67:3001–3012. https://doi.org/10.1109/TSP.2019.2907260
Zhang R, Yi C, Chen Y (2020a) Explainable machine learning for regime-based asset allocation. In: IEEE International conference on big data (Big Data), pp 5480–5485. https://doi.org/10.1109/BigData50022.2020.9378332
Zhang Y, Zhao H, Li X et al (2020b) Exchange traded fund clustering via metric learning. In: IEEE International conference on big data (Big Data), pp 5486–5495. https://doi.org/10.1109/BigData50022.2020.9378205
Zheng X, Zhu M, Li Q et al (2019) FinBrain: when finance meets AI 2.0. Front Inf Technol Electron Eng 20:914–924. https://doi.org/10.1631/FITEE.1700822
Zheng K, Cai S, Chua HR et al (2020) TRACER: A framework for facilitating accurate and interpretable analytics for high stakes applications. In: Proceedings of the 2020 ACM SIGMOD international conference on management of data. ACM, New York, NY, USA, pp 1747–1763. https://doi.org/10.1145/3318464.3389720
Zhu Y, Yi C, Chen Y (2020) Utilizing macroeconomic factors for sector rotation based on interpretable machine learning and explainable AI. In: IEEE International conference on big data (Big Data), pp 5505–5510. https://doi.org/10.1109/BigData50022.2020.9377954
Download references
Acknowledgements
We gratefully acknowledge research support from the efl–the Data Science Institute, Germany.
Funding from the efl–the Data Science Institute, Germany enabled the research project. Open Access funding enabled and organized by Projekt DEAL.
Author information
Authors and affiliations.
Chair of Information Systems and Information Management, Goethe University Frankfurt, Theodor-W-Adorno-Platz 4, 60629, Frankfurt am Main, Germany
Patrick Weber, K. Valerie Carl & Oliver Hinz
You can also search for this author in PubMed Google Scholar
Contributions
All authors contributed to the study conception and design, material preparation, data collection and analysis, and the first draft of the manuscript. All authors commented on previous versions of the manuscript. All authors read and approved the final manuscript.
Corresponding author
Correspondence to Patrick Weber .
Ethics declarations
Conflict of interest.
The authors have no relevant financial or non-financial interests to disclose.
Additional information
Publisher's note.
Springer Nature remains neutral with regard to jurisdictional claims in published maps and institutional affiliations.
Supplementary Information
Below is the link to the electronic supplementary material.
Supplementary file1 (PDF 179 KB)
Rights and permissions.
Open Access This article is licensed under a Creative Commons Attribution 4.0 International License, which permits use, sharing, adaptation, distribution and reproduction in any medium or format, as long as you give appropriate credit to the original author(s) and the source, provide a link to the Creative Commons licence, and indicate if changes were made. The images or other third party material in this article are included in the article's Creative Commons licence, unless indicated otherwise in a credit line to the material. If material is not included in the article's Creative Commons licence and your intended use is not permitted by statutory regulation or exceeds the permitted use, you will need to obtain permission directly from the copyright holder. To view a copy of this licence, visit http://creativecommons.org/licenses/by/4.0/ .
Reprints and permissions
About this article
Weber, P., Carl, K.V. & Hinz, O. Applications of Explainable Artificial Intelligence in Finance—a systematic review of Finance, Information Systems, and Computer Science literature. Manag Rev Q 74 , 867–907 (2024). https://doi.org/10.1007/s11301-023-00320-0
Download citation
Received : 16 November 2022
Accepted : 13 January 2023
Published : 28 February 2023
Issue Date : June 2024
DOI : https://doi.org/10.1007/s11301-023-00320-0
Share this article
Anyone you share the following link with will be able to read this content:
Sorry, a shareable link is not currently available for this article.
Provided by the Springer Nature SharedIt content-sharing initiative
- Explainable artificial intelligence
- Systematic literature review
- Machine learning
JEL Classification
- Find a journal
- Publish with us
- Track your research
This site uses cookies, including third-party cookies, to improve your experience and deliver personalized content.
By continuing to use this website, you agree to our use of all cookies. For more information visit IMA's Cookie Policy .
Change username?
Create a new account, forgot password, sign in to myima.
Multiple Categories
The Impact of Artificial Intelligence on Accounting and Finance
January 29, 2024
By: Qi "Susie" Duong , Ph.D., CMA, CPA, CIA, EA
From data analysis to decision-making processes, AI is reshaping the accounting and finance profession. To gain a deeper understanding of AI’s impact on the profession, IMA engaged global experts in the field and accounting and finance leaders to study the applications of AI, as well as the challenges of integrating it with management accounting work.
Although AI cannot fully substitute human expertise, it will profoundly influence the professional trajectory of those in accounting and finance. This transformation spans from the CFO to entry-level staff, emphasizing technology proficiency, data analytics, critical thinking, and cross-functional collaboration. To navigate this evolving landscape, organizations must proactively embrace AI-induced changes by fostering human-machine collaboration, focusing on upskilling and reskilling team members, and creating a culture of ongoing learning to understand AI’s strengths and weaknesses, steering toward its responsible and fruitful implementation.
Prerequisites for successful AI integration involve overcoming challenges in human, technological, operational, and ethical dimensions. This includes:
- Aligning AI strategies with organizational goals and ensuring adequate executive support, addressing the skills gap of the existing workforce through training and development.
- Fostering cross-functional collaboration, process reengineering, and scalability.
- Focusing on data quality and the digital preparedness of teams.
- Navigating AI-related risks and embracing ethical AI practices.
Findings from this research are valuable to any individual or organization navigating the evolving landscape of AI.
About the Authors
- Technology & Analytics
- Strategy, Planning & Performance
More Insights
Copyright Footer Message
- Asia Pacific
- Global Americas
- Middle East/Africa
More From Forbes
The impact of artificial intelligence on decentralized finance.
- Share to Facebook
- Share to Twitter
- Share to Linkedin
Sameera Devuruwan (Sam Dee): CEO at HEXXION. Venture Capitalist in elevating tech startups.
The convergence of artificial intelligence (AI) and decentralized finance (DeFi) is poised to revolutionize the financial landscape. DeFi, which leverages blockchain technology to offer decentralized financial services, has seen exponential growth over the past year. Meanwhile, AI continues to advance, offering sophisticated data analysis, predictive analytics and automation capabilities. Together, these technologies are transforming how financial services are created, delivered and consumed.
I mproving Security And Detecting Fraud
A major effect of AI on DeFi is the improvement of security measures. Since DeFi platforms operate based on decentralized systems and often do not require the provision of personal information, they are exposed to various cyber threats and frauds. Using AI, it is possible to process large sets of data regarding transactions and their characteristics in real time and recognize the tendencies that could indicate fraud. Machine learning models can be used to offer early warnings on possible security breaches to prevent the occurrence of attacks.
For instance, through the use of AI in security, one can identify any suspicious transactions or unusual behaviors. This is important in an environment where there is no governing body that can easily spot fraud.
Enhancing Trading And Investment Strategies
Trading and investment are also being transformed within the DeFi space with the help of AI. These traditional financial markets have been incorporating AI in their algorithmic trading, and so does DeFi. There are automated trading robots that can make trades within a very short time, exploit any market loopholes and manage investment portfolios through data analysis.
Trump Vs. Harris 2024 Polls: Harris Leads By 6 Points In Latest Likely Voter Survey
Viral ‘chase bank glitch’ isn’t the hack people thought it was, directv blacks out espn, abc, and other disney channels.
These AI systems can use historical data, news, social media sentiment and other information to make decisions on trading. Thus, they assist investors in achieving the greatest possible returns on their investments subject to acceptable levels of risk. Such complexity is most valuable in the highly unstable and rapidly evolving DeFi markets.
Optimizing Lending And Borrowing
In the DeFi ecosystem, the lending and borrowing processes are significant elements. AI has the potential to improve these processes through better credit scoring and risk evaluation. The conventional credit scoring systems may not capture the richness of DeFi users’ financial profiles. AI, however, can use transaction history, social behavior, network activity, etc. to more effectively determine creditworthiness.
This enhanced approach can result in more favorable interest rates and conditions for borrowers while at the same time lowering the risk to lenders. Also, the use of AI can help accelerate the loan approval process, thus enabling the DeFi space to sustain the current pace.
Enhancing User Experience
The use of DeFi platforms greatly depends on the experience that users have when interacting with the platform. As mentioned, AI can improve the quality of the user experience by offering recommendations, customer service and automation. Natural language processing (NLP) and chatbots can provide real-time help to users, navigate them through processes and provide relevant information to their queries.
Also, AI is capable of identifying the requirements of consumers and providing them with suitable financial solutions, which makes it more interactive. This can be useful in the process of acquiring and retaining users and thus the growth of DeFi platforms.
Facilitating Regulatory Compliance
One of the significant issues in DeFi is the matter of regulation because DeFi operates in a fully decentralized and borderless environment. AI can help address this scenario by supporting compliance checks and tracking the legal environment. It is possible to develop machine learning algorithms that will help recognize transactions that could potentially violate the law to prevent DeFi platforms from breaking the law.
AI can also inform DeFi projects regarding current legal changes and thus avoid legal problems. This feature is very important when it comes to building trust and credibility within the DeFi ecosystem.
DeFi with the integration of AI has the potential to revolutionize the financial industry. AI is powering the development of decentralized finance through the promotion of security, better trading and investment, better lending and borrowing solutions, user satisfaction and rule compliance. These technologies are still evolving and being integrated and hence they will create new possibilities and definitions in the future of finance. Thus, the incorporation of AI in DeFi is not a luxury but a need in a bid to stand out in the market and offer something new.
Forbes Business Council is the foremost growth and networking organization for business owners and leaders. Do I qualify?
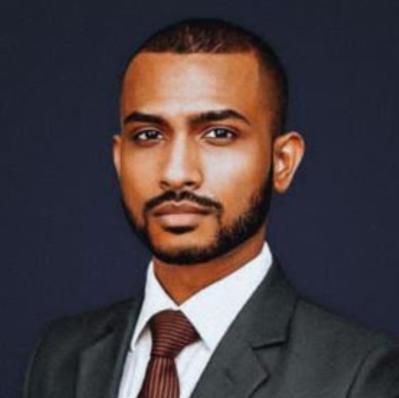
- Editorial Standards
- Reprints & Permissions
Thank you for visiting nature.com. You are using a browser version with limited support for CSS. To obtain the best experience, we recommend you use a more up to date browser (or turn off compatibility mode in Internet Explorer). In the meantime, to ensure continued support, we are displaying the site without styles and JavaScript.
- View all journals
- Explore content
- About the journal
- Publish with us
- Sign up for alerts
- Open access
- Published: 24 August 2024
Artificial intelligence investments reduce risks to critical mineral supply
- Joaquin Vespignani ORCID: orcid.org/0000-0003-0265-4377 1 , 2 &
- Russell Smyth ORCID: orcid.org/0000-0001-6593-5462 3
Nature Communications volume 15 , Article number: 7304 ( 2024 ) Cite this article
1298 Accesses
2 Altmetric
Metrics details
- Energy and society
- Energy economics
This paper employs insights from earth science on the financial risk of project developments to present an economic theory of critical minerals. Our theory posits that back-ended critical mineral projects that have unaddressed technical and non-technical barriers, such as those involving lithium and cobalt, exhibit an additional risk for investors which we term the “back-ended risk premium”. We show that the back-ended risk premium increases the cost of capital and, therefore, has the potential to reduce investment in the sector. We posit that the back-ended risk premium may also reduce the gains in productivity expected from artificial intelligence (AI) technologies in the mining sector. Progress in AI may, however, lessen the back-ended risk premium itself by shortening the duration of mining projects and the required rate of investment by reducing the associated risk. We conclude that the best way to reduce the costs associated with energy transition is for governments to invest heavily in AI mining technologies and research.
Similar content being viewed by others
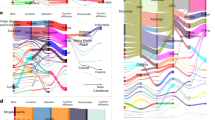
Reducing supply risk of critical materials for clean energy via foreign direct investment
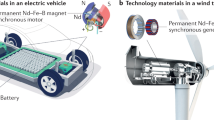
Towards sustainable extraction of technology materials through integrated approaches
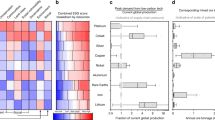
The social and environmental complexities of extracting energy transition metals
Introduction.
Unlike traditional energy sources, such as oil, coal, and gas, clean energy production and storage requires an unprecedented amount of critical minerals, such as copper, lithium, nickel, zinc, cobalt, and rare earth minerals 1 . Consequently, the shortage in the supply of critical minerals that are needed to achieve global net zero targets by 2050 has gained significant attention 1 . Recently, the International Energy Agency (IEA) estimated that the investment that will be required in critical minerals between 2022 and 2030 to realize global net zero by 2050 is ~360–450 USD billion, while the anticipated supply is between 180 and 220 USD billion, implying a shortfall of 180 to 230 USD billion 2 .
A key issue in meeting this shortfall is that there is a considerable time lag between investment and production. According to the IEA, exploring and producing some critical minerals can take 12.5 years 2 . One potential way to increase critical mineral production is via technological progress through artificial intelligence (AI), which can be used at all stages of the mining process. It has been argued that the mining industry is poised to reap the rewards of AI and data-driven approaches as it deals with a complex integrated value chain of exploration, extraction, and refining that has a history of incorporating high-technology systems in order to increase productivity 3 . Applying AI to mining databases may be useful in developing operations for decision-making support 4 . Automatization using AI could provide significant economic benefits for the mining industry through cost reduction, efficiency, and improving productivity 5 .
This paper uses insights from earth science about the financial risk of project developments for some key critical minerals to develop a theory that provides a less sanguine view of the potential for AI to address the shortfall in investment in critical minerals, at least in the short-to-medium term. Some key critical minerals have lower value to investors during the initial stages of project development due to both technical and non-technical risks 6 , 7 , 8 . This type of risk is called back-ended risk and contrasts with front-ended risk, which is present in more traditional mining projects in which investors place a high value on the project in the very early stages, reducing the cost of capital. Taking this observation as a starting point, in this work we develop an economic theory of what we call the back-ended risk premium. This theory states that for minerals that exhibit back-ended risk, the required rate of return by investors is higher than for front-ended risk mineral projects, leading to a back-ended risk premium. The required rate of return is defined as the minimum amount that an investor seeks when they embark on an investment or project 9 .
We show that the increase in the cost of capital due to this risk premium leads to a theoretical slowdown in technological progress. In the infancy of the AI revolution in the mining sector, the back-ended risk premium has the potential to reduce some of the expected gains from AI-inspired technologies. The extent to which this occurs will depend on whether, and to what extent, progress in AI can reduce the back-ended risk premium by shortening the duration of mining projects and lowering the risk faced by investors.
Results and discussion
Conceptualization of the back-ended risk premium.
Many of the minerals considered critical for energy transition exhibit back-ended risk in project development caused by technical and non-technical risks occurring in the later stages of the project (six to eight years after the exploration starts) 6 , 7 , 8 . Examples of critical minerals that exhibit back-ended risk are bauxite, cobalt, lithium, graphite, niobium, nickel laterite, tungsten, scandium, rare earth, vanadium, and zinc. The existence of such back-ended risk means that investment is insufficient for these minerals to increase production in response to an increase in demand. This contrasts with the risk faced by other minerals—so-called front-ended risk minerals—such as gold, copper, and iron ore, for which the value of the project increases rapidly during the exploration stage. For these minerals, most of the investment needed for production occurs in the early stages of the project in response to an increase in demand.
In Fig. 1 , we illustrate back-ended and front-ended risk project development and show how this relates to our proposed concept of the back-ended risk premium. The vertical axis represents the valuation (or share price) that the market places on the mineral project. The horizontal axis displays the different stages of development of the mining project (exploration, scoping, feasibility, development, and mining). At time \({T}_{1}\) the valuation (or share price) of front-ended risk minerals is \({V}_{2},\) which is much higher than the valuation of back-ended risk minerals ( \({V}_{1}\) ). Figure 2 shows that the valuations of back-ended and front-ended risk projects eventually converge after the development stage. The back-ended risk premium can be seen as the relative additional rate of return required by investors for back-ended minerals projects, relative to front-ended minerals projects, and in Fig. 1 is denoted by the gray-shaded area.
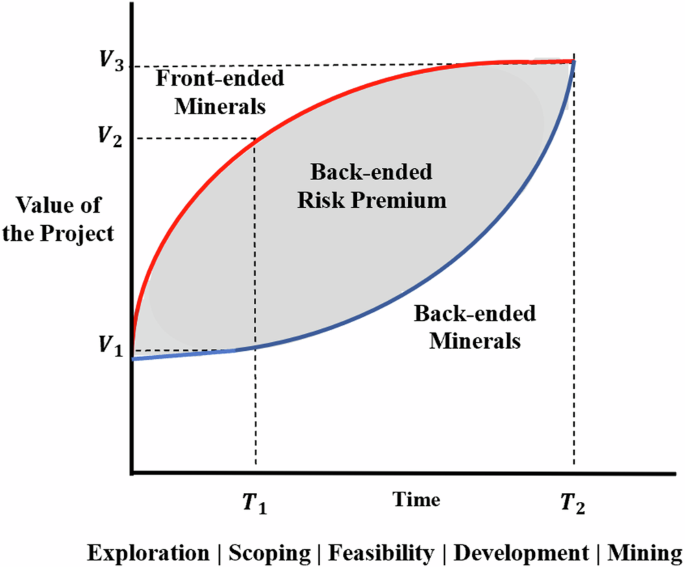
This figure illustrates the relationship between the value that investors attribute to the project at various stages of the development of the mining project. The red line represents this relationship for front-end minerals, while the blue line represents the corresponding relationship for back-end minerals. \({T}_{1}\) and \({T}_{2}\) denote the beginning and conclusion of the project development. \({V}_{1},\,{V}_{2}\) and \({V}_{3}\) are three alternative valuations attributed by investors to the project.
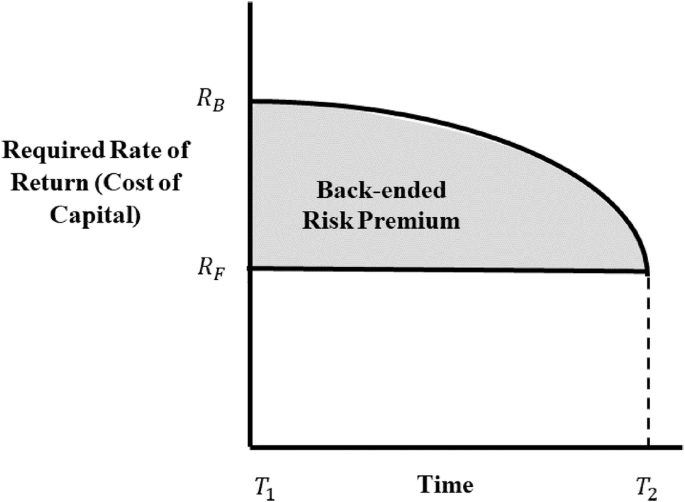
This figure illustrates the differences in the required rate of return to investors between front-ended and back-ended minerals. We define these differences as the back-ended risk premium. \({R}_{b}\) is the required rate of return for back-ended critical minerals and \({R}_{f}\) is the required rate of return for front-ended minerals. \({T}_{1}\) and \({T}_{2}\) denote the beginning and end of the project development.
Figure 1 suggests that for back-ended critical minerals, the value to shareholders only increases in the latter stages of the project development, meaning that investment (and production) will be lower than the market equilibrium requires. Figure 2 illustrates the difference in risk premium between front-ended and back-ended risk projects or the additional risk that investors would incur investing in back-ended minerals compared to front-ended minerals. The vertical axis represents the required rate of return by investors, and the horizontal axis denotes the time between \({T}_{1}\) and \({T}_{2}\) from Fig. 1 . From this figure, we can infer the following:
Investors require a relatively larger return to be compensated by the back-ended risk premium.
The back-ended risk premium decreases as a function of time.
The back-ended risk premium is equal to the risk of front-ended minerals after \({T}_{2}\) .
Risk premiums for critical minerals
Figure 3 presents the non-technical risk premium for each of the critical minerals for 2022. The measurement of the non-technical risk premium is described in the methods, Supplementary Information Section 2 , and in the Supplementary Data. Each of the critical minerals exhibits a non-technical risk premium, relative to the front-ended non-critical mineral benchmark, consisting of coal, gold, and iron ore. The non-technical risk premium varies from rare earth elements (30.6%) to nickel (2.9%), with the average being 15.7%. The heterogeneity in non-technical risk between critical minerals largely reflects the geographical distribution of specific critical minerals, with those exhibiting higher non-technical risk concentrated in countries having less attractive investment environments. In Supplementary Fig. 3 , we show these results are robust to including the only major front-ended critical mineral (copper) in addition to coal, gold and iron ore in the benchmark of front-ended minerals.
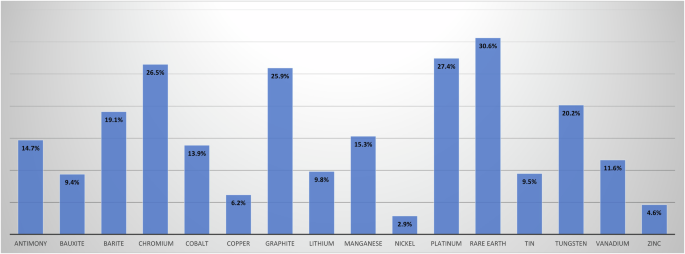
This figure represents the non-technical risk premium for 16 critical minerals relative to a front-ended minerals benchmark consisting of coal, gold, and iron ore for the period 2022. Details of the construction of this measure of the non-technical risk premium, data, and calculations are available in the methods, Supplementary Information, and Supplementary Data.
Figure 4 presents the technical risk premium for 14 critical minerals for which we have data. The measurement of the technical risk premium is described in the methods and Supplementary Information Section 3 . Tin has the highest technical risk premium, while copper and antimony have a technical risk discount, relative to the non-critical mineral benchmark. The average technical risk premium across the 14 critical minerals is 18.4%.
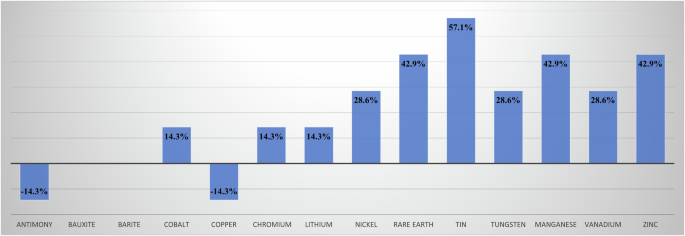
This measure of the technical risk premium is based on six characteristics (crustal abundance, crustal concentration, ease of mining, ease of processing, criticality of use, and diversity of use). Details of the construction of this measure of the technical risk premium, data, and calculations are available in the methods, Supplementary Information, and Supplementary Data.
In Fig. 5 , we present estimates of the total risk premium for the 13 critical minerals for which we have data on technical and non-technical risk. Rare earth elements, tin, manganese, tungsten, and zinc have the highest total risk premium. The only mineral showing a total risk premium discount is copper, reflecting its lower technical score relative to the benchmark.
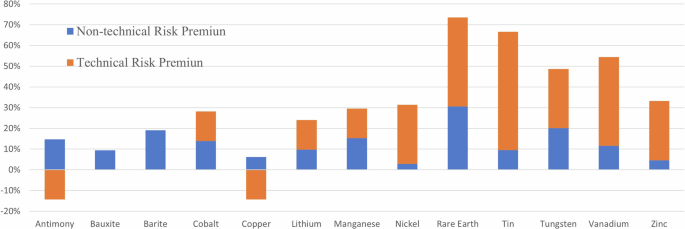
This figure illustrates the total risk premium, which is the sum of non-technical (Fig. 3 ) and technical (Fig. 4 ) risk premiums for 13 critical minerals for which we have data on both technical and non-technical risks.
Estimates of the back-ended risk premium
We provide conservative estimates of the cumulative back-ended risk premium depicted in Fig. 2 until 2035 under the IEA’s Sustainable Development Scenario (SDS) and Stated Policy Scenario (STEPS). STEPS is based on current policy settings, while SDS presents the best-case scenario for the clean energy transition. For each scenario, we calculate the total risk premium based on the weighted average of the market value of each critical mineral. Using the weighted average of the market value has the advantage that it considers differences in the relative importance of critical minerals to the clean energy transition. For the SDS, we calculate the total risk premium to be 32.5%, while for the STEPS the total risk premium is 31.6%.
Our estimates of the cumulative back-ended risk premium until 2035 are given in Fig. 6 . The cumulative back-ended risk premium by 2035 ranges between USD 660 billion and USD 678 billion in the STEPS and SDS scenarios, respectively. Based on estimates described in the methods section, in our main analysis, we use 13.49% as the Weighted Average Cost of Capital (WACC) for the benchmark front-ended minerals. In Supplementary Figs. 4 and 5 , as a sensitivity check on our main analysis, we alternatively assume that the WACC to calculate the back-ended risk premium is 12% or 16%. When the back-ended risk premium benchmark for front-ended minerals is estimated using a WACC of 12%, the cumulative back-ended risk premium decreases from USD 660-678 to USD 587-604, which can be considered a lower-bound estimate on the cumulative back-ended risk premium. In the upper bound case, when the back-ended risk premium benchmark for front-ended minerals is estimated based on 16%, the cumulative back-ended risk premium increases to the USD 775-804 billion range. This figure represents the additional cost of capital for back-ended projects and helps explain why investment in critical minerals is low even when long-term demand is high.
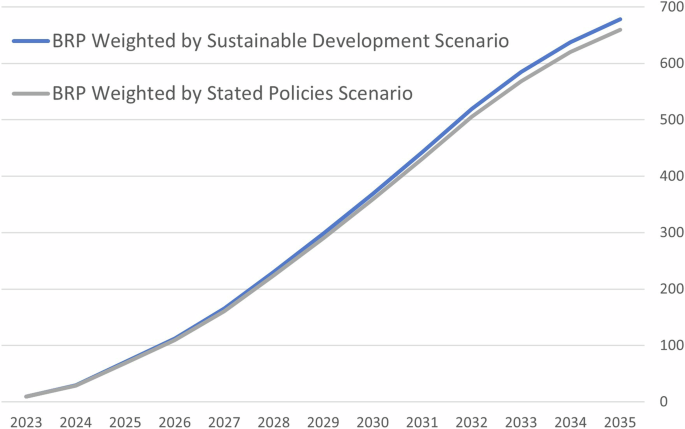
This figure presents the back-ended risk premium (BRP) for the Sustainable Development Scenario (SDS) and Stated Policies Scenario (STEPS), which are the two standard energy policy scenarios presented by the International Energy Agency.
Using AI to address the shortfall in critical minerals
A key feature of the AI revolution is that many roles will be automated, and machine learning procedures that heavily depend on capital will replace mundane labor tasks 10 . In a large survey of machine learning researchers, the consensus among respondents was that AI will outperform humans in many activities over the next decade 11 . The expected gains in productivity in critical minerals production that are hoped will meet the shortfall in supply will be in automation of the labor force and high-level machine intelligence, in which capital is the key component at all stages of the mining industry production process from exploration to extraction 5 .
The back-ended risk premium theory, presented above, implies that the cost of capital for back-ended minerals is higher than that for front-ended minerals. Our findings suggest that, on average, the WACC for front-ended minerals is 13.49%, whereas, for back-ended minerals, it is 4.26% and 4.44% higher, depending on the scenario (17.75% and 17.93%, respectively). Thus, in a capital-intensive industry in which productivity gains are expected to be made in AI developments, the increase in capital cost because of the back-ended risk premium is expected to be economically large, lowering productivity growth in the critical mineral sector.
In Fig. 7 , we employ a production possibility frontier (PPF) to show how the back-ended risk premium affects the potential to increase productivity in back-ended critical minerals via AI. The initial curve shows the combination between capital (K) and labor (L) that is required to produce the maximum amount of minerals for a firm or economy, in which the factors of production are any combination along the PPF of \({L}_{1}\) and \({K}_{1}\) . The green line represents a potential increase in productivity due to AI-technologies which requires more investment in capital (technology). Here capital increases from \({K}_{1}\) to \({K}_{2}\) and the initial PPF rotates outwards and to the right to \({L}_{1}\) and \({K}_{2}\) . The broken blue line represents the new PPF when the back-ended risk premium is considered. The blue line shows that some of the gains in production made by development in AI-technologies is lost due to the higher cost of capital, reflecting the back-ended risk premium. The loss in production is represented by the move from \({K}_{2}\) to \({K}_{3}\) .
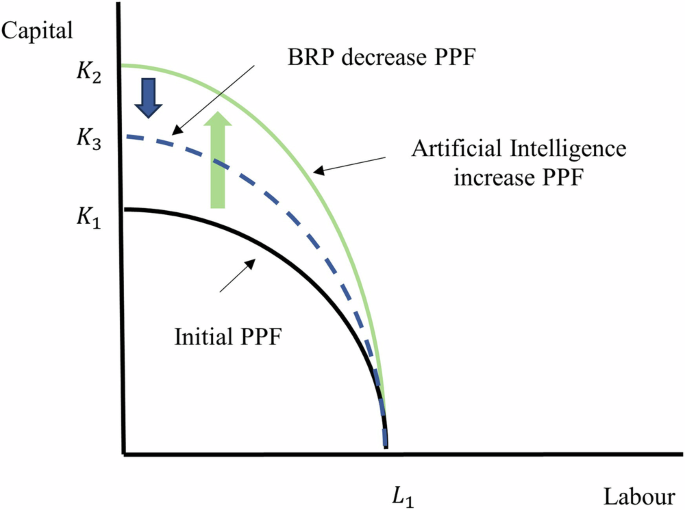
\({K}_{1}\) represents the initial level of capital at the initial Production Possibility Frontier (PPF), \({K}_{2}\) illustrates the level of capital after an increase in productivity due to technological advances in artificial intelligence in mining and \({K}_{3}\) denotes the level of capital considering the impact of the back-ended risk premium. \({L}_{1}\) is the amount of labor required for the three PPFs presented.
The analysis in Fig. 7 may be overly pessimistic. It overlooks the potential for AI to potentially reduce the back-ended risk premium by reducing the cost of capital for critical minerals mining projects. AI could reduce the back-ended risk premium by reducing the duration of mining projects and reducing the required rate of return on investment. We next consider the different ways in which AI could reduce the duration of mining projects and the required rate of return on investment, although we caution the reader that AI also has limitations, which we outline below. The potential benefits of AI for reducing the back-ended risk premium should be seen in light of those limitations.
There are multiple ways through which AI could potentially reduce the time from exploration to extraction in mining projects. One way is through improved mineral mapping. AI techniques, such as drone-based photogrammetry and remote sensing, can be used to automate the process of mineral mapping, making it possible to predict with greater accuracy regions with higher potential for new deposits 12 , 13 , 14 , 15 . Deep learning algorithms have been shown to provide a very high-level of accuracy in image recognition, which can be used for mineral resource mapping with surface and sub-surface image data 12 .
Supply-side risk stemming from the geographical concentration of critical minerals in a few countries has led to increased focus on the role of AI in detecting unconventional deposits of critical minerals in situ geological deposits of oil, gas, or coal mineral deposits from secondary by-products of anthropogenic processes 16 , 17 , 18 , 19 . An advantage AI has in such contexts is that a large amount of data has been collected through fossil fuel exploration on such geological deposits, which is well suited to machine learning 20 .
A second way in which AI could reduce the risk associated with duration from exploration to extraction is by making it possible to more accurately calculate the duration of the extraction period of the mine 14 . One of the most important risks for all mining projects relates to the orebody itself - ie. there is significant uncertainty about mineral resources 21 , 22 . One specific contributor to uncertainty being higher for critical minerals than conventional minerals in this early stage is that there are relatively few sources of data on critical mineral reserves. A second point of difference is that critical minerals are recovered as by-products from refining other metals. This means that the metal supply responds not only to the price of the by-product metal but also to the price of the host metal, which affects reserve estimates 23 . Uncertainty about the potential to recover critical minerals from mine waste, such as tailings, is particularly acute 24 . A third important way in which critical minerals differ from fossil fuels is that the former can be recycled, although the implications of recycling on future reserves is difficult to quantify, adding to uncertainty about available reserves 23 . Mining and extraction methods are dictated by the geology of the orebody or orebodies. AI can be used to provide a more accurate depiction of the geology of the orebody and its associated uncertainties 25 . Several AI methods have been developed to predict the grade and recovery of mineral deposits, reducing the associated technical risk 26 .
A third avenue for reducing the time from exploration to extraction is through employing AI to improve mining productivity. Risks associated with drilling and blasting performance depend on proper rock fragmentation. AI can be used to predict rock fragmentation and provide real-time evaluation of drilling performance that improves efficiency 12 . Automated drilling can be fitted with sensors that target specific ores; hence, reducing exploration time 27 .
AI can also be used to reduce the required rate of return on investment in two main ways. One way is through reducing uncertainty with the risk of a blowout in the cost, which is particularly important for back-ended minerals given that the initial cost of capital is higher than for front-ended minerals. For example, AI can be used to forecast the capital cost of open-pit mining projects 28 . Equipment selection is the most important phase during mine planning. The capital investment in selecting the right equipment represents a major risk. AI algorithms can be used to reduce the risk with equipment selection 12 . Once the mine is in operation, AI can be used for predictive maintenance and management of equipment, minimizing repairs 27 .
AI could also reduce the required rate of return on back-ended projects through reducing the risk, particularly environmental risks, associated with such projects. There is evidence which shows that AI algorithms can be effective in reducing disasters and environmental hazards associated with energy mining 29 . This is particularly important in the case of some key back-ended critical minerals, such as cobalt and lithium.
According to the U.S Geological Survey, more than 50% of the global proven lithium reserves are concentrated in the lithium triangle between Chile, Bolivia, and Argentina. Lithium in this region is mined from salt deserts or so-called salars 30 . Extracting lithium from salars has generated long-term environmental damage, with locals complaining that lithium mining has increased the prevalence of droughts, threatening livestock farming and drying out vegetation 31 . About half of the proven reserves of cobalt are located in Congo Kinshasa. Weak institutions, corruption, and conflict in the region exacerbate environmental risks associated with mining Cobalt. The technical risks associated with artisanal mining practices in Congo Kinshaha have caused environmental degradation, including deforestation, soil erosion, water pollution, and biodiversity loss. Unregulated mining activities, using mercury and other chemicals, and inadequate waste management practices can negatively affect ecosystems and local communities in the long term 32 .
The potential impact of AI on the rate of return and duration of the project are illustrated in Figs. 8 and 9 , respectively. In Fig. 8 , the potential impact of progress in AI on reducing the risk/rate on the back-ended risk premium is represented by the shift from \({R}_{B}\) to \({R}_{{AI}}\) while the volume of this reduction is represented by the area between the blue and green lines. Formally:
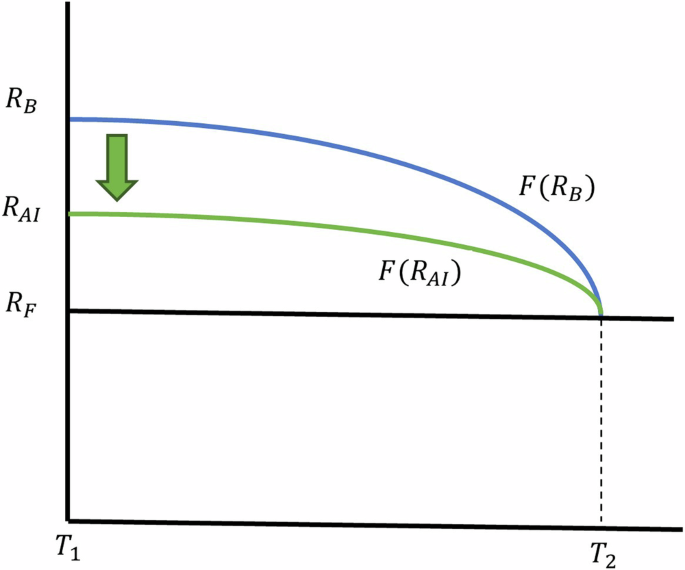
\({R}_{B}\) represents the required rate of return for back-ended critical minerals, \({R}_{F}\) denotes the required rate of return for front-ended minerals, and \({R}_{{AI}}\) indicates the required rate of return after considering advancements in artificial intelligence technology. \({{T}}_{1}\) and \({T}_{2}\) denote the start and end times of the project developments, respectively. \({f \left(\right. R}_{b}\) ) and \({f\left(\right.R}_{{AI}}\) ) are functions of \({R}_{B}\) and, \({R}_{{AI}}\) , respectively.
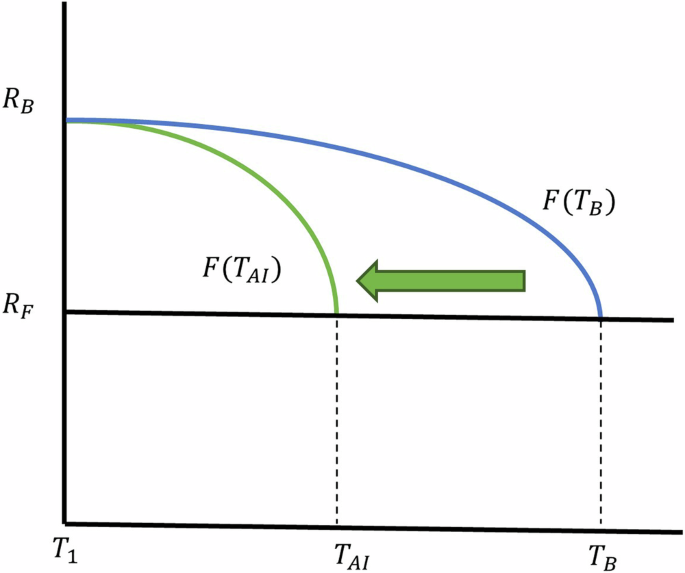
\({R}_{B}\) represents the required rate of return for back-ended critical minerals, and \({R}_{F}\) denotes the required rate of return for front-ended minerals. \({T}_{1}{{{\rm{and}}}}{T}_{B}\) denote the start and end times of the project developments, respective \({ly}.\,{T}_{{AI}}\,\) indicates the required rate of return after considering advancements in artificial intelligence technology. \({f\left(\right. T}_{B}\) ) and \({f\left(\right. T}_{{AI}}\) ) are functions of \({T}_{B}\) and, \({T}_{{AI}}\) , respectively.
In Fig. 9 , the impact of AI progress on reducing the mining project time is represented by the shift from \({T}_{B}\) to \({T}_{{AI}}\) (the area between the blue and the green lines represents the volume). Formally:
In Fig. 10 , we demonstrate the effect of lowering the rate of the back-ended risk premium and a reduction in the duration of mining projects because of advancements in AI technology. For simplicity, we assume a 50% reduction in risk. Since our estimates are proportional, readers can infer proportional reductions at different percentages. A 50% reduction in risk resulting from AI improvements leads to a corresponding decrease in the back-ended risk premium, falling within the range USD 330 (STEPS) to USD 341 billion, while a 50% shortening of project duration resulting from AI improvements leads to a corresponding decrease in the back-ended risk premium, falling within the range USD 330 billion (STEPS) to USD 334 billion (SDS).
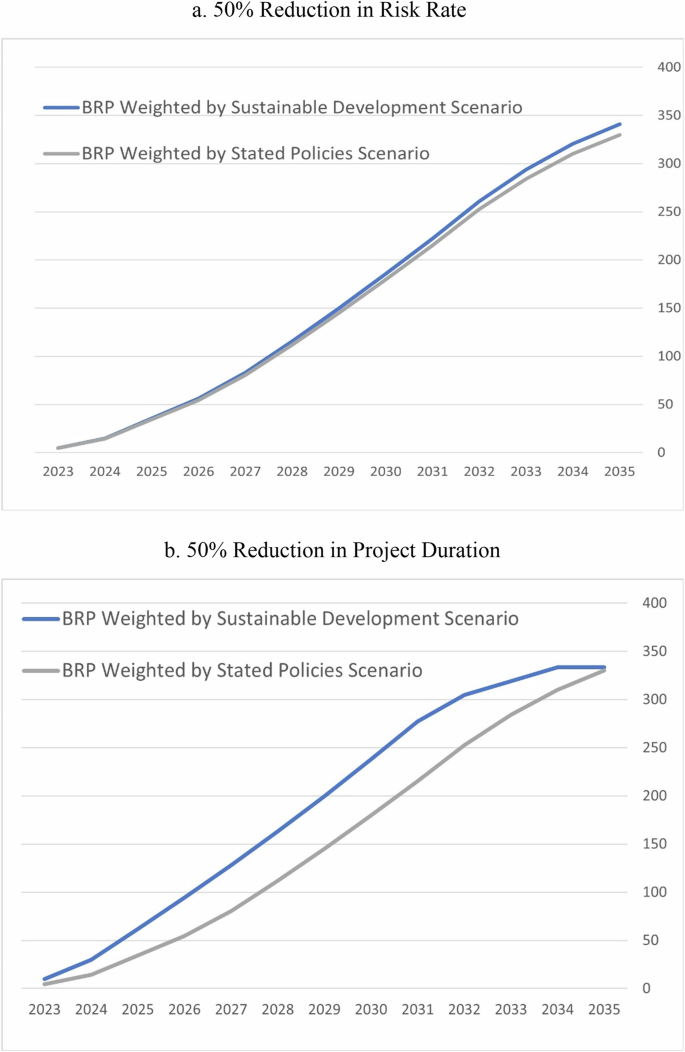
This figure describes the cumulative effect of artificial intelligence on the back-ended risk premium (BRP) for the Sustainable Development Scenario (SDS) and Stated Policy Scenario (STEPS), which are the two standard energy policy scenarios presented by the IEA. a shows the cumulative impact of a 50% reduction in the risk rate. b shows the cumulative impact of a 50% reduction in project duration.
Investment in AI can eliminate the negative impact on the back-ended risk premium on investment in and production of critical minerals, which is key to achieving net zero. Various combinations of reductions in the risk/return associated with back-ended projects, as well as the duration of mining project time, may achieve a zero back-ended risk premium. Again, conclusions about the potential for AI to address the back-ended risk premium are subject to AI’s limitations outlined below.
Subject to these limitations, the implication of this result for the energy transition is that an immediate large-scale direct investment in critical minerals’ projects by governments between USD 660 and USD 678 billion is needed over the next decade. This amount is consistent with the shortage in investment estimated by the IEA 2 . The back-ended risk premium is an additional cost to achieve net zero 2050 that policymakers, thus far, have not considered when estimating the shortfall in investment.
What if meeting carbon net zero by 2050 is not possible?
Some studies have posited that, given existing mining constraints and known reserves of critical minerals needed for clean energy transition, replacing existing fossil fuel sources for energy requirements with renewable alternatives will not be possible by 2050. For example, one study finds that of 29 necessary metals in the lifecycle of renewable energy technologies, known reserves of eight metals might be depleted by 2050 33 . Another study presents several scenarios for transition to carbon net zero, concluding that ultimately, global reserves of cobalt, nickel, and lithium may not be enough to resource the number of batteries needed to power the electric vehicles needed for clean energy transition 34 .
What does this mean for the back-ended risk premium and AI’s potential mitigation of this risk premium? Our interpretation is that the conclusion from such studies reinforces the importance of attracting investment in back-ended critical minerals to reduce the expected shortfall to realize the clean energy transition. It also highlights the importance of investment in AI applications in development and exploration to reduce the duration of mining projects and reduce the required rate of return on investment. Micheaux suggests that if existing critical mineral reserves are not sufficient to resource clean energy transition, a new social contract may be required that limits energy demand 34 . A pessimistic conclusion from this might be that if commitment to energy transition by 2050 is ignored this might erode the motivation for global support for net zero emissions from governments and lending agencies. In such circumstances, other solutions would need to be found, and financing investment in back-ended critical minerals would be less important, making the back-ended risk premium less important. However, this is unlikely. Given the global recognition of the threat posed by climate change, it is very unlikely that commitment to clean energy transition will be eroded even if meeting 2050 targets proves not to be feasible. If commitment to the clean energy transition did look like faltering, reducing expected demand for critical minerals, the added uncertainty would increase the back-ended risk premium by increasing the required rate of return on investment 35 . In this sense, the potential that political commitment to clean energy transition, broadly defined, could wane is a non-technical risk, which increases the uncertainty associated with investing in critical minerals, meaning investors require a higher rate of return. This applies a fortiori to critical minerals that have a high likelihood of running out before 2050. All things equal, the back-ended risk premium for these critical minerals will be higher because the uncertainty about their utility to the clean energy transition is particularly acute. However, it is important to note that the likelihood of a given mineral running out before 2050 is just one non-technical risk among many. Of the eight minerals that Moreau et al. 33 conclude might be depleted by 2050, we consider four in this study (cobalt, nickel, tin, and zinc). It is noteworthy that each of these four critical minerals has a relatively low non-technical risk premium (see Fig. 3 ). More generally, most likely, even if critical minerals do not provide the full solution to resourcing the clean energy transition, they will at least still provide some of the solution, in conjunction with other initiatives, and further investment in critical minerals will be needed to facilitate this.
Limitations on AI as a solution to the back-ended risk premium
We show that improvements in AI have the potential to reduce the back-ended risk premium. However, AI is going to require further investment to ensure the required gains and there is uncertainty about whether and when the benefits of AI will be realized. While it is often touted that AI will lead to improvements in productivity in mining and a reduction in mining project risks, there is a distribution of AI applications in mining and mineral processing in terms of maturity on the Gartner hype cycle for developing new technologies 36 , with many technologies still early on the curve (especially at ‘peak of inflated expectations’). AI has experienced a number of ‘hype cycles’ in which unrealistic expectations have preceded periods of under-delivery, funding cuts, and slowdowns in investment in R&D 37 .
Recent meta-studies have also revealed a worrying tendency for overly optimistic AI performance reporting, due to data leakage and lack of reproducibility 38 , 39 . Messeri and Crokett argue that “AI solutions can exploit our cognitive limitations, making us vulnerable to illusions of understanding in which we believe we understand more about the world than we actually do” 40 . AI faces specific challenges in mining and mineral processing projects. One of the main challenges facing the mining sector in exploiting the potential of AI is addressing the skills gap, with many workers requiring reskilling or upskilling to take advantage of AI 4 , 27 , 41 .
A second set of challenges facing AI in mining studies is the lack of high-quality training data 14 , 37 , 42 . The application of AI has been limited by the use of small datasets 26 , 42 . Cost forecasting applications are typically based on relatively few data points 4 . Given that the exploration of critical minerals is relatively new and that there are relatively few critical minerals (and particularly back-ended critical minerals), the lack of databases and experimental data to train and test AI models is particularly acute.
A third related problem is that mining operations often take place in remote locations making access difficult. Abrasive materials, dust, and humidity that are commonplace in mines do not create a congenial environment for the deployment of digital technologies. In underground mining, installed sensors need to be resistant not only to dust and humidity but also to blasting that can damage the senses. Transmitting the data can be problematic due to the limited bandwidth of communication networks employed in underground and open-pit mining. Connectivity can be particularly weak and unstable in deep mine sites 27 . This makes the storage and transmission of useful data challenging 43 .
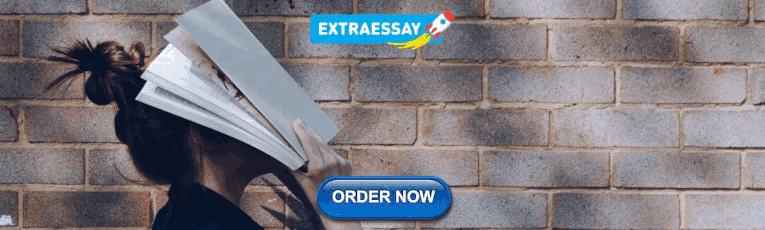
Policy recommendations
The main problem that back-ended critical minerals has is that they provide lower value to investors during the initial stages of project development and exploration, due to technical and non-technical risks. Our analysis suggests that the most important stages to invest in AI to reduce the back-end premium is in applications between exploration and development.
It is essential to highlight that investing in mining in these stages is distinct from investing in AI made by governments, the private sector, and global funding agencies in general. In 2021–2022, the United States Federal Government, as the global leader in AI investment, spent USD 3.3 billion on AI, while worldwide private sector investment in AI was USD 91.9 billion 44 . In comparison, mining companies were expected to spend just USD 218 million on AI globally in 2024 45 with only a fraction of this invested in AI in supporting development and exploration in back-ended critical minerals.
Our suggestions below for areas of investment in AI apply to governments, the private sector, and lending agencies such as the World Bank and IMF. The argument for government investment in AI applications is that it is broad-based, and the benefits of technical breakthroughs can extend beyond a single firm or sector. However, there is also a strong argument for the critical minerals sector to invest in AI to reduce the back-ended risk premium, given it is in their interests to attract investment. Improvements in AI in the development and exploration phase can reduce the back-ended risk premium by both reducing the duration of the project and reducing the required rate of return on investment.
Most AI applications in mining have focused on the development and exploration phase, which is where AI is particularly useful in mitigating technical risks 4 , 15 . One specific AI application in which governments and mining companies could invest is in data-driven prospectivity modeling, where random forest 46 and extreme learning machine models 47 have been used to more accurately predict recovery rates and reduce the time from exploration to extraction. A second related AI application is in mineral mapping in which drilling sensors, geophysical-geochemical-remote sensing surveys, and 3D geological modeling can be used to more accurately predict locations with the most potential for mineral deposits 48 , 49 , 50 , 51 .
A third area for potential investment would be in AI methods, such as artificial neural networks 52 and extreme learning models 53 , to predict the grade and recovery of mineral deposits. A fourth promising area for investment in AI is in the recovery of critical minerals from mining waste. AI has proved particularly useful in improving secondary recovery approaches, such as adsorption 54 , 55 .
Lithium extraction in Chile, Bolivia, and Argentina is plagued by water scarcity. Scarcity of water and water management is one of the main challenges of mineral processing plants that lead to delays 56 . Automated ‘dry’ or water-savvy sensing AI techniques can be employed to minimize these risks 25 . The benefits of using critical minerals, such as lithium, to facilitate the clean energy transition will be muted if they are extracted in an environmentally unfriendly way. More generally, investment in AI-powered environmental monitoring systems, such as smart earth technologies, could help mitigate the impact of minerals such as cobalt and lithium on the environment by detecting and addressing pollution in real time 56 . One of the biggest obstacles to the successful adoption of AI in addressing back-ended risk is the lack of data. Creating publicly available material datasets that can be used as a source of model training and testing is a common recommendation for further AI applications in mining 42 . Given the public goods nature of having open access material datasets, this is one area in which governments and international lending agencies could invest.
In this section, we describe how we constructed the non-technical and technical risk premiums and how we use this information to estimate the back-ended risk premium.
Non-technical risk premium
In Supplementary Section 2 , we construct indexes for the non-technical risk of 16 major critical minerals and a benchmark index consisting of the average for coal, iron ore, and gold, which are three representative non-critical front-ended minerals 6 . These 16 critical minerals account for more than 90% of the market value of the 50 critical minerals, as defined by the U.S. Department of Energy. There is no geological data on proven reserves for the other 34 critical minerals, although it is expected that more knowledge on global proven reserves will become available as AI applications improve, making it possible to calculate non-technical risks for other critical minerals in the future. The non-technical risk indexes are the product of the investment attractiveness index from the Annual Survey of Mining Companies 57 , weighted by country-proven reserves of each individual mineral (see Supplementary Tables 1 and 2 ) 30 , 58 .
Where \({w}_{c,{cm}}\) is the proven reserves of critical mineral m in country c as a percentage of the world’s proven reserves of minerals and \(S\) is the investment attractiveness index score for country c from the Annual Survey of Mining Companies 57 .
Using these indexes, we construct a measure of the non-technical risk premium, which is the critical mineral non-technical risk expressed as a percentage of the non-technical risk of the non-critical front-ended mineral benchmark.
Where \({IAI}\) is the investment attractiveness index and NC and C denote non-critical minerals and critical minerals respectively.
Technical risk premium
Sykes et al. 59 measured the technical risk of minerals for 49 minerals on the basis of six characteristics (crustal abundance, crustal concentration, ease of mining, ease of processing, criticality of use, and diversity of use). Details of these definitions are given in Supplementary Information Section 2 . Each of the minerals was given a score of either zero, 0.5, or 1 for each characteristic. Thus, technical risk for each mineral takes a value between zero (lowest) and six (highest), with higher values indicating lower technical risk. In Supplementary Fig. 2 , we show the scores of this measure of technical risk for 14 critical minerals and our benchmark of front-ended non-critical minerals, consisting of the average for coal, gold and iron ore. Using the technical risk index in Supplementary Information Section 3 , we construct a measure of the technical risk premium, which is expressed as the percentage difference between the critical mineral technical risk and the technical risk of the non-critical mineral benchmark.
Where TR is the technical risk of the non-critical mineral benchmark (NC) and critical minerals (C), from Sykes et al. 59 .
Back-ended risk premium
The back-ended risk premium is estimated as follows:
The total risk premium is the sum of the standardized technical and non-technical risk premium/discount with possible values in absolute values between zero and 1. WACC is the weighted average cost of capital for the average front-ended mining company. To estimate the WACC, we employ a random sample of 368 mining companies, spanning the period 2007 to 2023, giving a total of 2609 observations. The dataset consists of 368 mining companies engaged in mineral production worldwide, including but not limited to gold, copper, iron ore, and coal and is compiled from Bloomberg. In the Supplementary Data, we provide the dataset and all our calculations. For these firms, the WACC was 13.49%, which is consistent with the finding reported in Rasnosz 60 that the WACC of mining companies typically ranges from 8% to 20%, with an average of approximately 14%. In our main analysis, we use 13.49% as the WACC. In sensitivity analysis, we use a lower bound value of the WACC of 12% and an upper bound value of 16%. Our benchmark estimation from Eq. 4 for the back-ended risk premium is 4.44% for the SDS and 4.26% for the STEPS.
Data availability
The data is available in Excel format in the Source Data. Source data are provided with this paper.
Code availability
Calculations are coded in Excel format and are available in the Source Data.
Nature Editorial. The world’s costly and damaging fight for critical minerals. Nature 619 , 436 (2023).
Article Google Scholar
IEA. The role of critical minerals in clean energy transitions, IEA, Paris https://www.iea.org/reports/the-role-of-critical-minerals-in-clean-energy-transitions , (2021).
Sganzerla, C., Seixas, C. & Conti, A. Disruptive innovation in digital mining. Procedia Eng. 138 , 64–71 (2016).
Noriega, R. & Pourrahimian, Y. A systematic review of artificial intelligence and data-driven approaches in strategic open-pit mine planning. Resour. Policy 77 , 102727 (2022).
Hyder, Z., Siau, K. & Nah, F. Artificial intelligence, machine learning, and autonomous technologies in mining industry. J. Database Manag. 30 , 67–79 (2019).
Sykes, J. P. The future of rare earths project development (Australia Resources & Investment) 8 , 157–158 (2014).
Trench, A. & Packey, D. Austraia’s next top mining shares: understanding risk and value in minerals equities, Major Street Publishing, Highett, VIC, 336 (2021).
Trench, A., Packey, D. & Sykes, J. P. Non-technical risks and their impact on the mining industry. Miner. Resour. Ore Reserve Estimation: the AUSIMM guide to good practice, Monograph 30 , 605–618, http://hdl.handle.net/20.500.11937/22432 (2014).
Google Scholar
Drake, P. P. and Fabozzi, F. J. Foundations and applications of the time value of money . John Wiley & Sons (2009).
West, D. M. and Allen, J. R. How artificial intelligence is transforming the world . Brookings Institute. April, p. 24 (2018).
Grace, K., Salvatier, J., Dafoe, A., Zhang, B. & Evans, O. When will AI exceed human performance? Evidence from AI experts. J. Artif. Intell. Res. 62 , 729–754 (2018).
Article MathSciNet Google Scholar
Ali, D. & Frimpong, S. Artificial intelligence, machine learning and process automation: existing knowledge frontier and way forward for mining sector. Artif. Intell. Rev. 53 , 6025–6042 (2020).
Honarmand, M. & Shahriari, H. Geological mapping using drone-based photogrammetry: an application for exploration of vein-type Cu mineralization. Minerals 11 , 585 (2021).
Article ADS CAS Google Scholar
Jooshaki, M., Nad, A. & Michaux, S. A systematic review on the application of machine learning in exploiting mineralogical data in mining and mineral industry. Minerals 11 , 816 (2021).
Article ADS Google Scholar
Köhler, M. et al. Lithium potential mapping using artificial neural networks: a case study from Central Portugal. Minerals 11 , 1046 (2021).
Chatterjee, S., Mastalerz, M., Drobniak, A. & Karacan, C. Ö. Machine learning and data augmentation approach for identification of rare earth element potential in Indiana Coals, USA. Int. J. Coal Geol. 259 , 104054 (2022).
Article CAS Google Scholar
Creason, C. G. et al. A geo-data science method for assessing unconventional rare-earth element resources in sedimentary systems. Nat. Resour. Res. 32 , 855–878 (2023).
Presley, J. Exploring the unexpected: an AI model uncovers rare earth elements. J. Pet. Technol. 76 , 40–45 (2024).
Roth, E., Bank, T., Howard, B. & Granite, E. Rare earth elements in Alberta oil sand process streams. Energy Fuels 31 , 4714–4720 (2017).
Bishop, B. A. & Robbins, L. J. Using machine learning to identify indicators of rare earth element enrichment in sedimentary strata with applications for metal prospectivity. J. Geochem. Explor. 258 , 107388 (2024).
Al-Bakri, A. Y., Ahmed, H. A., Ahmed, H. M. & Hefni, M. A. Evaluation studies of the new mining projects. Open Geosci. 15 , 20220466 (2023).
Dominy, S. C., Noppé, M. A. & Annels, A. E. Errors and uncertainty in mineral resource and ore reserve estimation: The importance of getting it right. Explor. Min. Geol. 11 , 77–98 (2002).
Speirs, J., McGlade, C. & Slade, R. Uncertainty in the availability of natural resources: fossil fuels, critical metals and biomass. Energy Policy 87 , 654–664 (2015).
Suppes, R. & Heuss-Aßbichler, S. Resource potential of mine wastes: a conventional and sustainable perspective on a case study tailings mining project. J. Clean. Prod. 297 , 126446 (2021).
Nwaila, G. T. et al. The minerals industry in the era of digital transition: an energy-efficient and environmentally conscious approach. Resour. Policy 78 , 102851 (2022).
Gomez-Flores, A., Ilyas, S., Heyes, G. W. & Kim, H. A critical review of artificial intelligence in mineral concentration. Miner. Eng. 189 , 107884 (2022).
Onifade, M., Adebisi, J. A., Shivute, A. P. & Genc, B. Challenges and applications of digital technology in the mineral industry. Resour. Policy 85 , 103978 (2023).
Zhang, H. et al. Developing a novel artificial intelligence model to estimate the capital cost of mining projects using deep neural network-based ant colony optimization algorithm. Resour. Policy 66 , 101604 (2020).
You, M., Li, S., Li, D. & Xu, S. Applications of artificial intelligence for coal mine gas risk assessment. Saf. Sci. 143 , 105420 (2021).
United States Geological Survey (USGS). Mineral commodity summaries , 2010 – 2020 . In: Government Printing Office (2022).
Ortiz, E. M. The role of the state and the environmental impacts of lithium mining in Jujuy, Argentina. PhD thesis, University of California, Los Angeles (2021).
Agboola, O. et al. A review on the impact of mining operation: monitoring, assessment and management. Results Eng. 8 , 100181 (2020).
Moreau, V., Dos Reis, P. C. & Vuille, F. Enough metals? Resource constraints to supply a fully renewable energy system. Resources 8 , 29 (2019).
Michaux, S. P. Assessment of the extra capacity required of alternative energy electrical power systems to completely replace fossil fuels. Report 42 , 2021 (2021).
Carruth, A., Dickerson, A. & Henley, A. What do we know about investment under uncertainty? J. Econ. Surv. 14 , 119–154 (2000).
Linden, A. & Fenn, J. Understanding Gartner’s hype cycles. Strategic Anal. Rep. No R.-20-1971. Gart., Inc. 88 , 1423 (2003).
McCoy, J. T. & Auret, L. Machine learning applications in minerals processing: a review. Miner. Eng. 132 , 95–109 (2019).
Kapoor, S. & Narayanan, A. Leakage and the reproducibility crisis in machine-learning-based science. Patterns 4 , 100804 (2023).
Article PubMed PubMed Central Google Scholar
Rosenblatt, M. et al. Data leakage inflates prediction performance in connectome-based machine learning models. Nat. Commun. 15 , 1829 (2024).
Article ADS CAS PubMed PubMed Central Google Scholar
Messeri, L. & Crockett, M. J. Artificial intelligence and illusions of understanding in scientific research. Nature 627 , 49–58 (2024).
Article ADS CAS PubMed Google Scholar
Young, A. & Rogers, P. A review of digital transformation in mining. Min. Metall. Explor. 36 , 683–699 (2019).
Maleki, R., Asadnia, M. & Razmjou, A. Artificial intelligence‐based material discovery for clean energy future. Adv. Intell. Syst. 4 , 2200073 (2022).
Estay, H., Lois-Morales, P., Montes-Atenas, G. & Ruiz del Solar, J. On the challenges of applying machine learning in mineral processing and extractive metallurgy. Minerals 13 , 788 (2023).
Stanford. Artificial Intelligence Index Report 2023 . Stanford University Institute for Human Centred Artificial Intelligence (2023).
Mining Technology. Leading AI companies in the mining industry . https://www.mining-technology.com/buyers-guide/leading-ai-companies-mining/#:~:text=Mining%20firms%20will%20spend%20%24218,solutions%20is%20difficult%20to%20estimate (2024).
Flores, V., Keith, B. & Leiva, C. Using artificial intelligence techniques to improve the prediction of copper recovery by leaching. J. Sens. 2020 , 1–12 (2020).
Chen, Y. & Wu, W. Mapping mineral prospectivity using an extreme learning machine regression. Ore Geol. Rev. 80 , 200–213 (2017).
Acosta, I. C. C., Khodadadzadeh, M., Tusa, L., Ghamisi, P. & Gloaguen, R. A machine learning framework for drill-core mineral mapping using hyperspectral and high-resolution mineralogical data fusion. IEEE J. Sel. Top. Appl. Earth Obs. Remote Sens. 12 , 4829–4842 (2019).
Jindai, Z. et al. The main advance and achievements in the potential evaluation of uranium resource in China. Uranium Geol. 28 , 321–326 (2012).
Tusa, L. et al. Mineral mapping and vein detection in hyperspectral drill-core scans: application to porphyry-type mineralization. Minerals 9 , 122 (2019).
Wang, G. & Huang, L. 3D geological modeling for mineral resource assessment of the Tongshan Cu deposit, Heilongjiang Province, China. Geosci. Front. 3 , 483–491 (2012).
Chatterjee, S., Bandopadhyay, S. & Machuca, D. Ore grade prediction using a genetic algorithm and clustering based ensemble neural network model. Math. Geosci. 42 , 309–326 (2010).
Jain, G., Pathak, P., Bhatawdekar, R. M., Kainthola, A. & Srivastav, A. Evaluation of machine learning models for ore grade estimation. In: International Conference on Geotechnical Challenges in Mining, Tunneling and Underground Infrastructures . Singapore: Springer Nature Singapore, pp. 613–624 (2021).
de Vargas Brião, G., Franco, D. S. P., da Silva, F. V., da Silva, M. G. C. & Vieira, M. G. A. Critical rare earth metal adsorption onto expanded vermiculite: accurate modeling through response surface methodology and machine learning techniques. Sustain. Chem. Pharm. 31 , 100938 (2023).
Sadi, M. & Soleimani, M. Development of artificial intelligence-based models for prediction of vanadium adsorption onto activated carbon nanocomposites. J. Water Process Eng. 55 , 104220 (2023).
Dhanwani, R., Prajapati, A., Dimri, A., Varmora, A. & Shah, M. Smart earth technologies: a pressing need for abating pollution for a better tomorrow. Environ. Sci. Pollut. Res. 28 , 35406–35428 (2021).
Fraser Institute. Annual survey of mining companies . Fraser Institute: Vancouver, BC (2022).
BP Statistical Review of World Energy. British petroleum statistical review of world energy : Pureprint Group Limited, UK (2022).
Sykes, J. P., Wright, J. P., Trench, A. & Miller, P. An assessment of the potential for transformational market growth amongst the critical metals. Appl. Earth Sci. 125 , 21–56 (2016).
Ranosz, R. Analysis of the structure and cost of capital in mining enterprises. Gospod. Surowcami Miner alnymi Resour. Manag. 33 , 77–92 (2017).
Download references
Acknowledgements
We acknowledge the valuable comments of participants at the following conferences: Fourth Italian Workshop of Econometrics and Empirical Economics: “Climate and Energy Econometrics”, ERIA Working Group Meeting on the Supply Chain of Critical Minerals, Monash Business School, Climate Workshop: Navigating the Energy Transition, and presentations at Geoscience Australia, the Sustainable Minerals Institute of the University of Queensland and the School of Economics at Griffith University. We also acknowledge the help of our research assistants, Thanh Huong Bui and Lisa Chan.
Author information
Authors and affiliations.
Tasmanian School of Business and Economics, University of Tasmania, Sandy Bay, TAS, Australia
Joaquin Vespignani
Centre for Applied Macroeconomic Analysis, Australian National University, Canberra, ACT, Australia
Department of Economics, Monash Business School, Monash University, Clayton, NC, Australia
Russell Smyth
You can also search for this author in PubMed Google Scholar
Contributions
Both authors contributed equally to this work. Joaquin Vespignani and Russell Smyth collaboratively conceptualized the study, conducted the analysis, and wrote the manuscript. Both authors participated in data collection and provided critical feedback throughout the research process. Each author was involved in editing and approving the final manuscript.
Corresponding author
Correspondence to Joaquin Vespignani .
Ethics declarations
Competing interests.
The authors declare no competing interests
Peer review
Peer review information.
Nature Communications thanks Lidia Auret, Christopher Lawley and Shirui Luo for their contribution to the peer review of this work. A peer review file is available.
Additional information
Publisher’s note Springer Nature remains neutral with regard to jurisdictional claims in published maps and institutional affiliations.
Supplementary information
Supplementary information, peer review file, source data, source data, rights and permissions.
Open Access This article is licensed under a Creative Commons Attribution-NonCommercial-NoDerivatives 4.0 International License, which permits any non-commercial use, sharing, distribution and reproduction in any medium or format, as long as you give appropriate credit to the original author(s) and the source, provide a link to the Creative Commons licence, and indicate if you modified the licensed material. You do not have permission under this licence to share adapted material derived from this article or parts of it. The images or other third party material in this article are included in the article’s Creative Commons licence, unless indicated otherwise in a credit line to the material. If material is not included in the article’s Creative Commons licence and your intended use is not permitted by statutory regulation or exceeds the permitted use, you will need to obtain permission directly from the copyright holder. To view a copy of this licence, visit http://creativecommons.org/licenses/by-nc-nd/4.0/ .
Reprints and permissions
About this article
Cite this article.
Vespignani, J., Smyth, R. Artificial intelligence investments reduce risks to critical mineral supply. Nat Commun 15 , 7304 (2024). https://doi.org/10.1038/s41467-024-51661-7
Download citation
Received : 03 November 2023
Accepted : 14 August 2024
Published : 24 August 2024
DOI : https://doi.org/10.1038/s41467-024-51661-7
Share this article
Anyone you share the following link with will be able to read this content:
Sorry, a shareable link is not currently available for this article.
Provided by the Springer Nature SharedIt content-sharing initiative
By submitting a comment you agree to abide by our Terms and Community Guidelines . If you find something abusive or that does not comply with our terms or guidelines please flag it as inappropriate.
Quick links
- Explore articles by subject
- Guide to authors
- Editorial policies
Sign up for the Nature Briefing: Anthropocene newsletter — what matters in anthropocene research, free to your inbox weekly.

- Integrations
- Agent Coaching
- Improve Sales Skills
- Sales Training
- BPOs/Call Centers
- Case studies
- Customer Stories
- Contact Center Glossary
Tushar Jain
- August 26, 2024
Artificial Intelligence In Finance: Top AI Technologies
Discover how artificial intelligence in finance is revolutionizing operations, customer experiences, and operational efficiency for contact centers for financial services.
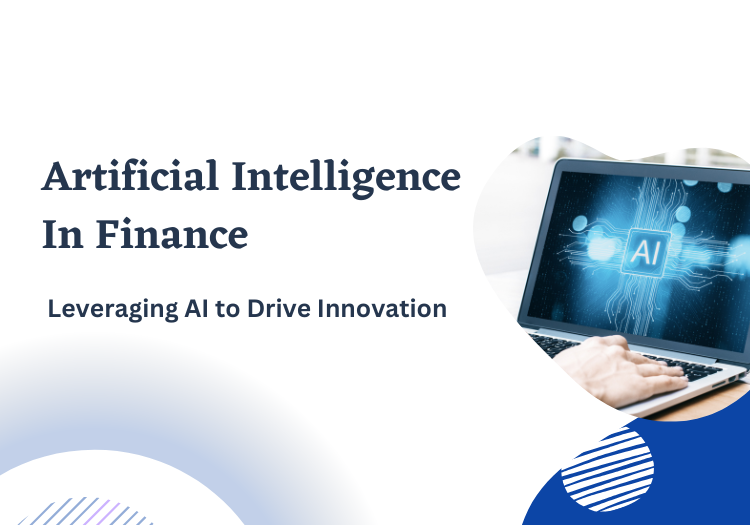
On this page
In today’s digital and competitive world, customers expect immediate support and personalized experiences from contact centers for financial institutions.
Often bogged down by outdated technology and manual processes, traditional contact centers need help to meet these rising expectations.
Long wait times, inconsistent service quality, and the inability to provide personalized interactions have become common pain points.
As a result, customer satisfaction drops, and operational costs soar, leaving financial institutions disadvantaged in a rapidly evolving market.
This is where AI steps in.
Artificial intelligence in finance addresses these pain points head-on by automating routine tasks, analyzing vast amounts of data in real-time, and providing insights into customer behavior.
A report found that 69% of banks and financial institutions are already investing in AI to maintain a competitive edge.
These organizations are leveraging AI technologies like predictive analytics, speech analytics, and virtual agents to streamline operations, deliver more personalized customer experiences, and ensure regulatory compliance.
But what if your financial contact center fails to embrace AI?
Without AI, your contact center may continue to struggle with inefficiencies, leading to frustrated customers and higher churn rates .
On the flip side, the opportunities presented by AI are immense.
In this article, we will explore how AI is revolutionizing financial contact centers by diving into the top AI technologies that are making an impact.
A. Role of artificial intelligence in finance
Artificial intelligence (AI) is transforming the finance industry by making processes smarter, faster, and more efficient.
When you think about the role of AI in finance, it’s all about enhancing the way financial institutions serve you and manage their operations.
AI helps in analyzing vast amounts of data quickly, allowing banks and financial services to offer personalized advice, detect fraud, and manage risks better.
For example, AI-powered chatbots can answer your queries 24/7, providing instant support.
AI can also help in processing loan applications faster by evaluating your creditworthiness in seconds rather than days.
Moreover, AI plays a crucial role in fraud detection.
By analyzing transaction patterns, AI can identify suspicious activities and alert you immediately, keeping your money safe.
In investment management, AI assists in making data-driven decisions, helping to predict market trends and manage your portfolio more effectively.
Overall, AI is reshaping finance by improving customer experience, increasing security, and driving efficiency.
Whether you’re managing your bank account, applying for a loan, or investing in stocks, AI is there, working behind the scenes to make things easier and more secure for you.
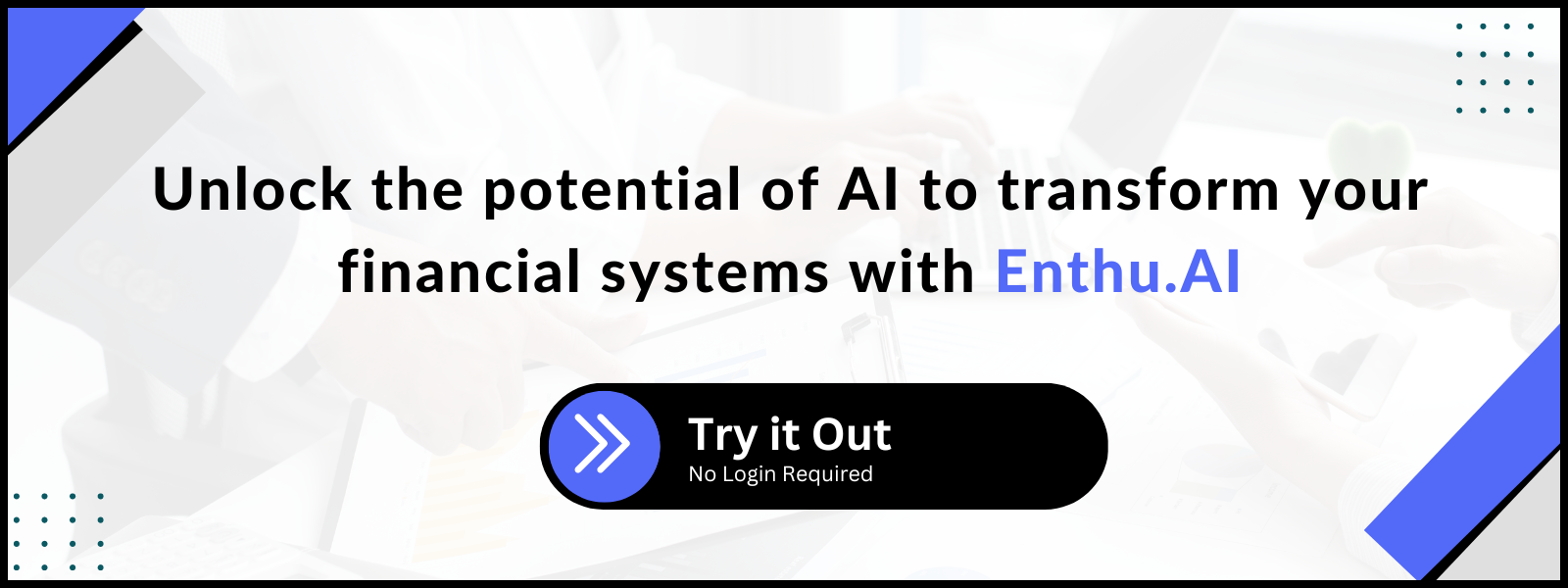
B. 9 AI technologies in financial contact centers
Based on our research and keeping the context of the year 2024, here’s a list of the top AI technologies in financial contact centers that any business can prefer:
1. Speech Analytics
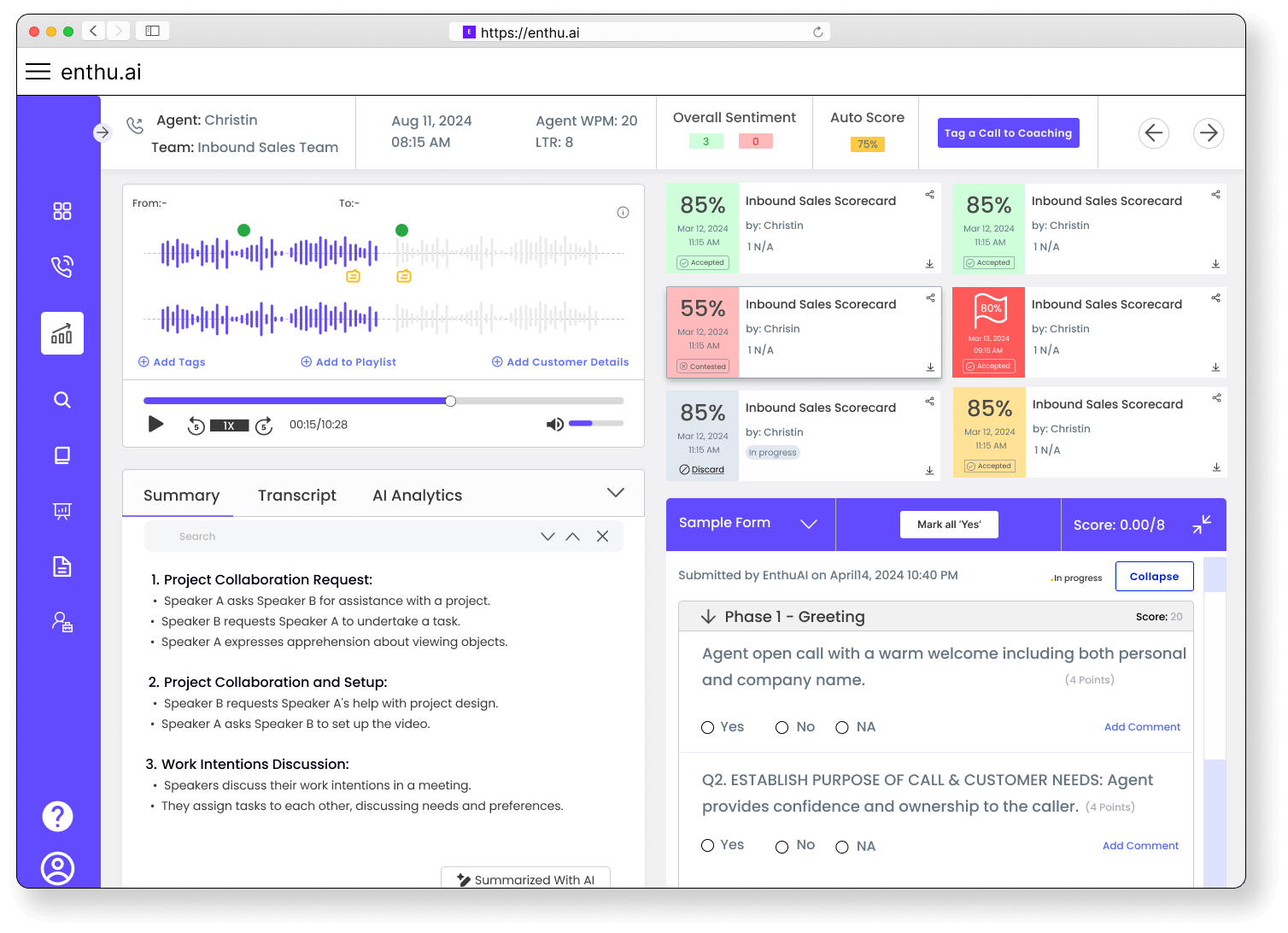
Speech analytics is one of financial contact centers’ most powerful AI tools .
It analyzes voice interactions between customers and agents, extracting valuable insights to improve customer service.
You can identify trends, understand customer sentiment, and even detect compliance issues with speech analytics.
For example, if a customer frequently calls about a specific problem, speech analytics can identify this pattern and help you address the issue proactively.
Moreover, it aids in quality assurance by monitoring calls for adherence to scripts and compliance requirements.
2. Predictive Call Routing
Predictive call routing uses AI to match customers with the most suitable agent based on historical data and real-time analytics.
This technology ensures that customers are connected to agents who are best equipped to handle their specific needs.
It helps you improve customer satisfaction and reduce call resolution times .
By analyzing previous interactions and customer data, predictive call routing can anticipate the customer’s needs and route them to the right agent.
It makes the process more efficient and personalized.
3. Sentiment Analysis
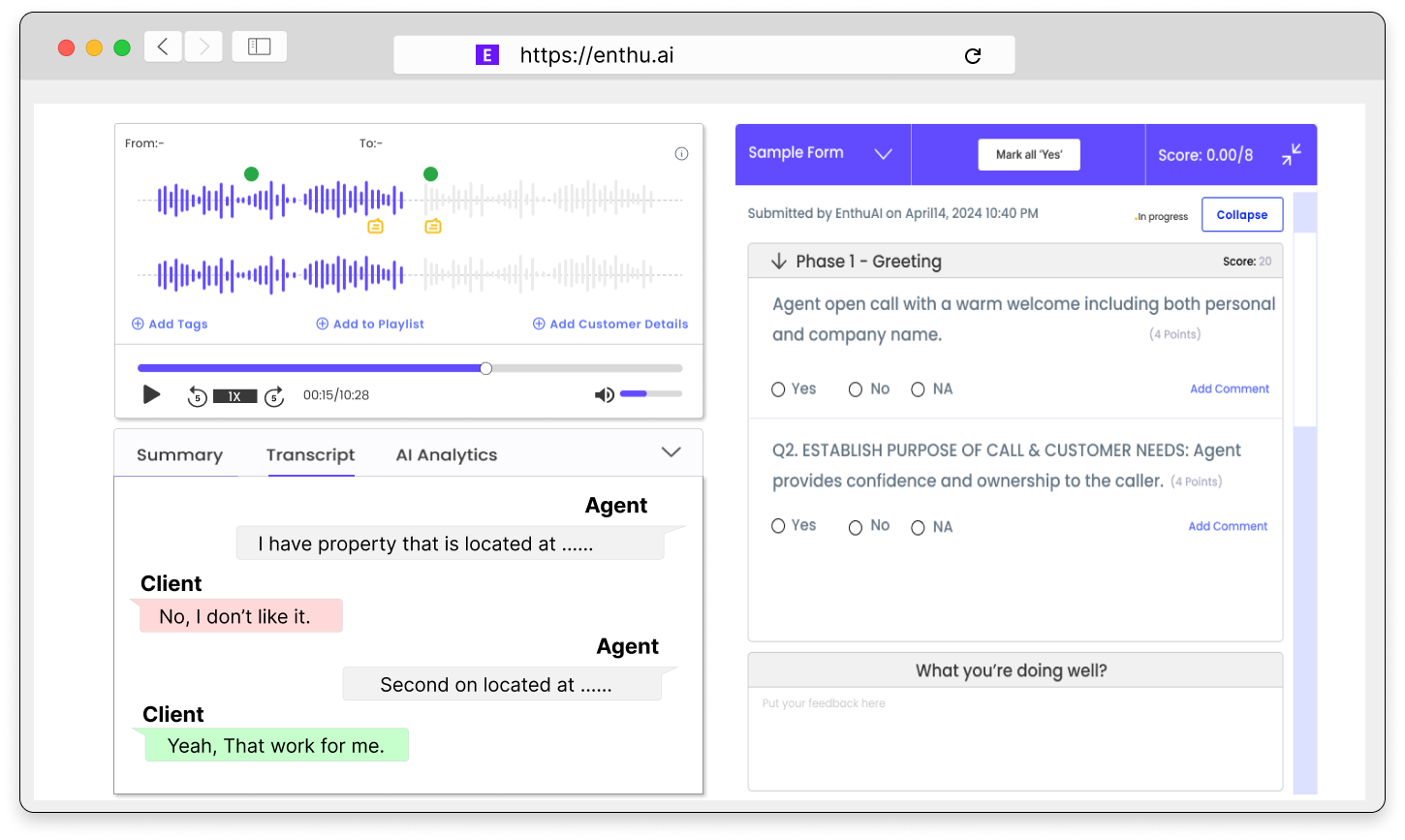
Sentiment analysis is another crucial AI technology in financial contact centers.
It involves analyzing customer interactions to determine their emotional tone.
This analysis helps understand customer satisfaction levels and identify areas where improvements are needed.
With sentiment analysis, you can gauge how customers feel about your services in real-time.
The system can alert agents or supervisors if a customer is frustrated or upset.
Therefore, it allows them to take immediate action to resolve the issue and improve the customer experience.
4. Predictive Analytics
Predictive analytics in finance uses historical data to forecast future outcomes.
In contact centers, this technology can predict call volumes, customer behavior, and even the likelihood of a customer churning.
By anticipating these trends, you can make informed decisions and implement strategies to enhance customer retention and service quality.
For example, if predictive analytics shows a spike in call volumes during a particular period, you can allocate additional resources to handle the increased demand, ensuring that customer service levels remain high.
5. Agent Quality Analysis and Training
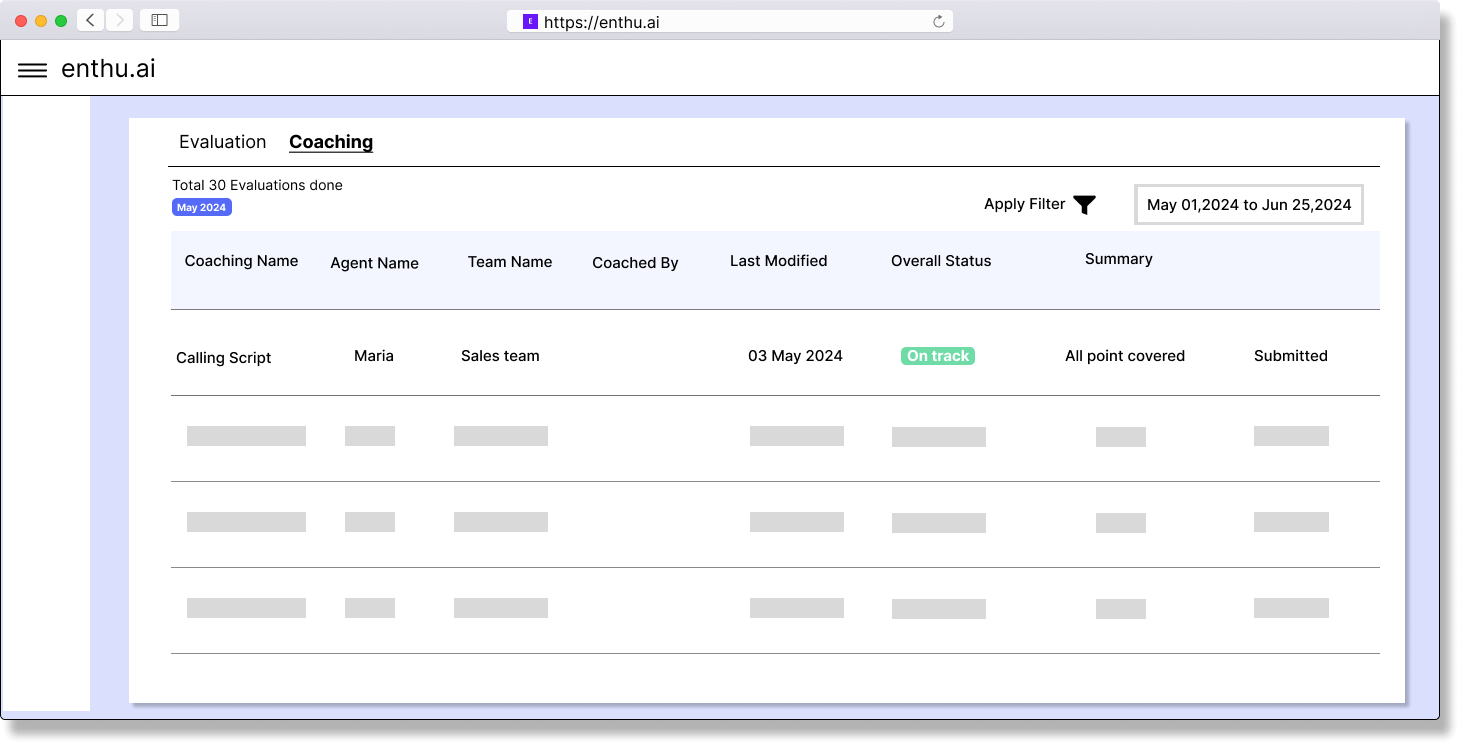
AI-driven agent quality analysis helps in evaluating agent performance by analyzing various metrics such as call handling time, customer satisfaction scores, and adherence to scripts.
This analysis provides insights into areas where agents excel and where they need improvement.
Based on these insights, you can design personalized training programs to enhance agent skills and ensure that they are well-equipped to meet customer expectations.
Continuous monitoring and training ensure that your team maintains high performance and delivers exceptional service.
6. Conversation Bots/Virtual Agents
Conversation bots, also known as virtual agents, are AI-powered tools that handle routine customer inquiries without human intervention.
These bots can manage tasks such as account inquiries, transaction history, and even basic troubleshooting.
This allows freeing up human agents to focus on more complex issues.
Since virtual agents are available 24/7, they can provide customers with immediate assistance.
They can handle multiple interactions simultaneously and ensure your contact center operates efficiently, even during peak times.
7. Customer Behavior Analysis
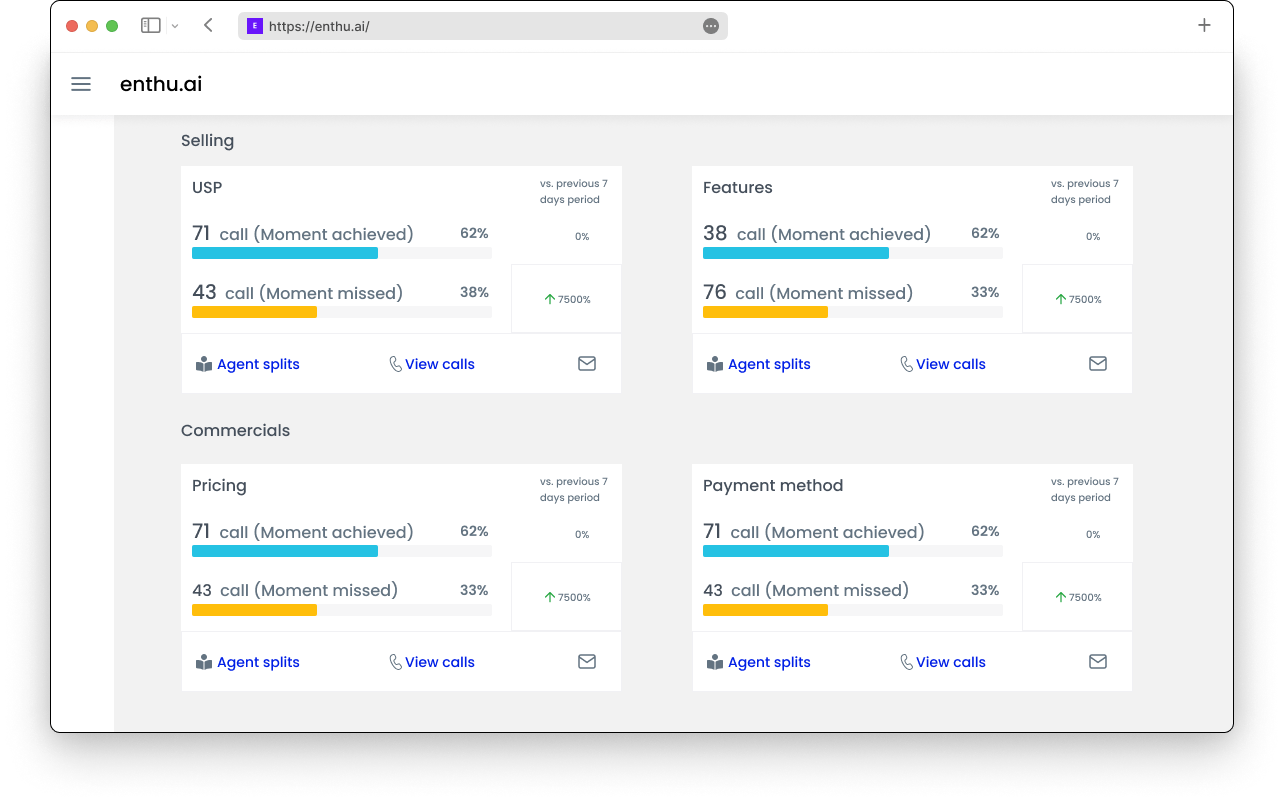
Customer behavior analysis uses AI to understand how customers interact with your services.
By financial data analysis using AI such as transaction history, browsing behavior, and interaction patterns, you can gain insights into customer preferences and tailor your services accordingly.
This technology helps you anticipate customer needs and provide personalized experiences, increasing customer satisfaction and loyalty.
For example, if customers frequently check mortgage rates, you can proactively offer them tailored mortgage products, enhancing their overall experience.
8. Fraud Detection
Fraud detection is a critical application of artificial intelligence finance.
AI algorithms can analyze large datasets to identify patterns and anomalies indicating fraudulent activity.
In contact centers, this technology helps monitor transactions and interactions for signs of fraud, ensuring the security of customer information.
Using AI for fraud detection, you can quickly identify and mitigate potential threats and protect your customers and your organization from financial loss.
9. Customer Experience Optimization
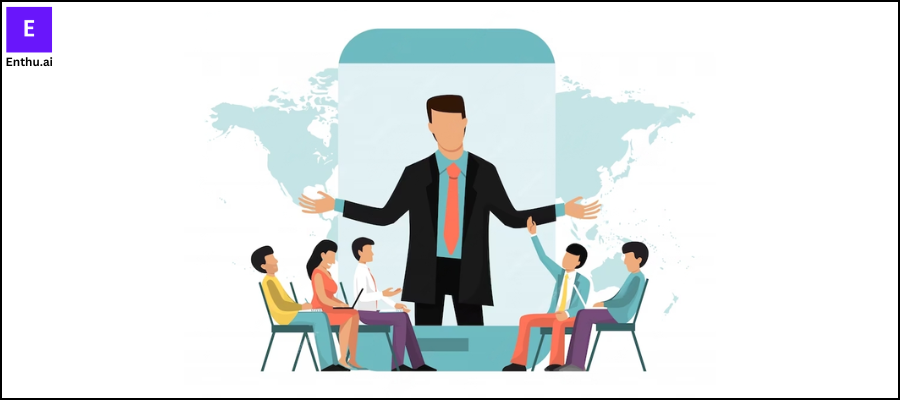
Customer experience optimization uses AI to enhance every aspect of the customer journey.
This includes personalizing interactions, predicting customer needs, and providing timely solutions.
By optimizing the customer experience, you can build stronger relationships with your customers and increase their loyalty to your brand.
AI technologies like predictive analytics, sentiment analysis, and conversation intelligence play a crucial role in optimizing customer experiences in financial contact centers.
C. Benefits of AI for the financial sector
Artificial intelligence in finance brings a wealth of advantages to the financial sector, transforming how institutions operate and serve their customers.
Here’s how AI can make a real difference for you:
- Automation: AI can streamline and automate repetitive tasks, such as verifying documents or answering routine questions. This means you can handle more tasks efficiently without manual intervention. For instance, AI can continuously monitor network traffic for cybersecurity or enhance digital banking experiences by personalizing services based on client needs.
- Accuracy: By minimizing manual errors, AI ensures that tasks like data processing, analytics, and document handling are done consistently and correctly every time. This reduces mistakes and provides more reliable outcomes in your operations.
- Efficiency: AI takes over routine, repetitive tasks, freeing up your team to focus on strategic activities. Whether it’s summarizing documents, transcribing calls , or handling simple customer inquiries, AI bots can handle these tasks, improving overall productivity.
- Speed: AI processes information faster than humans, quickly identifying patterns and relationships in data. This rapid analysis helps you make faster decisions, manage risks better, and respond to compliance requirements swiftly.
- Availability: AI works around the clock, allowing customers to access financial services and solutions whenever needed. Cloud-based AI systems can continuously handle tasks, ensuring you provide uninterrupted service.
- Innovation: With AI’s ability to analyze large volumes of data, you can develop new and innovative products and services. AI-driven predictive analytics can modernize customer experiences and keep you ahead of the competition.
D. How Enthu.AI drives more business to the financial sector
Running a contact center for a bank, lender, or credit union comes with its own set of challenges. It’s a tough job, from managing regulatory compliance to meeting customer expectations.
Enthu.AI is here to make it easier and help you drive more business. Here’s how:
- Boost Compliance: Enthu.AI ensures your agents are always on top of compliance requirements. It helps make sure they disclose all necessary information and adhere to banking regulations, keeping you safe and sound.
- Automate Quality Assurance: With Enthu.AI, you get 100% call QA coverage without needing extra manpower. This means you can identify coaching opportunities and improve agent performance effortlessly.
- Accelerate Training: Reviewing a 30-minute call used to take a lot of time. With Enthu.AI, you can analyze calls in seconds. This speeds up your training process and lets you focus more on coaching your agents.
- Increase Revenue: Enthu.AI helps you spot the winning behaviors of your top-performing agents. You can boost overall revenue performance by replicating these behaviors across your team.
- Improve Customer Experience: Identify and address your customers’ common concerns. Enthu.AI helps you group these issues and trains your agents to resolve them more efficiently, enhancing customer satisfaction.
- Reduce Agent Attrition: Working in financial sales and support can be stressful. Enthu.AI helps create a supportive environment by providing valuable feedback and fostering a culture of continuous learning, which can reduce agent turnover.
- Minimize Customer Churn: Understand why your customers leave and train your agents to handle these issues better. Enthu.AI helps you identify these reasons and take proactive measures to keep your customers happy.
In conclusion, embracing AI in your financial contact center isn’t just about keeping up with technology—it’s transforming how you serve customers and run operations.
Integrating AI tools can enhance efficiency, personalize experiences, and boost compliance.
The result?
Happier customers, more effective agents, and a competitive edge in the financial sector.
Investing in AI is a smart move if you want to stay ahead and meet your customers’ growing expectations.
With AI, you’re not just adapting but thriving in a fast-paced, digital world.
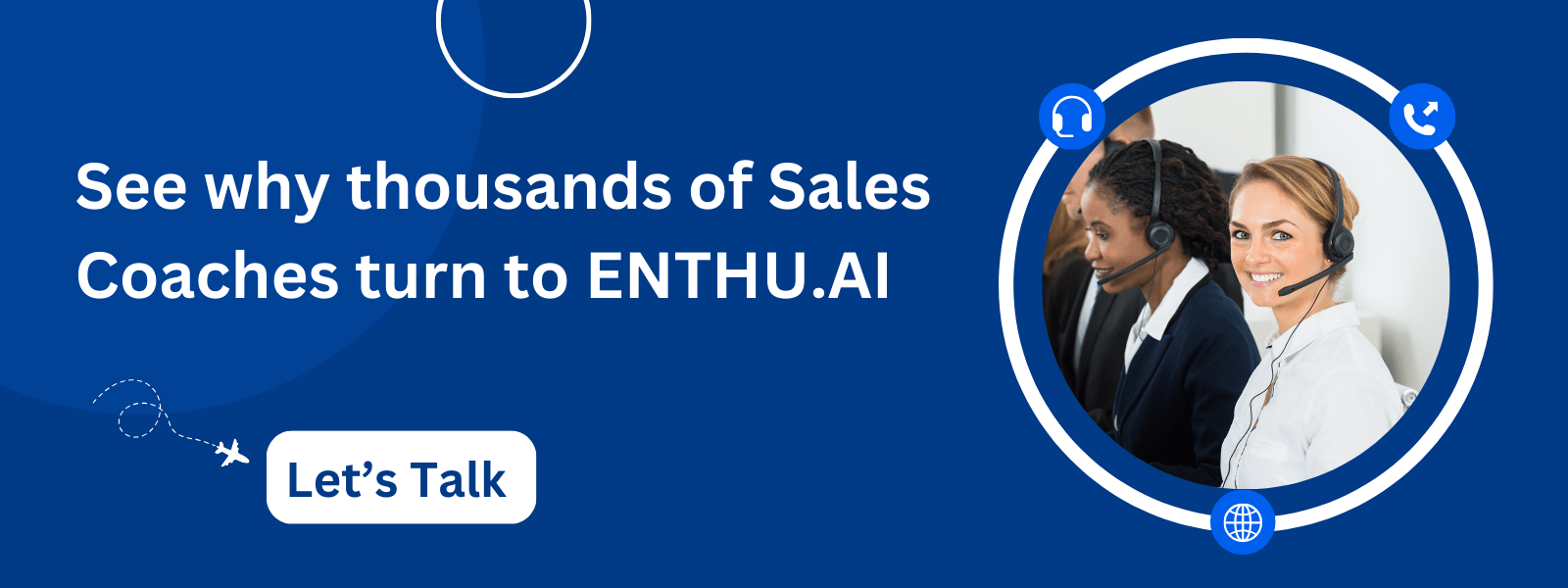
Tushar Jain is the co-founder and CEO at Enthu.AI. Tushar brings more than 15 years of leadership experience across contact center & sales function, including 5 years of experience building contact center specific SaaS solutions.
You can find Tushar Jain on Twitter & LinkedIn
More To Explore
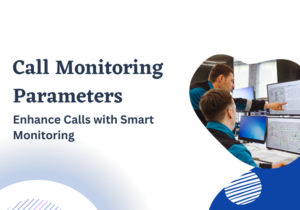
100+ Call Monitoring Parameters for Quality and Coaching
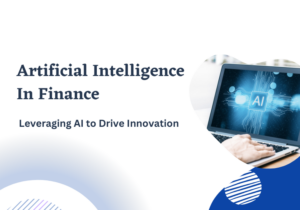
A Complete Guide on PII Redaction in Call Centers 2024
Leave a comment cancel reply.
Save my name, email, and website in this browser for the next time I comment.
Subscribe To Our Newsletter
Get updates and learn from the best.
- Request a demo
- [email protected]
- QA Managers
- Sales Leaders
- Sales Coaches
Get In Touch
- 651 N Broad St, Suite 201, Middletown, Delaware US - 19709
- C86, 5th Floor, IA Phase 7, Mohali, SAS Nagar, Punjab, India, 160055

COPYRIGHT © 2024 – enthu.ai | Privacy Policy | DPA | Terms
- Title & authors
Nandal, Neha, et al. "Healthcare Based Financial Decision Making System Using Artificial Intelligence." International journal of health sciences , vol. 6, no. S2, 2022, pp. 11255-11267, doi: 10.53730/ijhs.v6nS2.8025 .
Download citation file:
Artificial Intelligence is providing immense areas to work with and areas like Deep Learning and Machine Learning is taking over many research areas nowadays. The analysis and prediction of time series with machine and deep learning techniques are providing very promising results in the field of healthcare. The future values can be predicted with the help of time series. Therefore, the prediction of time series in healthcare based financial management provides organization with the useful information that supports in decision making. In this paper, the time series prediction on healthcare financial data is done by implementing Long Short Term Memory approach of Neural Networks for prediction of output for the time series data to predict business capabilities. Temporal characteristics of healthcare financial data are analyzed for time series forecasting. From the results, it is evident that this model is highly feasible to analyze the data with high precision and accuracy.
Table of contents
You're reading a free article with opinions that may differ from The Motley Fool's Premium Investing Services. Become a Motley Fool member today to get instant access to our top analyst recommendations, in-depth research, investing resources, and more. Learn More
Here's How Much Snowflake Spent on Research and Development Last Quarter
- Snowflake's Data Cloud is a revolutionary data unification and analytics platform.
- The company is now focusing on developing AI products, and it's spending heavily on R&D.
- Howevver, investors might be running out of patience with Snowflake's persistent losses.
- Motley Fool Issues Rare “All In” Buy Alert

Developing artificial intelligence products isn't cheap, and Snowflake certainly isn't holding back.
Snowflake 's ( SNOW 0.08% ) flagship product is the Data Cloud, which helps organizations break down data silos to extract more value from the information they harvest from their operations. Since data is the key to developing the most powerful artificial intelligence (AI) models, Snowflake is in the perfect position to build products for that industry.
The company launched its Cortex AI platform in 2023, which allows businesses to combine their data with ready-made large language models (like Meta Platforms ' Llama 3) to create AI software applications. Plus, Cortex comes with pre-built AI tools like Document AI, which allows developers to quickly extract valuable data from unstructured sources like contracts and invoices.
Snowflake loses money each quarter because it invests heavily in growth initiatives like research and development, which is its largest cost. While many tech companies have tried to manage their expenses over the last couple of years, that hasn't been so easy for Snowflake because of its focus on rolling out new AI products and services.
During the recent fiscal 2025 second quarter (ended July 31), Snowflake spent a record $437.6 million on research and development , which was a 40% increase from the year-ago period:
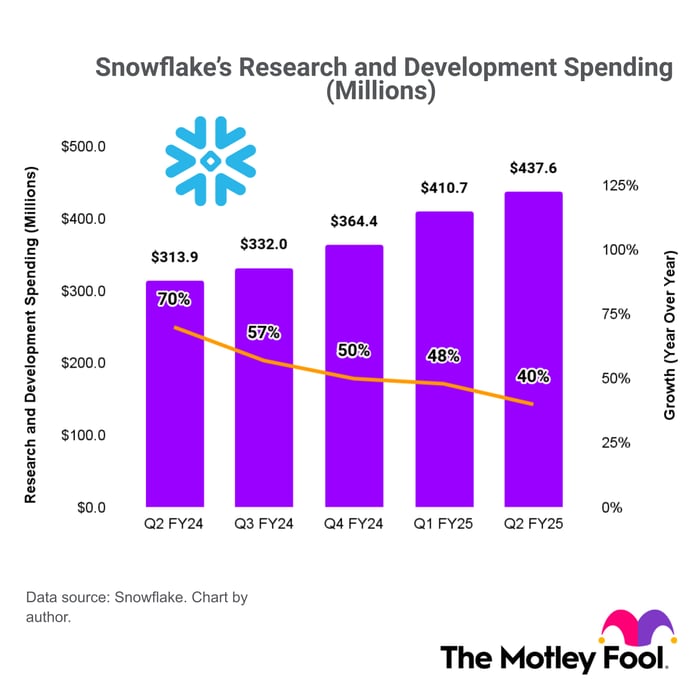
While that growth rate is decelerating, it's still much faster than the company's revenue growth, which came in at 30% in Q2 -- and that figure has also been decelerating over the last couple of years. An increase in operating costs overall led to a $316.9 million net loss for Snowflake during the quarter, which was a 40% jump from the year-ago period.
That trend is unlikely to reverse anytime soon because the company continues to hire more employees every quarter, with its headcount hitting a record high of 7,630 in Q2.
Investors will have to get used to persistent losses if Snowflake is to take a leadership position in the AI industry. However, they are clearly losing patience because the stock is down 39% this year already, and it's now down 71% from its all-time high. One investor that is no longer a shareholder is Berkshire Hathaway, which recently sold its shares .
Randi Zuckerberg, a former director of market development and spokeswoman for Facebook and sister to Meta Platforms CEO Mark Zuckerberg, is a member of The Motley Fool's board of directors. Anthony Di Pizio has no position in any of the stocks mentioned. The Motley Fool has positions in and recommends Meta Platforms and Snowflake. The Motley Fool has a disclosure policy .
Related Articles

Premium Investing Services
Invest better with The Motley Fool. Get stock recommendations, portfolio guidance, and more from The Motley Fool's premium services.
Try AI-powered search
- Why don’t women use artificial intelligence?
Even when in the same jobs, men are much more likely to turn to the tech

Your browser does not support the <audio> element.
B e more productive . That is how Chat GPT , a generative-artificial-intelligence tool from Open AI , sells itself to workers . But despite industry hopes that the technology will boost productivity across the workforce, not everyone is on board. According to two recent studies, women use Chat GPT between 16 and 20 percentage points less than their male peers, even when they are employed in the same jobs or read the same subject.

The first study, published as a working paper in June, explores Chat GPT at work. Anders Humlum of the University of Chicago and Emilie Vestergaard of the University of Copenhagen surveyed 100,000 Danes across 11 professions in which the technology could save workers time, including journalism, software-developing and teaching. The researchers asked respondents how often they turned to Chat GPT and what might keep them from adopting it. By exploiting Denmark’s extensive, hooked-up record-keeping, they were able to connect the answers with personal information, including income, wealth and education level.
Across all professions, women were less likely to use Chat GPT than men who worked in the same industry (see chart 1). For example, only a third of female teachers used it for work, compared with half of male teachers. Among software developers, almost two-thirds of men used it while less than half of women did. The gap shrank only slightly, to 16 percentage points, when directly comparing people in the same firms working on similar tasks. As such, the study concludes that a lack of female confidence may be in part to blame: women who did not use AI were more likely than men to highlight that they needed training to use the technology.

Another potential explanation for the gender imbalance comes from a survey of 486 students by Daniel Carvajal at Aalto University and Catalina Franco and Siri Isaksson at the Norwegian School of Economics ( NHH ). It also found a gender gap: female students enrolled in the NHH ’s only undergraduate programme were 18 percentage points less likely to use Chat GPT often. When the researchers separated students by admission grades, it became clear that the gap reflected the behaviour of mid- and high-performing women (see chart 2). Low performers were almost as likely as men to use the technology.
Why might this be? The researchers probed what was going on with some clever follow-up questions. They asked students whether they would use Chat GPT if their professor forbade it, and received a similar distribution of answers. However, in the context of explicit approval, everyone, including the better-performing women, reported that they would make use of the technology. In other words, the high-achieving women appeared to impose a ban on themselves. “It’s the ‘good girl’ thing,” reckons Ms Isaksson. “It’s this idea that ‘I have to go through this pain, I have to do it on my own and I shouldn’t cheat and take short-cuts’.”
A lack of experience with AI could carry a cost when students enter the labour market. In August the researchers added a survey of 1,143 hiring managers to their study, revealing that managers value high-performing women with AI expertise 8% more than those without. This sort of premium does not exist for men, suggesting that there are rewards for women who are willing to relax their self-imposed ban.
Tera Allas of McKinsey, a consultancy, worries that by the time AI is firmly embedded into modern working life, it might be designed to appeal more to men, who are its main users—potentially shutting women out in the long term. But not everyone is as concerned. Despite the fact that the early internet was dominated by men, for example, young American women were more online than their male counterparts by 2005. On top of this, Danielle Li of the Massachusetts Institute of Technology notes that the studies do not actually show whether men’s current Chat GPT use translates into better or more productive work. At the moment, the technology may be more of a digital toy, she says. Perhaps, then, high-achieving women are simply better at avoiding distraction. ■
For more expert analysis of the biggest stories in economics, finance and markets, sign up to Money Talks , our weekly subscriber-only newsletter.
Explore more
This article appeared in the Finance & economics section of the print edition under the headline “A new gender gap”
Finance & economics August 24th 2024
- Kamala Harris’s cost-of-living plan will end in failure
- America’s anti-price-gouging laws are too minor to be communist
- America’s recession signals are flashing red. Don’t believe them
- Why investors are not buying Europe’s revival
- Investors should avoid a new generation of rip-off ETFs
- Artificial intelligence is losing hype

From the August 24th 2024 edition
Discover stories from this section and more in the list of contents
More from Finance & economics

Can Japan’s zombie bond market be brought back to life?
Ueda Kazuo begins on a dangerous mission

The plasma trade is becoming ever-more hypocritical
Reliance on America grows, as other countries clutch their pearls

Are American rents rigged by algorithms?
That is what Department of Justice prosecutors allege
Inflation is down and a recession is unlikely. What went right?
A few years ago, nobody thought that a soft landing was possible
How Vladimir Putin hopes to transform Russian trade
He believes the country’s future lies with China and India. What could go wrong?
Vast government debts are riskier than they appear
A provocative new paper gets central bankers talking at Jackson Hole
arXiv's Accessibility Forum starts next month!
Help | Advanced Search
Quantum Physics
Title: quantum artificial intelligence: a brief survey.
Abstract: Quantum Artificial Intelligence (QAI) is the intersection of quantum computing and AI, a technological synergy with expected significant benefits for both. In this paper, we provide a brief overview of what has been achieved in QAI so far and point to some open questions for future research. In particular, we summarize some major key findings on the feasability and the potential of using quantum computing for solving computationally hard problems in various subfields of AI, and vice versa, the leveraging of AI methods for building and operating quantum computing devices.
Comments: | 21 pages, 5 figures |
Subjects: | Quantum Physics (quant-ph); Artificial Intelligence (cs.AI) |
Cite as: | [quant-ph] |
(or [quant-ph] for this version) | |
Focus to learn more arXiv-issued DOI via DataCite |
Submission history
Access paper:.
- Other Formats

References & Citations
- INSPIRE HEP
- Google Scholar
- Semantic Scholar
BibTeX formatted citation

Bibliographic and Citation Tools
Code, data and media associated with this article, recommenders and search tools.
- Institution
arXivLabs: experimental projects with community collaborators
arXivLabs is a framework that allows collaborators to develop and share new arXiv features directly on our website.
Both individuals and organizations that work with arXivLabs have embraced and accepted our values of openness, community, excellence, and user data privacy. arXiv is committed to these values and only works with partners that adhere to them.
Have an idea for a project that will add value for arXiv's community? Learn more about arXivLabs .
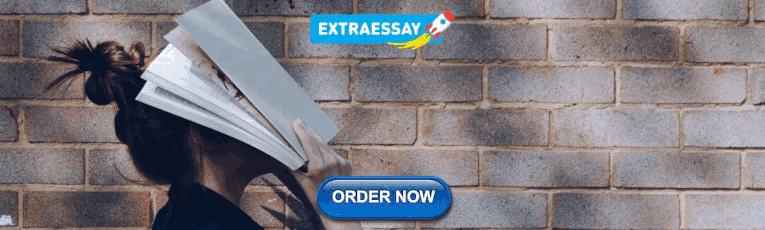
IMAGES
VIDEO
COMMENTS
Over the past two decades, artificial intelligence (AI) has experienced rapid development and is being used in a wide range of sectors and activities, including finance. In the meantime, a growing and heterogeneous strand of literature has explored the use of AI in finance. The aim of this study is to provide a comprehensive overview of the existing research on this topic and to identify which ...
1. Introduction. The emerging use of artificial intelligence (AI) and machine learning (ML) within financial systems is disrupting and transforming industries, and societies (Li and Tang, 2020, Wall, 2018).From traditional hedge fund management firms and investment and retail banks, to contemporary financial technology (FinTech) service providers, many financial firms today are heavily ...
The rapid expansion of artificial intelligence (AI) technologies presents novel technical solutions to traditional accounting and finance problems. Despite this, scholars in accounting and finance frequently encounter difficulties navigating the extensive and intricate domain knowledge of AI and its continuously evolving literature. To address this gap, this paper conducts a qualitative survey ...
Artificial Intelligence (AI) is revolutionizing the landscape of financial services, offering transformative opportunities for efficiency, innovation, and customer experience enhancement. This ...
Artificial intelligence (AI), ... (2021, p. 1) found three overarching clusters of AI and ML research in finance: "1) portfolio construction, valuation, and investor behavior; 2) financial fraud and distress; ... in each paper. Fig. 1 illustrates the distribution of the literature from 2011 to 2021, achieving an average growth rate of 34%. It ...
This review summarizes the lasting research on AI in finance and focuses on creating a comprehensive, multidimensional and economic-financial problem-driven research landscape of the roles, research directions and opportunities of AI in new-generation FinTech and finance. Keywords: Artificial intelligence, economics, finance, AI in FinTech ...
2)papers that focus on AI applications for finance and financial markets; and 3) sub-fields of finance and fin ancial markets where AI is being used. It is clarified that not all the 191 papers ...
This paper discusses the impact of the rapid adoption of artificial intelligence (AI) and machine learning (ML) in the financial sector. It highlights the benefits these technologies bring in terms of financial deepening and efficiency, while raising concerns about its potential in widening the digital divide between advanced and developing economies. The paper advances the discussion on the ...
Artificial intelligence (AI) in finance has been a research area of great interest for many decades. ... review summarizes the decades of AI research in finance from the financial application perspectives, ... analyzing and commenting on the application ecosystem. This paper complements this review but takes a technical perspective to further ...
View a PDF of the paper titled AI in Finance: Challenges, Techniques and Opportunities, by Longbing Cao. AI in finance broadly refers to the applications of AI techniques in financial businesses. This area has been lasting for decades with both classic and modern AI techniques applied to increasingly broader areas of finance, economy and society.
Artificial intelligence (AI) alongside one of its main subsets, machine learning (ML), is no longer a sheer propaganda, it has nearly become a household name, though the use of the term AI by the public and at times technologists is often a misnomer. This paper explores AI and ML, outlining the main categories of extensive ML algorithmic techniques. Importantly, it provides handy timeline and ...
Title: Powering the digital economy : opportunities and risks of artificial intelligence in finance / prepared by El Bachir Boukherouaa and Ghiath Shabsigh in collaboration with Khaled AlAjmi, Jose Deodoro, Aquiles Farias, Ebru S. Iskender, ... The Departmental Paper Series presents research by IMF staff on issues of broad regional or cross ...
A literature review. Abstract: In the 2020s, Artificial Intelligence (AI) has been increasingly becoming a domi nant. technology, and thanks to new computer technologies, M achine Learning (ML ...
With the increasing interest in artificial intelligence in recent years, this paper reviews the Artificial Intelligence (AI) techniques in the finance domain systematically and attempts to identify the current AI technologies used, major applications, challenges, and trends in Finance.
Artificial intelligence (AI) is transforming the global financial services industry. As a group of rapidly related technologies that include machine learning (ML) and deep learning(DL) , AI has the potential to disrupt and refine the existing financial services industry. I review the extant academic, practitioner and policy related literatureAI.
Abstract. Over the past two decades, artificial intelligence (AI) has experienced rapid develop-ment and is being used in a wide range of sectors and activities, including finance. In the meantime, a growing and heterogeneous strand of literature has explored the use of AI in finance. The aim of this study is to provide a comprehensive overview ...
Artificial Intelligence (AI) approaches have been increasingly used in financial markets as technology advances. In this research paper, we conduct a Systematic Literature Review (SLR) that studies financial trading approaches through AI techniques. It reviews 143 research articles that implemented AI techniques in financial trading markets.
The Finance industry is under constant development, always using and adapting to new technological opportunities (Gimpel et al. 2018)—like Artificial Intelligence (AI) and Data Analytics—that shape private and working lives worldwide.Financial institutions benefit from technological advances, recently from AI deployment (Alt et al. 2018; Goodell et al. 2021): Due to their strong predictive ...
From data analysis to decision-making processes, AI is reshaping the accounting and finance profession. To gain a deeper understanding of AI's impact on the profession, IMA engaged global experts in the field and accounting and finance leaders to study the applications of AI, as well as the challenges of integrating it with management ...
in financial decision making, enabling organizations to make more informed, efficient, and reliable decisions while maintaining ethical and regulatory compliance. The role of artificial intelligence (AI) in financial decision-making has resulted in several positive outcomes and decisions. Here are some key results and benefits:
The convergence of artificial intelligence (AI) and decentralized finance (DeFi) is poised to revolutionize the financial landscape. DeFi, which leverages blockchain technology to offer ...
This paper employs insights from earth science on the financial risk of project developments to present an economic theory of critical minerals. Our theory posits that back-ended critical mineral ...
A. Role of artificial intelligence in finance. Artificial intelligence (AI) is transforming the finance industry by making processes smarter, faster, and more efficient. ... Based on our research and keeping the context of the year 2024, here's a list of the top AI technologies in financial contact centers that any business can prefer: ...
Artificial Intelligence is providing immense areas to work with and areas like Deep Learning and Machine Learning is taking over many research areas nowadays. ... In this paper, the time series prediction on healthcare financial data is done by implementing Long Short Term Memory approach of Neural Networks for prediction of output for the time ...
This research paper aims to better define artificial intelligence (AI) and its current role in financial markets. All the while discussing the subgroups of AI, how machines learn, the pro's and ...
OpenAI Artificial intelligence Free exchange This article appeared in the Finance & economics section of the print edition under the headline "Hype about the hype cycle" Finance & economics ...
WASHINGTON, D.C. -- Americans continue to see few upsides to using artificial intelligence in business. The latest survey from Bentley University and Gallup shows that U.S. adults see more harm than good from the use of AI, believe it will cost U.S. jobs and do not trust businesses to use it responsibly.
Developing artificial intelligence products isn't cheap, and Snowflake certainly isn't holding back. ... The Motley Fool is a financial services company dedicated to making the world smarter ...
Artificial intelligence This article appeared in the Finance & economics section of the print edition under the headline "A new gender gap" Finance & economics August 24th 2024
Quantum Artificial Intelligence (QAI) is the intersection of quantum computing and AI, a technological synergy with expected significant benefits for both. In this paper, we provide a brief overview of what has been achieved in QAI so far and point to some open questions for future research. In particular, we summarize some major key findings on the feasability and the potential of using ...